Practical Strategies For Self-Hosting Large Language Models
Practical Strategies For Self-Hosting Large Language Models
Practical Strategies For Self-Hosting Large Language Models
Rehan Asif
Jun 12, 2024
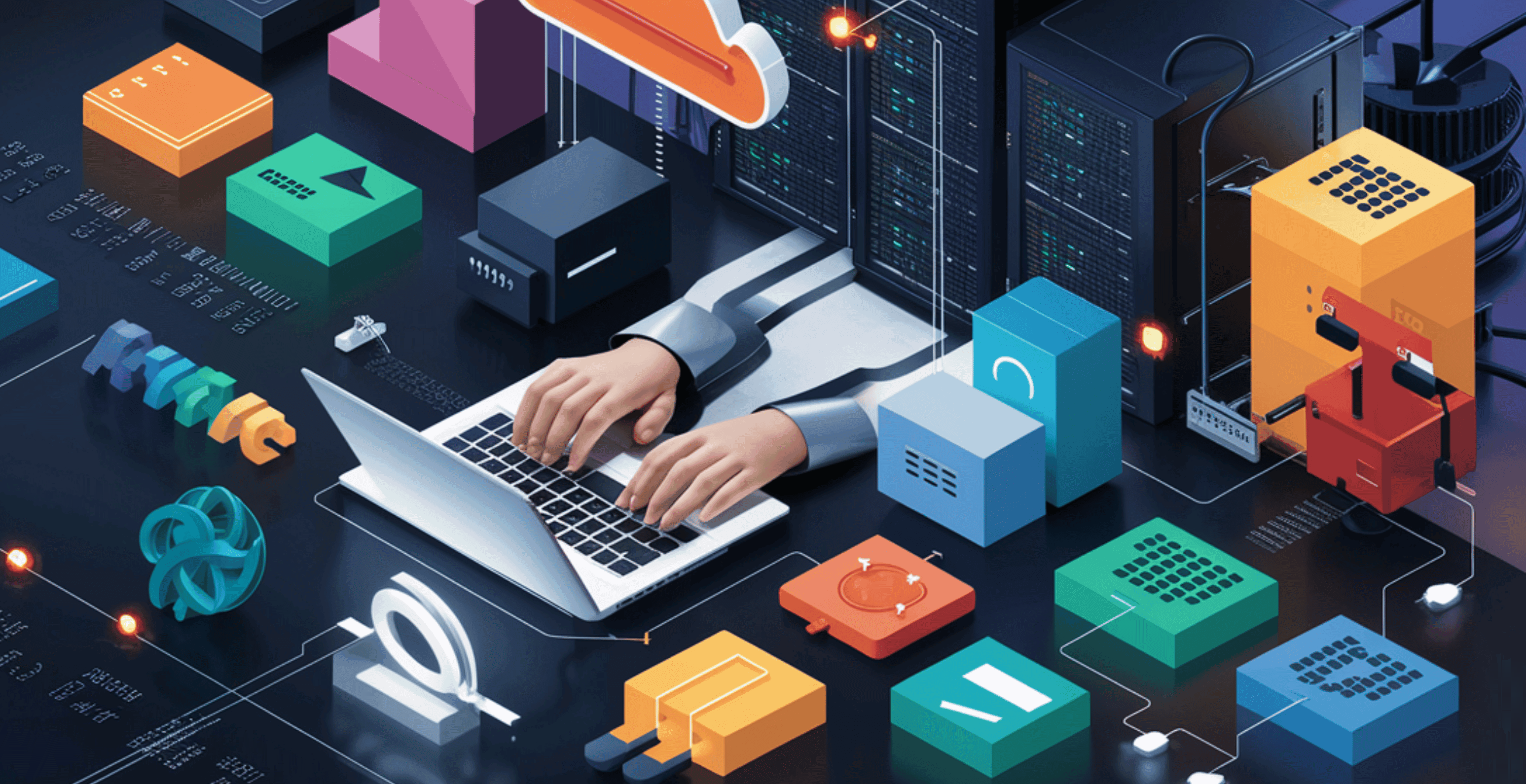
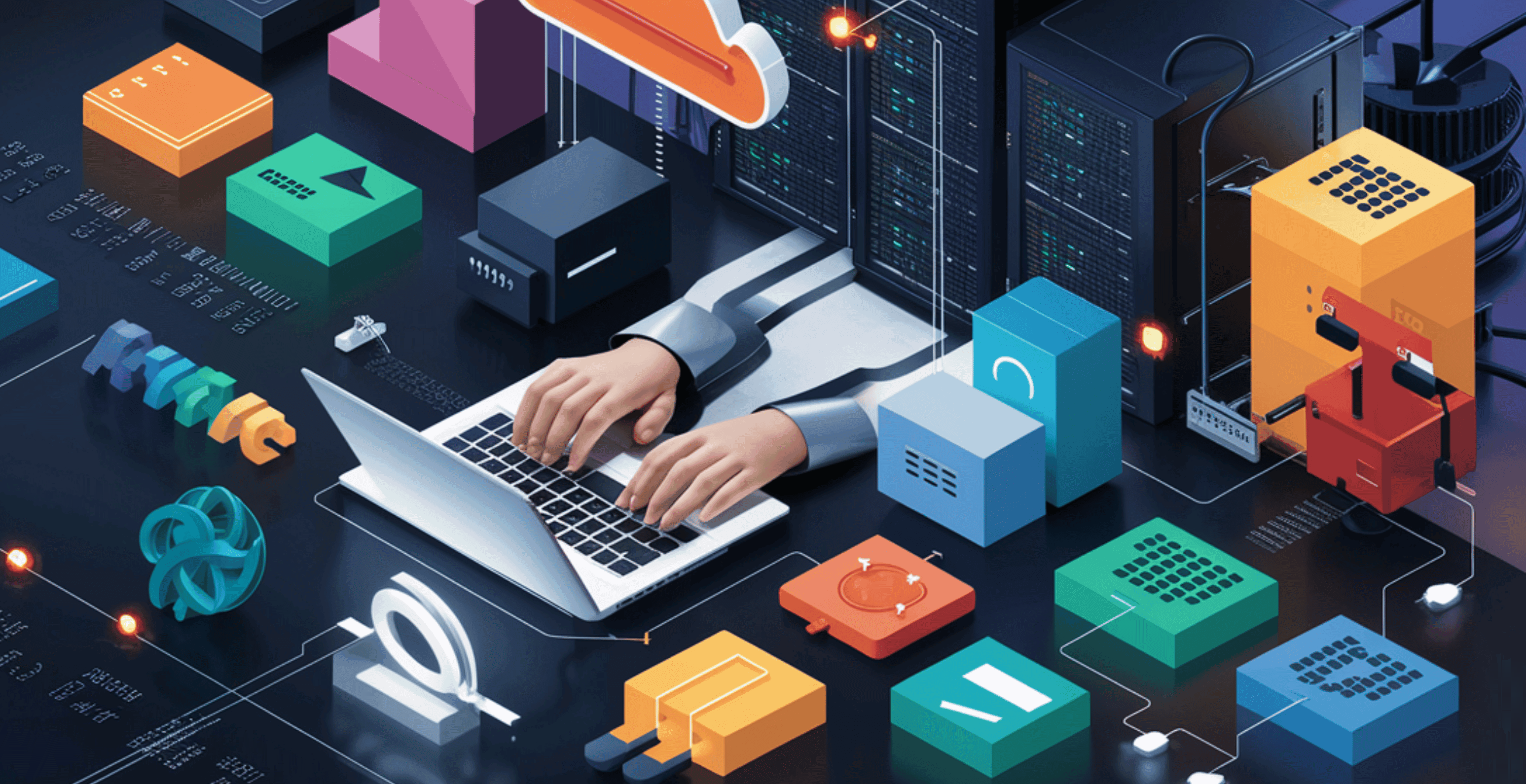
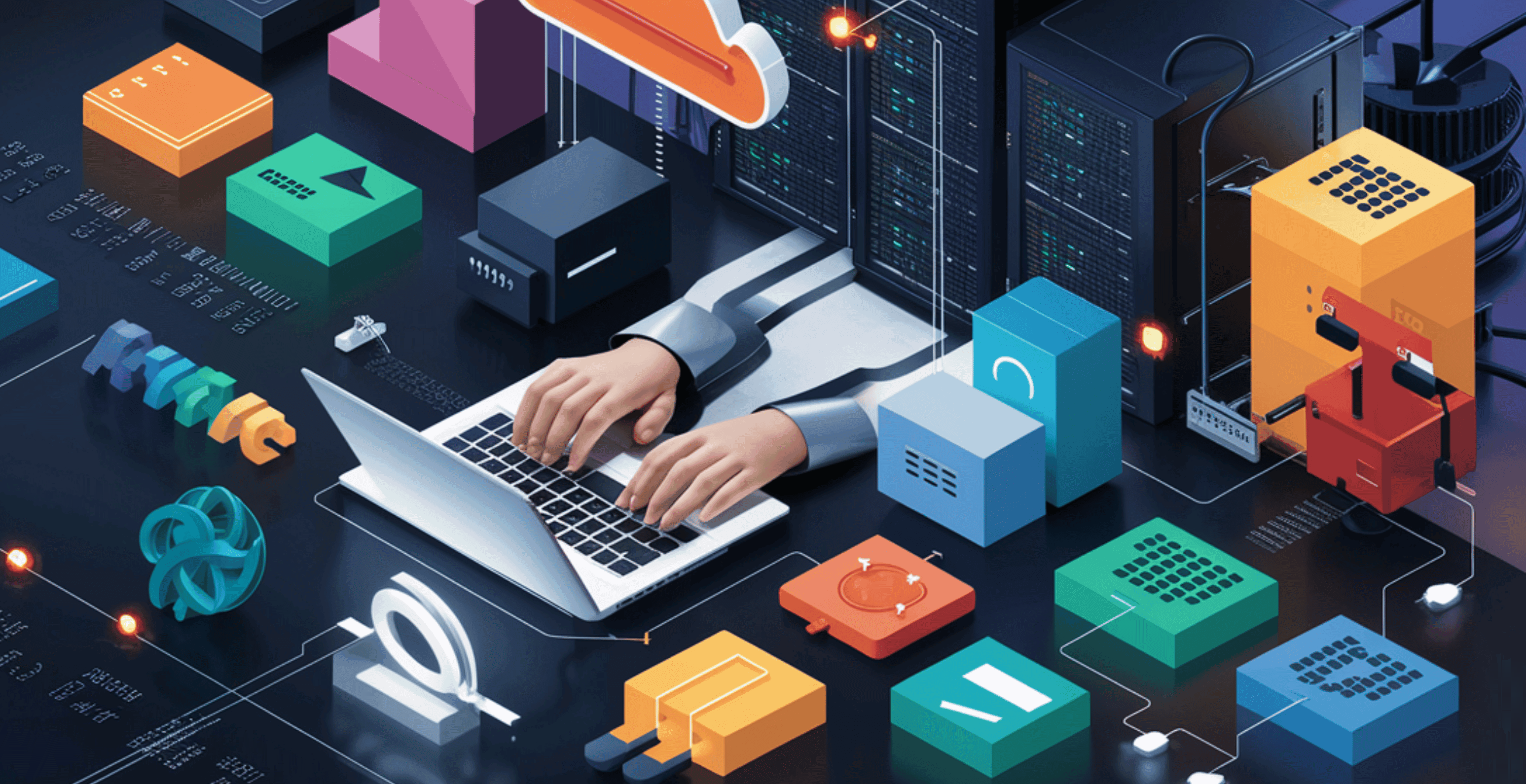
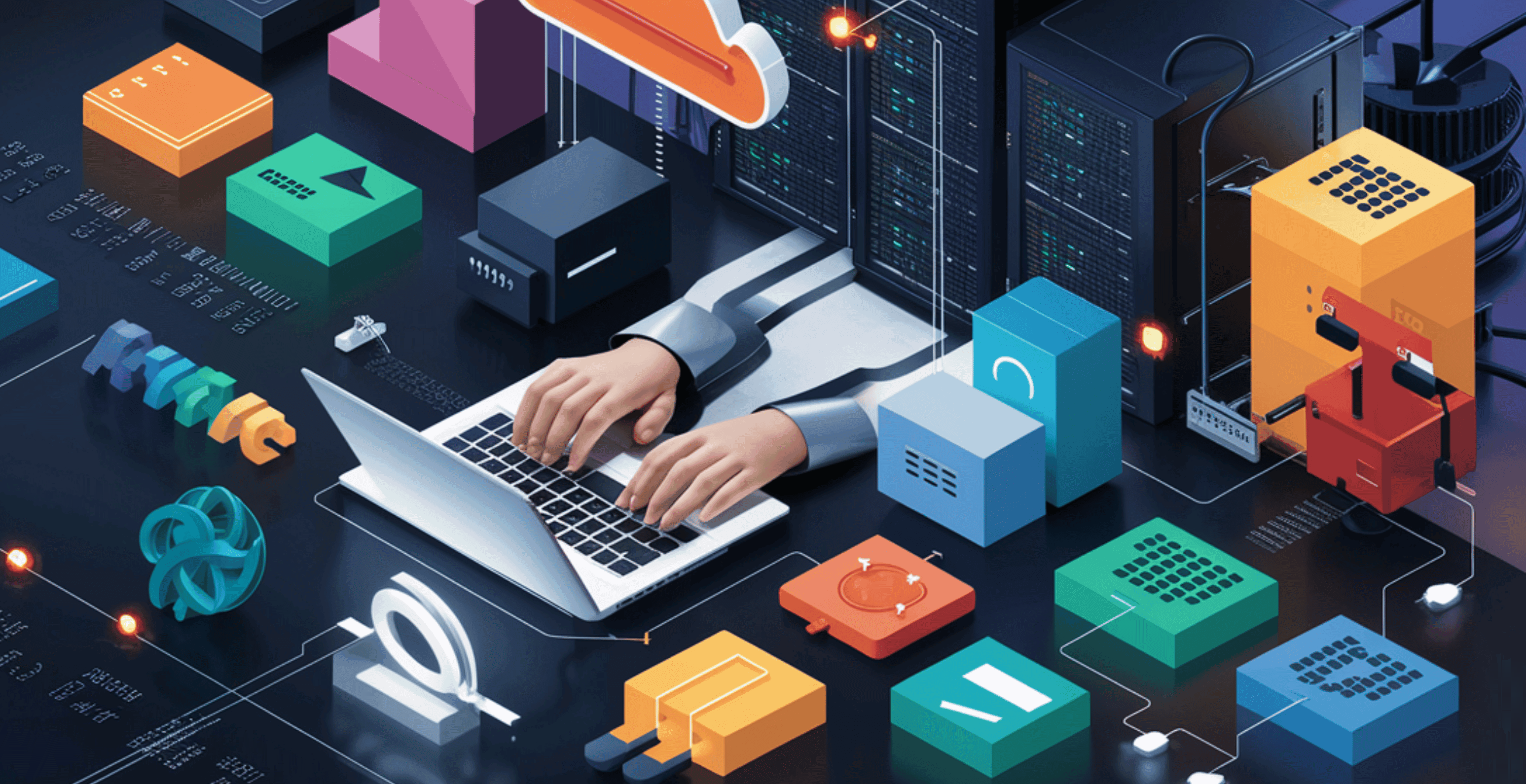
In today’s high-tech globe, Large Language Models (LLMs) are transforming industries by enabling sophisticated language comprehension and generation expertise.
From generating chatbots and virtual assistants to improving content formation and data analysis, the applications of LLMs are enormous and revolutionizing. However, while the potential of these models is enormous, running them effectively needs high-quality hardware, specifically GPUs, and substantial computational resources.
Many find self-hosting LLMs alluring, as it offers exceptional privacy, safety, and personalization advantages. But how do you determine the intricacies of setting up and handling your own LLM infrastructure?
And how do self-hosted fixes contrast to AI-as-a-Service (AIaaS) platforms such as OpenAI in terms of performance and expense? Let’s delve into practical strategies for self-hosting LLMs and discover the advantages and difficulties indulged.
Selecting the Right Model for Self-hosting
When you’re delving into the globe of self-hosted LLM, it is critical to make informed choices to ensure you get the most out of your speculation. Let’s discover how you can select the right model for your requirements:
Key Considerations for Choosing the Right Model
You need to equate numerous components to ensure the finest performance and cost-effectiveness when choosing an LLM for self-hosting. Here are the key considerations:
Performance per Dollar: You will want to assess how fine a model performs compared to its price. This indulges looking at the hardware requirements and the ongoing functioning costs. High-performing models might deliver spectacular outcomes, but they can also be costly to run. Locating an equation between performance and cost is necessary.
Latency: Low latency is crucial for real-time applications where rapid responses are significant. Make sure to select a model and hardware setup that can deliver the speed you require.
Payload Characteristics: Contemplate the kind of tasks you’ll be performing with the model. Distinct models are upgraded for various kinds of payloads–some might shine at managing huge documents, while others are better suited for short queries. Match the model to your precise use case to ensure effectiveness.
Licensing: Regarding utilization rights, not all LLMs are generated equally. Some models are open-source, while others demand licensing fees. Make sure you comprehend the licensing terms to avoid any legitimate difficulties down the time.
The Intricacy of Model Selection
Choosing the right LLM for self-hosting isn’t a direct task. It involves a deep comprehension of your precise requirements and the abilities of numerous models. Performance standard plays a pivotal role in this procedure. These criteria offer factual data on how distinct models perform under numerous circumstances, helping you make informed choices.
Also Read:- Evaluating Large Language Models: Methods And Metrics
Selecting the Right Hardware
The Necessity of GPUs and Their Cost
Running large models efficiently often needs the muscle of GPUs. GPUs manage the enormous computations required for instructing and inference, making them invaluable for LLMs. However, this power comes with a quoted price. Deep learning tasks often require high-end GPUs, which can be utterly costly. You’ll need to equate the performance gains against the expense connotation, specifically if you are operating with budget limitations.
Nvidia vs. AMD: The GPU Debate
When it comes to GPUs, NVIDIA is an ideal choice for a lot of people in the machine learning community. The consideration? CUDA technology. NVIDIA’s CUDA (Compute Unified Device Architecture) provides a sturdy and mature ecosystem that’s upgraded for deep learning tasks. While AMD GPUs can be prominent, they often lack in this phase due to less pragmatic support for deep learning structures. If you want to ensure conformity and boost performance, NVIDIA GPUs are the best choice.
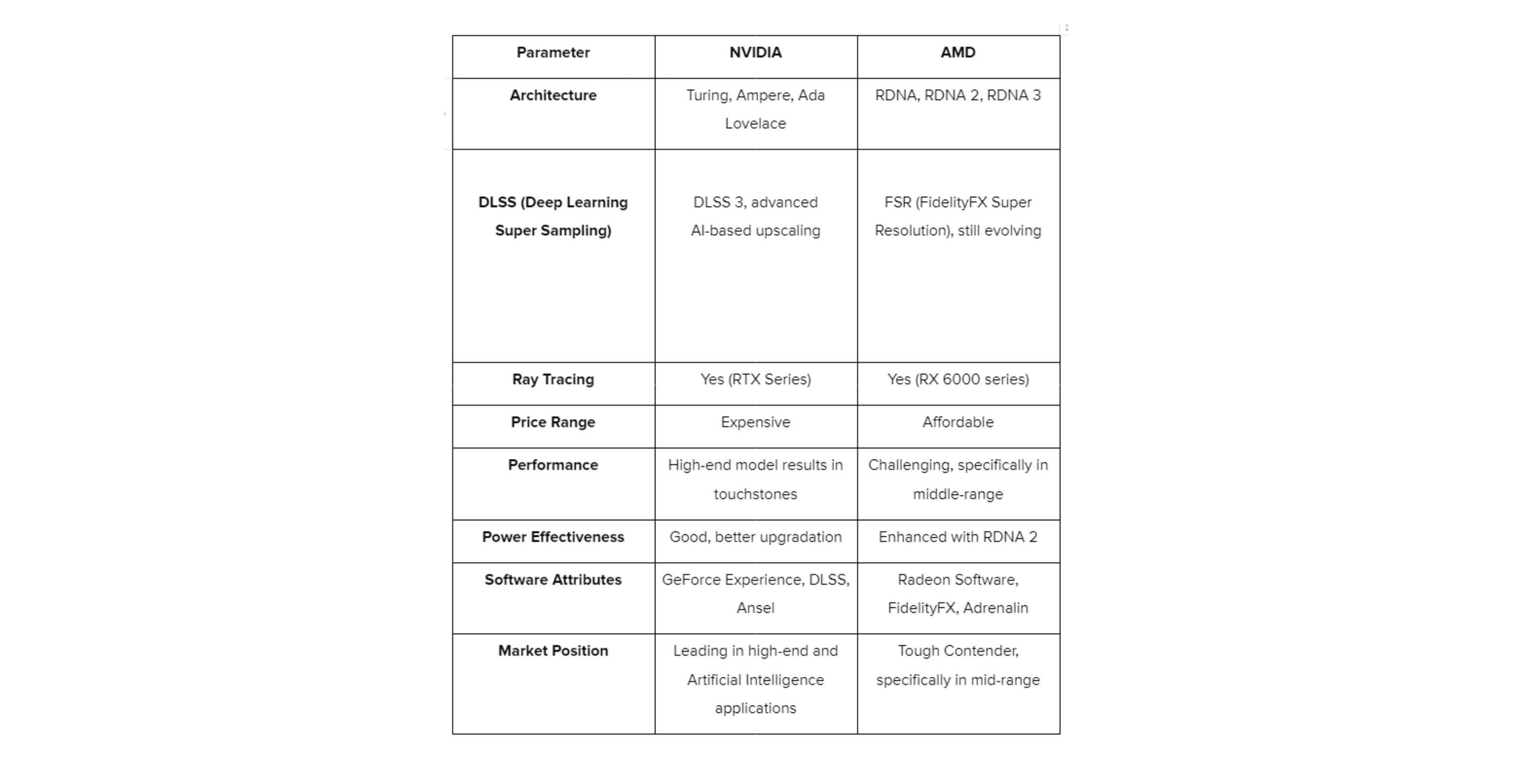
Alternatives to Buying Hardware
Contemplate alternatives such as leasing cloud hardware if putting money into high-end GPUs seems daunting. Cloud suppliers like AWS provide ductile GPU instances that let you compensate for what you utilize without the upfront costs of buying hardware. This adaptability can be groundbreaking, specifically for new ventures or smaller projects.
For less demanding tasks or smaller models, CPUs can sometimes satisfy. Contemporary CPUs are quite prominent and can manage smaller scale induction tasks. This can be an affordable solution if you are not handling extremely large models.
Suggested Hardware Options
For upgraded performance, specifically for self-hosting LLMs, you can’t go wrong with AWS and NVIDIA. AWS provides many options of GPU prototypes customized for deep learning, offering the adaptability to scale as your requirements grow. NVIDIA persists to lead the market with its advanced GPU mechanism, giving solutions that are substantial and hugely assimilated in the Artificial Intelligence community.
By selecting the right hardware, you ensure that your self-hosted LLM runs effectively, saving you time and certainly decreasing budget in the long run.Whether you choose high-end GPUs, lease cloud hardware, or select significant CPUs for minimal tasks, there’s a solution out to meet your requirements.
Also Read:- Comparing Different Large Language Models (LLM)
Deploying and Serving the Model
Deploying and serving your self-hosted LLM can be a groundbreaker for your applications. Let’s delve into some of the best techniques and tools attainable today, concentrating on containerized apps and utilizing Docker for streamlined deployment.
Approaches to Running a Model with a Focus on Containerized Applications
Containerized applications are ideal for adaptability and manageability when it comes to running a model. Containers cluster your application and its reliability into a single unit, ensuring compatible performance across distinct environments. You can run containers on your local machine, on-ground servers, or cloud platforms.
Using Docker, a prominent containerization tool, you can create a structured environment for your LLM. Docker images can summarize your model, its reliability, and any needed configurations, making it simpler to deploy and scale.
Benefits of Model Serving Interfaces like the Text Generation Interface (TGI)
Model serving interfaces, like the Text Generation Interface (TGI), streamline the procedure of deploying and communicating with your LLM. TGI gives a systematic API for serving models, permitting you to concentrate on evolving your apps rather than handling the complexities of model deployment.
With TGI, you acquire:
Operational Convenience: TGI outlines the intricacy of model serving, providing a user-friendly interface to handle your models.
Scalability: TGI sustains ductile deployments, making managing differing burdens easier and ensuring high attainability.
Adaptability: You can incorporate TGI with numerous extremity infrastructures, whether using Kubernetes, Docker Swarm, or other symmetry tools.
Detailed Example of Using Docker to Run and Serve a Model
Let’s explore an instance of using Docker to run and serve an LLM with precise configurations. Suppose you have a pre-trained model hoarded in a directory called my_model.
Create a Dockerfile: The file describes the environment for your model.
FROM python:3.9-slim
WORKDIR /app
COPY my_model /app/my_model
COPY requirements.txt /app/requirements.txt
RUN pip install --no-cache-dir -r requirements.txt
EXPOSE 5000
CMD ["python", "serve_model.py"]
Build the Docker Image: Use the Docker CLI to build your image.
docker build -t my_model_image
Run the Docker Container: Begin a container from your image.
docker run -d -p 5000:5000 --name my_model_container my_model_image
In this setup, serve_model.py is a scenario that establishes and serves your model using a website server. Your model is now operating in a container, attainable on port 5000.
For more information running and building Docker containers from machine learning models, you can refer here!
How to Interact with the Model Using REST API for Generating Predictions
Communicating with your model via Rest API is direct. Here’s how you can send requests to your deployed model to create forecasting:
Send a Post Request: Utilize tools such as curl or Postman to send information to your model .
curl -X POST "http://localhost:5000/predict" -H "Content-Type: application/json" -d '{"input": "Your text here"}'
Process to Response: The model will return a forecast in JSON format. For instance:
{
"output": "Generated text based on your input"
}
Using numerous programming languages, you can incorporate this Rest API call into your app code. Below given is a Python instance using the REQUESTS library:
import requests
url = "http://localhost:5000/predict"
data = {"input": "Your text here"}
response = requests.post(URL, json=data)
print(response.json())
This specifies how easy it is to communicate with your model once it’s ready to run in a containerized environment.
You can refer here for more detail regarding interacting with models using Rest API for generation predictions.
Optimizing Performance and Costs
Exploration of Self-Hosting Costs Versus Using Services Like OpenAI
When considering whether to self-host your large language model (LLM) or use a service like OpenAI, you need to consider the cost and advantages of each option.
Services such as OpenAI provide comfort, manageability, and sturdy infrastructure without requiring you to handle the hardware.
However, these services come at a premium, especially if you have a high utilization demand. Self-hosting can be more economical in the long run but requires an important upfront investment in hardware, setup, and ongoing maintenance.
For example, if you run multiple queries daily, the increasing cost of a service such as OpenAI might surpass the expenditures associated with buying and handling your own servers. The break-even point where self hosting becomes more cheap depends on numerous elements, including the loads of queries, the price of cloud services, and the criticism of your hardware.
Calculations Required to Find When Self-Hosting Becomes Viable
To recognize the feasibility of self-hosting, you need to execute a thorough cost inspection. Here’s a simplified approach:
Initial Investment: Compute the upfront costs for buying servers, GPUs, repositories, and any other significant hardware. For instance, high-end GPUs such as NVIDIA A100 can cost around $10,000 each.
Functional Costs: Indulges electricity, cooling, physical space, and sustained handling. Suppose you have a server that devours 2kW, with an average electricity expenditure of $0.12 per kWh. Over a year, this would amount to around $2,102 in electricity expenditure alone.
Cloud Service Expenditure: Assess the monthly cost of using a cloud service such as OpenAI. For example, OpenAI’s API costs might start from $0.02 to $0.06 per token, relying on the model and utilization tier. If you refine 1 million tokens per day, this can increase your monthly expenditure.
Break Even Analysis: Contrast the total annual expenditure of self-hosting to the paralleled cloud service costs. If the annual expense of utilizing a cloud service transcends the merged initial investment and functional costs of self-hosting within a coherent time frame (e.g., 2-3 years), then self-hosting might be a cheaper option.
Performance Optimization Strategies
To boost the performance of your self-hosted LLM, consider these strategies:
Load-Balancers: Enforce load balancers to supply incoming requests evenly across numerous servers. This precludes any single server from becoming a bottleneck and ensures effective utilization of resources. For instance, utilizing NGINX as a load balancer can help handle traffic and enhance feedback duration.
GPU Usage: Upgrade GPU utilization to manage the calculation requirements of LLMs. Utilize outlining tools to determine performance bottlenecks and adapt the work-load distribution appropriately. For example, using NVIDIA’s CUDA toolkit can help refine GPU performance.
Scalable Architecture:- Design your system to scale reclining, adding more servers as requirement accelerates. This permits you to maintain high performance during culminated utilization periods without overloading your existing infrastructure.
Comparison of HTTP Requests Speeds to LLM Processing Times and the Impact on User Experience
When contrasting HTTP request speeds to LLM processing times, it’s important to comprehend their effect on user experience. HTTPS request speeds usually rely on network suspension, server feedback duration, and the effectiveness of your backend infrastructure. In comparison, LLM processing times are impacted by the intricacies of the model, the hardware used, and the effectiveness of your execution.
For instance, if your HTTP request takes 100 milliseconds but the LLM refining time is 500 milliseconds, the general retaliation duration to the user will be around 600 milliseconds. This dawdle can impact the experience of the user, specifically in apps requiring real-time communications, such as virtual support and chatbots.
To alleviate this, you can enforce methods like:
Asynchronous Processing: Manage requests asynchronously to permit other tasks to proceed while waiting for the LLM to finish its refining.
Caching: Preserve regular feedback to curtail the requirement for recurring LLM refining.
Upgraded Models: Utilize smaller, upgraded models for less intricate questions to reduce refining times.
By meticulously balancing HTTP request managing and LLM refining, you can ensure a receptive and satisfying user experience.
Ensuring security and privacy is crucial when self-hosting LLMs, so let’s dive into the necessary steps to safeguard your data and user trust.
Try RagaAI LLM Hub which helps you get your applications 3X quicker and fix performance, safety and reliability issues across your LLM applications!
Ensuring Security and Privacy
Securing LLM Deployments for Sensitive Information
Safeguarding your large language model (LLM) deployments is uppermost, especially when handling sensitive data. When you self-host an LLM, you’re in charge of your data environment, but this also means you’re reliable for protecting the data. Envision you are handling esoteric client data, proprietary venture data, or personal information- a security infringement could lead to rigorous outcomes, including data stealing, financial loss and harm to your notoriety.
Contemplate the case of a healthcare supplier utilizing an LLM to refine patient data. Any susceptibility could uncover sensitive health data, leading to privacy infringement and legitimate compensation. This makes it important to enforce sturdy security measures to safeguard the data and ensure obedience with regulations such as GDPR and HIPAA.
Using HTTPS and SSL for Secure Connections
One rudimentary step to safeguard your LLM deployment is to utilize HTTPS and SSL for secure connections. HTTPS (Hypertext Transfer Protocol Secure) encodes the data exchanged between your server and clients, averting monitoring and invading. SSL (Secure Sockets Layer) is the fundamental technology that enables this encoding.
For example, when users communicate with your LLM via a website interface or API, HTTPS ensures that any information sent or received is enciphered. This is important for safeguarding login details, query information, and the LLMs replies from being seized by vicious actors. Enforcing HTTPS is direct- get an SSL certificate from a reputed and prominent certificate authority and configure your website server to use it.
Strategies for Maintaining Privacy in Data Processing and API Interactions
Maintaining data privacy during refining and API interactions indulges numerous plans. Initially, unidentified or unnamed private information to avert direct recognition. For instance, supersede names and social security numbers with unique codes before refining.
Next, employ encryption for information at rest and in transit. This ensures that even if information is seized or attained without consent, it stays illegible without the enciphered keys. In addition, execute strict attain control, authorizing data access only to those who need it for their work.
Contemplate also the principle of data minimization, only gather and refine the information significant for the task at hand. For example, if your LLM is used to dissect customer response, avoid gathering extraneous personal information that is not needed for the inspection.
Overview of Potential Security Vulnerabilities and Best Practices to Mitigate Them
Despite your best attempts, potential security vulnerabilities can still present risks. Prevalent risks include SQL injection, cross-site scripting (XSS), and illicit access. To alleviate these risks, adhere to best practices like:-
Frequently update and mend your software to solve known vulnerabilities.
Enforce input verification to avert SQL injection and XSS attacks. For instance, sanitizer user inputs before refining them.
Use strong, special passwords, and enable multi-factor validation (MFA) for accessing your systems.
Demeanor frequent security audits and penetration testing to determine and address vulnerabilities.
Real-world instances emphasize the significance of these practices. For example, a firm might loathe during a security audit that their LLM API was susceptible to an attack that could uncover sensitive customer feedback. By acknowledging the problems immediately and augmenting their security measures, they can avert potential infringement and maintain trust with their users.
By concentrating on these aspects, you can ensure that your self hosted LLM deployments are safe and privacy-compliant, securing both your data and your user’s trust.
Now that we’ve covered the critical aspects of security and privacy, let’s sum up the powerful benefits self-hosting LLMs can bring to your projects.
Conclusion
Self-hosting LLM provides substantial strategic advantages, from improved performance and cost savings to major control over security and personalization. However, equating these benefits needs cautious planning and enforcement.
Beginning with an AIaaS provider and altering to self-hosting as your requirements evolve can be a comprehensive approach.
Enfold the open-source ecosystem for LLM deployment, using community resources and inventiveness to stay at the leading-edge of AI technology. With the right plans, you can utilize the full potential of LLMs, driving inventiveness and accomplishing your aims effectively and safely.
Are you looking for more information on LLMs? Read our other guide on- Multimodal LLMs Using Image and Text.
In today’s high-tech globe, Large Language Models (LLMs) are transforming industries by enabling sophisticated language comprehension and generation expertise.
From generating chatbots and virtual assistants to improving content formation and data analysis, the applications of LLMs are enormous and revolutionizing. However, while the potential of these models is enormous, running them effectively needs high-quality hardware, specifically GPUs, and substantial computational resources.
Many find self-hosting LLMs alluring, as it offers exceptional privacy, safety, and personalization advantages. But how do you determine the intricacies of setting up and handling your own LLM infrastructure?
And how do self-hosted fixes contrast to AI-as-a-Service (AIaaS) platforms such as OpenAI in terms of performance and expense? Let’s delve into practical strategies for self-hosting LLMs and discover the advantages and difficulties indulged.
Selecting the Right Model for Self-hosting
When you’re delving into the globe of self-hosted LLM, it is critical to make informed choices to ensure you get the most out of your speculation. Let’s discover how you can select the right model for your requirements:
Key Considerations for Choosing the Right Model
You need to equate numerous components to ensure the finest performance and cost-effectiveness when choosing an LLM for self-hosting. Here are the key considerations:
Performance per Dollar: You will want to assess how fine a model performs compared to its price. This indulges looking at the hardware requirements and the ongoing functioning costs. High-performing models might deliver spectacular outcomes, but they can also be costly to run. Locating an equation between performance and cost is necessary.
Latency: Low latency is crucial for real-time applications where rapid responses are significant. Make sure to select a model and hardware setup that can deliver the speed you require.
Payload Characteristics: Contemplate the kind of tasks you’ll be performing with the model. Distinct models are upgraded for various kinds of payloads–some might shine at managing huge documents, while others are better suited for short queries. Match the model to your precise use case to ensure effectiveness.
Licensing: Regarding utilization rights, not all LLMs are generated equally. Some models are open-source, while others demand licensing fees. Make sure you comprehend the licensing terms to avoid any legitimate difficulties down the time.
The Intricacy of Model Selection
Choosing the right LLM for self-hosting isn’t a direct task. It involves a deep comprehension of your precise requirements and the abilities of numerous models. Performance standard plays a pivotal role in this procedure. These criteria offer factual data on how distinct models perform under numerous circumstances, helping you make informed choices.
Also Read:- Evaluating Large Language Models: Methods And Metrics
Selecting the Right Hardware
The Necessity of GPUs and Their Cost
Running large models efficiently often needs the muscle of GPUs. GPUs manage the enormous computations required for instructing and inference, making them invaluable for LLMs. However, this power comes with a quoted price. Deep learning tasks often require high-end GPUs, which can be utterly costly. You’ll need to equate the performance gains against the expense connotation, specifically if you are operating with budget limitations.
Nvidia vs. AMD: The GPU Debate
When it comes to GPUs, NVIDIA is an ideal choice for a lot of people in the machine learning community. The consideration? CUDA technology. NVIDIA’s CUDA (Compute Unified Device Architecture) provides a sturdy and mature ecosystem that’s upgraded for deep learning tasks. While AMD GPUs can be prominent, they often lack in this phase due to less pragmatic support for deep learning structures. If you want to ensure conformity and boost performance, NVIDIA GPUs are the best choice.
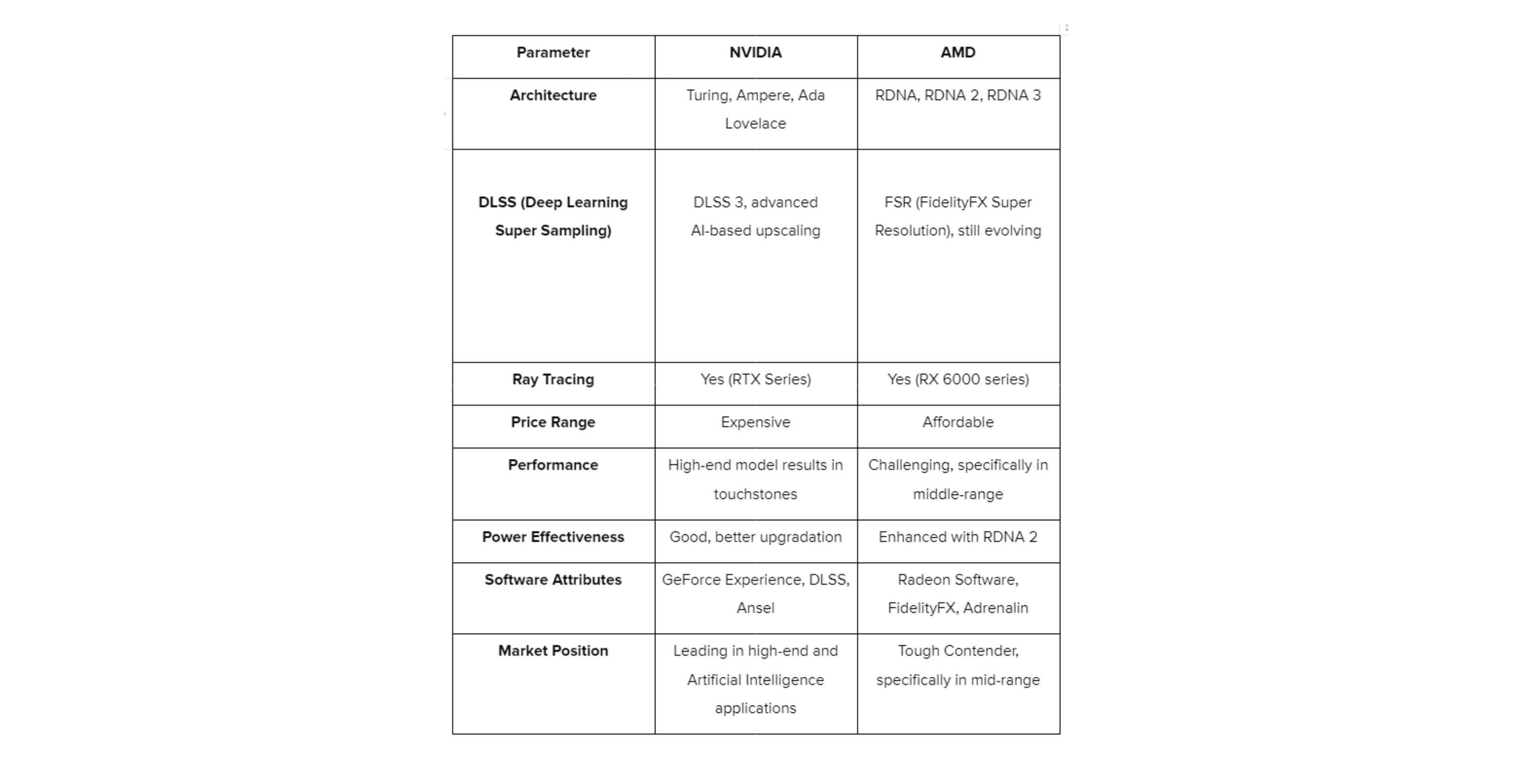
Alternatives to Buying Hardware
Contemplate alternatives such as leasing cloud hardware if putting money into high-end GPUs seems daunting. Cloud suppliers like AWS provide ductile GPU instances that let you compensate for what you utilize without the upfront costs of buying hardware. This adaptability can be groundbreaking, specifically for new ventures or smaller projects.
For less demanding tasks or smaller models, CPUs can sometimes satisfy. Contemporary CPUs are quite prominent and can manage smaller scale induction tasks. This can be an affordable solution if you are not handling extremely large models.
Suggested Hardware Options
For upgraded performance, specifically for self-hosting LLMs, you can’t go wrong with AWS and NVIDIA. AWS provides many options of GPU prototypes customized for deep learning, offering the adaptability to scale as your requirements grow. NVIDIA persists to lead the market with its advanced GPU mechanism, giving solutions that are substantial and hugely assimilated in the Artificial Intelligence community.
By selecting the right hardware, you ensure that your self-hosted LLM runs effectively, saving you time and certainly decreasing budget in the long run.Whether you choose high-end GPUs, lease cloud hardware, or select significant CPUs for minimal tasks, there’s a solution out to meet your requirements.
Also Read:- Comparing Different Large Language Models (LLM)
Deploying and Serving the Model
Deploying and serving your self-hosted LLM can be a groundbreaker for your applications. Let’s delve into some of the best techniques and tools attainable today, concentrating on containerized apps and utilizing Docker for streamlined deployment.
Approaches to Running a Model with a Focus on Containerized Applications
Containerized applications are ideal for adaptability and manageability when it comes to running a model. Containers cluster your application and its reliability into a single unit, ensuring compatible performance across distinct environments. You can run containers on your local machine, on-ground servers, or cloud platforms.
Using Docker, a prominent containerization tool, you can create a structured environment for your LLM. Docker images can summarize your model, its reliability, and any needed configurations, making it simpler to deploy and scale.
Benefits of Model Serving Interfaces like the Text Generation Interface (TGI)
Model serving interfaces, like the Text Generation Interface (TGI), streamline the procedure of deploying and communicating with your LLM. TGI gives a systematic API for serving models, permitting you to concentrate on evolving your apps rather than handling the complexities of model deployment.
With TGI, you acquire:
Operational Convenience: TGI outlines the intricacy of model serving, providing a user-friendly interface to handle your models.
Scalability: TGI sustains ductile deployments, making managing differing burdens easier and ensuring high attainability.
Adaptability: You can incorporate TGI with numerous extremity infrastructures, whether using Kubernetes, Docker Swarm, or other symmetry tools.
Detailed Example of Using Docker to Run and Serve a Model
Let’s explore an instance of using Docker to run and serve an LLM with precise configurations. Suppose you have a pre-trained model hoarded in a directory called my_model.
Create a Dockerfile: The file describes the environment for your model.
FROM python:3.9-slim
WORKDIR /app
COPY my_model /app/my_model
COPY requirements.txt /app/requirements.txt
RUN pip install --no-cache-dir -r requirements.txt
EXPOSE 5000
CMD ["python", "serve_model.py"]
Build the Docker Image: Use the Docker CLI to build your image.
docker build -t my_model_image
Run the Docker Container: Begin a container from your image.
docker run -d -p 5000:5000 --name my_model_container my_model_image
In this setup, serve_model.py is a scenario that establishes and serves your model using a website server. Your model is now operating in a container, attainable on port 5000.
For more information running and building Docker containers from machine learning models, you can refer here!
How to Interact with the Model Using REST API for Generating Predictions
Communicating with your model via Rest API is direct. Here’s how you can send requests to your deployed model to create forecasting:
Send a Post Request: Utilize tools such as curl or Postman to send information to your model .
curl -X POST "http://localhost:5000/predict" -H "Content-Type: application/json" -d '{"input": "Your text here"}'
Process to Response: The model will return a forecast in JSON format. For instance:
{
"output": "Generated text based on your input"
}
Using numerous programming languages, you can incorporate this Rest API call into your app code. Below given is a Python instance using the REQUESTS library:
import requests
url = "http://localhost:5000/predict"
data = {"input": "Your text here"}
response = requests.post(URL, json=data)
print(response.json())
This specifies how easy it is to communicate with your model once it’s ready to run in a containerized environment.
You can refer here for more detail regarding interacting with models using Rest API for generation predictions.
Optimizing Performance and Costs
Exploration of Self-Hosting Costs Versus Using Services Like OpenAI
When considering whether to self-host your large language model (LLM) or use a service like OpenAI, you need to consider the cost and advantages of each option.
Services such as OpenAI provide comfort, manageability, and sturdy infrastructure without requiring you to handle the hardware.
However, these services come at a premium, especially if you have a high utilization demand. Self-hosting can be more economical in the long run but requires an important upfront investment in hardware, setup, and ongoing maintenance.
For example, if you run multiple queries daily, the increasing cost of a service such as OpenAI might surpass the expenditures associated with buying and handling your own servers. The break-even point where self hosting becomes more cheap depends on numerous elements, including the loads of queries, the price of cloud services, and the criticism of your hardware.
Calculations Required to Find When Self-Hosting Becomes Viable
To recognize the feasibility of self-hosting, you need to execute a thorough cost inspection. Here’s a simplified approach:
Initial Investment: Compute the upfront costs for buying servers, GPUs, repositories, and any other significant hardware. For instance, high-end GPUs such as NVIDIA A100 can cost around $10,000 each.
Functional Costs: Indulges electricity, cooling, physical space, and sustained handling. Suppose you have a server that devours 2kW, with an average electricity expenditure of $0.12 per kWh. Over a year, this would amount to around $2,102 in electricity expenditure alone.
Cloud Service Expenditure: Assess the monthly cost of using a cloud service such as OpenAI. For example, OpenAI’s API costs might start from $0.02 to $0.06 per token, relying on the model and utilization tier. If you refine 1 million tokens per day, this can increase your monthly expenditure.
Break Even Analysis: Contrast the total annual expenditure of self-hosting to the paralleled cloud service costs. If the annual expense of utilizing a cloud service transcends the merged initial investment and functional costs of self-hosting within a coherent time frame (e.g., 2-3 years), then self-hosting might be a cheaper option.
Performance Optimization Strategies
To boost the performance of your self-hosted LLM, consider these strategies:
Load-Balancers: Enforce load balancers to supply incoming requests evenly across numerous servers. This precludes any single server from becoming a bottleneck and ensures effective utilization of resources. For instance, utilizing NGINX as a load balancer can help handle traffic and enhance feedback duration.
GPU Usage: Upgrade GPU utilization to manage the calculation requirements of LLMs. Utilize outlining tools to determine performance bottlenecks and adapt the work-load distribution appropriately. For example, using NVIDIA’s CUDA toolkit can help refine GPU performance.
Scalable Architecture:- Design your system to scale reclining, adding more servers as requirement accelerates. This permits you to maintain high performance during culminated utilization periods without overloading your existing infrastructure.
Comparison of HTTP Requests Speeds to LLM Processing Times and the Impact on User Experience
When contrasting HTTP request speeds to LLM processing times, it’s important to comprehend their effect on user experience. HTTPS request speeds usually rely on network suspension, server feedback duration, and the effectiveness of your backend infrastructure. In comparison, LLM processing times are impacted by the intricacies of the model, the hardware used, and the effectiveness of your execution.
For instance, if your HTTP request takes 100 milliseconds but the LLM refining time is 500 milliseconds, the general retaliation duration to the user will be around 600 milliseconds. This dawdle can impact the experience of the user, specifically in apps requiring real-time communications, such as virtual support and chatbots.
To alleviate this, you can enforce methods like:
Asynchronous Processing: Manage requests asynchronously to permit other tasks to proceed while waiting for the LLM to finish its refining.
Caching: Preserve regular feedback to curtail the requirement for recurring LLM refining.
Upgraded Models: Utilize smaller, upgraded models for less intricate questions to reduce refining times.
By meticulously balancing HTTP request managing and LLM refining, you can ensure a receptive and satisfying user experience.
Ensuring security and privacy is crucial when self-hosting LLMs, so let’s dive into the necessary steps to safeguard your data and user trust.
Try RagaAI LLM Hub which helps you get your applications 3X quicker and fix performance, safety and reliability issues across your LLM applications!
Ensuring Security and Privacy
Securing LLM Deployments for Sensitive Information
Safeguarding your large language model (LLM) deployments is uppermost, especially when handling sensitive data. When you self-host an LLM, you’re in charge of your data environment, but this also means you’re reliable for protecting the data. Envision you are handling esoteric client data, proprietary venture data, or personal information- a security infringement could lead to rigorous outcomes, including data stealing, financial loss and harm to your notoriety.
Contemplate the case of a healthcare supplier utilizing an LLM to refine patient data. Any susceptibility could uncover sensitive health data, leading to privacy infringement and legitimate compensation. This makes it important to enforce sturdy security measures to safeguard the data and ensure obedience with regulations such as GDPR and HIPAA.
Using HTTPS and SSL for Secure Connections
One rudimentary step to safeguard your LLM deployment is to utilize HTTPS and SSL for secure connections. HTTPS (Hypertext Transfer Protocol Secure) encodes the data exchanged between your server and clients, averting monitoring and invading. SSL (Secure Sockets Layer) is the fundamental technology that enables this encoding.
For example, when users communicate with your LLM via a website interface or API, HTTPS ensures that any information sent or received is enciphered. This is important for safeguarding login details, query information, and the LLMs replies from being seized by vicious actors. Enforcing HTTPS is direct- get an SSL certificate from a reputed and prominent certificate authority and configure your website server to use it.
Strategies for Maintaining Privacy in Data Processing and API Interactions
Maintaining data privacy during refining and API interactions indulges numerous plans. Initially, unidentified or unnamed private information to avert direct recognition. For instance, supersede names and social security numbers with unique codes before refining.
Next, employ encryption for information at rest and in transit. This ensures that even if information is seized or attained without consent, it stays illegible without the enciphered keys. In addition, execute strict attain control, authorizing data access only to those who need it for their work.
Contemplate also the principle of data minimization, only gather and refine the information significant for the task at hand. For example, if your LLM is used to dissect customer response, avoid gathering extraneous personal information that is not needed for the inspection.
Overview of Potential Security Vulnerabilities and Best Practices to Mitigate Them
Despite your best attempts, potential security vulnerabilities can still present risks. Prevalent risks include SQL injection, cross-site scripting (XSS), and illicit access. To alleviate these risks, adhere to best practices like:-
Frequently update and mend your software to solve known vulnerabilities.
Enforce input verification to avert SQL injection and XSS attacks. For instance, sanitizer user inputs before refining them.
Use strong, special passwords, and enable multi-factor validation (MFA) for accessing your systems.
Demeanor frequent security audits and penetration testing to determine and address vulnerabilities.
Real-world instances emphasize the significance of these practices. For example, a firm might loathe during a security audit that their LLM API was susceptible to an attack that could uncover sensitive customer feedback. By acknowledging the problems immediately and augmenting their security measures, they can avert potential infringement and maintain trust with their users.
By concentrating on these aspects, you can ensure that your self hosted LLM deployments are safe and privacy-compliant, securing both your data and your user’s trust.
Now that we’ve covered the critical aspects of security and privacy, let’s sum up the powerful benefits self-hosting LLMs can bring to your projects.
Conclusion
Self-hosting LLM provides substantial strategic advantages, from improved performance and cost savings to major control over security and personalization. However, equating these benefits needs cautious planning and enforcement.
Beginning with an AIaaS provider and altering to self-hosting as your requirements evolve can be a comprehensive approach.
Enfold the open-source ecosystem for LLM deployment, using community resources and inventiveness to stay at the leading-edge of AI technology. With the right plans, you can utilize the full potential of LLMs, driving inventiveness and accomplishing your aims effectively and safely.
Are you looking for more information on LLMs? Read our other guide on- Multimodal LLMs Using Image and Text.
In today’s high-tech globe, Large Language Models (LLMs) are transforming industries by enabling sophisticated language comprehension and generation expertise.
From generating chatbots and virtual assistants to improving content formation and data analysis, the applications of LLMs are enormous and revolutionizing. However, while the potential of these models is enormous, running them effectively needs high-quality hardware, specifically GPUs, and substantial computational resources.
Many find self-hosting LLMs alluring, as it offers exceptional privacy, safety, and personalization advantages. But how do you determine the intricacies of setting up and handling your own LLM infrastructure?
And how do self-hosted fixes contrast to AI-as-a-Service (AIaaS) platforms such as OpenAI in terms of performance and expense? Let’s delve into practical strategies for self-hosting LLMs and discover the advantages and difficulties indulged.
Selecting the Right Model for Self-hosting
When you’re delving into the globe of self-hosted LLM, it is critical to make informed choices to ensure you get the most out of your speculation. Let’s discover how you can select the right model for your requirements:
Key Considerations for Choosing the Right Model
You need to equate numerous components to ensure the finest performance and cost-effectiveness when choosing an LLM for self-hosting. Here are the key considerations:
Performance per Dollar: You will want to assess how fine a model performs compared to its price. This indulges looking at the hardware requirements and the ongoing functioning costs. High-performing models might deliver spectacular outcomes, but they can also be costly to run. Locating an equation between performance and cost is necessary.
Latency: Low latency is crucial for real-time applications where rapid responses are significant. Make sure to select a model and hardware setup that can deliver the speed you require.
Payload Characteristics: Contemplate the kind of tasks you’ll be performing with the model. Distinct models are upgraded for various kinds of payloads–some might shine at managing huge documents, while others are better suited for short queries. Match the model to your precise use case to ensure effectiveness.
Licensing: Regarding utilization rights, not all LLMs are generated equally. Some models are open-source, while others demand licensing fees. Make sure you comprehend the licensing terms to avoid any legitimate difficulties down the time.
The Intricacy of Model Selection
Choosing the right LLM for self-hosting isn’t a direct task. It involves a deep comprehension of your precise requirements and the abilities of numerous models. Performance standard plays a pivotal role in this procedure. These criteria offer factual data on how distinct models perform under numerous circumstances, helping you make informed choices.
Also Read:- Evaluating Large Language Models: Methods And Metrics
Selecting the Right Hardware
The Necessity of GPUs and Their Cost
Running large models efficiently often needs the muscle of GPUs. GPUs manage the enormous computations required for instructing and inference, making them invaluable for LLMs. However, this power comes with a quoted price. Deep learning tasks often require high-end GPUs, which can be utterly costly. You’ll need to equate the performance gains against the expense connotation, specifically if you are operating with budget limitations.
Nvidia vs. AMD: The GPU Debate
When it comes to GPUs, NVIDIA is an ideal choice for a lot of people in the machine learning community. The consideration? CUDA technology. NVIDIA’s CUDA (Compute Unified Device Architecture) provides a sturdy and mature ecosystem that’s upgraded for deep learning tasks. While AMD GPUs can be prominent, they often lack in this phase due to less pragmatic support for deep learning structures. If you want to ensure conformity and boost performance, NVIDIA GPUs are the best choice.
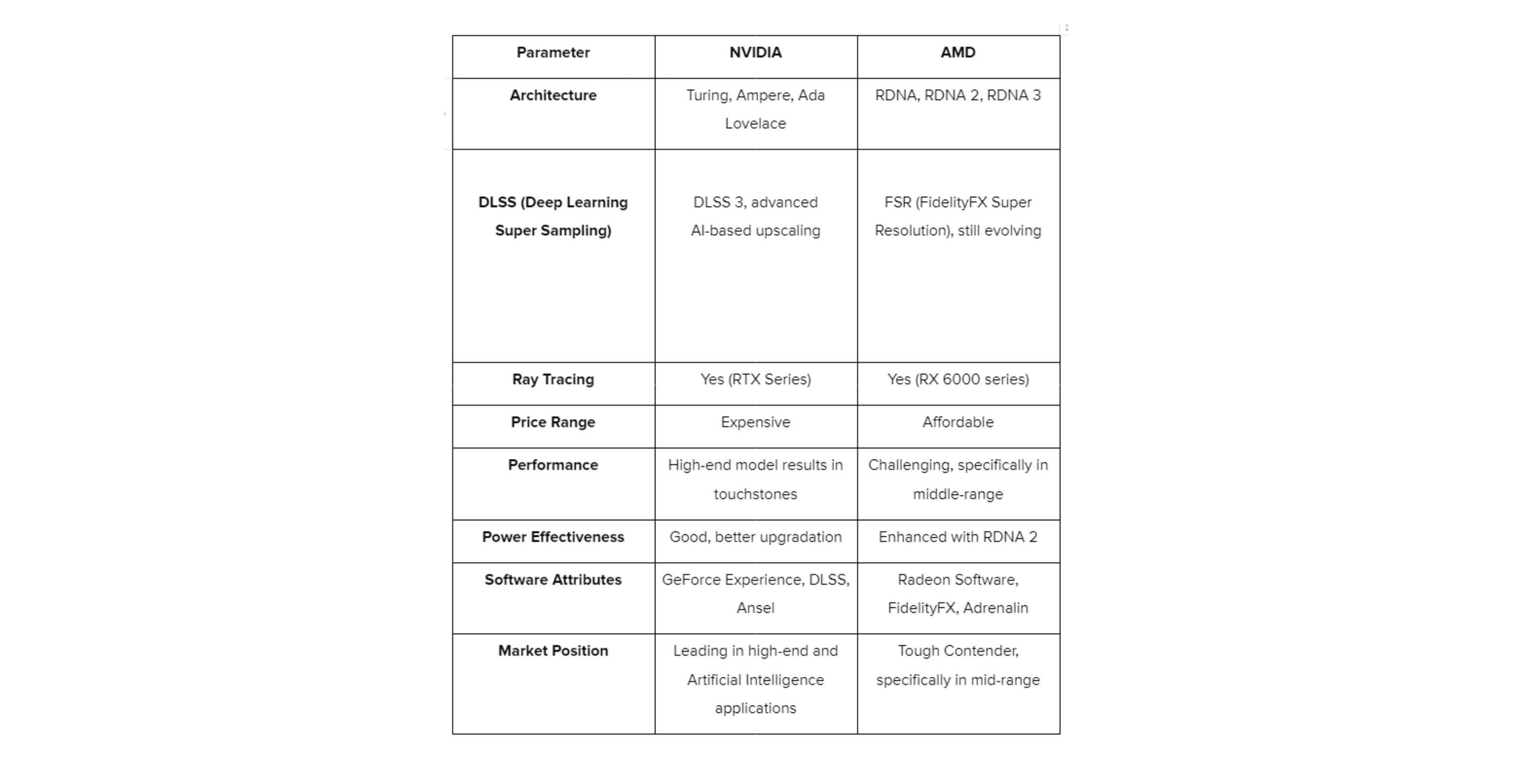
Alternatives to Buying Hardware
Contemplate alternatives such as leasing cloud hardware if putting money into high-end GPUs seems daunting. Cloud suppliers like AWS provide ductile GPU instances that let you compensate for what you utilize without the upfront costs of buying hardware. This adaptability can be groundbreaking, specifically for new ventures or smaller projects.
For less demanding tasks or smaller models, CPUs can sometimes satisfy. Contemporary CPUs are quite prominent and can manage smaller scale induction tasks. This can be an affordable solution if you are not handling extremely large models.
Suggested Hardware Options
For upgraded performance, specifically for self-hosting LLMs, you can’t go wrong with AWS and NVIDIA. AWS provides many options of GPU prototypes customized for deep learning, offering the adaptability to scale as your requirements grow. NVIDIA persists to lead the market with its advanced GPU mechanism, giving solutions that are substantial and hugely assimilated in the Artificial Intelligence community.
By selecting the right hardware, you ensure that your self-hosted LLM runs effectively, saving you time and certainly decreasing budget in the long run.Whether you choose high-end GPUs, lease cloud hardware, or select significant CPUs for minimal tasks, there’s a solution out to meet your requirements.
Also Read:- Comparing Different Large Language Models (LLM)
Deploying and Serving the Model
Deploying and serving your self-hosted LLM can be a groundbreaker for your applications. Let’s delve into some of the best techniques and tools attainable today, concentrating on containerized apps and utilizing Docker for streamlined deployment.
Approaches to Running a Model with a Focus on Containerized Applications
Containerized applications are ideal for adaptability and manageability when it comes to running a model. Containers cluster your application and its reliability into a single unit, ensuring compatible performance across distinct environments. You can run containers on your local machine, on-ground servers, or cloud platforms.
Using Docker, a prominent containerization tool, you can create a structured environment for your LLM. Docker images can summarize your model, its reliability, and any needed configurations, making it simpler to deploy and scale.
Benefits of Model Serving Interfaces like the Text Generation Interface (TGI)
Model serving interfaces, like the Text Generation Interface (TGI), streamline the procedure of deploying and communicating with your LLM. TGI gives a systematic API for serving models, permitting you to concentrate on evolving your apps rather than handling the complexities of model deployment.
With TGI, you acquire:
Operational Convenience: TGI outlines the intricacy of model serving, providing a user-friendly interface to handle your models.
Scalability: TGI sustains ductile deployments, making managing differing burdens easier and ensuring high attainability.
Adaptability: You can incorporate TGI with numerous extremity infrastructures, whether using Kubernetes, Docker Swarm, or other symmetry tools.
Detailed Example of Using Docker to Run and Serve a Model
Let’s explore an instance of using Docker to run and serve an LLM with precise configurations. Suppose you have a pre-trained model hoarded in a directory called my_model.
Create a Dockerfile: The file describes the environment for your model.
FROM python:3.9-slim
WORKDIR /app
COPY my_model /app/my_model
COPY requirements.txt /app/requirements.txt
RUN pip install --no-cache-dir -r requirements.txt
EXPOSE 5000
CMD ["python", "serve_model.py"]
Build the Docker Image: Use the Docker CLI to build your image.
docker build -t my_model_image
Run the Docker Container: Begin a container from your image.
docker run -d -p 5000:5000 --name my_model_container my_model_image
In this setup, serve_model.py is a scenario that establishes and serves your model using a website server. Your model is now operating in a container, attainable on port 5000.
For more information running and building Docker containers from machine learning models, you can refer here!
How to Interact with the Model Using REST API for Generating Predictions
Communicating with your model via Rest API is direct. Here’s how you can send requests to your deployed model to create forecasting:
Send a Post Request: Utilize tools such as curl or Postman to send information to your model .
curl -X POST "http://localhost:5000/predict" -H "Content-Type: application/json" -d '{"input": "Your text here"}'
Process to Response: The model will return a forecast in JSON format. For instance:
{
"output": "Generated text based on your input"
}
Using numerous programming languages, you can incorporate this Rest API call into your app code. Below given is a Python instance using the REQUESTS library:
import requests
url = "http://localhost:5000/predict"
data = {"input": "Your text here"}
response = requests.post(URL, json=data)
print(response.json())
This specifies how easy it is to communicate with your model once it’s ready to run in a containerized environment.
You can refer here for more detail regarding interacting with models using Rest API for generation predictions.
Optimizing Performance and Costs
Exploration of Self-Hosting Costs Versus Using Services Like OpenAI
When considering whether to self-host your large language model (LLM) or use a service like OpenAI, you need to consider the cost and advantages of each option.
Services such as OpenAI provide comfort, manageability, and sturdy infrastructure without requiring you to handle the hardware.
However, these services come at a premium, especially if you have a high utilization demand. Self-hosting can be more economical in the long run but requires an important upfront investment in hardware, setup, and ongoing maintenance.
For example, if you run multiple queries daily, the increasing cost of a service such as OpenAI might surpass the expenditures associated with buying and handling your own servers. The break-even point where self hosting becomes more cheap depends on numerous elements, including the loads of queries, the price of cloud services, and the criticism of your hardware.
Calculations Required to Find When Self-Hosting Becomes Viable
To recognize the feasibility of self-hosting, you need to execute a thorough cost inspection. Here’s a simplified approach:
Initial Investment: Compute the upfront costs for buying servers, GPUs, repositories, and any other significant hardware. For instance, high-end GPUs such as NVIDIA A100 can cost around $10,000 each.
Functional Costs: Indulges electricity, cooling, physical space, and sustained handling. Suppose you have a server that devours 2kW, with an average electricity expenditure of $0.12 per kWh. Over a year, this would amount to around $2,102 in electricity expenditure alone.
Cloud Service Expenditure: Assess the monthly cost of using a cloud service such as OpenAI. For example, OpenAI’s API costs might start from $0.02 to $0.06 per token, relying on the model and utilization tier. If you refine 1 million tokens per day, this can increase your monthly expenditure.
Break Even Analysis: Contrast the total annual expenditure of self-hosting to the paralleled cloud service costs. If the annual expense of utilizing a cloud service transcends the merged initial investment and functional costs of self-hosting within a coherent time frame (e.g., 2-3 years), then self-hosting might be a cheaper option.
Performance Optimization Strategies
To boost the performance of your self-hosted LLM, consider these strategies:
Load-Balancers: Enforce load balancers to supply incoming requests evenly across numerous servers. This precludes any single server from becoming a bottleneck and ensures effective utilization of resources. For instance, utilizing NGINX as a load balancer can help handle traffic and enhance feedback duration.
GPU Usage: Upgrade GPU utilization to manage the calculation requirements of LLMs. Utilize outlining tools to determine performance bottlenecks and adapt the work-load distribution appropriately. For example, using NVIDIA’s CUDA toolkit can help refine GPU performance.
Scalable Architecture:- Design your system to scale reclining, adding more servers as requirement accelerates. This permits you to maintain high performance during culminated utilization periods without overloading your existing infrastructure.
Comparison of HTTP Requests Speeds to LLM Processing Times and the Impact on User Experience
When contrasting HTTP request speeds to LLM processing times, it’s important to comprehend their effect on user experience. HTTPS request speeds usually rely on network suspension, server feedback duration, and the effectiveness of your backend infrastructure. In comparison, LLM processing times are impacted by the intricacies of the model, the hardware used, and the effectiveness of your execution.
For instance, if your HTTP request takes 100 milliseconds but the LLM refining time is 500 milliseconds, the general retaliation duration to the user will be around 600 milliseconds. This dawdle can impact the experience of the user, specifically in apps requiring real-time communications, such as virtual support and chatbots.
To alleviate this, you can enforce methods like:
Asynchronous Processing: Manage requests asynchronously to permit other tasks to proceed while waiting for the LLM to finish its refining.
Caching: Preserve regular feedback to curtail the requirement for recurring LLM refining.
Upgraded Models: Utilize smaller, upgraded models for less intricate questions to reduce refining times.
By meticulously balancing HTTP request managing and LLM refining, you can ensure a receptive and satisfying user experience.
Ensuring security and privacy is crucial when self-hosting LLMs, so let’s dive into the necessary steps to safeguard your data and user trust.
Try RagaAI LLM Hub which helps you get your applications 3X quicker and fix performance, safety and reliability issues across your LLM applications!
Ensuring Security and Privacy
Securing LLM Deployments for Sensitive Information
Safeguarding your large language model (LLM) deployments is uppermost, especially when handling sensitive data. When you self-host an LLM, you’re in charge of your data environment, but this also means you’re reliable for protecting the data. Envision you are handling esoteric client data, proprietary venture data, or personal information- a security infringement could lead to rigorous outcomes, including data stealing, financial loss and harm to your notoriety.
Contemplate the case of a healthcare supplier utilizing an LLM to refine patient data. Any susceptibility could uncover sensitive health data, leading to privacy infringement and legitimate compensation. This makes it important to enforce sturdy security measures to safeguard the data and ensure obedience with regulations such as GDPR and HIPAA.
Using HTTPS and SSL for Secure Connections
One rudimentary step to safeguard your LLM deployment is to utilize HTTPS and SSL for secure connections. HTTPS (Hypertext Transfer Protocol Secure) encodes the data exchanged between your server and clients, averting monitoring and invading. SSL (Secure Sockets Layer) is the fundamental technology that enables this encoding.
For example, when users communicate with your LLM via a website interface or API, HTTPS ensures that any information sent or received is enciphered. This is important for safeguarding login details, query information, and the LLMs replies from being seized by vicious actors. Enforcing HTTPS is direct- get an SSL certificate from a reputed and prominent certificate authority and configure your website server to use it.
Strategies for Maintaining Privacy in Data Processing and API Interactions
Maintaining data privacy during refining and API interactions indulges numerous plans. Initially, unidentified or unnamed private information to avert direct recognition. For instance, supersede names and social security numbers with unique codes before refining.
Next, employ encryption for information at rest and in transit. This ensures that even if information is seized or attained without consent, it stays illegible without the enciphered keys. In addition, execute strict attain control, authorizing data access only to those who need it for their work.
Contemplate also the principle of data minimization, only gather and refine the information significant for the task at hand. For example, if your LLM is used to dissect customer response, avoid gathering extraneous personal information that is not needed for the inspection.
Overview of Potential Security Vulnerabilities and Best Practices to Mitigate Them
Despite your best attempts, potential security vulnerabilities can still present risks. Prevalent risks include SQL injection, cross-site scripting (XSS), and illicit access. To alleviate these risks, adhere to best practices like:-
Frequently update and mend your software to solve known vulnerabilities.
Enforce input verification to avert SQL injection and XSS attacks. For instance, sanitizer user inputs before refining them.
Use strong, special passwords, and enable multi-factor validation (MFA) for accessing your systems.
Demeanor frequent security audits and penetration testing to determine and address vulnerabilities.
Real-world instances emphasize the significance of these practices. For example, a firm might loathe during a security audit that their LLM API was susceptible to an attack that could uncover sensitive customer feedback. By acknowledging the problems immediately and augmenting their security measures, they can avert potential infringement and maintain trust with their users.
By concentrating on these aspects, you can ensure that your self hosted LLM deployments are safe and privacy-compliant, securing both your data and your user’s trust.
Now that we’ve covered the critical aspects of security and privacy, let’s sum up the powerful benefits self-hosting LLMs can bring to your projects.
Conclusion
Self-hosting LLM provides substantial strategic advantages, from improved performance and cost savings to major control over security and personalization. However, equating these benefits needs cautious planning and enforcement.
Beginning with an AIaaS provider and altering to self-hosting as your requirements evolve can be a comprehensive approach.
Enfold the open-source ecosystem for LLM deployment, using community resources and inventiveness to stay at the leading-edge of AI technology. With the right plans, you can utilize the full potential of LLMs, driving inventiveness and accomplishing your aims effectively and safely.
Are you looking for more information on LLMs? Read our other guide on- Multimodal LLMs Using Image and Text.
In today’s high-tech globe, Large Language Models (LLMs) are transforming industries by enabling sophisticated language comprehension and generation expertise.
From generating chatbots and virtual assistants to improving content formation and data analysis, the applications of LLMs are enormous and revolutionizing. However, while the potential of these models is enormous, running them effectively needs high-quality hardware, specifically GPUs, and substantial computational resources.
Many find self-hosting LLMs alluring, as it offers exceptional privacy, safety, and personalization advantages. But how do you determine the intricacies of setting up and handling your own LLM infrastructure?
And how do self-hosted fixes contrast to AI-as-a-Service (AIaaS) platforms such as OpenAI in terms of performance and expense? Let’s delve into practical strategies for self-hosting LLMs and discover the advantages and difficulties indulged.
Selecting the Right Model for Self-hosting
When you’re delving into the globe of self-hosted LLM, it is critical to make informed choices to ensure you get the most out of your speculation. Let’s discover how you can select the right model for your requirements:
Key Considerations for Choosing the Right Model
You need to equate numerous components to ensure the finest performance and cost-effectiveness when choosing an LLM for self-hosting. Here are the key considerations:
Performance per Dollar: You will want to assess how fine a model performs compared to its price. This indulges looking at the hardware requirements and the ongoing functioning costs. High-performing models might deliver spectacular outcomes, but they can also be costly to run. Locating an equation between performance and cost is necessary.
Latency: Low latency is crucial for real-time applications where rapid responses are significant. Make sure to select a model and hardware setup that can deliver the speed you require.
Payload Characteristics: Contemplate the kind of tasks you’ll be performing with the model. Distinct models are upgraded for various kinds of payloads–some might shine at managing huge documents, while others are better suited for short queries. Match the model to your precise use case to ensure effectiveness.
Licensing: Regarding utilization rights, not all LLMs are generated equally. Some models are open-source, while others demand licensing fees. Make sure you comprehend the licensing terms to avoid any legitimate difficulties down the time.
The Intricacy of Model Selection
Choosing the right LLM for self-hosting isn’t a direct task. It involves a deep comprehension of your precise requirements and the abilities of numerous models. Performance standard plays a pivotal role in this procedure. These criteria offer factual data on how distinct models perform under numerous circumstances, helping you make informed choices.
Also Read:- Evaluating Large Language Models: Methods And Metrics
Selecting the Right Hardware
The Necessity of GPUs and Their Cost
Running large models efficiently often needs the muscle of GPUs. GPUs manage the enormous computations required for instructing and inference, making them invaluable for LLMs. However, this power comes with a quoted price. Deep learning tasks often require high-end GPUs, which can be utterly costly. You’ll need to equate the performance gains against the expense connotation, specifically if you are operating with budget limitations.
Nvidia vs. AMD: The GPU Debate
When it comes to GPUs, NVIDIA is an ideal choice for a lot of people in the machine learning community. The consideration? CUDA technology. NVIDIA’s CUDA (Compute Unified Device Architecture) provides a sturdy and mature ecosystem that’s upgraded for deep learning tasks. While AMD GPUs can be prominent, they often lack in this phase due to less pragmatic support for deep learning structures. If you want to ensure conformity and boost performance, NVIDIA GPUs are the best choice.
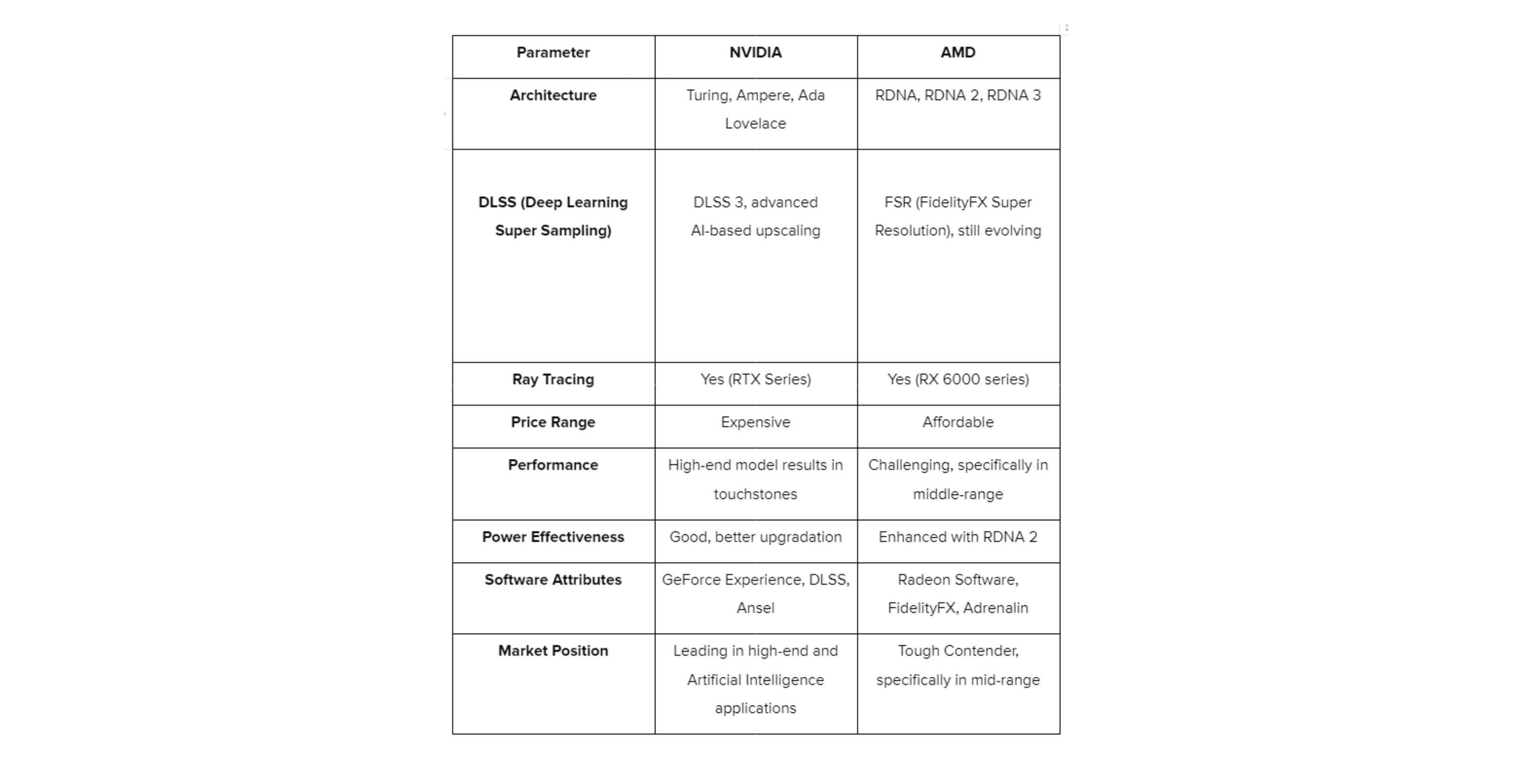
Alternatives to Buying Hardware
Contemplate alternatives such as leasing cloud hardware if putting money into high-end GPUs seems daunting. Cloud suppliers like AWS provide ductile GPU instances that let you compensate for what you utilize without the upfront costs of buying hardware. This adaptability can be groundbreaking, specifically for new ventures or smaller projects.
For less demanding tasks or smaller models, CPUs can sometimes satisfy. Contemporary CPUs are quite prominent and can manage smaller scale induction tasks. This can be an affordable solution if you are not handling extremely large models.
Suggested Hardware Options
For upgraded performance, specifically for self-hosting LLMs, you can’t go wrong with AWS and NVIDIA. AWS provides many options of GPU prototypes customized for deep learning, offering the adaptability to scale as your requirements grow. NVIDIA persists to lead the market with its advanced GPU mechanism, giving solutions that are substantial and hugely assimilated in the Artificial Intelligence community.
By selecting the right hardware, you ensure that your self-hosted LLM runs effectively, saving you time and certainly decreasing budget in the long run.Whether you choose high-end GPUs, lease cloud hardware, or select significant CPUs for minimal tasks, there’s a solution out to meet your requirements.
Also Read:- Comparing Different Large Language Models (LLM)
Deploying and Serving the Model
Deploying and serving your self-hosted LLM can be a groundbreaker for your applications. Let’s delve into some of the best techniques and tools attainable today, concentrating on containerized apps and utilizing Docker for streamlined deployment.
Approaches to Running a Model with a Focus on Containerized Applications
Containerized applications are ideal for adaptability and manageability when it comes to running a model. Containers cluster your application and its reliability into a single unit, ensuring compatible performance across distinct environments. You can run containers on your local machine, on-ground servers, or cloud platforms.
Using Docker, a prominent containerization tool, you can create a structured environment for your LLM. Docker images can summarize your model, its reliability, and any needed configurations, making it simpler to deploy and scale.
Benefits of Model Serving Interfaces like the Text Generation Interface (TGI)
Model serving interfaces, like the Text Generation Interface (TGI), streamline the procedure of deploying and communicating with your LLM. TGI gives a systematic API for serving models, permitting you to concentrate on evolving your apps rather than handling the complexities of model deployment.
With TGI, you acquire:
Operational Convenience: TGI outlines the intricacy of model serving, providing a user-friendly interface to handle your models.
Scalability: TGI sustains ductile deployments, making managing differing burdens easier and ensuring high attainability.
Adaptability: You can incorporate TGI with numerous extremity infrastructures, whether using Kubernetes, Docker Swarm, or other symmetry tools.
Detailed Example of Using Docker to Run and Serve a Model
Let’s explore an instance of using Docker to run and serve an LLM with precise configurations. Suppose you have a pre-trained model hoarded in a directory called my_model.
Create a Dockerfile: The file describes the environment for your model.
FROM python:3.9-slim
WORKDIR /app
COPY my_model /app/my_model
COPY requirements.txt /app/requirements.txt
RUN pip install --no-cache-dir -r requirements.txt
EXPOSE 5000
CMD ["python", "serve_model.py"]
Build the Docker Image: Use the Docker CLI to build your image.
docker build -t my_model_image
Run the Docker Container: Begin a container from your image.
docker run -d -p 5000:5000 --name my_model_container my_model_image
In this setup, serve_model.py is a scenario that establishes and serves your model using a website server. Your model is now operating in a container, attainable on port 5000.
For more information running and building Docker containers from machine learning models, you can refer here!
How to Interact with the Model Using REST API for Generating Predictions
Communicating with your model via Rest API is direct. Here’s how you can send requests to your deployed model to create forecasting:
Send a Post Request: Utilize tools such as curl or Postman to send information to your model .
curl -X POST "http://localhost:5000/predict" -H "Content-Type: application/json" -d '{"input": "Your text here"}'
Process to Response: The model will return a forecast in JSON format. For instance:
{
"output": "Generated text based on your input"
}
Using numerous programming languages, you can incorporate this Rest API call into your app code. Below given is a Python instance using the REQUESTS library:
import requests
url = "http://localhost:5000/predict"
data = {"input": "Your text here"}
response = requests.post(URL, json=data)
print(response.json())
This specifies how easy it is to communicate with your model once it’s ready to run in a containerized environment.
You can refer here for more detail regarding interacting with models using Rest API for generation predictions.
Optimizing Performance and Costs
Exploration of Self-Hosting Costs Versus Using Services Like OpenAI
When considering whether to self-host your large language model (LLM) or use a service like OpenAI, you need to consider the cost and advantages of each option.
Services such as OpenAI provide comfort, manageability, and sturdy infrastructure without requiring you to handle the hardware.
However, these services come at a premium, especially if you have a high utilization demand. Self-hosting can be more economical in the long run but requires an important upfront investment in hardware, setup, and ongoing maintenance.
For example, if you run multiple queries daily, the increasing cost of a service such as OpenAI might surpass the expenditures associated with buying and handling your own servers. The break-even point where self hosting becomes more cheap depends on numerous elements, including the loads of queries, the price of cloud services, and the criticism of your hardware.
Calculations Required to Find When Self-Hosting Becomes Viable
To recognize the feasibility of self-hosting, you need to execute a thorough cost inspection. Here’s a simplified approach:
Initial Investment: Compute the upfront costs for buying servers, GPUs, repositories, and any other significant hardware. For instance, high-end GPUs such as NVIDIA A100 can cost around $10,000 each.
Functional Costs: Indulges electricity, cooling, physical space, and sustained handling. Suppose you have a server that devours 2kW, with an average electricity expenditure of $0.12 per kWh. Over a year, this would amount to around $2,102 in electricity expenditure alone.
Cloud Service Expenditure: Assess the monthly cost of using a cloud service such as OpenAI. For example, OpenAI’s API costs might start from $0.02 to $0.06 per token, relying on the model and utilization tier. If you refine 1 million tokens per day, this can increase your monthly expenditure.
Break Even Analysis: Contrast the total annual expenditure of self-hosting to the paralleled cloud service costs. If the annual expense of utilizing a cloud service transcends the merged initial investment and functional costs of self-hosting within a coherent time frame (e.g., 2-3 years), then self-hosting might be a cheaper option.
Performance Optimization Strategies
To boost the performance of your self-hosted LLM, consider these strategies:
Load-Balancers: Enforce load balancers to supply incoming requests evenly across numerous servers. This precludes any single server from becoming a bottleneck and ensures effective utilization of resources. For instance, utilizing NGINX as a load balancer can help handle traffic and enhance feedback duration.
GPU Usage: Upgrade GPU utilization to manage the calculation requirements of LLMs. Utilize outlining tools to determine performance bottlenecks and adapt the work-load distribution appropriately. For example, using NVIDIA’s CUDA toolkit can help refine GPU performance.
Scalable Architecture:- Design your system to scale reclining, adding more servers as requirement accelerates. This permits you to maintain high performance during culminated utilization periods without overloading your existing infrastructure.
Comparison of HTTP Requests Speeds to LLM Processing Times and the Impact on User Experience
When contrasting HTTP request speeds to LLM processing times, it’s important to comprehend their effect on user experience. HTTPS request speeds usually rely on network suspension, server feedback duration, and the effectiveness of your backend infrastructure. In comparison, LLM processing times are impacted by the intricacies of the model, the hardware used, and the effectiveness of your execution.
For instance, if your HTTP request takes 100 milliseconds but the LLM refining time is 500 milliseconds, the general retaliation duration to the user will be around 600 milliseconds. This dawdle can impact the experience of the user, specifically in apps requiring real-time communications, such as virtual support and chatbots.
To alleviate this, you can enforce methods like:
Asynchronous Processing: Manage requests asynchronously to permit other tasks to proceed while waiting for the LLM to finish its refining.
Caching: Preserve regular feedback to curtail the requirement for recurring LLM refining.
Upgraded Models: Utilize smaller, upgraded models for less intricate questions to reduce refining times.
By meticulously balancing HTTP request managing and LLM refining, you can ensure a receptive and satisfying user experience.
Ensuring security and privacy is crucial when self-hosting LLMs, so let’s dive into the necessary steps to safeguard your data and user trust.
Try RagaAI LLM Hub which helps you get your applications 3X quicker and fix performance, safety and reliability issues across your LLM applications!
Ensuring Security and Privacy
Securing LLM Deployments for Sensitive Information
Safeguarding your large language model (LLM) deployments is uppermost, especially when handling sensitive data. When you self-host an LLM, you’re in charge of your data environment, but this also means you’re reliable for protecting the data. Envision you are handling esoteric client data, proprietary venture data, or personal information- a security infringement could lead to rigorous outcomes, including data stealing, financial loss and harm to your notoriety.
Contemplate the case of a healthcare supplier utilizing an LLM to refine patient data. Any susceptibility could uncover sensitive health data, leading to privacy infringement and legitimate compensation. This makes it important to enforce sturdy security measures to safeguard the data and ensure obedience with regulations such as GDPR and HIPAA.
Using HTTPS and SSL for Secure Connections
One rudimentary step to safeguard your LLM deployment is to utilize HTTPS and SSL for secure connections. HTTPS (Hypertext Transfer Protocol Secure) encodes the data exchanged between your server and clients, averting monitoring and invading. SSL (Secure Sockets Layer) is the fundamental technology that enables this encoding.
For example, when users communicate with your LLM via a website interface or API, HTTPS ensures that any information sent or received is enciphered. This is important for safeguarding login details, query information, and the LLMs replies from being seized by vicious actors. Enforcing HTTPS is direct- get an SSL certificate from a reputed and prominent certificate authority and configure your website server to use it.
Strategies for Maintaining Privacy in Data Processing and API Interactions
Maintaining data privacy during refining and API interactions indulges numerous plans. Initially, unidentified or unnamed private information to avert direct recognition. For instance, supersede names and social security numbers with unique codes before refining.
Next, employ encryption for information at rest and in transit. This ensures that even if information is seized or attained without consent, it stays illegible without the enciphered keys. In addition, execute strict attain control, authorizing data access only to those who need it for their work.
Contemplate also the principle of data minimization, only gather and refine the information significant for the task at hand. For example, if your LLM is used to dissect customer response, avoid gathering extraneous personal information that is not needed for the inspection.
Overview of Potential Security Vulnerabilities and Best Practices to Mitigate Them
Despite your best attempts, potential security vulnerabilities can still present risks. Prevalent risks include SQL injection, cross-site scripting (XSS), and illicit access. To alleviate these risks, adhere to best practices like:-
Frequently update and mend your software to solve known vulnerabilities.
Enforce input verification to avert SQL injection and XSS attacks. For instance, sanitizer user inputs before refining them.
Use strong, special passwords, and enable multi-factor validation (MFA) for accessing your systems.
Demeanor frequent security audits and penetration testing to determine and address vulnerabilities.
Real-world instances emphasize the significance of these practices. For example, a firm might loathe during a security audit that their LLM API was susceptible to an attack that could uncover sensitive customer feedback. By acknowledging the problems immediately and augmenting their security measures, they can avert potential infringement and maintain trust with their users.
By concentrating on these aspects, you can ensure that your self hosted LLM deployments are safe and privacy-compliant, securing both your data and your user’s trust.
Now that we’ve covered the critical aspects of security and privacy, let’s sum up the powerful benefits self-hosting LLMs can bring to your projects.
Conclusion
Self-hosting LLM provides substantial strategic advantages, from improved performance and cost savings to major control over security and personalization. However, equating these benefits needs cautious planning and enforcement.
Beginning with an AIaaS provider and altering to self-hosting as your requirements evolve can be a comprehensive approach.
Enfold the open-source ecosystem for LLM deployment, using community resources and inventiveness to stay at the leading-edge of AI technology. With the right plans, you can utilize the full potential of LLMs, driving inventiveness and accomplishing your aims effectively and safely.
Are you looking for more information on LLMs? Read our other guide on- Multimodal LLMs Using Image and Text.
In today’s high-tech globe, Large Language Models (LLMs) are transforming industries by enabling sophisticated language comprehension and generation expertise.
From generating chatbots and virtual assistants to improving content formation and data analysis, the applications of LLMs are enormous and revolutionizing. However, while the potential of these models is enormous, running them effectively needs high-quality hardware, specifically GPUs, and substantial computational resources.
Many find self-hosting LLMs alluring, as it offers exceptional privacy, safety, and personalization advantages. But how do you determine the intricacies of setting up and handling your own LLM infrastructure?
And how do self-hosted fixes contrast to AI-as-a-Service (AIaaS) platforms such as OpenAI in terms of performance and expense? Let’s delve into practical strategies for self-hosting LLMs and discover the advantages and difficulties indulged.
Selecting the Right Model for Self-hosting
When you’re delving into the globe of self-hosted LLM, it is critical to make informed choices to ensure you get the most out of your speculation. Let’s discover how you can select the right model for your requirements:
Key Considerations for Choosing the Right Model
You need to equate numerous components to ensure the finest performance and cost-effectiveness when choosing an LLM for self-hosting. Here are the key considerations:
Performance per Dollar: You will want to assess how fine a model performs compared to its price. This indulges looking at the hardware requirements and the ongoing functioning costs. High-performing models might deliver spectacular outcomes, but they can also be costly to run. Locating an equation between performance and cost is necessary.
Latency: Low latency is crucial for real-time applications where rapid responses are significant. Make sure to select a model and hardware setup that can deliver the speed you require.
Payload Characteristics: Contemplate the kind of tasks you’ll be performing with the model. Distinct models are upgraded for various kinds of payloads–some might shine at managing huge documents, while others are better suited for short queries. Match the model to your precise use case to ensure effectiveness.
Licensing: Regarding utilization rights, not all LLMs are generated equally. Some models are open-source, while others demand licensing fees. Make sure you comprehend the licensing terms to avoid any legitimate difficulties down the time.
The Intricacy of Model Selection
Choosing the right LLM for self-hosting isn’t a direct task. It involves a deep comprehension of your precise requirements and the abilities of numerous models. Performance standard plays a pivotal role in this procedure. These criteria offer factual data on how distinct models perform under numerous circumstances, helping you make informed choices.
Also Read:- Evaluating Large Language Models: Methods And Metrics
Selecting the Right Hardware
The Necessity of GPUs and Their Cost
Running large models efficiently often needs the muscle of GPUs. GPUs manage the enormous computations required for instructing and inference, making them invaluable for LLMs. However, this power comes with a quoted price. Deep learning tasks often require high-end GPUs, which can be utterly costly. You’ll need to equate the performance gains against the expense connotation, specifically if you are operating with budget limitations.
Nvidia vs. AMD: The GPU Debate
When it comes to GPUs, NVIDIA is an ideal choice for a lot of people in the machine learning community. The consideration? CUDA technology. NVIDIA’s CUDA (Compute Unified Device Architecture) provides a sturdy and mature ecosystem that’s upgraded for deep learning tasks. While AMD GPUs can be prominent, they often lack in this phase due to less pragmatic support for deep learning structures. If you want to ensure conformity and boost performance, NVIDIA GPUs are the best choice.
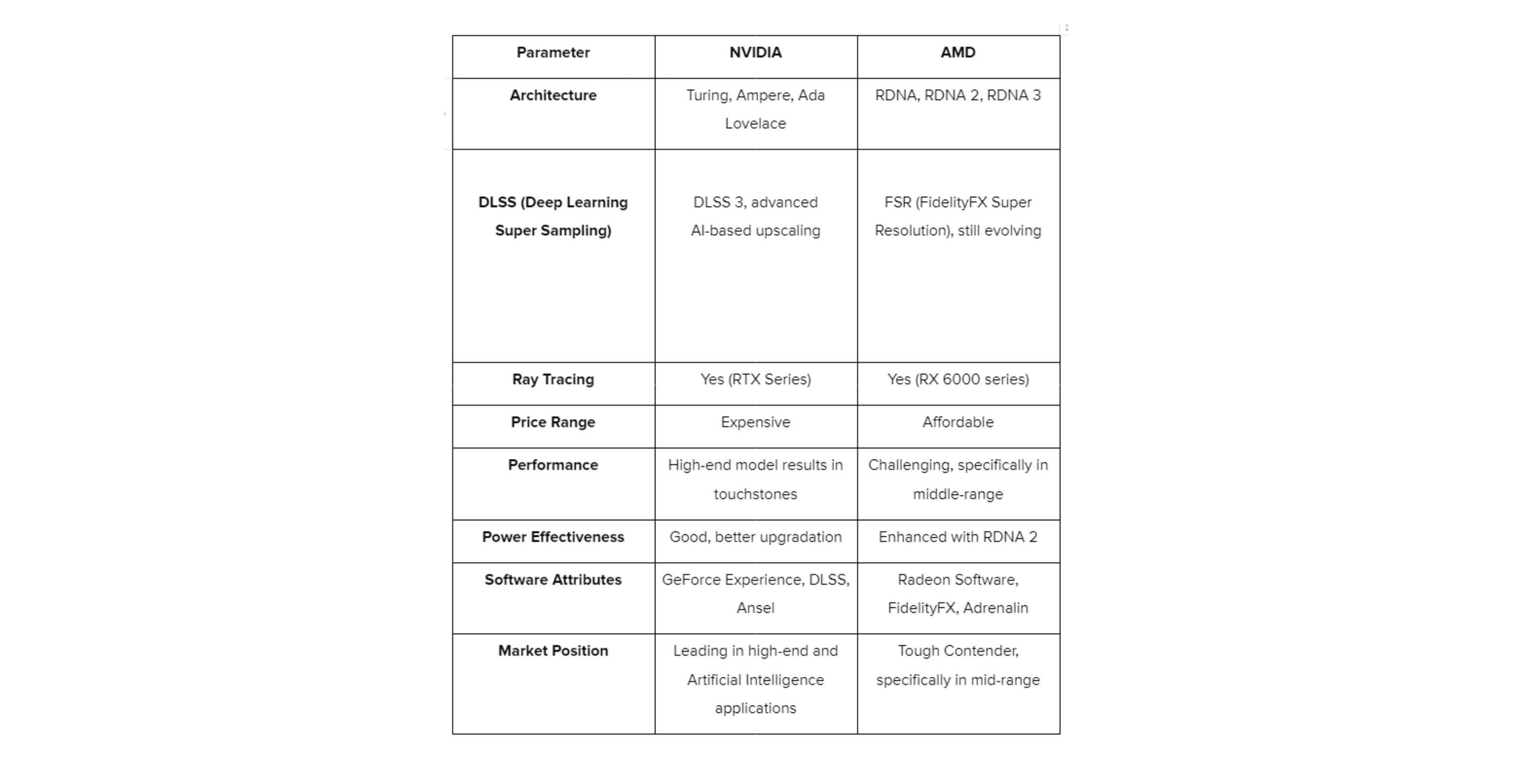
Alternatives to Buying Hardware
Contemplate alternatives such as leasing cloud hardware if putting money into high-end GPUs seems daunting. Cloud suppliers like AWS provide ductile GPU instances that let you compensate for what you utilize without the upfront costs of buying hardware. This adaptability can be groundbreaking, specifically for new ventures or smaller projects.
For less demanding tasks or smaller models, CPUs can sometimes satisfy. Contemporary CPUs are quite prominent and can manage smaller scale induction tasks. This can be an affordable solution if you are not handling extremely large models.
Suggested Hardware Options
For upgraded performance, specifically for self-hosting LLMs, you can’t go wrong with AWS and NVIDIA. AWS provides many options of GPU prototypes customized for deep learning, offering the adaptability to scale as your requirements grow. NVIDIA persists to lead the market with its advanced GPU mechanism, giving solutions that are substantial and hugely assimilated in the Artificial Intelligence community.
By selecting the right hardware, you ensure that your self-hosted LLM runs effectively, saving you time and certainly decreasing budget in the long run.Whether you choose high-end GPUs, lease cloud hardware, or select significant CPUs for minimal tasks, there’s a solution out to meet your requirements.
Also Read:- Comparing Different Large Language Models (LLM)
Deploying and Serving the Model
Deploying and serving your self-hosted LLM can be a groundbreaker for your applications. Let’s delve into some of the best techniques and tools attainable today, concentrating on containerized apps and utilizing Docker for streamlined deployment.
Approaches to Running a Model with a Focus on Containerized Applications
Containerized applications are ideal for adaptability and manageability when it comes to running a model. Containers cluster your application and its reliability into a single unit, ensuring compatible performance across distinct environments. You can run containers on your local machine, on-ground servers, or cloud platforms.
Using Docker, a prominent containerization tool, you can create a structured environment for your LLM. Docker images can summarize your model, its reliability, and any needed configurations, making it simpler to deploy and scale.
Benefits of Model Serving Interfaces like the Text Generation Interface (TGI)
Model serving interfaces, like the Text Generation Interface (TGI), streamline the procedure of deploying and communicating with your LLM. TGI gives a systematic API for serving models, permitting you to concentrate on evolving your apps rather than handling the complexities of model deployment.
With TGI, you acquire:
Operational Convenience: TGI outlines the intricacy of model serving, providing a user-friendly interface to handle your models.
Scalability: TGI sustains ductile deployments, making managing differing burdens easier and ensuring high attainability.
Adaptability: You can incorporate TGI with numerous extremity infrastructures, whether using Kubernetes, Docker Swarm, or other symmetry tools.
Detailed Example of Using Docker to Run and Serve a Model
Let’s explore an instance of using Docker to run and serve an LLM with precise configurations. Suppose you have a pre-trained model hoarded in a directory called my_model.
Create a Dockerfile: The file describes the environment for your model.
FROM python:3.9-slim
WORKDIR /app
COPY my_model /app/my_model
COPY requirements.txt /app/requirements.txt
RUN pip install --no-cache-dir -r requirements.txt
EXPOSE 5000
CMD ["python", "serve_model.py"]
Build the Docker Image: Use the Docker CLI to build your image.
docker build -t my_model_image
Run the Docker Container: Begin a container from your image.
docker run -d -p 5000:5000 --name my_model_container my_model_image
In this setup, serve_model.py is a scenario that establishes and serves your model using a website server. Your model is now operating in a container, attainable on port 5000.
For more information running and building Docker containers from machine learning models, you can refer here!
How to Interact with the Model Using REST API for Generating Predictions
Communicating with your model via Rest API is direct. Here’s how you can send requests to your deployed model to create forecasting:
Send a Post Request: Utilize tools such as curl or Postman to send information to your model .
curl -X POST "http://localhost:5000/predict" -H "Content-Type: application/json" -d '{"input": "Your text here"}'
Process to Response: The model will return a forecast in JSON format. For instance:
{
"output": "Generated text based on your input"
}
Using numerous programming languages, you can incorporate this Rest API call into your app code. Below given is a Python instance using the REQUESTS library:
import requests
url = "http://localhost:5000/predict"
data = {"input": "Your text here"}
response = requests.post(URL, json=data)
print(response.json())
This specifies how easy it is to communicate with your model once it’s ready to run in a containerized environment.
You can refer here for more detail regarding interacting with models using Rest API for generation predictions.
Optimizing Performance and Costs
Exploration of Self-Hosting Costs Versus Using Services Like OpenAI
When considering whether to self-host your large language model (LLM) or use a service like OpenAI, you need to consider the cost and advantages of each option.
Services such as OpenAI provide comfort, manageability, and sturdy infrastructure without requiring you to handle the hardware.
However, these services come at a premium, especially if you have a high utilization demand. Self-hosting can be more economical in the long run but requires an important upfront investment in hardware, setup, and ongoing maintenance.
For example, if you run multiple queries daily, the increasing cost of a service such as OpenAI might surpass the expenditures associated with buying and handling your own servers. The break-even point where self hosting becomes more cheap depends on numerous elements, including the loads of queries, the price of cloud services, and the criticism of your hardware.
Calculations Required to Find When Self-Hosting Becomes Viable
To recognize the feasibility of self-hosting, you need to execute a thorough cost inspection. Here’s a simplified approach:
Initial Investment: Compute the upfront costs for buying servers, GPUs, repositories, and any other significant hardware. For instance, high-end GPUs such as NVIDIA A100 can cost around $10,000 each.
Functional Costs: Indulges electricity, cooling, physical space, and sustained handling. Suppose you have a server that devours 2kW, with an average electricity expenditure of $0.12 per kWh. Over a year, this would amount to around $2,102 in electricity expenditure alone.
Cloud Service Expenditure: Assess the monthly cost of using a cloud service such as OpenAI. For example, OpenAI’s API costs might start from $0.02 to $0.06 per token, relying on the model and utilization tier. If you refine 1 million tokens per day, this can increase your monthly expenditure.
Break Even Analysis: Contrast the total annual expenditure of self-hosting to the paralleled cloud service costs. If the annual expense of utilizing a cloud service transcends the merged initial investment and functional costs of self-hosting within a coherent time frame (e.g., 2-3 years), then self-hosting might be a cheaper option.
Performance Optimization Strategies
To boost the performance of your self-hosted LLM, consider these strategies:
Load-Balancers: Enforce load balancers to supply incoming requests evenly across numerous servers. This precludes any single server from becoming a bottleneck and ensures effective utilization of resources. For instance, utilizing NGINX as a load balancer can help handle traffic and enhance feedback duration.
GPU Usage: Upgrade GPU utilization to manage the calculation requirements of LLMs. Utilize outlining tools to determine performance bottlenecks and adapt the work-load distribution appropriately. For example, using NVIDIA’s CUDA toolkit can help refine GPU performance.
Scalable Architecture:- Design your system to scale reclining, adding more servers as requirement accelerates. This permits you to maintain high performance during culminated utilization periods without overloading your existing infrastructure.
Comparison of HTTP Requests Speeds to LLM Processing Times and the Impact on User Experience
When contrasting HTTP request speeds to LLM processing times, it’s important to comprehend their effect on user experience. HTTPS request speeds usually rely on network suspension, server feedback duration, and the effectiveness of your backend infrastructure. In comparison, LLM processing times are impacted by the intricacies of the model, the hardware used, and the effectiveness of your execution.
For instance, if your HTTP request takes 100 milliseconds but the LLM refining time is 500 milliseconds, the general retaliation duration to the user will be around 600 milliseconds. This dawdle can impact the experience of the user, specifically in apps requiring real-time communications, such as virtual support and chatbots.
To alleviate this, you can enforce methods like:
Asynchronous Processing: Manage requests asynchronously to permit other tasks to proceed while waiting for the LLM to finish its refining.
Caching: Preserve regular feedback to curtail the requirement for recurring LLM refining.
Upgraded Models: Utilize smaller, upgraded models for less intricate questions to reduce refining times.
By meticulously balancing HTTP request managing and LLM refining, you can ensure a receptive and satisfying user experience.
Ensuring security and privacy is crucial when self-hosting LLMs, so let’s dive into the necessary steps to safeguard your data and user trust.
Try RagaAI LLM Hub which helps you get your applications 3X quicker and fix performance, safety and reliability issues across your LLM applications!
Ensuring Security and Privacy
Securing LLM Deployments for Sensitive Information
Safeguarding your large language model (LLM) deployments is uppermost, especially when handling sensitive data. When you self-host an LLM, you’re in charge of your data environment, but this also means you’re reliable for protecting the data. Envision you are handling esoteric client data, proprietary venture data, or personal information- a security infringement could lead to rigorous outcomes, including data stealing, financial loss and harm to your notoriety.
Contemplate the case of a healthcare supplier utilizing an LLM to refine patient data. Any susceptibility could uncover sensitive health data, leading to privacy infringement and legitimate compensation. This makes it important to enforce sturdy security measures to safeguard the data and ensure obedience with regulations such as GDPR and HIPAA.
Using HTTPS and SSL for Secure Connections
One rudimentary step to safeguard your LLM deployment is to utilize HTTPS and SSL for secure connections. HTTPS (Hypertext Transfer Protocol Secure) encodes the data exchanged between your server and clients, averting monitoring and invading. SSL (Secure Sockets Layer) is the fundamental technology that enables this encoding.
For example, when users communicate with your LLM via a website interface or API, HTTPS ensures that any information sent or received is enciphered. This is important for safeguarding login details, query information, and the LLMs replies from being seized by vicious actors. Enforcing HTTPS is direct- get an SSL certificate from a reputed and prominent certificate authority and configure your website server to use it.
Strategies for Maintaining Privacy in Data Processing and API Interactions
Maintaining data privacy during refining and API interactions indulges numerous plans. Initially, unidentified or unnamed private information to avert direct recognition. For instance, supersede names and social security numbers with unique codes before refining.
Next, employ encryption for information at rest and in transit. This ensures that even if information is seized or attained without consent, it stays illegible without the enciphered keys. In addition, execute strict attain control, authorizing data access only to those who need it for their work.
Contemplate also the principle of data minimization, only gather and refine the information significant for the task at hand. For example, if your LLM is used to dissect customer response, avoid gathering extraneous personal information that is not needed for the inspection.
Overview of Potential Security Vulnerabilities and Best Practices to Mitigate Them
Despite your best attempts, potential security vulnerabilities can still present risks. Prevalent risks include SQL injection, cross-site scripting (XSS), and illicit access. To alleviate these risks, adhere to best practices like:-
Frequently update and mend your software to solve known vulnerabilities.
Enforce input verification to avert SQL injection and XSS attacks. For instance, sanitizer user inputs before refining them.
Use strong, special passwords, and enable multi-factor validation (MFA) for accessing your systems.
Demeanor frequent security audits and penetration testing to determine and address vulnerabilities.
Real-world instances emphasize the significance of these practices. For example, a firm might loathe during a security audit that their LLM API was susceptible to an attack that could uncover sensitive customer feedback. By acknowledging the problems immediately and augmenting their security measures, they can avert potential infringement and maintain trust with their users.
By concentrating on these aspects, you can ensure that your self hosted LLM deployments are safe and privacy-compliant, securing both your data and your user’s trust.
Now that we’ve covered the critical aspects of security and privacy, let’s sum up the powerful benefits self-hosting LLMs can bring to your projects.
Conclusion
Self-hosting LLM provides substantial strategic advantages, from improved performance and cost savings to major control over security and personalization. However, equating these benefits needs cautious planning and enforcement.
Beginning with an AIaaS provider and altering to self-hosting as your requirements evolve can be a comprehensive approach.
Enfold the open-source ecosystem for LLM deployment, using community resources and inventiveness to stay at the leading-edge of AI technology. With the right plans, you can utilize the full potential of LLMs, driving inventiveness and accomplishing your aims effectively and safely.
Are you looking for more information on LLMs? Read our other guide on- Multimodal LLMs Using Image and Text.
Subscribe to our newsletter to never miss an update
Other articles


Agents Talk, Models Think: A2A + MCP for Enterprise Agentic AI

Riya Parikh, Nitai Agarwal
Apr 27, 2025
Read the article
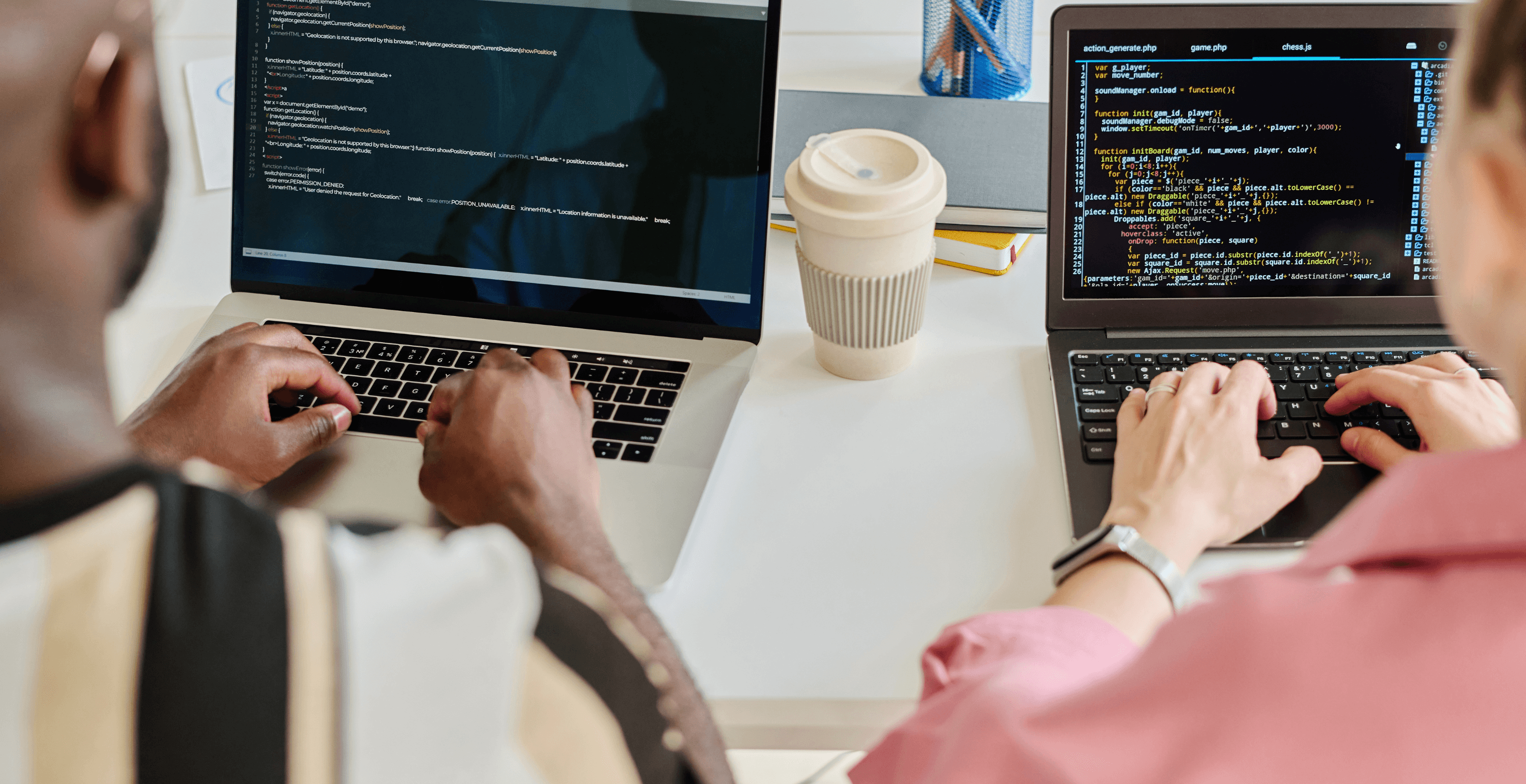
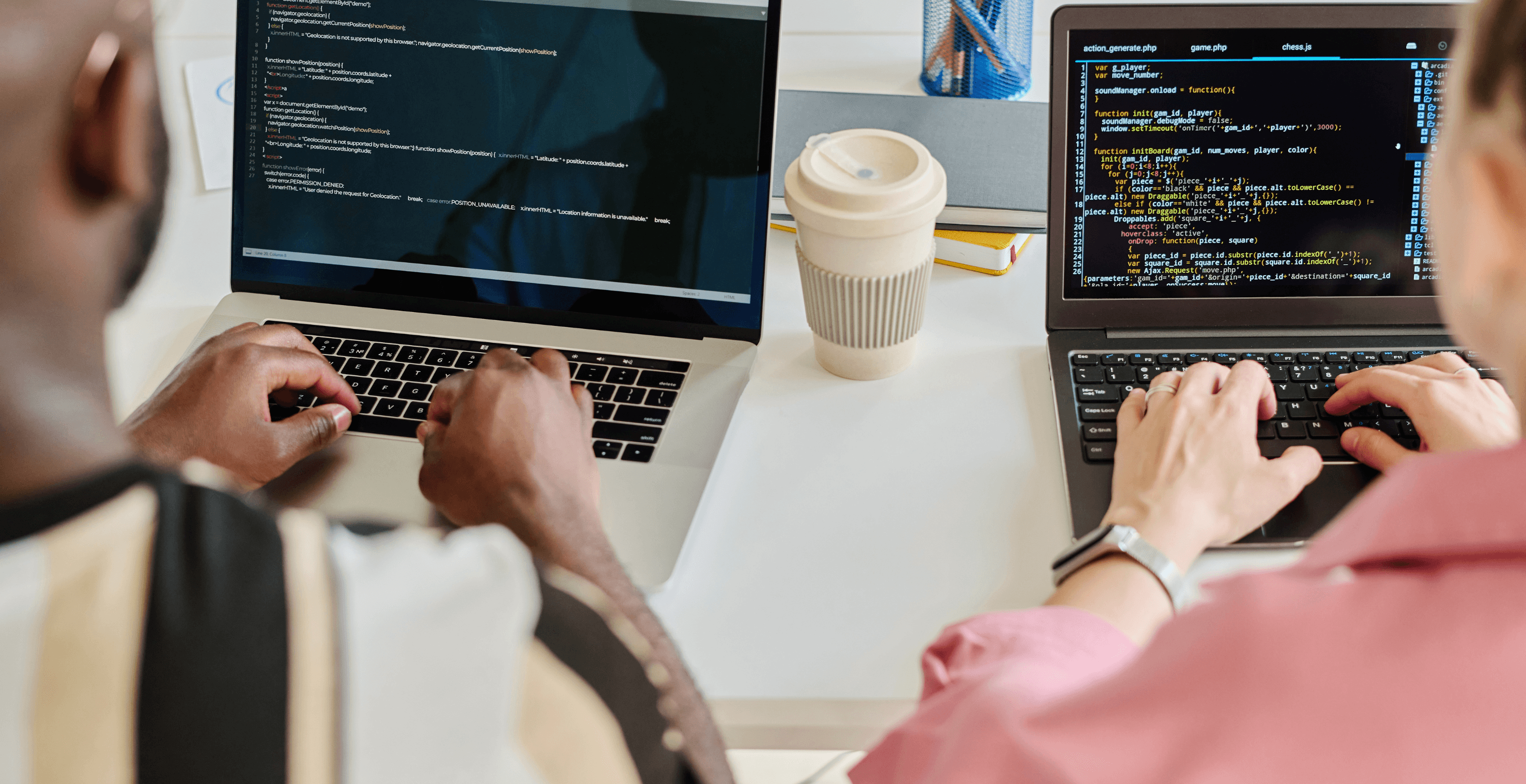
Understanding NeMo Guardrails: A Toolkit for LLM Security

Rehan Asif
Dec 24, 2024
Read the article


Understanding Differences in Large vs Small Language Models (LLM vs SLM)

Rehan Asif
Dec 21, 2024
Read the article