Agents Talk, Models Think: A2A + MCP for Enterprise Agentic AI
Agents Talk, Models Think: A2A + MCP for Enterprise Agentic AI
Agents Talk, Models Think: A2A + MCP for Enterprise Agentic AI
Riya Parikh, Nitai Agarwal
Apr 27, 2025




The hype surrounding Generative AI and Multi-Agent Systems often clashes with the messy reality of enterprise integration. We're rapidly moving beyond standalone AI models towards sophisticated, multi-agent systems designed to tackle complex, end-to-end enterprise workflows – a transition whose complexities we explored in our previous post, "Navigating the complexities of building and scaling Multi-Agent System". Yet, as these AI ecosystems grow in sophistication and scale, agent communication and context management have emerged as critical bottlenecks, hindering the very scalability, interoperability, and maintainability they promise.
The core problem?
A lack of standardization. We see bespoke integrations, inconsistent context handling between different AI models especially LLM, and brittle, ad-hoc communication methods between AI agents. This leads to complex, difficult-to-debug systems that struggle to scale and adapt.
Agent Communication Is the New Bottleneck
As we build more sophisticated AI systems composed of multiple specialized agents (e.g., one agent for data retrieval, another for analysis, a third for summarization), the interactions between these agents become paramount.
Challenges in Multi-Agent Systems (MAS)
Ad-hoc Communication: Teams often invent their own communication methods for each project, leading to inconsistency and integration nightmares.
Agent Coupling: Agents become overly dependent on the specific implementation details of others, making changes difficult and risky.
Lack of Discoverability: Agents can't easily find other agents that could help them, limiting dynamic collaboration.
Error Handling Complexity: Failures in one agent can cascade unpredictably without standardized error reporting and recovery protocols.
Debugging Hell: Tracing a request or workflow across multiple agents with different communication styles is incredibly difficult.
How Traditional Approaches Fail at Scale
Simple point-to-point integrations or basic API calls work for a few agents but break down quickly. Imagine dozens or hundreds of agents – the number of potential interactions explodes (N*(N-1)). Without standardization, this becomes an unmanageable web of dependencies.
Increased Latency: Poorly optimized or synchronous communication chains add significant delays.
High Failure Rates: Lack of standardized error handling leads to brittle systems where one agent failure brings down entire workflows.
Integration Effort: Time spent mapping data formats and communication protocols between agents becomes a major development cost.
Monitoring Complexity: Tracking performance and health across diverse communication channels is challenging.
Enter the power duo: MCP & A2A
These complementary protocols provide the standardized foundation needed to overcome these challenges. MCP focuses on standardizing how AI models receive and understand context, while A2A defines how independent AI agents interact and collaborate.
This comprehensive guide will dissect MCP and A2A, exploring their technical underpinnings, enterprise applications, and practical implementation strategies. We will delve into technical specifications, architectural patterns, real-world case studies, and developer best practices. By the end, you'll understand why MCP and A2A together form the foundation for truly scalable, interoperable multi-agent AI systems that can transform enterprise operations.
Understanding Model Context Protocol (MCP)
Model Context Protocol (MCP) is an open-source protocol developed by Anthropic (creators of Claude) that establishes a standardized framework for LLM integrations across the industry. As an open-source initiative, MCP aims to create a universal standard that benefits the entire AI ecosystem through collaborative development and widespread adoption.
At its core, MCP provides a standardized methodology for connecting LLM applications with external data sources, knowledge bases, and tools. Essentially, it defines implementation guidelines that create a universal interface layer between AI models and the contextual information they require to function effectively.
By establishing common patterns for data access, tool execution, and prompt management, MCP eliminates the need for developers to build custom integrations for each AI model they wish to incorporate. This standardization enables developers to focus on creating flexible, powerful AI applications with seamless access to files, databases, APIs, and other essential resources—all without being locked into proprietary implementations.

Fig 1: Model Context Protocol Extensibility
Architecture
At its core, MCP acts as an abstraction layer. Instead of applications directly formatting prompts or context windows in model-specific ways, they format context according to the MCP specification. An MCP-compliant layer then translates this standardized context into the format required by the target AI model.
MCP implements a robust client-server architecture comprised of five key components that work in concert to establish secure, standardized connections between LLMs and external resources:

Fig 2: MCP Architecture Diagram
MCP Hosts
These are applications requiring contextual AI capabilities, such as conversational assistants, AI-enhanced development environments, enterprise knowledge systems, or custom business applications. Hosts integrate with MCP clients to access data and leverage external tools through the standardized protocol, allowing them to focus on delivering value rather than managing integration complexities.
MCP Clients
Clients maintain dedicated 1:1 connections with MCP Servers and handle protocol-specific operations that the server doesn't need to manage. They serve as translators, converting host application requests into standardized MCP messages, managing connection states, and handling authentication and security with servers. This abstraction layer ensures hosts can communicate with any MCP-compliant server without modification.
MCP Servers
Servers form the operational core of the MCP ecosystem, exposing critical capabilities through the standardized interface. An MCP server might provide access to document repositories, specialized databases, analytical tools, or integrate with external APIs—all through a consistent, well-defined protocol. Servers implement the business logic necessary to fulfill client requests while maintaining security boundaries.
Let’s take an example of a Github MCP Server:
Git MCP Server : For Git repositories, an MCP server transforms technical version control systems into contextually aware knowledge bases:
Intelligent code context: Rather than forcing developers to manually specify file paths, commit hashes, and diffs, a Git MCP server can respond to requests like "Find who modified the authentication logic in the last month" by understanding code semantics beyond simple text patterns.
Cross-repository knowledge: When an LLM assistant needs context across multiple repositories or branches, the MCP server handles the complex task of assembling relevant code, commit messages, pull request discussions, and documentation into a coherent context window.
Development workflow integration: Instead of having AI tools make raw API calls to Git hosting services (GitHub, GitLab, etc.), the MCP server enforces secure, standardized patterns for repository interaction, ensuring consistent behavior across different AI systems while maintaining proper authentication and access controls.
Local Data Sources
These represent secure, organization-controlled resources such as files, databases, and internal services that MCP servers can access based on fine-grained permissions. The protocol implements comprehensive security measures ensuring AI models can only access authorized resources with appropriate authentication and access controls, protecting sensitive information while enabling useful functionality.
Remote Services
These encompass external, internet-accessible services available via APIs that MCP servers can connect to as needed. They might include public knowledge bases, code repositories, specialized computational tools, or third-party business services. MCP standardizes how these external services are discovered, authenticated, and utilized within the broader AI application ecosystem.
MCP vs LLM Context Limitations
LLMs have limited context windows. MCP helps by:
Standardized Summarization: Defining protocols for context summarization techniques.
Prioritization: Allowing context elements to be tagged with priority levels, ensuring critical information isn't truncated.
Retrieval Augmented Generation (RAG) Integration: Providing a standard way to represent retrieved documents or data snippets within the context payload.
Context Segmentation: Enabling large tasks to be broken down, with MCP managing the relevant context segment for each sub-task.
Enterprise Applications of MCP
Advantages of Adopting MCP
Adopting Model Context Protocol (MCP) provides enterprises with significant strategic advantages by establishing a standardized infrastructure for managing AI contexts across diverse applications and teams. With MCP, organizations can substantially reduce redundant efforts in prompt engineering and context management, as it introduces a common, universally understood schema. This standardization allows internal development teams to focus more on core application logic and less on model-specific context handling, significantly streamlining the onboarding process for new AI models. Additionally, MCP offers considerable benefits for external partners and vendors by clearly specifying integration standards, which promotes seamless compatibility and consistent governance across third-party solutions.
Key Enterprise Considerations
When integrating MCP into enterprise environments, several critical considerations must be addressed to ensure success and security. Governance and control become central, as MCP’s structured context enables fine-grained policy enforcement, precise masking or encryption of sensitive data, and consistent audit logging. Additionally, MCP promotes scalability across multiple departments by ensuring context representations remain compatible, facilitating smoother cross-functional workflows. Vendor interoperability is significantly improved, reducing the risk of vendor lock-in by isolating model-specific changes to the MCP adapter layer rather than the core application logic. Moreover, MCP provides essential future-proofing by abstracting application logic from underlying model changes, protecting investments as AI architectures evolve. Lastly, infrastructure security and access control are paramount, necessitating robust authentication and authorization mechanisms, rigorous input validation to mitigate injection attacks, and comprehensive hardening of MCP interfaces to protect sensitive enterprise resources.
Developers Guide for MCP Implementation
Implementing Model Context Protocol effectively requires careful schema design, robust context management logic, and well-defined integration points with AI models via adapters. This guide provides practical advice for developers tasked with building or utilizing MCP within their applications.
Best Practices
Schema Design - Start Simple, Iterate:
Define clear, well-documented schemas for your core context elements (e.g., user profile, chat history, document snippet, system prompt). Use standard formats like JSON Schema or Protobuf.
Start with essential fields and add complexity gradually. Avoid trying to create a universal schema for everything at once.
Versioning is Crucial: Implement versioning for your MCP schemas from day one to manage evolution and maintain backward compatibility.
Reusability: Design schemas to be composable and reusable across different applications or agents.
Modular Context Building:
Create dedicated functions or classes responsible for generating specific types of MCP context elements.
The main application logic should orchestrate calling these builders to assemble the final MCP payload, rather than embedding complex formatting logic everywhere.
Explicit Context Window Management:
Don't rely on downstream models to implicitly handle context limits. Implement strategies within your MCP layer or context builders:
Prioritization: Use metadata tags (e.g., priority: high/medium/low) within MCP elements to guide truncation or summarization.
Summarization: Integrate summarization techniques (e.g., calling a separate summarization model, using rule-based summarization) for long context elements like chat history or documents. Store summaries as distinct MCP elements.
RAG Integration: Standardize how retrieved chunks from Retrieval Augmented Generation are represented within the MCP structure (e.g., type: retrieved_document_chunk, with source and relevance score metadata).
Configurable Strategies: Allow configuration of how context limits are handled (e.g., "truncate oldest", "summarize history", "prioritize
Thin and Pluggable Adapters:
The MCP Adapter's sole responsibility should be translating the standardized MCP payload into the specific format required by the target AI model API.
Keep business logic out of adapters.
Design adapters to be easily swappable to support different models or API versions without changing the core application or context-building logic.
Standardized Output Parsing:
Define how model responses (content, function calls, metadata) are parsed back, potentially into a standardized MCP structure (e.g., type: model_response). This facilitates logging, evaluation, and using model output as context for subsequent calls.
Handle potential errors or malformed responses from the model gracefully.
Common Pitfalls
Over-Engineering Schemas: Creating excessively complex or rigid schemas early on that are hard to adapt. Start flexible and refine based on needs.
"Leaky" Adapters: Embedding model-specific logic or assumptions outside the adapter, breaking the abstraction MCP provides.
Inefficient Context Handling: Simply concatenating all available information without intelligent prioritization, summarization, or truncation, leading to poor model performance or hitting API limits unexpectedly.
Security/Compliance as an Afterthought: Failing to tag sensitive data properly during context creation or failing to enforce policies based on those tags before model interaction.
Lack of Testing for Context Logic: Bugs in context summarization, truncation, or prioritization can be subtle and significantly impact model quality and cost.
Monolithic Context Generation: Building context in one large, complex function instead of using modular, reusable builders, making maintenance difficult.
Poor Documentation: Undocumented schemas or context element types make it hard for other developers (or future you) to understand and use the MCP implementation correctly.
By following these best practices and being mindful of potential pitfalls, developers can effectively leverage MCP to build more robust, maintainable, and interoperable LLM applications.
Understanding Agent-to-Agent (A2A) Protocol
Agent-to-Agent (A2A) Protocol defines a standardized set of rules, message formats, and interaction patterns for communication and coordination between independent software agents, particularly AI agents within a multi-agent system (MAS).
A2A makes it easy for one agent (the “client”) to send tasks to another agent (the “remote”) and get them done. The client agent creates and sends the task, while the remote agent receives it and carries it out.

Fig 3: A2A Interaction Capabilities [1]
Architectural Principles
A2A protocols often borrow principles from established distributed systems and communication paradigms like microservices, REST, gRPC, and message queuing, but tailor them for the specific needs of autonomous or semi-autonomous agents. Key principles include:
Decentralization: Agents operate independently but can discover and interact with others.
Standardization: Common message structures and interaction protocols ensure interoperability.
Asynchronicity: Often favored to handle network latency and allow agents to work concurrently, though synchronous request/reply is also supported.
Discoverability: Mechanisms for agents to find other agents based on capabilities.
Negotiation: Protocols for agents to agree on tasks, data formats, or workflow steps.
Technical Specifications
Message Formats: Typically standardized formats like JSON or Protobuf are used. Messages usually include headers (sender ID, recipient ID, message ID, timestamp, intent/type) and a payload (task description, data, query, status update). MCP-structured context can often be part of the A2A payload.
Authentication Mechanisms: Ensures agents are who they claim to be. Options include API keys, OAuth tokens (e.g., client credentials flow), mutual TLS (mTLS), or JWTs signed by a trusted authority.
Routing Protocols: Defines how messages get from sender to recipient. This could involve direct HTTP calls, message queues (like RabbitMQ, Kafka), or dedicated agent communication platforms/meshes.
Agent Discovery: Mechanisms like a central registry, gossip protocols, or service discovery tools (e.g., Consul, Etcd) allow agents to find others offering needed capabilities.
Capability Negotiation: Standardized ways for agents to advertise their skills (e.g., "summarize text," "extract entities," "query database schema X") and for requesting agents to specify requirements.
Task Delegation & Coordination: Protocols for assigning tasks, tracking progress, handling errors, and coordinating multi-step workflows (e.g., using patterns like Saga or Choreography).
A2A vs Other Protocols
vs. REST/HTTP: A2A builds upon HTTP but adds agent-specific semantics (discovery, negotiation, standardized task types). Simple REST is often too generic for complex agent coordination.
vs. gRPC: Offers performance benefits (Protobuf, HTTP/2) but requires stricter schema definitions. A2A can leverage gRPC as a transport layer.
vs. Message Queues (Kafka, RabbitMQ): Excellent for asynchronous, decoupled communication. A2A protocols define the content and meaning of messages flowing through these queues, adding a layer of application-level understanding and not only this but conversation patterns, capability discovery, and error handling specific to agent interactions.
Enterprise Applications of A2A
Advantages of Adopting A2A
The A2A protocol offers enterprises a standardized framework that significantly enhances the interoperability and scalability of AI systems. By enabling seamless communication between AI agents, regardless of their underlying technologies or vendors, A2A facilitates the creation of dynamic, multi-agent ecosystems. This interoperability allows for the integration of specialized agents across various departments, promoting a more cohesive and efficient workflow. Moreover, A2A supports dynamic capability discovery through mechanisms like “Agent Cards,” enabling agents to advertise their functionalities and discover others based on capabilities, thus fostering a collaborative environment where agents can coordinate tasks effectively.
In addition to interoperability, A2A provides robust support for long-running tasks, offering mechanisms for real-time feedback, notifications, and state updates throughout the task lifecycle. This is particularly beneficial for complex business operations that require extended processing times. The protocol’s design, built upon existing standards such as HTTP, SSE, and JSON-RPC, ensures compatibility with current IT infrastructures, simplifying integration and reducing the need for custom solutions.
Furthermore, A2A incorporates enterprise-grade security features, including authentication and authorization mechanisms, ensuring secure communication between agents. By adopting A2A, enterprises can build modular and composable AI systems, where agents can be easily swapped, updated, or added with minimal friction, significantly accelerating development and improving maintainability
Key Enterprise Considerations
Implementing the A2A protocol within an enterprise setting necessitates careful consideration of several critical factors to ensure effective deployment and operation. Security and compliance are paramount; enterprises must establish robust authentication and authorization mechanisms, such as mutual TLS (mTLS) and OAuth tokens, to safeguard agent communications. Additionally, maintaining comprehensive audit trails and enforcing fine-grained access controls are essential for meeting regulatory requirements and ensuring data integrity.
Scalability and performance are also crucial considerations. As the number of interacting agents grows, enterprises need to ensure that the underlying infrastructure can scale accordingly. This includes optimizing message routing, load balancing, and monitoring to maintain performance. Integration with existing systems is another key aspect; A2A should be thoughtfully integrated with current enterprise applications and workflows, potentially involving the development of adapters or middleware to bridge between A2A-compliant agents and legacy systems. Furthermore, implementing governance frameworks to define and enforce policies around agent interactions, data sharing, and task delegation is vital for maintaining control and oversight. Deploying comprehensive monitoring and observability tools is also essential for tracking agent interactions, diagnosing issues, and ensuring the health of the multi-agent system. Finally, enterprises should invest in training and change management strategies to facilitate adoption and minimize disruption, as well as engage with the broader A2A community.
Developers Guide for A2A Implementation
Implementing robust A2A communication requires careful planning and adherence to best practices.
Best Practices
Choose the Right Transport:
Synchronous (Request/Reply): Use HTTP/S (REST-like) or gRPC for immediate responses when needed. Good for simple queries or commands.
Asynchronous (Fire & Forget/Events): Use Message Queues (RabbitMQ, Kafka, NATS, Pulsar) for decoupling, resilience, and workflows where immediate response isn't critical. Better for scalability and handling long-running tasks.
Define Clear Message Schemas: Use JSON Schema, Protobuf, or Avro to strictly define A2A message structures (headers and payload). Include versioning in your schemas.
Standardize Message Headers: Always include essential headers: unique message ID, correlation ID (to track workflows), sender/recipient IDs, timestamp, message type/intent.
Idempotency: Design message handlers to be idempotent where possible, especially with asynchronous systems. Processing the same message twice should not cause unintended side effects. Use the message ID for deduplication.
Error Handling & Resilience:
Define standard error message formats.
Implement retry mechanisms (e.g., exponential backoff) for transient network issues.
Use Dead Letter Queues (DLQs) in message brokers to handle messages that consistently fail processing.
Implement circuit breaker patterns to prevent cascading failures.
Agent Discovery: Use a service registry or a dedicated agent directory for dynamic discovery. Avoid hardcoding agent addresses.
Security First: Implement strong authentication and authorization from the start. Use standard protocols (OAuth 2.0, mTLS, JWT). Encrypt traffic (TLS).
Monitoring and Observability:
Logging: Log key information for each message sent/received (headers, size, timing). Use structured logging.
Tracing: Implement distributed tracing (e.g., OpenTelemetry) using the correlation ID to track requests across multiple agents.
Metrics: Monitor queue depths, message processing times, error rates, agent availability.
Common Pitfalls
Overly Chatty Communication: Designing agents that require excessive back-and-forth increases latency and complexity. Aim for well-defined, substantive interactions.
Ignoring Versioning: Failing to version message schemas leads to compatibility issues as agents evolve.
Lack of State Management: Assuming agents are stateless when workflows require state can lead to errors. Consider external state stores or stateful agent patterns if needed.
Inadequate Monitoring: Flying blind without proper logging, tracing, and metrics makes debugging and optimization impossible.
Security as an Afterthought: Adding security late in the process is much harder and riskier.
Performance Optimization
Asynchronous Communication: Prefer message queues for decoupling and parallelism.
Batching: Send/process messages in batches where appropriate (e.g., batch updates to a database).
Efficient Serialization: Use binary formats like Protobuf or MessagePack instead of JSON for performance-critical paths.
Caching: Cache frequently accessed data or results within agents (with appropriate invalidation).
Optimize Transport: Tune message broker configurations, use efficient network protocols (gRPC).
Enterprise Integration: Making MCP and A2A Work Together
MCP and A2A are highly synergistic. MCP standardizes the content (context) an agent needs or produces, while A2A standardizes the interaction (communication) between agents.
Integration
A common integration strategy between MCP and A2A involves embedding MCP-formatted context within the payload of A2A messages. In practice, an agent initiating a task via A2A includes the necessary input data structured according to MCP standards. The receiving agent completes the requested operation and returns its results, often formatted using MCP as well, ensuring consistent and standardized context communication. Additionally, enterprises can deploy a dedicated “Context Orchestration Agent” that leverages A2A protocols to coordinate interactions with various specialized data source agents, such as those interfacing with databases or document repositories. This orchestration agent assembles the collected data into a structured MCP payload, which can then be provided seamlessly to processing agents. Furthermore, A2A’s capability negotiation mechanism benefits significantly from MCP, as agents can clearly specify the MCP schemas they support for both input and output, streamlining discovery and collaboration processes across diverse multi-agent workflows.
Architectural Patterns for Deployment
For enterprise deployments of multi-agent systems, several architectural patterns are particularly effective. One common approach is the Agent Mesh or Fabric, which resembles traditional service meshes like Istio or Linkerd but is specifically optimized for agent-based communication. This model provides a dedicated infrastructure layer that manages A2A routing, agent discovery, security through mutual TLS (mTLS), observability, and policy enforcement, with agent communication handled through mesh sidecars or proxies. Alternatively, a Central Message Bus approach employs enterprise-grade message queues such as Kafka, Pulsar, or Solace to facilitate agent interactions via publish-subscribe patterns. Here, governance and access control are managed directly at the broker or topic level, enabling scalable and decoupled agent communication. Finally, the Agent Gateway architecture adapts the API Gateway pattern for agent interactions, where external requests and internal agents interact with a central gateway responsible for routing A2A messages to appropriate backend agents, enforcing security measures, and potentially translating between different communication protocols, thereby simplifying and standardizing access to agent services.
Technical Considerations
Successfully implementing MCP and A2A in an enterprise context involves careful attention to several critical technical considerations. Authentication and authorization require deliberate decisions around centralized versus decentralized enforcement mechanisms, robust token formats such as JWTs or OAuth, and secure management of keys and secrets to safeguard agent communications. Equally important is establishing comprehensive monitoring and observability practices, including standardized logging formats, consistent trace propagation across agent interactions, and clear definition of KPIs to measure and optimize agent effectiveness. Scalability and load balancing strategies will vary depending on the chosen A2A transport, such as employing Kubernetes Horizontal Pod Autoscalers for agent pods, utilizing Kafka consumer groups for message-driven workflows, or leveraging built-in load-balancing capabilities of gRPC. Additionally, robust error handling and recovery processes must be implemented, potentially employing distributed patterns like Saga or choreography for complex workflows, complemented by well-defined retry logic and effective use of Dead Letter Queues (DLQs) to handle persistent failures gracefully.
Complementing these technical foundations, security and governance require implementing centralized identity management (e.g., via an Identity Provider for issuing JWT or OAuth tokens) and strategically placed policy enforcement points within agent infrastructures. MCP tags further strengthen security by clearly marking sensitive data for targeted policy enforcement. Comprehensive auditing through centralized logging of all A2A interactions and MCP context usage ensures transparency, supports compliance, and facilitates effective oversight.
Future Directions & Enterprise Strategy
MCP and A2A are not static concepts; they represent an evolving approach to building complex AI systems.
Upcoming Developments
Formal Standardization Efforts: Expect industry groups or consortia (potentially involving major cloud providers, AI labs, and enterprise users) to propose more formal standards for both MCP and A2A, akin to OpenAPI for REST APIs or CloudEvents for eventing.
Richer Context Types (MCP): Support for multimodal context (images, audio, video), more sophisticated knowledge graph representations, and standardized ways to include agent reasoning traces within context.
Advanced A2A Capabilities: Protocols for complex negotiation, automated composition of agent services, decentralized trust and reputation systems for agents, and tighter integration with workflow orchestration engines.
AI Model Integration: MCP adapters will become more sophisticated, potentially handling automated context optimization (prompt compression, selective inclusion) based on model capabilities and cost.
Strategic Framework for Adoption
Acknowledge the Need: Recognize that ad-hoc integration is unsustainable for complex, mission-critical AI.
Invest in Standardization: Champion internal efforts to define and adopt MCP and A2A principles, even if formal external standards are still emerging. Start with core schemas and protocols relevant to your business.
Build Incrementally: Don't try to boil the ocean. Start with pilot projects, prove the value, and build out infrastructure and standards gradually.
Foster Collaboration: Encourage communication between AI/ML teams, platform engineering, security, and governance stakeholders.
Prioritize Interoperability: Design systems with the assumption that you will need to integrate agents and models from different sources (internal, vendor, open-source).
Stay Informed: Monitor developments in AI orchestration, agent frameworks (like LangChain, AutoGen, CrewAI), and emerging standardization efforts.
Future-proofing AI architectures hinge on building systems with clear abstraction and modularity, principles directly supported by MCP and A2A. By leveraging these protocols as foundational abstraction layers, enterprises can keep application logic centered on core business processes, delegating model-specific context management and agent communications entirely to MCP and A2A.
Conclusion
The era of standalone AI models solving isolated problems is giving way to a future of collaborative intelligence, where multi-agent systems tackle complex enterprise challenges. The primary obstacles to realizing this future at scale are the chaos of inconsistent context management and brittle, ad-hoc agent communication.
Model Context Protocol (MCP) and Agent-to-Agent (A2A) communication directly address these bottlenecks. MCP brings order to how AI models understand the world by standardizing context, overcoming limitations, and enabling governance. A2A brings order to how AI agents interact, enabling scalable, robust, and interoperable collaboration through standardized communication patterns.
Together, MCP and A2A form a powerful duo, providing the foundational layer for building the next generation of enterprise AI systems. They enable organizations to move beyond bespoke integrations towards truly scalable, maintainable, secure, and interoperable AI ecosystems. For enterprises serious about leveraging AI for strategic advantage, adopting and investing in MCP and A2A principles is not just a technical choice – it's a strategic imperative. The future of enterprise AI will be built on collaboration, and these protocols provide the blueprint for making that collaboration effective.
Case Study
HR Intelligent Document Processing System
Use Case: An enterprise needs to process thousands of job applications efficiently. The goal is to parse resumes, match candidates against job descriptions, evaluate qualifications, and flag top candidates for human review, integrating with the existing Applicant Tracking System (ATS).
Technical Architecture of the multi-agent system:
Document Ingestion Agent: Monitors application sources (email, web portal), retrieves new resumes/cover letters.
Document Parsing Agent: Uses OCR and layout analysis (potentially specialized models) to extract raw text and structure from various formats (PDF, DOCX, scanned images).
Information Extraction Agent: Employs an LLM or specialized NLP model to identify and extract key entities (skills, experience, education, contact info etc.)
Candidate Evaluation Agent: Compares extracted information against job description requirements, calculates match scores, and ranks candidates. Uses potentially different models or rule sets based on role complexity.
ATS Integration Agent: Formats evaluation results and updates the central Applicant Tracking System.
Human-in-the-Loop (HITL) Agent: Flags ambiguous cases or top candidates for review by HR personnel via a dedicated interface.
In the multi agent architecture above, adding standard protocols have the following effects.
MCP enables the following:
Consistent Context for Extraction: The Parsing Agent outputs text/structure in a standardized MCP format. The Information Extraction Agent receives this consistent context, regardless of the original document format or the specific parsing model used.
Handling Large Documents: For long resumes or complex cover letters exceeding LLM context limits, MCP facilitates segmentation. The Parsing Agent might break the document, and MCP provides the context linking these segments for the Extraction Agent.
Standardized Input/Output for ATS: The Evaluation Agent produces results (scores, rankings, extracted data) in an MCP-defined structure, which the ATS Integration Agent consumes, simplifying integration logic.
A2A facilitates the following:
Coordinated Workflow: A2A defines the handoffs. Ingestion Agent sends a "NEW_DOCUMENT" task via A2A message (e.g., on a Kafka topic) to the Parsing Agent pool. Parsing Agent sends "PARSE_COMPLETE" with MCP context payload to the Extraction Agent. Extraction Agent sends "EXTRACTION_COMPLETE" to the Evaluation Agent, and so on.
Clear Communication Protocols: Message types like REQUEST_PARSE, PARSE_RESULT, REQUEST_EVALUATION, EVALUATION_RESULT, UPDATE_ATS are defined within the A2A protocol, ensuring clarity. Error messages (PARSE_FAILED, EVALUATION_ERROR) follow a standard format.
Scalable Processing: Agents can be scaled independently. If parsing becomes a bottleneck, more Parsing Agents can be deployed; they pick up tasks via the A2A communication channel (e.g., message queue). A2A routing handles load balancing.
References
The following resources provide deeper technical insights and enterprise perspectives on Model Context Protocol (MCP) and Agent-to-Agent (A2A) communication.
MCP Documentation (Anthropic) – https://docs.anthropic.com/en/docs/agents-and-tools/mcp
MCP Servers (GitHub Repository) – https://github.com/modelcontextprotocol/servers
Enterprise Security for MCP (arXiv) – https://arxiv.org/abs/2504.08623
A2A Protocol Overview (a2aprotocol.ai) – https://a2aprotocol.ai
Multi-Agent Collaboration Study (arXiv) – https://arxiv.org/abs/2412.05449
The hype surrounding Generative AI and Multi-Agent Systems often clashes with the messy reality of enterprise integration. We're rapidly moving beyond standalone AI models towards sophisticated, multi-agent systems designed to tackle complex, end-to-end enterprise workflows – a transition whose complexities we explored in our previous post, "Navigating the complexities of building and scaling Multi-Agent System". Yet, as these AI ecosystems grow in sophistication and scale, agent communication and context management have emerged as critical bottlenecks, hindering the very scalability, interoperability, and maintainability they promise.
The core problem?
A lack of standardization. We see bespoke integrations, inconsistent context handling between different AI models especially LLM, and brittle, ad-hoc communication methods between AI agents. This leads to complex, difficult-to-debug systems that struggle to scale and adapt.
Agent Communication Is the New Bottleneck
As we build more sophisticated AI systems composed of multiple specialized agents (e.g., one agent for data retrieval, another for analysis, a third for summarization), the interactions between these agents become paramount.
Challenges in Multi-Agent Systems (MAS)
Ad-hoc Communication: Teams often invent their own communication methods for each project, leading to inconsistency and integration nightmares.
Agent Coupling: Agents become overly dependent on the specific implementation details of others, making changes difficult and risky.
Lack of Discoverability: Agents can't easily find other agents that could help them, limiting dynamic collaboration.
Error Handling Complexity: Failures in one agent can cascade unpredictably without standardized error reporting and recovery protocols.
Debugging Hell: Tracing a request or workflow across multiple agents with different communication styles is incredibly difficult.
How Traditional Approaches Fail at Scale
Simple point-to-point integrations or basic API calls work for a few agents but break down quickly. Imagine dozens or hundreds of agents – the number of potential interactions explodes (N*(N-1)). Without standardization, this becomes an unmanageable web of dependencies.
Increased Latency: Poorly optimized or synchronous communication chains add significant delays.
High Failure Rates: Lack of standardized error handling leads to brittle systems where one agent failure brings down entire workflows.
Integration Effort: Time spent mapping data formats and communication protocols between agents becomes a major development cost.
Monitoring Complexity: Tracking performance and health across diverse communication channels is challenging.
Enter the power duo: MCP & A2A
These complementary protocols provide the standardized foundation needed to overcome these challenges. MCP focuses on standardizing how AI models receive and understand context, while A2A defines how independent AI agents interact and collaborate.
This comprehensive guide will dissect MCP and A2A, exploring their technical underpinnings, enterprise applications, and practical implementation strategies. We will delve into technical specifications, architectural patterns, real-world case studies, and developer best practices. By the end, you'll understand why MCP and A2A together form the foundation for truly scalable, interoperable multi-agent AI systems that can transform enterprise operations.
Understanding Model Context Protocol (MCP)
Model Context Protocol (MCP) is an open-source protocol developed by Anthropic (creators of Claude) that establishes a standardized framework for LLM integrations across the industry. As an open-source initiative, MCP aims to create a universal standard that benefits the entire AI ecosystem through collaborative development and widespread adoption.
At its core, MCP provides a standardized methodology for connecting LLM applications with external data sources, knowledge bases, and tools. Essentially, it defines implementation guidelines that create a universal interface layer between AI models and the contextual information they require to function effectively.
By establishing common patterns for data access, tool execution, and prompt management, MCP eliminates the need for developers to build custom integrations for each AI model they wish to incorporate. This standardization enables developers to focus on creating flexible, powerful AI applications with seamless access to files, databases, APIs, and other essential resources—all without being locked into proprietary implementations.

Fig 1: Model Context Protocol Extensibility
Architecture
At its core, MCP acts as an abstraction layer. Instead of applications directly formatting prompts or context windows in model-specific ways, they format context according to the MCP specification. An MCP-compliant layer then translates this standardized context into the format required by the target AI model.
MCP implements a robust client-server architecture comprised of five key components that work in concert to establish secure, standardized connections between LLMs and external resources:

Fig 2: MCP Architecture Diagram
MCP Hosts
These are applications requiring contextual AI capabilities, such as conversational assistants, AI-enhanced development environments, enterprise knowledge systems, or custom business applications. Hosts integrate with MCP clients to access data and leverage external tools through the standardized protocol, allowing them to focus on delivering value rather than managing integration complexities.
MCP Clients
Clients maintain dedicated 1:1 connections with MCP Servers and handle protocol-specific operations that the server doesn't need to manage. They serve as translators, converting host application requests into standardized MCP messages, managing connection states, and handling authentication and security with servers. This abstraction layer ensures hosts can communicate with any MCP-compliant server without modification.
MCP Servers
Servers form the operational core of the MCP ecosystem, exposing critical capabilities through the standardized interface. An MCP server might provide access to document repositories, specialized databases, analytical tools, or integrate with external APIs—all through a consistent, well-defined protocol. Servers implement the business logic necessary to fulfill client requests while maintaining security boundaries.
Let’s take an example of a Github MCP Server:
Git MCP Server : For Git repositories, an MCP server transforms technical version control systems into contextually aware knowledge bases:
Intelligent code context: Rather than forcing developers to manually specify file paths, commit hashes, and diffs, a Git MCP server can respond to requests like "Find who modified the authentication logic in the last month" by understanding code semantics beyond simple text patterns.
Cross-repository knowledge: When an LLM assistant needs context across multiple repositories or branches, the MCP server handles the complex task of assembling relevant code, commit messages, pull request discussions, and documentation into a coherent context window.
Development workflow integration: Instead of having AI tools make raw API calls to Git hosting services (GitHub, GitLab, etc.), the MCP server enforces secure, standardized patterns for repository interaction, ensuring consistent behavior across different AI systems while maintaining proper authentication and access controls.
Local Data Sources
These represent secure, organization-controlled resources such as files, databases, and internal services that MCP servers can access based on fine-grained permissions. The protocol implements comprehensive security measures ensuring AI models can only access authorized resources with appropriate authentication and access controls, protecting sensitive information while enabling useful functionality.
Remote Services
These encompass external, internet-accessible services available via APIs that MCP servers can connect to as needed. They might include public knowledge bases, code repositories, specialized computational tools, or third-party business services. MCP standardizes how these external services are discovered, authenticated, and utilized within the broader AI application ecosystem.
MCP vs LLM Context Limitations
LLMs have limited context windows. MCP helps by:
Standardized Summarization: Defining protocols for context summarization techniques.
Prioritization: Allowing context elements to be tagged with priority levels, ensuring critical information isn't truncated.
Retrieval Augmented Generation (RAG) Integration: Providing a standard way to represent retrieved documents or data snippets within the context payload.
Context Segmentation: Enabling large tasks to be broken down, with MCP managing the relevant context segment for each sub-task.
Enterprise Applications of MCP
Advantages of Adopting MCP
Adopting Model Context Protocol (MCP) provides enterprises with significant strategic advantages by establishing a standardized infrastructure for managing AI contexts across diverse applications and teams. With MCP, organizations can substantially reduce redundant efforts in prompt engineering and context management, as it introduces a common, universally understood schema. This standardization allows internal development teams to focus more on core application logic and less on model-specific context handling, significantly streamlining the onboarding process for new AI models. Additionally, MCP offers considerable benefits for external partners and vendors by clearly specifying integration standards, which promotes seamless compatibility and consistent governance across third-party solutions.
Key Enterprise Considerations
When integrating MCP into enterprise environments, several critical considerations must be addressed to ensure success and security. Governance and control become central, as MCP’s structured context enables fine-grained policy enforcement, precise masking or encryption of sensitive data, and consistent audit logging. Additionally, MCP promotes scalability across multiple departments by ensuring context representations remain compatible, facilitating smoother cross-functional workflows. Vendor interoperability is significantly improved, reducing the risk of vendor lock-in by isolating model-specific changes to the MCP adapter layer rather than the core application logic. Moreover, MCP provides essential future-proofing by abstracting application logic from underlying model changes, protecting investments as AI architectures evolve. Lastly, infrastructure security and access control are paramount, necessitating robust authentication and authorization mechanisms, rigorous input validation to mitigate injection attacks, and comprehensive hardening of MCP interfaces to protect sensitive enterprise resources.
Developers Guide for MCP Implementation
Implementing Model Context Protocol effectively requires careful schema design, robust context management logic, and well-defined integration points with AI models via adapters. This guide provides practical advice for developers tasked with building or utilizing MCP within their applications.
Best Practices
Schema Design - Start Simple, Iterate:
Define clear, well-documented schemas for your core context elements (e.g., user profile, chat history, document snippet, system prompt). Use standard formats like JSON Schema or Protobuf.
Start with essential fields and add complexity gradually. Avoid trying to create a universal schema for everything at once.
Versioning is Crucial: Implement versioning for your MCP schemas from day one to manage evolution and maintain backward compatibility.
Reusability: Design schemas to be composable and reusable across different applications or agents.
Modular Context Building:
Create dedicated functions or classes responsible for generating specific types of MCP context elements.
The main application logic should orchestrate calling these builders to assemble the final MCP payload, rather than embedding complex formatting logic everywhere.
Explicit Context Window Management:
Don't rely on downstream models to implicitly handle context limits. Implement strategies within your MCP layer or context builders:
Prioritization: Use metadata tags (e.g., priority: high/medium/low) within MCP elements to guide truncation or summarization.
Summarization: Integrate summarization techniques (e.g., calling a separate summarization model, using rule-based summarization) for long context elements like chat history or documents. Store summaries as distinct MCP elements.
RAG Integration: Standardize how retrieved chunks from Retrieval Augmented Generation are represented within the MCP structure (e.g., type: retrieved_document_chunk, with source and relevance score metadata).
Configurable Strategies: Allow configuration of how context limits are handled (e.g., "truncate oldest", "summarize history", "prioritize
Thin and Pluggable Adapters:
The MCP Adapter's sole responsibility should be translating the standardized MCP payload into the specific format required by the target AI model API.
Keep business logic out of adapters.
Design adapters to be easily swappable to support different models or API versions without changing the core application or context-building logic.
Standardized Output Parsing:
Define how model responses (content, function calls, metadata) are parsed back, potentially into a standardized MCP structure (e.g., type: model_response). This facilitates logging, evaluation, and using model output as context for subsequent calls.
Handle potential errors or malformed responses from the model gracefully.
Common Pitfalls
Over-Engineering Schemas: Creating excessively complex or rigid schemas early on that are hard to adapt. Start flexible and refine based on needs.
"Leaky" Adapters: Embedding model-specific logic or assumptions outside the adapter, breaking the abstraction MCP provides.
Inefficient Context Handling: Simply concatenating all available information without intelligent prioritization, summarization, or truncation, leading to poor model performance or hitting API limits unexpectedly.
Security/Compliance as an Afterthought: Failing to tag sensitive data properly during context creation or failing to enforce policies based on those tags before model interaction.
Lack of Testing for Context Logic: Bugs in context summarization, truncation, or prioritization can be subtle and significantly impact model quality and cost.
Monolithic Context Generation: Building context in one large, complex function instead of using modular, reusable builders, making maintenance difficult.
Poor Documentation: Undocumented schemas or context element types make it hard for other developers (or future you) to understand and use the MCP implementation correctly.
By following these best practices and being mindful of potential pitfalls, developers can effectively leverage MCP to build more robust, maintainable, and interoperable LLM applications.
Understanding Agent-to-Agent (A2A) Protocol
Agent-to-Agent (A2A) Protocol defines a standardized set of rules, message formats, and interaction patterns for communication and coordination between independent software agents, particularly AI agents within a multi-agent system (MAS).
A2A makes it easy for one agent (the “client”) to send tasks to another agent (the “remote”) and get them done. The client agent creates and sends the task, while the remote agent receives it and carries it out.

Fig 3: A2A Interaction Capabilities [1]
Architectural Principles
A2A protocols often borrow principles from established distributed systems and communication paradigms like microservices, REST, gRPC, and message queuing, but tailor them for the specific needs of autonomous or semi-autonomous agents. Key principles include:
Decentralization: Agents operate independently but can discover and interact with others.
Standardization: Common message structures and interaction protocols ensure interoperability.
Asynchronicity: Often favored to handle network latency and allow agents to work concurrently, though synchronous request/reply is also supported.
Discoverability: Mechanisms for agents to find other agents based on capabilities.
Negotiation: Protocols for agents to agree on tasks, data formats, or workflow steps.
Technical Specifications
Message Formats: Typically standardized formats like JSON or Protobuf are used. Messages usually include headers (sender ID, recipient ID, message ID, timestamp, intent/type) and a payload (task description, data, query, status update). MCP-structured context can often be part of the A2A payload.
Authentication Mechanisms: Ensures agents are who they claim to be. Options include API keys, OAuth tokens (e.g., client credentials flow), mutual TLS (mTLS), or JWTs signed by a trusted authority.
Routing Protocols: Defines how messages get from sender to recipient. This could involve direct HTTP calls, message queues (like RabbitMQ, Kafka), or dedicated agent communication platforms/meshes.
Agent Discovery: Mechanisms like a central registry, gossip protocols, or service discovery tools (e.g., Consul, Etcd) allow agents to find others offering needed capabilities.
Capability Negotiation: Standardized ways for agents to advertise their skills (e.g., "summarize text," "extract entities," "query database schema X") and for requesting agents to specify requirements.
Task Delegation & Coordination: Protocols for assigning tasks, tracking progress, handling errors, and coordinating multi-step workflows (e.g., using patterns like Saga or Choreography).
A2A vs Other Protocols
vs. REST/HTTP: A2A builds upon HTTP but adds agent-specific semantics (discovery, negotiation, standardized task types). Simple REST is often too generic for complex agent coordination.
vs. gRPC: Offers performance benefits (Protobuf, HTTP/2) but requires stricter schema definitions. A2A can leverage gRPC as a transport layer.
vs. Message Queues (Kafka, RabbitMQ): Excellent for asynchronous, decoupled communication. A2A protocols define the content and meaning of messages flowing through these queues, adding a layer of application-level understanding and not only this but conversation patterns, capability discovery, and error handling specific to agent interactions.
Enterprise Applications of A2A
Advantages of Adopting A2A
The A2A protocol offers enterprises a standardized framework that significantly enhances the interoperability and scalability of AI systems. By enabling seamless communication between AI agents, regardless of their underlying technologies or vendors, A2A facilitates the creation of dynamic, multi-agent ecosystems. This interoperability allows for the integration of specialized agents across various departments, promoting a more cohesive and efficient workflow. Moreover, A2A supports dynamic capability discovery through mechanisms like “Agent Cards,” enabling agents to advertise their functionalities and discover others based on capabilities, thus fostering a collaborative environment where agents can coordinate tasks effectively.
In addition to interoperability, A2A provides robust support for long-running tasks, offering mechanisms for real-time feedback, notifications, and state updates throughout the task lifecycle. This is particularly beneficial for complex business operations that require extended processing times. The protocol’s design, built upon existing standards such as HTTP, SSE, and JSON-RPC, ensures compatibility with current IT infrastructures, simplifying integration and reducing the need for custom solutions.
Furthermore, A2A incorporates enterprise-grade security features, including authentication and authorization mechanisms, ensuring secure communication between agents. By adopting A2A, enterprises can build modular and composable AI systems, where agents can be easily swapped, updated, or added with minimal friction, significantly accelerating development and improving maintainability
Key Enterprise Considerations
Implementing the A2A protocol within an enterprise setting necessitates careful consideration of several critical factors to ensure effective deployment and operation. Security and compliance are paramount; enterprises must establish robust authentication and authorization mechanisms, such as mutual TLS (mTLS) and OAuth tokens, to safeguard agent communications. Additionally, maintaining comprehensive audit trails and enforcing fine-grained access controls are essential for meeting regulatory requirements and ensuring data integrity.
Scalability and performance are also crucial considerations. As the number of interacting agents grows, enterprises need to ensure that the underlying infrastructure can scale accordingly. This includes optimizing message routing, load balancing, and monitoring to maintain performance. Integration with existing systems is another key aspect; A2A should be thoughtfully integrated with current enterprise applications and workflows, potentially involving the development of adapters or middleware to bridge between A2A-compliant agents and legacy systems. Furthermore, implementing governance frameworks to define and enforce policies around agent interactions, data sharing, and task delegation is vital for maintaining control and oversight. Deploying comprehensive monitoring and observability tools is also essential for tracking agent interactions, diagnosing issues, and ensuring the health of the multi-agent system. Finally, enterprises should invest in training and change management strategies to facilitate adoption and minimize disruption, as well as engage with the broader A2A community.
Developers Guide for A2A Implementation
Implementing robust A2A communication requires careful planning and adherence to best practices.
Best Practices
Choose the Right Transport:
Synchronous (Request/Reply): Use HTTP/S (REST-like) or gRPC for immediate responses when needed. Good for simple queries or commands.
Asynchronous (Fire & Forget/Events): Use Message Queues (RabbitMQ, Kafka, NATS, Pulsar) for decoupling, resilience, and workflows where immediate response isn't critical. Better for scalability and handling long-running tasks.
Define Clear Message Schemas: Use JSON Schema, Protobuf, or Avro to strictly define A2A message structures (headers and payload). Include versioning in your schemas.
Standardize Message Headers: Always include essential headers: unique message ID, correlation ID (to track workflows), sender/recipient IDs, timestamp, message type/intent.
Idempotency: Design message handlers to be idempotent where possible, especially with asynchronous systems. Processing the same message twice should not cause unintended side effects. Use the message ID for deduplication.
Error Handling & Resilience:
Define standard error message formats.
Implement retry mechanisms (e.g., exponential backoff) for transient network issues.
Use Dead Letter Queues (DLQs) in message brokers to handle messages that consistently fail processing.
Implement circuit breaker patterns to prevent cascading failures.
Agent Discovery: Use a service registry or a dedicated agent directory for dynamic discovery. Avoid hardcoding agent addresses.
Security First: Implement strong authentication and authorization from the start. Use standard protocols (OAuth 2.0, mTLS, JWT). Encrypt traffic (TLS).
Monitoring and Observability:
Logging: Log key information for each message sent/received (headers, size, timing). Use structured logging.
Tracing: Implement distributed tracing (e.g., OpenTelemetry) using the correlation ID to track requests across multiple agents.
Metrics: Monitor queue depths, message processing times, error rates, agent availability.
Common Pitfalls
Overly Chatty Communication: Designing agents that require excessive back-and-forth increases latency and complexity. Aim for well-defined, substantive interactions.
Ignoring Versioning: Failing to version message schemas leads to compatibility issues as agents evolve.
Lack of State Management: Assuming agents are stateless when workflows require state can lead to errors. Consider external state stores or stateful agent patterns if needed.
Inadequate Monitoring: Flying blind without proper logging, tracing, and metrics makes debugging and optimization impossible.
Security as an Afterthought: Adding security late in the process is much harder and riskier.
Performance Optimization
Asynchronous Communication: Prefer message queues for decoupling and parallelism.
Batching: Send/process messages in batches where appropriate (e.g., batch updates to a database).
Efficient Serialization: Use binary formats like Protobuf or MessagePack instead of JSON for performance-critical paths.
Caching: Cache frequently accessed data or results within agents (with appropriate invalidation).
Optimize Transport: Tune message broker configurations, use efficient network protocols (gRPC).
Enterprise Integration: Making MCP and A2A Work Together
MCP and A2A are highly synergistic. MCP standardizes the content (context) an agent needs or produces, while A2A standardizes the interaction (communication) between agents.
Integration
A common integration strategy between MCP and A2A involves embedding MCP-formatted context within the payload of A2A messages. In practice, an agent initiating a task via A2A includes the necessary input data structured according to MCP standards. The receiving agent completes the requested operation and returns its results, often formatted using MCP as well, ensuring consistent and standardized context communication. Additionally, enterprises can deploy a dedicated “Context Orchestration Agent” that leverages A2A protocols to coordinate interactions with various specialized data source agents, such as those interfacing with databases or document repositories. This orchestration agent assembles the collected data into a structured MCP payload, which can then be provided seamlessly to processing agents. Furthermore, A2A’s capability negotiation mechanism benefits significantly from MCP, as agents can clearly specify the MCP schemas they support for both input and output, streamlining discovery and collaboration processes across diverse multi-agent workflows.
Architectural Patterns for Deployment
For enterprise deployments of multi-agent systems, several architectural patterns are particularly effective. One common approach is the Agent Mesh or Fabric, which resembles traditional service meshes like Istio or Linkerd but is specifically optimized for agent-based communication. This model provides a dedicated infrastructure layer that manages A2A routing, agent discovery, security through mutual TLS (mTLS), observability, and policy enforcement, with agent communication handled through mesh sidecars or proxies. Alternatively, a Central Message Bus approach employs enterprise-grade message queues such as Kafka, Pulsar, or Solace to facilitate agent interactions via publish-subscribe patterns. Here, governance and access control are managed directly at the broker or topic level, enabling scalable and decoupled agent communication. Finally, the Agent Gateway architecture adapts the API Gateway pattern for agent interactions, where external requests and internal agents interact with a central gateway responsible for routing A2A messages to appropriate backend agents, enforcing security measures, and potentially translating between different communication protocols, thereby simplifying and standardizing access to agent services.
Technical Considerations
Successfully implementing MCP and A2A in an enterprise context involves careful attention to several critical technical considerations. Authentication and authorization require deliberate decisions around centralized versus decentralized enforcement mechanisms, robust token formats such as JWTs or OAuth, and secure management of keys and secrets to safeguard agent communications. Equally important is establishing comprehensive monitoring and observability practices, including standardized logging formats, consistent trace propagation across agent interactions, and clear definition of KPIs to measure and optimize agent effectiveness. Scalability and load balancing strategies will vary depending on the chosen A2A transport, such as employing Kubernetes Horizontal Pod Autoscalers for agent pods, utilizing Kafka consumer groups for message-driven workflows, or leveraging built-in load-balancing capabilities of gRPC. Additionally, robust error handling and recovery processes must be implemented, potentially employing distributed patterns like Saga or choreography for complex workflows, complemented by well-defined retry logic and effective use of Dead Letter Queues (DLQs) to handle persistent failures gracefully.
Complementing these technical foundations, security and governance require implementing centralized identity management (e.g., via an Identity Provider for issuing JWT or OAuth tokens) and strategically placed policy enforcement points within agent infrastructures. MCP tags further strengthen security by clearly marking sensitive data for targeted policy enforcement. Comprehensive auditing through centralized logging of all A2A interactions and MCP context usage ensures transparency, supports compliance, and facilitates effective oversight.
Future Directions & Enterprise Strategy
MCP and A2A are not static concepts; they represent an evolving approach to building complex AI systems.
Upcoming Developments
Formal Standardization Efforts: Expect industry groups or consortia (potentially involving major cloud providers, AI labs, and enterprise users) to propose more formal standards for both MCP and A2A, akin to OpenAPI for REST APIs or CloudEvents for eventing.
Richer Context Types (MCP): Support for multimodal context (images, audio, video), more sophisticated knowledge graph representations, and standardized ways to include agent reasoning traces within context.
Advanced A2A Capabilities: Protocols for complex negotiation, automated composition of agent services, decentralized trust and reputation systems for agents, and tighter integration with workflow orchestration engines.
AI Model Integration: MCP adapters will become more sophisticated, potentially handling automated context optimization (prompt compression, selective inclusion) based on model capabilities and cost.
Strategic Framework for Adoption
Acknowledge the Need: Recognize that ad-hoc integration is unsustainable for complex, mission-critical AI.
Invest in Standardization: Champion internal efforts to define and adopt MCP and A2A principles, even if formal external standards are still emerging. Start with core schemas and protocols relevant to your business.
Build Incrementally: Don't try to boil the ocean. Start with pilot projects, prove the value, and build out infrastructure and standards gradually.
Foster Collaboration: Encourage communication between AI/ML teams, platform engineering, security, and governance stakeholders.
Prioritize Interoperability: Design systems with the assumption that you will need to integrate agents and models from different sources (internal, vendor, open-source).
Stay Informed: Monitor developments in AI orchestration, agent frameworks (like LangChain, AutoGen, CrewAI), and emerging standardization efforts.
Future-proofing AI architectures hinge on building systems with clear abstraction and modularity, principles directly supported by MCP and A2A. By leveraging these protocols as foundational abstraction layers, enterprises can keep application logic centered on core business processes, delegating model-specific context management and agent communications entirely to MCP and A2A.
Conclusion
The era of standalone AI models solving isolated problems is giving way to a future of collaborative intelligence, where multi-agent systems tackle complex enterprise challenges. The primary obstacles to realizing this future at scale are the chaos of inconsistent context management and brittle, ad-hoc agent communication.
Model Context Protocol (MCP) and Agent-to-Agent (A2A) communication directly address these bottlenecks. MCP brings order to how AI models understand the world by standardizing context, overcoming limitations, and enabling governance. A2A brings order to how AI agents interact, enabling scalable, robust, and interoperable collaboration through standardized communication patterns.
Together, MCP and A2A form a powerful duo, providing the foundational layer for building the next generation of enterprise AI systems. They enable organizations to move beyond bespoke integrations towards truly scalable, maintainable, secure, and interoperable AI ecosystems. For enterprises serious about leveraging AI for strategic advantage, adopting and investing in MCP and A2A principles is not just a technical choice – it's a strategic imperative. The future of enterprise AI will be built on collaboration, and these protocols provide the blueprint for making that collaboration effective.
Case Study
HR Intelligent Document Processing System
Use Case: An enterprise needs to process thousands of job applications efficiently. The goal is to parse resumes, match candidates against job descriptions, evaluate qualifications, and flag top candidates for human review, integrating with the existing Applicant Tracking System (ATS).
Technical Architecture of the multi-agent system:
Document Ingestion Agent: Monitors application sources (email, web portal), retrieves new resumes/cover letters.
Document Parsing Agent: Uses OCR and layout analysis (potentially specialized models) to extract raw text and structure from various formats (PDF, DOCX, scanned images).
Information Extraction Agent: Employs an LLM or specialized NLP model to identify and extract key entities (skills, experience, education, contact info etc.)
Candidate Evaluation Agent: Compares extracted information against job description requirements, calculates match scores, and ranks candidates. Uses potentially different models or rule sets based on role complexity.
ATS Integration Agent: Formats evaluation results and updates the central Applicant Tracking System.
Human-in-the-Loop (HITL) Agent: Flags ambiguous cases or top candidates for review by HR personnel via a dedicated interface.
In the multi agent architecture above, adding standard protocols have the following effects.
MCP enables the following:
Consistent Context for Extraction: The Parsing Agent outputs text/structure in a standardized MCP format. The Information Extraction Agent receives this consistent context, regardless of the original document format or the specific parsing model used.
Handling Large Documents: For long resumes or complex cover letters exceeding LLM context limits, MCP facilitates segmentation. The Parsing Agent might break the document, and MCP provides the context linking these segments for the Extraction Agent.
Standardized Input/Output for ATS: The Evaluation Agent produces results (scores, rankings, extracted data) in an MCP-defined structure, which the ATS Integration Agent consumes, simplifying integration logic.
A2A facilitates the following:
Coordinated Workflow: A2A defines the handoffs. Ingestion Agent sends a "NEW_DOCUMENT" task via A2A message (e.g., on a Kafka topic) to the Parsing Agent pool. Parsing Agent sends "PARSE_COMPLETE" with MCP context payload to the Extraction Agent. Extraction Agent sends "EXTRACTION_COMPLETE" to the Evaluation Agent, and so on.
Clear Communication Protocols: Message types like REQUEST_PARSE, PARSE_RESULT, REQUEST_EVALUATION, EVALUATION_RESULT, UPDATE_ATS are defined within the A2A protocol, ensuring clarity. Error messages (PARSE_FAILED, EVALUATION_ERROR) follow a standard format.
Scalable Processing: Agents can be scaled independently. If parsing becomes a bottleneck, more Parsing Agents can be deployed; they pick up tasks via the A2A communication channel (e.g., message queue). A2A routing handles load balancing.
References
The following resources provide deeper technical insights and enterprise perspectives on Model Context Protocol (MCP) and Agent-to-Agent (A2A) communication.
MCP Documentation (Anthropic) – https://docs.anthropic.com/en/docs/agents-and-tools/mcp
MCP Servers (GitHub Repository) – https://github.com/modelcontextprotocol/servers
Enterprise Security for MCP (arXiv) – https://arxiv.org/abs/2504.08623
A2A Protocol Overview (a2aprotocol.ai) – https://a2aprotocol.ai
Multi-Agent Collaboration Study (arXiv) – https://arxiv.org/abs/2412.05449
The hype surrounding Generative AI and Multi-Agent Systems often clashes with the messy reality of enterprise integration. We're rapidly moving beyond standalone AI models towards sophisticated, multi-agent systems designed to tackle complex, end-to-end enterprise workflows – a transition whose complexities we explored in our previous post, "Navigating the complexities of building and scaling Multi-Agent System". Yet, as these AI ecosystems grow in sophistication and scale, agent communication and context management have emerged as critical bottlenecks, hindering the very scalability, interoperability, and maintainability they promise.
The core problem?
A lack of standardization. We see bespoke integrations, inconsistent context handling between different AI models especially LLM, and brittle, ad-hoc communication methods between AI agents. This leads to complex, difficult-to-debug systems that struggle to scale and adapt.
Agent Communication Is the New Bottleneck
As we build more sophisticated AI systems composed of multiple specialized agents (e.g., one agent for data retrieval, another for analysis, a third for summarization), the interactions between these agents become paramount.
Challenges in Multi-Agent Systems (MAS)
Ad-hoc Communication: Teams often invent their own communication methods for each project, leading to inconsistency and integration nightmares.
Agent Coupling: Agents become overly dependent on the specific implementation details of others, making changes difficult and risky.
Lack of Discoverability: Agents can't easily find other agents that could help them, limiting dynamic collaboration.
Error Handling Complexity: Failures in one agent can cascade unpredictably without standardized error reporting and recovery protocols.
Debugging Hell: Tracing a request or workflow across multiple agents with different communication styles is incredibly difficult.
How Traditional Approaches Fail at Scale
Simple point-to-point integrations or basic API calls work for a few agents but break down quickly. Imagine dozens or hundreds of agents – the number of potential interactions explodes (N*(N-1)). Without standardization, this becomes an unmanageable web of dependencies.
Increased Latency: Poorly optimized or synchronous communication chains add significant delays.
High Failure Rates: Lack of standardized error handling leads to brittle systems where one agent failure brings down entire workflows.
Integration Effort: Time spent mapping data formats and communication protocols between agents becomes a major development cost.
Monitoring Complexity: Tracking performance and health across diverse communication channels is challenging.
Enter the power duo: MCP & A2A
These complementary protocols provide the standardized foundation needed to overcome these challenges. MCP focuses on standardizing how AI models receive and understand context, while A2A defines how independent AI agents interact and collaborate.
This comprehensive guide will dissect MCP and A2A, exploring their technical underpinnings, enterprise applications, and practical implementation strategies. We will delve into technical specifications, architectural patterns, real-world case studies, and developer best practices. By the end, you'll understand why MCP and A2A together form the foundation for truly scalable, interoperable multi-agent AI systems that can transform enterprise operations.
Understanding Model Context Protocol (MCP)
Model Context Protocol (MCP) is an open-source protocol developed by Anthropic (creators of Claude) that establishes a standardized framework for LLM integrations across the industry. As an open-source initiative, MCP aims to create a universal standard that benefits the entire AI ecosystem through collaborative development and widespread adoption.
At its core, MCP provides a standardized methodology for connecting LLM applications with external data sources, knowledge bases, and tools. Essentially, it defines implementation guidelines that create a universal interface layer between AI models and the contextual information they require to function effectively.
By establishing common patterns for data access, tool execution, and prompt management, MCP eliminates the need for developers to build custom integrations for each AI model they wish to incorporate. This standardization enables developers to focus on creating flexible, powerful AI applications with seamless access to files, databases, APIs, and other essential resources—all without being locked into proprietary implementations.

Fig 1: Model Context Protocol Extensibility
Architecture
At its core, MCP acts as an abstraction layer. Instead of applications directly formatting prompts or context windows in model-specific ways, they format context according to the MCP specification. An MCP-compliant layer then translates this standardized context into the format required by the target AI model.
MCP implements a robust client-server architecture comprised of five key components that work in concert to establish secure, standardized connections between LLMs and external resources:

Fig 2: MCP Architecture Diagram
MCP Hosts
These are applications requiring contextual AI capabilities, such as conversational assistants, AI-enhanced development environments, enterprise knowledge systems, or custom business applications. Hosts integrate with MCP clients to access data and leverage external tools through the standardized protocol, allowing them to focus on delivering value rather than managing integration complexities.
MCP Clients
Clients maintain dedicated 1:1 connections with MCP Servers and handle protocol-specific operations that the server doesn't need to manage. They serve as translators, converting host application requests into standardized MCP messages, managing connection states, and handling authentication and security with servers. This abstraction layer ensures hosts can communicate with any MCP-compliant server without modification.
MCP Servers
Servers form the operational core of the MCP ecosystem, exposing critical capabilities through the standardized interface. An MCP server might provide access to document repositories, specialized databases, analytical tools, or integrate with external APIs—all through a consistent, well-defined protocol. Servers implement the business logic necessary to fulfill client requests while maintaining security boundaries.
Let’s take an example of a Github MCP Server:
Git MCP Server : For Git repositories, an MCP server transforms technical version control systems into contextually aware knowledge bases:
Intelligent code context: Rather than forcing developers to manually specify file paths, commit hashes, and diffs, a Git MCP server can respond to requests like "Find who modified the authentication logic in the last month" by understanding code semantics beyond simple text patterns.
Cross-repository knowledge: When an LLM assistant needs context across multiple repositories or branches, the MCP server handles the complex task of assembling relevant code, commit messages, pull request discussions, and documentation into a coherent context window.
Development workflow integration: Instead of having AI tools make raw API calls to Git hosting services (GitHub, GitLab, etc.), the MCP server enforces secure, standardized patterns for repository interaction, ensuring consistent behavior across different AI systems while maintaining proper authentication and access controls.
Local Data Sources
These represent secure, organization-controlled resources such as files, databases, and internal services that MCP servers can access based on fine-grained permissions. The protocol implements comprehensive security measures ensuring AI models can only access authorized resources with appropriate authentication and access controls, protecting sensitive information while enabling useful functionality.
Remote Services
These encompass external, internet-accessible services available via APIs that MCP servers can connect to as needed. They might include public knowledge bases, code repositories, specialized computational tools, or third-party business services. MCP standardizes how these external services are discovered, authenticated, and utilized within the broader AI application ecosystem.
MCP vs LLM Context Limitations
LLMs have limited context windows. MCP helps by:
Standardized Summarization: Defining protocols for context summarization techniques.
Prioritization: Allowing context elements to be tagged with priority levels, ensuring critical information isn't truncated.
Retrieval Augmented Generation (RAG) Integration: Providing a standard way to represent retrieved documents or data snippets within the context payload.
Context Segmentation: Enabling large tasks to be broken down, with MCP managing the relevant context segment for each sub-task.
Enterprise Applications of MCP
Advantages of Adopting MCP
Adopting Model Context Protocol (MCP) provides enterprises with significant strategic advantages by establishing a standardized infrastructure for managing AI contexts across diverse applications and teams. With MCP, organizations can substantially reduce redundant efforts in prompt engineering and context management, as it introduces a common, universally understood schema. This standardization allows internal development teams to focus more on core application logic and less on model-specific context handling, significantly streamlining the onboarding process for new AI models. Additionally, MCP offers considerable benefits for external partners and vendors by clearly specifying integration standards, which promotes seamless compatibility and consistent governance across third-party solutions.
Key Enterprise Considerations
When integrating MCP into enterprise environments, several critical considerations must be addressed to ensure success and security. Governance and control become central, as MCP’s structured context enables fine-grained policy enforcement, precise masking or encryption of sensitive data, and consistent audit logging. Additionally, MCP promotes scalability across multiple departments by ensuring context representations remain compatible, facilitating smoother cross-functional workflows. Vendor interoperability is significantly improved, reducing the risk of vendor lock-in by isolating model-specific changes to the MCP adapter layer rather than the core application logic. Moreover, MCP provides essential future-proofing by abstracting application logic from underlying model changes, protecting investments as AI architectures evolve. Lastly, infrastructure security and access control are paramount, necessitating robust authentication and authorization mechanisms, rigorous input validation to mitigate injection attacks, and comprehensive hardening of MCP interfaces to protect sensitive enterprise resources.
Developers Guide for MCP Implementation
Implementing Model Context Protocol effectively requires careful schema design, robust context management logic, and well-defined integration points with AI models via adapters. This guide provides practical advice for developers tasked with building or utilizing MCP within their applications.
Best Practices
Schema Design - Start Simple, Iterate:
Define clear, well-documented schemas for your core context elements (e.g., user profile, chat history, document snippet, system prompt). Use standard formats like JSON Schema or Protobuf.
Start with essential fields and add complexity gradually. Avoid trying to create a universal schema for everything at once.
Versioning is Crucial: Implement versioning for your MCP schemas from day one to manage evolution and maintain backward compatibility.
Reusability: Design schemas to be composable and reusable across different applications or agents.
Modular Context Building:
Create dedicated functions or classes responsible for generating specific types of MCP context elements.
The main application logic should orchestrate calling these builders to assemble the final MCP payload, rather than embedding complex formatting logic everywhere.
Explicit Context Window Management:
Don't rely on downstream models to implicitly handle context limits. Implement strategies within your MCP layer or context builders:
Prioritization: Use metadata tags (e.g., priority: high/medium/low) within MCP elements to guide truncation or summarization.
Summarization: Integrate summarization techniques (e.g., calling a separate summarization model, using rule-based summarization) for long context elements like chat history or documents. Store summaries as distinct MCP elements.
RAG Integration: Standardize how retrieved chunks from Retrieval Augmented Generation are represented within the MCP structure (e.g., type: retrieved_document_chunk, with source and relevance score metadata).
Configurable Strategies: Allow configuration of how context limits are handled (e.g., "truncate oldest", "summarize history", "prioritize
Thin and Pluggable Adapters:
The MCP Adapter's sole responsibility should be translating the standardized MCP payload into the specific format required by the target AI model API.
Keep business logic out of adapters.
Design adapters to be easily swappable to support different models or API versions without changing the core application or context-building logic.
Standardized Output Parsing:
Define how model responses (content, function calls, metadata) are parsed back, potentially into a standardized MCP structure (e.g., type: model_response). This facilitates logging, evaluation, and using model output as context for subsequent calls.
Handle potential errors or malformed responses from the model gracefully.
Common Pitfalls
Over-Engineering Schemas: Creating excessively complex or rigid schemas early on that are hard to adapt. Start flexible and refine based on needs.
"Leaky" Adapters: Embedding model-specific logic or assumptions outside the adapter, breaking the abstraction MCP provides.
Inefficient Context Handling: Simply concatenating all available information without intelligent prioritization, summarization, or truncation, leading to poor model performance or hitting API limits unexpectedly.
Security/Compliance as an Afterthought: Failing to tag sensitive data properly during context creation or failing to enforce policies based on those tags before model interaction.
Lack of Testing for Context Logic: Bugs in context summarization, truncation, or prioritization can be subtle and significantly impact model quality and cost.
Monolithic Context Generation: Building context in one large, complex function instead of using modular, reusable builders, making maintenance difficult.
Poor Documentation: Undocumented schemas or context element types make it hard for other developers (or future you) to understand and use the MCP implementation correctly.
By following these best practices and being mindful of potential pitfalls, developers can effectively leverage MCP to build more robust, maintainable, and interoperable LLM applications.
Understanding Agent-to-Agent (A2A) Protocol
Agent-to-Agent (A2A) Protocol defines a standardized set of rules, message formats, and interaction patterns for communication and coordination between independent software agents, particularly AI agents within a multi-agent system (MAS).
A2A makes it easy for one agent (the “client”) to send tasks to another agent (the “remote”) and get them done. The client agent creates and sends the task, while the remote agent receives it and carries it out.

Fig 3: A2A Interaction Capabilities [1]
Architectural Principles
A2A protocols often borrow principles from established distributed systems and communication paradigms like microservices, REST, gRPC, and message queuing, but tailor them for the specific needs of autonomous or semi-autonomous agents. Key principles include:
Decentralization: Agents operate independently but can discover and interact with others.
Standardization: Common message structures and interaction protocols ensure interoperability.
Asynchronicity: Often favored to handle network latency and allow agents to work concurrently, though synchronous request/reply is also supported.
Discoverability: Mechanisms for agents to find other agents based on capabilities.
Negotiation: Protocols for agents to agree on tasks, data formats, or workflow steps.
Technical Specifications
Message Formats: Typically standardized formats like JSON or Protobuf are used. Messages usually include headers (sender ID, recipient ID, message ID, timestamp, intent/type) and a payload (task description, data, query, status update). MCP-structured context can often be part of the A2A payload.
Authentication Mechanisms: Ensures agents are who they claim to be. Options include API keys, OAuth tokens (e.g., client credentials flow), mutual TLS (mTLS), or JWTs signed by a trusted authority.
Routing Protocols: Defines how messages get from sender to recipient. This could involve direct HTTP calls, message queues (like RabbitMQ, Kafka), or dedicated agent communication platforms/meshes.
Agent Discovery: Mechanisms like a central registry, gossip protocols, or service discovery tools (e.g., Consul, Etcd) allow agents to find others offering needed capabilities.
Capability Negotiation: Standardized ways for agents to advertise their skills (e.g., "summarize text," "extract entities," "query database schema X") and for requesting agents to specify requirements.
Task Delegation & Coordination: Protocols for assigning tasks, tracking progress, handling errors, and coordinating multi-step workflows (e.g., using patterns like Saga or Choreography).
A2A vs Other Protocols
vs. REST/HTTP: A2A builds upon HTTP but adds agent-specific semantics (discovery, negotiation, standardized task types). Simple REST is often too generic for complex agent coordination.
vs. gRPC: Offers performance benefits (Protobuf, HTTP/2) but requires stricter schema definitions. A2A can leverage gRPC as a transport layer.
vs. Message Queues (Kafka, RabbitMQ): Excellent for asynchronous, decoupled communication. A2A protocols define the content and meaning of messages flowing through these queues, adding a layer of application-level understanding and not only this but conversation patterns, capability discovery, and error handling specific to agent interactions.
Enterprise Applications of A2A
Advantages of Adopting A2A
The A2A protocol offers enterprises a standardized framework that significantly enhances the interoperability and scalability of AI systems. By enabling seamless communication between AI agents, regardless of their underlying technologies or vendors, A2A facilitates the creation of dynamic, multi-agent ecosystems. This interoperability allows for the integration of specialized agents across various departments, promoting a more cohesive and efficient workflow. Moreover, A2A supports dynamic capability discovery through mechanisms like “Agent Cards,” enabling agents to advertise their functionalities and discover others based on capabilities, thus fostering a collaborative environment where agents can coordinate tasks effectively.
In addition to interoperability, A2A provides robust support for long-running tasks, offering mechanisms for real-time feedback, notifications, and state updates throughout the task lifecycle. This is particularly beneficial for complex business operations that require extended processing times. The protocol’s design, built upon existing standards such as HTTP, SSE, and JSON-RPC, ensures compatibility with current IT infrastructures, simplifying integration and reducing the need for custom solutions.
Furthermore, A2A incorporates enterprise-grade security features, including authentication and authorization mechanisms, ensuring secure communication between agents. By adopting A2A, enterprises can build modular and composable AI systems, where agents can be easily swapped, updated, or added with minimal friction, significantly accelerating development and improving maintainability
Key Enterprise Considerations
Implementing the A2A protocol within an enterprise setting necessitates careful consideration of several critical factors to ensure effective deployment and operation. Security and compliance are paramount; enterprises must establish robust authentication and authorization mechanisms, such as mutual TLS (mTLS) and OAuth tokens, to safeguard agent communications. Additionally, maintaining comprehensive audit trails and enforcing fine-grained access controls are essential for meeting regulatory requirements and ensuring data integrity.
Scalability and performance are also crucial considerations. As the number of interacting agents grows, enterprises need to ensure that the underlying infrastructure can scale accordingly. This includes optimizing message routing, load balancing, and monitoring to maintain performance. Integration with existing systems is another key aspect; A2A should be thoughtfully integrated with current enterprise applications and workflows, potentially involving the development of adapters or middleware to bridge between A2A-compliant agents and legacy systems. Furthermore, implementing governance frameworks to define and enforce policies around agent interactions, data sharing, and task delegation is vital for maintaining control and oversight. Deploying comprehensive monitoring and observability tools is also essential for tracking agent interactions, diagnosing issues, and ensuring the health of the multi-agent system. Finally, enterprises should invest in training and change management strategies to facilitate adoption and minimize disruption, as well as engage with the broader A2A community.
Developers Guide for A2A Implementation
Implementing robust A2A communication requires careful planning and adherence to best practices.
Best Practices
Choose the Right Transport:
Synchronous (Request/Reply): Use HTTP/S (REST-like) or gRPC for immediate responses when needed. Good for simple queries or commands.
Asynchronous (Fire & Forget/Events): Use Message Queues (RabbitMQ, Kafka, NATS, Pulsar) for decoupling, resilience, and workflows where immediate response isn't critical. Better for scalability and handling long-running tasks.
Define Clear Message Schemas: Use JSON Schema, Protobuf, or Avro to strictly define A2A message structures (headers and payload). Include versioning in your schemas.
Standardize Message Headers: Always include essential headers: unique message ID, correlation ID (to track workflows), sender/recipient IDs, timestamp, message type/intent.
Idempotency: Design message handlers to be idempotent where possible, especially with asynchronous systems. Processing the same message twice should not cause unintended side effects. Use the message ID for deduplication.
Error Handling & Resilience:
Define standard error message formats.
Implement retry mechanisms (e.g., exponential backoff) for transient network issues.
Use Dead Letter Queues (DLQs) in message brokers to handle messages that consistently fail processing.
Implement circuit breaker patterns to prevent cascading failures.
Agent Discovery: Use a service registry or a dedicated agent directory for dynamic discovery. Avoid hardcoding agent addresses.
Security First: Implement strong authentication and authorization from the start. Use standard protocols (OAuth 2.0, mTLS, JWT). Encrypt traffic (TLS).
Monitoring and Observability:
Logging: Log key information for each message sent/received (headers, size, timing). Use structured logging.
Tracing: Implement distributed tracing (e.g., OpenTelemetry) using the correlation ID to track requests across multiple agents.
Metrics: Monitor queue depths, message processing times, error rates, agent availability.
Common Pitfalls
Overly Chatty Communication: Designing agents that require excessive back-and-forth increases latency and complexity. Aim for well-defined, substantive interactions.
Ignoring Versioning: Failing to version message schemas leads to compatibility issues as agents evolve.
Lack of State Management: Assuming agents are stateless when workflows require state can lead to errors. Consider external state stores or stateful agent patterns if needed.
Inadequate Monitoring: Flying blind without proper logging, tracing, and metrics makes debugging and optimization impossible.
Security as an Afterthought: Adding security late in the process is much harder and riskier.
Performance Optimization
Asynchronous Communication: Prefer message queues for decoupling and parallelism.
Batching: Send/process messages in batches where appropriate (e.g., batch updates to a database).
Efficient Serialization: Use binary formats like Protobuf or MessagePack instead of JSON for performance-critical paths.
Caching: Cache frequently accessed data or results within agents (with appropriate invalidation).
Optimize Transport: Tune message broker configurations, use efficient network protocols (gRPC).
Enterprise Integration: Making MCP and A2A Work Together
MCP and A2A are highly synergistic. MCP standardizes the content (context) an agent needs or produces, while A2A standardizes the interaction (communication) between agents.
Integration
A common integration strategy between MCP and A2A involves embedding MCP-formatted context within the payload of A2A messages. In practice, an agent initiating a task via A2A includes the necessary input data structured according to MCP standards. The receiving agent completes the requested operation and returns its results, often formatted using MCP as well, ensuring consistent and standardized context communication. Additionally, enterprises can deploy a dedicated “Context Orchestration Agent” that leverages A2A protocols to coordinate interactions with various specialized data source agents, such as those interfacing with databases or document repositories. This orchestration agent assembles the collected data into a structured MCP payload, which can then be provided seamlessly to processing agents. Furthermore, A2A’s capability negotiation mechanism benefits significantly from MCP, as agents can clearly specify the MCP schemas they support for both input and output, streamlining discovery and collaboration processes across diverse multi-agent workflows.
Architectural Patterns for Deployment
For enterprise deployments of multi-agent systems, several architectural patterns are particularly effective. One common approach is the Agent Mesh or Fabric, which resembles traditional service meshes like Istio or Linkerd but is specifically optimized for agent-based communication. This model provides a dedicated infrastructure layer that manages A2A routing, agent discovery, security through mutual TLS (mTLS), observability, and policy enforcement, with agent communication handled through mesh sidecars or proxies. Alternatively, a Central Message Bus approach employs enterprise-grade message queues such as Kafka, Pulsar, or Solace to facilitate agent interactions via publish-subscribe patterns. Here, governance and access control are managed directly at the broker or topic level, enabling scalable and decoupled agent communication. Finally, the Agent Gateway architecture adapts the API Gateway pattern for agent interactions, where external requests and internal agents interact with a central gateway responsible for routing A2A messages to appropriate backend agents, enforcing security measures, and potentially translating between different communication protocols, thereby simplifying and standardizing access to agent services.
Technical Considerations
Successfully implementing MCP and A2A in an enterprise context involves careful attention to several critical technical considerations. Authentication and authorization require deliberate decisions around centralized versus decentralized enforcement mechanisms, robust token formats such as JWTs or OAuth, and secure management of keys and secrets to safeguard agent communications. Equally important is establishing comprehensive monitoring and observability practices, including standardized logging formats, consistent trace propagation across agent interactions, and clear definition of KPIs to measure and optimize agent effectiveness. Scalability and load balancing strategies will vary depending on the chosen A2A transport, such as employing Kubernetes Horizontal Pod Autoscalers for agent pods, utilizing Kafka consumer groups for message-driven workflows, or leveraging built-in load-balancing capabilities of gRPC. Additionally, robust error handling and recovery processes must be implemented, potentially employing distributed patterns like Saga or choreography for complex workflows, complemented by well-defined retry logic and effective use of Dead Letter Queues (DLQs) to handle persistent failures gracefully.
Complementing these technical foundations, security and governance require implementing centralized identity management (e.g., via an Identity Provider for issuing JWT or OAuth tokens) and strategically placed policy enforcement points within agent infrastructures. MCP tags further strengthen security by clearly marking sensitive data for targeted policy enforcement. Comprehensive auditing through centralized logging of all A2A interactions and MCP context usage ensures transparency, supports compliance, and facilitates effective oversight.
Future Directions & Enterprise Strategy
MCP and A2A are not static concepts; they represent an evolving approach to building complex AI systems.
Upcoming Developments
Formal Standardization Efforts: Expect industry groups or consortia (potentially involving major cloud providers, AI labs, and enterprise users) to propose more formal standards for both MCP and A2A, akin to OpenAPI for REST APIs or CloudEvents for eventing.
Richer Context Types (MCP): Support for multimodal context (images, audio, video), more sophisticated knowledge graph representations, and standardized ways to include agent reasoning traces within context.
Advanced A2A Capabilities: Protocols for complex negotiation, automated composition of agent services, decentralized trust and reputation systems for agents, and tighter integration with workflow orchestration engines.
AI Model Integration: MCP adapters will become more sophisticated, potentially handling automated context optimization (prompt compression, selective inclusion) based on model capabilities and cost.
Strategic Framework for Adoption
Acknowledge the Need: Recognize that ad-hoc integration is unsustainable for complex, mission-critical AI.
Invest in Standardization: Champion internal efforts to define and adopt MCP and A2A principles, even if formal external standards are still emerging. Start with core schemas and protocols relevant to your business.
Build Incrementally: Don't try to boil the ocean. Start with pilot projects, prove the value, and build out infrastructure and standards gradually.
Foster Collaboration: Encourage communication between AI/ML teams, platform engineering, security, and governance stakeholders.
Prioritize Interoperability: Design systems with the assumption that you will need to integrate agents and models from different sources (internal, vendor, open-source).
Stay Informed: Monitor developments in AI orchestration, agent frameworks (like LangChain, AutoGen, CrewAI), and emerging standardization efforts.
Future-proofing AI architectures hinge on building systems with clear abstraction and modularity, principles directly supported by MCP and A2A. By leveraging these protocols as foundational abstraction layers, enterprises can keep application logic centered on core business processes, delegating model-specific context management and agent communications entirely to MCP and A2A.
Conclusion
The era of standalone AI models solving isolated problems is giving way to a future of collaborative intelligence, where multi-agent systems tackle complex enterprise challenges. The primary obstacles to realizing this future at scale are the chaos of inconsistent context management and brittle, ad-hoc agent communication.
Model Context Protocol (MCP) and Agent-to-Agent (A2A) communication directly address these bottlenecks. MCP brings order to how AI models understand the world by standardizing context, overcoming limitations, and enabling governance. A2A brings order to how AI agents interact, enabling scalable, robust, and interoperable collaboration through standardized communication patterns.
Together, MCP and A2A form a powerful duo, providing the foundational layer for building the next generation of enterprise AI systems. They enable organizations to move beyond bespoke integrations towards truly scalable, maintainable, secure, and interoperable AI ecosystems. For enterprises serious about leveraging AI for strategic advantage, adopting and investing in MCP and A2A principles is not just a technical choice – it's a strategic imperative. The future of enterprise AI will be built on collaboration, and these protocols provide the blueprint for making that collaboration effective.
Case Study
HR Intelligent Document Processing System
Use Case: An enterprise needs to process thousands of job applications efficiently. The goal is to parse resumes, match candidates against job descriptions, evaluate qualifications, and flag top candidates for human review, integrating with the existing Applicant Tracking System (ATS).
Technical Architecture of the multi-agent system:
Document Ingestion Agent: Monitors application sources (email, web portal), retrieves new resumes/cover letters.
Document Parsing Agent: Uses OCR and layout analysis (potentially specialized models) to extract raw text and structure from various formats (PDF, DOCX, scanned images).
Information Extraction Agent: Employs an LLM or specialized NLP model to identify and extract key entities (skills, experience, education, contact info etc.)
Candidate Evaluation Agent: Compares extracted information against job description requirements, calculates match scores, and ranks candidates. Uses potentially different models or rule sets based on role complexity.
ATS Integration Agent: Formats evaluation results and updates the central Applicant Tracking System.
Human-in-the-Loop (HITL) Agent: Flags ambiguous cases or top candidates for review by HR personnel via a dedicated interface.
In the multi agent architecture above, adding standard protocols have the following effects.
MCP enables the following:
Consistent Context for Extraction: The Parsing Agent outputs text/structure in a standardized MCP format. The Information Extraction Agent receives this consistent context, regardless of the original document format or the specific parsing model used.
Handling Large Documents: For long resumes or complex cover letters exceeding LLM context limits, MCP facilitates segmentation. The Parsing Agent might break the document, and MCP provides the context linking these segments for the Extraction Agent.
Standardized Input/Output for ATS: The Evaluation Agent produces results (scores, rankings, extracted data) in an MCP-defined structure, which the ATS Integration Agent consumes, simplifying integration logic.
A2A facilitates the following:
Coordinated Workflow: A2A defines the handoffs. Ingestion Agent sends a "NEW_DOCUMENT" task via A2A message (e.g., on a Kafka topic) to the Parsing Agent pool. Parsing Agent sends "PARSE_COMPLETE" with MCP context payload to the Extraction Agent. Extraction Agent sends "EXTRACTION_COMPLETE" to the Evaluation Agent, and so on.
Clear Communication Protocols: Message types like REQUEST_PARSE, PARSE_RESULT, REQUEST_EVALUATION, EVALUATION_RESULT, UPDATE_ATS are defined within the A2A protocol, ensuring clarity. Error messages (PARSE_FAILED, EVALUATION_ERROR) follow a standard format.
Scalable Processing: Agents can be scaled independently. If parsing becomes a bottleneck, more Parsing Agents can be deployed; they pick up tasks via the A2A communication channel (e.g., message queue). A2A routing handles load balancing.
References
The following resources provide deeper technical insights and enterprise perspectives on Model Context Protocol (MCP) and Agent-to-Agent (A2A) communication.
MCP Documentation (Anthropic) – https://docs.anthropic.com/en/docs/agents-and-tools/mcp
MCP Servers (GitHub Repository) – https://github.com/modelcontextprotocol/servers
Enterprise Security for MCP (arXiv) – https://arxiv.org/abs/2504.08623
A2A Protocol Overview (a2aprotocol.ai) – https://a2aprotocol.ai
Multi-Agent Collaboration Study (arXiv) – https://arxiv.org/abs/2412.05449
The hype surrounding Generative AI and Multi-Agent Systems often clashes with the messy reality of enterprise integration. We're rapidly moving beyond standalone AI models towards sophisticated, multi-agent systems designed to tackle complex, end-to-end enterprise workflows – a transition whose complexities we explored in our previous post, "Navigating the complexities of building and scaling Multi-Agent System". Yet, as these AI ecosystems grow in sophistication and scale, agent communication and context management have emerged as critical bottlenecks, hindering the very scalability, interoperability, and maintainability they promise.
The core problem?
A lack of standardization. We see bespoke integrations, inconsistent context handling between different AI models especially LLM, and brittle, ad-hoc communication methods between AI agents. This leads to complex, difficult-to-debug systems that struggle to scale and adapt.
Agent Communication Is the New Bottleneck
As we build more sophisticated AI systems composed of multiple specialized agents (e.g., one agent for data retrieval, another for analysis, a third for summarization), the interactions between these agents become paramount.
Challenges in Multi-Agent Systems (MAS)
Ad-hoc Communication: Teams often invent their own communication methods for each project, leading to inconsistency and integration nightmares.
Agent Coupling: Agents become overly dependent on the specific implementation details of others, making changes difficult and risky.
Lack of Discoverability: Agents can't easily find other agents that could help them, limiting dynamic collaboration.
Error Handling Complexity: Failures in one agent can cascade unpredictably without standardized error reporting and recovery protocols.
Debugging Hell: Tracing a request or workflow across multiple agents with different communication styles is incredibly difficult.
How Traditional Approaches Fail at Scale
Simple point-to-point integrations or basic API calls work for a few agents but break down quickly. Imagine dozens or hundreds of agents – the number of potential interactions explodes (N*(N-1)). Without standardization, this becomes an unmanageable web of dependencies.
Increased Latency: Poorly optimized or synchronous communication chains add significant delays.
High Failure Rates: Lack of standardized error handling leads to brittle systems where one agent failure brings down entire workflows.
Integration Effort: Time spent mapping data formats and communication protocols between agents becomes a major development cost.
Monitoring Complexity: Tracking performance and health across diverse communication channels is challenging.
Enter the power duo: MCP & A2A
These complementary protocols provide the standardized foundation needed to overcome these challenges. MCP focuses on standardizing how AI models receive and understand context, while A2A defines how independent AI agents interact and collaborate.
This comprehensive guide will dissect MCP and A2A, exploring their technical underpinnings, enterprise applications, and practical implementation strategies. We will delve into technical specifications, architectural patterns, real-world case studies, and developer best practices. By the end, you'll understand why MCP and A2A together form the foundation for truly scalable, interoperable multi-agent AI systems that can transform enterprise operations.
Understanding Model Context Protocol (MCP)
Model Context Protocol (MCP) is an open-source protocol developed by Anthropic (creators of Claude) that establishes a standardized framework for LLM integrations across the industry. As an open-source initiative, MCP aims to create a universal standard that benefits the entire AI ecosystem through collaborative development and widespread adoption.
At its core, MCP provides a standardized methodology for connecting LLM applications with external data sources, knowledge bases, and tools. Essentially, it defines implementation guidelines that create a universal interface layer between AI models and the contextual information they require to function effectively.
By establishing common patterns for data access, tool execution, and prompt management, MCP eliminates the need for developers to build custom integrations for each AI model they wish to incorporate. This standardization enables developers to focus on creating flexible, powerful AI applications with seamless access to files, databases, APIs, and other essential resources—all without being locked into proprietary implementations.

Fig 1: Model Context Protocol Extensibility
Architecture
At its core, MCP acts as an abstraction layer. Instead of applications directly formatting prompts or context windows in model-specific ways, they format context according to the MCP specification. An MCP-compliant layer then translates this standardized context into the format required by the target AI model.
MCP implements a robust client-server architecture comprised of five key components that work in concert to establish secure, standardized connections between LLMs and external resources:

Fig 2: MCP Architecture Diagram
MCP Hosts
These are applications requiring contextual AI capabilities, such as conversational assistants, AI-enhanced development environments, enterprise knowledge systems, or custom business applications. Hosts integrate with MCP clients to access data and leverage external tools through the standardized protocol, allowing them to focus on delivering value rather than managing integration complexities.
MCP Clients
Clients maintain dedicated 1:1 connections with MCP Servers and handle protocol-specific operations that the server doesn't need to manage. They serve as translators, converting host application requests into standardized MCP messages, managing connection states, and handling authentication and security with servers. This abstraction layer ensures hosts can communicate with any MCP-compliant server without modification.
MCP Servers
Servers form the operational core of the MCP ecosystem, exposing critical capabilities through the standardized interface. An MCP server might provide access to document repositories, specialized databases, analytical tools, or integrate with external APIs—all through a consistent, well-defined protocol. Servers implement the business logic necessary to fulfill client requests while maintaining security boundaries.
Let’s take an example of a Github MCP Server:
Git MCP Server : For Git repositories, an MCP server transforms technical version control systems into contextually aware knowledge bases:
Intelligent code context: Rather than forcing developers to manually specify file paths, commit hashes, and diffs, a Git MCP server can respond to requests like "Find who modified the authentication logic in the last month" by understanding code semantics beyond simple text patterns.
Cross-repository knowledge: When an LLM assistant needs context across multiple repositories or branches, the MCP server handles the complex task of assembling relevant code, commit messages, pull request discussions, and documentation into a coherent context window.
Development workflow integration: Instead of having AI tools make raw API calls to Git hosting services (GitHub, GitLab, etc.), the MCP server enforces secure, standardized patterns for repository interaction, ensuring consistent behavior across different AI systems while maintaining proper authentication and access controls.
Local Data Sources
These represent secure, organization-controlled resources such as files, databases, and internal services that MCP servers can access based on fine-grained permissions. The protocol implements comprehensive security measures ensuring AI models can only access authorized resources with appropriate authentication and access controls, protecting sensitive information while enabling useful functionality.
Remote Services
These encompass external, internet-accessible services available via APIs that MCP servers can connect to as needed. They might include public knowledge bases, code repositories, specialized computational tools, or third-party business services. MCP standardizes how these external services are discovered, authenticated, and utilized within the broader AI application ecosystem.
MCP vs LLM Context Limitations
LLMs have limited context windows. MCP helps by:
Standardized Summarization: Defining protocols for context summarization techniques.
Prioritization: Allowing context elements to be tagged with priority levels, ensuring critical information isn't truncated.
Retrieval Augmented Generation (RAG) Integration: Providing a standard way to represent retrieved documents or data snippets within the context payload.
Context Segmentation: Enabling large tasks to be broken down, with MCP managing the relevant context segment for each sub-task.
Enterprise Applications of MCP
Advantages of Adopting MCP
Adopting Model Context Protocol (MCP) provides enterprises with significant strategic advantages by establishing a standardized infrastructure for managing AI contexts across diverse applications and teams. With MCP, organizations can substantially reduce redundant efforts in prompt engineering and context management, as it introduces a common, universally understood schema. This standardization allows internal development teams to focus more on core application logic and less on model-specific context handling, significantly streamlining the onboarding process for new AI models. Additionally, MCP offers considerable benefits for external partners and vendors by clearly specifying integration standards, which promotes seamless compatibility and consistent governance across third-party solutions.
Key Enterprise Considerations
When integrating MCP into enterprise environments, several critical considerations must be addressed to ensure success and security. Governance and control become central, as MCP’s structured context enables fine-grained policy enforcement, precise masking or encryption of sensitive data, and consistent audit logging. Additionally, MCP promotes scalability across multiple departments by ensuring context representations remain compatible, facilitating smoother cross-functional workflows. Vendor interoperability is significantly improved, reducing the risk of vendor lock-in by isolating model-specific changes to the MCP adapter layer rather than the core application logic. Moreover, MCP provides essential future-proofing by abstracting application logic from underlying model changes, protecting investments as AI architectures evolve. Lastly, infrastructure security and access control are paramount, necessitating robust authentication and authorization mechanisms, rigorous input validation to mitigate injection attacks, and comprehensive hardening of MCP interfaces to protect sensitive enterprise resources.
Developers Guide for MCP Implementation
Implementing Model Context Protocol effectively requires careful schema design, robust context management logic, and well-defined integration points with AI models via adapters. This guide provides practical advice for developers tasked with building or utilizing MCP within their applications.
Best Practices
Schema Design - Start Simple, Iterate:
Define clear, well-documented schemas for your core context elements (e.g., user profile, chat history, document snippet, system prompt). Use standard formats like JSON Schema or Protobuf.
Start with essential fields and add complexity gradually. Avoid trying to create a universal schema for everything at once.
Versioning is Crucial: Implement versioning for your MCP schemas from day one to manage evolution and maintain backward compatibility.
Reusability: Design schemas to be composable and reusable across different applications or agents.
Modular Context Building:
Create dedicated functions or classes responsible for generating specific types of MCP context elements.
The main application logic should orchestrate calling these builders to assemble the final MCP payload, rather than embedding complex formatting logic everywhere.
Explicit Context Window Management:
Don't rely on downstream models to implicitly handle context limits. Implement strategies within your MCP layer or context builders:
Prioritization: Use metadata tags (e.g., priority: high/medium/low) within MCP elements to guide truncation or summarization.
Summarization: Integrate summarization techniques (e.g., calling a separate summarization model, using rule-based summarization) for long context elements like chat history or documents. Store summaries as distinct MCP elements.
RAG Integration: Standardize how retrieved chunks from Retrieval Augmented Generation are represented within the MCP structure (e.g., type: retrieved_document_chunk, with source and relevance score metadata).
Configurable Strategies: Allow configuration of how context limits are handled (e.g., "truncate oldest", "summarize history", "prioritize
Thin and Pluggable Adapters:
The MCP Adapter's sole responsibility should be translating the standardized MCP payload into the specific format required by the target AI model API.
Keep business logic out of adapters.
Design adapters to be easily swappable to support different models or API versions without changing the core application or context-building logic.
Standardized Output Parsing:
Define how model responses (content, function calls, metadata) are parsed back, potentially into a standardized MCP structure (e.g., type: model_response). This facilitates logging, evaluation, and using model output as context for subsequent calls.
Handle potential errors or malformed responses from the model gracefully.
Common Pitfalls
Over-Engineering Schemas: Creating excessively complex or rigid schemas early on that are hard to adapt. Start flexible and refine based on needs.
"Leaky" Adapters: Embedding model-specific logic or assumptions outside the adapter, breaking the abstraction MCP provides.
Inefficient Context Handling: Simply concatenating all available information without intelligent prioritization, summarization, or truncation, leading to poor model performance or hitting API limits unexpectedly.
Security/Compliance as an Afterthought: Failing to tag sensitive data properly during context creation or failing to enforce policies based on those tags before model interaction.
Lack of Testing for Context Logic: Bugs in context summarization, truncation, or prioritization can be subtle and significantly impact model quality and cost.
Monolithic Context Generation: Building context in one large, complex function instead of using modular, reusable builders, making maintenance difficult.
Poor Documentation: Undocumented schemas or context element types make it hard for other developers (or future you) to understand and use the MCP implementation correctly.
By following these best practices and being mindful of potential pitfalls, developers can effectively leverage MCP to build more robust, maintainable, and interoperable LLM applications.
Understanding Agent-to-Agent (A2A) Protocol
Agent-to-Agent (A2A) Protocol defines a standardized set of rules, message formats, and interaction patterns for communication and coordination between independent software agents, particularly AI agents within a multi-agent system (MAS).
A2A makes it easy for one agent (the “client”) to send tasks to another agent (the “remote”) and get them done. The client agent creates and sends the task, while the remote agent receives it and carries it out.

Fig 3: A2A Interaction Capabilities [1]
Architectural Principles
A2A protocols often borrow principles from established distributed systems and communication paradigms like microservices, REST, gRPC, and message queuing, but tailor them for the specific needs of autonomous or semi-autonomous agents. Key principles include:
Decentralization: Agents operate independently but can discover and interact with others.
Standardization: Common message structures and interaction protocols ensure interoperability.
Asynchronicity: Often favored to handle network latency and allow agents to work concurrently, though synchronous request/reply is also supported.
Discoverability: Mechanisms for agents to find other agents based on capabilities.
Negotiation: Protocols for agents to agree on tasks, data formats, or workflow steps.
Technical Specifications
Message Formats: Typically standardized formats like JSON or Protobuf are used. Messages usually include headers (sender ID, recipient ID, message ID, timestamp, intent/type) and a payload (task description, data, query, status update). MCP-structured context can often be part of the A2A payload.
Authentication Mechanisms: Ensures agents are who they claim to be. Options include API keys, OAuth tokens (e.g., client credentials flow), mutual TLS (mTLS), or JWTs signed by a trusted authority.
Routing Protocols: Defines how messages get from sender to recipient. This could involve direct HTTP calls, message queues (like RabbitMQ, Kafka), or dedicated agent communication platforms/meshes.
Agent Discovery: Mechanisms like a central registry, gossip protocols, or service discovery tools (e.g., Consul, Etcd) allow agents to find others offering needed capabilities.
Capability Negotiation: Standardized ways for agents to advertise their skills (e.g., "summarize text," "extract entities," "query database schema X") and for requesting agents to specify requirements.
Task Delegation & Coordination: Protocols for assigning tasks, tracking progress, handling errors, and coordinating multi-step workflows (e.g., using patterns like Saga or Choreography).
A2A vs Other Protocols
vs. REST/HTTP: A2A builds upon HTTP but adds agent-specific semantics (discovery, negotiation, standardized task types). Simple REST is often too generic for complex agent coordination.
vs. gRPC: Offers performance benefits (Protobuf, HTTP/2) but requires stricter schema definitions. A2A can leverage gRPC as a transport layer.
vs. Message Queues (Kafka, RabbitMQ): Excellent for asynchronous, decoupled communication. A2A protocols define the content and meaning of messages flowing through these queues, adding a layer of application-level understanding and not only this but conversation patterns, capability discovery, and error handling specific to agent interactions.
Enterprise Applications of A2A
Advantages of Adopting A2A
The A2A protocol offers enterprises a standardized framework that significantly enhances the interoperability and scalability of AI systems. By enabling seamless communication between AI agents, regardless of their underlying technologies or vendors, A2A facilitates the creation of dynamic, multi-agent ecosystems. This interoperability allows for the integration of specialized agents across various departments, promoting a more cohesive and efficient workflow. Moreover, A2A supports dynamic capability discovery through mechanisms like “Agent Cards,” enabling agents to advertise their functionalities and discover others based on capabilities, thus fostering a collaborative environment where agents can coordinate tasks effectively.
In addition to interoperability, A2A provides robust support for long-running tasks, offering mechanisms for real-time feedback, notifications, and state updates throughout the task lifecycle. This is particularly beneficial for complex business operations that require extended processing times. The protocol’s design, built upon existing standards such as HTTP, SSE, and JSON-RPC, ensures compatibility with current IT infrastructures, simplifying integration and reducing the need for custom solutions.
Furthermore, A2A incorporates enterprise-grade security features, including authentication and authorization mechanisms, ensuring secure communication between agents. By adopting A2A, enterprises can build modular and composable AI systems, where agents can be easily swapped, updated, or added with minimal friction, significantly accelerating development and improving maintainability
Key Enterprise Considerations
Implementing the A2A protocol within an enterprise setting necessitates careful consideration of several critical factors to ensure effective deployment and operation. Security and compliance are paramount; enterprises must establish robust authentication and authorization mechanisms, such as mutual TLS (mTLS) and OAuth tokens, to safeguard agent communications. Additionally, maintaining comprehensive audit trails and enforcing fine-grained access controls are essential for meeting regulatory requirements and ensuring data integrity.
Scalability and performance are also crucial considerations. As the number of interacting agents grows, enterprises need to ensure that the underlying infrastructure can scale accordingly. This includes optimizing message routing, load balancing, and monitoring to maintain performance. Integration with existing systems is another key aspect; A2A should be thoughtfully integrated with current enterprise applications and workflows, potentially involving the development of adapters or middleware to bridge between A2A-compliant agents and legacy systems. Furthermore, implementing governance frameworks to define and enforce policies around agent interactions, data sharing, and task delegation is vital for maintaining control and oversight. Deploying comprehensive monitoring and observability tools is also essential for tracking agent interactions, diagnosing issues, and ensuring the health of the multi-agent system. Finally, enterprises should invest in training and change management strategies to facilitate adoption and minimize disruption, as well as engage with the broader A2A community.
Developers Guide for A2A Implementation
Implementing robust A2A communication requires careful planning and adherence to best practices.
Best Practices
Choose the Right Transport:
Synchronous (Request/Reply): Use HTTP/S (REST-like) or gRPC for immediate responses when needed. Good for simple queries or commands.
Asynchronous (Fire & Forget/Events): Use Message Queues (RabbitMQ, Kafka, NATS, Pulsar) for decoupling, resilience, and workflows where immediate response isn't critical. Better for scalability and handling long-running tasks.
Define Clear Message Schemas: Use JSON Schema, Protobuf, or Avro to strictly define A2A message structures (headers and payload). Include versioning in your schemas.
Standardize Message Headers: Always include essential headers: unique message ID, correlation ID (to track workflows), sender/recipient IDs, timestamp, message type/intent.
Idempotency: Design message handlers to be idempotent where possible, especially with asynchronous systems. Processing the same message twice should not cause unintended side effects. Use the message ID for deduplication.
Error Handling & Resilience:
Define standard error message formats.
Implement retry mechanisms (e.g., exponential backoff) for transient network issues.
Use Dead Letter Queues (DLQs) in message brokers to handle messages that consistently fail processing.
Implement circuit breaker patterns to prevent cascading failures.
Agent Discovery: Use a service registry or a dedicated agent directory for dynamic discovery. Avoid hardcoding agent addresses.
Security First: Implement strong authentication and authorization from the start. Use standard protocols (OAuth 2.0, mTLS, JWT). Encrypt traffic (TLS).
Monitoring and Observability:
Logging: Log key information for each message sent/received (headers, size, timing). Use structured logging.
Tracing: Implement distributed tracing (e.g., OpenTelemetry) using the correlation ID to track requests across multiple agents.
Metrics: Monitor queue depths, message processing times, error rates, agent availability.
Common Pitfalls
Overly Chatty Communication: Designing agents that require excessive back-and-forth increases latency and complexity. Aim for well-defined, substantive interactions.
Ignoring Versioning: Failing to version message schemas leads to compatibility issues as agents evolve.
Lack of State Management: Assuming agents are stateless when workflows require state can lead to errors. Consider external state stores or stateful agent patterns if needed.
Inadequate Monitoring: Flying blind without proper logging, tracing, and metrics makes debugging and optimization impossible.
Security as an Afterthought: Adding security late in the process is much harder and riskier.
Performance Optimization
Asynchronous Communication: Prefer message queues for decoupling and parallelism.
Batching: Send/process messages in batches where appropriate (e.g., batch updates to a database).
Efficient Serialization: Use binary formats like Protobuf or MessagePack instead of JSON for performance-critical paths.
Caching: Cache frequently accessed data or results within agents (with appropriate invalidation).
Optimize Transport: Tune message broker configurations, use efficient network protocols (gRPC).
Enterprise Integration: Making MCP and A2A Work Together
MCP and A2A are highly synergistic. MCP standardizes the content (context) an agent needs or produces, while A2A standardizes the interaction (communication) between agents.
Integration
A common integration strategy between MCP and A2A involves embedding MCP-formatted context within the payload of A2A messages. In practice, an agent initiating a task via A2A includes the necessary input data structured according to MCP standards. The receiving agent completes the requested operation and returns its results, often formatted using MCP as well, ensuring consistent and standardized context communication. Additionally, enterprises can deploy a dedicated “Context Orchestration Agent” that leverages A2A protocols to coordinate interactions with various specialized data source agents, such as those interfacing with databases or document repositories. This orchestration agent assembles the collected data into a structured MCP payload, which can then be provided seamlessly to processing agents. Furthermore, A2A’s capability negotiation mechanism benefits significantly from MCP, as agents can clearly specify the MCP schemas they support for both input and output, streamlining discovery and collaboration processes across diverse multi-agent workflows.
Architectural Patterns for Deployment
For enterprise deployments of multi-agent systems, several architectural patterns are particularly effective. One common approach is the Agent Mesh or Fabric, which resembles traditional service meshes like Istio or Linkerd but is specifically optimized for agent-based communication. This model provides a dedicated infrastructure layer that manages A2A routing, agent discovery, security through mutual TLS (mTLS), observability, and policy enforcement, with agent communication handled through mesh sidecars or proxies. Alternatively, a Central Message Bus approach employs enterprise-grade message queues such as Kafka, Pulsar, or Solace to facilitate agent interactions via publish-subscribe patterns. Here, governance and access control are managed directly at the broker or topic level, enabling scalable and decoupled agent communication. Finally, the Agent Gateway architecture adapts the API Gateway pattern for agent interactions, where external requests and internal agents interact with a central gateway responsible for routing A2A messages to appropriate backend agents, enforcing security measures, and potentially translating between different communication protocols, thereby simplifying and standardizing access to agent services.
Technical Considerations
Successfully implementing MCP and A2A in an enterprise context involves careful attention to several critical technical considerations. Authentication and authorization require deliberate decisions around centralized versus decentralized enforcement mechanisms, robust token formats such as JWTs or OAuth, and secure management of keys and secrets to safeguard agent communications. Equally important is establishing comprehensive monitoring and observability practices, including standardized logging formats, consistent trace propagation across agent interactions, and clear definition of KPIs to measure and optimize agent effectiveness. Scalability and load balancing strategies will vary depending on the chosen A2A transport, such as employing Kubernetes Horizontal Pod Autoscalers for agent pods, utilizing Kafka consumer groups for message-driven workflows, or leveraging built-in load-balancing capabilities of gRPC. Additionally, robust error handling and recovery processes must be implemented, potentially employing distributed patterns like Saga or choreography for complex workflows, complemented by well-defined retry logic and effective use of Dead Letter Queues (DLQs) to handle persistent failures gracefully.
Complementing these technical foundations, security and governance require implementing centralized identity management (e.g., via an Identity Provider for issuing JWT or OAuth tokens) and strategically placed policy enforcement points within agent infrastructures. MCP tags further strengthen security by clearly marking sensitive data for targeted policy enforcement. Comprehensive auditing through centralized logging of all A2A interactions and MCP context usage ensures transparency, supports compliance, and facilitates effective oversight.
Future Directions & Enterprise Strategy
MCP and A2A are not static concepts; they represent an evolving approach to building complex AI systems.
Upcoming Developments
Formal Standardization Efforts: Expect industry groups or consortia (potentially involving major cloud providers, AI labs, and enterprise users) to propose more formal standards for both MCP and A2A, akin to OpenAPI for REST APIs or CloudEvents for eventing.
Richer Context Types (MCP): Support for multimodal context (images, audio, video), more sophisticated knowledge graph representations, and standardized ways to include agent reasoning traces within context.
Advanced A2A Capabilities: Protocols for complex negotiation, automated composition of agent services, decentralized trust and reputation systems for agents, and tighter integration with workflow orchestration engines.
AI Model Integration: MCP adapters will become more sophisticated, potentially handling automated context optimization (prompt compression, selective inclusion) based on model capabilities and cost.
Strategic Framework for Adoption
Acknowledge the Need: Recognize that ad-hoc integration is unsustainable for complex, mission-critical AI.
Invest in Standardization: Champion internal efforts to define and adopt MCP and A2A principles, even if formal external standards are still emerging. Start with core schemas and protocols relevant to your business.
Build Incrementally: Don't try to boil the ocean. Start with pilot projects, prove the value, and build out infrastructure and standards gradually.
Foster Collaboration: Encourage communication between AI/ML teams, platform engineering, security, and governance stakeholders.
Prioritize Interoperability: Design systems with the assumption that you will need to integrate agents and models from different sources (internal, vendor, open-source).
Stay Informed: Monitor developments in AI orchestration, agent frameworks (like LangChain, AutoGen, CrewAI), and emerging standardization efforts.
Future-proofing AI architectures hinge on building systems with clear abstraction and modularity, principles directly supported by MCP and A2A. By leveraging these protocols as foundational abstraction layers, enterprises can keep application logic centered on core business processes, delegating model-specific context management and agent communications entirely to MCP and A2A.
Conclusion
The era of standalone AI models solving isolated problems is giving way to a future of collaborative intelligence, where multi-agent systems tackle complex enterprise challenges. The primary obstacles to realizing this future at scale are the chaos of inconsistent context management and brittle, ad-hoc agent communication.
Model Context Protocol (MCP) and Agent-to-Agent (A2A) communication directly address these bottlenecks. MCP brings order to how AI models understand the world by standardizing context, overcoming limitations, and enabling governance. A2A brings order to how AI agents interact, enabling scalable, robust, and interoperable collaboration through standardized communication patterns.
Together, MCP and A2A form a powerful duo, providing the foundational layer for building the next generation of enterprise AI systems. They enable organizations to move beyond bespoke integrations towards truly scalable, maintainable, secure, and interoperable AI ecosystems. For enterprises serious about leveraging AI for strategic advantage, adopting and investing in MCP and A2A principles is not just a technical choice – it's a strategic imperative. The future of enterprise AI will be built on collaboration, and these protocols provide the blueprint for making that collaboration effective.
Case Study
HR Intelligent Document Processing System
Use Case: An enterprise needs to process thousands of job applications efficiently. The goal is to parse resumes, match candidates against job descriptions, evaluate qualifications, and flag top candidates for human review, integrating with the existing Applicant Tracking System (ATS).
Technical Architecture of the multi-agent system:
Document Ingestion Agent: Monitors application sources (email, web portal), retrieves new resumes/cover letters.
Document Parsing Agent: Uses OCR and layout analysis (potentially specialized models) to extract raw text and structure from various formats (PDF, DOCX, scanned images).
Information Extraction Agent: Employs an LLM or specialized NLP model to identify and extract key entities (skills, experience, education, contact info etc.)
Candidate Evaluation Agent: Compares extracted information against job description requirements, calculates match scores, and ranks candidates. Uses potentially different models or rule sets based on role complexity.
ATS Integration Agent: Formats evaluation results and updates the central Applicant Tracking System.
Human-in-the-Loop (HITL) Agent: Flags ambiguous cases or top candidates for review by HR personnel via a dedicated interface.
In the multi agent architecture above, adding standard protocols have the following effects.
MCP enables the following:
Consistent Context for Extraction: The Parsing Agent outputs text/structure in a standardized MCP format. The Information Extraction Agent receives this consistent context, regardless of the original document format or the specific parsing model used.
Handling Large Documents: For long resumes or complex cover letters exceeding LLM context limits, MCP facilitates segmentation. The Parsing Agent might break the document, and MCP provides the context linking these segments for the Extraction Agent.
Standardized Input/Output for ATS: The Evaluation Agent produces results (scores, rankings, extracted data) in an MCP-defined structure, which the ATS Integration Agent consumes, simplifying integration logic.
A2A facilitates the following:
Coordinated Workflow: A2A defines the handoffs. Ingestion Agent sends a "NEW_DOCUMENT" task via A2A message (e.g., on a Kafka topic) to the Parsing Agent pool. Parsing Agent sends "PARSE_COMPLETE" with MCP context payload to the Extraction Agent. Extraction Agent sends "EXTRACTION_COMPLETE" to the Evaluation Agent, and so on.
Clear Communication Protocols: Message types like REQUEST_PARSE, PARSE_RESULT, REQUEST_EVALUATION, EVALUATION_RESULT, UPDATE_ATS are defined within the A2A protocol, ensuring clarity. Error messages (PARSE_FAILED, EVALUATION_ERROR) follow a standard format.
Scalable Processing: Agents can be scaled independently. If parsing becomes a bottleneck, more Parsing Agents can be deployed; they pick up tasks via the A2A communication channel (e.g., message queue). A2A routing handles load balancing.
References
The following resources provide deeper technical insights and enterprise perspectives on Model Context Protocol (MCP) and Agent-to-Agent (A2A) communication.
MCP Documentation (Anthropic) – https://docs.anthropic.com/en/docs/agents-and-tools/mcp
MCP Servers (GitHub Repository) – https://github.com/modelcontextprotocol/servers
Enterprise Security for MCP (arXiv) – https://arxiv.org/abs/2504.08623
A2A Protocol Overview (a2aprotocol.ai) – https://a2aprotocol.ai
Multi-Agent Collaboration Study (arXiv) – https://arxiv.org/abs/2412.05449
The hype surrounding Generative AI and Multi-Agent Systems often clashes with the messy reality of enterprise integration. We're rapidly moving beyond standalone AI models towards sophisticated, multi-agent systems designed to tackle complex, end-to-end enterprise workflows – a transition whose complexities we explored in our previous post, "Navigating the complexities of building and scaling Multi-Agent System". Yet, as these AI ecosystems grow in sophistication and scale, agent communication and context management have emerged as critical bottlenecks, hindering the very scalability, interoperability, and maintainability they promise.
The core problem?
A lack of standardization. We see bespoke integrations, inconsistent context handling between different AI models especially LLM, and brittle, ad-hoc communication methods between AI agents. This leads to complex, difficult-to-debug systems that struggle to scale and adapt.
Agent Communication Is the New Bottleneck
As we build more sophisticated AI systems composed of multiple specialized agents (e.g., one agent for data retrieval, another for analysis, a third for summarization), the interactions between these agents become paramount.
Challenges in Multi-Agent Systems (MAS)
Ad-hoc Communication: Teams often invent their own communication methods for each project, leading to inconsistency and integration nightmares.
Agent Coupling: Agents become overly dependent on the specific implementation details of others, making changes difficult and risky.
Lack of Discoverability: Agents can't easily find other agents that could help them, limiting dynamic collaboration.
Error Handling Complexity: Failures in one agent can cascade unpredictably without standardized error reporting and recovery protocols.
Debugging Hell: Tracing a request or workflow across multiple agents with different communication styles is incredibly difficult.
How Traditional Approaches Fail at Scale
Simple point-to-point integrations or basic API calls work for a few agents but break down quickly. Imagine dozens or hundreds of agents – the number of potential interactions explodes (N*(N-1)). Without standardization, this becomes an unmanageable web of dependencies.
Increased Latency: Poorly optimized or synchronous communication chains add significant delays.
High Failure Rates: Lack of standardized error handling leads to brittle systems where one agent failure brings down entire workflows.
Integration Effort: Time spent mapping data formats and communication protocols between agents becomes a major development cost.
Monitoring Complexity: Tracking performance and health across diverse communication channels is challenging.
Enter the power duo: MCP & A2A
These complementary protocols provide the standardized foundation needed to overcome these challenges. MCP focuses on standardizing how AI models receive and understand context, while A2A defines how independent AI agents interact and collaborate.
This comprehensive guide will dissect MCP and A2A, exploring their technical underpinnings, enterprise applications, and practical implementation strategies. We will delve into technical specifications, architectural patterns, real-world case studies, and developer best practices. By the end, you'll understand why MCP and A2A together form the foundation for truly scalable, interoperable multi-agent AI systems that can transform enterprise operations.
Understanding Model Context Protocol (MCP)
Model Context Protocol (MCP) is an open-source protocol developed by Anthropic (creators of Claude) that establishes a standardized framework for LLM integrations across the industry. As an open-source initiative, MCP aims to create a universal standard that benefits the entire AI ecosystem through collaborative development and widespread adoption.
At its core, MCP provides a standardized methodology for connecting LLM applications with external data sources, knowledge bases, and tools. Essentially, it defines implementation guidelines that create a universal interface layer between AI models and the contextual information they require to function effectively.
By establishing common patterns for data access, tool execution, and prompt management, MCP eliminates the need for developers to build custom integrations for each AI model they wish to incorporate. This standardization enables developers to focus on creating flexible, powerful AI applications with seamless access to files, databases, APIs, and other essential resources—all without being locked into proprietary implementations.

Fig 1: Model Context Protocol Extensibility
Architecture
At its core, MCP acts as an abstraction layer. Instead of applications directly formatting prompts or context windows in model-specific ways, they format context according to the MCP specification. An MCP-compliant layer then translates this standardized context into the format required by the target AI model.
MCP implements a robust client-server architecture comprised of five key components that work in concert to establish secure, standardized connections between LLMs and external resources:

Fig 2: MCP Architecture Diagram
MCP Hosts
These are applications requiring contextual AI capabilities, such as conversational assistants, AI-enhanced development environments, enterprise knowledge systems, or custom business applications. Hosts integrate with MCP clients to access data and leverage external tools through the standardized protocol, allowing them to focus on delivering value rather than managing integration complexities.
MCP Clients
Clients maintain dedicated 1:1 connections with MCP Servers and handle protocol-specific operations that the server doesn't need to manage. They serve as translators, converting host application requests into standardized MCP messages, managing connection states, and handling authentication and security with servers. This abstraction layer ensures hosts can communicate with any MCP-compliant server without modification.
MCP Servers
Servers form the operational core of the MCP ecosystem, exposing critical capabilities through the standardized interface. An MCP server might provide access to document repositories, specialized databases, analytical tools, or integrate with external APIs—all through a consistent, well-defined protocol. Servers implement the business logic necessary to fulfill client requests while maintaining security boundaries.
Let’s take an example of a Github MCP Server:
Git MCP Server : For Git repositories, an MCP server transforms technical version control systems into contextually aware knowledge bases:
Intelligent code context: Rather than forcing developers to manually specify file paths, commit hashes, and diffs, a Git MCP server can respond to requests like "Find who modified the authentication logic in the last month" by understanding code semantics beyond simple text patterns.
Cross-repository knowledge: When an LLM assistant needs context across multiple repositories or branches, the MCP server handles the complex task of assembling relevant code, commit messages, pull request discussions, and documentation into a coherent context window.
Development workflow integration: Instead of having AI tools make raw API calls to Git hosting services (GitHub, GitLab, etc.), the MCP server enforces secure, standardized patterns for repository interaction, ensuring consistent behavior across different AI systems while maintaining proper authentication and access controls.
Local Data Sources
These represent secure, organization-controlled resources such as files, databases, and internal services that MCP servers can access based on fine-grained permissions. The protocol implements comprehensive security measures ensuring AI models can only access authorized resources with appropriate authentication and access controls, protecting sensitive information while enabling useful functionality.
Remote Services
These encompass external, internet-accessible services available via APIs that MCP servers can connect to as needed. They might include public knowledge bases, code repositories, specialized computational tools, or third-party business services. MCP standardizes how these external services are discovered, authenticated, and utilized within the broader AI application ecosystem.
MCP vs LLM Context Limitations
LLMs have limited context windows. MCP helps by:
Standardized Summarization: Defining protocols for context summarization techniques.
Prioritization: Allowing context elements to be tagged with priority levels, ensuring critical information isn't truncated.
Retrieval Augmented Generation (RAG) Integration: Providing a standard way to represent retrieved documents or data snippets within the context payload.
Context Segmentation: Enabling large tasks to be broken down, with MCP managing the relevant context segment for each sub-task.
Enterprise Applications of MCP
Advantages of Adopting MCP
Adopting Model Context Protocol (MCP) provides enterprises with significant strategic advantages by establishing a standardized infrastructure for managing AI contexts across diverse applications and teams. With MCP, organizations can substantially reduce redundant efforts in prompt engineering and context management, as it introduces a common, universally understood schema. This standardization allows internal development teams to focus more on core application logic and less on model-specific context handling, significantly streamlining the onboarding process for new AI models. Additionally, MCP offers considerable benefits for external partners and vendors by clearly specifying integration standards, which promotes seamless compatibility and consistent governance across third-party solutions.
Key Enterprise Considerations
When integrating MCP into enterprise environments, several critical considerations must be addressed to ensure success and security. Governance and control become central, as MCP’s structured context enables fine-grained policy enforcement, precise masking or encryption of sensitive data, and consistent audit logging. Additionally, MCP promotes scalability across multiple departments by ensuring context representations remain compatible, facilitating smoother cross-functional workflows. Vendor interoperability is significantly improved, reducing the risk of vendor lock-in by isolating model-specific changes to the MCP adapter layer rather than the core application logic. Moreover, MCP provides essential future-proofing by abstracting application logic from underlying model changes, protecting investments as AI architectures evolve. Lastly, infrastructure security and access control are paramount, necessitating robust authentication and authorization mechanisms, rigorous input validation to mitigate injection attacks, and comprehensive hardening of MCP interfaces to protect sensitive enterprise resources.
Developers Guide for MCP Implementation
Implementing Model Context Protocol effectively requires careful schema design, robust context management logic, and well-defined integration points with AI models via adapters. This guide provides practical advice for developers tasked with building or utilizing MCP within their applications.
Best Practices
Schema Design - Start Simple, Iterate:
Define clear, well-documented schemas for your core context elements (e.g., user profile, chat history, document snippet, system prompt). Use standard formats like JSON Schema or Protobuf.
Start with essential fields and add complexity gradually. Avoid trying to create a universal schema for everything at once.
Versioning is Crucial: Implement versioning for your MCP schemas from day one to manage evolution and maintain backward compatibility.
Reusability: Design schemas to be composable and reusable across different applications or agents.
Modular Context Building:
Create dedicated functions or classes responsible for generating specific types of MCP context elements.
The main application logic should orchestrate calling these builders to assemble the final MCP payload, rather than embedding complex formatting logic everywhere.
Explicit Context Window Management:
Don't rely on downstream models to implicitly handle context limits. Implement strategies within your MCP layer or context builders:
Prioritization: Use metadata tags (e.g., priority: high/medium/low) within MCP elements to guide truncation or summarization.
Summarization: Integrate summarization techniques (e.g., calling a separate summarization model, using rule-based summarization) for long context elements like chat history or documents. Store summaries as distinct MCP elements.
RAG Integration: Standardize how retrieved chunks from Retrieval Augmented Generation are represented within the MCP structure (e.g., type: retrieved_document_chunk, with source and relevance score metadata).
Configurable Strategies: Allow configuration of how context limits are handled (e.g., "truncate oldest", "summarize history", "prioritize
Thin and Pluggable Adapters:
The MCP Adapter's sole responsibility should be translating the standardized MCP payload into the specific format required by the target AI model API.
Keep business logic out of adapters.
Design adapters to be easily swappable to support different models or API versions without changing the core application or context-building logic.
Standardized Output Parsing:
Define how model responses (content, function calls, metadata) are parsed back, potentially into a standardized MCP structure (e.g., type: model_response). This facilitates logging, evaluation, and using model output as context for subsequent calls.
Handle potential errors or malformed responses from the model gracefully.
Common Pitfalls
Over-Engineering Schemas: Creating excessively complex or rigid schemas early on that are hard to adapt. Start flexible and refine based on needs.
"Leaky" Adapters: Embedding model-specific logic or assumptions outside the adapter, breaking the abstraction MCP provides.
Inefficient Context Handling: Simply concatenating all available information without intelligent prioritization, summarization, or truncation, leading to poor model performance or hitting API limits unexpectedly.
Security/Compliance as an Afterthought: Failing to tag sensitive data properly during context creation or failing to enforce policies based on those tags before model interaction.
Lack of Testing for Context Logic: Bugs in context summarization, truncation, or prioritization can be subtle and significantly impact model quality and cost.
Monolithic Context Generation: Building context in one large, complex function instead of using modular, reusable builders, making maintenance difficult.
Poor Documentation: Undocumented schemas or context element types make it hard for other developers (or future you) to understand and use the MCP implementation correctly.
By following these best practices and being mindful of potential pitfalls, developers can effectively leverage MCP to build more robust, maintainable, and interoperable LLM applications.
Understanding Agent-to-Agent (A2A) Protocol
Agent-to-Agent (A2A) Protocol defines a standardized set of rules, message formats, and interaction patterns for communication and coordination between independent software agents, particularly AI agents within a multi-agent system (MAS).
A2A makes it easy for one agent (the “client”) to send tasks to another agent (the “remote”) and get them done. The client agent creates and sends the task, while the remote agent receives it and carries it out.

Fig 3: A2A Interaction Capabilities [1]
Architectural Principles
A2A protocols often borrow principles from established distributed systems and communication paradigms like microservices, REST, gRPC, and message queuing, but tailor them for the specific needs of autonomous or semi-autonomous agents. Key principles include:
Decentralization: Agents operate independently but can discover and interact with others.
Standardization: Common message structures and interaction protocols ensure interoperability.
Asynchronicity: Often favored to handle network latency and allow agents to work concurrently, though synchronous request/reply is also supported.
Discoverability: Mechanisms for agents to find other agents based on capabilities.
Negotiation: Protocols for agents to agree on tasks, data formats, or workflow steps.
Technical Specifications
Message Formats: Typically standardized formats like JSON or Protobuf are used. Messages usually include headers (sender ID, recipient ID, message ID, timestamp, intent/type) and a payload (task description, data, query, status update). MCP-structured context can often be part of the A2A payload.
Authentication Mechanisms: Ensures agents are who they claim to be. Options include API keys, OAuth tokens (e.g., client credentials flow), mutual TLS (mTLS), or JWTs signed by a trusted authority.
Routing Protocols: Defines how messages get from sender to recipient. This could involve direct HTTP calls, message queues (like RabbitMQ, Kafka), or dedicated agent communication platforms/meshes.
Agent Discovery: Mechanisms like a central registry, gossip protocols, or service discovery tools (e.g., Consul, Etcd) allow agents to find others offering needed capabilities.
Capability Negotiation: Standardized ways for agents to advertise their skills (e.g., "summarize text," "extract entities," "query database schema X") and for requesting agents to specify requirements.
Task Delegation & Coordination: Protocols for assigning tasks, tracking progress, handling errors, and coordinating multi-step workflows (e.g., using patterns like Saga or Choreography).
A2A vs Other Protocols
vs. REST/HTTP: A2A builds upon HTTP but adds agent-specific semantics (discovery, negotiation, standardized task types). Simple REST is often too generic for complex agent coordination.
vs. gRPC: Offers performance benefits (Protobuf, HTTP/2) but requires stricter schema definitions. A2A can leverage gRPC as a transport layer.
vs. Message Queues (Kafka, RabbitMQ): Excellent for asynchronous, decoupled communication. A2A protocols define the content and meaning of messages flowing through these queues, adding a layer of application-level understanding and not only this but conversation patterns, capability discovery, and error handling specific to agent interactions.
Enterprise Applications of A2A
Advantages of Adopting A2A
The A2A protocol offers enterprises a standardized framework that significantly enhances the interoperability and scalability of AI systems. By enabling seamless communication between AI agents, regardless of their underlying technologies or vendors, A2A facilitates the creation of dynamic, multi-agent ecosystems. This interoperability allows for the integration of specialized agents across various departments, promoting a more cohesive and efficient workflow. Moreover, A2A supports dynamic capability discovery through mechanisms like “Agent Cards,” enabling agents to advertise their functionalities and discover others based on capabilities, thus fostering a collaborative environment where agents can coordinate tasks effectively.
In addition to interoperability, A2A provides robust support for long-running tasks, offering mechanisms for real-time feedback, notifications, and state updates throughout the task lifecycle. This is particularly beneficial for complex business operations that require extended processing times. The protocol’s design, built upon existing standards such as HTTP, SSE, and JSON-RPC, ensures compatibility with current IT infrastructures, simplifying integration and reducing the need for custom solutions.
Furthermore, A2A incorporates enterprise-grade security features, including authentication and authorization mechanisms, ensuring secure communication between agents. By adopting A2A, enterprises can build modular and composable AI systems, where agents can be easily swapped, updated, or added with minimal friction, significantly accelerating development and improving maintainability
Key Enterprise Considerations
Implementing the A2A protocol within an enterprise setting necessitates careful consideration of several critical factors to ensure effective deployment and operation. Security and compliance are paramount; enterprises must establish robust authentication and authorization mechanisms, such as mutual TLS (mTLS) and OAuth tokens, to safeguard agent communications. Additionally, maintaining comprehensive audit trails and enforcing fine-grained access controls are essential for meeting regulatory requirements and ensuring data integrity.
Scalability and performance are also crucial considerations. As the number of interacting agents grows, enterprises need to ensure that the underlying infrastructure can scale accordingly. This includes optimizing message routing, load balancing, and monitoring to maintain performance. Integration with existing systems is another key aspect; A2A should be thoughtfully integrated with current enterprise applications and workflows, potentially involving the development of adapters or middleware to bridge between A2A-compliant agents and legacy systems. Furthermore, implementing governance frameworks to define and enforce policies around agent interactions, data sharing, and task delegation is vital for maintaining control and oversight. Deploying comprehensive monitoring and observability tools is also essential for tracking agent interactions, diagnosing issues, and ensuring the health of the multi-agent system. Finally, enterprises should invest in training and change management strategies to facilitate adoption and minimize disruption, as well as engage with the broader A2A community.
Developers Guide for A2A Implementation
Implementing robust A2A communication requires careful planning and adherence to best practices.
Best Practices
Choose the Right Transport:
Synchronous (Request/Reply): Use HTTP/S (REST-like) or gRPC for immediate responses when needed. Good for simple queries or commands.
Asynchronous (Fire & Forget/Events): Use Message Queues (RabbitMQ, Kafka, NATS, Pulsar) for decoupling, resilience, and workflows where immediate response isn't critical. Better for scalability and handling long-running tasks.
Define Clear Message Schemas: Use JSON Schema, Protobuf, or Avro to strictly define A2A message structures (headers and payload). Include versioning in your schemas.
Standardize Message Headers: Always include essential headers: unique message ID, correlation ID (to track workflows), sender/recipient IDs, timestamp, message type/intent.
Idempotency: Design message handlers to be idempotent where possible, especially with asynchronous systems. Processing the same message twice should not cause unintended side effects. Use the message ID for deduplication.
Error Handling & Resilience:
Define standard error message formats.
Implement retry mechanisms (e.g., exponential backoff) for transient network issues.
Use Dead Letter Queues (DLQs) in message brokers to handle messages that consistently fail processing.
Implement circuit breaker patterns to prevent cascading failures.
Agent Discovery: Use a service registry or a dedicated agent directory for dynamic discovery. Avoid hardcoding agent addresses.
Security First: Implement strong authentication and authorization from the start. Use standard protocols (OAuth 2.0, mTLS, JWT). Encrypt traffic (TLS).
Monitoring and Observability:
Logging: Log key information for each message sent/received (headers, size, timing). Use structured logging.
Tracing: Implement distributed tracing (e.g., OpenTelemetry) using the correlation ID to track requests across multiple agents.
Metrics: Monitor queue depths, message processing times, error rates, agent availability.
Common Pitfalls
Overly Chatty Communication: Designing agents that require excessive back-and-forth increases latency and complexity. Aim for well-defined, substantive interactions.
Ignoring Versioning: Failing to version message schemas leads to compatibility issues as agents evolve.
Lack of State Management: Assuming agents are stateless when workflows require state can lead to errors. Consider external state stores or stateful agent patterns if needed.
Inadequate Monitoring: Flying blind without proper logging, tracing, and metrics makes debugging and optimization impossible.
Security as an Afterthought: Adding security late in the process is much harder and riskier.
Performance Optimization
Asynchronous Communication: Prefer message queues for decoupling and parallelism.
Batching: Send/process messages in batches where appropriate (e.g., batch updates to a database).
Efficient Serialization: Use binary formats like Protobuf or MessagePack instead of JSON for performance-critical paths.
Caching: Cache frequently accessed data or results within agents (with appropriate invalidation).
Optimize Transport: Tune message broker configurations, use efficient network protocols (gRPC).
Enterprise Integration: Making MCP and A2A Work Together
MCP and A2A are highly synergistic. MCP standardizes the content (context) an agent needs or produces, while A2A standardizes the interaction (communication) between agents.
Integration
A common integration strategy between MCP and A2A involves embedding MCP-formatted context within the payload of A2A messages. In practice, an agent initiating a task via A2A includes the necessary input data structured according to MCP standards. The receiving agent completes the requested operation and returns its results, often formatted using MCP as well, ensuring consistent and standardized context communication. Additionally, enterprises can deploy a dedicated “Context Orchestration Agent” that leverages A2A protocols to coordinate interactions with various specialized data source agents, such as those interfacing with databases or document repositories. This orchestration agent assembles the collected data into a structured MCP payload, which can then be provided seamlessly to processing agents. Furthermore, A2A’s capability negotiation mechanism benefits significantly from MCP, as agents can clearly specify the MCP schemas they support for both input and output, streamlining discovery and collaboration processes across diverse multi-agent workflows.
Architectural Patterns for Deployment
For enterprise deployments of multi-agent systems, several architectural patterns are particularly effective. One common approach is the Agent Mesh or Fabric, which resembles traditional service meshes like Istio or Linkerd but is specifically optimized for agent-based communication. This model provides a dedicated infrastructure layer that manages A2A routing, agent discovery, security through mutual TLS (mTLS), observability, and policy enforcement, with agent communication handled through mesh sidecars or proxies. Alternatively, a Central Message Bus approach employs enterprise-grade message queues such as Kafka, Pulsar, or Solace to facilitate agent interactions via publish-subscribe patterns. Here, governance and access control are managed directly at the broker or topic level, enabling scalable and decoupled agent communication. Finally, the Agent Gateway architecture adapts the API Gateway pattern for agent interactions, where external requests and internal agents interact with a central gateway responsible for routing A2A messages to appropriate backend agents, enforcing security measures, and potentially translating between different communication protocols, thereby simplifying and standardizing access to agent services.
Technical Considerations
Successfully implementing MCP and A2A in an enterprise context involves careful attention to several critical technical considerations. Authentication and authorization require deliberate decisions around centralized versus decentralized enforcement mechanisms, robust token formats such as JWTs or OAuth, and secure management of keys and secrets to safeguard agent communications. Equally important is establishing comprehensive monitoring and observability practices, including standardized logging formats, consistent trace propagation across agent interactions, and clear definition of KPIs to measure and optimize agent effectiveness. Scalability and load balancing strategies will vary depending on the chosen A2A transport, such as employing Kubernetes Horizontal Pod Autoscalers for agent pods, utilizing Kafka consumer groups for message-driven workflows, or leveraging built-in load-balancing capabilities of gRPC. Additionally, robust error handling and recovery processes must be implemented, potentially employing distributed patterns like Saga or choreography for complex workflows, complemented by well-defined retry logic and effective use of Dead Letter Queues (DLQs) to handle persistent failures gracefully.
Complementing these technical foundations, security and governance require implementing centralized identity management (e.g., via an Identity Provider for issuing JWT or OAuth tokens) and strategically placed policy enforcement points within agent infrastructures. MCP tags further strengthen security by clearly marking sensitive data for targeted policy enforcement. Comprehensive auditing through centralized logging of all A2A interactions and MCP context usage ensures transparency, supports compliance, and facilitates effective oversight.
Future Directions & Enterprise Strategy
MCP and A2A are not static concepts; they represent an evolving approach to building complex AI systems.
Upcoming Developments
Formal Standardization Efforts: Expect industry groups or consortia (potentially involving major cloud providers, AI labs, and enterprise users) to propose more formal standards for both MCP and A2A, akin to OpenAPI for REST APIs or CloudEvents for eventing.
Richer Context Types (MCP): Support for multimodal context (images, audio, video), more sophisticated knowledge graph representations, and standardized ways to include agent reasoning traces within context.
Advanced A2A Capabilities: Protocols for complex negotiation, automated composition of agent services, decentralized trust and reputation systems for agents, and tighter integration with workflow orchestration engines.
AI Model Integration: MCP adapters will become more sophisticated, potentially handling automated context optimization (prompt compression, selective inclusion) based on model capabilities and cost.
Strategic Framework for Adoption
Acknowledge the Need: Recognize that ad-hoc integration is unsustainable for complex, mission-critical AI.
Invest in Standardization: Champion internal efforts to define and adopt MCP and A2A principles, even if formal external standards are still emerging. Start with core schemas and protocols relevant to your business.
Build Incrementally: Don't try to boil the ocean. Start with pilot projects, prove the value, and build out infrastructure and standards gradually.
Foster Collaboration: Encourage communication between AI/ML teams, platform engineering, security, and governance stakeholders.
Prioritize Interoperability: Design systems with the assumption that you will need to integrate agents and models from different sources (internal, vendor, open-source).
Stay Informed: Monitor developments in AI orchestration, agent frameworks (like LangChain, AutoGen, CrewAI), and emerging standardization efforts.
Future-proofing AI architectures hinge on building systems with clear abstraction and modularity, principles directly supported by MCP and A2A. By leveraging these protocols as foundational abstraction layers, enterprises can keep application logic centered on core business processes, delegating model-specific context management and agent communications entirely to MCP and A2A.
Conclusion
The era of standalone AI models solving isolated problems is giving way to a future of collaborative intelligence, where multi-agent systems tackle complex enterprise challenges. The primary obstacles to realizing this future at scale are the chaos of inconsistent context management and brittle, ad-hoc agent communication.
Model Context Protocol (MCP) and Agent-to-Agent (A2A) communication directly address these bottlenecks. MCP brings order to how AI models understand the world by standardizing context, overcoming limitations, and enabling governance. A2A brings order to how AI agents interact, enabling scalable, robust, and interoperable collaboration through standardized communication patterns.
Together, MCP and A2A form a powerful duo, providing the foundational layer for building the next generation of enterprise AI systems. They enable organizations to move beyond bespoke integrations towards truly scalable, maintainable, secure, and interoperable AI ecosystems. For enterprises serious about leveraging AI for strategic advantage, adopting and investing in MCP and A2A principles is not just a technical choice – it's a strategic imperative. The future of enterprise AI will be built on collaboration, and these protocols provide the blueprint for making that collaboration effective.
Case Study
HR Intelligent Document Processing System
Use Case: An enterprise needs to process thousands of job applications efficiently. The goal is to parse resumes, match candidates against job descriptions, evaluate qualifications, and flag top candidates for human review, integrating with the existing Applicant Tracking System (ATS).
Technical Architecture of the multi-agent system:
Document Ingestion Agent: Monitors application sources (email, web portal), retrieves new resumes/cover letters.
Document Parsing Agent: Uses OCR and layout analysis (potentially specialized models) to extract raw text and structure from various formats (PDF, DOCX, scanned images).
Information Extraction Agent: Employs an LLM or specialized NLP model to identify and extract key entities (skills, experience, education, contact info etc.)
Candidate Evaluation Agent: Compares extracted information against job description requirements, calculates match scores, and ranks candidates. Uses potentially different models or rule sets based on role complexity.
ATS Integration Agent: Formats evaluation results and updates the central Applicant Tracking System.
Human-in-the-Loop (HITL) Agent: Flags ambiguous cases or top candidates for review by HR personnel via a dedicated interface.
In the multi agent architecture above, adding standard protocols have the following effects.
MCP enables the following:
Consistent Context for Extraction: The Parsing Agent outputs text/structure in a standardized MCP format. The Information Extraction Agent receives this consistent context, regardless of the original document format or the specific parsing model used.
Handling Large Documents: For long resumes or complex cover letters exceeding LLM context limits, MCP facilitates segmentation. The Parsing Agent might break the document, and MCP provides the context linking these segments for the Extraction Agent.
Standardized Input/Output for ATS: The Evaluation Agent produces results (scores, rankings, extracted data) in an MCP-defined structure, which the ATS Integration Agent consumes, simplifying integration logic.
A2A facilitates the following:
Coordinated Workflow: A2A defines the handoffs. Ingestion Agent sends a "NEW_DOCUMENT" task via A2A message (e.g., on a Kafka topic) to the Parsing Agent pool. Parsing Agent sends "PARSE_COMPLETE" with MCP context payload to the Extraction Agent. Extraction Agent sends "EXTRACTION_COMPLETE" to the Evaluation Agent, and so on.
Clear Communication Protocols: Message types like REQUEST_PARSE, PARSE_RESULT, REQUEST_EVALUATION, EVALUATION_RESULT, UPDATE_ATS are defined within the A2A protocol, ensuring clarity. Error messages (PARSE_FAILED, EVALUATION_ERROR) follow a standard format.
Scalable Processing: Agents can be scaled independently. If parsing becomes a bottleneck, more Parsing Agents can be deployed; they pick up tasks via the A2A communication channel (e.g., message queue). A2A routing handles load balancing.
References
The following resources provide deeper technical insights and enterprise perspectives on Model Context Protocol (MCP) and Agent-to-Agent (A2A) communication.
MCP Documentation (Anthropic) – https://docs.anthropic.com/en/docs/agents-and-tools/mcp
MCP Servers (GitHub Repository) – https://github.com/modelcontextprotocol/servers
Enterprise Security for MCP (arXiv) – https://arxiv.org/abs/2504.08623
A2A Protocol Overview (a2aprotocol.ai) – https://a2aprotocol.ai
Multi-Agent Collaboration Study (arXiv) – https://arxiv.org/abs/2412.05449
Subscribe to our newsletter to never miss an update
Other articles


Agents Talk, Models Think: A2A + MCP for Enterprise Agentic AI

Riya Parikh, Nitai Agarwal
Apr 27, 2025
Read the article
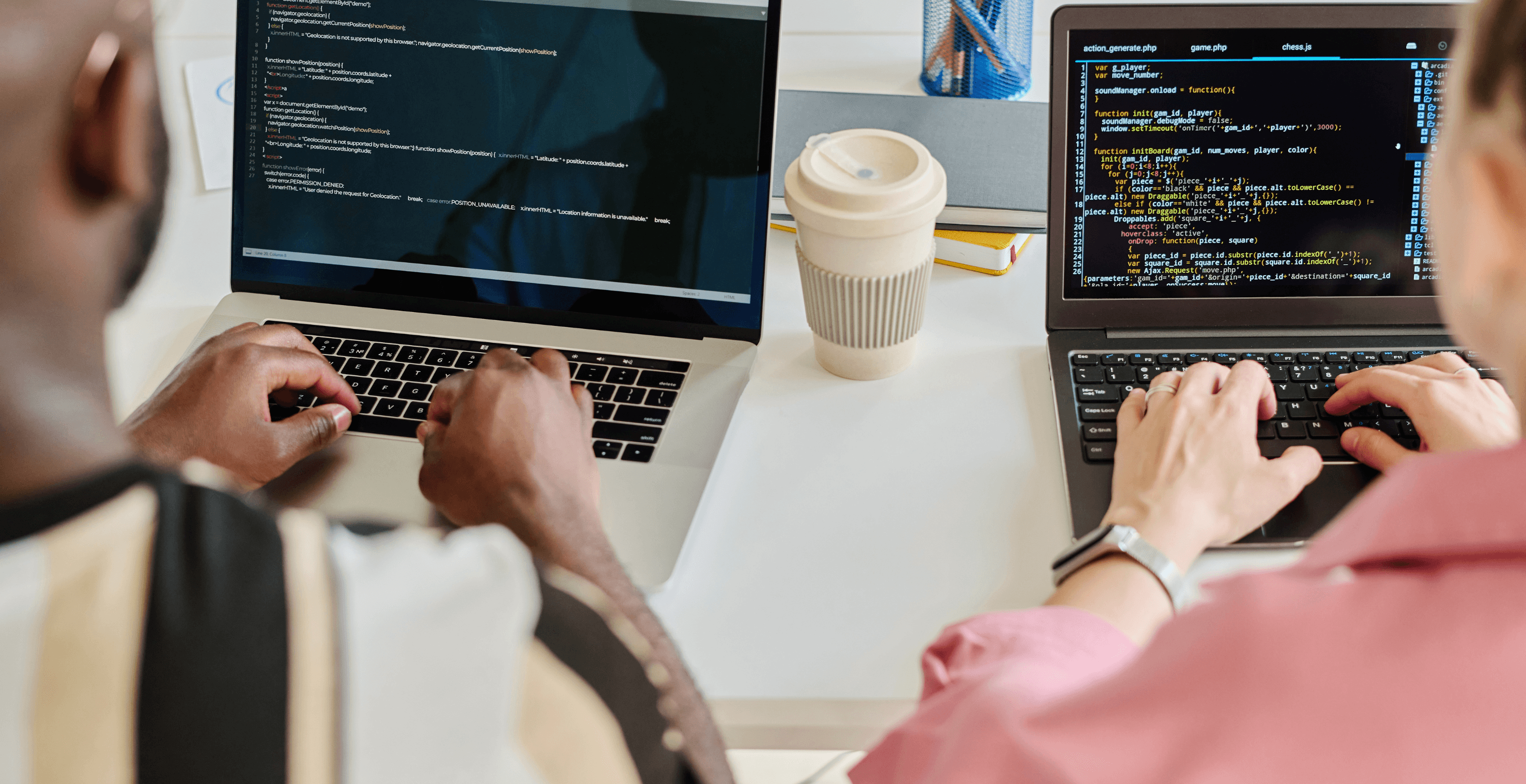
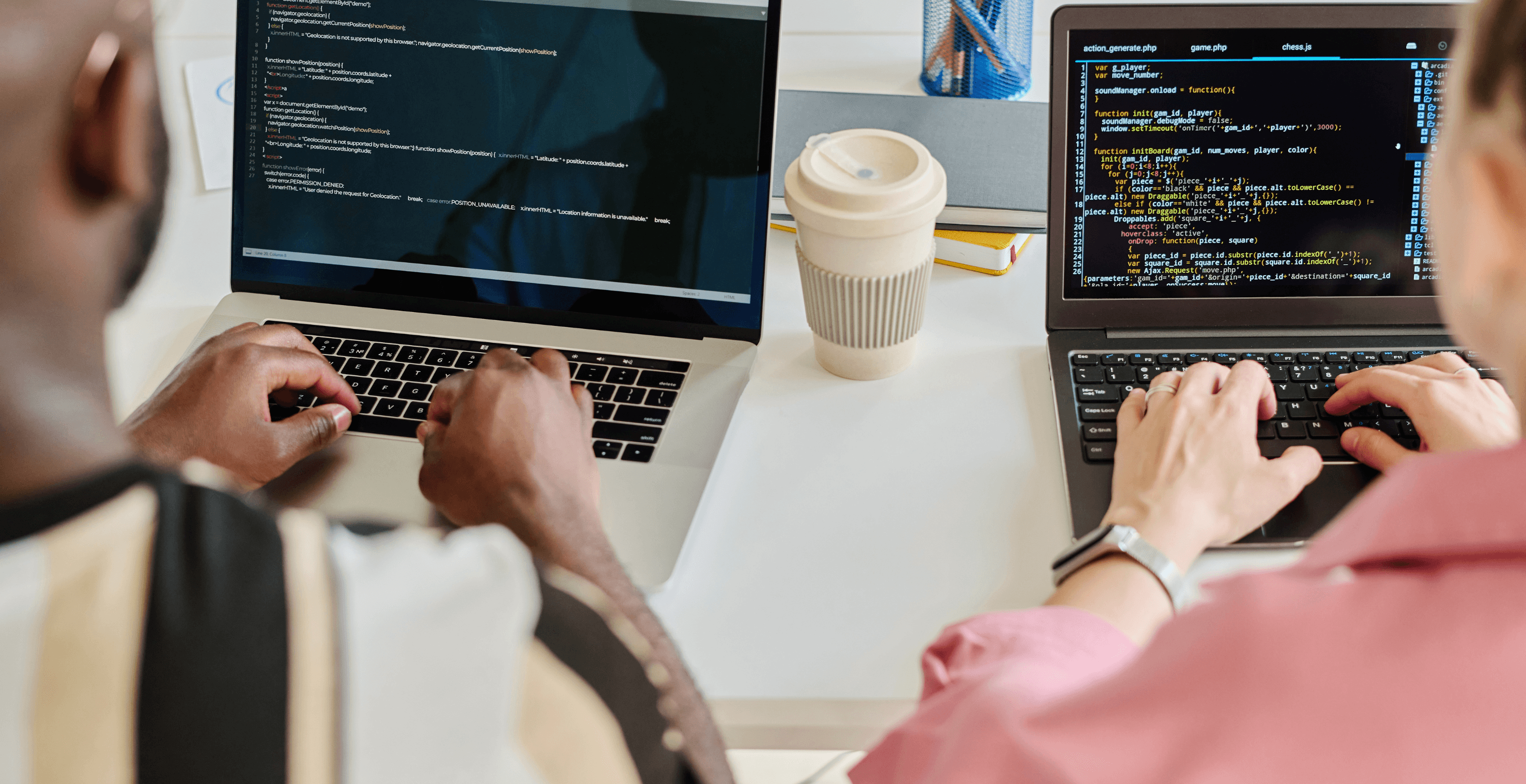
Understanding NeMo Guardrails: A Toolkit for LLM Security

Rehan Asif
Dec 24, 2024
Read the article


Understanding Differences in Large vs Small Language Models (LLM vs SLM)

Rehan Asif
Dec 21, 2024
Read the article