Understanding Differences in Large vs Small Language Models (LLM vs SLM)
Rehan Asif
Dec 21, 2024




Artificial Intelligence has significantly transformed the way we interact with technology, and nowhere is this more evident than in the field of Natural Language Processing (NLP). From smart assistants to advanced text generation, NLP advancements have reshaped our digital experiences. Whether you're a data scientist, a mid-level manager, or an AI enthusiast, grasping the distinctions between LLMs and SLMs is crucial for making informed decisions.
In this article, you'll learn the basics of LLMs and SLMs, their differences and how to choose them for your AI needs. Now, let’s dive deeper into understanding Large Language Models (LLMs).
Understanding Large Language Models (LLMs)
Large Language Models (LLMs) have emerged as a cornerstone in the field of NLP, enabling a range of applications from chatbots to content generation. These models handle vast amounts of data, making them exceptionally powerful for understanding and generating human-like text. Let's break down what makes LLMs unique and explore their key characteristics.
Definition and Characteristics of LLMs
LLMs are neural networks that are trained on extensive datasets, allowing them to learn the nuances of human language. Here’s what defines them:
Massive Datasets: LLMs are trained on billions of words from diverse sources.
High Number of Parameters: They contain millions to billions of parameters, which are weights and biases that the model learns during training.
Complex Architectures: LLMs use advanced architectures like transformers to process and generate text efficiently.
Versatile Applications: They are used in various applications, including translation, summarization, and content generation.
Human-Like Text Generation: LLMs excel at producing coherent and contextually accurate human-like text.
Training Datasets and Parameter Scale
The effectiveness of LLMs is largely due to the size and quality of their training datasets. These models process a variety of texts, from books and articles to websites, helping them understand context and generate coherent text.
Datasets: LLMs train on a diverse range of text sources, including books, articles, websites, and social media posts. This variety ensures the models are exposed to different writing styles, contexts, and topics, enhancing their ability to understand and generate text across multiple domains. The inclusion of diverse datasets is crucial for the models to learn the nuances of human language and produce contextually relevant outputs.
Parameter Scale: LLMs have a parameter scale ranging from millions to billions of parameters. These parameters represent the weights and biases the model learns during training, allowing it to understand and generate text with high accuracy. The larger the number of parameters, the better the model can capture complex patterns and relationships within the data. This extensive parameter scale contributes to the model's capability to produce sophisticated and contextually appropriate text, making LLMs powerful tools for various natural language processing tasks.
Transformer Architecture
LLMs utilize the Transformer architecture, which consists of encoder and decoder components:
Encoder: The encoder processes the input text to understand its context. It breaks down the input into smaller components, analyzes each part, and captures the underlying meaning and relationships within the text. This process involves converting the input text into a series of numerical representations that encapsulate the context and nuances of the original text, making it easier for the model to understand and work with.
Decoder: The decoder generates the output text based on the processed input. Using the numerical representations created by the encoder, the decoder constructs coherent and contextually relevant text. It systematically assembles words and phrases to form meaningful sentences that reflect the input's original context and intent. This step is crucial for producing accurate and human-like responses or translations in various natural language processing applications.
Although keep in mind that not all LLMs have the encoder component. For example, LLMs like GPT-3 work only on decoder components.
Here's a simple example of how a Transformer might be coded in Python using the ‘transformers’ library:
from transformers import GPT2Tokenizer, GPT2LMHeadModel
# Load pre-trained model and tokenizer
model_name = 'gpt2'
tokenizer = GPT2Tokenizer.from_pretrained(model_name)
model = GPT2LMHeadModel.from_pretrained(model_name)
# Encode input text
input_text = "The future of AI is"
input_ids = tokenizer.encode(input_text, return_tensors='pt')
# Generate text
output = model.generate(input_ids, max_length=50, num_return_sequences=1)
# Decode output
generated_text = tokenizer.decode(output[0], skip_special_tokens=True)
print(generated_text)
Capabilities and Common Applications
LLMs excel in several areas, making them highly versatile:
Contextual Text Generation: They can generate text that is contextually relevant and coherent.
Multimodal Abilities: Capable of processing and generating text, images, and even audio.
Advantages of LLMs
LLMs offer several advantages, including:
Complex Pattern Recognition: They can identify and understand intricate patterns in data.
High Performance in Precision Tasks: Ideal for tasks requiring high accuracy and detailed understanding.
Ethical Considerations
While powerful, LLMs also pose ethical challenges:
Misinformation: They can generate convincing but false information.
Bias: They might perpetuate biases present in their training data.
Privacy Risks: Handling of sensitive data needs careful management.
Now that you have a solid understanding of Large Language Models, let's explore the characteristics and advantages of Small Language Models (SLMs).
For a deeper dive into enhancing reliability with guardrails for LLMs, check out Raga AI’s case study on safeguarding enterprise LLM applications.
Understanding Small Language Models (SLMs)
Small Language Models (SLMs) offer a more streamlined approach to NLP, focusing on efficiency and specific task performance. They handle less data and require fewer resources, making them an excellent choice for many applications. Let's explore what makes SLMs distinct and their key benefits.
Definition and Characteristics of SLMs
SLMs are neural networks trained on smaller datasets compared to LLMs, focusing on efficiency and specific applications. Here’s what defines them:
Smaller Datasets: SLMs are trained on millions to tens of millions of words, often sourced from specific domains or industries. This focused training allows them to perform well within particular contexts or specialized tasks. By concentrating on a narrower range of data, SLMs can quickly and effectively learn the relevant patterns and nuances necessary for their specific applications.
Fewer Parameters: SLMs contain significantly fewer parameters than LLMs, usually ranging from a few million to a few hundred million. This reduction in parameters makes SLMs less resource-intensive, allowing them to run efficiently on less powerful hardware. Despite having fewer parameters, these models can still deliver strong performance within their specialized domains by leveraging the high-quality, targeted datasets they are trained on.
Efficient Computation: Due to their smaller size, SLMs require less computational power and memory, making them suitable for deployment in environments with limited resources. This efficiency is particularly advantageous for applications that need to run on edge devices or mobile platforms where computational capacity is restricted.
Faster Training and Inference: The reduced complexity of SLMs leads to faster training times and quicker inference speeds. This speed allows for rapid development cycles and real-time application scenarios, where timely responses are critical. The ability to quickly train and deploy these models makes them an attractive choice for many practical applications.
Specific Applications: SLMs are often designed for specific applications where domain knowledge is paramount. Examples include medical diagnosis, financial forecasting, and customer service automation. By tailoring the model to a particular use case, SLMs can achieve high accuracy and reliability, making them valuable tools in specialized fields.
Training Datasets and Parameter Scale
SLMs are effective due to their focused training datasets and manageable parameter scales. These models prioritize relevance and specificity over sheer size.
Datasets: SLMs are often trained on domain-specific datasets, ensuring their relevance to particular tasks. These datasets are carefully curated to include texts from specialized areas such as medical records, financial reports, technical manuals, or customer service interactions. By focusing on domain-specific data, SLMs can develop a deep understanding of the specific language, terminology, and context required for their target applications. This targeted training helps the models perform accurately and effectively within their specialized areas, providing reliable and contextually appropriate outputs.
Parameter Scale: SLMs typically have a parameter scale that ranges from millions to tens of millions of parameters. This relatively smaller scale compared to LLMs makes SLMs lighter and faster, requiring less computational power and memory. The reduced number of parameters allows these models to be more efficient, enabling quicker training times and faster inference speeds. Despite their smaller size, SLMs maintain the ability to capture essential patterns and relationships within their specialized domains, delivering high-performance results for specific tasks without the need for extensive computational resources. This efficiency makes SLMs an ideal choice for applications where speed and resource management are crucial.
Simpler Architecture Compared to LLMs
SLMs employ a simpler architecture, which contributes to their efficiency: They use fewer layers and parameters, reducing computational requirements.
Advantages of SLMs
SLMs offer several advantages, including:
Speed: Faster training and inference times.
Memory and Resource Efficiency: Require less computational power and memory, making them suitable for edge devices.
Limitations of SLMs
Despite their benefits, SLMs have some limitations:
Scalability Issues: Struggle with larger, more complex datasets.
Lower Performance in Intricate Tasks: May not perform as well in tasks requiring deep understanding and context.
Example Code for a Simple SLM
Here's a simple example of an SLM coded in Python using the ‘transformers’ library:
from transformers import DistilBertTokenizer, DistilBertModel
# Load pre-trained model and tokenizer
model_name = 'distilbert-base-uncased'
tokenizer = DistilBertTokenizer.from_pretrained(model_name)
model = DistilBertModel.from_pretrained(model_name)
# Encode input text
input_text = "Efficient NLP with small models"
input_ids = tokenizer.encode(input_text, return_tensors='pt')
# Generate embeddings
outputs = model(input_ids)
embeddings = outputs.last_hidden_state
print(embeddings)
For a comprehensive guide on testing AI models like SLMs, explore Raga AI’s guide to evaluating LLM applications and enabling guardrails.
Understanding the characteristics and advantages of Small Language Models sets the stage for comparing them with Large Language Models. Let's now examine the key differences between LLMs and SLMs.
Key Differences Between LLMs and SLMs
Understanding the distinctions between Large Language Models (LLMs) and Small Language Models (SLMs) is essential for using their strengths effectively. Let's break down the key differences to help you determine which model suits your needs best.

For further insights on AI models' implementation and optimization, explore Raga AI’s blog on enhancing edge AI with RagaAI integration on NVIDIA Metropolis.
Next, we will explore the resource requirements of LLMs and SLMs.
Resource Requirements
When choosing between Large Language Models (LLMs) and Small Language Models (SLMs), it's crucial to consider the resource requirements. These factors play a significant role in determining the feasibility and efficiency of deploying these models.
Resource Requirements of LLMs
LLMs are known for their high computational and memory demands. Here’s a breakdown of what they typically require:
High Memory Consumption: LLMs need substantial RAM to store and process large amounts of data.
Computational Power: They require powerful GPUs or TPUs to handle the complex calculations involved in training and inference.
Energy Usage: The extensive computational processes lead to high energy consumption, impacting both cost and environmental footprint.
Resource Requirements of SLMs
SLMs are designed with efficiency in mind, making them more accessible for various applications. Here’s what they typically require:
Lower Memory Consumption: SLMs need less RAM, making them suitable for devices with limited memory.
Reduced Computational Power: They can run on standard CPUs and require fewer GPUs, making them more cost-effective.
Energy Efficiency: Due to their simpler architecture, SLMs consume less energy, which reduces operational costs and environmental impact.
Efficiency Techniques
SLMs often employ efficiency techniques to maximize their performance within resource constraints:
Knowledge Distillation: Transferring knowledge from a larger model to a smaller one to maintain performance while reducing size.
Quantization: Reducing the precision of the model’s weights to decrease memory and computational requirements without significantly impacting accuracy.
Here's an example of how quantization can be implemented in Python using the ‘transformers’ library:
from transformers import DistilBertModel, DistilBertTokenizer
from transformers import pipeline
# Load pre-trained model and tokenizer
model_name = 'distilbert-base-uncased'
tokenizer = DistilBertTokenizer.from_pretrained(model_name)
model = DistilBertModel.from_pretrained(model_name)
# Quantize the model
quantized_model = torch.quantization.quantize_dynamic(
model, {torch.nn.Linear}, dtype=torch.qint8
)
# Use the quantized model in a pipeline
nlp_pipeline = pipeline('feature-extraction', model=quantized_model, tokenizer=tokenizer)
output = nlp_pipeline("Efficient NLP with quantized models")
print(output)
For a detailed look at optimizing AI models with resource-efficient techniques, check out Raga AI’s blog on LLM evaluation.
Along with resource utilization, LLMs and SLMs vary in training and fine-tuning, too. Let’s see how.
Training and Fine-Tuning
Training and fine-tuning Large Language Models (LLMs) and Small Language Models (SLMs) involve different processes and resource investments. Understanding these differences can help you choose the right approach for your specific needs.
Training Large Language Models (LLMs)
Training LLMs is a resource-intensive process that requires substantial computational power and time. Here’s a closer look at what’s involved:
Time and Cost: Training an LLM from scratch can take weeks or months and requires significant financial investment due to the computational resources needed.
Datasets: LLMs are trained on massive datasets, often comprising billions of words from diverse sources, which helps them generalize across various contexts.
Infrastructure: High-performance GPUs or TPUs are essential to manage extensive computations. Cloud platforms are commonly used to facilitate this process.
Fine-Tuning Large Language Models
Fine-tuning LLMs involves adjusting the pre-trained model to specific tasks or domains, which is typically less resource-intensive than training from scratch but still demanding:
Specific Domains: Fine-tuning allows LLMs to adapt to particular domains, improving performance on specialized tasks.
Cost and Time: Fine-tuning can still be costly and time-consuming but is more manageable compared to full training.
Example Code:
from transformers import GPT2Tokenizer, GPT2LMHeadModel, Trainer, TrainingArguments
# Load pre-trained model and tokenizer
model_name = 'gpt2'
tokenizer = GPT2Tokenizer.from_pretrained(model_name)
model = GPT2LMHeadModel.from_pretrained(model_name)
# Prepare dataset (assuming 'train_dataset' is already prepared)
training_args = TrainingArguments(
output_dir='./results',
num_train_epochs=3,
per_device_train_batch_size=4,
save_steps=10_000,
save_total_limit=2,
)
trainer = Trainer(
model=model,
args=training_args,
train_dataset=train_dataset,
)
# Fine-tune model
trainer.train()
Training Small Language Models (SLMs)
Training SLMs is more feasible for many organizations due to their lower resource requirements:
Time and Cost: Training SLMs can be completed in a shorter timeframe and at a lower cost.
Datasets: SLMs use smaller, often domain-specific datasets, which reduce training complexity and duration.
Infrastructure: Standard CPUs or lower-end GPUs are usually sufficient, making it accessible for smaller setups.
Fine-Tuning Small Language Models
Fine-tuning SLMs is relatively straightforward and cost-effective:
Efficiency: The process is faster and requires fewer resources, making it ideal for iterative improvements and specific task adaptation.
Specialization: Fine-tuning allows SLMs to excel in niche areas without the overhead of extensive retraining.
For a deeper dive into the training processes and best practices for AI models, check out Raga AI’s guide on fine-tuning LLM applications.
Next, we will compare the inference speed and deployment flexibility of LLMs and SLMs.
Inference Speed and Deployment Flexibility
When considering Large Language Models (LLMs) and Small Language Models (SLMs), understanding their inference speed and deployment flexibility significantly impacts the practical application and efficiency of the models.
Inference Speed
Inference speed refers to how quickly a model can generate predictions or responses after being deployed.
LLMs: Due to their large size and complexity, LLMs typically have slower inference speeds. They require substantial computational power, which can lead to latency issues, especially in real-time applications.
Example:
import torch
from transformers import GPT2Tokenizer, GPT2LMHeadModel
# Load pre-trained model and tokenizer
model_name = 'gpt2'
tokenizer = GPT2Tokenizer.from_pretrained(model_name)
model = GPT2LMHeadModel.from_pretrained(model_name)
# Encode input text
input_text = "What is the capital of France?"
input_ids = tokenizer.encode(input_text, return_tensors='pt')
# Measure inference time
import time
start_time = time.time()
output = model.generate(input_ids, max_length=50)
end_time = time.time()
# Calculate inference time
inference_time = end_time - start_time
print(f"Inference time: {inference_time:.2f} seconds")
SLMs: SLMs are designed for speed and efficiency. Their simpler architecture and smaller size enable them to deliver faster inference speeds, making them suitable for applications requiring quick responses.
Deployment Flexibility
Deployment flexibility is about how easily a model can be deployed across different platforms and environments.
LLMs: LLMs are best suited for cloud-based deployment due to their high computational needs. Cloud platforms can provide the necessary resources for running these models efficiently, but this limits their use in environments with restricted or no internet access.
Cloud Deployment:
High computational power
Suitable for large-scale applications
High operational costs
SLMs: SLMs excel in deployment flexibility. They can be deployed on various platforms, including local machines, edge devices, and mobile applications, due to their lower resource requirements. This makes them ideal for environments where quick and efficient processing is needed without relying heavily on cloud infrastructure.
Edge Deployment:
Lower computational power
Suitable for real-time, on-device applications
Cost-effective and accessible
For more on optimizing AI deployment, see Raga AI’s blog on AI-driven predictive analytics in retail inventory.
Comparison Table

Understanding these aspects helps in selecting the right model based on the specific needs and constraints of your application.
Next, let’s explore various use cases and applications for LLMs and SLMs.
Use Cases and Applications
The distinct characteristics of Large Language Models (LLMs) and Small Language Models (SLMs) make them suitable for different types of applications. Understanding these use cases can help you benefit each model's strengths for your specific needs.
Use Cases for Large Language Models (LLMs)
LLMs excel in tasks that require deep understanding, complex pattern recognition, and high accuracy. Here are some common applications:
Chatbots and Virtual Assistants: LLMs can handle sophisticated conversations, providing accurate and contextually relevant responses.
Content Generation: Ideal for creating articles, reports, and other text-based content that requires a nuanced understanding of language and context.
Translation Services: LLMs can accurately translate text between languages, capturing the nuances and subtleties of the original content.
Research and Analysis: Useful for extracting insights from large datasets, summarizing information, and generating comprehensive reports.
Example Code for Content Generation
Here's a simple example of using an LLM for generating content:
from transformers import GPT2Tokenizer, GPT2LMHeadModel
# Load pre-trained model and tokenizer
model_name = 'gpt2'
tokenizer = GPT2Tokenizer.from_pretrained(model_name)
model = GPT2LMHeadModel.from_pretrained(model_name)
# Encode input text
input_text = "Write an introduction for a blog about AI advancements."
input_ids = tokenizer.encode(input_text, return_tensors='pt')
# Generate text
output = model.generate(input_ids, max_length=100)
generated_text = tokenizer.decode(output[0], skip_special_tokens=True)
print(generated_text)
Use Cases for Small Language Models (SLMs)
SLMs are well-suited for applications where speed, efficiency, and resource constraints are critical. Here are some common applications:
Specialized Knowledge Tasks: Ideal for tasks requiring domain-specific knowledge, such as technical support or legal document analysis.
Real-Time Language Processing: Suitable for mobile apps, voice assistants, and IoT devices where quick responses are essential.
Voice Assistants: Efficient for running on devices with limited computational power, providing quick and accurate responses.
IoT Applications: Useful for real-time data processing and decision-making in edge computing environments.
Example Code for a Voice Assistant
Here's a simple example of using an SLM for a voice assistant application:
from transformers import DistilBertTokenizer, DistilBertForQuestionAnswering
import torch
# Load pre-trained model and tokenizer
model_name = 'distilbert-base-uncased'
tokenizer = DistilBertTokenizer.from_pretrained(model_name)
model = DistilBertForQuestionAnswering.from_pretrained(model_name)
# Sample question and context
question = "What is the capital of France?"
context = "France is a country in Europe. The capital of France is Paris."
# Encode input
inputs = tokenizer.encode_plus(question, context, return_tensors='pt')
# Get answer
with torch.no_grad():
outputs = model(**inputs)
answer_start = torch.argmax(outputs.start_logits)
answer_end = torch.argmax(outputs.end_logits) + 1
answer = tokenizer.convert_tokens_to_ids(inputs.input_ids[answer_start:answer_end])
answer_text = tokenizer.decode(answer)
print(answer_text)
For detailed insights on optimizing quality and performance in AI applications, check out Raga AI’s blog on optimizing quality and performance in LLM apps.
Next, we will discuss the factors influencing the choice between LLMs and SLMs.
Choosing Between LLMs and SLMs
Selecting the right language model for your needs involves considering various factors that align with your specific business applications, resource availability, and task complexity. Here’s a guide to help you make an informed choice between Large Language Models (LLMs) and Small Language Models (SLMs).
Factors Influencing the Choice
1. Specific Business Applications
LLMs: Suitable for applications requiring deep understanding and high accuracy, such as complex text generation, sophisticated chatbots, and detailed data analysis.
SLMs: Best for tasks needing speed and efficiency, such as real-time language processing, voice assistants, and domain-specific knowledge tasks.
2. Resource Availability
LLMs: Require substantial computational resources, including powerful GPUs and significant memory. Ideal for organizations with access to cloud infrastructure.
SLMs: More resource-efficient, capable of running on standard CPUs and edge devices. Suitable for environments with limited computational resources.
3. Task Complexity
LLMs: Excel in handling complex tasks that involve nuanced understanding and context, making them ideal for research, content generation, and translation.
SLMs: Effective for simpler, specialized tasks where quick and efficient processing is essential, such as technical support and IoT applications.
Balanced Approach
Starting with LLMs for Broad Applications: LLMs provide a comprehensive understanding of language and can be a good starting point for broad applications. They can handle diverse and complex tasks, offering versatility and high performance.
Optimizing with SLMs for Efficiency: Once the broader application requirements are understood, optimizing with SLMs can enhance efficiency. SLMs are faster and more cost-effective, making them ideal for specific tasks within the broader application scope.
Example Scenario
Imagine a customer service application:
LLM: Used to train an advanced chatbot that understands and generates responses across various topics, providing high-quality customer interactions.
SLM: Deployed on local devices to handle specific queries quickly and efficiently, ensuring real-time responses and improved user experience.
For a detailed exploration of AI models' performance in real-world applications, explore Raga AI’s blog on enhancing edge AI with RagaAI integration.
Verdict
Choosing between LLMs and SLMs depends on your specific needs and resources. By understanding their strengths and limitations, you can strategically deploy these models to achieve optimal performance and efficiency. This approach ensures you utilize the full potential of AI tailored to your organizational requirements.
Conclusion
Large Language Models (LLMs) and Small Language Models (SLMs) each offer unique strengths and are suited to different applications. LLMs excel in complex, context-rich tasks like advanced text generation and comprehensive data analysis, delivering high accuracy and performance.
In contrast, SLMs are designed for speed and efficiency, making them ideal for real-time applications and domain-specific tasks. Understanding these differences helps you make informed decisions based on your organizational needs and resource availability.
Raga AI stands at the forefront of these advancements, offering state-of-the-art solutions for AI testing, governance, and optimization. With tools like the RagaAI Catalyst, Raga AI Prism, and Governance Hub, Raga AI ensures the quality, reliability, and efficiency of your AI applications. Whether you're dealing with LLMs or SLMs, Raga AI provides comprehensive support to enhance your AI strategies and achieve your goals.
Explore how Raga AI can transform your AI initiatives by visiting our Raga AI platform. Discover the full potential of your AI projects with Raga AI today!
Artificial Intelligence has significantly transformed the way we interact with technology, and nowhere is this more evident than in the field of Natural Language Processing (NLP). From smart assistants to advanced text generation, NLP advancements have reshaped our digital experiences. Whether you're a data scientist, a mid-level manager, or an AI enthusiast, grasping the distinctions between LLMs and SLMs is crucial for making informed decisions.
In this article, you'll learn the basics of LLMs and SLMs, their differences and how to choose them for your AI needs. Now, let’s dive deeper into understanding Large Language Models (LLMs).
Understanding Large Language Models (LLMs)
Large Language Models (LLMs) have emerged as a cornerstone in the field of NLP, enabling a range of applications from chatbots to content generation. These models handle vast amounts of data, making them exceptionally powerful for understanding and generating human-like text. Let's break down what makes LLMs unique and explore their key characteristics.
Definition and Characteristics of LLMs
LLMs are neural networks that are trained on extensive datasets, allowing them to learn the nuances of human language. Here’s what defines them:
Massive Datasets: LLMs are trained on billions of words from diverse sources.
High Number of Parameters: They contain millions to billions of parameters, which are weights and biases that the model learns during training.
Complex Architectures: LLMs use advanced architectures like transformers to process and generate text efficiently.
Versatile Applications: They are used in various applications, including translation, summarization, and content generation.
Human-Like Text Generation: LLMs excel at producing coherent and contextually accurate human-like text.
Training Datasets and Parameter Scale
The effectiveness of LLMs is largely due to the size and quality of their training datasets. These models process a variety of texts, from books and articles to websites, helping them understand context and generate coherent text.
Datasets: LLMs train on a diverse range of text sources, including books, articles, websites, and social media posts. This variety ensures the models are exposed to different writing styles, contexts, and topics, enhancing their ability to understand and generate text across multiple domains. The inclusion of diverse datasets is crucial for the models to learn the nuances of human language and produce contextually relevant outputs.
Parameter Scale: LLMs have a parameter scale ranging from millions to billions of parameters. These parameters represent the weights and biases the model learns during training, allowing it to understand and generate text with high accuracy. The larger the number of parameters, the better the model can capture complex patterns and relationships within the data. This extensive parameter scale contributes to the model's capability to produce sophisticated and contextually appropriate text, making LLMs powerful tools for various natural language processing tasks.
Transformer Architecture
LLMs utilize the Transformer architecture, which consists of encoder and decoder components:
Encoder: The encoder processes the input text to understand its context. It breaks down the input into smaller components, analyzes each part, and captures the underlying meaning and relationships within the text. This process involves converting the input text into a series of numerical representations that encapsulate the context and nuances of the original text, making it easier for the model to understand and work with.
Decoder: The decoder generates the output text based on the processed input. Using the numerical representations created by the encoder, the decoder constructs coherent and contextually relevant text. It systematically assembles words and phrases to form meaningful sentences that reflect the input's original context and intent. This step is crucial for producing accurate and human-like responses or translations in various natural language processing applications.
Although keep in mind that not all LLMs have the encoder component. For example, LLMs like GPT-3 work only on decoder components.
Here's a simple example of how a Transformer might be coded in Python using the ‘transformers’ library:
from transformers import GPT2Tokenizer, GPT2LMHeadModel
# Load pre-trained model and tokenizer
model_name = 'gpt2'
tokenizer = GPT2Tokenizer.from_pretrained(model_name)
model = GPT2LMHeadModel.from_pretrained(model_name)
# Encode input text
input_text = "The future of AI is"
input_ids = tokenizer.encode(input_text, return_tensors='pt')
# Generate text
output = model.generate(input_ids, max_length=50, num_return_sequences=1)
# Decode output
generated_text = tokenizer.decode(output[0], skip_special_tokens=True)
print(generated_text)
Capabilities and Common Applications
LLMs excel in several areas, making them highly versatile:
Contextual Text Generation: They can generate text that is contextually relevant and coherent.
Multimodal Abilities: Capable of processing and generating text, images, and even audio.
Advantages of LLMs
LLMs offer several advantages, including:
Complex Pattern Recognition: They can identify and understand intricate patterns in data.
High Performance in Precision Tasks: Ideal for tasks requiring high accuracy and detailed understanding.
Ethical Considerations
While powerful, LLMs also pose ethical challenges:
Misinformation: They can generate convincing but false information.
Bias: They might perpetuate biases present in their training data.
Privacy Risks: Handling of sensitive data needs careful management.
Now that you have a solid understanding of Large Language Models, let's explore the characteristics and advantages of Small Language Models (SLMs).
For a deeper dive into enhancing reliability with guardrails for LLMs, check out Raga AI’s case study on safeguarding enterprise LLM applications.
Understanding Small Language Models (SLMs)
Small Language Models (SLMs) offer a more streamlined approach to NLP, focusing on efficiency and specific task performance. They handle less data and require fewer resources, making them an excellent choice for many applications. Let's explore what makes SLMs distinct and their key benefits.
Definition and Characteristics of SLMs
SLMs are neural networks trained on smaller datasets compared to LLMs, focusing on efficiency and specific applications. Here’s what defines them:
Smaller Datasets: SLMs are trained on millions to tens of millions of words, often sourced from specific domains or industries. This focused training allows them to perform well within particular contexts or specialized tasks. By concentrating on a narrower range of data, SLMs can quickly and effectively learn the relevant patterns and nuances necessary for their specific applications.
Fewer Parameters: SLMs contain significantly fewer parameters than LLMs, usually ranging from a few million to a few hundred million. This reduction in parameters makes SLMs less resource-intensive, allowing them to run efficiently on less powerful hardware. Despite having fewer parameters, these models can still deliver strong performance within their specialized domains by leveraging the high-quality, targeted datasets they are trained on.
Efficient Computation: Due to their smaller size, SLMs require less computational power and memory, making them suitable for deployment in environments with limited resources. This efficiency is particularly advantageous for applications that need to run on edge devices or mobile platforms where computational capacity is restricted.
Faster Training and Inference: The reduced complexity of SLMs leads to faster training times and quicker inference speeds. This speed allows for rapid development cycles and real-time application scenarios, where timely responses are critical. The ability to quickly train and deploy these models makes them an attractive choice for many practical applications.
Specific Applications: SLMs are often designed for specific applications where domain knowledge is paramount. Examples include medical diagnosis, financial forecasting, and customer service automation. By tailoring the model to a particular use case, SLMs can achieve high accuracy and reliability, making them valuable tools in specialized fields.
Training Datasets and Parameter Scale
SLMs are effective due to their focused training datasets and manageable parameter scales. These models prioritize relevance and specificity over sheer size.
Datasets: SLMs are often trained on domain-specific datasets, ensuring their relevance to particular tasks. These datasets are carefully curated to include texts from specialized areas such as medical records, financial reports, technical manuals, or customer service interactions. By focusing on domain-specific data, SLMs can develop a deep understanding of the specific language, terminology, and context required for their target applications. This targeted training helps the models perform accurately and effectively within their specialized areas, providing reliable and contextually appropriate outputs.
Parameter Scale: SLMs typically have a parameter scale that ranges from millions to tens of millions of parameters. This relatively smaller scale compared to LLMs makes SLMs lighter and faster, requiring less computational power and memory. The reduced number of parameters allows these models to be more efficient, enabling quicker training times and faster inference speeds. Despite their smaller size, SLMs maintain the ability to capture essential patterns and relationships within their specialized domains, delivering high-performance results for specific tasks without the need for extensive computational resources. This efficiency makes SLMs an ideal choice for applications where speed and resource management are crucial.
Simpler Architecture Compared to LLMs
SLMs employ a simpler architecture, which contributes to their efficiency: They use fewer layers and parameters, reducing computational requirements.
Advantages of SLMs
SLMs offer several advantages, including:
Speed: Faster training and inference times.
Memory and Resource Efficiency: Require less computational power and memory, making them suitable for edge devices.
Limitations of SLMs
Despite their benefits, SLMs have some limitations:
Scalability Issues: Struggle with larger, more complex datasets.
Lower Performance in Intricate Tasks: May not perform as well in tasks requiring deep understanding and context.
Example Code for a Simple SLM
Here's a simple example of an SLM coded in Python using the ‘transformers’ library:
from transformers import DistilBertTokenizer, DistilBertModel
# Load pre-trained model and tokenizer
model_name = 'distilbert-base-uncased'
tokenizer = DistilBertTokenizer.from_pretrained(model_name)
model = DistilBertModel.from_pretrained(model_name)
# Encode input text
input_text = "Efficient NLP with small models"
input_ids = tokenizer.encode(input_text, return_tensors='pt')
# Generate embeddings
outputs = model(input_ids)
embeddings = outputs.last_hidden_state
print(embeddings)
For a comprehensive guide on testing AI models like SLMs, explore Raga AI’s guide to evaluating LLM applications and enabling guardrails.
Understanding the characteristics and advantages of Small Language Models sets the stage for comparing them with Large Language Models. Let's now examine the key differences between LLMs and SLMs.
Key Differences Between LLMs and SLMs
Understanding the distinctions between Large Language Models (LLMs) and Small Language Models (SLMs) is essential for using their strengths effectively. Let's break down the key differences to help you determine which model suits your needs best.

For further insights on AI models' implementation and optimization, explore Raga AI’s blog on enhancing edge AI with RagaAI integration on NVIDIA Metropolis.
Next, we will explore the resource requirements of LLMs and SLMs.
Resource Requirements
When choosing between Large Language Models (LLMs) and Small Language Models (SLMs), it's crucial to consider the resource requirements. These factors play a significant role in determining the feasibility and efficiency of deploying these models.
Resource Requirements of LLMs
LLMs are known for their high computational and memory demands. Here’s a breakdown of what they typically require:
High Memory Consumption: LLMs need substantial RAM to store and process large amounts of data.
Computational Power: They require powerful GPUs or TPUs to handle the complex calculations involved in training and inference.
Energy Usage: The extensive computational processes lead to high energy consumption, impacting both cost and environmental footprint.
Resource Requirements of SLMs
SLMs are designed with efficiency in mind, making them more accessible for various applications. Here’s what they typically require:
Lower Memory Consumption: SLMs need less RAM, making them suitable for devices with limited memory.
Reduced Computational Power: They can run on standard CPUs and require fewer GPUs, making them more cost-effective.
Energy Efficiency: Due to their simpler architecture, SLMs consume less energy, which reduces operational costs and environmental impact.
Efficiency Techniques
SLMs often employ efficiency techniques to maximize their performance within resource constraints:
Knowledge Distillation: Transferring knowledge from a larger model to a smaller one to maintain performance while reducing size.
Quantization: Reducing the precision of the model’s weights to decrease memory and computational requirements without significantly impacting accuracy.
Here's an example of how quantization can be implemented in Python using the ‘transformers’ library:
from transformers import DistilBertModel, DistilBertTokenizer
from transformers import pipeline
# Load pre-trained model and tokenizer
model_name = 'distilbert-base-uncased'
tokenizer = DistilBertTokenizer.from_pretrained(model_name)
model = DistilBertModel.from_pretrained(model_name)
# Quantize the model
quantized_model = torch.quantization.quantize_dynamic(
model, {torch.nn.Linear}, dtype=torch.qint8
)
# Use the quantized model in a pipeline
nlp_pipeline = pipeline('feature-extraction', model=quantized_model, tokenizer=tokenizer)
output = nlp_pipeline("Efficient NLP with quantized models")
print(output)
For a detailed look at optimizing AI models with resource-efficient techniques, check out Raga AI’s blog on LLM evaluation.
Along with resource utilization, LLMs and SLMs vary in training and fine-tuning, too. Let’s see how.
Training and Fine-Tuning
Training and fine-tuning Large Language Models (LLMs) and Small Language Models (SLMs) involve different processes and resource investments. Understanding these differences can help you choose the right approach for your specific needs.
Training Large Language Models (LLMs)
Training LLMs is a resource-intensive process that requires substantial computational power and time. Here’s a closer look at what’s involved:
Time and Cost: Training an LLM from scratch can take weeks or months and requires significant financial investment due to the computational resources needed.
Datasets: LLMs are trained on massive datasets, often comprising billions of words from diverse sources, which helps them generalize across various contexts.
Infrastructure: High-performance GPUs or TPUs are essential to manage extensive computations. Cloud platforms are commonly used to facilitate this process.
Fine-Tuning Large Language Models
Fine-tuning LLMs involves adjusting the pre-trained model to specific tasks or domains, which is typically less resource-intensive than training from scratch but still demanding:
Specific Domains: Fine-tuning allows LLMs to adapt to particular domains, improving performance on specialized tasks.
Cost and Time: Fine-tuning can still be costly and time-consuming but is more manageable compared to full training.
Example Code:
from transformers import GPT2Tokenizer, GPT2LMHeadModel, Trainer, TrainingArguments
# Load pre-trained model and tokenizer
model_name = 'gpt2'
tokenizer = GPT2Tokenizer.from_pretrained(model_name)
model = GPT2LMHeadModel.from_pretrained(model_name)
# Prepare dataset (assuming 'train_dataset' is already prepared)
training_args = TrainingArguments(
output_dir='./results',
num_train_epochs=3,
per_device_train_batch_size=4,
save_steps=10_000,
save_total_limit=2,
)
trainer = Trainer(
model=model,
args=training_args,
train_dataset=train_dataset,
)
# Fine-tune model
trainer.train()
Training Small Language Models (SLMs)
Training SLMs is more feasible for many organizations due to their lower resource requirements:
Time and Cost: Training SLMs can be completed in a shorter timeframe and at a lower cost.
Datasets: SLMs use smaller, often domain-specific datasets, which reduce training complexity and duration.
Infrastructure: Standard CPUs or lower-end GPUs are usually sufficient, making it accessible for smaller setups.
Fine-Tuning Small Language Models
Fine-tuning SLMs is relatively straightforward and cost-effective:
Efficiency: The process is faster and requires fewer resources, making it ideal for iterative improvements and specific task adaptation.
Specialization: Fine-tuning allows SLMs to excel in niche areas without the overhead of extensive retraining.
For a deeper dive into the training processes and best practices for AI models, check out Raga AI’s guide on fine-tuning LLM applications.
Next, we will compare the inference speed and deployment flexibility of LLMs and SLMs.
Inference Speed and Deployment Flexibility
When considering Large Language Models (LLMs) and Small Language Models (SLMs), understanding their inference speed and deployment flexibility significantly impacts the practical application and efficiency of the models.
Inference Speed
Inference speed refers to how quickly a model can generate predictions or responses after being deployed.
LLMs: Due to their large size and complexity, LLMs typically have slower inference speeds. They require substantial computational power, which can lead to latency issues, especially in real-time applications.
Example:
import torch
from transformers import GPT2Tokenizer, GPT2LMHeadModel
# Load pre-trained model and tokenizer
model_name = 'gpt2'
tokenizer = GPT2Tokenizer.from_pretrained(model_name)
model = GPT2LMHeadModel.from_pretrained(model_name)
# Encode input text
input_text = "What is the capital of France?"
input_ids = tokenizer.encode(input_text, return_tensors='pt')
# Measure inference time
import time
start_time = time.time()
output = model.generate(input_ids, max_length=50)
end_time = time.time()
# Calculate inference time
inference_time = end_time - start_time
print(f"Inference time: {inference_time:.2f} seconds")
SLMs: SLMs are designed for speed and efficiency. Their simpler architecture and smaller size enable them to deliver faster inference speeds, making them suitable for applications requiring quick responses.
Deployment Flexibility
Deployment flexibility is about how easily a model can be deployed across different platforms and environments.
LLMs: LLMs are best suited for cloud-based deployment due to their high computational needs. Cloud platforms can provide the necessary resources for running these models efficiently, but this limits their use in environments with restricted or no internet access.
Cloud Deployment:
High computational power
Suitable for large-scale applications
High operational costs
SLMs: SLMs excel in deployment flexibility. They can be deployed on various platforms, including local machines, edge devices, and mobile applications, due to their lower resource requirements. This makes them ideal for environments where quick and efficient processing is needed without relying heavily on cloud infrastructure.
Edge Deployment:
Lower computational power
Suitable for real-time, on-device applications
Cost-effective and accessible
For more on optimizing AI deployment, see Raga AI’s blog on AI-driven predictive analytics in retail inventory.
Comparison Table

Understanding these aspects helps in selecting the right model based on the specific needs and constraints of your application.
Next, let’s explore various use cases and applications for LLMs and SLMs.
Use Cases and Applications
The distinct characteristics of Large Language Models (LLMs) and Small Language Models (SLMs) make them suitable for different types of applications. Understanding these use cases can help you benefit each model's strengths for your specific needs.
Use Cases for Large Language Models (LLMs)
LLMs excel in tasks that require deep understanding, complex pattern recognition, and high accuracy. Here are some common applications:
Chatbots and Virtual Assistants: LLMs can handle sophisticated conversations, providing accurate and contextually relevant responses.
Content Generation: Ideal for creating articles, reports, and other text-based content that requires a nuanced understanding of language and context.
Translation Services: LLMs can accurately translate text between languages, capturing the nuances and subtleties of the original content.
Research and Analysis: Useful for extracting insights from large datasets, summarizing information, and generating comprehensive reports.
Example Code for Content Generation
Here's a simple example of using an LLM for generating content:
from transformers import GPT2Tokenizer, GPT2LMHeadModel
# Load pre-trained model and tokenizer
model_name = 'gpt2'
tokenizer = GPT2Tokenizer.from_pretrained(model_name)
model = GPT2LMHeadModel.from_pretrained(model_name)
# Encode input text
input_text = "Write an introduction for a blog about AI advancements."
input_ids = tokenizer.encode(input_text, return_tensors='pt')
# Generate text
output = model.generate(input_ids, max_length=100)
generated_text = tokenizer.decode(output[0], skip_special_tokens=True)
print(generated_text)
Use Cases for Small Language Models (SLMs)
SLMs are well-suited for applications where speed, efficiency, and resource constraints are critical. Here are some common applications:
Specialized Knowledge Tasks: Ideal for tasks requiring domain-specific knowledge, such as technical support or legal document analysis.
Real-Time Language Processing: Suitable for mobile apps, voice assistants, and IoT devices where quick responses are essential.
Voice Assistants: Efficient for running on devices with limited computational power, providing quick and accurate responses.
IoT Applications: Useful for real-time data processing and decision-making in edge computing environments.
Example Code for a Voice Assistant
Here's a simple example of using an SLM for a voice assistant application:
from transformers import DistilBertTokenizer, DistilBertForQuestionAnswering
import torch
# Load pre-trained model and tokenizer
model_name = 'distilbert-base-uncased'
tokenizer = DistilBertTokenizer.from_pretrained(model_name)
model = DistilBertForQuestionAnswering.from_pretrained(model_name)
# Sample question and context
question = "What is the capital of France?"
context = "France is a country in Europe. The capital of France is Paris."
# Encode input
inputs = tokenizer.encode_plus(question, context, return_tensors='pt')
# Get answer
with torch.no_grad():
outputs = model(**inputs)
answer_start = torch.argmax(outputs.start_logits)
answer_end = torch.argmax(outputs.end_logits) + 1
answer = tokenizer.convert_tokens_to_ids(inputs.input_ids[answer_start:answer_end])
answer_text = tokenizer.decode(answer)
print(answer_text)
For detailed insights on optimizing quality and performance in AI applications, check out Raga AI’s blog on optimizing quality and performance in LLM apps.
Next, we will discuss the factors influencing the choice between LLMs and SLMs.
Choosing Between LLMs and SLMs
Selecting the right language model for your needs involves considering various factors that align with your specific business applications, resource availability, and task complexity. Here’s a guide to help you make an informed choice between Large Language Models (LLMs) and Small Language Models (SLMs).
Factors Influencing the Choice
1. Specific Business Applications
LLMs: Suitable for applications requiring deep understanding and high accuracy, such as complex text generation, sophisticated chatbots, and detailed data analysis.
SLMs: Best for tasks needing speed and efficiency, such as real-time language processing, voice assistants, and domain-specific knowledge tasks.
2. Resource Availability
LLMs: Require substantial computational resources, including powerful GPUs and significant memory. Ideal for organizations with access to cloud infrastructure.
SLMs: More resource-efficient, capable of running on standard CPUs and edge devices. Suitable for environments with limited computational resources.
3. Task Complexity
LLMs: Excel in handling complex tasks that involve nuanced understanding and context, making them ideal for research, content generation, and translation.
SLMs: Effective for simpler, specialized tasks where quick and efficient processing is essential, such as technical support and IoT applications.
Balanced Approach
Starting with LLMs for Broad Applications: LLMs provide a comprehensive understanding of language and can be a good starting point for broad applications. They can handle diverse and complex tasks, offering versatility and high performance.
Optimizing with SLMs for Efficiency: Once the broader application requirements are understood, optimizing with SLMs can enhance efficiency. SLMs are faster and more cost-effective, making them ideal for specific tasks within the broader application scope.
Example Scenario
Imagine a customer service application:
LLM: Used to train an advanced chatbot that understands and generates responses across various topics, providing high-quality customer interactions.
SLM: Deployed on local devices to handle specific queries quickly and efficiently, ensuring real-time responses and improved user experience.
For a detailed exploration of AI models' performance in real-world applications, explore Raga AI’s blog on enhancing edge AI with RagaAI integration.
Verdict
Choosing between LLMs and SLMs depends on your specific needs and resources. By understanding their strengths and limitations, you can strategically deploy these models to achieve optimal performance and efficiency. This approach ensures you utilize the full potential of AI tailored to your organizational requirements.
Conclusion
Large Language Models (LLMs) and Small Language Models (SLMs) each offer unique strengths and are suited to different applications. LLMs excel in complex, context-rich tasks like advanced text generation and comprehensive data analysis, delivering high accuracy and performance.
In contrast, SLMs are designed for speed and efficiency, making them ideal for real-time applications and domain-specific tasks. Understanding these differences helps you make informed decisions based on your organizational needs and resource availability.
Raga AI stands at the forefront of these advancements, offering state-of-the-art solutions for AI testing, governance, and optimization. With tools like the RagaAI Catalyst, Raga AI Prism, and Governance Hub, Raga AI ensures the quality, reliability, and efficiency of your AI applications. Whether you're dealing with LLMs or SLMs, Raga AI provides comprehensive support to enhance your AI strategies and achieve your goals.
Explore how Raga AI can transform your AI initiatives by visiting our Raga AI platform. Discover the full potential of your AI projects with Raga AI today!
Artificial Intelligence has significantly transformed the way we interact with technology, and nowhere is this more evident than in the field of Natural Language Processing (NLP). From smart assistants to advanced text generation, NLP advancements have reshaped our digital experiences. Whether you're a data scientist, a mid-level manager, or an AI enthusiast, grasping the distinctions between LLMs and SLMs is crucial for making informed decisions.
In this article, you'll learn the basics of LLMs and SLMs, their differences and how to choose them for your AI needs. Now, let’s dive deeper into understanding Large Language Models (LLMs).
Understanding Large Language Models (LLMs)
Large Language Models (LLMs) have emerged as a cornerstone in the field of NLP, enabling a range of applications from chatbots to content generation. These models handle vast amounts of data, making them exceptionally powerful for understanding and generating human-like text. Let's break down what makes LLMs unique and explore their key characteristics.
Definition and Characteristics of LLMs
LLMs are neural networks that are trained on extensive datasets, allowing them to learn the nuances of human language. Here’s what defines them:
Massive Datasets: LLMs are trained on billions of words from diverse sources.
High Number of Parameters: They contain millions to billions of parameters, which are weights and biases that the model learns during training.
Complex Architectures: LLMs use advanced architectures like transformers to process and generate text efficiently.
Versatile Applications: They are used in various applications, including translation, summarization, and content generation.
Human-Like Text Generation: LLMs excel at producing coherent and contextually accurate human-like text.
Training Datasets and Parameter Scale
The effectiveness of LLMs is largely due to the size and quality of their training datasets. These models process a variety of texts, from books and articles to websites, helping them understand context and generate coherent text.
Datasets: LLMs train on a diverse range of text sources, including books, articles, websites, and social media posts. This variety ensures the models are exposed to different writing styles, contexts, and topics, enhancing their ability to understand and generate text across multiple domains. The inclusion of diverse datasets is crucial for the models to learn the nuances of human language and produce contextually relevant outputs.
Parameter Scale: LLMs have a parameter scale ranging from millions to billions of parameters. These parameters represent the weights and biases the model learns during training, allowing it to understand and generate text with high accuracy. The larger the number of parameters, the better the model can capture complex patterns and relationships within the data. This extensive parameter scale contributes to the model's capability to produce sophisticated and contextually appropriate text, making LLMs powerful tools for various natural language processing tasks.
Transformer Architecture
LLMs utilize the Transformer architecture, which consists of encoder and decoder components:
Encoder: The encoder processes the input text to understand its context. It breaks down the input into smaller components, analyzes each part, and captures the underlying meaning and relationships within the text. This process involves converting the input text into a series of numerical representations that encapsulate the context and nuances of the original text, making it easier for the model to understand and work with.
Decoder: The decoder generates the output text based on the processed input. Using the numerical representations created by the encoder, the decoder constructs coherent and contextually relevant text. It systematically assembles words and phrases to form meaningful sentences that reflect the input's original context and intent. This step is crucial for producing accurate and human-like responses or translations in various natural language processing applications.
Although keep in mind that not all LLMs have the encoder component. For example, LLMs like GPT-3 work only on decoder components.
Here's a simple example of how a Transformer might be coded in Python using the ‘transformers’ library:
from transformers import GPT2Tokenizer, GPT2LMHeadModel
# Load pre-trained model and tokenizer
model_name = 'gpt2'
tokenizer = GPT2Tokenizer.from_pretrained(model_name)
model = GPT2LMHeadModel.from_pretrained(model_name)
# Encode input text
input_text = "The future of AI is"
input_ids = tokenizer.encode(input_text, return_tensors='pt')
# Generate text
output = model.generate(input_ids, max_length=50, num_return_sequences=1)
# Decode output
generated_text = tokenizer.decode(output[0], skip_special_tokens=True)
print(generated_text)
Capabilities and Common Applications
LLMs excel in several areas, making them highly versatile:
Contextual Text Generation: They can generate text that is contextually relevant and coherent.
Multimodal Abilities: Capable of processing and generating text, images, and even audio.
Advantages of LLMs
LLMs offer several advantages, including:
Complex Pattern Recognition: They can identify and understand intricate patterns in data.
High Performance in Precision Tasks: Ideal for tasks requiring high accuracy and detailed understanding.
Ethical Considerations
While powerful, LLMs also pose ethical challenges:
Misinformation: They can generate convincing but false information.
Bias: They might perpetuate biases present in their training data.
Privacy Risks: Handling of sensitive data needs careful management.
Now that you have a solid understanding of Large Language Models, let's explore the characteristics and advantages of Small Language Models (SLMs).
For a deeper dive into enhancing reliability with guardrails for LLMs, check out Raga AI’s case study on safeguarding enterprise LLM applications.
Understanding Small Language Models (SLMs)
Small Language Models (SLMs) offer a more streamlined approach to NLP, focusing on efficiency and specific task performance. They handle less data and require fewer resources, making them an excellent choice for many applications. Let's explore what makes SLMs distinct and their key benefits.
Definition and Characteristics of SLMs
SLMs are neural networks trained on smaller datasets compared to LLMs, focusing on efficiency and specific applications. Here’s what defines them:
Smaller Datasets: SLMs are trained on millions to tens of millions of words, often sourced from specific domains or industries. This focused training allows them to perform well within particular contexts or specialized tasks. By concentrating on a narrower range of data, SLMs can quickly and effectively learn the relevant patterns and nuances necessary for their specific applications.
Fewer Parameters: SLMs contain significantly fewer parameters than LLMs, usually ranging from a few million to a few hundred million. This reduction in parameters makes SLMs less resource-intensive, allowing them to run efficiently on less powerful hardware. Despite having fewer parameters, these models can still deliver strong performance within their specialized domains by leveraging the high-quality, targeted datasets they are trained on.
Efficient Computation: Due to their smaller size, SLMs require less computational power and memory, making them suitable for deployment in environments with limited resources. This efficiency is particularly advantageous for applications that need to run on edge devices or mobile platforms where computational capacity is restricted.
Faster Training and Inference: The reduced complexity of SLMs leads to faster training times and quicker inference speeds. This speed allows for rapid development cycles and real-time application scenarios, where timely responses are critical. The ability to quickly train and deploy these models makes them an attractive choice for many practical applications.
Specific Applications: SLMs are often designed for specific applications where domain knowledge is paramount. Examples include medical diagnosis, financial forecasting, and customer service automation. By tailoring the model to a particular use case, SLMs can achieve high accuracy and reliability, making them valuable tools in specialized fields.
Training Datasets and Parameter Scale
SLMs are effective due to their focused training datasets and manageable parameter scales. These models prioritize relevance and specificity over sheer size.
Datasets: SLMs are often trained on domain-specific datasets, ensuring their relevance to particular tasks. These datasets are carefully curated to include texts from specialized areas such as medical records, financial reports, technical manuals, or customer service interactions. By focusing on domain-specific data, SLMs can develop a deep understanding of the specific language, terminology, and context required for their target applications. This targeted training helps the models perform accurately and effectively within their specialized areas, providing reliable and contextually appropriate outputs.
Parameter Scale: SLMs typically have a parameter scale that ranges from millions to tens of millions of parameters. This relatively smaller scale compared to LLMs makes SLMs lighter and faster, requiring less computational power and memory. The reduced number of parameters allows these models to be more efficient, enabling quicker training times and faster inference speeds. Despite their smaller size, SLMs maintain the ability to capture essential patterns and relationships within their specialized domains, delivering high-performance results for specific tasks without the need for extensive computational resources. This efficiency makes SLMs an ideal choice for applications where speed and resource management are crucial.
Simpler Architecture Compared to LLMs
SLMs employ a simpler architecture, which contributes to their efficiency: They use fewer layers and parameters, reducing computational requirements.
Advantages of SLMs
SLMs offer several advantages, including:
Speed: Faster training and inference times.
Memory and Resource Efficiency: Require less computational power and memory, making them suitable for edge devices.
Limitations of SLMs
Despite their benefits, SLMs have some limitations:
Scalability Issues: Struggle with larger, more complex datasets.
Lower Performance in Intricate Tasks: May not perform as well in tasks requiring deep understanding and context.
Example Code for a Simple SLM
Here's a simple example of an SLM coded in Python using the ‘transformers’ library:
from transformers import DistilBertTokenizer, DistilBertModel
# Load pre-trained model and tokenizer
model_name = 'distilbert-base-uncased'
tokenizer = DistilBertTokenizer.from_pretrained(model_name)
model = DistilBertModel.from_pretrained(model_name)
# Encode input text
input_text = "Efficient NLP with small models"
input_ids = tokenizer.encode(input_text, return_tensors='pt')
# Generate embeddings
outputs = model(input_ids)
embeddings = outputs.last_hidden_state
print(embeddings)
For a comprehensive guide on testing AI models like SLMs, explore Raga AI’s guide to evaluating LLM applications and enabling guardrails.
Understanding the characteristics and advantages of Small Language Models sets the stage for comparing them with Large Language Models. Let's now examine the key differences between LLMs and SLMs.
Key Differences Between LLMs and SLMs
Understanding the distinctions between Large Language Models (LLMs) and Small Language Models (SLMs) is essential for using their strengths effectively. Let's break down the key differences to help you determine which model suits your needs best.

For further insights on AI models' implementation and optimization, explore Raga AI’s blog on enhancing edge AI with RagaAI integration on NVIDIA Metropolis.
Next, we will explore the resource requirements of LLMs and SLMs.
Resource Requirements
When choosing between Large Language Models (LLMs) and Small Language Models (SLMs), it's crucial to consider the resource requirements. These factors play a significant role in determining the feasibility and efficiency of deploying these models.
Resource Requirements of LLMs
LLMs are known for their high computational and memory demands. Here’s a breakdown of what they typically require:
High Memory Consumption: LLMs need substantial RAM to store and process large amounts of data.
Computational Power: They require powerful GPUs or TPUs to handle the complex calculations involved in training and inference.
Energy Usage: The extensive computational processes lead to high energy consumption, impacting both cost and environmental footprint.
Resource Requirements of SLMs
SLMs are designed with efficiency in mind, making them more accessible for various applications. Here’s what they typically require:
Lower Memory Consumption: SLMs need less RAM, making them suitable for devices with limited memory.
Reduced Computational Power: They can run on standard CPUs and require fewer GPUs, making them more cost-effective.
Energy Efficiency: Due to their simpler architecture, SLMs consume less energy, which reduces operational costs and environmental impact.
Efficiency Techniques
SLMs often employ efficiency techniques to maximize their performance within resource constraints:
Knowledge Distillation: Transferring knowledge from a larger model to a smaller one to maintain performance while reducing size.
Quantization: Reducing the precision of the model’s weights to decrease memory and computational requirements without significantly impacting accuracy.
Here's an example of how quantization can be implemented in Python using the ‘transformers’ library:
from transformers import DistilBertModel, DistilBertTokenizer
from transformers import pipeline
# Load pre-trained model and tokenizer
model_name = 'distilbert-base-uncased'
tokenizer = DistilBertTokenizer.from_pretrained(model_name)
model = DistilBertModel.from_pretrained(model_name)
# Quantize the model
quantized_model = torch.quantization.quantize_dynamic(
model, {torch.nn.Linear}, dtype=torch.qint8
)
# Use the quantized model in a pipeline
nlp_pipeline = pipeline('feature-extraction', model=quantized_model, tokenizer=tokenizer)
output = nlp_pipeline("Efficient NLP with quantized models")
print(output)
For a detailed look at optimizing AI models with resource-efficient techniques, check out Raga AI’s blog on LLM evaluation.
Along with resource utilization, LLMs and SLMs vary in training and fine-tuning, too. Let’s see how.
Training and Fine-Tuning
Training and fine-tuning Large Language Models (LLMs) and Small Language Models (SLMs) involve different processes and resource investments. Understanding these differences can help you choose the right approach for your specific needs.
Training Large Language Models (LLMs)
Training LLMs is a resource-intensive process that requires substantial computational power and time. Here’s a closer look at what’s involved:
Time and Cost: Training an LLM from scratch can take weeks or months and requires significant financial investment due to the computational resources needed.
Datasets: LLMs are trained on massive datasets, often comprising billions of words from diverse sources, which helps them generalize across various contexts.
Infrastructure: High-performance GPUs or TPUs are essential to manage extensive computations. Cloud platforms are commonly used to facilitate this process.
Fine-Tuning Large Language Models
Fine-tuning LLMs involves adjusting the pre-trained model to specific tasks or domains, which is typically less resource-intensive than training from scratch but still demanding:
Specific Domains: Fine-tuning allows LLMs to adapt to particular domains, improving performance on specialized tasks.
Cost and Time: Fine-tuning can still be costly and time-consuming but is more manageable compared to full training.
Example Code:
from transformers import GPT2Tokenizer, GPT2LMHeadModel, Trainer, TrainingArguments
# Load pre-trained model and tokenizer
model_name = 'gpt2'
tokenizer = GPT2Tokenizer.from_pretrained(model_name)
model = GPT2LMHeadModel.from_pretrained(model_name)
# Prepare dataset (assuming 'train_dataset' is already prepared)
training_args = TrainingArguments(
output_dir='./results',
num_train_epochs=3,
per_device_train_batch_size=4,
save_steps=10_000,
save_total_limit=2,
)
trainer = Trainer(
model=model,
args=training_args,
train_dataset=train_dataset,
)
# Fine-tune model
trainer.train()
Training Small Language Models (SLMs)
Training SLMs is more feasible for many organizations due to their lower resource requirements:
Time and Cost: Training SLMs can be completed in a shorter timeframe and at a lower cost.
Datasets: SLMs use smaller, often domain-specific datasets, which reduce training complexity and duration.
Infrastructure: Standard CPUs or lower-end GPUs are usually sufficient, making it accessible for smaller setups.
Fine-Tuning Small Language Models
Fine-tuning SLMs is relatively straightforward and cost-effective:
Efficiency: The process is faster and requires fewer resources, making it ideal for iterative improvements and specific task adaptation.
Specialization: Fine-tuning allows SLMs to excel in niche areas without the overhead of extensive retraining.
For a deeper dive into the training processes and best practices for AI models, check out Raga AI’s guide on fine-tuning LLM applications.
Next, we will compare the inference speed and deployment flexibility of LLMs and SLMs.
Inference Speed and Deployment Flexibility
When considering Large Language Models (LLMs) and Small Language Models (SLMs), understanding their inference speed and deployment flexibility significantly impacts the practical application and efficiency of the models.
Inference Speed
Inference speed refers to how quickly a model can generate predictions or responses after being deployed.
LLMs: Due to their large size and complexity, LLMs typically have slower inference speeds. They require substantial computational power, which can lead to latency issues, especially in real-time applications.
Example:
import torch
from transformers import GPT2Tokenizer, GPT2LMHeadModel
# Load pre-trained model and tokenizer
model_name = 'gpt2'
tokenizer = GPT2Tokenizer.from_pretrained(model_name)
model = GPT2LMHeadModel.from_pretrained(model_name)
# Encode input text
input_text = "What is the capital of France?"
input_ids = tokenizer.encode(input_text, return_tensors='pt')
# Measure inference time
import time
start_time = time.time()
output = model.generate(input_ids, max_length=50)
end_time = time.time()
# Calculate inference time
inference_time = end_time - start_time
print(f"Inference time: {inference_time:.2f} seconds")
SLMs: SLMs are designed for speed and efficiency. Their simpler architecture and smaller size enable them to deliver faster inference speeds, making them suitable for applications requiring quick responses.
Deployment Flexibility
Deployment flexibility is about how easily a model can be deployed across different platforms and environments.
LLMs: LLMs are best suited for cloud-based deployment due to their high computational needs. Cloud platforms can provide the necessary resources for running these models efficiently, but this limits their use in environments with restricted or no internet access.
Cloud Deployment:
High computational power
Suitable for large-scale applications
High operational costs
SLMs: SLMs excel in deployment flexibility. They can be deployed on various platforms, including local machines, edge devices, and mobile applications, due to their lower resource requirements. This makes them ideal for environments where quick and efficient processing is needed without relying heavily on cloud infrastructure.
Edge Deployment:
Lower computational power
Suitable for real-time, on-device applications
Cost-effective and accessible
For more on optimizing AI deployment, see Raga AI’s blog on AI-driven predictive analytics in retail inventory.
Comparison Table

Understanding these aspects helps in selecting the right model based on the specific needs and constraints of your application.
Next, let’s explore various use cases and applications for LLMs and SLMs.
Use Cases and Applications
The distinct characteristics of Large Language Models (LLMs) and Small Language Models (SLMs) make them suitable for different types of applications. Understanding these use cases can help you benefit each model's strengths for your specific needs.
Use Cases for Large Language Models (LLMs)
LLMs excel in tasks that require deep understanding, complex pattern recognition, and high accuracy. Here are some common applications:
Chatbots and Virtual Assistants: LLMs can handle sophisticated conversations, providing accurate and contextually relevant responses.
Content Generation: Ideal for creating articles, reports, and other text-based content that requires a nuanced understanding of language and context.
Translation Services: LLMs can accurately translate text between languages, capturing the nuances and subtleties of the original content.
Research and Analysis: Useful for extracting insights from large datasets, summarizing information, and generating comprehensive reports.
Example Code for Content Generation
Here's a simple example of using an LLM for generating content:
from transformers import GPT2Tokenizer, GPT2LMHeadModel
# Load pre-trained model and tokenizer
model_name = 'gpt2'
tokenizer = GPT2Tokenizer.from_pretrained(model_name)
model = GPT2LMHeadModel.from_pretrained(model_name)
# Encode input text
input_text = "Write an introduction for a blog about AI advancements."
input_ids = tokenizer.encode(input_text, return_tensors='pt')
# Generate text
output = model.generate(input_ids, max_length=100)
generated_text = tokenizer.decode(output[0], skip_special_tokens=True)
print(generated_text)
Use Cases for Small Language Models (SLMs)
SLMs are well-suited for applications where speed, efficiency, and resource constraints are critical. Here are some common applications:
Specialized Knowledge Tasks: Ideal for tasks requiring domain-specific knowledge, such as technical support or legal document analysis.
Real-Time Language Processing: Suitable for mobile apps, voice assistants, and IoT devices where quick responses are essential.
Voice Assistants: Efficient for running on devices with limited computational power, providing quick and accurate responses.
IoT Applications: Useful for real-time data processing and decision-making in edge computing environments.
Example Code for a Voice Assistant
Here's a simple example of using an SLM for a voice assistant application:
from transformers import DistilBertTokenizer, DistilBertForQuestionAnswering
import torch
# Load pre-trained model and tokenizer
model_name = 'distilbert-base-uncased'
tokenizer = DistilBertTokenizer.from_pretrained(model_name)
model = DistilBertForQuestionAnswering.from_pretrained(model_name)
# Sample question and context
question = "What is the capital of France?"
context = "France is a country in Europe. The capital of France is Paris."
# Encode input
inputs = tokenizer.encode_plus(question, context, return_tensors='pt')
# Get answer
with torch.no_grad():
outputs = model(**inputs)
answer_start = torch.argmax(outputs.start_logits)
answer_end = torch.argmax(outputs.end_logits) + 1
answer = tokenizer.convert_tokens_to_ids(inputs.input_ids[answer_start:answer_end])
answer_text = tokenizer.decode(answer)
print(answer_text)
For detailed insights on optimizing quality and performance in AI applications, check out Raga AI’s blog on optimizing quality and performance in LLM apps.
Next, we will discuss the factors influencing the choice between LLMs and SLMs.
Choosing Between LLMs and SLMs
Selecting the right language model for your needs involves considering various factors that align with your specific business applications, resource availability, and task complexity. Here’s a guide to help you make an informed choice between Large Language Models (LLMs) and Small Language Models (SLMs).
Factors Influencing the Choice
1. Specific Business Applications
LLMs: Suitable for applications requiring deep understanding and high accuracy, such as complex text generation, sophisticated chatbots, and detailed data analysis.
SLMs: Best for tasks needing speed and efficiency, such as real-time language processing, voice assistants, and domain-specific knowledge tasks.
2. Resource Availability
LLMs: Require substantial computational resources, including powerful GPUs and significant memory. Ideal for organizations with access to cloud infrastructure.
SLMs: More resource-efficient, capable of running on standard CPUs and edge devices. Suitable for environments with limited computational resources.
3. Task Complexity
LLMs: Excel in handling complex tasks that involve nuanced understanding and context, making them ideal for research, content generation, and translation.
SLMs: Effective for simpler, specialized tasks where quick and efficient processing is essential, such as technical support and IoT applications.
Balanced Approach
Starting with LLMs for Broad Applications: LLMs provide a comprehensive understanding of language and can be a good starting point for broad applications. They can handle diverse and complex tasks, offering versatility and high performance.
Optimizing with SLMs for Efficiency: Once the broader application requirements are understood, optimizing with SLMs can enhance efficiency. SLMs are faster and more cost-effective, making them ideal for specific tasks within the broader application scope.
Example Scenario
Imagine a customer service application:
LLM: Used to train an advanced chatbot that understands and generates responses across various topics, providing high-quality customer interactions.
SLM: Deployed on local devices to handle specific queries quickly and efficiently, ensuring real-time responses and improved user experience.
For a detailed exploration of AI models' performance in real-world applications, explore Raga AI’s blog on enhancing edge AI with RagaAI integration.
Verdict
Choosing between LLMs and SLMs depends on your specific needs and resources. By understanding their strengths and limitations, you can strategically deploy these models to achieve optimal performance and efficiency. This approach ensures you utilize the full potential of AI tailored to your organizational requirements.
Conclusion
Large Language Models (LLMs) and Small Language Models (SLMs) each offer unique strengths and are suited to different applications. LLMs excel in complex, context-rich tasks like advanced text generation and comprehensive data analysis, delivering high accuracy and performance.
In contrast, SLMs are designed for speed and efficiency, making them ideal for real-time applications and domain-specific tasks. Understanding these differences helps you make informed decisions based on your organizational needs and resource availability.
Raga AI stands at the forefront of these advancements, offering state-of-the-art solutions for AI testing, governance, and optimization. With tools like the RagaAI Catalyst, Raga AI Prism, and Governance Hub, Raga AI ensures the quality, reliability, and efficiency of your AI applications. Whether you're dealing with LLMs or SLMs, Raga AI provides comprehensive support to enhance your AI strategies and achieve your goals.
Explore how Raga AI can transform your AI initiatives by visiting our Raga AI platform. Discover the full potential of your AI projects with Raga AI today!
Artificial Intelligence has significantly transformed the way we interact with technology, and nowhere is this more evident than in the field of Natural Language Processing (NLP). From smart assistants to advanced text generation, NLP advancements have reshaped our digital experiences. Whether you're a data scientist, a mid-level manager, or an AI enthusiast, grasping the distinctions between LLMs and SLMs is crucial for making informed decisions.
In this article, you'll learn the basics of LLMs and SLMs, their differences and how to choose them for your AI needs. Now, let’s dive deeper into understanding Large Language Models (LLMs).
Understanding Large Language Models (LLMs)
Large Language Models (LLMs) have emerged as a cornerstone in the field of NLP, enabling a range of applications from chatbots to content generation. These models handle vast amounts of data, making them exceptionally powerful for understanding and generating human-like text. Let's break down what makes LLMs unique and explore their key characteristics.
Definition and Characteristics of LLMs
LLMs are neural networks that are trained on extensive datasets, allowing them to learn the nuances of human language. Here’s what defines them:
Massive Datasets: LLMs are trained on billions of words from diverse sources.
High Number of Parameters: They contain millions to billions of parameters, which are weights and biases that the model learns during training.
Complex Architectures: LLMs use advanced architectures like transformers to process and generate text efficiently.
Versatile Applications: They are used in various applications, including translation, summarization, and content generation.
Human-Like Text Generation: LLMs excel at producing coherent and contextually accurate human-like text.
Training Datasets and Parameter Scale
The effectiveness of LLMs is largely due to the size and quality of their training datasets. These models process a variety of texts, from books and articles to websites, helping them understand context and generate coherent text.
Datasets: LLMs train on a diverse range of text sources, including books, articles, websites, and social media posts. This variety ensures the models are exposed to different writing styles, contexts, and topics, enhancing their ability to understand and generate text across multiple domains. The inclusion of diverse datasets is crucial for the models to learn the nuances of human language and produce contextually relevant outputs.
Parameter Scale: LLMs have a parameter scale ranging from millions to billions of parameters. These parameters represent the weights and biases the model learns during training, allowing it to understand and generate text with high accuracy. The larger the number of parameters, the better the model can capture complex patterns and relationships within the data. This extensive parameter scale contributes to the model's capability to produce sophisticated and contextually appropriate text, making LLMs powerful tools for various natural language processing tasks.
Transformer Architecture
LLMs utilize the Transformer architecture, which consists of encoder and decoder components:
Encoder: The encoder processes the input text to understand its context. It breaks down the input into smaller components, analyzes each part, and captures the underlying meaning and relationships within the text. This process involves converting the input text into a series of numerical representations that encapsulate the context and nuances of the original text, making it easier for the model to understand and work with.
Decoder: The decoder generates the output text based on the processed input. Using the numerical representations created by the encoder, the decoder constructs coherent and contextually relevant text. It systematically assembles words and phrases to form meaningful sentences that reflect the input's original context and intent. This step is crucial for producing accurate and human-like responses or translations in various natural language processing applications.
Although keep in mind that not all LLMs have the encoder component. For example, LLMs like GPT-3 work only on decoder components.
Here's a simple example of how a Transformer might be coded in Python using the ‘transformers’ library:
from transformers import GPT2Tokenizer, GPT2LMHeadModel
# Load pre-trained model and tokenizer
model_name = 'gpt2'
tokenizer = GPT2Tokenizer.from_pretrained(model_name)
model = GPT2LMHeadModel.from_pretrained(model_name)
# Encode input text
input_text = "The future of AI is"
input_ids = tokenizer.encode(input_text, return_tensors='pt')
# Generate text
output = model.generate(input_ids, max_length=50, num_return_sequences=1)
# Decode output
generated_text = tokenizer.decode(output[0], skip_special_tokens=True)
print(generated_text)
Capabilities and Common Applications
LLMs excel in several areas, making them highly versatile:
Contextual Text Generation: They can generate text that is contextually relevant and coherent.
Multimodal Abilities: Capable of processing and generating text, images, and even audio.
Advantages of LLMs
LLMs offer several advantages, including:
Complex Pattern Recognition: They can identify and understand intricate patterns in data.
High Performance in Precision Tasks: Ideal for tasks requiring high accuracy and detailed understanding.
Ethical Considerations
While powerful, LLMs also pose ethical challenges:
Misinformation: They can generate convincing but false information.
Bias: They might perpetuate biases present in their training data.
Privacy Risks: Handling of sensitive data needs careful management.
Now that you have a solid understanding of Large Language Models, let's explore the characteristics and advantages of Small Language Models (SLMs).
For a deeper dive into enhancing reliability with guardrails for LLMs, check out Raga AI’s case study on safeguarding enterprise LLM applications.
Understanding Small Language Models (SLMs)
Small Language Models (SLMs) offer a more streamlined approach to NLP, focusing on efficiency and specific task performance. They handle less data and require fewer resources, making them an excellent choice for many applications. Let's explore what makes SLMs distinct and their key benefits.
Definition and Characteristics of SLMs
SLMs are neural networks trained on smaller datasets compared to LLMs, focusing on efficiency and specific applications. Here’s what defines them:
Smaller Datasets: SLMs are trained on millions to tens of millions of words, often sourced from specific domains or industries. This focused training allows them to perform well within particular contexts or specialized tasks. By concentrating on a narrower range of data, SLMs can quickly and effectively learn the relevant patterns and nuances necessary for their specific applications.
Fewer Parameters: SLMs contain significantly fewer parameters than LLMs, usually ranging from a few million to a few hundred million. This reduction in parameters makes SLMs less resource-intensive, allowing them to run efficiently on less powerful hardware. Despite having fewer parameters, these models can still deliver strong performance within their specialized domains by leveraging the high-quality, targeted datasets they are trained on.
Efficient Computation: Due to their smaller size, SLMs require less computational power and memory, making them suitable for deployment in environments with limited resources. This efficiency is particularly advantageous for applications that need to run on edge devices or mobile platforms where computational capacity is restricted.
Faster Training and Inference: The reduced complexity of SLMs leads to faster training times and quicker inference speeds. This speed allows for rapid development cycles and real-time application scenarios, where timely responses are critical. The ability to quickly train and deploy these models makes them an attractive choice for many practical applications.
Specific Applications: SLMs are often designed for specific applications where domain knowledge is paramount. Examples include medical diagnosis, financial forecasting, and customer service automation. By tailoring the model to a particular use case, SLMs can achieve high accuracy and reliability, making them valuable tools in specialized fields.
Training Datasets and Parameter Scale
SLMs are effective due to their focused training datasets and manageable parameter scales. These models prioritize relevance and specificity over sheer size.
Datasets: SLMs are often trained on domain-specific datasets, ensuring their relevance to particular tasks. These datasets are carefully curated to include texts from specialized areas such as medical records, financial reports, technical manuals, or customer service interactions. By focusing on domain-specific data, SLMs can develop a deep understanding of the specific language, terminology, and context required for their target applications. This targeted training helps the models perform accurately and effectively within their specialized areas, providing reliable and contextually appropriate outputs.
Parameter Scale: SLMs typically have a parameter scale that ranges from millions to tens of millions of parameters. This relatively smaller scale compared to LLMs makes SLMs lighter and faster, requiring less computational power and memory. The reduced number of parameters allows these models to be more efficient, enabling quicker training times and faster inference speeds. Despite their smaller size, SLMs maintain the ability to capture essential patterns and relationships within their specialized domains, delivering high-performance results for specific tasks without the need for extensive computational resources. This efficiency makes SLMs an ideal choice for applications where speed and resource management are crucial.
Simpler Architecture Compared to LLMs
SLMs employ a simpler architecture, which contributes to their efficiency: They use fewer layers and parameters, reducing computational requirements.
Advantages of SLMs
SLMs offer several advantages, including:
Speed: Faster training and inference times.
Memory and Resource Efficiency: Require less computational power and memory, making them suitable for edge devices.
Limitations of SLMs
Despite their benefits, SLMs have some limitations:
Scalability Issues: Struggle with larger, more complex datasets.
Lower Performance in Intricate Tasks: May not perform as well in tasks requiring deep understanding and context.
Example Code for a Simple SLM
Here's a simple example of an SLM coded in Python using the ‘transformers’ library:
from transformers import DistilBertTokenizer, DistilBertModel
# Load pre-trained model and tokenizer
model_name = 'distilbert-base-uncased'
tokenizer = DistilBertTokenizer.from_pretrained(model_name)
model = DistilBertModel.from_pretrained(model_name)
# Encode input text
input_text = "Efficient NLP with small models"
input_ids = tokenizer.encode(input_text, return_tensors='pt')
# Generate embeddings
outputs = model(input_ids)
embeddings = outputs.last_hidden_state
print(embeddings)
For a comprehensive guide on testing AI models like SLMs, explore Raga AI’s guide to evaluating LLM applications and enabling guardrails.
Understanding the characteristics and advantages of Small Language Models sets the stage for comparing them with Large Language Models. Let's now examine the key differences between LLMs and SLMs.
Key Differences Between LLMs and SLMs
Understanding the distinctions between Large Language Models (LLMs) and Small Language Models (SLMs) is essential for using their strengths effectively. Let's break down the key differences to help you determine which model suits your needs best.

For further insights on AI models' implementation and optimization, explore Raga AI’s blog on enhancing edge AI with RagaAI integration on NVIDIA Metropolis.
Next, we will explore the resource requirements of LLMs and SLMs.
Resource Requirements
When choosing between Large Language Models (LLMs) and Small Language Models (SLMs), it's crucial to consider the resource requirements. These factors play a significant role in determining the feasibility and efficiency of deploying these models.
Resource Requirements of LLMs
LLMs are known for their high computational and memory demands. Here’s a breakdown of what they typically require:
High Memory Consumption: LLMs need substantial RAM to store and process large amounts of data.
Computational Power: They require powerful GPUs or TPUs to handle the complex calculations involved in training and inference.
Energy Usage: The extensive computational processes lead to high energy consumption, impacting both cost and environmental footprint.
Resource Requirements of SLMs
SLMs are designed with efficiency in mind, making them more accessible for various applications. Here’s what they typically require:
Lower Memory Consumption: SLMs need less RAM, making them suitable for devices with limited memory.
Reduced Computational Power: They can run on standard CPUs and require fewer GPUs, making them more cost-effective.
Energy Efficiency: Due to their simpler architecture, SLMs consume less energy, which reduces operational costs and environmental impact.
Efficiency Techniques
SLMs often employ efficiency techniques to maximize their performance within resource constraints:
Knowledge Distillation: Transferring knowledge from a larger model to a smaller one to maintain performance while reducing size.
Quantization: Reducing the precision of the model’s weights to decrease memory and computational requirements without significantly impacting accuracy.
Here's an example of how quantization can be implemented in Python using the ‘transformers’ library:
from transformers import DistilBertModel, DistilBertTokenizer
from transformers import pipeline
# Load pre-trained model and tokenizer
model_name = 'distilbert-base-uncased'
tokenizer = DistilBertTokenizer.from_pretrained(model_name)
model = DistilBertModel.from_pretrained(model_name)
# Quantize the model
quantized_model = torch.quantization.quantize_dynamic(
model, {torch.nn.Linear}, dtype=torch.qint8
)
# Use the quantized model in a pipeline
nlp_pipeline = pipeline('feature-extraction', model=quantized_model, tokenizer=tokenizer)
output = nlp_pipeline("Efficient NLP with quantized models")
print(output)
For a detailed look at optimizing AI models with resource-efficient techniques, check out Raga AI’s blog on LLM evaluation.
Along with resource utilization, LLMs and SLMs vary in training and fine-tuning, too. Let’s see how.
Training and Fine-Tuning
Training and fine-tuning Large Language Models (LLMs) and Small Language Models (SLMs) involve different processes and resource investments. Understanding these differences can help you choose the right approach for your specific needs.
Training Large Language Models (LLMs)
Training LLMs is a resource-intensive process that requires substantial computational power and time. Here’s a closer look at what’s involved:
Time and Cost: Training an LLM from scratch can take weeks or months and requires significant financial investment due to the computational resources needed.
Datasets: LLMs are trained on massive datasets, often comprising billions of words from diverse sources, which helps them generalize across various contexts.
Infrastructure: High-performance GPUs or TPUs are essential to manage extensive computations. Cloud platforms are commonly used to facilitate this process.
Fine-Tuning Large Language Models
Fine-tuning LLMs involves adjusting the pre-trained model to specific tasks or domains, which is typically less resource-intensive than training from scratch but still demanding:
Specific Domains: Fine-tuning allows LLMs to adapt to particular domains, improving performance on specialized tasks.
Cost and Time: Fine-tuning can still be costly and time-consuming but is more manageable compared to full training.
Example Code:
from transformers import GPT2Tokenizer, GPT2LMHeadModel, Trainer, TrainingArguments
# Load pre-trained model and tokenizer
model_name = 'gpt2'
tokenizer = GPT2Tokenizer.from_pretrained(model_name)
model = GPT2LMHeadModel.from_pretrained(model_name)
# Prepare dataset (assuming 'train_dataset' is already prepared)
training_args = TrainingArguments(
output_dir='./results',
num_train_epochs=3,
per_device_train_batch_size=4,
save_steps=10_000,
save_total_limit=2,
)
trainer = Trainer(
model=model,
args=training_args,
train_dataset=train_dataset,
)
# Fine-tune model
trainer.train()
Training Small Language Models (SLMs)
Training SLMs is more feasible for many organizations due to their lower resource requirements:
Time and Cost: Training SLMs can be completed in a shorter timeframe and at a lower cost.
Datasets: SLMs use smaller, often domain-specific datasets, which reduce training complexity and duration.
Infrastructure: Standard CPUs or lower-end GPUs are usually sufficient, making it accessible for smaller setups.
Fine-Tuning Small Language Models
Fine-tuning SLMs is relatively straightforward and cost-effective:
Efficiency: The process is faster and requires fewer resources, making it ideal for iterative improvements and specific task adaptation.
Specialization: Fine-tuning allows SLMs to excel in niche areas without the overhead of extensive retraining.
For a deeper dive into the training processes and best practices for AI models, check out Raga AI’s guide on fine-tuning LLM applications.
Next, we will compare the inference speed and deployment flexibility of LLMs and SLMs.
Inference Speed and Deployment Flexibility
When considering Large Language Models (LLMs) and Small Language Models (SLMs), understanding their inference speed and deployment flexibility significantly impacts the practical application and efficiency of the models.
Inference Speed
Inference speed refers to how quickly a model can generate predictions or responses after being deployed.
LLMs: Due to their large size and complexity, LLMs typically have slower inference speeds. They require substantial computational power, which can lead to latency issues, especially in real-time applications.
Example:
import torch
from transformers import GPT2Tokenizer, GPT2LMHeadModel
# Load pre-trained model and tokenizer
model_name = 'gpt2'
tokenizer = GPT2Tokenizer.from_pretrained(model_name)
model = GPT2LMHeadModel.from_pretrained(model_name)
# Encode input text
input_text = "What is the capital of France?"
input_ids = tokenizer.encode(input_text, return_tensors='pt')
# Measure inference time
import time
start_time = time.time()
output = model.generate(input_ids, max_length=50)
end_time = time.time()
# Calculate inference time
inference_time = end_time - start_time
print(f"Inference time: {inference_time:.2f} seconds")
SLMs: SLMs are designed for speed and efficiency. Their simpler architecture and smaller size enable them to deliver faster inference speeds, making them suitable for applications requiring quick responses.
Deployment Flexibility
Deployment flexibility is about how easily a model can be deployed across different platforms and environments.
LLMs: LLMs are best suited for cloud-based deployment due to their high computational needs. Cloud platforms can provide the necessary resources for running these models efficiently, but this limits their use in environments with restricted or no internet access.
Cloud Deployment:
High computational power
Suitable for large-scale applications
High operational costs
SLMs: SLMs excel in deployment flexibility. They can be deployed on various platforms, including local machines, edge devices, and mobile applications, due to their lower resource requirements. This makes them ideal for environments where quick and efficient processing is needed without relying heavily on cloud infrastructure.
Edge Deployment:
Lower computational power
Suitable for real-time, on-device applications
Cost-effective and accessible
For more on optimizing AI deployment, see Raga AI’s blog on AI-driven predictive analytics in retail inventory.
Comparison Table

Understanding these aspects helps in selecting the right model based on the specific needs and constraints of your application.
Next, let’s explore various use cases and applications for LLMs and SLMs.
Use Cases and Applications
The distinct characteristics of Large Language Models (LLMs) and Small Language Models (SLMs) make them suitable for different types of applications. Understanding these use cases can help you benefit each model's strengths for your specific needs.
Use Cases for Large Language Models (LLMs)
LLMs excel in tasks that require deep understanding, complex pattern recognition, and high accuracy. Here are some common applications:
Chatbots and Virtual Assistants: LLMs can handle sophisticated conversations, providing accurate and contextually relevant responses.
Content Generation: Ideal for creating articles, reports, and other text-based content that requires a nuanced understanding of language and context.
Translation Services: LLMs can accurately translate text between languages, capturing the nuances and subtleties of the original content.
Research and Analysis: Useful for extracting insights from large datasets, summarizing information, and generating comprehensive reports.
Example Code for Content Generation
Here's a simple example of using an LLM for generating content:
from transformers import GPT2Tokenizer, GPT2LMHeadModel
# Load pre-trained model and tokenizer
model_name = 'gpt2'
tokenizer = GPT2Tokenizer.from_pretrained(model_name)
model = GPT2LMHeadModel.from_pretrained(model_name)
# Encode input text
input_text = "Write an introduction for a blog about AI advancements."
input_ids = tokenizer.encode(input_text, return_tensors='pt')
# Generate text
output = model.generate(input_ids, max_length=100)
generated_text = tokenizer.decode(output[0], skip_special_tokens=True)
print(generated_text)
Use Cases for Small Language Models (SLMs)
SLMs are well-suited for applications where speed, efficiency, and resource constraints are critical. Here are some common applications:
Specialized Knowledge Tasks: Ideal for tasks requiring domain-specific knowledge, such as technical support or legal document analysis.
Real-Time Language Processing: Suitable for mobile apps, voice assistants, and IoT devices where quick responses are essential.
Voice Assistants: Efficient for running on devices with limited computational power, providing quick and accurate responses.
IoT Applications: Useful for real-time data processing and decision-making in edge computing environments.
Example Code for a Voice Assistant
Here's a simple example of using an SLM for a voice assistant application:
from transformers import DistilBertTokenizer, DistilBertForQuestionAnswering
import torch
# Load pre-trained model and tokenizer
model_name = 'distilbert-base-uncased'
tokenizer = DistilBertTokenizer.from_pretrained(model_name)
model = DistilBertForQuestionAnswering.from_pretrained(model_name)
# Sample question and context
question = "What is the capital of France?"
context = "France is a country in Europe. The capital of France is Paris."
# Encode input
inputs = tokenizer.encode_plus(question, context, return_tensors='pt')
# Get answer
with torch.no_grad():
outputs = model(**inputs)
answer_start = torch.argmax(outputs.start_logits)
answer_end = torch.argmax(outputs.end_logits) + 1
answer = tokenizer.convert_tokens_to_ids(inputs.input_ids[answer_start:answer_end])
answer_text = tokenizer.decode(answer)
print(answer_text)
For detailed insights on optimizing quality and performance in AI applications, check out Raga AI’s blog on optimizing quality and performance in LLM apps.
Next, we will discuss the factors influencing the choice between LLMs and SLMs.
Choosing Between LLMs and SLMs
Selecting the right language model for your needs involves considering various factors that align with your specific business applications, resource availability, and task complexity. Here’s a guide to help you make an informed choice between Large Language Models (LLMs) and Small Language Models (SLMs).
Factors Influencing the Choice
1. Specific Business Applications
LLMs: Suitable for applications requiring deep understanding and high accuracy, such as complex text generation, sophisticated chatbots, and detailed data analysis.
SLMs: Best for tasks needing speed and efficiency, such as real-time language processing, voice assistants, and domain-specific knowledge tasks.
2. Resource Availability
LLMs: Require substantial computational resources, including powerful GPUs and significant memory. Ideal for organizations with access to cloud infrastructure.
SLMs: More resource-efficient, capable of running on standard CPUs and edge devices. Suitable for environments with limited computational resources.
3. Task Complexity
LLMs: Excel in handling complex tasks that involve nuanced understanding and context, making them ideal for research, content generation, and translation.
SLMs: Effective for simpler, specialized tasks where quick and efficient processing is essential, such as technical support and IoT applications.
Balanced Approach
Starting with LLMs for Broad Applications: LLMs provide a comprehensive understanding of language and can be a good starting point for broad applications. They can handle diverse and complex tasks, offering versatility and high performance.
Optimizing with SLMs for Efficiency: Once the broader application requirements are understood, optimizing with SLMs can enhance efficiency. SLMs are faster and more cost-effective, making them ideal for specific tasks within the broader application scope.
Example Scenario
Imagine a customer service application:
LLM: Used to train an advanced chatbot that understands and generates responses across various topics, providing high-quality customer interactions.
SLM: Deployed on local devices to handle specific queries quickly and efficiently, ensuring real-time responses and improved user experience.
For a detailed exploration of AI models' performance in real-world applications, explore Raga AI’s blog on enhancing edge AI with RagaAI integration.
Verdict
Choosing between LLMs and SLMs depends on your specific needs and resources. By understanding their strengths and limitations, you can strategically deploy these models to achieve optimal performance and efficiency. This approach ensures you utilize the full potential of AI tailored to your organizational requirements.
Conclusion
Large Language Models (LLMs) and Small Language Models (SLMs) each offer unique strengths and are suited to different applications. LLMs excel in complex, context-rich tasks like advanced text generation and comprehensive data analysis, delivering high accuracy and performance.
In contrast, SLMs are designed for speed and efficiency, making them ideal for real-time applications and domain-specific tasks. Understanding these differences helps you make informed decisions based on your organizational needs and resource availability.
Raga AI stands at the forefront of these advancements, offering state-of-the-art solutions for AI testing, governance, and optimization. With tools like the RagaAI Catalyst, Raga AI Prism, and Governance Hub, Raga AI ensures the quality, reliability, and efficiency of your AI applications. Whether you're dealing with LLMs or SLMs, Raga AI provides comprehensive support to enhance your AI strategies and achieve your goals.
Explore how Raga AI can transform your AI initiatives by visiting our Raga AI platform. Discover the full potential of your AI projects with Raga AI today!
Artificial Intelligence has significantly transformed the way we interact with technology, and nowhere is this more evident than in the field of Natural Language Processing (NLP). From smart assistants to advanced text generation, NLP advancements have reshaped our digital experiences. Whether you're a data scientist, a mid-level manager, or an AI enthusiast, grasping the distinctions between LLMs and SLMs is crucial for making informed decisions.
In this article, you'll learn the basics of LLMs and SLMs, their differences and how to choose them for your AI needs. Now, let’s dive deeper into understanding Large Language Models (LLMs).
Understanding Large Language Models (LLMs)
Large Language Models (LLMs) have emerged as a cornerstone in the field of NLP, enabling a range of applications from chatbots to content generation. These models handle vast amounts of data, making them exceptionally powerful for understanding and generating human-like text. Let's break down what makes LLMs unique and explore their key characteristics.
Definition and Characteristics of LLMs
LLMs are neural networks that are trained on extensive datasets, allowing them to learn the nuances of human language. Here’s what defines them:
Massive Datasets: LLMs are trained on billions of words from diverse sources.
High Number of Parameters: They contain millions to billions of parameters, which are weights and biases that the model learns during training.
Complex Architectures: LLMs use advanced architectures like transformers to process and generate text efficiently.
Versatile Applications: They are used in various applications, including translation, summarization, and content generation.
Human-Like Text Generation: LLMs excel at producing coherent and contextually accurate human-like text.
Training Datasets and Parameter Scale
The effectiveness of LLMs is largely due to the size and quality of their training datasets. These models process a variety of texts, from books and articles to websites, helping them understand context and generate coherent text.
Datasets: LLMs train on a diverse range of text sources, including books, articles, websites, and social media posts. This variety ensures the models are exposed to different writing styles, contexts, and topics, enhancing their ability to understand and generate text across multiple domains. The inclusion of diverse datasets is crucial for the models to learn the nuances of human language and produce contextually relevant outputs.
Parameter Scale: LLMs have a parameter scale ranging from millions to billions of parameters. These parameters represent the weights and biases the model learns during training, allowing it to understand and generate text with high accuracy. The larger the number of parameters, the better the model can capture complex patterns and relationships within the data. This extensive parameter scale contributes to the model's capability to produce sophisticated and contextually appropriate text, making LLMs powerful tools for various natural language processing tasks.
Transformer Architecture
LLMs utilize the Transformer architecture, which consists of encoder and decoder components:
Encoder: The encoder processes the input text to understand its context. It breaks down the input into smaller components, analyzes each part, and captures the underlying meaning and relationships within the text. This process involves converting the input text into a series of numerical representations that encapsulate the context and nuances of the original text, making it easier for the model to understand and work with.
Decoder: The decoder generates the output text based on the processed input. Using the numerical representations created by the encoder, the decoder constructs coherent and contextually relevant text. It systematically assembles words and phrases to form meaningful sentences that reflect the input's original context and intent. This step is crucial for producing accurate and human-like responses or translations in various natural language processing applications.
Although keep in mind that not all LLMs have the encoder component. For example, LLMs like GPT-3 work only on decoder components.
Here's a simple example of how a Transformer might be coded in Python using the ‘transformers’ library:
from transformers import GPT2Tokenizer, GPT2LMHeadModel
# Load pre-trained model and tokenizer
model_name = 'gpt2'
tokenizer = GPT2Tokenizer.from_pretrained(model_name)
model = GPT2LMHeadModel.from_pretrained(model_name)
# Encode input text
input_text = "The future of AI is"
input_ids = tokenizer.encode(input_text, return_tensors='pt')
# Generate text
output = model.generate(input_ids, max_length=50, num_return_sequences=1)
# Decode output
generated_text = tokenizer.decode(output[0], skip_special_tokens=True)
print(generated_text)
Capabilities and Common Applications
LLMs excel in several areas, making them highly versatile:
Contextual Text Generation: They can generate text that is contextually relevant and coherent.
Multimodal Abilities: Capable of processing and generating text, images, and even audio.
Advantages of LLMs
LLMs offer several advantages, including:
Complex Pattern Recognition: They can identify and understand intricate patterns in data.
High Performance in Precision Tasks: Ideal for tasks requiring high accuracy and detailed understanding.
Ethical Considerations
While powerful, LLMs also pose ethical challenges:
Misinformation: They can generate convincing but false information.
Bias: They might perpetuate biases present in their training data.
Privacy Risks: Handling of sensitive data needs careful management.
Now that you have a solid understanding of Large Language Models, let's explore the characteristics and advantages of Small Language Models (SLMs).
For a deeper dive into enhancing reliability with guardrails for LLMs, check out Raga AI’s case study on safeguarding enterprise LLM applications.
Understanding Small Language Models (SLMs)
Small Language Models (SLMs) offer a more streamlined approach to NLP, focusing on efficiency and specific task performance. They handle less data and require fewer resources, making them an excellent choice for many applications. Let's explore what makes SLMs distinct and their key benefits.
Definition and Characteristics of SLMs
SLMs are neural networks trained on smaller datasets compared to LLMs, focusing on efficiency and specific applications. Here’s what defines them:
Smaller Datasets: SLMs are trained on millions to tens of millions of words, often sourced from specific domains or industries. This focused training allows them to perform well within particular contexts or specialized tasks. By concentrating on a narrower range of data, SLMs can quickly and effectively learn the relevant patterns and nuances necessary for their specific applications.
Fewer Parameters: SLMs contain significantly fewer parameters than LLMs, usually ranging from a few million to a few hundred million. This reduction in parameters makes SLMs less resource-intensive, allowing them to run efficiently on less powerful hardware. Despite having fewer parameters, these models can still deliver strong performance within their specialized domains by leveraging the high-quality, targeted datasets they are trained on.
Efficient Computation: Due to their smaller size, SLMs require less computational power and memory, making them suitable for deployment in environments with limited resources. This efficiency is particularly advantageous for applications that need to run on edge devices or mobile platforms where computational capacity is restricted.
Faster Training and Inference: The reduced complexity of SLMs leads to faster training times and quicker inference speeds. This speed allows for rapid development cycles and real-time application scenarios, where timely responses are critical. The ability to quickly train and deploy these models makes them an attractive choice for many practical applications.
Specific Applications: SLMs are often designed for specific applications where domain knowledge is paramount. Examples include medical diagnosis, financial forecasting, and customer service automation. By tailoring the model to a particular use case, SLMs can achieve high accuracy and reliability, making them valuable tools in specialized fields.
Training Datasets and Parameter Scale
SLMs are effective due to their focused training datasets and manageable parameter scales. These models prioritize relevance and specificity over sheer size.
Datasets: SLMs are often trained on domain-specific datasets, ensuring their relevance to particular tasks. These datasets are carefully curated to include texts from specialized areas such as medical records, financial reports, technical manuals, or customer service interactions. By focusing on domain-specific data, SLMs can develop a deep understanding of the specific language, terminology, and context required for their target applications. This targeted training helps the models perform accurately and effectively within their specialized areas, providing reliable and contextually appropriate outputs.
Parameter Scale: SLMs typically have a parameter scale that ranges from millions to tens of millions of parameters. This relatively smaller scale compared to LLMs makes SLMs lighter and faster, requiring less computational power and memory. The reduced number of parameters allows these models to be more efficient, enabling quicker training times and faster inference speeds. Despite their smaller size, SLMs maintain the ability to capture essential patterns and relationships within their specialized domains, delivering high-performance results for specific tasks without the need for extensive computational resources. This efficiency makes SLMs an ideal choice for applications where speed and resource management are crucial.
Simpler Architecture Compared to LLMs
SLMs employ a simpler architecture, which contributes to their efficiency: They use fewer layers and parameters, reducing computational requirements.
Advantages of SLMs
SLMs offer several advantages, including:
Speed: Faster training and inference times.
Memory and Resource Efficiency: Require less computational power and memory, making them suitable for edge devices.
Limitations of SLMs
Despite their benefits, SLMs have some limitations:
Scalability Issues: Struggle with larger, more complex datasets.
Lower Performance in Intricate Tasks: May not perform as well in tasks requiring deep understanding and context.
Example Code for a Simple SLM
Here's a simple example of an SLM coded in Python using the ‘transformers’ library:
from transformers import DistilBertTokenizer, DistilBertModel
# Load pre-trained model and tokenizer
model_name = 'distilbert-base-uncased'
tokenizer = DistilBertTokenizer.from_pretrained(model_name)
model = DistilBertModel.from_pretrained(model_name)
# Encode input text
input_text = "Efficient NLP with small models"
input_ids = tokenizer.encode(input_text, return_tensors='pt')
# Generate embeddings
outputs = model(input_ids)
embeddings = outputs.last_hidden_state
print(embeddings)
For a comprehensive guide on testing AI models like SLMs, explore Raga AI’s guide to evaluating LLM applications and enabling guardrails.
Understanding the characteristics and advantages of Small Language Models sets the stage for comparing them with Large Language Models. Let's now examine the key differences between LLMs and SLMs.
Key Differences Between LLMs and SLMs
Understanding the distinctions between Large Language Models (LLMs) and Small Language Models (SLMs) is essential for using their strengths effectively. Let's break down the key differences to help you determine which model suits your needs best.

For further insights on AI models' implementation and optimization, explore Raga AI’s blog on enhancing edge AI with RagaAI integration on NVIDIA Metropolis.
Next, we will explore the resource requirements of LLMs and SLMs.
Resource Requirements
When choosing between Large Language Models (LLMs) and Small Language Models (SLMs), it's crucial to consider the resource requirements. These factors play a significant role in determining the feasibility and efficiency of deploying these models.
Resource Requirements of LLMs
LLMs are known for their high computational and memory demands. Here’s a breakdown of what they typically require:
High Memory Consumption: LLMs need substantial RAM to store and process large amounts of data.
Computational Power: They require powerful GPUs or TPUs to handle the complex calculations involved in training and inference.
Energy Usage: The extensive computational processes lead to high energy consumption, impacting both cost and environmental footprint.
Resource Requirements of SLMs
SLMs are designed with efficiency in mind, making them more accessible for various applications. Here’s what they typically require:
Lower Memory Consumption: SLMs need less RAM, making them suitable for devices with limited memory.
Reduced Computational Power: They can run on standard CPUs and require fewer GPUs, making them more cost-effective.
Energy Efficiency: Due to their simpler architecture, SLMs consume less energy, which reduces operational costs and environmental impact.
Efficiency Techniques
SLMs often employ efficiency techniques to maximize their performance within resource constraints:
Knowledge Distillation: Transferring knowledge from a larger model to a smaller one to maintain performance while reducing size.
Quantization: Reducing the precision of the model’s weights to decrease memory and computational requirements without significantly impacting accuracy.
Here's an example of how quantization can be implemented in Python using the ‘transformers’ library:
from transformers import DistilBertModel, DistilBertTokenizer
from transformers import pipeline
# Load pre-trained model and tokenizer
model_name = 'distilbert-base-uncased'
tokenizer = DistilBertTokenizer.from_pretrained(model_name)
model = DistilBertModel.from_pretrained(model_name)
# Quantize the model
quantized_model = torch.quantization.quantize_dynamic(
model, {torch.nn.Linear}, dtype=torch.qint8
)
# Use the quantized model in a pipeline
nlp_pipeline = pipeline('feature-extraction', model=quantized_model, tokenizer=tokenizer)
output = nlp_pipeline("Efficient NLP with quantized models")
print(output)
For a detailed look at optimizing AI models with resource-efficient techniques, check out Raga AI’s blog on LLM evaluation.
Along with resource utilization, LLMs and SLMs vary in training and fine-tuning, too. Let’s see how.
Training and Fine-Tuning
Training and fine-tuning Large Language Models (LLMs) and Small Language Models (SLMs) involve different processes and resource investments. Understanding these differences can help you choose the right approach for your specific needs.
Training Large Language Models (LLMs)
Training LLMs is a resource-intensive process that requires substantial computational power and time. Here’s a closer look at what’s involved:
Time and Cost: Training an LLM from scratch can take weeks or months and requires significant financial investment due to the computational resources needed.
Datasets: LLMs are trained on massive datasets, often comprising billions of words from diverse sources, which helps them generalize across various contexts.
Infrastructure: High-performance GPUs or TPUs are essential to manage extensive computations. Cloud platforms are commonly used to facilitate this process.
Fine-Tuning Large Language Models
Fine-tuning LLMs involves adjusting the pre-trained model to specific tasks or domains, which is typically less resource-intensive than training from scratch but still demanding:
Specific Domains: Fine-tuning allows LLMs to adapt to particular domains, improving performance on specialized tasks.
Cost and Time: Fine-tuning can still be costly and time-consuming but is more manageable compared to full training.
Example Code:
from transformers import GPT2Tokenizer, GPT2LMHeadModel, Trainer, TrainingArguments
# Load pre-trained model and tokenizer
model_name = 'gpt2'
tokenizer = GPT2Tokenizer.from_pretrained(model_name)
model = GPT2LMHeadModel.from_pretrained(model_name)
# Prepare dataset (assuming 'train_dataset' is already prepared)
training_args = TrainingArguments(
output_dir='./results',
num_train_epochs=3,
per_device_train_batch_size=4,
save_steps=10_000,
save_total_limit=2,
)
trainer = Trainer(
model=model,
args=training_args,
train_dataset=train_dataset,
)
# Fine-tune model
trainer.train()
Training Small Language Models (SLMs)
Training SLMs is more feasible for many organizations due to their lower resource requirements:
Time and Cost: Training SLMs can be completed in a shorter timeframe and at a lower cost.
Datasets: SLMs use smaller, often domain-specific datasets, which reduce training complexity and duration.
Infrastructure: Standard CPUs or lower-end GPUs are usually sufficient, making it accessible for smaller setups.
Fine-Tuning Small Language Models
Fine-tuning SLMs is relatively straightforward and cost-effective:
Efficiency: The process is faster and requires fewer resources, making it ideal for iterative improvements and specific task adaptation.
Specialization: Fine-tuning allows SLMs to excel in niche areas without the overhead of extensive retraining.
For a deeper dive into the training processes and best practices for AI models, check out Raga AI’s guide on fine-tuning LLM applications.
Next, we will compare the inference speed and deployment flexibility of LLMs and SLMs.
Inference Speed and Deployment Flexibility
When considering Large Language Models (LLMs) and Small Language Models (SLMs), understanding their inference speed and deployment flexibility significantly impacts the practical application and efficiency of the models.
Inference Speed
Inference speed refers to how quickly a model can generate predictions or responses after being deployed.
LLMs: Due to their large size and complexity, LLMs typically have slower inference speeds. They require substantial computational power, which can lead to latency issues, especially in real-time applications.
Example:
import torch
from transformers import GPT2Tokenizer, GPT2LMHeadModel
# Load pre-trained model and tokenizer
model_name = 'gpt2'
tokenizer = GPT2Tokenizer.from_pretrained(model_name)
model = GPT2LMHeadModel.from_pretrained(model_name)
# Encode input text
input_text = "What is the capital of France?"
input_ids = tokenizer.encode(input_text, return_tensors='pt')
# Measure inference time
import time
start_time = time.time()
output = model.generate(input_ids, max_length=50)
end_time = time.time()
# Calculate inference time
inference_time = end_time - start_time
print(f"Inference time: {inference_time:.2f} seconds")
SLMs: SLMs are designed for speed and efficiency. Their simpler architecture and smaller size enable them to deliver faster inference speeds, making them suitable for applications requiring quick responses.
Deployment Flexibility
Deployment flexibility is about how easily a model can be deployed across different platforms and environments.
LLMs: LLMs are best suited for cloud-based deployment due to their high computational needs. Cloud platforms can provide the necessary resources for running these models efficiently, but this limits their use in environments with restricted or no internet access.
Cloud Deployment:
High computational power
Suitable for large-scale applications
High operational costs
SLMs: SLMs excel in deployment flexibility. They can be deployed on various platforms, including local machines, edge devices, and mobile applications, due to their lower resource requirements. This makes them ideal for environments where quick and efficient processing is needed without relying heavily on cloud infrastructure.
Edge Deployment:
Lower computational power
Suitable for real-time, on-device applications
Cost-effective and accessible
For more on optimizing AI deployment, see Raga AI’s blog on AI-driven predictive analytics in retail inventory.
Comparison Table

Understanding these aspects helps in selecting the right model based on the specific needs and constraints of your application.
Next, let’s explore various use cases and applications for LLMs and SLMs.
Use Cases and Applications
The distinct characteristics of Large Language Models (LLMs) and Small Language Models (SLMs) make them suitable for different types of applications. Understanding these use cases can help you benefit each model's strengths for your specific needs.
Use Cases for Large Language Models (LLMs)
LLMs excel in tasks that require deep understanding, complex pattern recognition, and high accuracy. Here are some common applications:
Chatbots and Virtual Assistants: LLMs can handle sophisticated conversations, providing accurate and contextually relevant responses.
Content Generation: Ideal for creating articles, reports, and other text-based content that requires a nuanced understanding of language and context.
Translation Services: LLMs can accurately translate text between languages, capturing the nuances and subtleties of the original content.
Research and Analysis: Useful for extracting insights from large datasets, summarizing information, and generating comprehensive reports.
Example Code for Content Generation
Here's a simple example of using an LLM for generating content:
from transformers import GPT2Tokenizer, GPT2LMHeadModel
# Load pre-trained model and tokenizer
model_name = 'gpt2'
tokenizer = GPT2Tokenizer.from_pretrained(model_name)
model = GPT2LMHeadModel.from_pretrained(model_name)
# Encode input text
input_text = "Write an introduction for a blog about AI advancements."
input_ids = tokenizer.encode(input_text, return_tensors='pt')
# Generate text
output = model.generate(input_ids, max_length=100)
generated_text = tokenizer.decode(output[0], skip_special_tokens=True)
print(generated_text)
Use Cases for Small Language Models (SLMs)
SLMs are well-suited for applications where speed, efficiency, and resource constraints are critical. Here are some common applications:
Specialized Knowledge Tasks: Ideal for tasks requiring domain-specific knowledge, such as technical support or legal document analysis.
Real-Time Language Processing: Suitable for mobile apps, voice assistants, and IoT devices where quick responses are essential.
Voice Assistants: Efficient for running on devices with limited computational power, providing quick and accurate responses.
IoT Applications: Useful for real-time data processing and decision-making in edge computing environments.
Example Code for a Voice Assistant
Here's a simple example of using an SLM for a voice assistant application:
from transformers import DistilBertTokenizer, DistilBertForQuestionAnswering
import torch
# Load pre-trained model and tokenizer
model_name = 'distilbert-base-uncased'
tokenizer = DistilBertTokenizer.from_pretrained(model_name)
model = DistilBertForQuestionAnswering.from_pretrained(model_name)
# Sample question and context
question = "What is the capital of France?"
context = "France is a country in Europe. The capital of France is Paris."
# Encode input
inputs = tokenizer.encode_plus(question, context, return_tensors='pt')
# Get answer
with torch.no_grad():
outputs = model(**inputs)
answer_start = torch.argmax(outputs.start_logits)
answer_end = torch.argmax(outputs.end_logits) + 1
answer = tokenizer.convert_tokens_to_ids(inputs.input_ids[answer_start:answer_end])
answer_text = tokenizer.decode(answer)
print(answer_text)
For detailed insights on optimizing quality and performance in AI applications, check out Raga AI’s blog on optimizing quality and performance in LLM apps.
Next, we will discuss the factors influencing the choice between LLMs and SLMs.
Choosing Between LLMs and SLMs
Selecting the right language model for your needs involves considering various factors that align with your specific business applications, resource availability, and task complexity. Here’s a guide to help you make an informed choice between Large Language Models (LLMs) and Small Language Models (SLMs).
Factors Influencing the Choice
1. Specific Business Applications
LLMs: Suitable for applications requiring deep understanding and high accuracy, such as complex text generation, sophisticated chatbots, and detailed data analysis.
SLMs: Best for tasks needing speed and efficiency, such as real-time language processing, voice assistants, and domain-specific knowledge tasks.
2. Resource Availability
LLMs: Require substantial computational resources, including powerful GPUs and significant memory. Ideal for organizations with access to cloud infrastructure.
SLMs: More resource-efficient, capable of running on standard CPUs and edge devices. Suitable for environments with limited computational resources.
3. Task Complexity
LLMs: Excel in handling complex tasks that involve nuanced understanding and context, making them ideal for research, content generation, and translation.
SLMs: Effective for simpler, specialized tasks where quick and efficient processing is essential, such as technical support and IoT applications.
Balanced Approach
Starting with LLMs for Broad Applications: LLMs provide a comprehensive understanding of language and can be a good starting point for broad applications. They can handle diverse and complex tasks, offering versatility and high performance.
Optimizing with SLMs for Efficiency: Once the broader application requirements are understood, optimizing with SLMs can enhance efficiency. SLMs are faster and more cost-effective, making them ideal for specific tasks within the broader application scope.
Example Scenario
Imagine a customer service application:
LLM: Used to train an advanced chatbot that understands and generates responses across various topics, providing high-quality customer interactions.
SLM: Deployed on local devices to handle specific queries quickly and efficiently, ensuring real-time responses and improved user experience.
For a detailed exploration of AI models' performance in real-world applications, explore Raga AI’s blog on enhancing edge AI with RagaAI integration.
Verdict
Choosing between LLMs and SLMs depends on your specific needs and resources. By understanding their strengths and limitations, you can strategically deploy these models to achieve optimal performance and efficiency. This approach ensures you utilize the full potential of AI tailored to your organizational requirements.
Conclusion
Large Language Models (LLMs) and Small Language Models (SLMs) each offer unique strengths and are suited to different applications. LLMs excel in complex, context-rich tasks like advanced text generation and comprehensive data analysis, delivering high accuracy and performance.
In contrast, SLMs are designed for speed and efficiency, making them ideal for real-time applications and domain-specific tasks. Understanding these differences helps you make informed decisions based on your organizational needs and resource availability.
Raga AI stands at the forefront of these advancements, offering state-of-the-art solutions for AI testing, governance, and optimization. With tools like the RagaAI Catalyst, Raga AI Prism, and Governance Hub, Raga AI ensures the quality, reliability, and efficiency of your AI applications. Whether you're dealing with LLMs or SLMs, Raga AI provides comprehensive support to enhance your AI strategies and achieve your goals.
Explore how Raga AI can transform your AI initiatives by visiting our Raga AI platform. Discover the full potential of your AI projects with Raga AI today!
Subscribe to our newsletter to never miss an update
Subscribe to our newsletter to never miss an update
Other articles
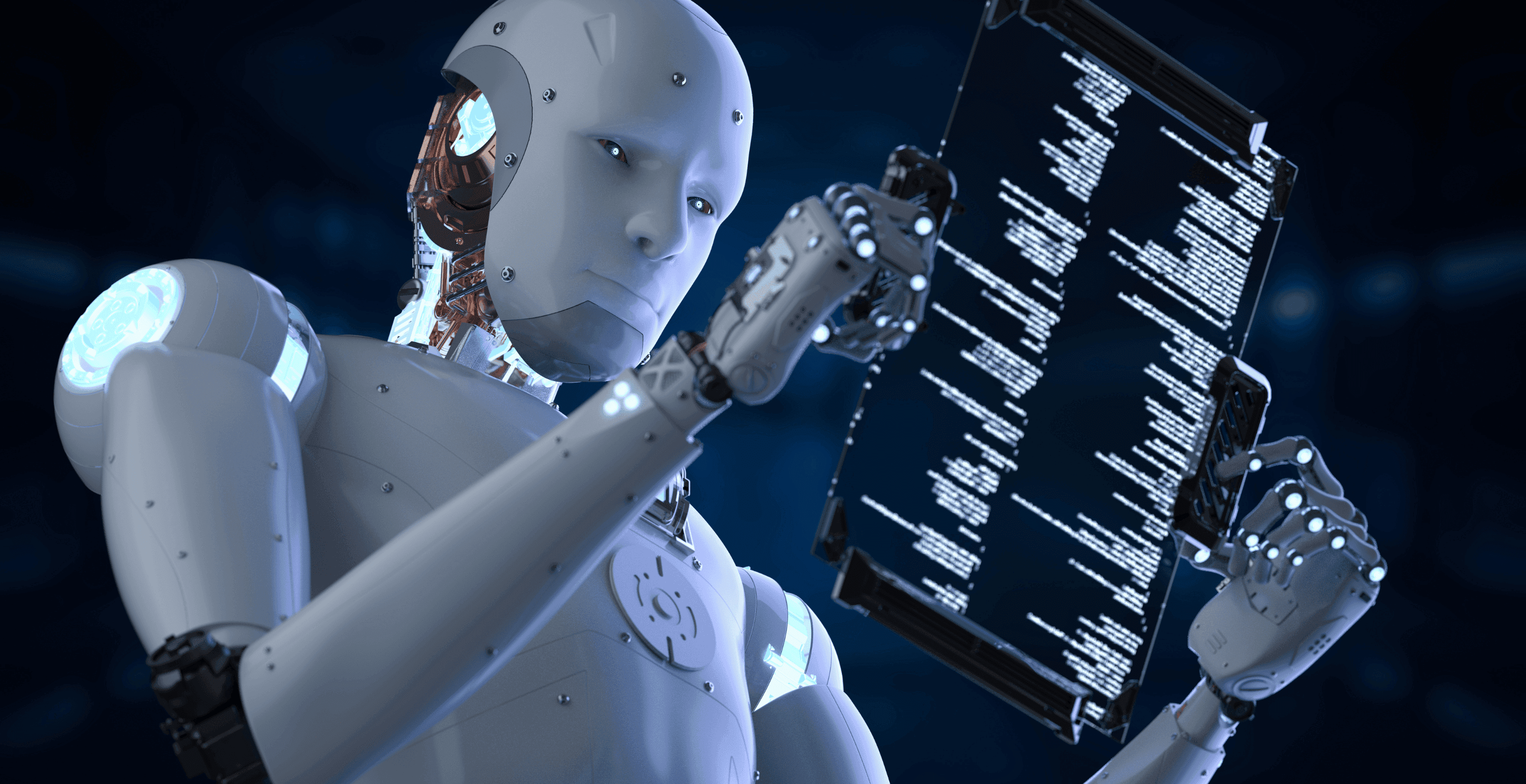
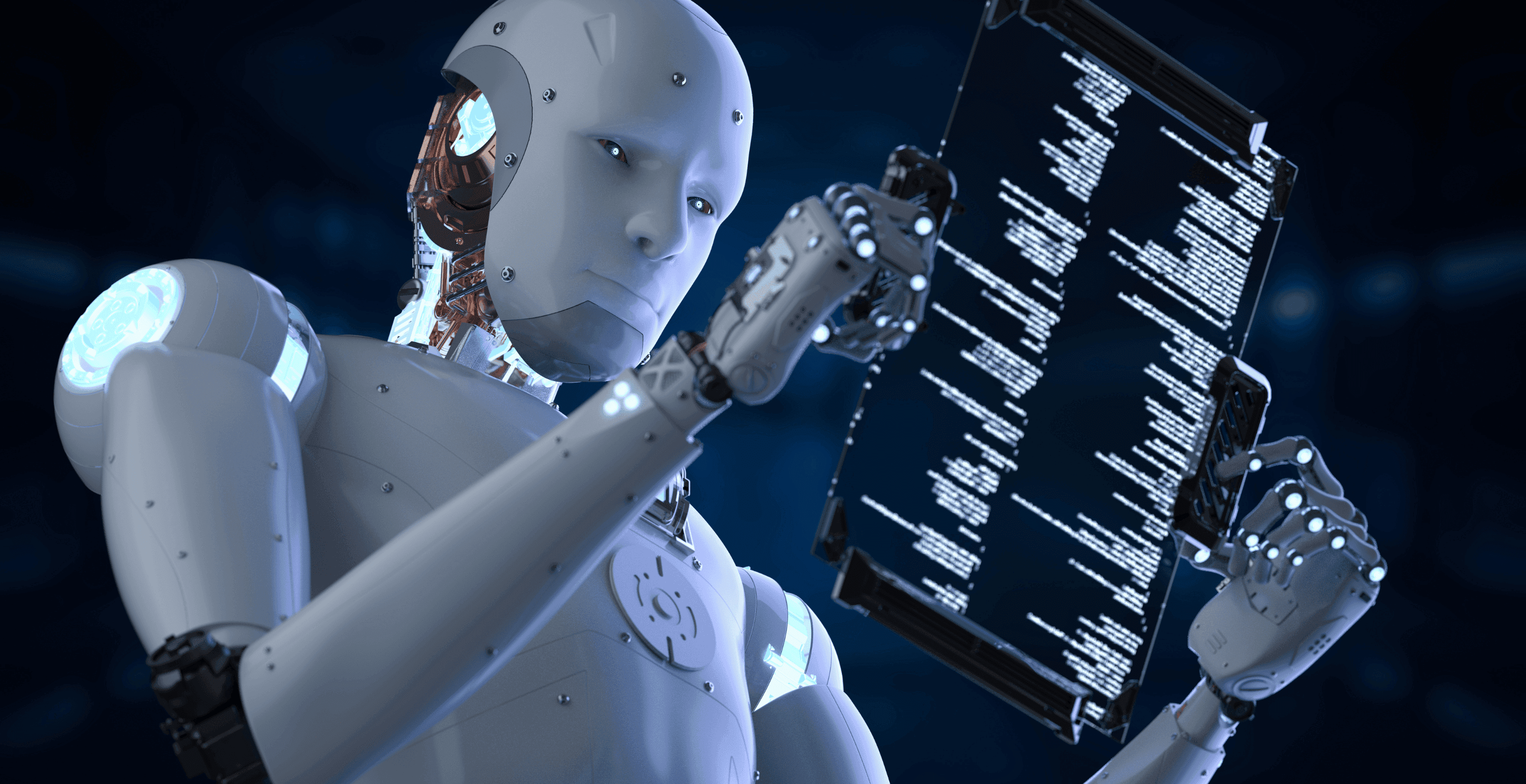
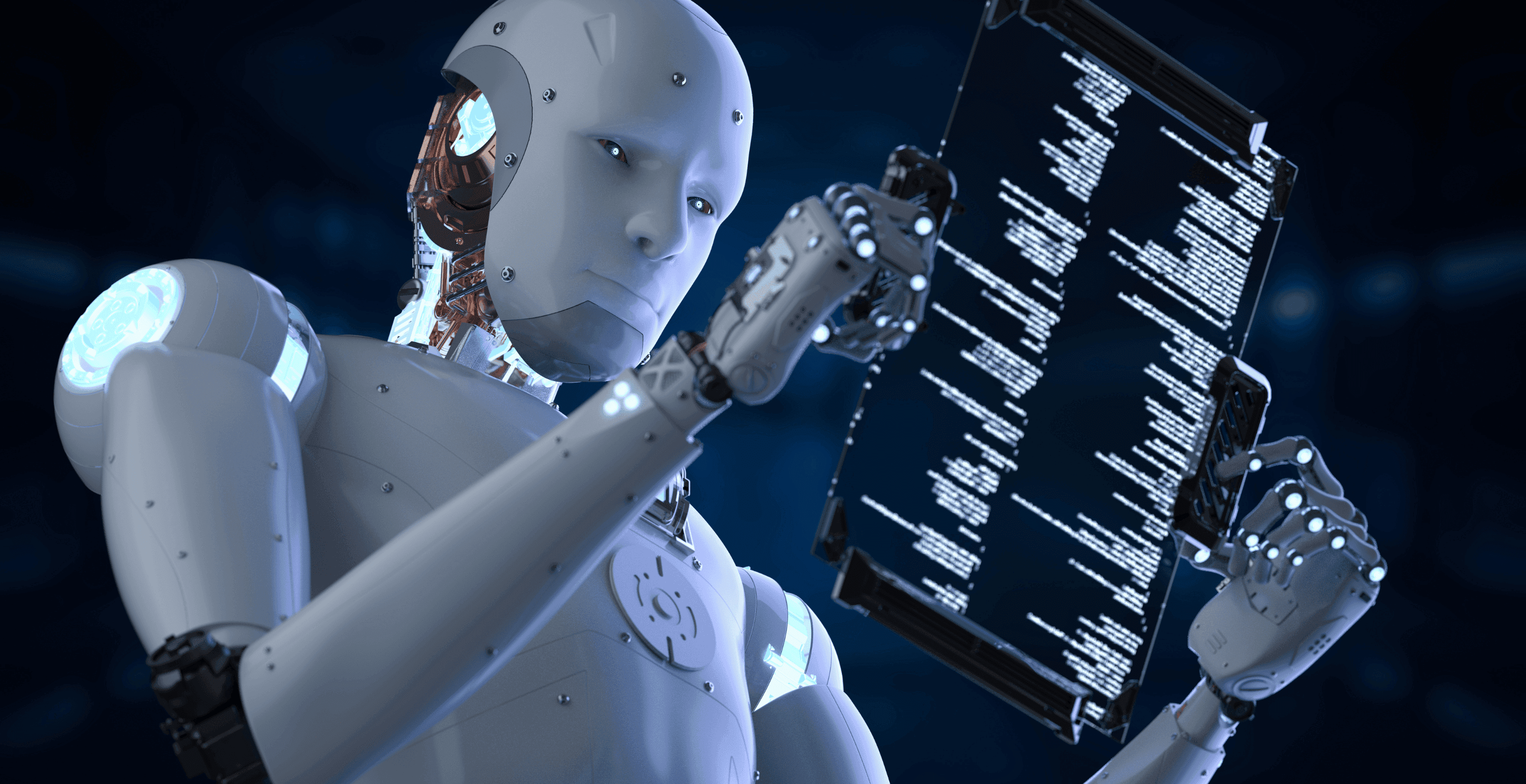
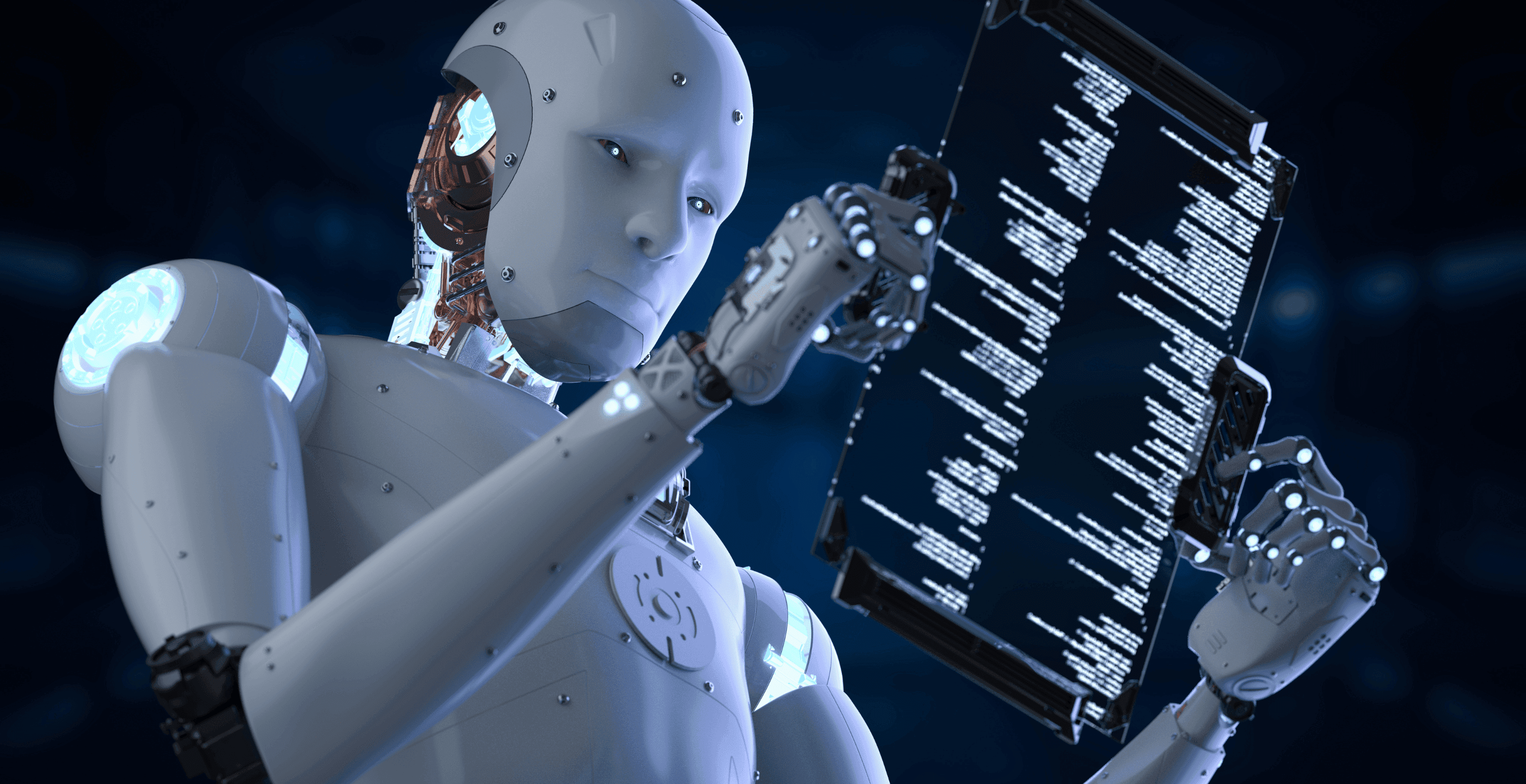
Exploring Intelligent Agents in AI

Rehan Asif
Jan 3, 2025
Read the article
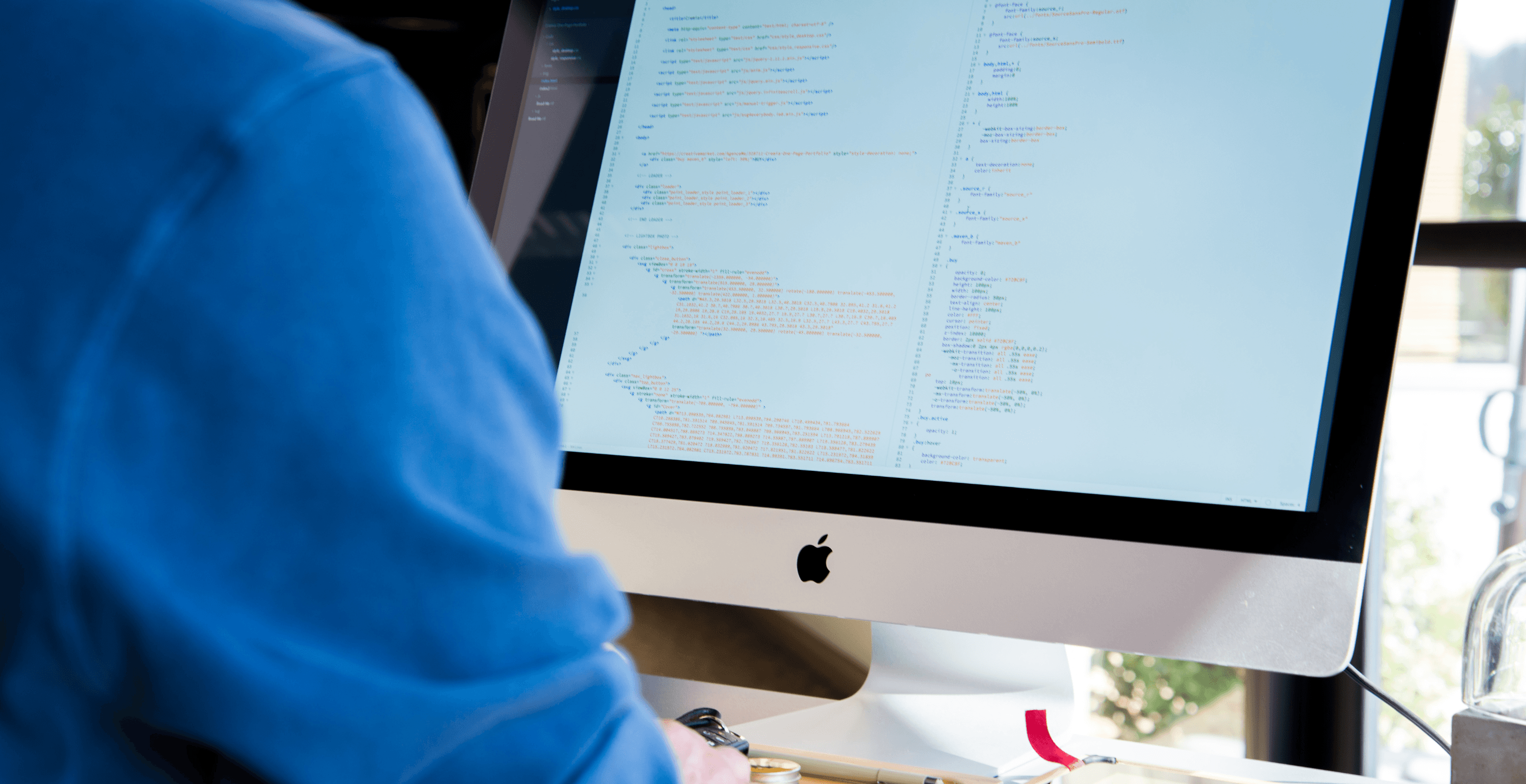
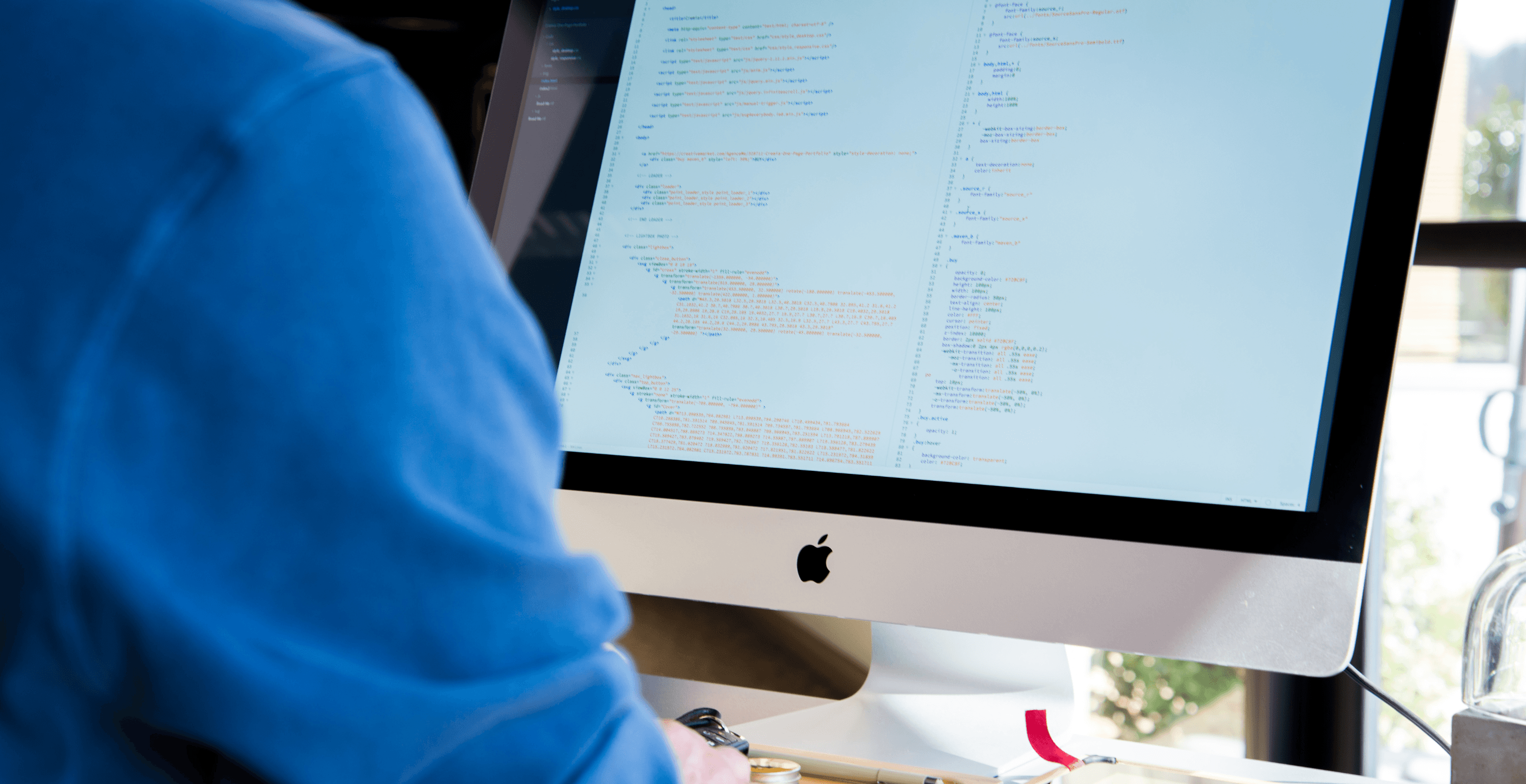
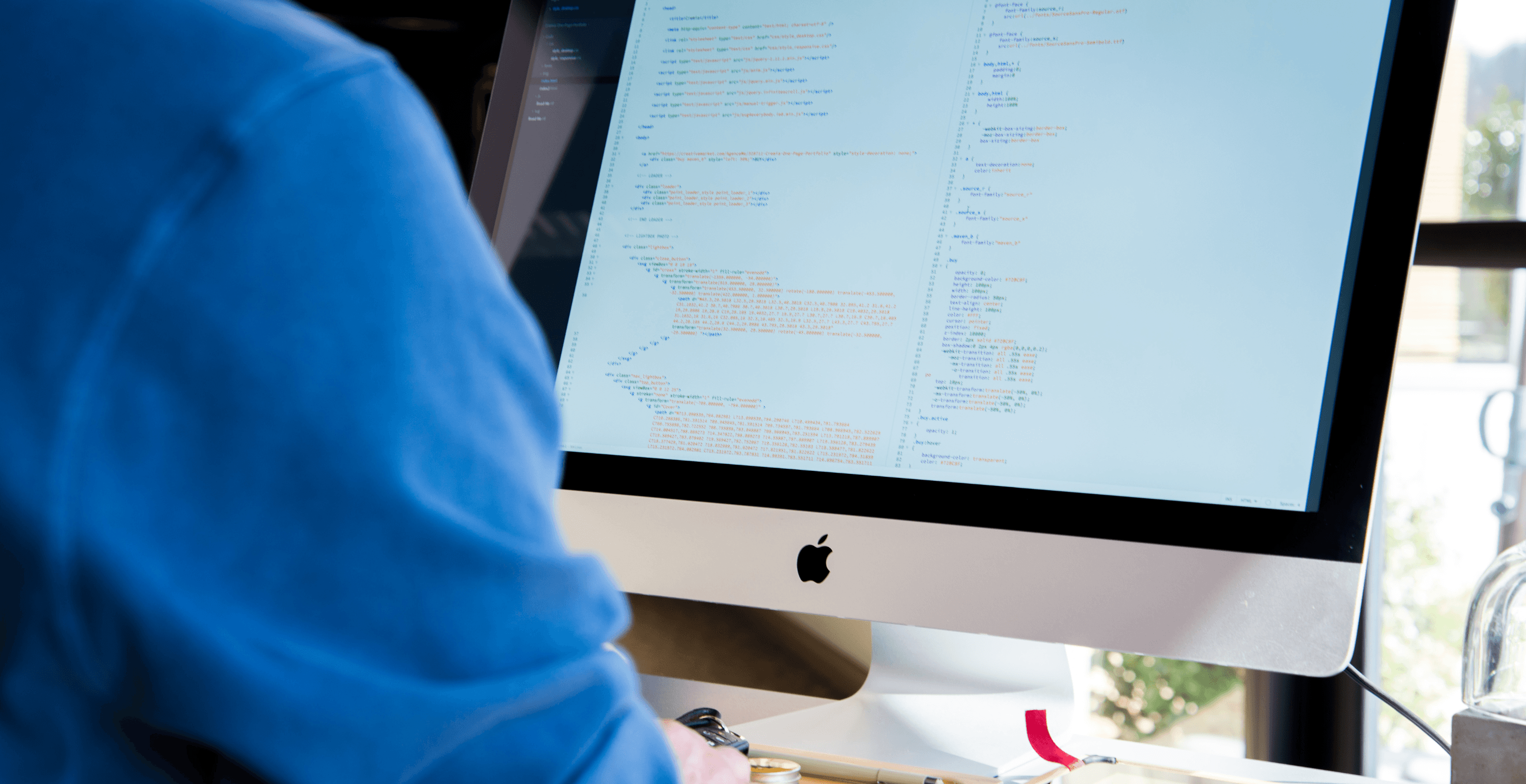
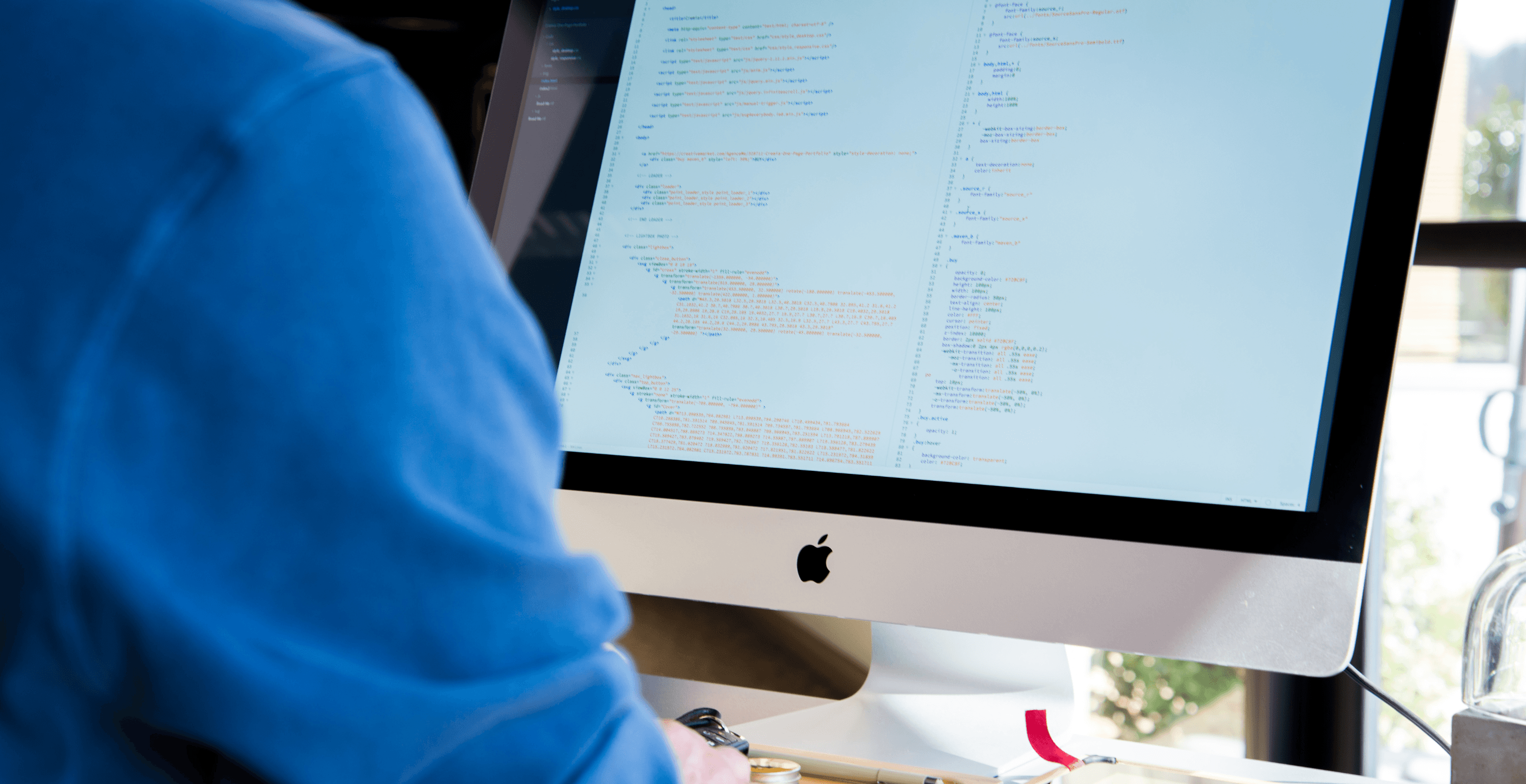
Understanding What AI Red Teaming Means for Generative Models
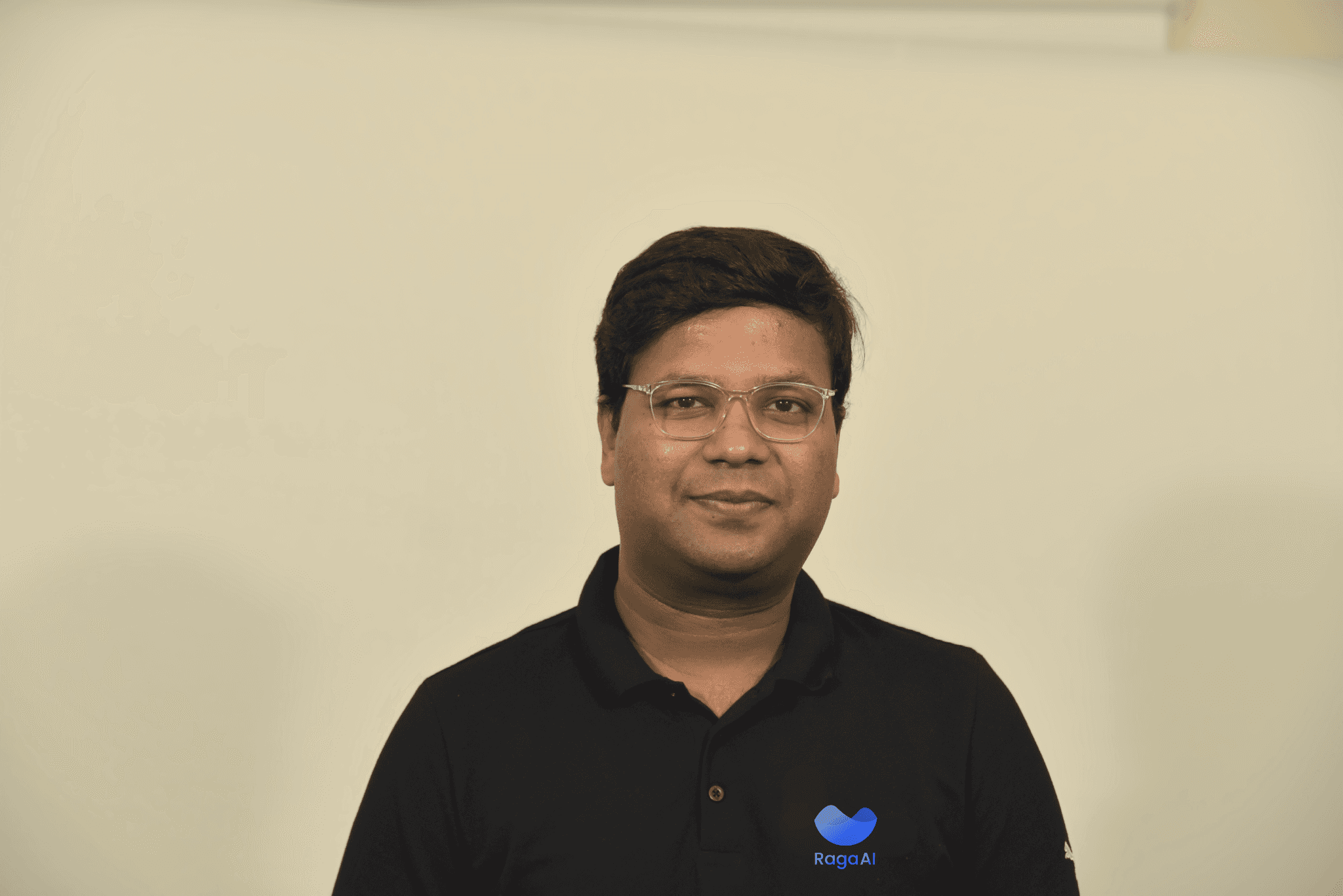
Jigar Gupta
Dec 30, 2024
Read the article
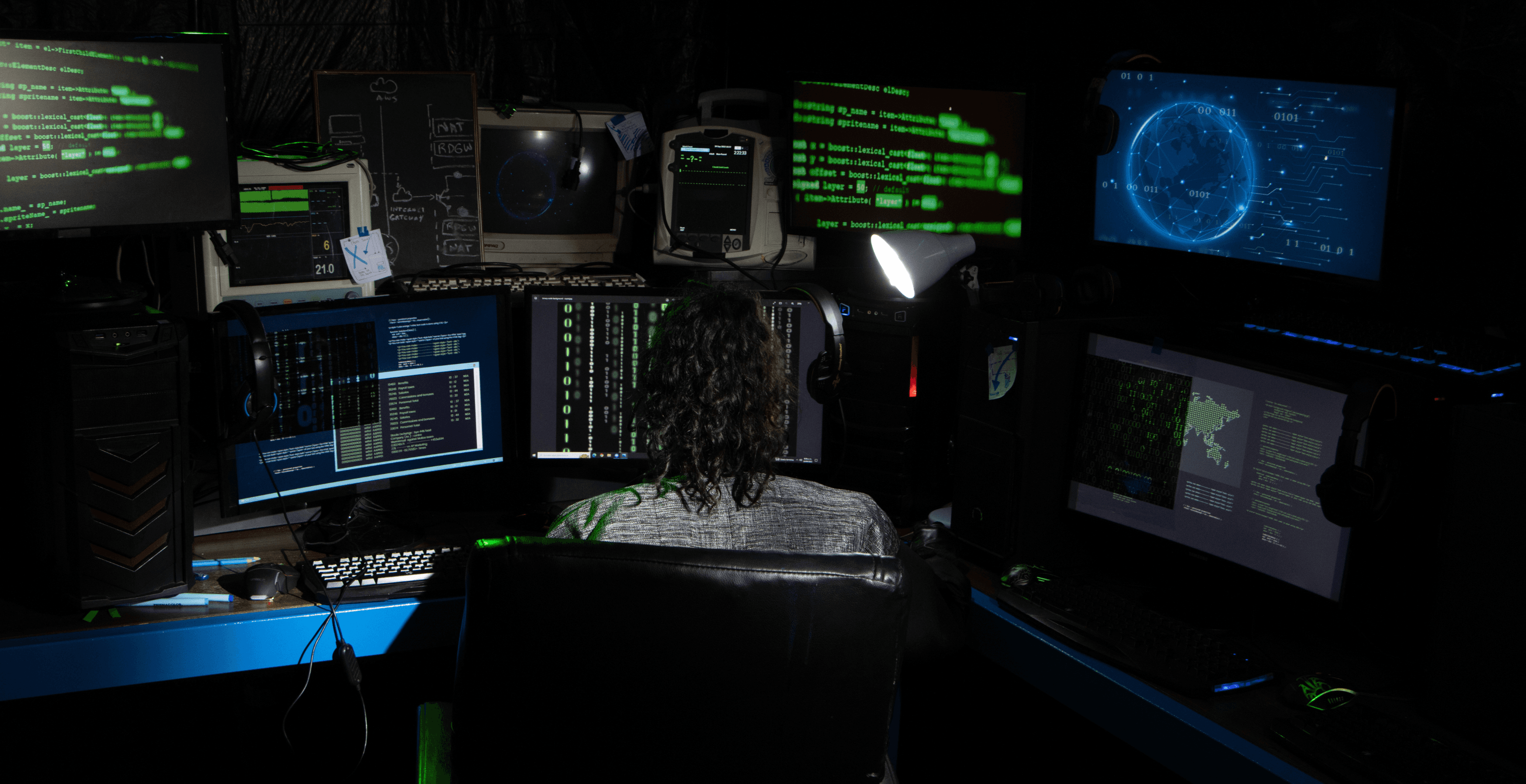
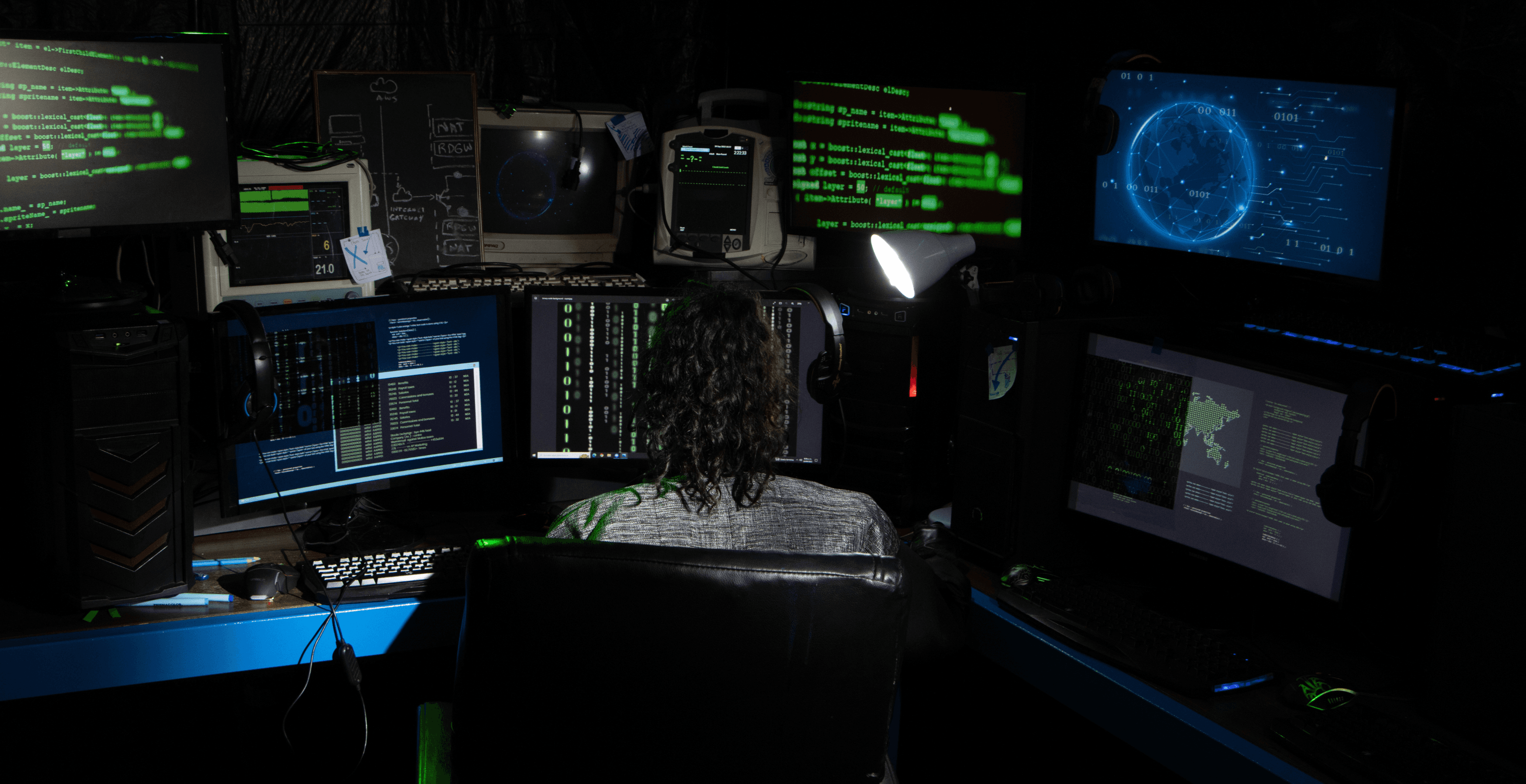
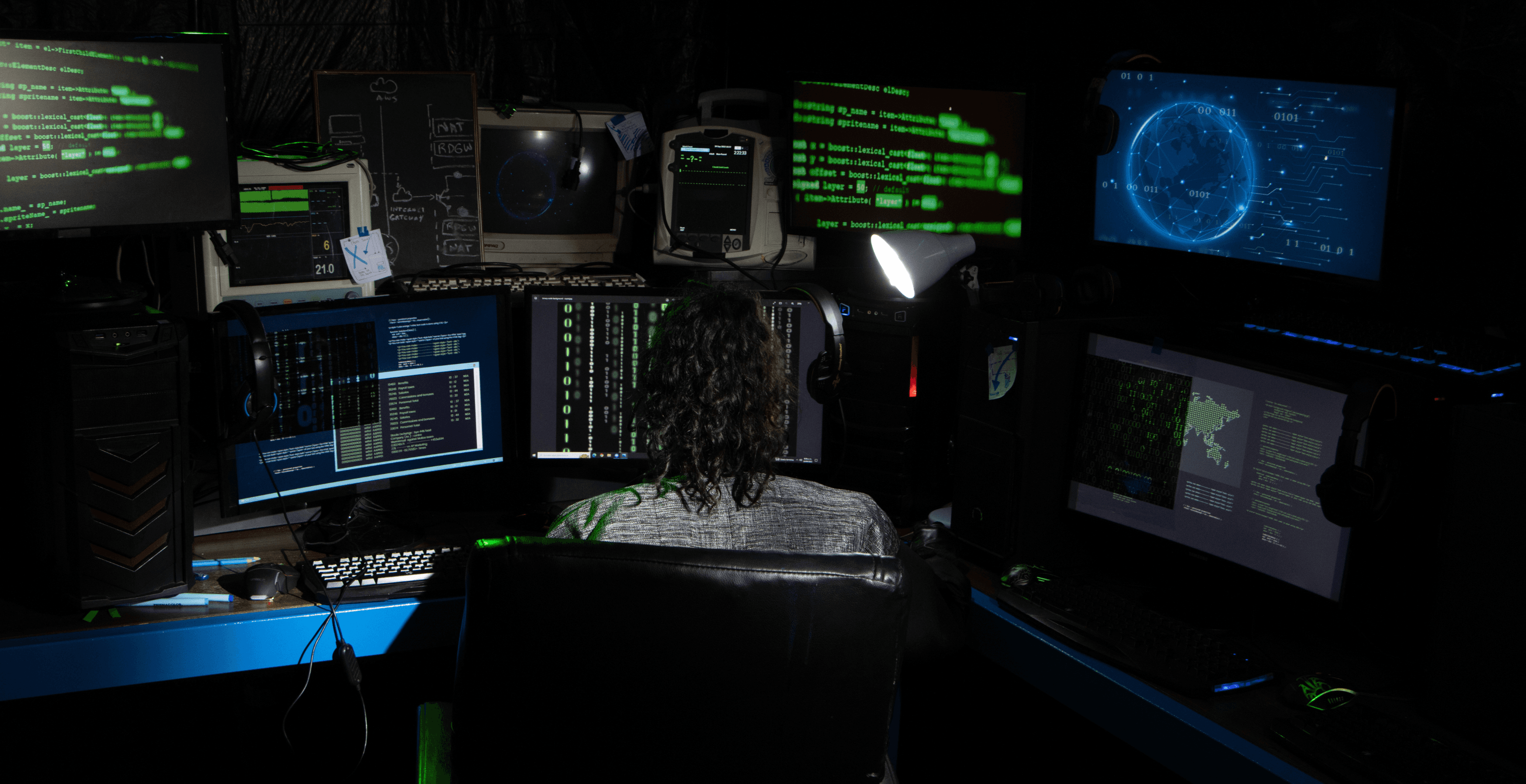
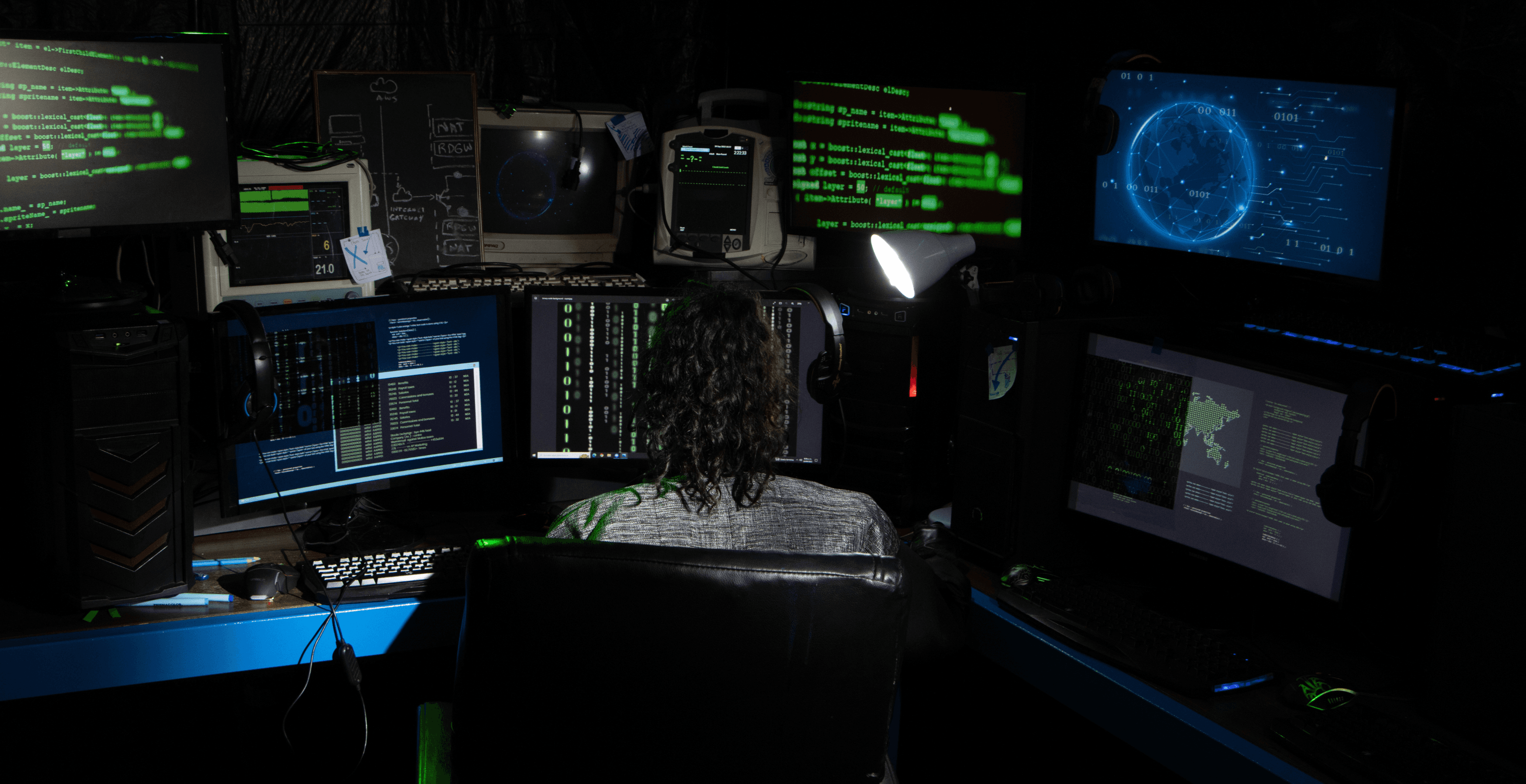
RAG vs Fine-Tuning: Choosing the Best AI Learning Technique
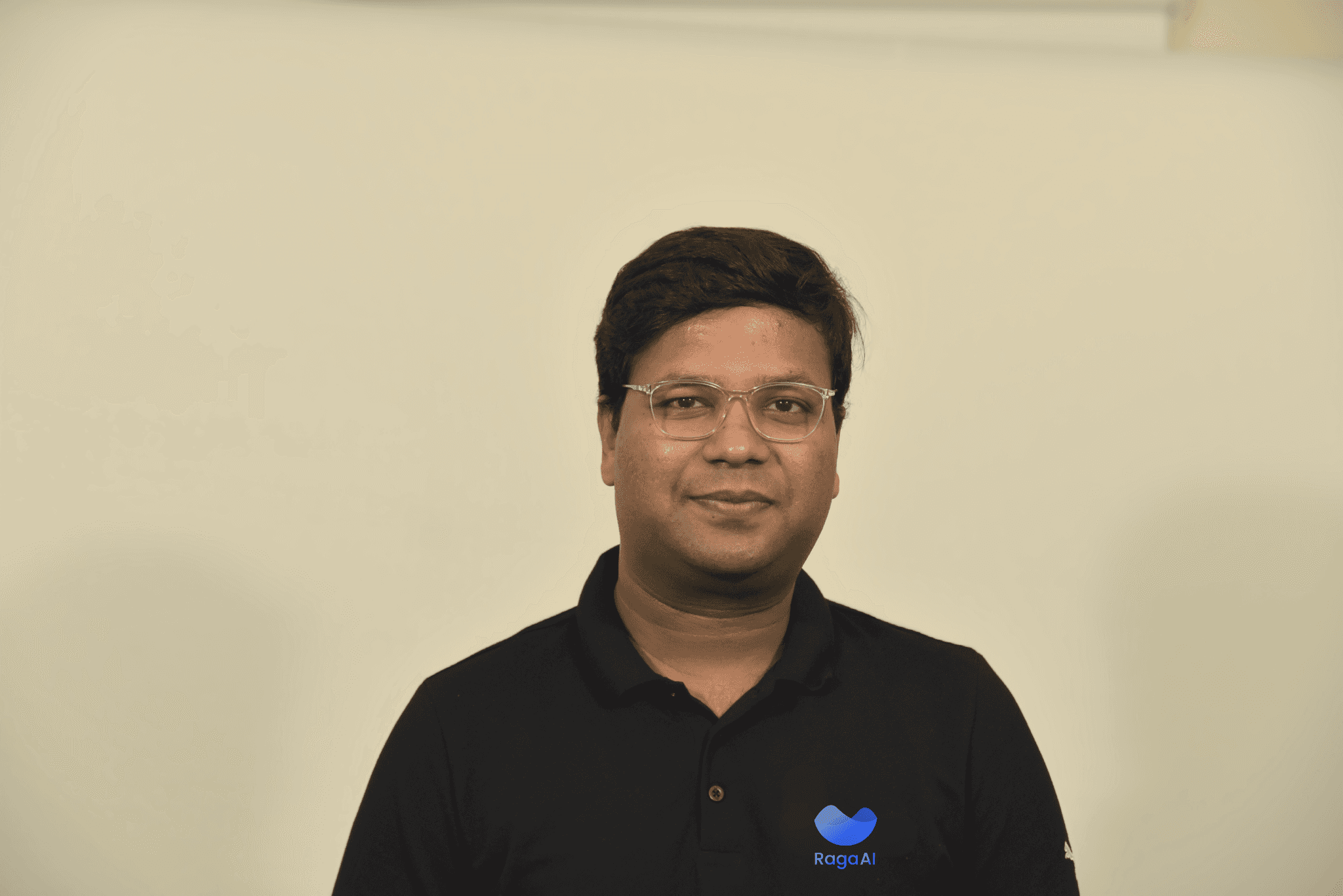
Jigar Gupta
Dec 27, 2024
Read the article
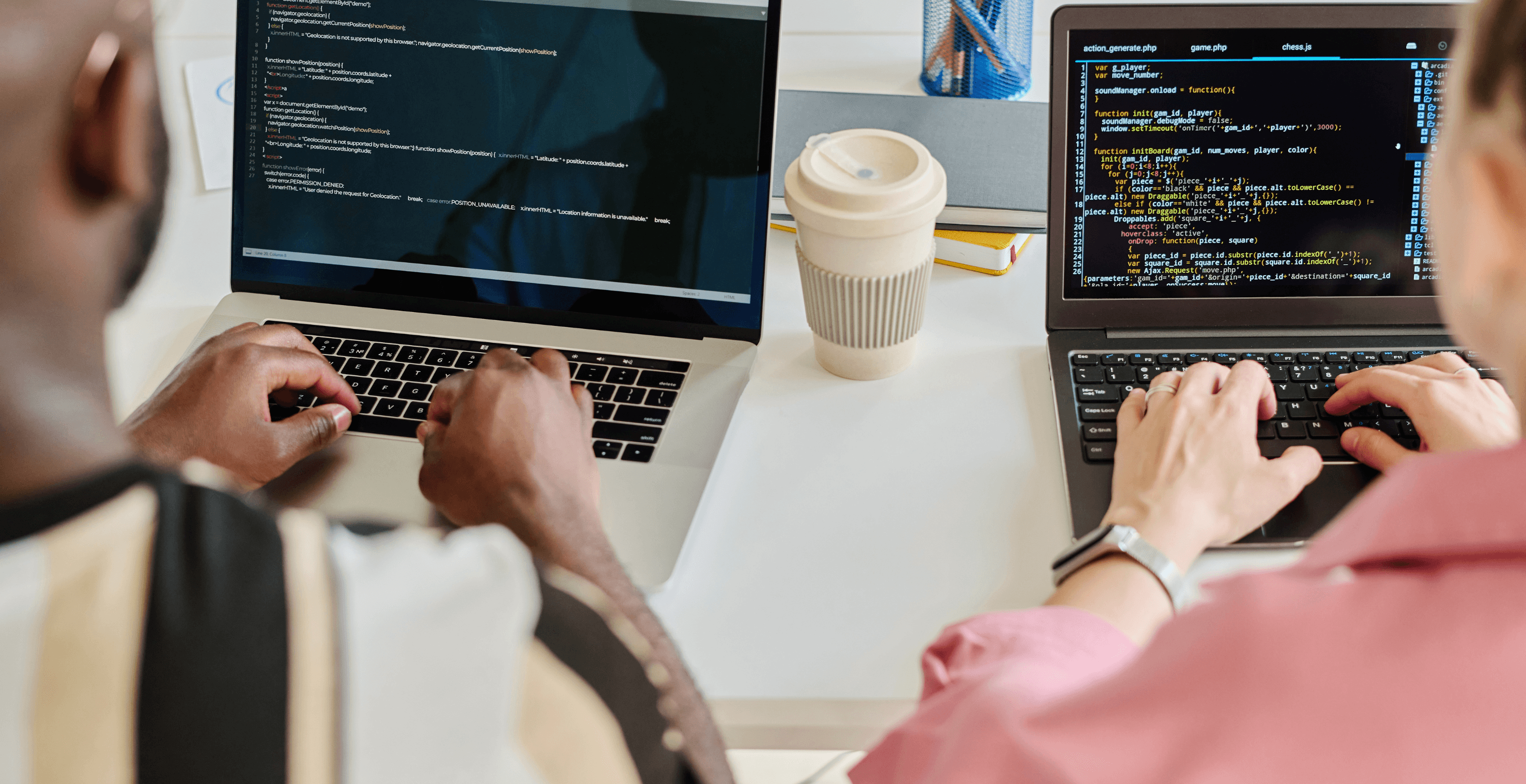
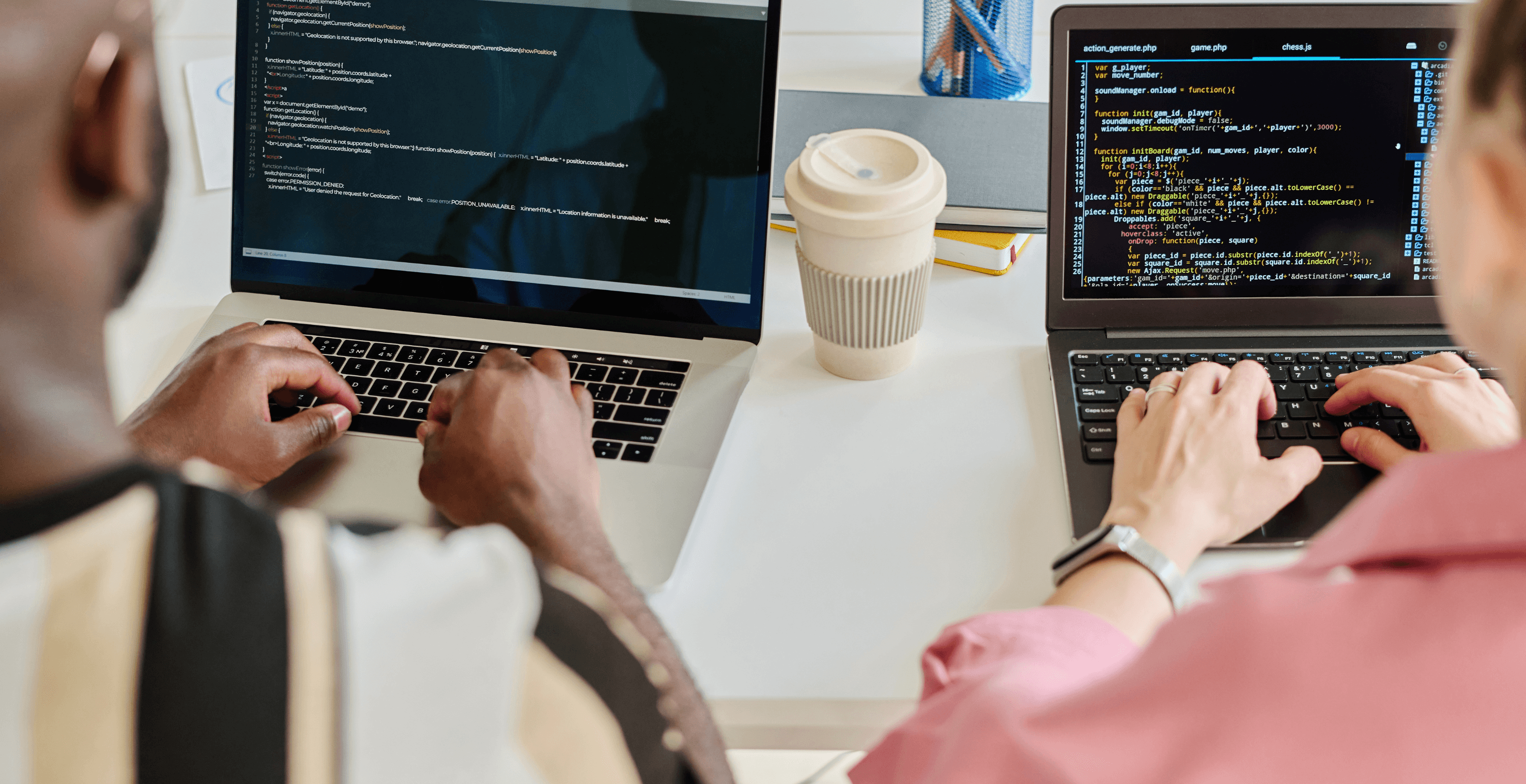
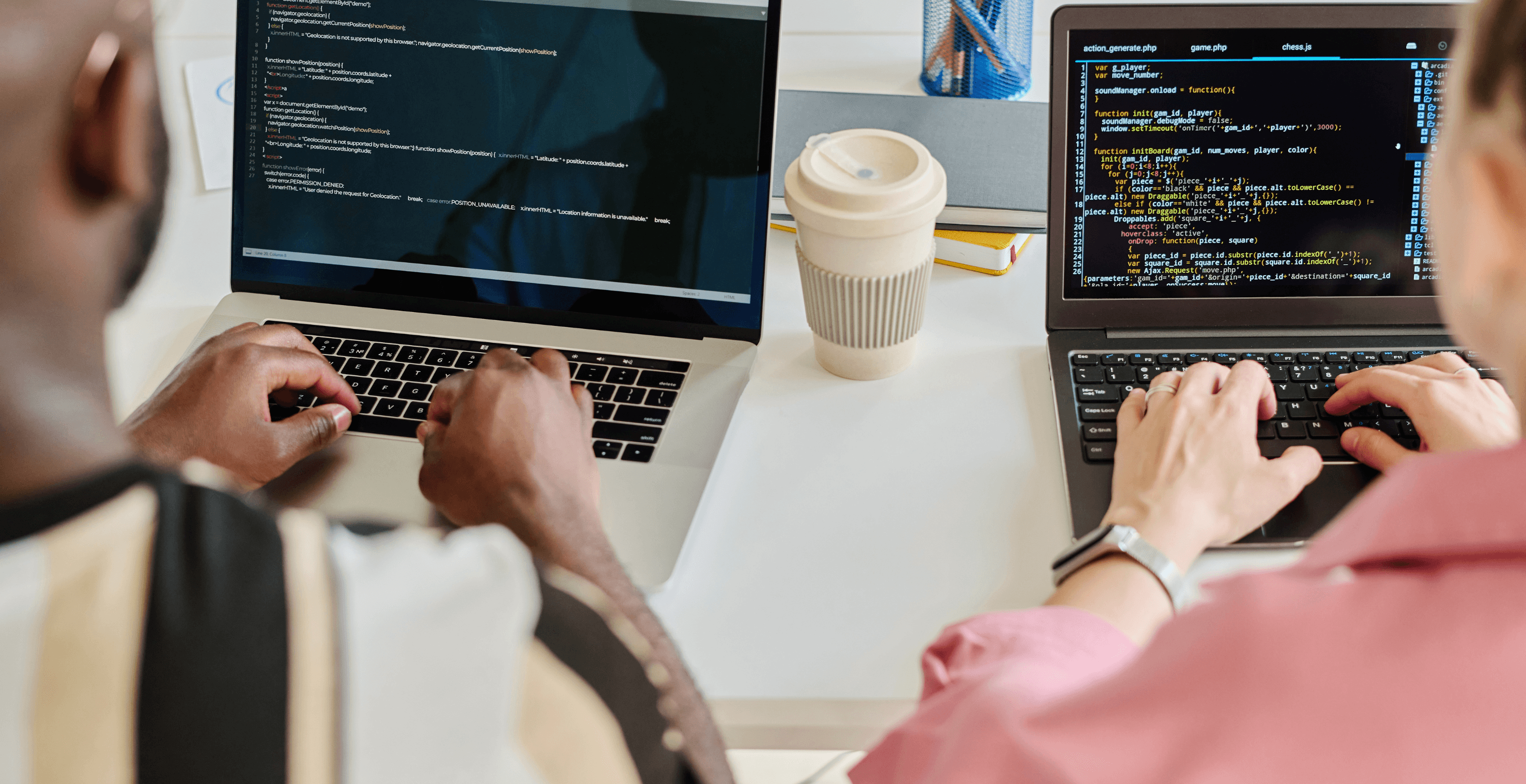
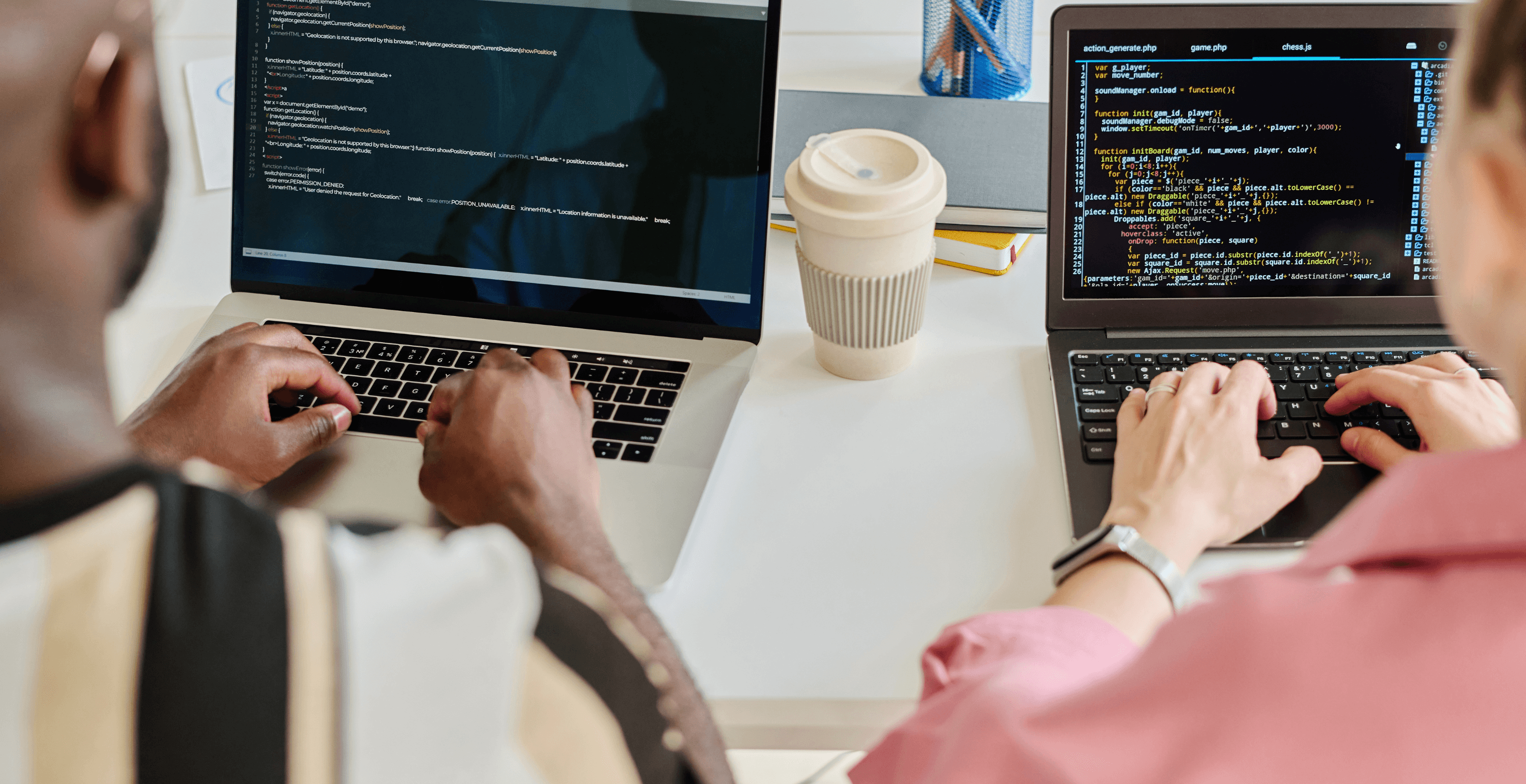
Understanding NeMo Guardrails: A Toolkit for LLM Security

Rehan Asif
Dec 24, 2024
Read the article




Understanding Differences in Large vs Small Language Models (LLM vs SLM)

Rehan Asif
Dec 21, 2024
Read the article




Understanding What an AI Agent is: Key Applications and Examples
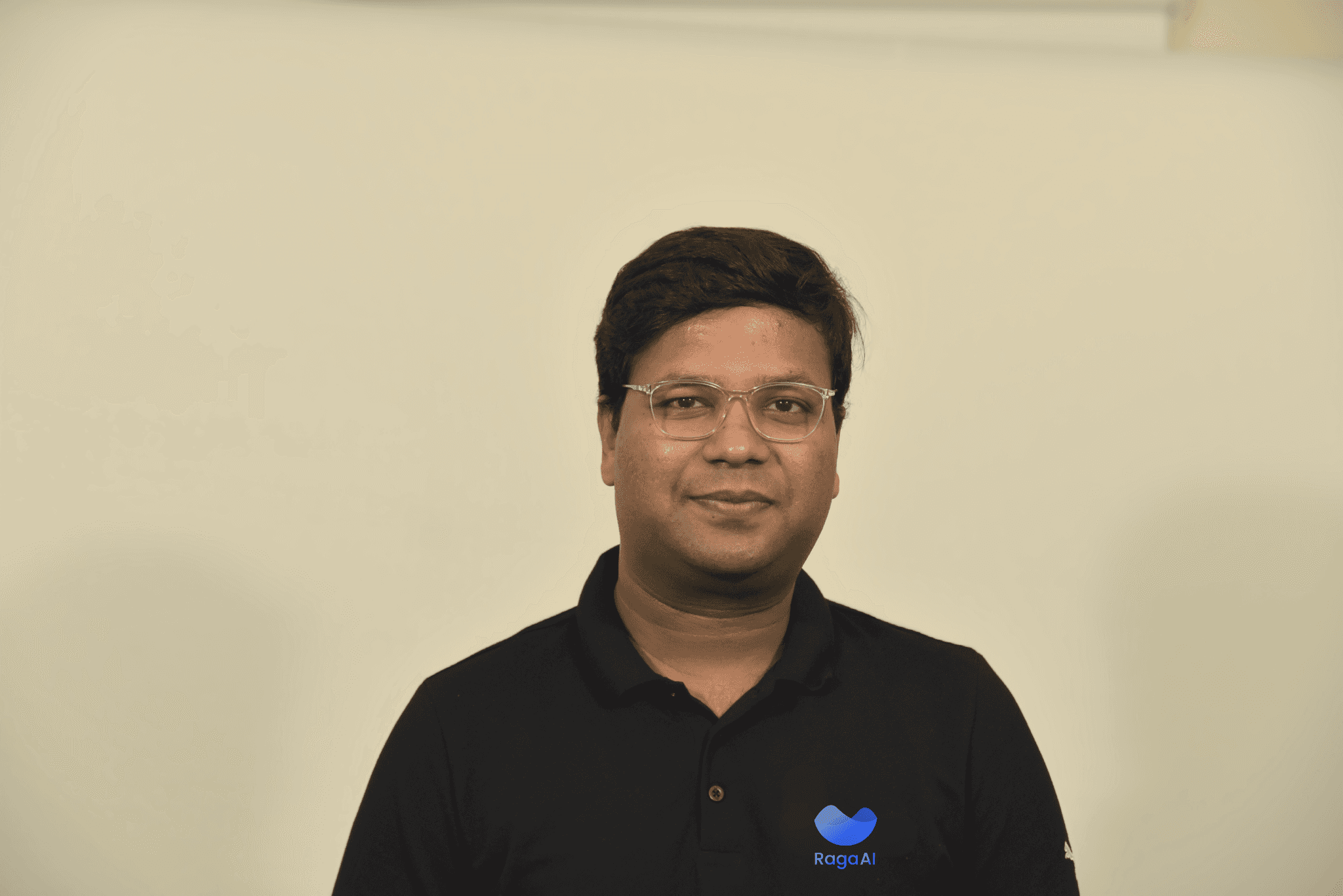
Jigar Gupta
Dec 17, 2024
Read the article




Prompt Engineering and Retrieval Augmented Generation (RAG)
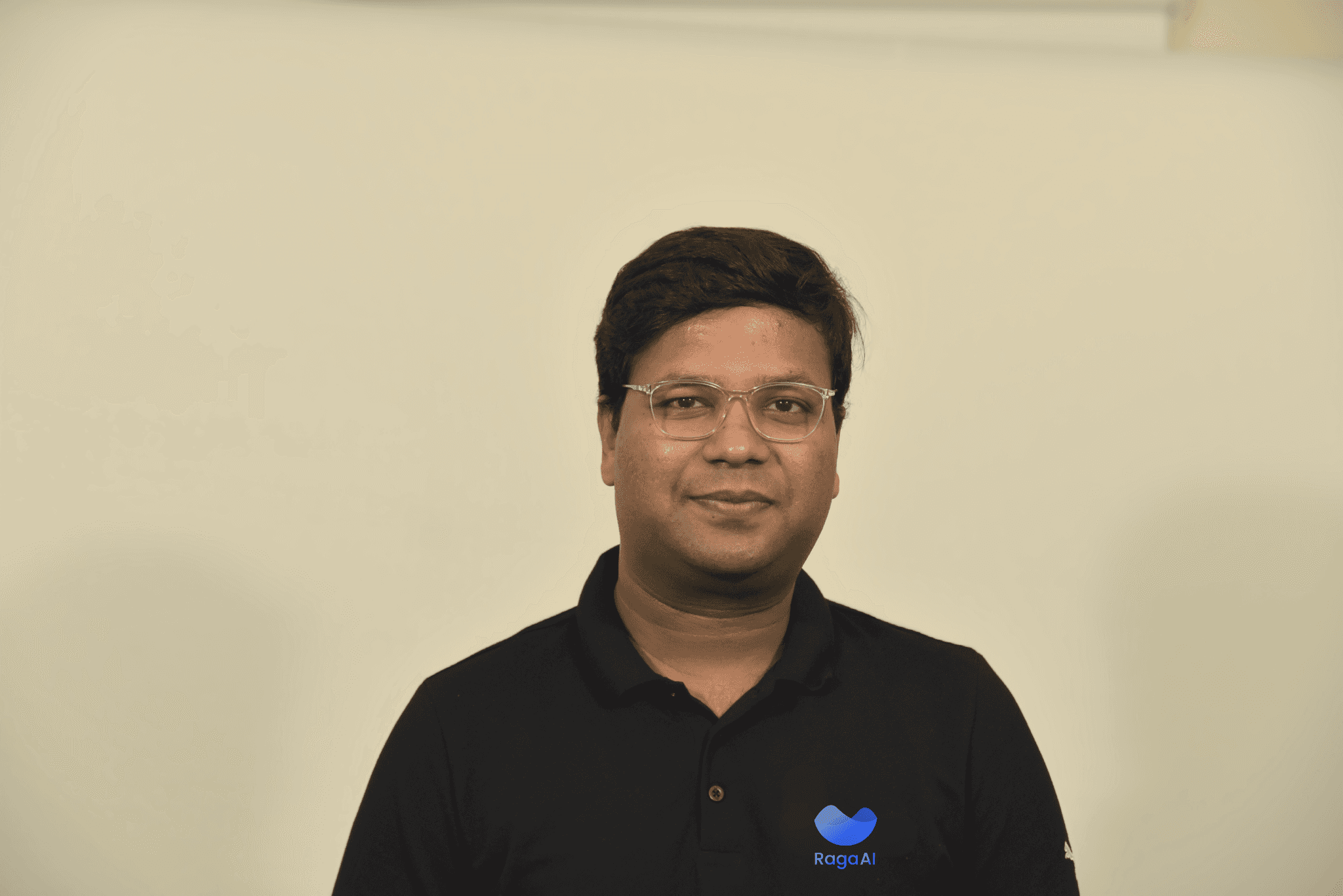
Jigar Gupta
Dec 12, 2024
Read the article




Exploring How Multimodal Large Language Models Work

Rehan Asif
Dec 9, 2024
Read the article
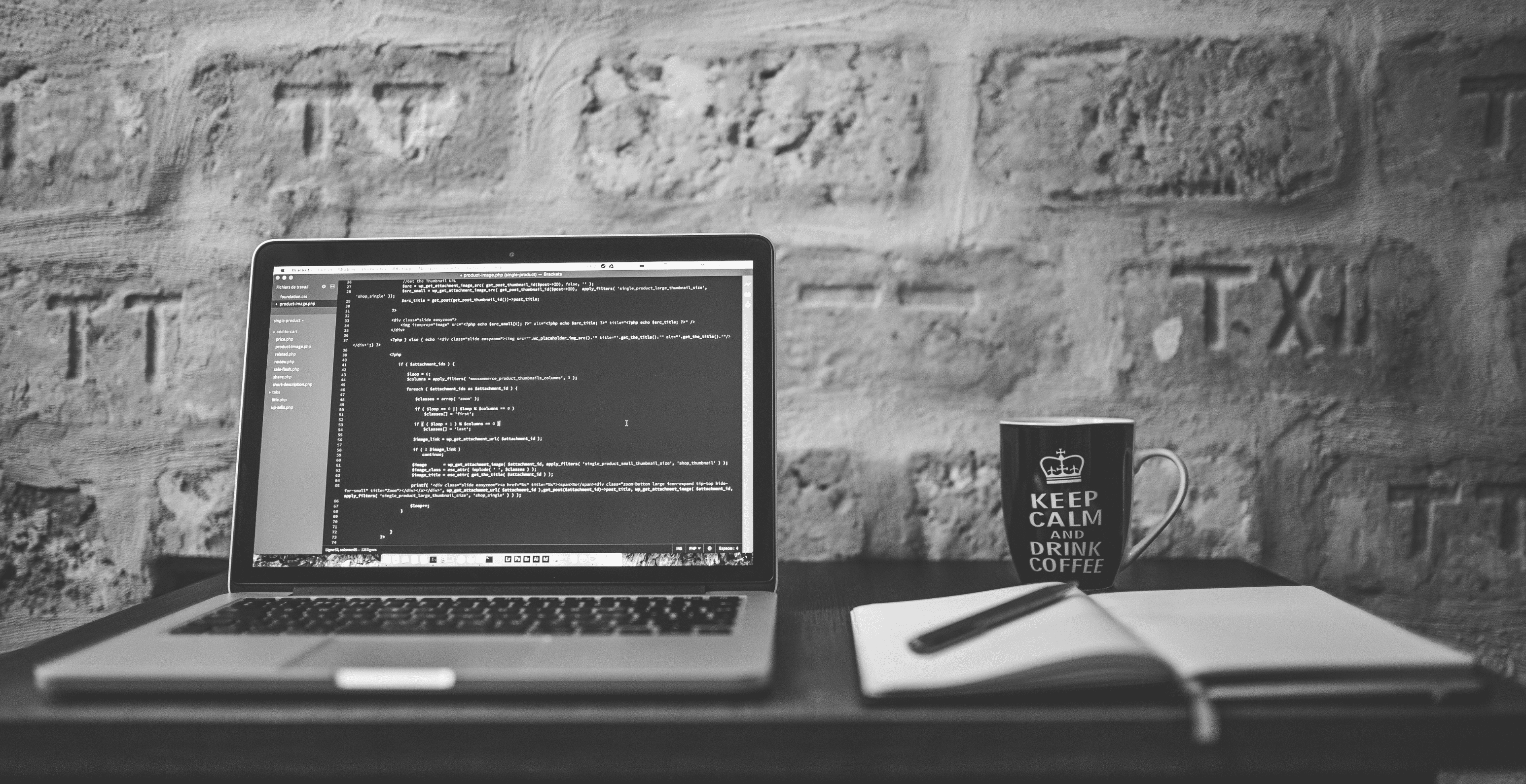
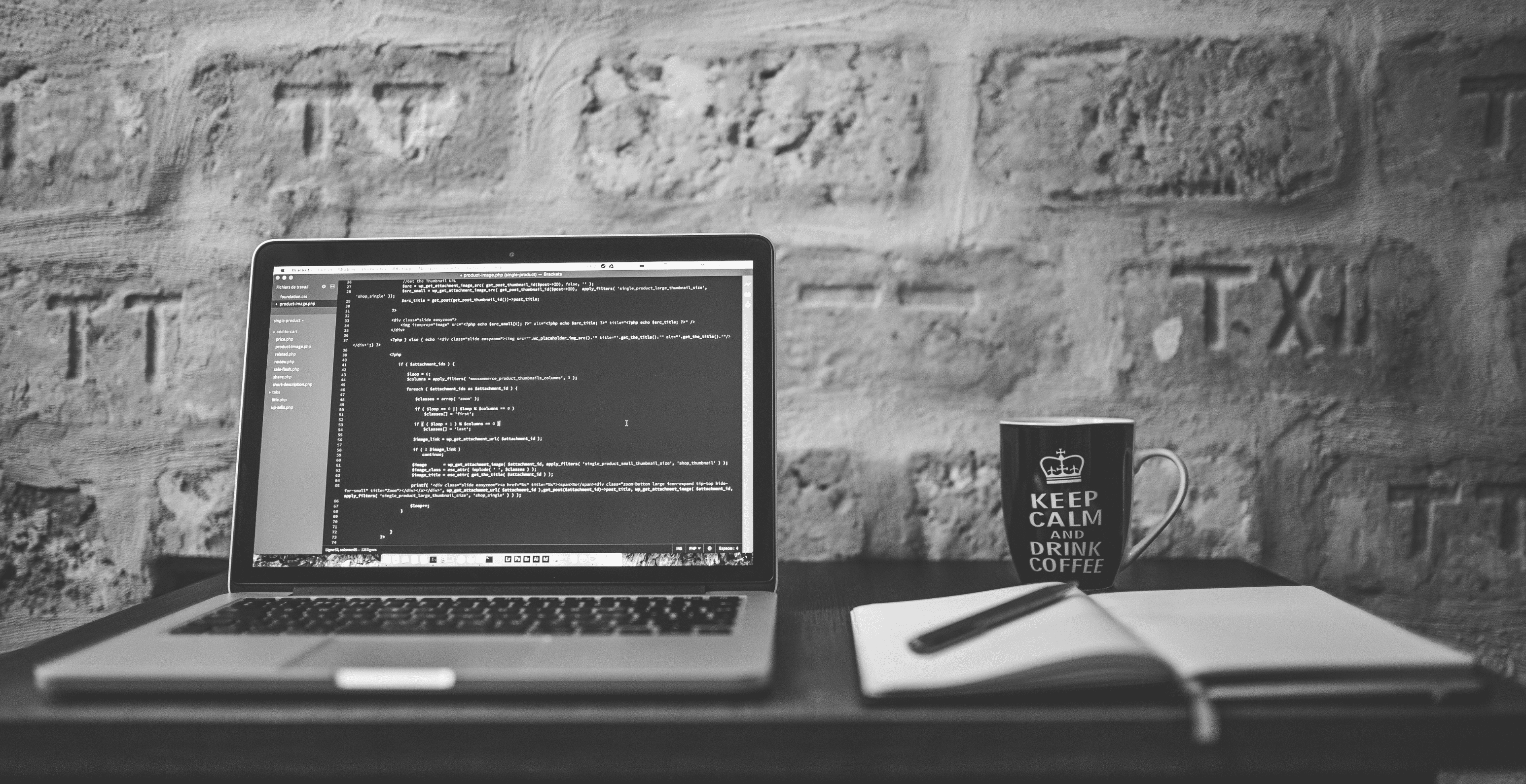
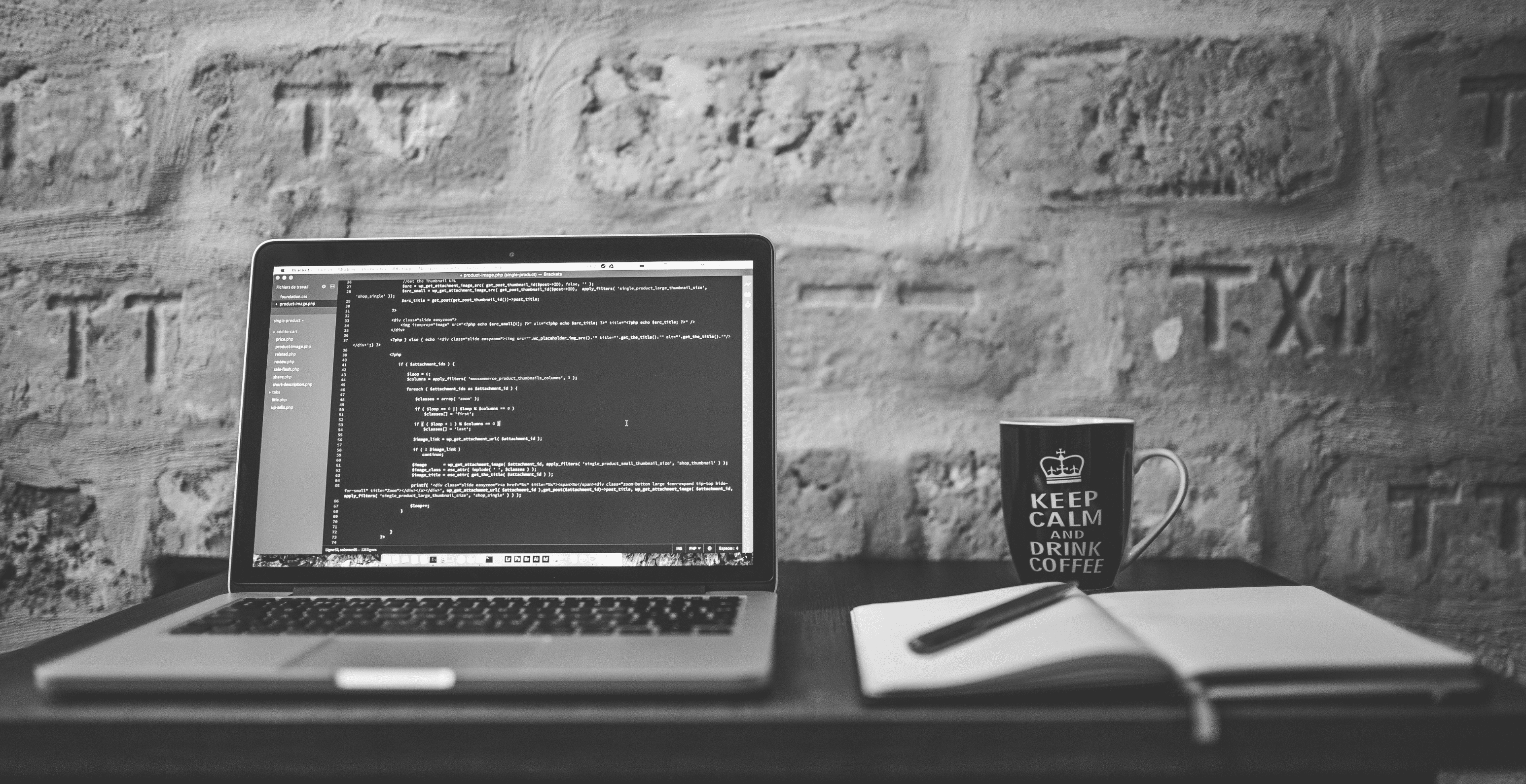
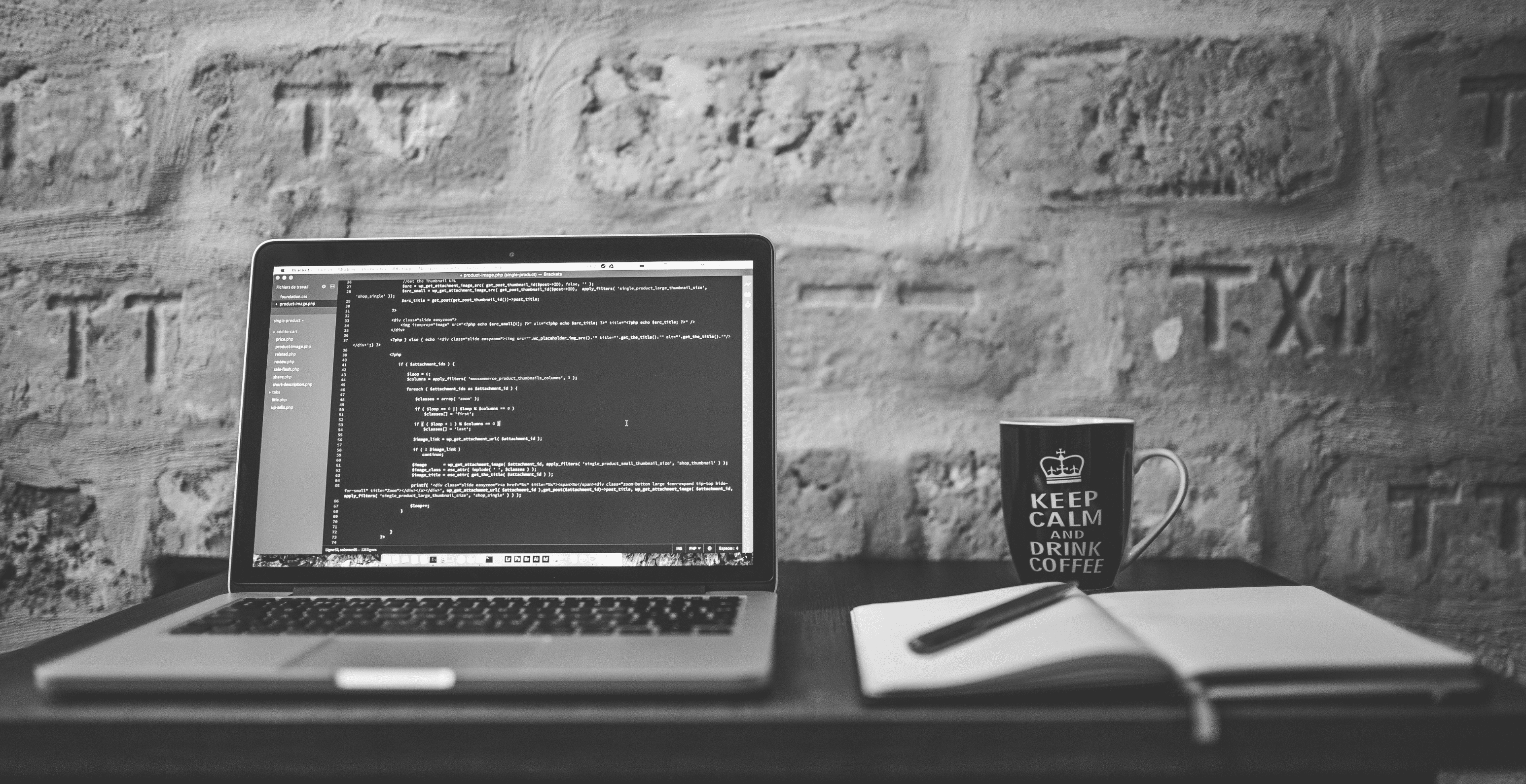
Evaluating and Enhancing LLM-as-a-Judge with Automated Tools

Rehan Asif
Dec 6, 2024
Read the article




Optimizing Performance and Cost by Caching LLM Queries

Rehan Asif
Dec 3, 2024
Read the article




LoRA vs RAG: Full Model Fine-Tuning in Large Language Models
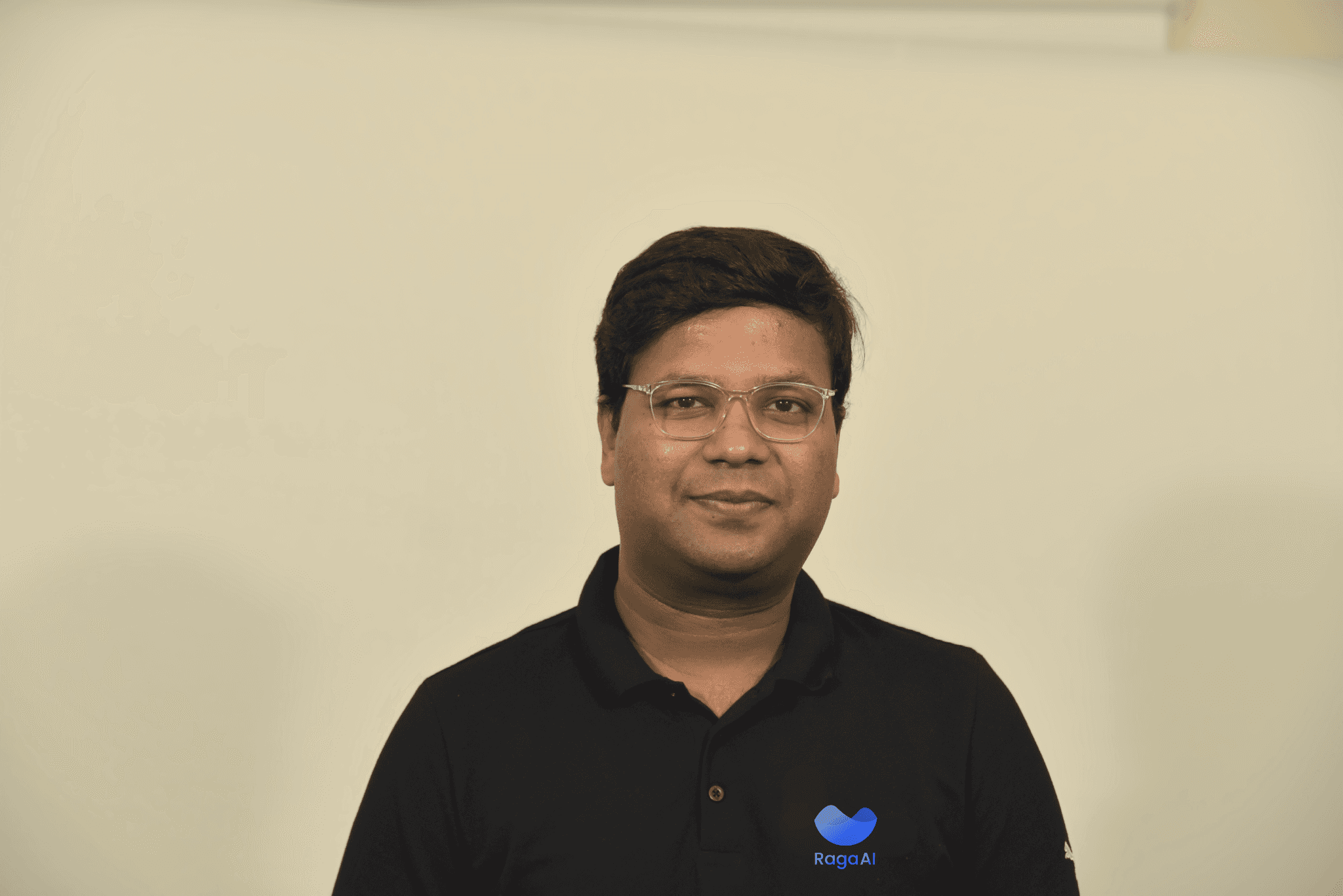
Jigar Gupta
Nov 30, 2024
Read the article
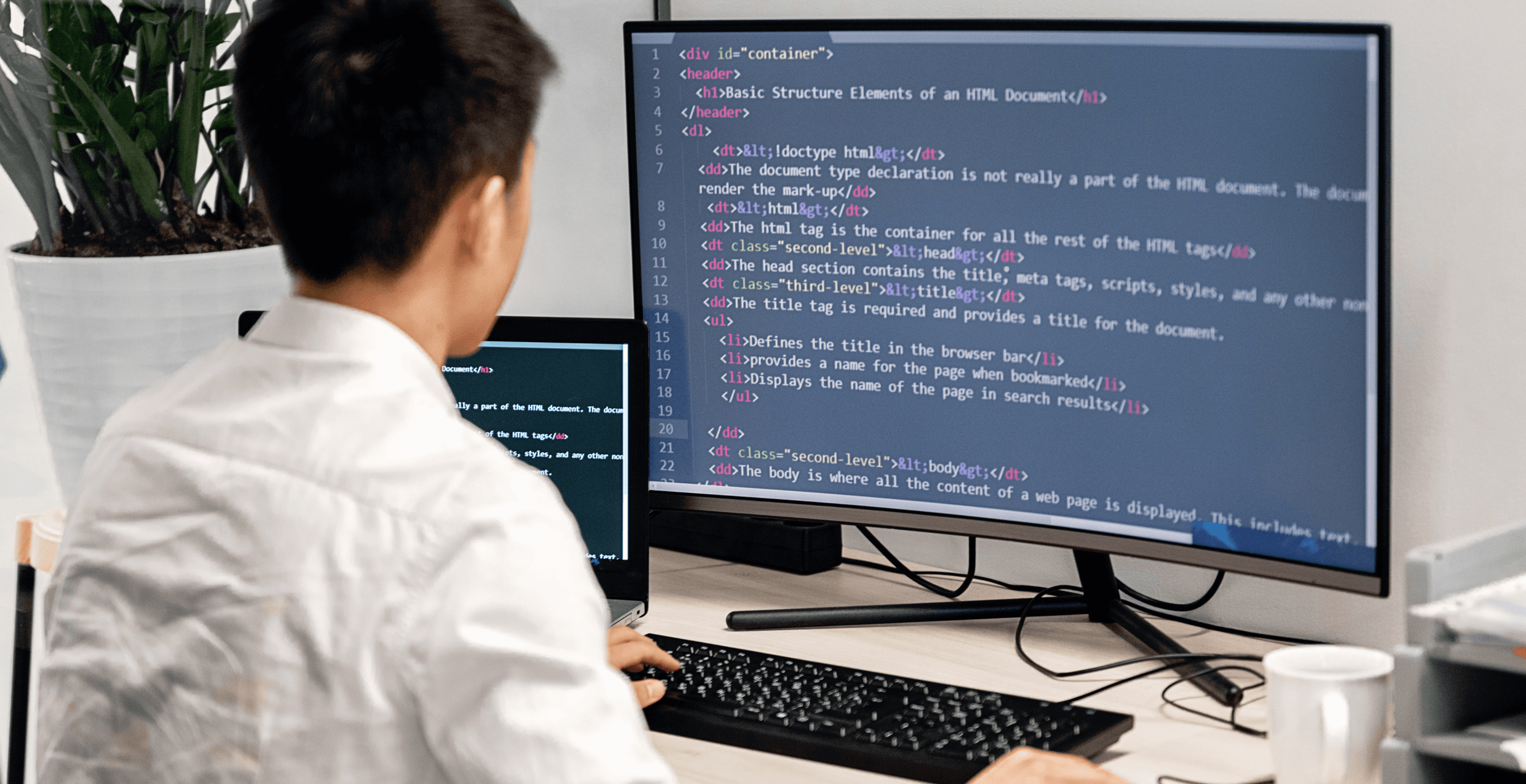
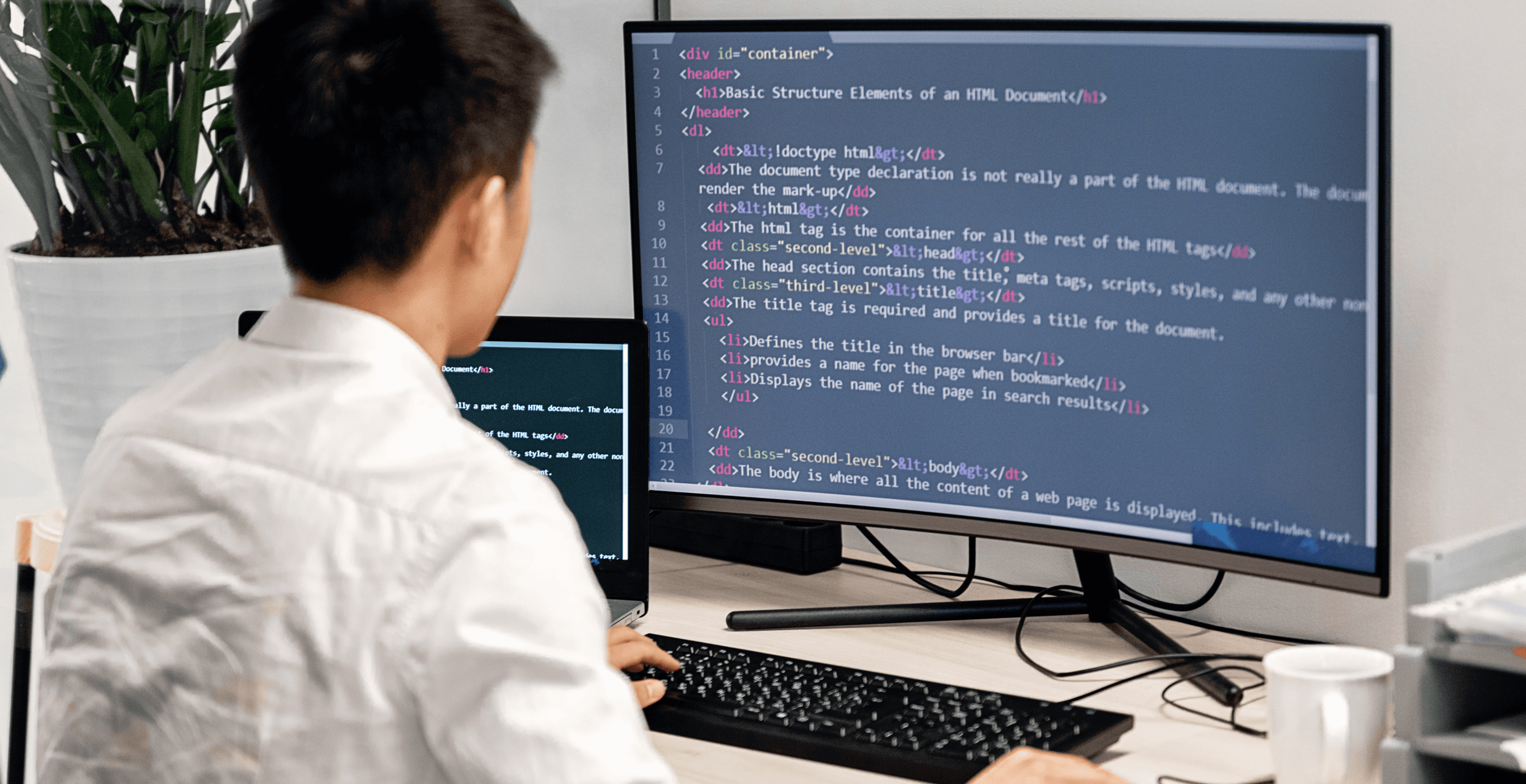
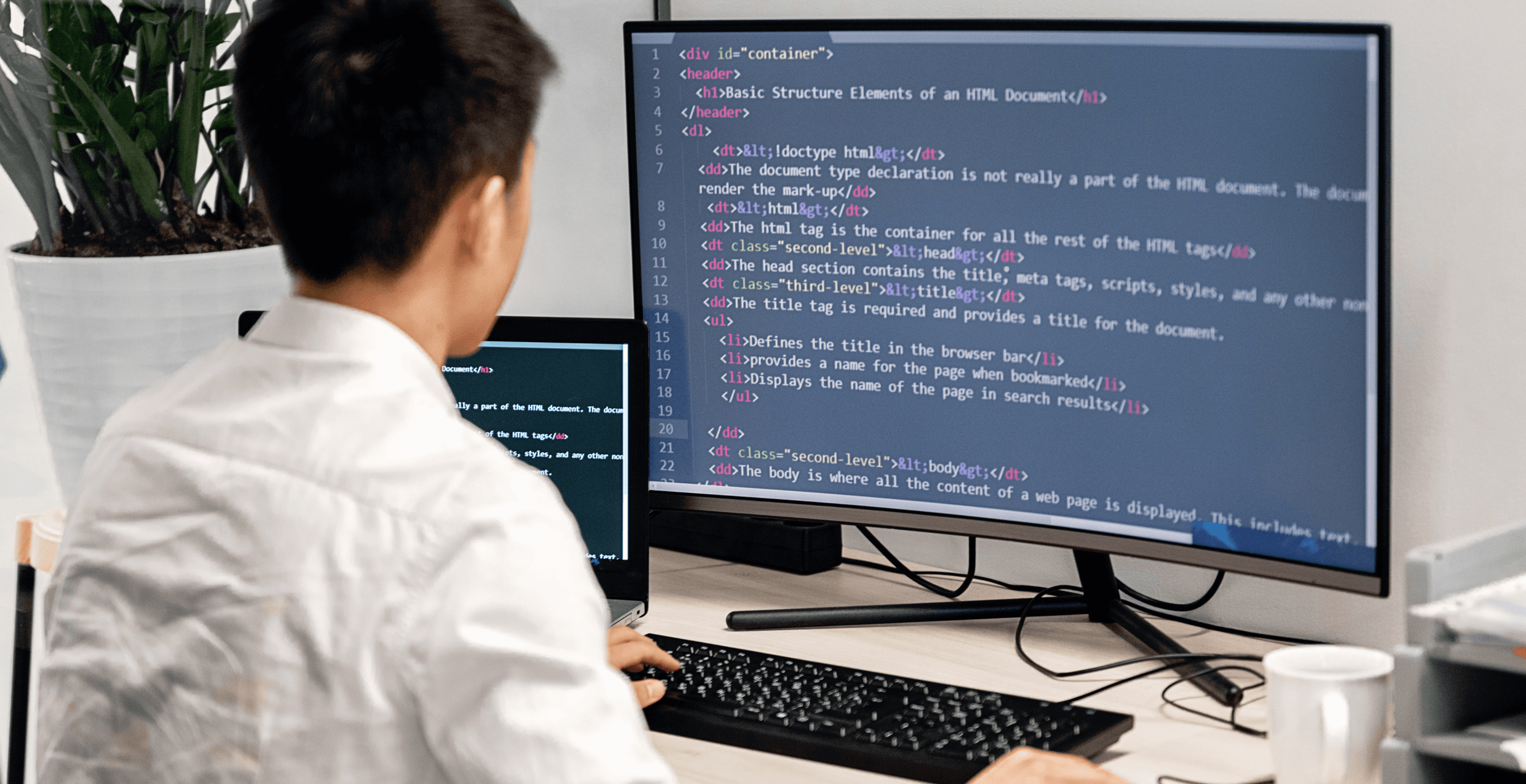
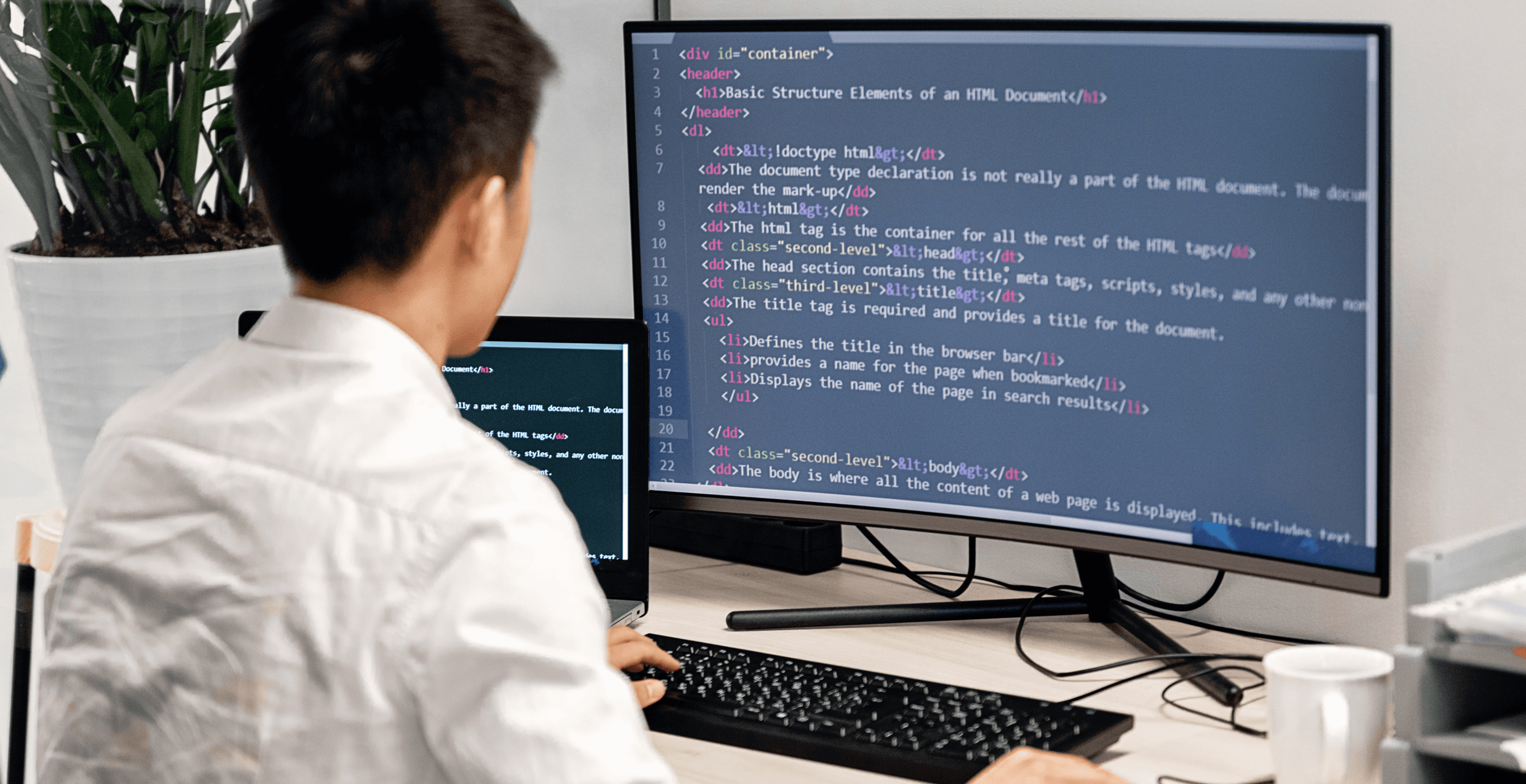
Steps to Train LLM on Personal Data

Rehan Asif
Nov 28, 2024
Read the article




Step by Step Guide to Building RAG-based LLM Applications with Examples

Rehan Asif
Nov 27, 2024
Read the article
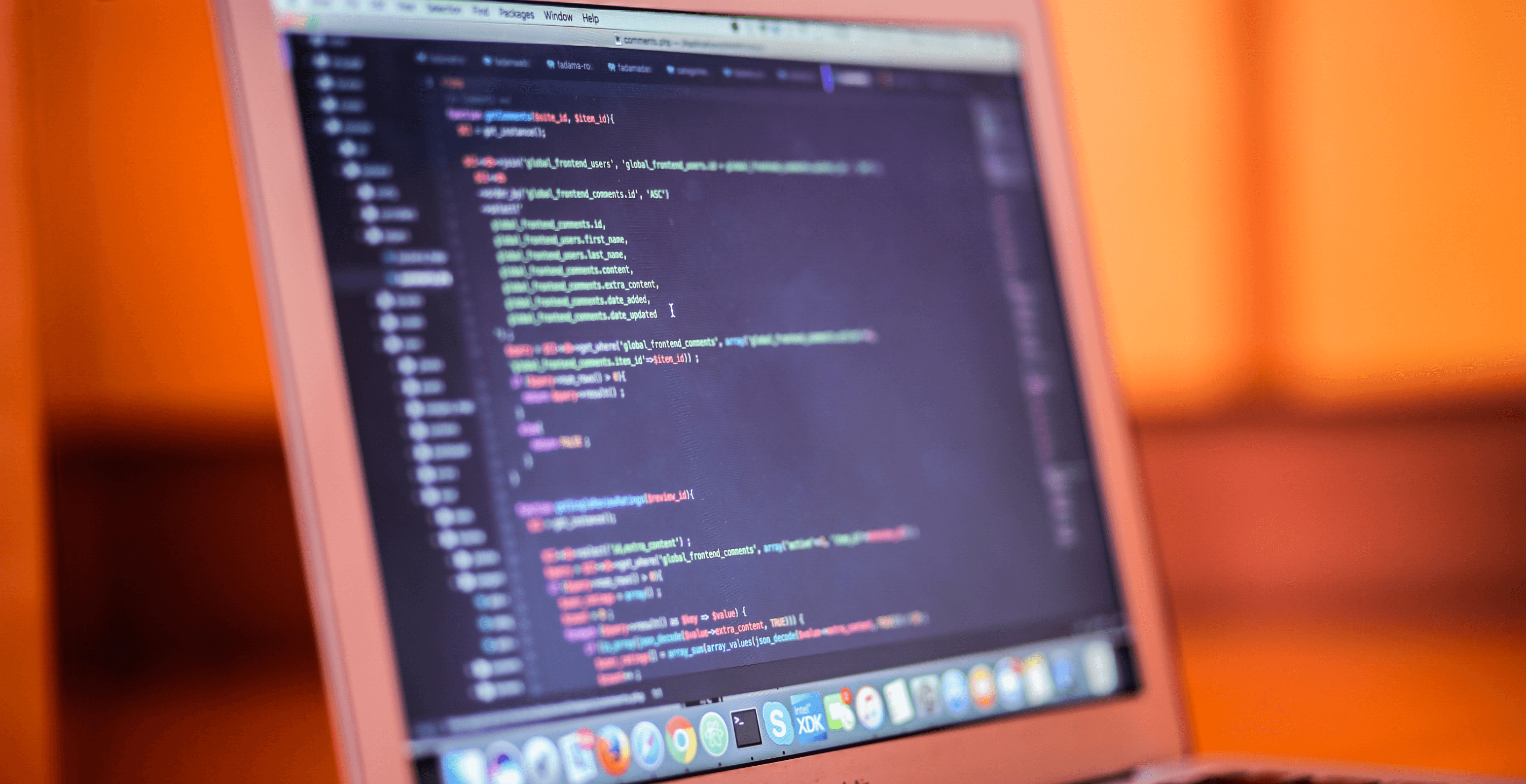
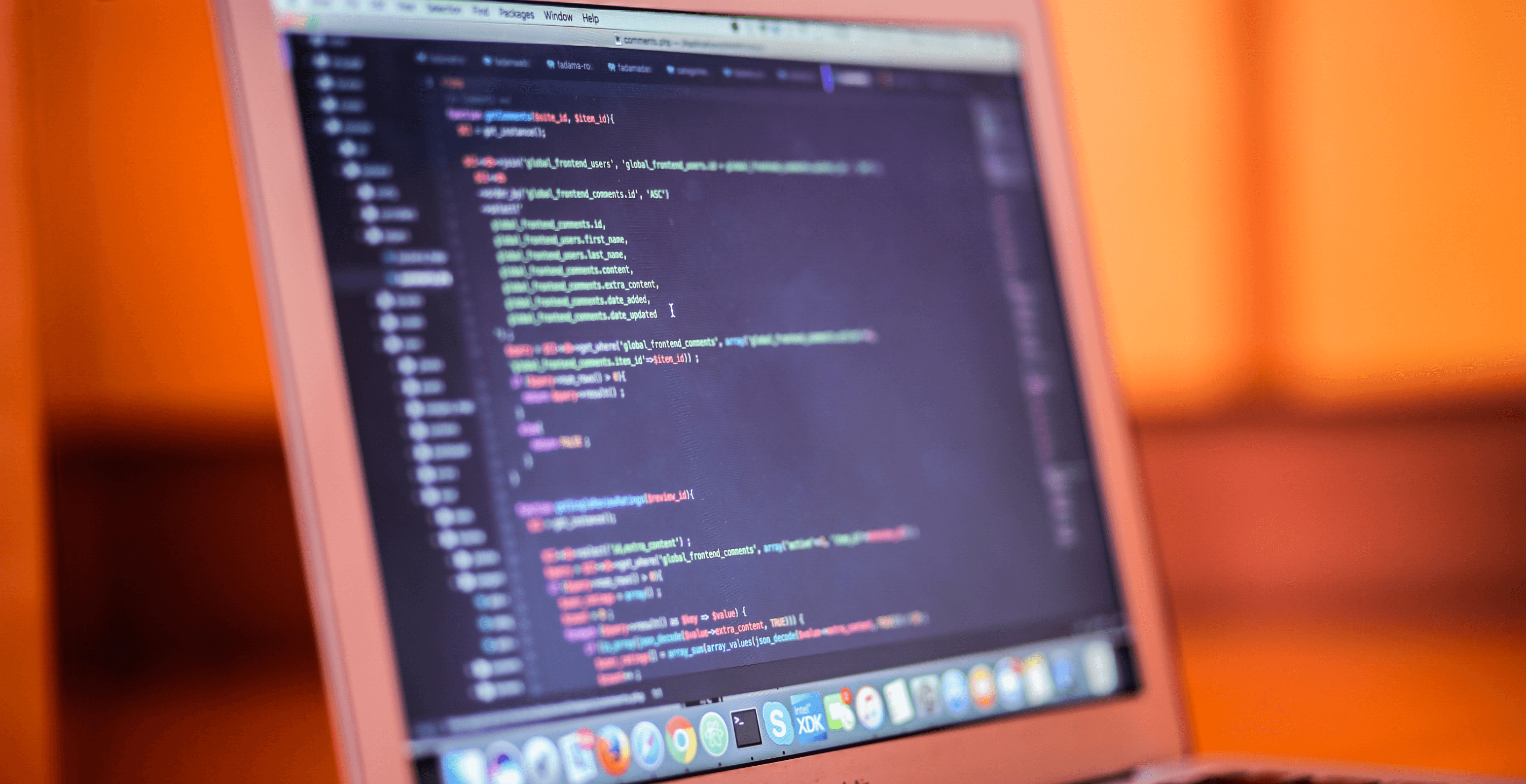
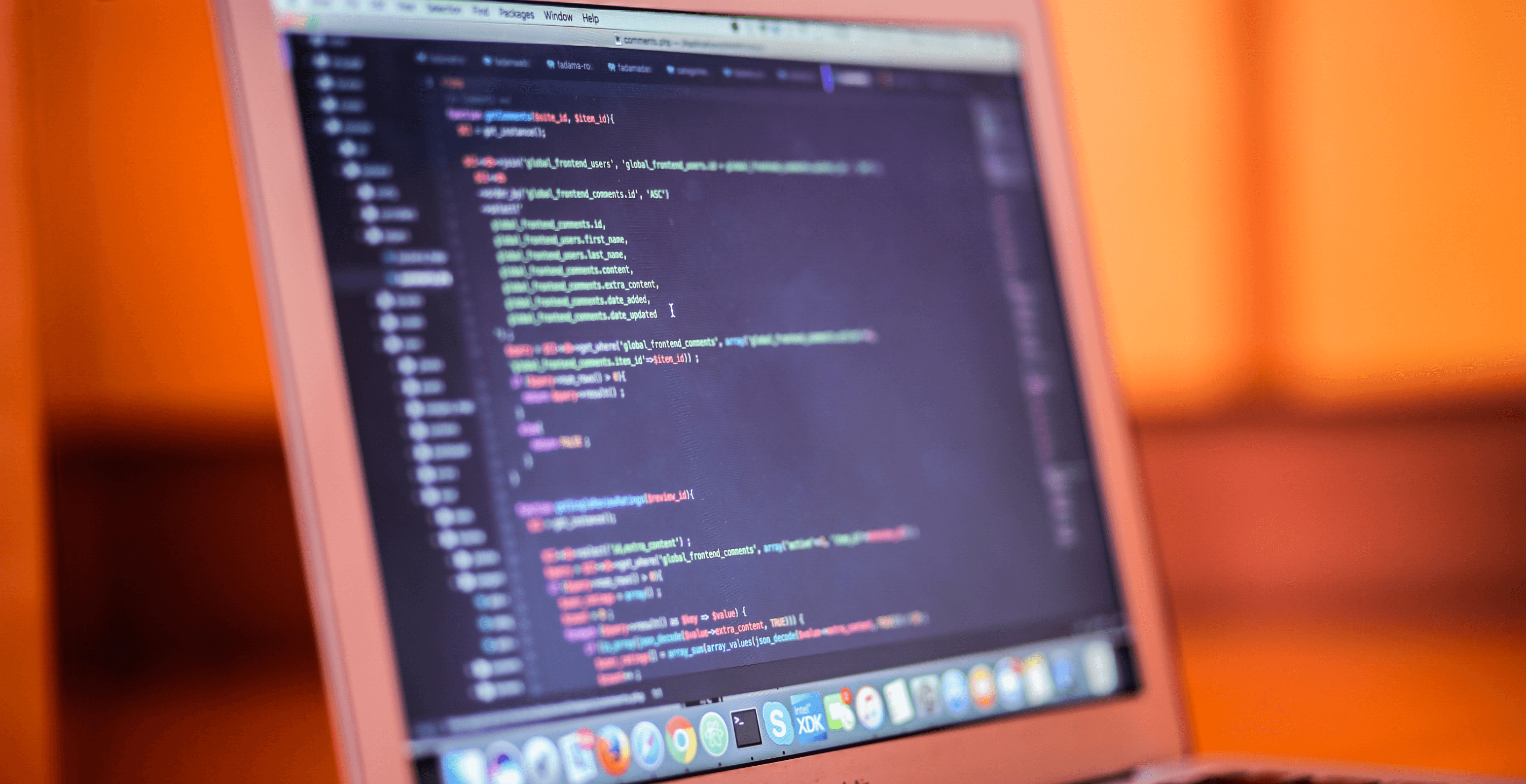
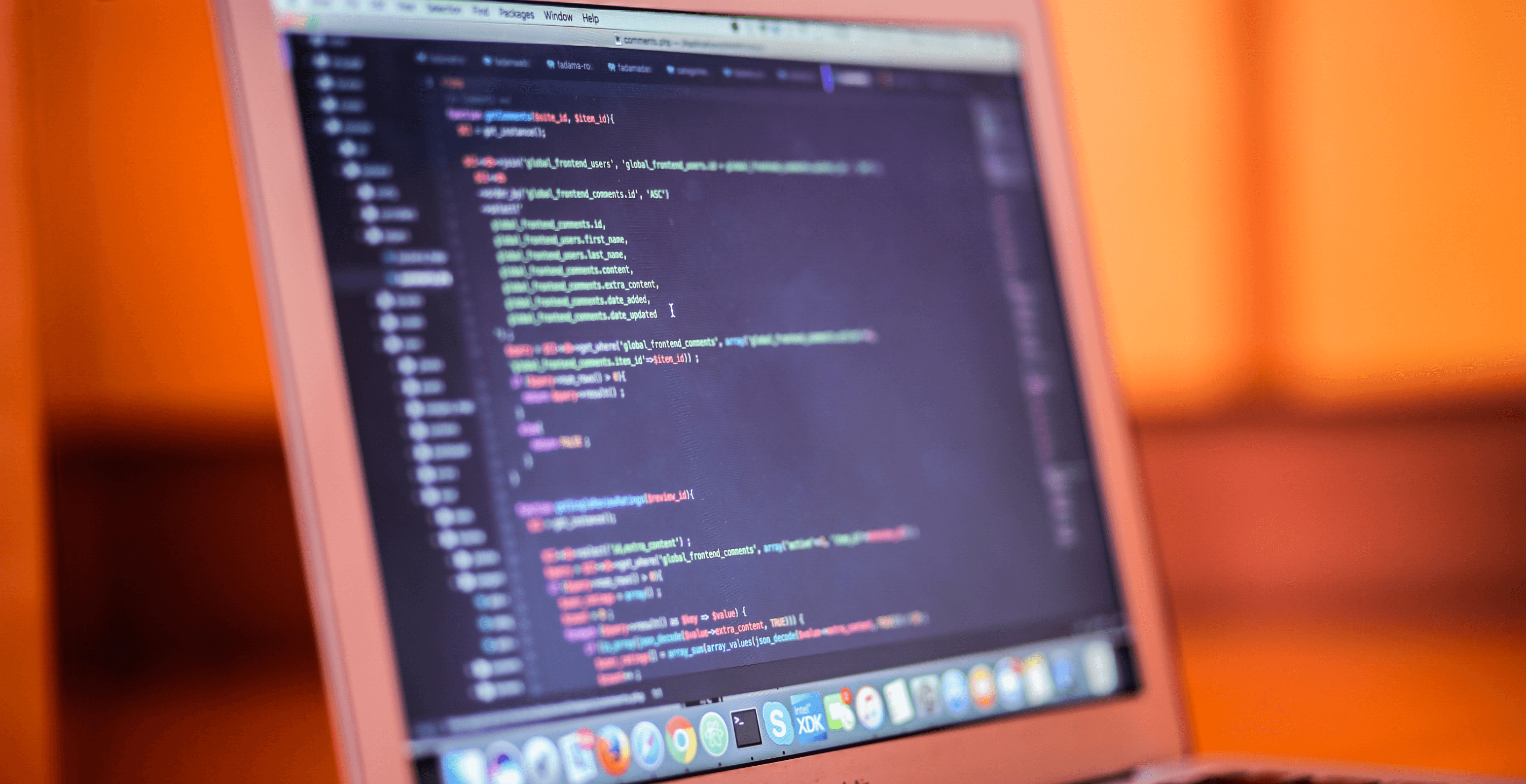
Building AI Agentic Workflows with Multi-Agent Collaboration
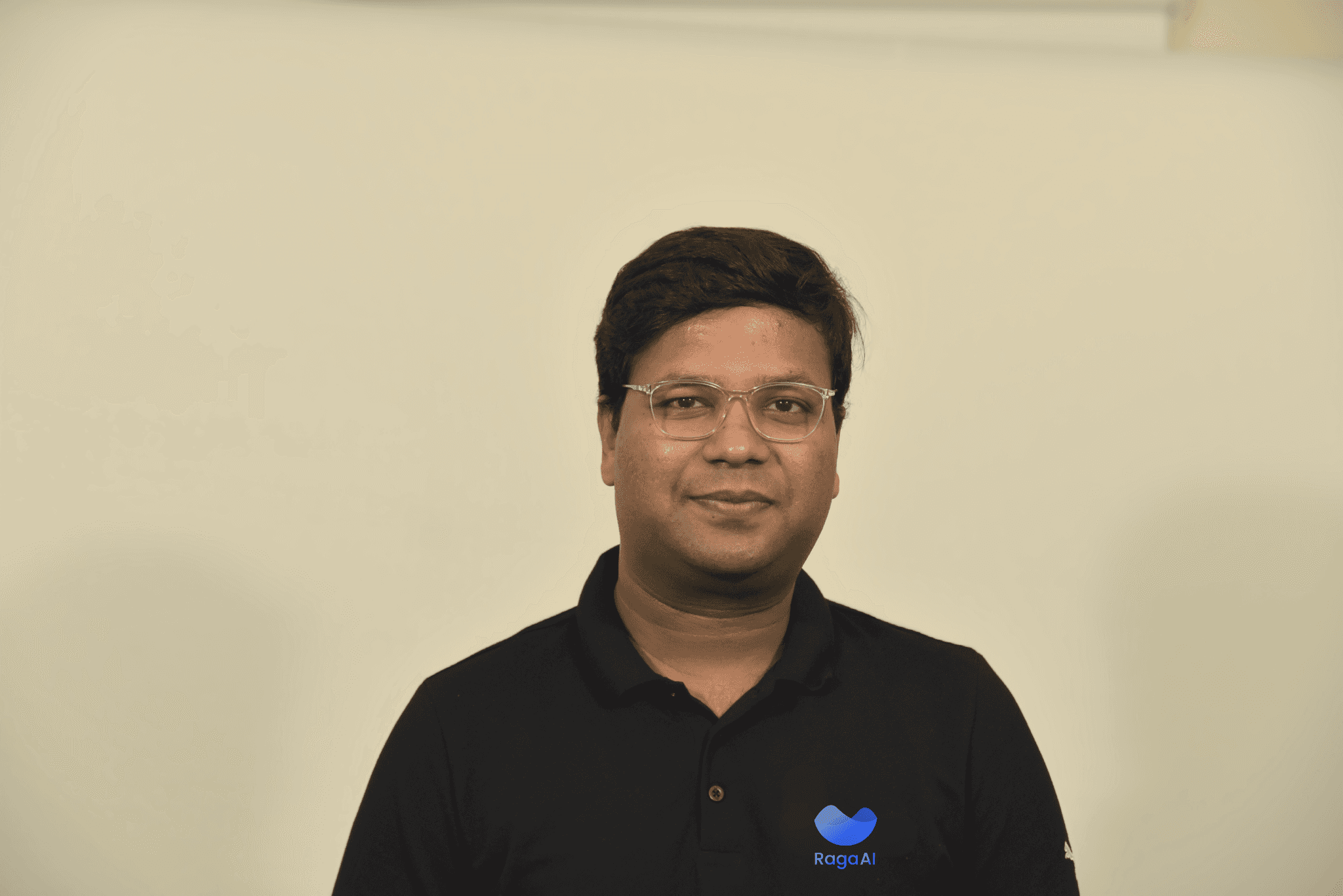
Jigar Gupta
Nov 25, 2024
Read the article




Top Large Language Models (LLMs) in 2024

Rehan Asif
Nov 22, 2024
Read the article
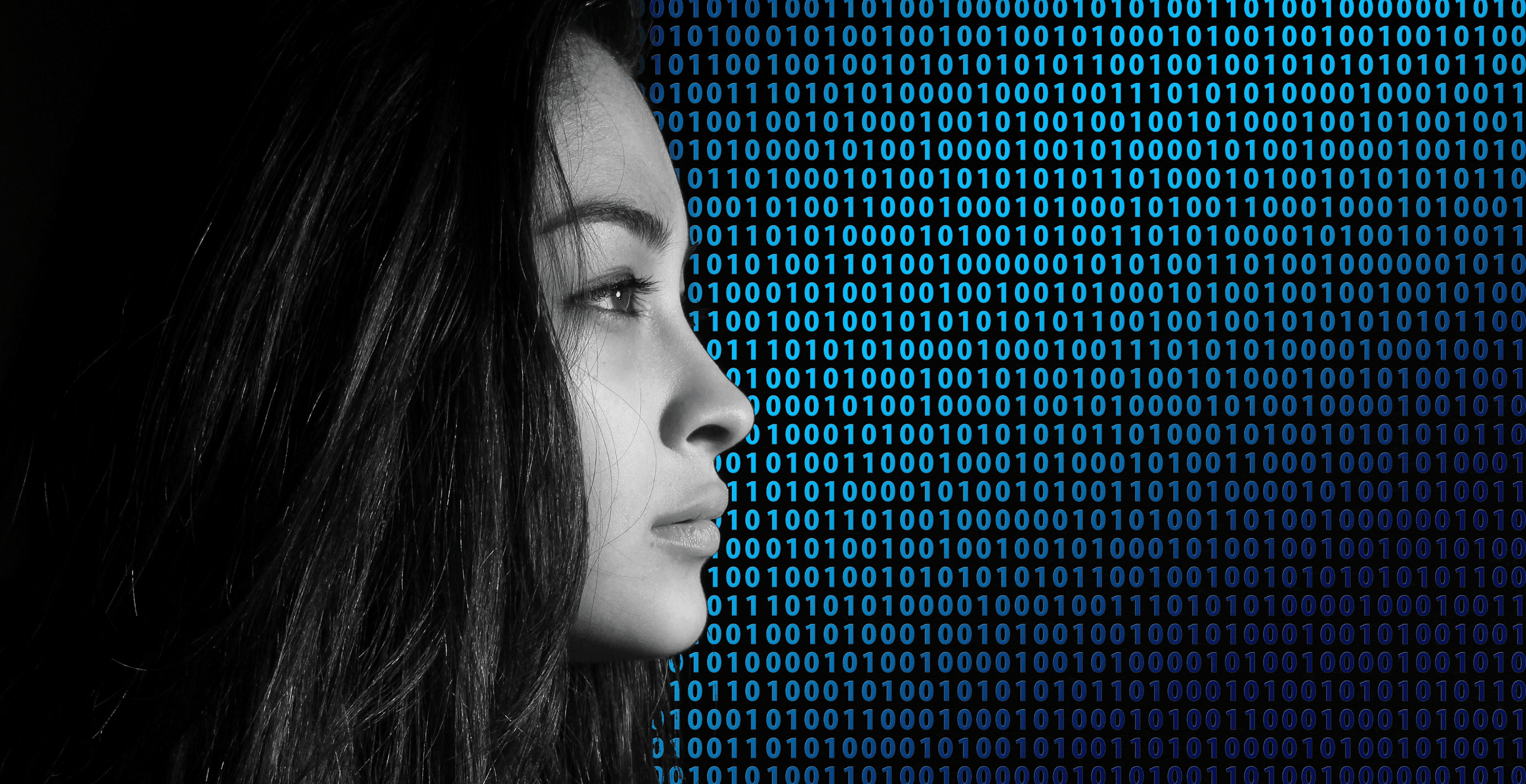
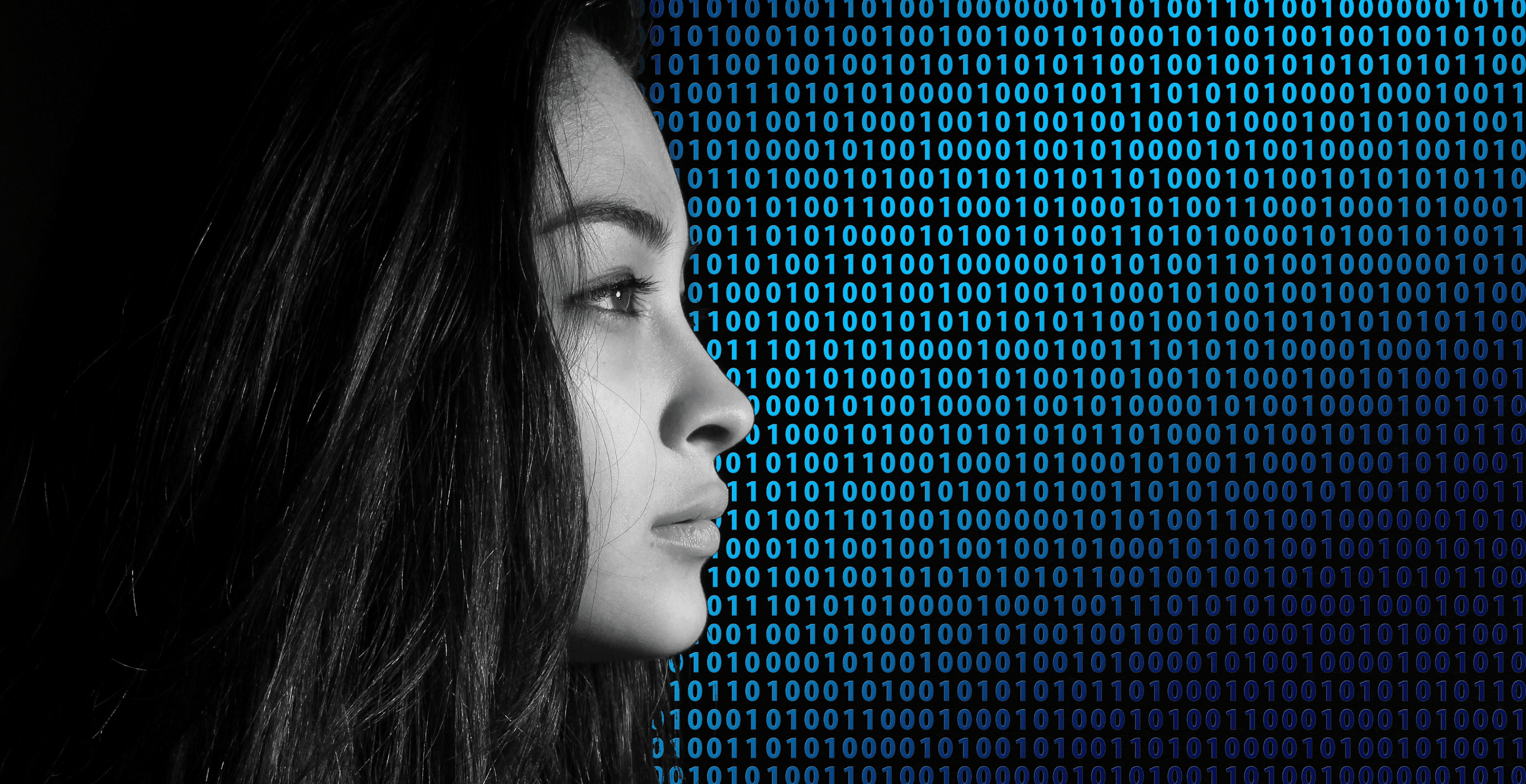
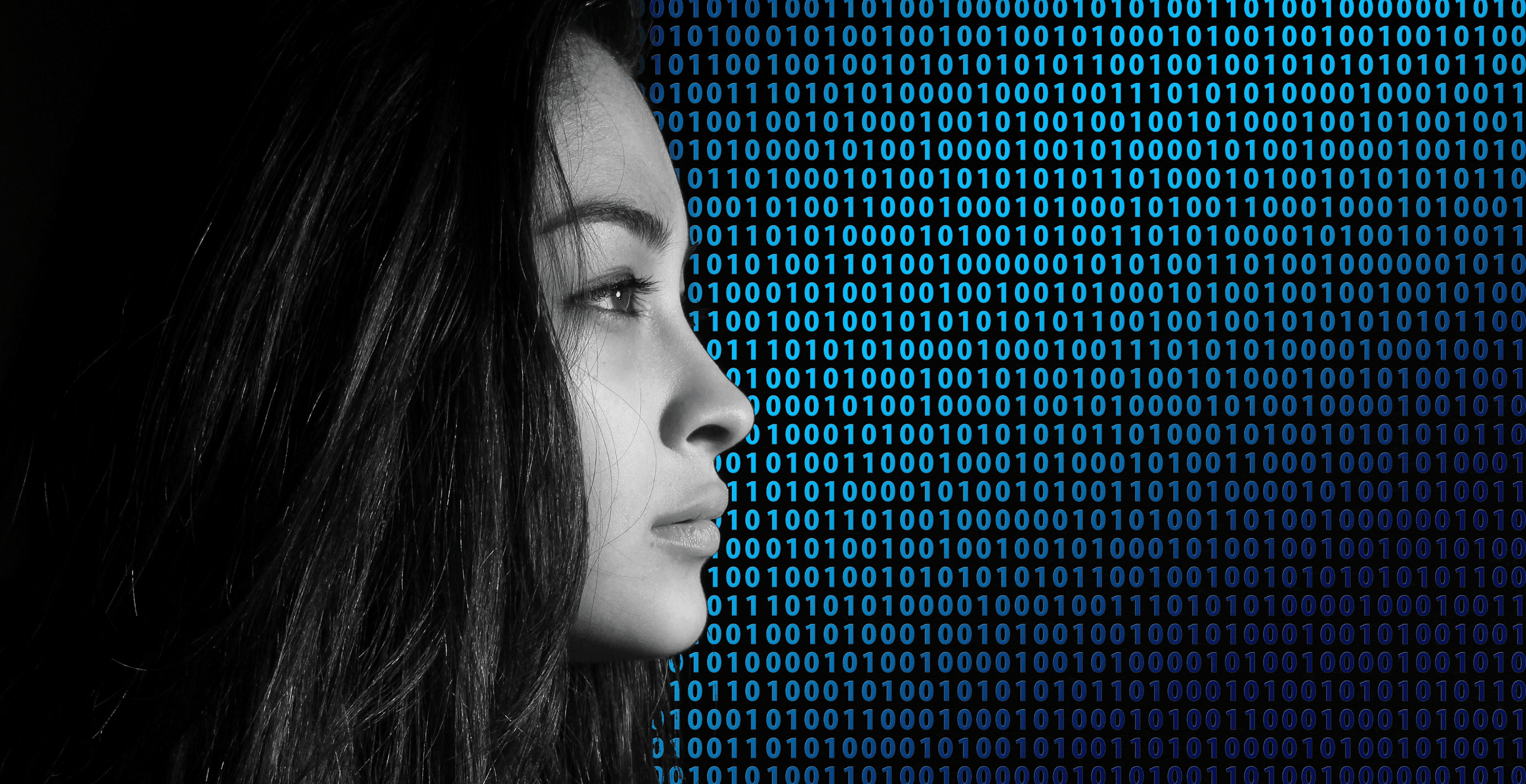
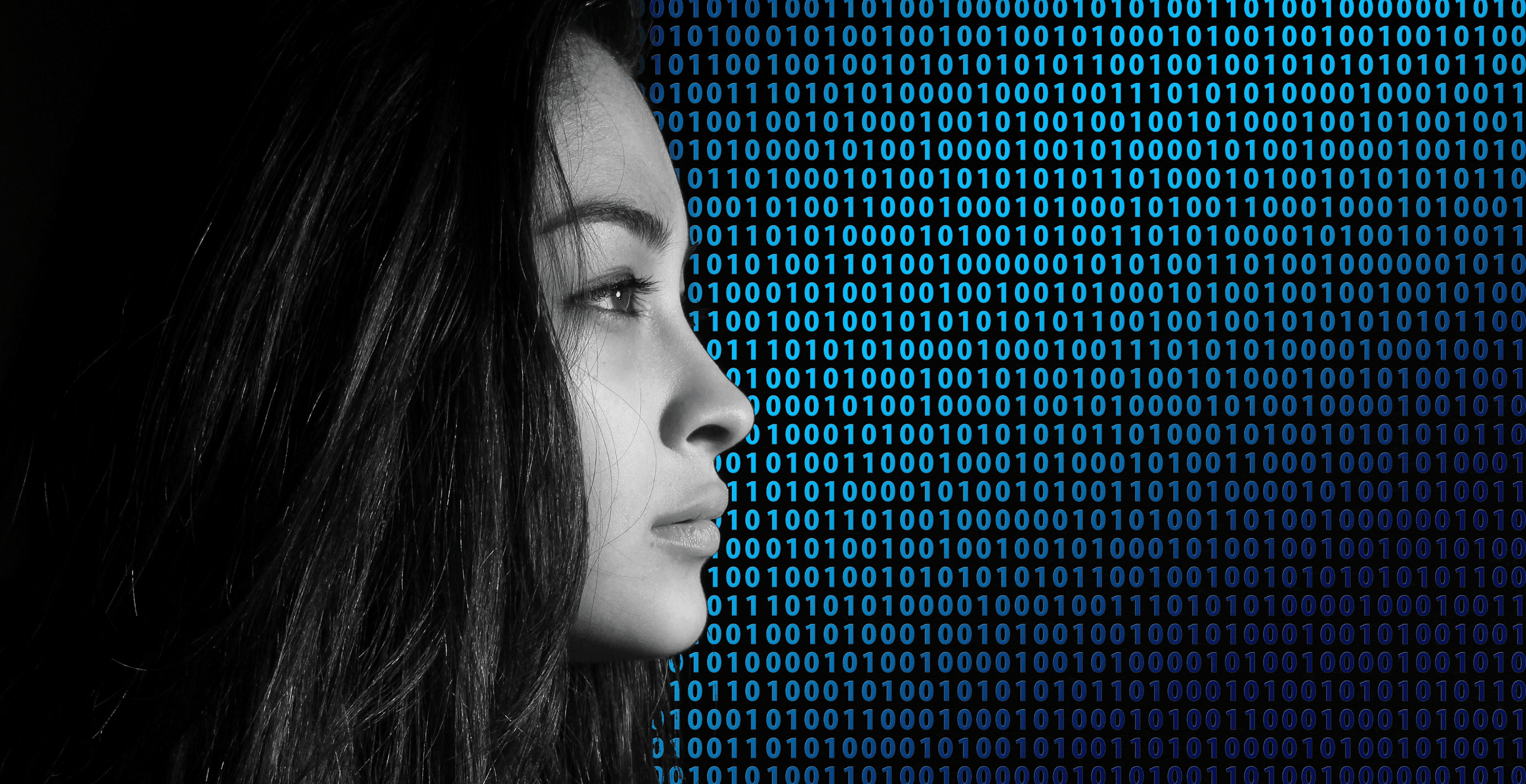
Creating Apps with Large Language Models

Rehan Asif
Nov 21, 2024
Read the article
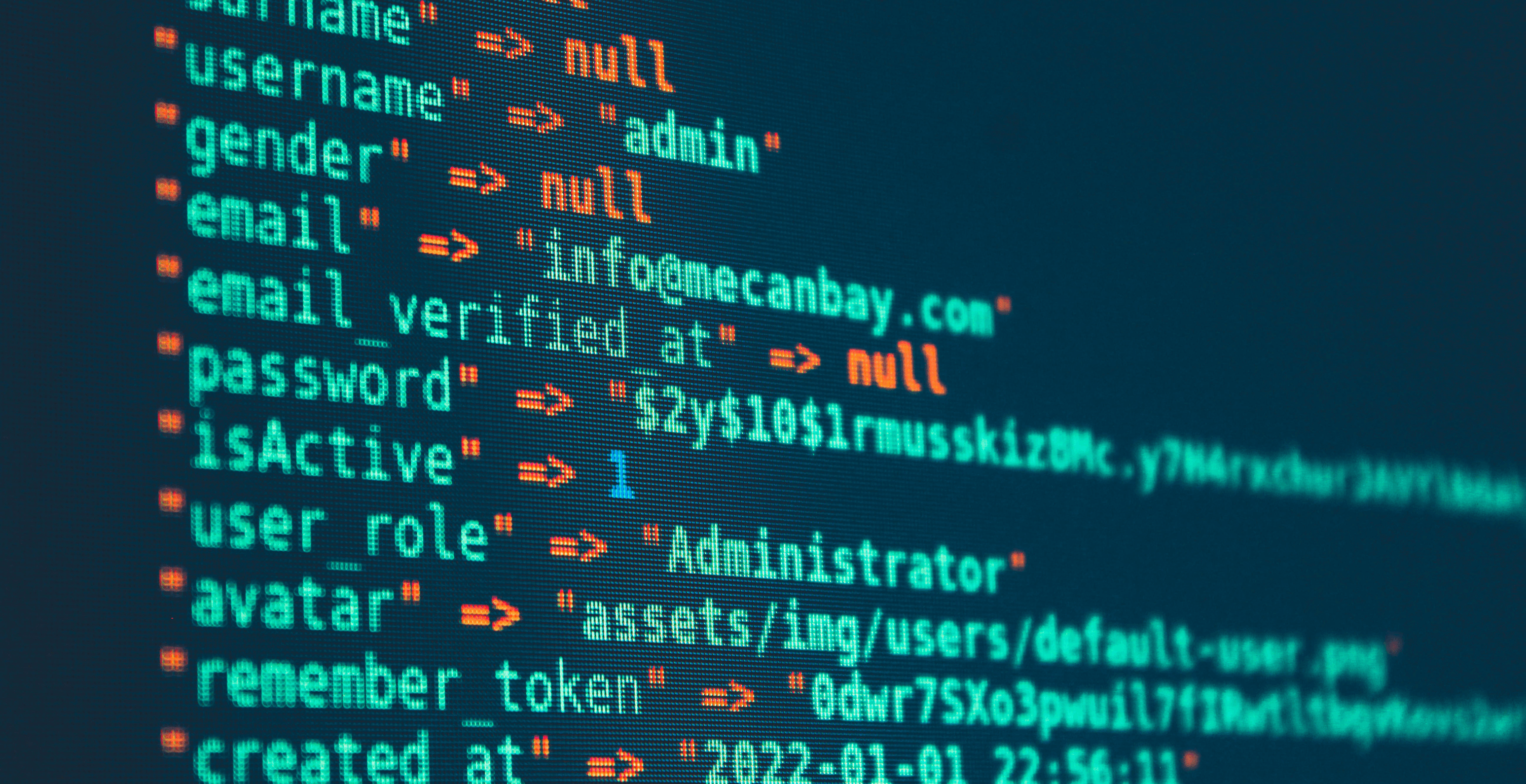
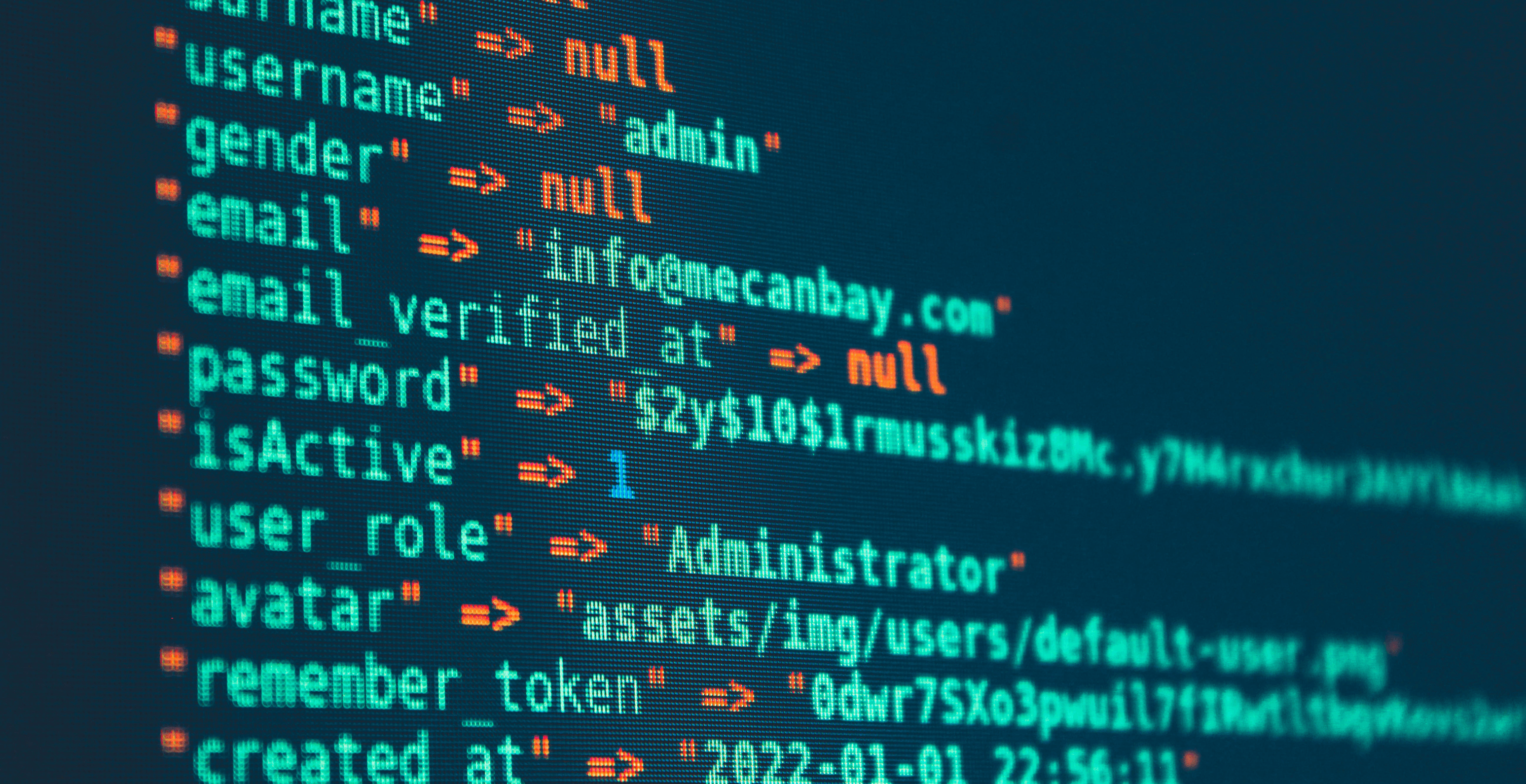
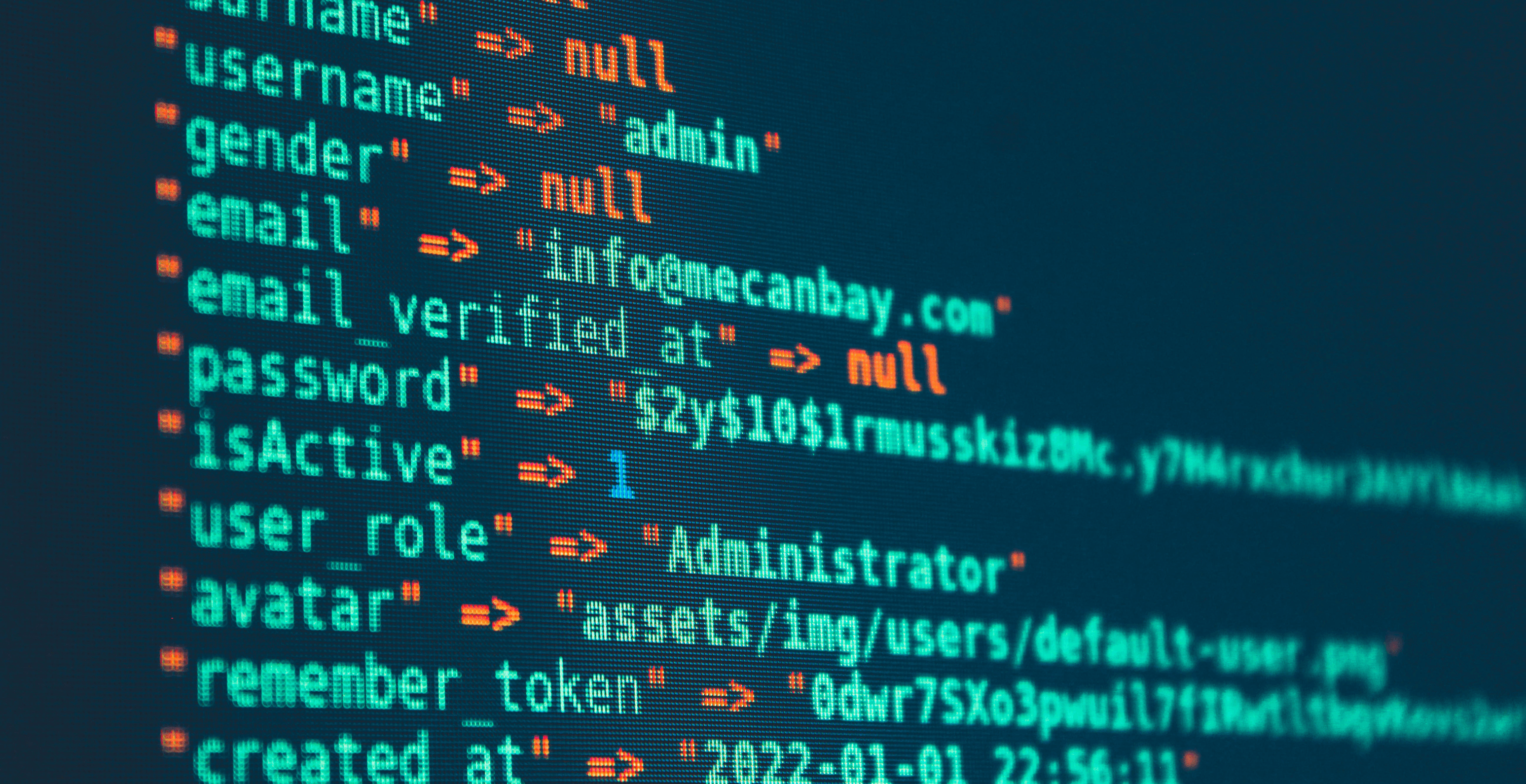
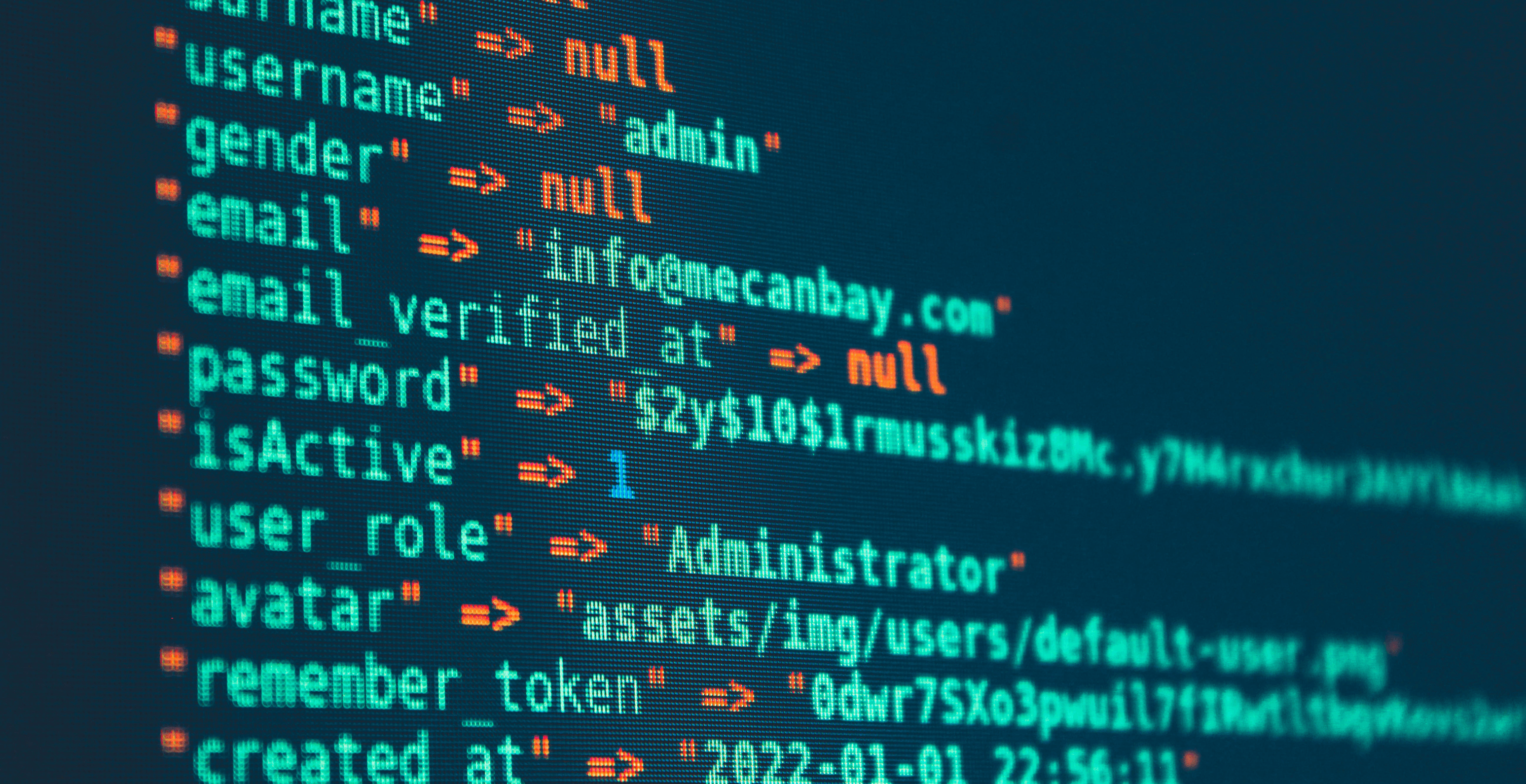
Best Practices In Data Governance For AI
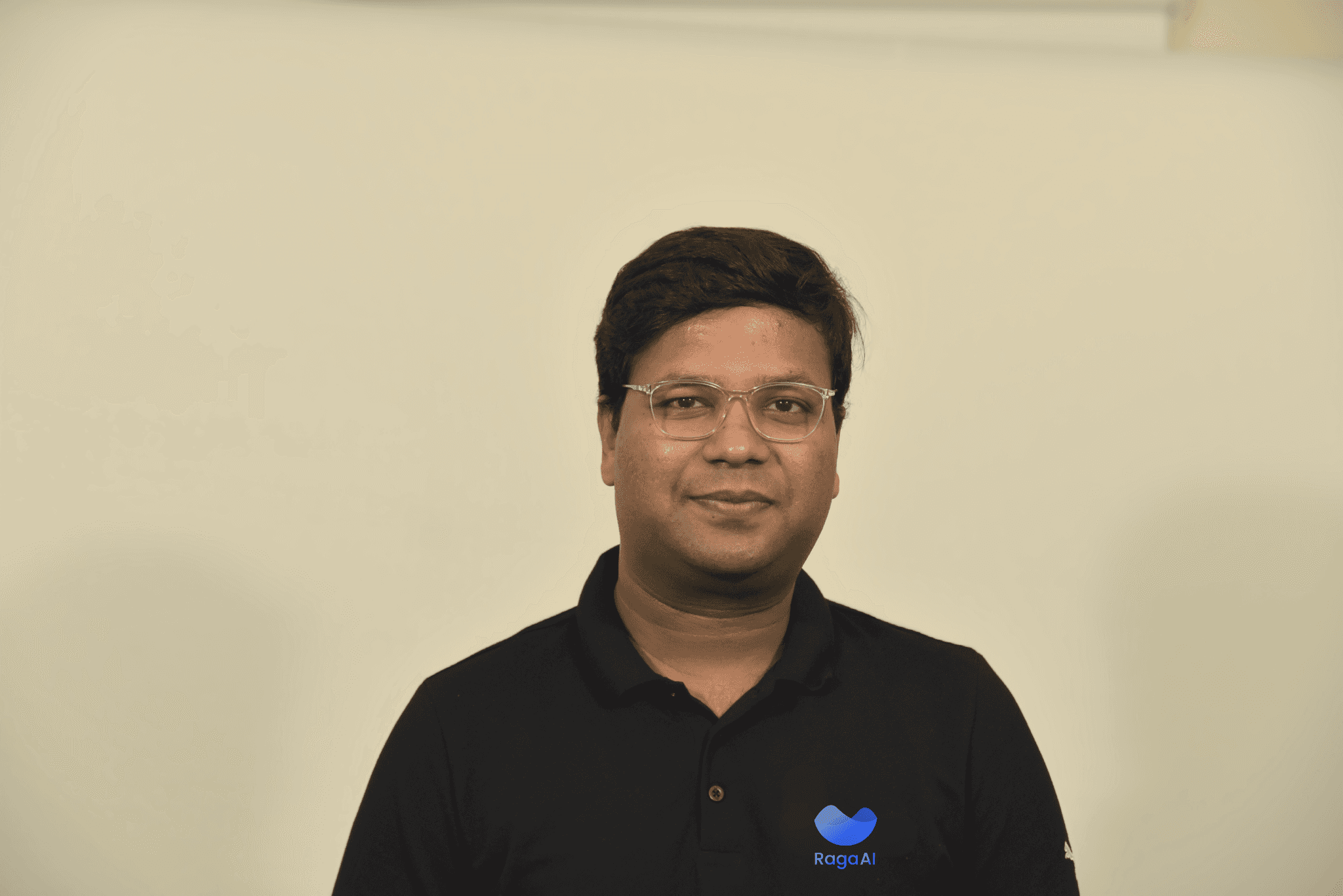
Jigar Gupta
Nov 17, 2024
Read the article
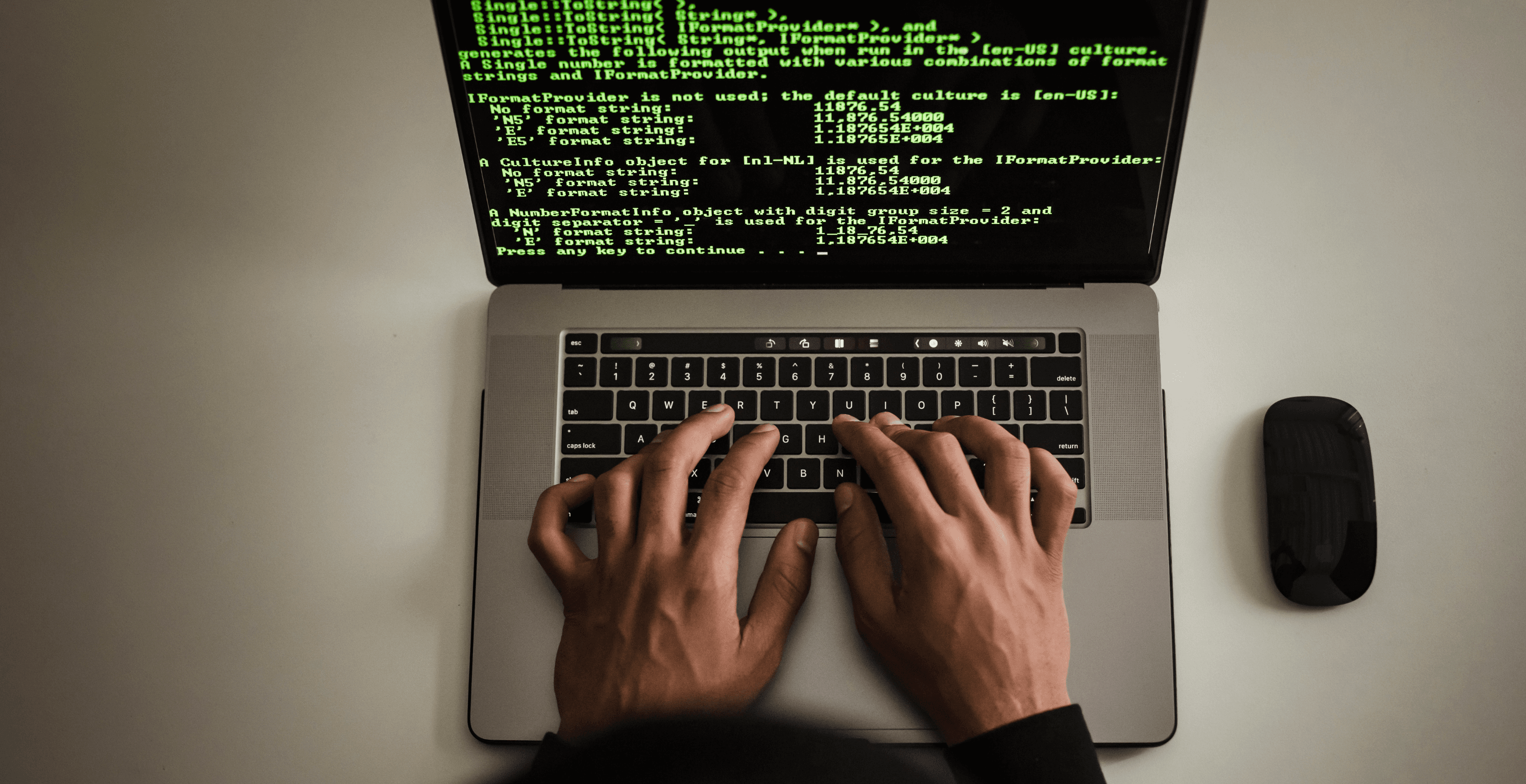
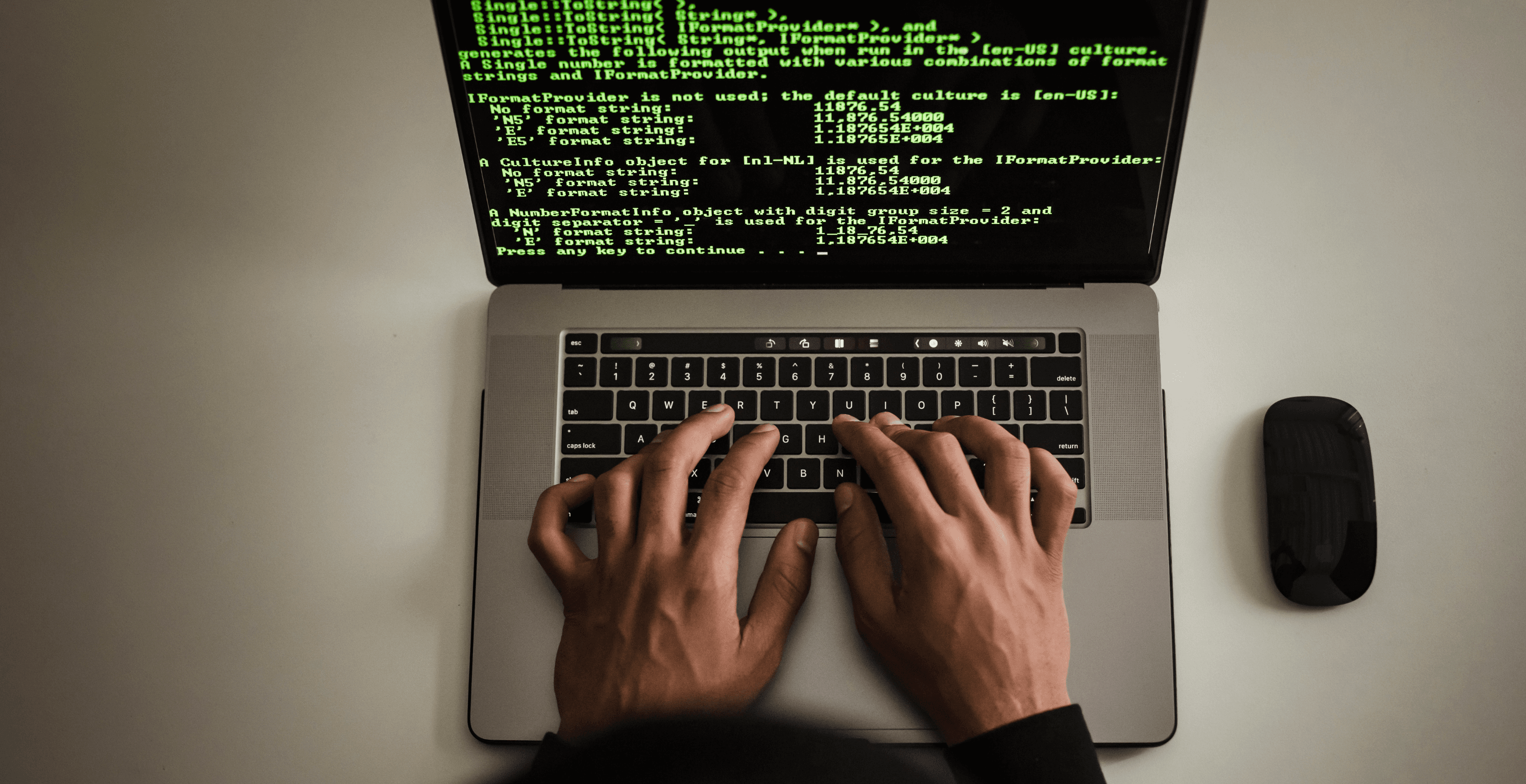
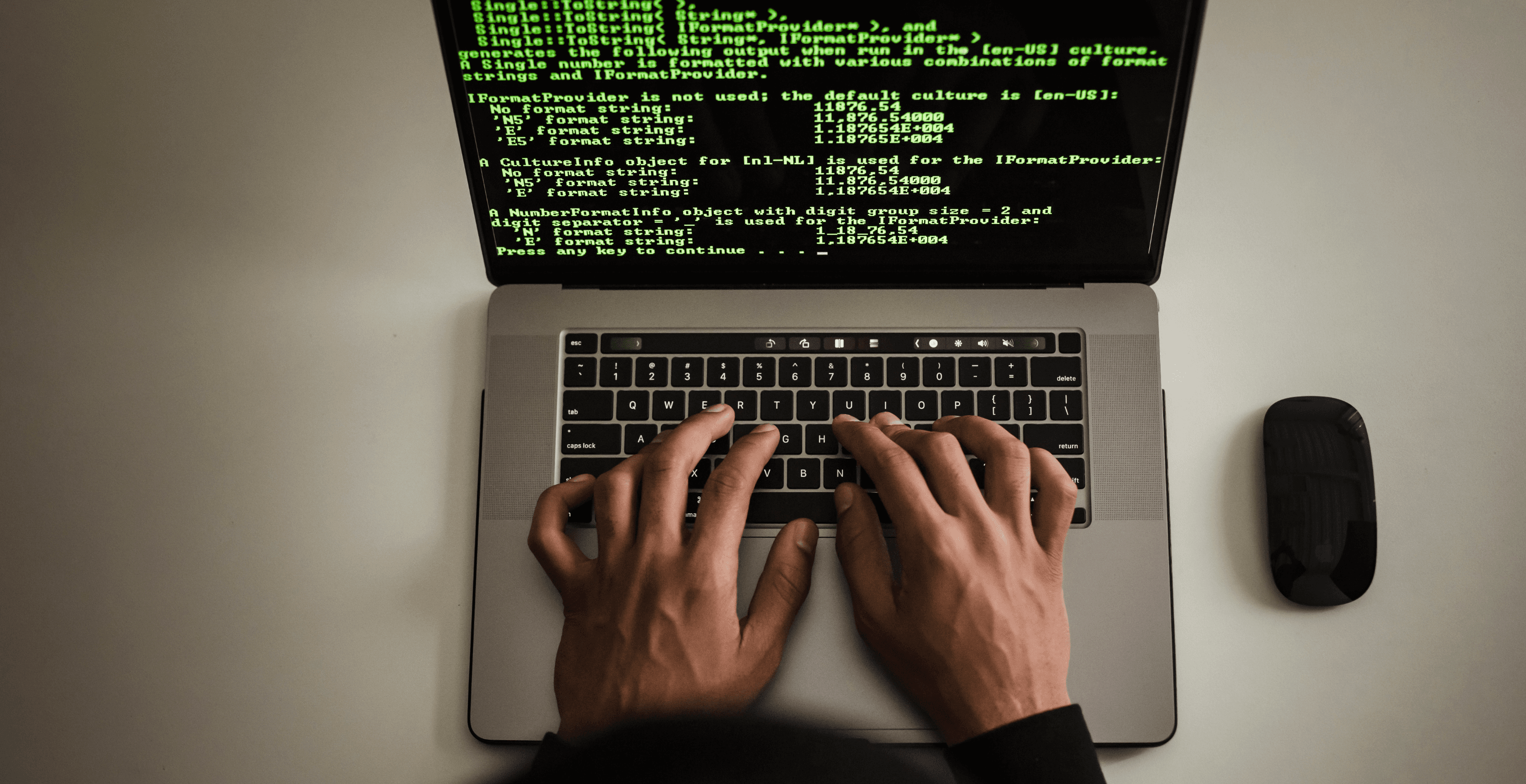
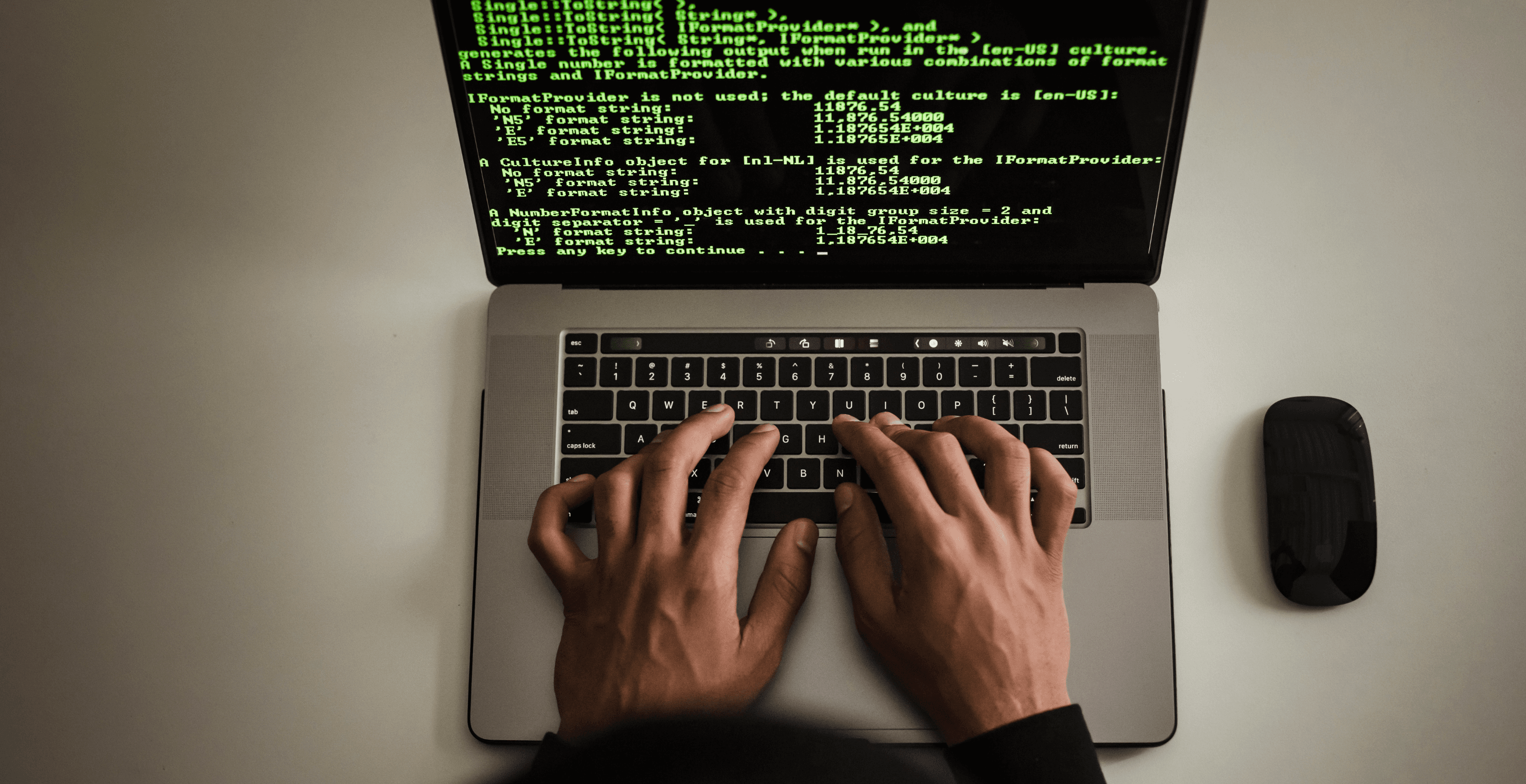
Transforming Conversational AI with Large Language Models

Rehan Asif
Nov 15, 2024
Read the article
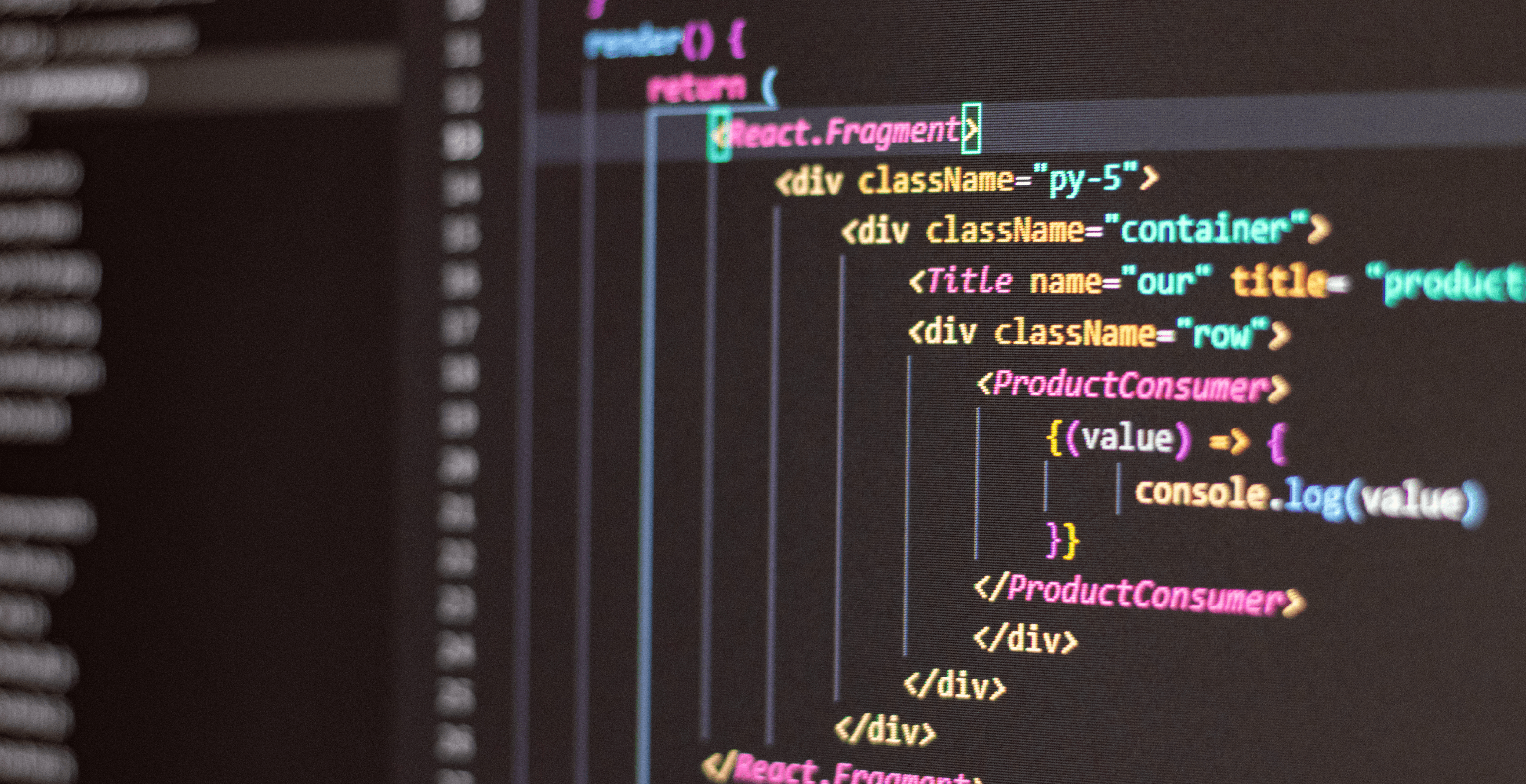
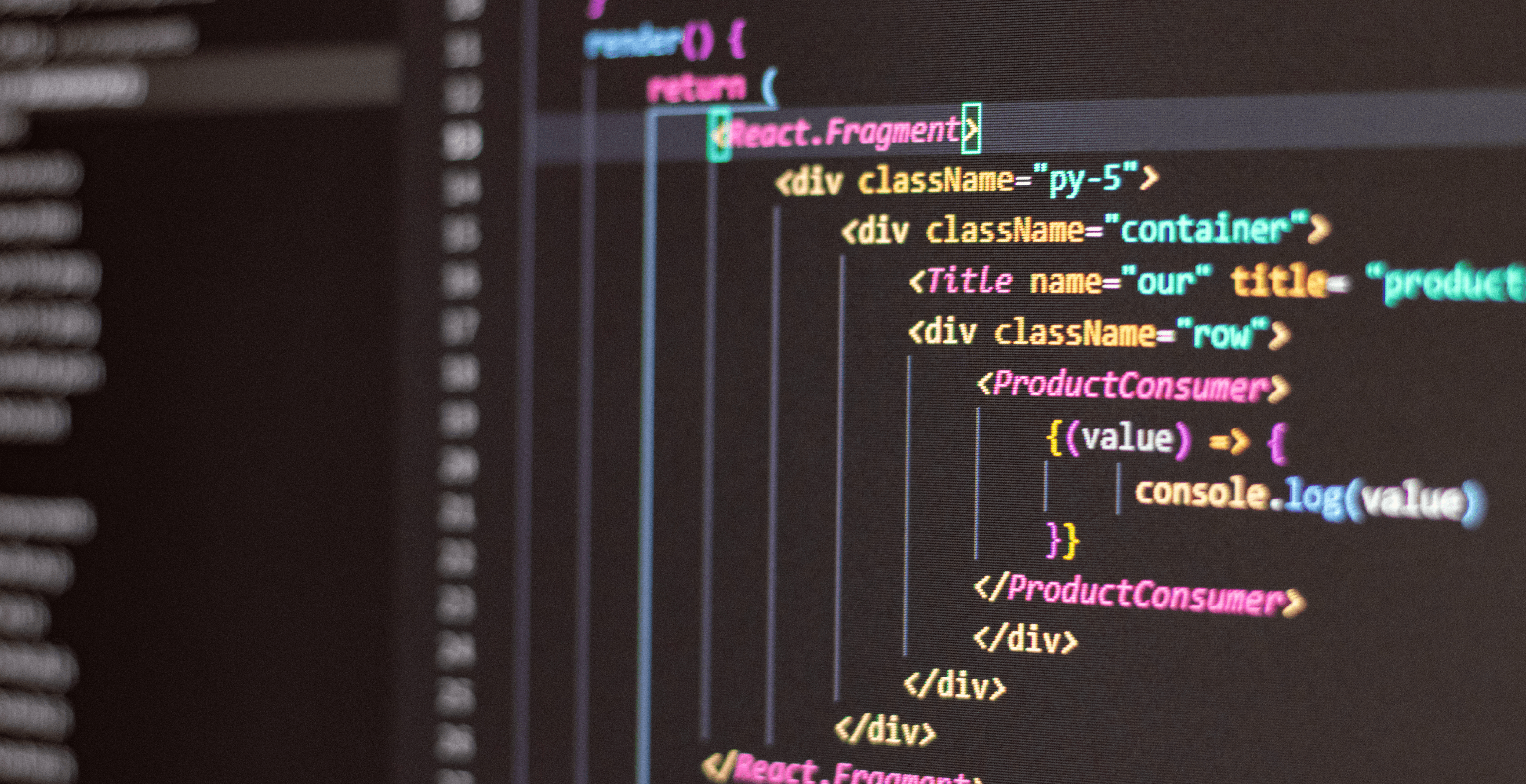
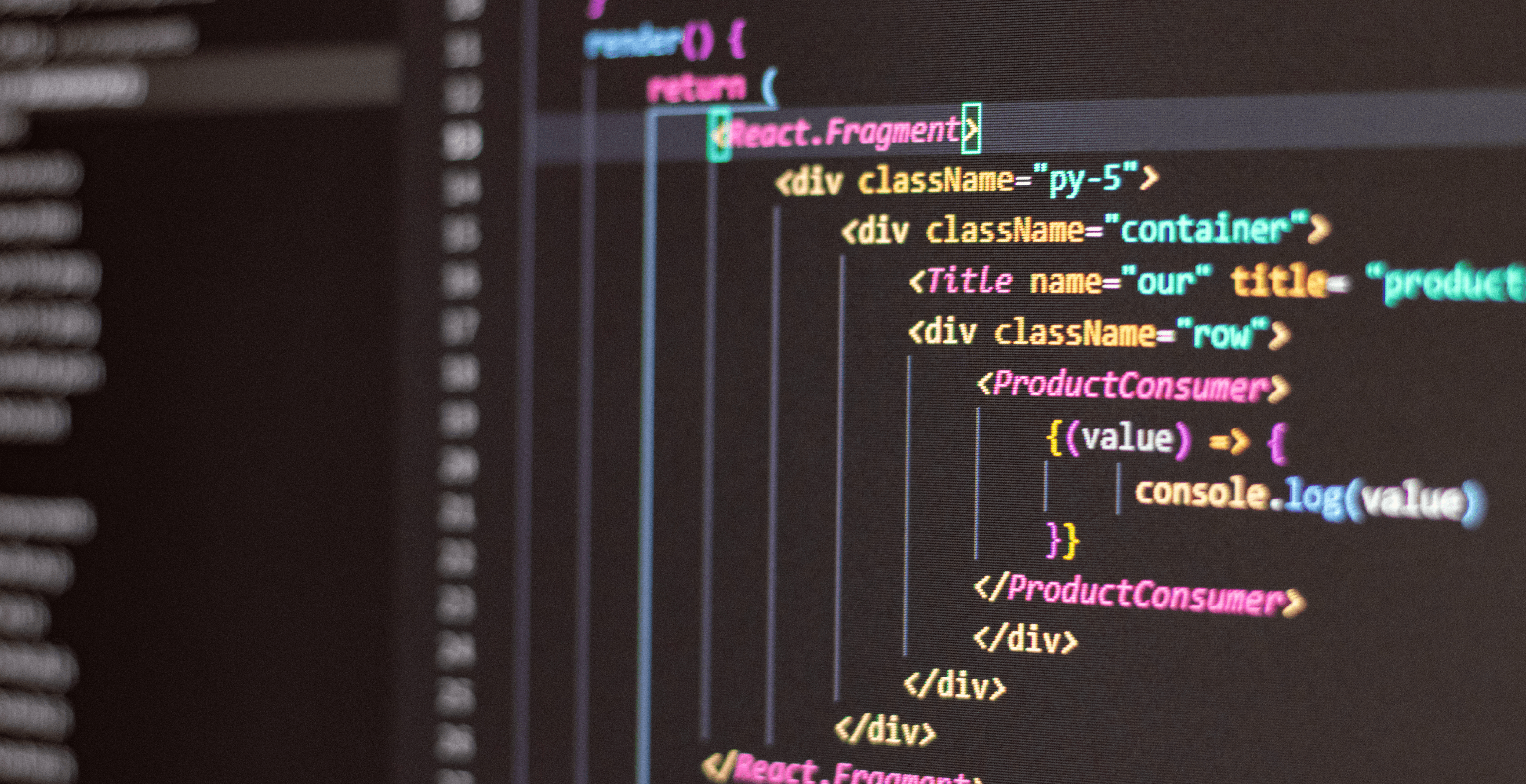
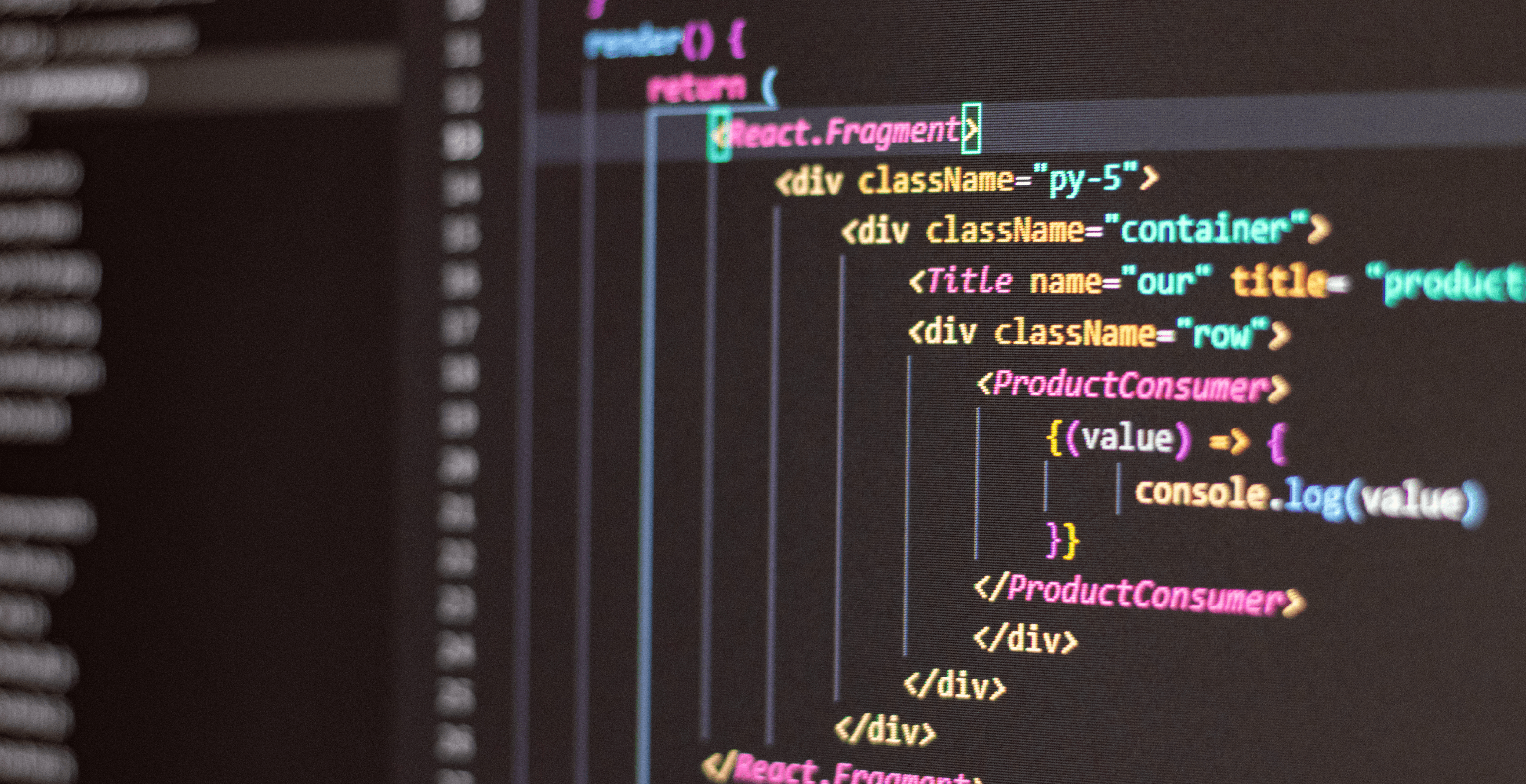
Deploying Generative AI Agents with Local LLMs

Rehan Asif
Nov 13, 2024
Read the article




Exploring Different Types of AI Agents with Key Examples
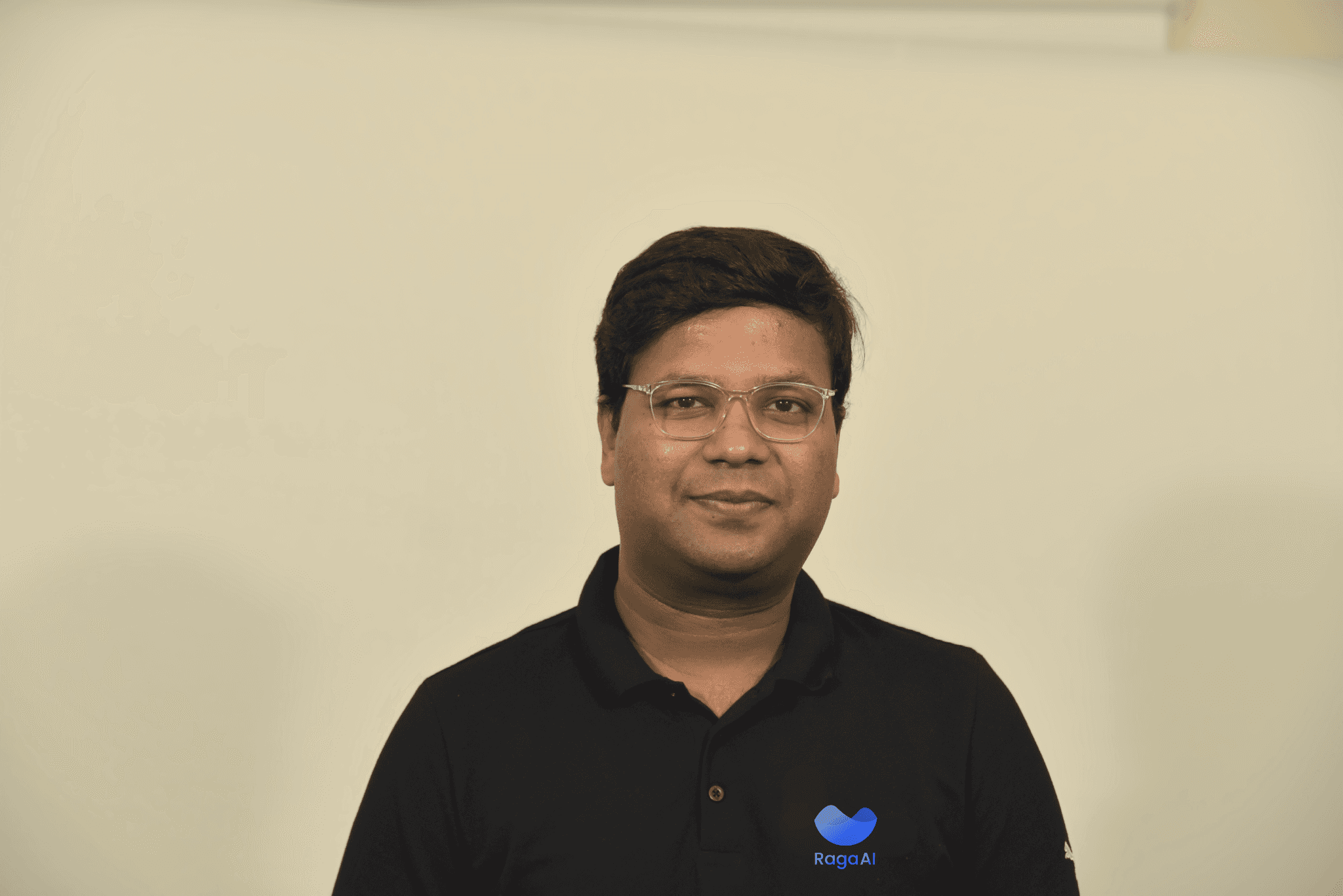
Jigar Gupta
Nov 11, 2024
Read the article




Creating Your Own Personal LLM Agents: Introduction to Implementation

Rehan Asif
Nov 8, 2024
Read the article




Exploring Agentic AI Architecture and Design Patterns
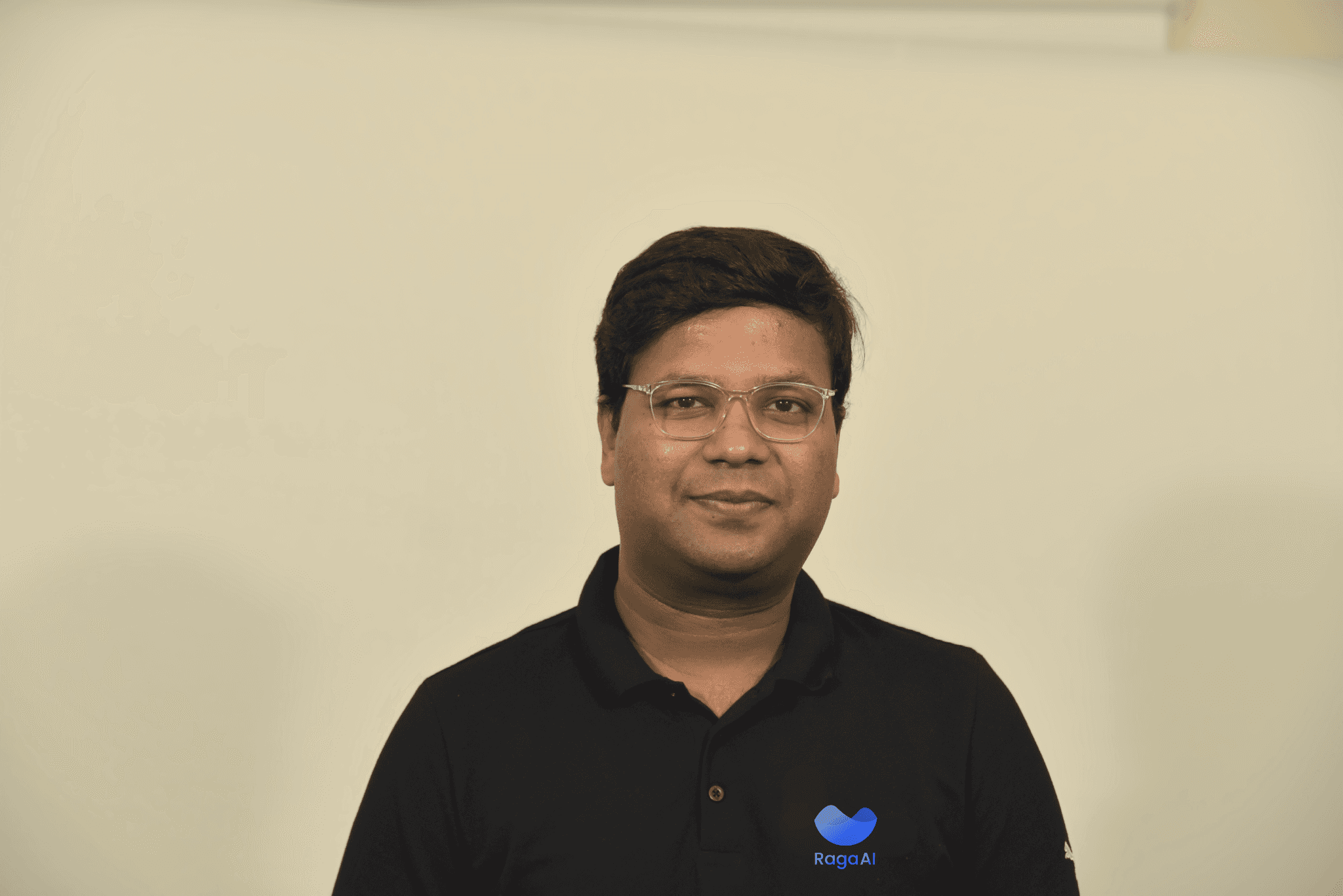
Jigar Gupta
Nov 6, 2024
Read the article
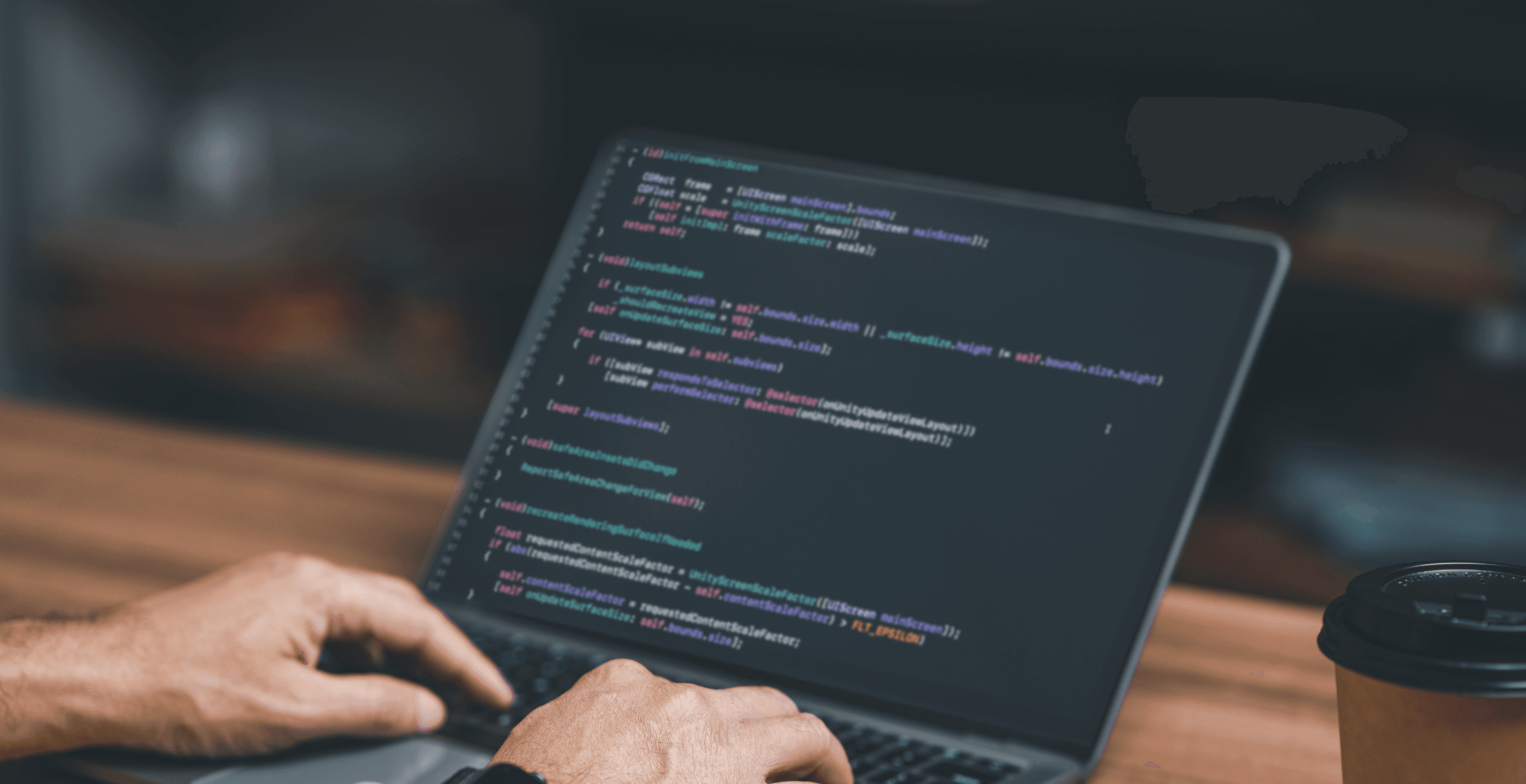
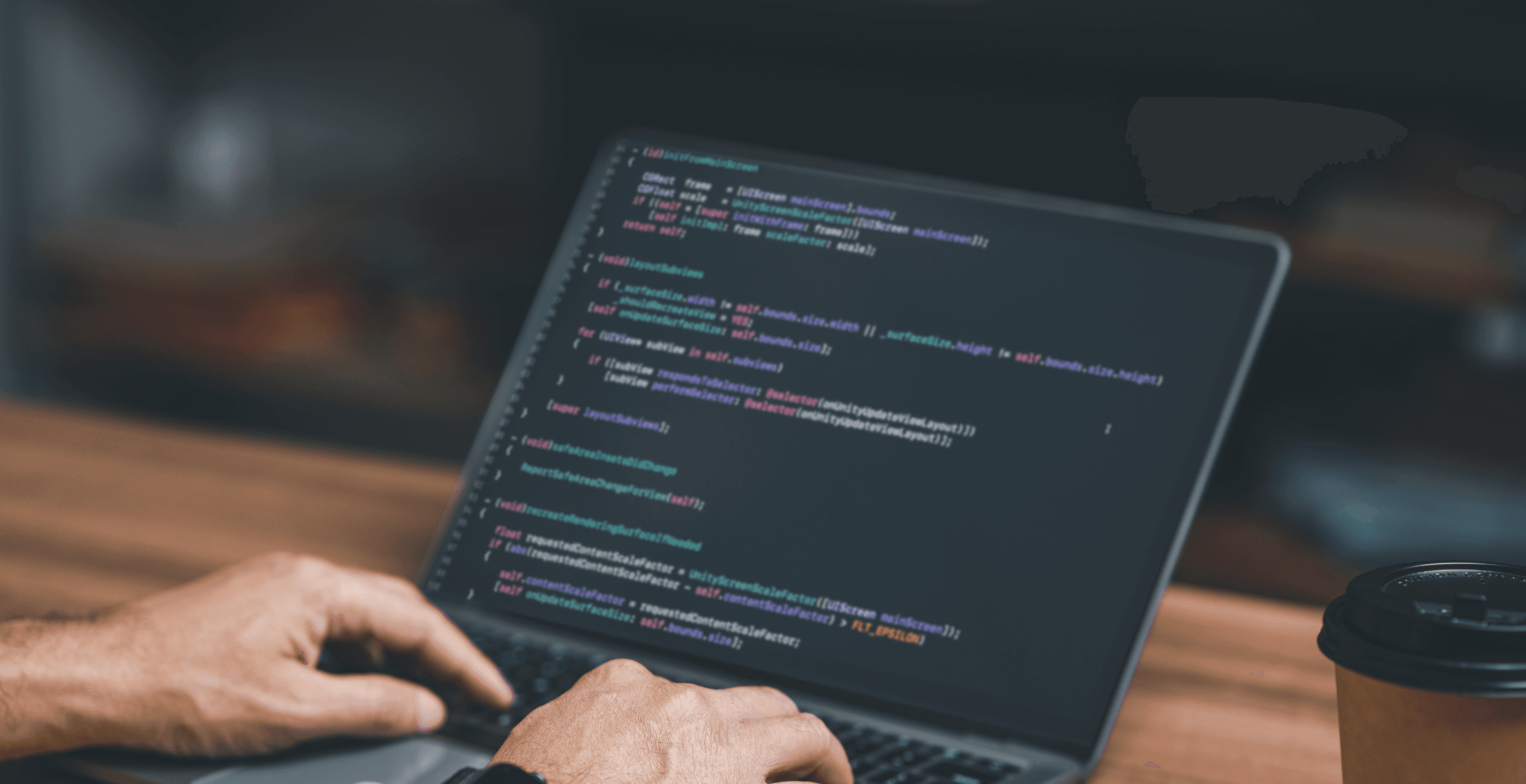
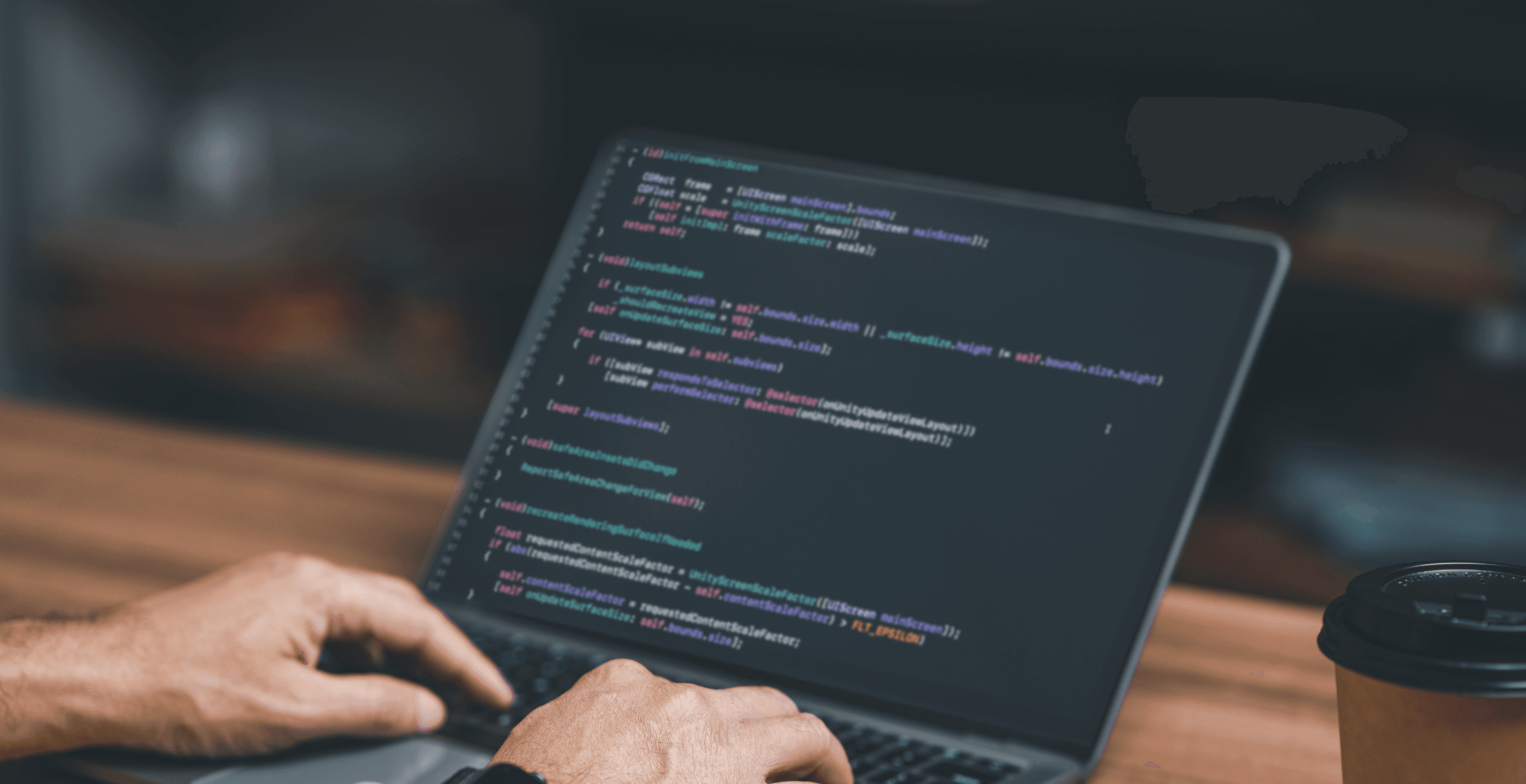
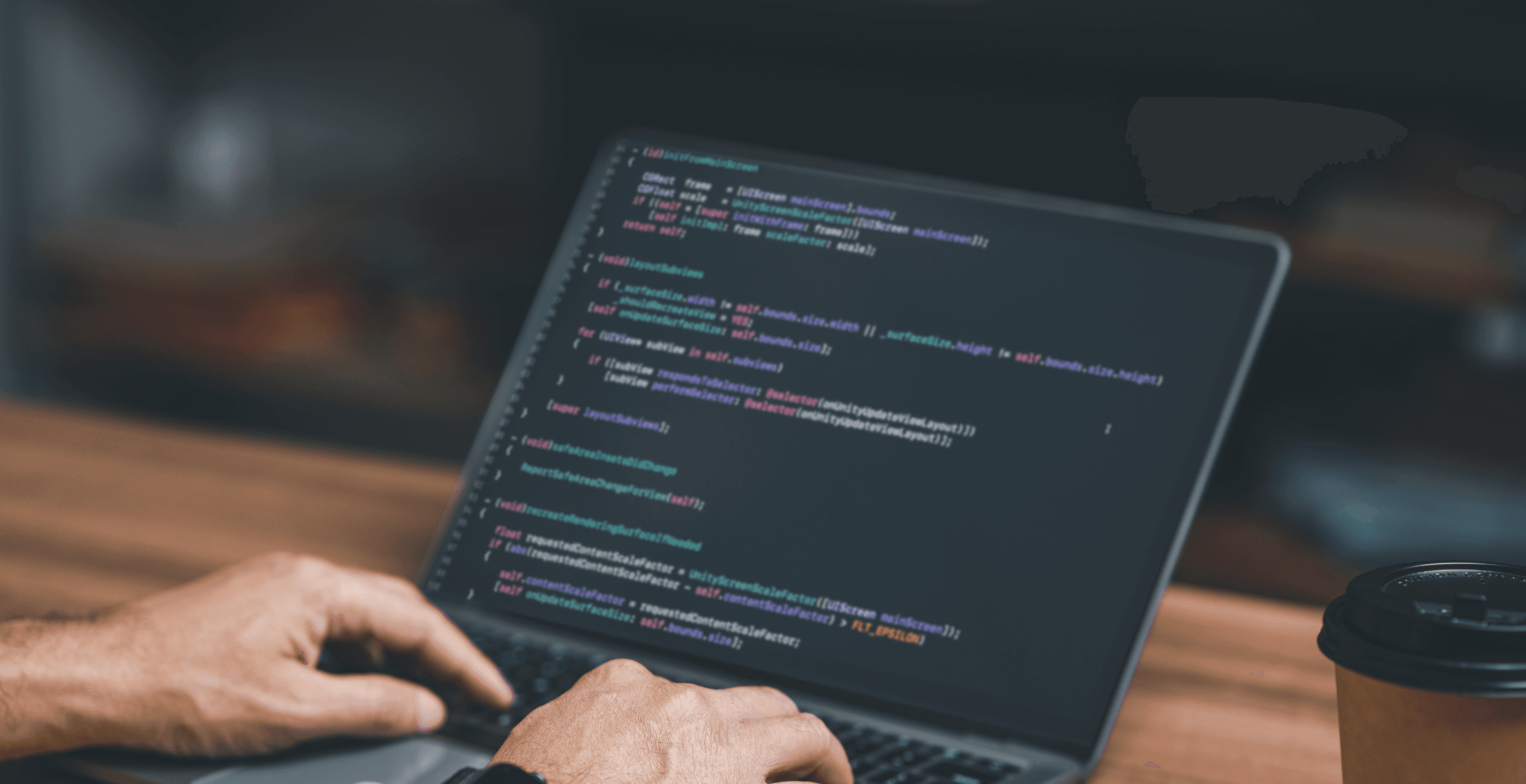
Building Your First LLM Agent Framework Application

Rehan Asif
Nov 4, 2024
Read the article




Multi-Agent Design and Collaboration Patterns

Rehan Asif
Nov 1, 2024
Read the article




Creating Your Own LLM Agent Application from Scratch

Rehan Asif
Oct 30, 2024
Read the article




Solving LLM Token Limit Issues: Understanding and Approaches

Rehan Asif
Oct 27, 2024
Read the article
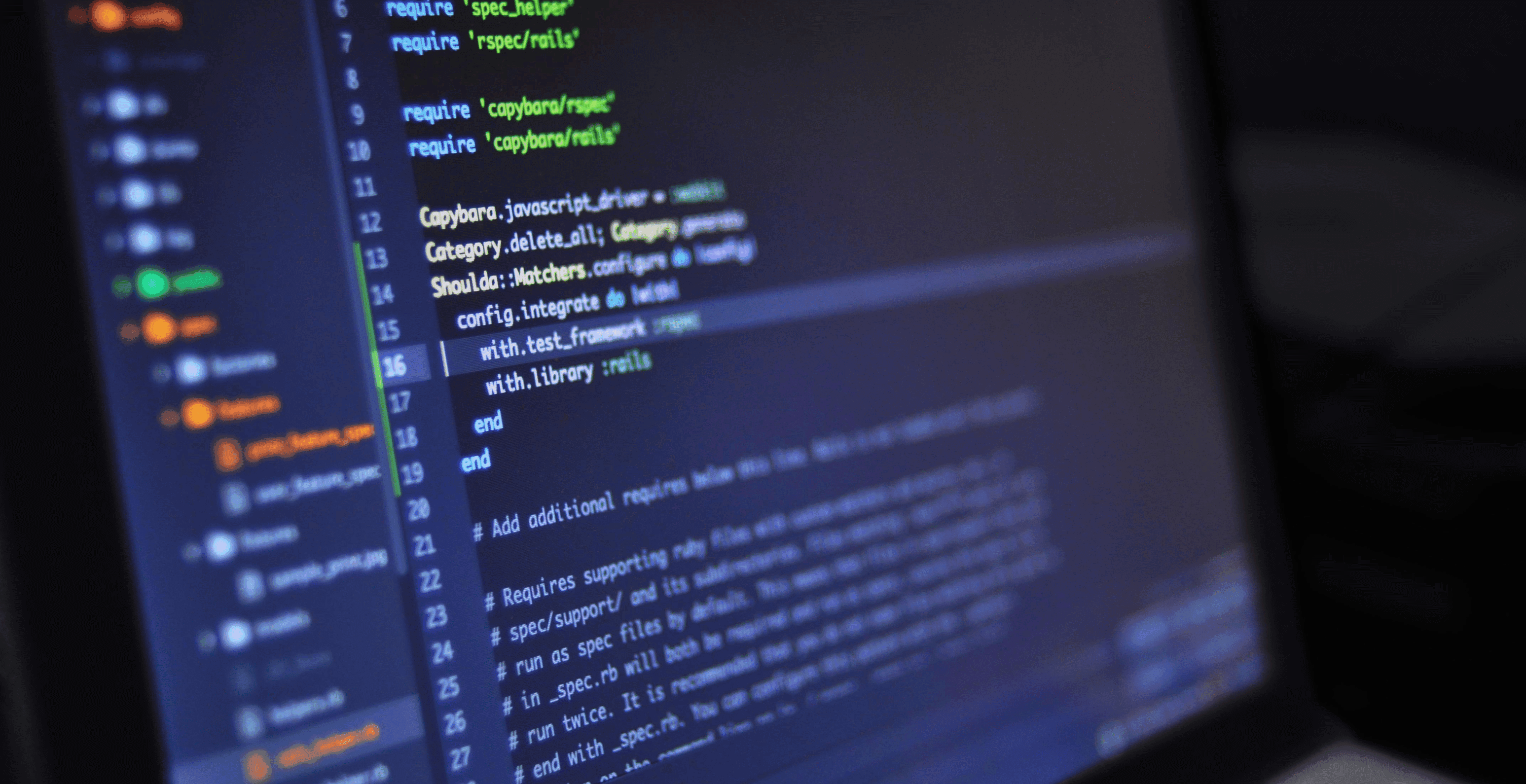
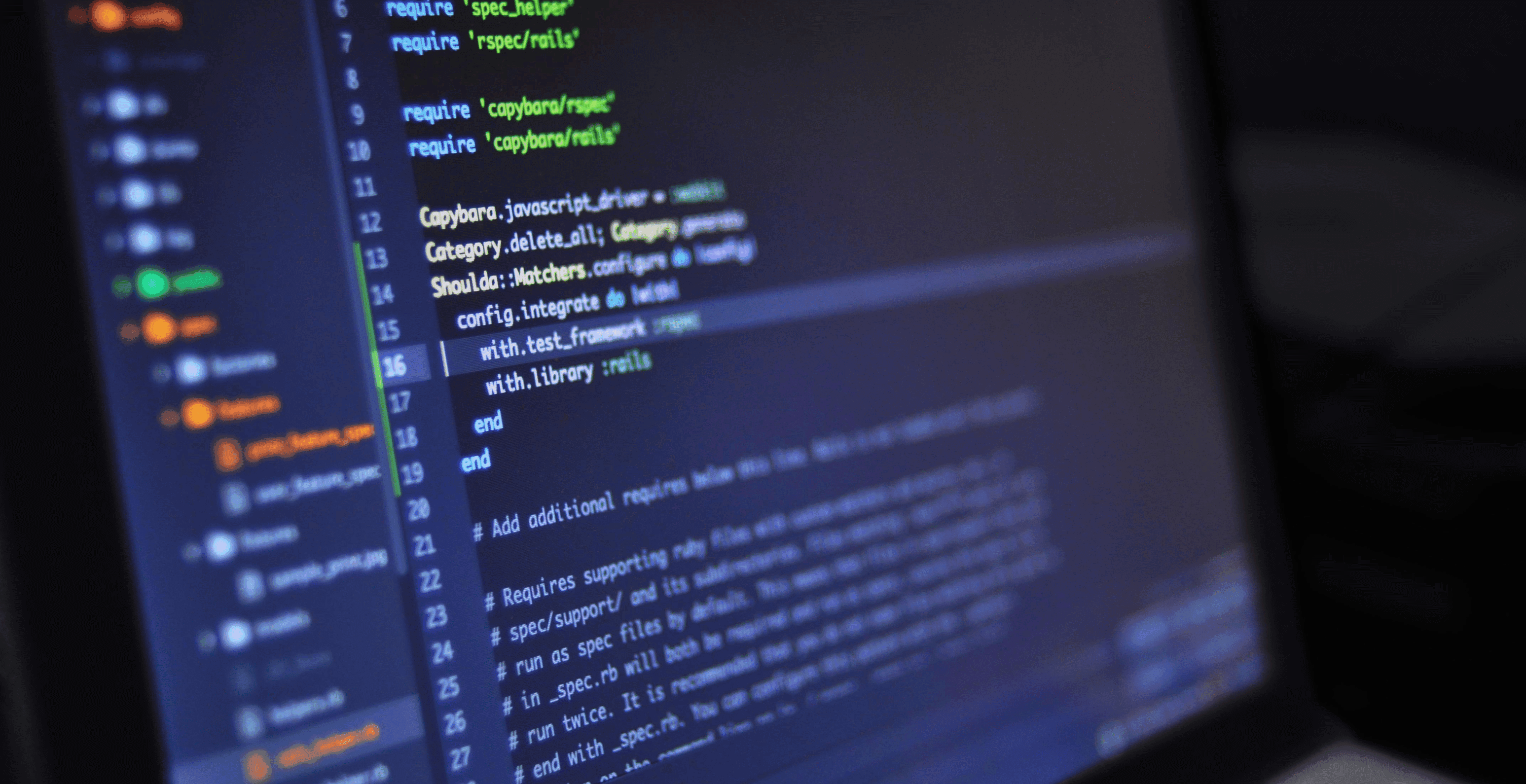
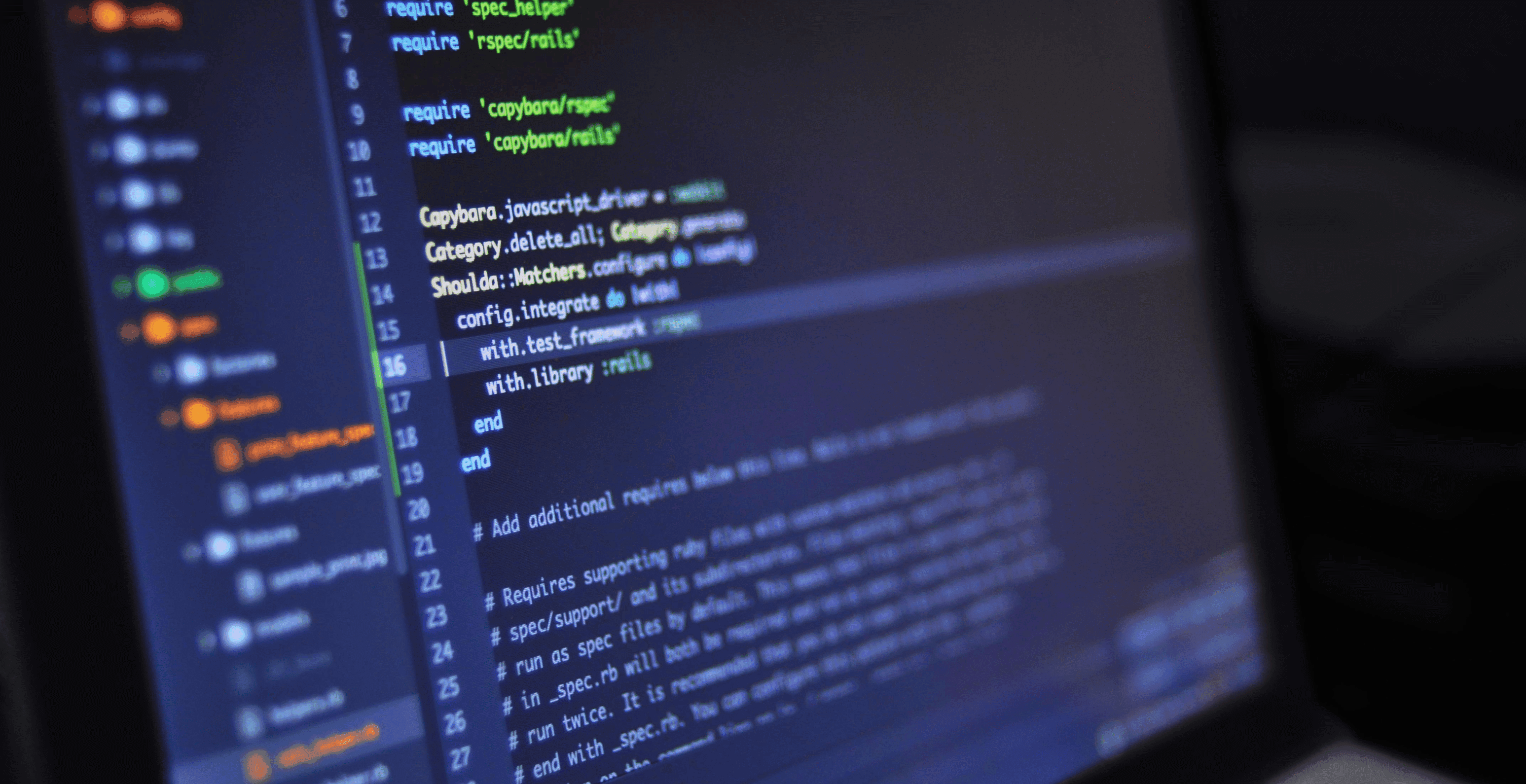
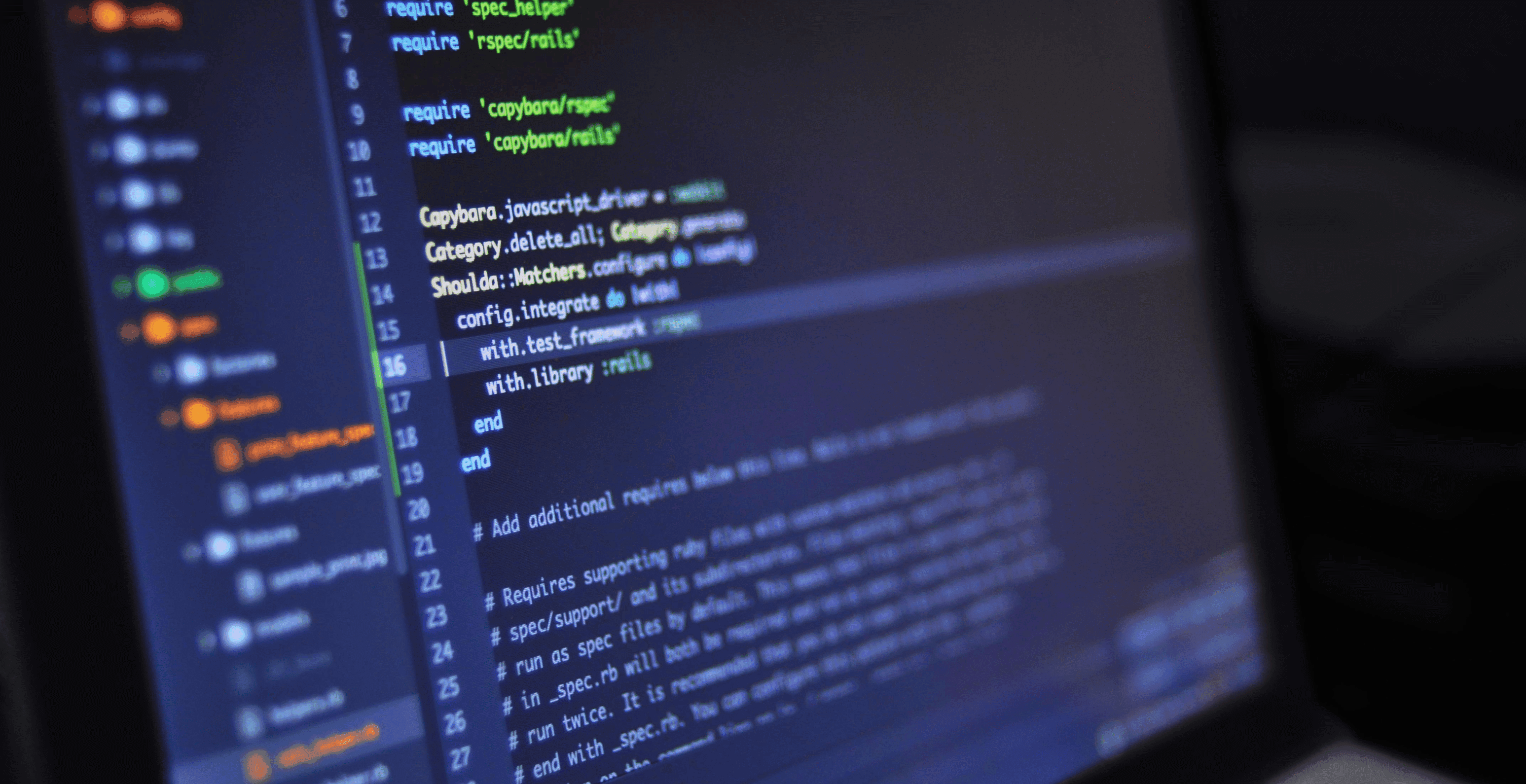
Understanding the Impact of Inference Cost on Generative AI Adoption
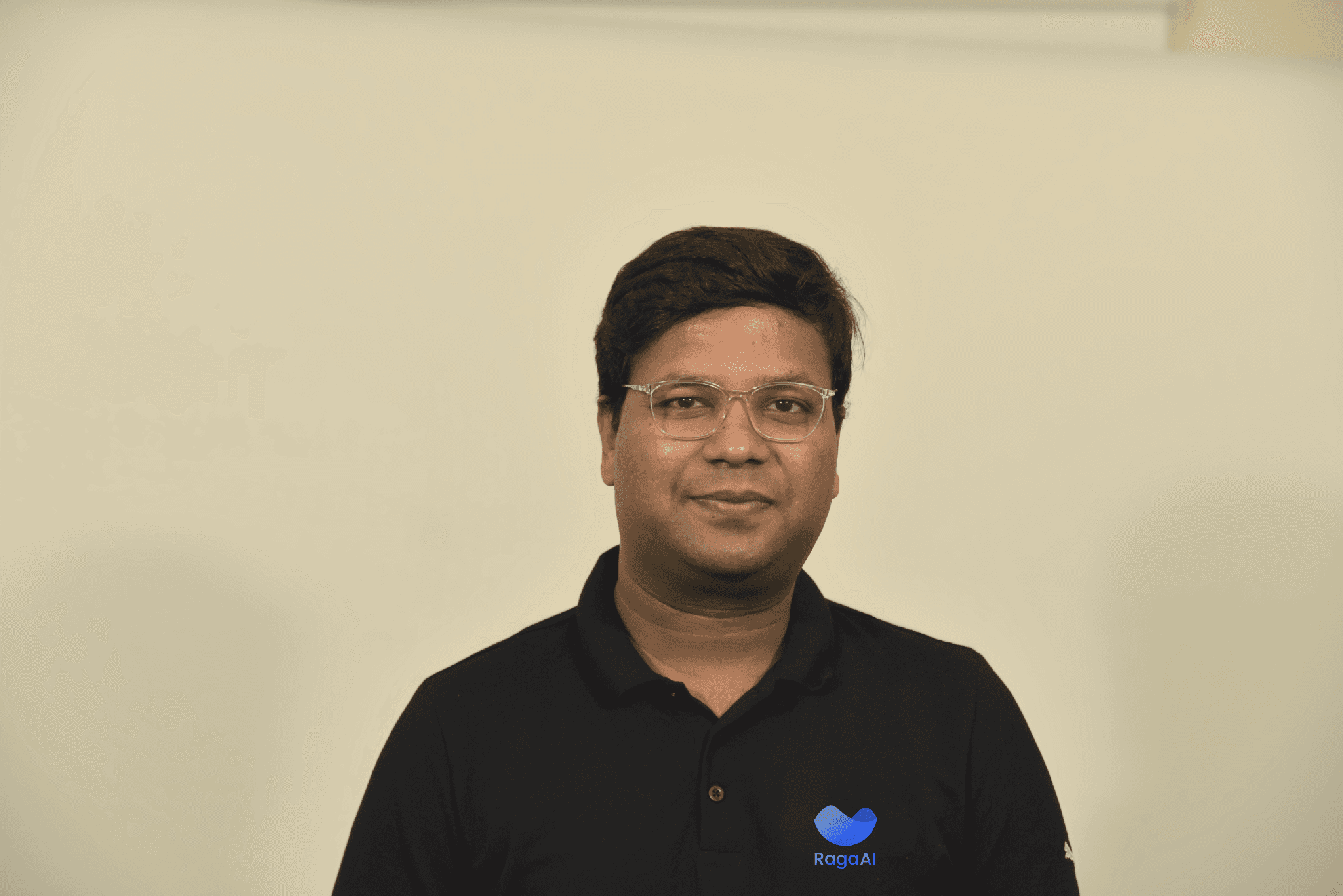
Jigar Gupta
Oct 24, 2024
Read the article




Data Security: Risks, Solutions, Types and Best Practices
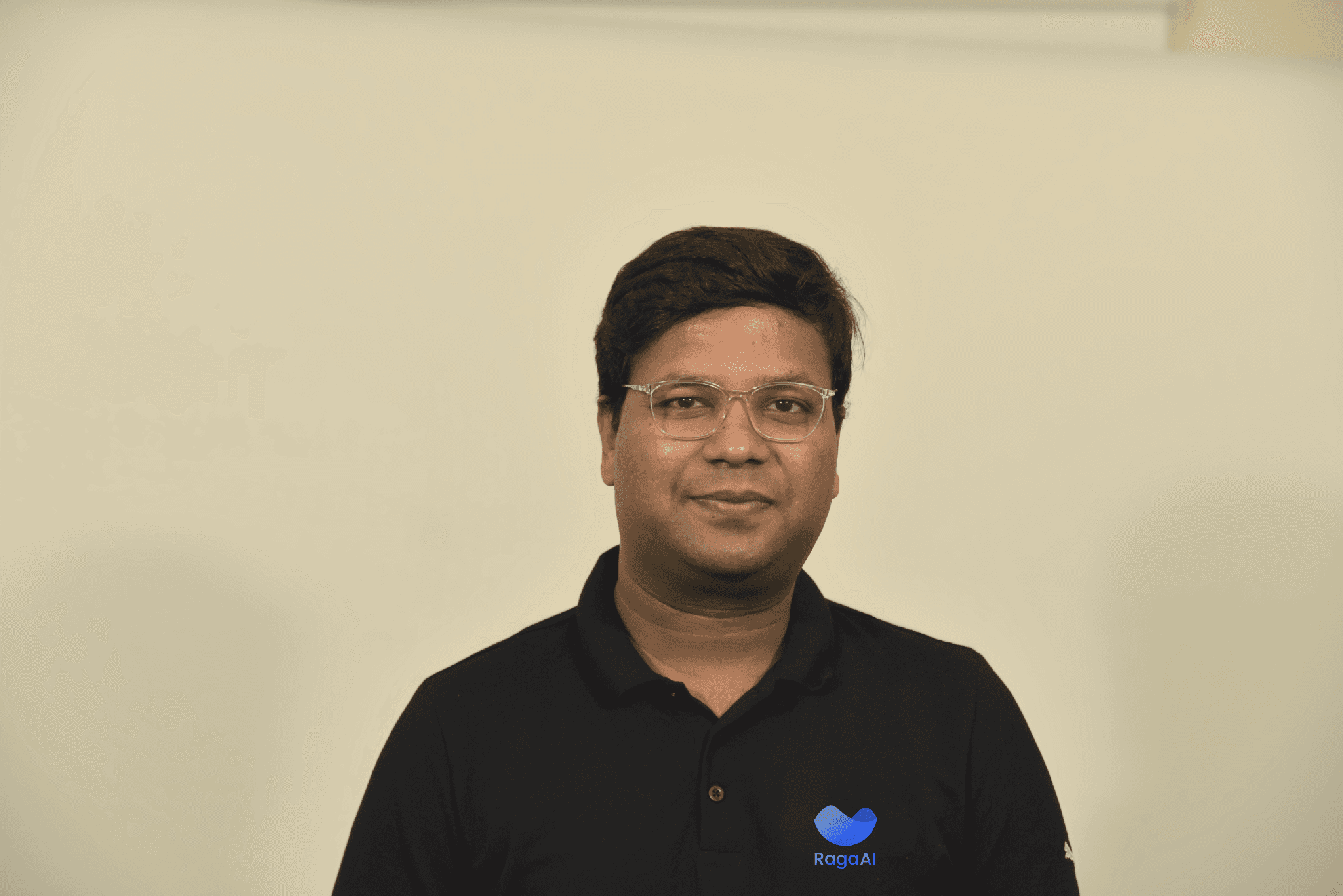
Jigar Gupta
Oct 21, 2024
Read the article
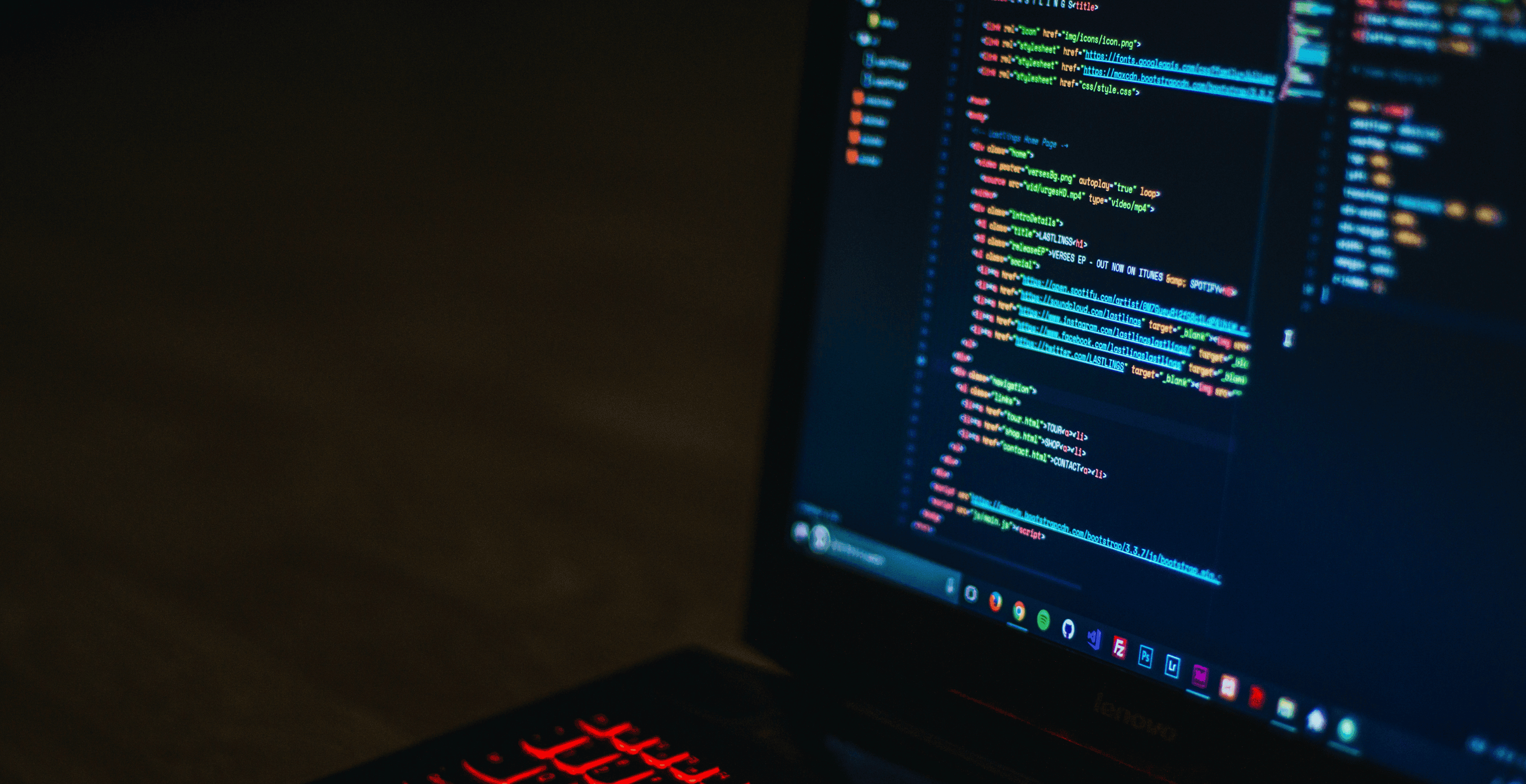
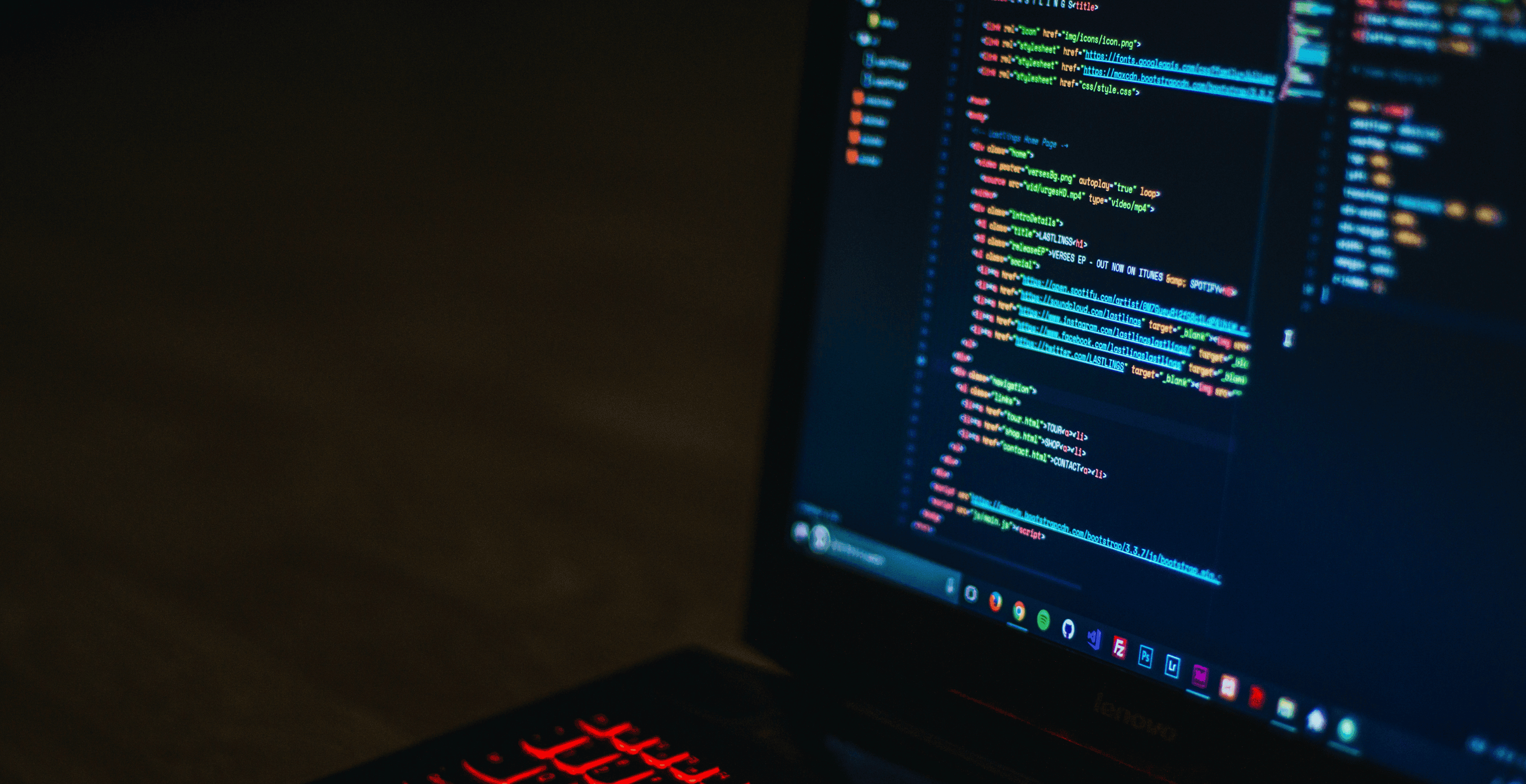
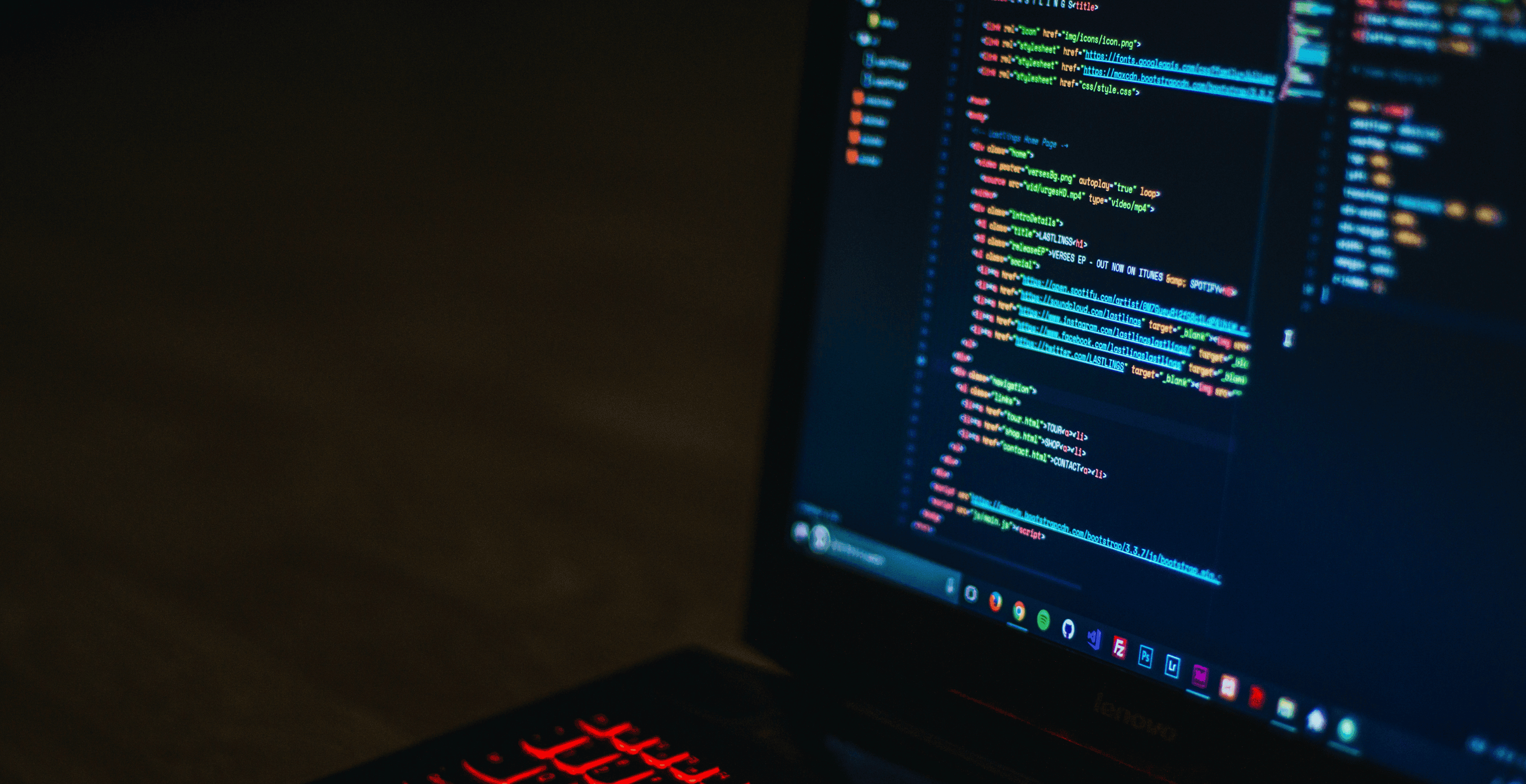
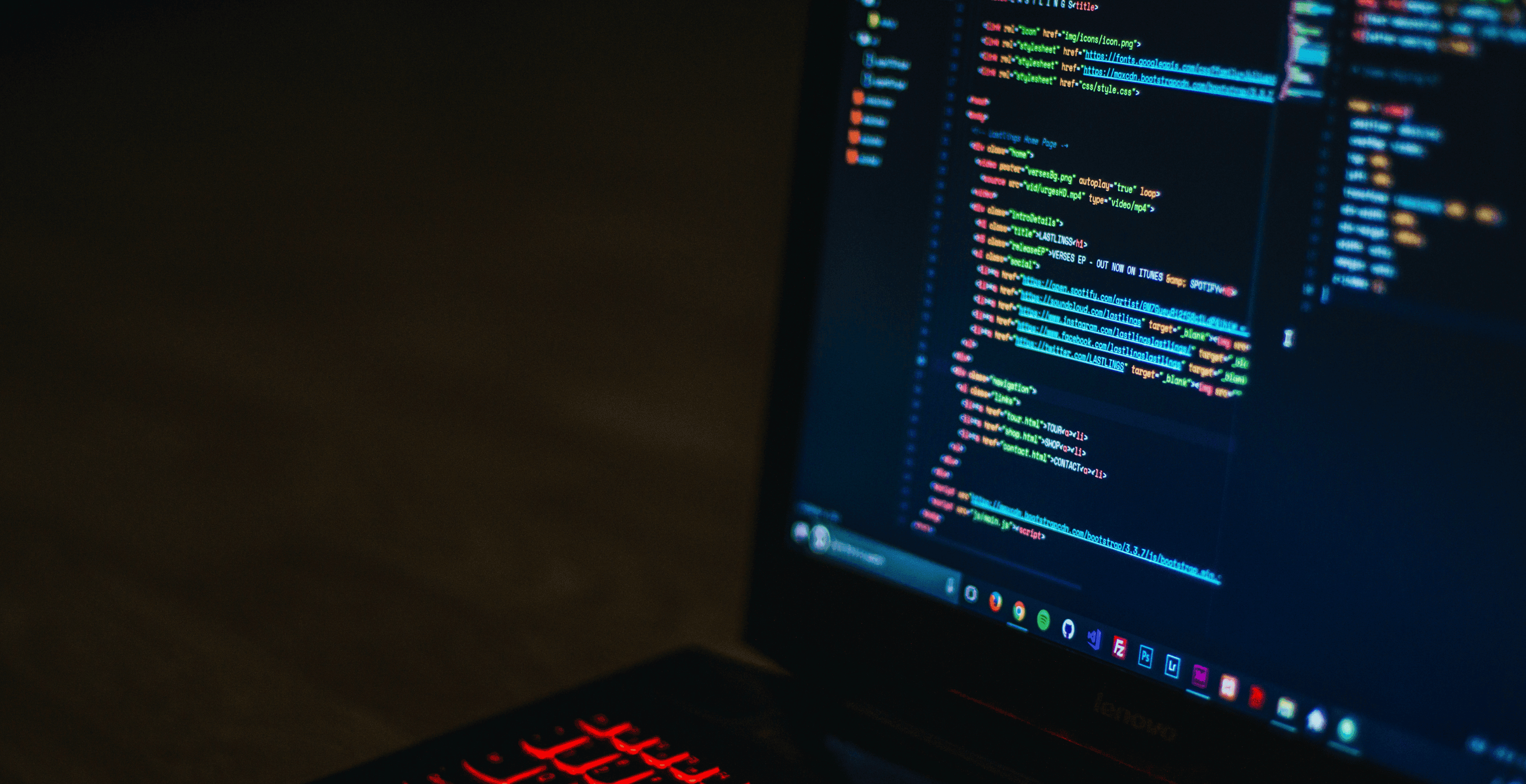
Getting Contextual Understanding Right for RAG Applications
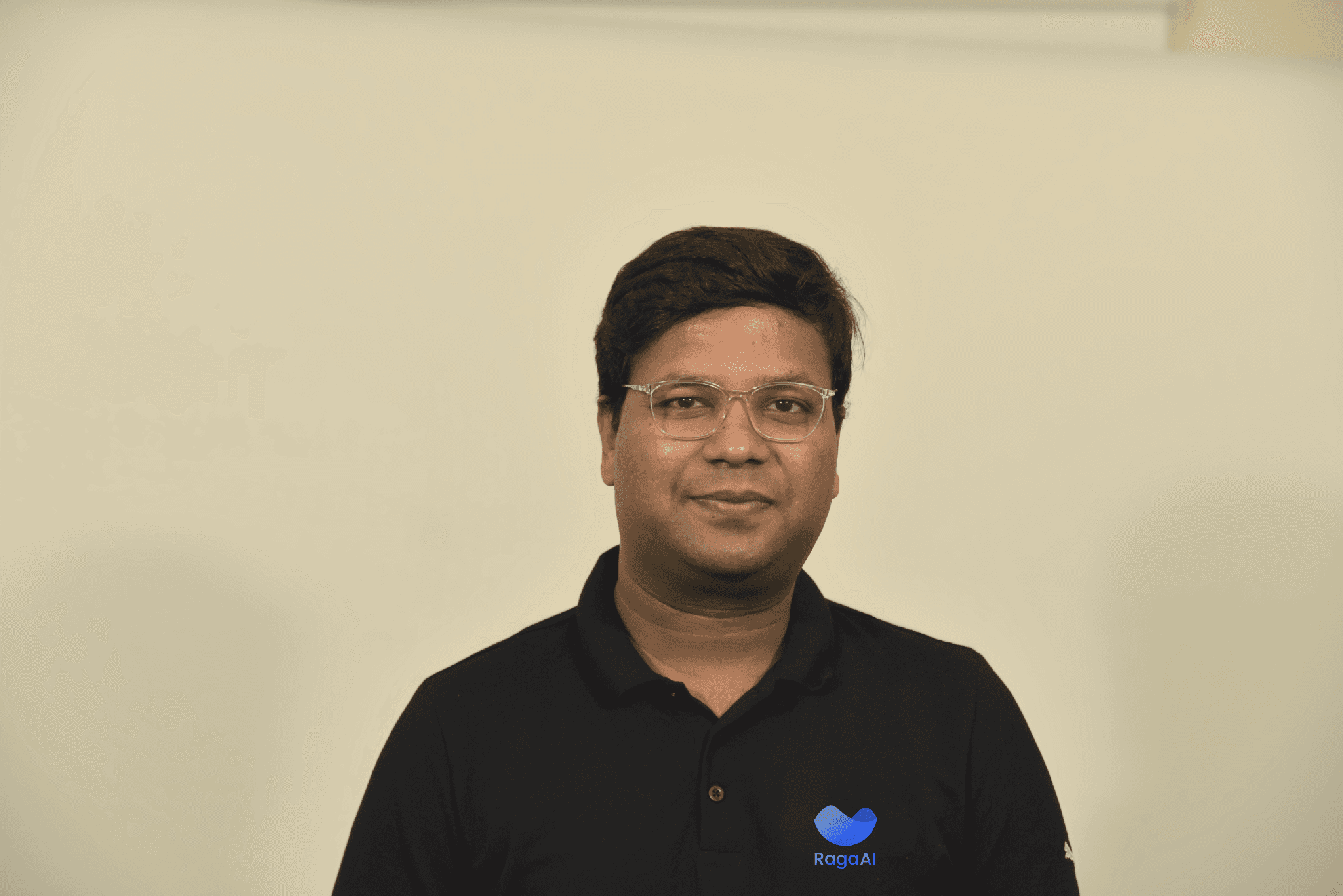
Jigar Gupta
Oct 19, 2024
Read the article
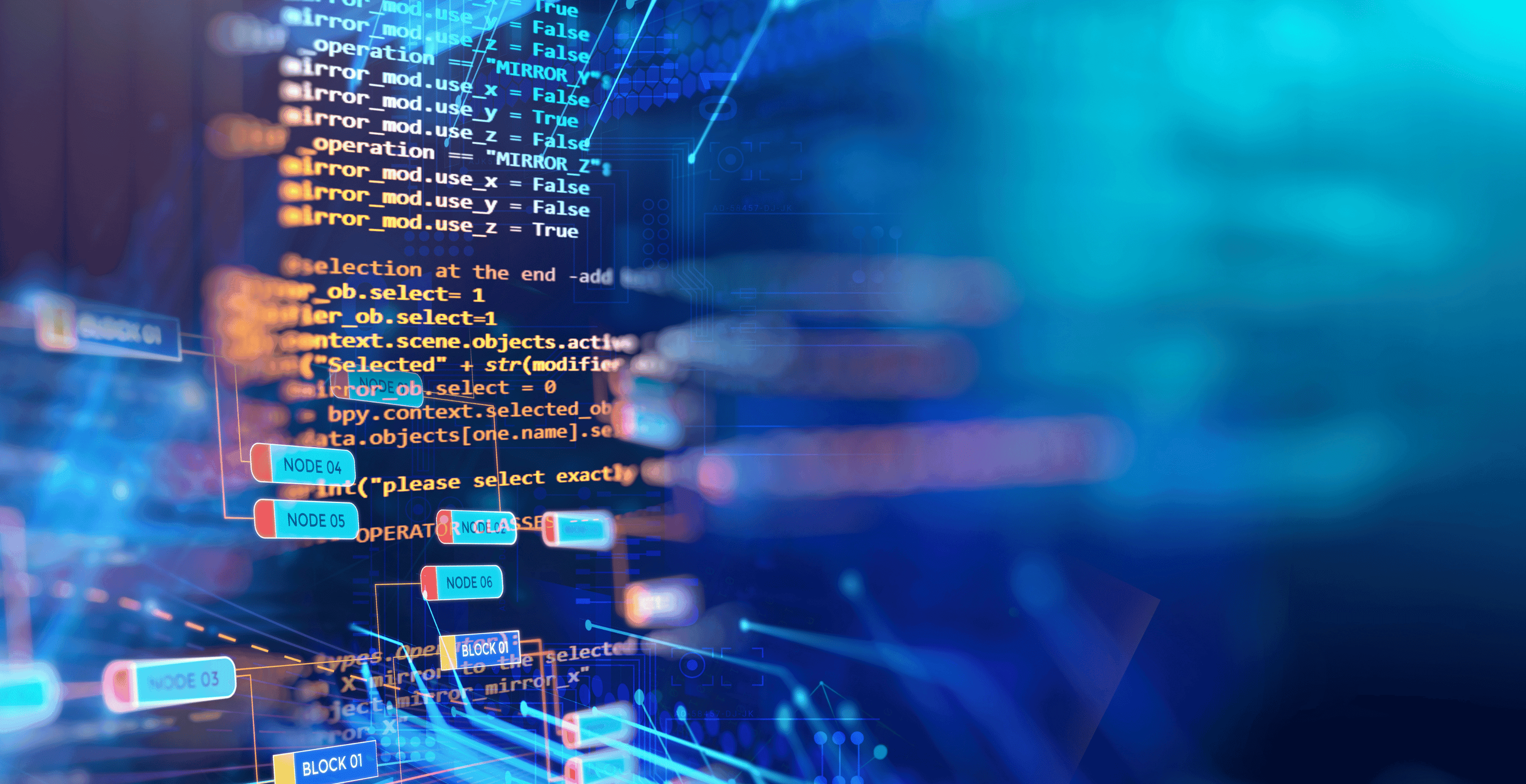
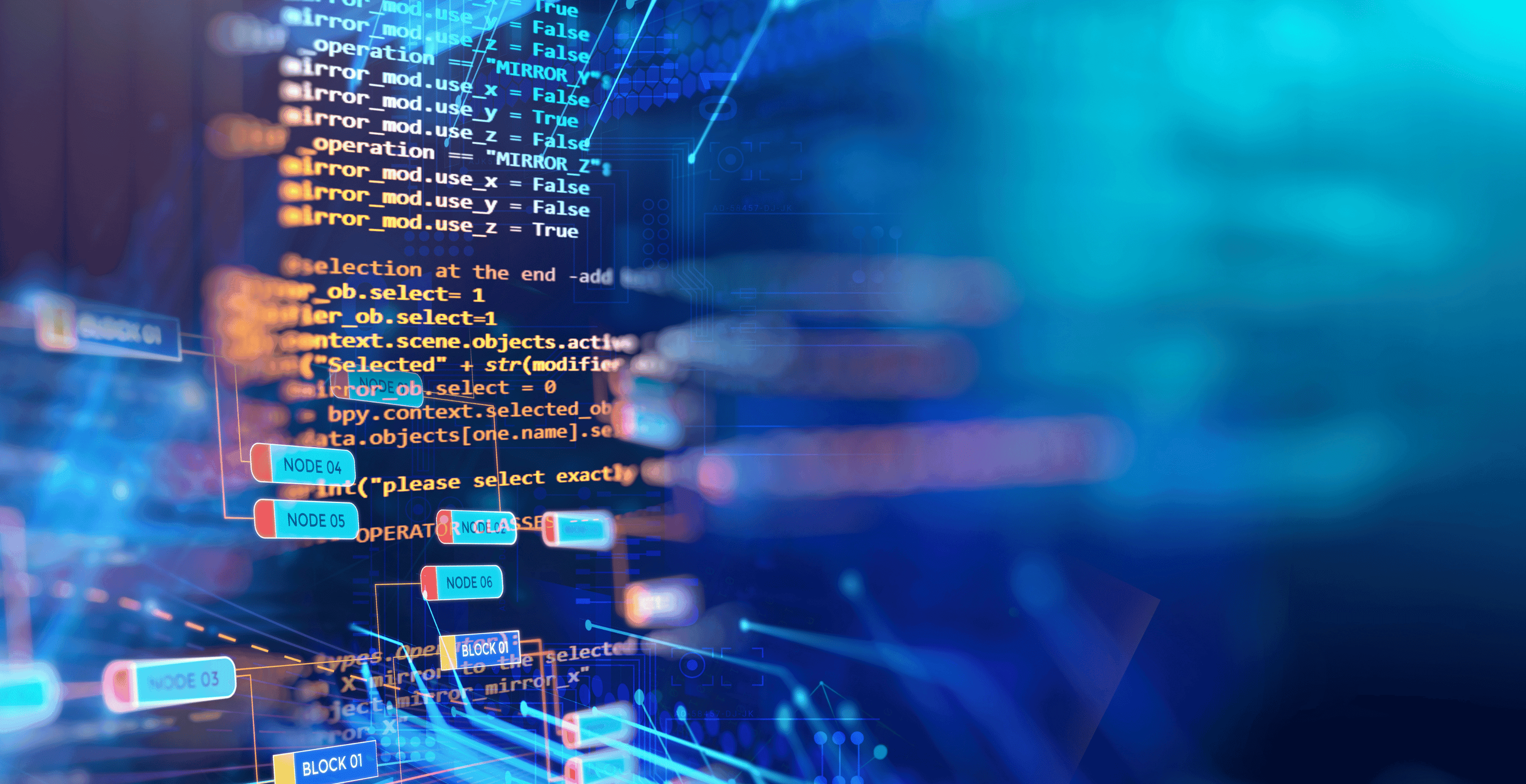
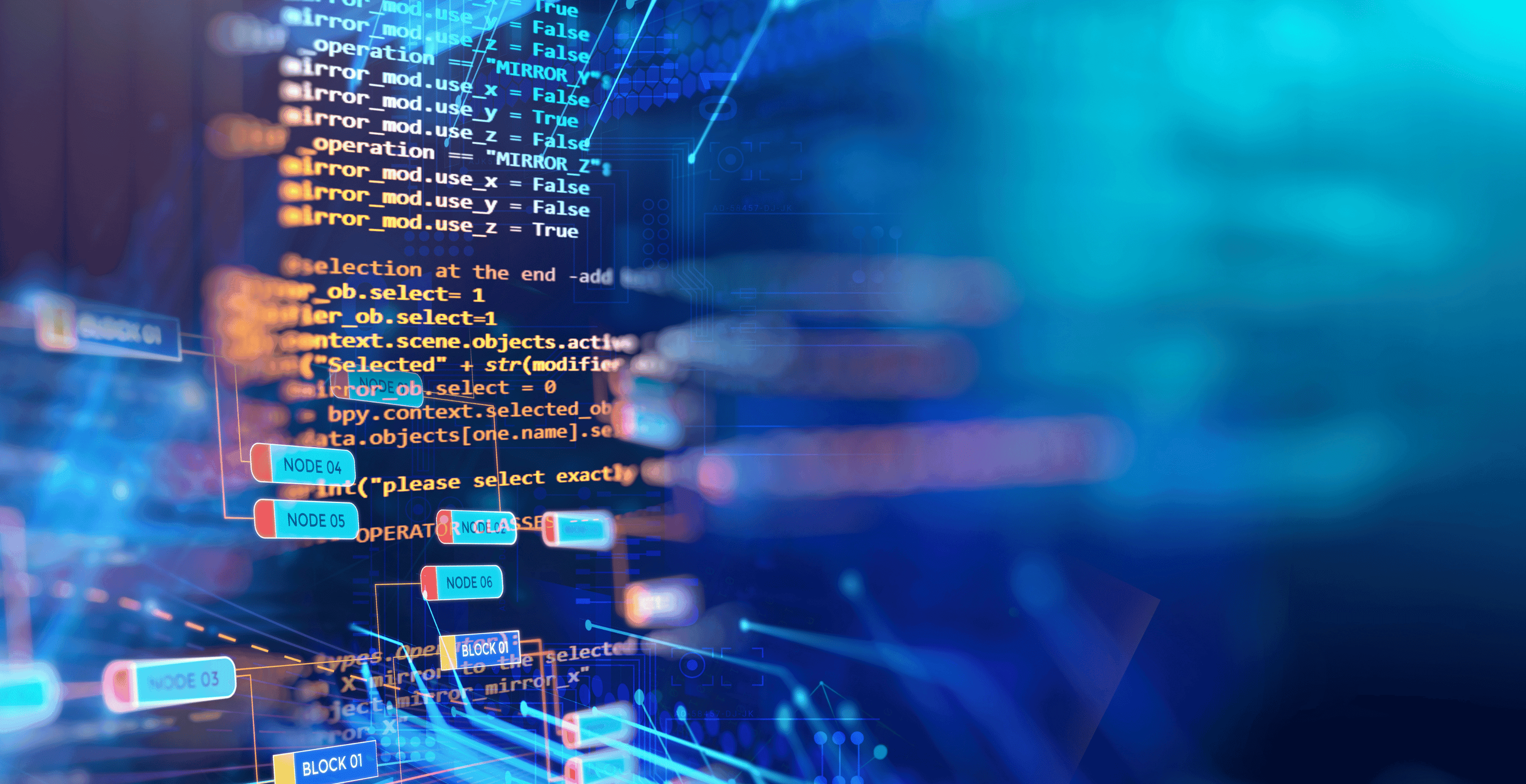
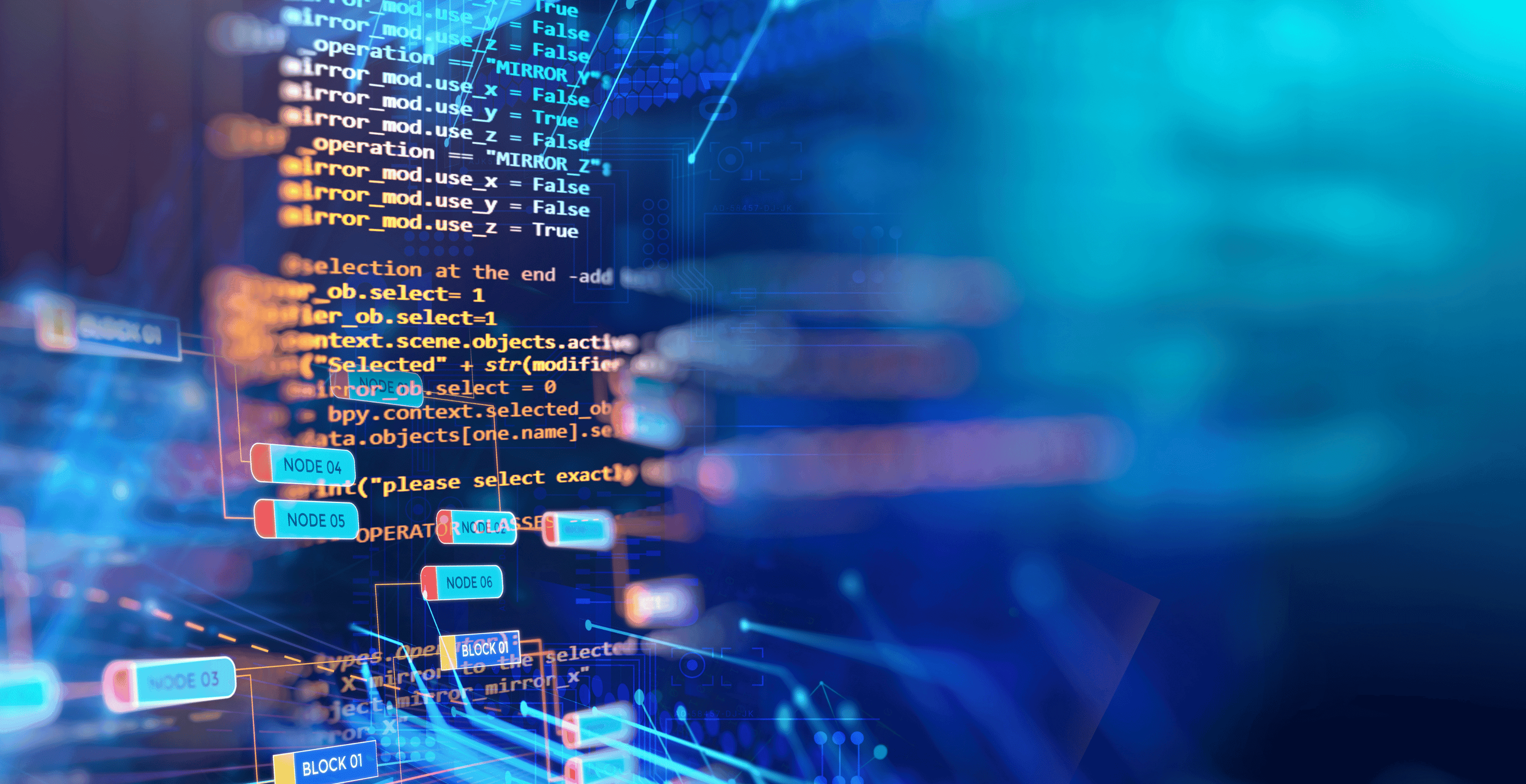
Understanding Data Fragmentation and Strategies to Overcome It
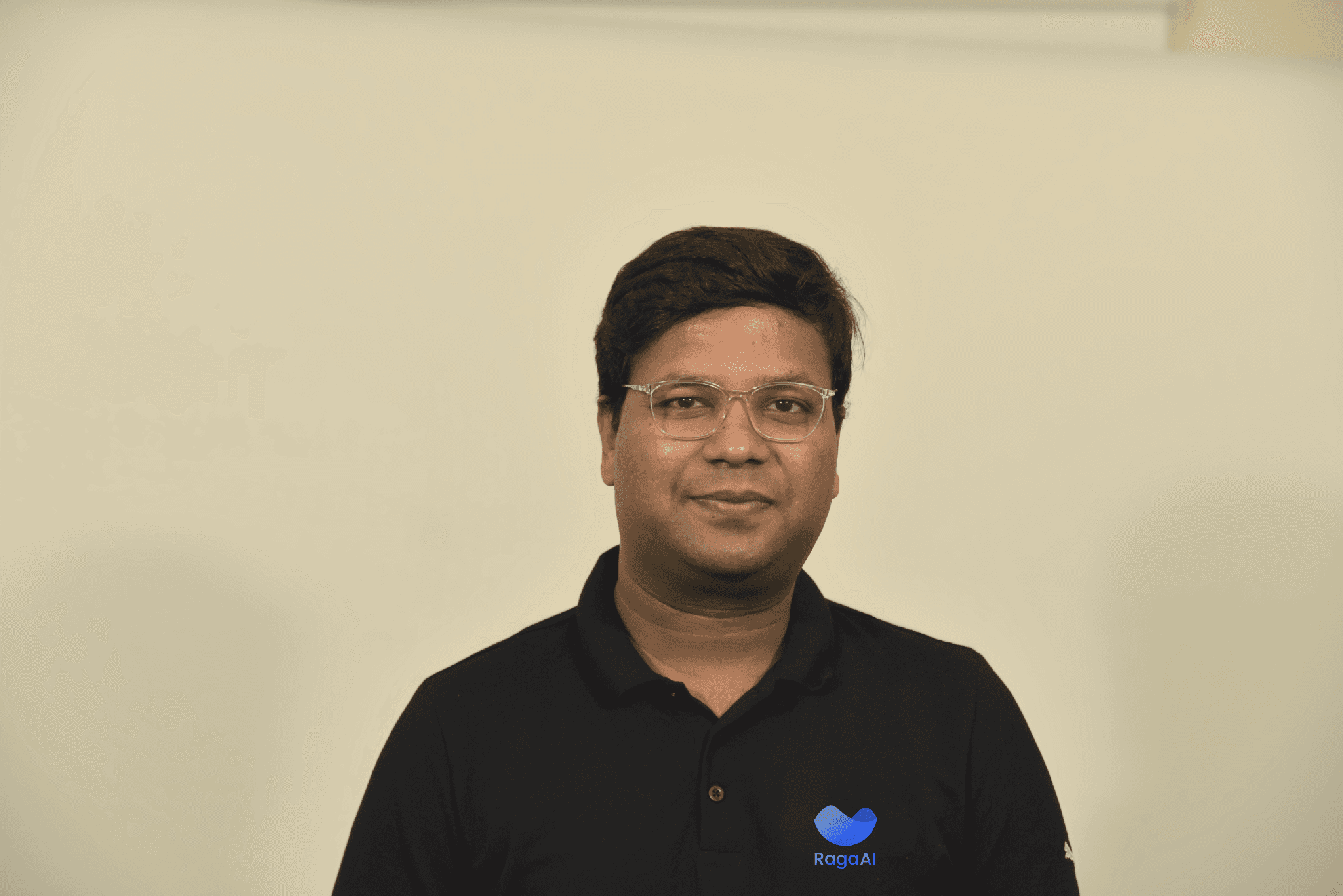
Jigar Gupta
Oct 16, 2024
Read the article
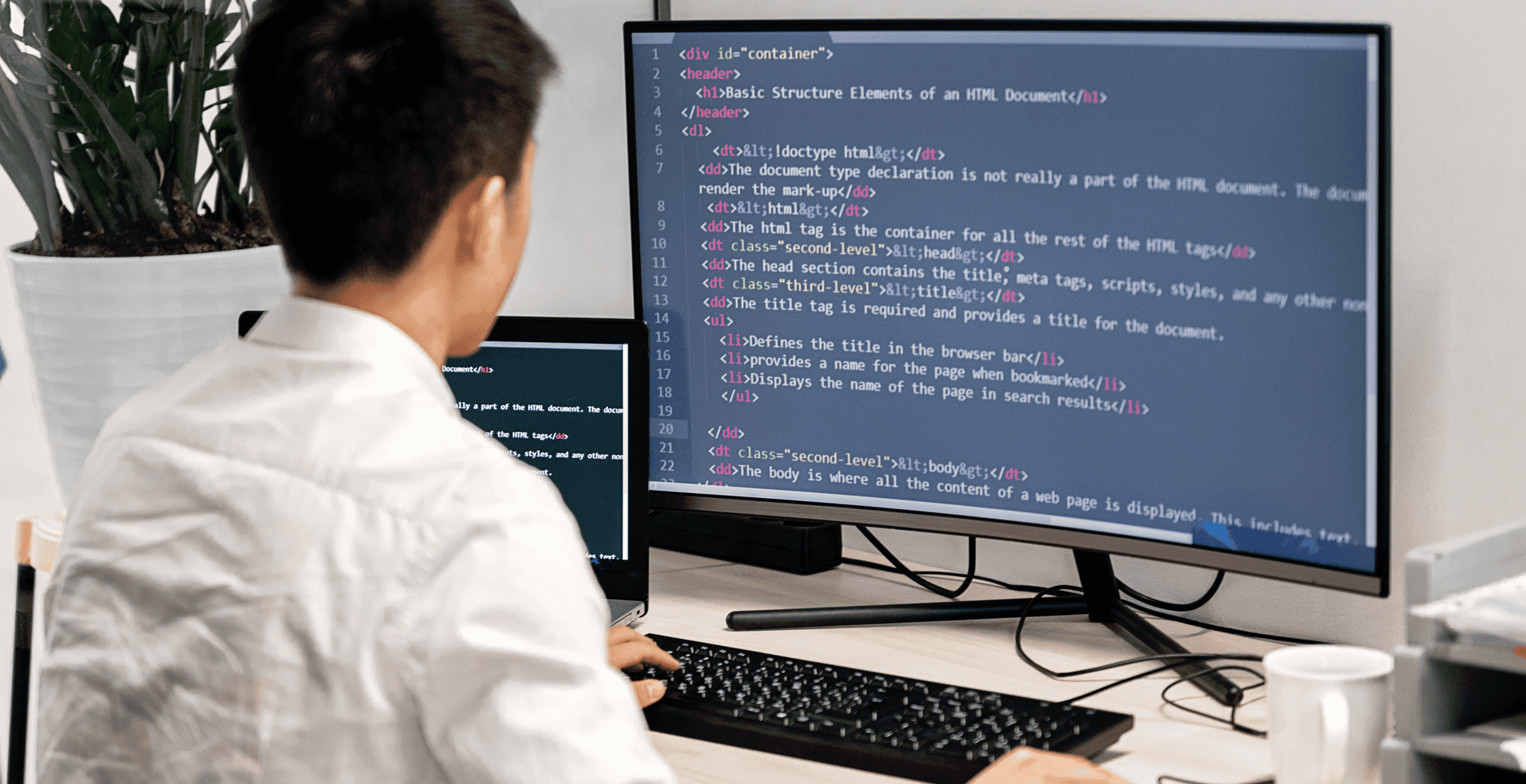
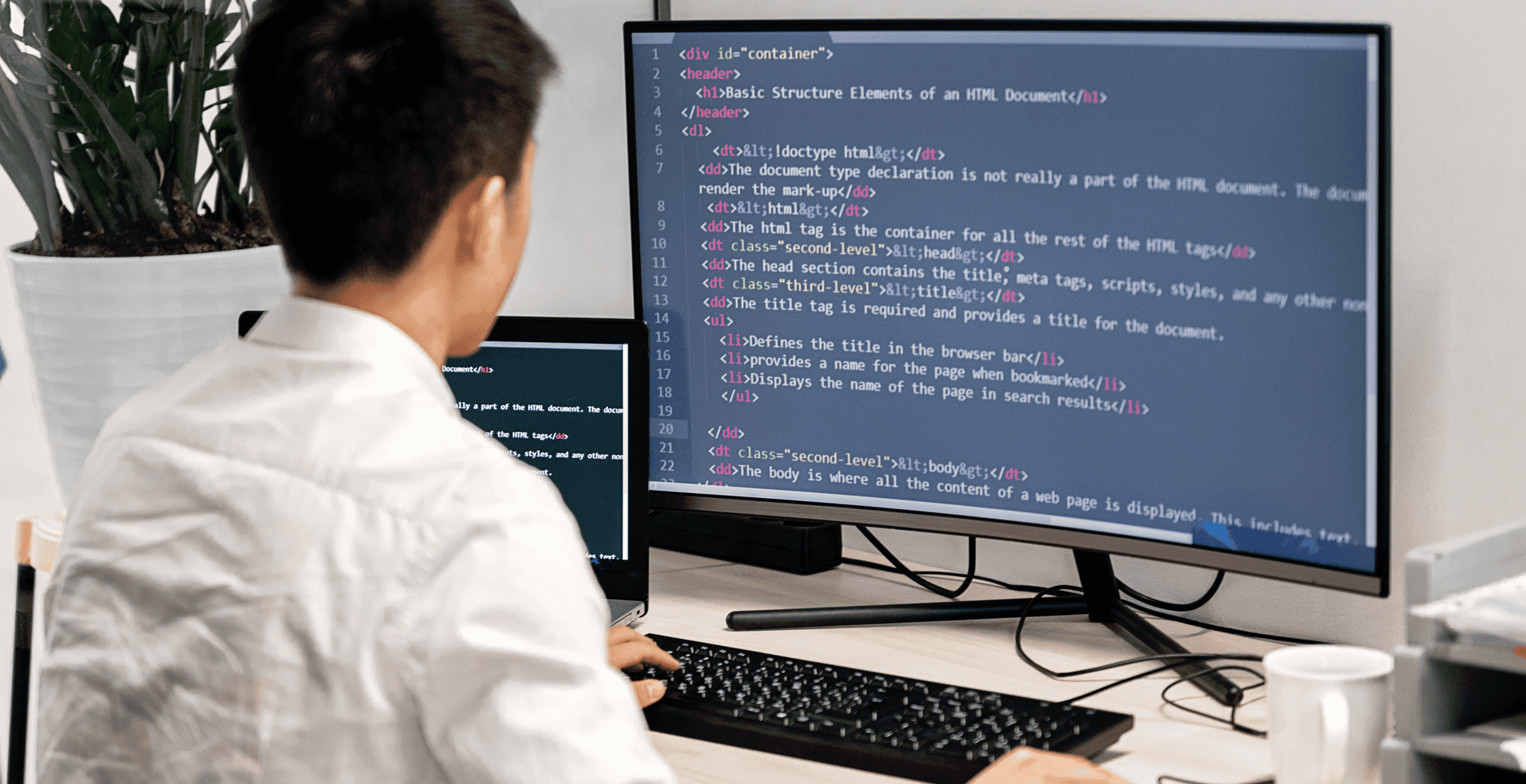
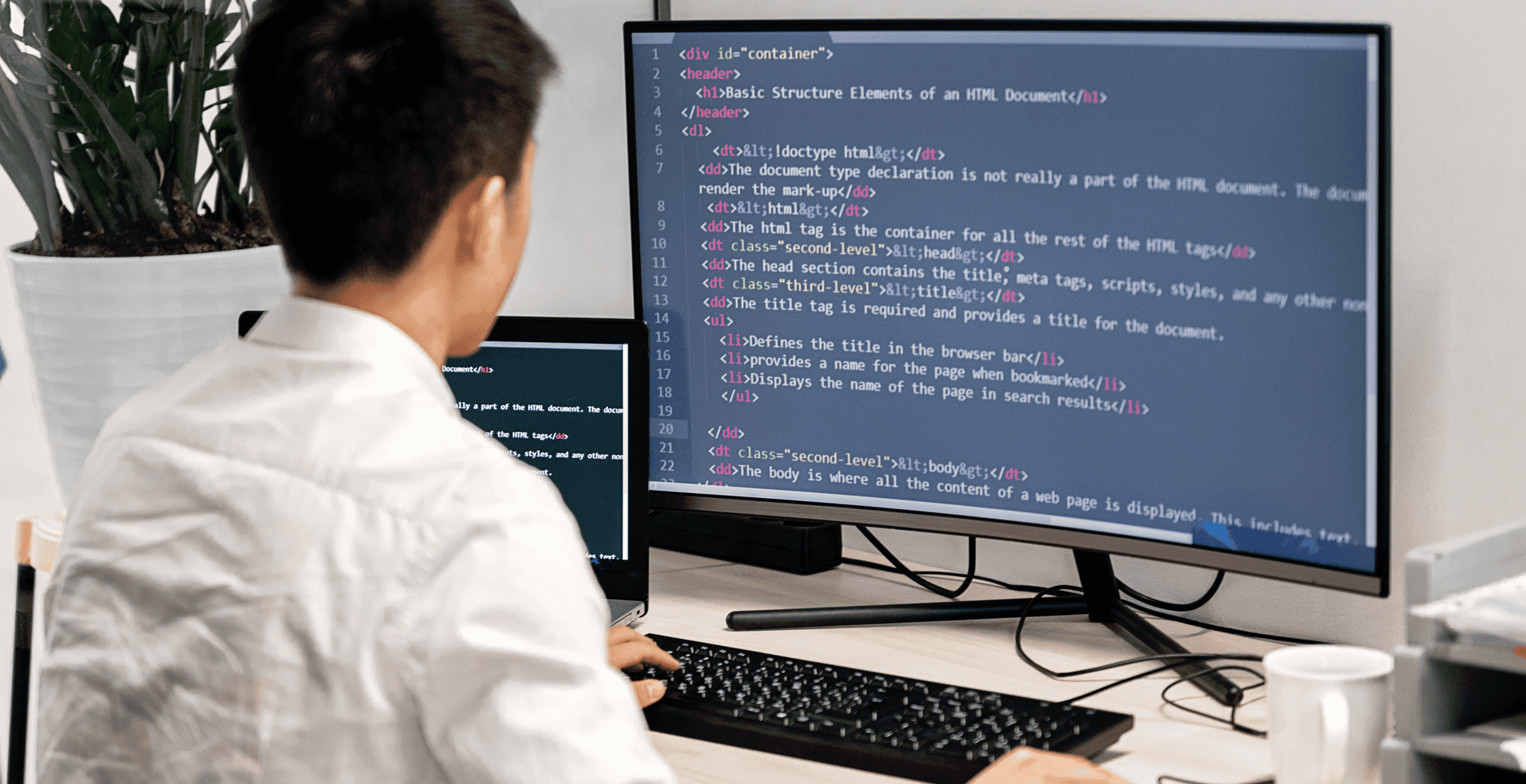
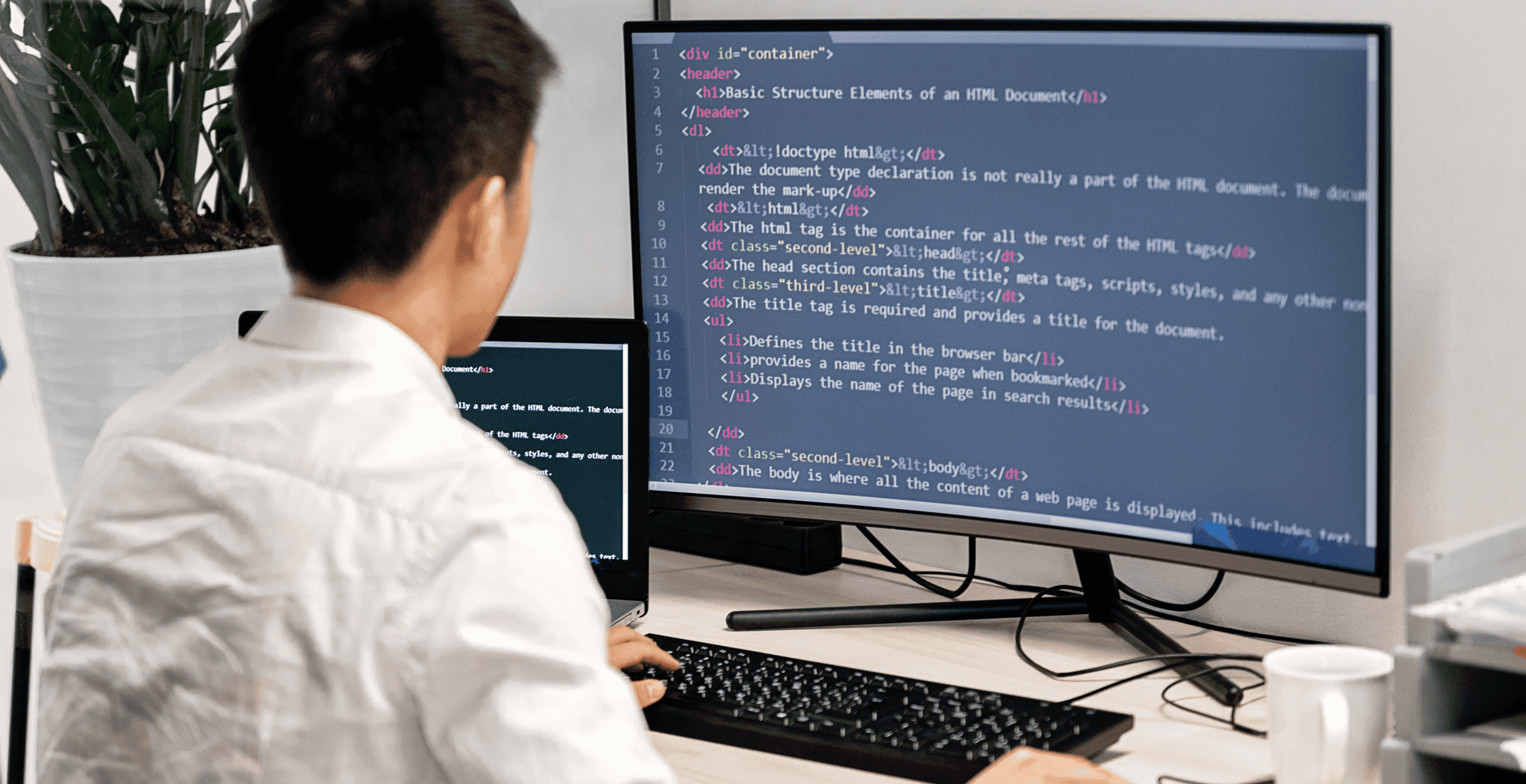
Understanding Techniques and Applications for Grounding LLMs in Data

Rehan Asif
Oct 13, 2024
Read the article




Advantages Of Using LLMs For Rapid Application Development

Rehan Asif
Oct 10, 2024
Read the article
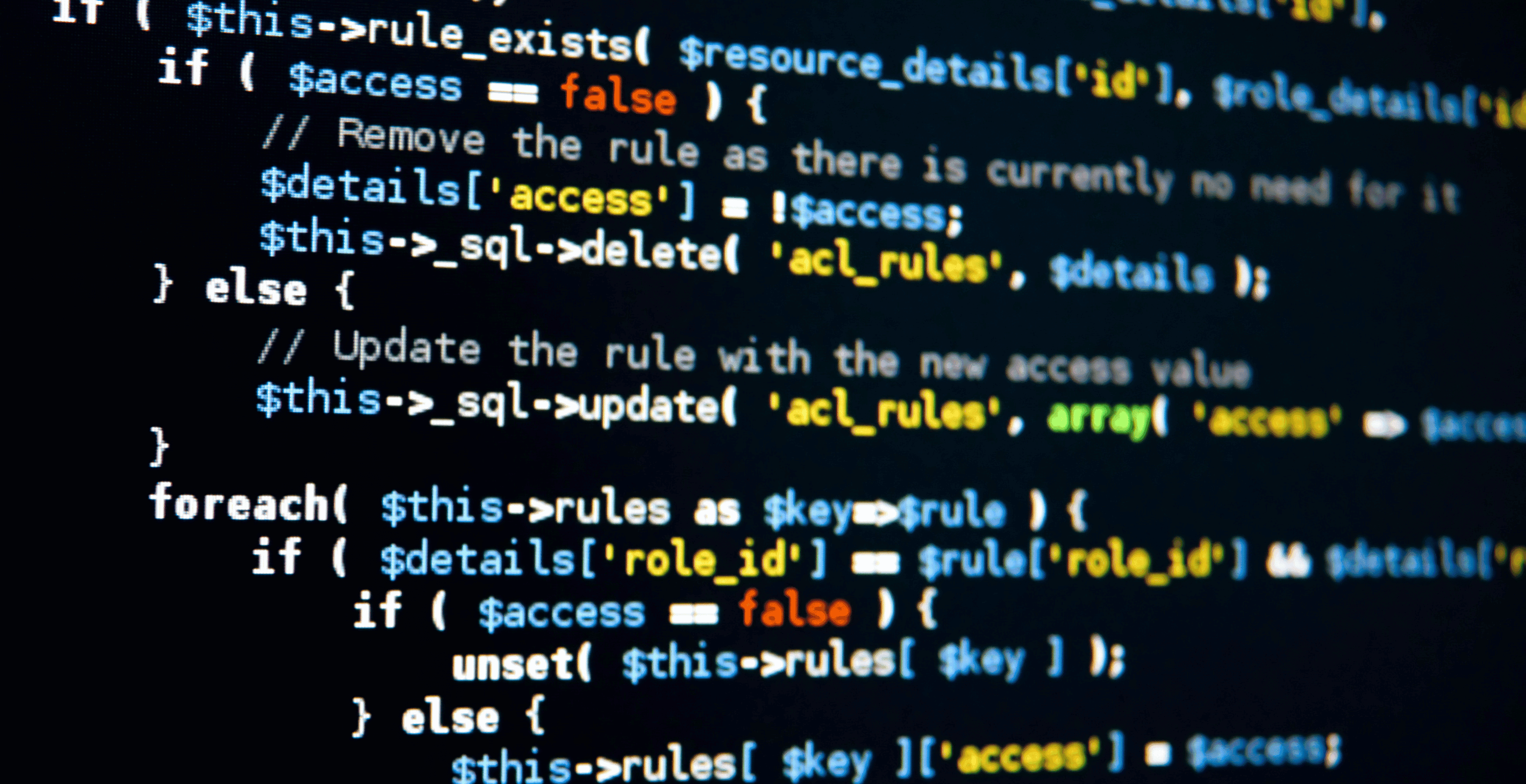
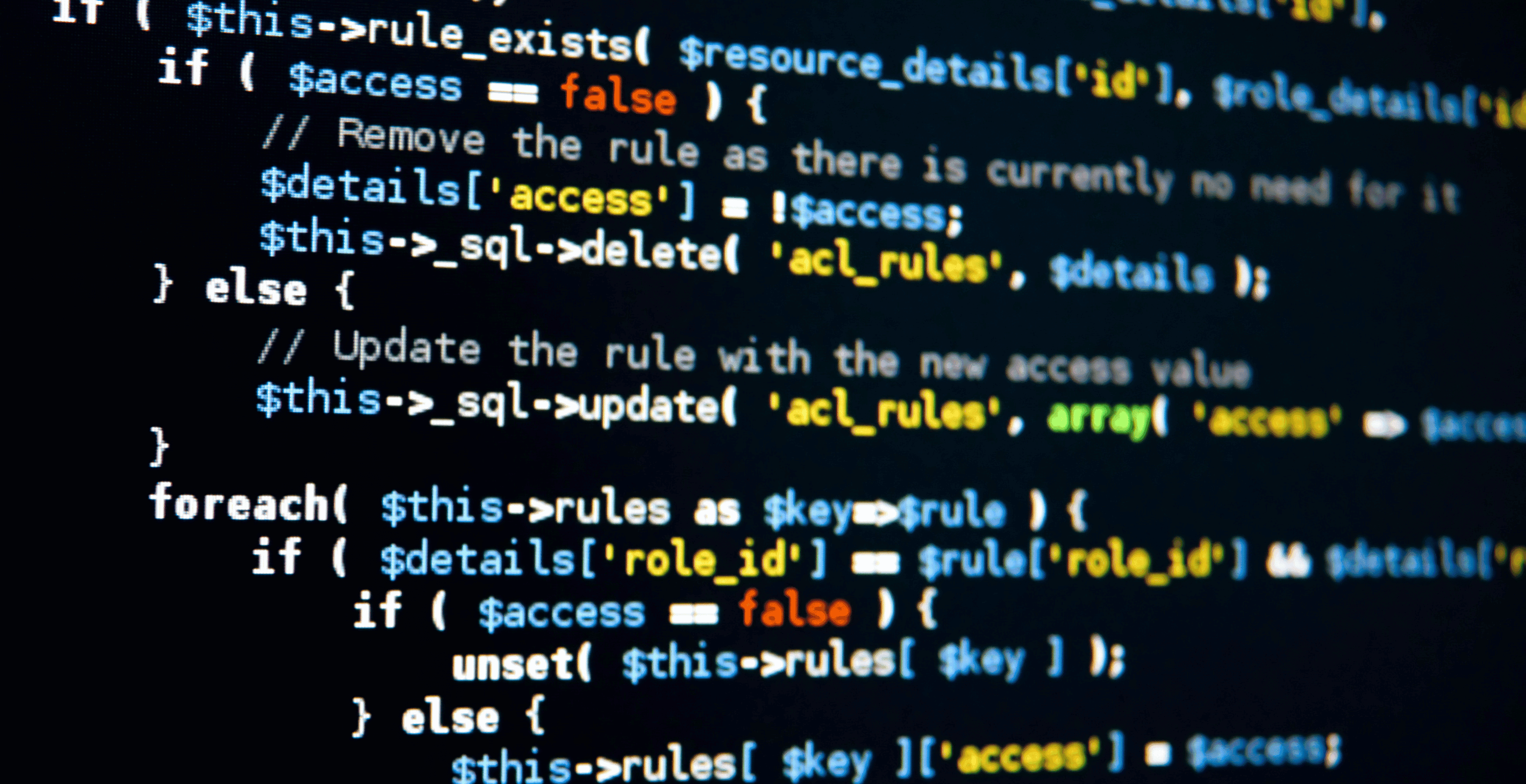
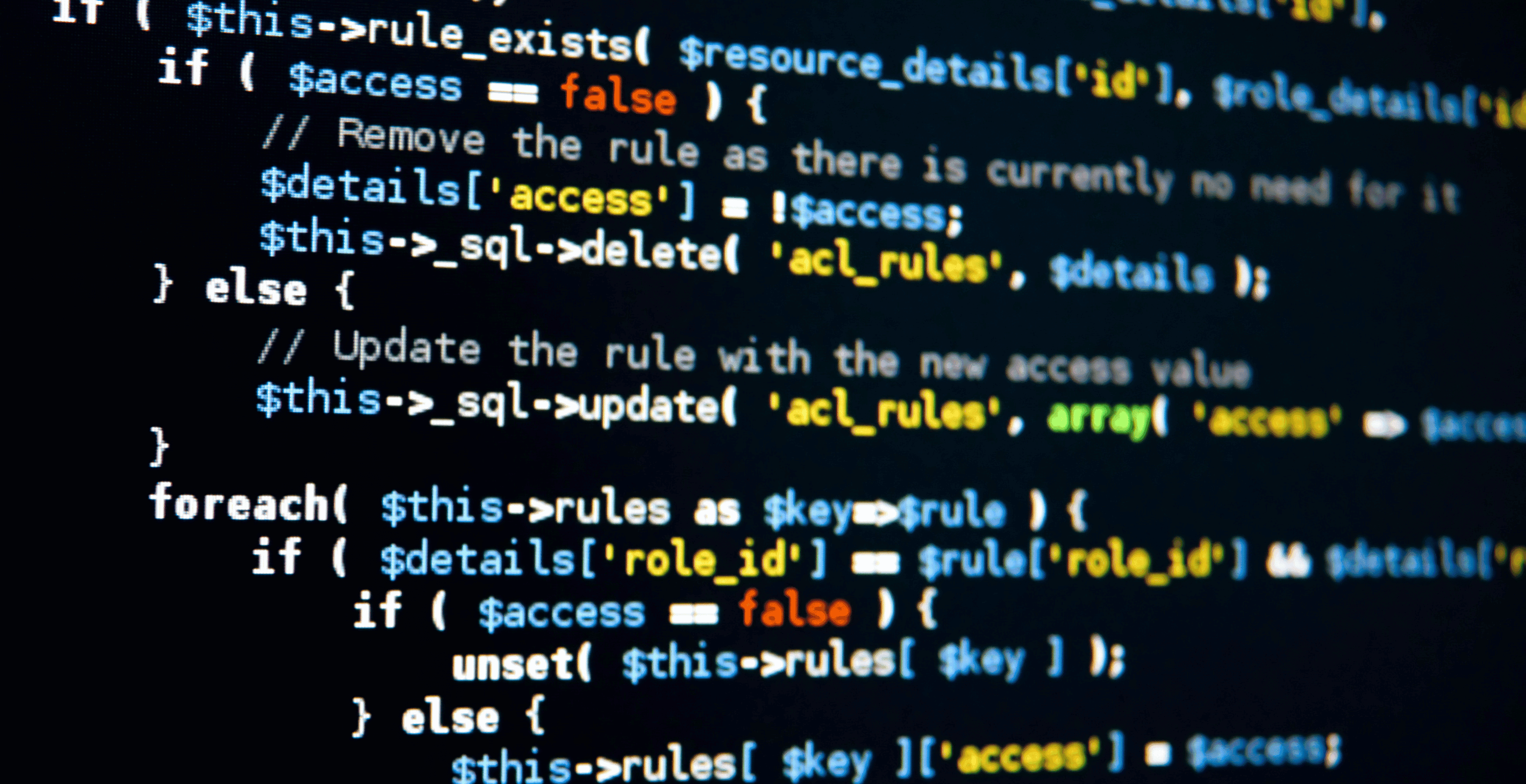
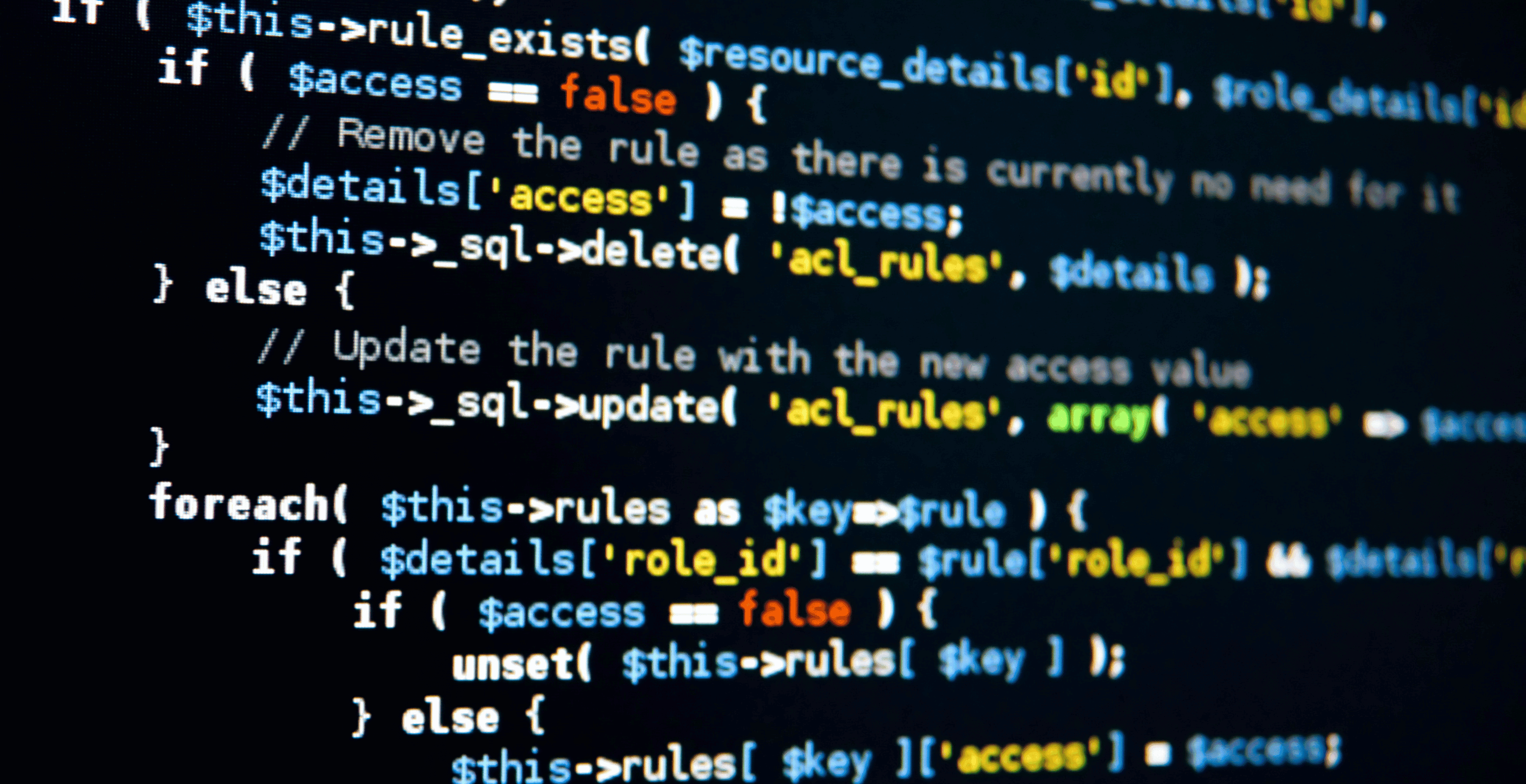
Understanding React Agent in LangChain Engineering

Rehan Asif
Oct 7, 2024
Read the article
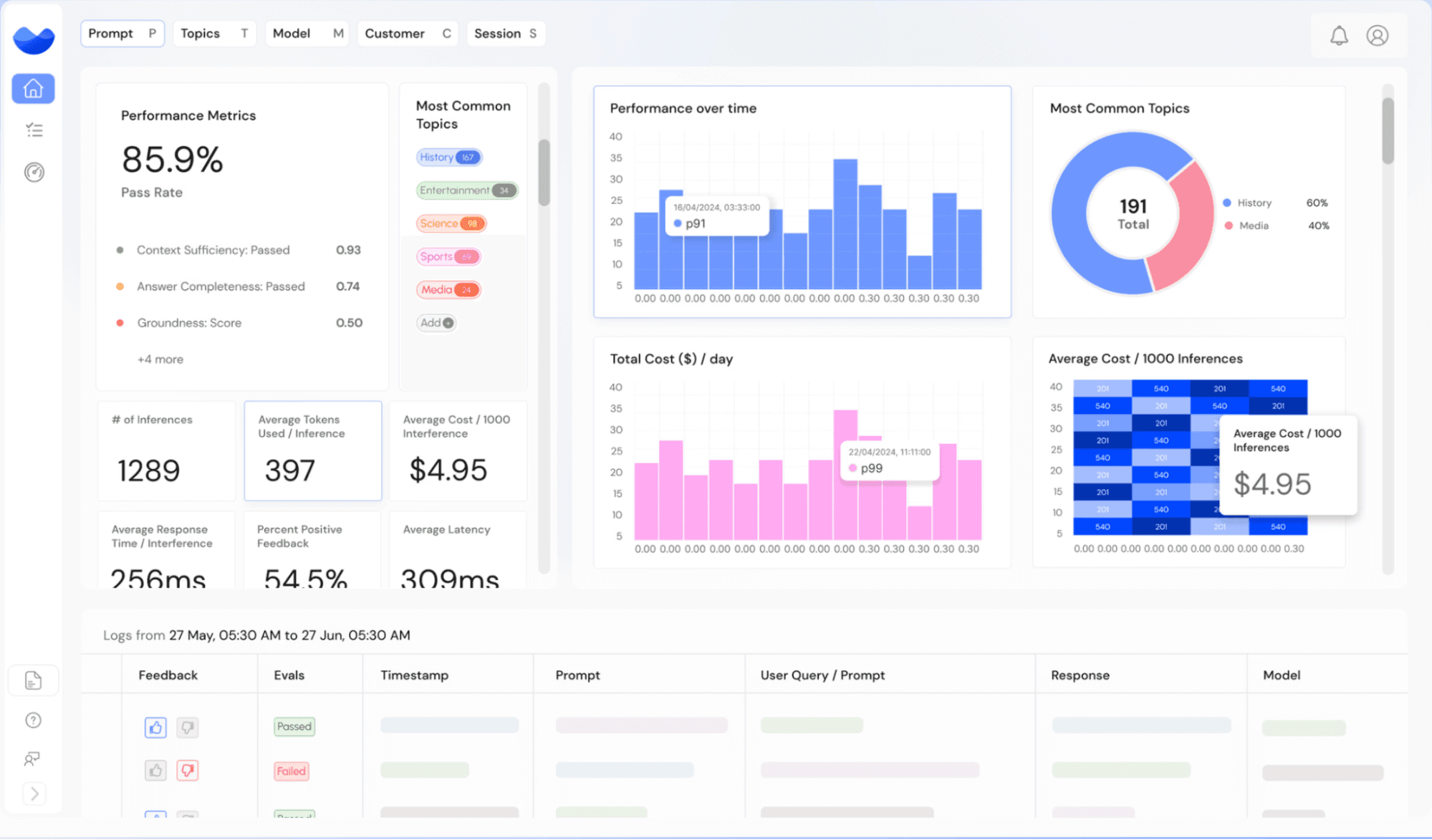
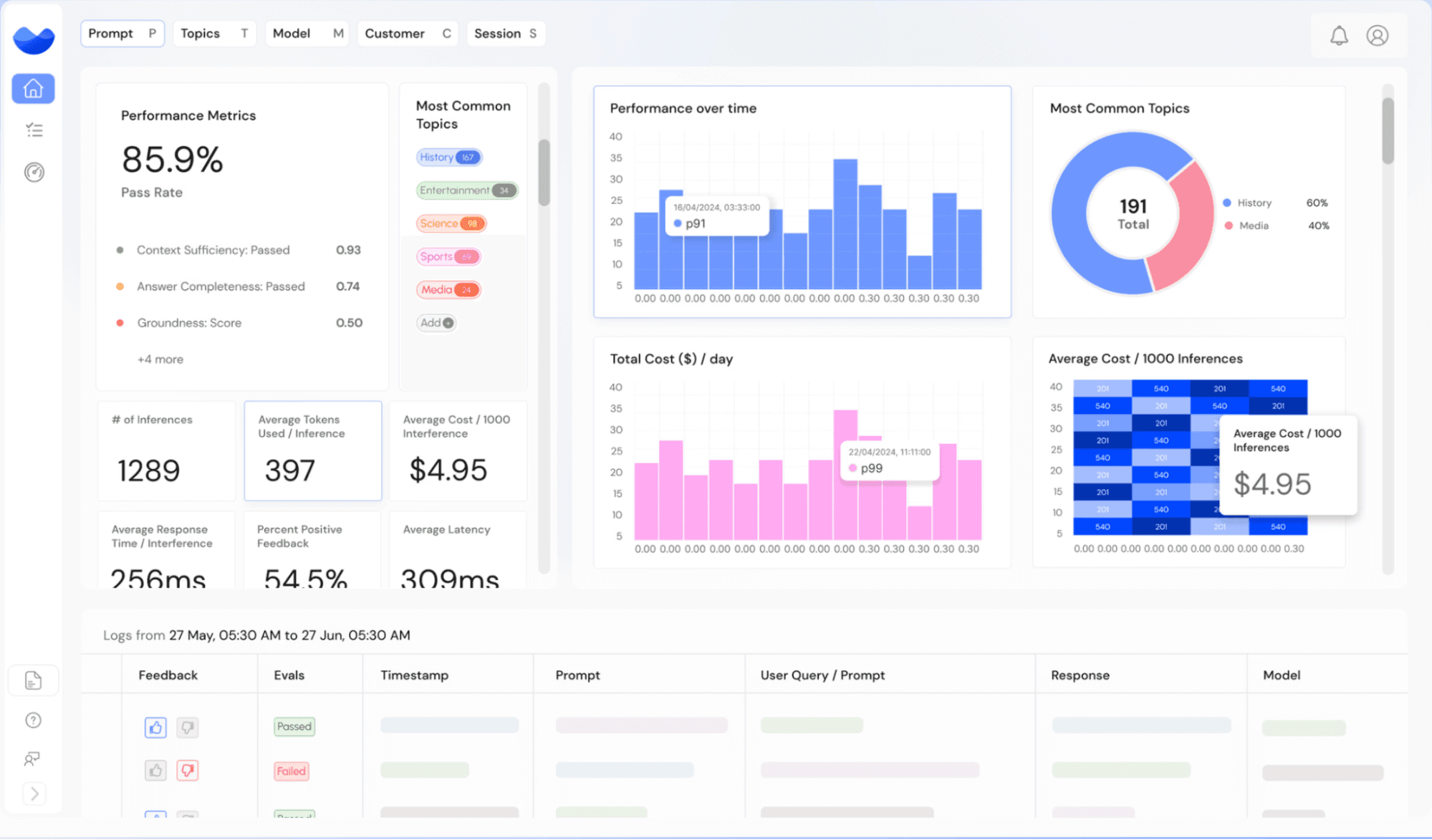
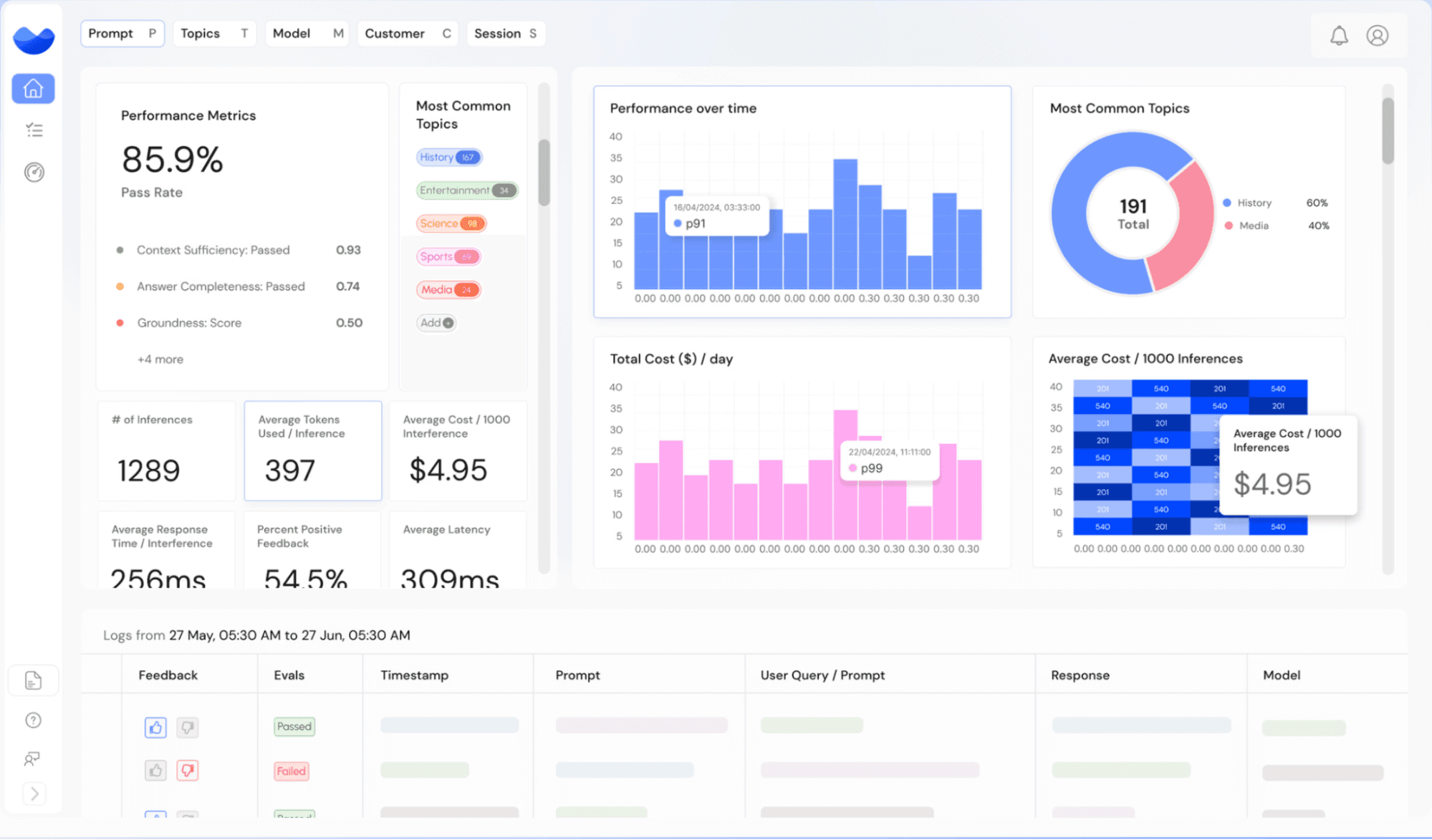
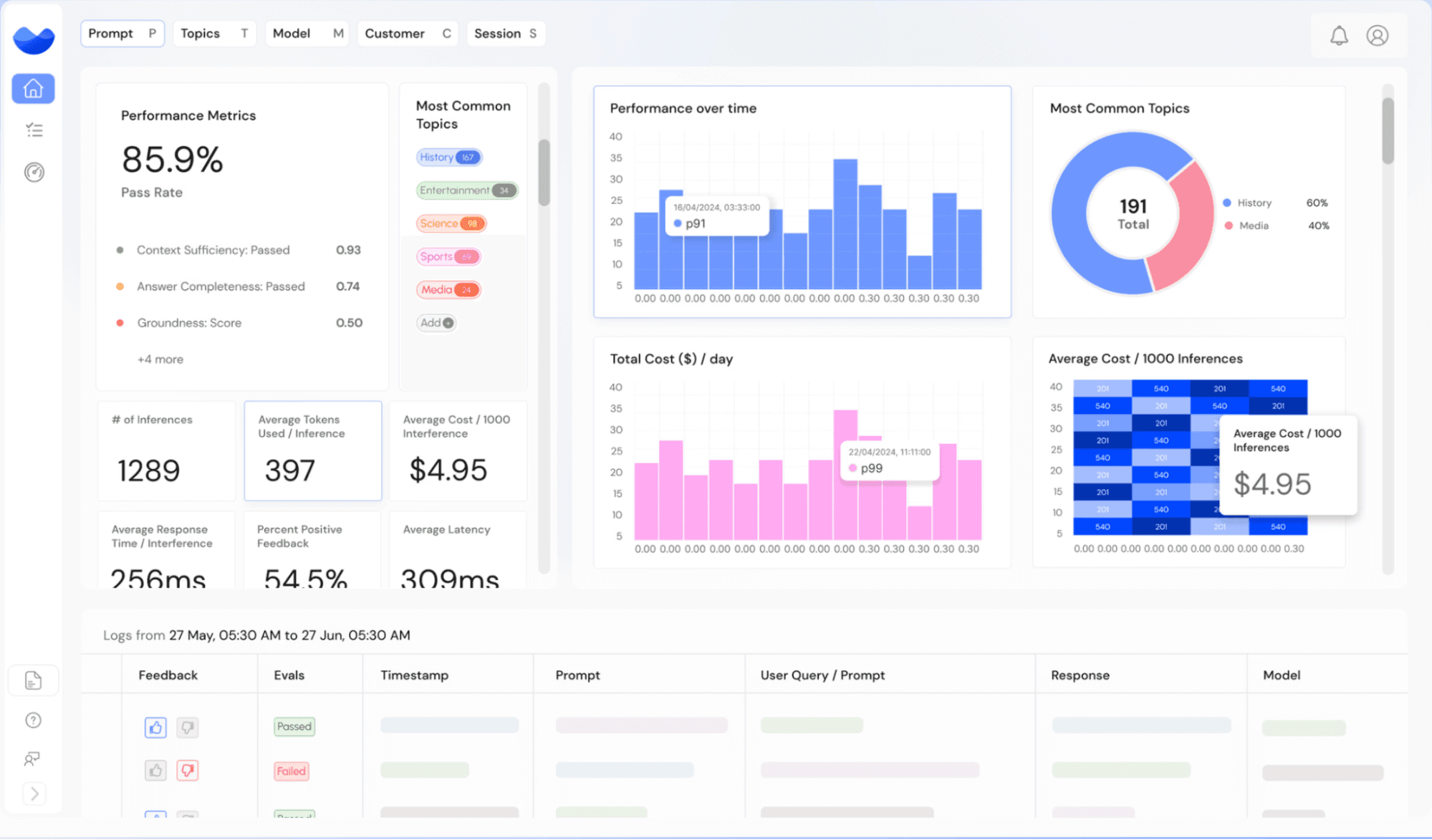
Using RagaAI Catalyst to Evaluate LLM Applications

Gaurav Agarwal
Oct 4, 2024
Read the article




Step-by-Step Guide on Training Large Language Models

Rehan Asif
Oct 1, 2024
Read the article
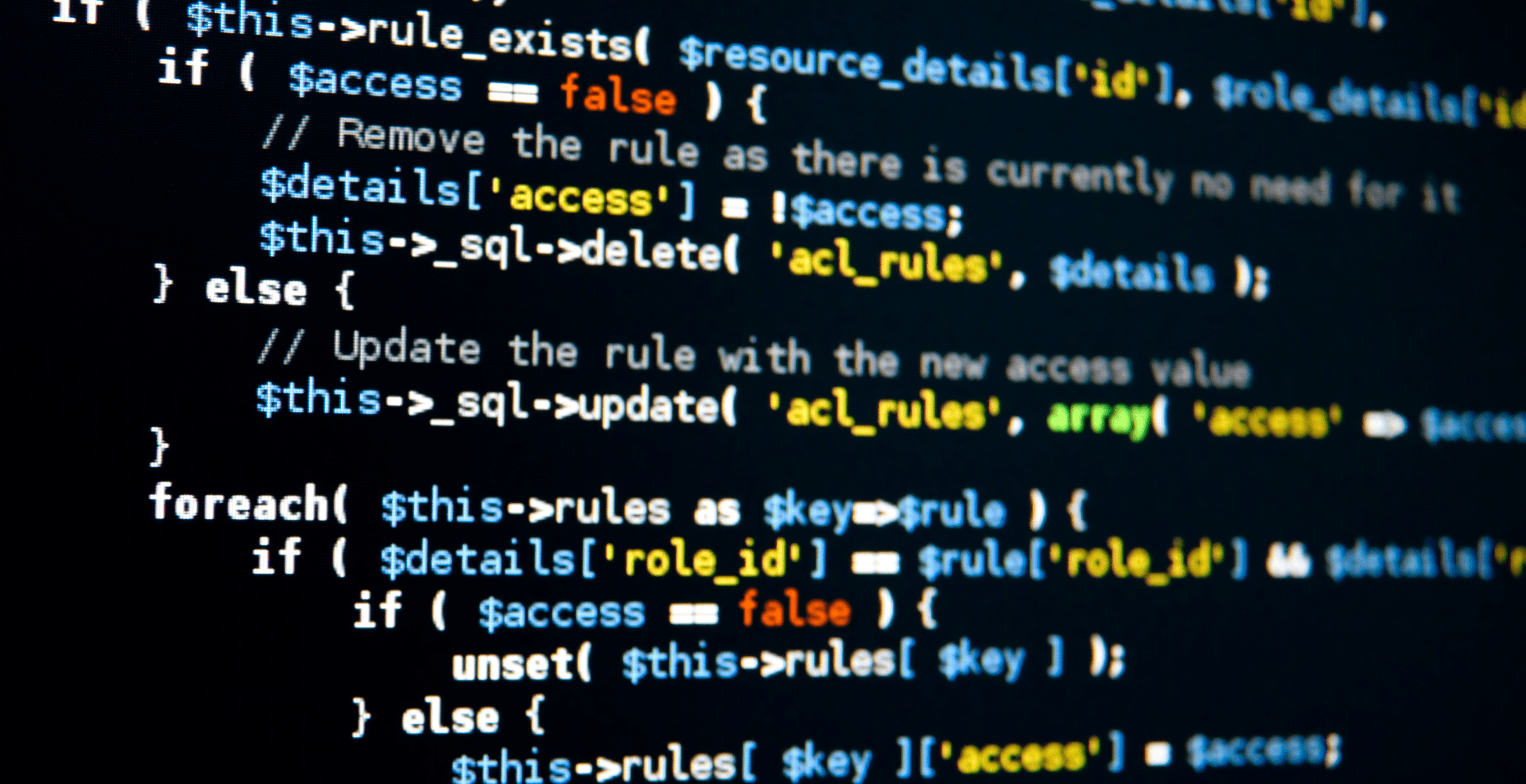
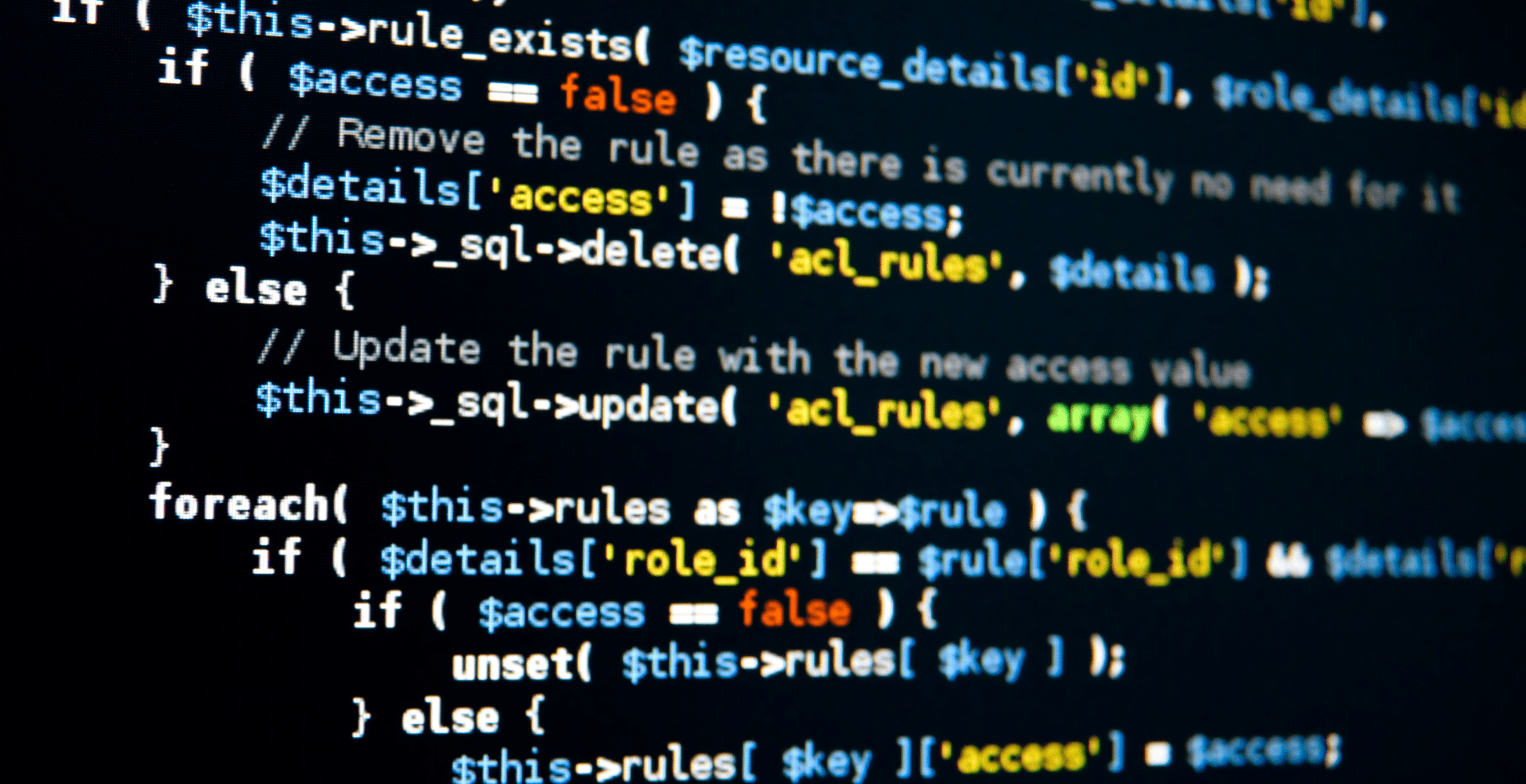
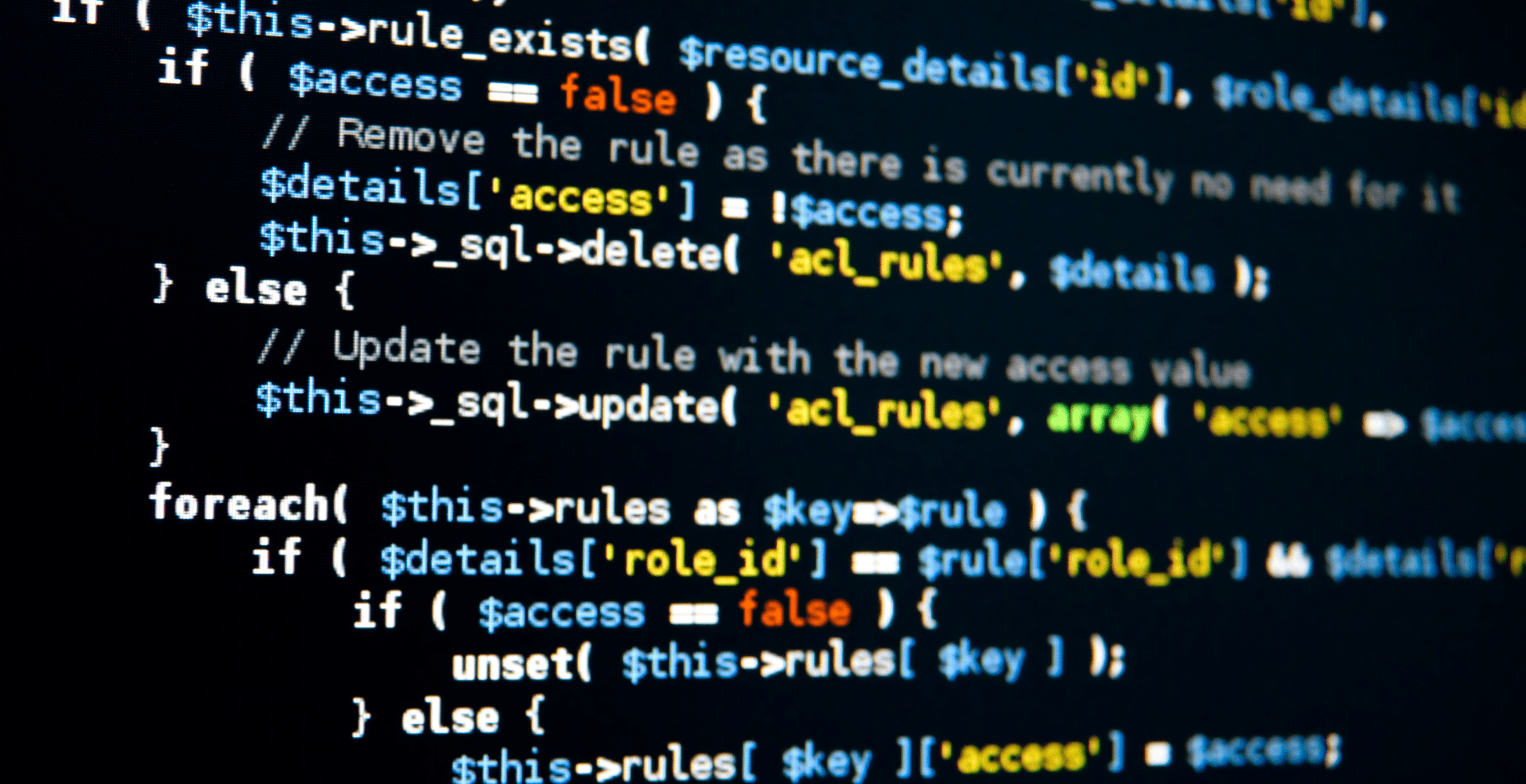
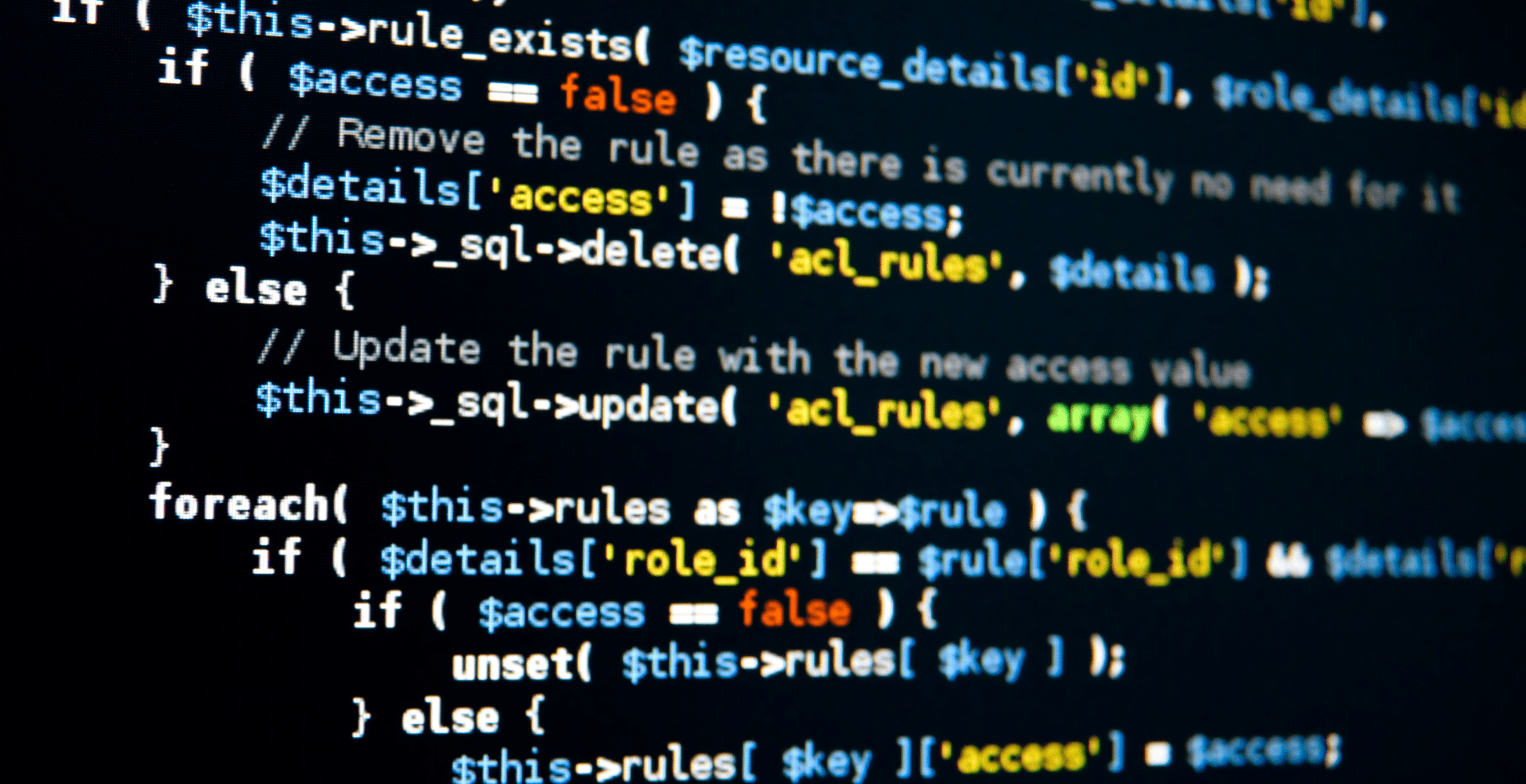
Understanding LLM Agent Architecture

Rehan Asif
Aug 19, 2024
Read the article




Understanding the Need and Possibilities of AI Guardrails Today
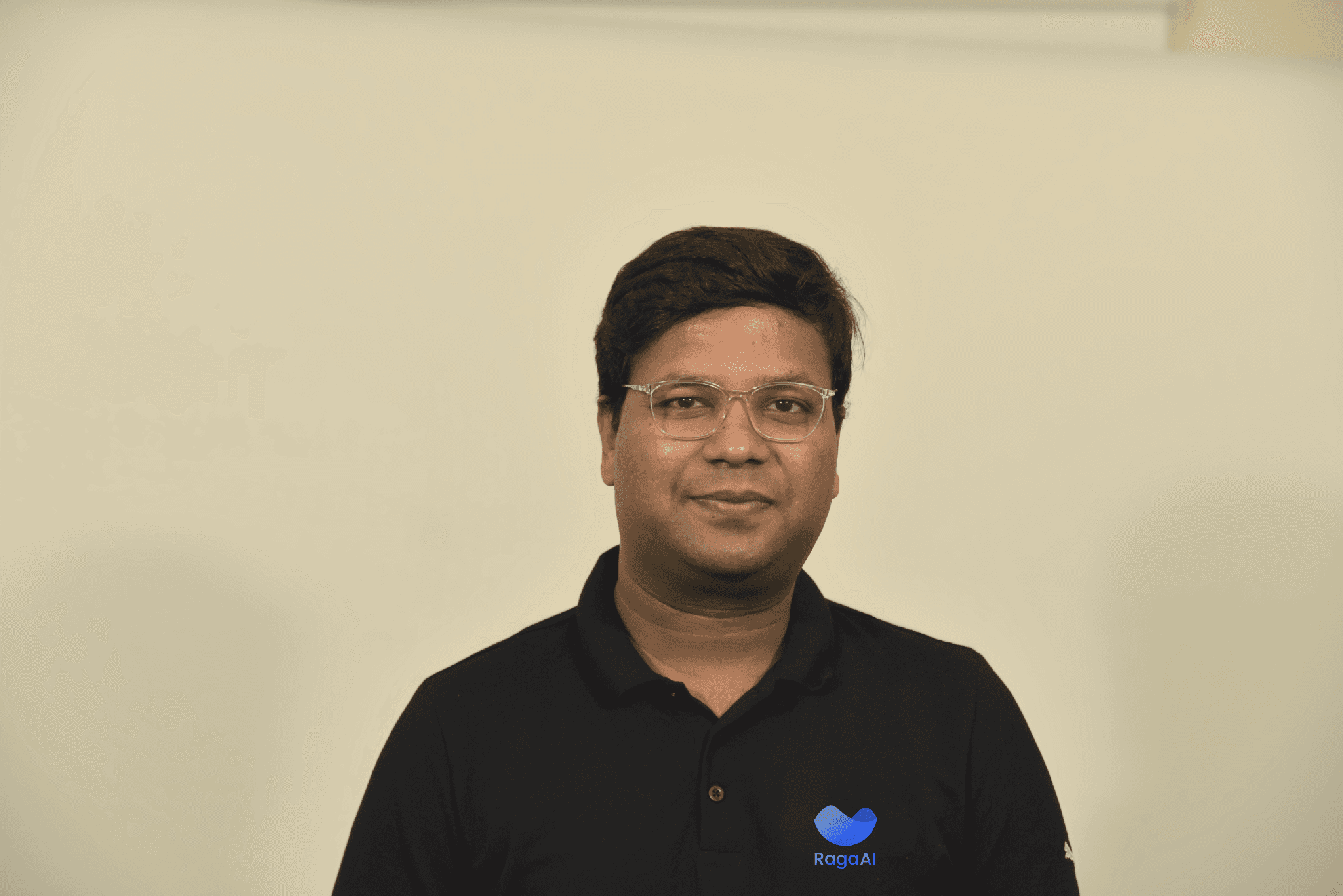
Jigar Gupta
Aug 19, 2024
Read the article




How to Prepare Quality Dataset for LLM Training

Rehan Asif
Aug 14, 2024
Read the article




Understanding Multi-Agent LLM Framework and Its Performance Scaling

Rehan Asif
Aug 15, 2024
Read the article
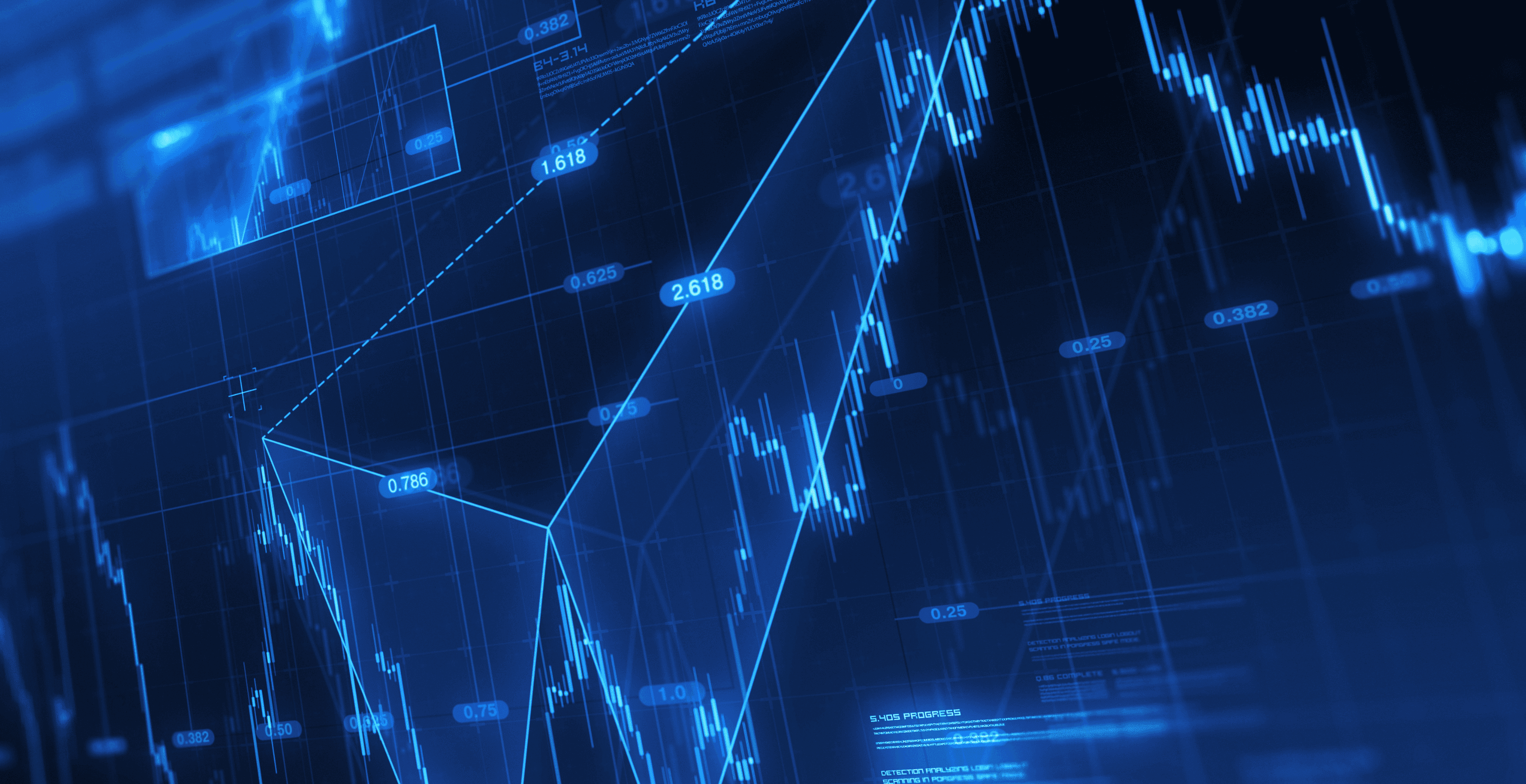
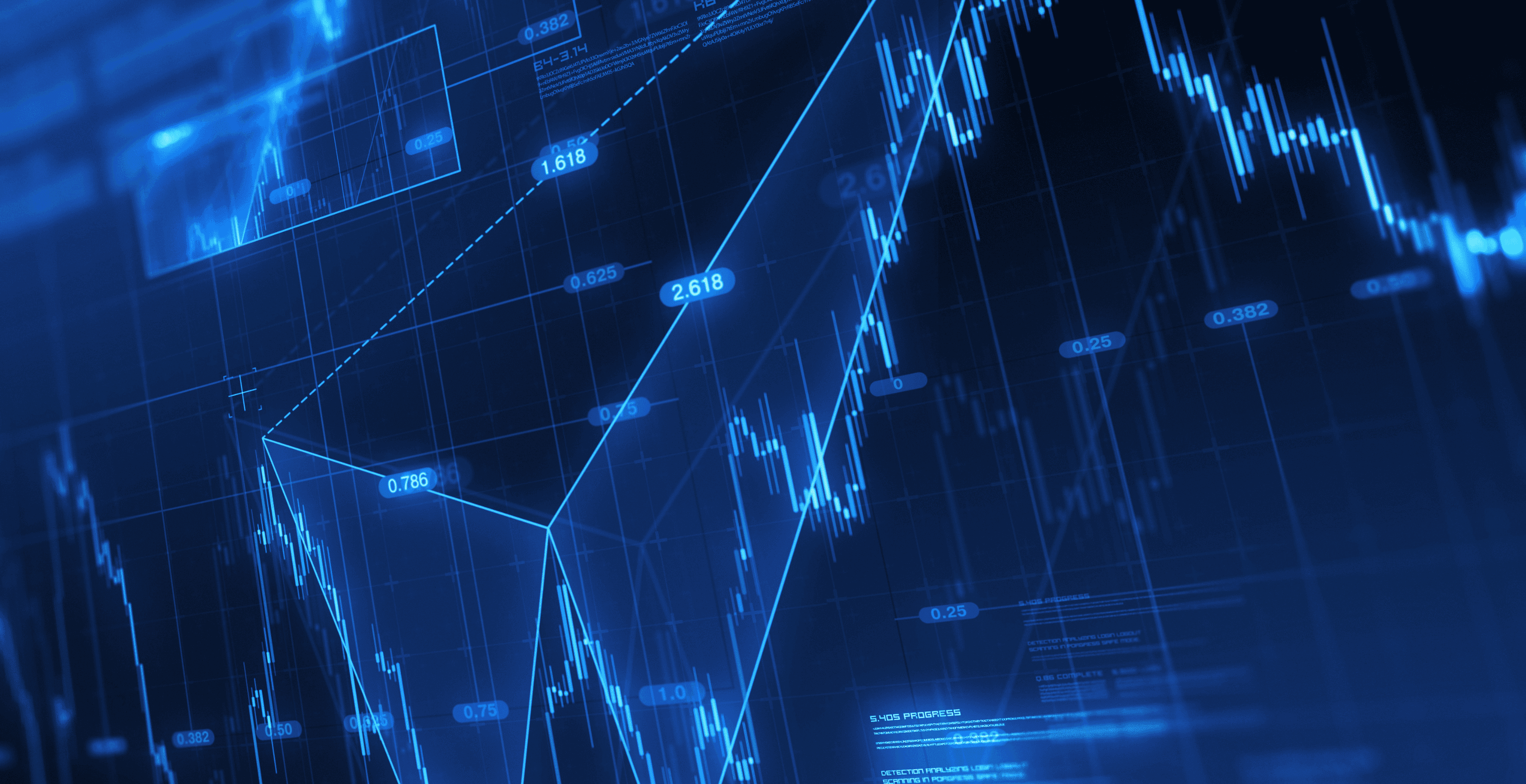
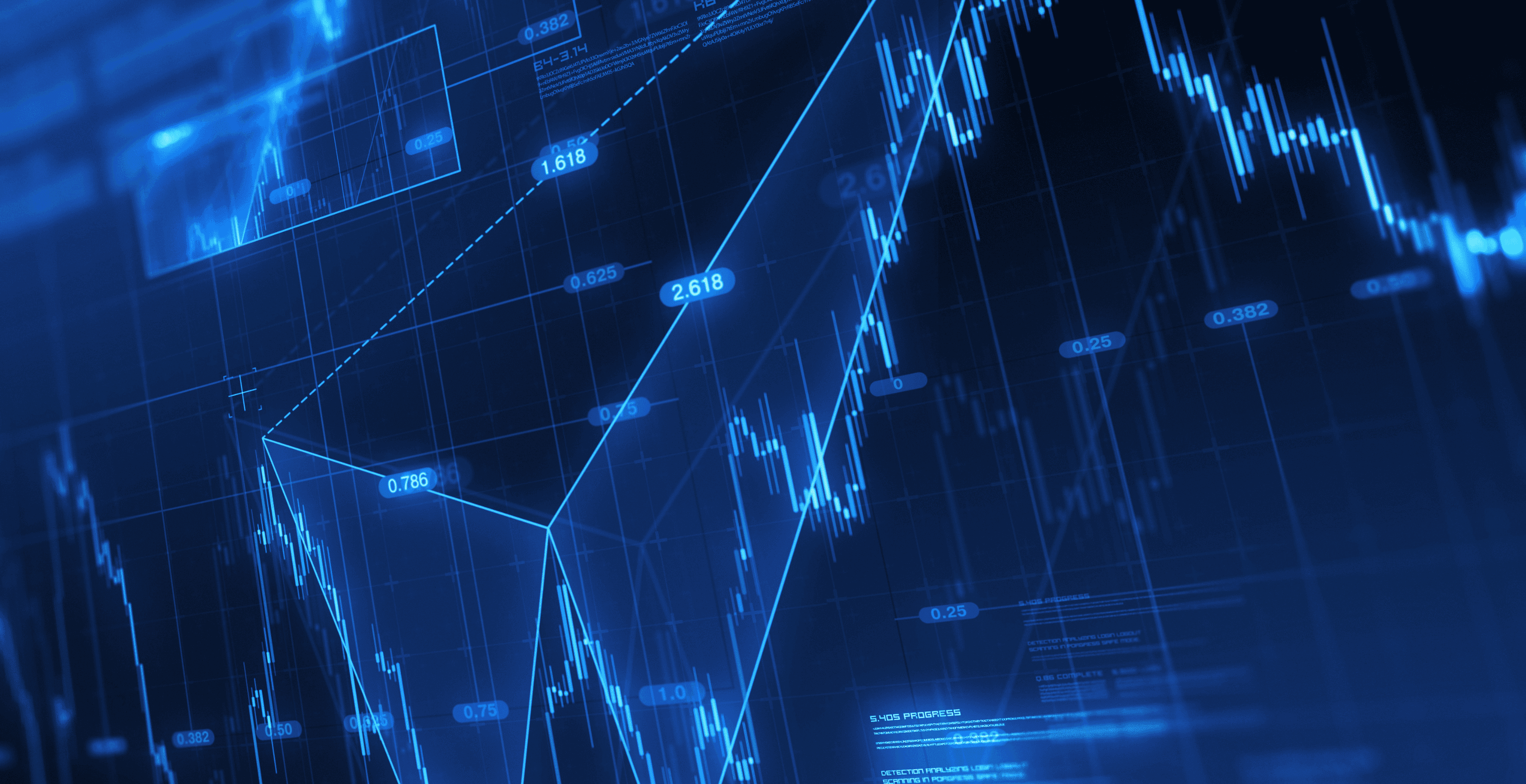
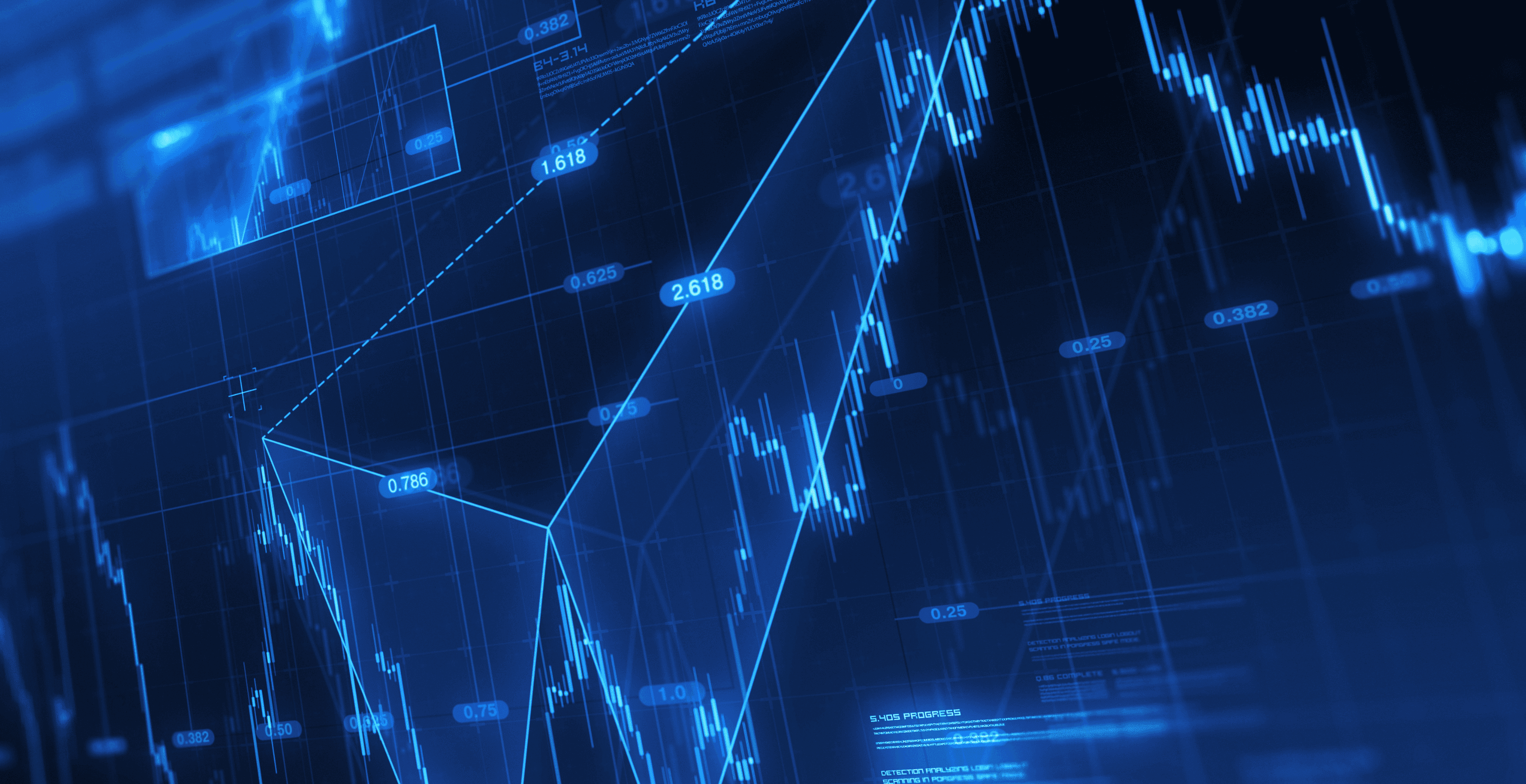
Understanding and Tackling Data Drift: Causes, Impact, and Automation Strategies
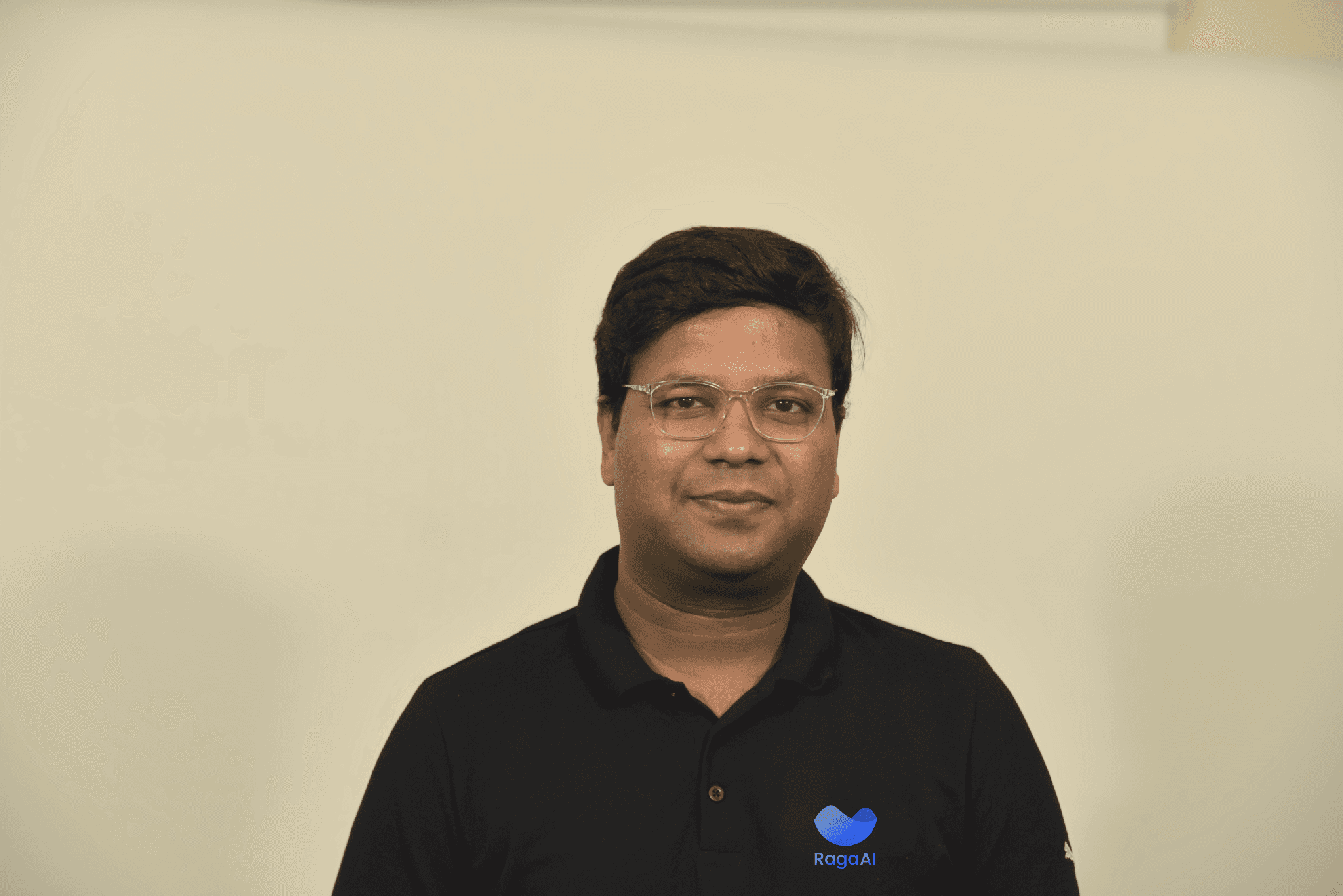
Jigar Gupta
Aug 14, 2024
Read the article




Introducing RagaAI Catalyst: Best in class automated LLM evaluation with 93% Human Alignment
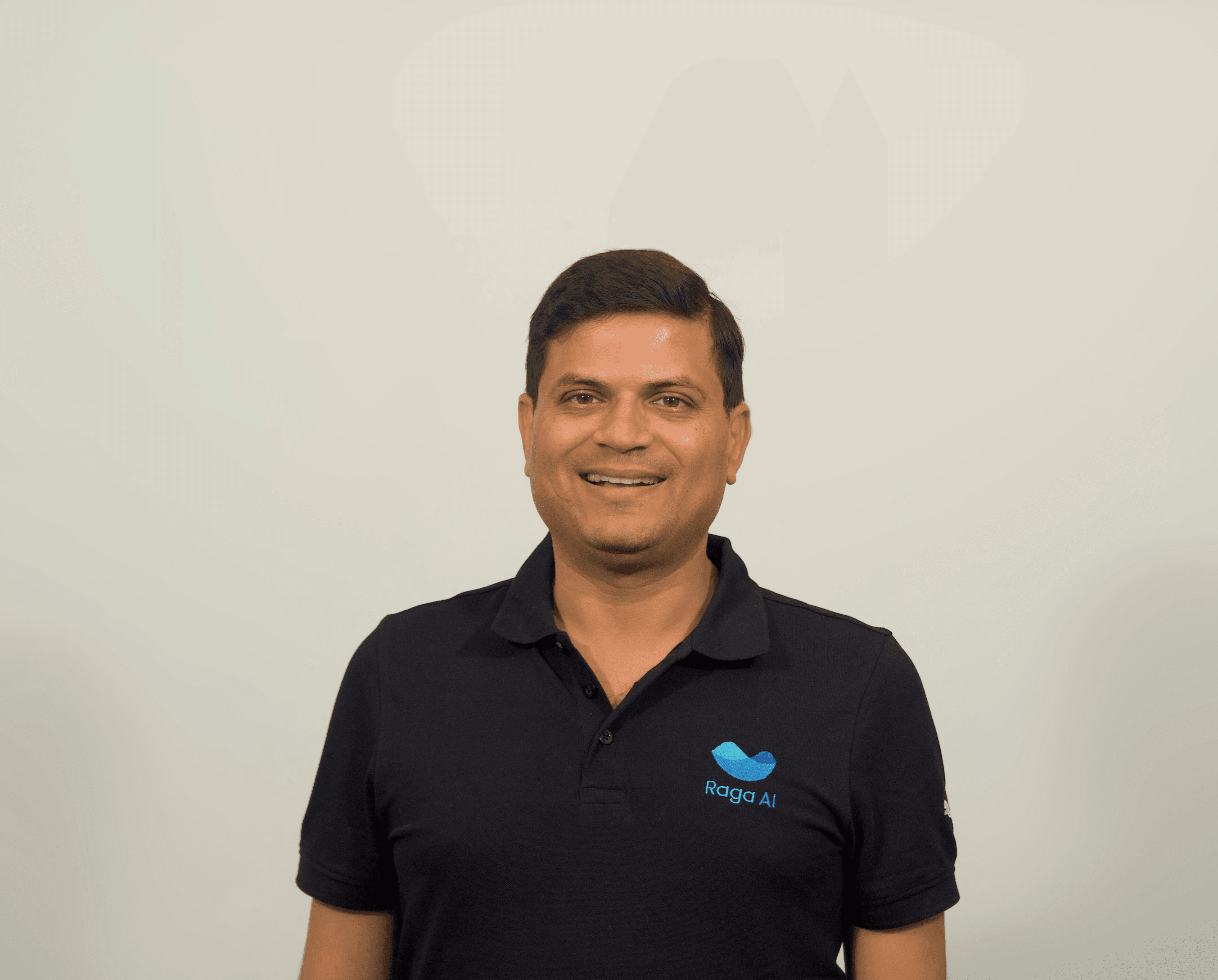
Gaurav Agarwal
Jul 15, 2024
Read the article




Key Pillars and Techniques for LLM Observability and Monitoring

Rehan Asif
Jul 24, 2024
Read the article
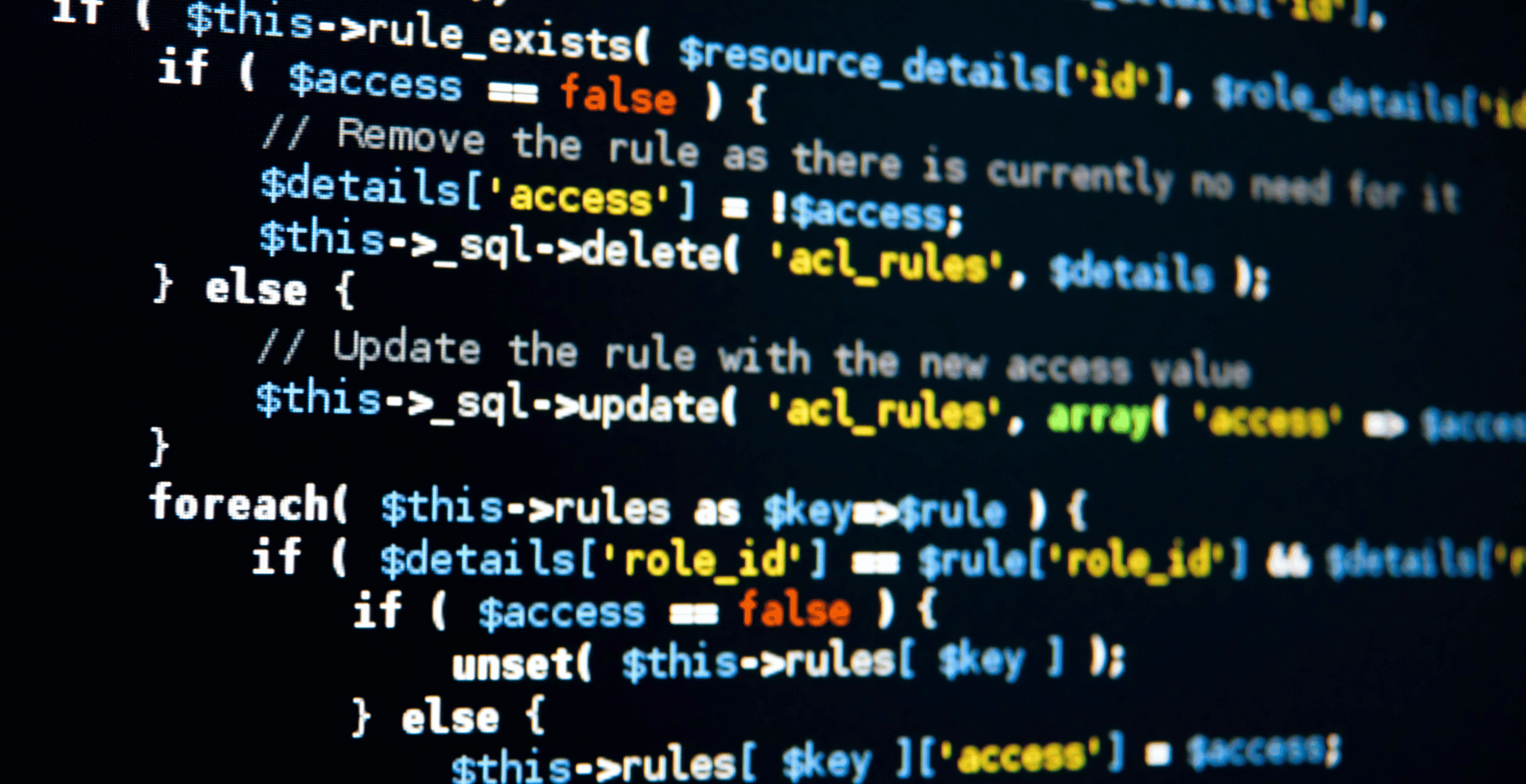
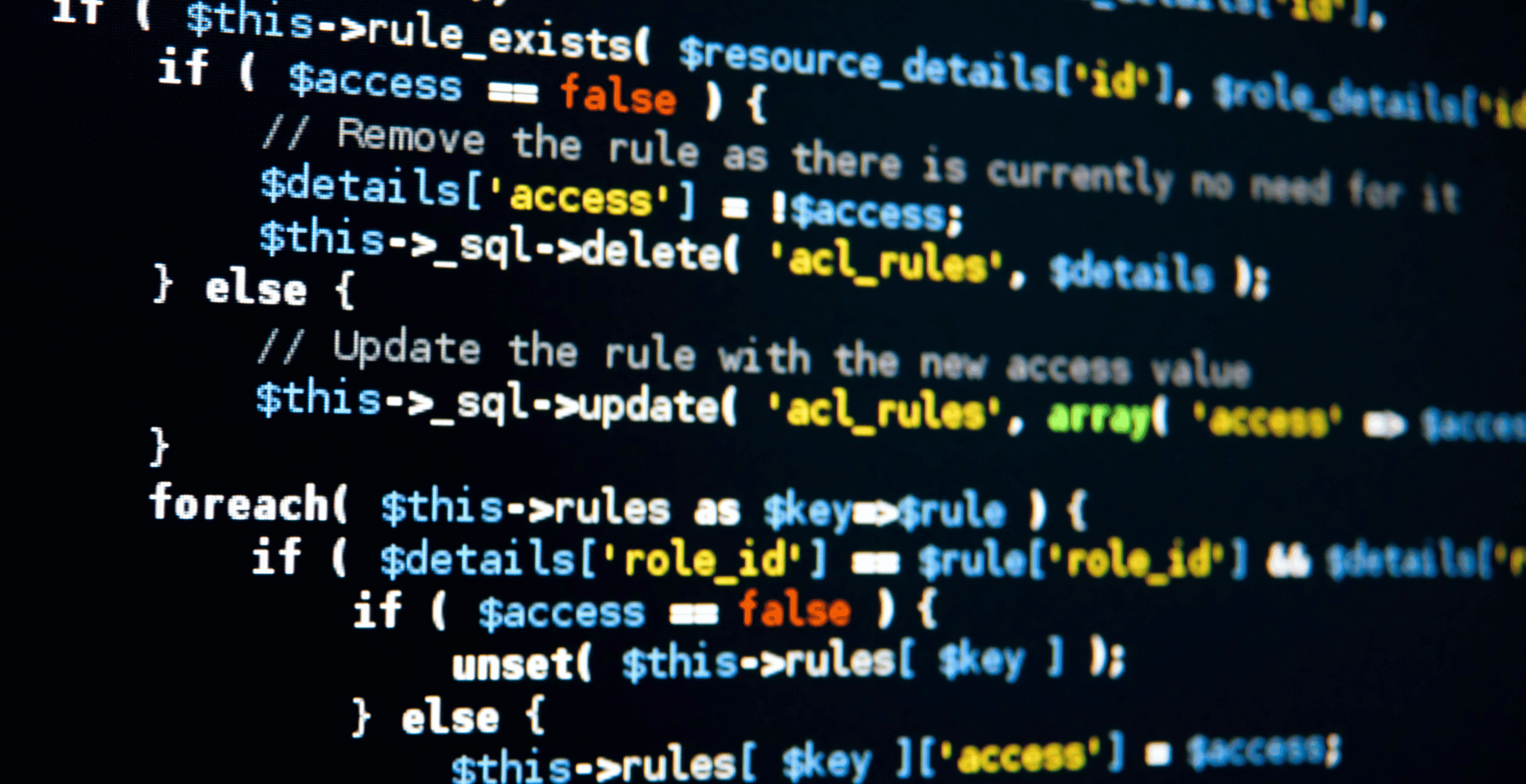
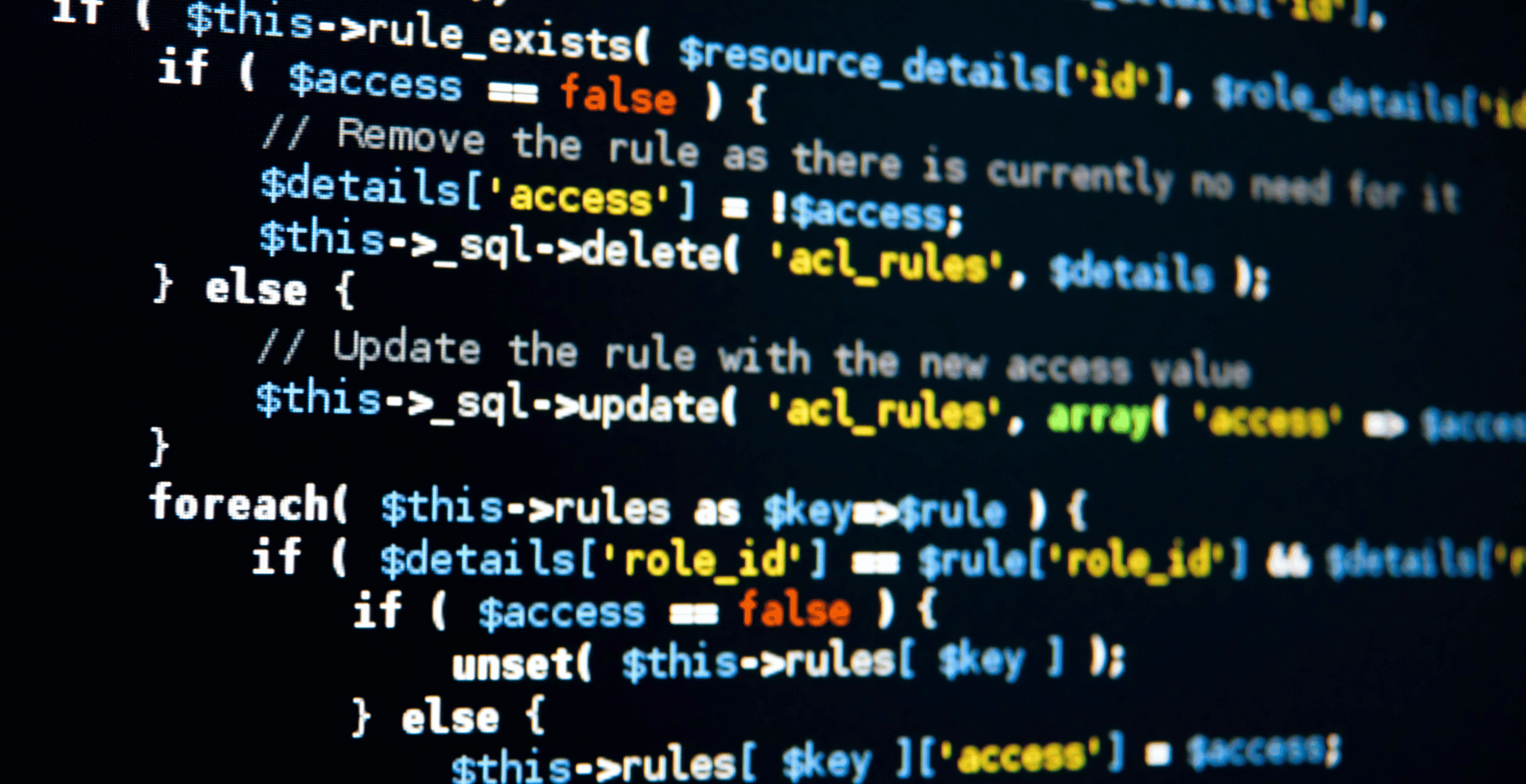
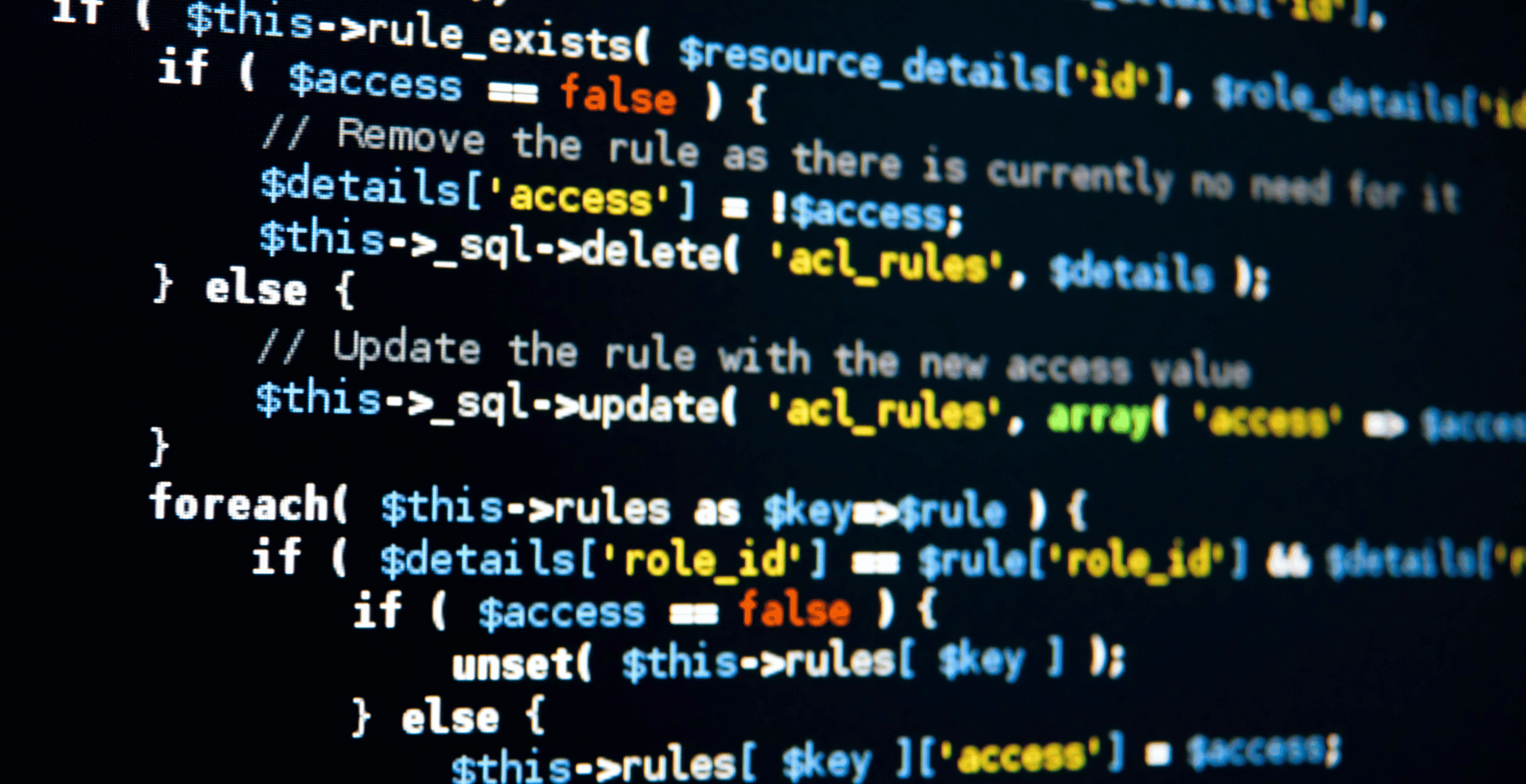
Introduction to What is LLM Agents and How They Work?

Rehan Asif
Jul 24, 2024
Read the article




Analysis of the Large Language Model Landscape Evolution

Rehan Asif
Jul 24, 2024
Read the article




Marketing Success With Retrieval Augmented Generation (RAG) Platforms
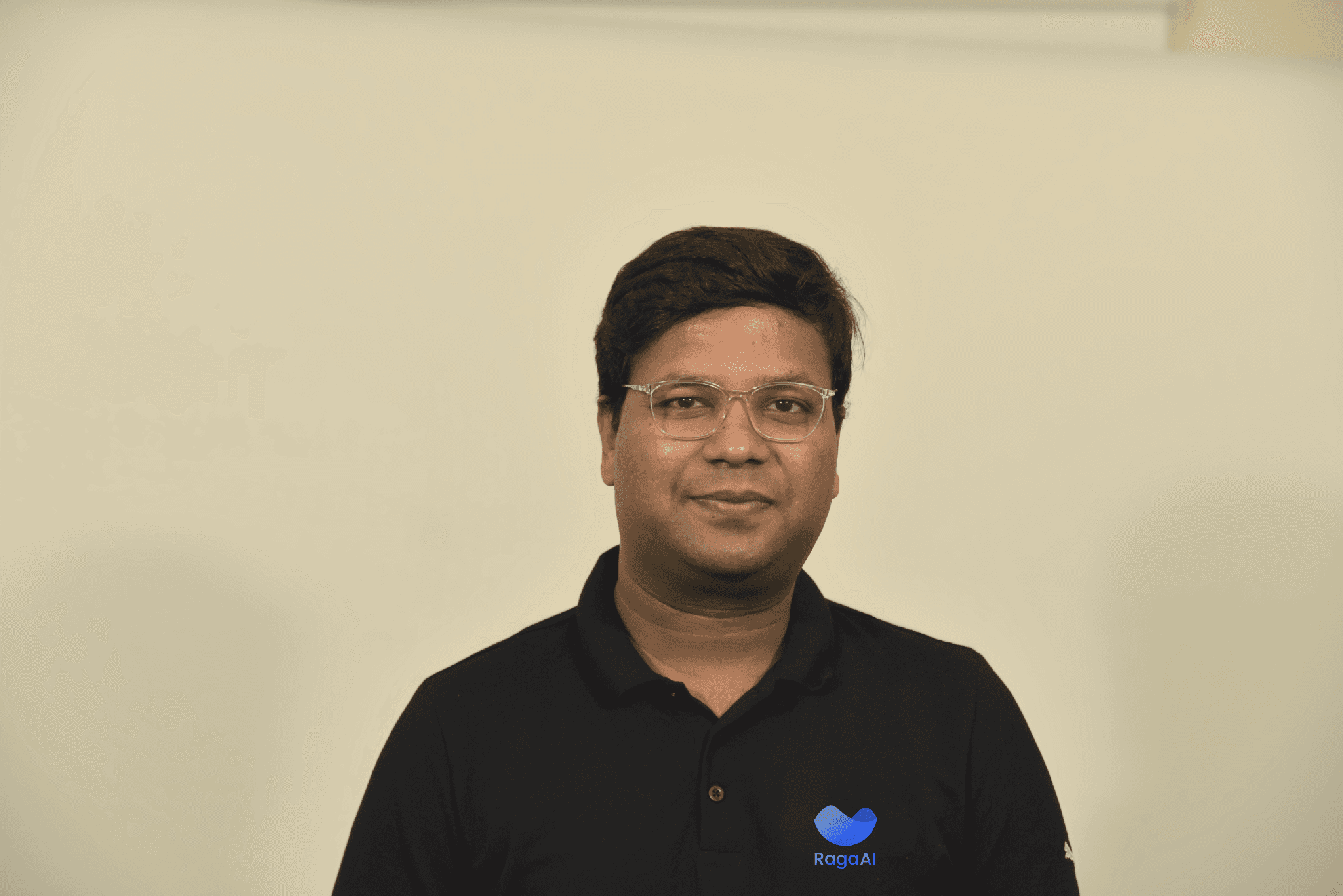
Jigar Gupta
Jul 24, 2024
Read the article




Developing AI Agent Strategies Using GPT
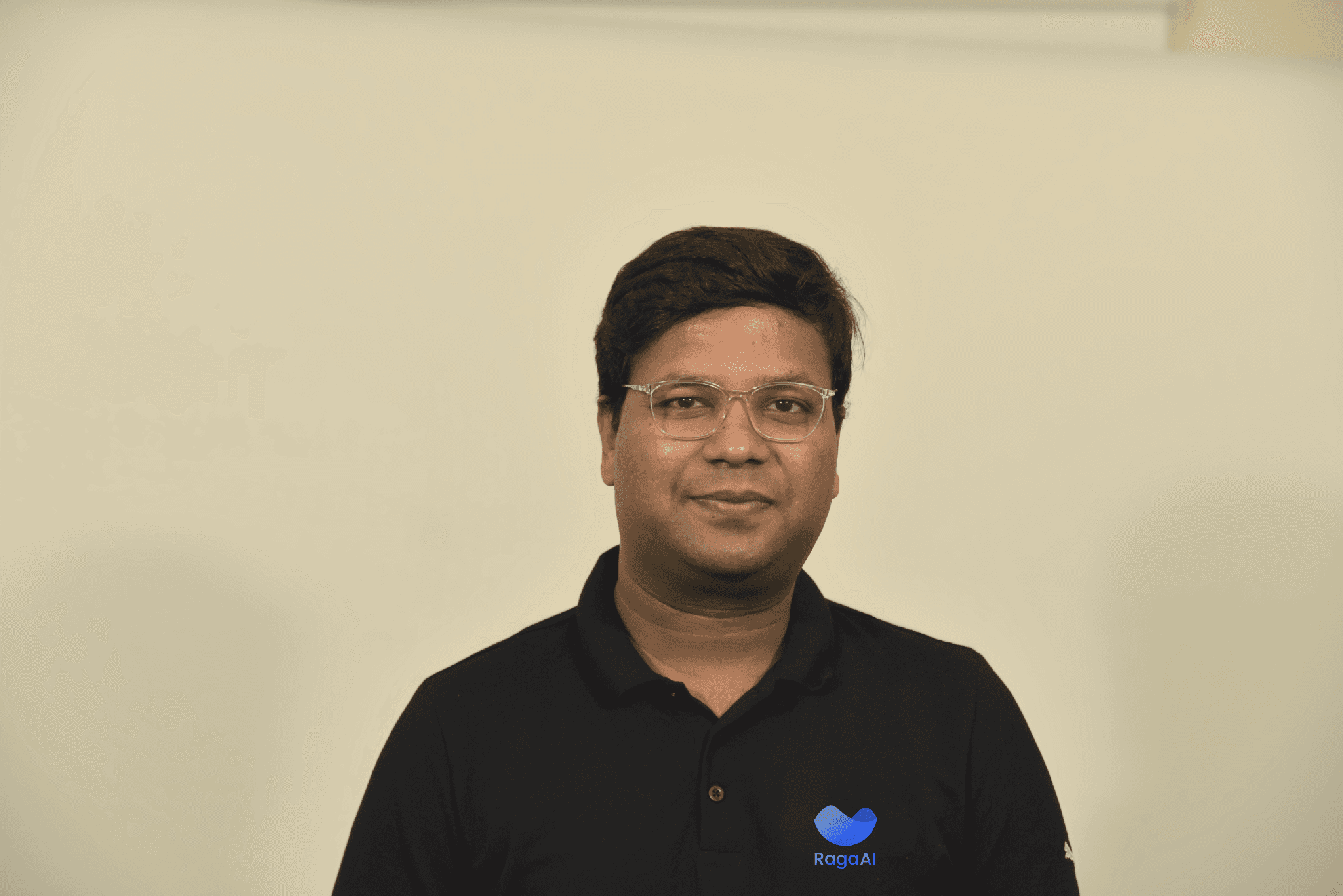
Jigar Gupta
Jul 24, 2024
Read the article




Identifying Triggers for Retraining AI Models to Maintain Performance
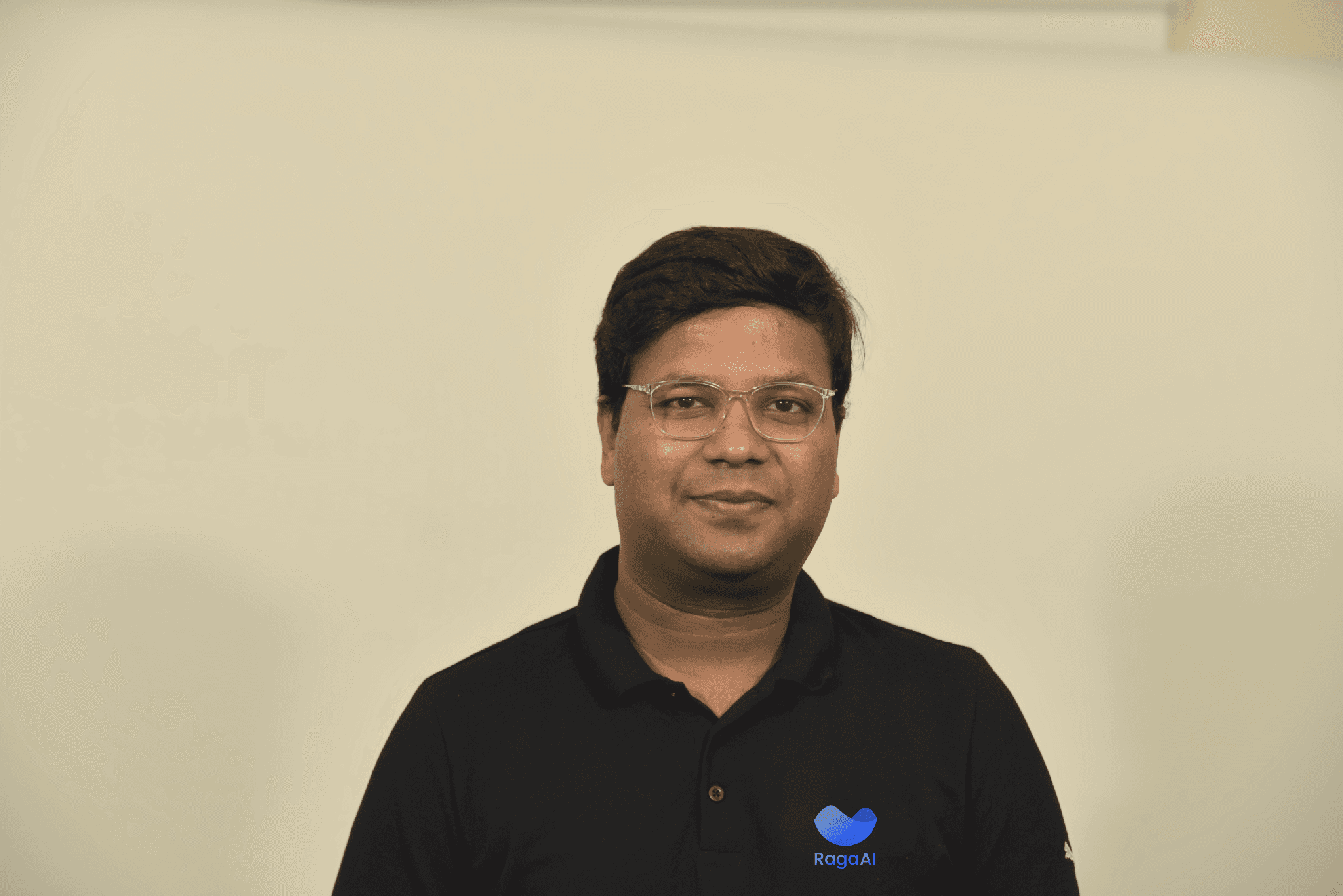
Jigar Gupta
Jul 16, 2024
Read the article
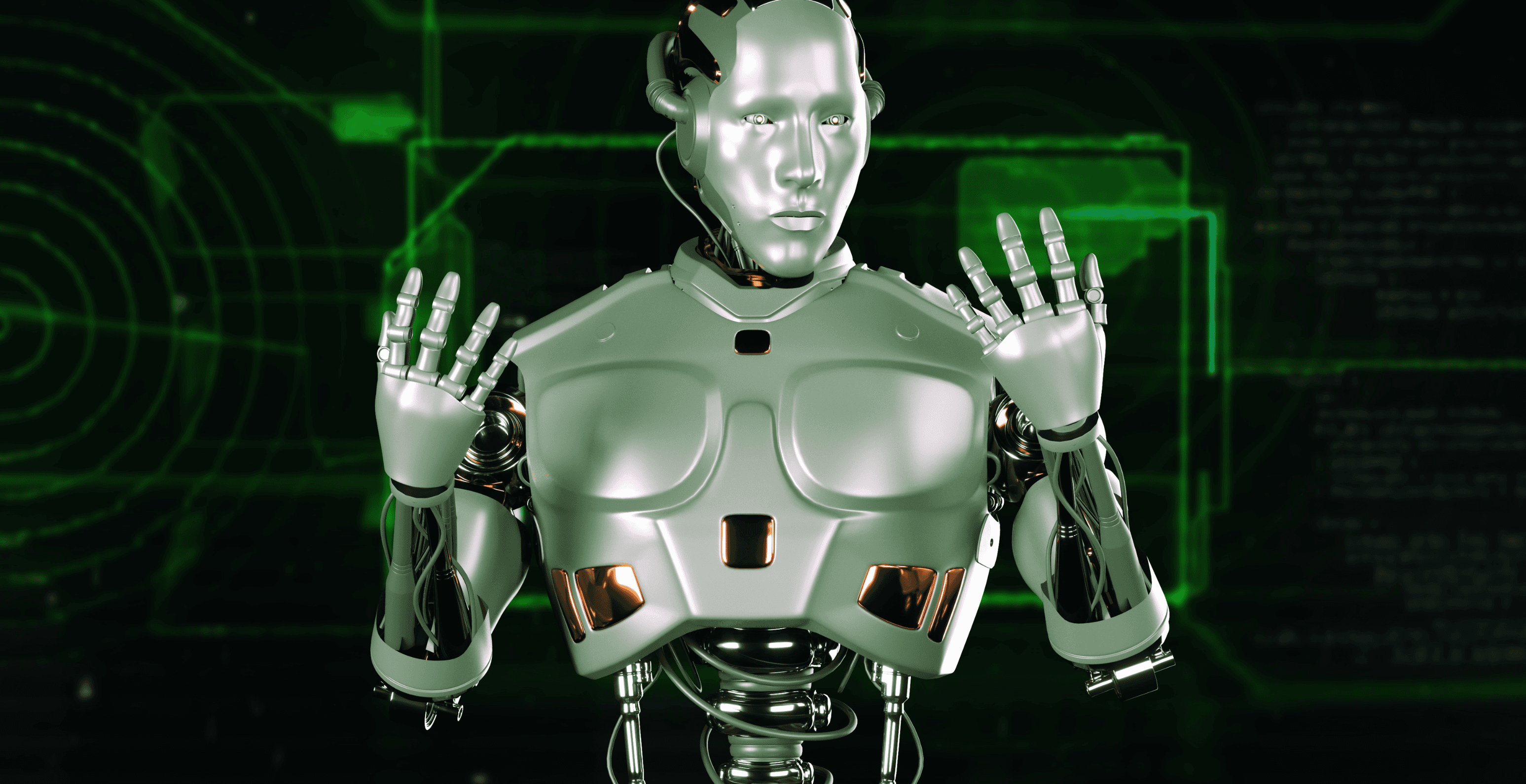
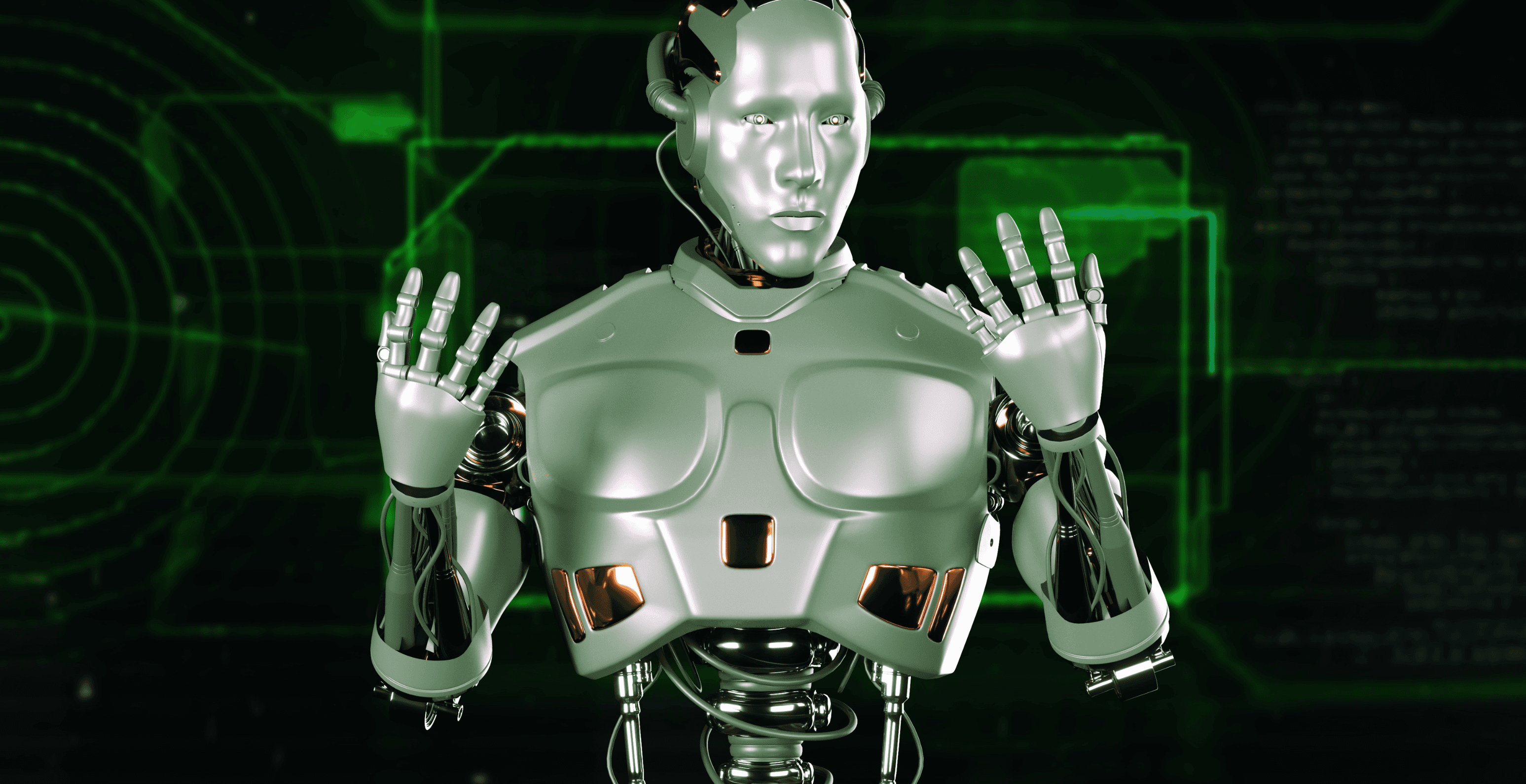
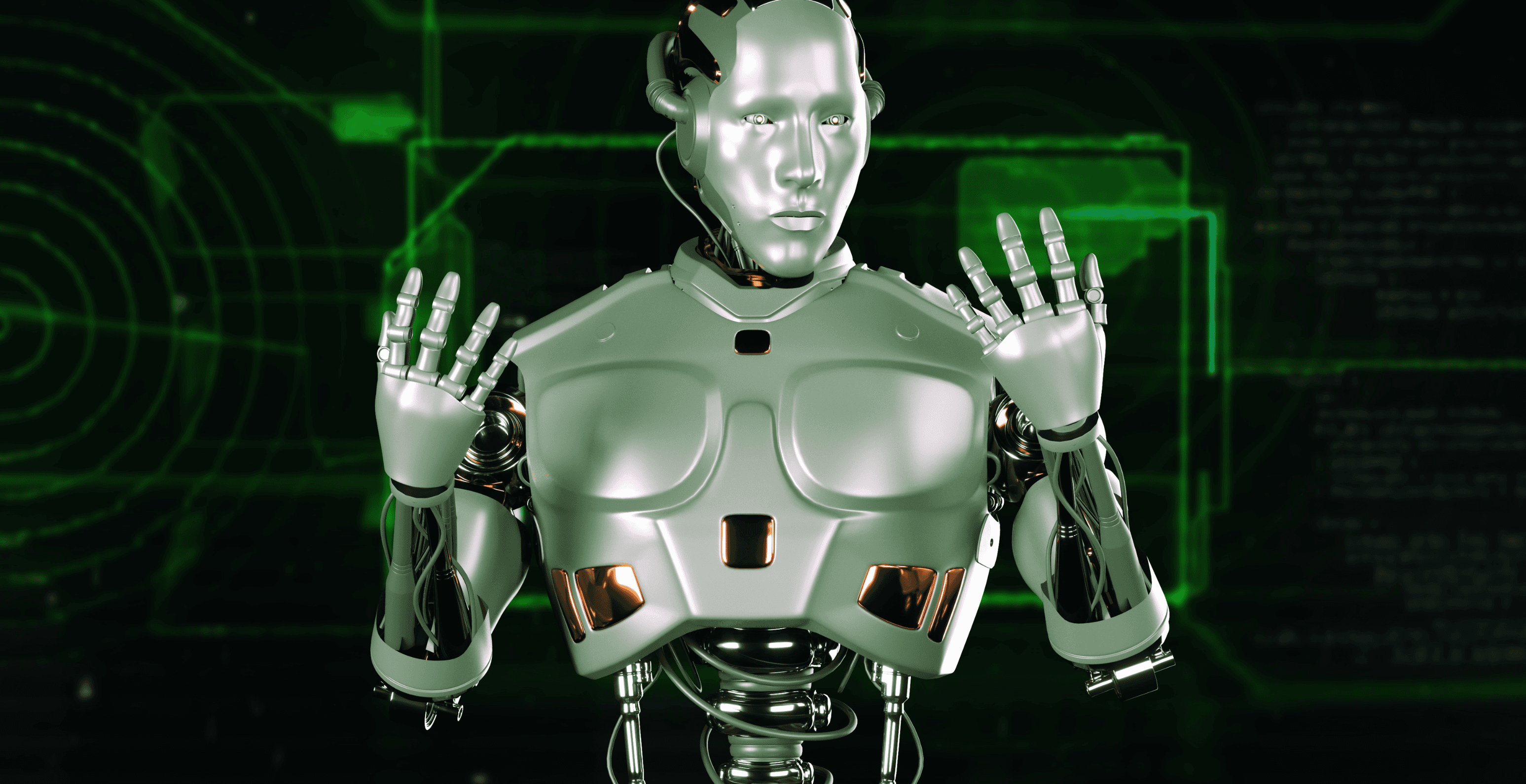
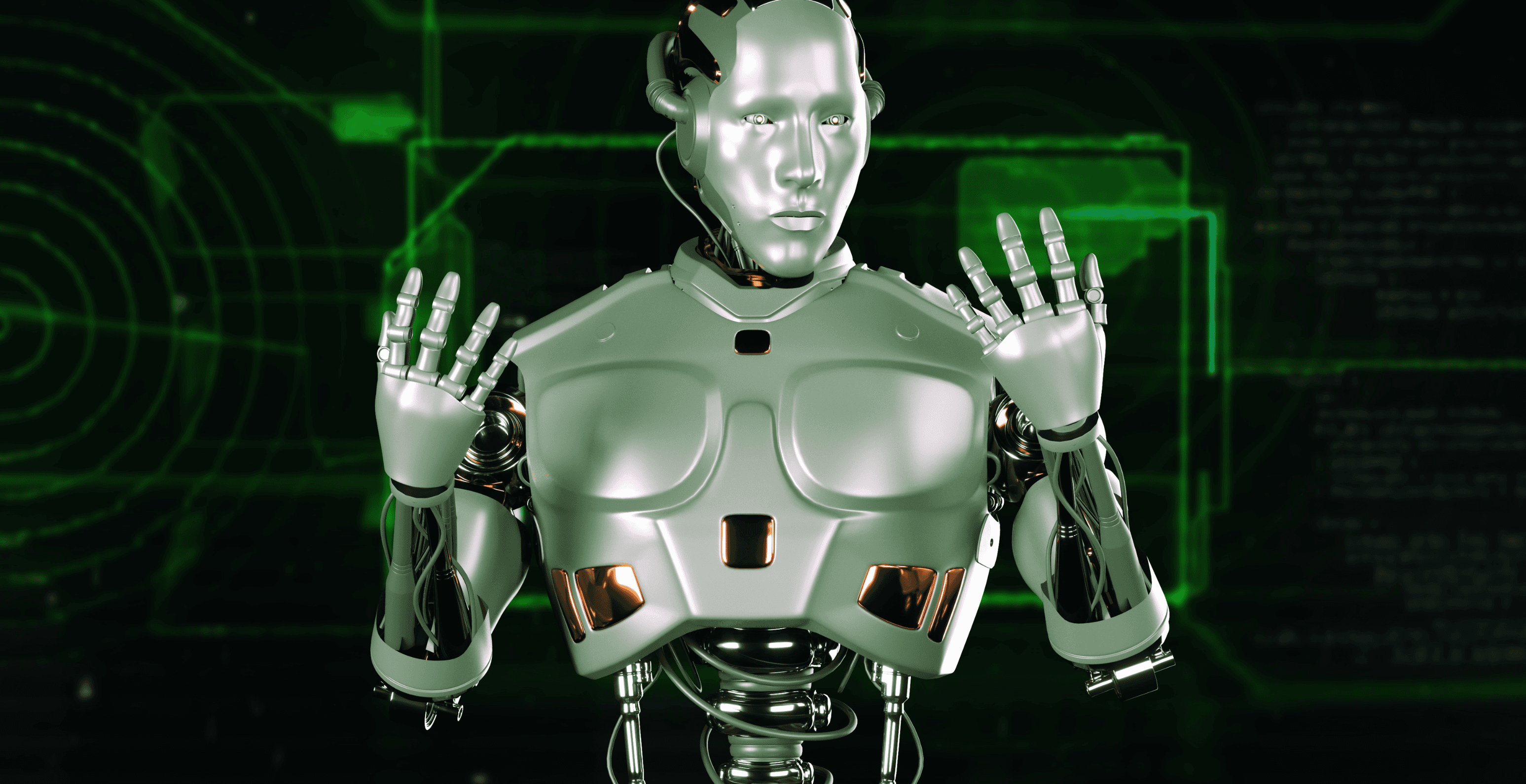
Agentic Design Patterns In LLM-Based Applications

Rehan Asif
Jul 16, 2024
Read the article
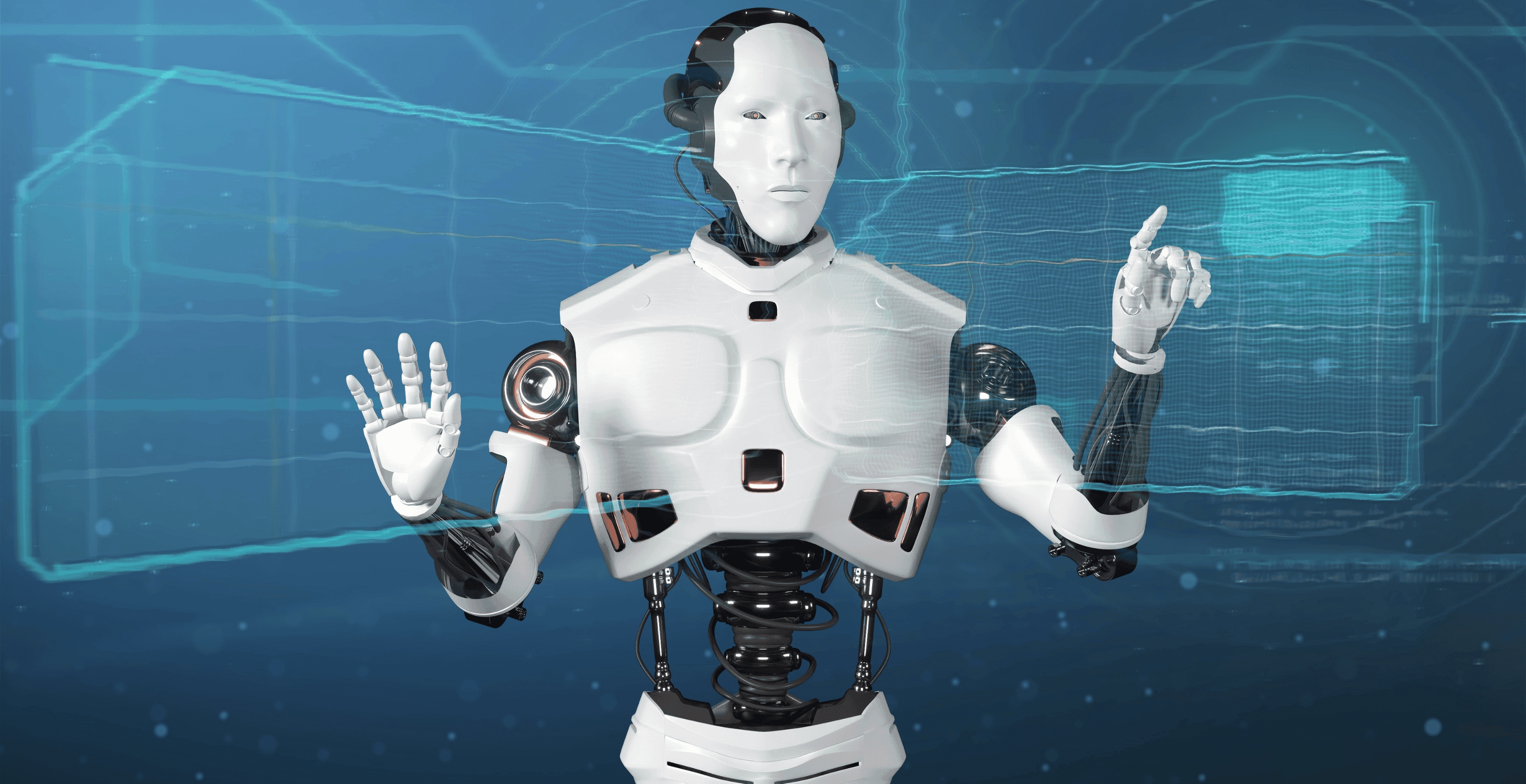
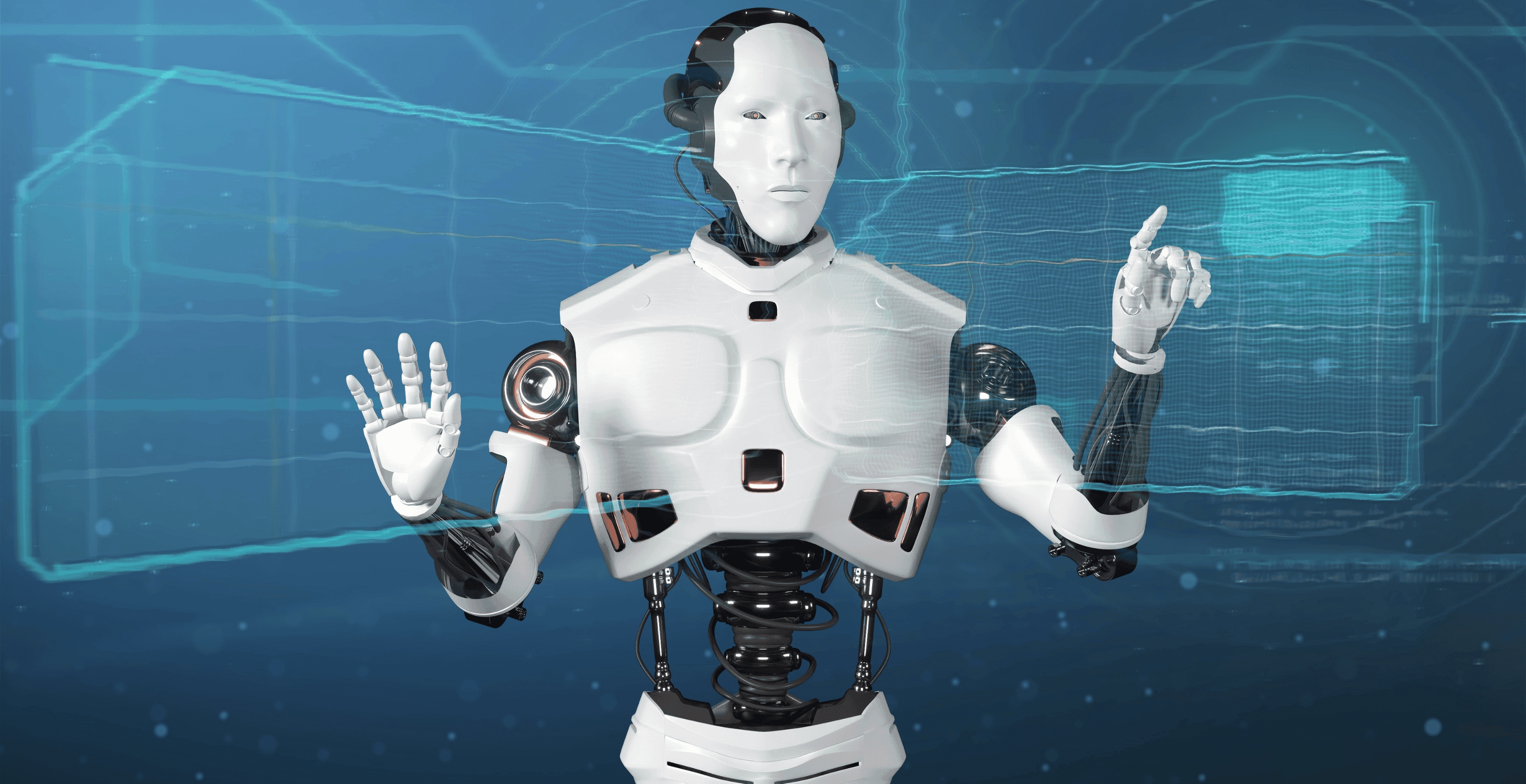
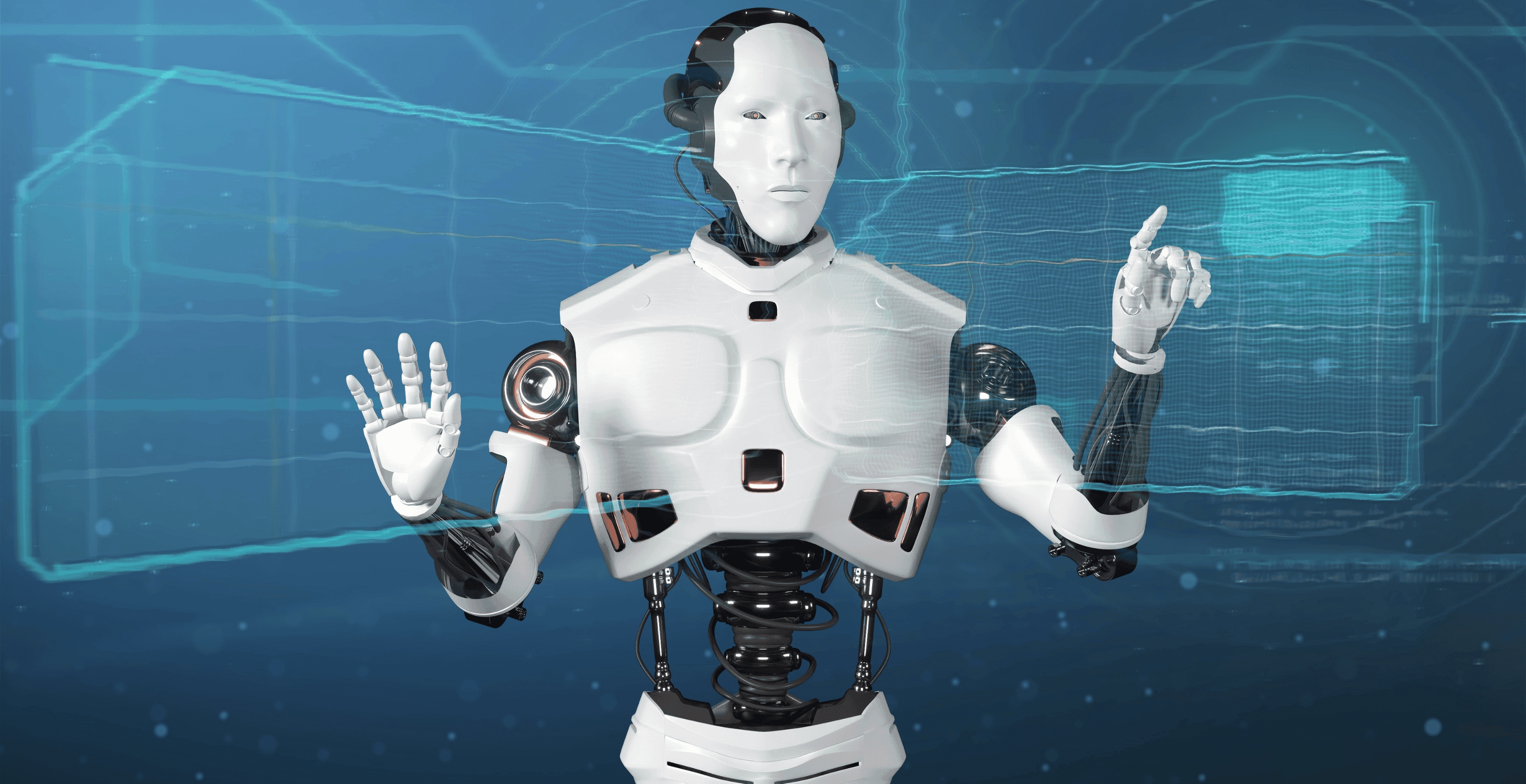
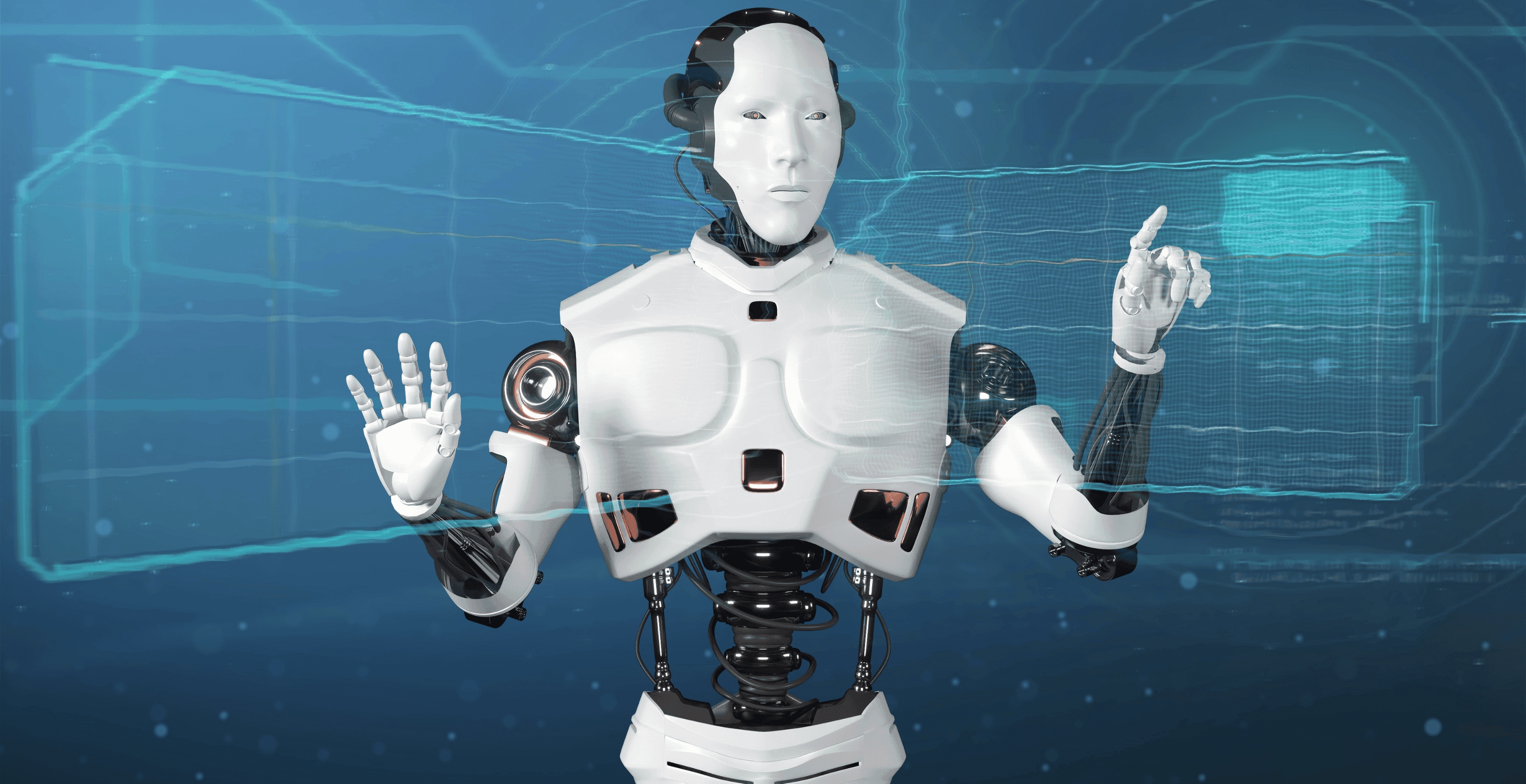
Generative AI And Document Question Answering With LLMs
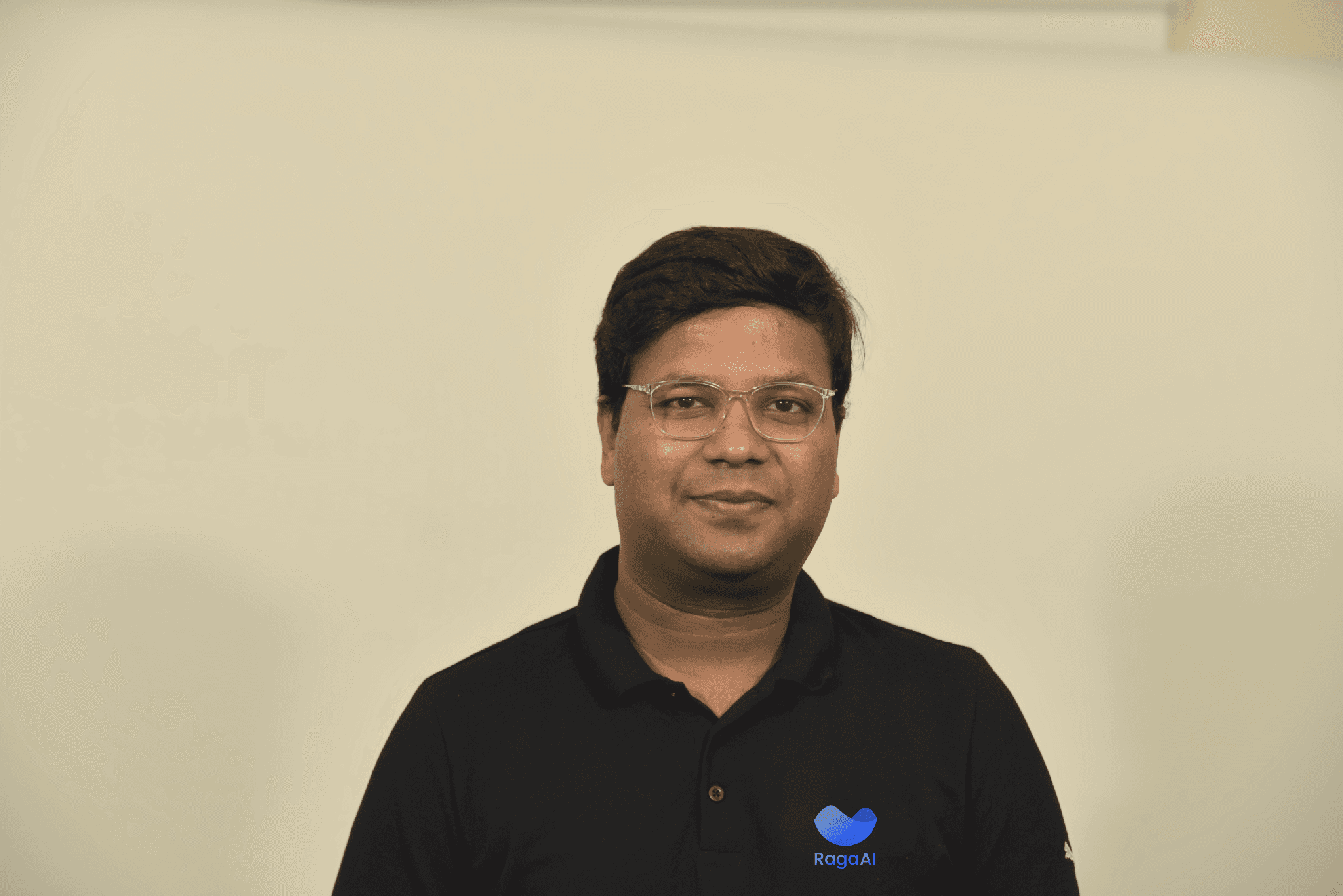
Jigar Gupta
Jul 15, 2024
Read the article




How to Fine-Tune ChatGPT for Your Use Case - Step by Step Guide
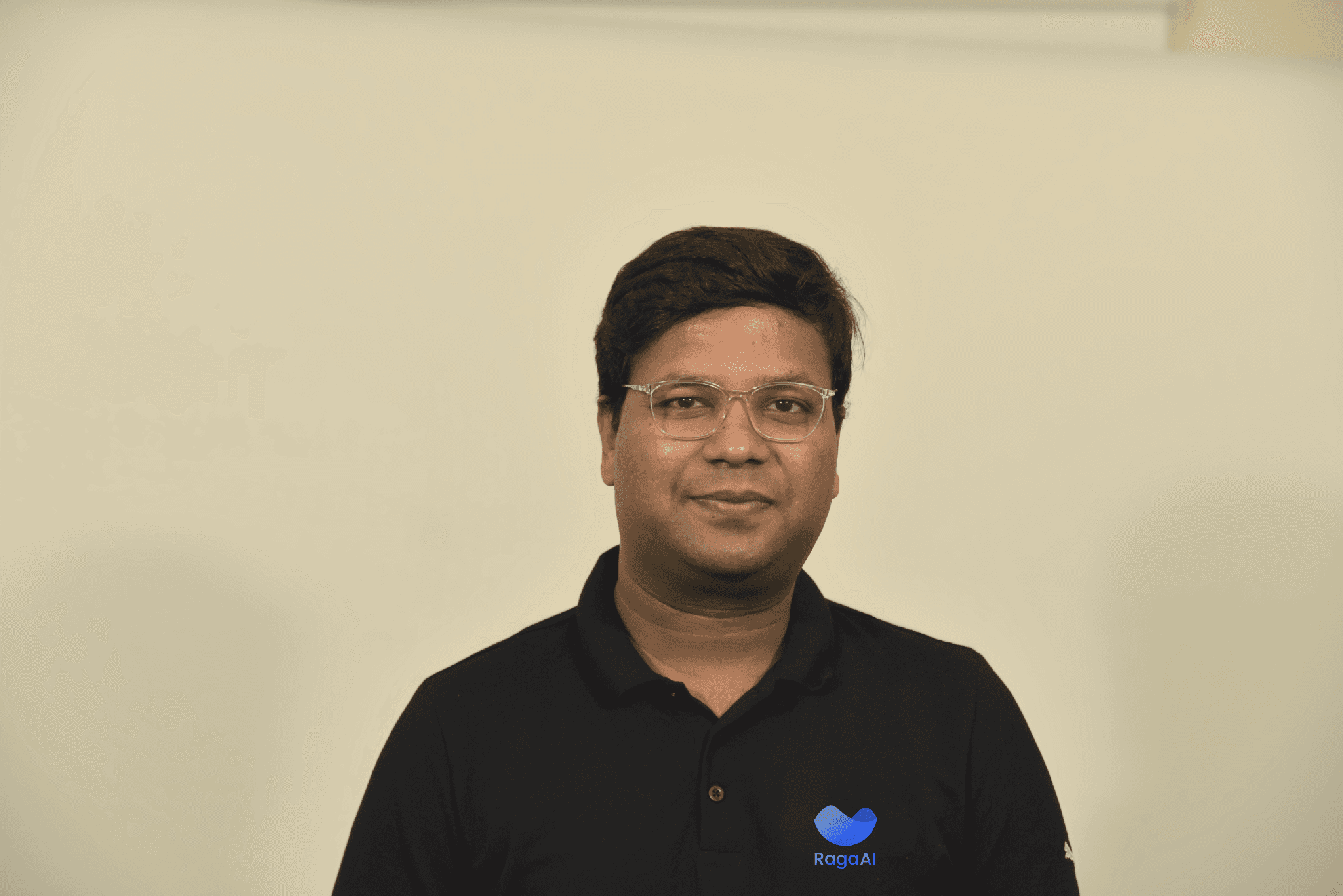
Jigar Gupta
Jul 15, 2024
Read the article




Security and LLM Firewall Controls

Rehan Asif
Jul 15, 2024
Read the article
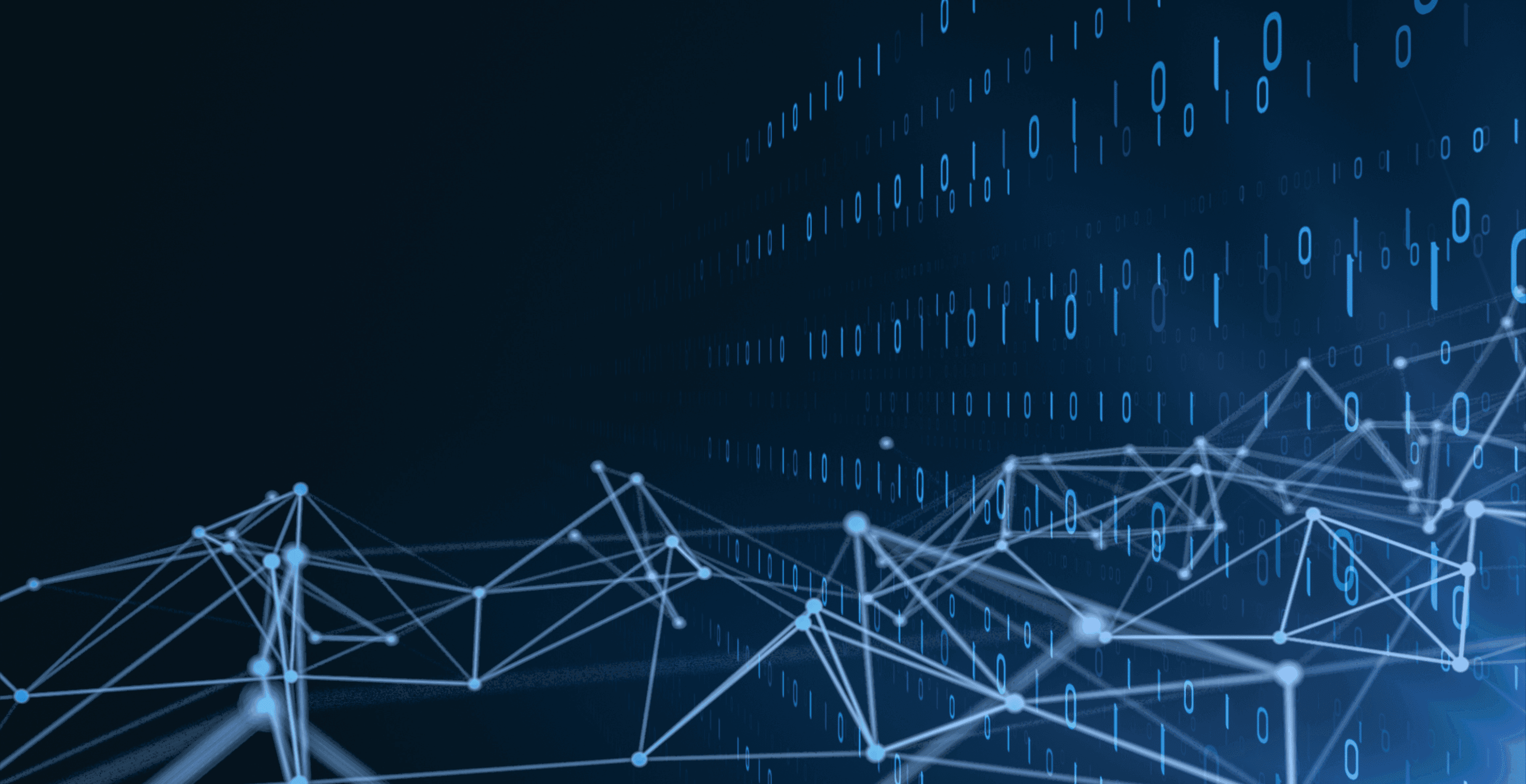
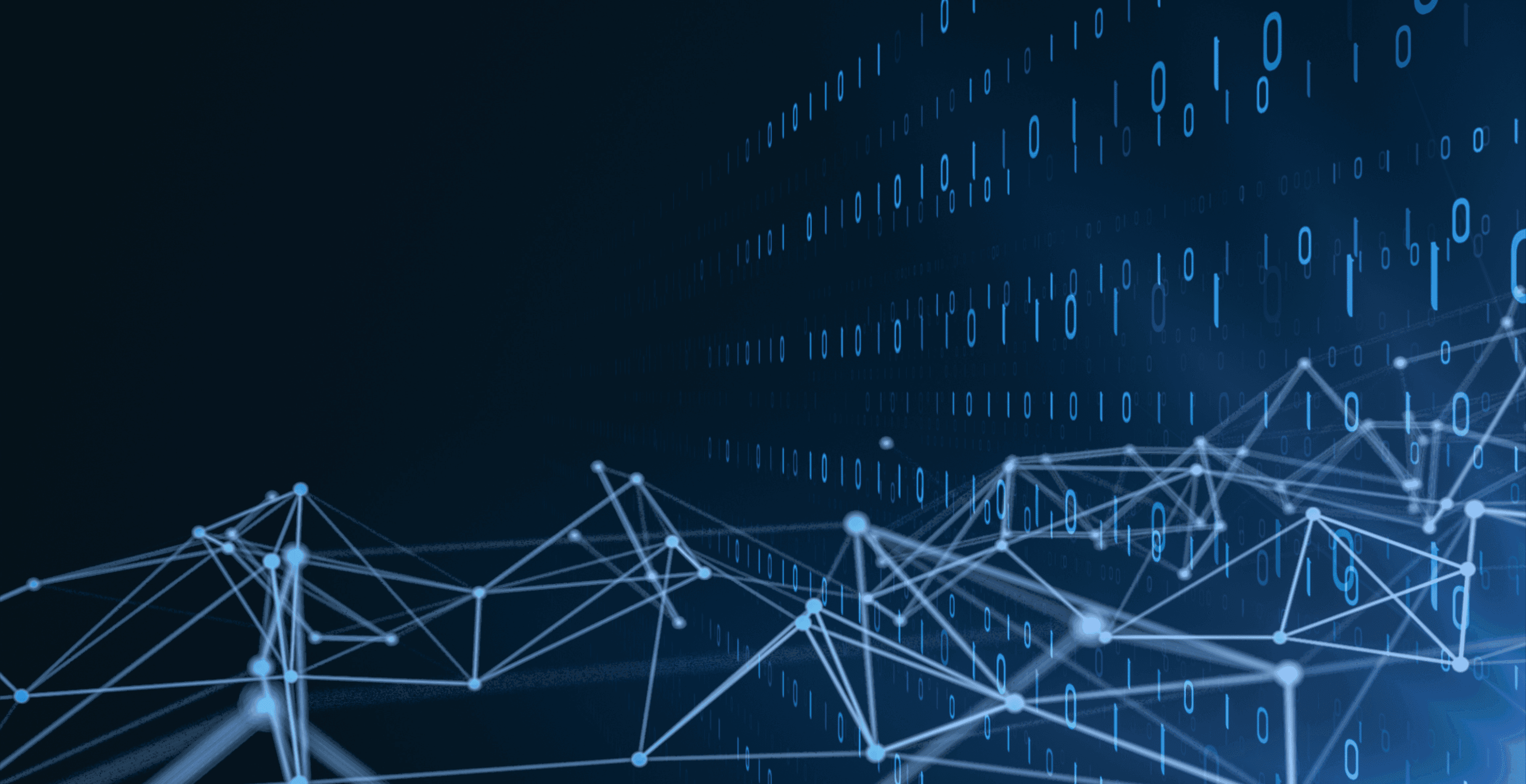
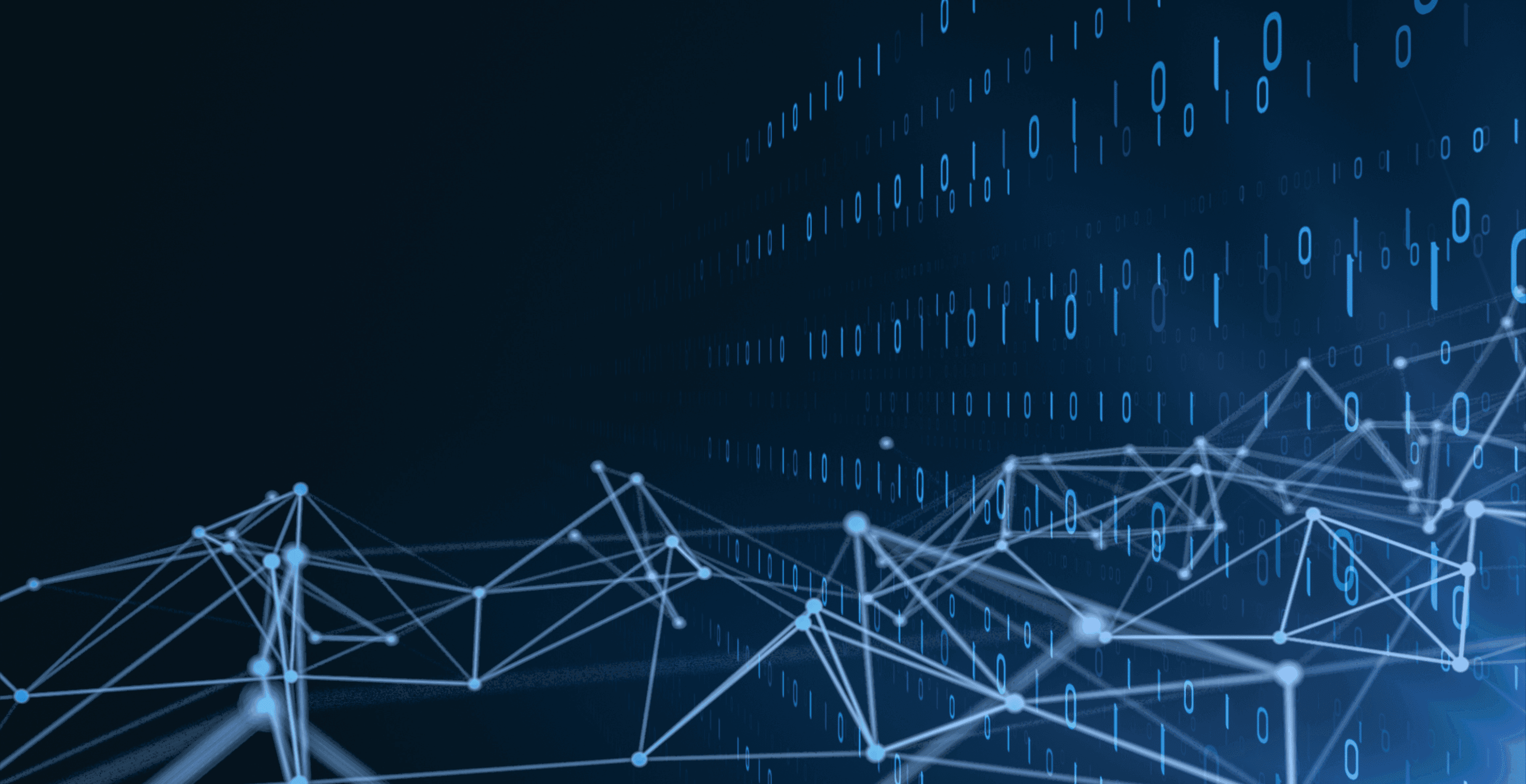
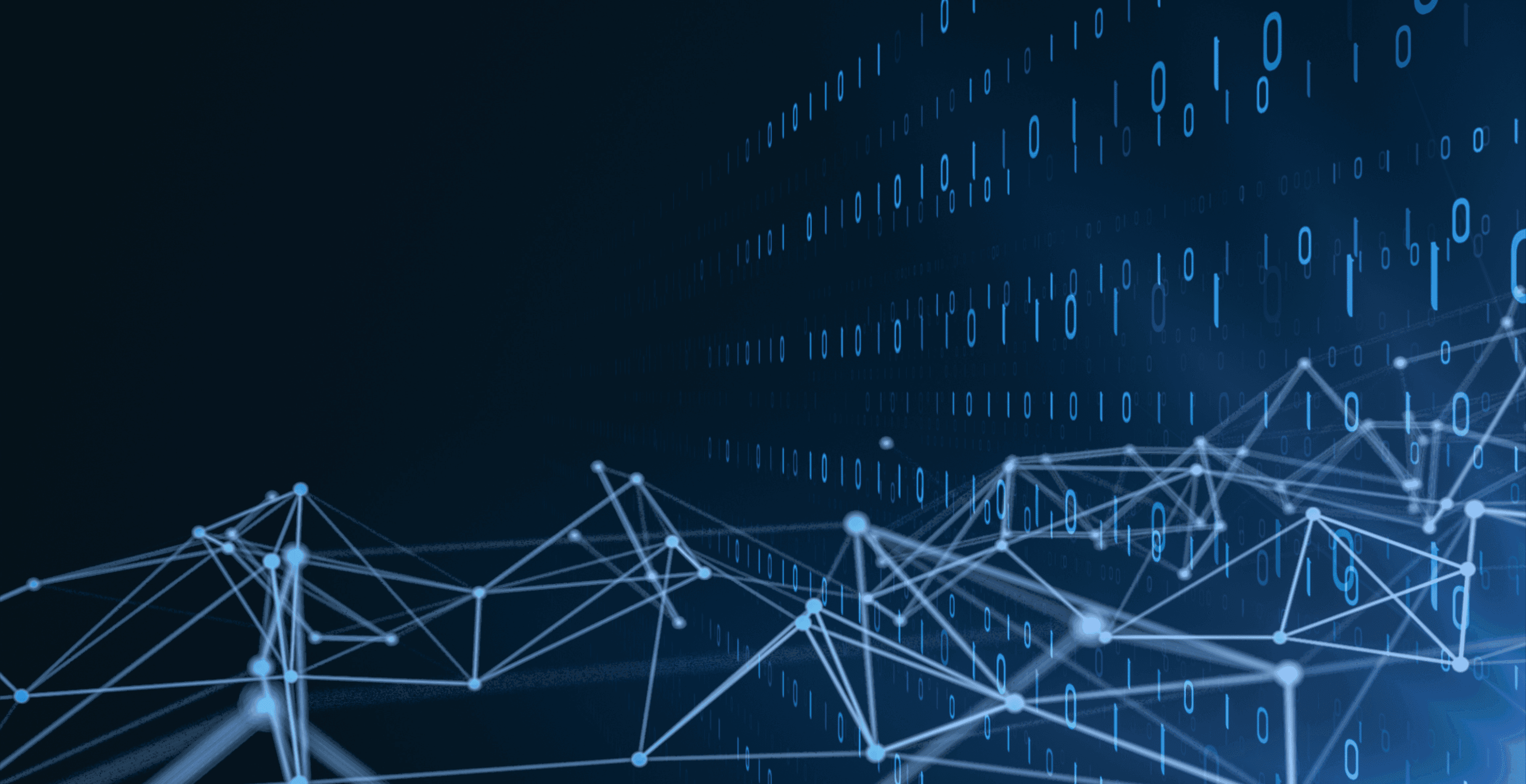
Understanding the Use of Guardrail Metrics in Ensuring LLM Safety

Rehan Asif
Jul 13, 2024
Read the article
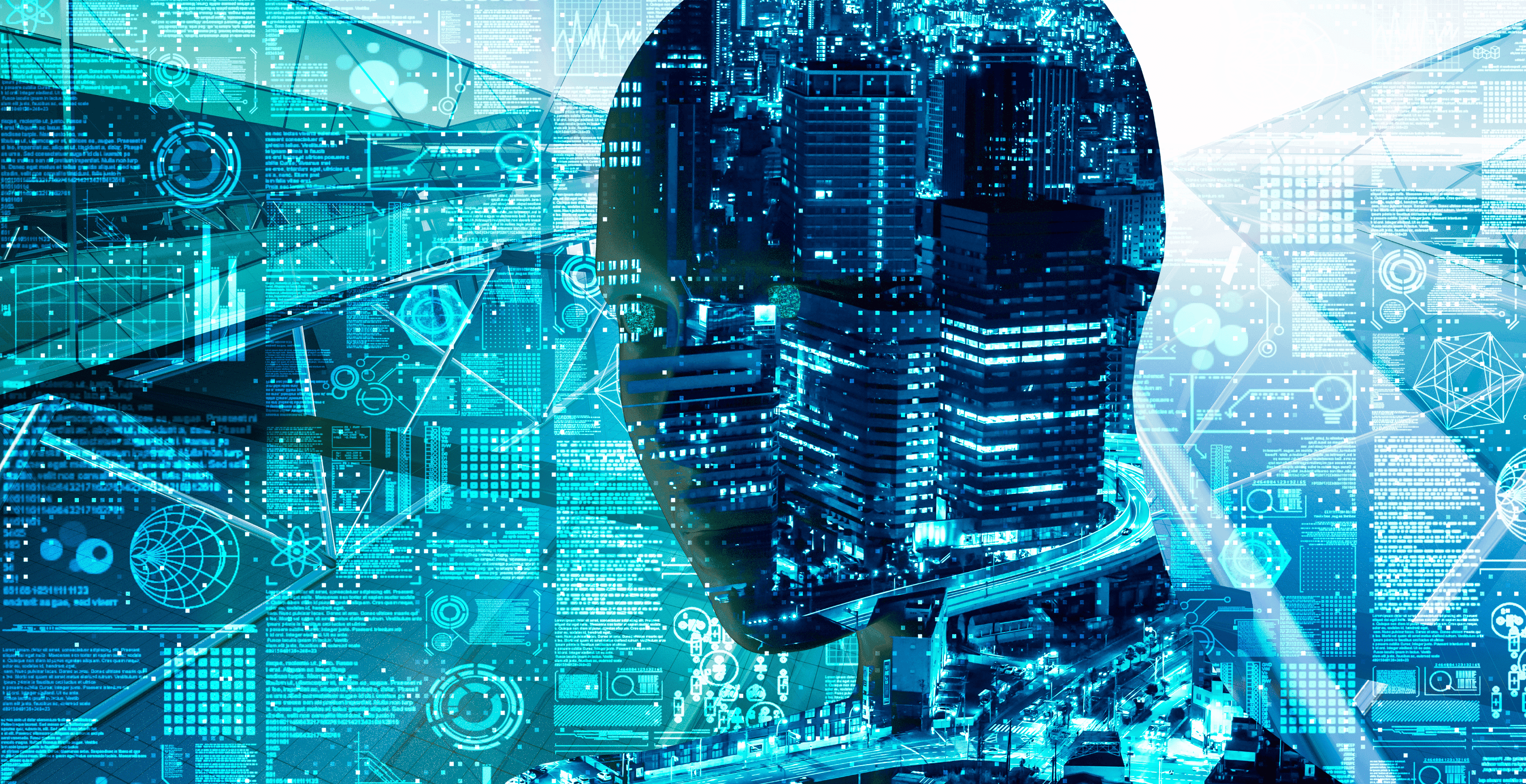
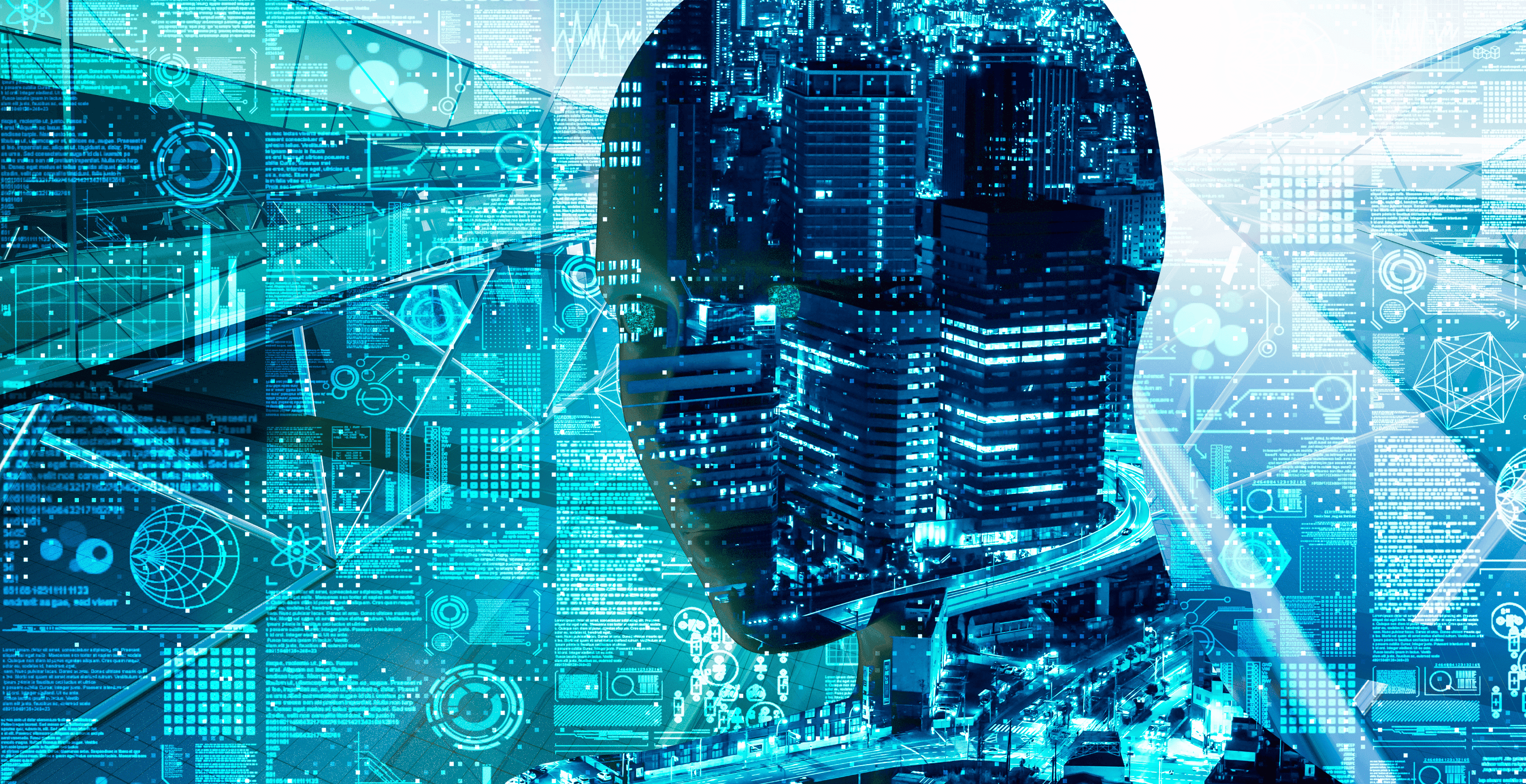
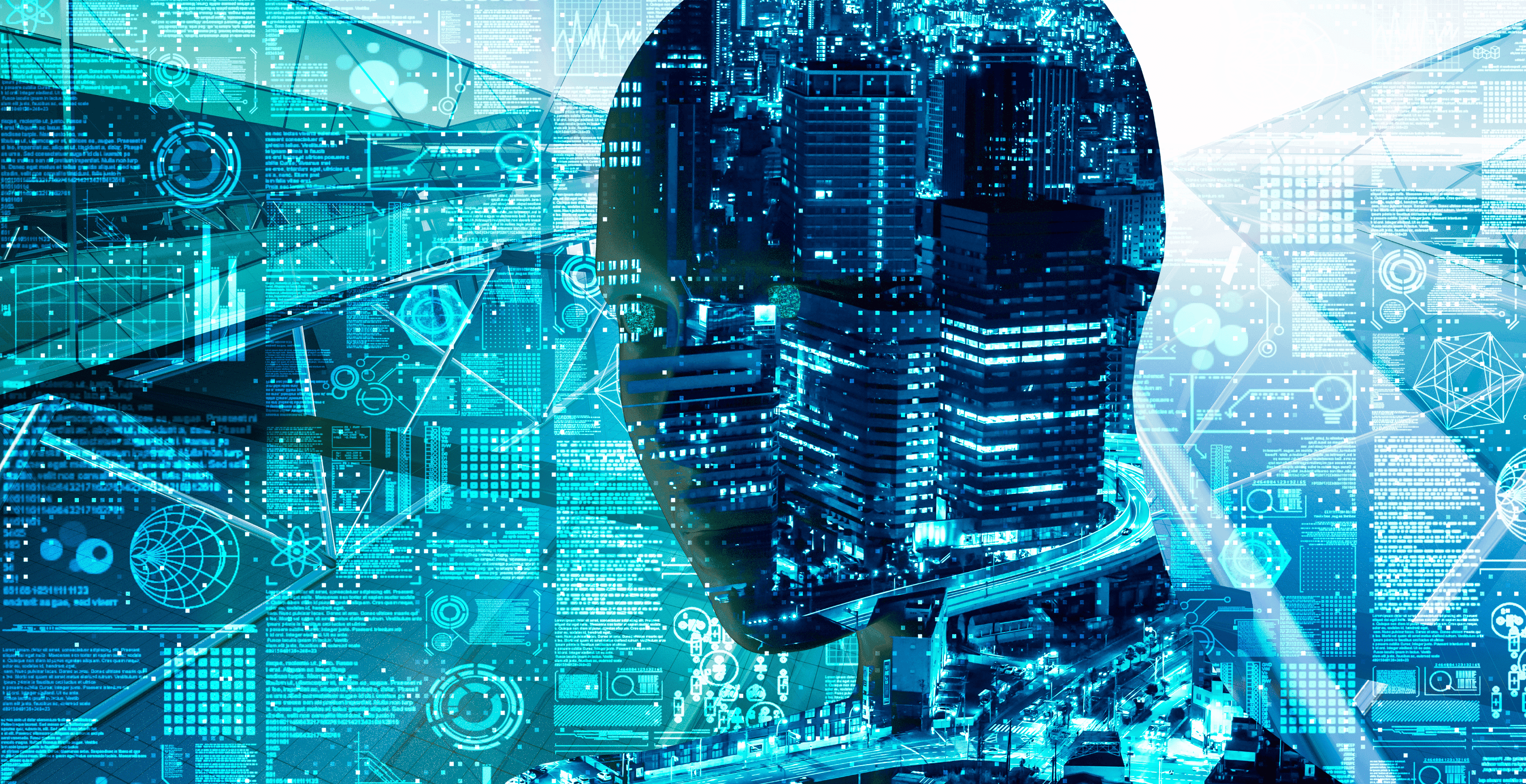
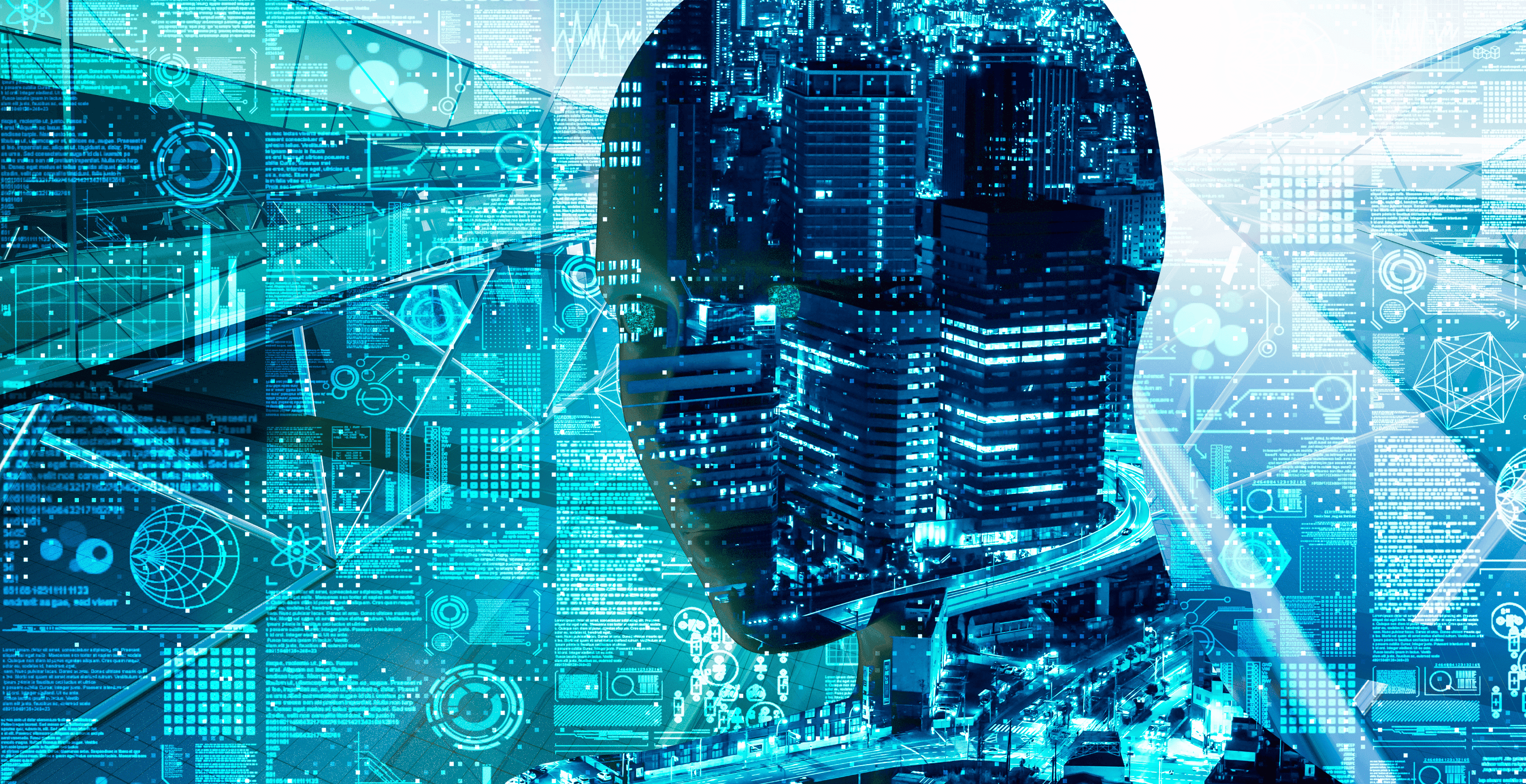
Exploring the Future of LLM and Generative AI Infrastructure

Rehan Asif
Jul 13, 2024
Read the article




Comprehensive Guide to RLHF and Fine Tuning LLMs from Scratch

Rehan Asif
Jul 13, 2024
Read the article
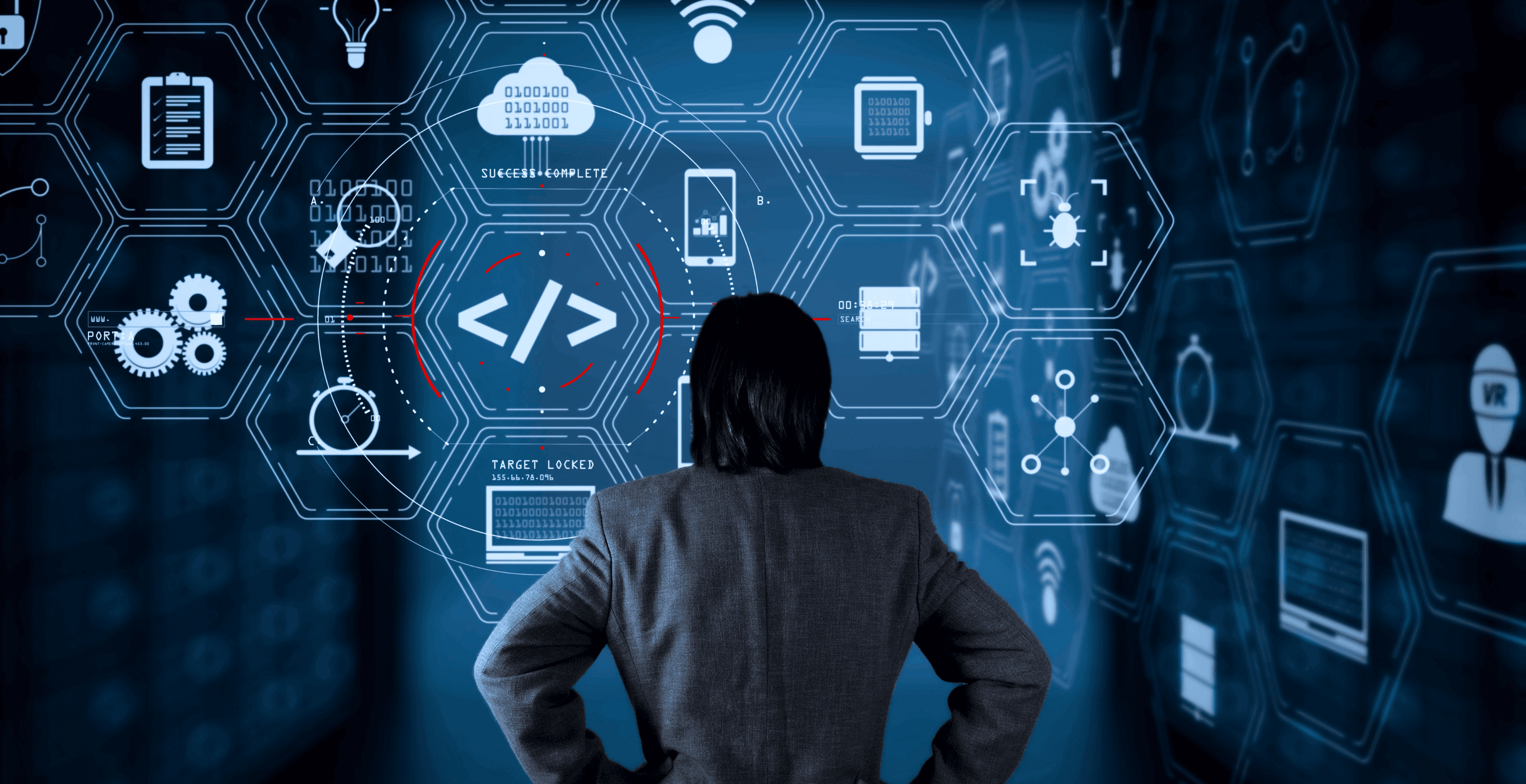
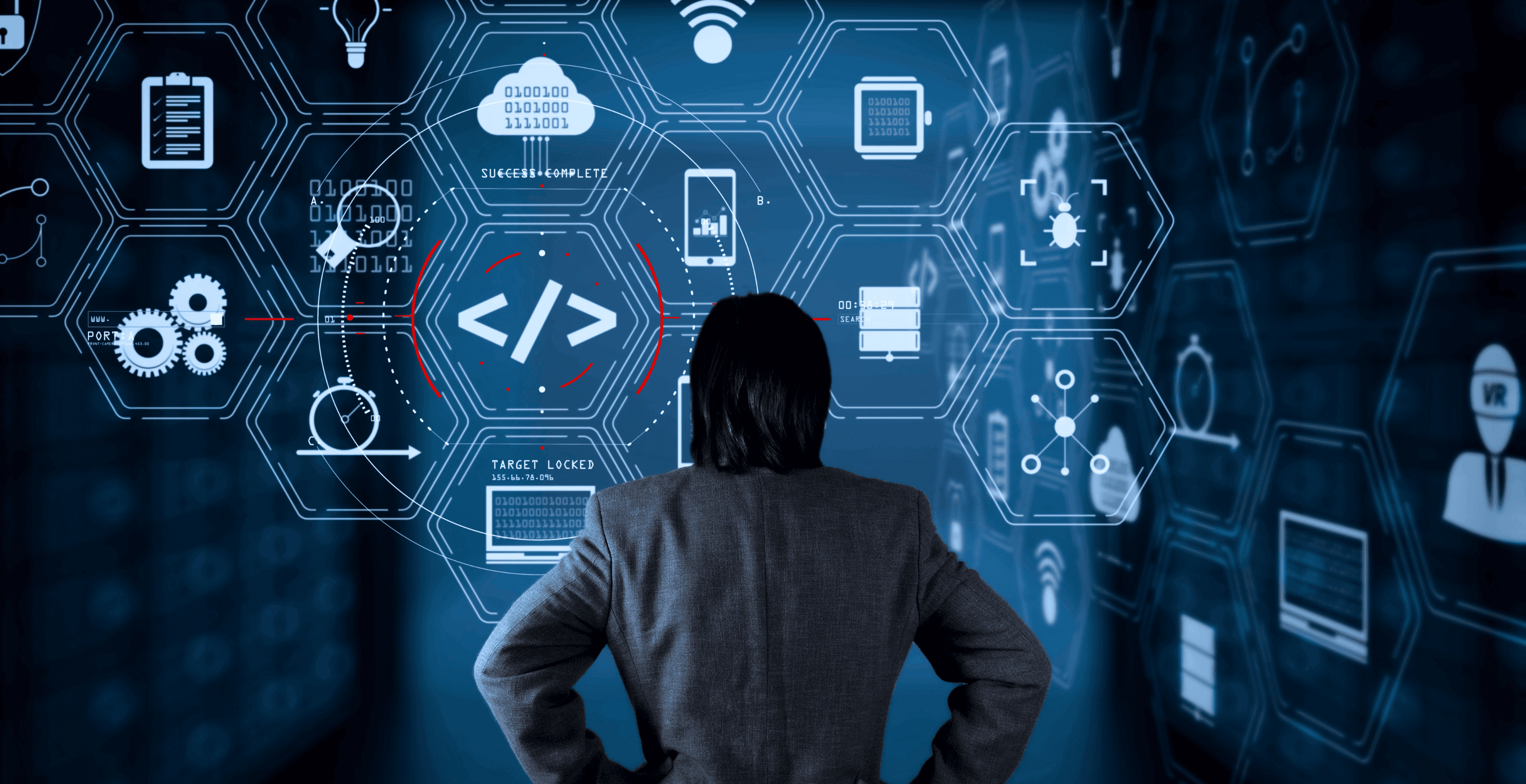
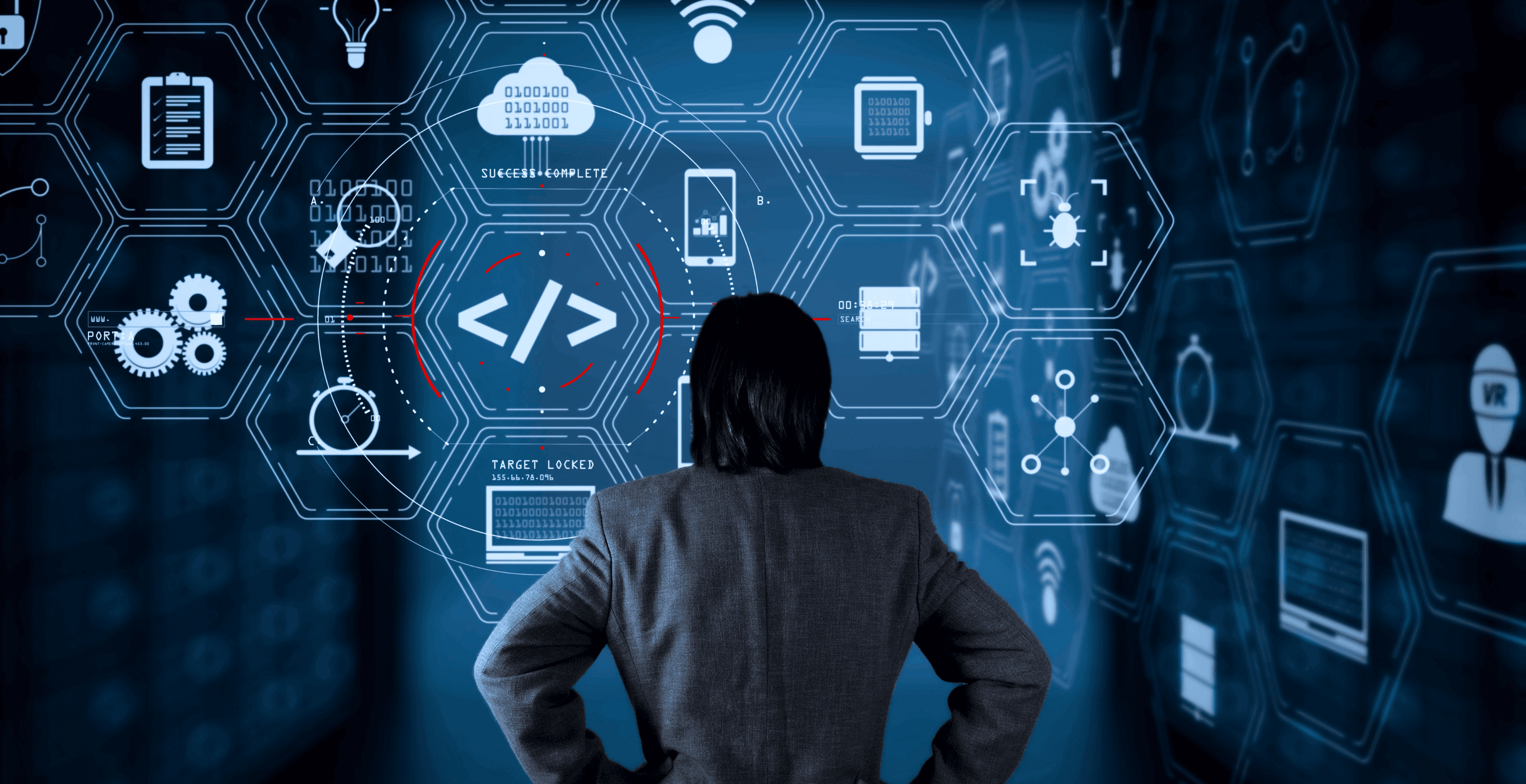
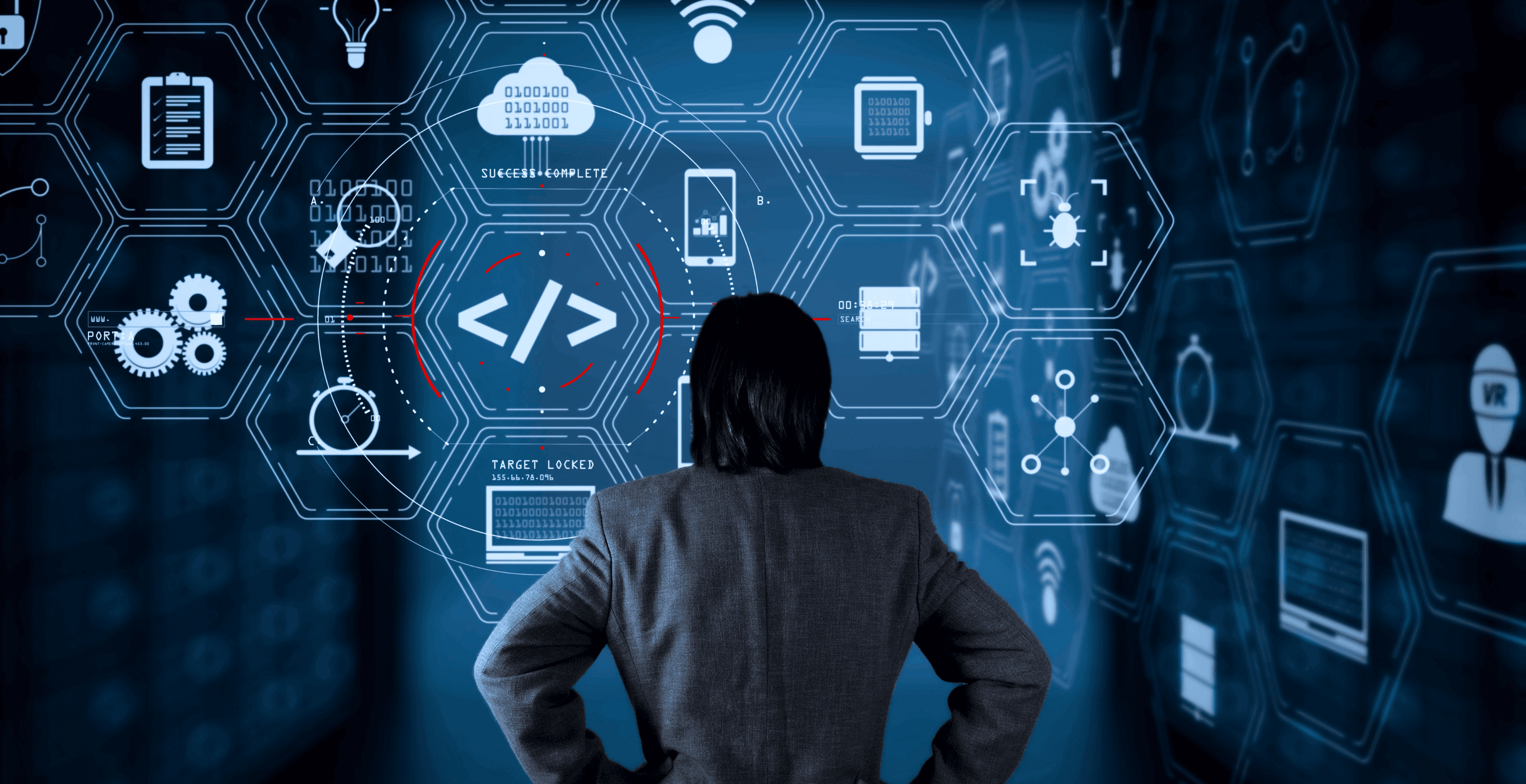
Using Synthetic Data To Enrich RAG Applications
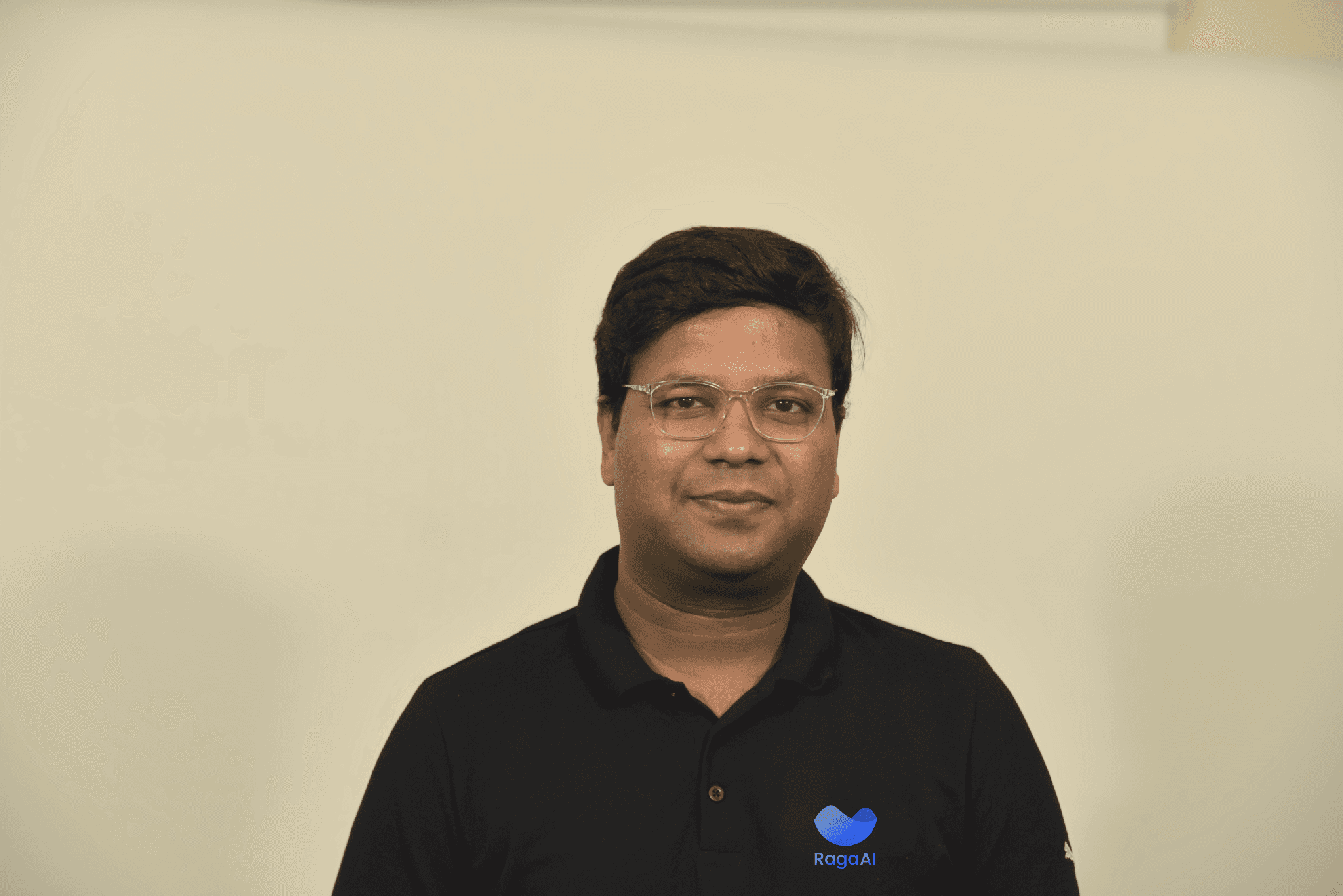
Jigar Gupta
Jul 13, 2024
Read the article




Comparing Different Large Language Model (LLM) Frameworks

Rehan Asif
Jul 12, 2024
Read the article
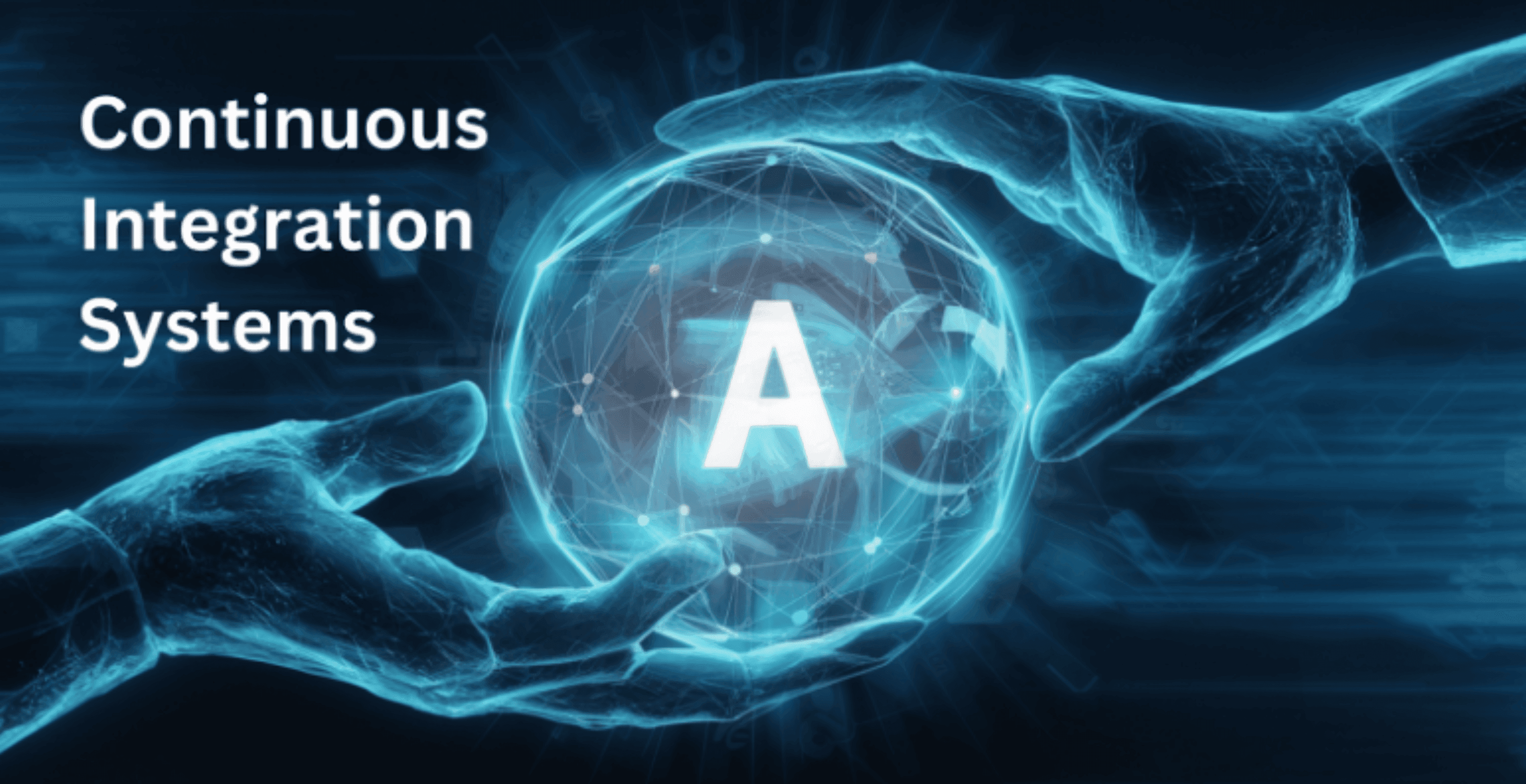
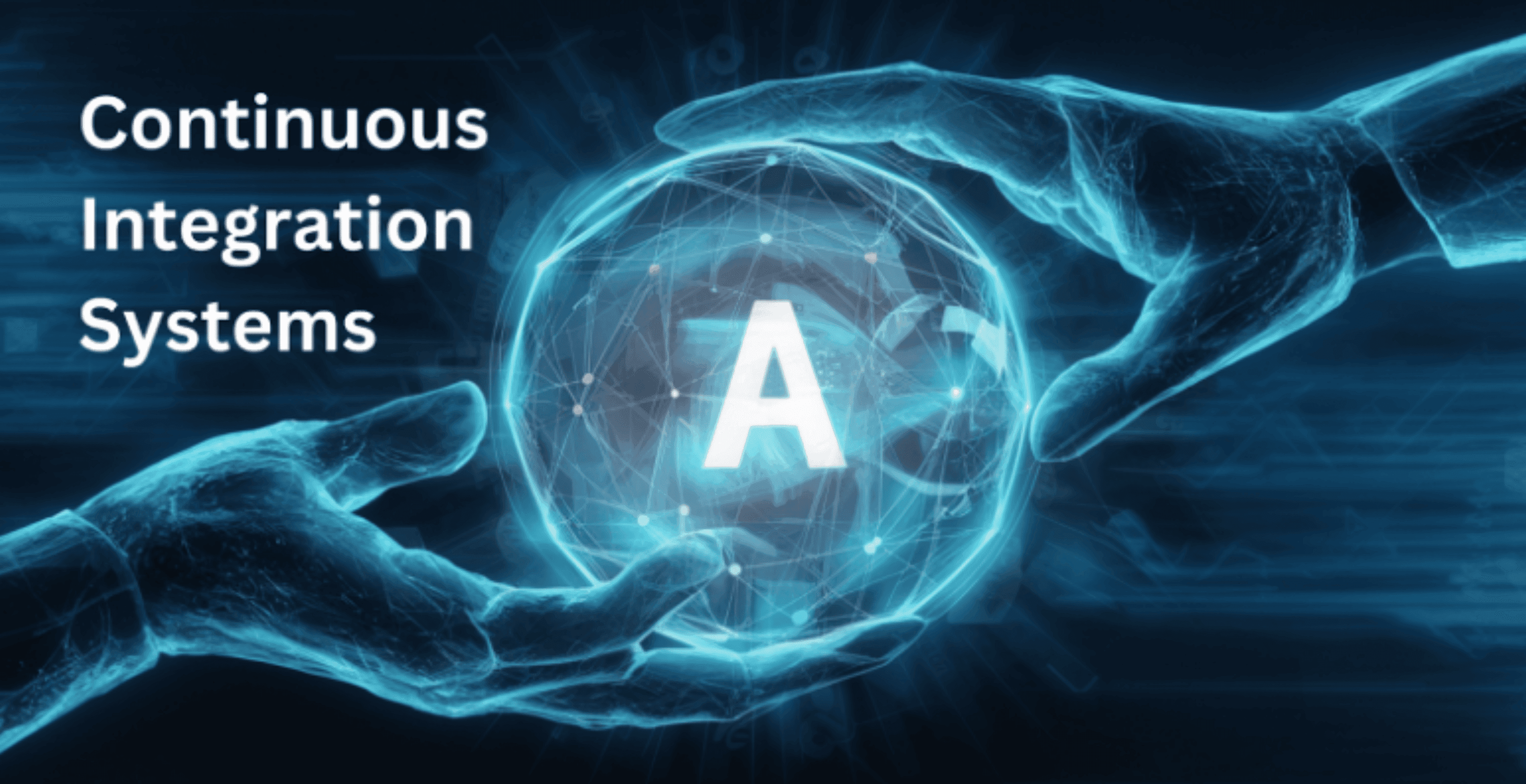
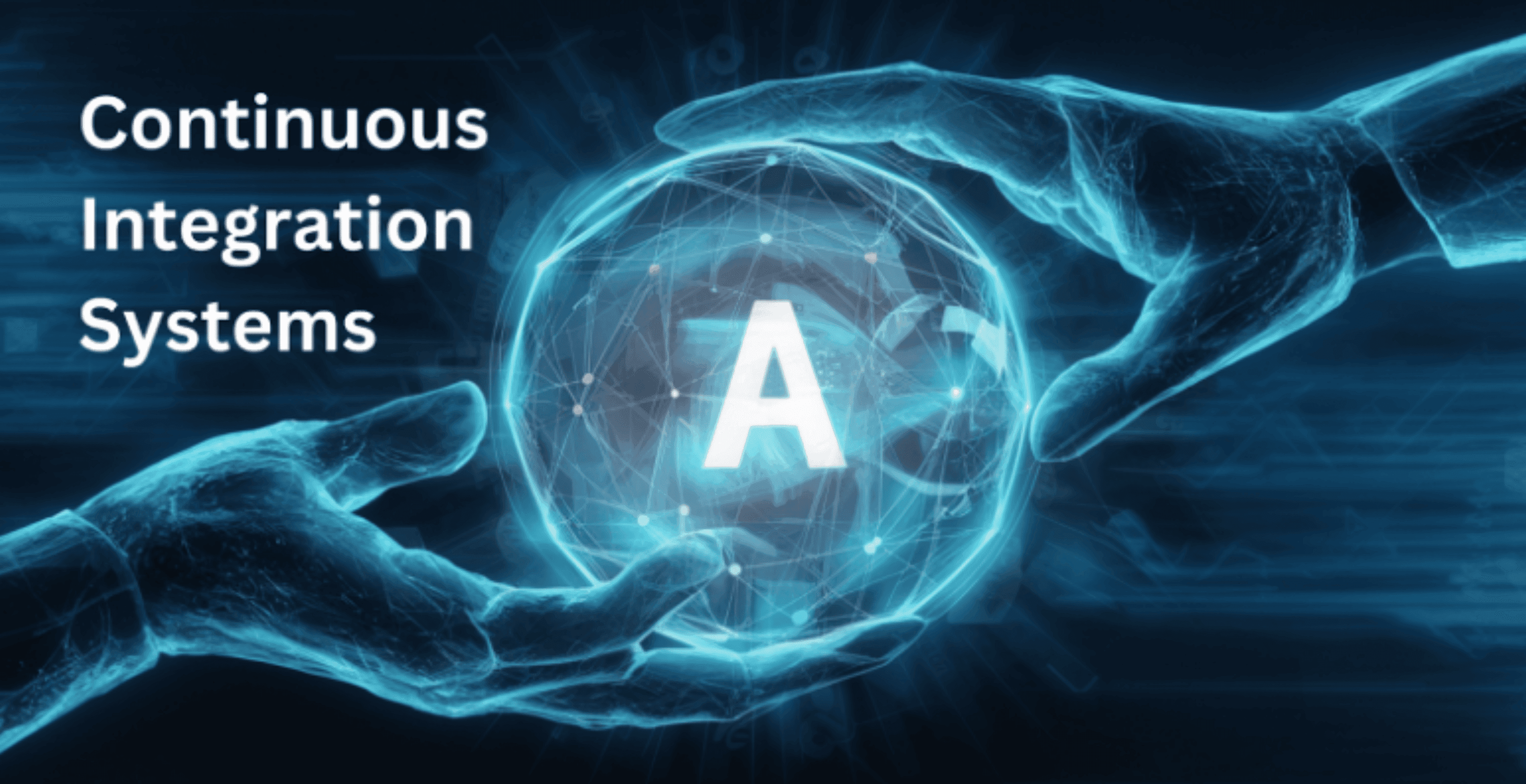
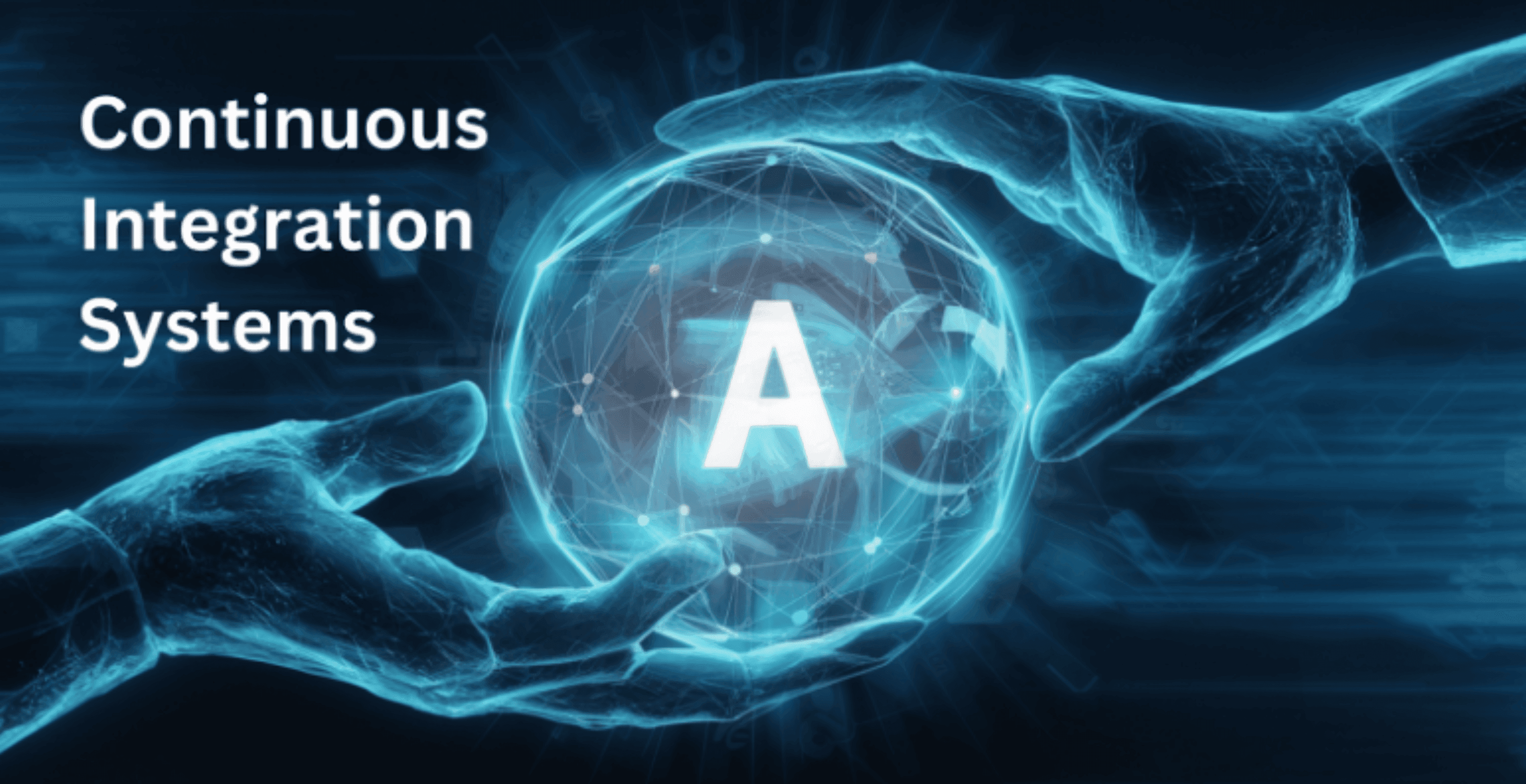
Integrating AI Models with Continuous Integration Systems
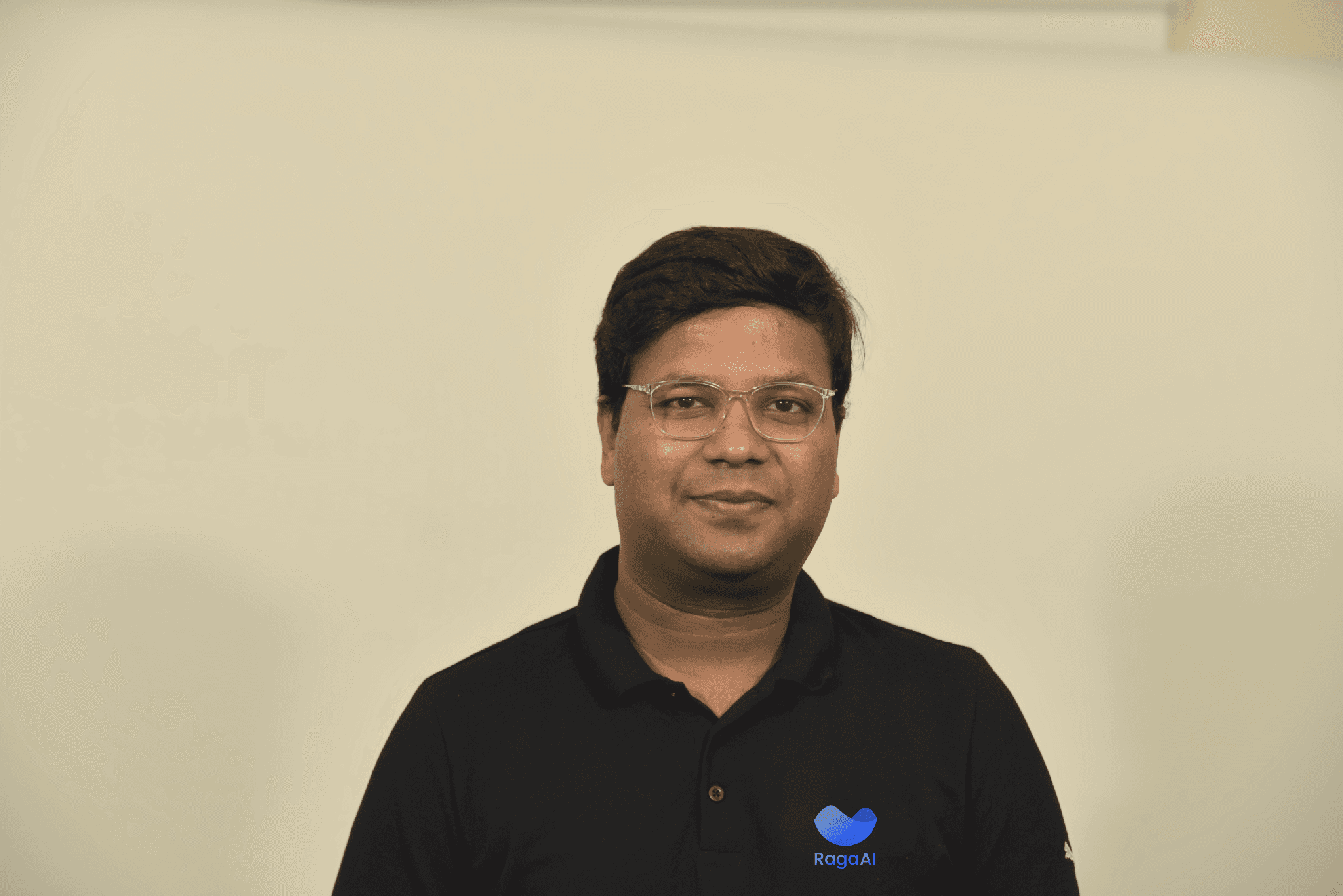
Jigar Gupta
Jul 12, 2024
Read the article




Understanding Retrieval Augmented Generation for Large Language Models: A Survey
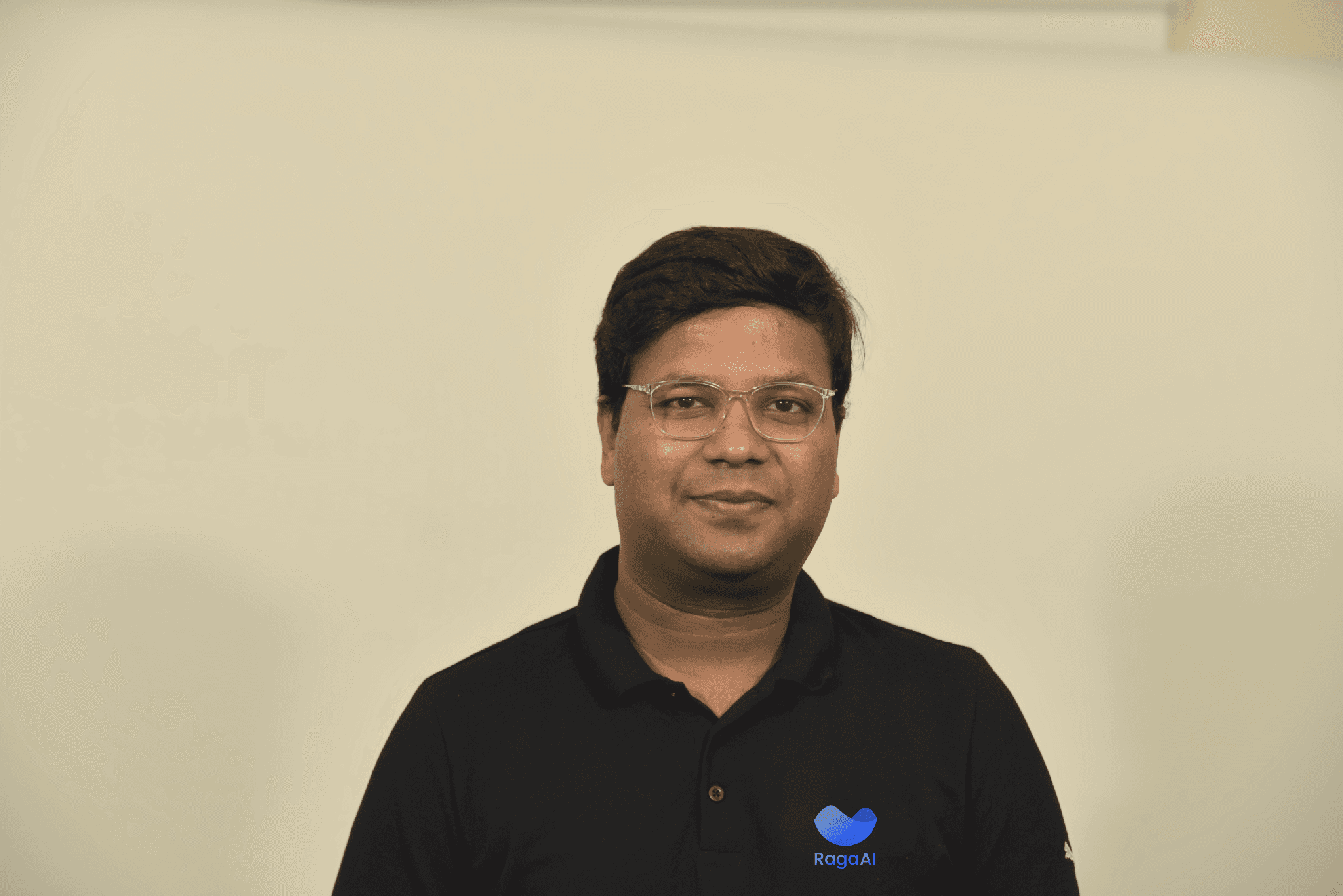
Jigar Gupta
Jul 12, 2024
Read the article




Leveraging AI For Enhanced Retail Customer Experiences
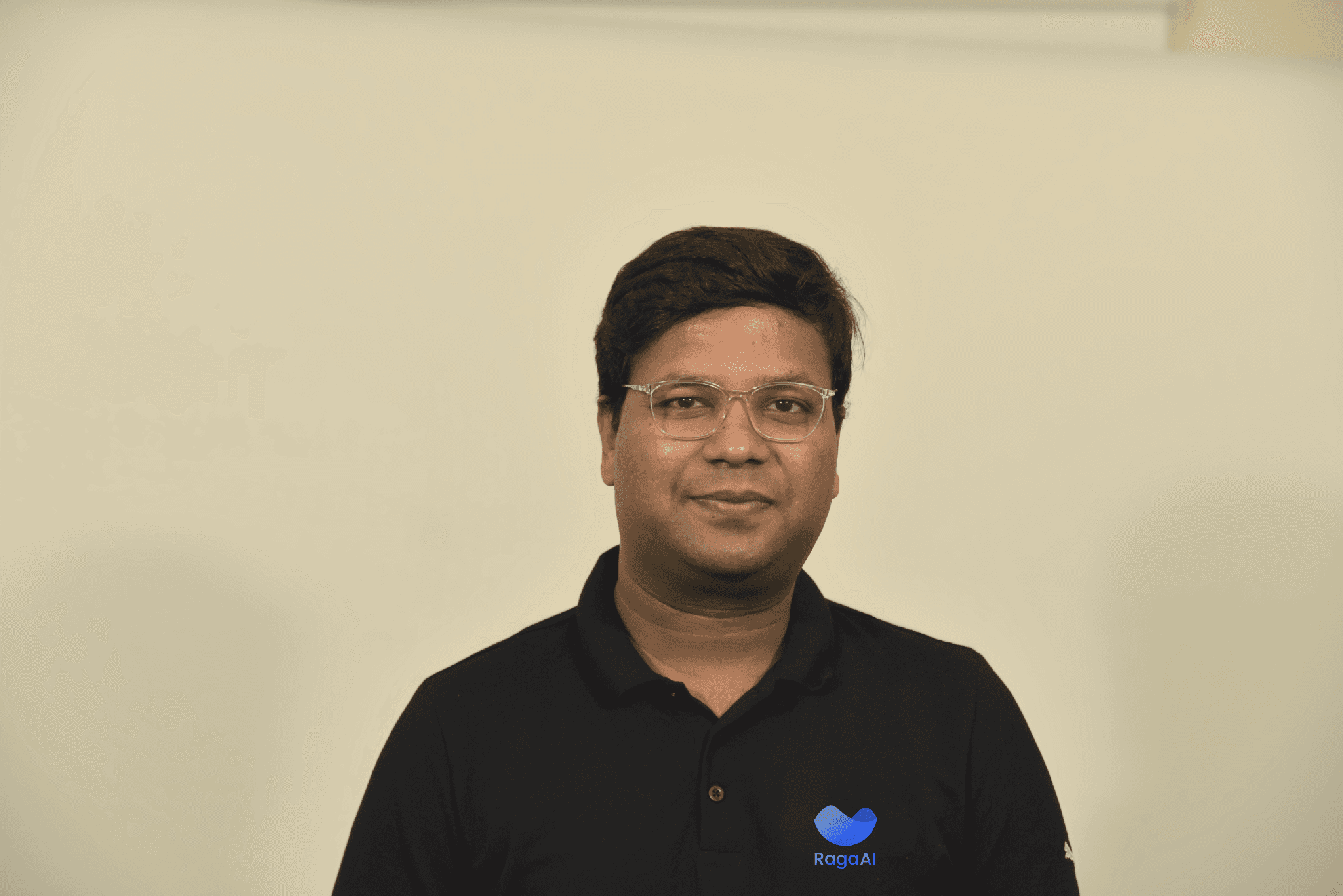
Jigar Gupta
Jul 1, 2024
Read the article
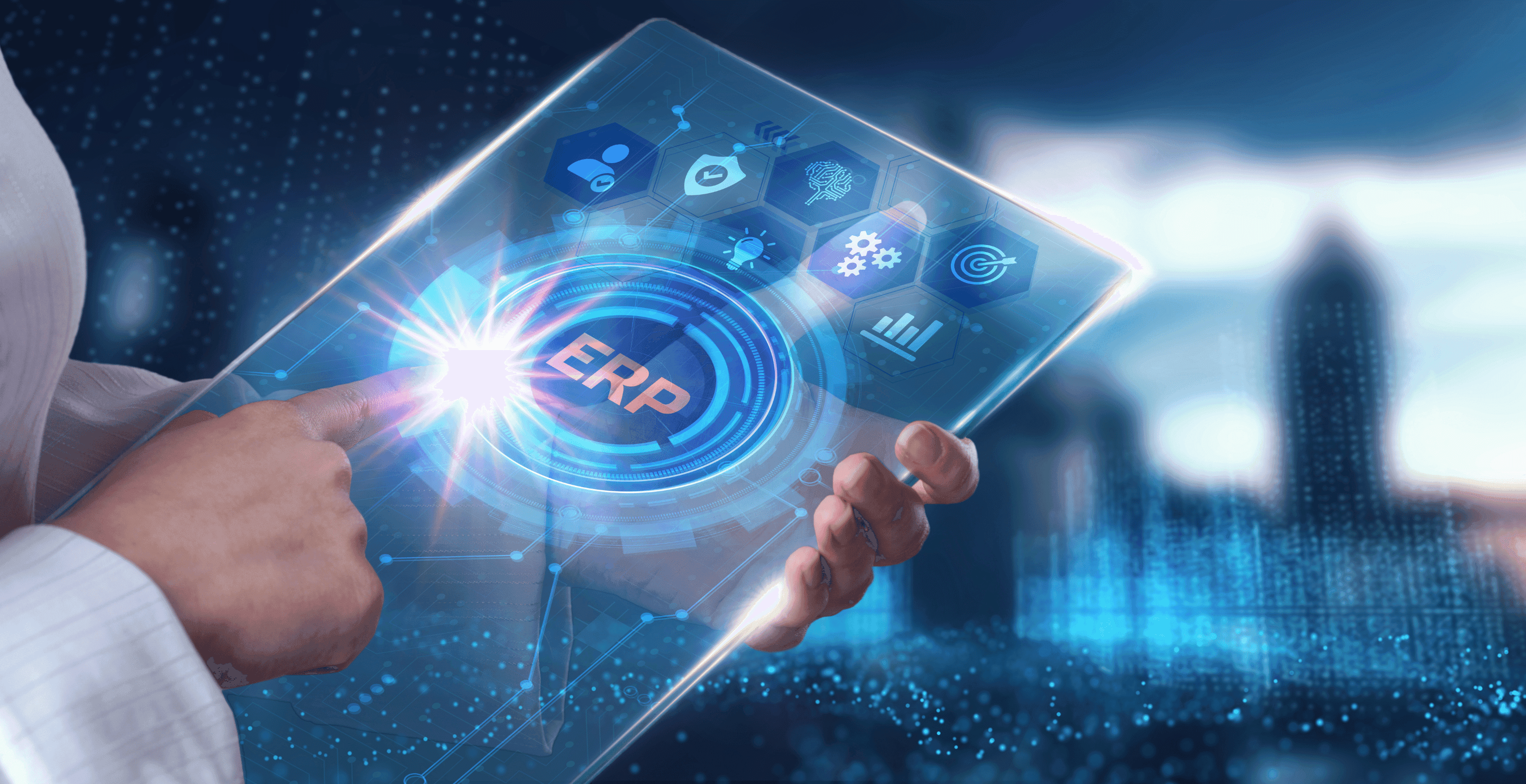
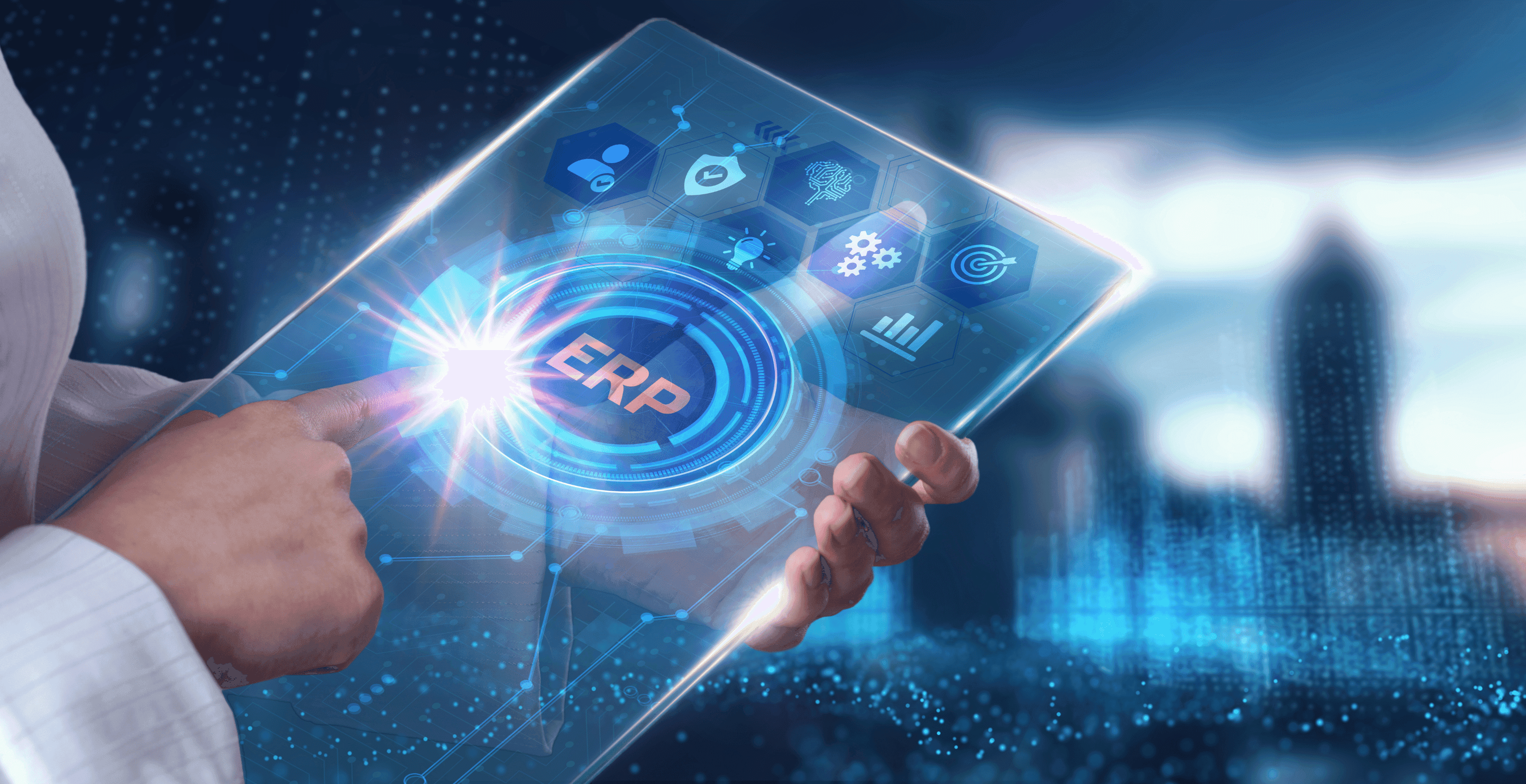
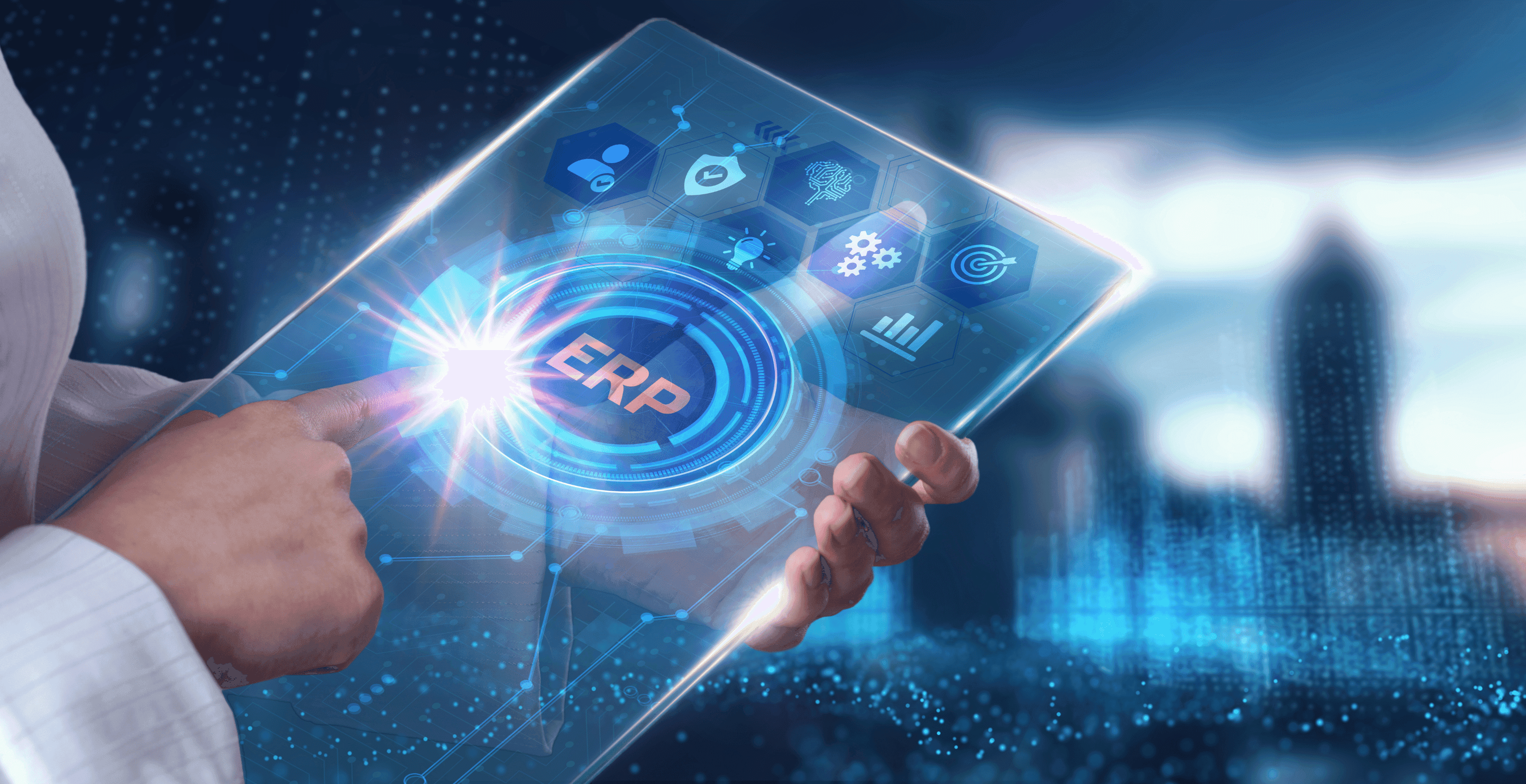
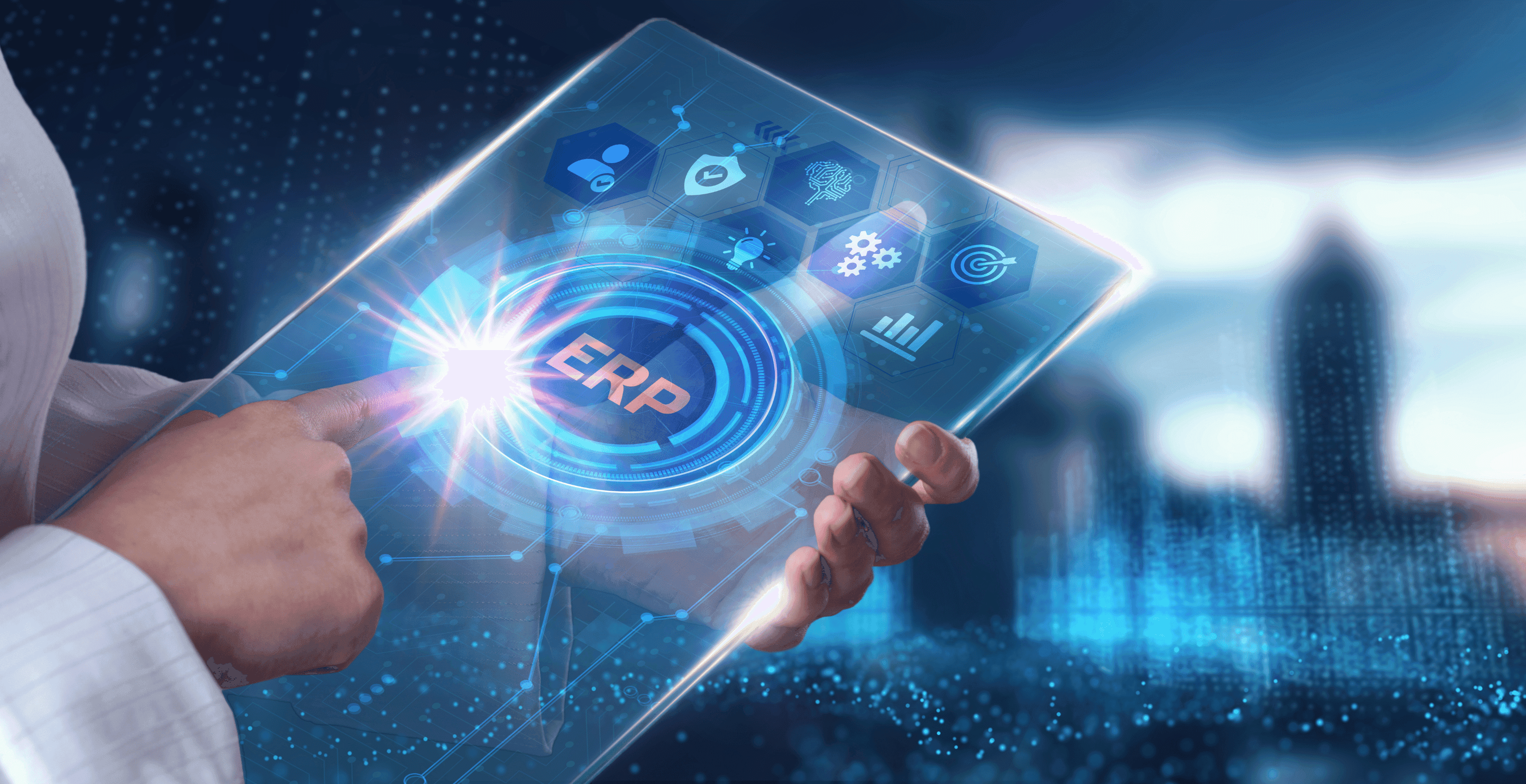
Enhancing Enterprise Search Using RAG and LLMs

Rehan Asif
Jul 1, 2024
Read the article
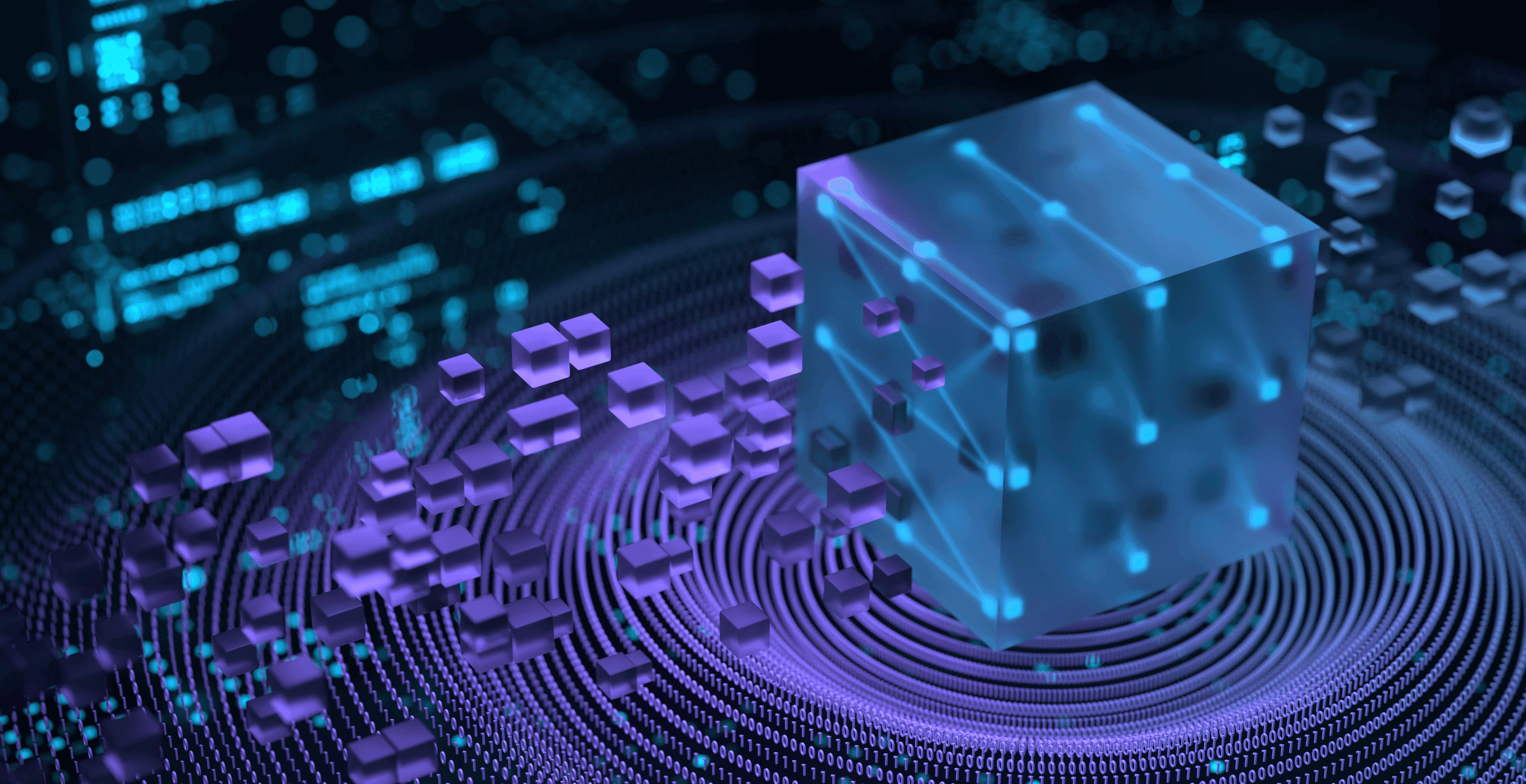
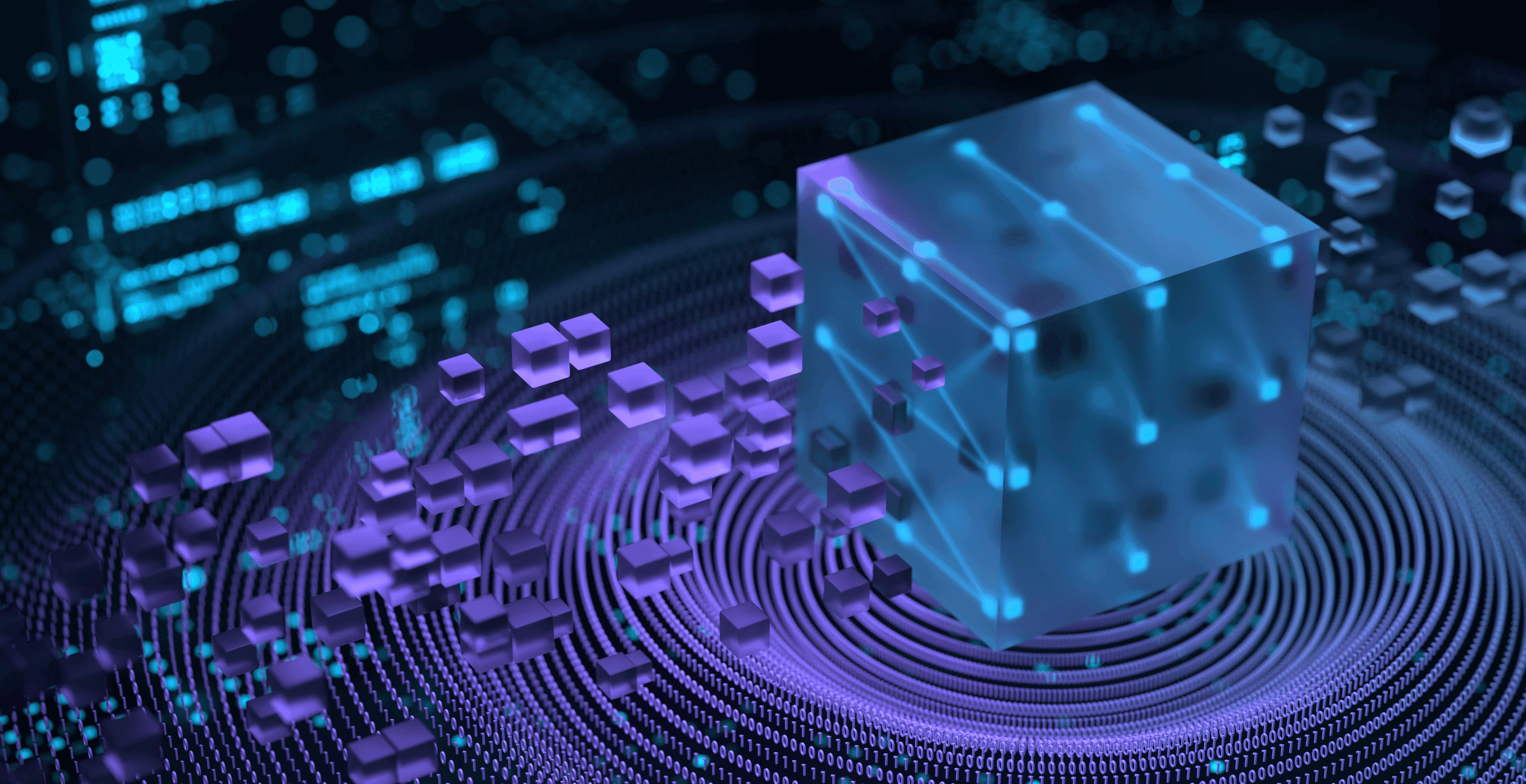
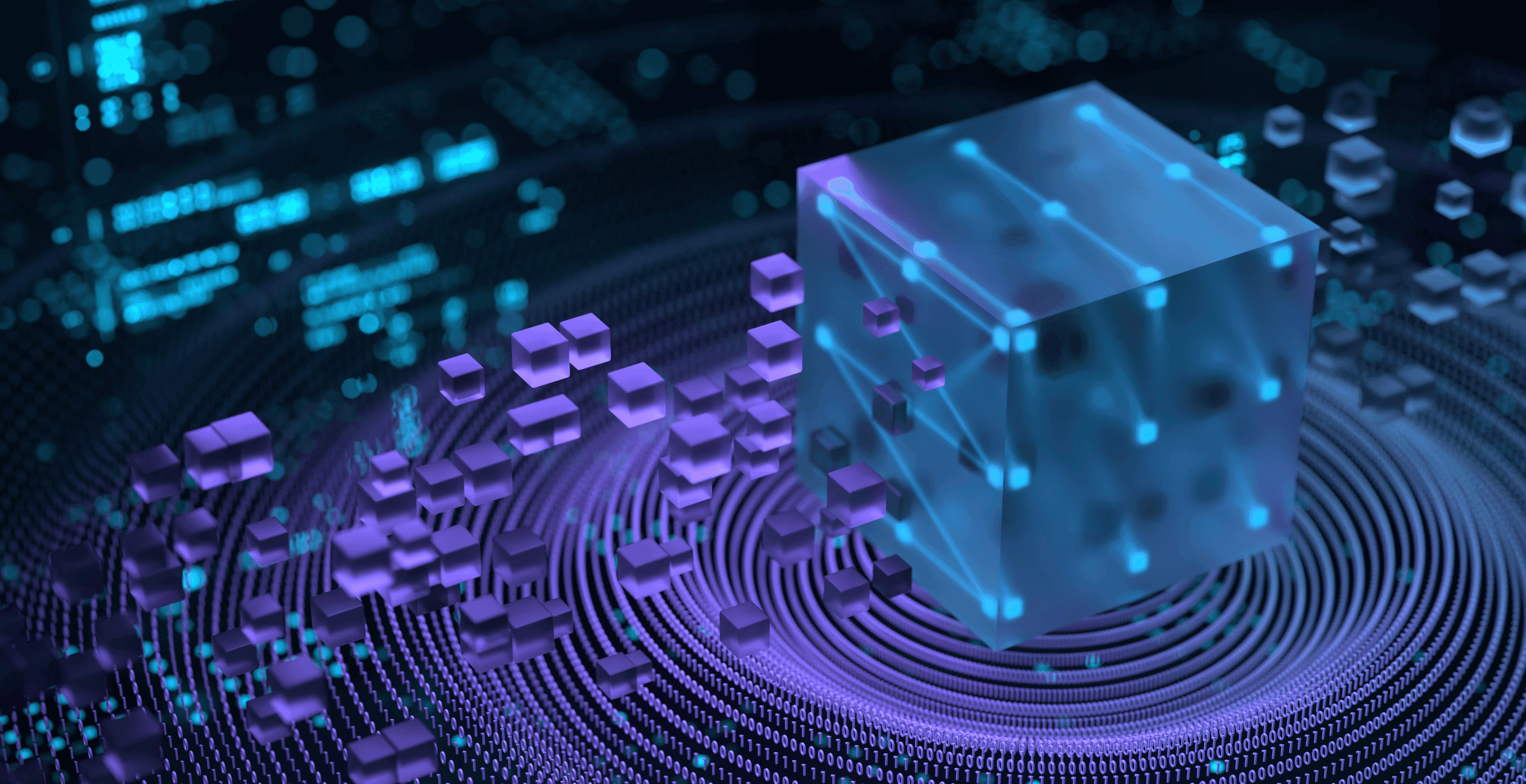
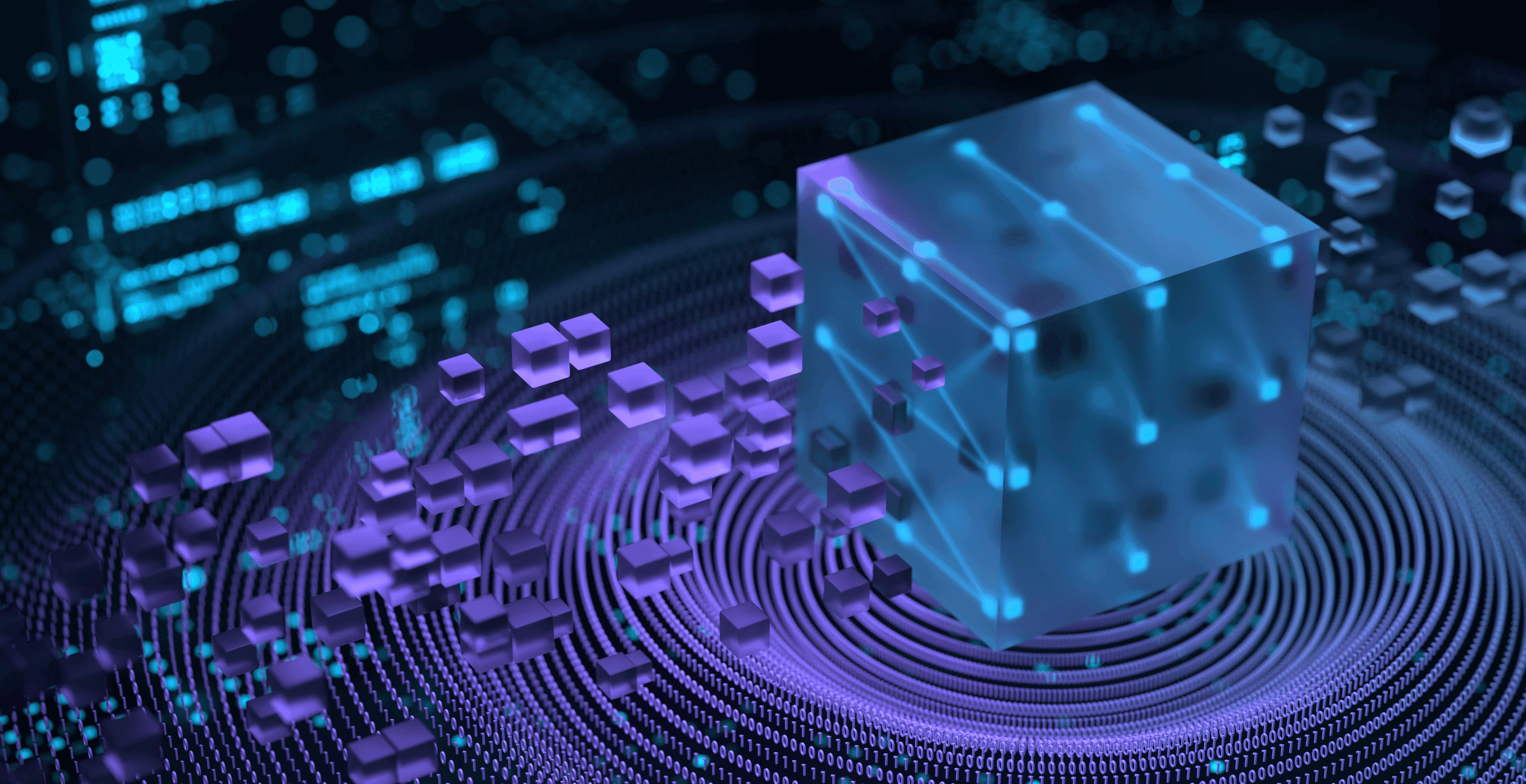
Importance of Accuracy and Reliability in Tabular Data Models
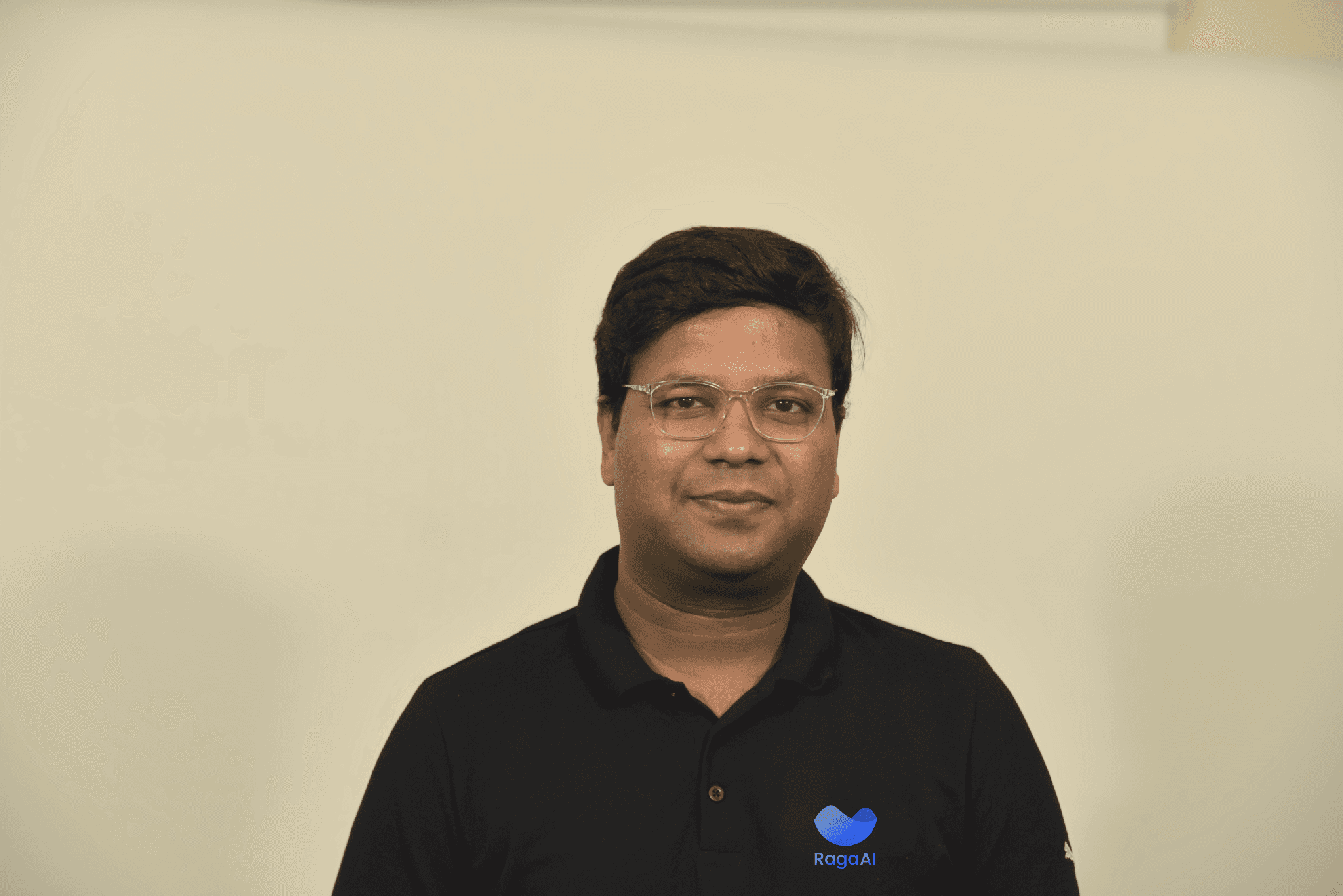
Jigar Gupta
Jul 1, 2024
Read the article
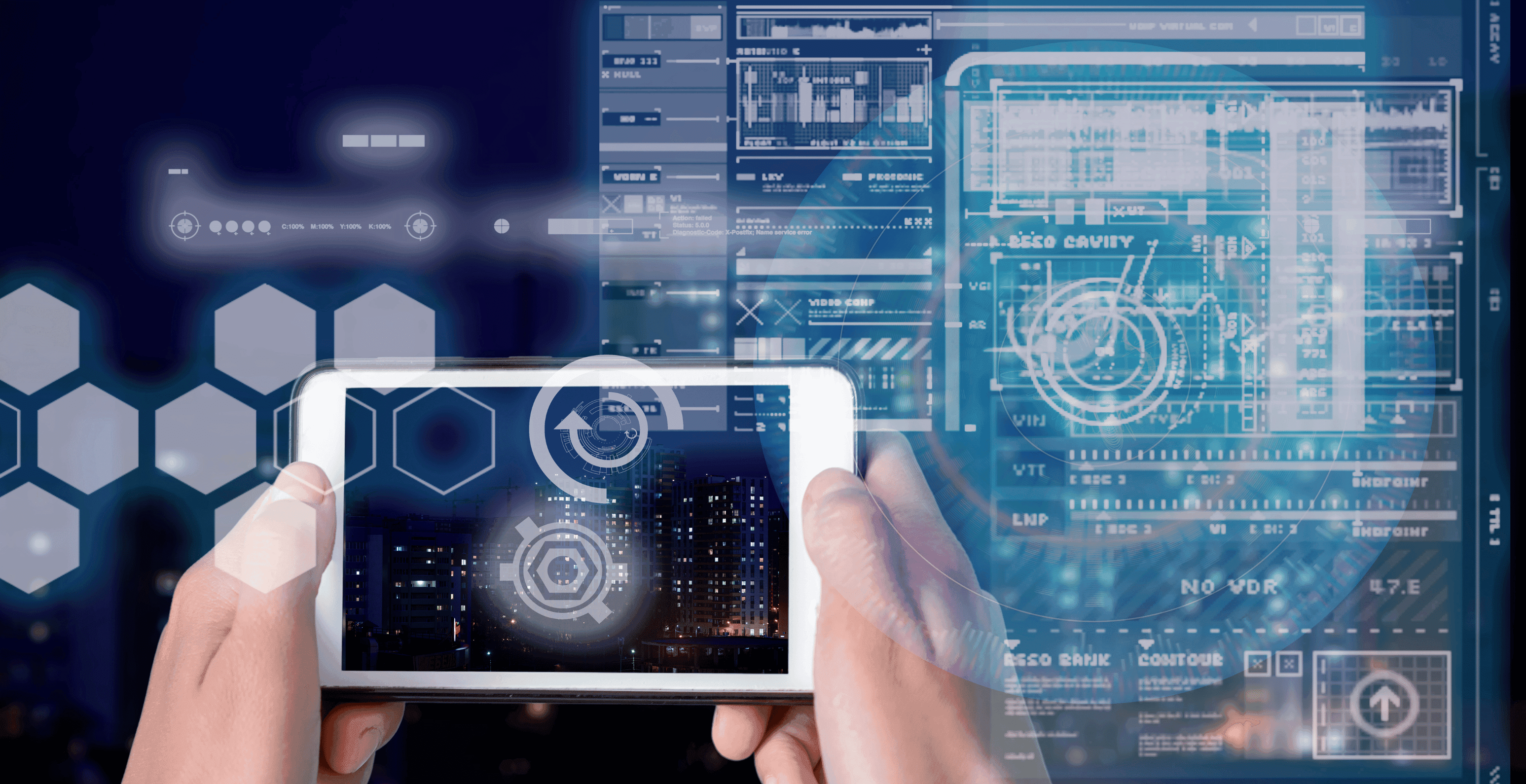
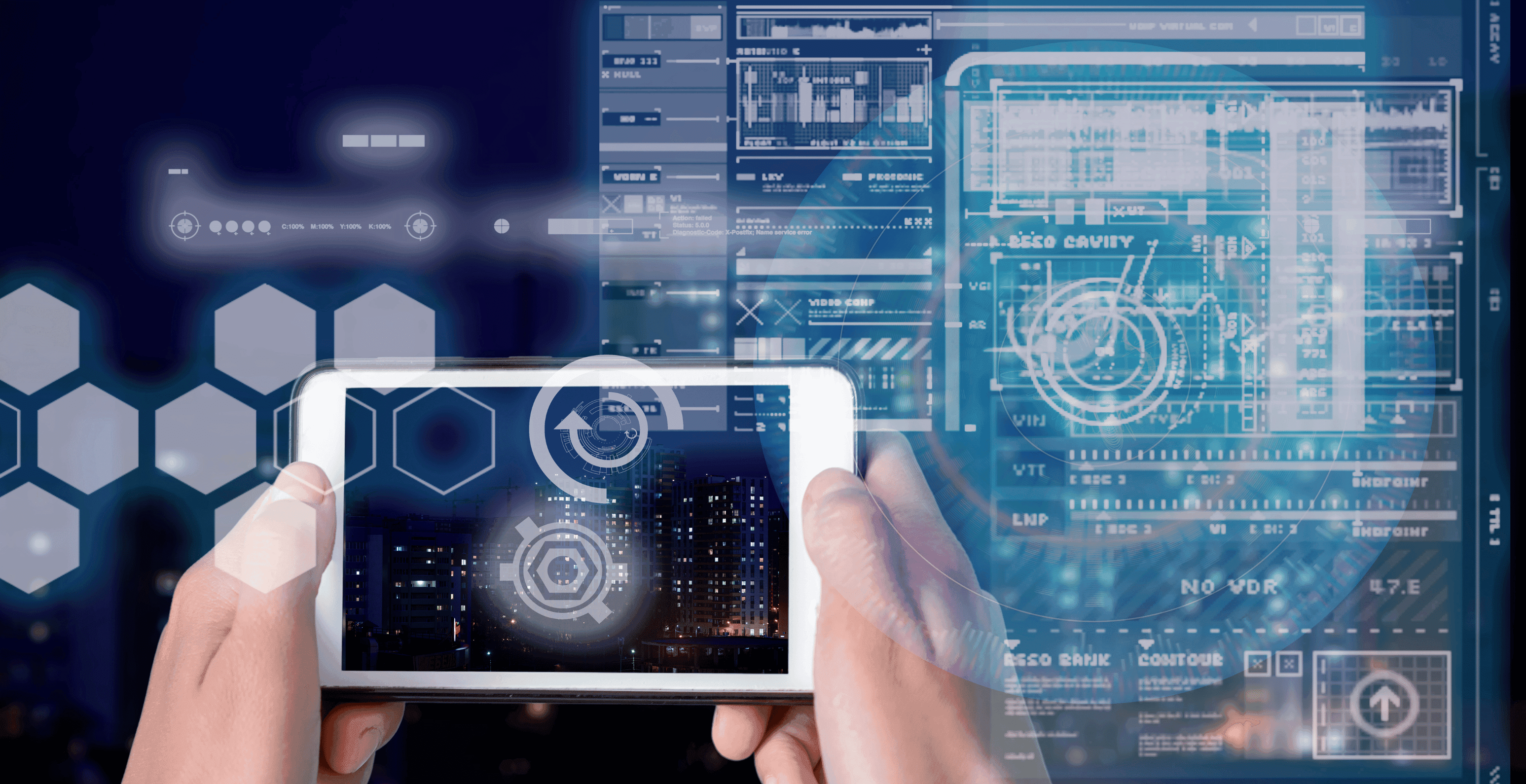
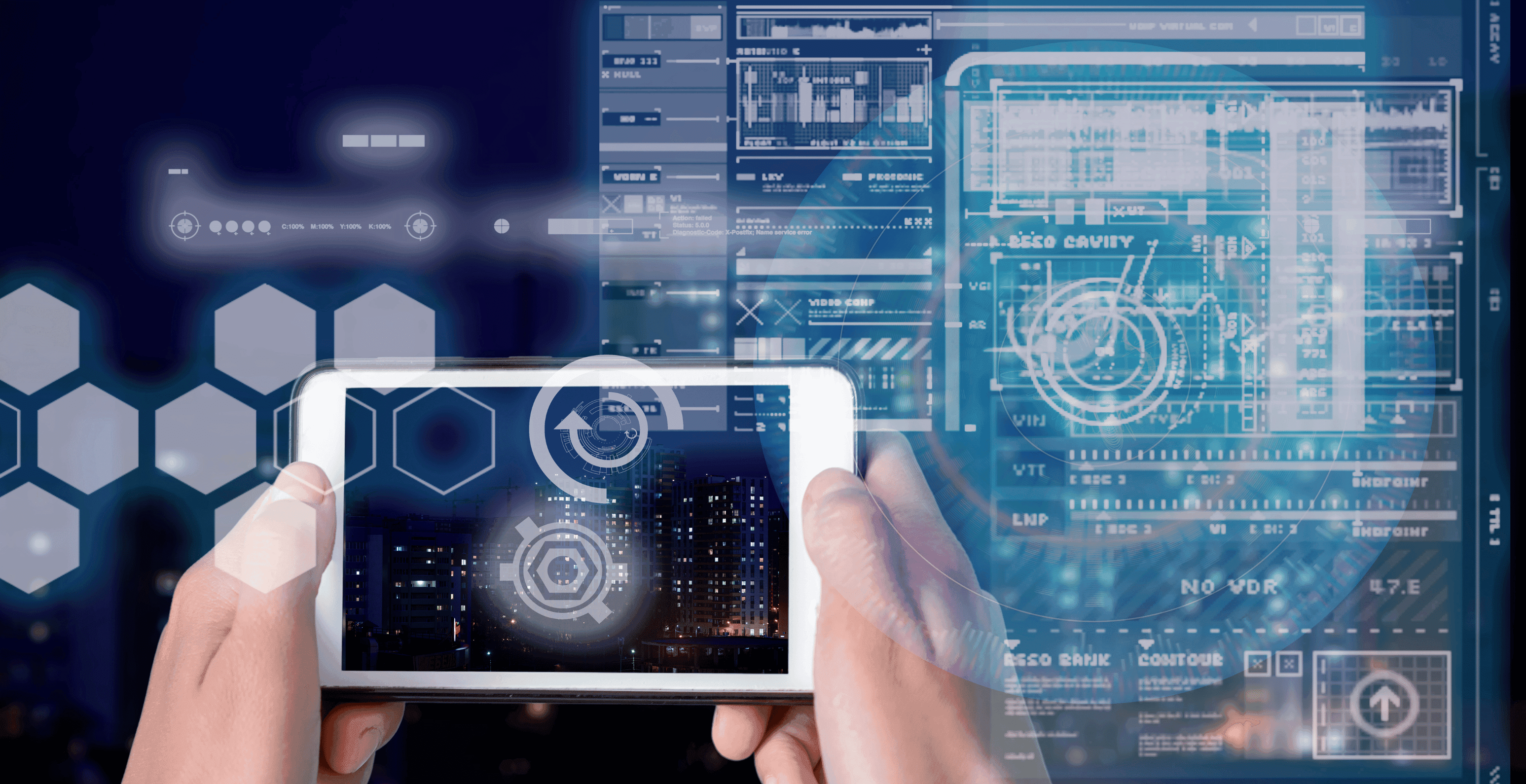
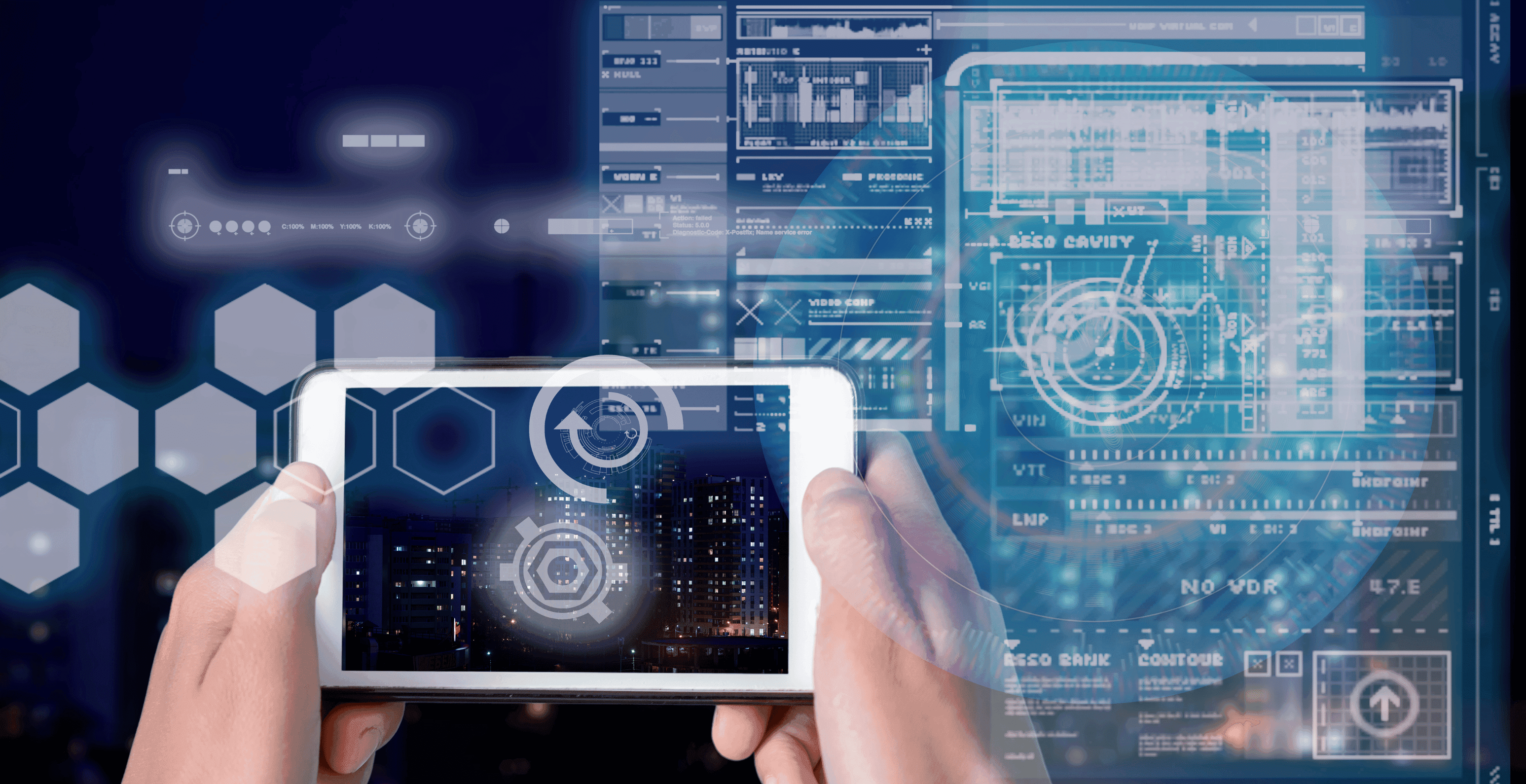
Information Retrieval And LLMs: RAG Explained

Rehan Asif
Jul 1, 2024
Read the article




Introduction to LLM Powered Autonomous Agents

Rehan Asif
Jul 1, 2024
Read the article
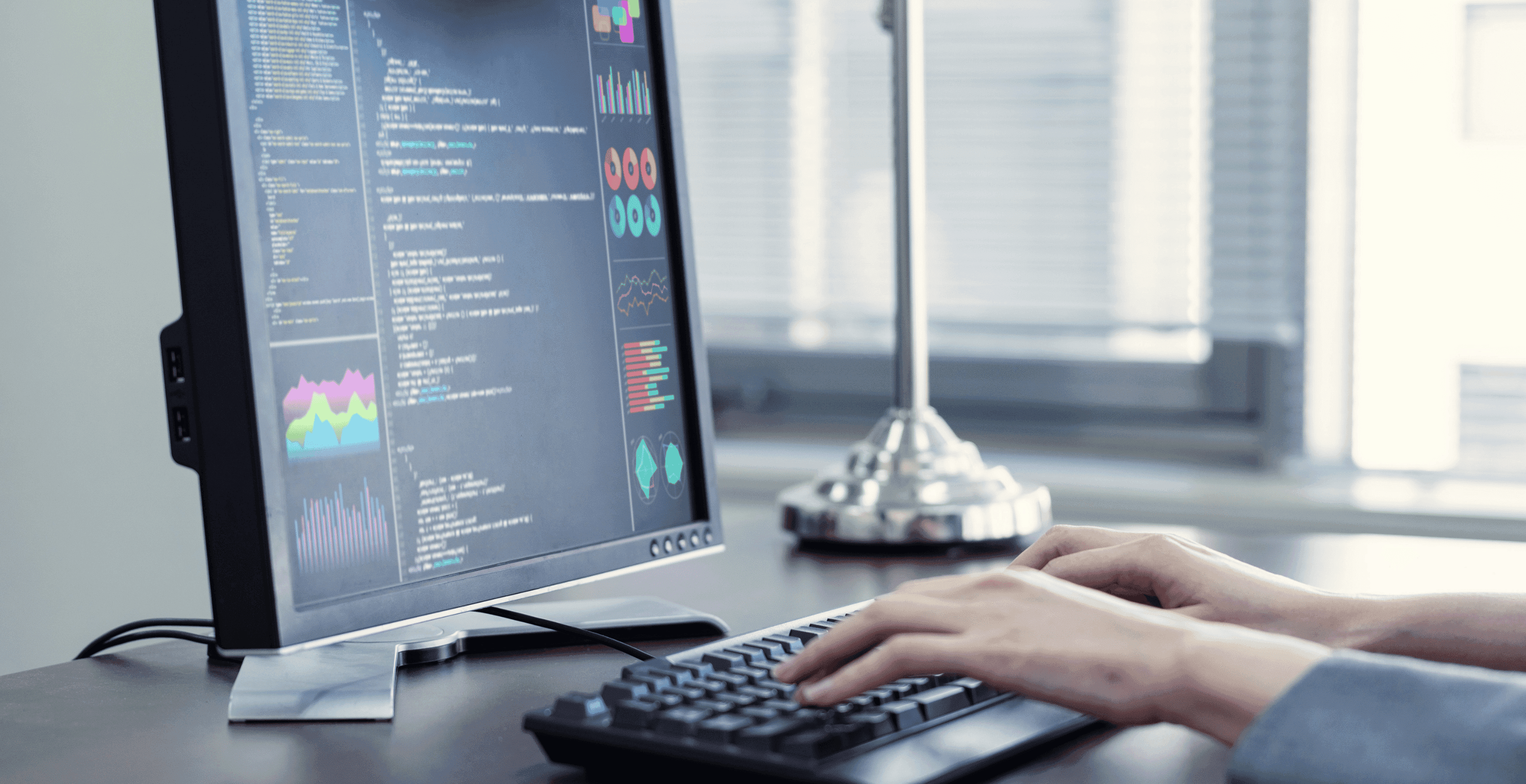
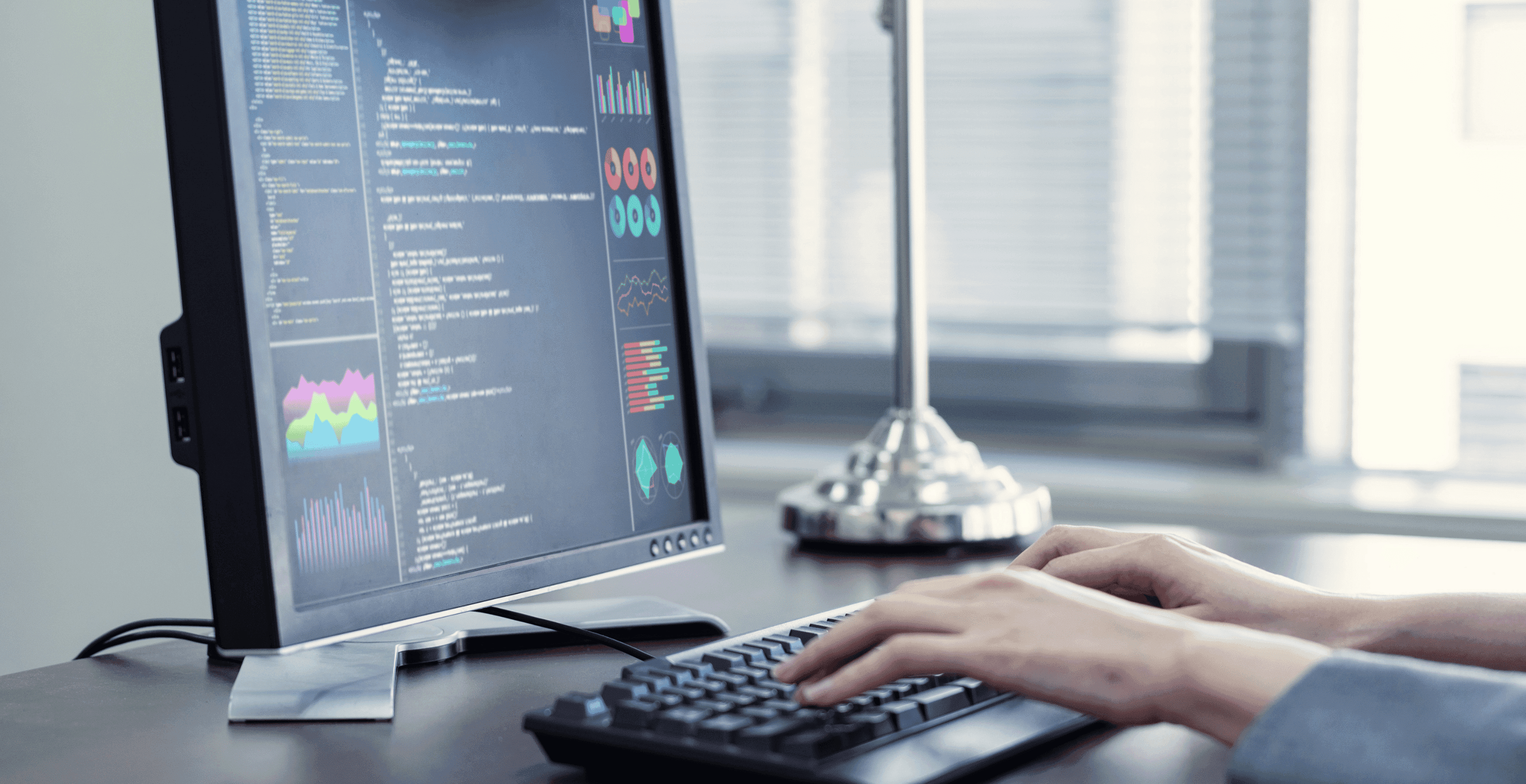
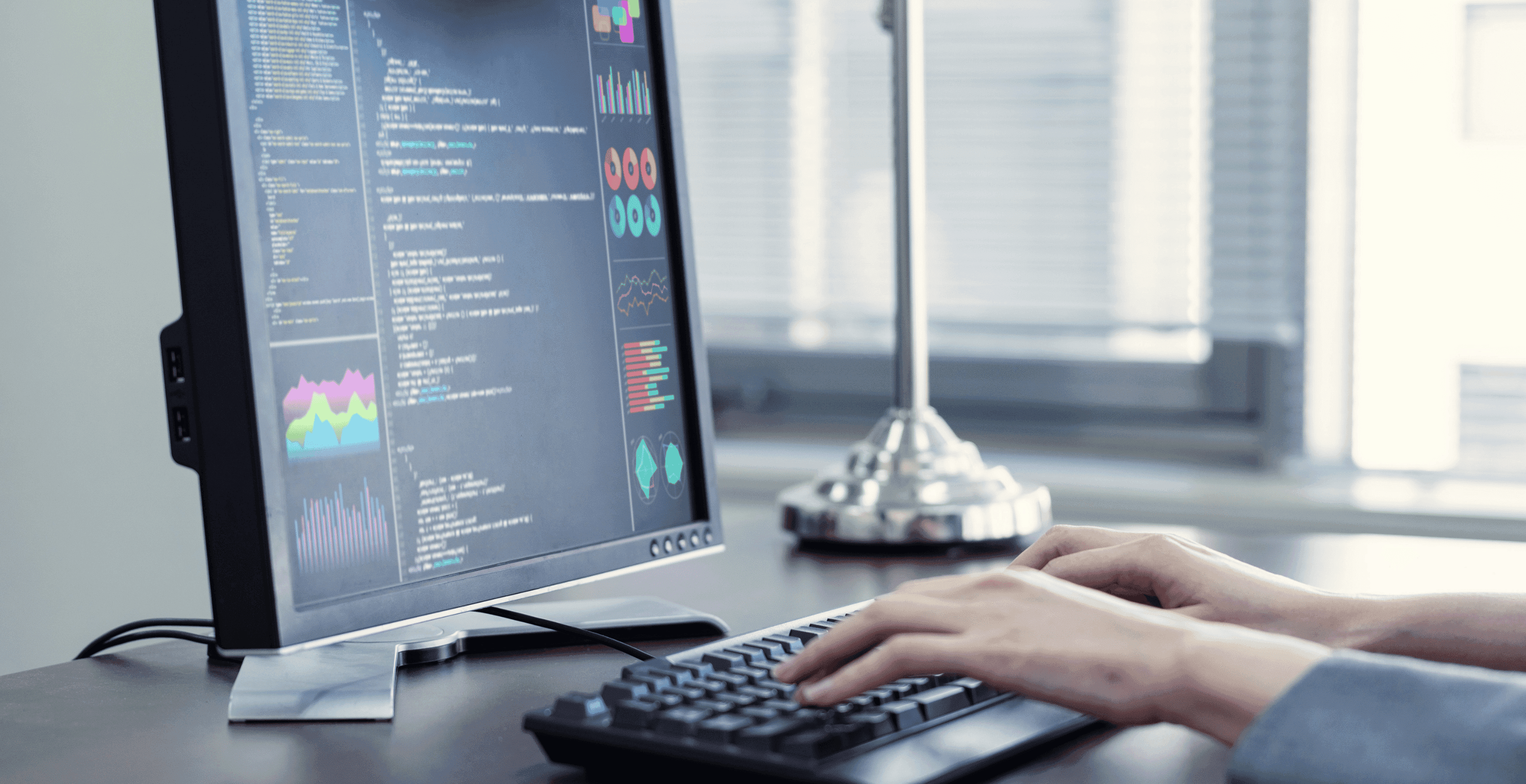
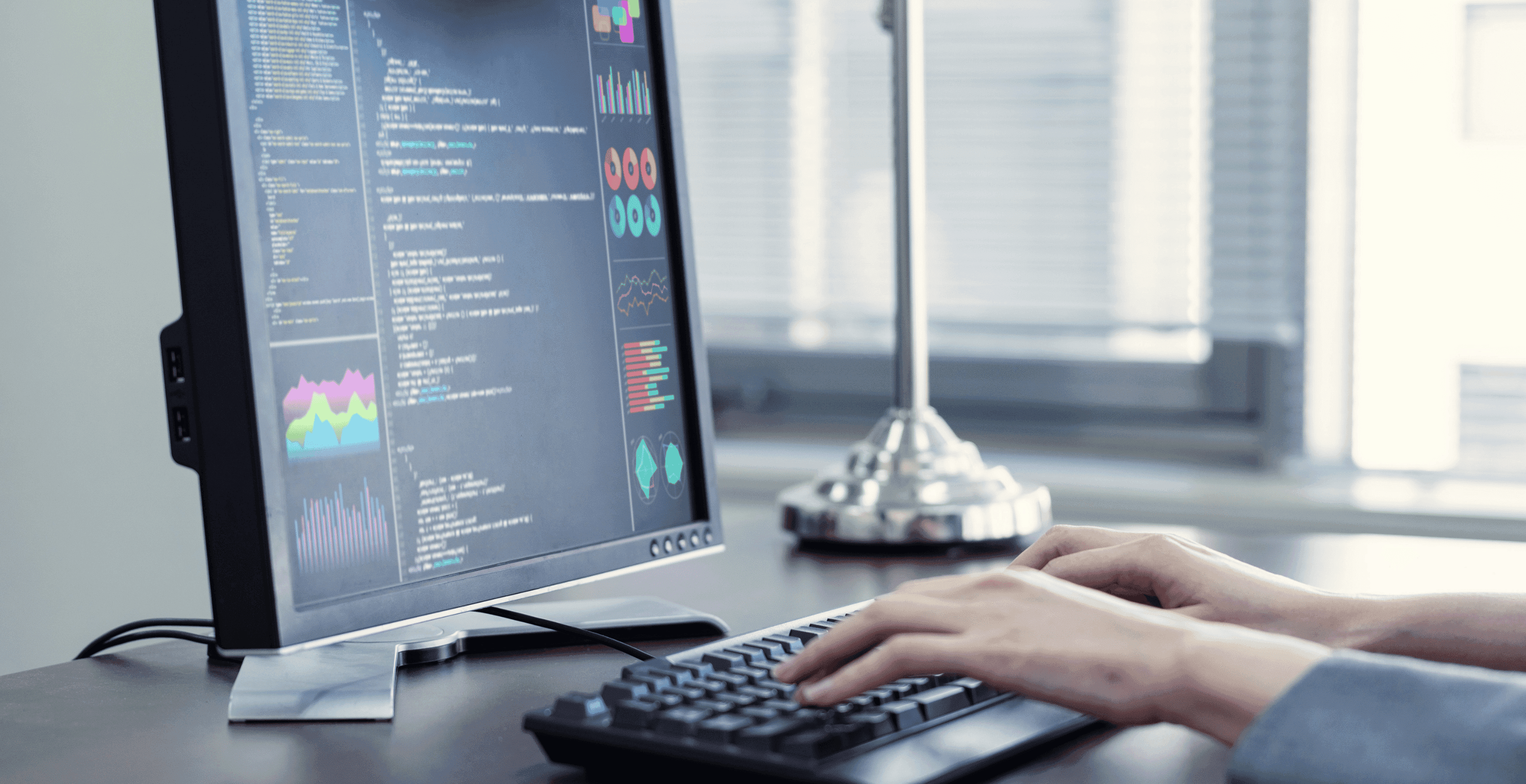
Guide on Unified Multi-Dimensional LLM Evaluation and Benchmark Metrics

Rehan Asif
Jul 1, 2024
Read the article




Innovations In AI For Healthcare
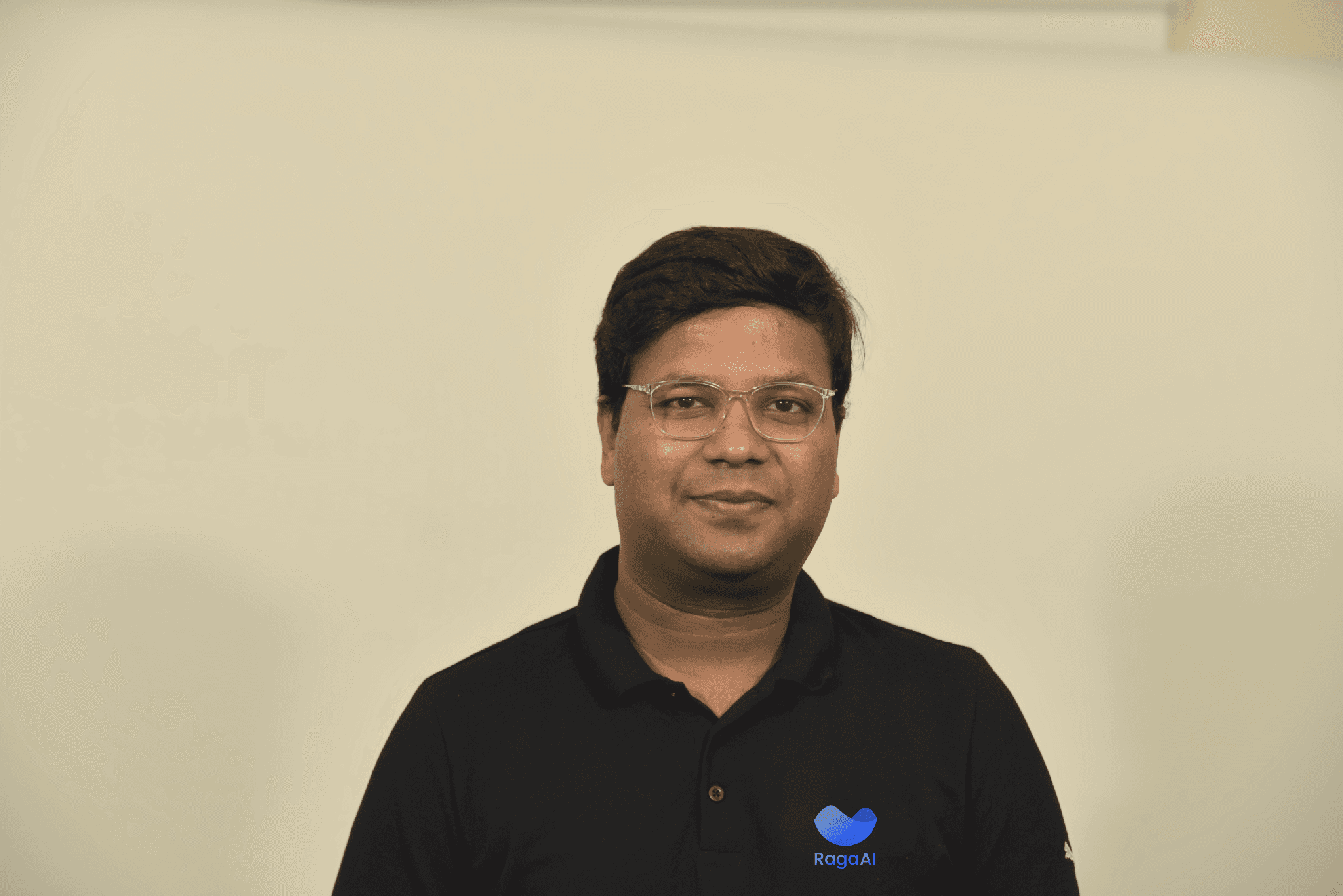
Jigar Gupta
Jun 24, 2024
Read the article




Implementing AI-Driven Inventory Management For The Retail Industry
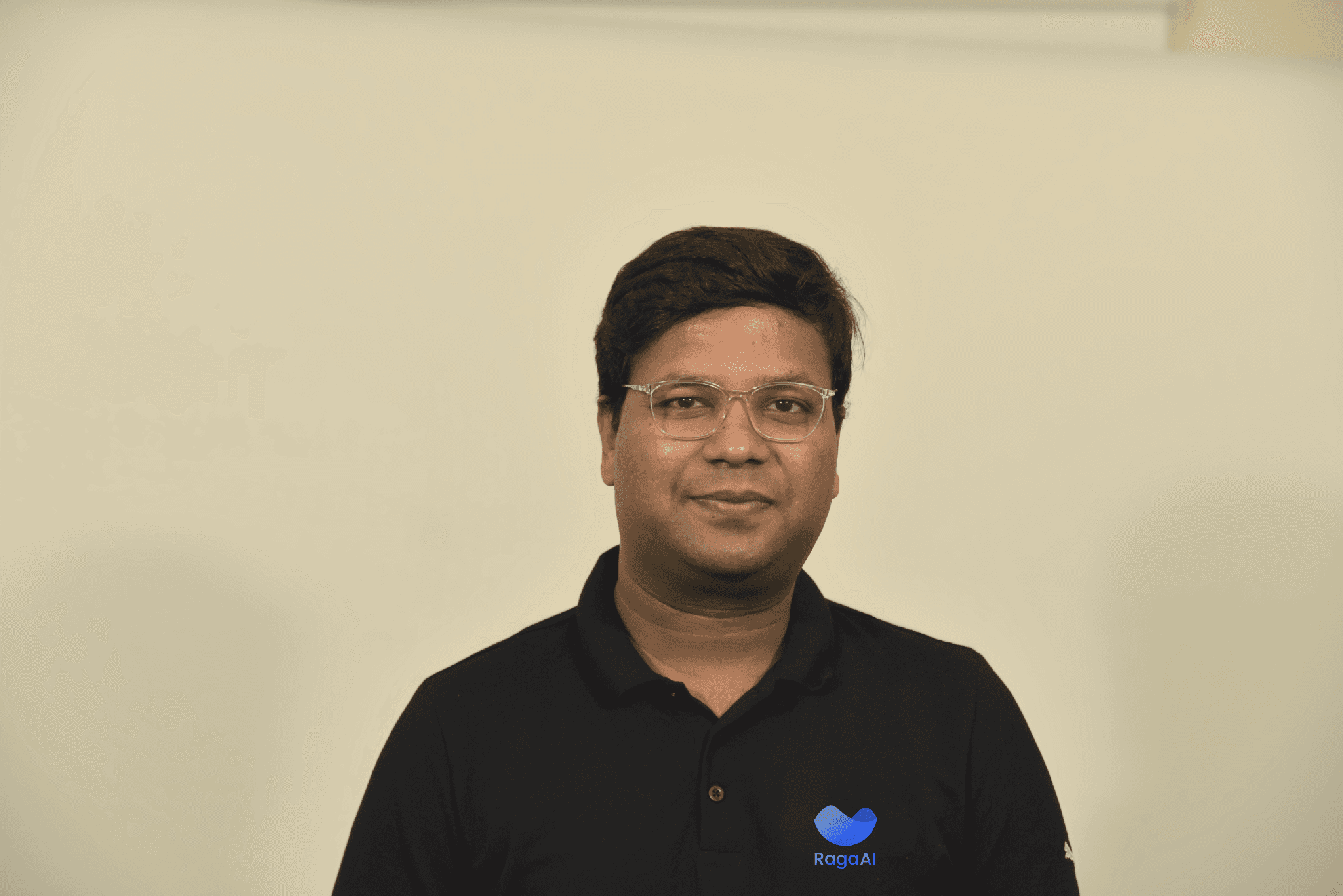
Jigar Gupta
Jun 24, 2024
Read the article




Practical Retrieval Augmented Generation: Use Cases And Impact
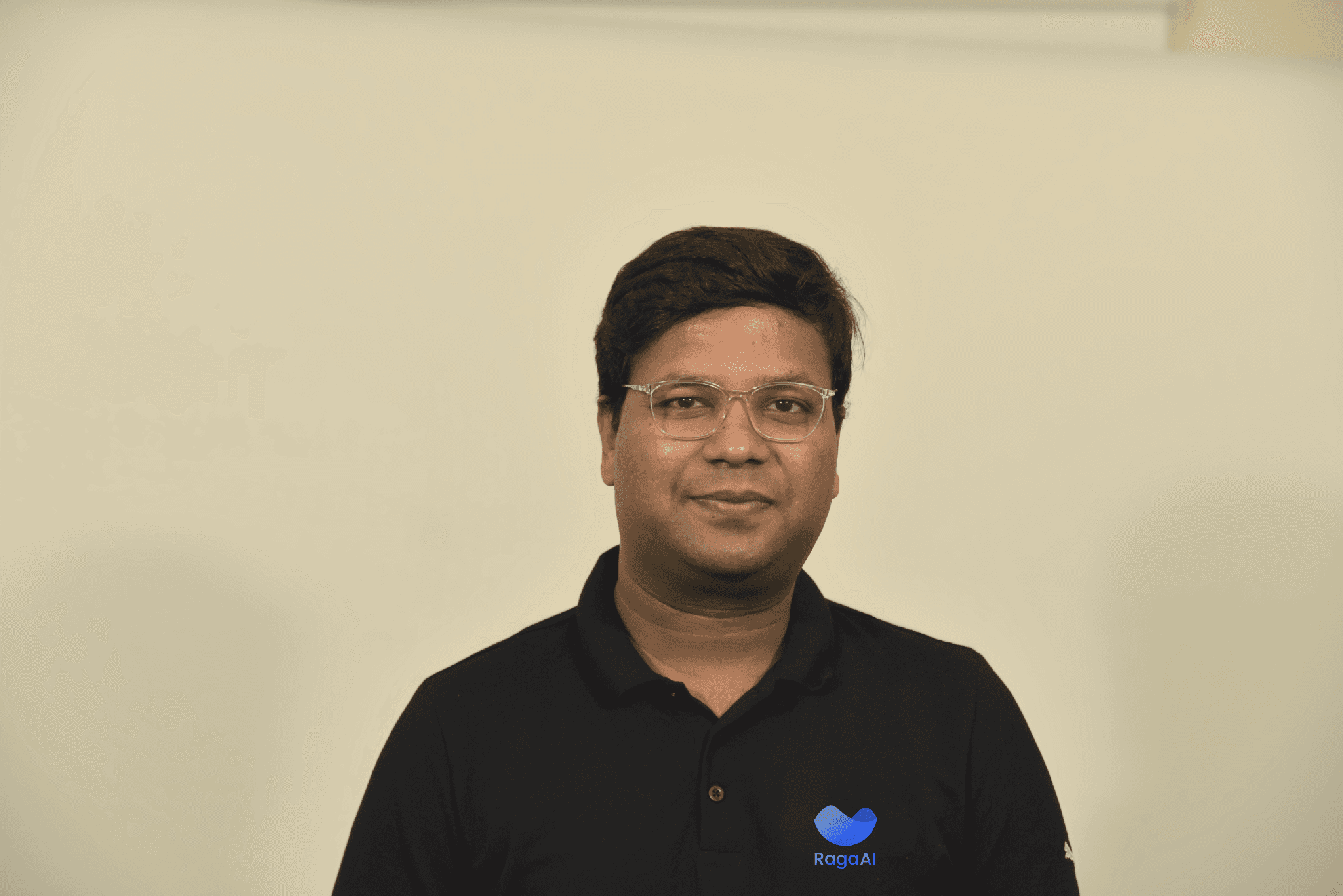
Jigar Gupta
Jun 24, 2024
Read the article




LLM Pre-Training and Fine-Tuning Differences

Rehan Asif
Jun 23, 2024
Read the article




20 LLM Project Ideas For Beginners Using Large Language Models

Rehan Asif
Jun 23, 2024
Read the article




Understanding LLM Parameters: Tuning Top-P, Temperature And Tokens

Rehan Asif
Jun 23, 2024
Read the article




Understanding Large Action Models In AI

Rehan Asif
Jun 23, 2024
Read the article




Building And Implementing Custom LLM Guardrails

Rehan Asif
Jun 12, 2024
Read the article




Understanding LLM Alignment: A Simple Guide

Rehan Asif
Jun 12, 2024
Read the article
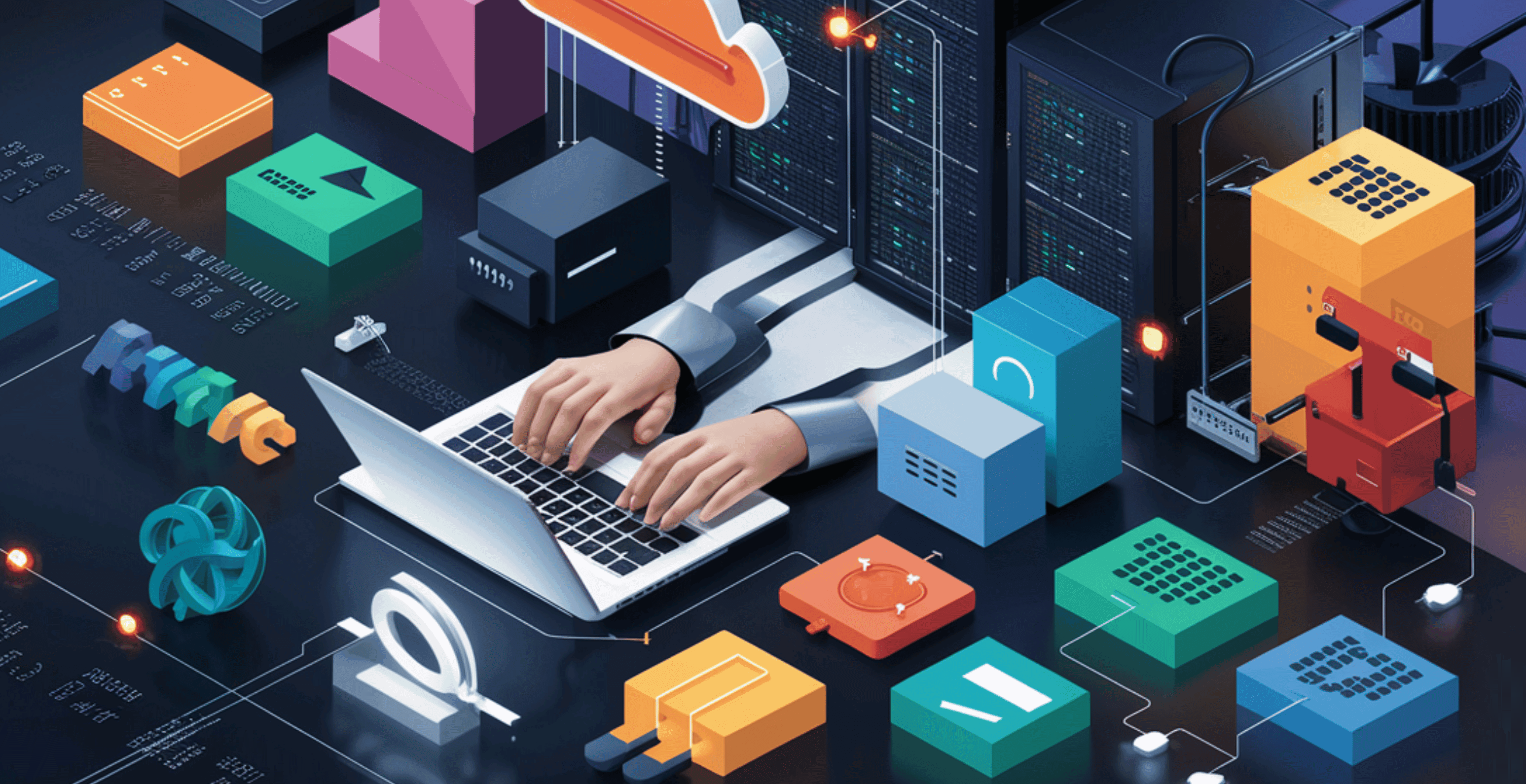
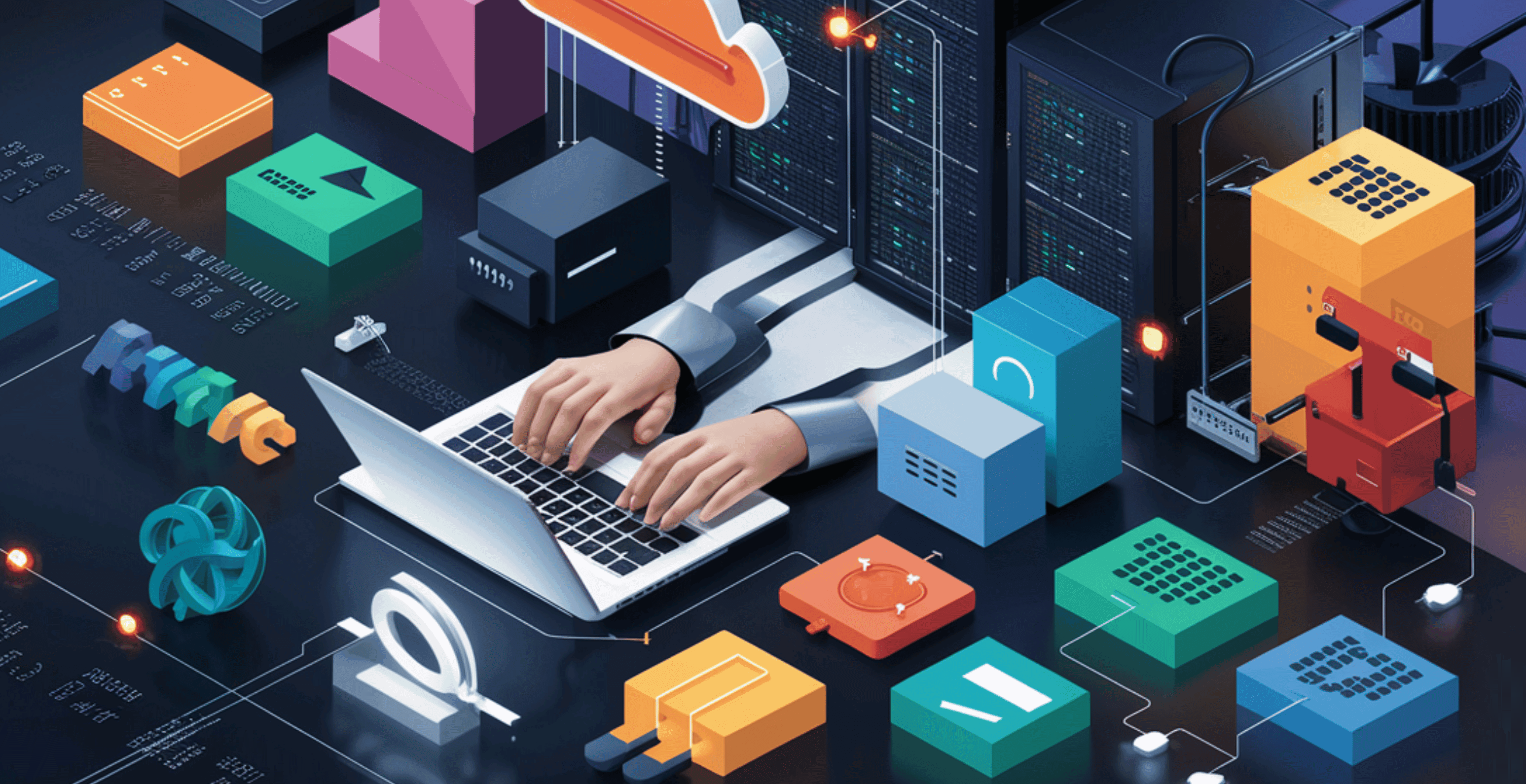
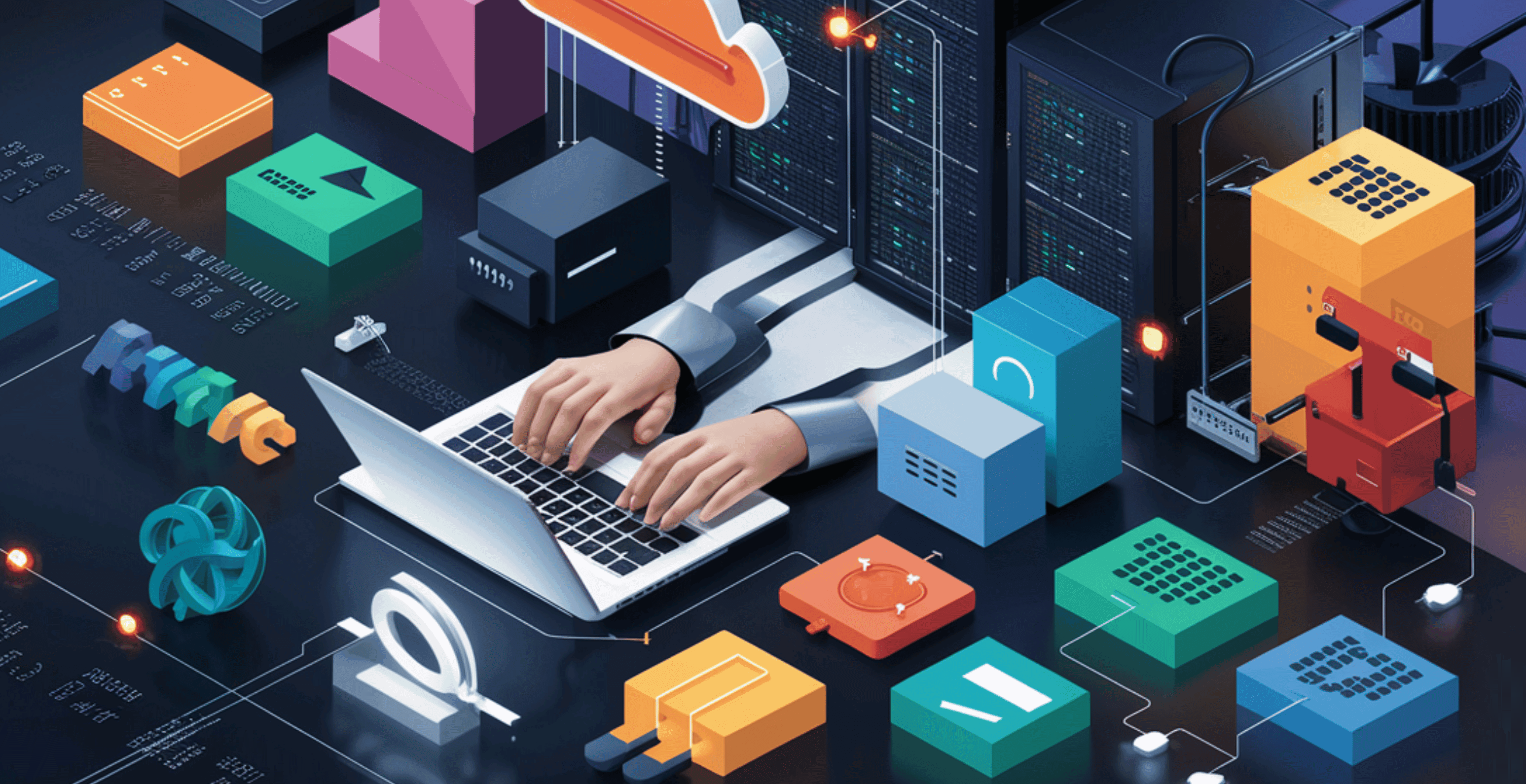
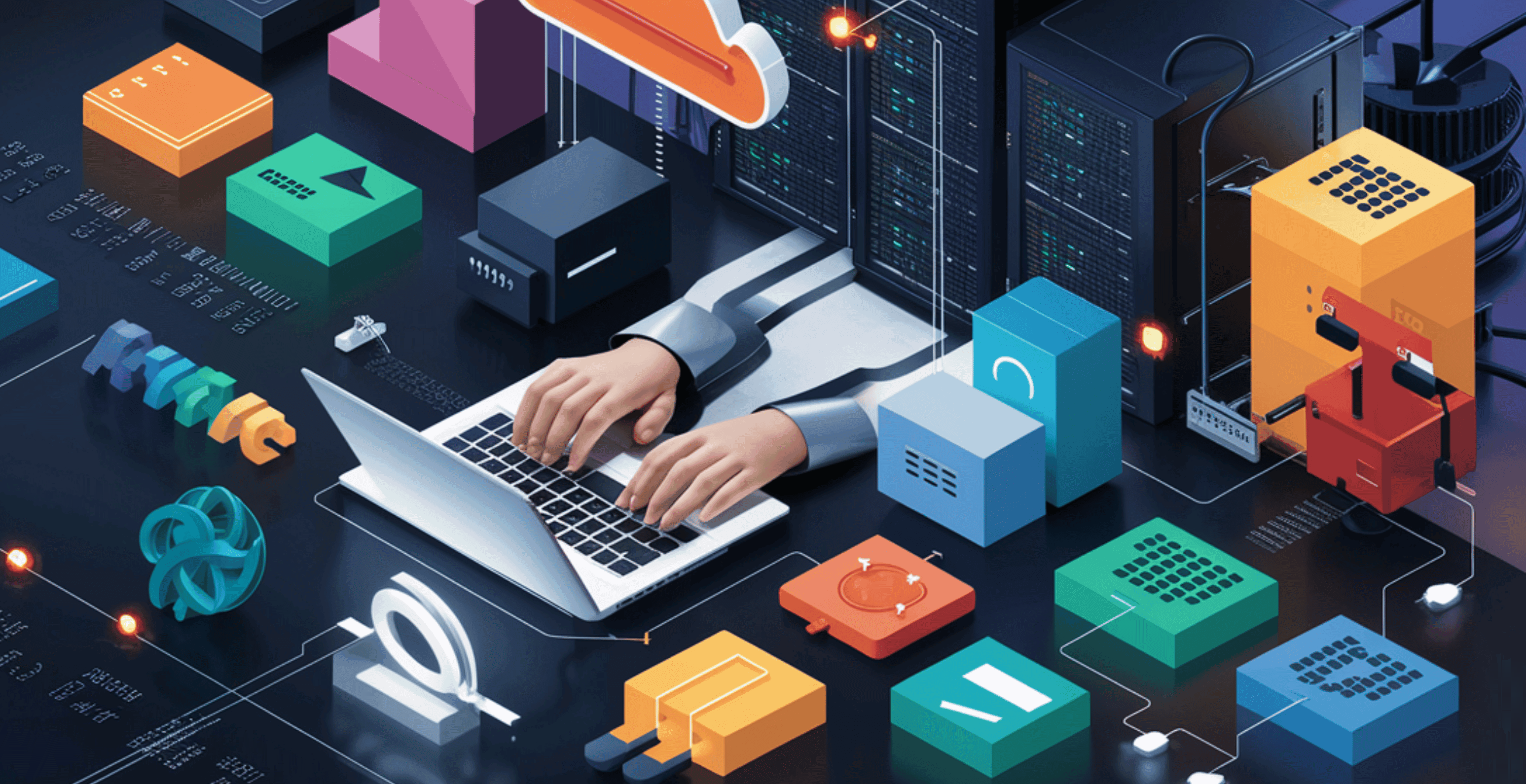
Practical Strategies For Self-Hosting Large Language Models

Rehan Asif
Jun 12, 2024
Read the article
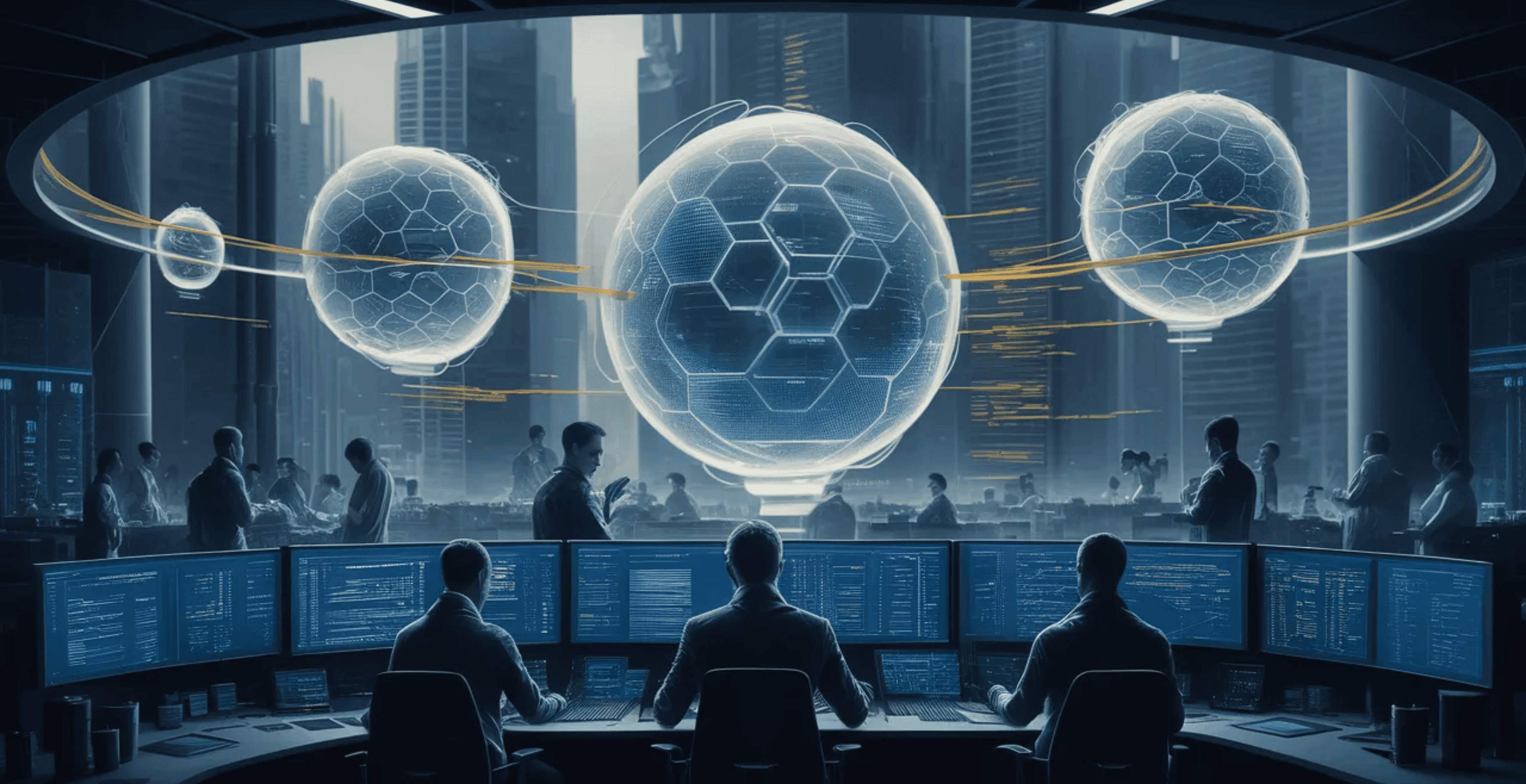
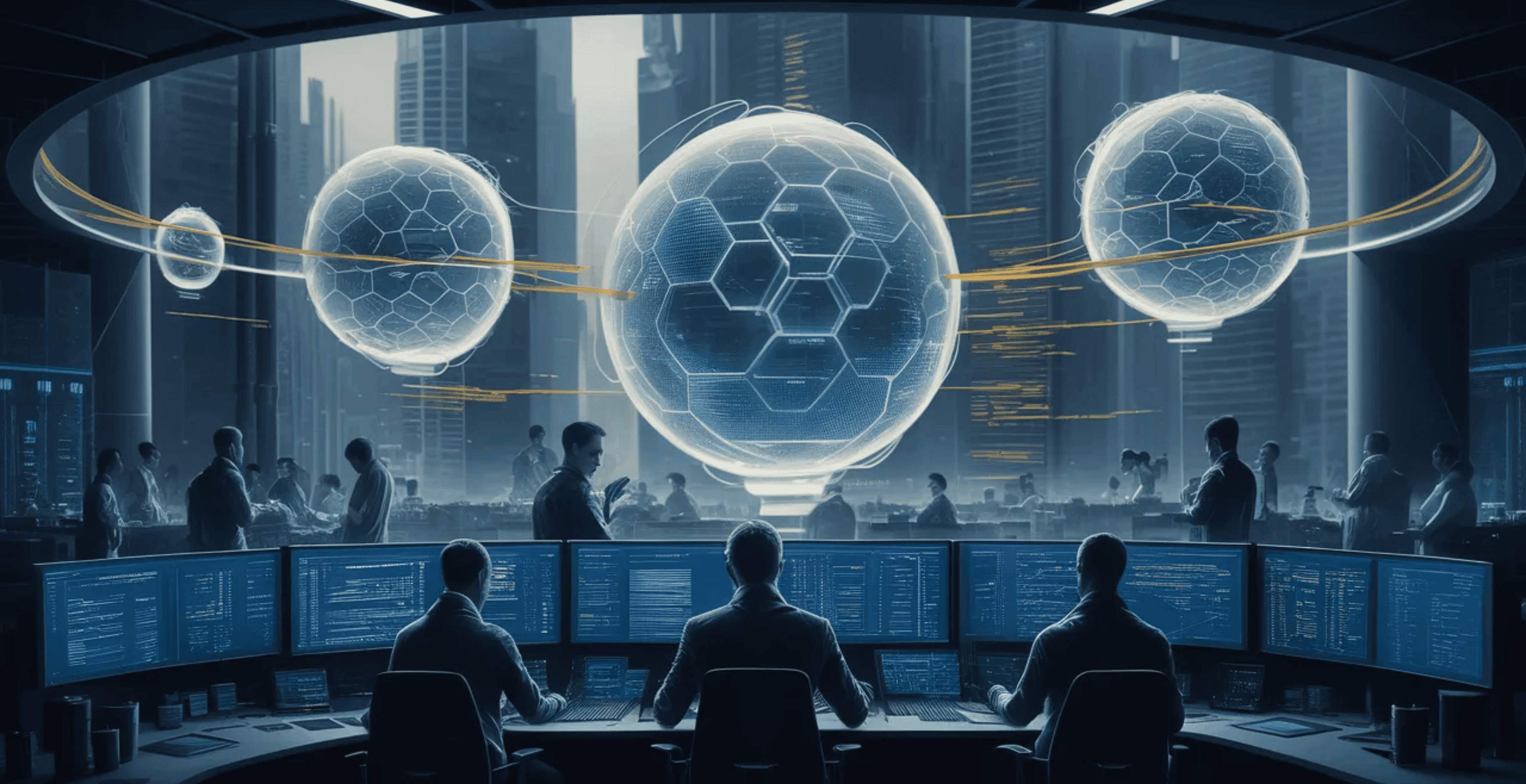
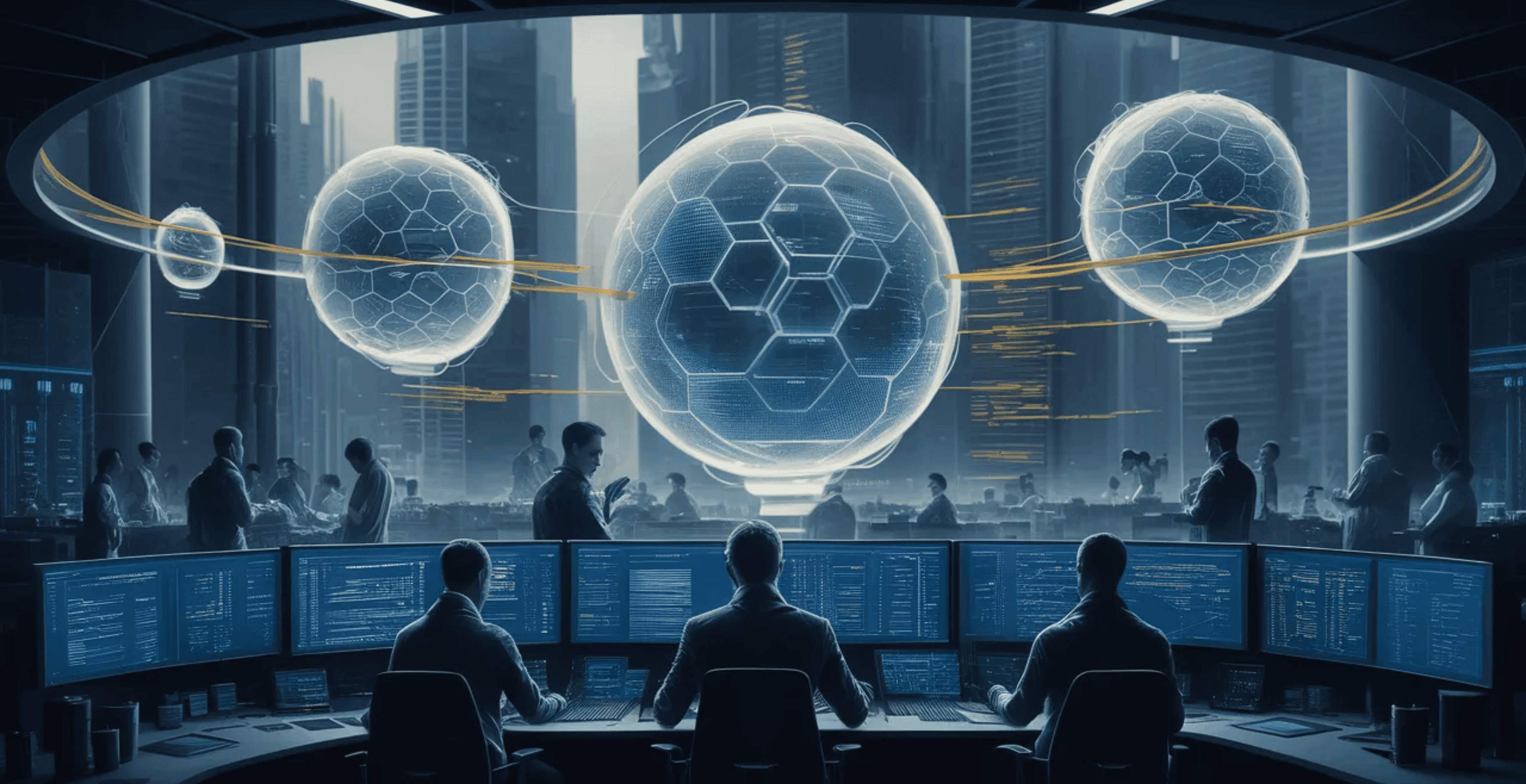
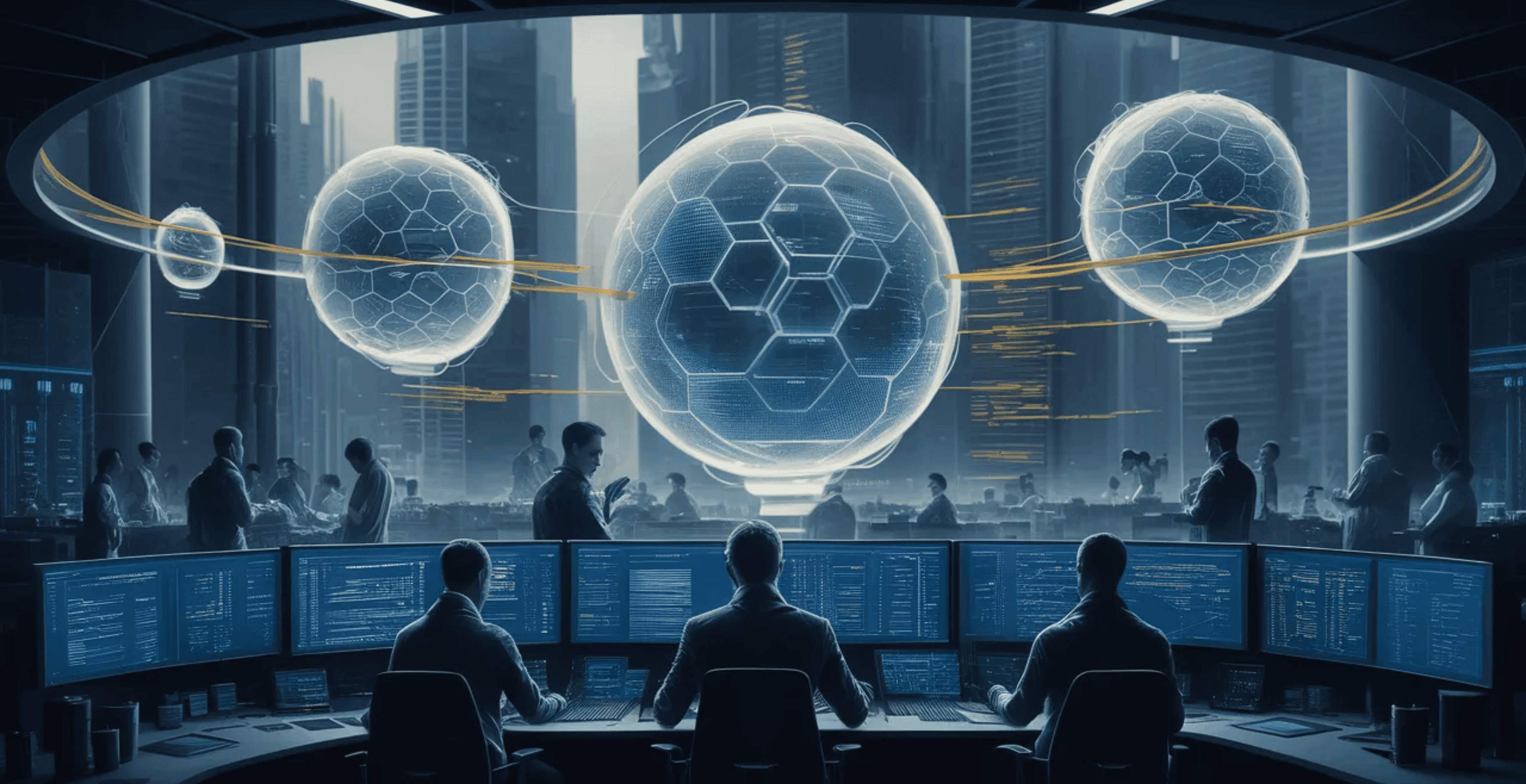
Practical Guide For Deploying LLMs In Production

Rehan Asif
Jun 12, 2024
Read the article
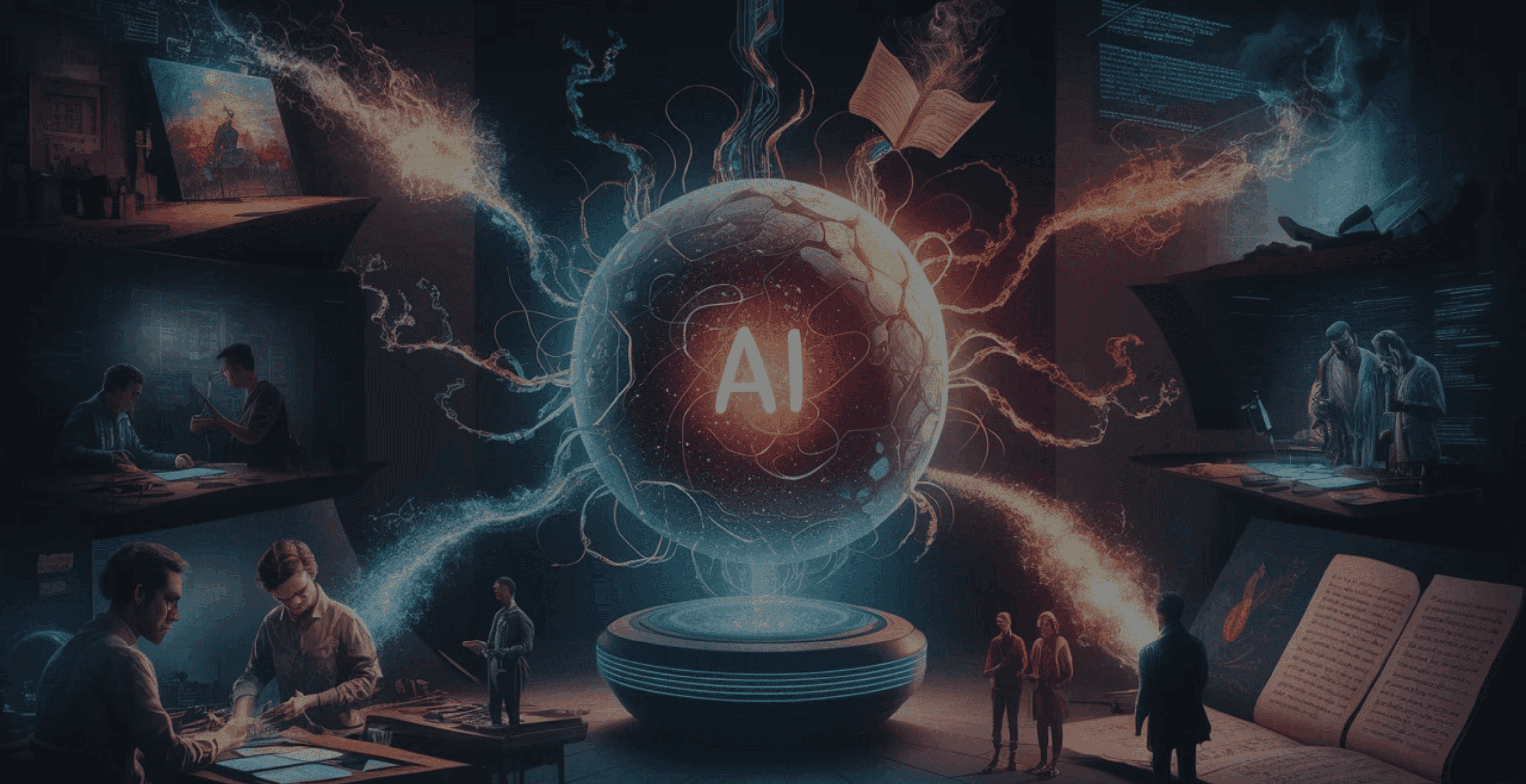
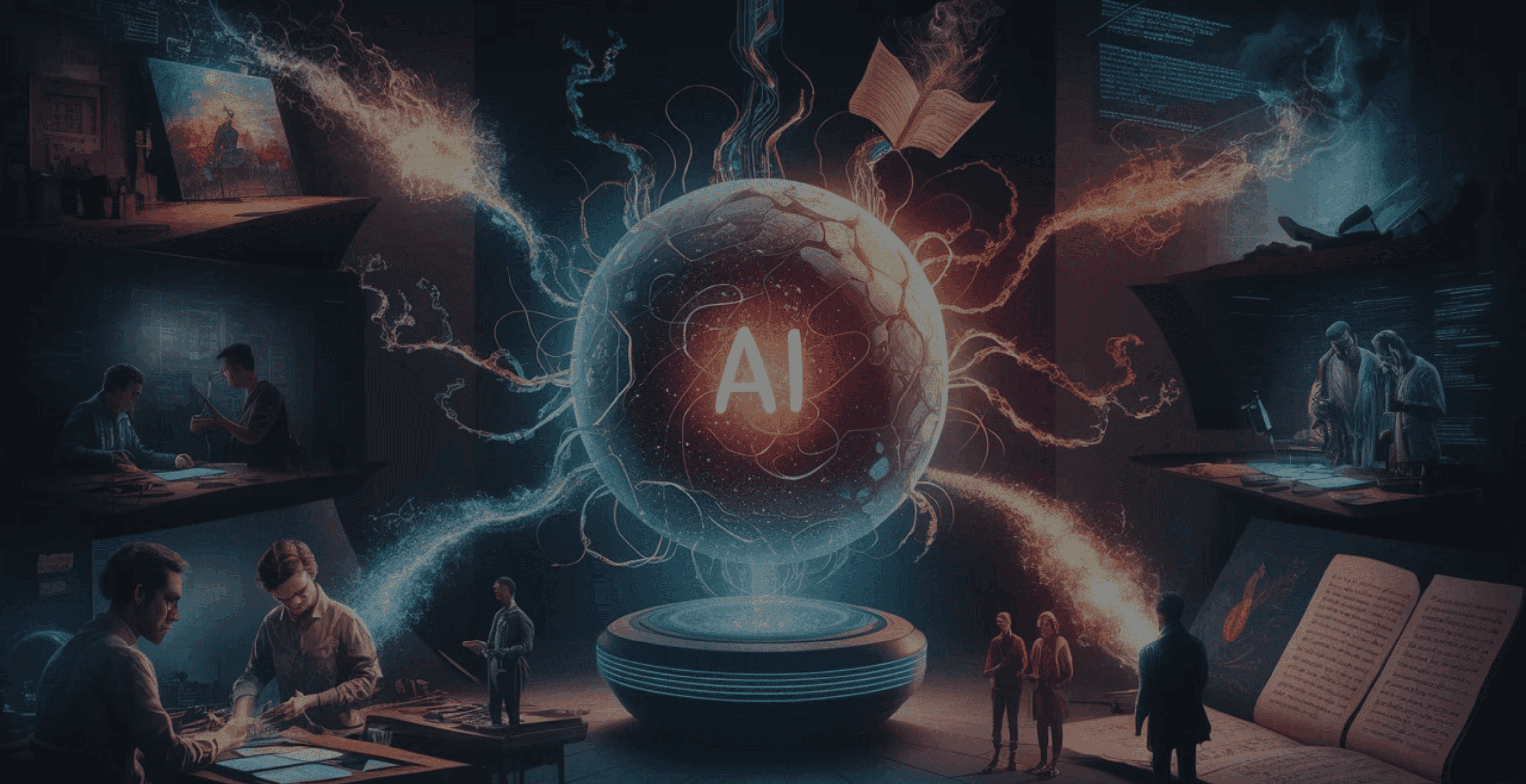
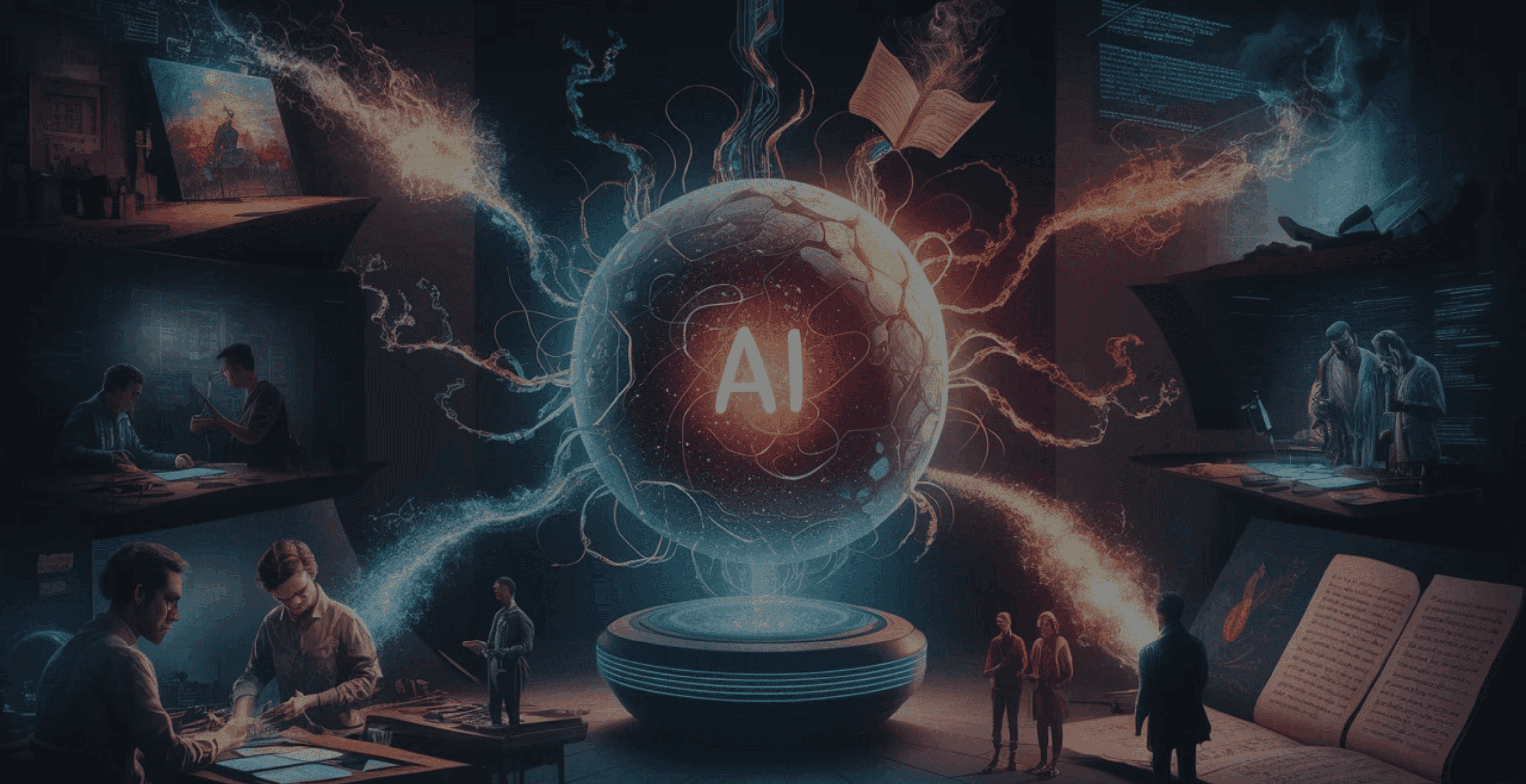
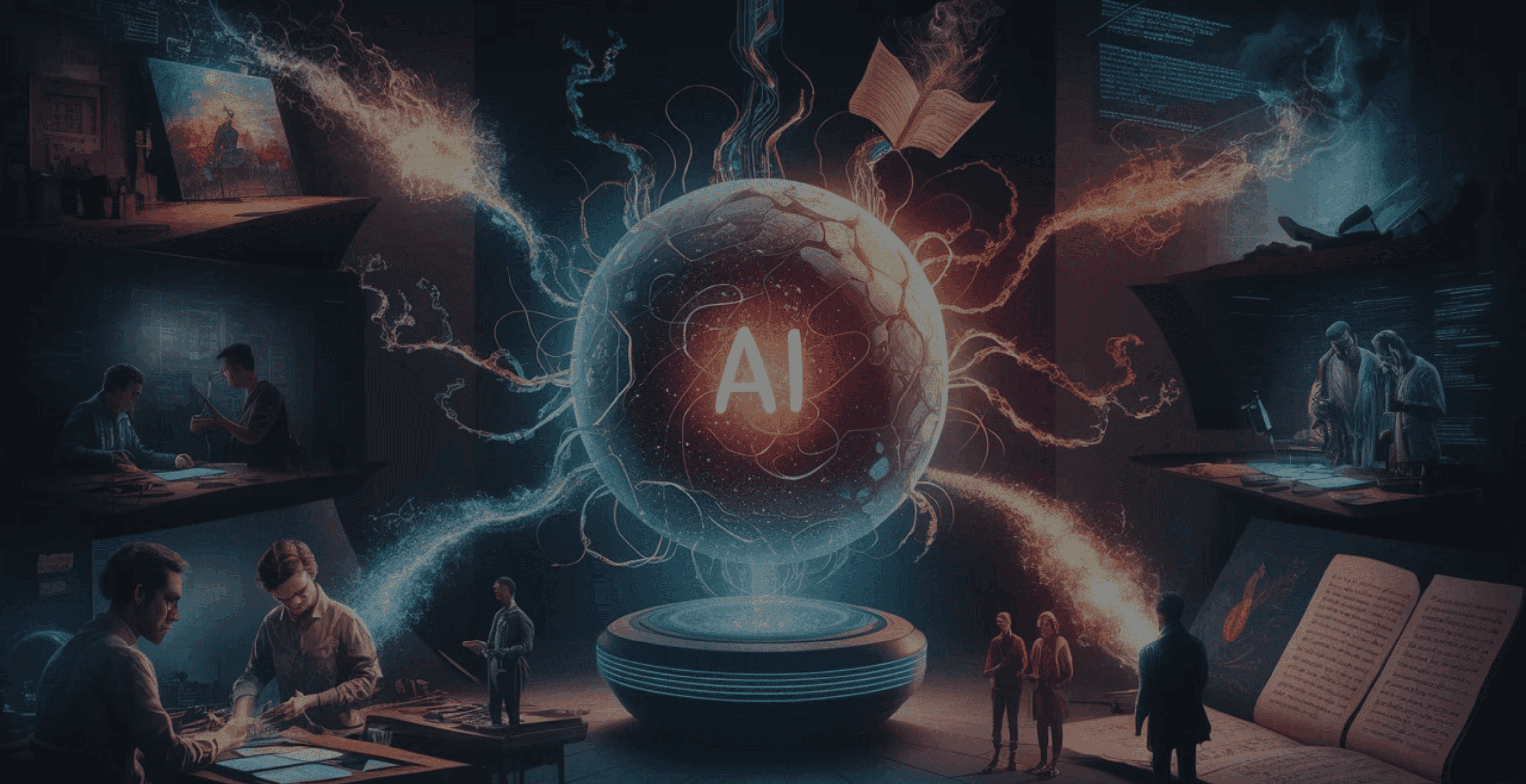
The Impact Of Generative Models On Content Creation
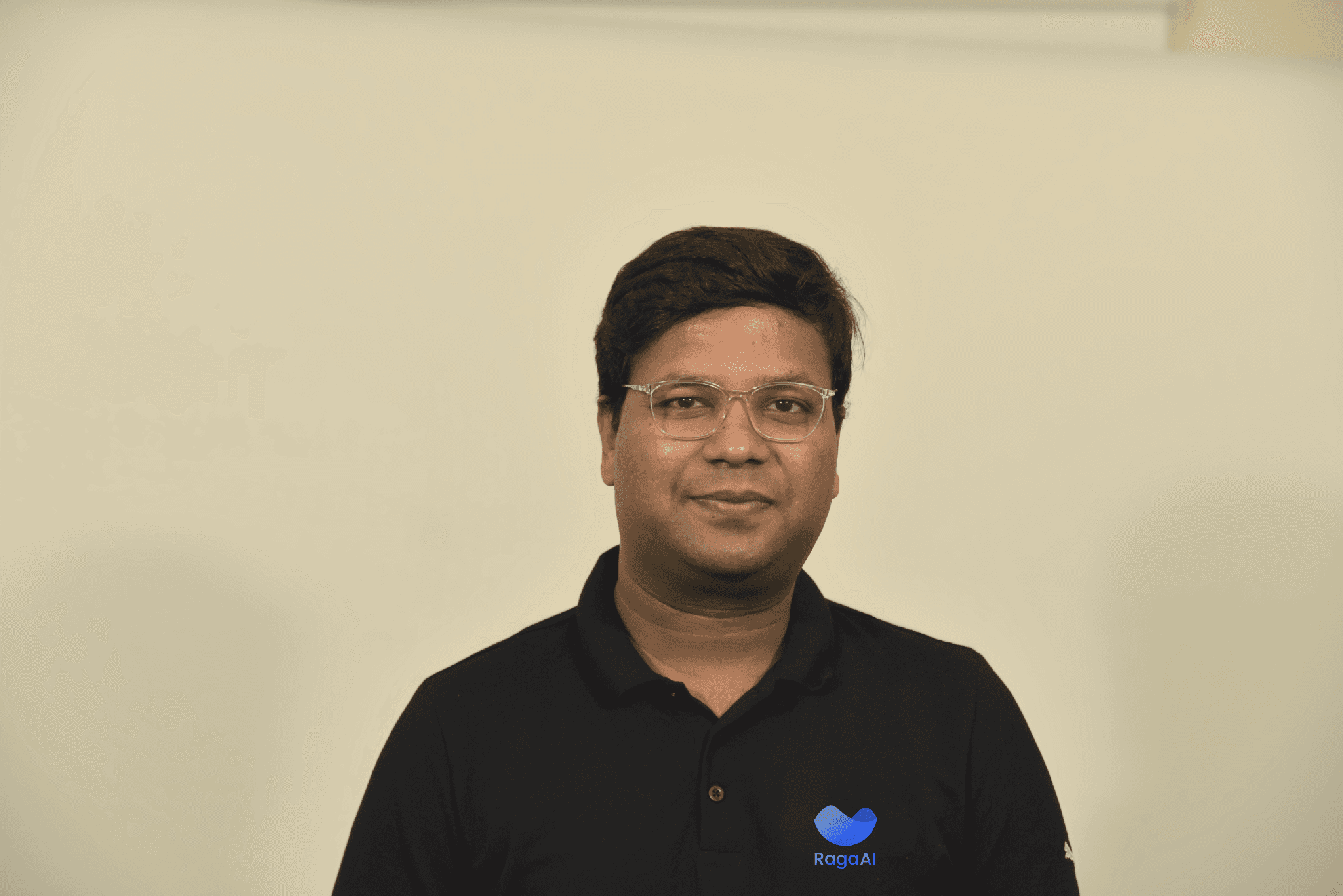
Jigar Gupta
Jun 12, 2024
Read the article




Implementing Regression Tests In AI Development
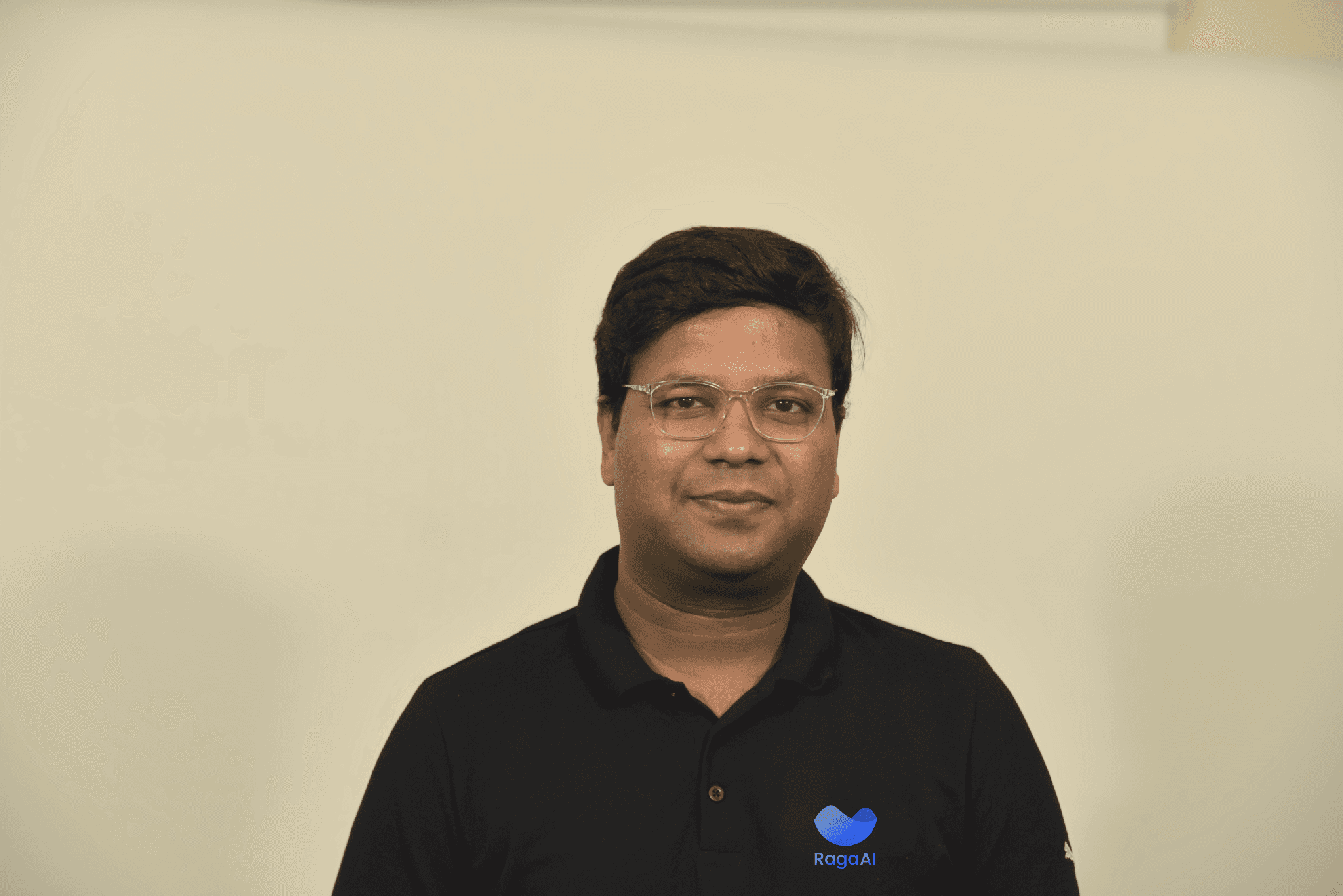
Jigar Gupta
Jun 12, 2024
Read the article
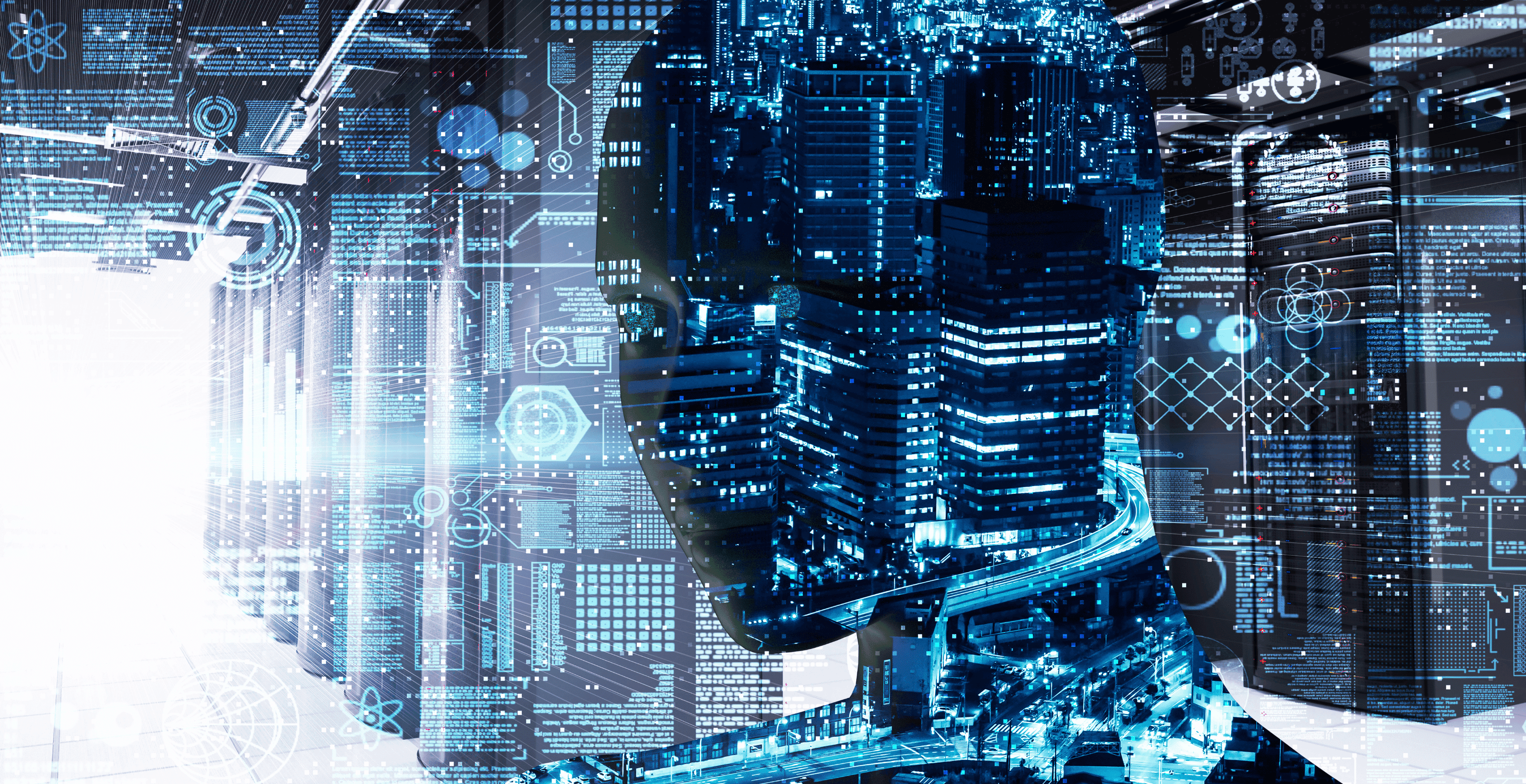
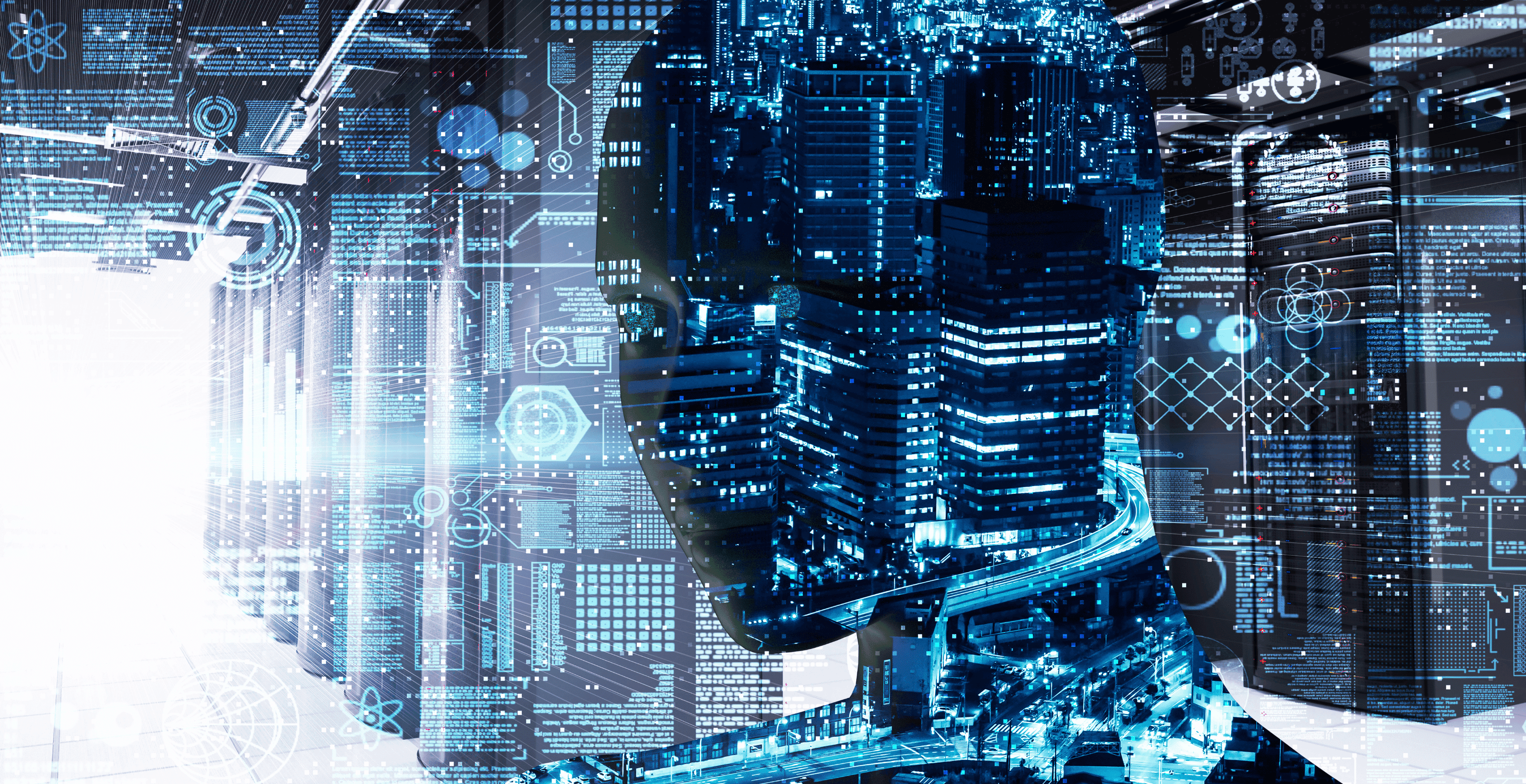
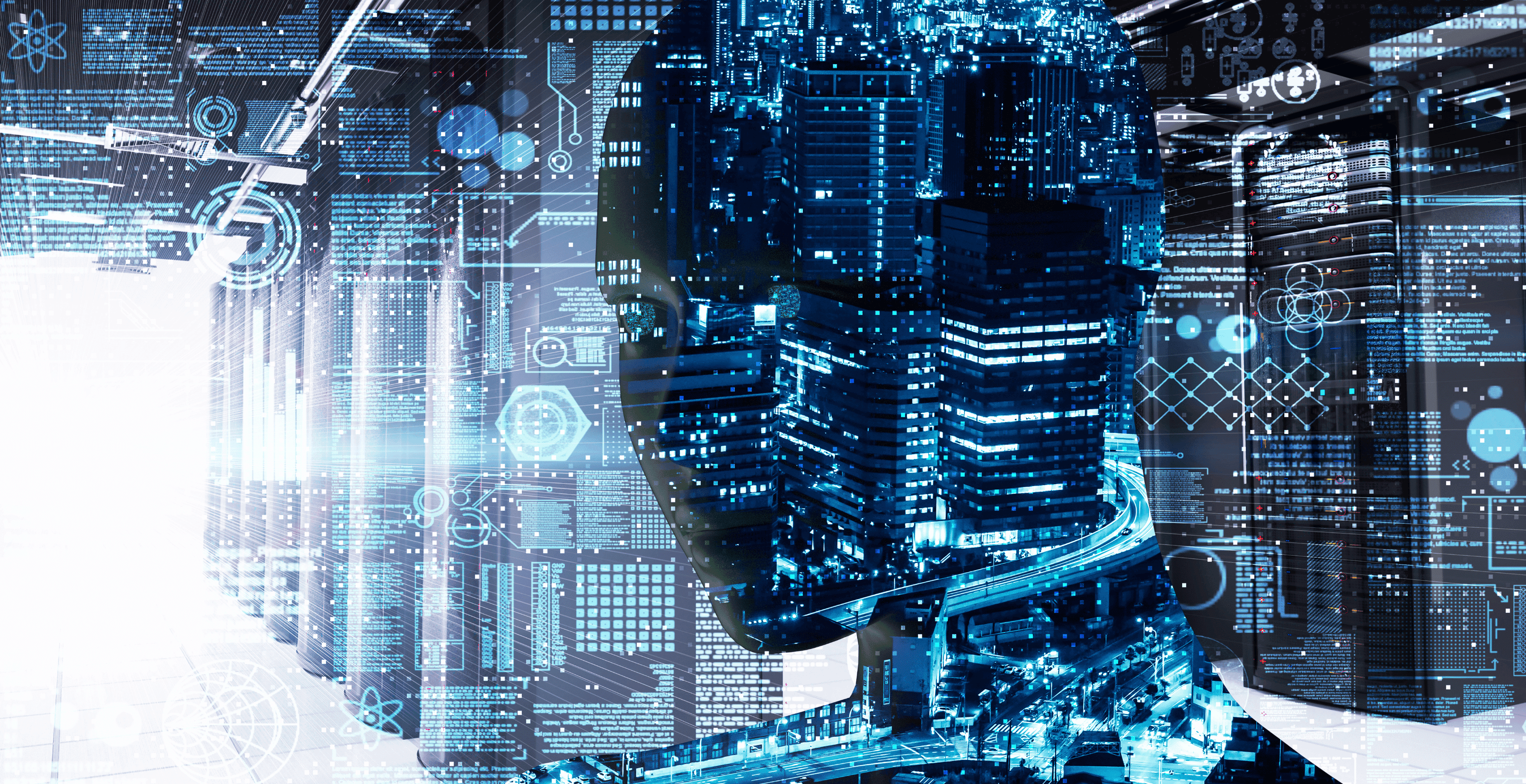
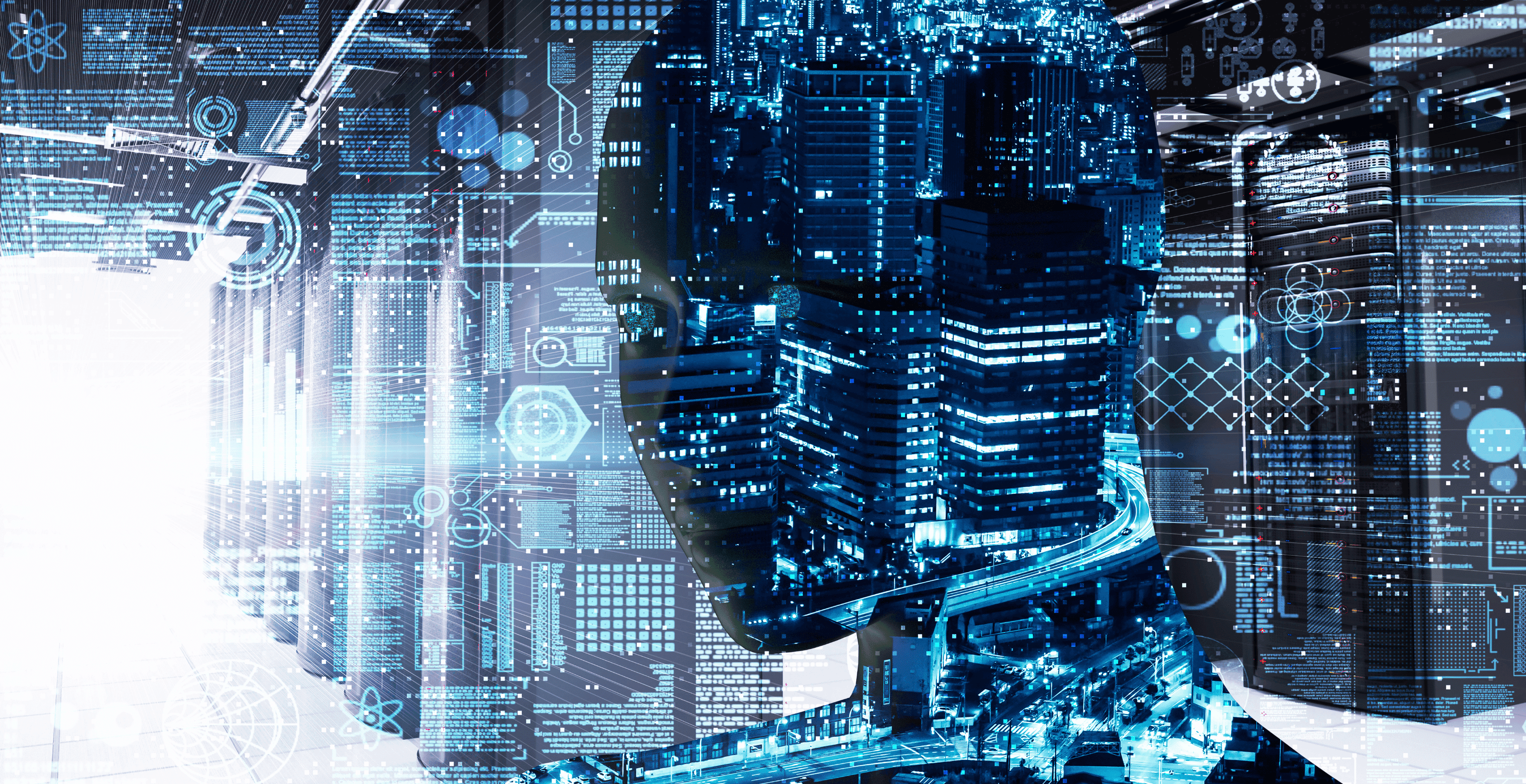
In-Depth Case Studies in AI Model Testing: Exploring Real-World Applications and Insights
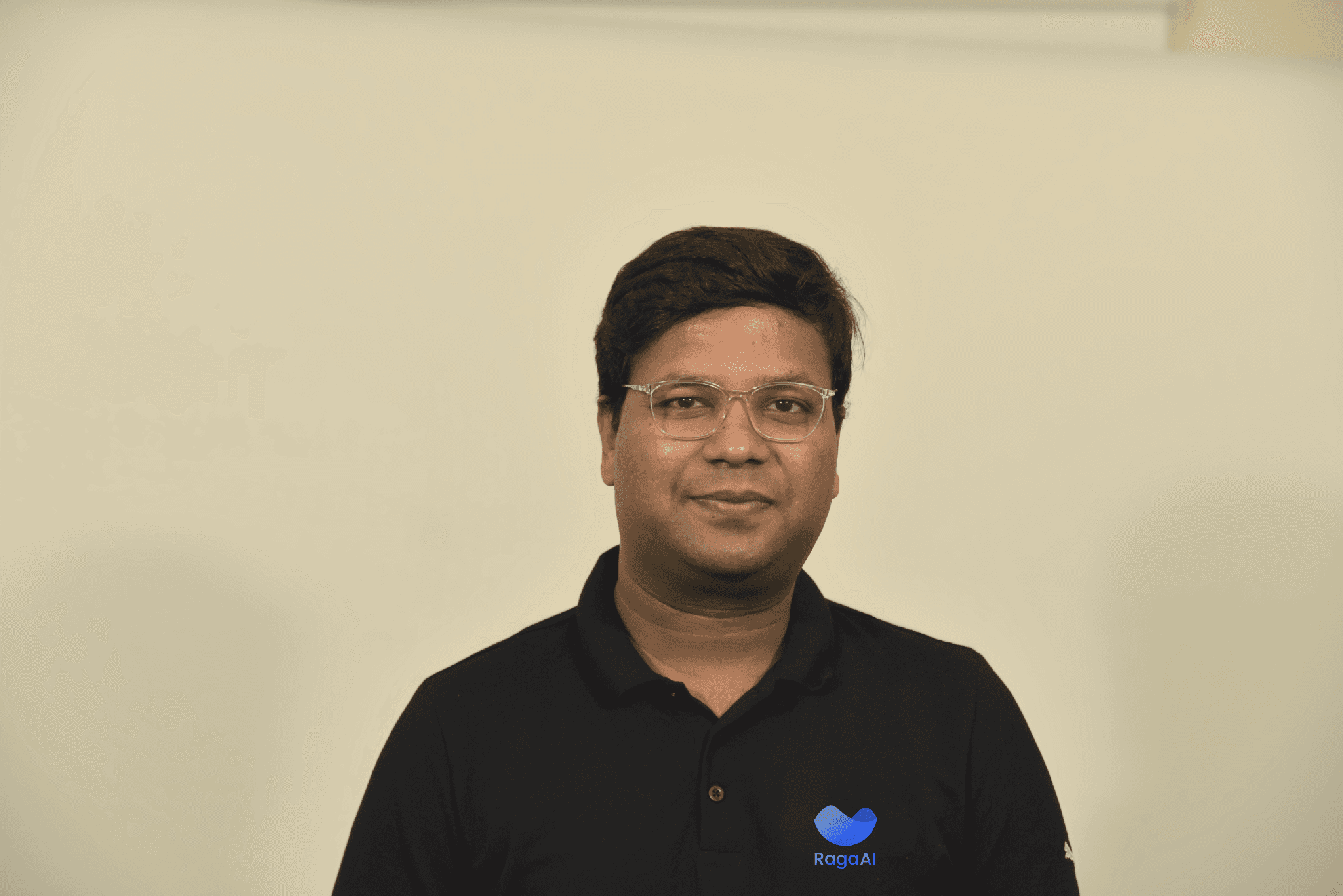
Jigar Gupta
Jun 11, 2024
Read the article




Techniques and Importance of Stress Testing AI Systems
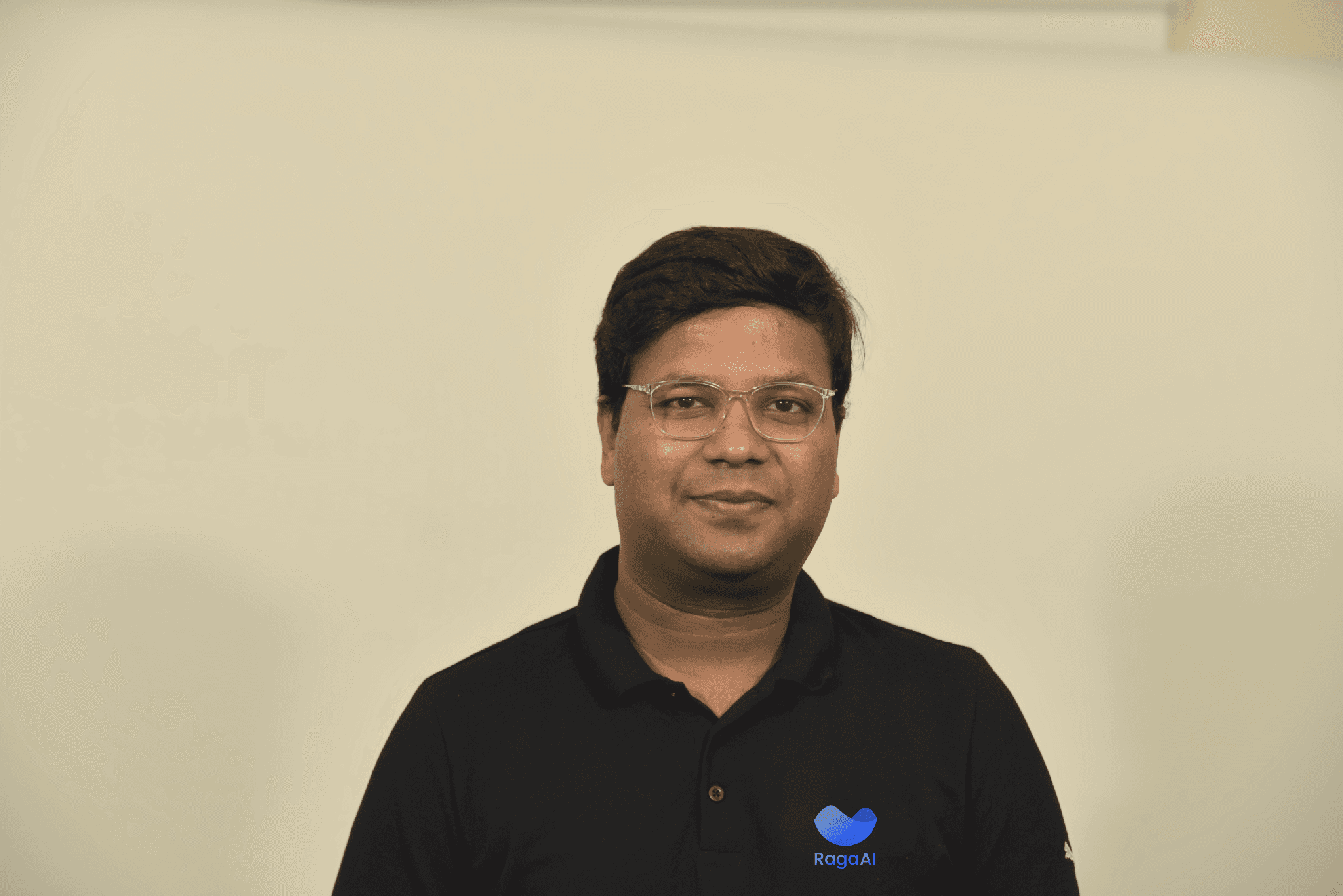
Jigar Gupta
Jun 11, 2024
Read the article
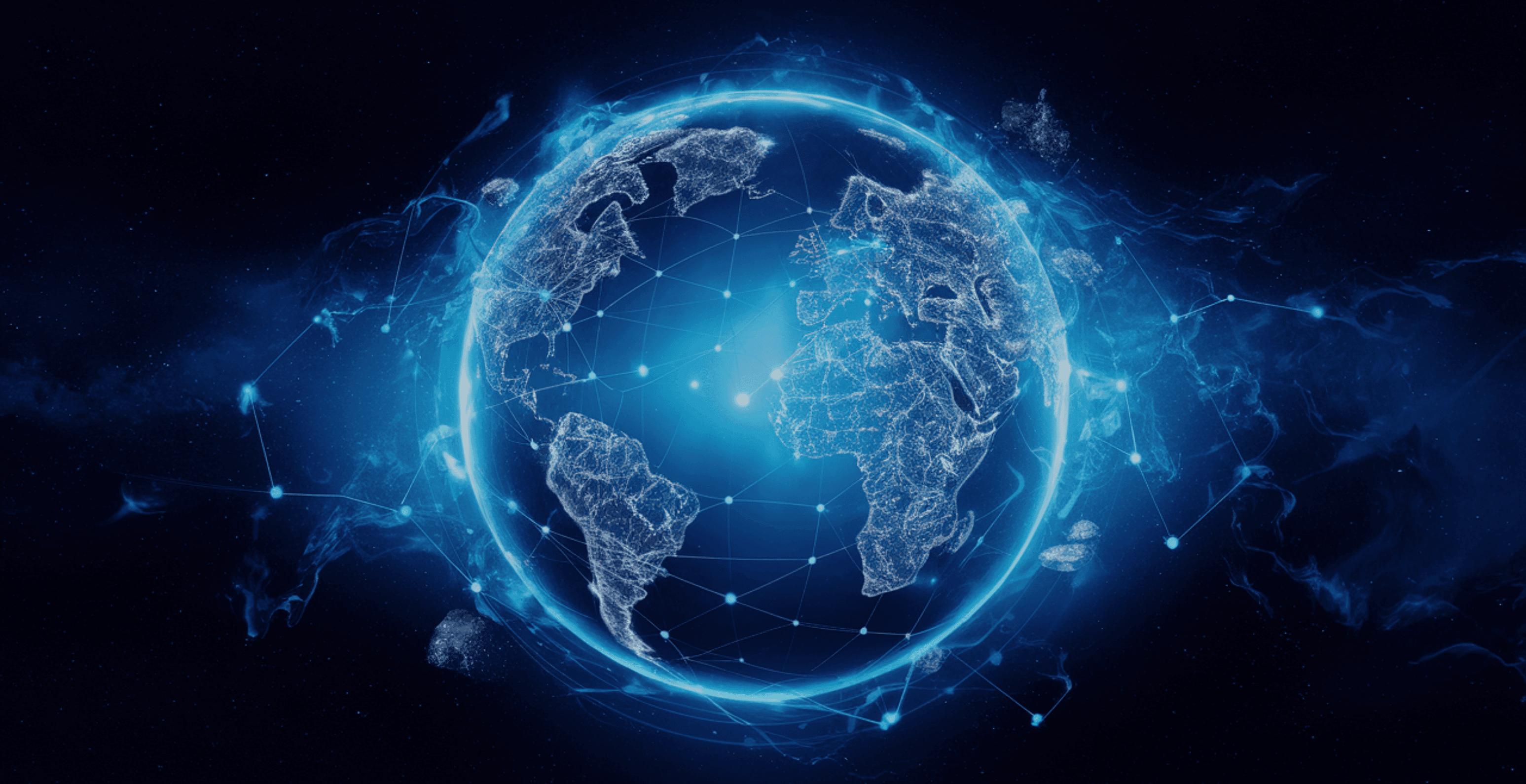
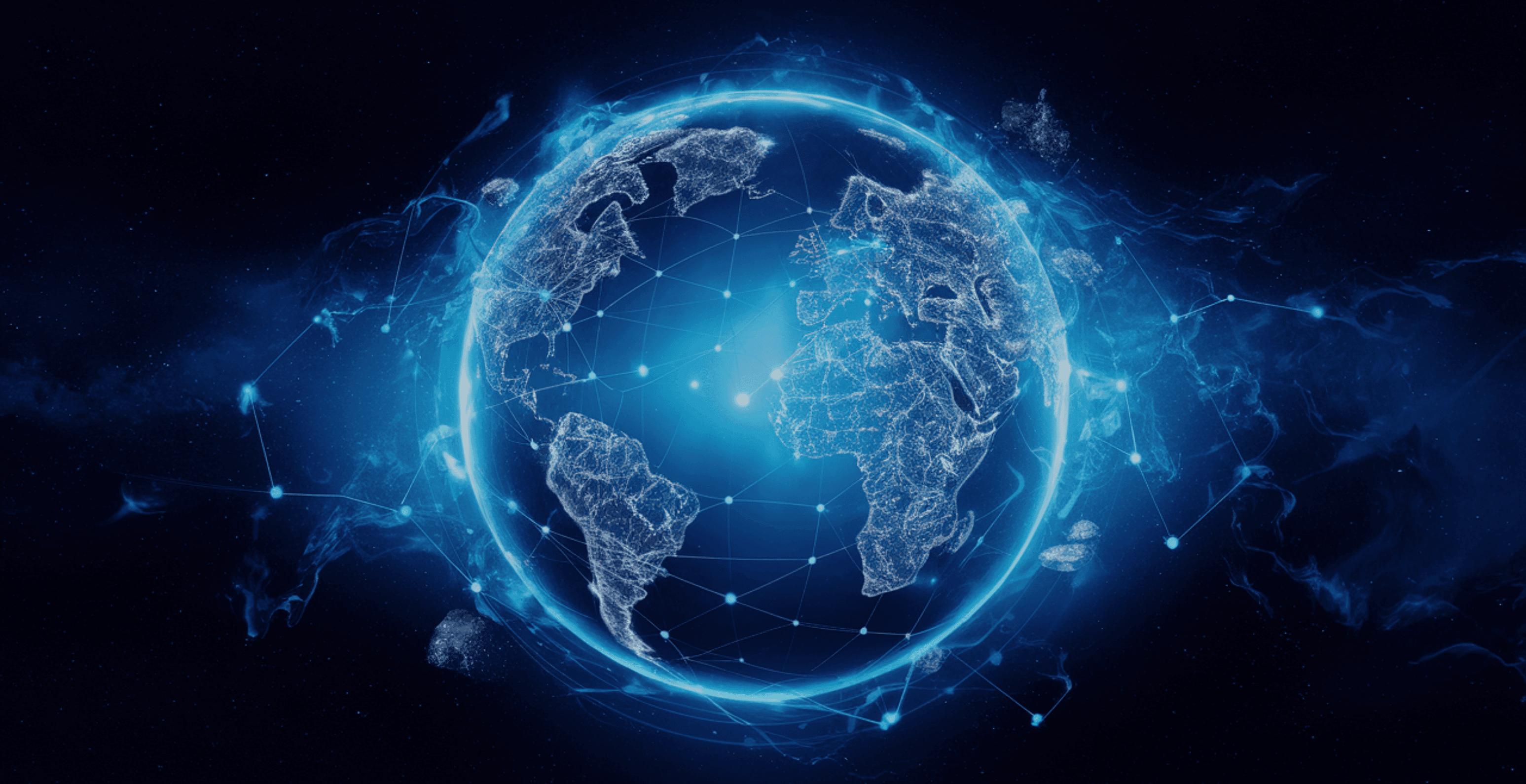
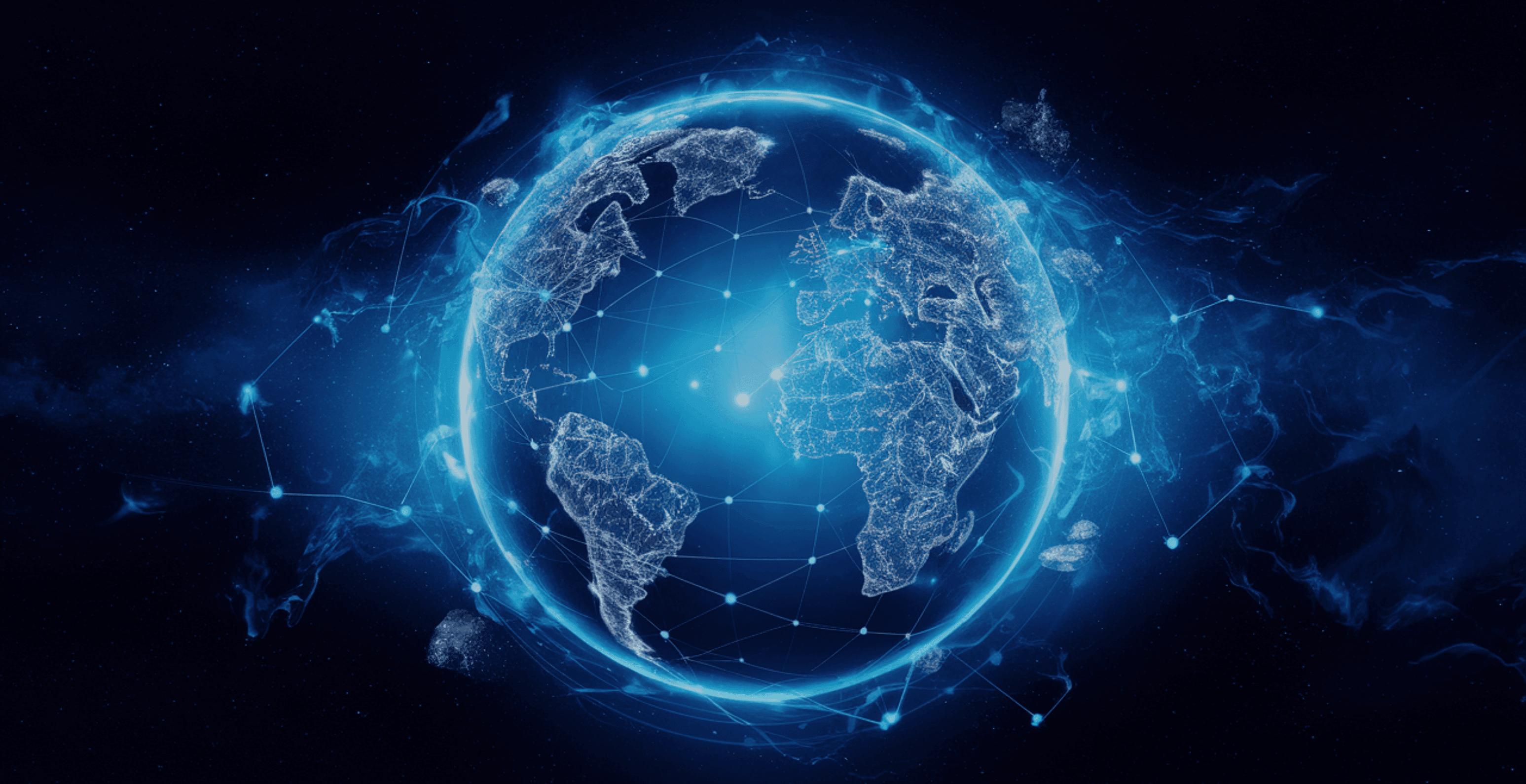
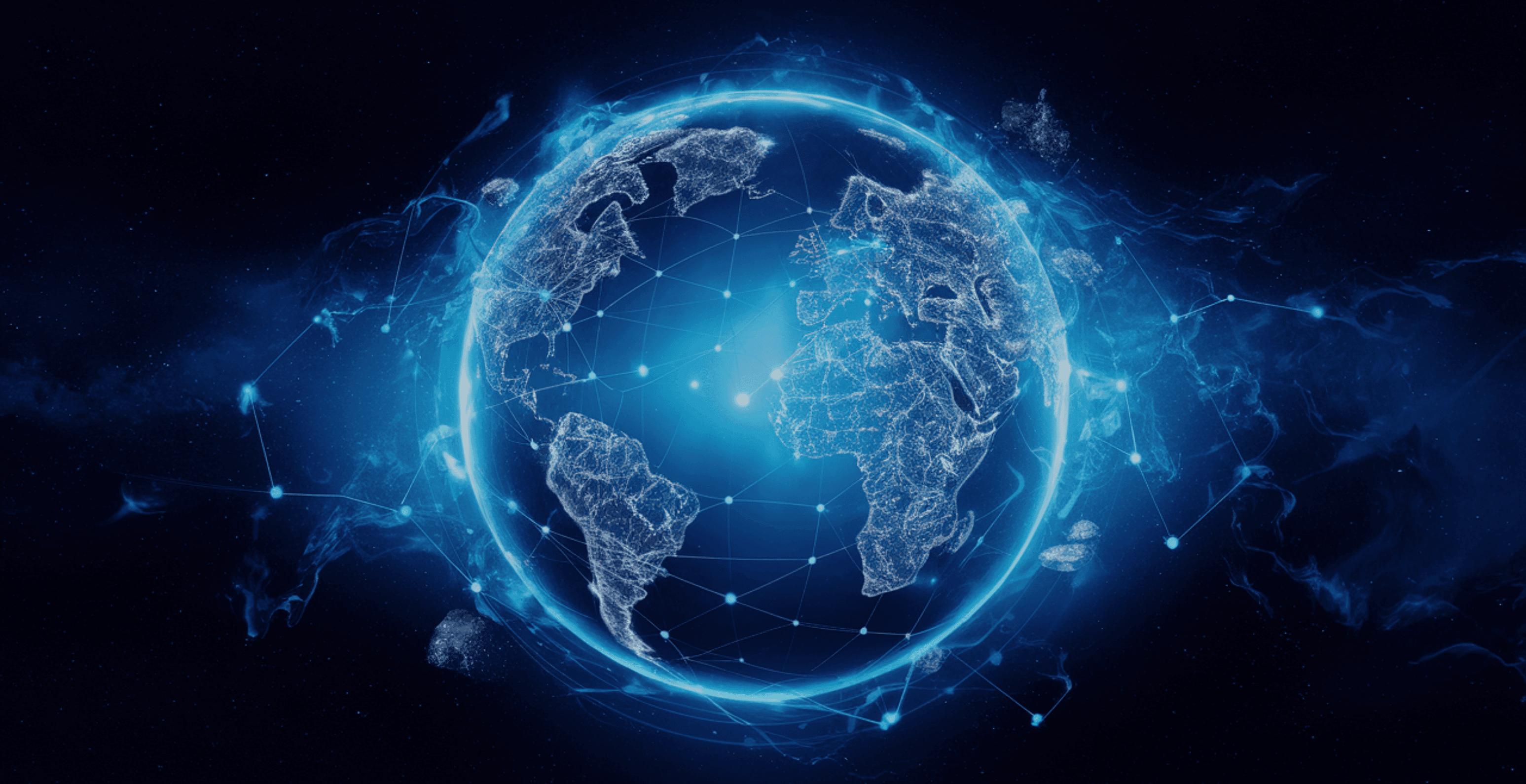
Navigating Global AI Regulations and Standards

Rehan Asif
Jun 10, 2024
Read the article




The Cost of Errors In AI Application Development

Rehan Asif
Jun 10, 2024
Read the article
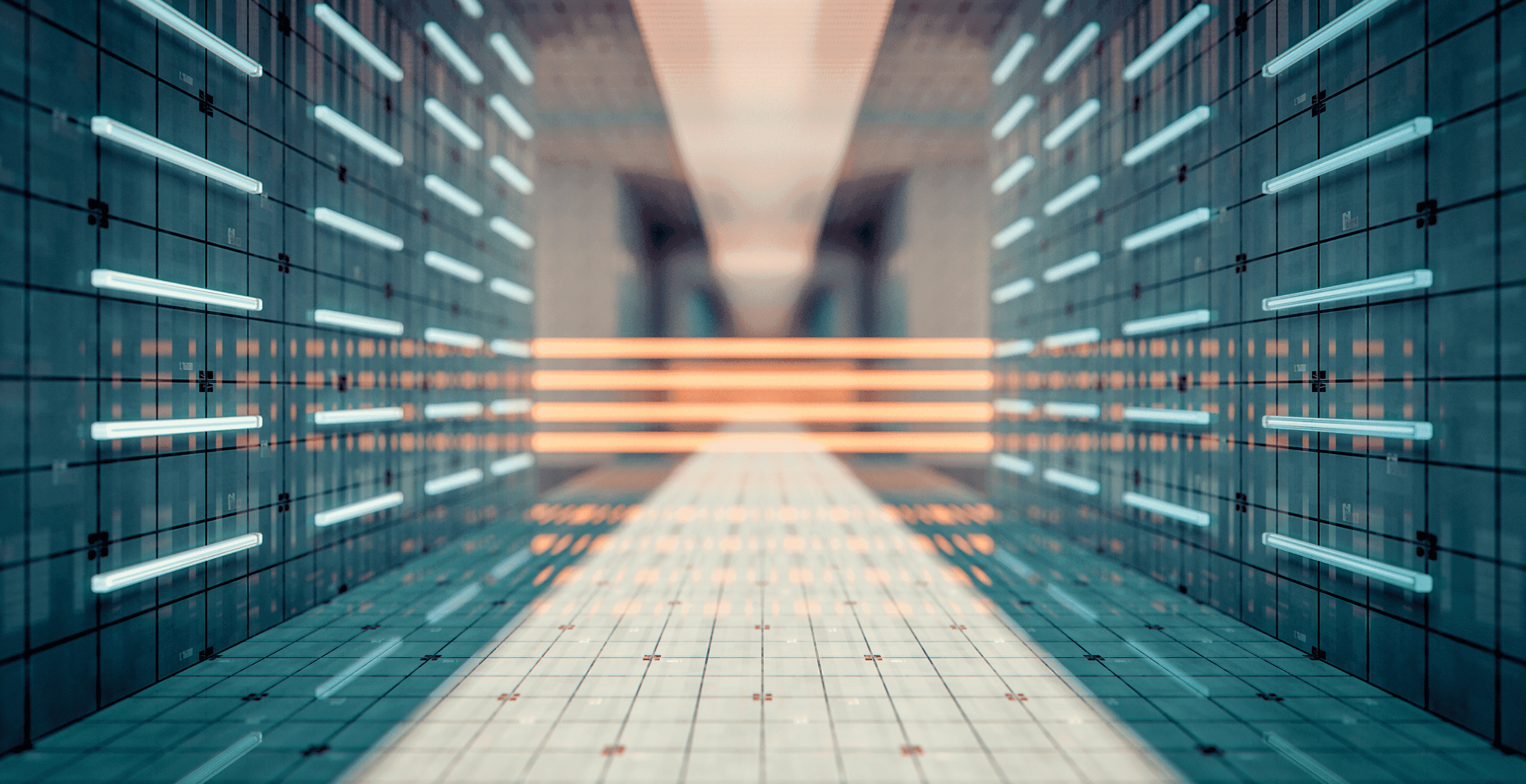
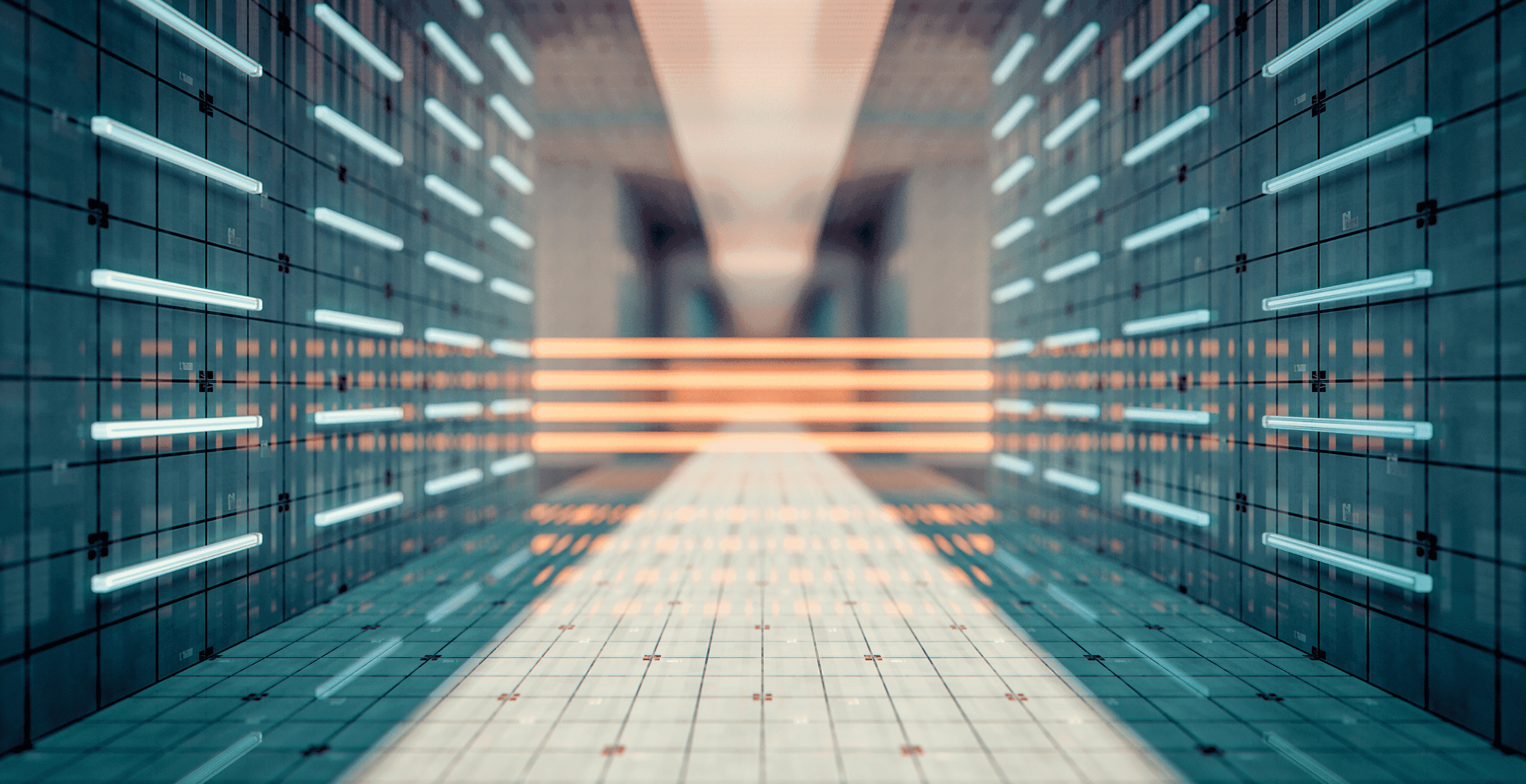
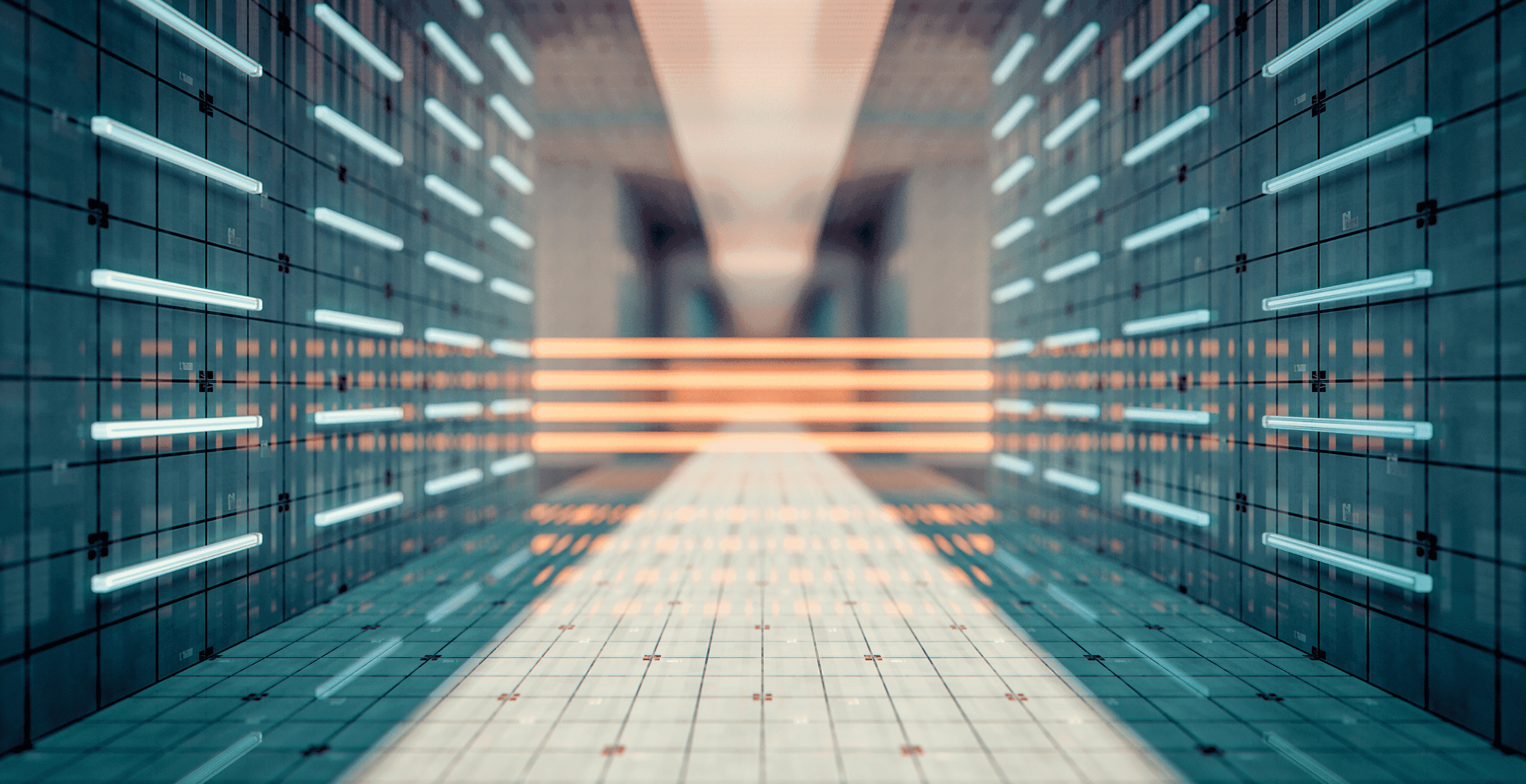
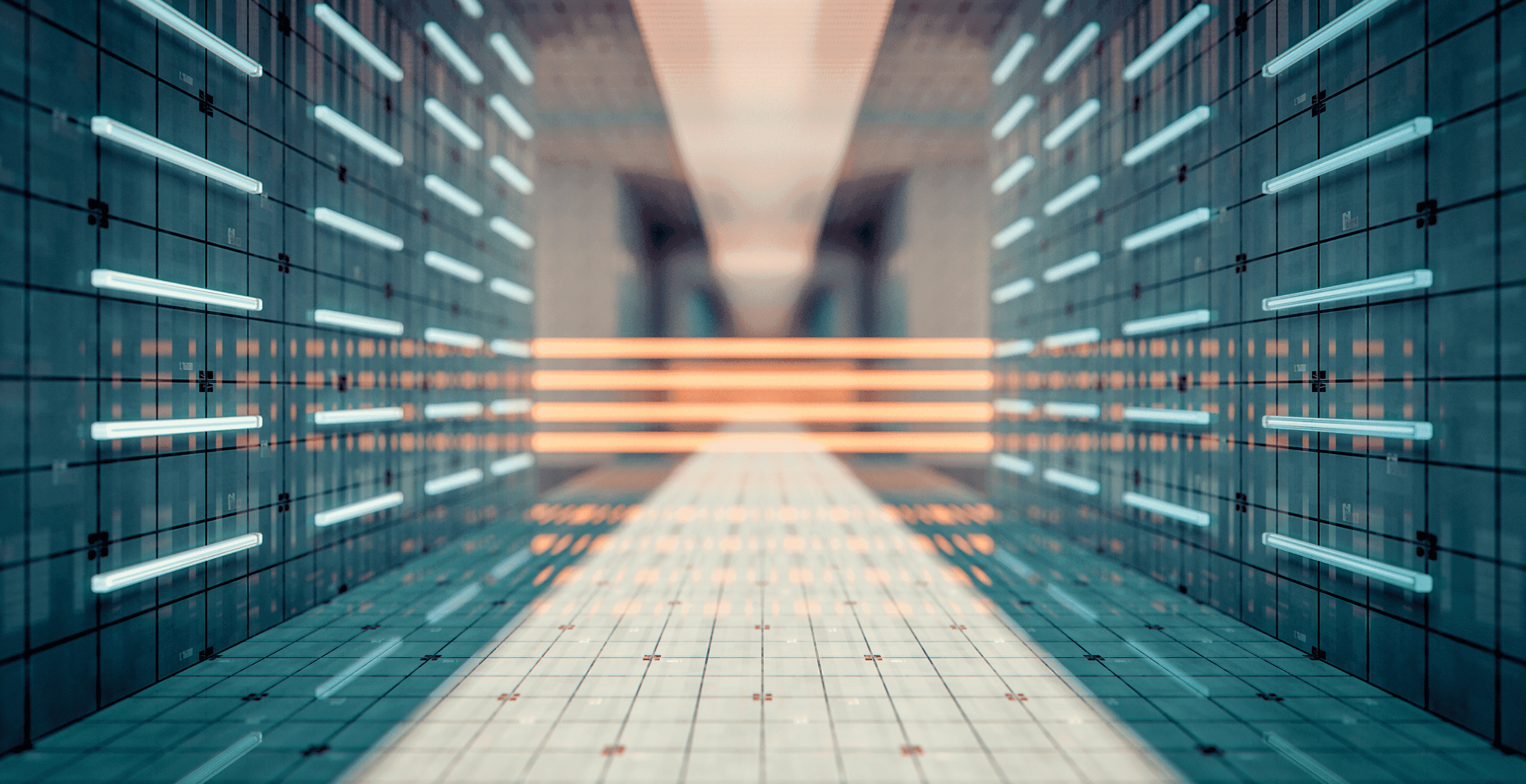
Best Practices In Data Governance For AI

Rehan Asif
Jun 10, 2024
Read the article




Success Stories And Case Studies Of AI Adoption Across Industries
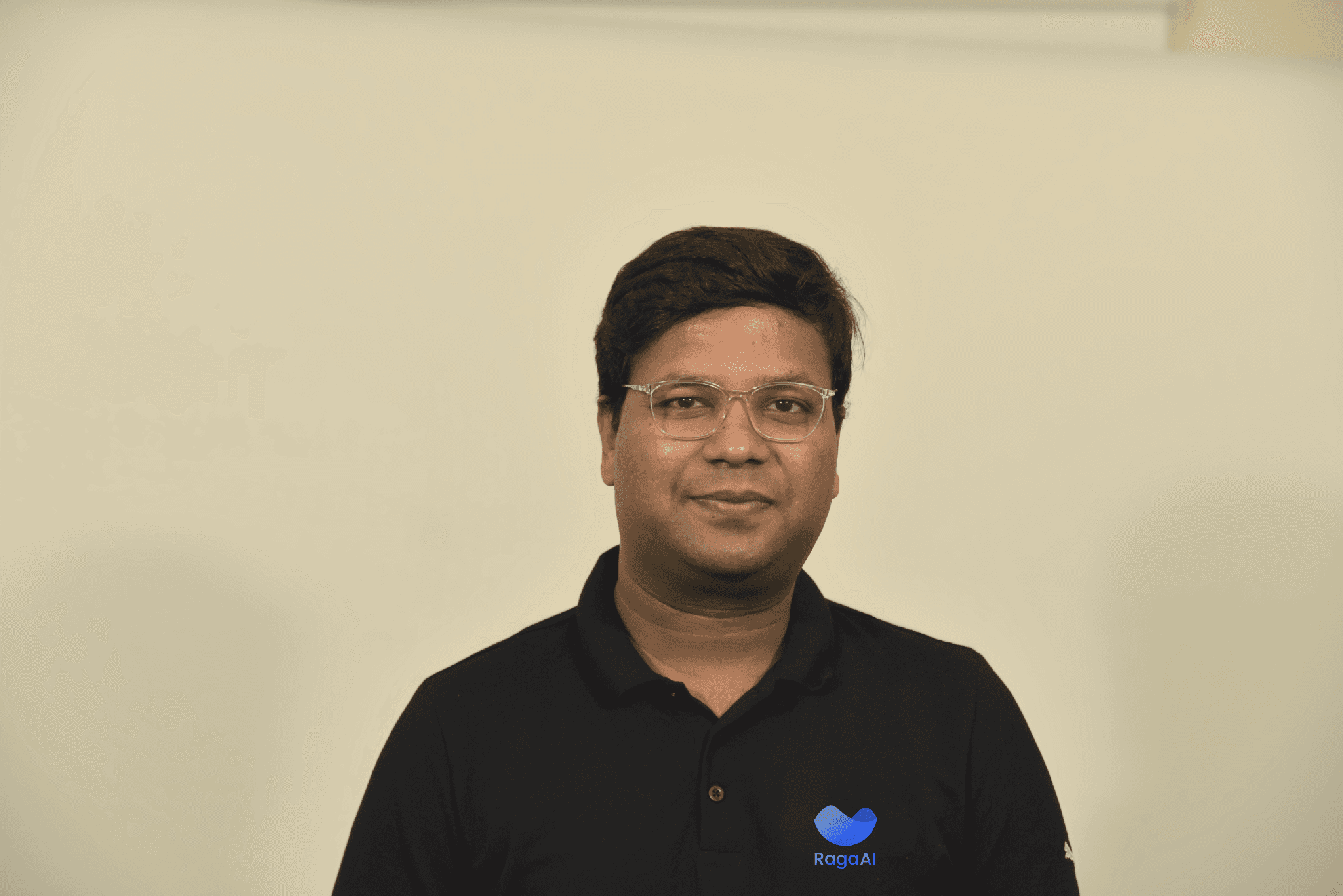
Jigar Gupta
May 1, 2024
Read the article
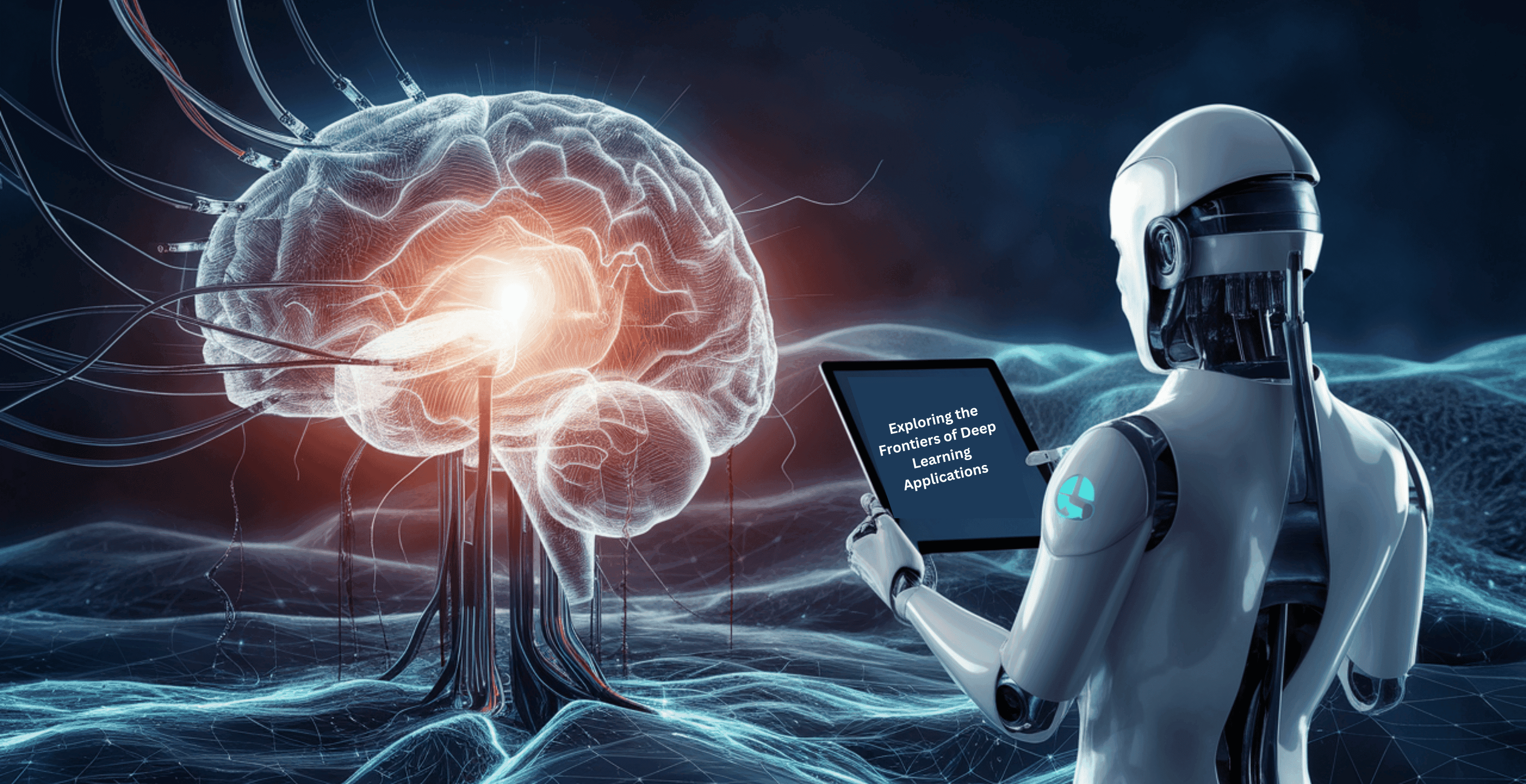
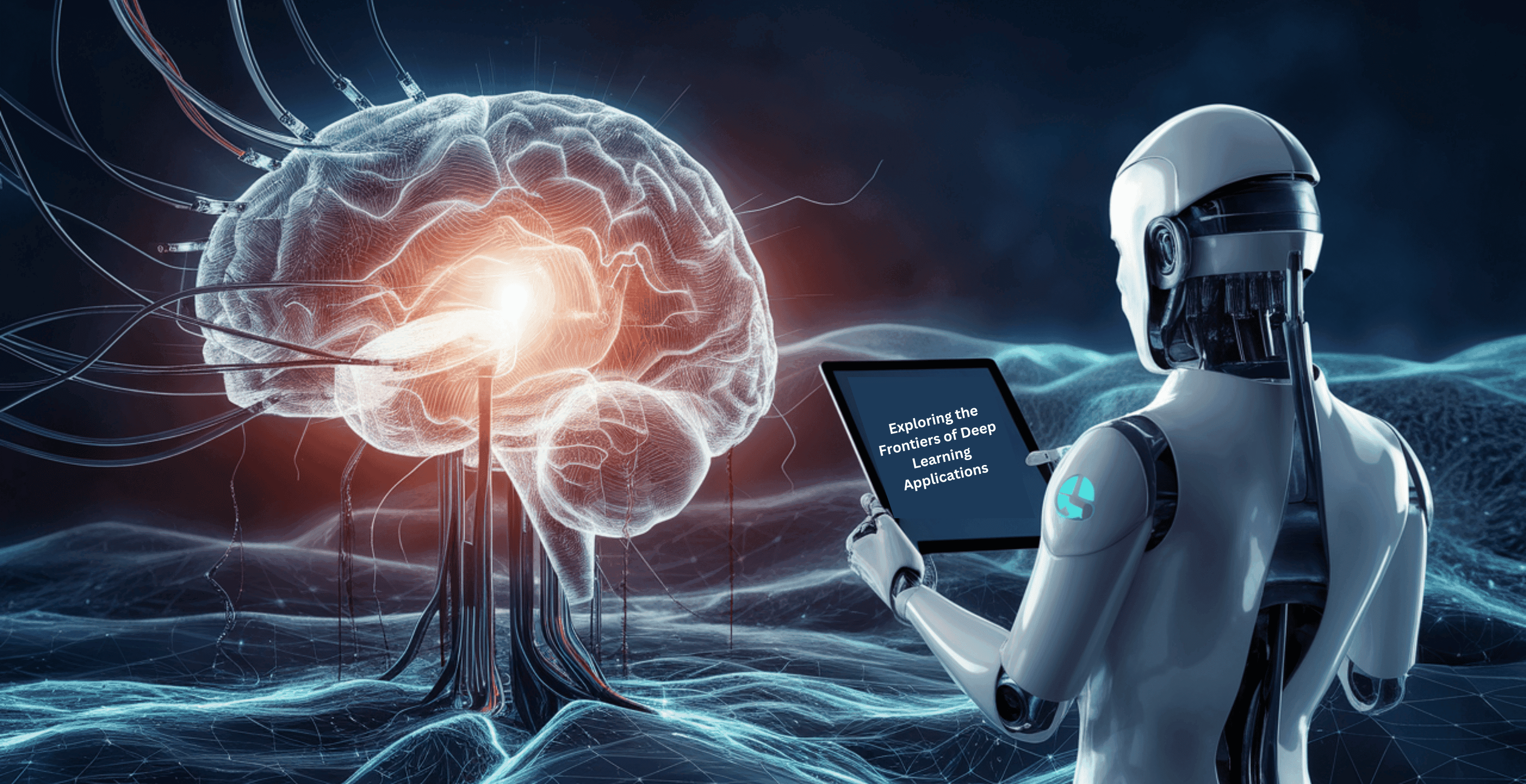
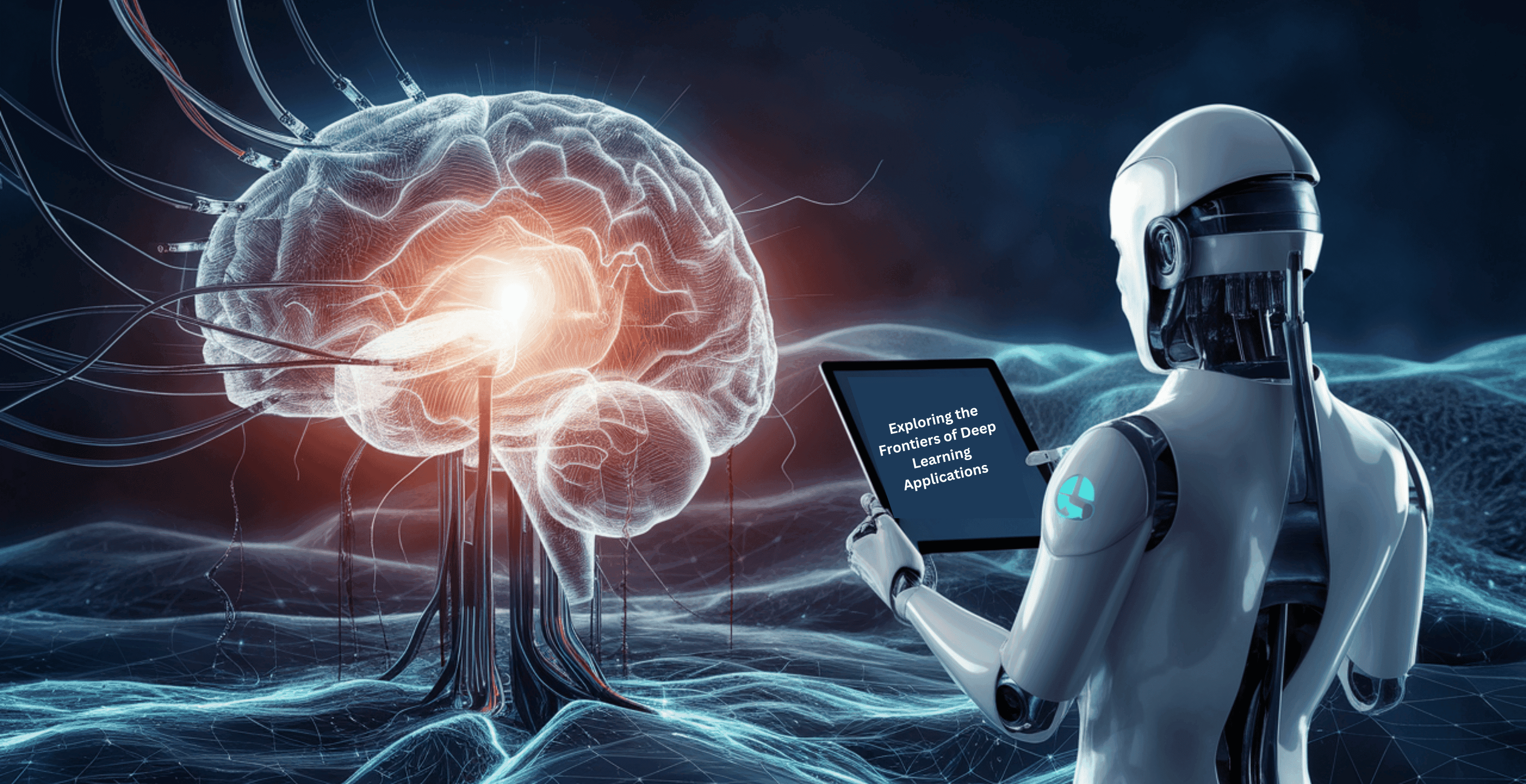
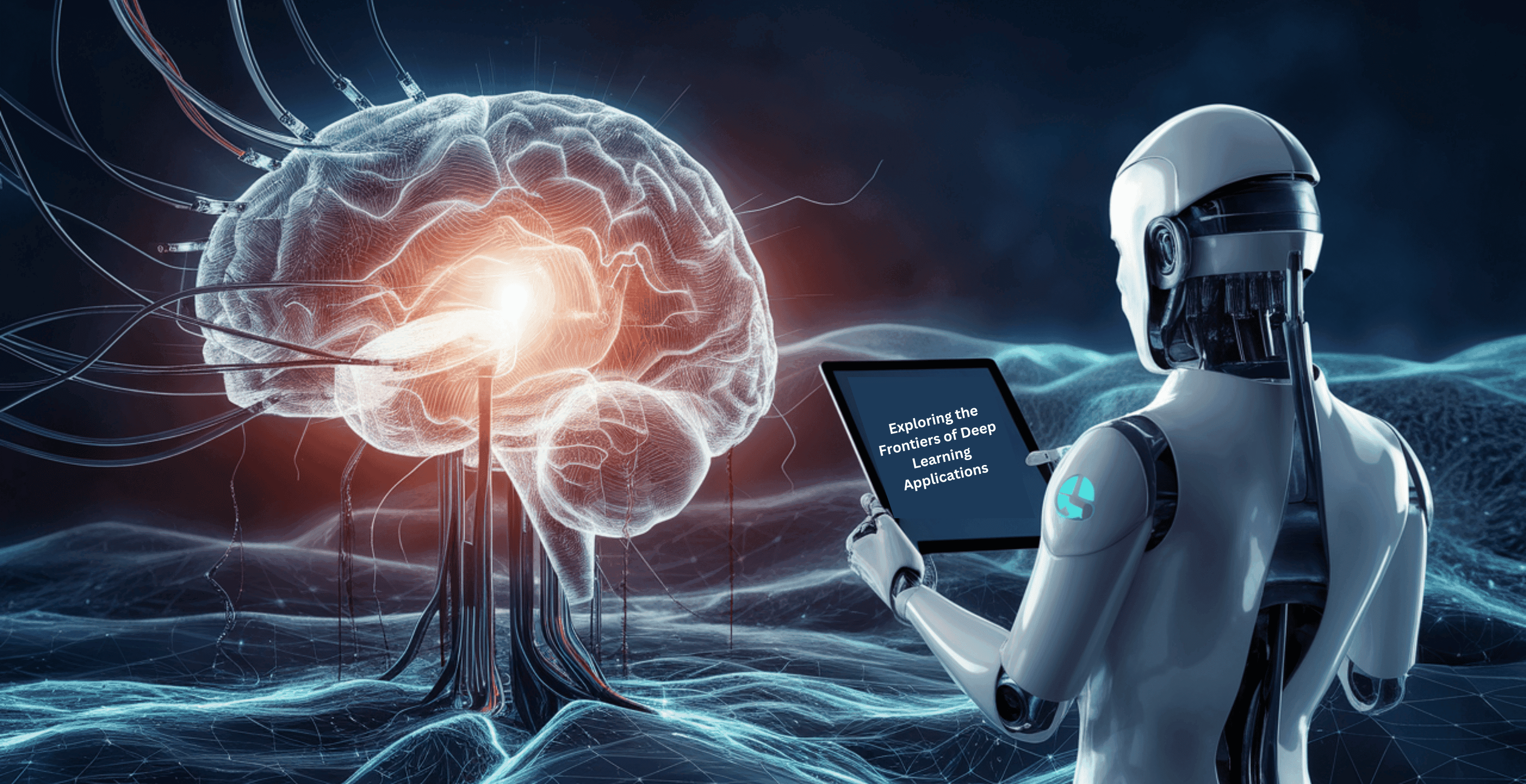
Exploring The Frontiers Of Deep Learning Applications
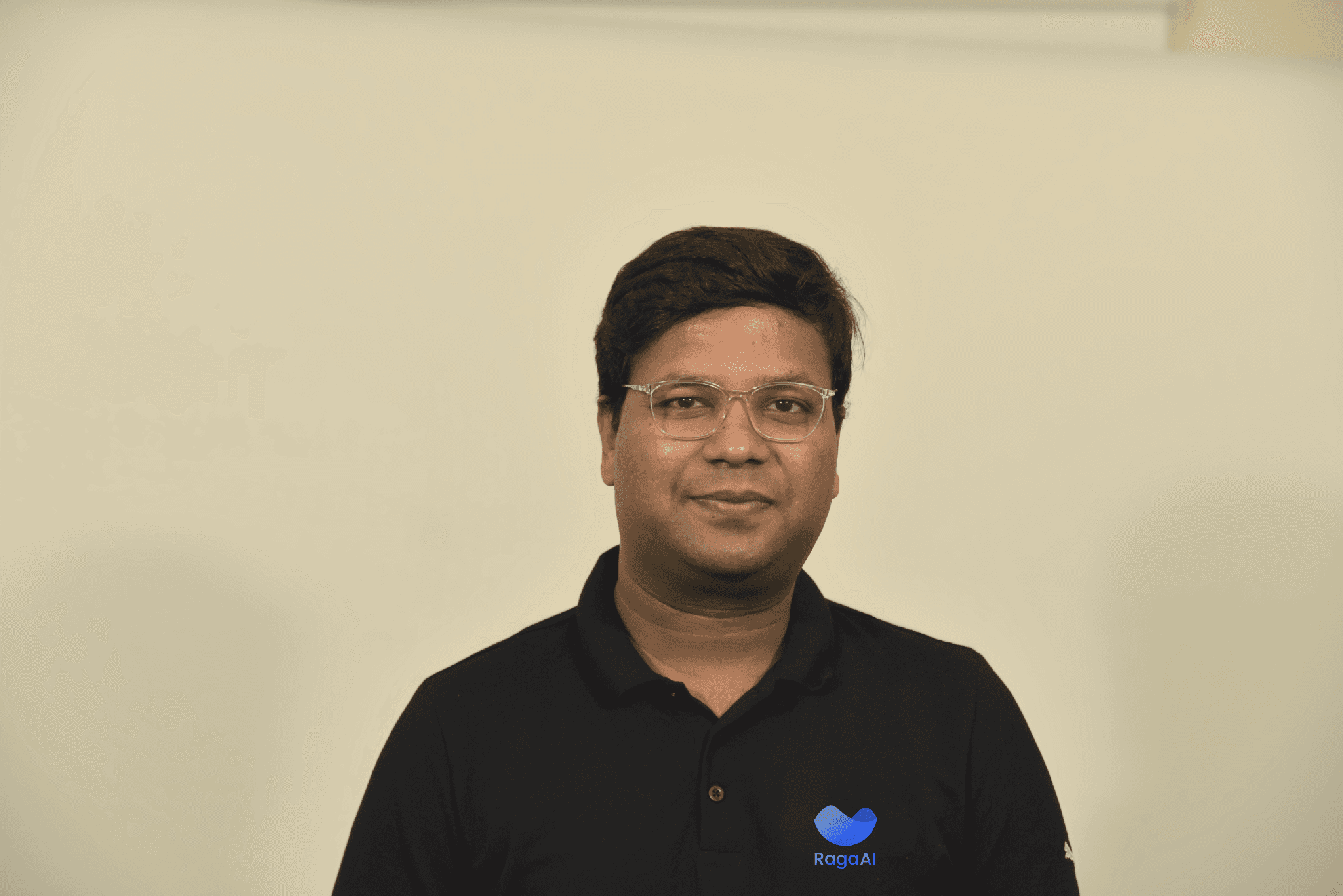
Jigar Gupta
May 1, 2024
Read the article




Integration Of RAG Platforms With Existing Enterprise Systems
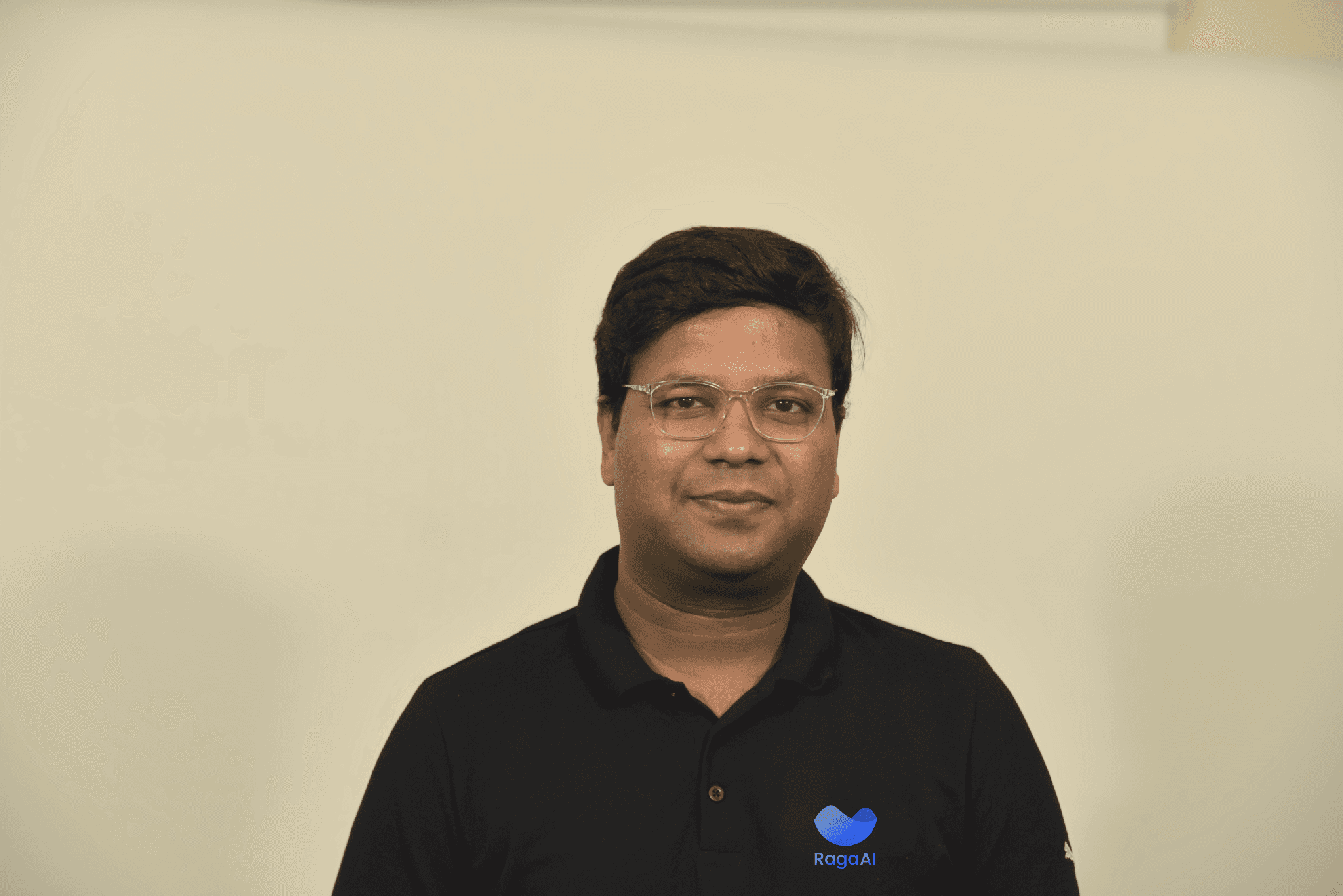
Jigar Gupta
Apr 30, 2024
Read the article




Multimodal LLMS Using Image And Text

Rehan Asif
Apr 30, 2024
Read the article




Understanding ML Model Monitoring In Production

Rehan Asif
Apr 30, 2024
Read the article
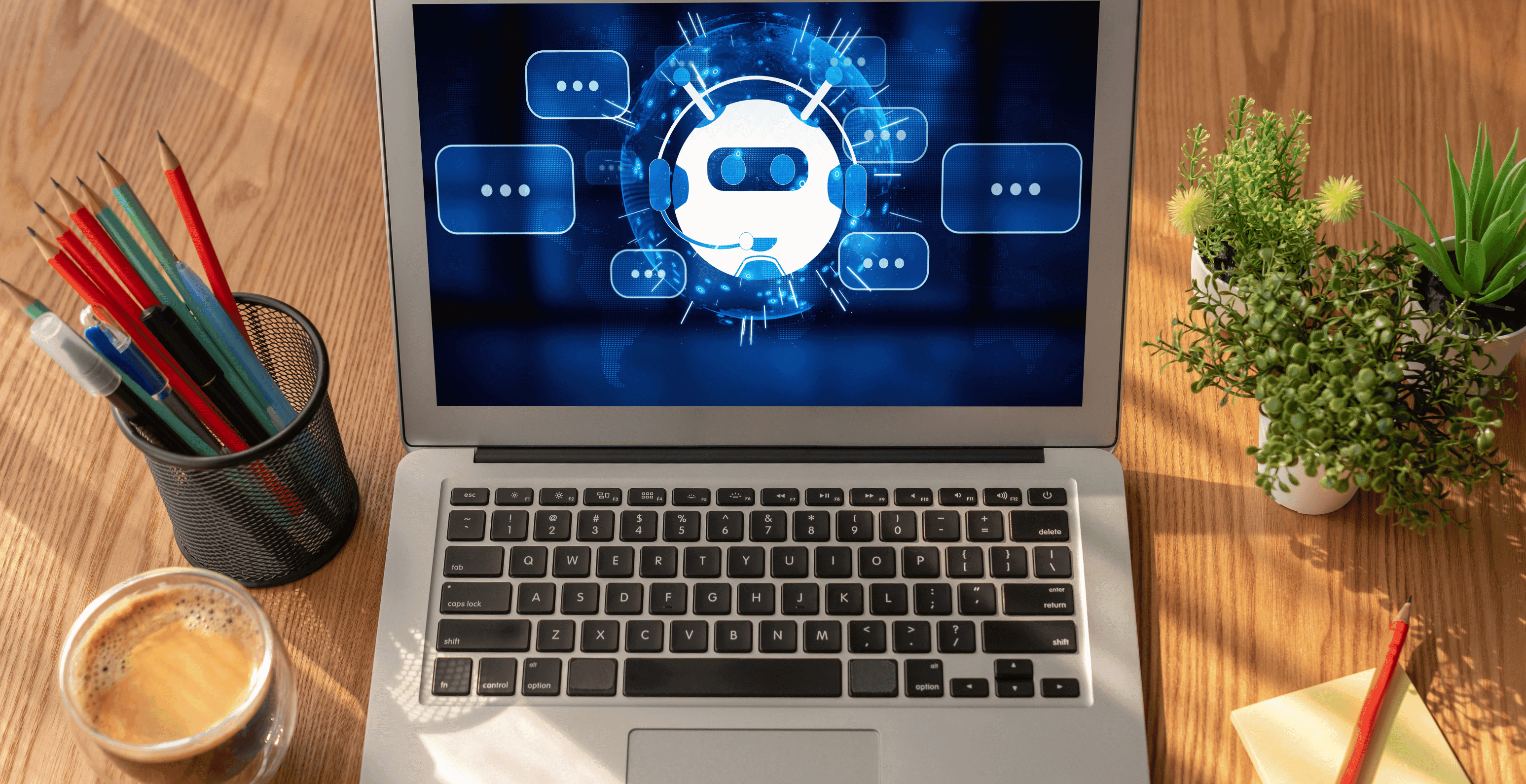
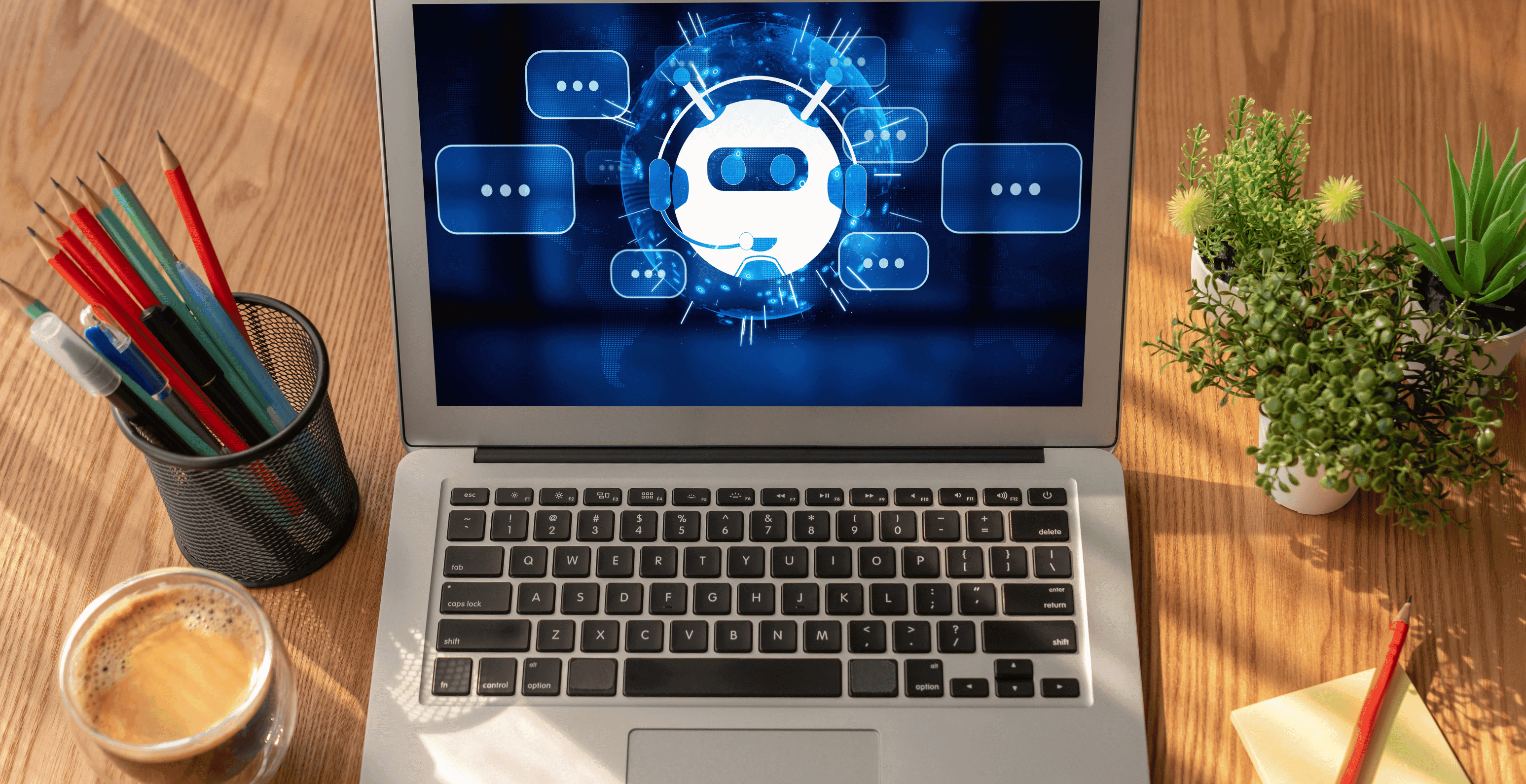
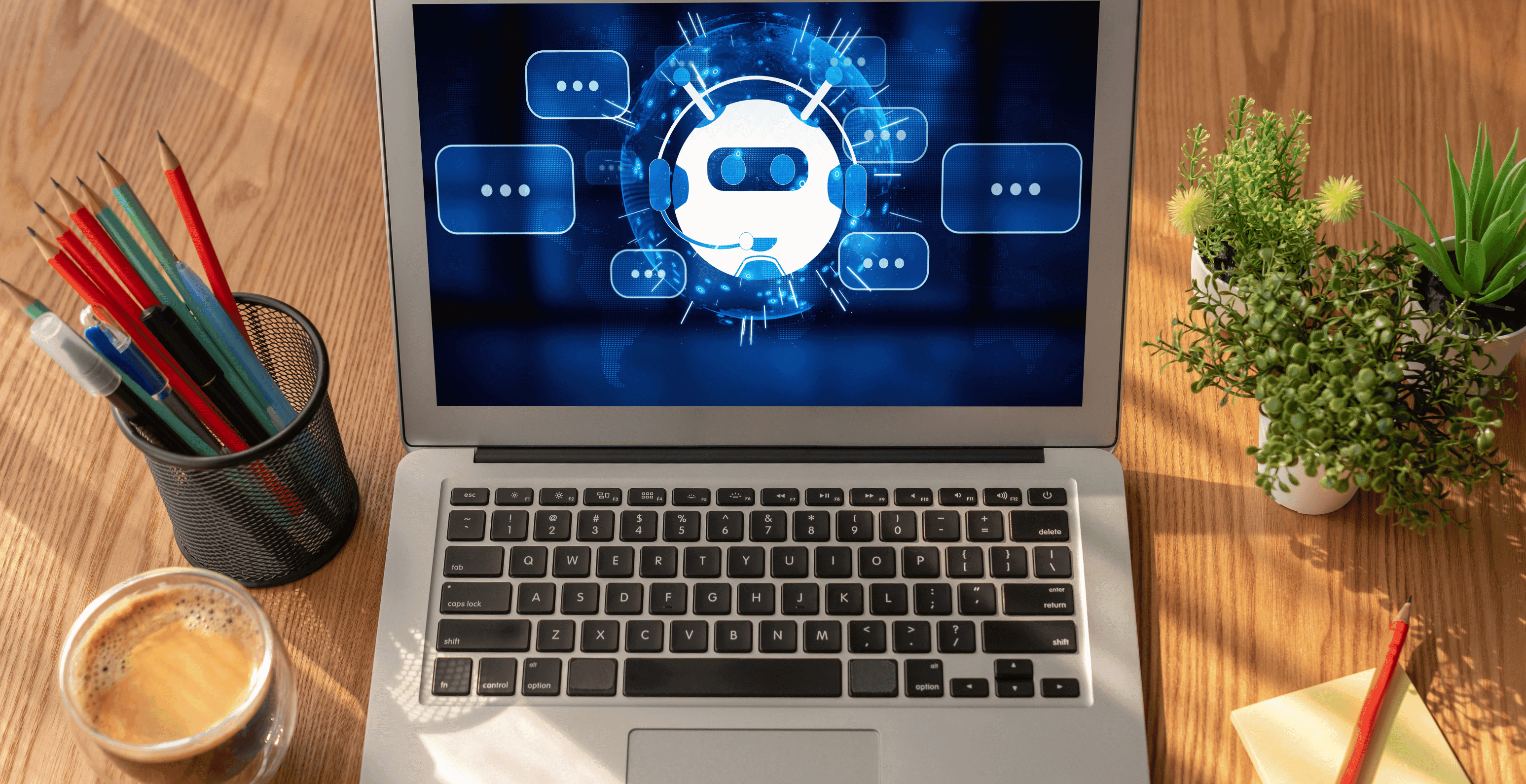
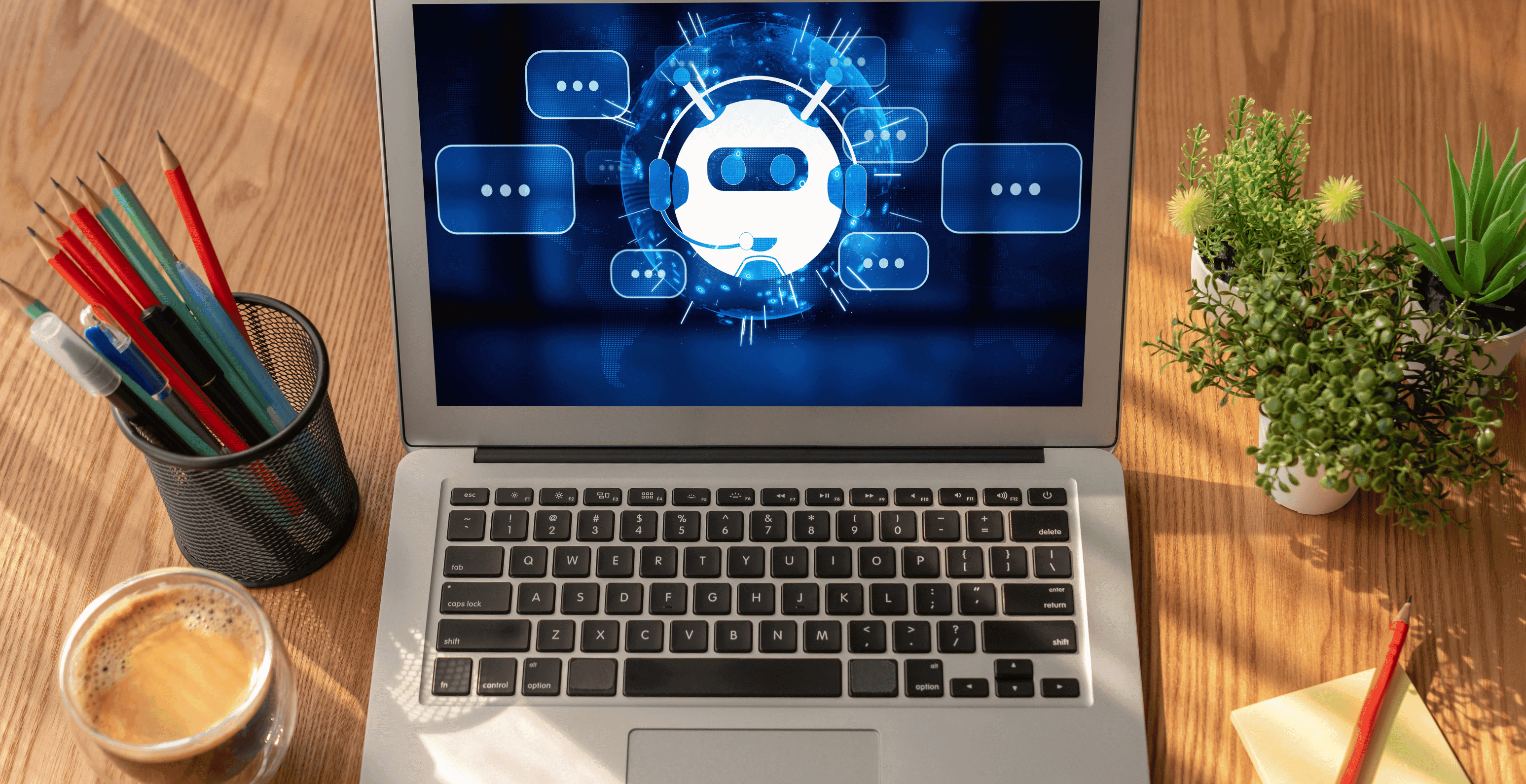
Strategic Approach To Testing AI-Powered Applications And Systems

Rehan Asif
Apr 30, 2024
Read the article




Navigating GDPR Compliance for AI Applications

Rehan Asif
Apr 26, 2024
Read the article
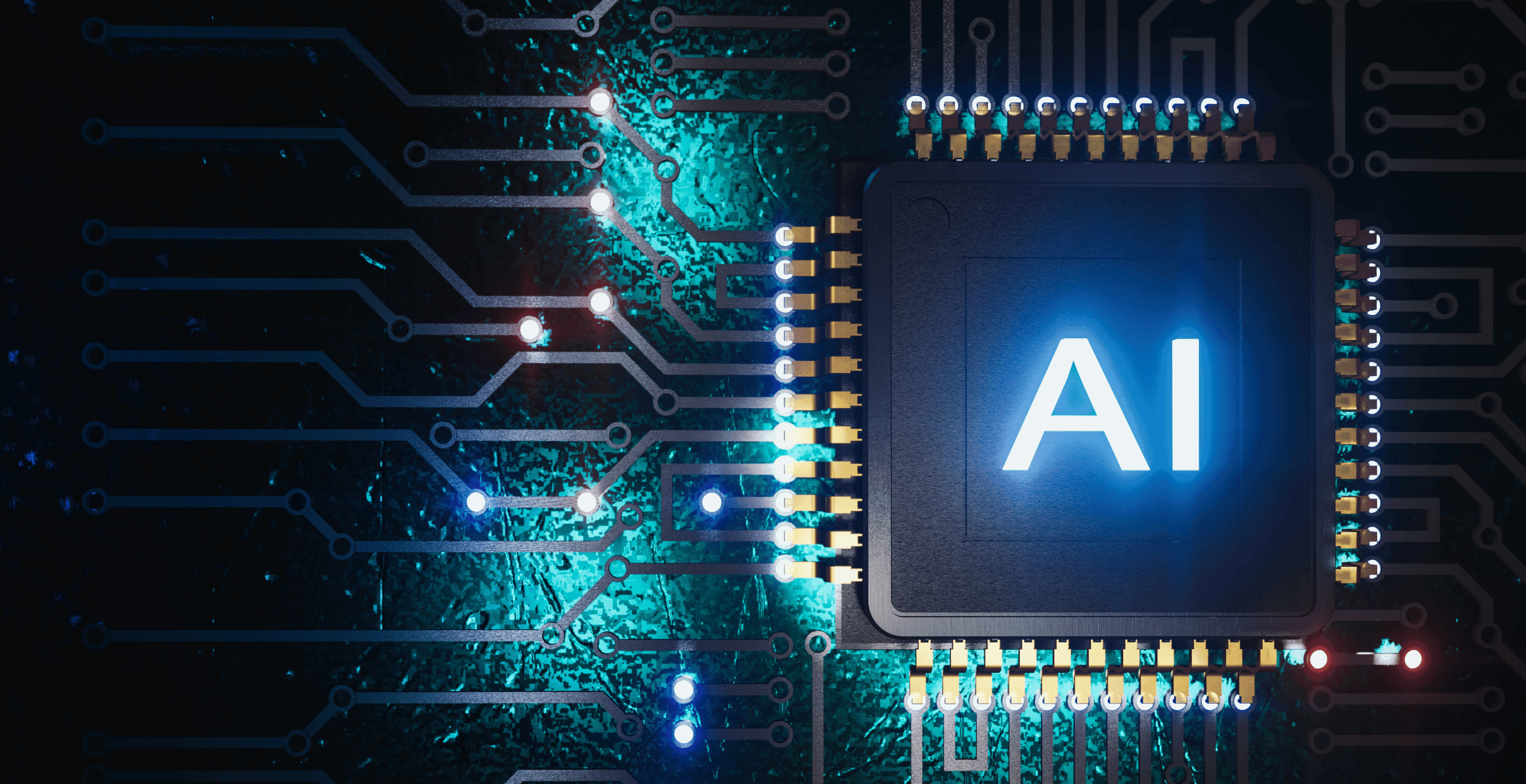
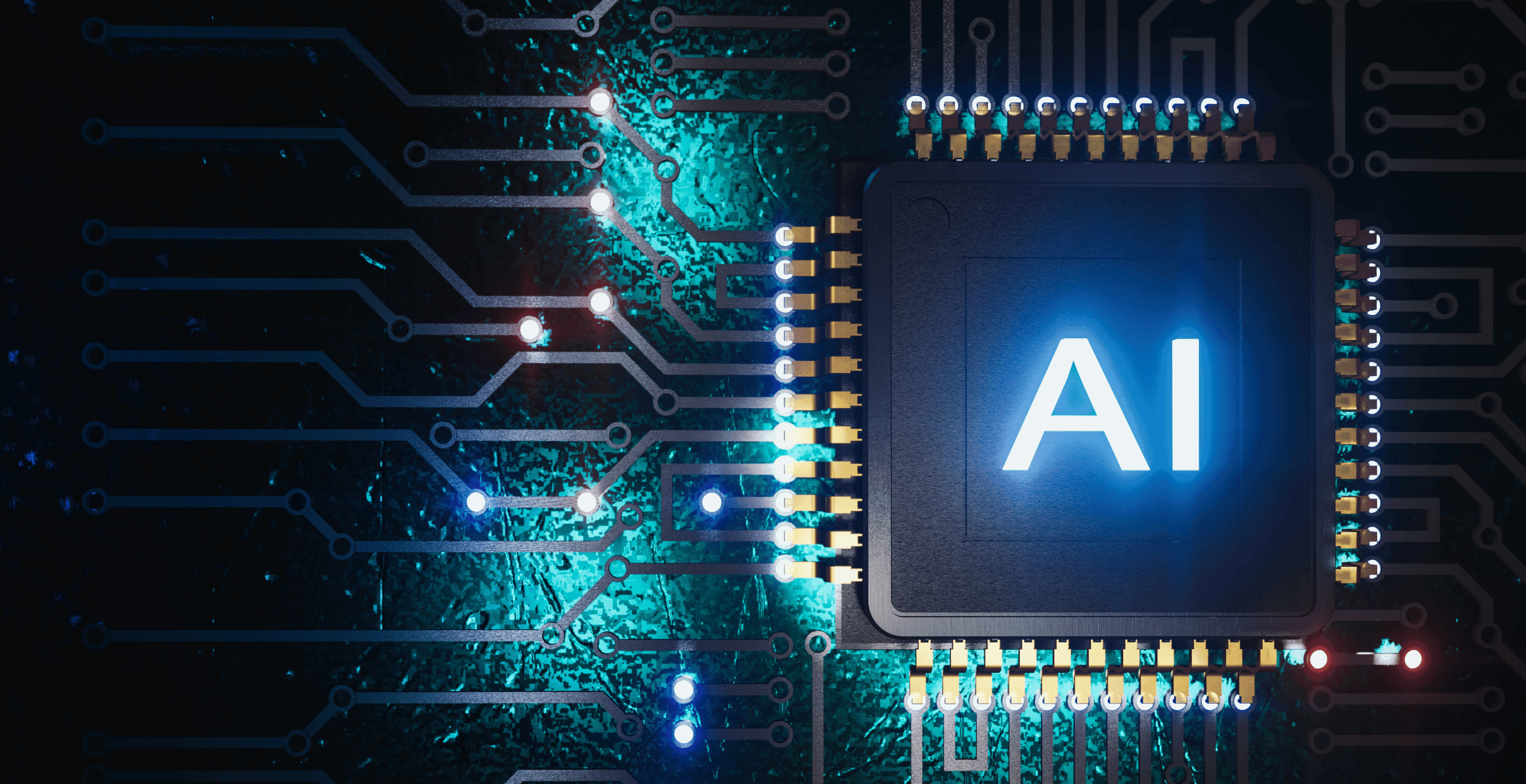
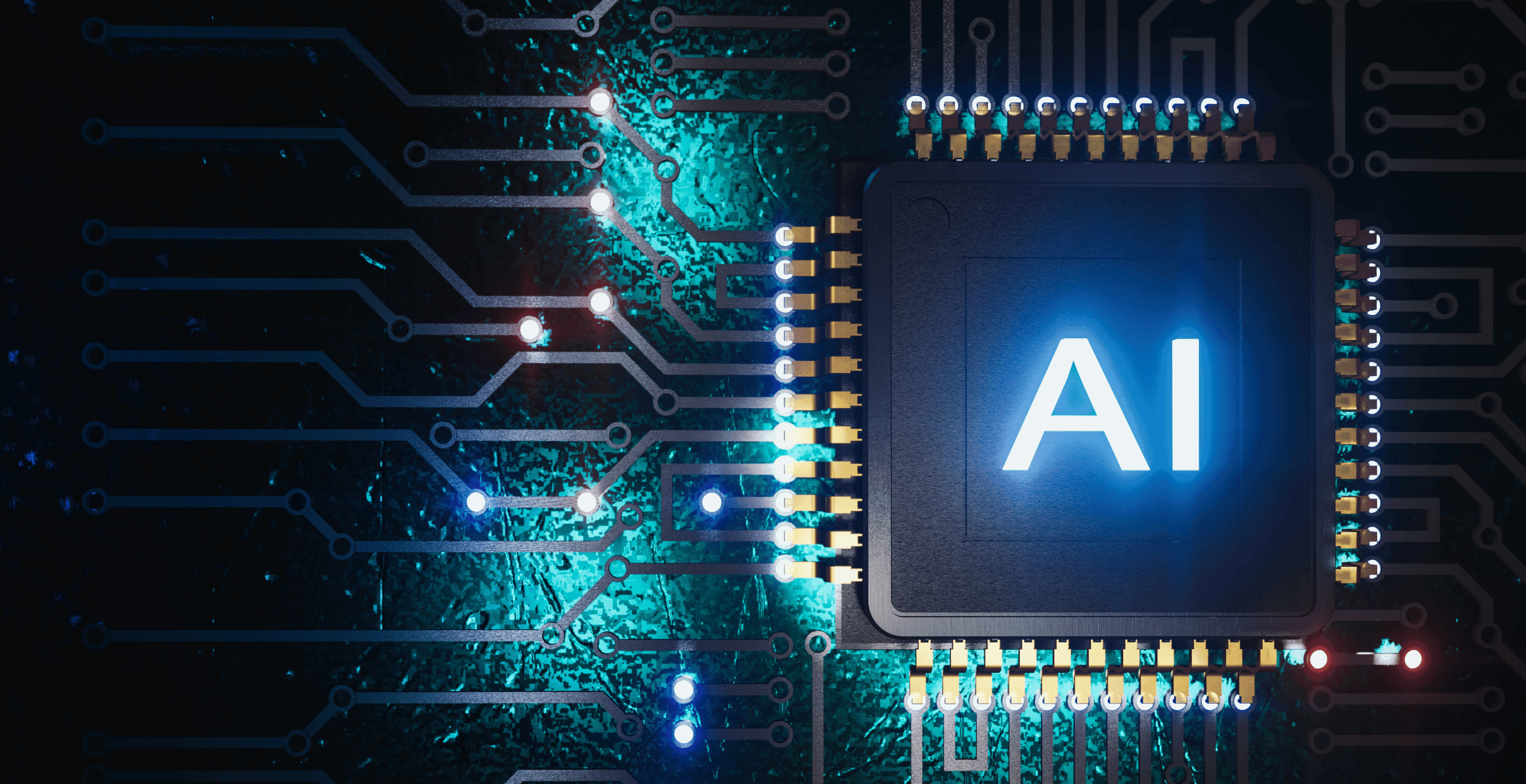
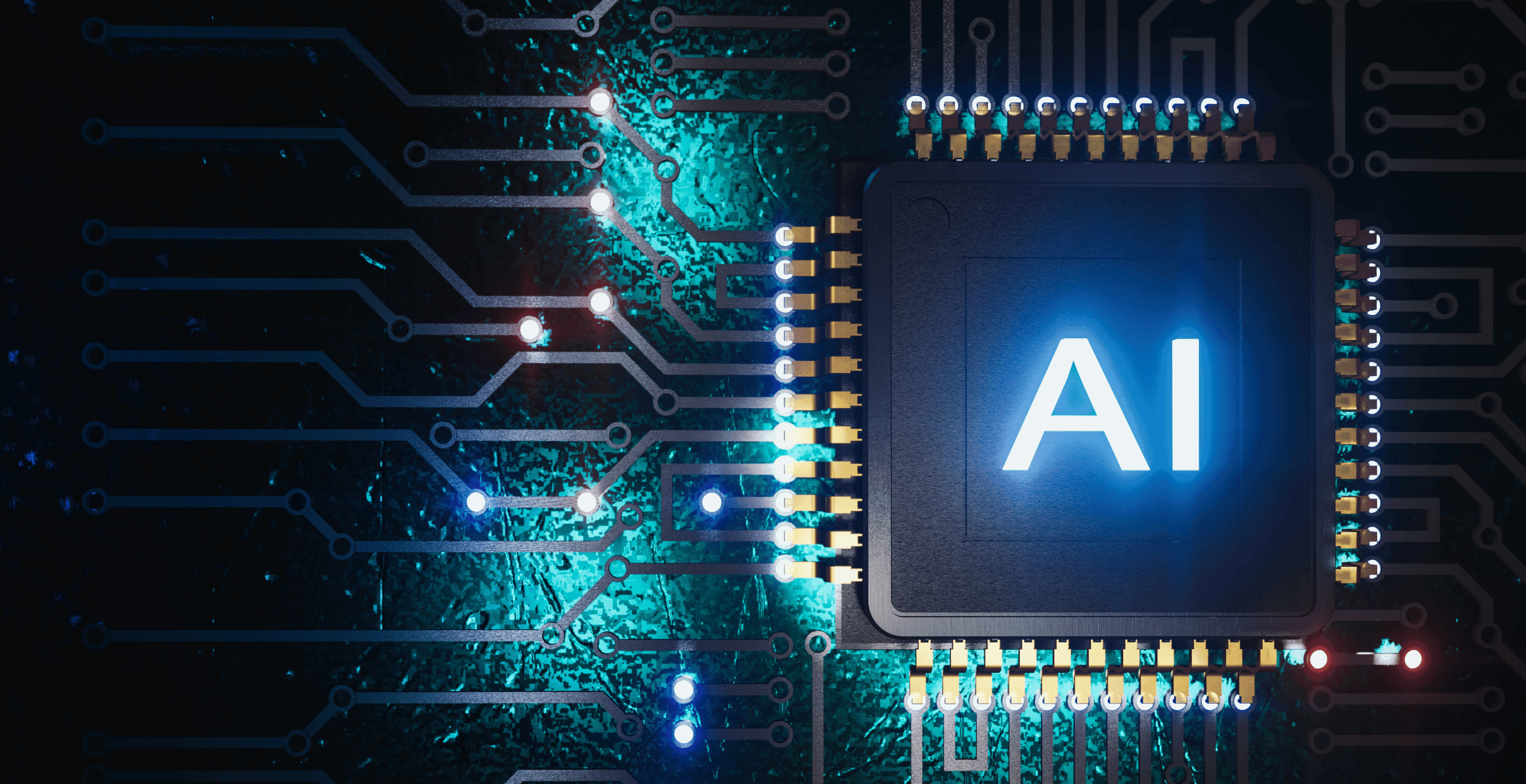
The Impact of AI Governance on Innovation and Development Speed

Rehan Asif
Apr 26, 2024
Read the article
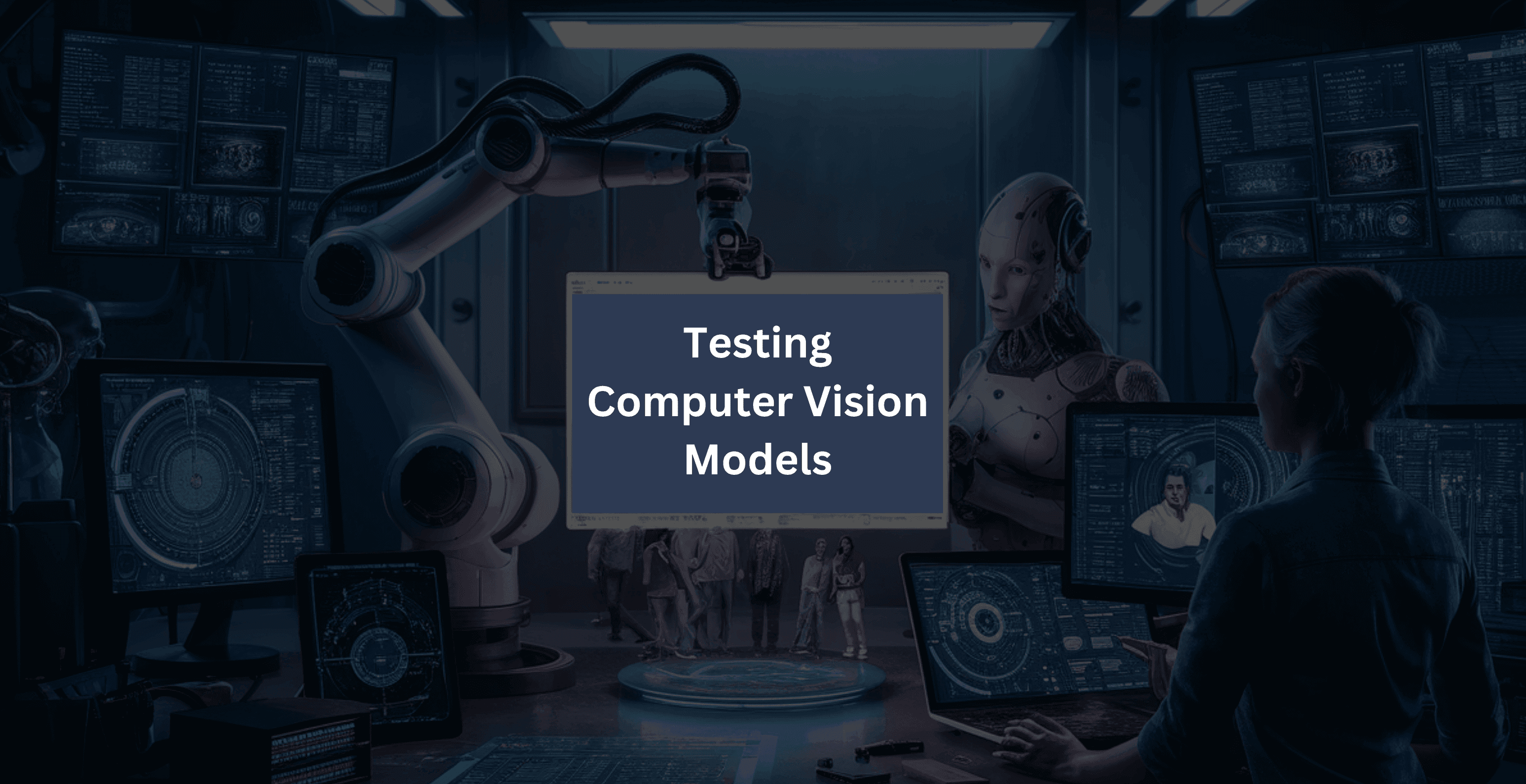
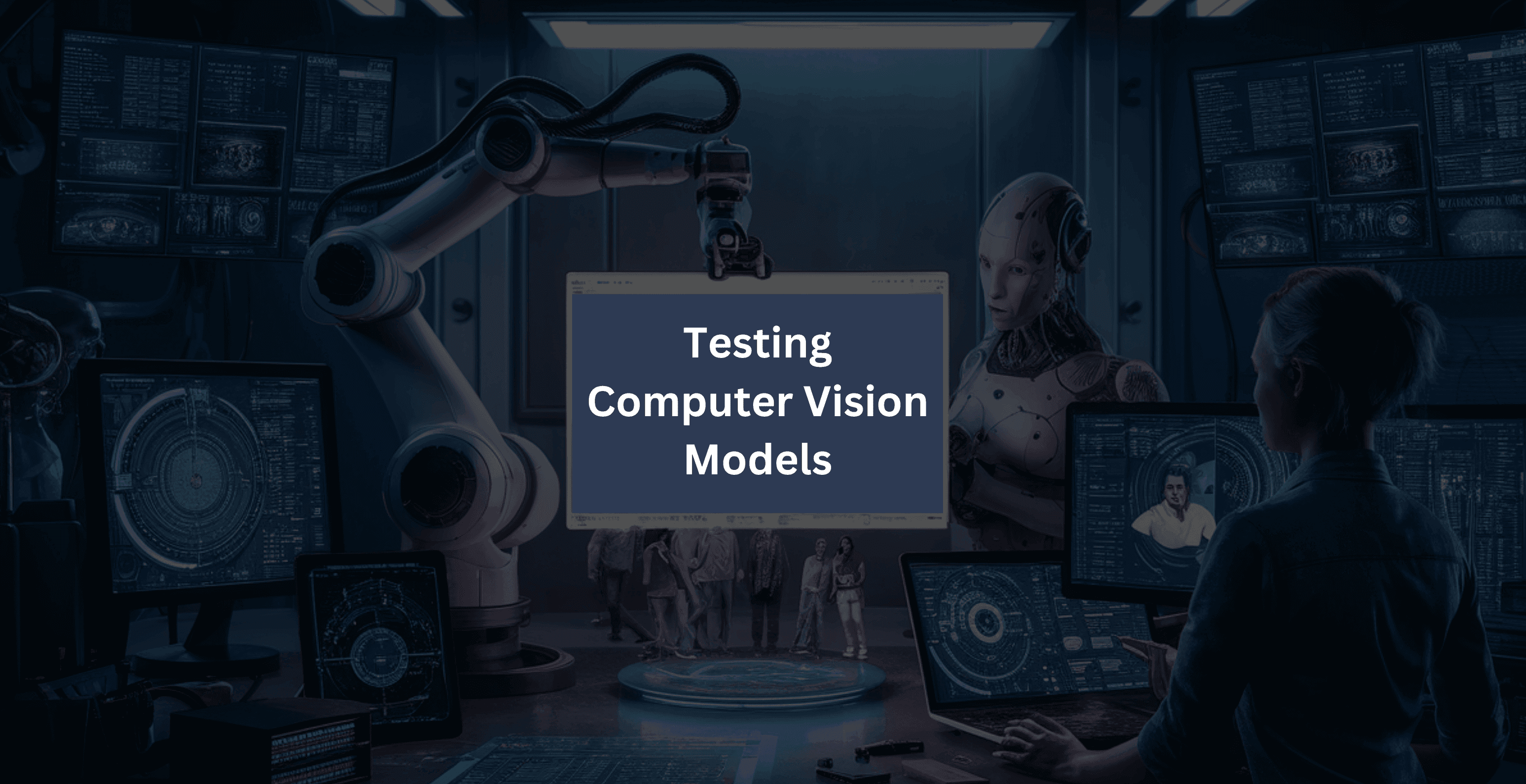
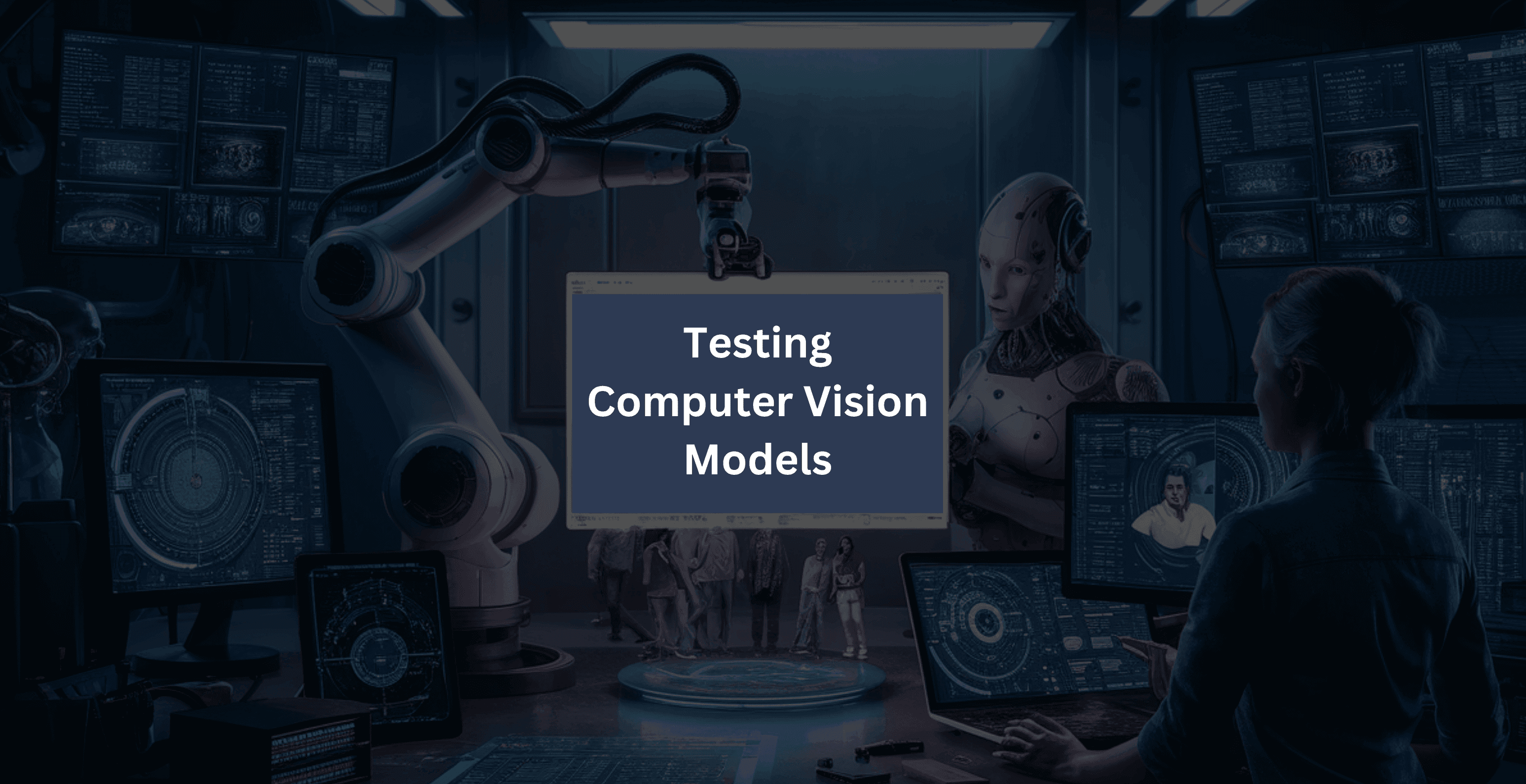
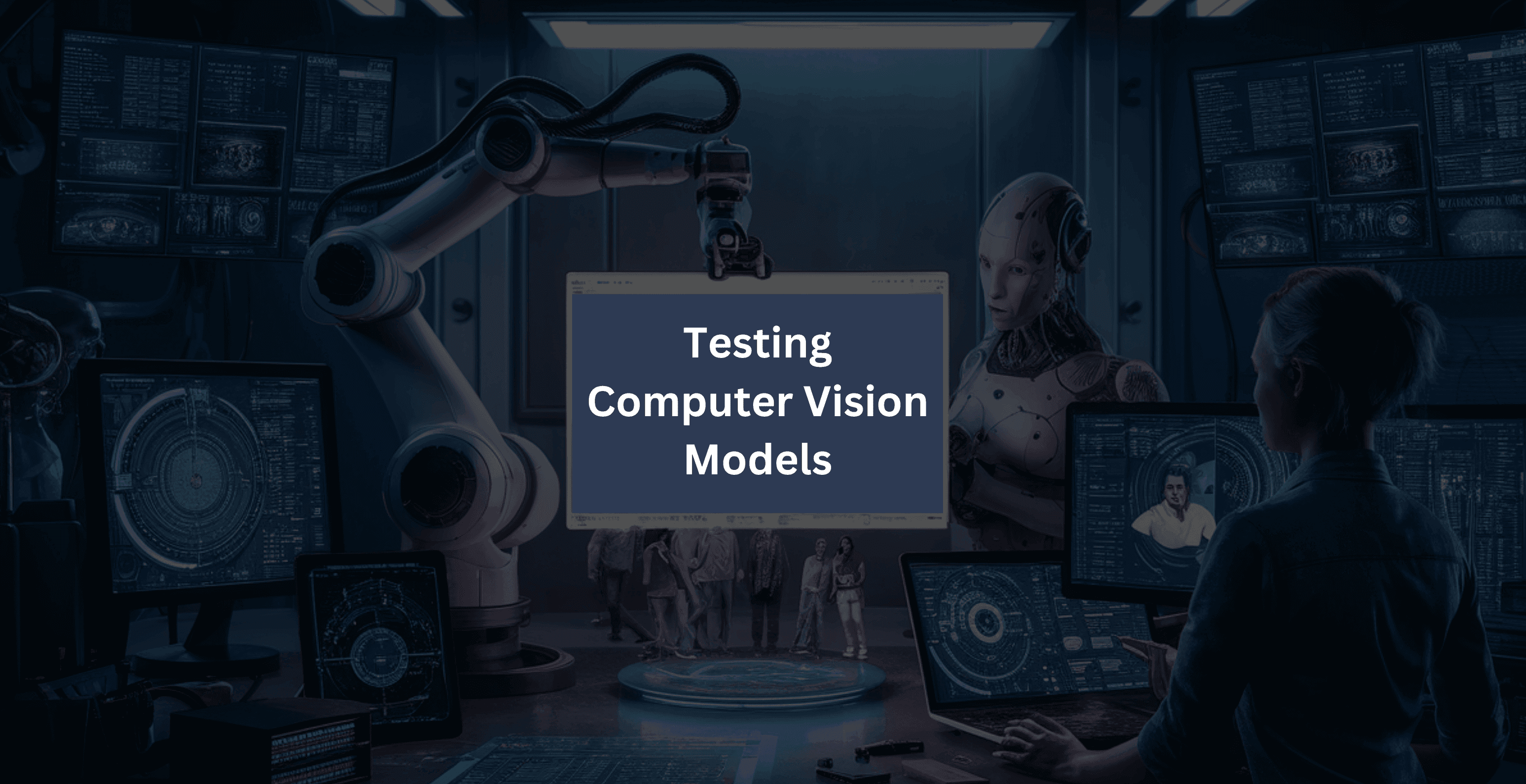
Best Practices For Testing Computer Vision Models
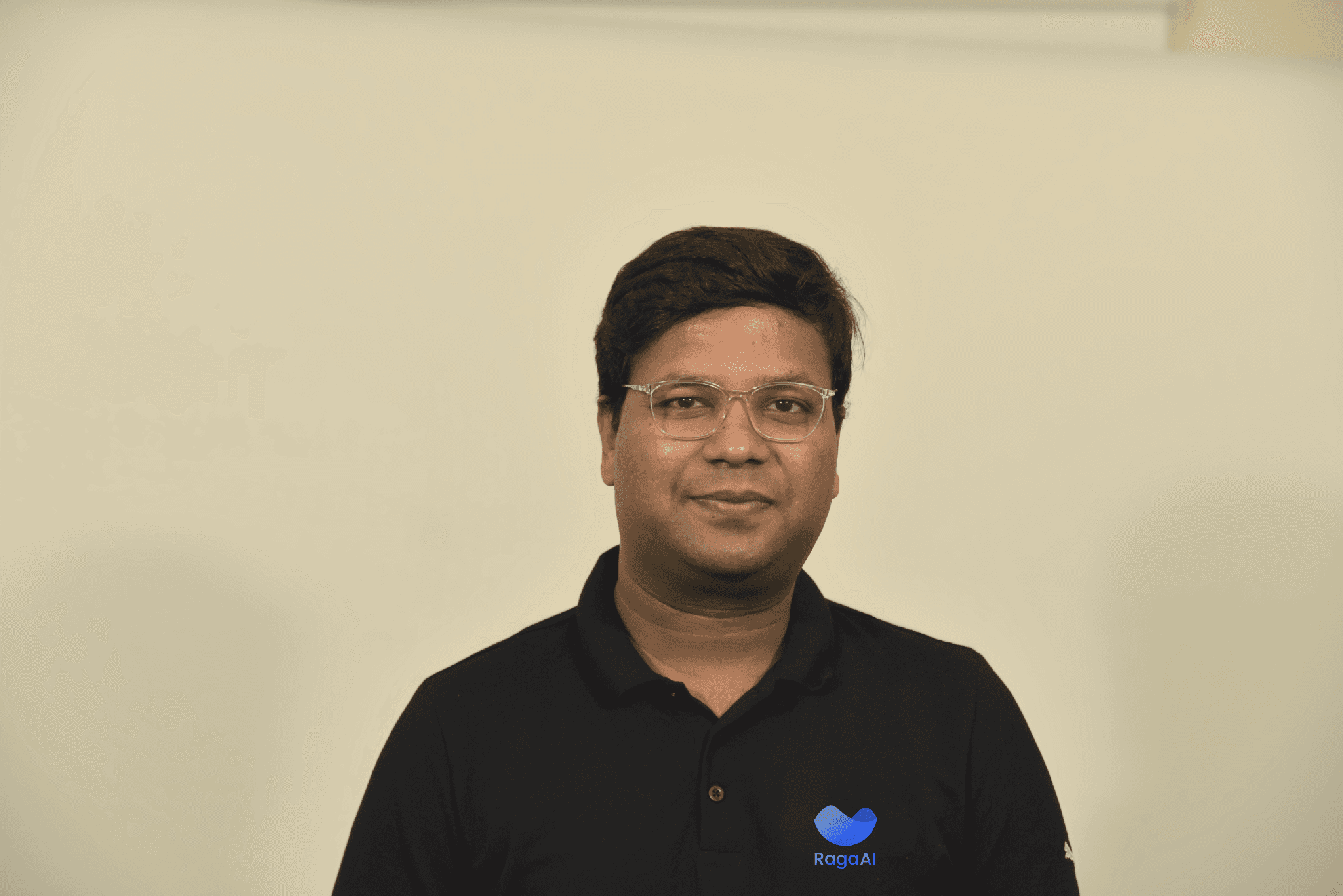
Jigar Gupta
Apr 25, 2024
Read the article
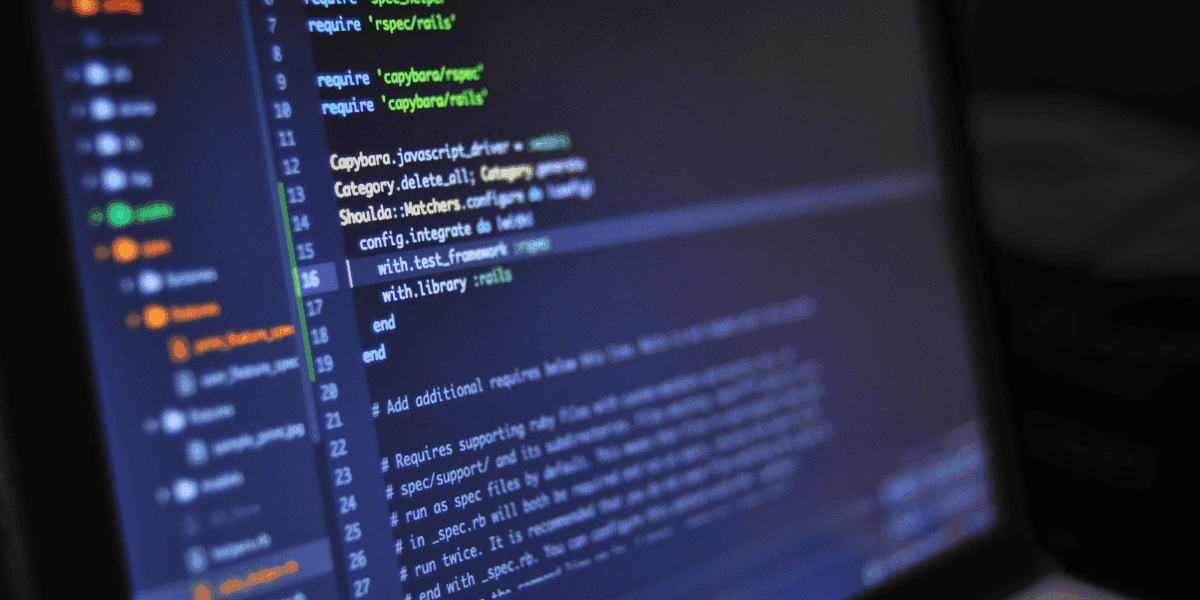
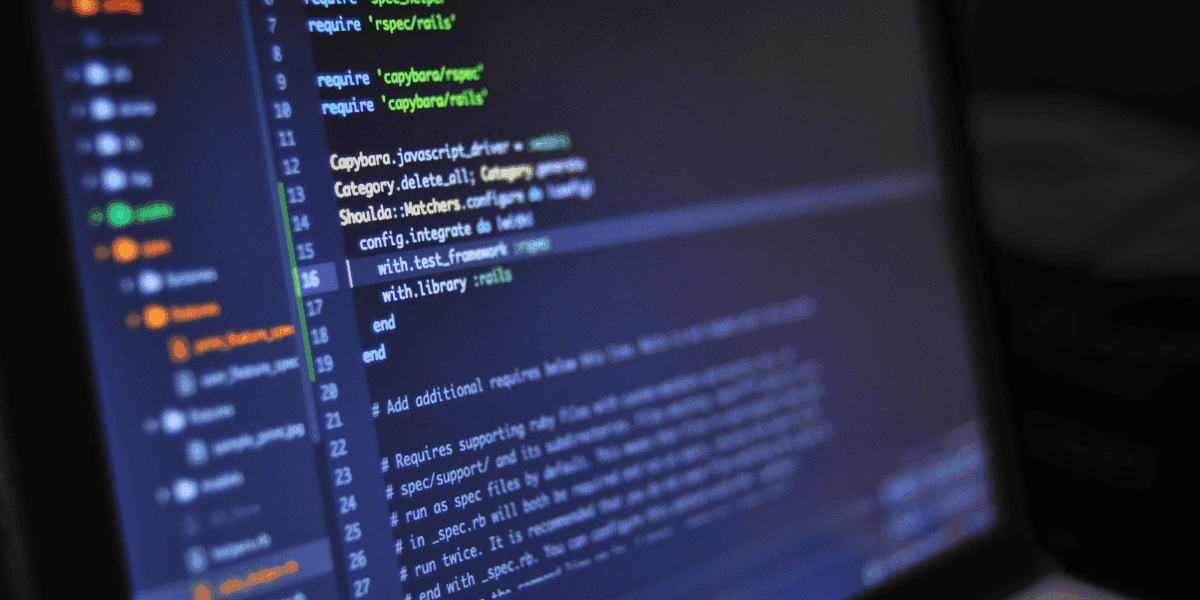
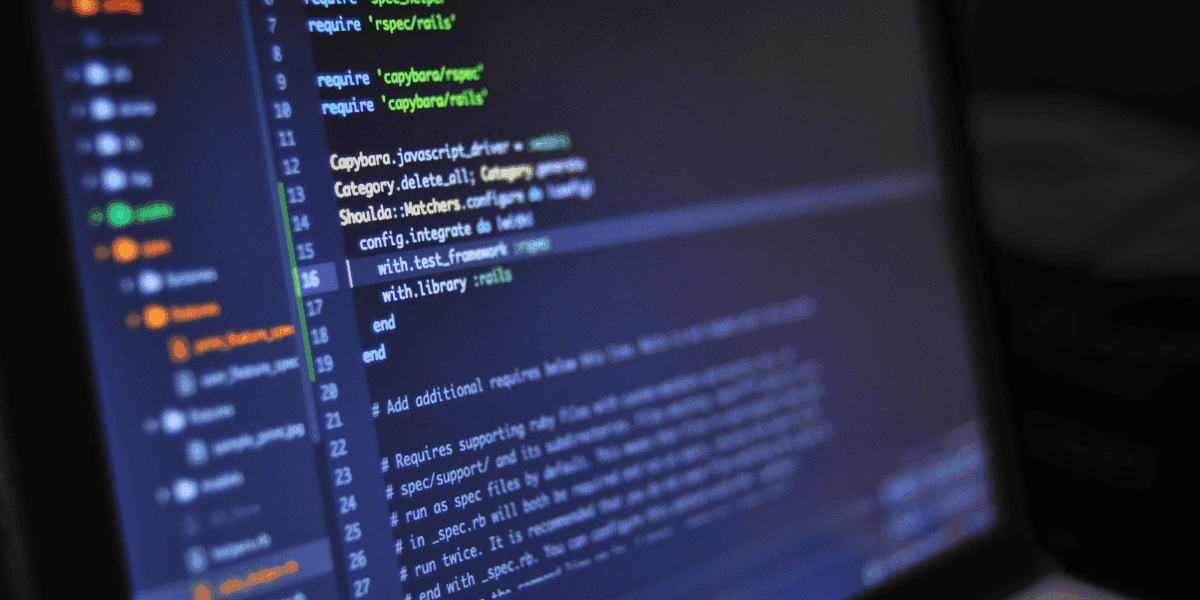
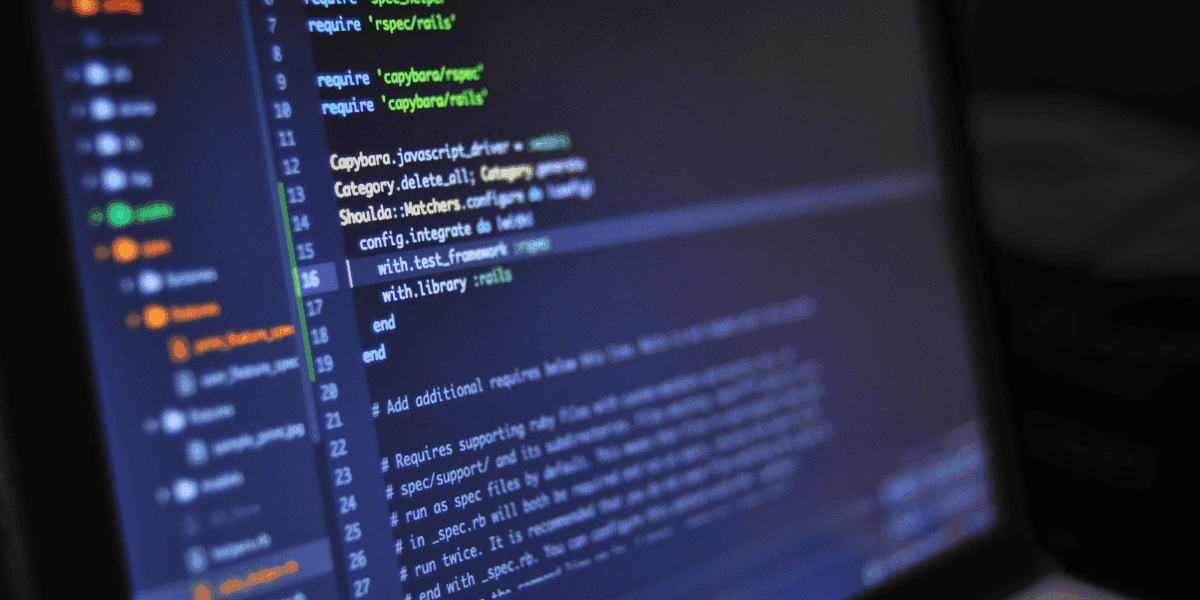
Building Low-Code LLM Apps with Visual Programming

Rehan Asif
Apr 26, 2024
Read the article




Understanding AI regulations In Finance

Akshat Gupta
Apr 26, 2024
Read the article




Compliance Automation: Getting Started with Regulatory Management

Akshat Gupta
Apr 25, 2024
Read the article
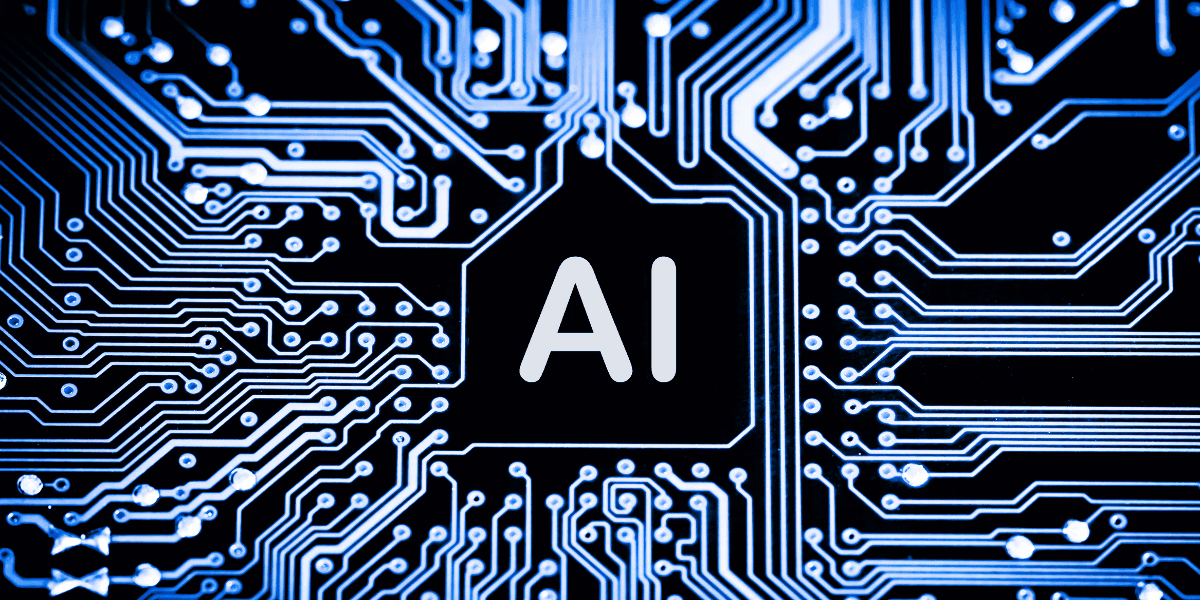
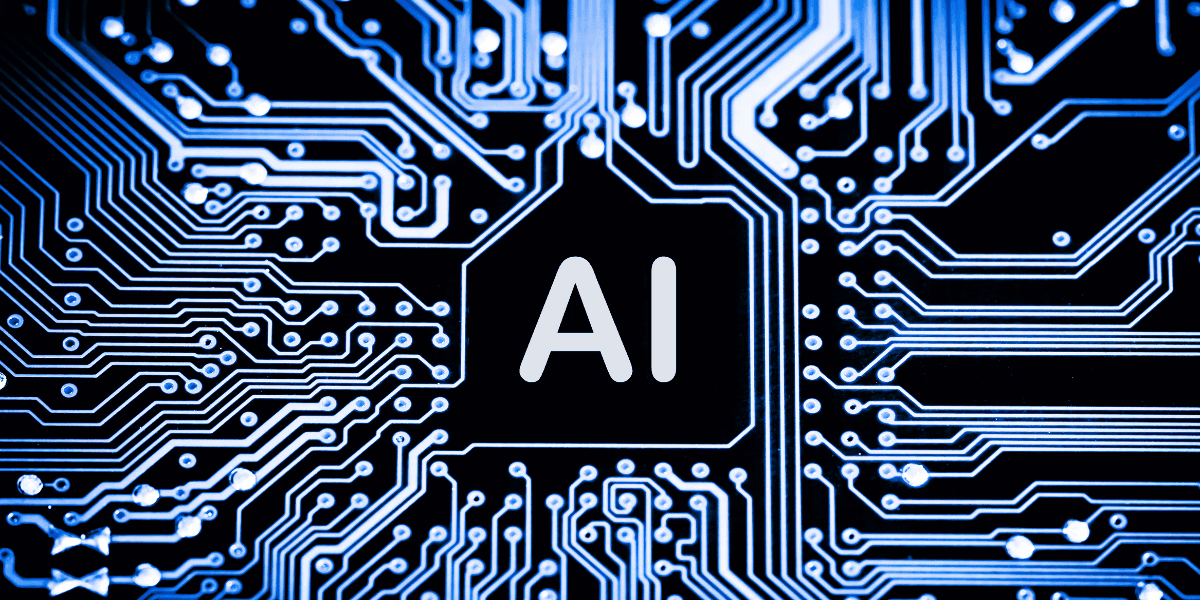
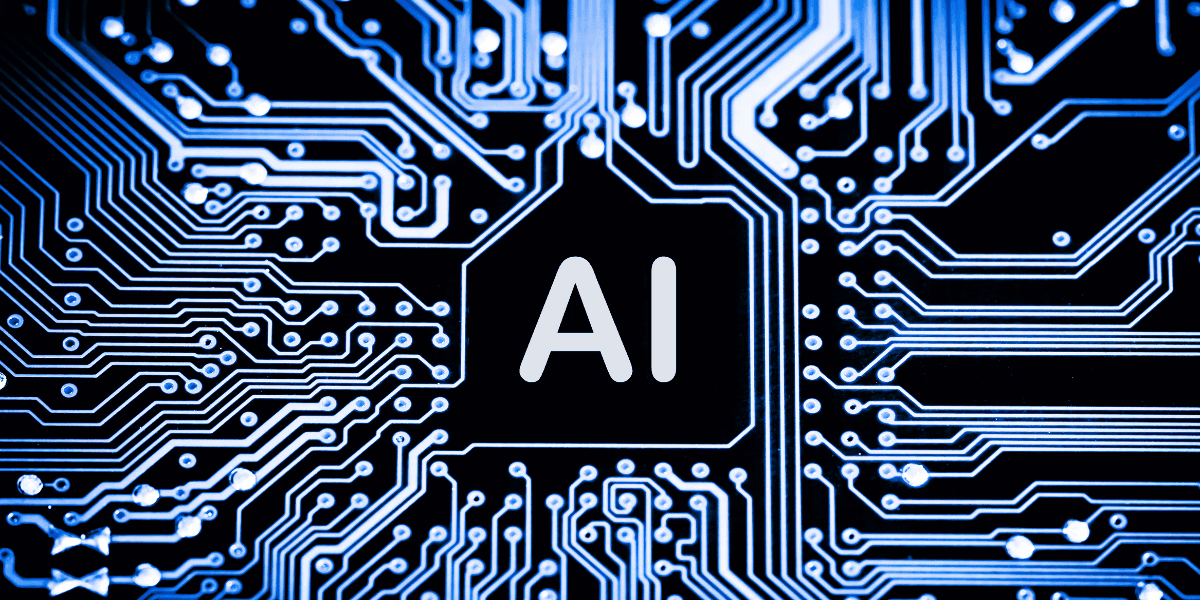
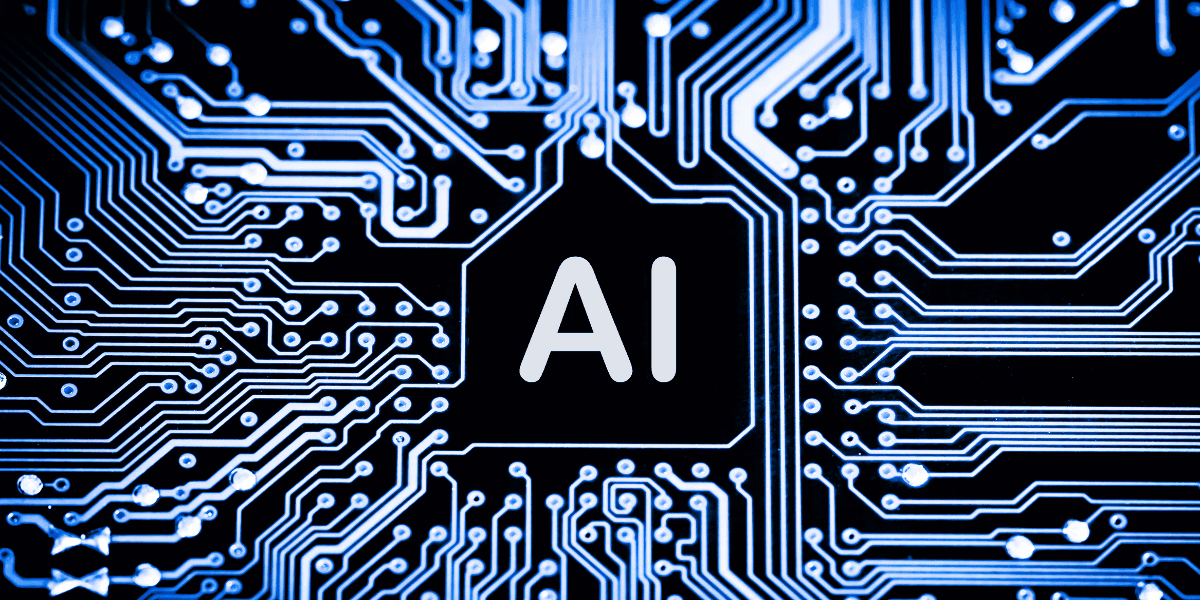
Practical Guide to Fine-Tuning OpenAI GPT Models Using Python

Rehan Asif
Apr 24, 2024
Read the article




Comparing Different Large Language Models (LLM)

Rehan Asif
Apr 23, 2024
Read the article




Evaluating Large Language Models: Methods And Metrics

Rehan Asif
Apr 22, 2024
Read the article




Significant AI Errors, Mistakes, Failures, and Flaws Companies Encounter

Akshat Gupta
Apr 21, 2024
Read the article




Challenges and Strategies for Implementing Enterprise LLM

Rehan Asif
Apr 20, 2024
Read the article




Enhancing Computer Vision with Synthetic Data: Advantages and Generation Techniques
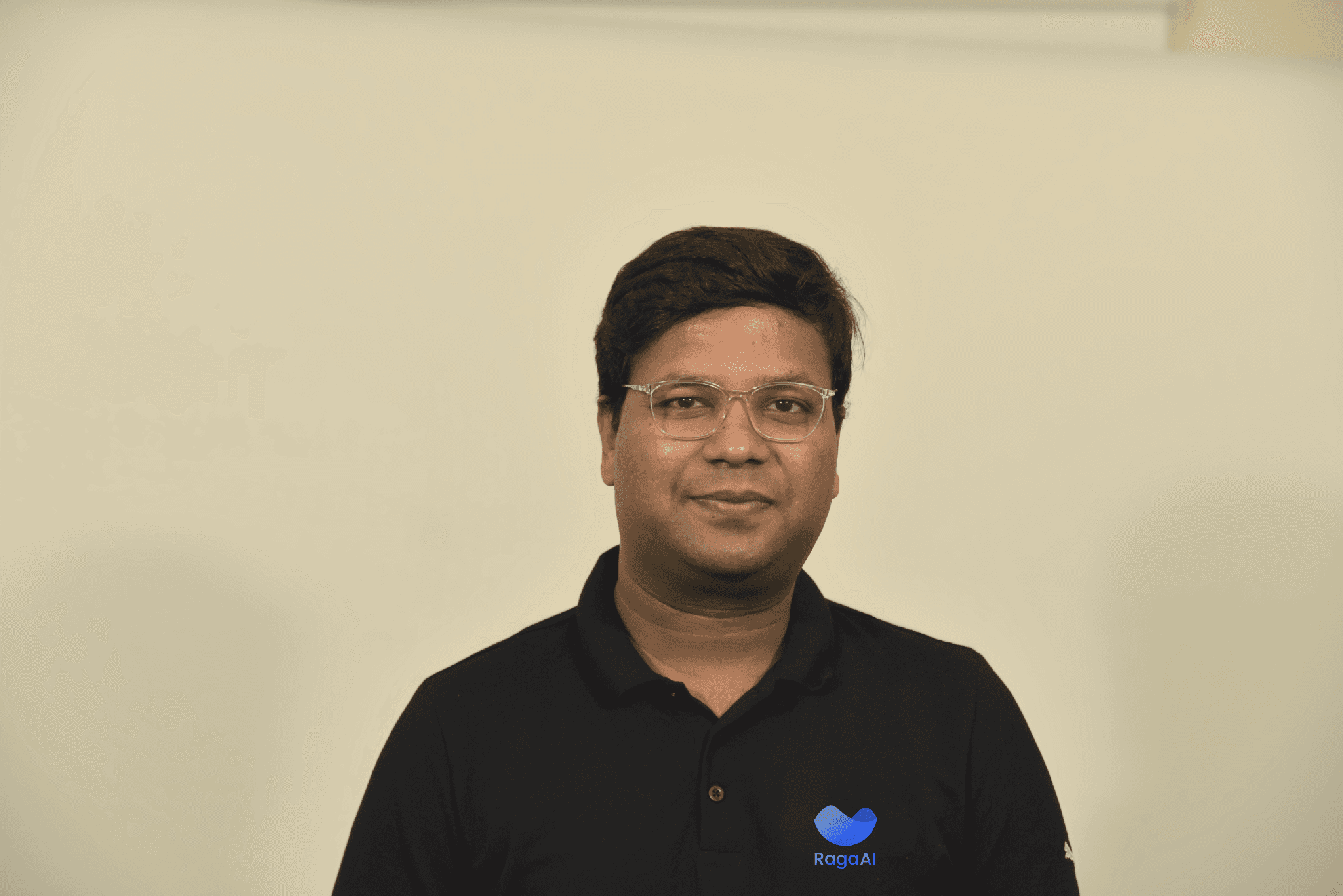
Jigar Gupta
Apr 20, 2024
Read the article
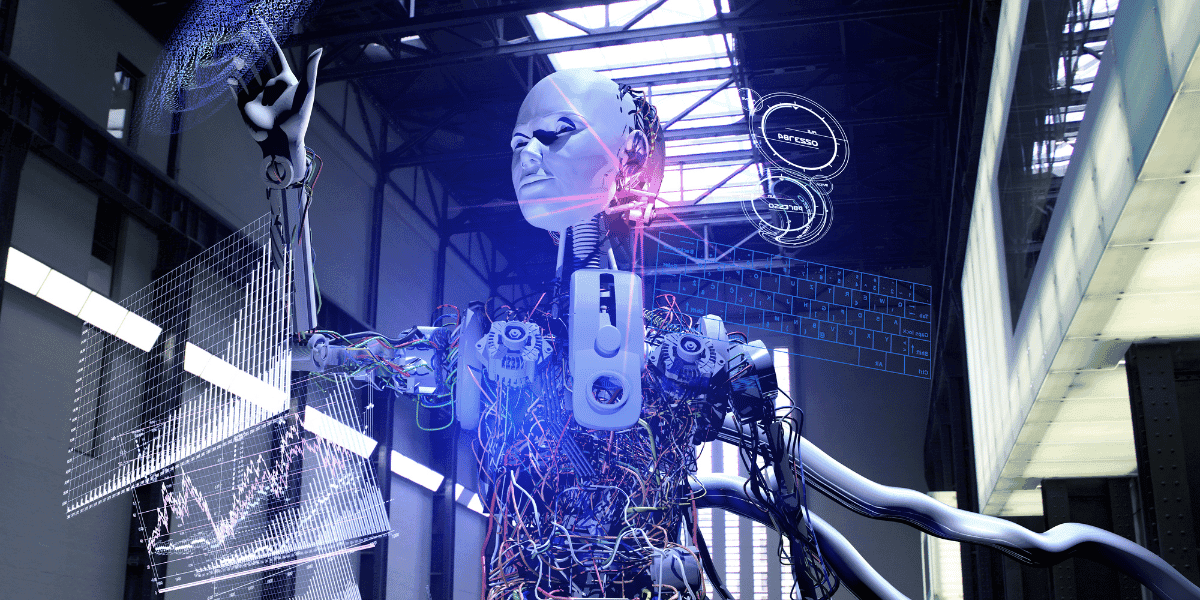
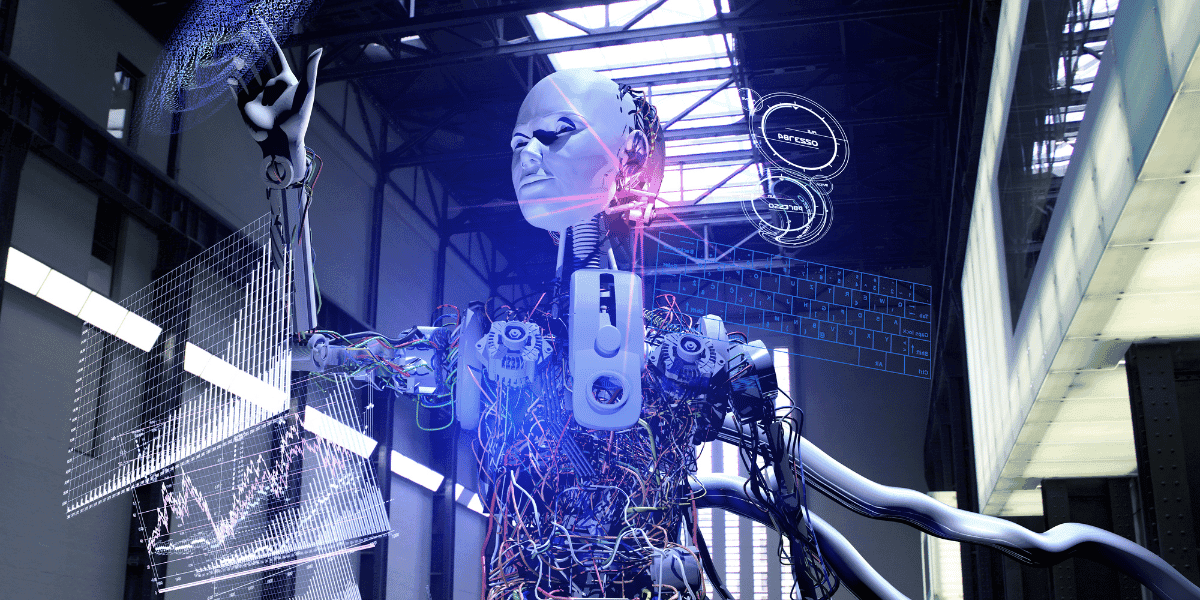
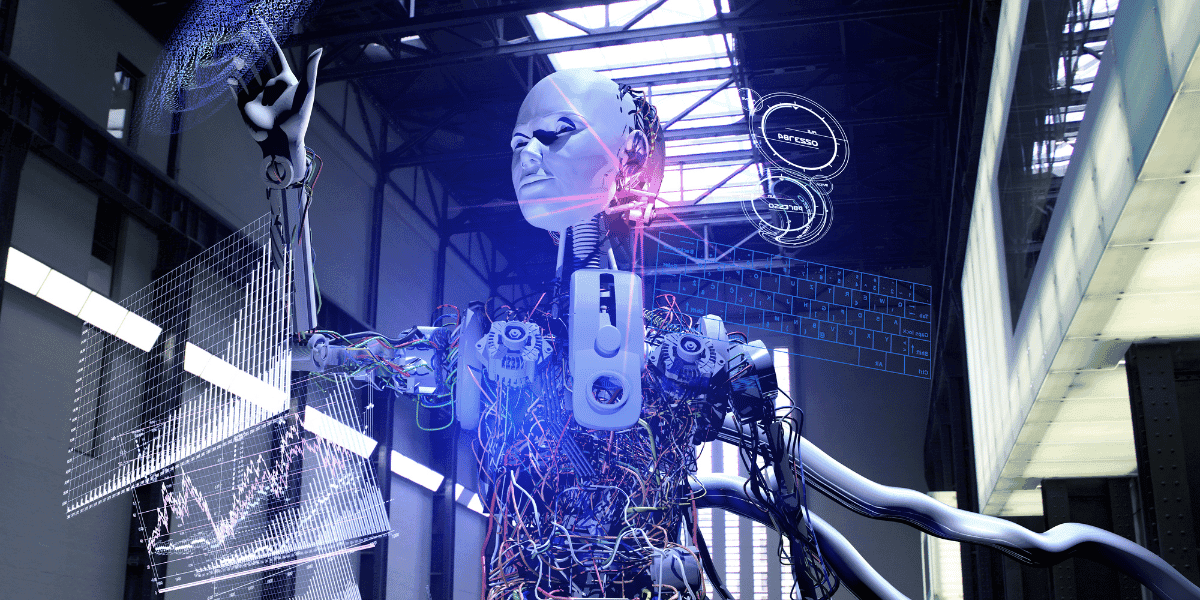
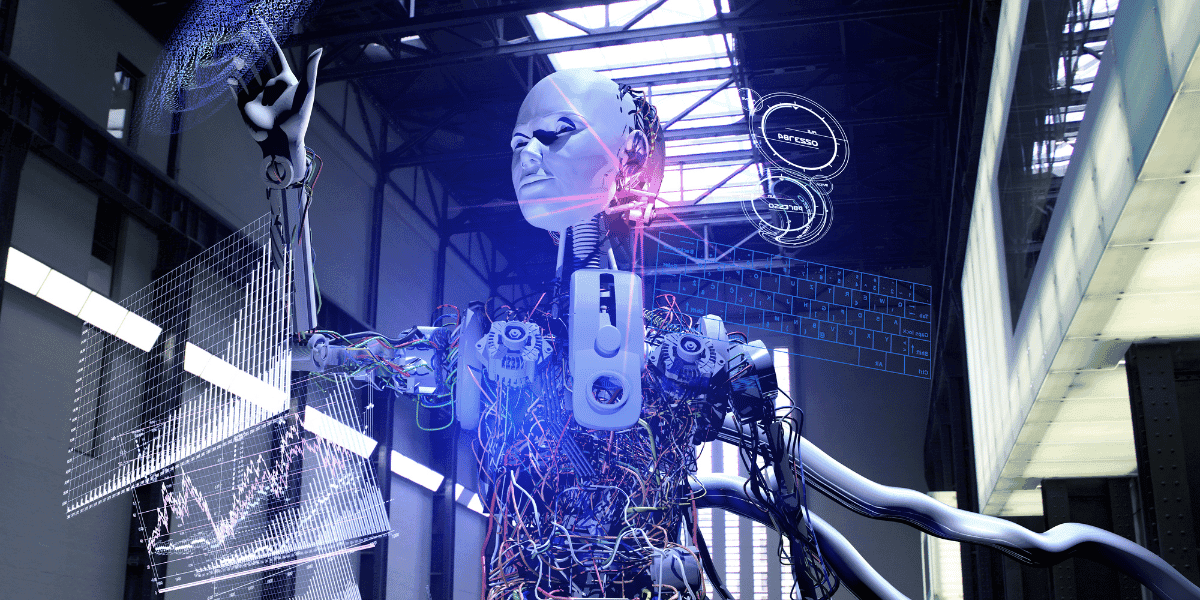
Building Trust In Artificial Intelligence Systems

Akshat Gupta
Apr 19, 2024
Read the article




A Brief Guide To LLM Parameters: Tuning and Optimization

Rehan Asif
Apr 18, 2024
Read the article




Unlocking The Potential Of Computer Vision Testing: Key Techniques And Tools
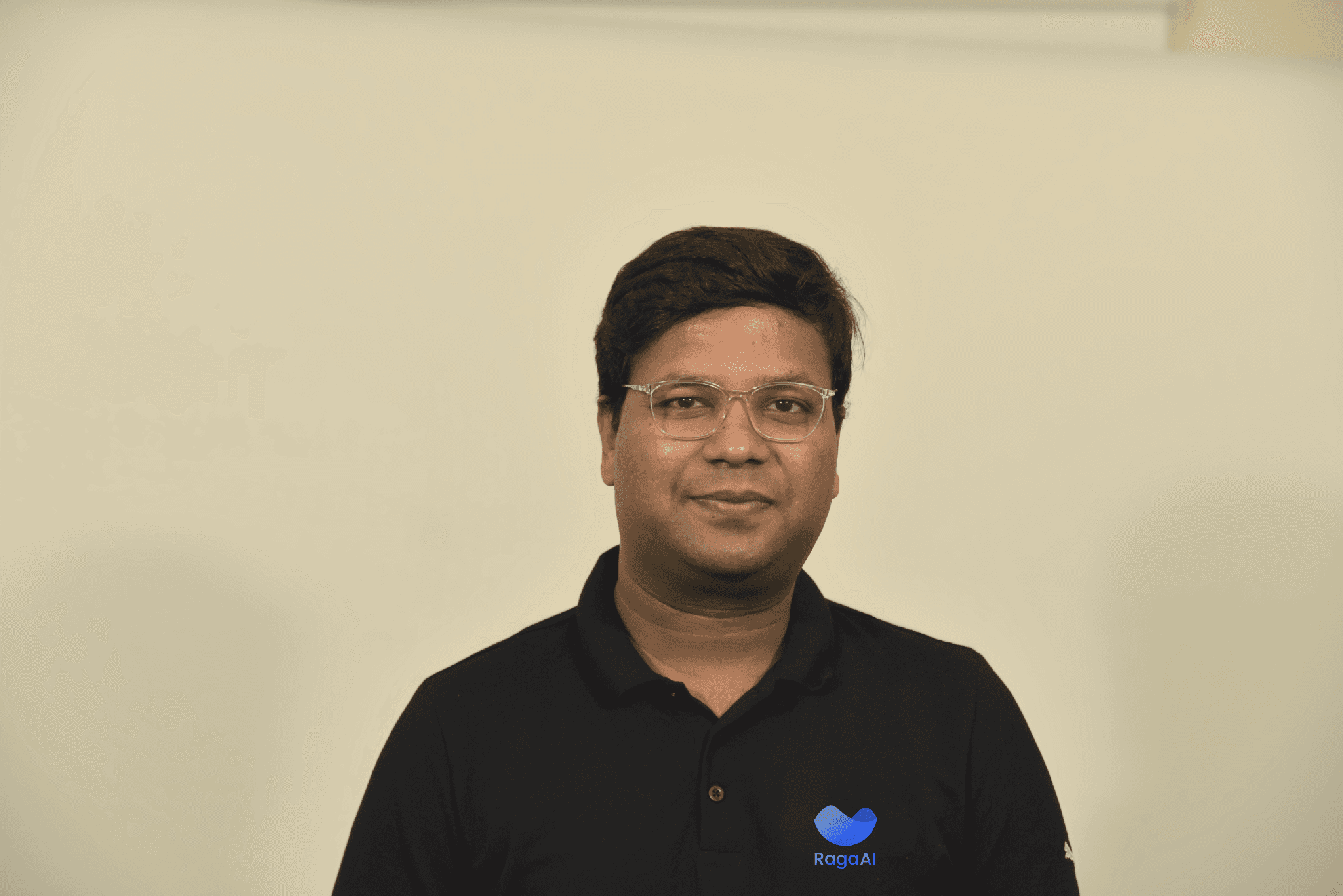
Jigar Gupta
Apr 17, 2024
Read the article




Understanding AI Regulatory Compliance And Its Importance

Akshat Gupta
Apr 16, 2024
Read the article
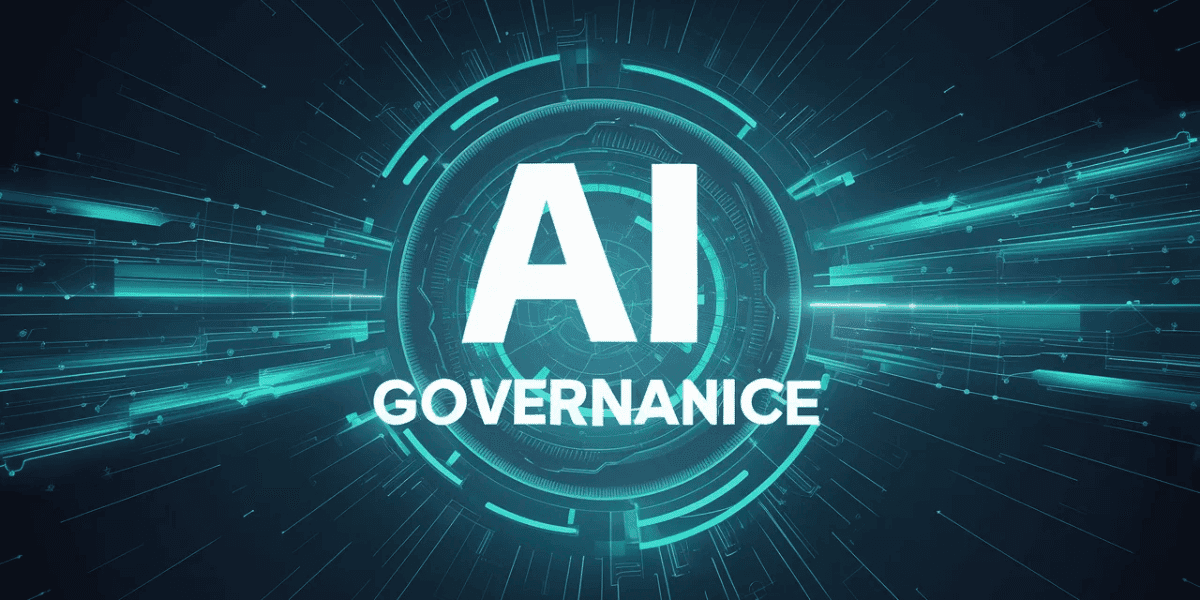
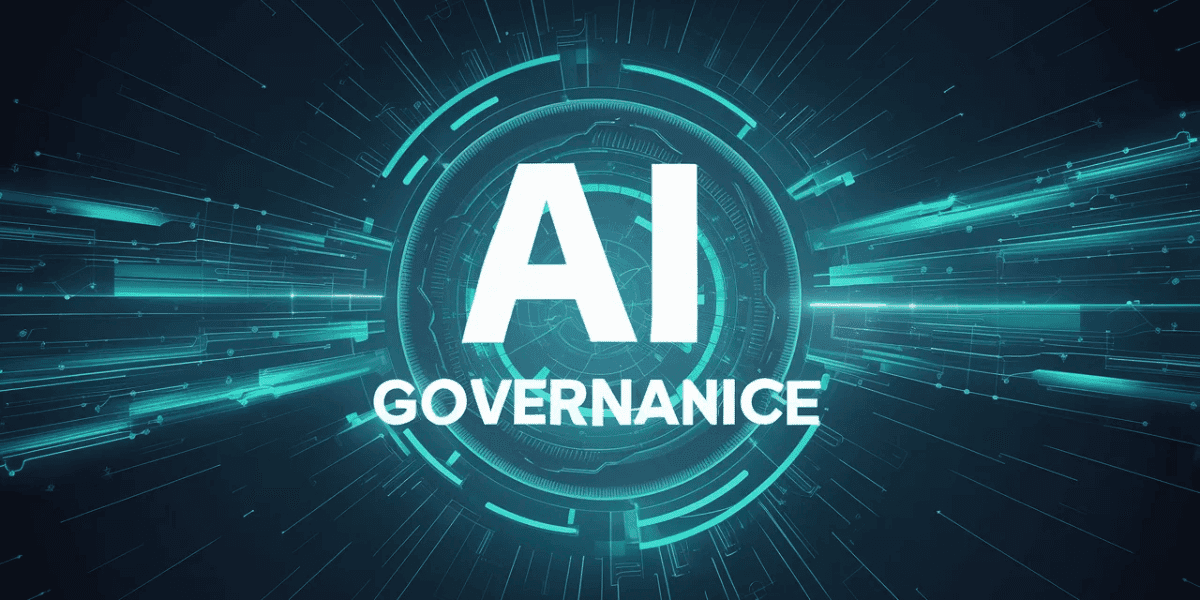
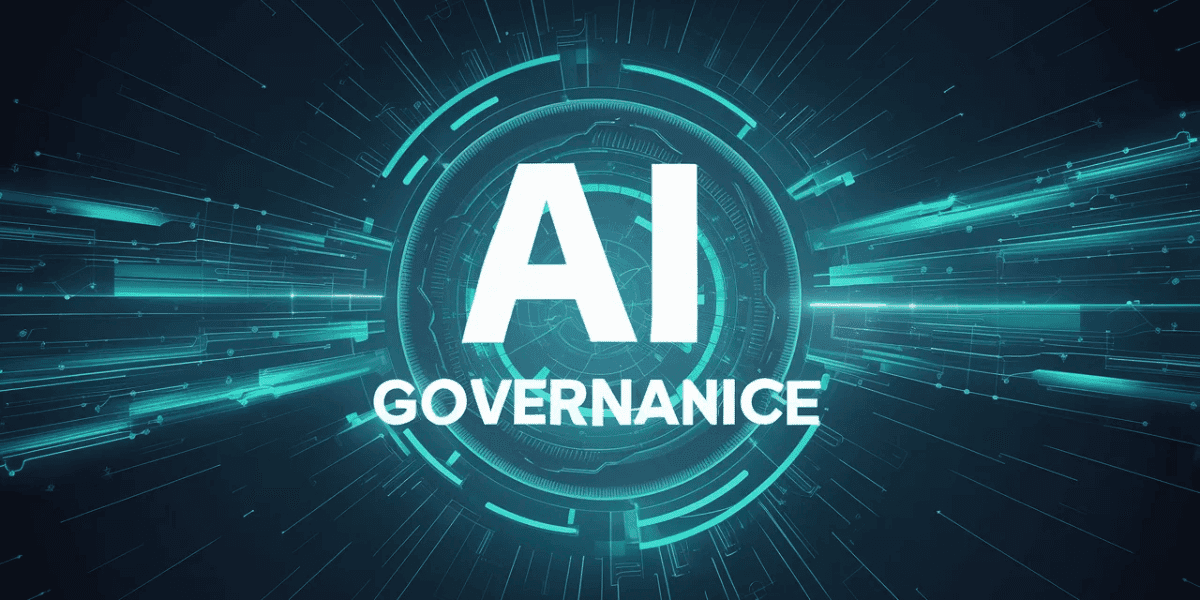
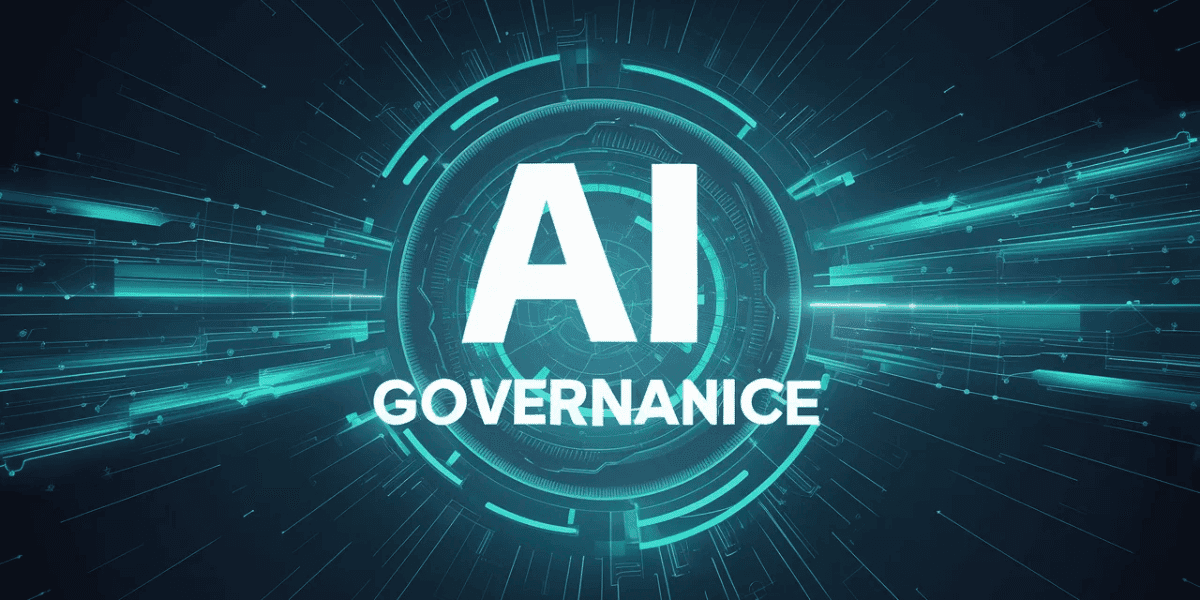
Understanding The Basics Of AI Governance

Akshat Gupta
Apr 15, 2024
Read the article




Understanding Prompt Engineering: A Guide

Rehan Asif
Apr 15, 2024
Read the article
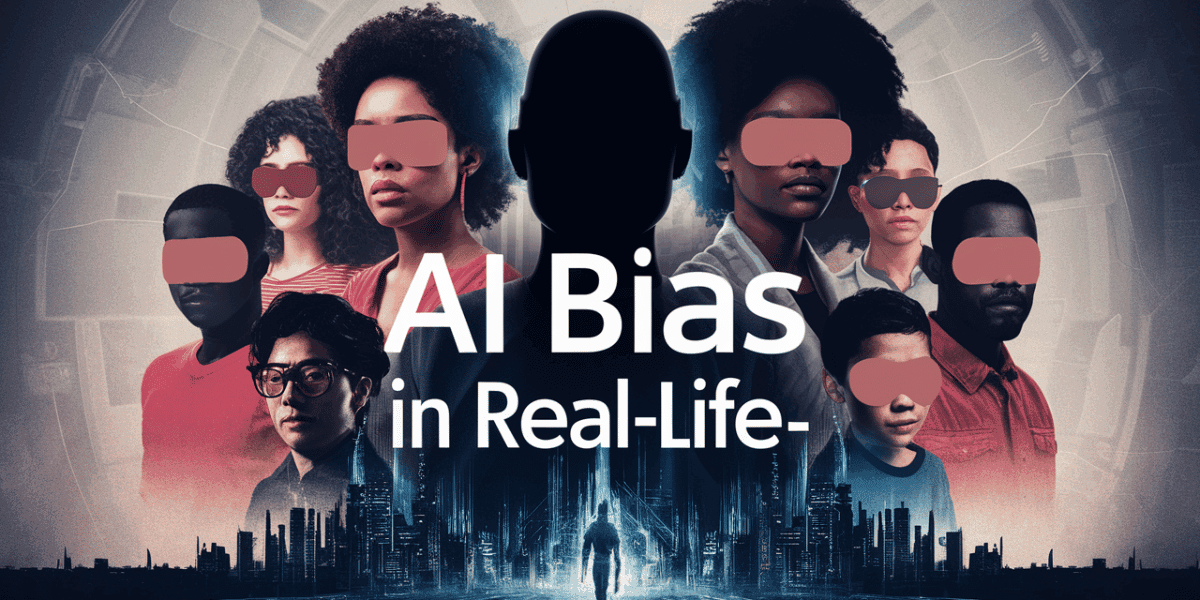
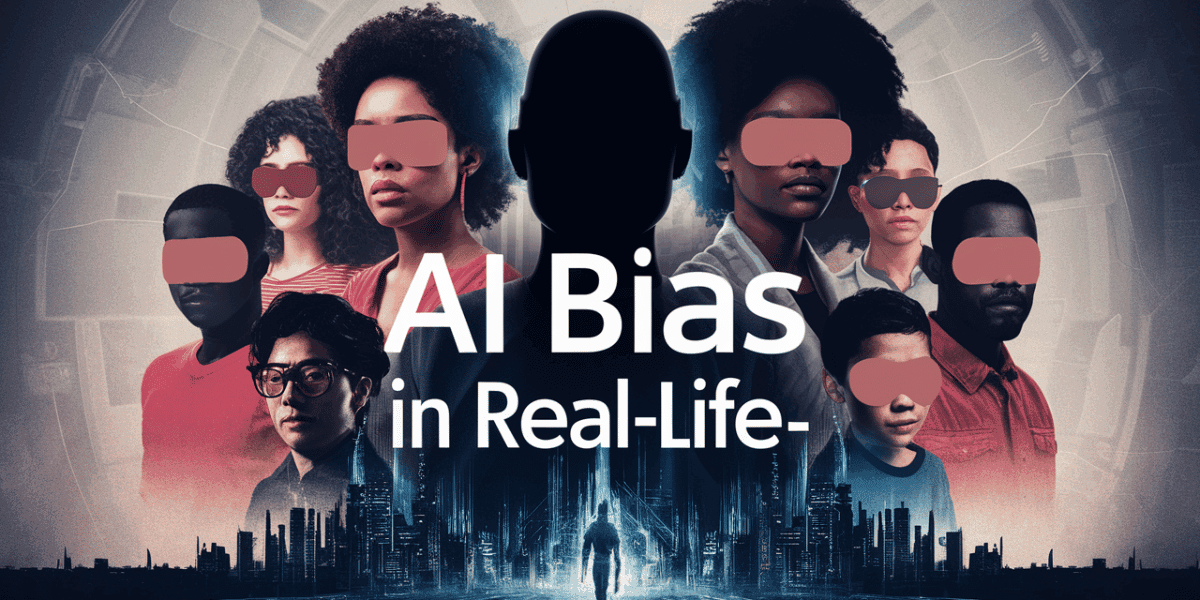
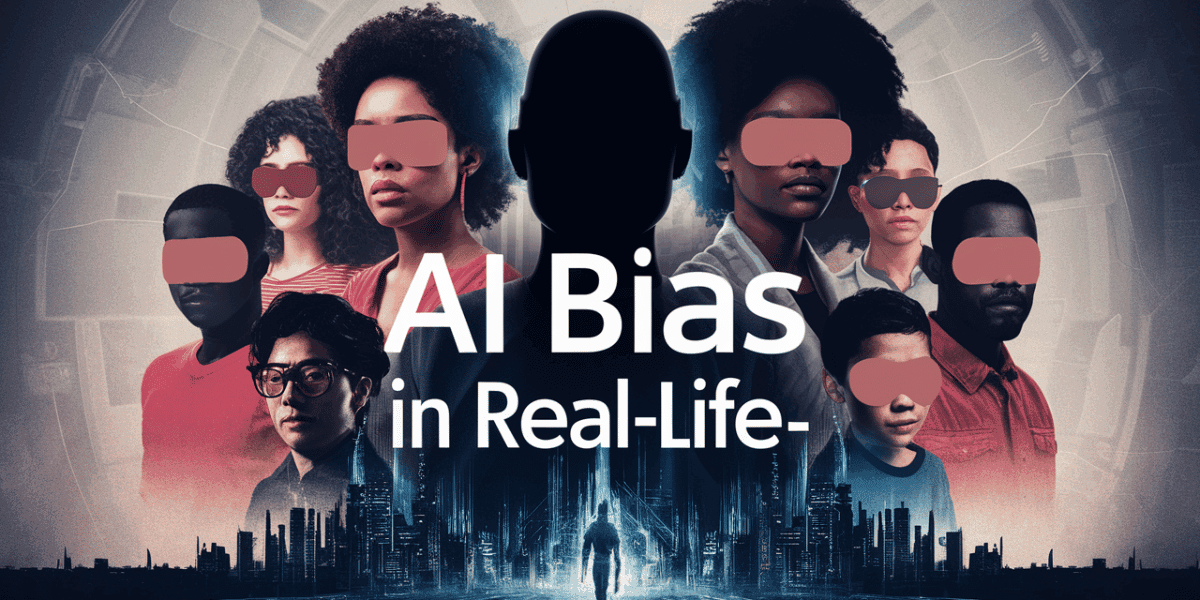
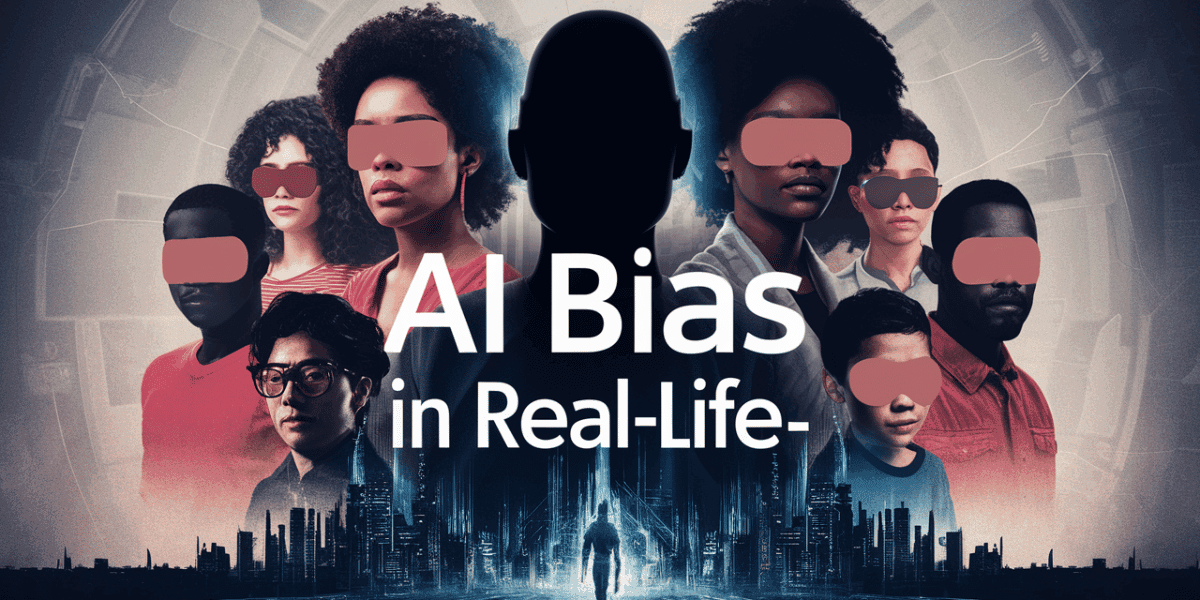
Examples And Strategies To Mitigate AI Bias In Real-Life

Akshat Gupta
Apr 14, 2024
Read the article




Understanding The Basics Of LLM Fine-tuning With Custom Data

Rehan Asif
Apr 13, 2024
Read the article
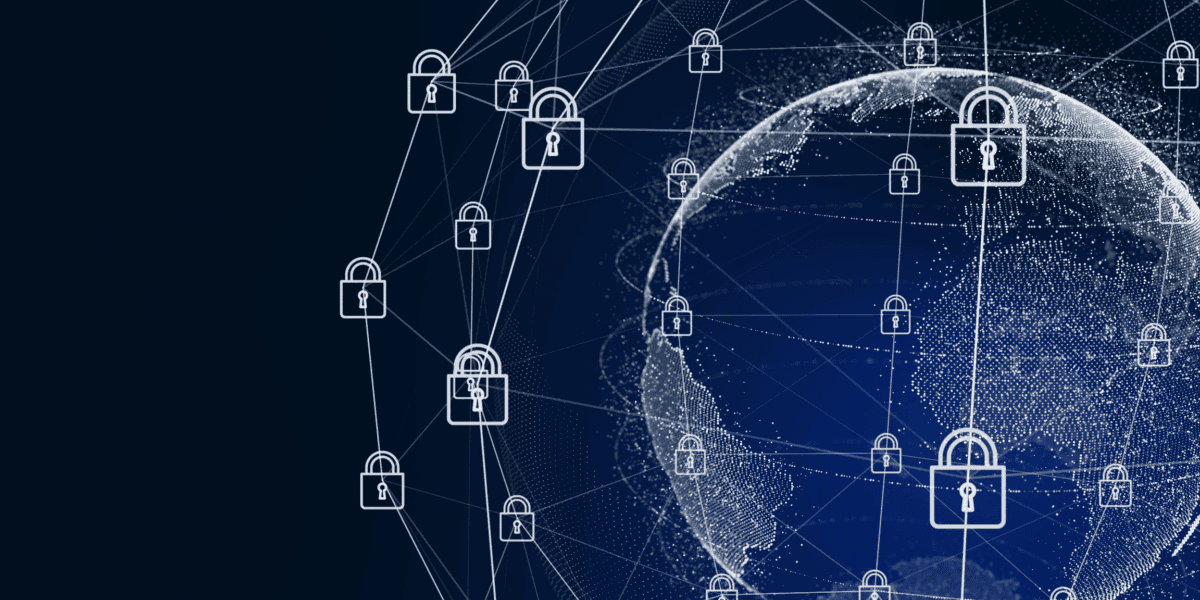
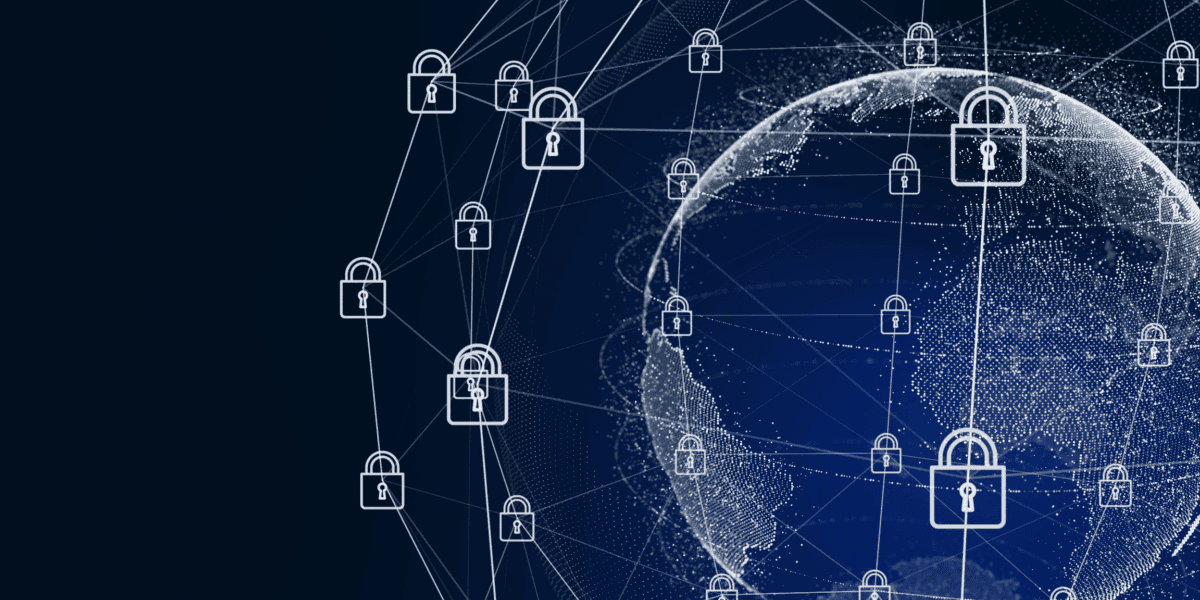
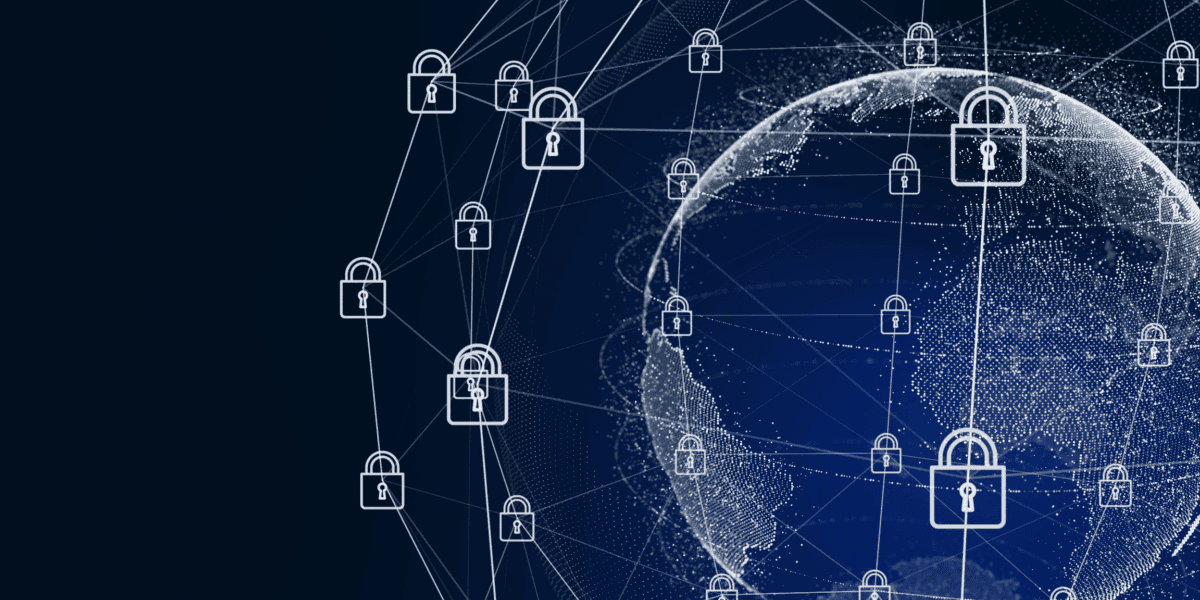
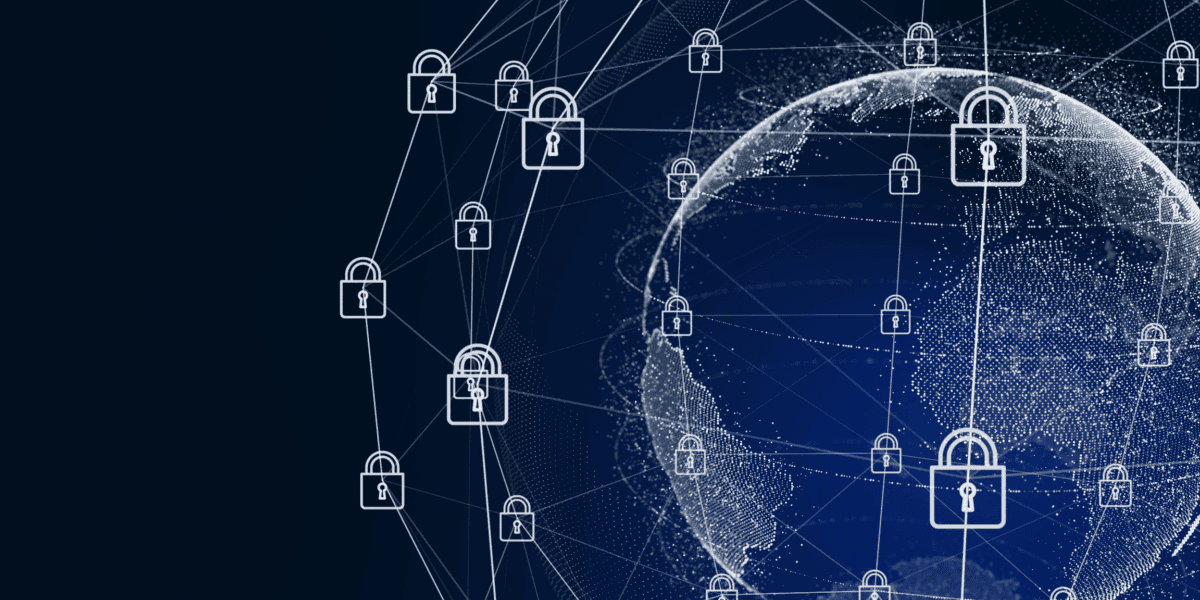
Overview Of Key Concepts In AI Safety And Security
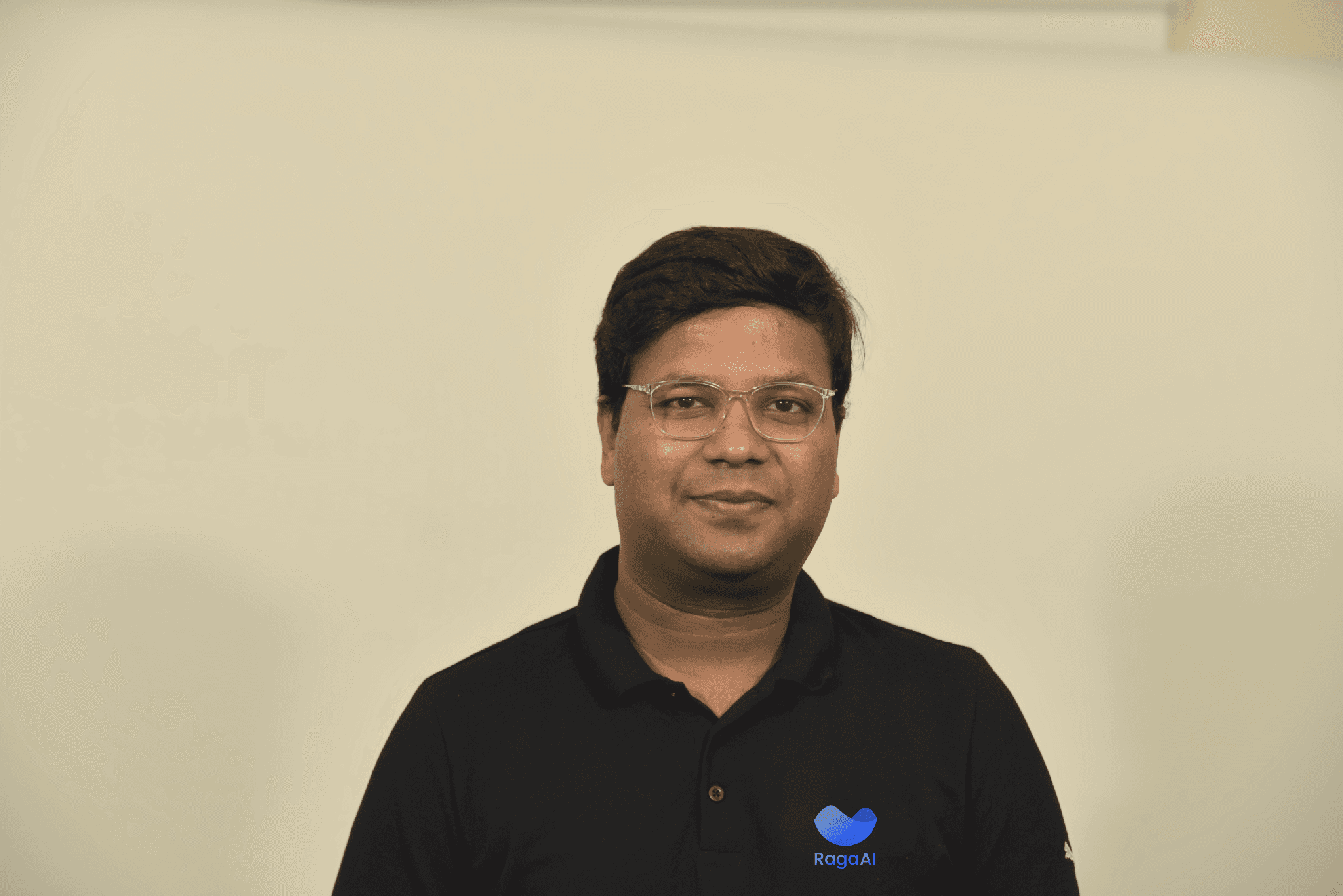
Jigar Gupta
Apr 12, 2024
Read the article




Understanding Hallucinations In LLMs

Rehan Asif
Apr 7, 2024
Read the article




Demystifying FDA's Approach to AI/ML in Healthcare: Your Ultimate Guide
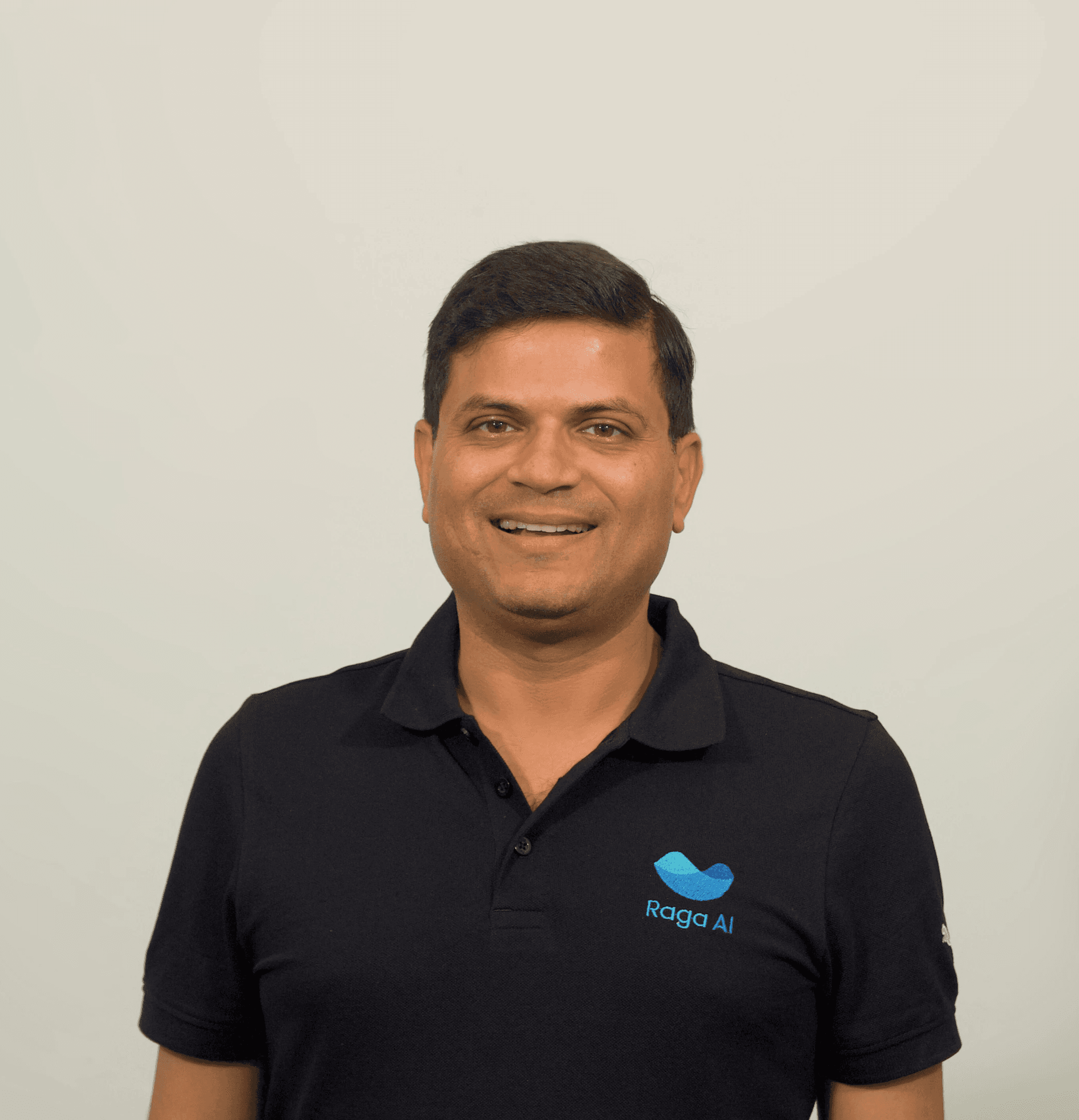
Gaurav Agarwal
Apr 4, 2024
Read the article




Navigating AI Governance in Aerospace Industry

Akshat Gupta
Apr 3, 2024
Read the article




The White House Executive Order on Safe and Trustworthy AI

Jigar Gupta
Mar 29, 2024
Read the article




The EU AI Act - All you need to know
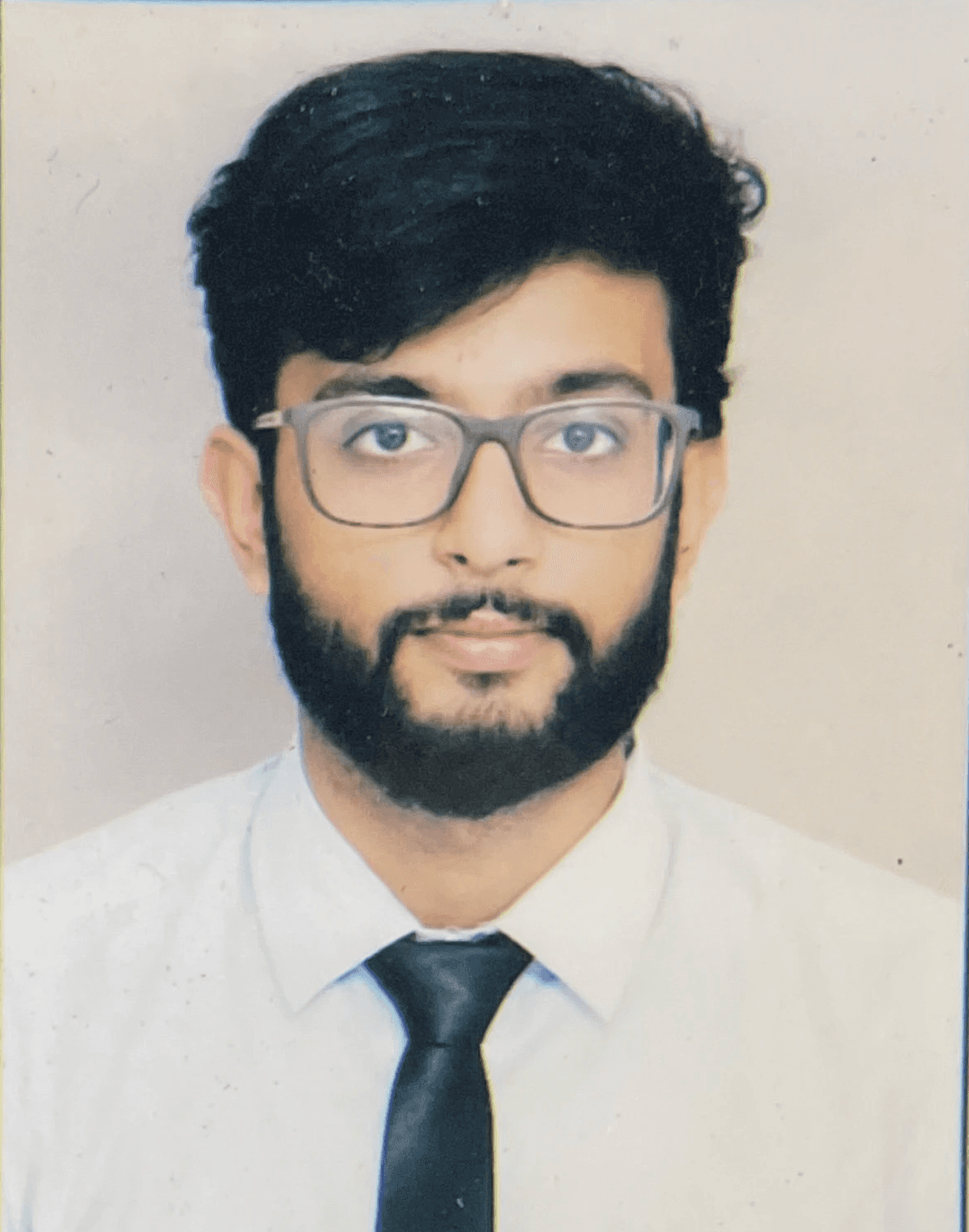
Akshat Gupta
Mar 27, 2024
Read the article
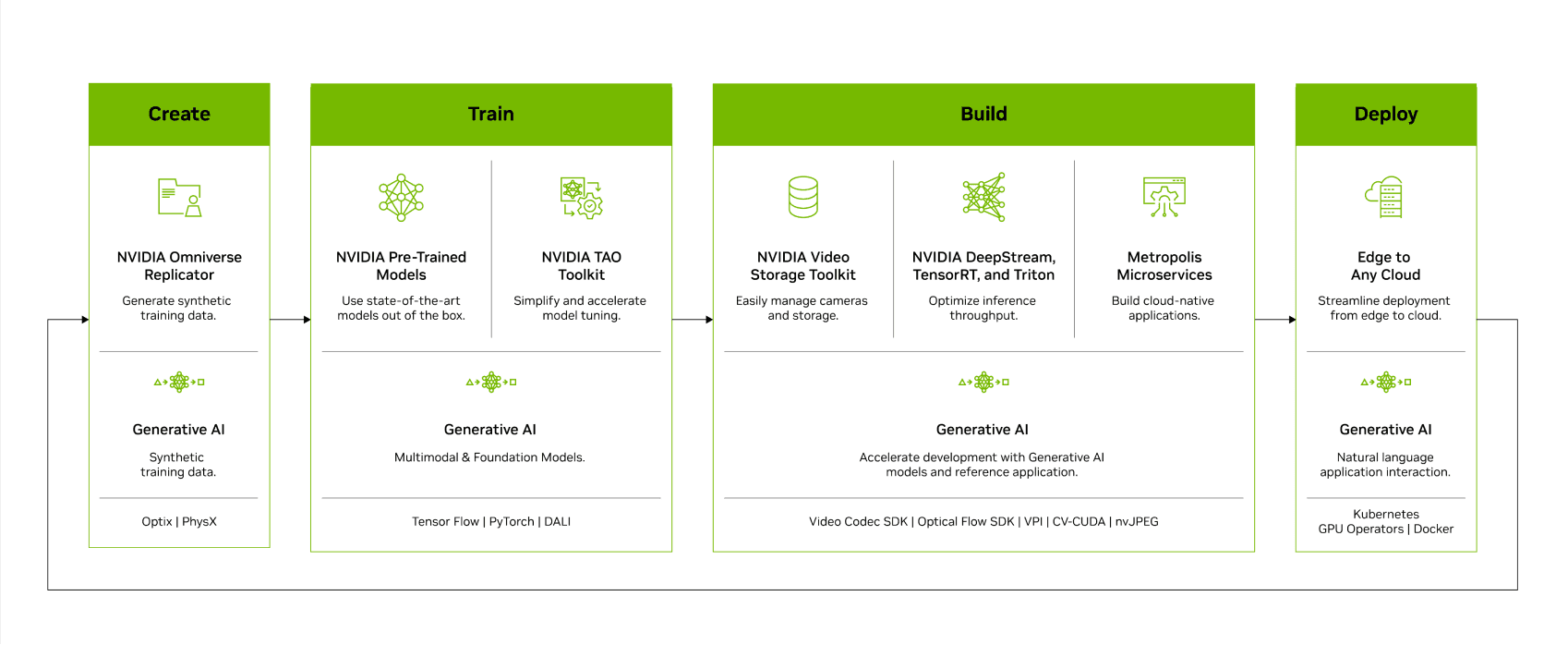
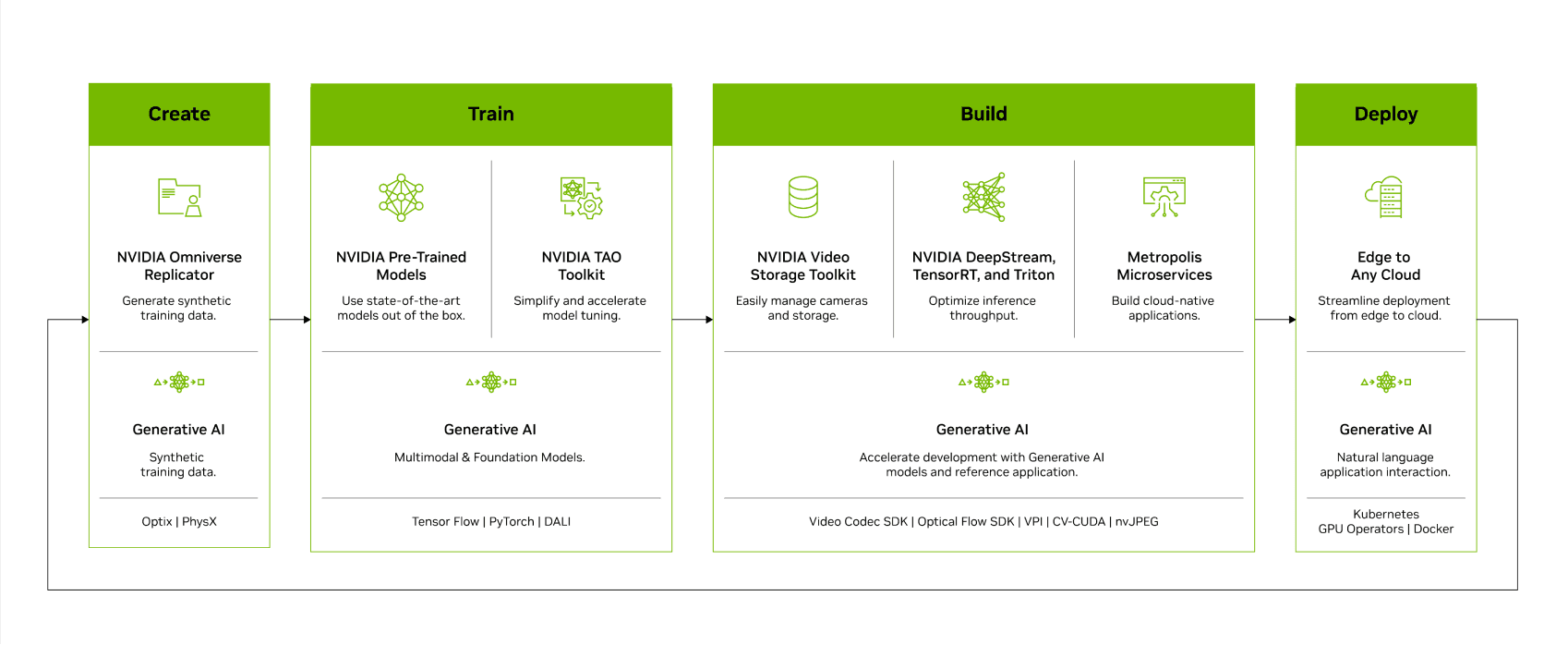
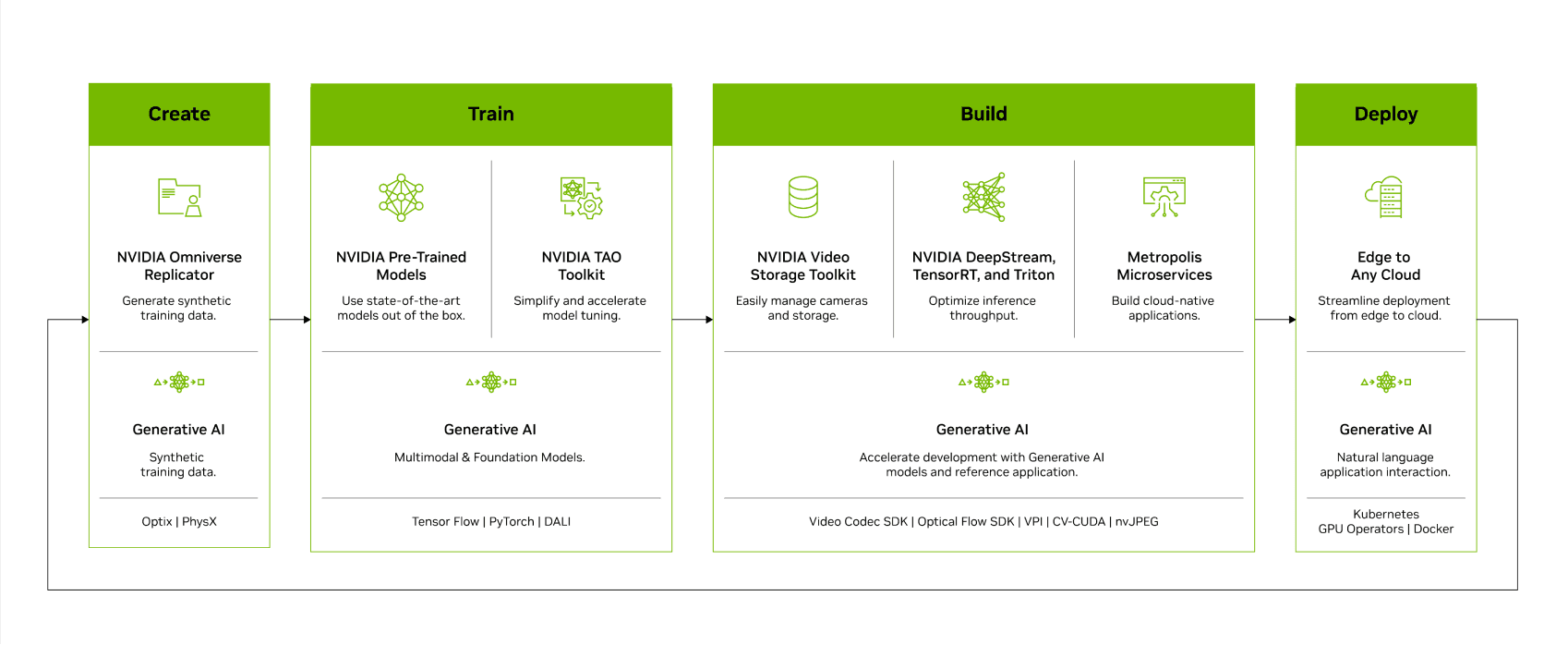
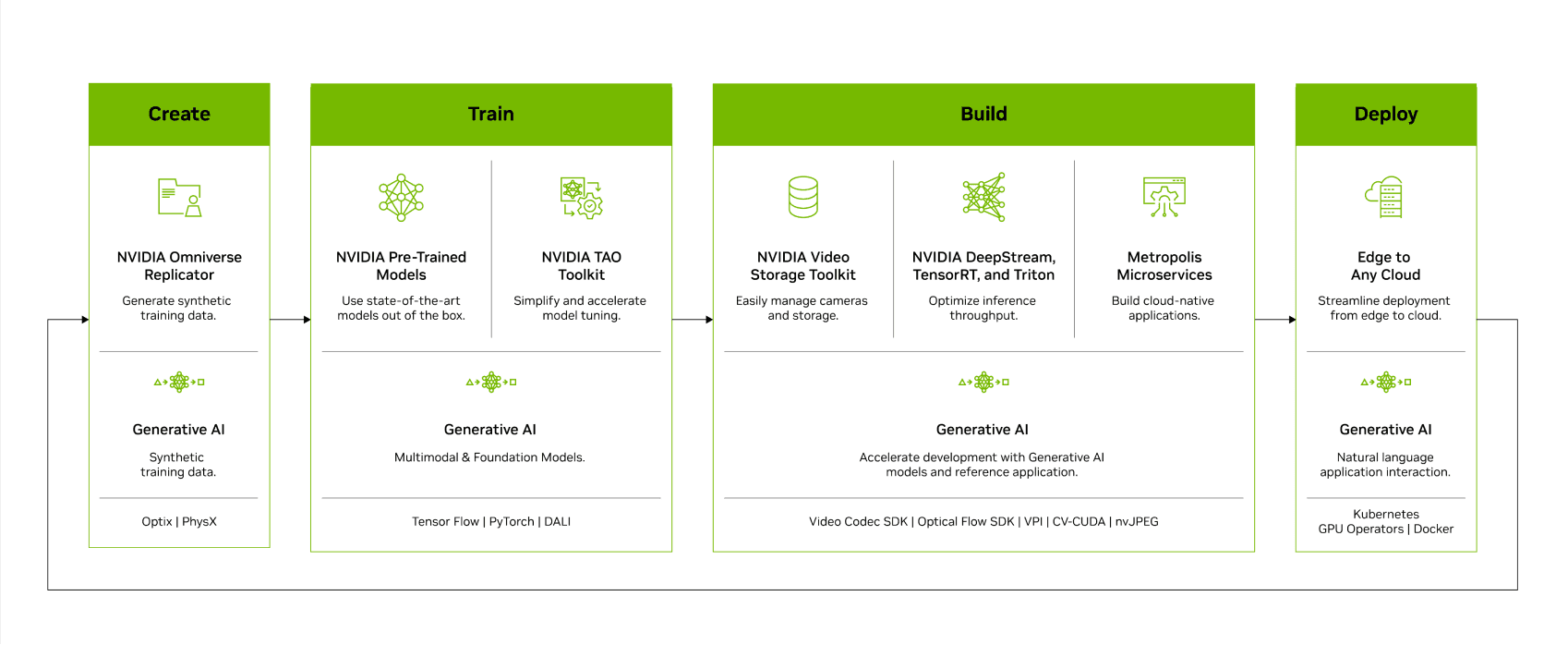
Enhancing Edge AI with RagaAI Integration on NVIDIA Metropolis

Siddharth Jain
Mar 15, 2024
Read the article




RagaAI releases the most comprehensive open-source LLM Evaluation and Guardrails package

Gaurav Agarwal
Mar 7, 2024
Read the article
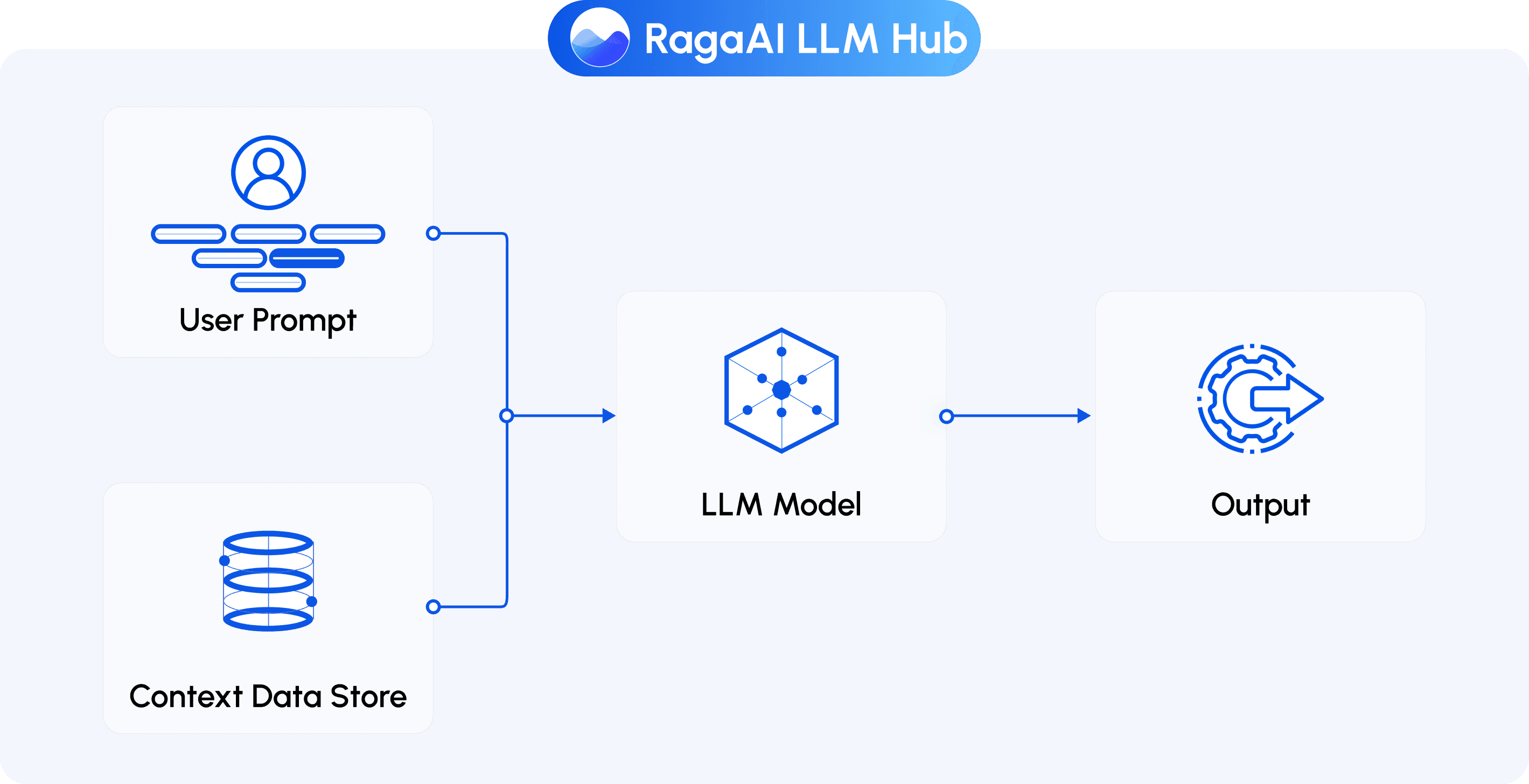
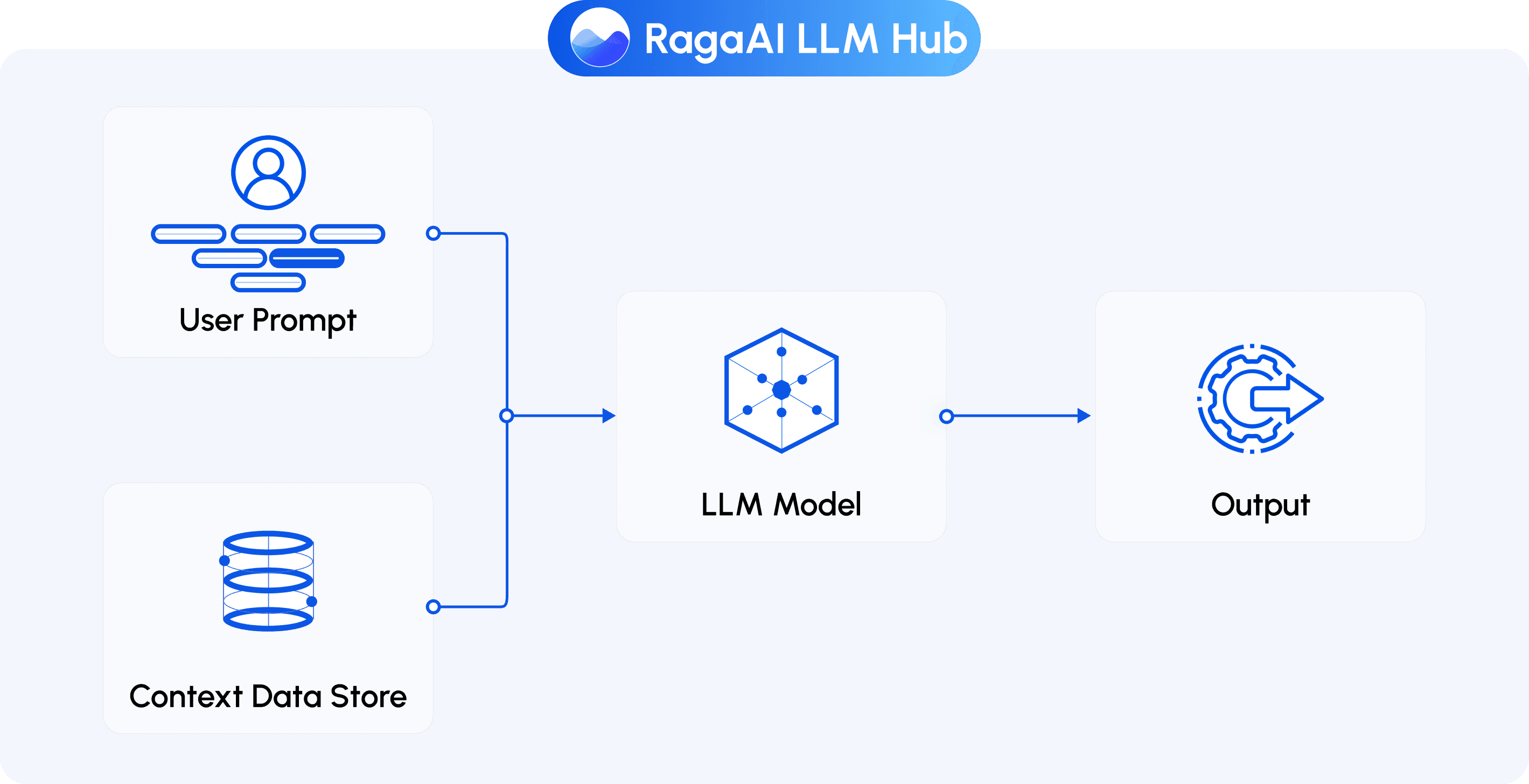
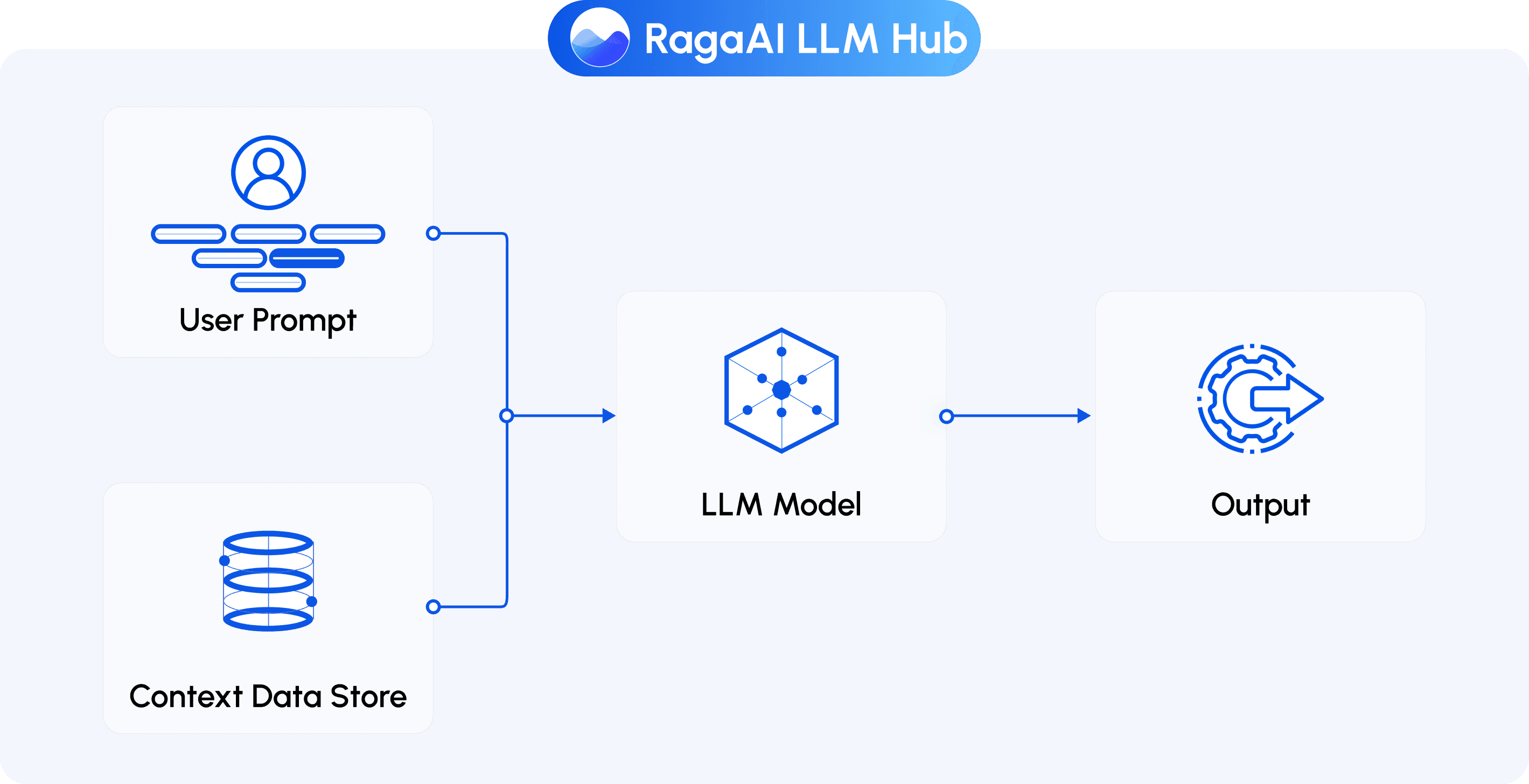
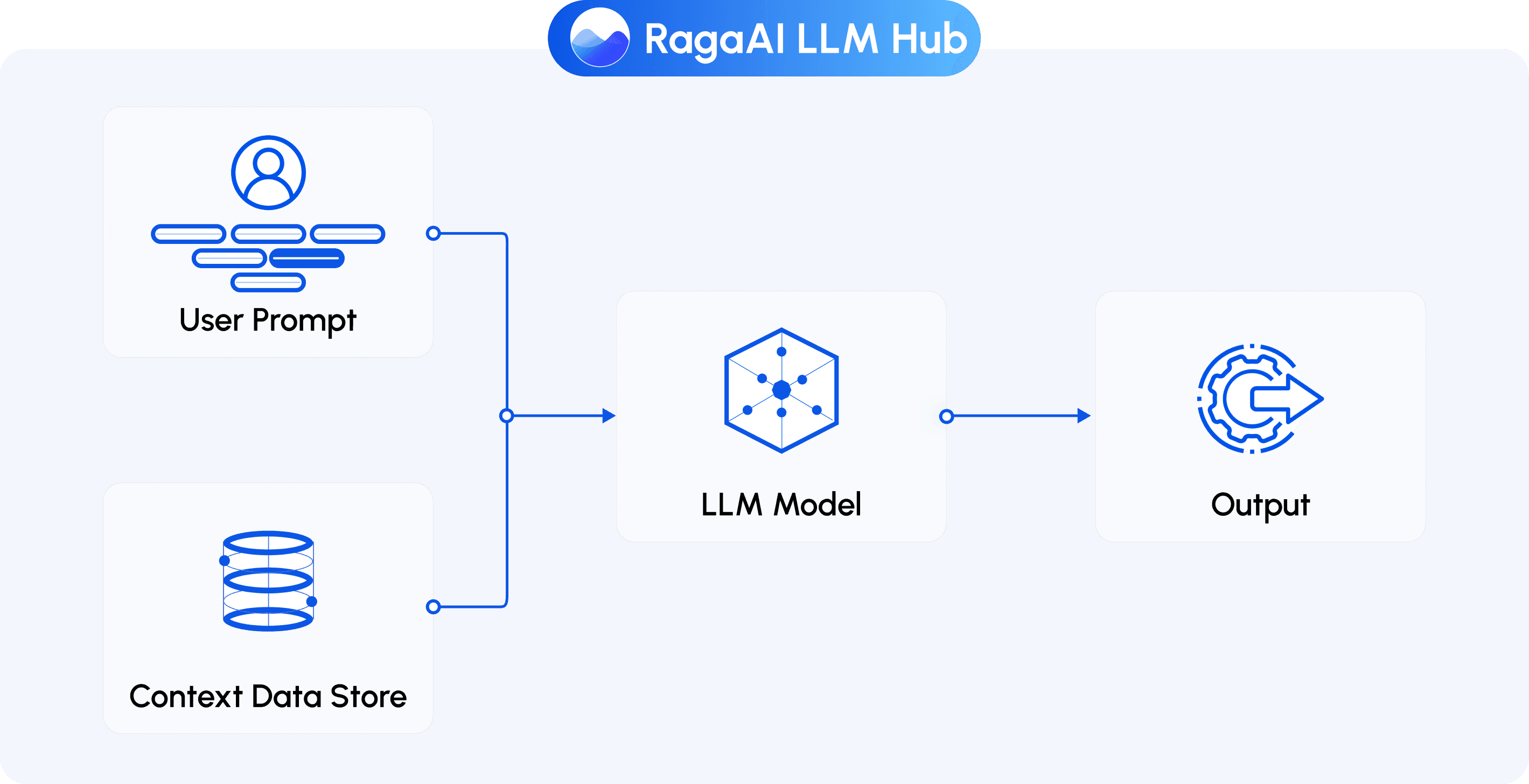
A Guide to Evaluating LLM Applications and enabling Guardrails using Raga-LLM-Hub

Rehan Asif
Mar 7, 2024
Read the article




Identifying edge cases within CelebA Dataset using RagaAI testing Platform

Rehan Asif
Feb 15, 2024
Read the article
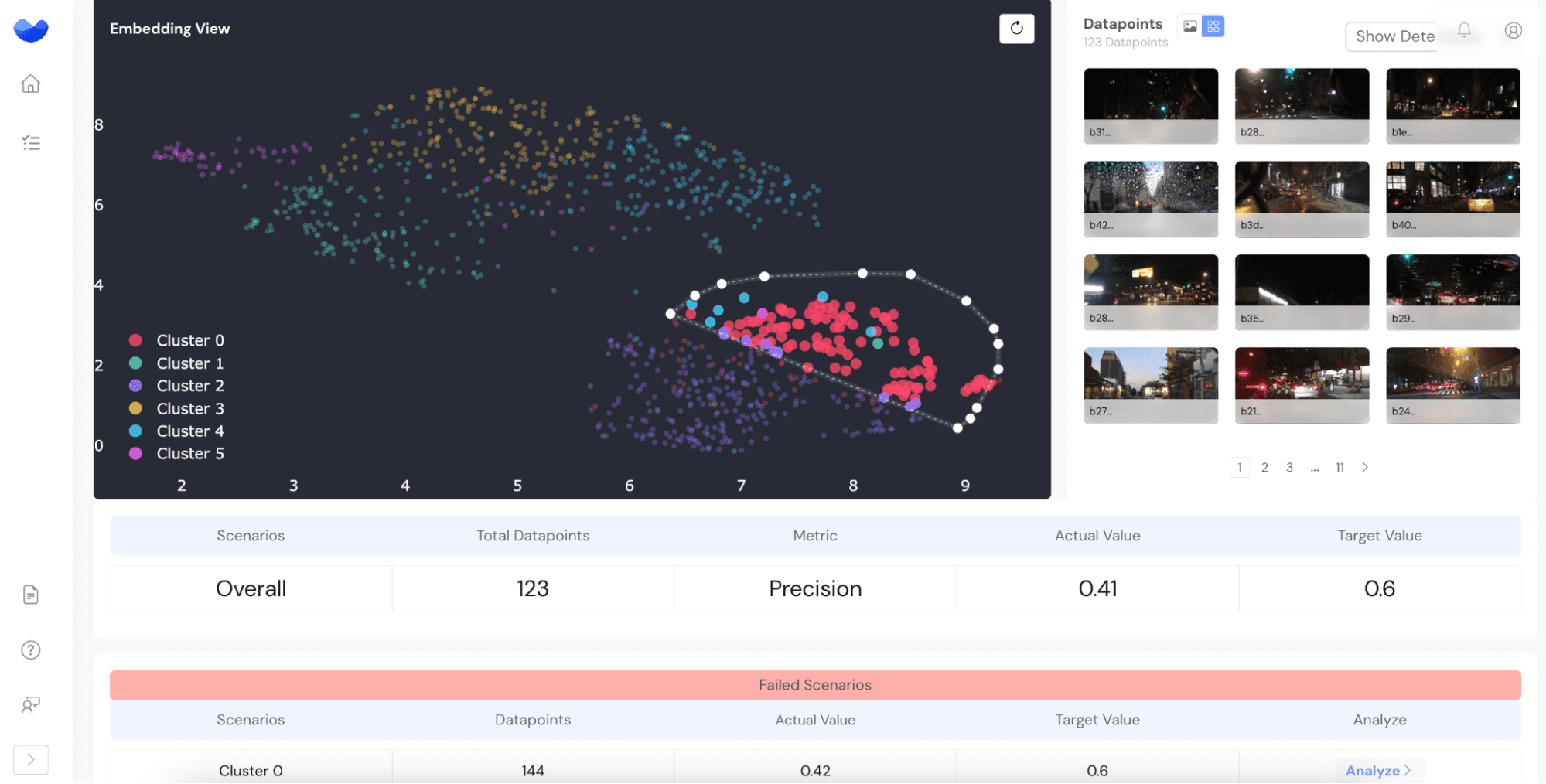
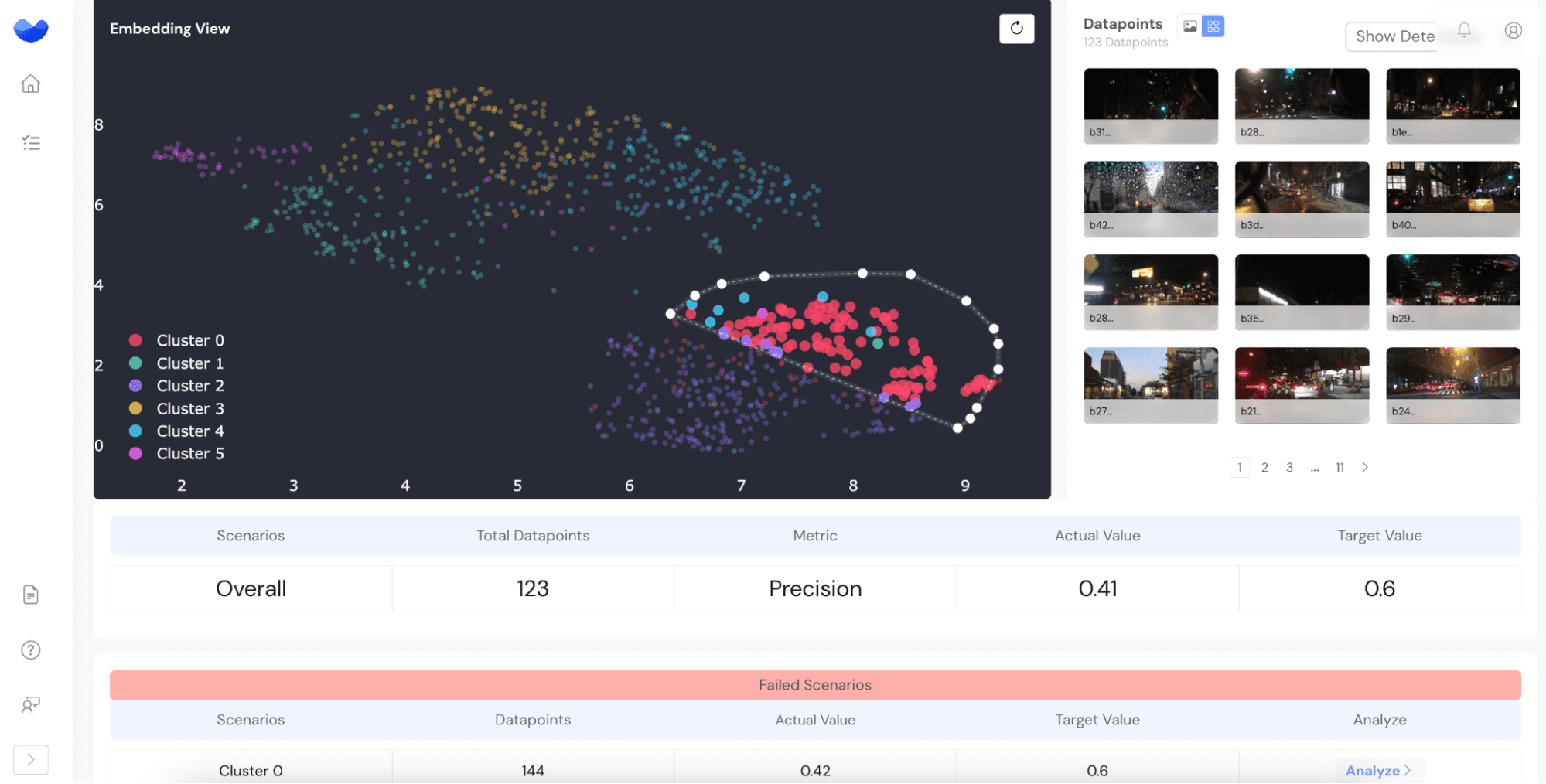
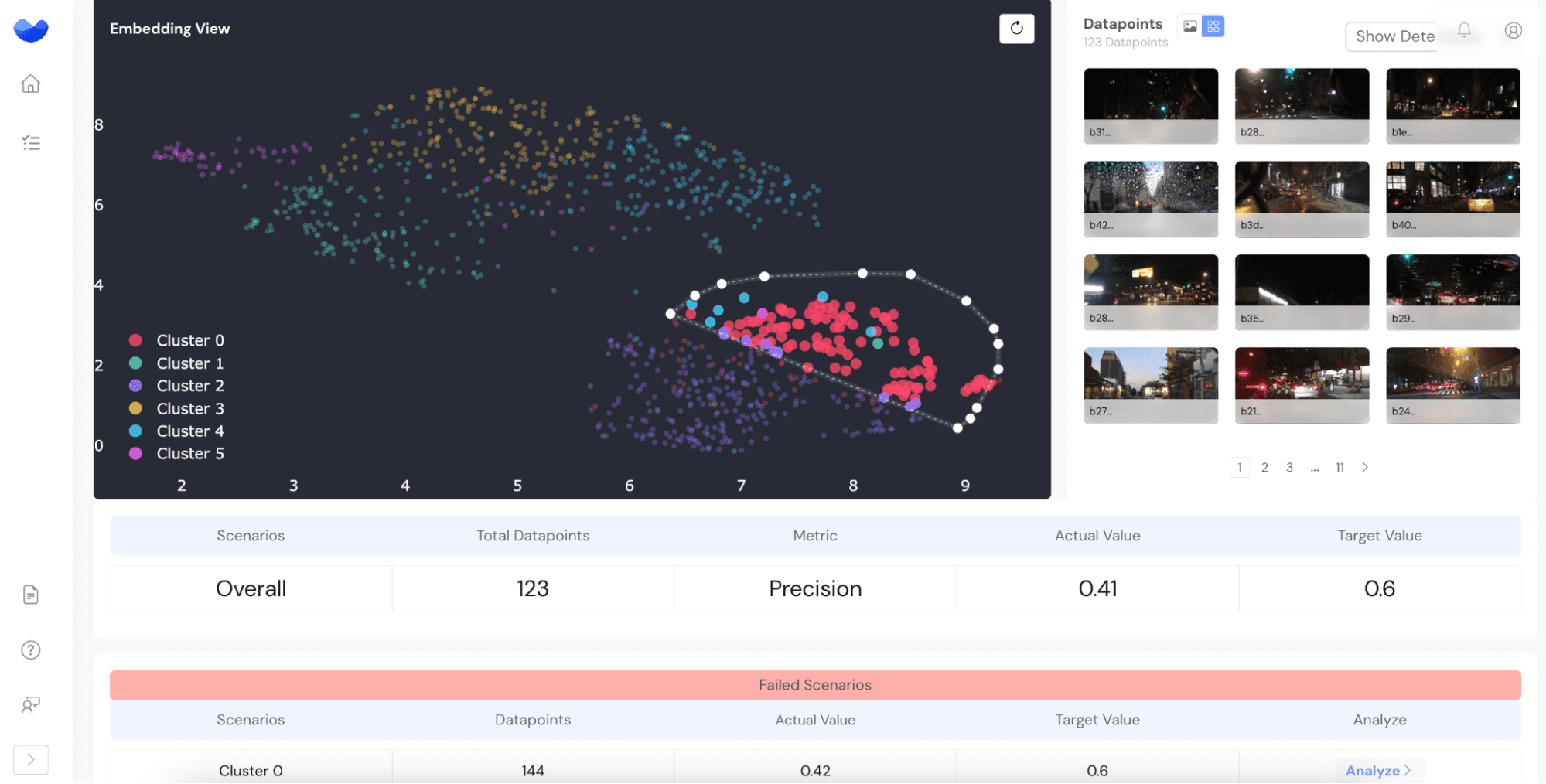
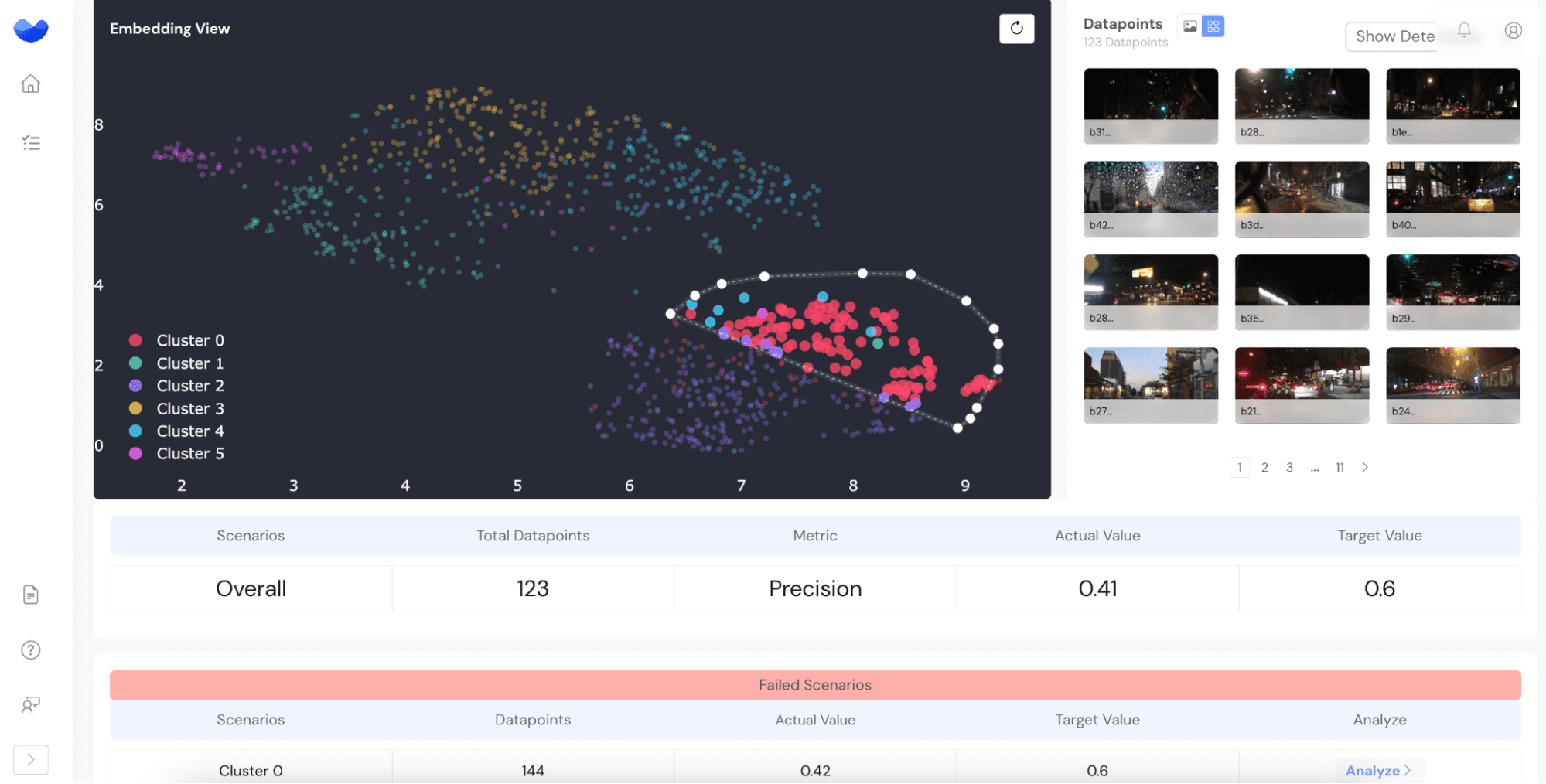
How to Detect and Fix AI Issues with RagaAI
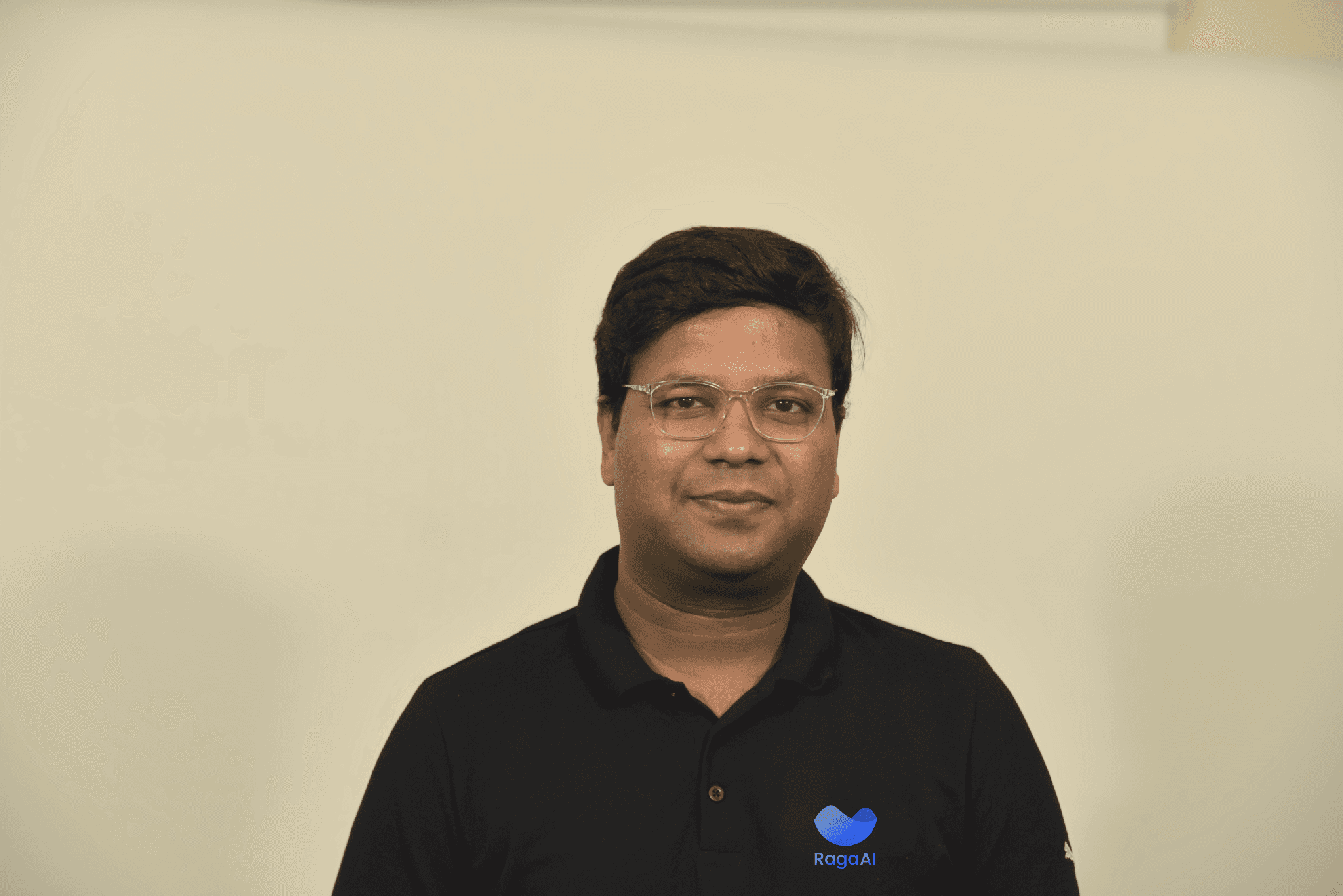
Jigar Gupta
Feb 16, 2024
Read the article
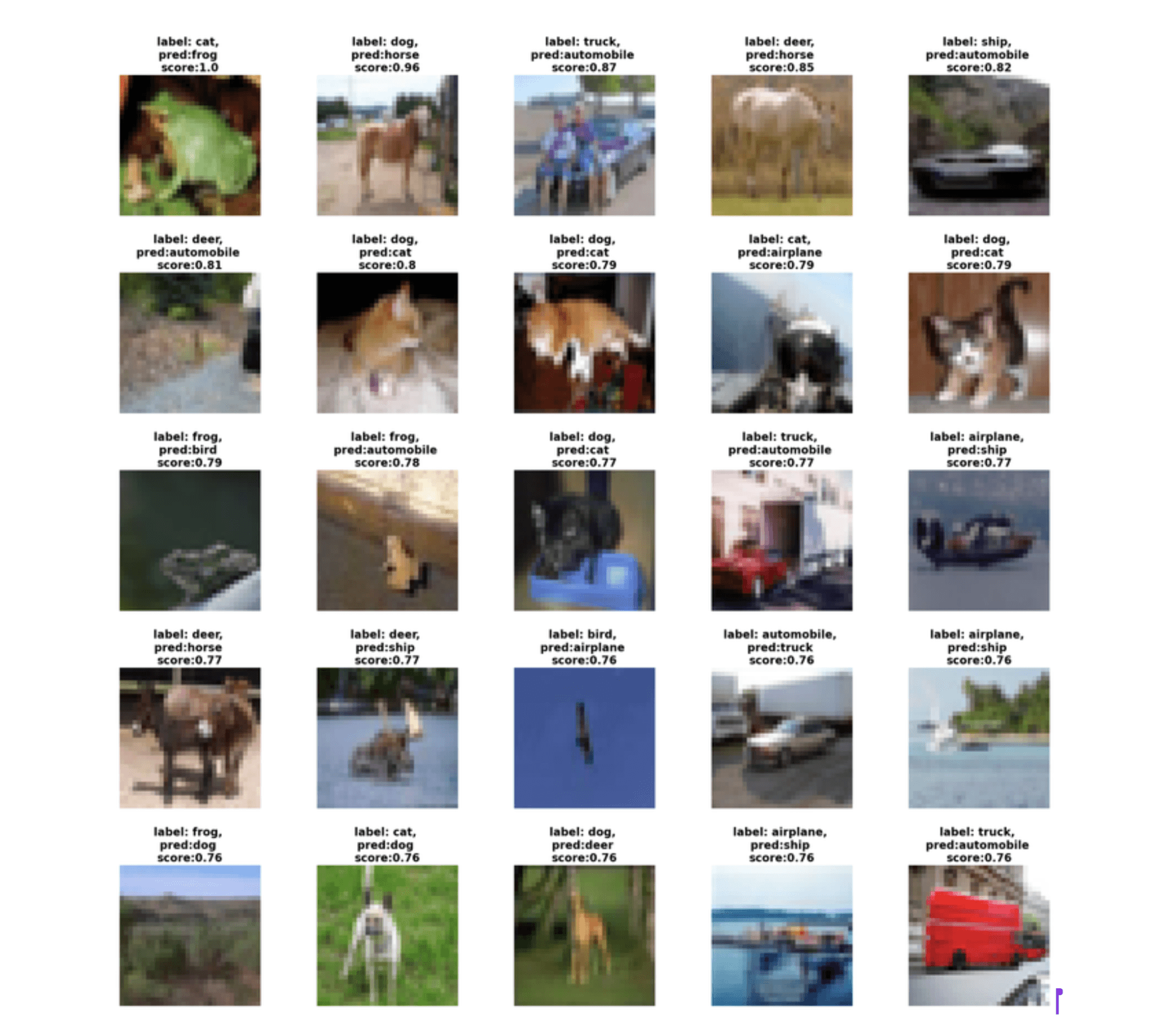
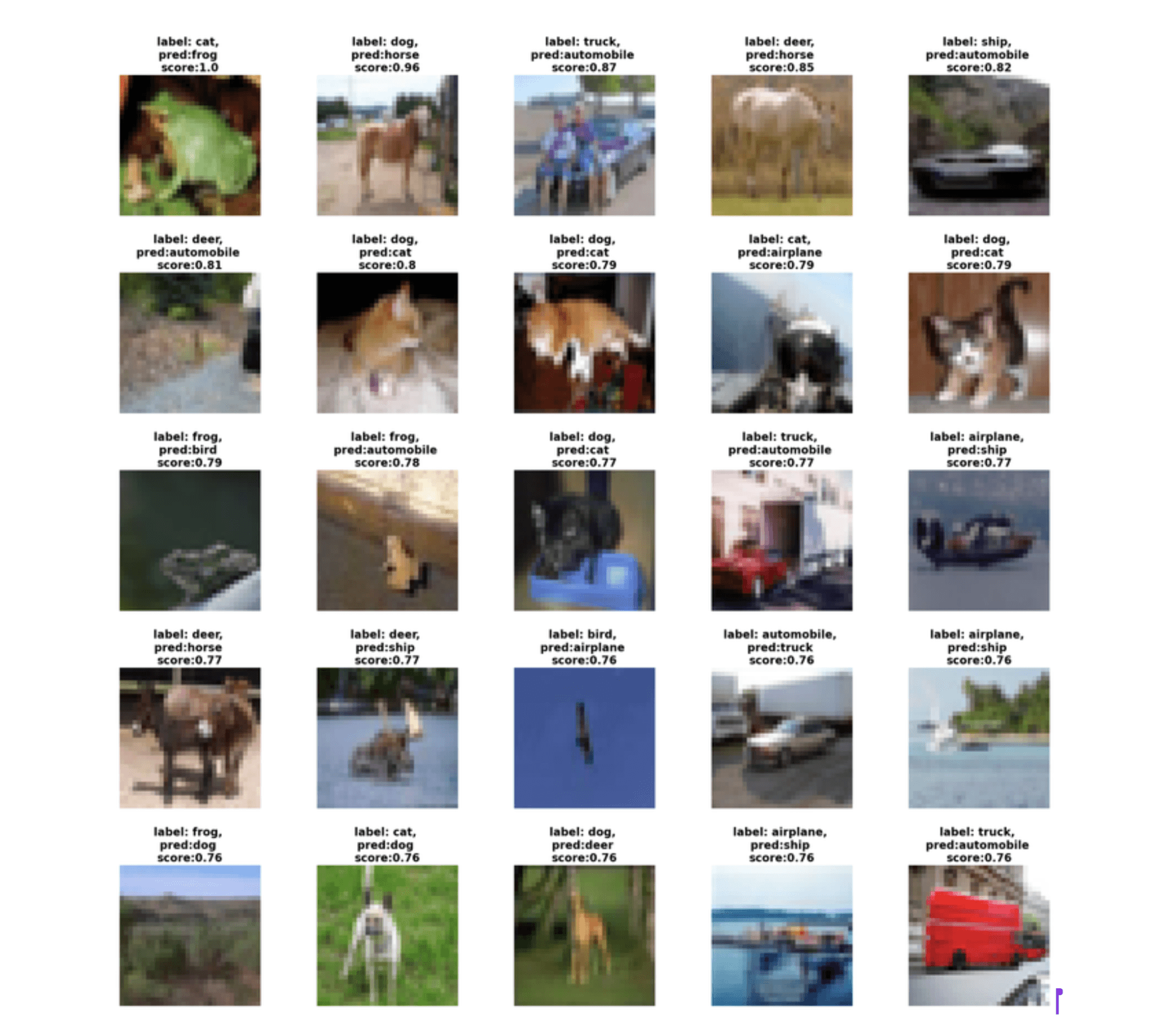
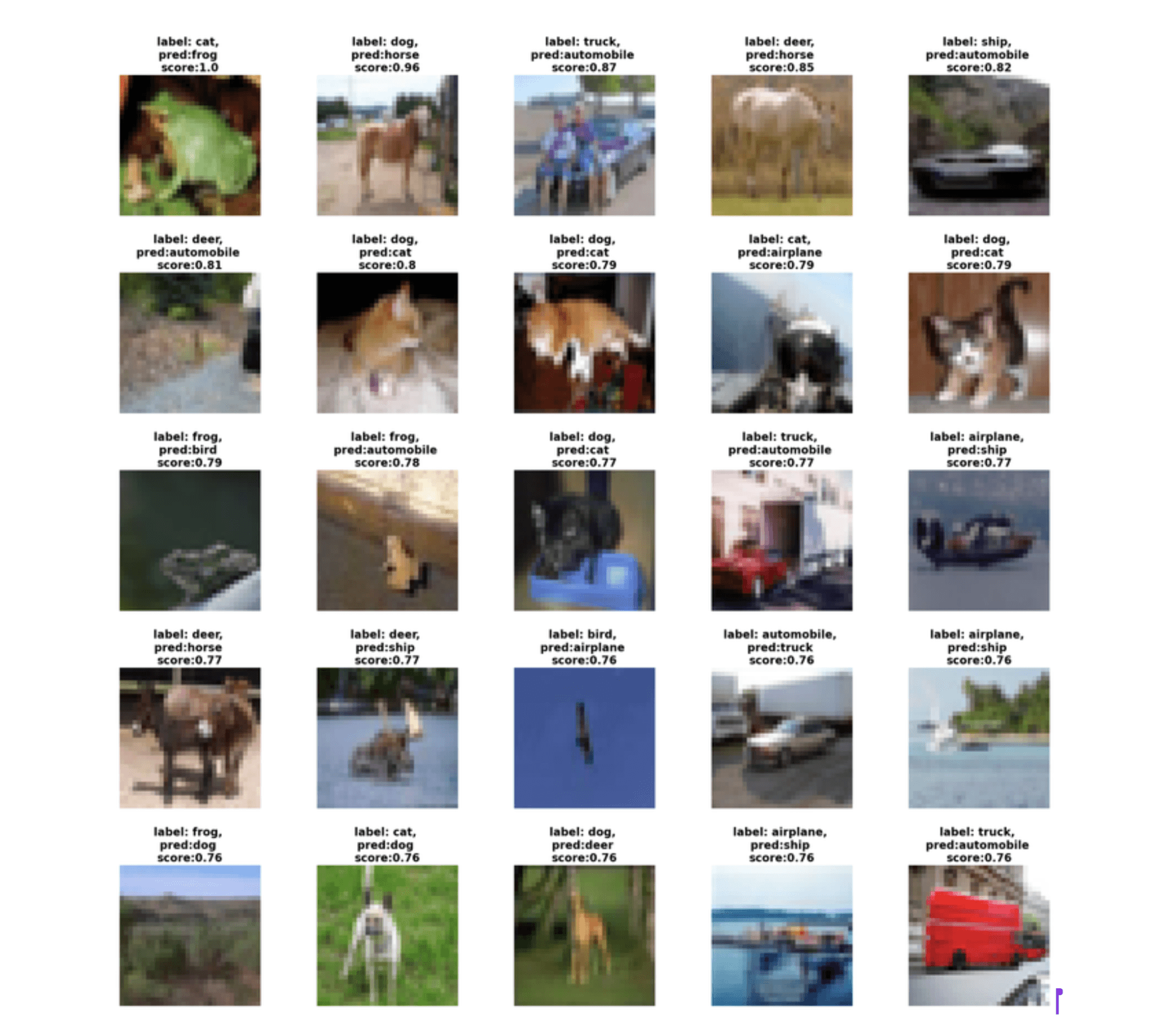
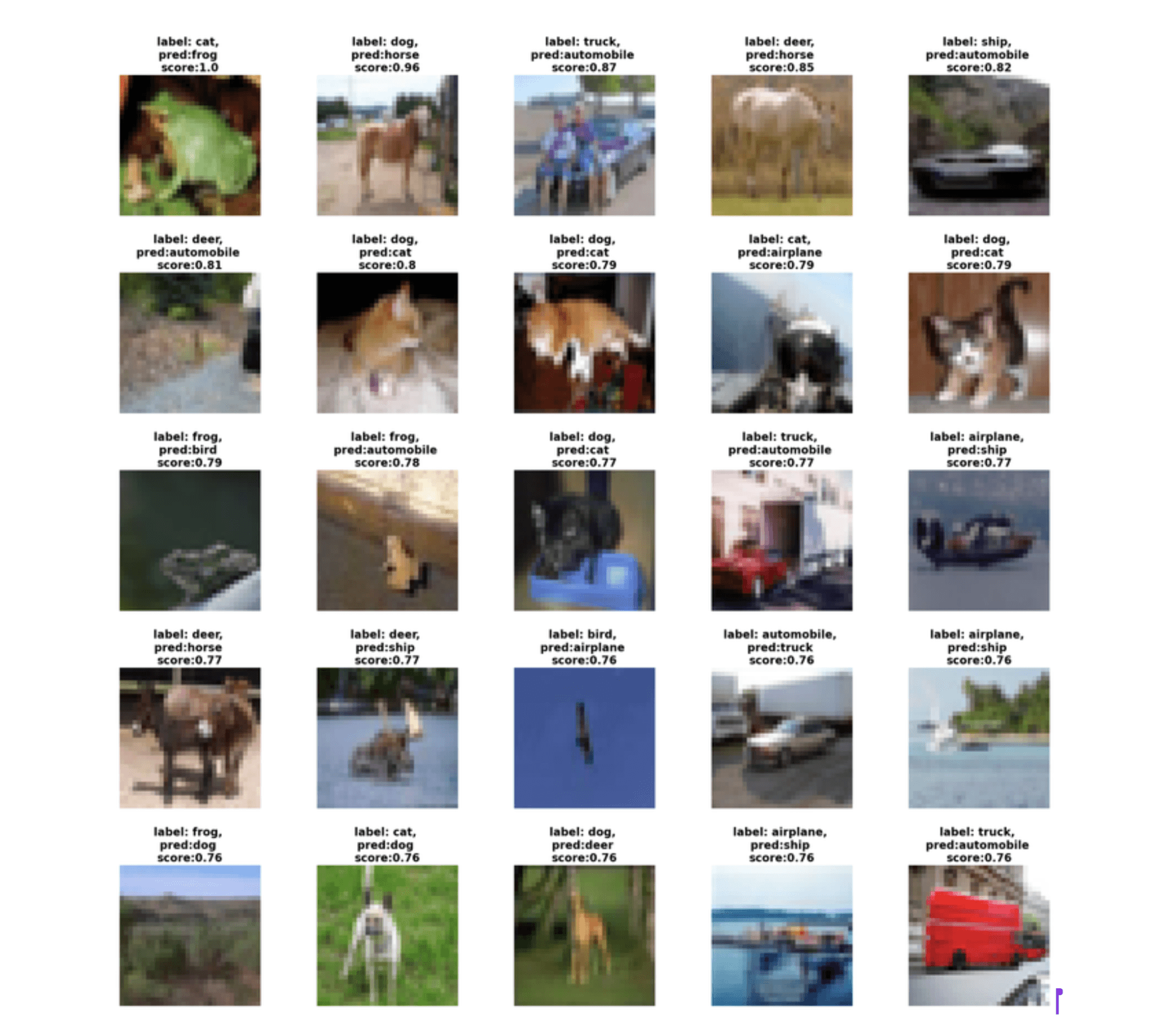
Detection of Labelling Issue in CIFAR-10 Dataset using RagaAI Platform
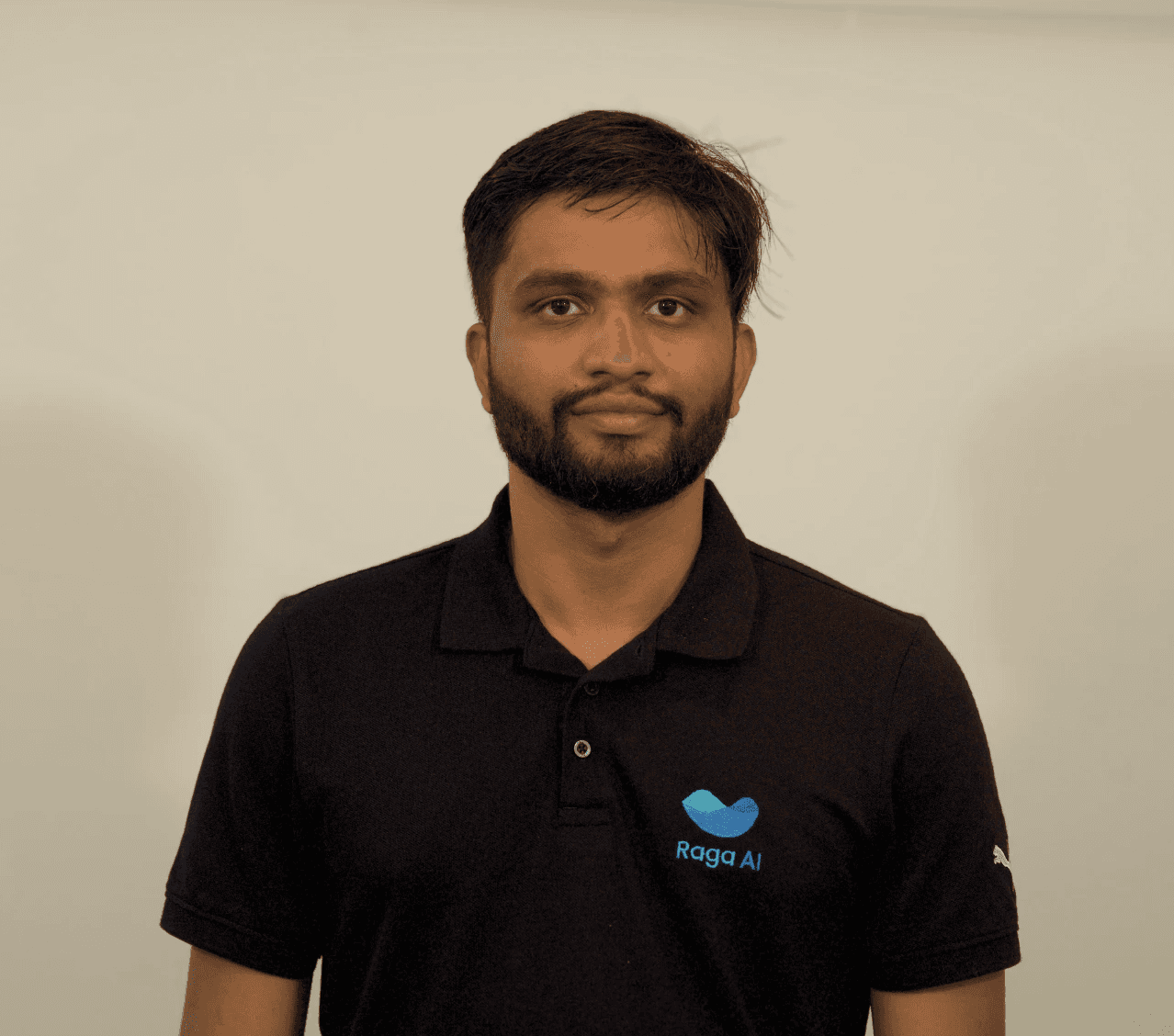
Rehan Asif
Feb 5, 2024
Read the article
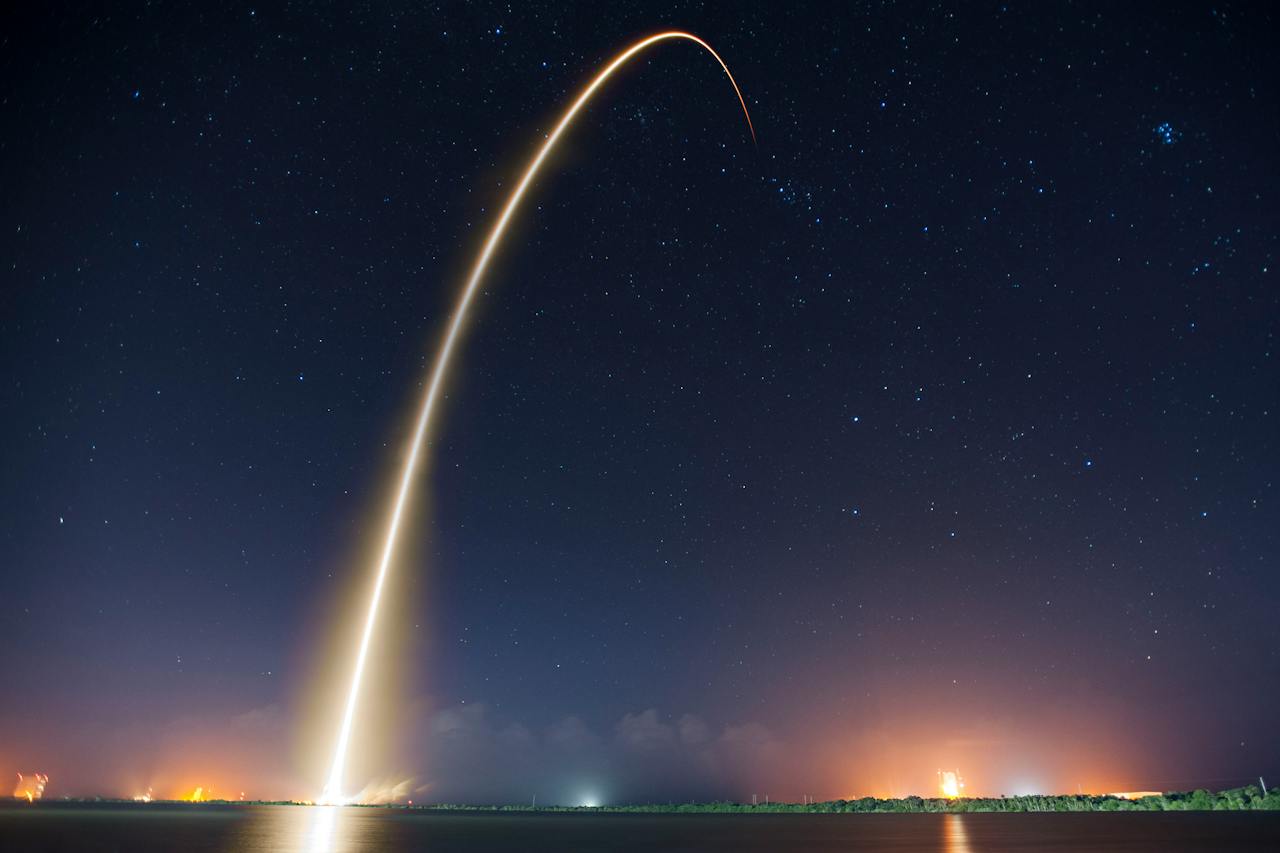
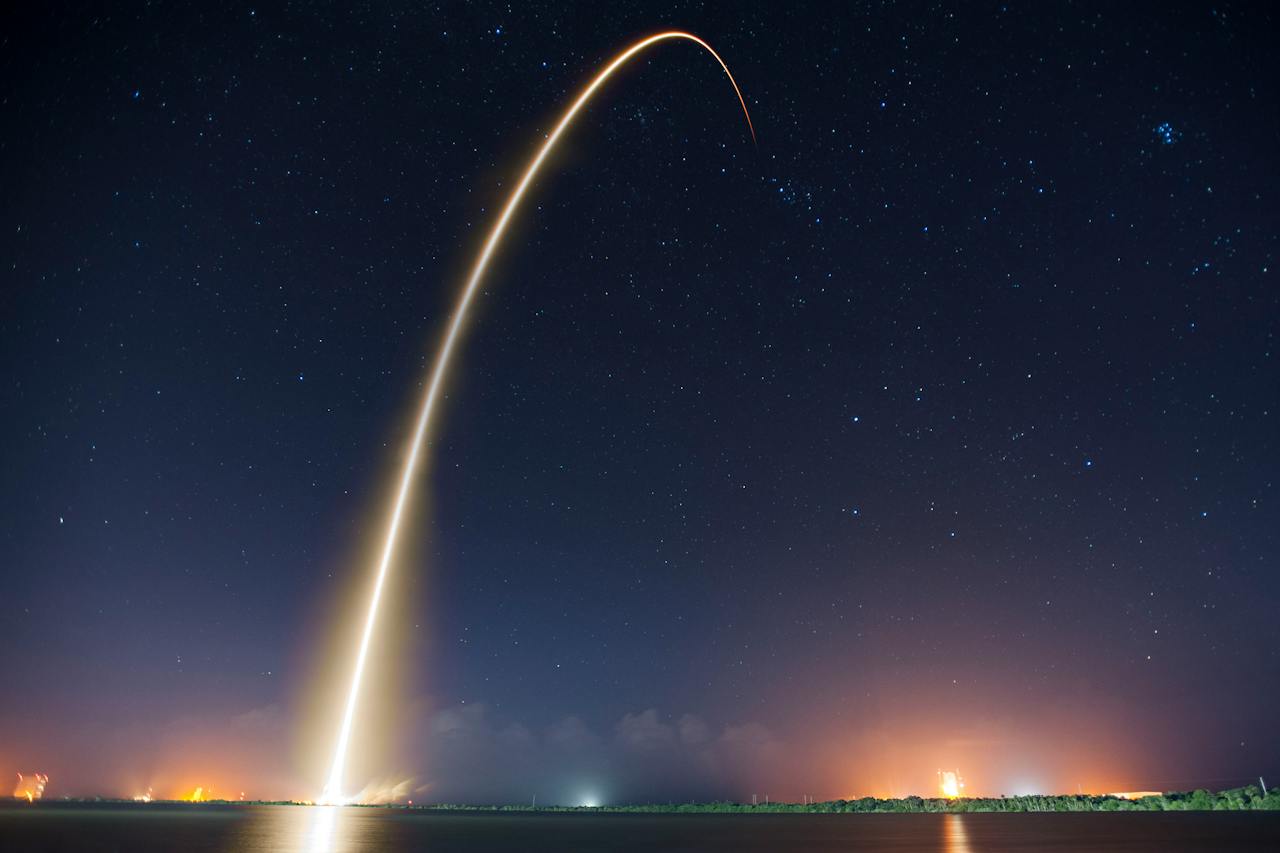
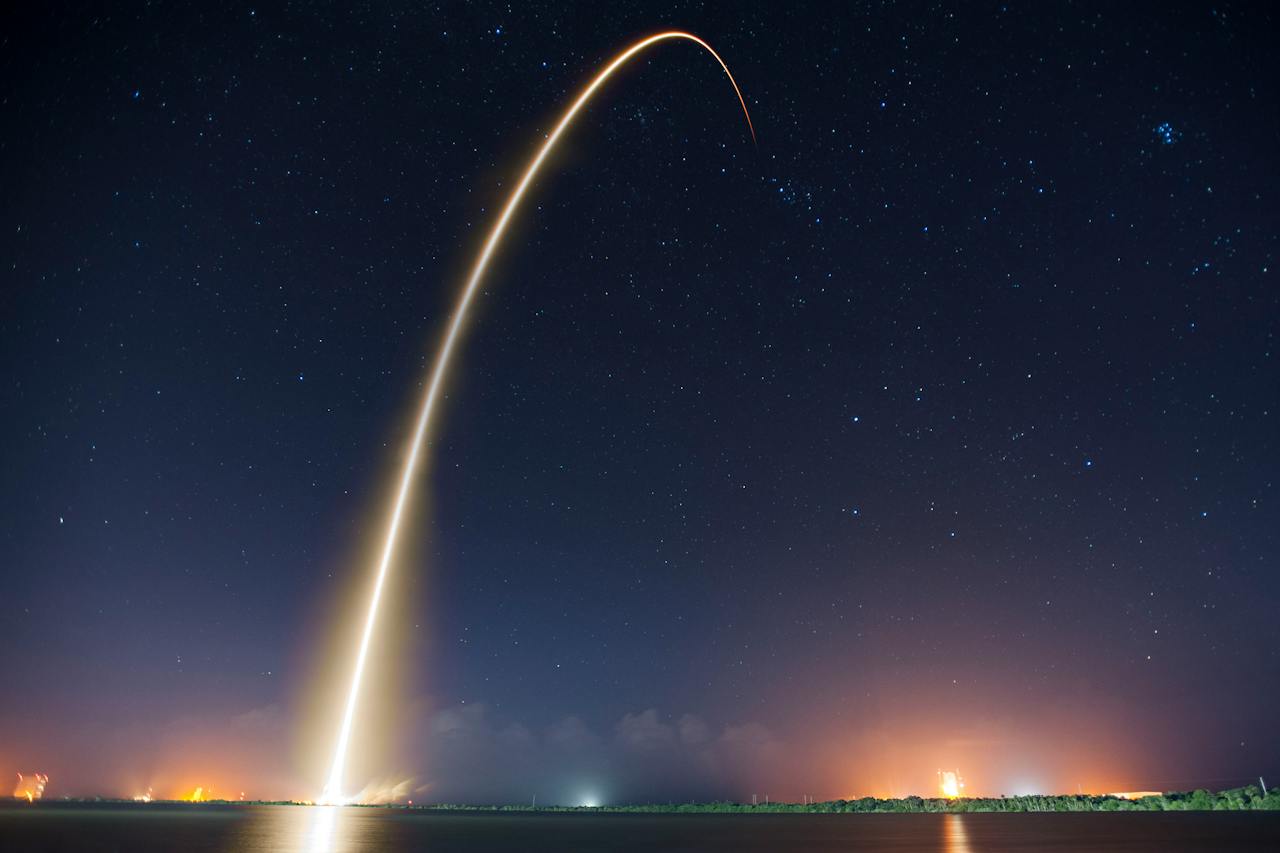
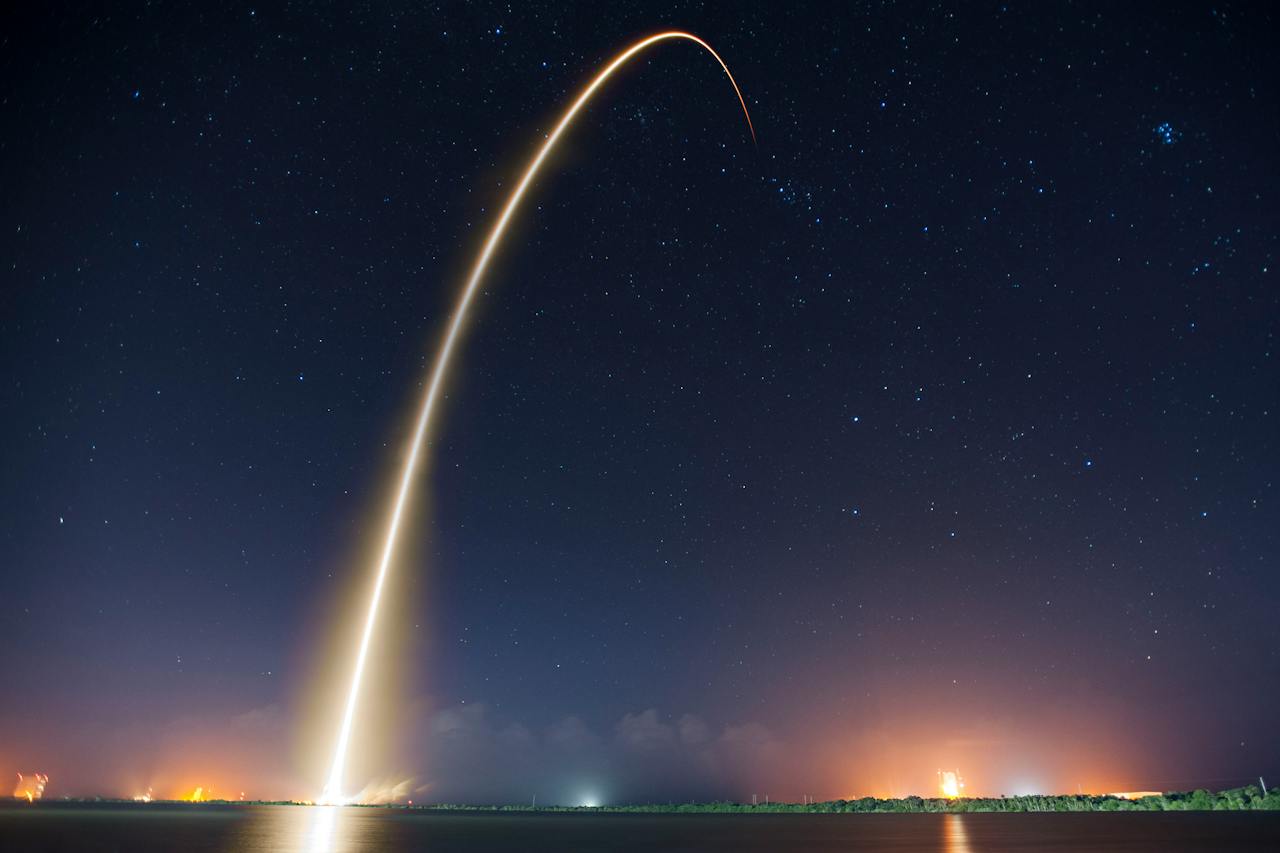
RagaAI emerges from Stealth with the most Comprehensive Testing Platform for AI
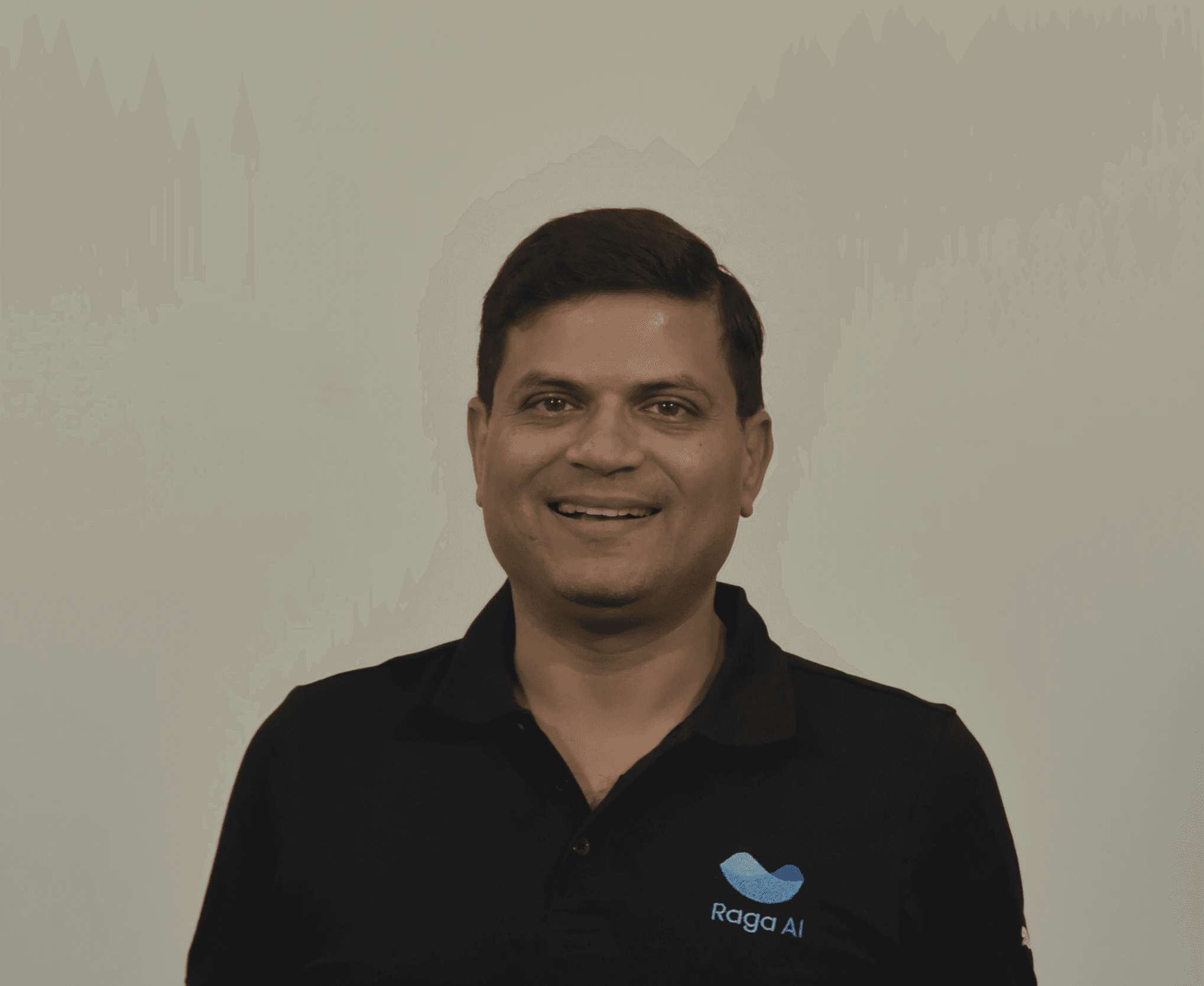
Gaurav Agarwal
Jan 23, 2024
Read the article




AI’s Missing Piece: Comprehensive AI Testing

Gaurav Agarwal
Jan 11, 2024
Read the article




Introducing RagaAI - The Future of AI Testing
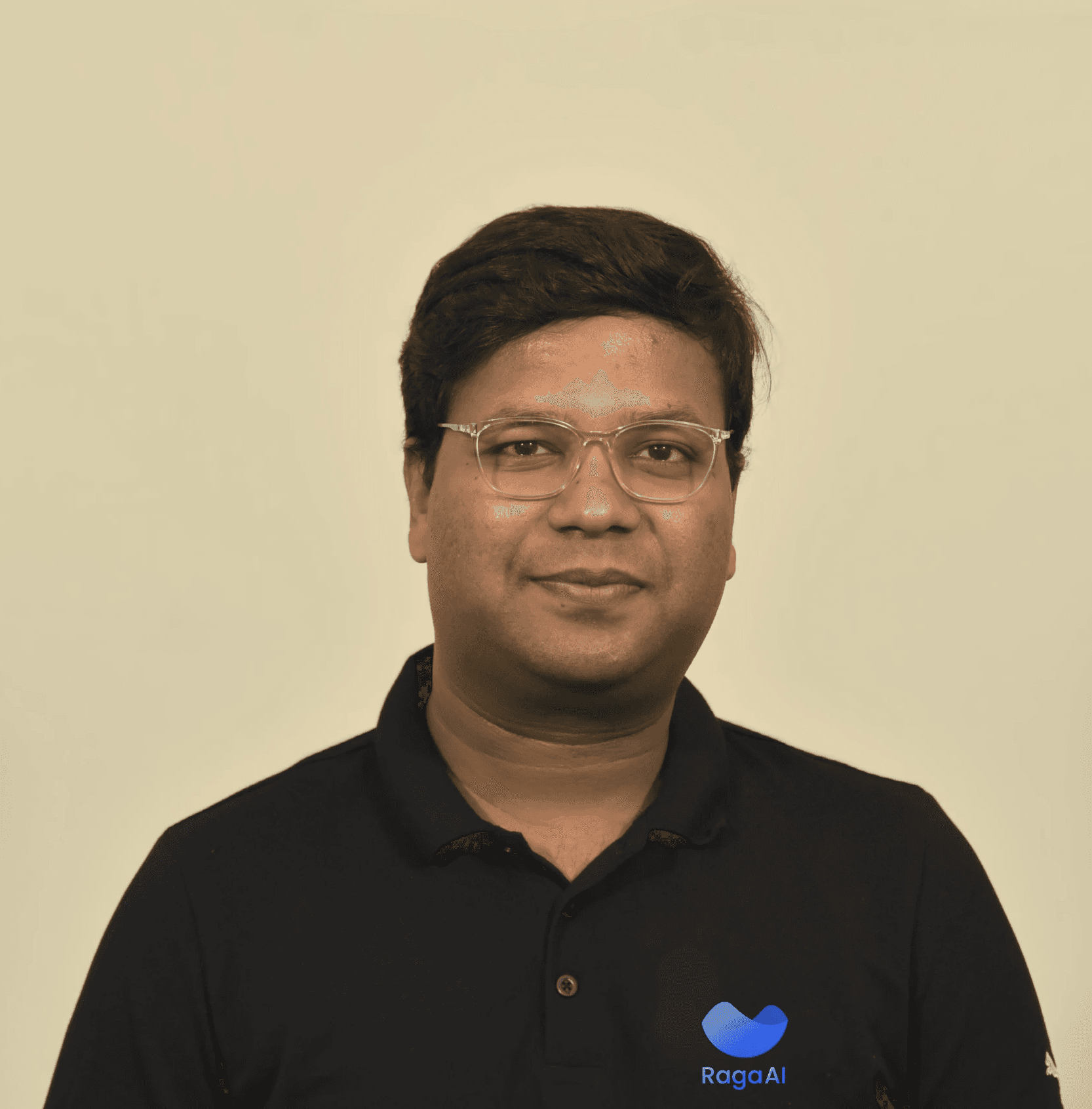
Jigar Gupta
Jan 14, 2024
Read the article




Introducing RagaAI DNA: The Multi-modal Foundation Model for AI Testing
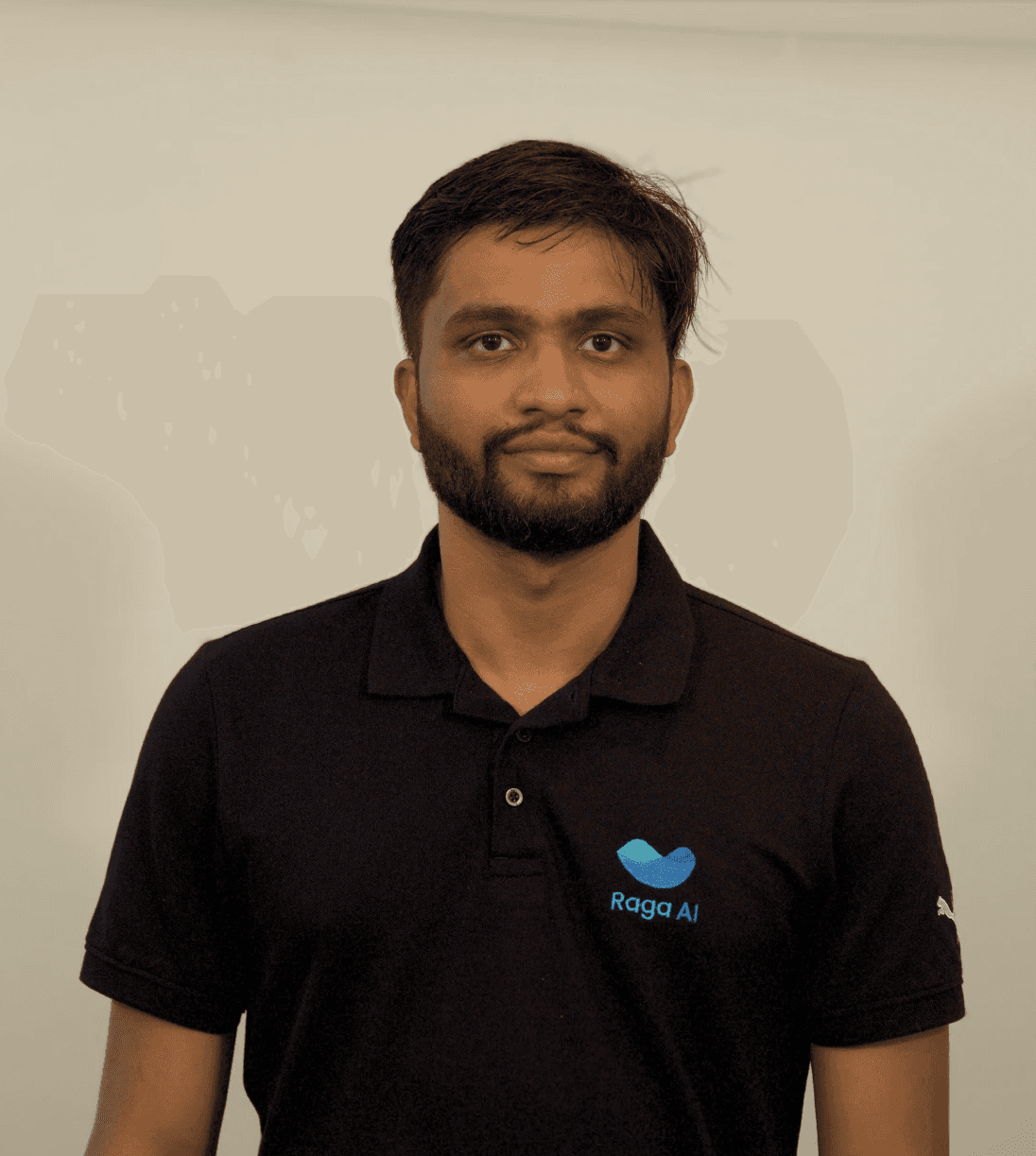
Rehan Asif
Jan 13, 2024
Read the article
Get Started With RagaAI®
Book a Demo
Schedule a call with AI Testing Experts

Get Started With RagaAI®
Book a Demo
Schedule a call with AI Testing Experts
