A Guide to Evaluating LLM Applications and enabling Guardrails using Raga-LLM-Hub
A Guide to Evaluating LLM Applications and enabling Guardrails using Raga-LLM-Hub
A Guide to Evaluating LLM Applications and enabling Guardrails using Raga-LLM-Hub
Rehan Asif
Mar 7, 2024
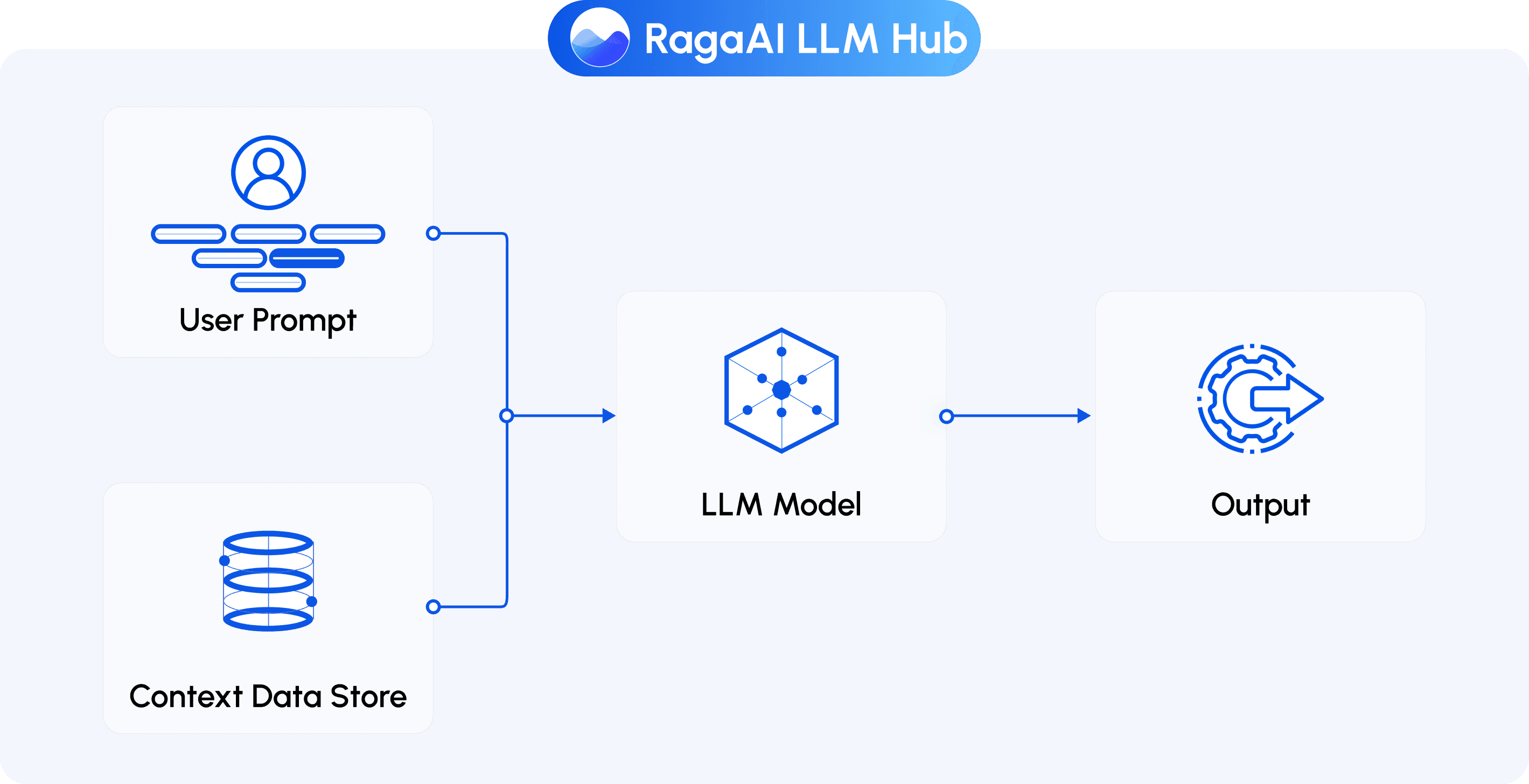
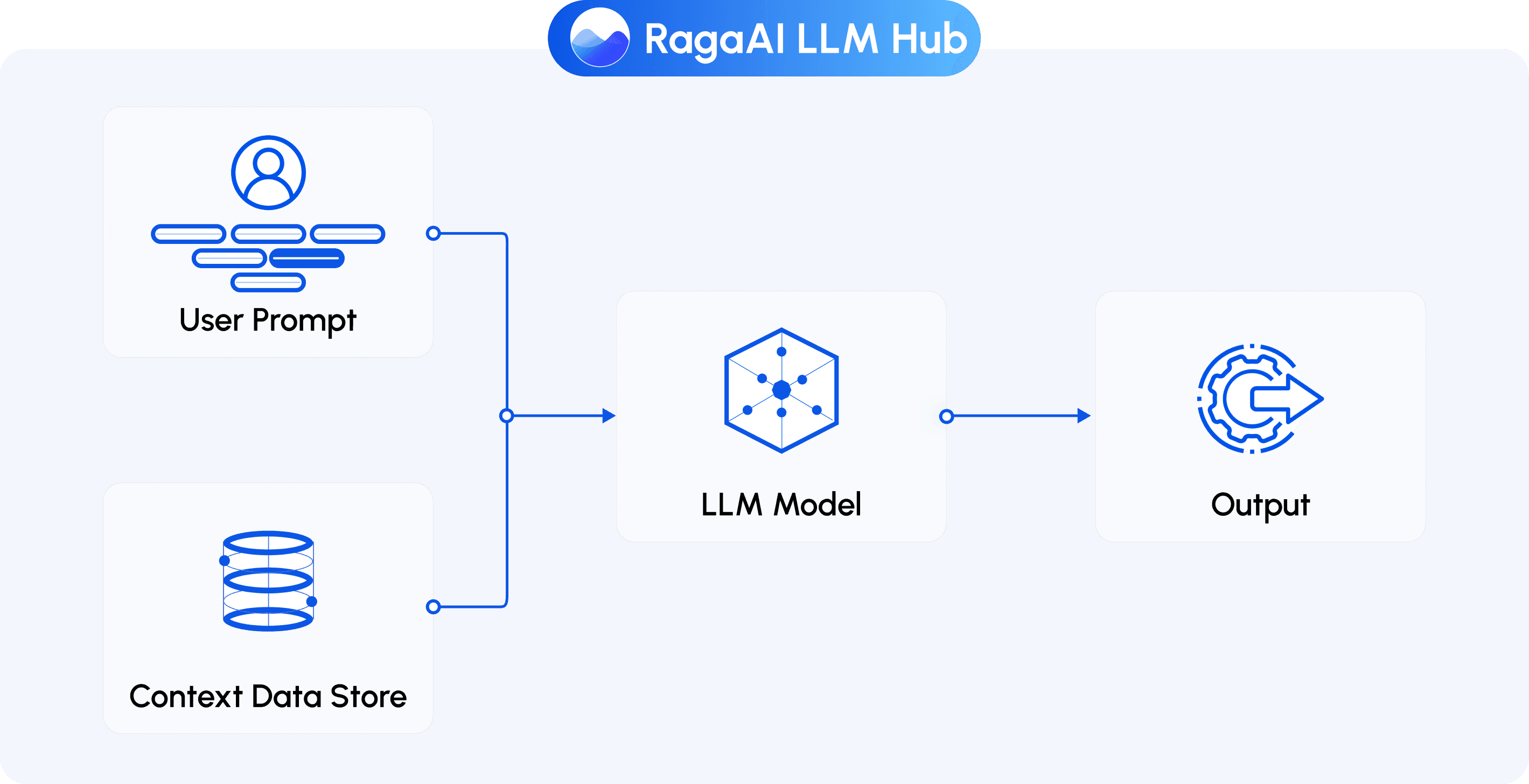
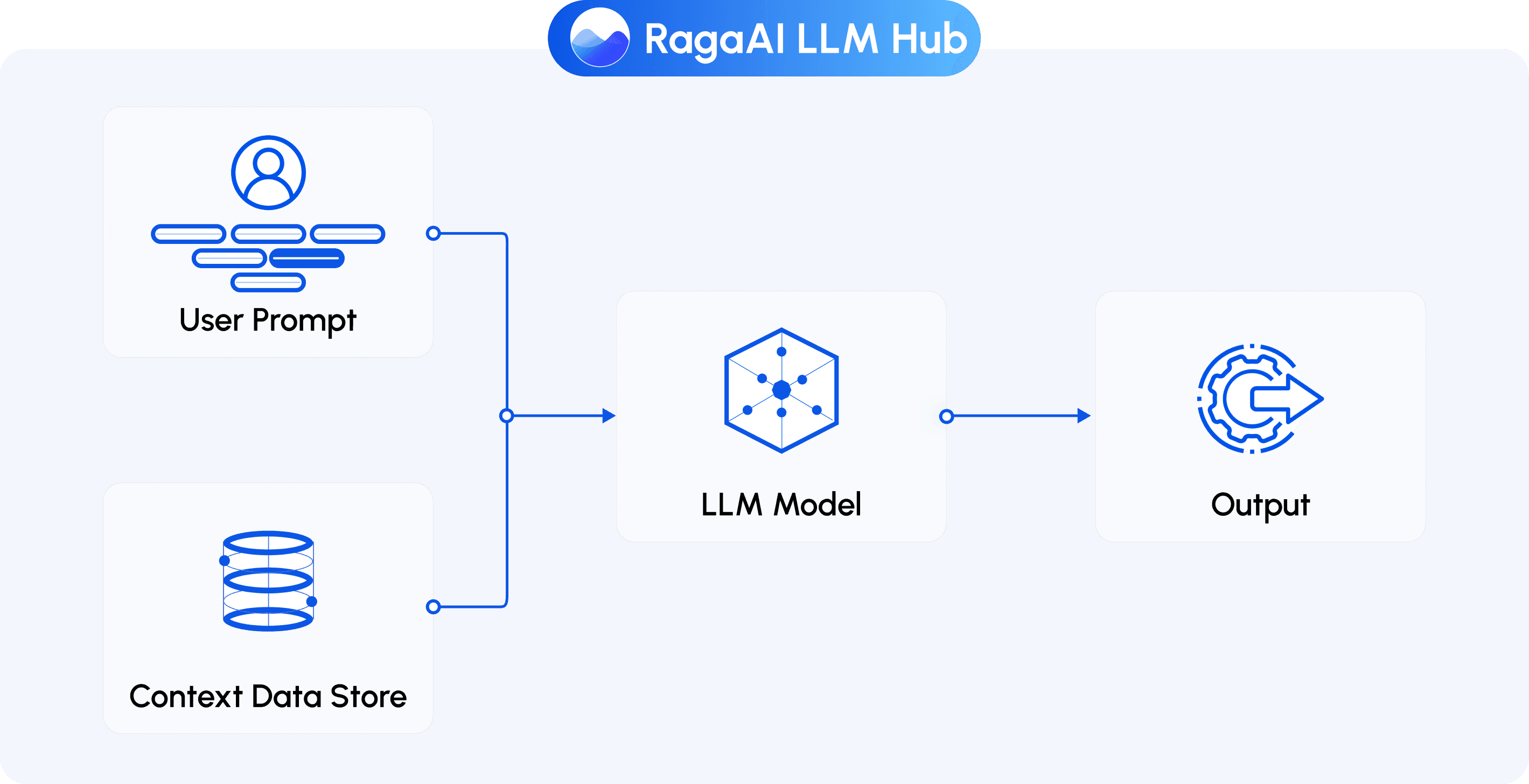
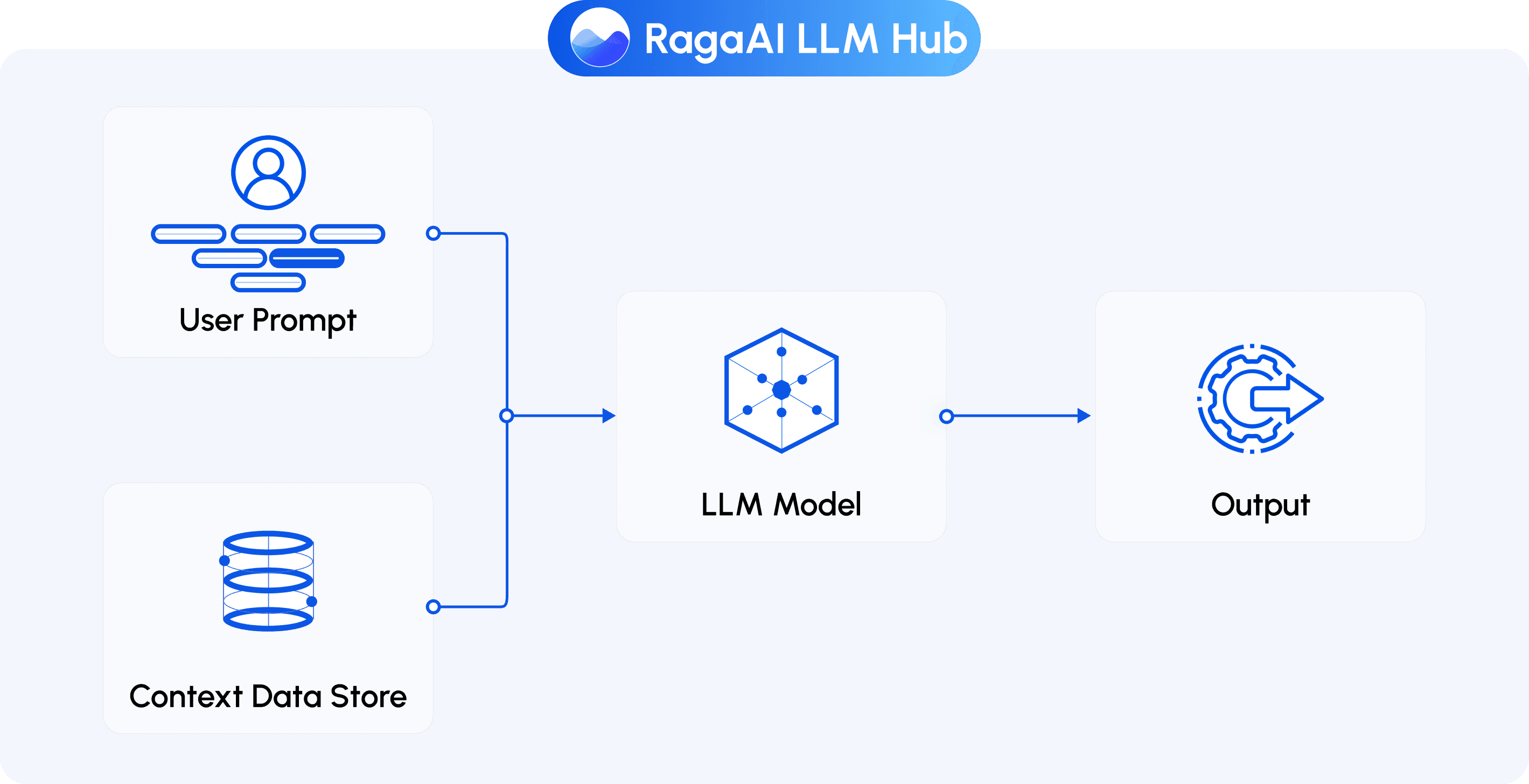
In the rapidly evolving field of large language models (LLMs), ensuring the safety, reliability, and quality of model outputs is paramount. Raga-LLM-Hub emerges as a cutting-edge Python library designed to tackle these challenges head-on. This guide delves into the intricate world of LLM applications, focusing on evaluating their performance and integrating necessary guardrails with the help of Raga-LLM-Hub.
Understanding the RAG Architecture
Retrieval Augmented Generation (RAG) is making AI much smarter by using information from big databases to help language models like OpenAI's GPT give better and more accurate answers. This is really helpful because usually, these AI models are good at making up stories but sometimes struggle with getting the facts right. RAG fixes this by mixing the AI's ability to create stories with real, accurate information from databases. This is making a big difference in many areas where we use AI to talk to people, answer questions, create content, and translate languages. Now, thanks to RAG, these AI systems can offer answers that are not just smooth and natural but also true and based on real facts.
The RAG framework operates through a series of steps designed to enhance the relevancy and accuracy of information generated by language models:
Prompt: The process begins with a user's prompt, which outlines the expected response.
Contextual Search: An external mechanism then augments the original prompt with relevant information sourced from databases, documents, or APIs, enriching the query with factual data.
Prompt Augmentation: This retrieved information is integrated into the original prompt, providing a richer context for the language model to operate within.
Inference: Armed with the augmented prompt, the language model processes both the original query and the added context to generate responses that are more accurate and contextually relevant.
Response: Finally, the model delivers a response that incorporates the newly integrated, factual information, ensuring that the output is both reliable and informative.
By following these steps, RAG overcomes the traditional limitations of language models, such as reliance on outdated information and the inability to verify the accuracy of generated content. This innovative framework significantly improves the quality of NLP applications, offering a pathway to more reliable, accurate, and informative AI-generated content.
Need of Evaluation and Guardrails
The RAG architecture combines retrieval mechanisms with generative models to leverage external knowledge, enhancing response quality. This necessitates robust evaluation to ensure the relevance and accuracy of incorporated external data, and the implementation of guardrails to manage risks associated with misinformation, biases, and inappropriate content from these vast, uncontrolled external sources. Evaluation processes are critical for verifying the effective integration of this data, optimizing performance across tasks. Guardrails, meanwhile, serve to filter and mitigate potential harms, ensuring the model's outputs remain trustworthy and ethically compliant. These measures are essential for maintaining the integrity and reliability of RAG models, addressing both technical and ethical challenges in their deployment.
Raga-LLM-Hub :- Framework for Evaluation and Guardrails
Enter Raga-LLM-Hub, a Python library designed to bridge this gap, providing tools for both evaluation and the integration of safety guardrails. Let’s dive deeper into the functionalities offered by Raga-LLM-Hub, exploring how it revolutionises the handling of LLM applications.

Raga-LLM-Hub stands out by offering a multifaceted suite designed for rigorous evaluation and robust guardrails. Here’s an in-depth look at how Raga-LLM-Hub accomplishes this:
Sophisticated Evaluation Metrics:
Hallucination Detection: This metric is crucial for identifying instances where the LLM fabricates information that lacks factual basis or logical coherence. By quantifying hallucination, developers can fine-tune their models to prioritize accuracy and reliability in generated content.
Chunk Impact Analysis: Understanding the influence of different input segments on the output is vital for model tuning. Chunk Impact Analysis provides granular insights into how each part of the input contributes to the final score, facilitating targeted improvements in model sensitivity and response quality.
Contextual Relevance: Maintaining context is a cornerstone of effective communication. This evaluation metric ensures that the model's outputs remain pertinent to the given context, enhancing the overall coherence and applicability of responses.
Summarization Quality: For applications requiring concise summarization, this metric assesses the summaries for clarity, completeness, and conciseness, ensuring that the essence of the original content is captured accurately and efficiently.
Beyond quantitative metrics, explainability sheds light on the "why" behind a model's outputs, offering reasons and sources for evaluation results. This transparency is crucial for trust and understanding in LLM applications.
Comprehensive Guardrails:
Preventing PII Leakage: The safeguarding of personally identifiable information cannot be overstated. Raga-LLM-Hub's PII leakage checks are essential for complying with privacy regulations and maintaining user trust.
Competitor Content Management: In competitive landscapes, inadvertently promoting or favouring competitors’ content could be detrimental. This guardrail ensures that outputs are neutral and unbiased.
Security and Sensitivity Checks: By scanning for vulnerabilities and sensitive information, Raga-LLM-Hub protects against security threats and ensures that outputs align with ethical standards and societal norms.
Vulnerability Scanner for Adversarial Attacks: The internet is rife with adversarial inputs aimed at misleading AI systems. The vulnerability scanner is a proactive measure to identify and mitigate such threats, enhancing the model's resilience.
Easy to install and run
# Example Test
#pip install raga-llm-hub
from raga_llm_hub import RagaLLMEval
evaluator = RagaLLMEval(api_keys={"OPENAI_API_KEY": "Your Key"})
evaluator.add_test(
test_names=["faithfulness_test"],
data={"prompt": prompt,
"response": pos_response,
"context": context_string,
},
arguments={"model": "gpt-4", "threshold": 0.5},
).run()
evaluator.print_results()
Learn More about 100s of Tests and Guardrails
For those keen on exploring the full capabilities of Raga-LLM-Hub and its enterprise solutions, a visit to docs.raga.ai offers a gateway to a wealth of resources tailored for enhancing LLM applications with unparalleled precision and safety.
In the rapidly evolving field of large language models (LLMs), ensuring the safety, reliability, and quality of model outputs is paramount. Raga-LLM-Hub emerges as a cutting-edge Python library designed to tackle these challenges head-on. This guide delves into the intricate world of LLM applications, focusing on evaluating their performance and integrating necessary guardrails with the help of Raga-LLM-Hub.
Understanding the RAG Architecture
Retrieval Augmented Generation (RAG) is making AI much smarter by using information from big databases to help language models like OpenAI's GPT give better and more accurate answers. This is really helpful because usually, these AI models are good at making up stories but sometimes struggle with getting the facts right. RAG fixes this by mixing the AI's ability to create stories with real, accurate information from databases. This is making a big difference in many areas where we use AI to talk to people, answer questions, create content, and translate languages. Now, thanks to RAG, these AI systems can offer answers that are not just smooth and natural but also true and based on real facts.
The RAG framework operates through a series of steps designed to enhance the relevancy and accuracy of information generated by language models:
Prompt: The process begins with a user's prompt, which outlines the expected response.
Contextual Search: An external mechanism then augments the original prompt with relevant information sourced from databases, documents, or APIs, enriching the query with factual data.
Prompt Augmentation: This retrieved information is integrated into the original prompt, providing a richer context for the language model to operate within.
Inference: Armed with the augmented prompt, the language model processes both the original query and the added context to generate responses that are more accurate and contextually relevant.
Response: Finally, the model delivers a response that incorporates the newly integrated, factual information, ensuring that the output is both reliable and informative.
By following these steps, RAG overcomes the traditional limitations of language models, such as reliance on outdated information and the inability to verify the accuracy of generated content. This innovative framework significantly improves the quality of NLP applications, offering a pathway to more reliable, accurate, and informative AI-generated content.
Need of Evaluation and Guardrails
The RAG architecture combines retrieval mechanisms with generative models to leverage external knowledge, enhancing response quality. This necessitates robust evaluation to ensure the relevance and accuracy of incorporated external data, and the implementation of guardrails to manage risks associated with misinformation, biases, and inappropriate content from these vast, uncontrolled external sources. Evaluation processes are critical for verifying the effective integration of this data, optimizing performance across tasks. Guardrails, meanwhile, serve to filter and mitigate potential harms, ensuring the model's outputs remain trustworthy and ethically compliant. These measures are essential for maintaining the integrity and reliability of RAG models, addressing both technical and ethical challenges in their deployment.
Raga-LLM-Hub :- Framework for Evaluation and Guardrails
Enter Raga-LLM-Hub, a Python library designed to bridge this gap, providing tools for both evaluation and the integration of safety guardrails. Let’s dive deeper into the functionalities offered by Raga-LLM-Hub, exploring how it revolutionises the handling of LLM applications.

Raga-LLM-Hub stands out by offering a multifaceted suite designed for rigorous evaluation and robust guardrails. Here’s an in-depth look at how Raga-LLM-Hub accomplishes this:
Sophisticated Evaluation Metrics:
Hallucination Detection: This metric is crucial for identifying instances where the LLM fabricates information that lacks factual basis or logical coherence. By quantifying hallucination, developers can fine-tune their models to prioritize accuracy and reliability in generated content.
Chunk Impact Analysis: Understanding the influence of different input segments on the output is vital for model tuning. Chunk Impact Analysis provides granular insights into how each part of the input contributes to the final score, facilitating targeted improvements in model sensitivity and response quality.
Contextual Relevance: Maintaining context is a cornerstone of effective communication. This evaluation metric ensures that the model's outputs remain pertinent to the given context, enhancing the overall coherence and applicability of responses.
Summarization Quality: For applications requiring concise summarization, this metric assesses the summaries for clarity, completeness, and conciseness, ensuring that the essence of the original content is captured accurately and efficiently.
Beyond quantitative metrics, explainability sheds light on the "why" behind a model's outputs, offering reasons and sources for evaluation results. This transparency is crucial for trust and understanding in LLM applications.
Comprehensive Guardrails:
Preventing PII Leakage: The safeguarding of personally identifiable information cannot be overstated. Raga-LLM-Hub's PII leakage checks are essential for complying with privacy regulations and maintaining user trust.
Competitor Content Management: In competitive landscapes, inadvertently promoting or favouring competitors’ content could be detrimental. This guardrail ensures that outputs are neutral and unbiased.
Security and Sensitivity Checks: By scanning for vulnerabilities and sensitive information, Raga-LLM-Hub protects against security threats and ensures that outputs align with ethical standards and societal norms.
Vulnerability Scanner for Adversarial Attacks: The internet is rife with adversarial inputs aimed at misleading AI systems. The vulnerability scanner is a proactive measure to identify and mitigate such threats, enhancing the model's resilience.
Easy to install and run
# Example Test
#pip install raga-llm-hub
from raga_llm_hub import RagaLLMEval
evaluator = RagaLLMEval(api_keys={"OPENAI_API_KEY": "Your Key"})
evaluator.add_test(
test_names=["faithfulness_test"],
data={"prompt": prompt,
"response": pos_response,
"context": context_string,
},
arguments={"model": "gpt-4", "threshold": 0.5},
).run()
evaluator.print_results()
Learn More about 100s of Tests and Guardrails
For those keen on exploring the full capabilities of Raga-LLM-Hub and its enterprise solutions, a visit to docs.raga.ai offers a gateway to a wealth of resources tailored for enhancing LLM applications with unparalleled precision and safety.
In the rapidly evolving field of large language models (LLMs), ensuring the safety, reliability, and quality of model outputs is paramount. Raga-LLM-Hub emerges as a cutting-edge Python library designed to tackle these challenges head-on. This guide delves into the intricate world of LLM applications, focusing on evaluating their performance and integrating necessary guardrails with the help of Raga-LLM-Hub.
Understanding the RAG Architecture
Retrieval Augmented Generation (RAG) is making AI much smarter by using information from big databases to help language models like OpenAI's GPT give better and more accurate answers. This is really helpful because usually, these AI models are good at making up stories but sometimes struggle with getting the facts right. RAG fixes this by mixing the AI's ability to create stories with real, accurate information from databases. This is making a big difference in many areas where we use AI to talk to people, answer questions, create content, and translate languages. Now, thanks to RAG, these AI systems can offer answers that are not just smooth and natural but also true and based on real facts.
The RAG framework operates through a series of steps designed to enhance the relevancy and accuracy of information generated by language models:
Prompt: The process begins with a user's prompt, which outlines the expected response.
Contextual Search: An external mechanism then augments the original prompt with relevant information sourced from databases, documents, or APIs, enriching the query with factual data.
Prompt Augmentation: This retrieved information is integrated into the original prompt, providing a richer context for the language model to operate within.
Inference: Armed with the augmented prompt, the language model processes both the original query and the added context to generate responses that are more accurate and contextually relevant.
Response: Finally, the model delivers a response that incorporates the newly integrated, factual information, ensuring that the output is both reliable and informative.
By following these steps, RAG overcomes the traditional limitations of language models, such as reliance on outdated information and the inability to verify the accuracy of generated content. This innovative framework significantly improves the quality of NLP applications, offering a pathway to more reliable, accurate, and informative AI-generated content.
Need of Evaluation and Guardrails
The RAG architecture combines retrieval mechanisms with generative models to leverage external knowledge, enhancing response quality. This necessitates robust evaluation to ensure the relevance and accuracy of incorporated external data, and the implementation of guardrails to manage risks associated with misinformation, biases, and inappropriate content from these vast, uncontrolled external sources. Evaluation processes are critical for verifying the effective integration of this data, optimizing performance across tasks. Guardrails, meanwhile, serve to filter and mitigate potential harms, ensuring the model's outputs remain trustworthy and ethically compliant. These measures are essential for maintaining the integrity and reliability of RAG models, addressing both technical and ethical challenges in their deployment.
Raga-LLM-Hub :- Framework for Evaluation and Guardrails
Enter Raga-LLM-Hub, a Python library designed to bridge this gap, providing tools for both evaluation and the integration of safety guardrails. Let’s dive deeper into the functionalities offered by Raga-LLM-Hub, exploring how it revolutionises the handling of LLM applications.

Raga-LLM-Hub stands out by offering a multifaceted suite designed for rigorous evaluation and robust guardrails. Here’s an in-depth look at how Raga-LLM-Hub accomplishes this:
Sophisticated Evaluation Metrics:
Hallucination Detection: This metric is crucial for identifying instances where the LLM fabricates information that lacks factual basis or logical coherence. By quantifying hallucination, developers can fine-tune their models to prioritize accuracy and reliability in generated content.
Chunk Impact Analysis: Understanding the influence of different input segments on the output is vital for model tuning. Chunk Impact Analysis provides granular insights into how each part of the input contributes to the final score, facilitating targeted improvements in model sensitivity and response quality.
Contextual Relevance: Maintaining context is a cornerstone of effective communication. This evaluation metric ensures that the model's outputs remain pertinent to the given context, enhancing the overall coherence and applicability of responses.
Summarization Quality: For applications requiring concise summarization, this metric assesses the summaries for clarity, completeness, and conciseness, ensuring that the essence of the original content is captured accurately and efficiently.
Beyond quantitative metrics, explainability sheds light on the "why" behind a model's outputs, offering reasons and sources for evaluation results. This transparency is crucial for trust and understanding in LLM applications.
Comprehensive Guardrails:
Preventing PII Leakage: The safeguarding of personally identifiable information cannot be overstated. Raga-LLM-Hub's PII leakage checks are essential for complying with privacy regulations and maintaining user trust.
Competitor Content Management: In competitive landscapes, inadvertently promoting or favouring competitors’ content could be detrimental. This guardrail ensures that outputs are neutral and unbiased.
Security and Sensitivity Checks: By scanning for vulnerabilities and sensitive information, Raga-LLM-Hub protects against security threats and ensures that outputs align with ethical standards and societal norms.
Vulnerability Scanner for Adversarial Attacks: The internet is rife with adversarial inputs aimed at misleading AI systems. The vulnerability scanner is a proactive measure to identify and mitigate such threats, enhancing the model's resilience.
Easy to install and run
# Example Test
#pip install raga-llm-hub
from raga_llm_hub import RagaLLMEval
evaluator = RagaLLMEval(api_keys={"OPENAI_API_KEY": "Your Key"})
evaluator.add_test(
test_names=["faithfulness_test"],
data={"prompt": prompt,
"response": pos_response,
"context": context_string,
},
arguments={"model": "gpt-4", "threshold": 0.5},
).run()
evaluator.print_results()
Learn More about 100s of Tests and Guardrails
For those keen on exploring the full capabilities of Raga-LLM-Hub and its enterprise solutions, a visit to docs.raga.ai offers a gateway to a wealth of resources tailored for enhancing LLM applications with unparalleled precision and safety.
In the rapidly evolving field of large language models (LLMs), ensuring the safety, reliability, and quality of model outputs is paramount. Raga-LLM-Hub emerges as a cutting-edge Python library designed to tackle these challenges head-on. This guide delves into the intricate world of LLM applications, focusing on evaluating their performance and integrating necessary guardrails with the help of Raga-LLM-Hub.
Understanding the RAG Architecture
Retrieval Augmented Generation (RAG) is making AI much smarter by using information from big databases to help language models like OpenAI's GPT give better and more accurate answers. This is really helpful because usually, these AI models are good at making up stories but sometimes struggle with getting the facts right. RAG fixes this by mixing the AI's ability to create stories with real, accurate information from databases. This is making a big difference in many areas where we use AI to talk to people, answer questions, create content, and translate languages. Now, thanks to RAG, these AI systems can offer answers that are not just smooth and natural but also true and based on real facts.
The RAG framework operates through a series of steps designed to enhance the relevancy and accuracy of information generated by language models:
Prompt: The process begins with a user's prompt, which outlines the expected response.
Contextual Search: An external mechanism then augments the original prompt with relevant information sourced from databases, documents, or APIs, enriching the query with factual data.
Prompt Augmentation: This retrieved information is integrated into the original prompt, providing a richer context for the language model to operate within.
Inference: Armed with the augmented prompt, the language model processes both the original query and the added context to generate responses that are more accurate and contextually relevant.
Response: Finally, the model delivers a response that incorporates the newly integrated, factual information, ensuring that the output is both reliable and informative.
By following these steps, RAG overcomes the traditional limitations of language models, such as reliance on outdated information and the inability to verify the accuracy of generated content. This innovative framework significantly improves the quality of NLP applications, offering a pathway to more reliable, accurate, and informative AI-generated content.
Need of Evaluation and Guardrails
The RAG architecture combines retrieval mechanisms with generative models to leverage external knowledge, enhancing response quality. This necessitates robust evaluation to ensure the relevance and accuracy of incorporated external data, and the implementation of guardrails to manage risks associated with misinformation, biases, and inappropriate content from these vast, uncontrolled external sources. Evaluation processes are critical for verifying the effective integration of this data, optimizing performance across tasks. Guardrails, meanwhile, serve to filter and mitigate potential harms, ensuring the model's outputs remain trustworthy and ethically compliant. These measures are essential for maintaining the integrity and reliability of RAG models, addressing both technical and ethical challenges in their deployment.
Raga-LLM-Hub :- Framework for Evaluation and Guardrails
Enter Raga-LLM-Hub, a Python library designed to bridge this gap, providing tools for both evaluation and the integration of safety guardrails. Let’s dive deeper into the functionalities offered by Raga-LLM-Hub, exploring how it revolutionises the handling of LLM applications.

Raga-LLM-Hub stands out by offering a multifaceted suite designed for rigorous evaluation and robust guardrails. Here’s an in-depth look at how Raga-LLM-Hub accomplishes this:
Sophisticated Evaluation Metrics:
Hallucination Detection: This metric is crucial for identifying instances where the LLM fabricates information that lacks factual basis or logical coherence. By quantifying hallucination, developers can fine-tune their models to prioritize accuracy and reliability in generated content.
Chunk Impact Analysis: Understanding the influence of different input segments on the output is vital for model tuning. Chunk Impact Analysis provides granular insights into how each part of the input contributes to the final score, facilitating targeted improvements in model sensitivity and response quality.
Contextual Relevance: Maintaining context is a cornerstone of effective communication. This evaluation metric ensures that the model's outputs remain pertinent to the given context, enhancing the overall coherence and applicability of responses.
Summarization Quality: For applications requiring concise summarization, this metric assesses the summaries for clarity, completeness, and conciseness, ensuring that the essence of the original content is captured accurately and efficiently.
Beyond quantitative metrics, explainability sheds light on the "why" behind a model's outputs, offering reasons and sources for evaluation results. This transparency is crucial for trust and understanding in LLM applications.
Comprehensive Guardrails:
Preventing PII Leakage: The safeguarding of personally identifiable information cannot be overstated. Raga-LLM-Hub's PII leakage checks are essential for complying with privacy regulations and maintaining user trust.
Competitor Content Management: In competitive landscapes, inadvertently promoting or favouring competitors’ content could be detrimental. This guardrail ensures that outputs are neutral and unbiased.
Security and Sensitivity Checks: By scanning for vulnerabilities and sensitive information, Raga-LLM-Hub protects against security threats and ensures that outputs align with ethical standards and societal norms.
Vulnerability Scanner for Adversarial Attacks: The internet is rife with adversarial inputs aimed at misleading AI systems. The vulnerability scanner is a proactive measure to identify and mitigate such threats, enhancing the model's resilience.
Easy to install and run
# Example Test
#pip install raga-llm-hub
from raga_llm_hub import RagaLLMEval
evaluator = RagaLLMEval(api_keys={"OPENAI_API_KEY": "Your Key"})
evaluator.add_test(
test_names=["faithfulness_test"],
data={"prompt": prompt,
"response": pos_response,
"context": context_string,
},
arguments={"model": "gpt-4", "threshold": 0.5},
).run()
evaluator.print_results()
Learn More about 100s of Tests and Guardrails
For those keen on exploring the full capabilities of Raga-LLM-Hub and its enterprise solutions, a visit to docs.raga.ai offers a gateway to a wealth of resources tailored for enhancing LLM applications with unparalleled precision and safety.
In the rapidly evolving field of large language models (LLMs), ensuring the safety, reliability, and quality of model outputs is paramount. Raga-LLM-Hub emerges as a cutting-edge Python library designed to tackle these challenges head-on. This guide delves into the intricate world of LLM applications, focusing on evaluating their performance and integrating necessary guardrails with the help of Raga-LLM-Hub.
Understanding the RAG Architecture
Retrieval Augmented Generation (RAG) is making AI much smarter by using information from big databases to help language models like OpenAI's GPT give better and more accurate answers. This is really helpful because usually, these AI models are good at making up stories but sometimes struggle with getting the facts right. RAG fixes this by mixing the AI's ability to create stories with real, accurate information from databases. This is making a big difference in many areas where we use AI to talk to people, answer questions, create content, and translate languages. Now, thanks to RAG, these AI systems can offer answers that are not just smooth and natural but also true and based on real facts.
The RAG framework operates through a series of steps designed to enhance the relevancy and accuracy of information generated by language models:
Prompt: The process begins with a user's prompt, which outlines the expected response.
Contextual Search: An external mechanism then augments the original prompt with relevant information sourced from databases, documents, or APIs, enriching the query with factual data.
Prompt Augmentation: This retrieved information is integrated into the original prompt, providing a richer context for the language model to operate within.
Inference: Armed with the augmented prompt, the language model processes both the original query and the added context to generate responses that are more accurate and contextually relevant.
Response: Finally, the model delivers a response that incorporates the newly integrated, factual information, ensuring that the output is both reliable and informative.
By following these steps, RAG overcomes the traditional limitations of language models, such as reliance on outdated information and the inability to verify the accuracy of generated content. This innovative framework significantly improves the quality of NLP applications, offering a pathway to more reliable, accurate, and informative AI-generated content.
Need of Evaluation and Guardrails
The RAG architecture combines retrieval mechanisms with generative models to leverage external knowledge, enhancing response quality. This necessitates robust evaluation to ensure the relevance and accuracy of incorporated external data, and the implementation of guardrails to manage risks associated with misinformation, biases, and inappropriate content from these vast, uncontrolled external sources. Evaluation processes are critical for verifying the effective integration of this data, optimizing performance across tasks. Guardrails, meanwhile, serve to filter and mitigate potential harms, ensuring the model's outputs remain trustworthy and ethically compliant. These measures are essential for maintaining the integrity and reliability of RAG models, addressing both technical and ethical challenges in their deployment.
Raga-LLM-Hub :- Framework for Evaluation and Guardrails
Enter Raga-LLM-Hub, a Python library designed to bridge this gap, providing tools for both evaluation and the integration of safety guardrails. Let’s dive deeper into the functionalities offered by Raga-LLM-Hub, exploring how it revolutionises the handling of LLM applications.

Raga-LLM-Hub stands out by offering a multifaceted suite designed for rigorous evaluation and robust guardrails. Here’s an in-depth look at how Raga-LLM-Hub accomplishes this:
Sophisticated Evaluation Metrics:
Hallucination Detection: This metric is crucial for identifying instances where the LLM fabricates information that lacks factual basis or logical coherence. By quantifying hallucination, developers can fine-tune their models to prioritize accuracy and reliability in generated content.
Chunk Impact Analysis: Understanding the influence of different input segments on the output is vital for model tuning. Chunk Impact Analysis provides granular insights into how each part of the input contributes to the final score, facilitating targeted improvements in model sensitivity and response quality.
Contextual Relevance: Maintaining context is a cornerstone of effective communication. This evaluation metric ensures that the model's outputs remain pertinent to the given context, enhancing the overall coherence and applicability of responses.
Summarization Quality: For applications requiring concise summarization, this metric assesses the summaries for clarity, completeness, and conciseness, ensuring that the essence of the original content is captured accurately and efficiently.
Beyond quantitative metrics, explainability sheds light on the "why" behind a model's outputs, offering reasons and sources for evaluation results. This transparency is crucial for trust and understanding in LLM applications.
Comprehensive Guardrails:
Preventing PII Leakage: The safeguarding of personally identifiable information cannot be overstated. Raga-LLM-Hub's PII leakage checks are essential for complying with privacy regulations and maintaining user trust.
Competitor Content Management: In competitive landscapes, inadvertently promoting or favouring competitors’ content could be detrimental. This guardrail ensures that outputs are neutral and unbiased.
Security and Sensitivity Checks: By scanning for vulnerabilities and sensitive information, Raga-LLM-Hub protects against security threats and ensures that outputs align with ethical standards and societal norms.
Vulnerability Scanner for Adversarial Attacks: The internet is rife with adversarial inputs aimed at misleading AI systems. The vulnerability scanner is a proactive measure to identify and mitigate such threats, enhancing the model's resilience.
Easy to install and run
# Example Test
#pip install raga-llm-hub
from raga_llm_hub import RagaLLMEval
evaluator = RagaLLMEval(api_keys={"OPENAI_API_KEY": "Your Key"})
evaluator.add_test(
test_names=["faithfulness_test"],
data={"prompt": prompt,
"response": pos_response,
"context": context_string,
},
arguments={"model": "gpt-4", "threshold": 0.5},
).run()
evaluator.print_results()
Learn More about 100s of Tests and Guardrails
For those keen on exploring the full capabilities of Raga-LLM-Hub and its enterprise solutions, a visit to docs.raga.ai offers a gateway to a wealth of resources tailored for enhancing LLM applications with unparalleled precision and safety.
Subscribe to our newsletter to never miss an update
Other articles


Agents Talk, Models Think: A2A + MCP for Enterprise Agentic AI

Riya Parikh, Nitai Agarwal
Apr 27, 2025
Read the article
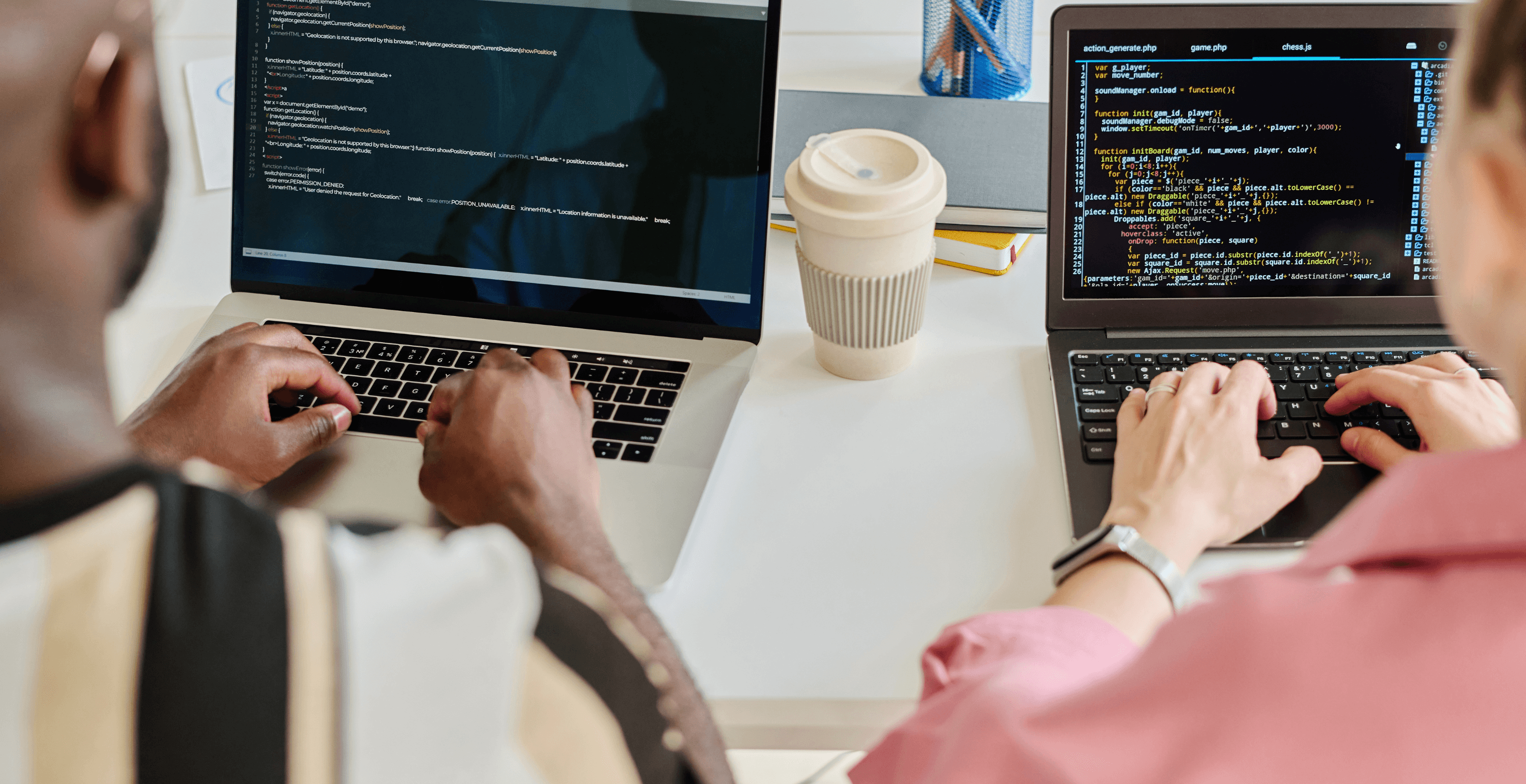
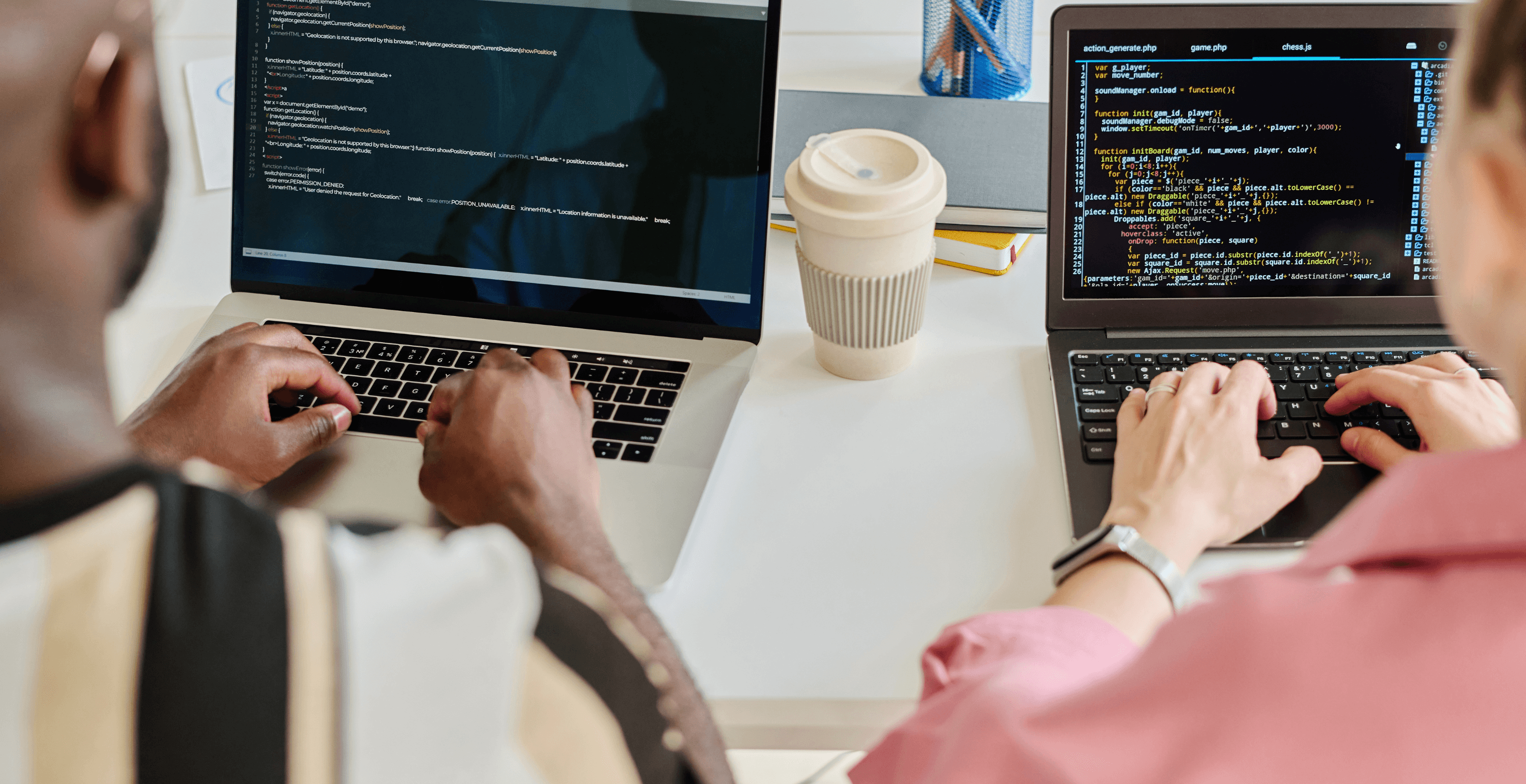
Understanding NeMo Guardrails: A Toolkit for LLM Security

Rehan Asif
Dec 24, 2024
Read the article


Understanding Differences in Large vs Small Language Models (LLM vs SLM)

Rehan Asif
Dec 21, 2024
Read the article