Multimodal LLMS Using Image And Text
Multimodal LLMS Using Image And Text
Multimodal LLMS Using Image And Text
Rehan Asif
Apr 30, 2024




Imagine having a conversation in which you can describe a scene and show a picture of it to convey your message more vividly. That's what multimodal prompts do for LLMs.
These prompts feed AI not just text but also other forms of data like images, enabling a richer, more context-aware response. This capability transforms how LLMs understand and interact with the world, making them readers, writers, and comprehensive interpreters of information.
Integrating image and text inputs allows LLMs to process and generate information that reflects a deeper understanding of textual descriptions and visual representations.
This integration involves sophisticated models that can analyze an image's content, relate it to textual data, and generate coherent outputs that blend insights from both.
For example, given a picture of a street scene and a query about the weather, the LLM can recognize visual cues in the image to determine whether it’s rainy, sunny, or cloudy and respond appropriately.
Understanding Multimodal Inputs
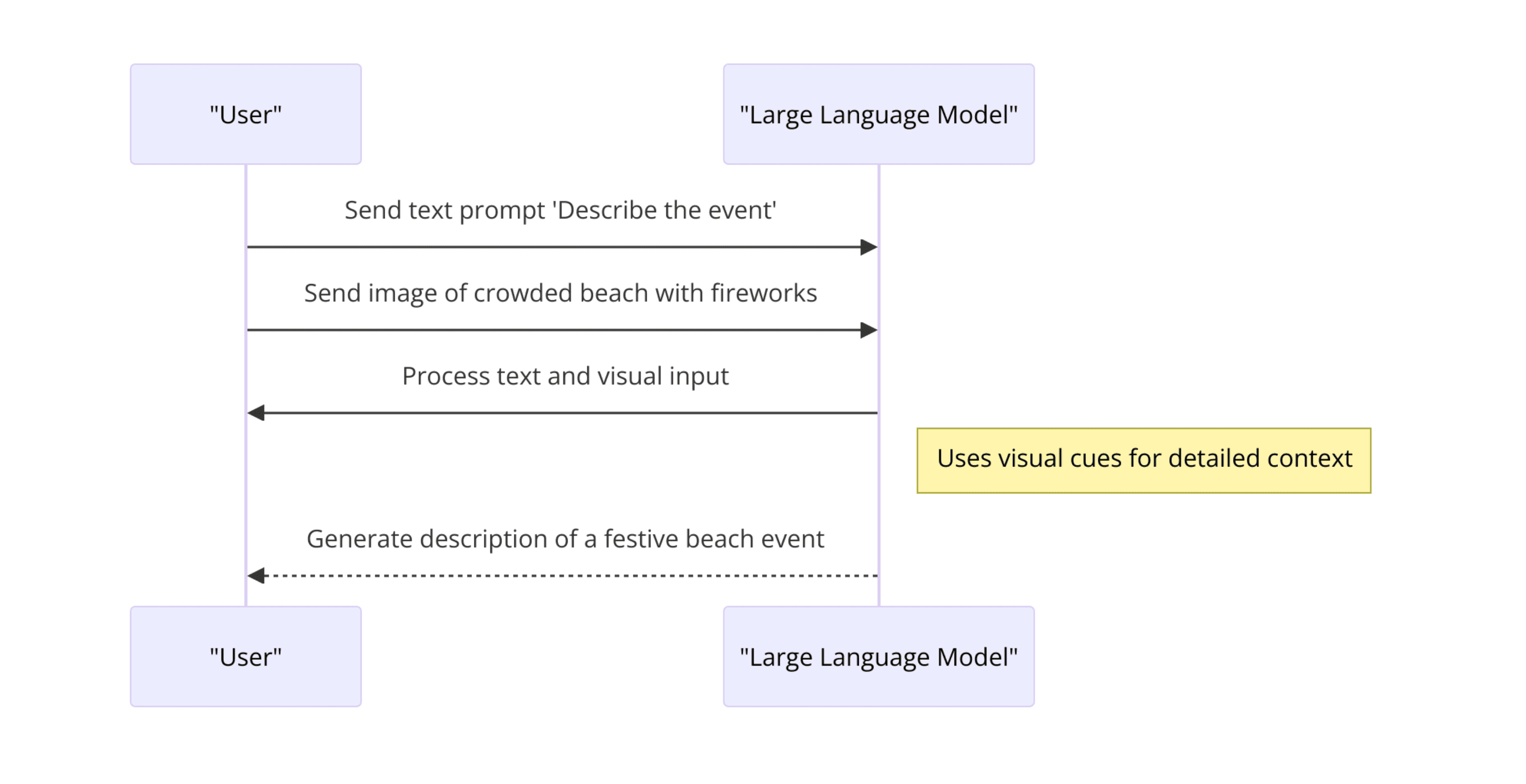
Multimodal prompting in the context of Large Language Models refers to using more than one type of data—such as text and images—to elicit a response from the model.
This approach allows the LLM to draw from a richer information set, enhancing its ability to provide more accurate and contextually relevant outputs. The model can make connections and inferences that are impossible with text alone by processing textual descriptions and visual elements.
Consider a scenario where an LLM is given a text prompt, "Describe the event," and an image of a crowded beach with fireworks. The model uses the visual cues from the image—such as the presence of people, the setting, and the fireworks—to generate a detailed description that complements the textual query, potentially describing a festive beach event celebrating a national holiday.
Read more on RagaAI’s Multimodal Input Capabilities
Text-Only Response Mechanism
The Gemini model exemplifies a sophisticated approach within multimodal systems. It focuses exclusively on generating text-based responses by leveraging dual mechanisms—one that processes text and another that translates visual information into textual concepts. Even when only text output is required, Gemini utilizes the visual data to enrich the context of its responses, making it especially powerful in scenarios where the text alone might not convey the full picture.
Overview of Multimodal LLM Architecture
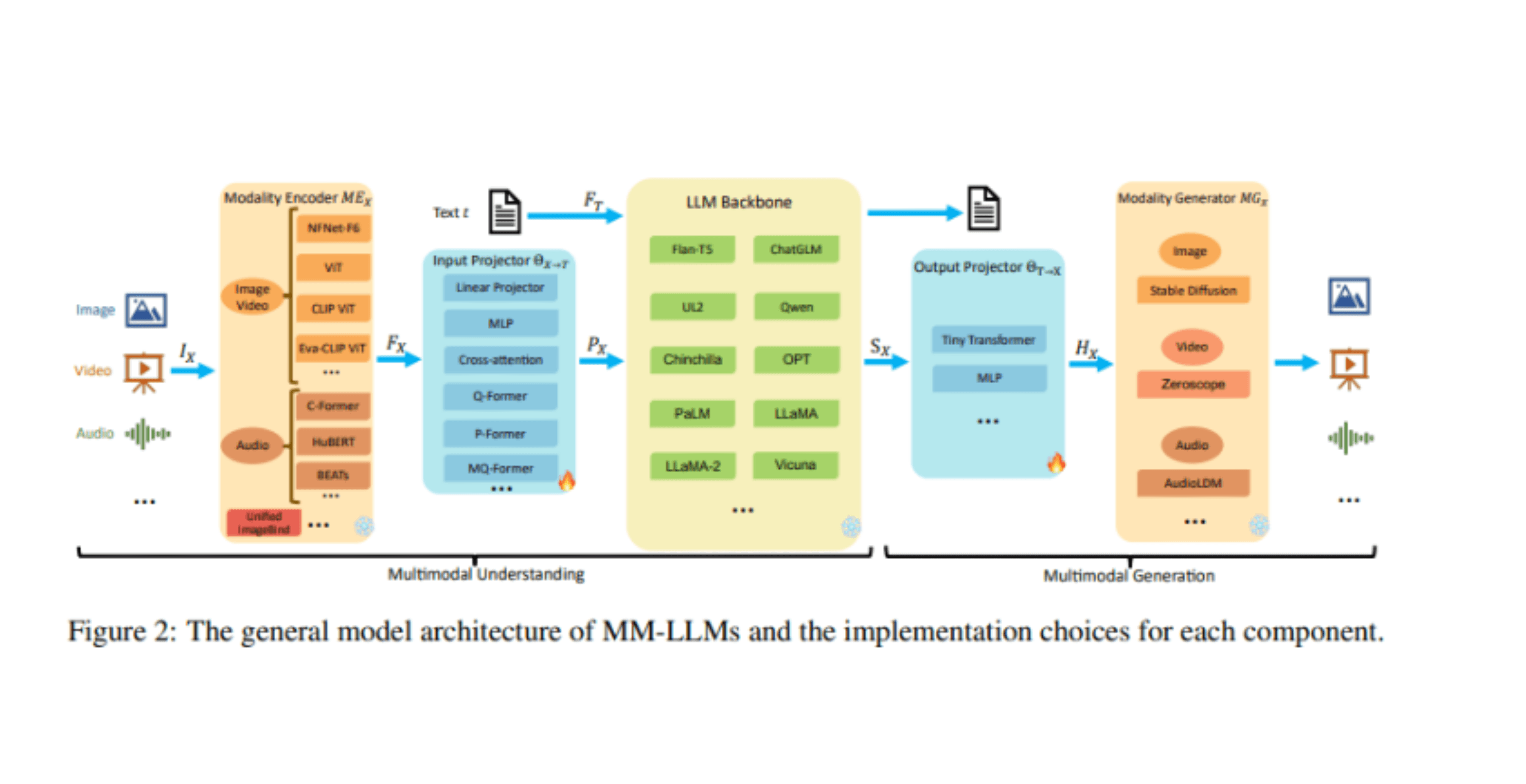
Source: Website
The architecture of Multimodal LLMs (MM-LLMs) is composed of five main components, each playing a crucial role in the model's functionality:
Modality Encoder
Input Projector
LLM Backbone
Output Projector
Modality Generator
1. Modality Encoder
The Modality Encoder (ME) encodes inputs from various modalities (image, video, audio, 3D, etc.) into corresponding features FXF_XFX. This is formulated as: FX=MEX(IX)F_X = ME_X(I_X)FX=MEX(IX)
Visual Modality
For images, multiple encoders can be used, such as NFNet-F6, ViT, CLIP ViT, Eva-CLIP ViT, and others. For videos, frames are uniformly sampled and processed similarly to images.
Audio Modality
Common encoders include C-Former, HuBERT, BEATs, Whisper, and CLAP.
3D Point Cloud Modality
Typically encoded by ULIP-2 with a PointBERT backbone.
Unified encoders like ImageBind handle multiple modalities, including image/video, text, audio, heat maps, inertial measurement units, and depth.
2. Input Projector
The Input Projector aligns encoded features FXF_XFX with the text feature space TTT. The aligned features PXP_XPX are fed into the LLM Backbone alongside textual features FTF_TFT. The goal is to minimize the X-conditioned text generation loss. The alignment can be achieved using Linear Projectors, Multi-Layer Perceptrons (MLPs), or more complex methods like Cross-attention, Q-Former, P-Former, and MQ-Former.
3. LLM Backbone
The LLM Backbone is the core of MM-LLMs, inheriting properties like zero-shot generalization, few-shot in-context learning (ICL), Chain-of-Thought (CoT), and instruction following. It processes representations from various modalities, engaging in semantic understanding, reasoning, and decision-making. The backbone outputs direct textual responses and signal tokens SXS_XSX for other modalities, guiding the Modality Generator.
Popular LLMs include Flan-T5, ChatGLM, UL2, Qwen, Chinchilla, OPT, PaLM, LLaMA, and Vicuna.
4. Output Projector
The Output Projector maps signal token representations from the LLM Backbone into features understandable by the Modality Generator. The goal is to align HXH_XHX with the conditional text representations of MGXMG_XMGX. Implementations include Tiny Transformers or MLPs.
5. Modality Generator
The Modality Generator MGXMG_XMGX produces outputs in various modalities. Commonly used generators include Latent Diffusion Models (LDMs) like Stable Diffusion for images, Zeroscope for videos, and AudioLDM for audio. These generators use features HXH_XHX from the Output Projector as conditional inputs to generate multimodal content.
During training, the ground truth content is transformed into a latent feature z0z_0z0 by a pre-trained VAE, noise ϵ\epsilonϵ is added, and a pre-trained UNet computes the conditional LDM loss LX−genL_{X-gen}LX−gen.
Read more on CLIP Integration in RagaAI
Methodology for Enhancing LLM with Multimodal Inputs
These methodologies enhance the functional capabilities of LLMs and ensure that the outputs are deeply aligned with the input contexts, leading to more effective and user-centric applications.
Prompt Parsing and Extension Agent Roles
In multimodal LLMs, prompt parsing plays a crucial role. This process involves breaking down the input prompts into understandable segments the model can process.
The extension agent's role is to expand these prompts based on the context provided by multimodal data, effectively enhancing the model's understanding and response capability.
For instance, if the text prompt is ambiguous or lacks detail, the extension agent can use information from image or audio inputs to add specificity and relevance to the prompt, guiding the model toward a more accurate output.
Tree of Thought of Models and its Construction
The Tree of Thought is a conceptual framework used in some advanced LLMs to organize and process complex inputs. By constructing a decision tree-like structure, the model can navigate through different layers of information, from general to specific, integrating insights from various modalities.
This structure helps the LLM maintain context and coherence across extended interactions or complex query sequences, improving its problem-solving capabilities and response accuracy.
Model Selection
Model selection is often guided by human feedback and data from 'advantage databases'—repositories of interactions and outcomes highlighting which model configurations perform best under specific conditions. By analyzing this data, developers can fine-tune the model selection process, choosing or adjusting LLM configurations that maximize performance for particular types of multimodal inputs.
Execution of Generation for Personalized Image and Text Outputs
The final step in the methodology involves executing the generation of outputs tailored to the specific requirements of the task at hand. Whether generating a text description based on an image or creating a personalized response to a multimodal query, the LLM utilizes its integrated understanding of textual, visual, and auditory data to produce precise and contextually relevant outputs.descriptive text from an image or responding to a complex query.
Experimentation and Results
Let's delve into the experimental setup and results that evaluate the effectiveness of multimodal Large Language Models (LLMs), examining how these models perform in practical applications and the improvements achieved through sophisticated multimodal integration.
To rigorously test the efficacy of multimodal LLMs, researchers set up controlled experiments comparing different model configurations.
These settings often vary in terms of the types and amounts of multimodal data used and the specific tasks being tested, such as image-text correlation or audio-text integration.
These experiments use standardized datasets and transparent performance metrics to objectively assess how well multimodal LLMs handle complex, real-world tasks compared to their unimodal counterparts or earlier model versions.
Semantic Alignment and Aesthetic Comparison in Images
One key area of experimentation involves assessing semantic alignment—how accurately the model's outputs align with the meanings conveyed in the multimodal inputs.
For example, if an image shows a rainy street, does the text generated by the model accurately describe the scene? Aesthetic comparison tests might evaluate how pleasing or contextually appropriate generated images are when the model is prompted to create visual content based on textual descriptions.
Improvements in Image Reward and Aesthetic Score
Results from these experiments often show significant improvements in 'image reward'—a metric that evaluates how well the generated images meet the user-defined objectives—and 'aesthetic score,' which assesses the visual appeal and relevance of images generated by the LLM.
These improvements highlight the model's enhanced ability to effectively interpret and integrate multimodal data.
User Study
Finally, user studies provide invaluable feedback on how users perceive and value the outputs from multimodal LLMs. Participants might be asked to rate the relevance, coherence, and usefulness of the model's responses in various scenarios, providing direct insights into the user experience.
These studies often reveal a strong preference for outputs generated by enhanced multimodal systems, confirming their practical benefits.
These experimental findings play a crucial role in validating the advancements in multimodal LLMs, demonstrating their enhanced capabilities and their tangible benefits to users. As we progress, we will explore the applications of these powerful tools across various domains.
Applications of Multimodal LLMs
Let’s explore the diverse applications of multimodal Large Language Models (LLMs), showcasing how they are effectively utilized in various scenarios, from simple tasks to complex cognitive functions.
Simple and Advanced Multimodal Prompts
Multimodal LLMs are adept at handling simple and advanced prompts that integrate text, images, or audio to create more engaging and informative responses. For instance, a simple application generates a text description for an uploaded image, while more advanced uses include developing a story based on a series of pictures and text prompts, demonstrating the model's ability to weave together narrative elements from different modalities.
Classification, Recognition, and Counting Using Multimodal Inputs
In more structured tasks, multimodal LLMs excel at classification, recognition, and counting within complex environments. For example, in a medical diagnosis application, an LLM might analyze X-ray images alongside clinical notes to accurately identify and classify medical conditions. Similarly, in retail or surveillance settings, these models can count objects and recognize specific activities or items from video footage accompanied by descriptive audio or text data.
Creative Storytelling and Logical Reasoning Through Image-Text Synthesis
One of the most exciting applications of multimodal LLMs lies in creative storytelling and logical reasoning. These models can generate imaginative stories or detailed explanations based on a mix of visual and textual cues, engaging users with content that is both creative and contextually relevant. This capability is not only entertaining but also useful in educational settings where blending visual learning materials with explanatory text can help illustrate complex concepts more clearly.# Exam
These applications illustrate the versatility and power of multimodal LLMs, enabling them to operate effectively across a wide range of domains and scenarios. Next, we will discuss these technologies' challenges and future directions, considering the ongoing developments and potential for further advancements.
Challenges and Future Directions
Despite the challenges, the potential for multimodal LLMs to transform various industries and applications remains substantial.
As we continue to advance these technologies, their integration into everyday tools and platforms will likely become more pervasive, driving innovation and creating new opportunities for interaction and automation.
Limitations of Current Stable Diffusion Models and DiffusionGPT
While multimodal LLMs like DiffusionGPT offer impressive capabilities, they face limitations in terms of the stability and fidelity of generated outputs, particularly under complex or nuanced scenarios. Current diffusion models may need to help to maintain consistency in long sequences or detailed scenarios, which can result in less coherent or visually disconnected outputs.
Addressing these challenges requires ongoing research and development to refine the models' understanding and processing of multimodal data.
Read more on Integrating DiffusionGPT at RagaAI
Feedback-Driven Optimization
Feedback-driven optimization represents a promising approach to enhancing multimodal LLMs. By systematically incorporating user feedback into the training loop, developers can fine-tune the models to better meet user expectations and improve performance across varied tasks.
This process helps identify and correct specific areas where the models may fall short, such as handling subtleties of human emotion or complex logical reasoning.
Expansion of Model Candidates
The future of multimodal LLMs also lies in expanding the pool of model candidates beyond the current mainstream options. Exploring alternative architectures or hybrid models that combine different types of neural networks might offer new ways to handle the intricacies of multimodal data more effectively. This expansion could lead to breakthroughs in how these models process and integrate diverse inputs, potentially unlocking new applications and improving existing functionalities.
Conclusion
The integration of image and text inputs into LLMs has significantly expanded their capabilities, making them more efficient in processing information and vastly more versatile in their applications.
In conclusion, integrating multimodal inputs into LLMs represents a significant step forward in the evolution of AI technologies.
By continuing to develop and experiment with these models, we can unlock a future where AI assists us more seamlessly in our daily lives, enhancing our abilities and enriching our understanding of the world around us.
Ready to transform your approach to AI with cutting-edge insights and tools? Explore RagaAI's comprehensive suite of AI solutions — from enhancing AI reliability with our guardrails to navigating the complexities of AI governance. Whether you're diving into prompt engineering, seeking to mitigate AI biases, or exploring the frontier of AI testing
Imagine having a conversation in which you can describe a scene and show a picture of it to convey your message more vividly. That's what multimodal prompts do for LLMs.
These prompts feed AI not just text but also other forms of data like images, enabling a richer, more context-aware response. This capability transforms how LLMs understand and interact with the world, making them readers, writers, and comprehensive interpreters of information.
Integrating image and text inputs allows LLMs to process and generate information that reflects a deeper understanding of textual descriptions and visual representations.
This integration involves sophisticated models that can analyze an image's content, relate it to textual data, and generate coherent outputs that blend insights from both.
For example, given a picture of a street scene and a query about the weather, the LLM can recognize visual cues in the image to determine whether it’s rainy, sunny, or cloudy and respond appropriately.
Understanding Multimodal Inputs
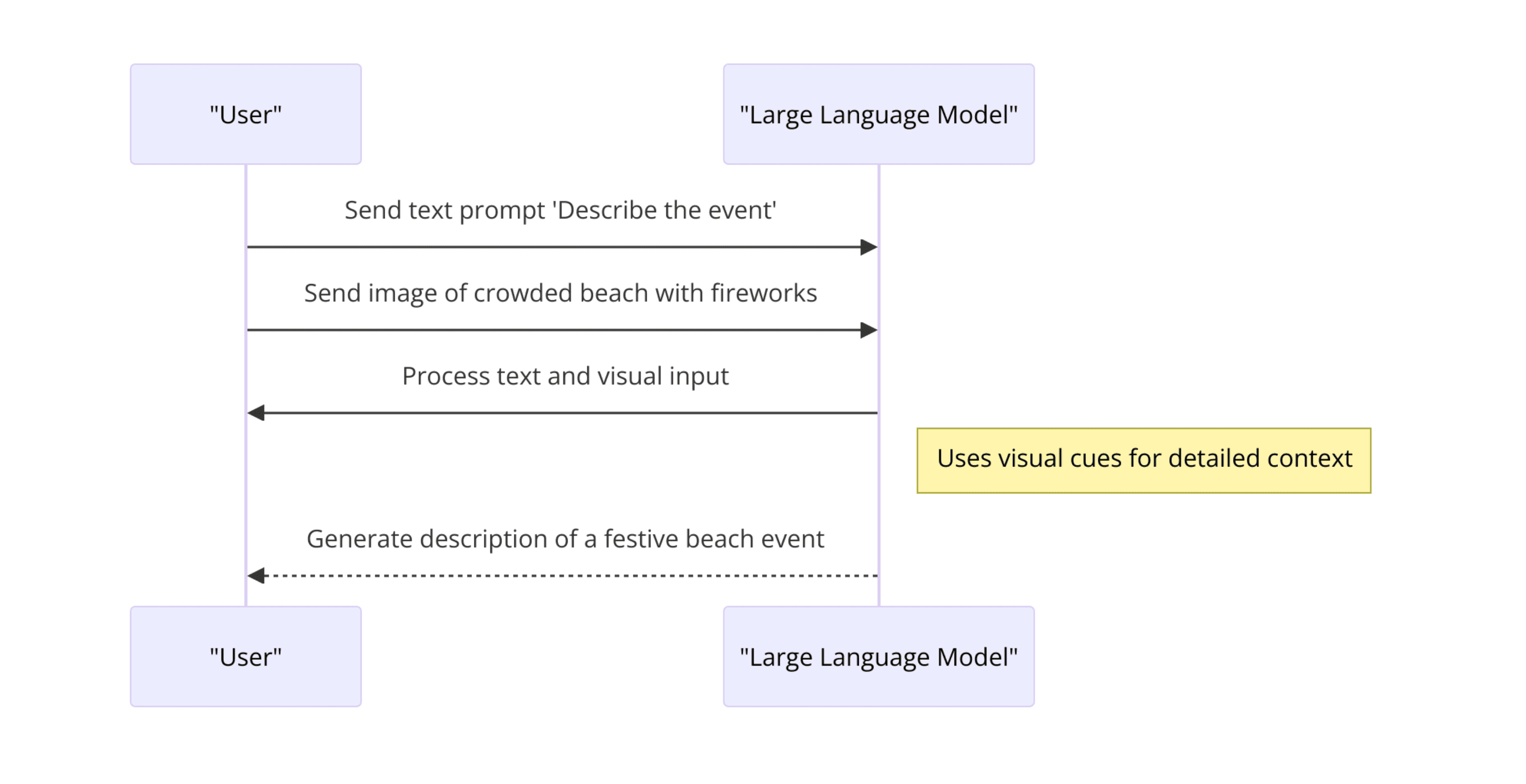
Multimodal prompting in the context of Large Language Models refers to using more than one type of data—such as text and images—to elicit a response from the model.
This approach allows the LLM to draw from a richer information set, enhancing its ability to provide more accurate and contextually relevant outputs. The model can make connections and inferences that are impossible with text alone by processing textual descriptions and visual elements.
Consider a scenario where an LLM is given a text prompt, "Describe the event," and an image of a crowded beach with fireworks. The model uses the visual cues from the image—such as the presence of people, the setting, and the fireworks—to generate a detailed description that complements the textual query, potentially describing a festive beach event celebrating a national holiday.
Read more on RagaAI’s Multimodal Input Capabilities
Text-Only Response Mechanism
The Gemini model exemplifies a sophisticated approach within multimodal systems. It focuses exclusively on generating text-based responses by leveraging dual mechanisms—one that processes text and another that translates visual information into textual concepts. Even when only text output is required, Gemini utilizes the visual data to enrich the context of its responses, making it especially powerful in scenarios where the text alone might not convey the full picture.
Overview of Multimodal LLM Architecture
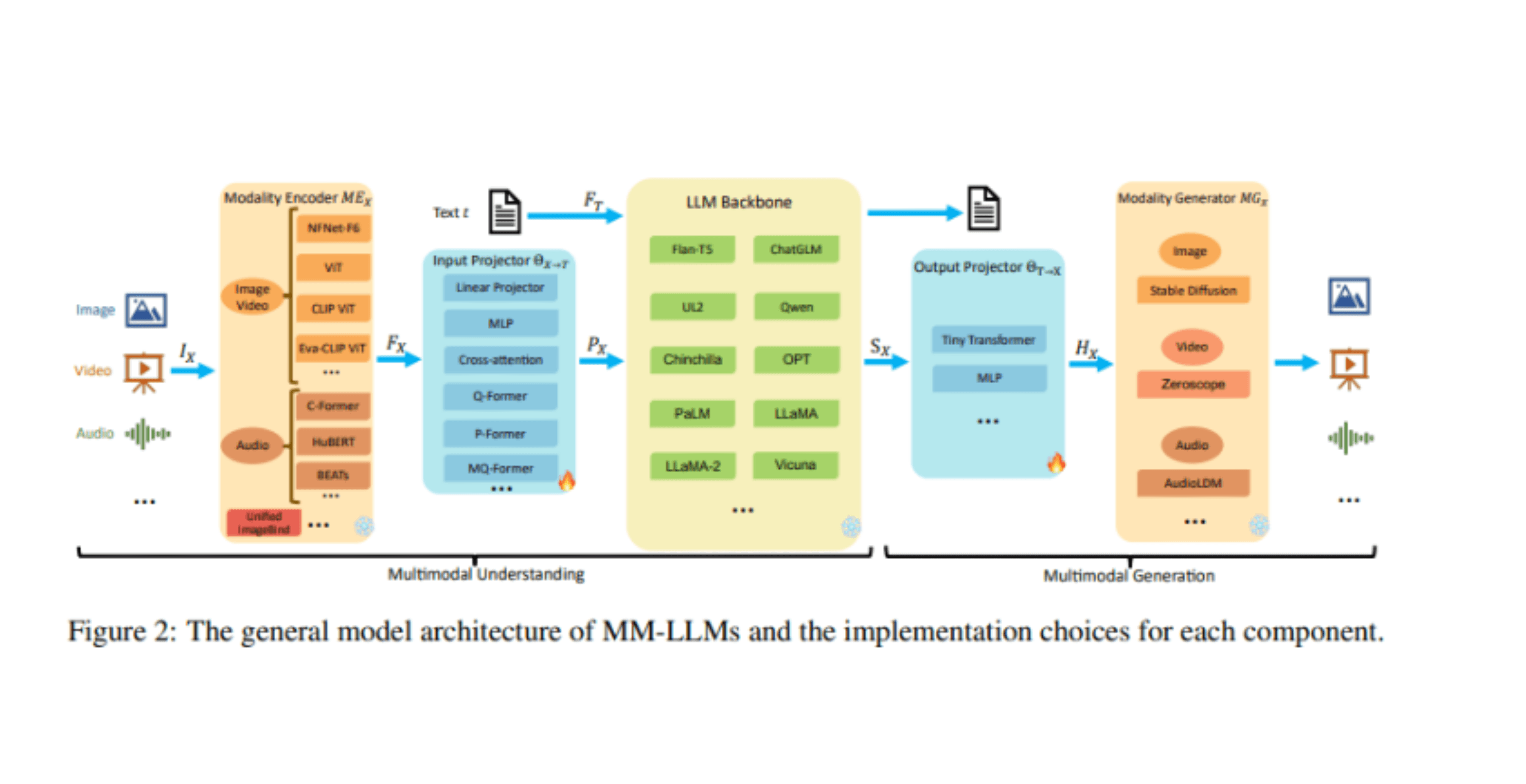
Source: Website
The architecture of Multimodal LLMs (MM-LLMs) is composed of five main components, each playing a crucial role in the model's functionality:
Modality Encoder
Input Projector
LLM Backbone
Output Projector
Modality Generator
1. Modality Encoder
The Modality Encoder (ME) encodes inputs from various modalities (image, video, audio, 3D, etc.) into corresponding features FXF_XFX. This is formulated as: FX=MEX(IX)F_X = ME_X(I_X)FX=MEX(IX)
Visual Modality
For images, multiple encoders can be used, such as NFNet-F6, ViT, CLIP ViT, Eva-CLIP ViT, and others. For videos, frames are uniformly sampled and processed similarly to images.
Audio Modality
Common encoders include C-Former, HuBERT, BEATs, Whisper, and CLAP.
3D Point Cloud Modality
Typically encoded by ULIP-2 with a PointBERT backbone.
Unified encoders like ImageBind handle multiple modalities, including image/video, text, audio, heat maps, inertial measurement units, and depth.
2. Input Projector
The Input Projector aligns encoded features FXF_XFX with the text feature space TTT. The aligned features PXP_XPX are fed into the LLM Backbone alongside textual features FTF_TFT. The goal is to minimize the X-conditioned text generation loss. The alignment can be achieved using Linear Projectors, Multi-Layer Perceptrons (MLPs), or more complex methods like Cross-attention, Q-Former, P-Former, and MQ-Former.
3. LLM Backbone
The LLM Backbone is the core of MM-LLMs, inheriting properties like zero-shot generalization, few-shot in-context learning (ICL), Chain-of-Thought (CoT), and instruction following. It processes representations from various modalities, engaging in semantic understanding, reasoning, and decision-making. The backbone outputs direct textual responses and signal tokens SXS_XSX for other modalities, guiding the Modality Generator.
Popular LLMs include Flan-T5, ChatGLM, UL2, Qwen, Chinchilla, OPT, PaLM, LLaMA, and Vicuna.
4. Output Projector
The Output Projector maps signal token representations from the LLM Backbone into features understandable by the Modality Generator. The goal is to align HXH_XHX with the conditional text representations of MGXMG_XMGX. Implementations include Tiny Transformers or MLPs.
5. Modality Generator
The Modality Generator MGXMG_XMGX produces outputs in various modalities. Commonly used generators include Latent Diffusion Models (LDMs) like Stable Diffusion for images, Zeroscope for videos, and AudioLDM for audio. These generators use features HXH_XHX from the Output Projector as conditional inputs to generate multimodal content.
During training, the ground truth content is transformed into a latent feature z0z_0z0 by a pre-trained VAE, noise ϵ\epsilonϵ is added, and a pre-trained UNet computes the conditional LDM loss LX−genL_{X-gen}LX−gen.
Read more on CLIP Integration in RagaAI
Methodology for Enhancing LLM with Multimodal Inputs
These methodologies enhance the functional capabilities of LLMs and ensure that the outputs are deeply aligned with the input contexts, leading to more effective and user-centric applications.
Prompt Parsing and Extension Agent Roles
In multimodal LLMs, prompt parsing plays a crucial role. This process involves breaking down the input prompts into understandable segments the model can process.
The extension agent's role is to expand these prompts based on the context provided by multimodal data, effectively enhancing the model's understanding and response capability.
For instance, if the text prompt is ambiguous or lacks detail, the extension agent can use information from image or audio inputs to add specificity and relevance to the prompt, guiding the model toward a more accurate output.
Tree of Thought of Models and its Construction
The Tree of Thought is a conceptual framework used in some advanced LLMs to organize and process complex inputs. By constructing a decision tree-like structure, the model can navigate through different layers of information, from general to specific, integrating insights from various modalities.
This structure helps the LLM maintain context and coherence across extended interactions or complex query sequences, improving its problem-solving capabilities and response accuracy.
Model Selection
Model selection is often guided by human feedback and data from 'advantage databases'—repositories of interactions and outcomes highlighting which model configurations perform best under specific conditions. By analyzing this data, developers can fine-tune the model selection process, choosing or adjusting LLM configurations that maximize performance for particular types of multimodal inputs.
Execution of Generation for Personalized Image and Text Outputs
The final step in the methodology involves executing the generation of outputs tailored to the specific requirements of the task at hand. Whether generating a text description based on an image or creating a personalized response to a multimodal query, the LLM utilizes its integrated understanding of textual, visual, and auditory data to produce precise and contextually relevant outputs.descriptive text from an image or responding to a complex query.
Experimentation and Results
Let's delve into the experimental setup and results that evaluate the effectiveness of multimodal Large Language Models (LLMs), examining how these models perform in practical applications and the improvements achieved through sophisticated multimodal integration.
To rigorously test the efficacy of multimodal LLMs, researchers set up controlled experiments comparing different model configurations.
These settings often vary in terms of the types and amounts of multimodal data used and the specific tasks being tested, such as image-text correlation or audio-text integration.
These experiments use standardized datasets and transparent performance metrics to objectively assess how well multimodal LLMs handle complex, real-world tasks compared to their unimodal counterparts or earlier model versions.
Semantic Alignment and Aesthetic Comparison in Images
One key area of experimentation involves assessing semantic alignment—how accurately the model's outputs align with the meanings conveyed in the multimodal inputs.
For example, if an image shows a rainy street, does the text generated by the model accurately describe the scene? Aesthetic comparison tests might evaluate how pleasing or contextually appropriate generated images are when the model is prompted to create visual content based on textual descriptions.
Improvements in Image Reward and Aesthetic Score
Results from these experiments often show significant improvements in 'image reward'—a metric that evaluates how well the generated images meet the user-defined objectives—and 'aesthetic score,' which assesses the visual appeal and relevance of images generated by the LLM.
These improvements highlight the model's enhanced ability to effectively interpret and integrate multimodal data.
User Study
Finally, user studies provide invaluable feedback on how users perceive and value the outputs from multimodal LLMs. Participants might be asked to rate the relevance, coherence, and usefulness of the model's responses in various scenarios, providing direct insights into the user experience.
These studies often reveal a strong preference for outputs generated by enhanced multimodal systems, confirming their practical benefits.
These experimental findings play a crucial role in validating the advancements in multimodal LLMs, demonstrating their enhanced capabilities and their tangible benefits to users. As we progress, we will explore the applications of these powerful tools across various domains.
Applications of Multimodal LLMs
Let’s explore the diverse applications of multimodal Large Language Models (LLMs), showcasing how they are effectively utilized in various scenarios, from simple tasks to complex cognitive functions.
Simple and Advanced Multimodal Prompts
Multimodal LLMs are adept at handling simple and advanced prompts that integrate text, images, or audio to create more engaging and informative responses. For instance, a simple application generates a text description for an uploaded image, while more advanced uses include developing a story based on a series of pictures and text prompts, demonstrating the model's ability to weave together narrative elements from different modalities.
Classification, Recognition, and Counting Using Multimodal Inputs
In more structured tasks, multimodal LLMs excel at classification, recognition, and counting within complex environments. For example, in a medical diagnosis application, an LLM might analyze X-ray images alongside clinical notes to accurately identify and classify medical conditions. Similarly, in retail or surveillance settings, these models can count objects and recognize specific activities or items from video footage accompanied by descriptive audio or text data.
Creative Storytelling and Logical Reasoning Through Image-Text Synthesis
One of the most exciting applications of multimodal LLMs lies in creative storytelling and logical reasoning. These models can generate imaginative stories or detailed explanations based on a mix of visual and textual cues, engaging users with content that is both creative and contextually relevant. This capability is not only entertaining but also useful in educational settings where blending visual learning materials with explanatory text can help illustrate complex concepts more clearly.# Exam
These applications illustrate the versatility and power of multimodal LLMs, enabling them to operate effectively across a wide range of domains and scenarios. Next, we will discuss these technologies' challenges and future directions, considering the ongoing developments and potential for further advancements.
Challenges and Future Directions
Despite the challenges, the potential for multimodal LLMs to transform various industries and applications remains substantial.
As we continue to advance these technologies, their integration into everyday tools and platforms will likely become more pervasive, driving innovation and creating new opportunities for interaction and automation.
Limitations of Current Stable Diffusion Models and DiffusionGPT
While multimodal LLMs like DiffusionGPT offer impressive capabilities, they face limitations in terms of the stability and fidelity of generated outputs, particularly under complex or nuanced scenarios. Current diffusion models may need to help to maintain consistency in long sequences or detailed scenarios, which can result in less coherent or visually disconnected outputs.
Addressing these challenges requires ongoing research and development to refine the models' understanding and processing of multimodal data.
Read more on Integrating DiffusionGPT at RagaAI
Feedback-Driven Optimization
Feedback-driven optimization represents a promising approach to enhancing multimodal LLMs. By systematically incorporating user feedback into the training loop, developers can fine-tune the models to better meet user expectations and improve performance across varied tasks.
This process helps identify and correct specific areas where the models may fall short, such as handling subtleties of human emotion or complex logical reasoning.
Expansion of Model Candidates
The future of multimodal LLMs also lies in expanding the pool of model candidates beyond the current mainstream options. Exploring alternative architectures or hybrid models that combine different types of neural networks might offer new ways to handle the intricacies of multimodal data more effectively. This expansion could lead to breakthroughs in how these models process and integrate diverse inputs, potentially unlocking new applications and improving existing functionalities.
Conclusion
The integration of image and text inputs into LLMs has significantly expanded their capabilities, making them more efficient in processing information and vastly more versatile in their applications.
In conclusion, integrating multimodal inputs into LLMs represents a significant step forward in the evolution of AI technologies.
By continuing to develop and experiment with these models, we can unlock a future where AI assists us more seamlessly in our daily lives, enhancing our abilities and enriching our understanding of the world around us.
Ready to transform your approach to AI with cutting-edge insights and tools? Explore RagaAI's comprehensive suite of AI solutions — from enhancing AI reliability with our guardrails to navigating the complexities of AI governance. Whether you're diving into prompt engineering, seeking to mitigate AI biases, or exploring the frontier of AI testing
Imagine having a conversation in which you can describe a scene and show a picture of it to convey your message more vividly. That's what multimodal prompts do for LLMs.
These prompts feed AI not just text but also other forms of data like images, enabling a richer, more context-aware response. This capability transforms how LLMs understand and interact with the world, making them readers, writers, and comprehensive interpreters of information.
Integrating image and text inputs allows LLMs to process and generate information that reflects a deeper understanding of textual descriptions and visual representations.
This integration involves sophisticated models that can analyze an image's content, relate it to textual data, and generate coherent outputs that blend insights from both.
For example, given a picture of a street scene and a query about the weather, the LLM can recognize visual cues in the image to determine whether it’s rainy, sunny, or cloudy and respond appropriately.
Understanding Multimodal Inputs
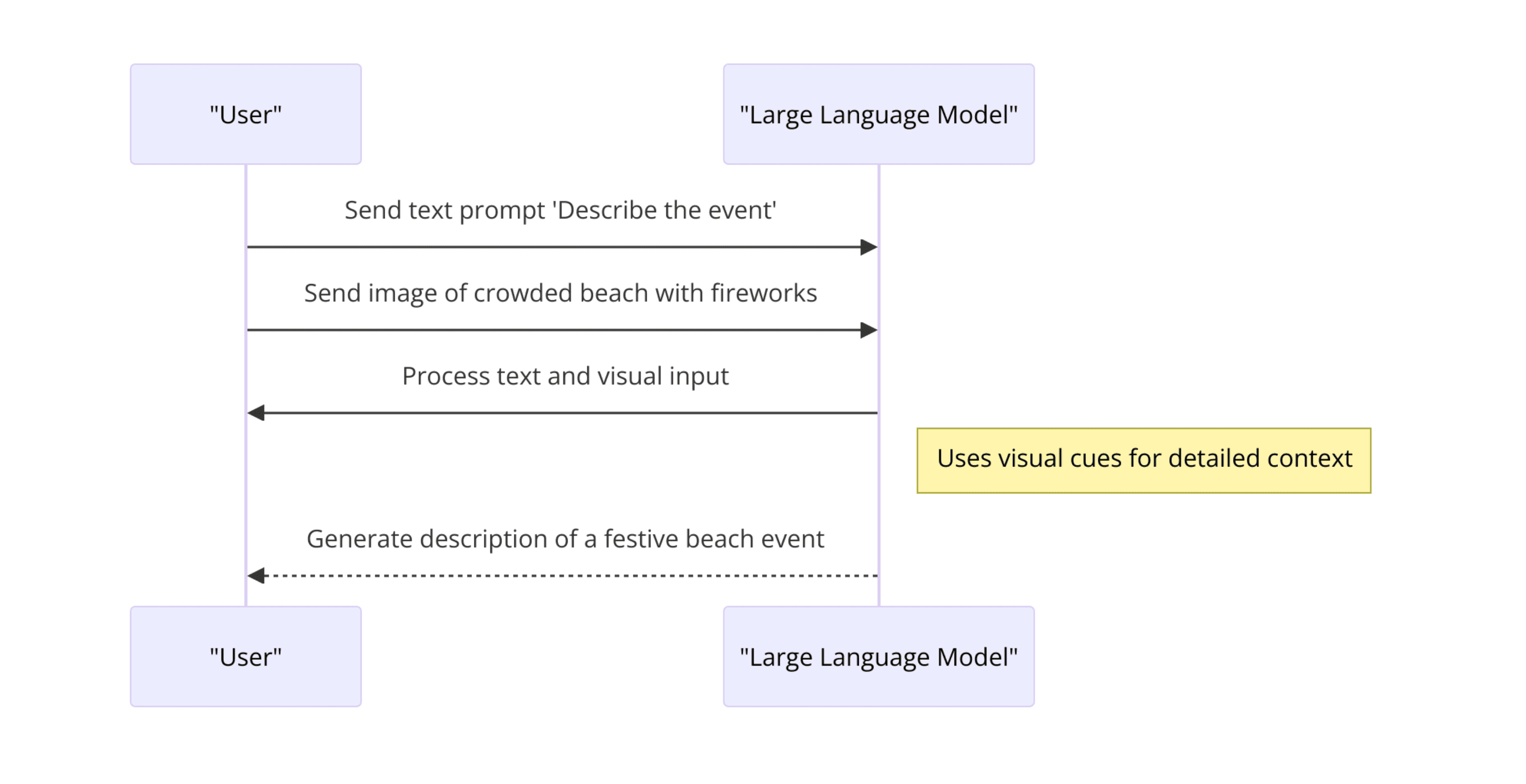
Multimodal prompting in the context of Large Language Models refers to using more than one type of data—such as text and images—to elicit a response from the model.
This approach allows the LLM to draw from a richer information set, enhancing its ability to provide more accurate and contextually relevant outputs. The model can make connections and inferences that are impossible with text alone by processing textual descriptions and visual elements.
Consider a scenario where an LLM is given a text prompt, "Describe the event," and an image of a crowded beach with fireworks. The model uses the visual cues from the image—such as the presence of people, the setting, and the fireworks—to generate a detailed description that complements the textual query, potentially describing a festive beach event celebrating a national holiday.
Read more on RagaAI’s Multimodal Input Capabilities
Text-Only Response Mechanism
The Gemini model exemplifies a sophisticated approach within multimodal systems. It focuses exclusively on generating text-based responses by leveraging dual mechanisms—one that processes text and another that translates visual information into textual concepts. Even when only text output is required, Gemini utilizes the visual data to enrich the context of its responses, making it especially powerful in scenarios where the text alone might not convey the full picture.
Overview of Multimodal LLM Architecture
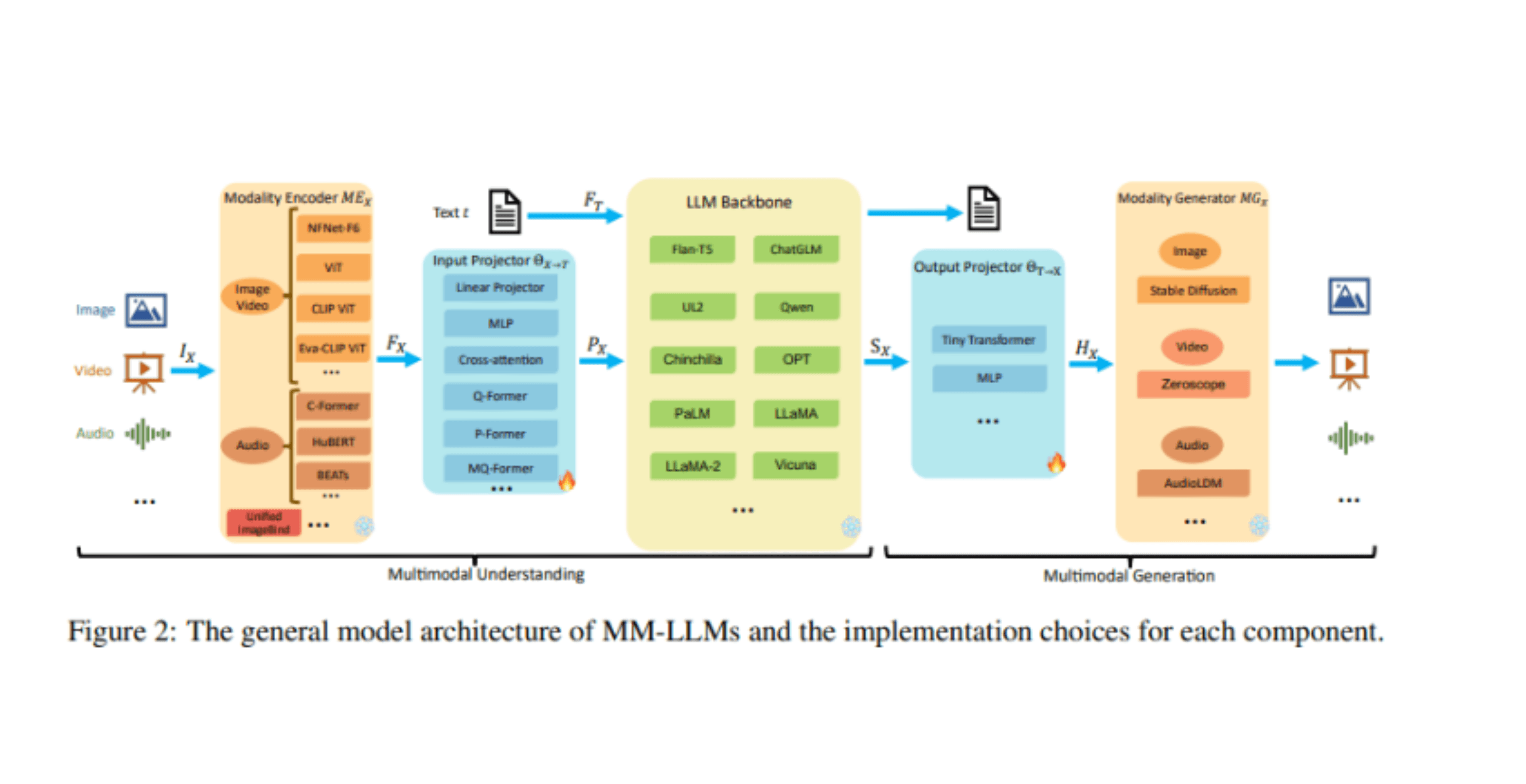
Source: Website
The architecture of Multimodal LLMs (MM-LLMs) is composed of five main components, each playing a crucial role in the model's functionality:
Modality Encoder
Input Projector
LLM Backbone
Output Projector
Modality Generator
1. Modality Encoder
The Modality Encoder (ME) encodes inputs from various modalities (image, video, audio, 3D, etc.) into corresponding features FXF_XFX. This is formulated as: FX=MEX(IX)F_X = ME_X(I_X)FX=MEX(IX)
Visual Modality
For images, multiple encoders can be used, such as NFNet-F6, ViT, CLIP ViT, Eva-CLIP ViT, and others. For videos, frames are uniformly sampled and processed similarly to images.
Audio Modality
Common encoders include C-Former, HuBERT, BEATs, Whisper, and CLAP.
3D Point Cloud Modality
Typically encoded by ULIP-2 with a PointBERT backbone.
Unified encoders like ImageBind handle multiple modalities, including image/video, text, audio, heat maps, inertial measurement units, and depth.
2. Input Projector
The Input Projector aligns encoded features FXF_XFX with the text feature space TTT. The aligned features PXP_XPX are fed into the LLM Backbone alongside textual features FTF_TFT. The goal is to minimize the X-conditioned text generation loss. The alignment can be achieved using Linear Projectors, Multi-Layer Perceptrons (MLPs), or more complex methods like Cross-attention, Q-Former, P-Former, and MQ-Former.
3. LLM Backbone
The LLM Backbone is the core of MM-LLMs, inheriting properties like zero-shot generalization, few-shot in-context learning (ICL), Chain-of-Thought (CoT), and instruction following. It processes representations from various modalities, engaging in semantic understanding, reasoning, and decision-making. The backbone outputs direct textual responses and signal tokens SXS_XSX for other modalities, guiding the Modality Generator.
Popular LLMs include Flan-T5, ChatGLM, UL2, Qwen, Chinchilla, OPT, PaLM, LLaMA, and Vicuna.
4. Output Projector
The Output Projector maps signal token representations from the LLM Backbone into features understandable by the Modality Generator. The goal is to align HXH_XHX with the conditional text representations of MGXMG_XMGX. Implementations include Tiny Transformers or MLPs.
5. Modality Generator
The Modality Generator MGXMG_XMGX produces outputs in various modalities. Commonly used generators include Latent Diffusion Models (LDMs) like Stable Diffusion for images, Zeroscope for videos, and AudioLDM for audio. These generators use features HXH_XHX from the Output Projector as conditional inputs to generate multimodal content.
During training, the ground truth content is transformed into a latent feature z0z_0z0 by a pre-trained VAE, noise ϵ\epsilonϵ is added, and a pre-trained UNet computes the conditional LDM loss LX−genL_{X-gen}LX−gen.
Read more on CLIP Integration in RagaAI
Methodology for Enhancing LLM with Multimodal Inputs
These methodologies enhance the functional capabilities of LLMs and ensure that the outputs are deeply aligned with the input contexts, leading to more effective and user-centric applications.
Prompt Parsing and Extension Agent Roles
In multimodal LLMs, prompt parsing plays a crucial role. This process involves breaking down the input prompts into understandable segments the model can process.
The extension agent's role is to expand these prompts based on the context provided by multimodal data, effectively enhancing the model's understanding and response capability.
For instance, if the text prompt is ambiguous or lacks detail, the extension agent can use information from image or audio inputs to add specificity and relevance to the prompt, guiding the model toward a more accurate output.
Tree of Thought of Models and its Construction
The Tree of Thought is a conceptual framework used in some advanced LLMs to organize and process complex inputs. By constructing a decision tree-like structure, the model can navigate through different layers of information, from general to specific, integrating insights from various modalities.
This structure helps the LLM maintain context and coherence across extended interactions or complex query sequences, improving its problem-solving capabilities and response accuracy.
Model Selection
Model selection is often guided by human feedback and data from 'advantage databases'—repositories of interactions and outcomes highlighting which model configurations perform best under specific conditions. By analyzing this data, developers can fine-tune the model selection process, choosing or adjusting LLM configurations that maximize performance for particular types of multimodal inputs.
Execution of Generation for Personalized Image and Text Outputs
The final step in the methodology involves executing the generation of outputs tailored to the specific requirements of the task at hand. Whether generating a text description based on an image or creating a personalized response to a multimodal query, the LLM utilizes its integrated understanding of textual, visual, and auditory data to produce precise and contextually relevant outputs.descriptive text from an image or responding to a complex query.
Experimentation and Results
Let's delve into the experimental setup and results that evaluate the effectiveness of multimodal Large Language Models (LLMs), examining how these models perform in practical applications and the improvements achieved through sophisticated multimodal integration.
To rigorously test the efficacy of multimodal LLMs, researchers set up controlled experiments comparing different model configurations.
These settings often vary in terms of the types and amounts of multimodal data used and the specific tasks being tested, such as image-text correlation or audio-text integration.
These experiments use standardized datasets and transparent performance metrics to objectively assess how well multimodal LLMs handle complex, real-world tasks compared to their unimodal counterparts or earlier model versions.
Semantic Alignment and Aesthetic Comparison in Images
One key area of experimentation involves assessing semantic alignment—how accurately the model's outputs align with the meanings conveyed in the multimodal inputs.
For example, if an image shows a rainy street, does the text generated by the model accurately describe the scene? Aesthetic comparison tests might evaluate how pleasing or contextually appropriate generated images are when the model is prompted to create visual content based on textual descriptions.
Improvements in Image Reward and Aesthetic Score
Results from these experiments often show significant improvements in 'image reward'—a metric that evaluates how well the generated images meet the user-defined objectives—and 'aesthetic score,' which assesses the visual appeal and relevance of images generated by the LLM.
These improvements highlight the model's enhanced ability to effectively interpret and integrate multimodal data.
User Study
Finally, user studies provide invaluable feedback on how users perceive and value the outputs from multimodal LLMs. Participants might be asked to rate the relevance, coherence, and usefulness of the model's responses in various scenarios, providing direct insights into the user experience.
These studies often reveal a strong preference for outputs generated by enhanced multimodal systems, confirming their practical benefits.
These experimental findings play a crucial role in validating the advancements in multimodal LLMs, demonstrating their enhanced capabilities and their tangible benefits to users. As we progress, we will explore the applications of these powerful tools across various domains.
Applications of Multimodal LLMs
Let’s explore the diverse applications of multimodal Large Language Models (LLMs), showcasing how they are effectively utilized in various scenarios, from simple tasks to complex cognitive functions.
Simple and Advanced Multimodal Prompts
Multimodal LLMs are adept at handling simple and advanced prompts that integrate text, images, or audio to create more engaging and informative responses. For instance, a simple application generates a text description for an uploaded image, while more advanced uses include developing a story based on a series of pictures and text prompts, demonstrating the model's ability to weave together narrative elements from different modalities.
Classification, Recognition, and Counting Using Multimodal Inputs
In more structured tasks, multimodal LLMs excel at classification, recognition, and counting within complex environments. For example, in a medical diagnosis application, an LLM might analyze X-ray images alongside clinical notes to accurately identify and classify medical conditions. Similarly, in retail or surveillance settings, these models can count objects and recognize specific activities or items from video footage accompanied by descriptive audio or text data.
Creative Storytelling and Logical Reasoning Through Image-Text Synthesis
One of the most exciting applications of multimodal LLMs lies in creative storytelling and logical reasoning. These models can generate imaginative stories or detailed explanations based on a mix of visual and textual cues, engaging users with content that is both creative and contextually relevant. This capability is not only entertaining but also useful in educational settings where blending visual learning materials with explanatory text can help illustrate complex concepts more clearly.# Exam
These applications illustrate the versatility and power of multimodal LLMs, enabling them to operate effectively across a wide range of domains and scenarios. Next, we will discuss these technologies' challenges and future directions, considering the ongoing developments and potential for further advancements.
Challenges and Future Directions
Despite the challenges, the potential for multimodal LLMs to transform various industries and applications remains substantial.
As we continue to advance these technologies, their integration into everyday tools and platforms will likely become more pervasive, driving innovation and creating new opportunities for interaction and automation.
Limitations of Current Stable Diffusion Models and DiffusionGPT
While multimodal LLMs like DiffusionGPT offer impressive capabilities, they face limitations in terms of the stability and fidelity of generated outputs, particularly under complex or nuanced scenarios. Current diffusion models may need to help to maintain consistency in long sequences or detailed scenarios, which can result in less coherent or visually disconnected outputs.
Addressing these challenges requires ongoing research and development to refine the models' understanding and processing of multimodal data.
Read more on Integrating DiffusionGPT at RagaAI
Feedback-Driven Optimization
Feedback-driven optimization represents a promising approach to enhancing multimodal LLMs. By systematically incorporating user feedback into the training loop, developers can fine-tune the models to better meet user expectations and improve performance across varied tasks.
This process helps identify and correct specific areas where the models may fall short, such as handling subtleties of human emotion or complex logical reasoning.
Expansion of Model Candidates
The future of multimodal LLMs also lies in expanding the pool of model candidates beyond the current mainstream options. Exploring alternative architectures or hybrid models that combine different types of neural networks might offer new ways to handle the intricacies of multimodal data more effectively. This expansion could lead to breakthroughs in how these models process and integrate diverse inputs, potentially unlocking new applications and improving existing functionalities.
Conclusion
The integration of image and text inputs into LLMs has significantly expanded their capabilities, making them more efficient in processing information and vastly more versatile in their applications.
In conclusion, integrating multimodal inputs into LLMs represents a significant step forward in the evolution of AI technologies.
By continuing to develop and experiment with these models, we can unlock a future where AI assists us more seamlessly in our daily lives, enhancing our abilities and enriching our understanding of the world around us.
Ready to transform your approach to AI with cutting-edge insights and tools? Explore RagaAI's comprehensive suite of AI solutions — from enhancing AI reliability with our guardrails to navigating the complexities of AI governance. Whether you're diving into prompt engineering, seeking to mitigate AI biases, or exploring the frontier of AI testing
Imagine having a conversation in which you can describe a scene and show a picture of it to convey your message more vividly. That's what multimodal prompts do for LLMs.
These prompts feed AI not just text but also other forms of data like images, enabling a richer, more context-aware response. This capability transforms how LLMs understand and interact with the world, making them readers, writers, and comprehensive interpreters of information.
Integrating image and text inputs allows LLMs to process and generate information that reflects a deeper understanding of textual descriptions and visual representations.
This integration involves sophisticated models that can analyze an image's content, relate it to textual data, and generate coherent outputs that blend insights from both.
For example, given a picture of a street scene and a query about the weather, the LLM can recognize visual cues in the image to determine whether it’s rainy, sunny, or cloudy and respond appropriately.
Understanding Multimodal Inputs
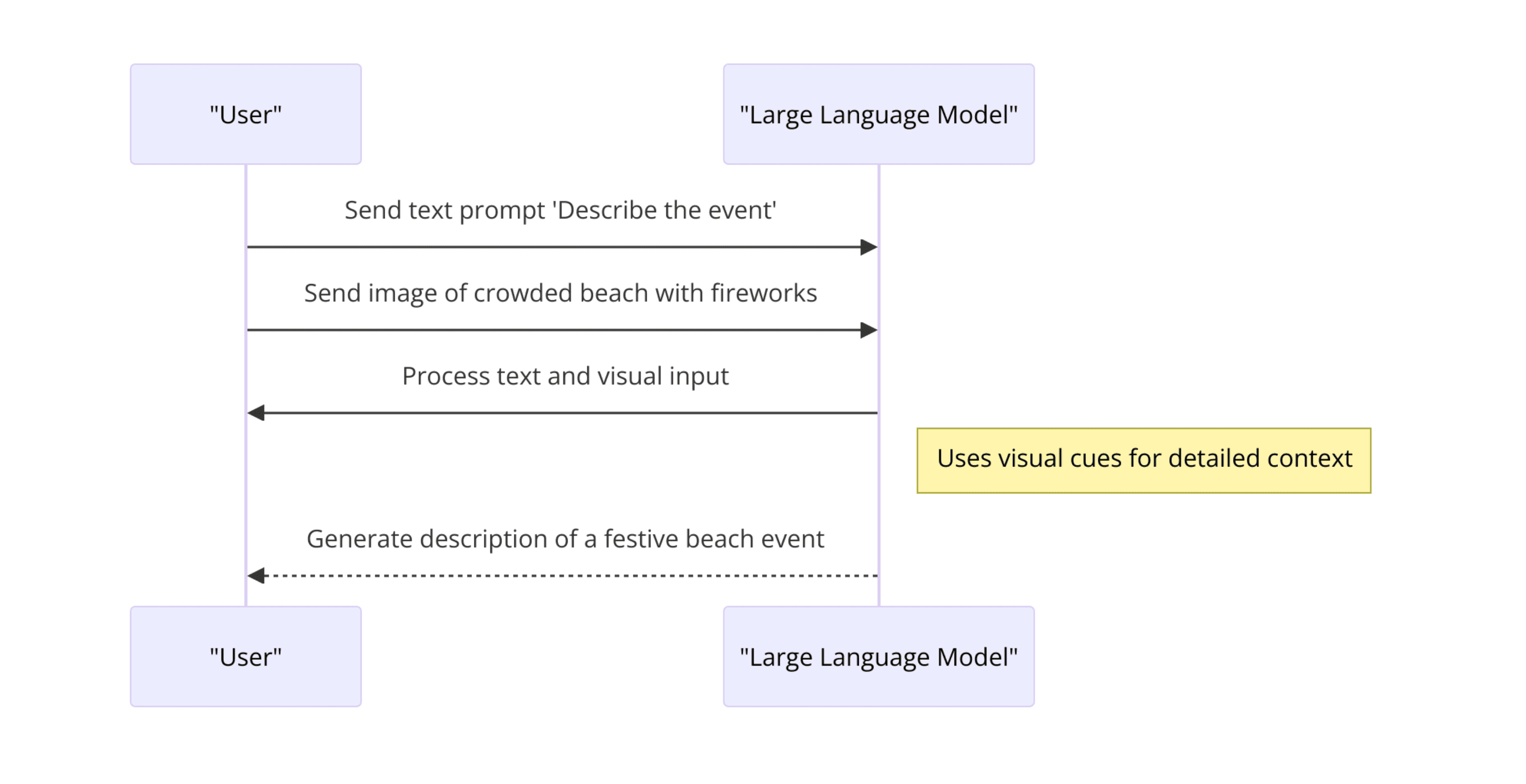
Multimodal prompting in the context of Large Language Models refers to using more than one type of data—such as text and images—to elicit a response from the model.
This approach allows the LLM to draw from a richer information set, enhancing its ability to provide more accurate and contextually relevant outputs. The model can make connections and inferences that are impossible with text alone by processing textual descriptions and visual elements.
Consider a scenario where an LLM is given a text prompt, "Describe the event," and an image of a crowded beach with fireworks. The model uses the visual cues from the image—such as the presence of people, the setting, and the fireworks—to generate a detailed description that complements the textual query, potentially describing a festive beach event celebrating a national holiday.
Read more on RagaAI’s Multimodal Input Capabilities
Text-Only Response Mechanism
The Gemini model exemplifies a sophisticated approach within multimodal systems. It focuses exclusively on generating text-based responses by leveraging dual mechanisms—one that processes text and another that translates visual information into textual concepts. Even when only text output is required, Gemini utilizes the visual data to enrich the context of its responses, making it especially powerful in scenarios where the text alone might not convey the full picture.
Overview of Multimodal LLM Architecture
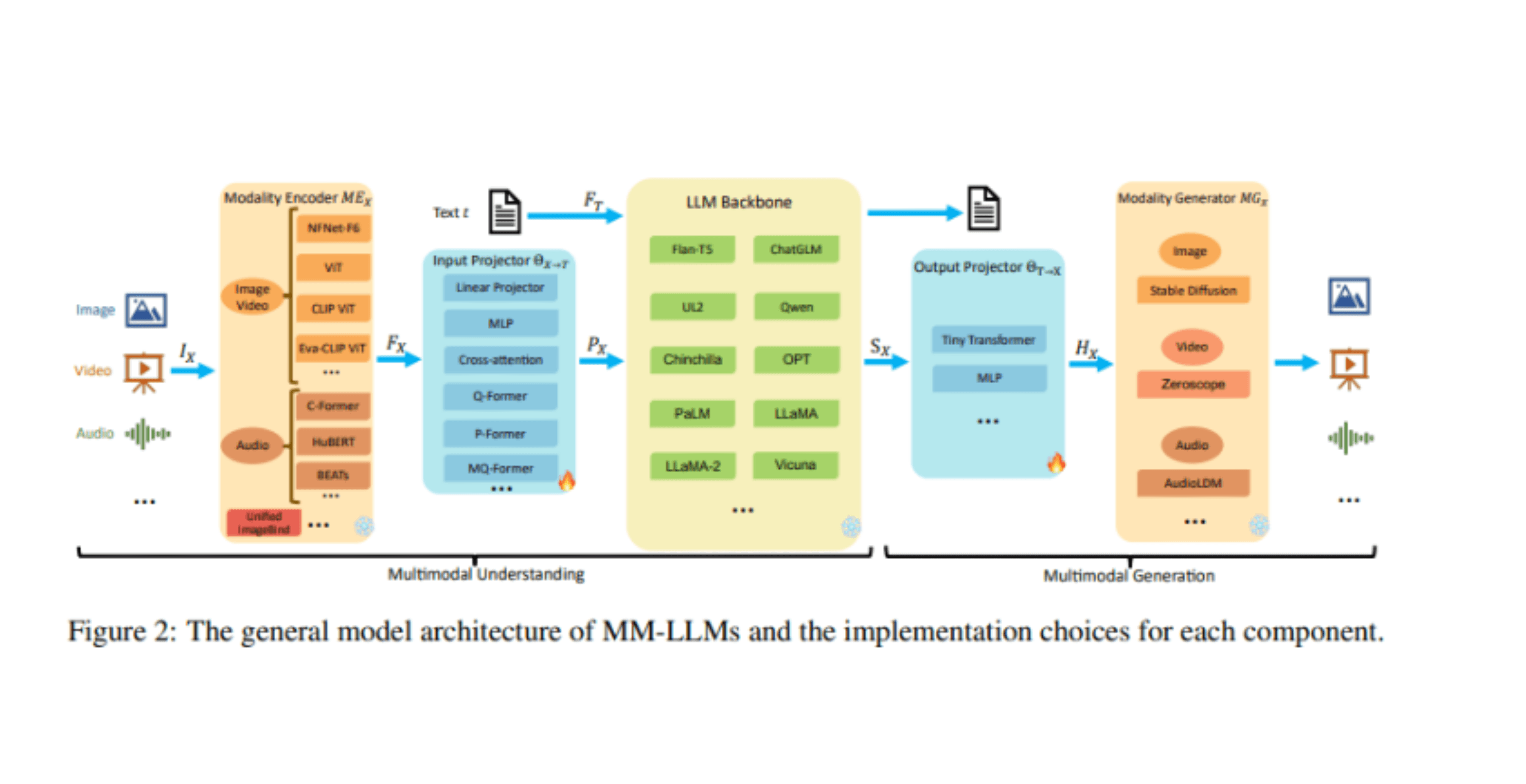
Source: Website
The architecture of Multimodal LLMs (MM-LLMs) is composed of five main components, each playing a crucial role in the model's functionality:
Modality Encoder
Input Projector
LLM Backbone
Output Projector
Modality Generator
1. Modality Encoder
The Modality Encoder (ME) encodes inputs from various modalities (image, video, audio, 3D, etc.) into corresponding features FXF_XFX. This is formulated as: FX=MEX(IX)F_X = ME_X(I_X)FX=MEX(IX)
Visual Modality
For images, multiple encoders can be used, such as NFNet-F6, ViT, CLIP ViT, Eva-CLIP ViT, and others. For videos, frames are uniformly sampled and processed similarly to images.
Audio Modality
Common encoders include C-Former, HuBERT, BEATs, Whisper, and CLAP.
3D Point Cloud Modality
Typically encoded by ULIP-2 with a PointBERT backbone.
Unified encoders like ImageBind handle multiple modalities, including image/video, text, audio, heat maps, inertial measurement units, and depth.
2. Input Projector
The Input Projector aligns encoded features FXF_XFX with the text feature space TTT. The aligned features PXP_XPX are fed into the LLM Backbone alongside textual features FTF_TFT. The goal is to minimize the X-conditioned text generation loss. The alignment can be achieved using Linear Projectors, Multi-Layer Perceptrons (MLPs), or more complex methods like Cross-attention, Q-Former, P-Former, and MQ-Former.
3. LLM Backbone
The LLM Backbone is the core of MM-LLMs, inheriting properties like zero-shot generalization, few-shot in-context learning (ICL), Chain-of-Thought (CoT), and instruction following. It processes representations from various modalities, engaging in semantic understanding, reasoning, and decision-making. The backbone outputs direct textual responses and signal tokens SXS_XSX for other modalities, guiding the Modality Generator.
Popular LLMs include Flan-T5, ChatGLM, UL2, Qwen, Chinchilla, OPT, PaLM, LLaMA, and Vicuna.
4. Output Projector
The Output Projector maps signal token representations from the LLM Backbone into features understandable by the Modality Generator. The goal is to align HXH_XHX with the conditional text representations of MGXMG_XMGX. Implementations include Tiny Transformers or MLPs.
5. Modality Generator
The Modality Generator MGXMG_XMGX produces outputs in various modalities. Commonly used generators include Latent Diffusion Models (LDMs) like Stable Diffusion for images, Zeroscope for videos, and AudioLDM for audio. These generators use features HXH_XHX from the Output Projector as conditional inputs to generate multimodal content.
During training, the ground truth content is transformed into a latent feature z0z_0z0 by a pre-trained VAE, noise ϵ\epsilonϵ is added, and a pre-trained UNet computes the conditional LDM loss LX−genL_{X-gen}LX−gen.
Read more on CLIP Integration in RagaAI
Methodology for Enhancing LLM with Multimodal Inputs
These methodologies enhance the functional capabilities of LLMs and ensure that the outputs are deeply aligned with the input contexts, leading to more effective and user-centric applications.
Prompt Parsing and Extension Agent Roles
In multimodal LLMs, prompt parsing plays a crucial role. This process involves breaking down the input prompts into understandable segments the model can process.
The extension agent's role is to expand these prompts based on the context provided by multimodal data, effectively enhancing the model's understanding and response capability.
For instance, if the text prompt is ambiguous or lacks detail, the extension agent can use information from image or audio inputs to add specificity and relevance to the prompt, guiding the model toward a more accurate output.
Tree of Thought of Models and its Construction
The Tree of Thought is a conceptual framework used in some advanced LLMs to organize and process complex inputs. By constructing a decision tree-like structure, the model can navigate through different layers of information, from general to specific, integrating insights from various modalities.
This structure helps the LLM maintain context and coherence across extended interactions or complex query sequences, improving its problem-solving capabilities and response accuracy.
Model Selection
Model selection is often guided by human feedback and data from 'advantage databases'—repositories of interactions and outcomes highlighting which model configurations perform best under specific conditions. By analyzing this data, developers can fine-tune the model selection process, choosing or adjusting LLM configurations that maximize performance for particular types of multimodal inputs.
Execution of Generation for Personalized Image and Text Outputs
The final step in the methodology involves executing the generation of outputs tailored to the specific requirements of the task at hand. Whether generating a text description based on an image or creating a personalized response to a multimodal query, the LLM utilizes its integrated understanding of textual, visual, and auditory data to produce precise and contextually relevant outputs.descriptive text from an image or responding to a complex query.
Experimentation and Results
Let's delve into the experimental setup and results that evaluate the effectiveness of multimodal Large Language Models (LLMs), examining how these models perform in practical applications and the improvements achieved through sophisticated multimodal integration.
To rigorously test the efficacy of multimodal LLMs, researchers set up controlled experiments comparing different model configurations.
These settings often vary in terms of the types and amounts of multimodal data used and the specific tasks being tested, such as image-text correlation or audio-text integration.
These experiments use standardized datasets and transparent performance metrics to objectively assess how well multimodal LLMs handle complex, real-world tasks compared to their unimodal counterparts or earlier model versions.
Semantic Alignment and Aesthetic Comparison in Images
One key area of experimentation involves assessing semantic alignment—how accurately the model's outputs align with the meanings conveyed in the multimodal inputs.
For example, if an image shows a rainy street, does the text generated by the model accurately describe the scene? Aesthetic comparison tests might evaluate how pleasing or contextually appropriate generated images are when the model is prompted to create visual content based on textual descriptions.
Improvements in Image Reward and Aesthetic Score
Results from these experiments often show significant improvements in 'image reward'—a metric that evaluates how well the generated images meet the user-defined objectives—and 'aesthetic score,' which assesses the visual appeal and relevance of images generated by the LLM.
These improvements highlight the model's enhanced ability to effectively interpret and integrate multimodal data.
User Study
Finally, user studies provide invaluable feedback on how users perceive and value the outputs from multimodal LLMs. Participants might be asked to rate the relevance, coherence, and usefulness of the model's responses in various scenarios, providing direct insights into the user experience.
These studies often reveal a strong preference for outputs generated by enhanced multimodal systems, confirming their practical benefits.
These experimental findings play a crucial role in validating the advancements in multimodal LLMs, demonstrating their enhanced capabilities and their tangible benefits to users. As we progress, we will explore the applications of these powerful tools across various domains.
Applications of Multimodal LLMs
Let’s explore the diverse applications of multimodal Large Language Models (LLMs), showcasing how they are effectively utilized in various scenarios, from simple tasks to complex cognitive functions.
Simple and Advanced Multimodal Prompts
Multimodal LLMs are adept at handling simple and advanced prompts that integrate text, images, or audio to create more engaging and informative responses. For instance, a simple application generates a text description for an uploaded image, while more advanced uses include developing a story based on a series of pictures and text prompts, demonstrating the model's ability to weave together narrative elements from different modalities.
Classification, Recognition, and Counting Using Multimodal Inputs
In more structured tasks, multimodal LLMs excel at classification, recognition, and counting within complex environments. For example, in a medical diagnosis application, an LLM might analyze X-ray images alongside clinical notes to accurately identify and classify medical conditions. Similarly, in retail or surveillance settings, these models can count objects and recognize specific activities or items from video footage accompanied by descriptive audio or text data.
Creative Storytelling and Logical Reasoning Through Image-Text Synthesis
One of the most exciting applications of multimodal LLMs lies in creative storytelling and logical reasoning. These models can generate imaginative stories or detailed explanations based on a mix of visual and textual cues, engaging users with content that is both creative and contextually relevant. This capability is not only entertaining but also useful in educational settings where blending visual learning materials with explanatory text can help illustrate complex concepts more clearly.# Exam
These applications illustrate the versatility and power of multimodal LLMs, enabling them to operate effectively across a wide range of domains and scenarios. Next, we will discuss these technologies' challenges and future directions, considering the ongoing developments and potential for further advancements.
Challenges and Future Directions
Despite the challenges, the potential for multimodal LLMs to transform various industries and applications remains substantial.
As we continue to advance these technologies, their integration into everyday tools and platforms will likely become more pervasive, driving innovation and creating new opportunities for interaction and automation.
Limitations of Current Stable Diffusion Models and DiffusionGPT
While multimodal LLMs like DiffusionGPT offer impressive capabilities, they face limitations in terms of the stability and fidelity of generated outputs, particularly under complex or nuanced scenarios. Current diffusion models may need to help to maintain consistency in long sequences or detailed scenarios, which can result in less coherent or visually disconnected outputs.
Addressing these challenges requires ongoing research and development to refine the models' understanding and processing of multimodal data.
Read more on Integrating DiffusionGPT at RagaAI
Feedback-Driven Optimization
Feedback-driven optimization represents a promising approach to enhancing multimodal LLMs. By systematically incorporating user feedback into the training loop, developers can fine-tune the models to better meet user expectations and improve performance across varied tasks.
This process helps identify and correct specific areas where the models may fall short, such as handling subtleties of human emotion or complex logical reasoning.
Expansion of Model Candidates
The future of multimodal LLMs also lies in expanding the pool of model candidates beyond the current mainstream options. Exploring alternative architectures or hybrid models that combine different types of neural networks might offer new ways to handle the intricacies of multimodal data more effectively. This expansion could lead to breakthroughs in how these models process and integrate diverse inputs, potentially unlocking new applications and improving existing functionalities.
Conclusion
The integration of image and text inputs into LLMs has significantly expanded their capabilities, making them more efficient in processing information and vastly more versatile in their applications.
In conclusion, integrating multimodal inputs into LLMs represents a significant step forward in the evolution of AI technologies.
By continuing to develop and experiment with these models, we can unlock a future where AI assists us more seamlessly in our daily lives, enhancing our abilities and enriching our understanding of the world around us.
Ready to transform your approach to AI with cutting-edge insights and tools? Explore RagaAI's comprehensive suite of AI solutions — from enhancing AI reliability with our guardrails to navigating the complexities of AI governance. Whether you're diving into prompt engineering, seeking to mitigate AI biases, or exploring the frontier of AI testing
Imagine having a conversation in which you can describe a scene and show a picture of it to convey your message more vividly. That's what multimodal prompts do for LLMs.
These prompts feed AI not just text but also other forms of data like images, enabling a richer, more context-aware response. This capability transforms how LLMs understand and interact with the world, making them readers, writers, and comprehensive interpreters of information.
Integrating image and text inputs allows LLMs to process and generate information that reflects a deeper understanding of textual descriptions and visual representations.
This integration involves sophisticated models that can analyze an image's content, relate it to textual data, and generate coherent outputs that blend insights from both.
For example, given a picture of a street scene and a query about the weather, the LLM can recognize visual cues in the image to determine whether it’s rainy, sunny, or cloudy and respond appropriately.
Understanding Multimodal Inputs
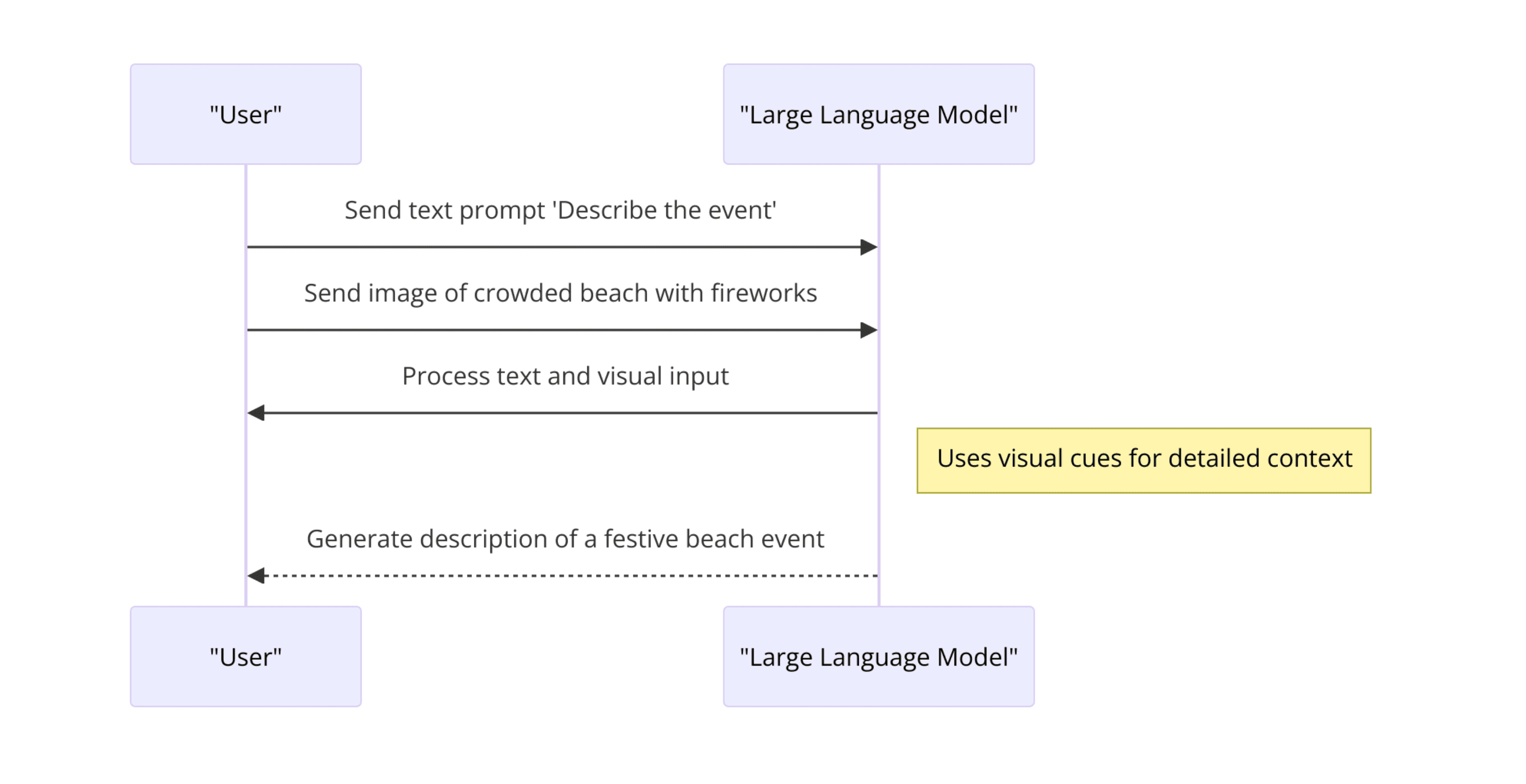
Multimodal prompting in the context of Large Language Models refers to using more than one type of data—such as text and images—to elicit a response from the model.
This approach allows the LLM to draw from a richer information set, enhancing its ability to provide more accurate and contextually relevant outputs. The model can make connections and inferences that are impossible with text alone by processing textual descriptions and visual elements.
Consider a scenario where an LLM is given a text prompt, "Describe the event," and an image of a crowded beach with fireworks. The model uses the visual cues from the image—such as the presence of people, the setting, and the fireworks—to generate a detailed description that complements the textual query, potentially describing a festive beach event celebrating a national holiday.
Read more on RagaAI’s Multimodal Input Capabilities
Text-Only Response Mechanism
The Gemini model exemplifies a sophisticated approach within multimodal systems. It focuses exclusively on generating text-based responses by leveraging dual mechanisms—one that processes text and another that translates visual information into textual concepts. Even when only text output is required, Gemini utilizes the visual data to enrich the context of its responses, making it especially powerful in scenarios where the text alone might not convey the full picture.
Overview of Multimodal LLM Architecture
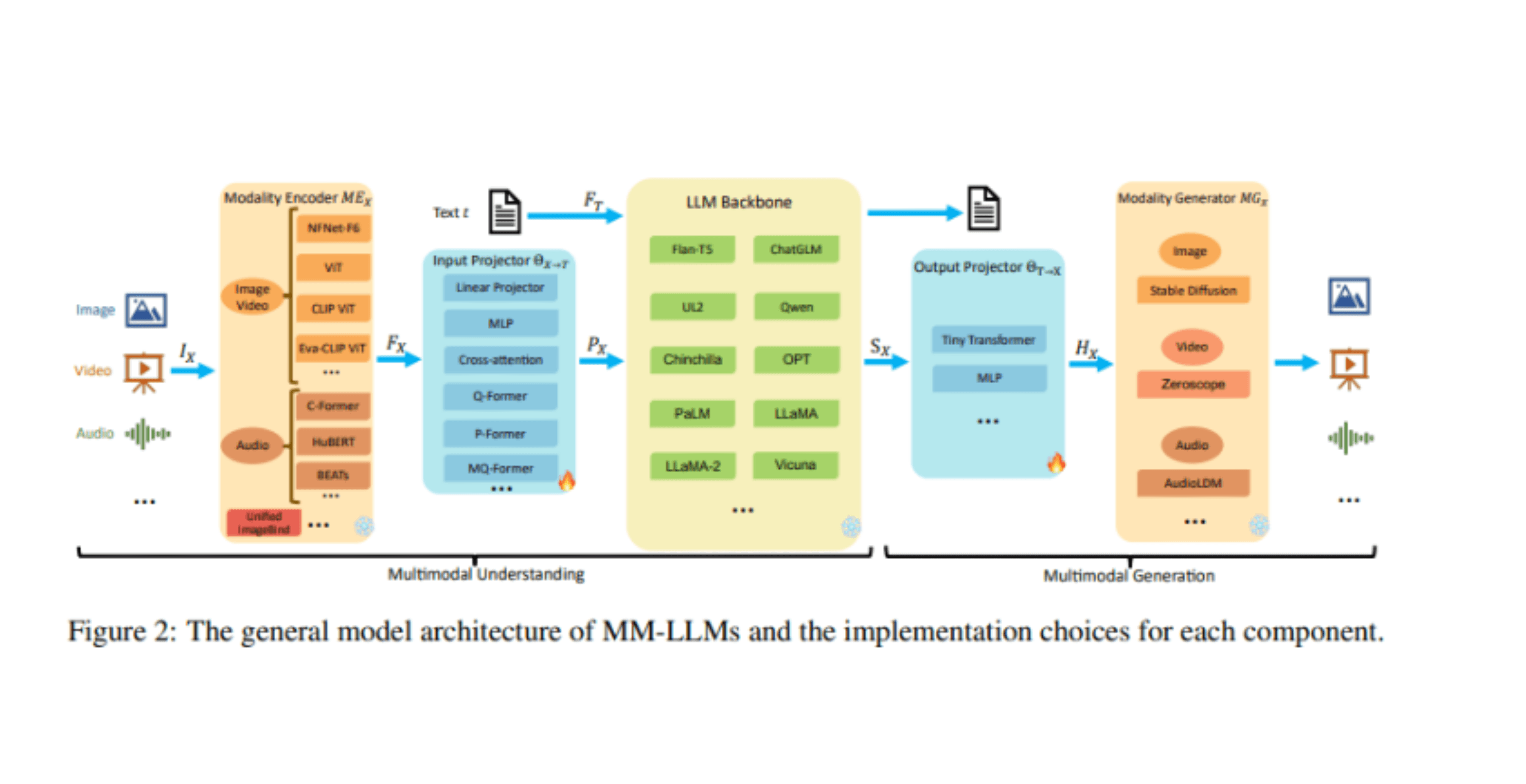
Source: Website
The architecture of Multimodal LLMs (MM-LLMs) is composed of five main components, each playing a crucial role in the model's functionality:
Modality Encoder
Input Projector
LLM Backbone
Output Projector
Modality Generator
1. Modality Encoder
The Modality Encoder (ME) encodes inputs from various modalities (image, video, audio, 3D, etc.) into corresponding features FXF_XFX. This is formulated as: FX=MEX(IX)F_X = ME_X(I_X)FX=MEX(IX)
Visual Modality
For images, multiple encoders can be used, such as NFNet-F6, ViT, CLIP ViT, Eva-CLIP ViT, and others. For videos, frames are uniformly sampled and processed similarly to images.
Audio Modality
Common encoders include C-Former, HuBERT, BEATs, Whisper, and CLAP.
3D Point Cloud Modality
Typically encoded by ULIP-2 with a PointBERT backbone.
Unified encoders like ImageBind handle multiple modalities, including image/video, text, audio, heat maps, inertial measurement units, and depth.
2. Input Projector
The Input Projector aligns encoded features FXF_XFX with the text feature space TTT. The aligned features PXP_XPX are fed into the LLM Backbone alongside textual features FTF_TFT. The goal is to minimize the X-conditioned text generation loss. The alignment can be achieved using Linear Projectors, Multi-Layer Perceptrons (MLPs), or more complex methods like Cross-attention, Q-Former, P-Former, and MQ-Former.
3. LLM Backbone
The LLM Backbone is the core of MM-LLMs, inheriting properties like zero-shot generalization, few-shot in-context learning (ICL), Chain-of-Thought (CoT), and instruction following. It processes representations from various modalities, engaging in semantic understanding, reasoning, and decision-making. The backbone outputs direct textual responses and signal tokens SXS_XSX for other modalities, guiding the Modality Generator.
Popular LLMs include Flan-T5, ChatGLM, UL2, Qwen, Chinchilla, OPT, PaLM, LLaMA, and Vicuna.
4. Output Projector
The Output Projector maps signal token representations from the LLM Backbone into features understandable by the Modality Generator. The goal is to align HXH_XHX with the conditional text representations of MGXMG_XMGX. Implementations include Tiny Transformers or MLPs.
5. Modality Generator
The Modality Generator MGXMG_XMGX produces outputs in various modalities. Commonly used generators include Latent Diffusion Models (LDMs) like Stable Diffusion for images, Zeroscope for videos, and AudioLDM for audio. These generators use features HXH_XHX from the Output Projector as conditional inputs to generate multimodal content.
During training, the ground truth content is transformed into a latent feature z0z_0z0 by a pre-trained VAE, noise ϵ\epsilonϵ is added, and a pre-trained UNet computes the conditional LDM loss LX−genL_{X-gen}LX−gen.
Read more on CLIP Integration in RagaAI
Methodology for Enhancing LLM with Multimodal Inputs
These methodologies enhance the functional capabilities of LLMs and ensure that the outputs are deeply aligned with the input contexts, leading to more effective and user-centric applications.
Prompt Parsing and Extension Agent Roles
In multimodal LLMs, prompt parsing plays a crucial role. This process involves breaking down the input prompts into understandable segments the model can process.
The extension agent's role is to expand these prompts based on the context provided by multimodal data, effectively enhancing the model's understanding and response capability.
For instance, if the text prompt is ambiguous or lacks detail, the extension agent can use information from image or audio inputs to add specificity and relevance to the prompt, guiding the model toward a more accurate output.
Tree of Thought of Models and its Construction
The Tree of Thought is a conceptual framework used in some advanced LLMs to organize and process complex inputs. By constructing a decision tree-like structure, the model can navigate through different layers of information, from general to specific, integrating insights from various modalities.
This structure helps the LLM maintain context and coherence across extended interactions or complex query sequences, improving its problem-solving capabilities and response accuracy.
Model Selection
Model selection is often guided by human feedback and data from 'advantage databases'—repositories of interactions and outcomes highlighting which model configurations perform best under specific conditions. By analyzing this data, developers can fine-tune the model selection process, choosing or adjusting LLM configurations that maximize performance for particular types of multimodal inputs.
Execution of Generation for Personalized Image and Text Outputs
The final step in the methodology involves executing the generation of outputs tailored to the specific requirements of the task at hand. Whether generating a text description based on an image or creating a personalized response to a multimodal query, the LLM utilizes its integrated understanding of textual, visual, and auditory data to produce precise and contextually relevant outputs.descriptive text from an image or responding to a complex query.
Experimentation and Results
Let's delve into the experimental setup and results that evaluate the effectiveness of multimodal Large Language Models (LLMs), examining how these models perform in practical applications and the improvements achieved through sophisticated multimodal integration.
To rigorously test the efficacy of multimodal LLMs, researchers set up controlled experiments comparing different model configurations.
These settings often vary in terms of the types and amounts of multimodal data used and the specific tasks being tested, such as image-text correlation or audio-text integration.
These experiments use standardized datasets and transparent performance metrics to objectively assess how well multimodal LLMs handle complex, real-world tasks compared to their unimodal counterparts or earlier model versions.
Semantic Alignment and Aesthetic Comparison in Images
One key area of experimentation involves assessing semantic alignment—how accurately the model's outputs align with the meanings conveyed in the multimodal inputs.
For example, if an image shows a rainy street, does the text generated by the model accurately describe the scene? Aesthetic comparison tests might evaluate how pleasing or contextually appropriate generated images are when the model is prompted to create visual content based on textual descriptions.
Improvements in Image Reward and Aesthetic Score
Results from these experiments often show significant improvements in 'image reward'—a metric that evaluates how well the generated images meet the user-defined objectives—and 'aesthetic score,' which assesses the visual appeal and relevance of images generated by the LLM.
These improvements highlight the model's enhanced ability to effectively interpret and integrate multimodal data.
User Study
Finally, user studies provide invaluable feedback on how users perceive and value the outputs from multimodal LLMs. Participants might be asked to rate the relevance, coherence, and usefulness of the model's responses in various scenarios, providing direct insights into the user experience.
These studies often reveal a strong preference for outputs generated by enhanced multimodal systems, confirming their practical benefits.
These experimental findings play a crucial role in validating the advancements in multimodal LLMs, demonstrating their enhanced capabilities and their tangible benefits to users. As we progress, we will explore the applications of these powerful tools across various domains.
Applications of Multimodal LLMs
Let’s explore the diverse applications of multimodal Large Language Models (LLMs), showcasing how they are effectively utilized in various scenarios, from simple tasks to complex cognitive functions.
Simple and Advanced Multimodal Prompts
Multimodal LLMs are adept at handling simple and advanced prompts that integrate text, images, or audio to create more engaging and informative responses. For instance, a simple application generates a text description for an uploaded image, while more advanced uses include developing a story based on a series of pictures and text prompts, demonstrating the model's ability to weave together narrative elements from different modalities.
Classification, Recognition, and Counting Using Multimodal Inputs
In more structured tasks, multimodal LLMs excel at classification, recognition, and counting within complex environments. For example, in a medical diagnosis application, an LLM might analyze X-ray images alongside clinical notes to accurately identify and classify medical conditions. Similarly, in retail or surveillance settings, these models can count objects and recognize specific activities or items from video footage accompanied by descriptive audio or text data.
Creative Storytelling and Logical Reasoning Through Image-Text Synthesis
One of the most exciting applications of multimodal LLMs lies in creative storytelling and logical reasoning. These models can generate imaginative stories or detailed explanations based on a mix of visual and textual cues, engaging users with content that is both creative and contextually relevant. This capability is not only entertaining but also useful in educational settings where blending visual learning materials with explanatory text can help illustrate complex concepts more clearly.# Exam
These applications illustrate the versatility and power of multimodal LLMs, enabling them to operate effectively across a wide range of domains and scenarios. Next, we will discuss these technologies' challenges and future directions, considering the ongoing developments and potential for further advancements.
Challenges and Future Directions
Despite the challenges, the potential for multimodal LLMs to transform various industries and applications remains substantial.
As we continue to advance these technologies, their integration into everyday tools and platforms will likely become more pervasive, driving innovation and creating new opportunities for interaction and automation.
Limitations of Current Stable Diffusion Models and DiffusionGPT
While multimodal LLMs like DiffusionGPT offer impressive capabilities, they face limitations in terms of the stability and fidelity of generated outputs, particularly under complex or nuanced scenarios. Current diffusion models may need to help to maintain consistency in long sequences or detailed scenarios, which can result in less coherent or visually disconnected outputs.
Addressing these challenges requires ongoing research and development to refine the models' understanding and processing of multimodal data.
Read more on Integrating DiffusionGPT at RagaAI
Feedback-Driven Optimization
Feedback-driven optimization represents a promising approach to enhancing multimodal LLMs. By systematically incorporating user feedback into the training loop, developers can fine-tune the models to better meet user expectations and improve performance across varied tasks.
This process helps identify and correct specific areas where the models may fall short, such as handling subtleties of human emotion or complex logical reasoning.
Expansion of Model Candidates
The future of multimodal LLMs also lies in expanding the pool of model candidates beyond the current mainstream options. Exploring alternative architectures or hybrid models that combine different types of neural networks might offer new ways to handle the intricacies of multimodal data more effectively. This expansion could lead to breakthroughs in how these models process and integrate diverse inputs, potentially unlocking new applications and improving existing functionalities.
Conclusion
The integration of image and text inputs into LLMs has significantly expanded their capabilities, making them more efficient in processing information and vastly more versatile in their applications.
In conclusion, integrating multimodal inputs into LLMs represents a significant step forward in the evolution of AI technologies.
By continuing to develop and experiment with these models, we can unlock a future where AI assists us more seamlessly in our daily lives, enhancing our abilities and enriching our understanding of the world around us.
Ready to transform your approach to AI with cutting-edge insights and tools? Explore RagaAI's comprehensive suite of AI solutions — from enhancing AI reliability with our guardrails to navigating the complexities of AI governance. Whether you're diving into prompt engineering, seeking to mitigate AI biases, or exploring the frontier of AI testing
Subscribe to our newsletter to never miss an update
Other articles


Agents Talk, Models Think: A2A + MCP for Enterprise Agentic AI

Riya Parikh, Nitai Agarwal
Apr 27, 2025
Read the article
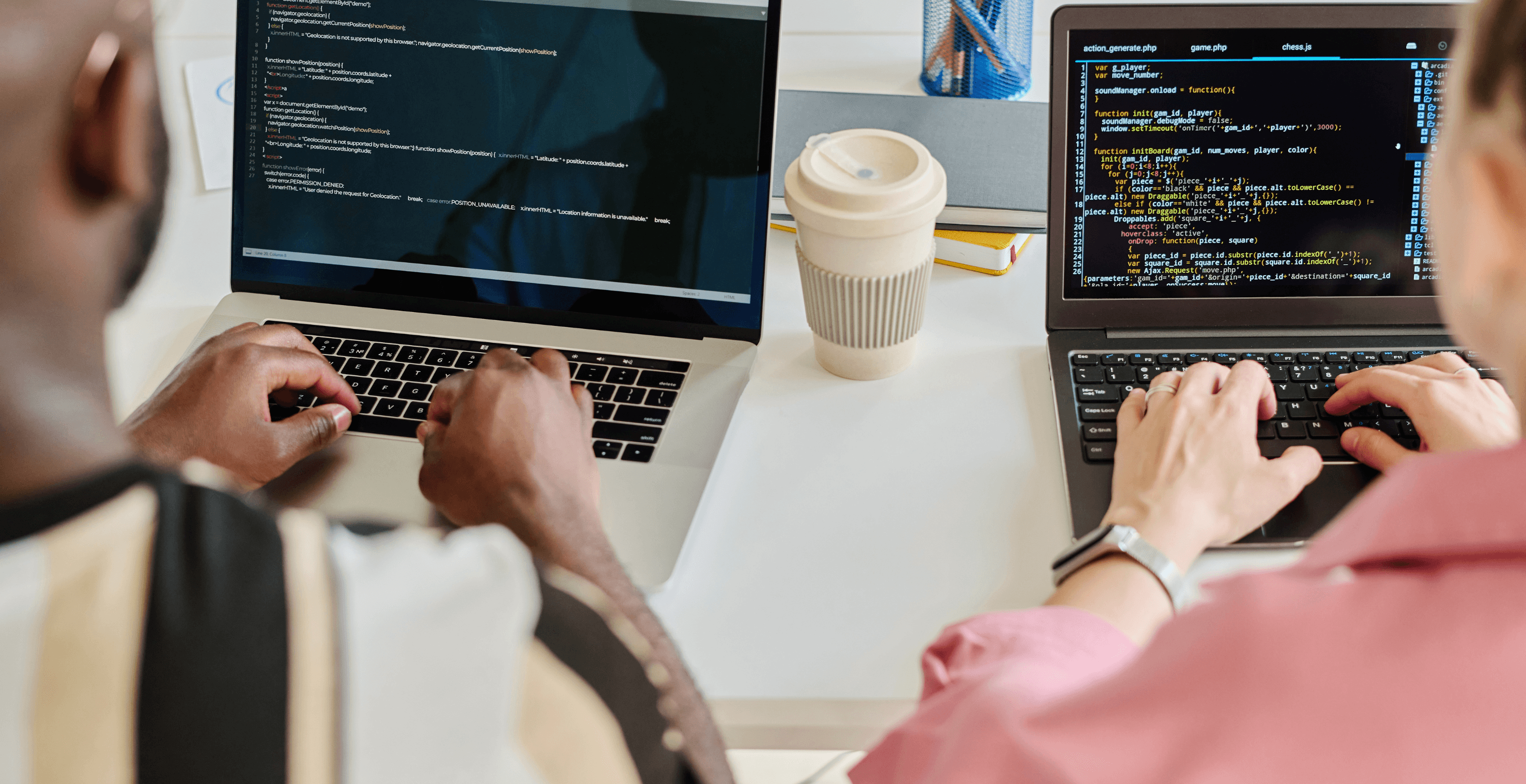
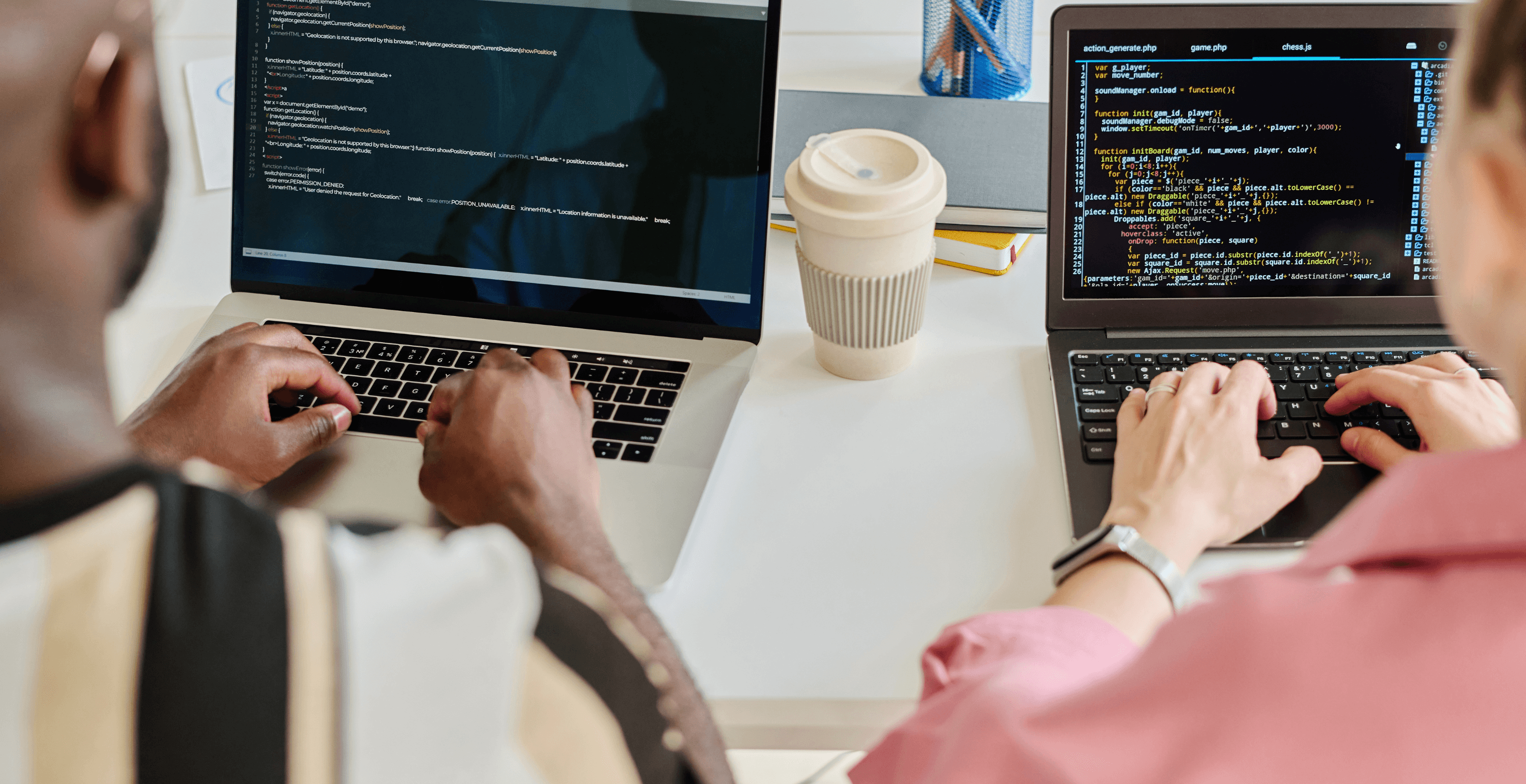
Understanding NeMo Guardrails: A Toolkit for LLM Security

Rehan Asif
Dec 24, 2024
Read the article


Understanding Differences in Large vs Small Language Models (LLM vs SLM)

Rehan Asif
Dec 21, 2024
Read the article