Identifying Triggers for Retraining AI Models to Maintain Performance
Identifying Triggers for Retraining AI Models to Maintain Performance
Identifying Triggers for Retraining AI Models to Maintain Performance
Jigar Gupta
Jul 16, 2024




Ever wonder why your AI models can underperform over time? Knowing why model retraining matters is key in AI. Your AI models need to adapt to new information and changes. You need to identify when retraining your AI models is needed.
Now that you understand why retraining is crucial let’s dive into the specifics of when and how to approach it.
Discover Real-World AI Model Testing Perceptions with Our In-Depth Case Studies in "In-Depth Case Studies in AI Model Testing: Exploring Real-World Applications and Insights."
The Necessity of Retraining AI Models
Updating your AI models is crucial for their efficiency. Let's look at two main reasons to retrain them.
Maintaining Predictive Accuracy Amid Data and Concept Drift
Think of your AI model as a detective spotting clues. However, the globe keeps altering, and new types of testimony pop up that our detective has seen before. This is what we call information and concept drift. Your model’s auguring precision can take a hit if it doesn't adjust to these alterations. Retraining your AI model ensures it stays fine-edged and pertinent, ready to tackle the newest challenges and deliver precise forecasts.
Incorporating Current Data
Data terrains are always progressing. New information comes in, reflecting the latest trends, behaviors and motifs. If your AI model doesn’t integrate this fresh information, it becomes outdated, such as an old map to traverse a modern city. By retraining your AI model, you ensure it remains current and proficient in making choices based on the most recent data. This perpetual learning process keeps your model affiliated with the real globe, improving its performance and dependability.
Retraining your AI model is not just a technical requisite; it’s a way to ensure your model remains a valuable asset in a volatile environment. Stay proactive with model retraining triggers to keep your models precise and up-to-date.
Let’s break down two of the most significant challenges you’ll face: concept drift and data drift.
Ready to ensure your AI tasks are sturdy and dependable? Begin Implementing Regression Tests in AI Development today to protect your AI solutions from regression and ensure constant performance. Contact us now to locate how our expert AI testing services can upgrade your evolution process.
Understanding Concept Drift and Data Drift
Concept drift and data drift are crucial factors that can affect the execution of your AI models. Let’s learn what these terms mean and how they affect your models.
Definition and Implications of Concept Drift
Concept drift refers to the phenomenon where the statistical properties of the target volatile alteration over time in unanticipated ways. In simpler terms, the motifs of your AI model learned initially may no longer hold true as new information appears. This can lead to a subside in forecast precision and dependability. For instance, in a financial predictive model, alterations in market behavior or regulations could indulge concept drift.
Challenges of Data Drift and Its Impact on Model Accuracy
Data drift occurs when the distribution of your input data alters over time, causing your model to become less efficient. This can happen due to numerous reasons like alterations in user behavior, environmental factors, or data gathering techniques. For example, if you’re using an AI model to forecast customer preferences based on social media information, shifts in user demographics or platform algorithms can introduce data drift.
Maintaining Model Performance

To maintain optimal performance, it's important to observe for signs of concept drift and data drift frequently. Here are the steps you take:
Continuous Monitoring: Execute monitoring systems that trace model performance metrics and locate divergence that may specify drift.
Flexible Learning: Integrate techniques such as online learning or gradual training that permit your model to adjust new motifs without full retraining.
Trigger-Based Retraining: Set verges or triggers based on performance metrics. When these thresholds are crossed, it beacons the need for retraining to capture new motifs efficiently.
By comprehending and proactively acknowledging concept drift and data drift, you can ensure that your AI model sustains their precision and pertinence over time. Frequent updates and adjustments will keep your models affiliated with the progressing real-globe synopsis they are designed to elucidate.
Speaking of staying ahead, let’s explore how to identify the key triggers that signal when it’s time to retrain your models.
Ready to boost your AI-powered applications and systems? Discover the Strategic Approach To Testing AI-powered applications And Systems. Don’t leave your AI solutions to chance; take command with our expert guidance.
Identifying Retraining Triggers
Keeping your AI models performing at their best needs alertness and prompt mediation. Knowing when to preserve your models is critical. Here are some key triggers to watch for:
Performance Metrics as Indicators for Retraining
Pay close attention to your performance metrics. Metrics such as precision, FI score and RMSE (Root Mean Square Error) give clear indications of your model’s health. If you observe a substantial drop in these numbers, it’s a strong sign that your model might require retraining. Frequently monitoring these metrics helps you catch problems early, ensuring your AI persists in delivering dependable outcomes.
Data Distribution Statistics and Prediction Difference Rate
Alterations in data distribution can be delicate. Monitor your data for shifts in distribution statistics, like mean, variance, or class proportions. In addition, trace the forecast distinction rate, which gauges the variance between forecasted and actual values over time. Sudden spikes or continuous drifts in these indicators suggest that your model’s assumptions about the information may no longer hold true, beckoning the requirement for retraining.
Human-in-Loop Signals and Flagged Predictions
Human-in-the-loop systems give valuable responses. When users constantly flag certain forecasts as incorrect or questionable, it’s a clear sign that the model might be losing its edge. Integrate this response loop to determine difficult areas that need retraining. Frequently retrospecting and acting on these flagged forecasts helps sustain your model’s pertinence and precision.
Monitoring for Changes in Statistical Distribution in Data
Statistical alterations in your data can creep in discreetly, impacting model’s performance. Enforce constant monitoring systems to locate any shifts in data distribution. Look for alterations in key statistical measures such as mean, standard deviation, and distribution shapes. By determining these alterations early, you can decide whether your model needs to be retrained to sustain the new data attributes.
By staying proactive and observing these triggers, you can ensure your AI models stay efficient and precise. Frequent retraining based on these cues will aid you.
So, when exactly should you retrain, and which methods should you consider? Let’s take a closer look.
Discover Best Practices For Testing Computer Vision Models – Improve Precision Today!
Approaches to Model Retraining
In the hasty world of Artificial Intelligence, keeping your model performing at its best requires frequent attention. One critical aspect of sustaining the model's performance is knowing when to retrain it. Let’s learn about the distinct approaches to model retraining, concentrating on the distinction between fixed retraining tempo and dynamic retraining, the advantages and disadvantages of schedule-based retraining, and the merits of trigger-based retraining.
Difference Between Fixed Retraining Cadence and Dynamic Retraining
You have two predominant strategies when it comes to retraining your models: fixed retraining cadence and dynamic retraining.
Fixed Retraining Cadence: This approach indulges retraining your models at standard, predetermined intervals. For instance, you might choose to retrain every month, quarter, or year, regardless of how your model is working. The benefit here is modesty and consistency. You know exactly when the next retraining session will occur, making it easier to strategize and distribute resources.
Dynamic Retraining: Unlike the fixed schedule, dynamic retraining is more adaptable and receptive to your model’s performance. You observe your model’s performance metrics constantly and retrain only when required. This approach ensures that you acknowledge performance dips or alterations in data allocation instantly. It needs more sophisticated monitoring and evaluation procedures but can result in more effective resource use and better model performance.
Schedule-Based Retraining: Its Simplicity and Limitations
Schedule-Based Retraining is elementary and easy to enforce. By sticking to a fixed schedule, you can strategize your resources and outlines without any amazements. Here’s why it is appealing:
Simplicity: You don’t require intricate monitoring systems. Just mark your calendar and retrain on the set date.
Predictability: You can forecast time and resources well in advance, ensuring that your team is ready for each retraining session.
Routine Maintenance: Frequent retraining can help catch any steady performance degradation over time.
However there are renowned limitations:
Inefficiency: You might retrain models even when it’s not mandatory, frittering time and resources.
Delayed Response: If you model performance drops substantially between anticipated retrainings, you can suffer from poor outcomes until the next session.
Static Approach: This technique doesn’t adjust to the real-time requirements of your models, certainly resulting in periods of unacceptable performance.
Trigger-Based Retraining: Responsive and Adaptive Approach to Retraining
Trigger-Based Retraining is a more sophisticated and receptive method. Instead of following a fixed schedule, you retrain your models based on precise triggers or performance metrics. This approach is highly flexible and ensures your models remain in top shape.
Responsiveness: You retrain your models only where there’s a clear requirement, like a drop in precision, alterations in data motifs, or the introduction of new data attributes. This keeps your model up-to-date and executing well.
Effectiveness: By retraining only when significant, you upgrade the use of your resources, saving time and effort.
Flexibility: This technique permits you to swiftly adjust to new trends or information shifts, sustaining high performance in changing environments.
However, enforcing trigger-based retraining requires:
Continuous Monitoring: You need sturdy systems to trace your model’s performance metrics and locate when they fall below adequate thresholds.
Complex Infrastructure: Setting up and sustaining the essential infrastructure for trigger-based retraining can be intricate and resource-intensive.
Selecting the right approach to model retraining.relies on your precise requirements and resources. Schedule-based retraining provides clarity and consistency but may lead to ineffectiveness. On the contrary, trigger-based retraining offers a receptive and compatible solution, ensuring your models perform perfectly at all times. By comprehending these approaches, you make informed decisions to sustain and improve the performance of your AI models.
Knowing the approaches is great, but let’s ensure you’re following the best practices to maximize the efficiency of your model retraining.
Want to know about Deep learning applications? Check out our detailed guide on Exploring The Frontiers Of Deep Learning Applications.
Best Practices in Retraining AI Models
Retraining AI models is a crucial practice to ensure their precision, pertinence, and performance over time. Here are some best practices to contemplate:
Monitor Multiple Trigger Metrics to Robustly Detect Drift
You can observe numerous metrics to detect drift efficiently. Don’t just depend on a single metric; an amalgamation gives you a coherent picture. Think of it like observing distinct integral signs for a patient. If one goes off, it could specify an issue, but if numerous do, you know something is surely wrong.
Minimize Operational Impact with Asynchronous Retraining
Retraining models can be resource-intensive, but you can avoid disrupting your daily functioning by doing it asynchronously. Schedule training during off-peak hours or run it in the background. This way, you predominant systems stay genuine, and you sustain smooth functioning.
Continuous Testing of Retrained Models in Pre-Production
Before you roll out a retrained model, put it through rigid testing in a pre-production environment. This step ensures that the new model works well under numerous synopsis and doesn’t acquaint any unanticipated problems. It’s like going on a test drive before purchasing the car.
Maintain Dataset Snapshots for Reproducible Retraining
Always save screenshots of your training datasets. This practice ensures that you can reproduce the training process if required. Suppose it is saving distinct versions of a document you’re operating on; if something goes erroneous, you can always switch to the previous version.
Validate Retrained Models for Stability Before Deployment
Before deploying a retrained model, verify it comprehensively. This step assures that the model is steady and dependable. Think of it as cross-verifying your work before handing over an important report; you want to make sure everything is precise.
Automate Retraining for Efficiency and Scalability
Automation is your best buddy when it comes to retraining AI models. Set up automated assignments to handle retraining tasks. This approach not only saves time but also ensures steadiness and configurability. It’s like having a dependable assistant who takes care of repetitive tasks for you.
By following these best practices, you will keep your AI models precise, dependable and ready to adjust to new data and altering circumstances.
Now that you’re equipped with best practices, let’s outline the strategies for effectively implementing retraining triggers.
Unleash smooth incorporation: Your Practical Guide to Deploying LLMs in Production.
Strategies for Implementing Retraining Triggers

Implementing retraining triggers efficiently indulges numerous key strategies to ensure that your AI models remain precise, dependable and up-to-date. Here are some strategies to contemplate:
Choose Relevant Metrics
To keep your AI model on track, you need to choose the right metric. Concentrate on those that directly affect your model’s performance. Perfection, accuracy, recall, and FI score are great starting points. Frequently monitor these metrics to spot any dips in performance, which signify the duration of retraining.
Spot the Need for Retraining
Information alters over time, and so should your model. Set up alerts for substantial shifts in data motifs or distributions. Use methods such as concept drift detection to recognize when your model’s forecasts are becoming less dependable. This dynamic approach helps you stay ahead and retrain before problems intensify.
Test and Verify Comprehensively
Before rolling out a new model and version, rigorously experiment with it. Separate your information into training and verifying sets and use cross-verification to ensure sturdy performance. Contrast the new model against the old one in a restrained environment. Once it clears all tests, you can positively deploy it to production, knowing it’s ready to handle real-globe information.
Discover the power of Artificial Intelligence: Practical Strategies for Self-Hosting Large Language Models Today!
Conclusion
Determining triggers for retraining is a crucial step in sustaining AI model performance. By constantly observing performance metrics, data distribution methods, and human-in-the-loop signals, you can ensure your models stay precise and efficient. Enfold a constant cycle of monitoring, triggering, and retraining to keep your AI models at the top of their game, ensuring endurance and dependability in a continuously developing environment.
Revolutionize your testing productivity today with RagaAI’s instinctive platform. Sign up now for a free trial!
Ever wonder why your AI models can underperform over time? Knowing why model retraining matters is key in AI. Your AI models need to adapt to new information and changes. You need to identify when retraining your AI models is needed.
Now that you understand why retraining is crucial let’s dive into the specifics of when and how to approach it.
Discover Real-World AI Model Testing Perceptions with Our In-Depth Case Studies in "In-Depth Case Studies in AI Model Testing: Exploring Real-World Applications and Insights."
The Necessity of Retraining AI Models
Updating your AI models is crucial for their efficiency. Let's look at two main reasons to retrain them.
Maintaining Predictive Accuracy Amid Data and Concept Drift
Think of your AI model as a detective spotting clues. However, the globe keeps altering, and new types of testimony pop up that our detective has seen before. This is what we call information and concept drift. Your model’s auguring precision can take a hit if it doesn't adjust to these alterations. Retraining your AI model ensures it stays fine-edged and pertinent, ready to tackle the newest challenges and deliver precise forecasts.
Incorporating Current Data
Data terrains are always progressing. New information comes in, reflecting the latest trends, behaviors and motifs. If your AI model doesn’t integrate this fresh information, it becomes outdated, such as an old map to traverse a modern city. By retraining your AI model, you ensure it remains current and proficient in making choices based on the most recent data. This perpetual learning process keeps your model affiliated with the real globe, improving its performance and dependability.
Retraining your AI model is not just a technical requisite; it’s a way to ensure your model remains a valuable asset in a volatile environment. Stay proactive with model retraining triggers to keep your models precise and up-to-date.
Let’s break down two of the most significant challenges you’ll face: concept drift and data drift.
Ready to ensure your AI tasks are sturdy and dependable? Begin Implementing Regression Tests in AI Development today to protect your AI solutions from regression and ensure constant performance. Contact us now to locate how our expert AI testing services can upgrade your evolution process.
Understanding Concept Drift and Data Drift
Concept drift and data drift are crucial factors that can affect the execution of your AI models. Let’s learn what these terms mean and how they affect your models.
Definition and Implications of Concept Drift
Concept drift refers to the phenomenon where the statistical properties of the target volatile alteration over time in unanticipated ways. In simpler terms, the motifs of your AI model learned initially may no longer hold true as new information appears. This can lead to a subside in forecast precision and dependability. For instance, in a financial predictive model, alterations in market behavior or regulations could indulge concept drift.
Challenges of Data Drift and Its Impact on Model Accuracy
Data drift occurs when the distribution of your input data alters over time, causing your model to become less efficient. This can happen due to numerous reasons like alterations in user behavior, environmental factors, or data gathering techniques. For example, if you’re using an AI model to forecast customer preferences based on social media information, shifts in user demographics or platform algorithms can introduce data drift.
Maintaining Model Performance

To maintain optimal performance, it's important to observe for signs of concept drift and data drift frequently. Here are the steps you take:
Continuous Monitoring: Execute monitoring systems that trace model performance metrics and locate divergence that may specify drift.
Flexible Learning: Integrate techniques such as online learning or gradual training that permit your model to adjust new motifs without full retraining.
Trigger-Based Retraining: Set verges or triggers based on performance metrics. When these thresholds are crossed, it beacons the need for retraining to capture new motifs efficiently.
By comprehending and proactively acknowledging concept drift and data drift, you can ensure that your AI model sustains their precision and pertinence over time. Frequent updates and adjustments will keep your models affiliated with the progressing real-globe synopsis they are designed to elucidate.
Speaking of staying ahead, let’s explore how to identify the key triggers that signal when it’s time to retrain your models.
Ready to boost your AI-powered applications and systems? Discover the Strategic Approach To Testing AI-powered applications And Systems. Don’t leave your AI solutions to chance; take command with our expert guidance.
Identifying Retraining Triggers
Keeping your AI models performing at their best needs alertness and prompt mediation. Knowing when to preserve your models is critical. Here are some key triggers to watch for:
Performance Metrics as Indicators for Retraining
Pay close attention to your performance metrics. Metrics such as precision, FI score and RMSE (Root Mean Square Error) give clear indications of your model’s health. If you observe a substantial drop in these numbers, it’s a strong sign that your model might require retraining. Frequently monitoring these metrics helps you catch problems early, ensuring your AI persists in delivering dependable outcomes.
Data Distribution Statistics and Prediction Difference Rate
Alterations in data distribution can be delicate. Monitor your data for shifts in distribution statistics, like mean, variance, or class proportions. In addition, trace the forecast distinction rate, which gauges the variance between forecasted and actual values over time. Sudden spikes or continuous drifts in these indicators suggest that your model’s assumptions about the information may no longer hold true, beckoning the requirement for retraining.
Human-in-Loop Signals and Flagged Predictions
Human-in-the-loop systems give valuable responses. When users constantly flag certain forecasts as incorrect or questionable, it’s a clear sign that the model might be losing its edge. Integrate this response loop to determine difficult areas that need retraining. Frequently retrospecting and acting on these flagged forecasts helps sustain your model’s pertinence and precision.
Monitoring for Changes in Statistical Distribution in Data
Statistical alterations in your data can creep in discreetly, impacting model’s performance. Enforce constant monitoring systems to locate any shifts in data distribution. Look for alterations in key statistical measures such as mean, standard deviation, and distribution shapes. By determining these alterations early, you can decide whether your model needs to be retrained to sustain the new data attributes.
By staying proactive and observing these triggers, you can ensure your AI models stay efficient and precise. Frequent retraining based on these cues will aid you.
So, when exactly should you retrain, and which methods should you consider? Let’s take a closer look.
Discover Best Practices For Testing Computer Vision Models – Improve Precision Today!
Approaches to Model Retraining
In the hasty world of Artificial Intelligence, keeping your model performing at its best requires frequent attention. One critical aspect of sustaining the model's performance is knowing when to retrain it. Let’s learn about the distinct approaches to model retraining, concentrating on the distinction between fixed retraining tempo and dynamic retraining, the advantages and disadvantages of schedule-based retraining, and the merits of trigger-based retraining.
Difference Between Fixed Retraining Cadence and Dynamic Retraining
You have two predominant strategies when it comes to retraining your models: fixed retraining cadence and dynamic retraining.
Fixed Retraining Cadence: This approach indulges retraining your models at standard, predetermined intervals. For instance, you might choose to retrain every month, quarter, or year, regardless of how your model is working. The benefit here is modesty and consistency. You know exactly when the next retraining session will occur, making it easier to strategize and distribute resources.
Dynamic Retraining: Unlike the fixed schedule, dynamic retraining is more adaptable and receptive to your model’s performance. You observe your model’s performance metrics constantly and retrain only when required. This approach ensures that you acknowledge performance dips or alterations in data allocation instantly. It needs more sophisticated monitoring and evaluation procedures but can result in more effective resource use and better model performance.
Schedule-Based Retraining: Its Simplicity and Limitations
Schedule-Based Retraining is elementary and easy to enforce. By sticking to a fixed schedule, you can strategize your resources and outlines without any amazements. Here’s why it is appealing:
Simplicity: You don’t require intricate monitoring systems. Just mark your calendar and retrain on the set date.
Predictability: You can forecast time and resources well in advance, ensuring that your team is ready for each retraining session.
Routine Maintenance: Frequent retraining can help catch any steady performance degradation over time.
However there are renowned limitations:
Inefficiency: You might retrain models even when it’s not mandatory, frittering time and resources.
Delayed Response: If you model performance drops substantially between anticipated retrainings, you can suffer from poor outcomes until the next session.
Static Approach: This technique doesn’t adjust to the real-time requirements of your models, certainly resulting in periods of unacceptable performance.
Trigger-Based Retraining: Responsive and Adaptive Approach to Retraining
Trigger-Based Retraining is a more sophisticated and receptive method. Instead of following a fixed schedule, you retrain your models based on precise triggers or performance metrics. This approach is highly flexible and ensures your models remain in top shape.
Responsiveness: You retrain your models only where there’s a clear requirement, like a drop in precision, alterations in data motifs, or the introduction of new data attributes. This keeps your model up-to-date and executing well.
Effectiveness: By retraining only when significant, you upgrade the use of your resources, saving time and effort.
Flexibility: This technique permits you to swiftly adjust to new trends or information shifts, sustaining high performance in changing environments.
However, enforcing trigger-based retraining requires:
Continuous Monitoring: You need sturdy systems to trace your model’s performance metrics and locate when they fall below adequate thresholds.
Complex Infrastructure: Setting up and sustaining the essential infrastructure for trigger-based retraining can be intricate and resource-intensive.
Selecting the right approach to model retraining.relies on your precise requirements and resources. Schedule-based retraining provides clarity and consistency but may lead to ineffectiveness. On the contrary, trigger-based retraining offers a receptive and compatible solution, ensuring your models perform perfectly at all times. By comprehending these approaches, you make informed decisions to sustain and improve the performance of your AI models.
Knowing the approaches is great, but let’s ensure you’re following the best practices to maximize the efficiency of your model retraining.
Want to know about Deep learning applications? Check out our detailed guide on Exploring The Frontiers Of Deep Learning Applications.
Best Practices in Retraining AI Models
Retraining AI models is a crucial practice to ensure their precision, pertinence, and performance over time. Here are some best practices to contemplate:
Monitor Multiple Trigger Metrics to Robustly Detect Drift
You can observe numerous metrics to detect drift efficiently. Don’t just depend on a single metric; an amalgamation gives you a coherent picture. Think of it like observing distinct integral signs for a patient. If one goes off, it could specify an issue, but if numerous do, you know something is surely wrong.
Minimize Operational Impact with Asynchronous Retraining
Retraining models can be resource-intensive, but you can avoid disrupting your daily functioning by doing it asynchronously. Schedule training during off-peak hours or run it in the background. This way, you predominant systems stay genuine, and you sustain smooth functioning.
Continuous Testing of Retrained Models in Pre-Production
Before you roll out a retrained model, put it through rigid testing in a pre-production environment. This step ensures that the new model works well under numerous synopsis and doesn’t acquaint any unanticipated problems. It’s like going on a test drive before purchasing the car.
Maintain Dataset Snapshots for Reproducible Retraining
Always save screenshots of your training datasets. This practice ensures that you can reproduce the training process if required. Suppose it is saving distinct versions of a document you’re operating on; if something goes erroneous, you can always switch to the previous version.
Validate Retrained Models for Stability Before Deployment
Before deploying a retrained model, verify it comprehensively. This step assures that the model is steady and dependable. Think of it as cross-verifying your work before handing over an important report; you want to make sure everything is precise.
Automate Retraining for Efficiency and Scalability
Automation is your best buddy when it comes to retraining AI models. Set up automated assignments to handle retraining tasks. This approach not only saves time but also ensures steadiness and configurability. It’s like having a dependable assistant who takes care of repetitive tasks for you.
By following these best practices, you will keep your AI models precise, dependable and ready to adjust to new data and altering circumstances.
Now that you’re equipped with best practices, let’s outline the strategies for effectively implementing retraining triggers.
Unleash smooth incorporation: Your Practical Guide to Deploying LLMs in Production.
Strategies for Implementing Retraining Triggers

Implementing retraining triggers efficiently indulges numerous key strategies to ensure that your AI models remain precise, dependable and up-to-date. Here are some strategies to contemplate:
Choose Relevant Metrics
To keep your AI model on track, you need to choose the right metric. Concentrate on those that directly affect your model’s performance. Perfection, accuracy, recall, and FI score are great starting points. Frequently monitor these metrics to spot any dips in performance, which signify the duration of retraining.
Spot the Need for Retraining
Information alters over time, and so should your model. Set up alerts for substantial shifts in data motifs or distributions. Use methods such as concept drift detection to recognize when your model’s forecasts are becoming less dependable. This dynamic approach helps you stay ahead and retrain before problems intensify.
Test and Verify Comprehensively
Before rolling out a new model and version, rigorously experiment with it. Separate your information into training and verifying sets and use cross-verification to ensure sturdy performance. Contrast the new model against the old one in a restrained environment. Once it clears all tests, you can positively deploy it to production, knowing it’s ready to handle real-globe information.
Discover the power of Artificial Intelligence: Practical Strategies for Self-Hosting Large Language Models Today!
Conclusion
Determining triggers for retraining is a crucial step in sustaining AI model performance. By constantly observing performance metrics, data distribution methods, and human-in-the-loop signals, you can ensure your models stay precise and efficient. Enfold a constant cycle of monitoring, triggering, and retraining to keep your AI models at the top of their game, ensuring endurance and dependability in a continuously developing environment.
Revolutionize your testing productivity today with RagaAI’s instinctive platform. Sign up now for a free trial!
Ever wonder why your AI models can underperform over time? Knowing why model retraining matters is key in AI. Your AI models need to adapt to new information and changes. You need to identify when retraining your AI models is needed.
Now that you understand why retraining is crucial let’s dive into the specifics of when and how to approach it.
Discover Real-World AI Model Testing Perceptions with Our In-Depth Case Studies in "In-Depth Case Studies in AI Model Testing: Exploring Real-World Applications and Insights."
The Necessity of Retraining AI Models
Updating your AI models is crucial for their efficiency. Let's look at two main reasons to retrain them.
Maintaining Predictive Accuracy Amid Data and Concept Drift
Think of your AI model as a detective spotting clues. However, the globe keeps altering, and new types of testimony pop up that our detective has seen before. This is what we call information and concept drift. Your model’s auguring precision can take a hit if it doesn't adjust to these alterations. Retraining your AI model ensures it stays fine-edged and pertinent, ready to tackle the newest challenges and deliver precise forecasts.
Incorporating Current Data
Data terrains are always progressing. New information comes in, reflecting the latest trends, behaviors and motifs. If your AI model doesn’t integrate this fresh information, it becomes outdated, such as an old map to traverse a modern city. By retraining your AI model, you ensure it remains current and proficient in making choices based on the most recent data. This perpetual learning process keeps your model affiliated with the real globe, improving its performance and dependability.
Retraining your AI model is not just a technical requisite; it’s a way to ensure your model remains a valuable asset in a volatile environment. Stay proactive with model retraining triggers to keep your models precise and up-to-date.
Let’s break down two of the most significant challenges you’ll face: concept drift and data drift.
Ready to ensure your AI tasks are sturdy and dependable? Begin Implementing Regression Tests in AI Development today to protect your AI solutions from regression and ensure constant performance. Contact us now to locate how our expert AI testing services can upgrade your evolution process.
Understanding Concept Drift and Data Drift
Concept drift and data drift are crucial factors that can affect the execution of your AI models. Let’s learn what these terms mean and how they affect your models.
Definition and Implications of Concept Drift
Concept drift refers to the phenomenon where the statistical properties of the target volatile alteration over time in unanticipated ways. In simpler terms, the motifs of your AI model learned initially may no longer hold true as new information appears. This can lead to a subside in forecast precision and dependability. For instance, in a financial predictive model, alterations in market behavior or regulations could indulge concept drift.
Challenges of Data Drift and Its Impact on Model Accuracy
Data drift occurs when the distribution of your input data alters over time, causing your model to become less efficient. This can happen due to numerous reasons like alterations in user behavior, environmental factors, or data gathering techniques. For example, if you’re using an AI model to forecast customer preferences based on social media information, shifts in user demographics or platform algorithms can introduce data drift.
Maintaining Model Performance

To maintain optimal performance, it's important to observe for signs of concept drift and data drift frequently. Here are the steps you take:
Continuous Monitoring: Execute monitoring systems that trace model performance metrics and locate divergence that may specify drift.
Flexible Learning: Integrate techniques such as online learning or gradual training that permit your model to adjust new motifs without full retraining.
Trigger-Based Retraining: Set verges or triggers based on performance metrics. When these thresholds are crossed, it beacons the need for retraining to capture new motifs efficiently.
By comprehending and proactively acknowledging concept drift and data drift, you can ensure that your AI model sustains their precision and pertinence over time. Frequent updates and adjustments will keep your models affiliated with the progressing real-globe synopsis they are designed to elucidate.
Speaking of staying ahead, let’s explore how to identify the key triggers that signal when it’s time to retrain your models.
Ready to boost your AI-powered applications and systems? Discover the Strategic Approach To Testing AI-powered applications And Systems. Don’t leave your AI solutions to chance; take command with our expert guidance.
Identifying Retraining Triggers
Keeping your AI models performing at their best needs alertness and prompt mediation. Knowing when to preserve your models is critical. Here are some key triggers to watch for:
Performance Metrics as Indicators for Retraining
Pay close attention to your performance metrics. Metrics such as precision, FI score and RMSE (Root Mean Square Error) give clear indications of your model’s health. If you observe a substantial drop in these numbers, it’s a strong sign that your model might require retraining. Frequently monitoring these metrics helps you catch problems early, ensuring your AI persists in delivering dependable outcomes.
Data Distribution Statistics and Prediction Difference Rate
Alterations in data distribution can be delicate. Monitor your data for shifts in distribution statistics, like mean, variance, or class proportions. In addition, trace the forecast distinction rate, which gauges the variance between forecasted and actual values over time. Sudden spikes or continuous drifts in these indicators suggest that your model’s assumptions about the information may no longer hold true, beckoning the requirement for retraining.
Human-in-Loop Signals and Flagged Predictions
Human-in-the-loop systems give valuable responses. When users constantly flag certain forecasts as incorrect or questionable, it’s a clear sign that the model might be losing its edge. Integrate this response loop to determine difficult areas that need retraining. Frequently retrospecting and acting on these flagged forecasts helps sustain your model’s pertinence and precision.
Monitoring for Changes in Statistical Distribution in Data
Statistical alterations in your data can creep in discreetly, impacting model’s performance. Enforce constant monitoring systems to locate any shifts in data distribution. Look for alterations in key statistical measures such as mean, standard deviation, and distribution shapes. By determining these alterations early, you can decide whether your model needs to be retrained to sustain the new data attributes.
By staying proactive and observing these triggers, you can ensure your AI models stay efficient and precise. Frequent retraining based on these cues will aid you.
So, when exactly should you retrain, and which methods should you consider? Let’s take a closer look.
Discover Best Practices For Testing Computer Vision Models – Improve Precision Today!
Approaches to Model Retraining
In the hasty world of Artificial Intelligence, keeping your model performing at its best requires frequent attention. One critical aspect of sustaining the model's performance is knowing when to retrain it. Let’s learn about the distinct approaches to model retraining, concentrating on the distinction between fixed retraining tempo and dynamic retraining, the advantages and disadvantages of schedule-based retraining, and the merits of trigger-based retraining.
Difference Between Fixed Retraining Cadence and Dynamic Retraining
You have two predominant strategies when it comes to retraining your models: fixed retraining cadence and dynamic retraining.
Fixed Retraining Cadence: This approach indulges retraining your models at standard, predetermined intervals. For instance, you might choose to retrain every month, quarter, or year, regardless of how your model is working. The benefit here is modesty and consistency. You know exactly when the next retraining session will occur, making it easier to strategize and distribute resources.
Dynamic Retraining: Unlike the fixed schedule, dynamic retraining is more adaptable and receptive to your model’s performance. You observe your model’s performance metrics constantly and retrain only when required. This approach ensures that you acknowledge performance dips or alterations in data allocation instantly. It needs more sophisticated monitoring and evaluation procedures but can result in more effective resource use and better model performance.
Schedule-Based Retraining: Its Simplicity and Limitations
Schedule-Based Retraining is elementary and easy to enforce. By sticking to a fixed schedule, you can strategize your resources and outlines without any amazements. Here’s why it is appealing:
Simplicity: You don’t require intricate monitoring systems. Just mark your calendar and retrain on the set date.
Predictability: You can forecast time and resources well in advance, ensuring that your team is ready for each retraining session.
Routine Maintenance: Frequent retraining can help catch any steady performance degradation over time.
However there are renowned limitations:
Inefficiency: You might retrain models even when it’s not mandatory, frittering time and resources.
Delayed Response: If you model performance drops substantially between anticipated retrainings, you can suffer from poor outcomes until the next session.
Static Approach: This technique doesn’t adjust to the real-time requirements of your models, certainly resulting in periods of unacceptable performance.
Trigger-Based Retraining: Responsive and Adaptive Approach to Retraining
Trigger-Based Retraining is a more sophisticated and receptive method. Instead of following a fixed schedule, you retrain your models based on precise triggers or performance metrics. This approach is highly flexible and ensures your models remain in top shape.
Responsiveness: You retrain your models only where there’s a clear requirement, like a drop in precision, alterations in data motifs, or the introduction of new data attributes. This keeps your model up-to-date and executing well.
Effectiveness: By retraining only when significant, you upgrade the use of your resources, saving time and effort.
Flexibility: This technique permits you to swiftly adjust to new trends or information shifts, sustaining high performance in changing environments.
However, enforcing trigger-based retraining requires:
Continuous Monitoring: You need sturdy systems to trace your model’s performance metrics and locate when they fall below adequate thresholds.
Complex Infrastructure: Setting up and sustaining the essential infrastructure for trigger-based retraining can be intricate and resource-intensive.
Selecting the right approach to model retraining.relies on your precise requirements and resources. Schedule-based retraining provides clarity and consistency but may lead to ineffectiveness. On the contrary, trigger-based retraining offers a receptive and compatible solution, ensuring your models perform perfectly at all times. By comprehending these approaches, you make informed decisions to sustain and improve the performance of your AI models.
Knowing the approaches is great, but let’s ensure you’re following the best practices to maximize the efficiency of your model retraining.
Want to know about Deep learning applications? Check out our detailed guide on Exploring The Frontiers Of Deep Learning Applications.
Best Practices in Retraining AI Models
Retraining AI models is a crucial practice to ensure their precision, pertinence, and performance over time. Here are some best practices to contemplate:
Monitor Multiple Trigger Metrics to Robustly Detect Drift
You can observe numerous metrics to detect drift efficiently. Don’t just depend on a single metric; an amalgamation gives you a coherent picture. Think of it like observing distinct integral signs for a patient. If one goes off, it could specify an issue, but if numerous do, you know something is surely wrong.
Minimize Operational Impact with Asynchronous Retraining
Retraining models can be resource-intensive, but you can avoid disrupting your daily functioning by doing it asynchronously. Schedule training during off-peak hours or run it in the background. This way, you predominant systems stay genuine, and you sustain smooth functioning.
Continuous Testing of Retrained Models in Pre-Production
Before you roll out a retrained model, put it through rigid testing in a pre-production environment. This step ensures that the new model works well under numerous synopsis and doesn’t acquaint any unanticipated problems. It’s like going on a test drive before purchasing the car.
Maintain Dataset Snapshots for Reproducible Retraining
Always save screenshots of your training datasets. This practice ensures that you can reproduce the training process if required. Suppose it is saving distinct versions of a document you’re operating on; if something goes erroneous, you can always switch to the previous version.
Validate Retrained Models for Stability Before Deployment
Before deploying a retrained model, verify it comprehensively. This step assures that the model is steady and dependable. Think of it as cross-verifying your work before handing over an important report; you want to make sure everything is precise.
Automate Retraining for Efficiency and Scalability
Automation is your best buddy when it comes to retraining AI models. Set up automated assignments to handle retraining tasks. This approach not only saves time but also ensures steadiness and configurability. It’s like having a dependable assistant who takes care of repetitive tasks for you.
By following these best practices, you will keep your AI models precise, dependable and ready to adjust to new data and altering circumstances.
Now that you’re equipped with best practices, let’s outline the strategies for effectively implementing retraining triggers.
Unleash smooth incorporation: Your Practical Guide to Deploying LLMs in Production.
Strategies for Implementing Retraining Triggers

Implementing retraining triggers efficiently indulges numerous key strategies to ensure that your AI models remain precise, dependable and up-to-date. Here are some strategies to contemplate:
Choose Relevant Metrics
To keep your AI model on track, you need to choose the right metric. Concentrate on those that directly affect your model’s performance. Perfection, accuracy, recall, and FI score are great starting points. Frequently monitor these metrics to spot any dips in performance, which signify the duration of retraining.
Spot the Need for Retraining
Information alters over time, and so should your model. Set up alerts for substantial shifts in data motifs or distributions. Use methods such as concept drift detection to recognize when your model’s forecasts are becoming less dependable. This dynamic approach helps you stay ahead and retrain before problems intensify.
Test and Verify Comprehensively
Before rolling out a new model and version, rigorously experiment with it. Separate your information into training and verifying sets and use cross-verification to ensure sturdy performance. Contrast the new model against the old one in a restrained environment. Once it clears all tests, you can positively deploy it to production, knowing it’s ready to handle real-globe information.
Discover the power of Artificial Intelligence: Practical Strategies for Self-Hosting Large Language Models Today!
Conclusion
Determining triggers for retraining is a crucial step in sustaining AI model performance. By constantly observing performance metrics, data distribution methods, and human-in-the-loop signals, you can ensure your models stay precise and efficient. Enfold a constant cycle of monitoring, triggering, and retraining to keep your AI models at the top of their game, ensuring endurance and dependability in a continuously developing environment.
Revolutionize your testing productivity today with RagaAI’s instinctive platform. Sign up now for a free trial!
Ever wonder why your AI models can underperform over time? Knowing why model retraining matters is key in AI. Your AI models need to adapt to new information and changes. You need to identify when retraining your AI models is needed.
Now that you understand why retraining is crucial let’s dive into the specifics of when and how to approach it.
Discover Real-World AI Model Testing Perceptions with Our In-Depth Case Studies in "In-Depth Case Studies in AI Model Testing: Exploring Real-World Applications and Insights."
The Necessity of Retraining AI Models
Updating your AI models is crucial for their efficiency. Let's look at two main reasons to retrain them.
Maintaining Predictive Accuracy Amid Data and Concept Drift
Think of your AI model as a detective spotting clues. However, the globe keeps altering, and new types of testimony pop up that our detective has seen before. This is what we call information and concept drift. Your model’s auguring precision can take a hit if it doesn't adjust to these alterations. Retraining your AI model ensures it stays fine-edged and pertinent, ready to tackle the newest challenges and deliver precise forecasts.
Incorporating Current Data
Data terrains are always progressing. New information comes in, reflecting the latest trends, behaviors and motifs. If your AI model doesn’t integrate this fresh information, it becomes outdated, such as an old map to traverse a modern city. By retraining your AI model, you ensure it remains current and proficient in making choices based on the most recent data. This perpetual learning process keeps your model affiliated with the real globe, improving its performance and dependability.
Retraining your AI model is not just a technical requisite; it’s a way to ensure your model remains a valuable asset in a volatile environment. Stay proactive with model retraining triggers to keep your models precise and up-to-date.
Let’s break down two of the most significant challenges you’ll face: concept drift and data drift.
Ready to ensure your AI tasks are sturdy and dependable? Begin Implementing Regression Tests in AI Development today to protect your AI solutions from regression and ensure constant performance. Contact us now to locate how our expert AI testing services can upgrade your evolution process.
Understanding Concept Drift and Data Drift
Concept drift and data drift are crucial factors that can affect the execution of your AI models. Let’s learn what these terms mean and how they affect your models.
Definition and Implications of Concept Drift
Concept drift refers to the phenomenon where the statistical properties of the target volatile alteration over time in unanticipated ways. In simpler terms, the motifs of your AI model learned initially may no longer hold true as new information appears. This can lead to a subside in forecast precision and dependability. For instance, in a financial predictive model, alterations in market behavior or regulations could indulge concept drift.
Challenges of Data Drift and Its Impact on Model Accuracy
Data drift occurs when the distribution of your input data alters over time, causing your model to become less efficient. This can happen due to numerous reasons like alterations in user behavior, environmental factors, or data gathering techniques. For example, if you’re using an AI model to forecast customer preferences based on social media information, shifts in user demographics or platform algorithms can introduce data drift.
Maintaining Model Performance

To maintain optimal performance, it's important to observe for signs of concept drift and data drift frequently. Here are the steps you take:
Continuous Monitoring: Execute monitoring systems that trace model performance metrics and locate divergence that may specify drift.
Flexible Learning: Integrate techniques such as online learning or gradual training that permit your model to adjust new motifs without full retraining.
Trigger-Based Retraining: Set verges or triggers based on performance metrics. When these thresholds are crossed, it beacons the need for retraining to capture new motifs efficiently.
By comprehending and proactively acknowledging concept drift and data drift, you can ensure that your AI model sustains their precision and pertinence over time. Frequent updates and adjustments will keep your models affiliated with the progressing real-globe synopsis they are designed to elucidate.
Speaking of staying ahead, let’s explore how to identify the key triggers that signal when it’s time to retrain your models.
Ready to boost your AI-powered applications and systems? Discover the Strategic Approach To Testing AI-powered applications And Systems. Don’t leave your AI solutions to chance; take command with our expert guidance.
Identifying Retraining Triggers
Keeping your AI models performing at their best needs alertness and prompt mediation. Knowing when to preserve your models is critical. Here are some key triggers to watch for:
Performance Metrics as Indicators for Retraining
Pay close attention to your performance metrics. Metrics such as precision, FI score and RMSE (Root Mean Square Error) give clear indications of your model’s health. If you observe a substantial drop in these numbers, it’s a strong sign that your model might require retraining. Frequently monitoring these metrics helps you catch problems early, ensuring your AI persists in delivering dependable outcomes.
Data Distribution Statistics and Prediction Difference Rate
Alterations in data distribution can be delicate. Monitor your data for shifts in distribution statistics, like mean, variance, or class proportions. In addition, trace the forecast distinction rate, which gauges the variance between forecasted and actual values over time. Sudden spikes or continuous drifts in these indicators suggest that your model’s assumptions about the information may no longer hold true, beckoning the requirement for retraining.
Human-in-Loop Signals and Flagged Predictions
Human-in-the-loop systems give valuable responses. When users constantly flag certain forecasts as incorrect or questionable, it’s a clear sign that the model might be losing its edge. Integrate this response loop to determine difficult areas that need retraining. Frequently retrospecting and acting on these flagged forecasts helps sustain your model’s pertinence and precision.
Monitoring for Changes in Statistical Distribution in Data
Statistical alterations in your data can creep in discreetly, impacting model’s performance. Enforce constant monitoring systems to locate any shifts in data distribution. Look for alterations in key statistical measures such as mean, standard deviation, and distribution shapes. By determining these alterations early, you can decide whether your model needs to be retrained to sustain the new data attributes.
By staying proactive and observing these triggers, you can ensure your AI models stay efficient and precise. Frequent retraining based on these cues will aid you.
So, when exactly should you retrain, and which methods should you consider? Let’s take a closer look.
Discover Best Practices For Testing Computer Vision Models – Improve Precision Today!
Approaches to Model Retraining
In the hasty world of Artificial Intelligence, keeping your model performing at its best requires frequent attention. One critical aspect of sustaining the model's performance is knowing when to retrain it. Let’s learn about the distinct approaches to model retraining, concentrating on the distinction between fixed retraining tempo and dynamic retraining, the advantages and disadvantages of schedule-based retraining, and the merits of trigger-based retraining.
Difference Between Fixed Retraining Cadence and Dynamic Retraining
You have two predominant strategies when it comes to retraining your models: fixed retraining cadence and dynamic retraining.
Fixed Retraining Cadence: This approach indulges retraining your models at standard, predetermined intervals. For instance, you might choose to retrain every month, quarter, or year, regardless of how your model is working. The benefit here is modesty and consistency. You know exactly when the next retraining session will occur, making it easier to strategize and distribute resources.
Dynamic Retraining: Unlike the fixed schedule, dynamic retraining is more adaptable and receptive to your model’s performance. You observe your model’s performance metrics constantly and retrain only when required. This approach ensures that you acknowledge performance dips or alterations in data allocation instantly. It needs more sophisticated monitoring and evaluation procedures but can result in more effective resource use and better model performance.
Schedule-Based Retraining: Its Simplicity and Limitations
Schedule-Based Retraining is elementary and easy to enforce. By sticking to a fixed schedule, you can strategize your resources and outlines without any amazements. Here’s why it is appealing:
Simplicity: You don’t require intricate monitoring systems. Just mark your calendar and retrain on the set date.
Predictability: You can forecast time and resources well in advance, ensuring that your team is ready for each retraining session.
Routine Maintenance: Frequent retraining can help catch any steady performance degradation over time.
However there are renowned limitations:
Inefficiency: You might retrain models even when it’s not mandatory, frittering time and resources.
Delayed Response: If you model performance drops substantially between anticipated retrainings, you can suffer from poor outcomes until the next session.
Static Approach: This technique doesn’t adjust to the real-time requirements of your models, certainly resulting in periods of unacceptable performance.
Trigger-Based Retraining: Responsive and Adaptive Approach to Retraining
Trigger-Based Retraining is a more sophisticated and receptive method. Instead of following a fixed schedule, you retrain your models based on precise triggers or performance metrics. This approach is highly flexible and ensures your models remain in top shape.
Responsiveness: You retrain your models only where there’s a clear requirement, like a drop in precision, alterations in data motifs, or the introduction of new data attributes. This keeps your model up-to-date and executing well.
Effectiveness: By retraining only when significant, you upgrade the use of your resources, saving time and effort.
Flexibility: This technique permits you to swiftly adjust to new trends or information shifts, sustaining high performance in changing environments.
However, enforcing trigger-based retraining requires:
Continuous Monitoring: You need sturdy systems to trace your model’s performance metrics and locate when they fall below adequate thresholds.
Complex Infrastructure: Setting up and sustaining the essential infrastructure for trigger-based retraining can be intricate and resource-intensive.
Selecting the right approach to model retraining.relies on your precise requirements and resources. Schedule-based retraining provides clarity and consistency but may lead to ineffectiveness. On the contrary, trigger-based retraining offers a receptive and compatible solution, ensuring your models perform perfectly at all times. By comprehending these approaches, you make informed decisions to sustain and improve the performance of your AI models.
Knowing the approaches is great, but let’s ensure you’re following the best practices to maximize the efficiency of your model retraining.
Want to know about Deep learning applications? Check out our detailed guide on Exploring The Frontiers Of Deep Learning Applications.
Best Practices in Retraining AI Models
Retraining AI models is a crucial practice to ensure their precision, pertinence, and performance over time. Here are some best practices to contemplate:
Monitor Multiple Trigger Metrics to Robustly Detect Drift
You can observe numerous metrics to detect drift efficiently. Don’t just depend on a single metric; an amalgamation gives you a coherent picture. Think of it like observing distinct integral signs for a patient. If one goes off, it could specify an issue, but if numerous do, you know something is surely wrong.
Minimize Operational Impact with Asynchronous Retraining
Retraining models can be resource-intensive, but you can avoid disrupting your daily functioning by doing it asynchronously. Schedule training during off-peak hours or run it in the background. This way, you predominant systems stay genuine, and you sustain smooth functioning.
Continuous Testing of Retrained Models in Pre-Production
Before you roll out a retrained model, put it through rigid testing in a pre-production environment. This step ensures that the new model works well under numerous synopsis and doesn’t acquaint any unanticipated problems. It’s like going on a test drive before purchasing the car.
Maintain Dataset Snapshots for Reproducible Retraining
Always save screenshots of your training datasets. This practice ensures that you can reproduce the training process if required. Suppose it is saving distinct versions of a document you’re operating on; if something goes erroneous, you can always switch to the previous version.
Validate Retrained Models for Stability Before Deployment
Before deploying a retrained model, verify it comprehensively. This step assures that the model is steady and dependable. Think of it as cross-verifying your work before handing over an important report; you want to make sure everything is precise.
Automate Retraining for Efficiency and Scalability
Automation is your best buddy when it comes to retraining AI models. Set up automated assignments to handle retraining tasks. This approach not only saves time but also ensures steadiness and configurability. It’s like having a dependable assistant who takes care of repetitive tasks for you.
By following these best practices, you will keep your AI models precise, dependable and ready to adjust to new data and altering circumstances.
Now that you’re equipped with best practices, let’s outline the strategies for effectively implementing retraining triggers.
Unleash smooth incorporation: Your Practical Guide to Deploying LLMs in Production.
Strategies for Implementing Retraining Triggers

Implementing retraining triggers efficiently indulges numerous key strategies to ensure that your AI models remain precise, dependable and up-to-date. Here are some strategies to contemplate:
Choose Relevant Metrics
To keep your AI model on track, you need to choose the right metric. Concentrate on those that directly affect your model’s performance. Perfection, accuracy, recall, and FI score are great starting points. Frequently monitor these metrics to spot any dips in performance, which signify the duration of retraining.
Spot the Need for Retraining
Information alters over time, and so should your model. Set up alerts for substantial shifts in data motifs or distributions. Use methods such as concept drift detection to recognize when your model’s forecasts are becoming less dependable. This dynamic approach helps you stay ahead and retrain before problems intensify.
Test and Verify Comprehensively
Before rolling out a new model and version, rigorously experiment with it. Separate your information into training and verifying sets and use cross-verification to ensure sturdy performance. Contrast the new model against the old one in a restrained environment. Once it clears all tests, you can positively deploy it to production, knowing it’s ready to handle real-globe information.
Discover the power of Artificial Intelligence: Practical Strategies for Self-Hosting Large Language Models Today!
Conclusion
Determining triggers for retraining is a crucial step in sustaining AI model performance. By constantly observing performance metrics, data distribution methods, and human-in-the-loop signals, you can ensure your models stay precise and efficient. Enfold a constant cycle of monitoring, triggering, and retraining to keep your AI models at the top of their game, ensuring endurance and dependability in a continuously developing environment.
Revolutionize your testing productivity today with RagaAI’s instinctive platform. Sign up now for a free trial!
Ever wonder why your AI models can underperform over time? Knowing why model retraining matters is key in AI. Your AI models need to adapt to new information and changes. You need to identify when retraining your AI models is needed.
Now that you understand why retraining is crucial let’s dive into the specifics of when and how to approach it.
Discover Real-World AI Model Testing Perceptions with Our In-Depth Case Studies in "In-Depth Case Studies in AI Model Testing: Exploring Real-World Applications and Insights."
The Necessity of Retraining AI Models
Updating your AI models is crucial for their efficiency. Let's look at two main reasons to retrain them.
Maintaining Predictive Accuracy Amid Data and Concept Drift
Think of your AI model as a detective spotting clues. However, the globe keeps altering, and new types of testimony pop up that our detective has seen before. This is what we call information and concept drift. Your model’s auguring precision can take a hit if it doesn't adjust to these alterations. Retraining your AI model ensures it stays fine-edged and pertinent, ready to tackle the newest challenges and deliver precise forecasts.
Incorporating Current Data
Data terrains are always progressing. New information comes in, reflecting the latest trends, behaviors and motifs. If your AI model doesn’t integrate this fresh information, it becomes outdated, such as an old map to traverse a modern city. By retraining your AI model, you ensure it remains current and proficient in making choices based on the most recent data. This perpetual learning process keeps your model affiliated with the real globe, improving its performance and dependability.
Retraining your AI model is not just a technical requisite; it’s a way to ensure your model remains a valuable asset in a volatile environment. Stay proactive with model retraining triggers to keep your models precise and up-to-date.
Let’s break down two of the most significant challenges you’ll face: concept drift and data drift.
Ready to ensure your AI tasks are sturdy and dependable? Begin Implementing Regression Tests in AI Development today to protect your AI solutions from regression and ensure constant performance. Contact us now to locate how our expert AI testing services can upgrade your evolution process.
Understanding Concept Drift and Data Drift
Concept drift and data drift are crucial factors that can affect the execution of your AI models. Let’s learn what these terms mean and how they affect your models.
Definition and Implications of Concept Drift
Concept drift refers to the phenomenon where the statistical properties of the target volatile alteration over time in unanticipated ways. In simpler terms, the motifs of your AI model learned initially may no longer hold true as new information appears. This can lead to a subside in forecast precision and dependability. For instance, in a financial predictive model, alterations in market behavior or regulations could indulge concept drift.
Challenges of Data Drift and Its Impact on Model Accuracy
Data drift occurs when the distribution of your input data alters over time, causing your model to become less efficient. This can happen due to numerous reasons like alterations in user behavior, environmental factors, or data gathering techniques. For example, if you’re using an AI model to forecast customer preferences based on social media information, shifts in user demographics or platform algorithms can introduce data drift.
Maintaining Model Performance

To maintain optimal performance, it's important to observe for signs of concept drift and data drift frequently. Here are the steps you take:
Continuous Monitoring: Execute monitoring systems that trace model performance metrics and locate divergence that may specify drift.
Flexible Learning: Integrate techniques such as online learning or gradual training that permit your model to adjust new motifs without full retraining.
Trigger-Based Retraining: Set verges or triggers based on performance metrics. When these thresholds are crossed, it beacons the need for retraining to capture new motifs efficiently.
By comprehending and proactively acknowledging concept drift and data drift, you can ensure that your AI model sustains their precision and pertinence over time. Frequent updates and adjustments will keep your models affiliated with the progressing real-globe synopsis they are designed to elucidate.
Speaking of staying ahead, let’s explore how to identify the key triggers that signal when it’s time to retrain your models.
Ready to boost your AI-powered applications and systems? Discover the Strategic Approach To Testing AI-powered applications And Systems. Don’t leave your AI solutions to chance; take command with our expert guidance.
Identifying Retraining Triggers
Keeping your AI models performing at their best needs alertness and prompt mediation. Knowing when to preserve your models is critical. Here are some key triggers to watch for:
Performance Metrics as Indicators for Retraining
Pay close attention to your performance metrics. Metrics such as precision, FI score and RMSE (Root Mean Square Error) give clear indications of your model’s health. If you observe a substantial drop in these numbers, it’s a strong sign that your model might require retraining. Frequently monitoring these metrics helps you catch problems early, ensuring your AI persists in delivering dependable outcomes.
Data Distribution Statistics and Prediction Difference Rate
Alterations in data distribution can be delicate. Monitor your data for shifts in distribution statistics, like mean, variance, or class proportions. In addition, trace the forecast distinction rate, which gauges the variance between forecasted and actual values over time. Sudden spikes or continuous drifts in these indicators suggest that your model’s assumptions about the information may no longer hold true, beckoning the requirement for retraining.
Human-in-Loop Signals and Flagged Predictions
Human-in-the-loop systems give valuable responses. When users constantly flag certain forecasts as incorrect or questionable, it’s a clear sign that the model might be losing its edge. Integrate this response loop to determine difficult areas that need retraining. Frequently retrospecting and acting on these flagged forecasts helps sustain your model’s pertinence and precision.
Monitoring for Changes in Statistical Distribution in Data
Statistical alterations in your data can creep in discreetly, impacting model’s performance. Enforce constant monitoring systems to locate any shifts in data distribution. Look for alterations in key statistical measures such as mean, standard deviation, and distribution shapes. By determining these alterations early, you can decide whether your model needs to be retrained to sustain the new data attributes.
By staying proactive and observing these triggers, you can ensure your AI models stay efficient and precise. Frequent retraining based on these cues will aid you.
So, when exactly should you retrain, and which methods should you consider? Let’s take a closer look.
Discover Best Practices For Testing Computer Vision Models – Improve Precision Today!
Approaches to Model Retraining
In the hasty world of Artificial Intelligence, keeping your model performing at its best requires frequent attention. One critical aspect of sustaining the model's performance is knowing when to retrain it. Let’s learn about the distinct approaches to model retraining, concentrating on the distinction between fixed retraining tempo and dynamic retraining, the advantages and disadvantages of schedule-based retraining, and the merits of trigger-based retraining.
Difference Between Fixed Retraining Cadence and Dynamic Retraining
You have two predominant strategies when it comes to retraining your models: fixed retraining cadence and dynamic retraining.
Fixed Retraining Cadence: This approach indulges retraining your models at standard, predetermined intervals. For instance, you might choose to retrain every month, quarter, or year, regardless of how your model is working. The benefit here is modesty and consistency. You know exactly when the next retraining session will occur, making it easier to strategize and distribute resources.
Dynamic Retraining: Unlike the fixed schedule, dynamic retraining is more adaptable and receptive to your model’s performance. You observe your model’s performance metrics constantly and retrain only when required. This approach ensures that you acknowledge performance dips or alterations in data allocation instantly. It needs more sophisticated monitoring and evaluation procedures but can result in more effective resource use and better model performance.
Schedule-Based Retraining: Its Simplicity and Limitations
Schedule-Based Retraining is elementary and easy to enforce. By sticking to a fixed schedule, you can strategize your resources and outlines without any amazements. Here’s why it is appealing:
Simplicity: You don’t require intricate monitoring systems. Just mark your calendar and retrain on the set date.
Predictability: You can forecast time and resources well in advance, ensuring that your team is ready for each retraining session.
Routine Maintenance: Frequent retraining can help catch any steady performance degradation over time.
However there are renowned limitations:
Inefficiency: You might retrain models even when it’s not mandatory, frittering time and resources.
Delayed Response: If you model performance drops substantially between anticipated retrainings, you can suffer from poor outcomes until the next session.
Static Approach: This technique doesn’t adjust to the real-time requirements of your models, certainly resulting in periods of unacceptable performance.
Trigger-Based Retraining: Responsive and Adaptive Approach to Retraining
Trigger-Based Retraining is a more sophisticated and receptive method. Instead of following a fixed schedule, you retrain your models based on precise triggers or performance metrics. This approach is highly flexible and ensures your models remain in top shape.
Responsiveness: You retrain your models only where there’s a clear requirement, like a drop in precision, alterations in data motifs, or the introduction of new data attributes. This keeps your model up-to-date and executing well.
Effectiveness: By retraining only when significant, you upgrade the use of your resources, saving time and effort.
Flexibility: This technique permits you to swiftly adjust to new trends or information shifts, sustaining high performance in changing environments.
However, enforcing trigger-based retraining requires:
Continuous Monitoring: You need sturdy systems to trace your model’s performance metrics and locate when they fall below adequate thresholds.
Complex Infrastructure: Setting up and sustaining the essential infrastructure for trigger-based retraining can be intricate and resource-intensive.
Selecting the right approach to model retraining.relies on your precise requirements and resources. Schedule-based retraining provides clarity and consistency but may lead to ineffectiveness. On the contrary, trigger-based retraining offers a receptive and compatible solution, ensuring your models perform perfectly at all times. By comprehending these approaches, you make informed decisions to sustain and improve the performance of your AI models.
Knowing the approaches is great, but let’s ensure you’re following the best practices to maximize the efficiency of your model retraining.
Want to know about Deep learning applications? Check out our detailed guide on Exploring The Frontiers Of Deep Learning Applications.
Best Practices in Retraining AI Models
Retraining AI models is a crucial practice to ensure their precision, pertinence, and performance over time. Here are some best practices to contemplate:
Monitor Multiple Trigger Metrics to Robustly Detect Drift
You can observe numerous metrics to detect drift efficiently. Don’t just depend on a single metric; an amalgamation gives you a coherent picture. Think of it like observing distinct integral signs for a patient. If one goes off, it could specify an issue, but if numerous do, you know something is surely wrong.
Minimize Operational Impact with Asynchronous Retraining
Retraining models can be resource-intensive, but you can avoid disrupting your daily functioning by doing it asynchronously. Schedule training during off-peak hours or run it in the background. This way, you predominant systems stay genuine, and you sustain smooth functioning.
Continuous Testing of Retrained Models in Pre-Production
Before you roll out a retrained model, put it through rigid testing in a pre-production environment. This step ensures that the new model works well under numerous synopsis and doesn’t acquaint any unanticipated problems. It’s like going on a test drive before purchasing the car.
Maintain Dataset Snapshots for Reproducible Retraining
Always save screenshots of your training datasets. This practice ensures that you can reproduce the training process if required. Suppose it is saving distinct versions of a document you’re operating on; if something goes erroneous, you can always switch to the previous version.
Validate Retrained Models for Stability Before Deployment
Before deploying a retrained model, verify it comprehensively. This step assures that the model is steady and dependable. Think of it as cross-verifying your work before handing over an important report; you want to make sure everything is precise.
Automate Retraining for Efficiency and Scalability
Automation is your best buddy when it comes to retraining AI models. Set up automated assignments to handle retraining tasks. This approach not only saves time but also ensures steadiness and configurability. It’s like having a dependable assistant who takes care of repetitive tasks for you.
By following these best practices, you will keep your AI models precise, dependable and ready to adjust to new data and altering circumstances.
Now that you’re equipped with best practices, let’s outline the strategies for effectively implementing retraining triggers.
Unleash smooth incorporation: Your Practical Guide to Deploying LLMs in Production.
Strategies for Implementing Retraining Triggers

Implementing retraining triggers efficiently indulges numerous key strategies to ensure that your AI models remain precise, dependable and up-to-date. Here are some strategies to contemplate:
Choose Relevant Metrics
To keep your AI model on track, you need to choose the right metric. Concentrate on those that directly affect your model’s performance. Perfection, accuracy, recall, and FI score are great starting points. Frequently monitor these metrics to spot any dips in performance, which signify the duration of retraining.
Spot the Need for Retraining
Information alters over time, and so should your model. Set up alerts for substantial shifts in data motifs or distributions. Use methods such as concept drift detection to recognize when your model’s forecasts are becoming less dependable. This dynamic approach helps you stay ahead and retrain before problems intensify.
Test and Verify Comprehensively
Before rolling out a new model and version, rigorously experiment with it. Separate your information into training and verifying sets and use cross-verification to ensure sturdy performance. Contrast the new model against the old one in a restrained environment. Once it clears all tests, you can positively deploy it to production, knowing it’s ready to handle real-globe information.
Discover the power of Artificial Intelligence: Practical Strategies for Self-Hosting Large Language Models Today!
Conclusion
Determining triggers for retraining is a crucial step in sustaining AI model performance. By constantly observing performance metrics, data distribution methods, and human-in-the-loop signals, you can ensure your models stay precise and efficient. Enfold a constant cycle of monitoring, triggering, and retraining to keep your AI models at the top of their game, ensuring endurance and dependability in a continuously developing environment.
Revolutionize your testing productivity today with RagaAI’s instinctive platform. Sign up now for a free trial!
Subscribe to our newsletter to never miss an update
Other articles


Agents Talk, Models Think: A2A + MCP for Enterprise Agentic AI

Riya Parikh, Nitai Agarwal
Apr 27, 2025
Read the article
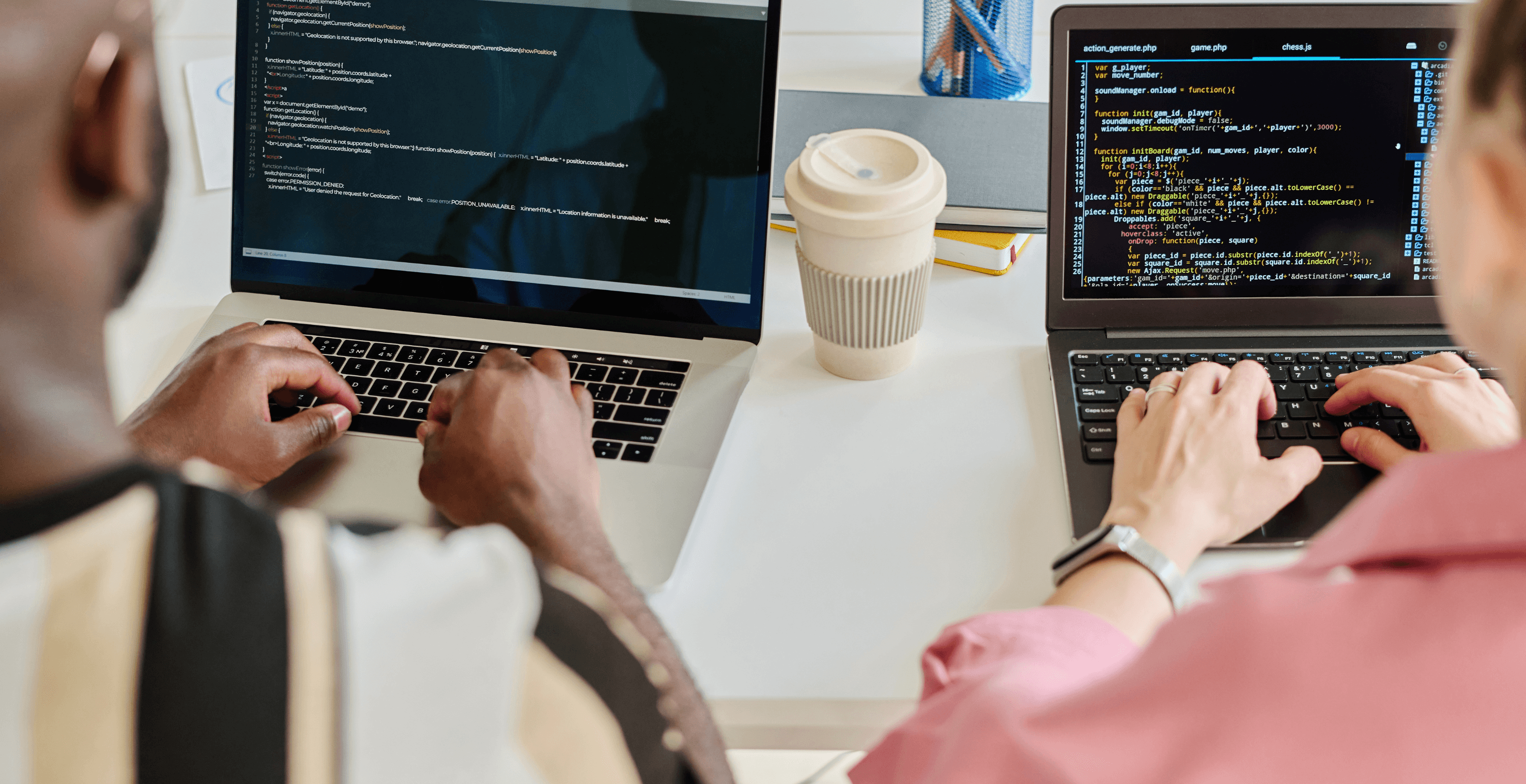
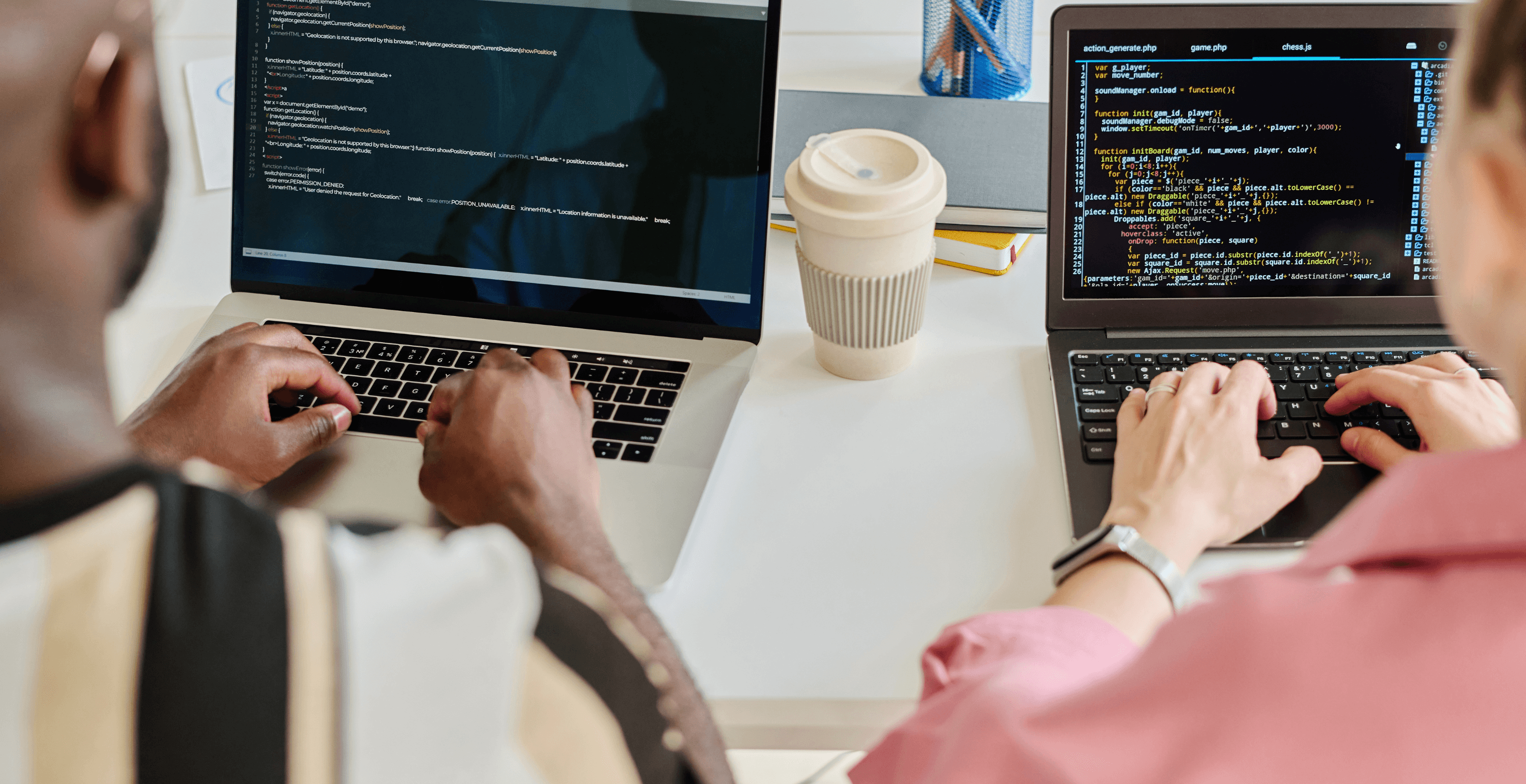
Understanding NeMo Guardrails: A Toolkit for LLM Security

Rehan Asif
Dec 24, 2024
Read the article


Understanding Differences in Large vs Small Language Models (LLM vs SLM)

Rehan Asif
Dec 21, 2024
Read the article