Understanding The Basics Of LLM Fine-tuning With Custom Data
Understanding The Basics Of LLM Fine-tuning With Custom Data
Understanding The Basics Of LLM Fine-tuning With Custom Data
Rehan Asif
Apr 13, 2024




The advent of Large Language Models (LLMs) like GPT (Generative Pre-trained Transformer), Mistral, Llama, etc. has revolutionized the field of natural language processing (NLP). By understanding and generating human-like text, these models have become indispensable for various applications, from chatbots to content generation.
Understanding Pre-trained Large Language Models
LLMs utilize the Transformers architecture to comprehend and generate text, and they are trained on extensive datasets. Models like GPT have simplified the integration of NLP features into various applications.
Popular Datasets for Fine-tuning
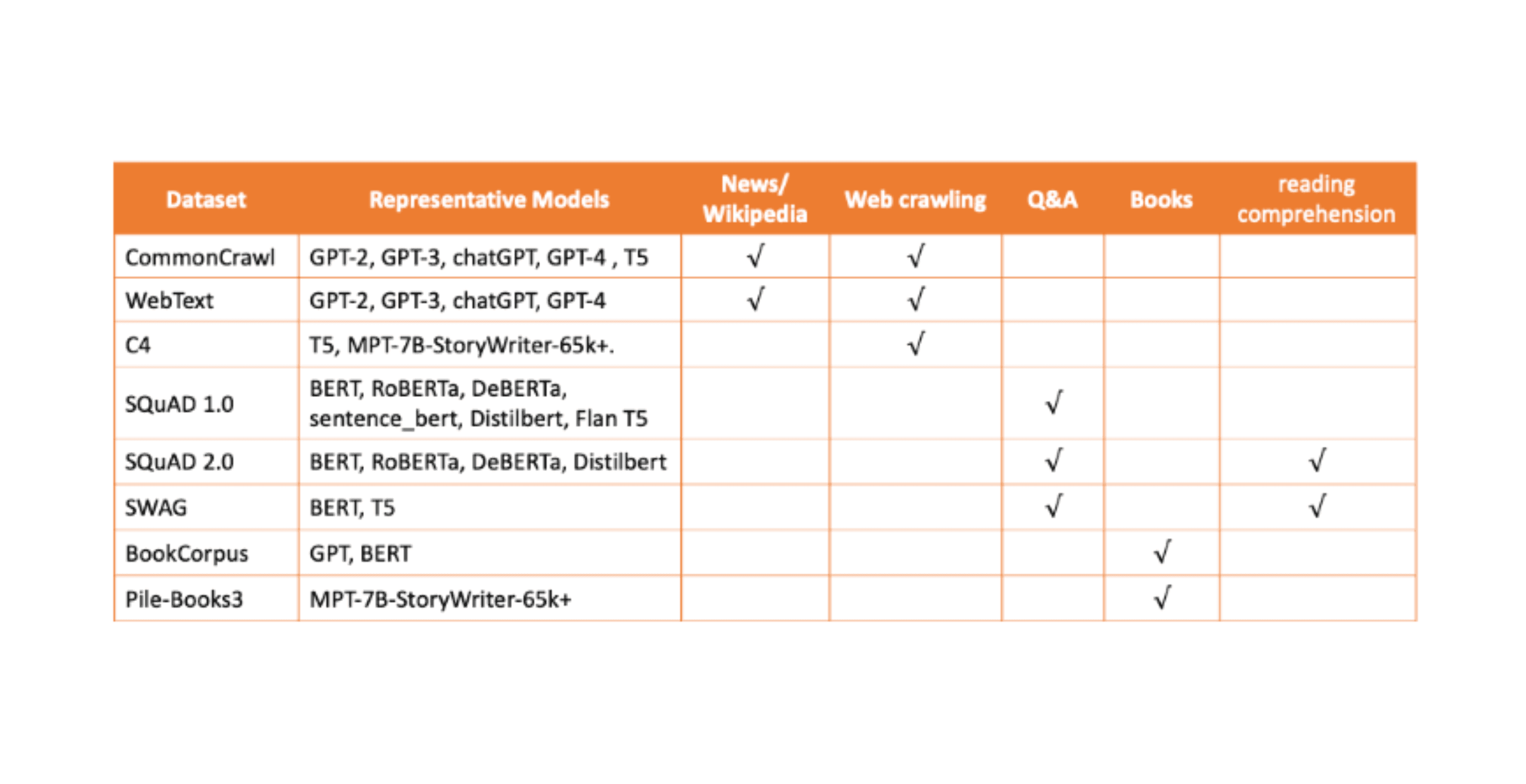
Source: Medium
GLUE: A collection of nine NLP tasks designed to evaluate model performance across various tasks.
SQUAD: A reading comprehension dataset consisting of questions posed on Wikipedia articles.
Common Crawl: A massive collection of web-crawled data that is highly diverse and useful for general domain fine-tuning.
More datasets for fine-tuning can be found here
GPT and similar models are at the forefront of NLP, offering simple text generation capabilities to complex dialogue systems. Pre-trained models are readily available through APIs, allowing developers to integrate advanced NLP features into their applications with minimal setup.
Loading A Pre-Trained Model
# Initialize tokenizer and model
From transformers import GPT2Model, GPT2Tokenizer
tokenizer = GPT2Tokenizer.from_pretrained('gpt2')
model = GPT2Model.from_pretrained('gpt2')
# Tokenize input text
inputs = tokenizer("Hello, world!", return_tensors="pt")
# Generate model output
outputs = model(**inputs)
Critical Steps in the Fine-tuning Process
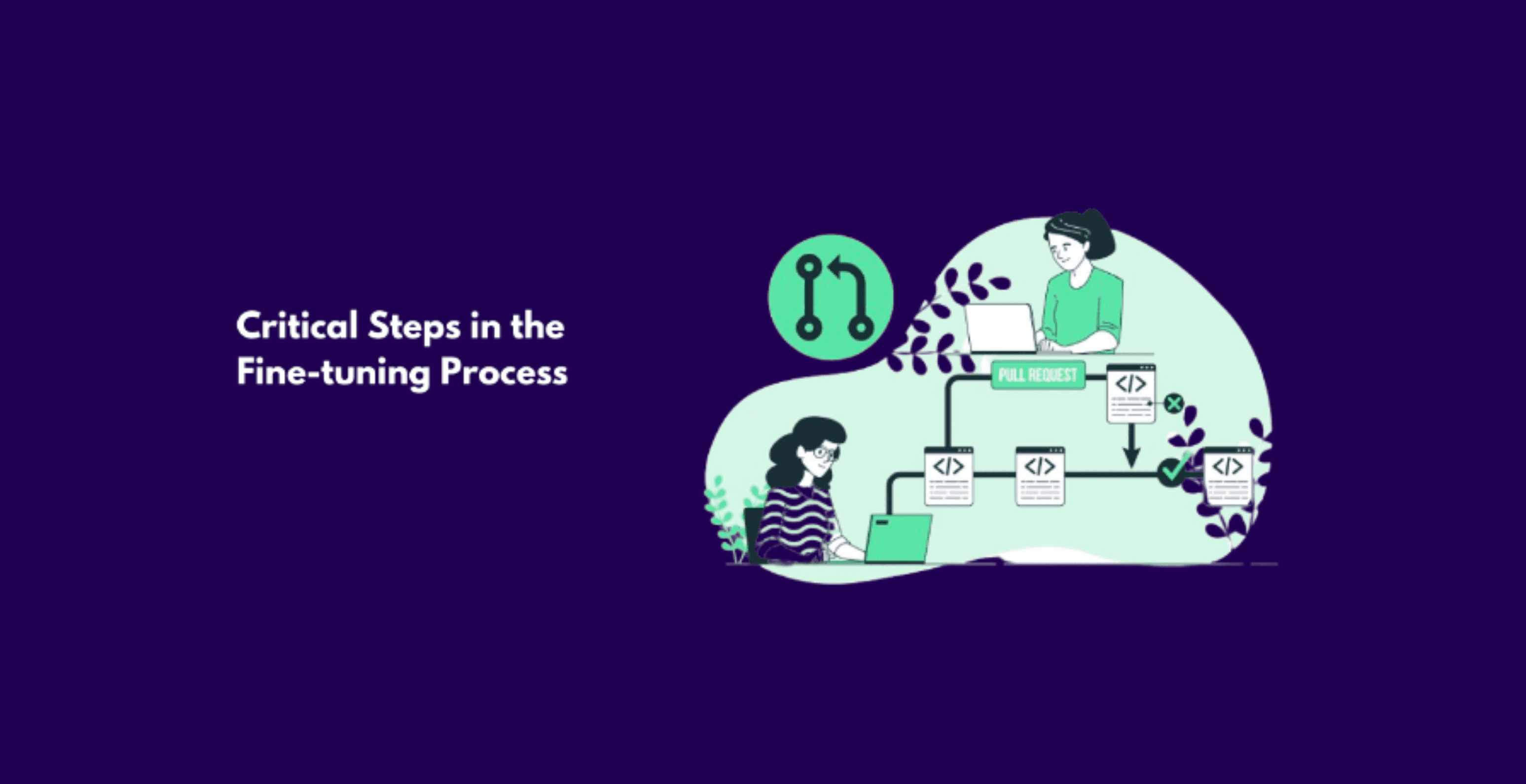
Selecting an Appropriate Model
The first step in fine-tuning involves selecting a suitable pre-trained model. This decision is pivotal because the chosen model's architecture, size, and initial training data significantly influence its adaptability to your specific task.
Architecture Consideration: Select a model architecture that is conducive to your task. For instance, GPT-3 is preferred for generative tasks, while BERT is better for classification tasks.
Size and Capacity: Larger models can achieve higher accuracy but require more computational resources for fine-tuning and inference.
Initial Pre-training Data: Consider what data the model was initially trained on; models pre-trained on domain-similar data might require less fine-tuning.
Preparing the Dataset
Proper dataset preparation is essential. It involves collecting, cleaning, and formatting your data so that it's suitable for training the model.
Dataset Collection: Compile a dataset that closely represents the task you're fine-tuning the model.
Cleaning and Preprocessing: Remove noise from the data and perform necessary preprocessing steps, including tokenization, truncation, and padding.
Splitting Data: Divide your dataset into training, validation, and test sets to ensure your model can be trained and evaluated effectively.
Executing the Fine-tuning Procedure
After preparing your dataset and selecting the appropriate model, you can proceed to fine-tune your model. This step adjusts the model's weights based on your custom dataset, improving its performance for your specific task.
Training Configuration: Define your training parameters, including learning rate, number of epochs, and batch size. These parameters significantly influence the fine-tuning outcome.
Model Training: Utilize the Trainer API or a custom training loop to fine-tune your model on the prepared dataset.
Evaluation and Adjustment: Regularly evaluate the model's performance on a validation set to adjust hyperparameters and prevent overfitting.
from transformers import GPT2LMHeadModel, GPT2Tokenizer, Trainer, TrainingArguments
model = GPT2LMHeadModel.from_pretrained('gpt2')
tokenizer = GPT2Tokenizer.from_pretrained('gpt2')# Prepare dataset
train_dataset = # Your custom dataset loading and processing logic here
# Training arguments
training_args = TrainingArguments(
output_dir='./results', # output directory
num_train_epochs=3, # total number of training epochs
per_device_train_batch_size=4, # batch size per device during training
warmup_steps=500, # number of warmup steps for learning rate scheduler
weight_decay=0.01, # strength of weight decay
)
# Initialize Trainer
trainer = Trainer(
model=model,
args=training_args,
train_dataset=train_dataset,
)
# Start training
trainer.train()
Best Practices for Successful Fine-tuning Hyperparameter Tuning: Experiment with different hyperparameters to find the best combination for your specific task. Tools like Hugging Face's Trainer offer hyperparameter search capabilities.
Monitoring and Evaluation: Use the validation set to monitor the model's performance during training. Adjust training parameters as necessary to improve results and avoid overfitting.
Incremental Training: Start with a small amount of data and gradually add more data or increase model complexity as needed. This approach can save computational resources and help identify the best training strategy.
Fine-tuning Methods and Approaches
Supervised Fine-tuning (SFT): This approach uses task-specific labeled data to guide the model's learning process.
Instruction Fine-tuning and Full Fine-tuning: These methods involve adjusting all model weights to align with the new task's requirements.
Parameter-efficient Fine-tuning (PEFT) and Quantized LoRA: These techniques aim for efficient adaptation, minimizing the resources required for fine-tuning.
Packages for Fine-tuning
Transformers by Hugging Face: A comprehensive library offering pre-trained models and utilities for NLP tasks.
Datasets by Hugging Face: A tool for loading and preparing datasets, making it easier to manage data for fine-tuning.
from datasets import load_dataset
# Load your dataset
dataset = load_dataset('path_to_your_dataset', split='train')
# Preprocess the dataset
def preprocess_function(examples):
return tokenizer(examples['text'], truncation=True, padding='max_length', max_length=512)
tokenized_dataset = dataset.map(preprocess_function, batched=True)
Selecting Datasets for Chatbot Fine-tuning
Fine-tuning a chatbot involves training the pre-existing LLM on a dataset that reflects the specific conversational context and user intents it will encounter. Here are some criteria and sources for selecting such datasets:
Task-Specific Data: Collect dialogues or conversational datasets closely aligned with the chatbot’s intended function.
Domain-Specific Interactions: For chatbots serving specific industries (e.g., healthcare, finance), incorporating industry-specific dialogue can drastically improve relevance and accuracy.
Fine-tuning Methods for Chatbots
Fine-tuning for chatbots focuses on adjusting the LLM to better understand and respond to user queries and statements in a conversational context. This involves several key methods:
Supervised Learning: Training the model on a labeled conversational dataset where inputs (user queries) and correct outputs (bot responses) are clearly defined.
Reinforcement Learning from Human Feedback (RLHF): A method where the model is fine-tuned based on feedback from human interactions, refining its responses to be more aligned with user expectations.
Data Requirement and Preparation for Fine-tuning
Need for High-quality Data
The foundation of effective fine-tuning lies in the dataset used. High-quality data that is clean, relevant, and representative of the task is crucial. This ensures the model learns the correct patterns and nuances of the specific domain or application. The following aspects are essential when considering data quality:
Relevance: The data should closely align with the task or domain the model is fine-tuning for.
Diversity: A varied dataset helps generalize the model's understanding and reduces biases.
Label Accuracy: For supervised learning tasks, the accuracy of labels directly impacts the model's performance.
Strategies for Addressing Challenges
Data Augmentation: Increase the size and diversity of your dataset through techniques such as paraphrasing, back-translation, or leveraging generative models.
Efficient Computing Resources: Utilise cloud computing services that offer scalable GPU resources to manage costs effectively. Additionally, it explores techniques like quantization and pruning to reduce model size and computational requirements.
Automated Hyperparameter Tuning: Employ tools and frameworks that support automated hyperparameter search to streamline the optimization process.
Incremental Learning: Regularly update your model with new data in smaller batches to keep it current and reduce the need for extensive retraining.
Conclusion
Fine-tuning LLMs with custom datasets is a robust process that significantly enhances the model's relevance, accuracy, and efficiency for specific tasks.
Despite its challenges, the benefits of fine-tuning—such as increased specificity and improved user interactions—make it an essential practice for anyone looking to leverage the full capabilities of Large Language Models.
As the field of NLP continues to evolve, fine-tuning remains crucial in adapting general-purpose models to meet the ever-changing needs of applications and users.
Ready to elevate your AI applications to new heights of efficiency and reliability? Join the forefront of AI innovation with RagaAI.
Whether you're working on LLMs, Computer Vision, or Tabular Data, RagaAI is designed to streamline your AI development, ensuring your projects are production-ready 3X faster. With RagaAI, you'll experience a remarkable 90% reduction in AI failures and a 50% reduction in operational costs. Try Raga today.
FAQ:
How do I choose the suitable pre-trained model for fine-tuning?
Consider the model's architecture, the dataset's size, and your application's specific requirements to select the most suitable pre-trained model.
What are the main challenges of fine-tuning LLMs?
The main challenges include acquiring high-quality, representative datasets, managing the costs of computational resources, and the need for continuous adjustments and updates to the model.
The advent of Large Language Models (LLMs) like GPT (Generative Pre-trained Transformer), Mistral, Llama, etc. has revolutionized the field of natural language processing (NLP). By understanding and generating human-like text, these models have become indispensable for various applications, from chatbots to content generation.
Understanding Pre-trained Large Language Models
LLMs utilize the Transformers architecture to comprehend and generate text, and they are trained on extensive datasets. Models like GPT have simplified the integration of NLP features into various applications.
Popular Datasets for Fine-tuning
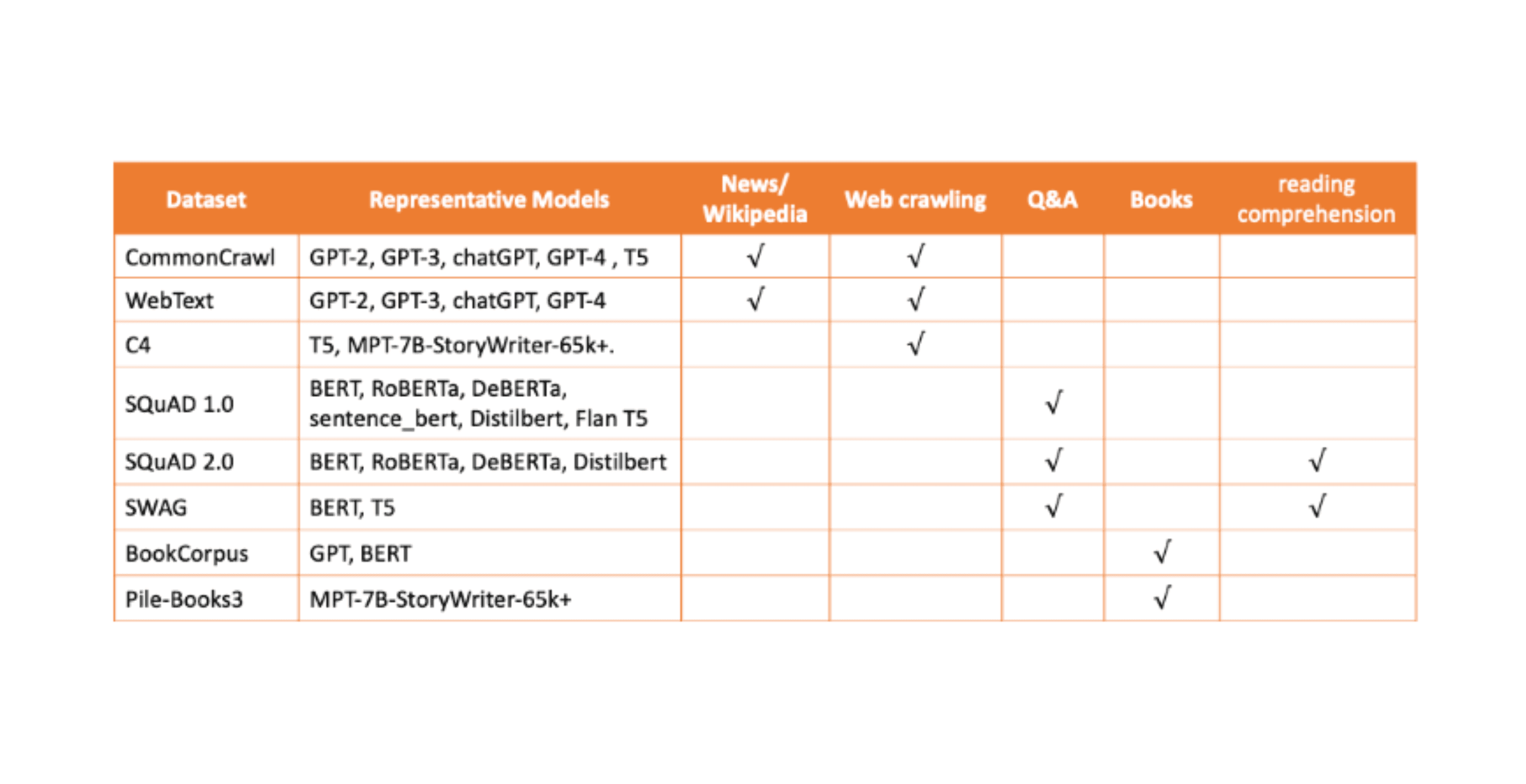
Source: Medium
GLUE: A collection of nine NLP tasks designed to evaluate model performance across various tasks.
SQUAD: A reading comprehension dataset consisting of questions posed on Wikipedia articles.
Common Crawl: A massive collection of web-crawled data that is highly diverse and useful for general domain fine-tuning.
More datasets for fine-tuning can be found here
GPT and similar models are at the forefront of NLP, offering simple text generation capabilities to complex dialogue systems. Pre-trained models are readily available through APIs, allowing developers to integrate advanced NLP features into their applications with minimal setup.
Loading A Pre-Trained Model
# Initialize tokenizer and model
From transformers import GPT2Model, GPT2Tokenizer
tokenizer = GPT2Tokenizer.from_pretrained('gpt2')
model = GPT2Model.from_pretrained('gpt2')
# Tokenize input text
inputs = tokenizer("Hello, world!", return_tensors="pt")
# Generate model output
outputs = model(**inputs)
Critical Steps in the Fine-tuning Process
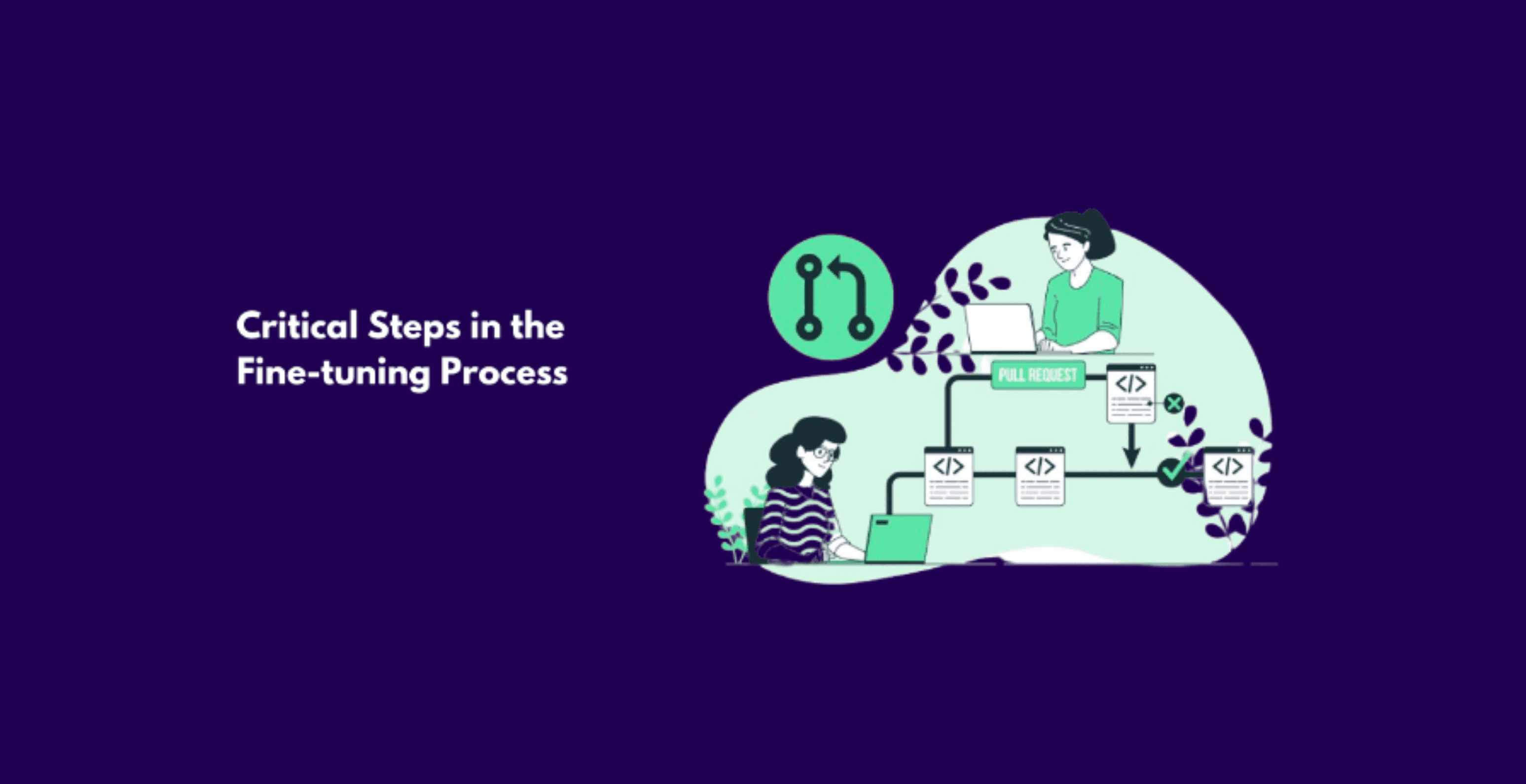
Selecting an Appropriate Model
The first step in fine-tuning involves selecting a suitable pre-trained model. This decision is pivotal because the chosen model's architecture, size, and initial training data significantly influence its adaptability to your specific task.
Architecture Consideration: Select a model architecture that is conducive to your task. For instance, GPT-3 is preferred for generative tasks, while BERT is better for classification tasks.
Size and Capacity: Larger models can achieve higher accuracy but require more computational resources for fine-tuning and inference.
Initial Pre-training Data: Consider what data the model was initially trained on; models pre-trained on domain-similar data might require less fine-tuning.
Preparing the Dataset
Proper dataset preparation is essential. It involves collecting, cleaning, and formatting your data so that it's suitable for training the model.
Dataset Collection: Compile a dataset that closely represents the task you're fine-tuning the model.
Cleaning and Preprocessing: Remove noise from the data and perform necessary preprocessing steps, including tokenization, truncation, and padding.
Splitting Data: Divide your dataset into training, validation, and test sets to ensure your model can be trained and evaluated effectively.
Executing the Fine-tuning Procedure
After preparing your dataset and selecting the appropriate model, you can proceed to fine-tune your model. This step adjusts the model's weights based on your custom dataset, improving its performance for your specific task.
Training Configuration: Define your training parameters, including learning rate, number of epochs, and batch size. These parameters significantly influence the fine-tuning outcome.
Model Training: Utilize the Trainer API or a custom training loop to fine-tune your model on the prepared dataset.
Evaluation and Adjustment: Regularly evaluate the model's performance on a validation set to adjust hyperparameters and prevent overfitting.
from transformers import GPT2LMHeadModel, GPT2Tokenizer, Trainer, TrainingArguments
model = GPT2LMHeadModel.from_pretrained('gpt2')
tokenizer = GPT2Tokenizer.from_pretrained('gpt2')# Prepare dataset
train_dataset = # Your custom dataset loading and processing logic here
# Training arguments
training_args = TrainingArguments(
output_dir='./results', # output directory
num_train_epochs=3, # total number of training epochs
per_device_train_batch_size=4, # batch size per device during training
warmup_steps=500, # number of warmup steps for learning rate scheduler
weight_decay=0.01, # strength of weight decay
)
# Initialize Trainer
trainer = Trainer(
model=model,
args=training_args,
train_dataset=train_dataset,
)
# Start training
trainer.train()
Best Practices for Successful Fine-tuning Hyperparameter Tuning: Experiment with different hyperparameters to find the best combination for your specific task. Tools like Hugging Face's Trainer offer hyperparameter search capabilities.
Monitoring and Evaluation: Use the validation set to monitor the model's performance during training. Adjust training parameters as necessary to improve results and avoid overfitting.
Incremental Training: Start with a small amount of data and gradually add more data or increase model complexity as needed. This approach can save computational resources and help identify the best training strategy.
Fine-tuning Methods and Approaches
Supervised Fine-tuning (SFT): This approach uses task-specific labeled data to guide the model's learning process.
Instruction Fine-tuning and Full Fine-tuning: These methods involve adjusting all model weights to align with the new task's requirements.
Parameter-efficient Fine-tuning (PEFT) and Quantized LoRA: These techniques aim for efficient adaptation, minimizing the resources required for fine-tuning.
Packages for Fine-tuning
Transformers by Hugging Face: A comprehensive library offering pre-trained models and utilities for NLP tasks.
Datasets by Hugging Face: A tool for loading and preparing datasets, making it easier to manage data for fine-tuning.
from datasets import load_dataset
# Load your dataset
dataset = load_dataset('path_to_your_dataset', split='train')
# Preprocess the dataset
def preprocess_function(examples):
return tokenizer(examples['text'], truncation=True, padding='max_length', max_length=512)
tokenized_dataset = dataset.map(preprocess_function, batched=True)
Selecting Datasets for Chatbot Fine-tuning
Fine-tuning a chatbot involves training the pre-existing LLM on a dataset that reflects the specific conversational context and user intents it will encounter. Here are some criteria and sources for selecting such datasets:
Task-Specific Data: Collect dialogues or conversational datasets closely aligned with the chatbot’s intended function.
Domain-Specific Interactions: For chatbots serving specific industries (e.g., healthcare, finance), incorporating industry-specific dialogue can drastically improve relevance and accuracy.
Fine-tuning Methods for Chatbots
Fine-tuning for chatbots focuses on adjusting the LLM to better understand and respond to user queries and statements in a conversational context. This involves several key methods:
Supervised Learning: Training the model on a labeled conversational dataset where inputs (user queries) and correct outputs (bot responses) are clearly defined.
Reinforcement Learning from Human Feedback (RLHF): A method where the model is fine-tuned based on feedback from human interactions, refining its responses to be more aligned with user expectations.
Data Requirement and Preparation for Fine-tuning
Need for High-quality Data
The foundation of effective fine-tuning lies in the dataset used. High-quality data that is clean, relevant, and representative of the task is crucial. This ensures the model learns the correct patterns and nuances of the specific domain or application. The following aspects are essential when considering data quality:
Relevance: The data should closely align with the task or domain the model is fine-tuning for.
Diversity: A varied dataset helps generalize the model's understanding and reduces biases.
Label Accuracy: For supervised learning tasks, the accuracy of labels directly impacts the model's performance.
Strategies for Addressing Challenges
Data Augmentation: Increase the size and diversity of your dataset through techniques such as paraphrasing, back-translation, or leveraging generative models.
Efficient Computing Resources: Utilise cloud computing services that offer scalable GPU resources to manage costs effectively. Additionally, it explores techniques like quantization and pruning to reduce model size and computational requirements.
Automated Hyperparameter Tuning: Employ tools and frameworks that support automated hyperparameter search to streamline the optimization process.
Incremental Learning: Regularly update your model with new data in smaller batches to keep it current and reduce the need for extensive retraining.
Conclusion
Fine-tuning LLMs with custom datasets is a robust process that significantly enhances the model's relevance, accuracy, and efficiency for specific tasks.
Despite its challenges, the benefits of fine-tuning—such as increased specificity and improved user interactions—make it an essential practice for anyone looking to leverage the full capabilities of Large Language Models.
As the field of NLP continues to evolve, fine-tuning remains crucial in adapting general-purpose models to meet the ever-changing needs of applications and users.
Ready to elevate your AI applications to new heights of efficiency and reliability? Join the forefront of AI innovation with RagaAI.
Whether you're working on LLMs, Computer Vision, or Tabular Data, RagaAI is designed to streamline your AI development, ensuring your projects are production-ready 3X faster. With RagaAI, you'll experience a remarkable 90% reduction in AI failures and a 50% reduction in operational costs. Try Raga today.
FAQ:
How do I choose the suitable pre-trained model for fine-tuning?
Consider the model's architecture, the dataset's size, and your application's specific requirements to select the most suitable pre-trained model.
What are the main challenges of fine-tuning LLMs?
The main challenges include acquiring high-quality, representative datasets, managing the costs of computational resources, and the need for continuous adjustments and updates to the model.
The advent of Large Language Models (LLMs) like GPT (Generative Pre-trained Transformer), Mistral, Llama, etc. has revolutionized the field of natural language processing (NLP). By understanding and generating human-like text, these models have become indispensable for various applications, from chatbots to content generation.
Understanding Pre-trained Large Language Models
LLMs utilize the Transformers architecture to comprehend and generate text, and they are trained on extensive datasets. Models like GPT have simplified the integration of NLP features into various applications.
Popular Datasets for Fine-tuning
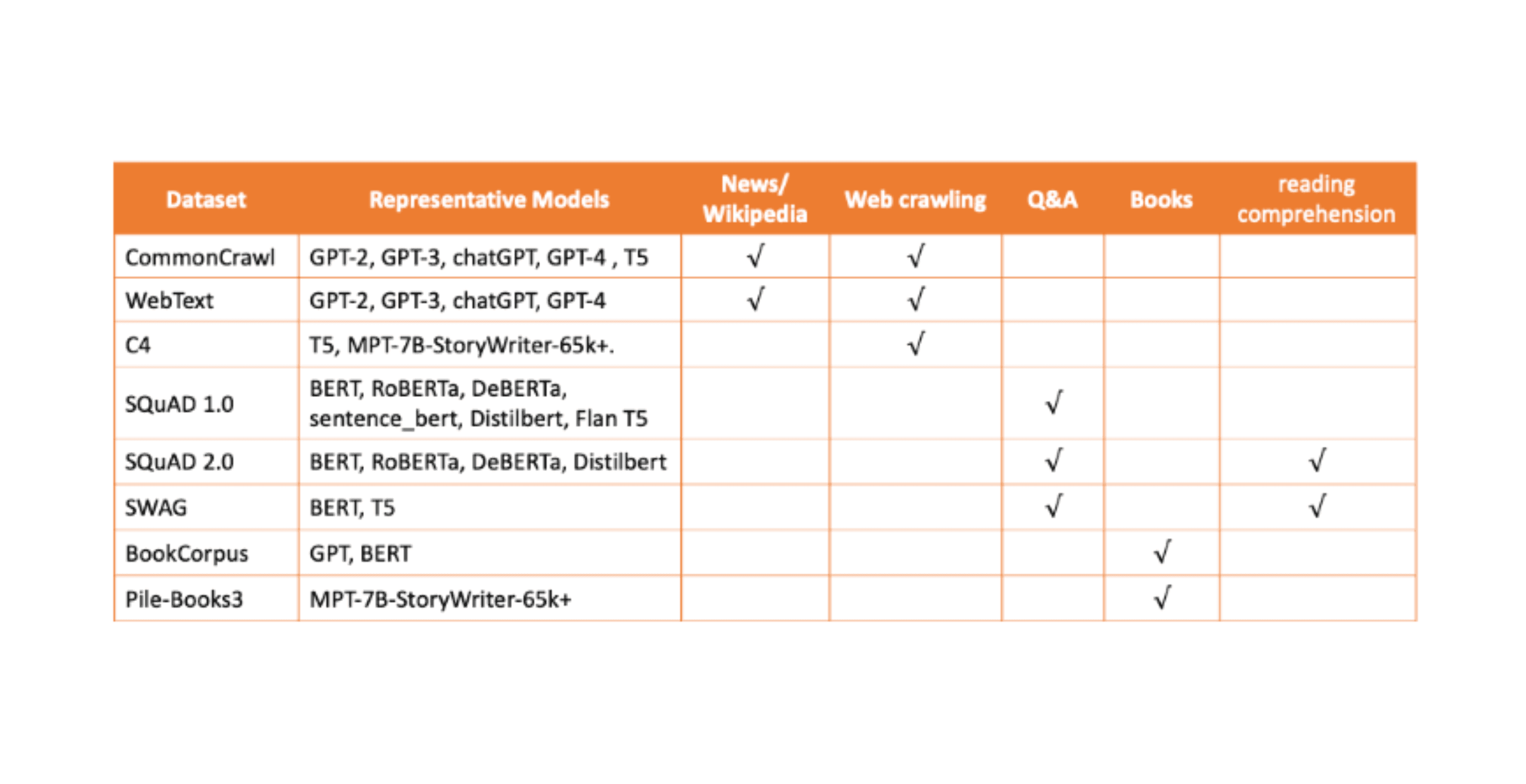
Source: Medium
GLUE: A collection of nine NLP tasks designed to evaluate model performance across various tasks.
SQUAD: A reading comprehension dataset consisting of questions posed on Wikipedia articles.
Common Crawl: A massive collection of web-crawled data that is highly diverse and useful for general domain fine-tuning.
More datasets for fine-tuning can be found here
GPT and similar models are at the forefront of NLP, offering simple text generation capabilities to complex dialogue systems. Pre-trained models are readily available through APIs, allowing developers to integrate advanced NLP features into their applications with minimal setup.
Loading A Pre-Trained Model
# Initialize tokenizer and model
From transformers import GPT2Model, GPT2Tokenizer
tokenizer = GPT2Tokenizer.from_pretrained('gpt2')
model = GPT2Model.from_pretrained('gpt2')
# Tokenize input text
inputs = tokenizer("Hello, world!", return_tensors="pt")
# Generate model output
outputs = model(**inputs)
Critical Steps in the Fine-tuning Process
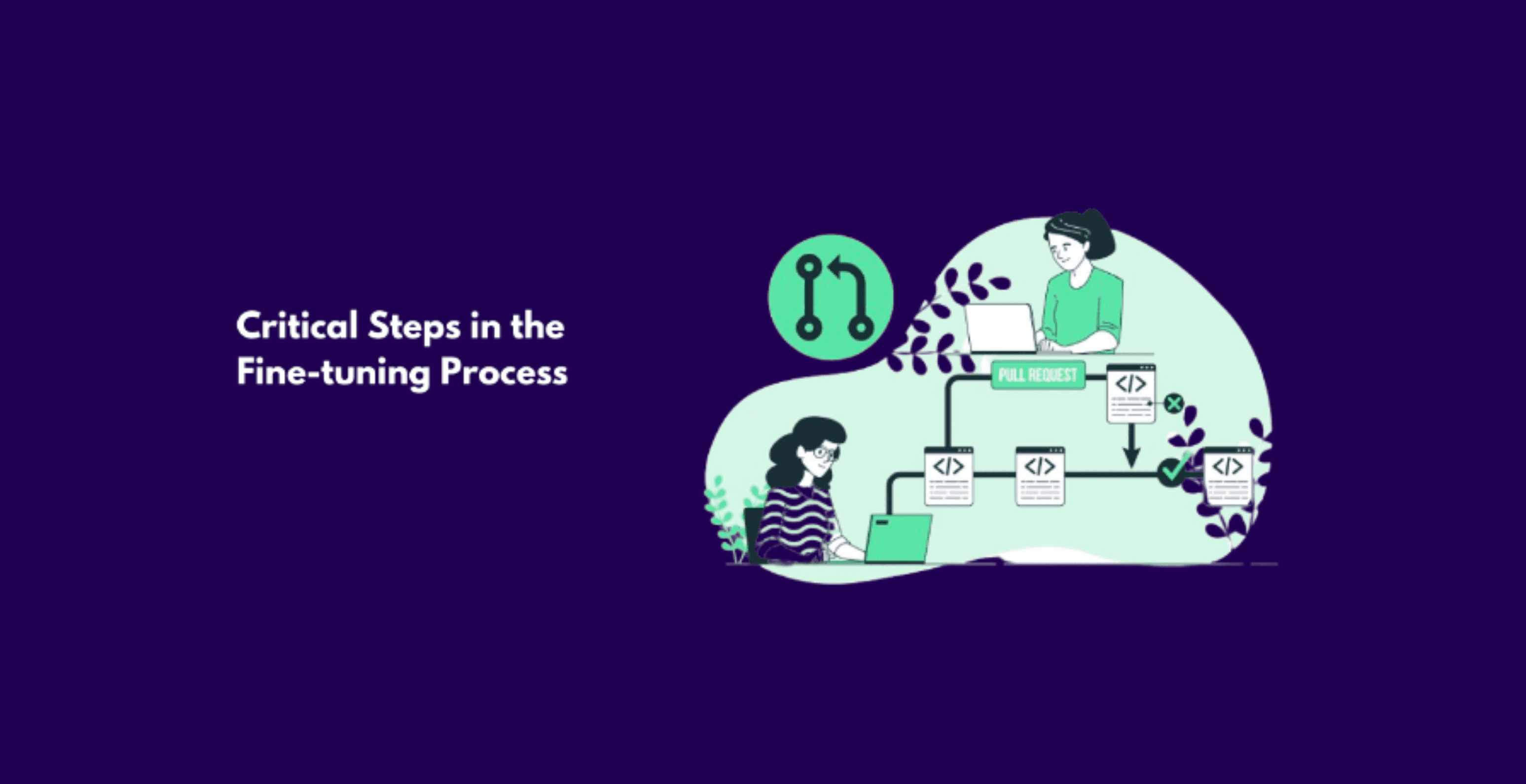
Selecting an Appropriate Model
The first step in fine-tuning involves selecting a suitable pre-trained model. This decision is pivotal because the chosen model's architecture, size, and initial training data significantly influence its adaptability to your specific task.
Architecture Consideration: Select a model architecture that is conducive to your task. For instance, GPT-3 is preferred for generative tasks, while BERT is better for classification tasks.
Size and Capacity: Larger models can achieve higher accuracy but require more computational resources for fine-tuning and inference.
Initial Pre-training Data: Consider what data the model was initially trained on; models pre-trained on domain-similar data might require less fine-tuning.
Preparing the Dataset
Proper dataset preparation is essential. It involves collecting, cleaning, and formatting your data so that it's suitable for training the model.
Dataset Collection: Compile a dataset that closely represents the task you're fine-tuning the model.
Cleaning and Preprocessing: Remove noise from the data and perform necessary preprocessing steps, including tokenization, truncation, and padding.
Splitting Data: Divide your dataset into training, validation, and test sets to ensure your model can be trained and evaluated effectively.
Executing the Fine-tuning Procedure
After preparing your dataset and selecting the appropriate model, you can proceed to fine-tune your model. This step adjusts the model's weights based on your custom dataset, improving its performance for your specific task.
Training Configuration: Define your training parameters, including learning rate, number of epochs, and batch size. These parameters significantly influence the fine-tuning outcome.
Model Training: Utilize the Trainer API or a custom training loop to fine-tune your model on the prepared dataset.
Evaluation and Adjustment: Regularly evaluate the model's performance on a validation set to adjust hyperparameters and prevent overfitting.
from transformers import GPT2LMHeadModel, GPT2Tokenizer, Trainer, TrainingArguments
model = GPT2LMHeadModel.from_pretrained('gpt2')
tokenizer = GPT2Tokenizer.from_pretrained('gpt2')# Prepare dataset
train_dataset = # Your custom dataset loading and processing logic here
# Training arguments
training_args = TrainingArguments(
output_dir='./results', # output directory
num_train_epochs=3, # total number of training epochs
per_device_train_batch_size=4, # batch size per device during training
warmup_steps=500, # number of warmup steps for learning rate scheduler
weight_decay=0.01, # strength of weight decay
)
# Initialize Trainer
trainer = Trainer(
model=model,
args=training_args,
train_dataset=train_dataset,
)
# Start training
trainer.train()
Best Practices for Successful Fine-tuning Hyperparameter Tuning: Experiment with different hyperparameters to find the best combination for your specific task. Tools like Hugging Face's Trainer offer hyperparameter search capabilities.
Monitoring and Evaluation: Use the validation set to monitor the model's performance during training. Adjust training parameters as necessary to improve results and avoid overfitting.
Incremental Training: Start with a small amount of data and gradually add more data or increase model complexity as needed. This approach can save computational resources and help identify the best training strategy.
Fine-tuning Methods and Approaches
Supervised Fine-tuning (SFT): This approach uses task-specific labeled data to guide the model's learning process.
Instruction Fine-tuning and Full Fine-tuning: These methods involve adjusting all model weights to align with the new task's requirements.
Parameter-efficient Fine-tuning (PEFT) and Quantized LoRA: These techniques aim for efficient adaptation, minimizing the resources required for fine-tuning.
Packages for Fine-tuning
Transformers by Hugging Face: A comprehensive library offering pre-trained models and utilities for NLP tasks.
Datasets by Hugging Face: A tool for loading and preparing datasets, making it easier to manage data for fine-tuning.
from datasets import load_dataset
# Load your dataset
dataset = load_dataset('path_to_your_dataset', split='train')
# Preprocess the dataset
def preprocess_function(examples):
return tokenizer(examples['text'], truncation=True, padding='max_length', max_length=512)
tokenized_dataset = dataset.map(preprocess_function, batched=True)
Selecting Datasets for Chatbot Fine-tuning
Fine-tuning a chatbot involves training the pre-existing LLM on a dataset that reflects the specific conversational context and user intents it will encounter. Here are some criteria and sources for selecting such datasets:
Task-Specific Data: Collect dialogues or conversational datasets closely aligned with the chatbot’s intended function.
Domain-Specific Interactions: For chatbots serving specific industries (e.g., healthcare, finance), incorporating industry-specific dialogue can drastically improve relevance and accuracy.
Fine-tuning Methods for Chatbots
Fine-tuning for chatbots focuses on adjusting the LLM to better understand and respond to user queries and statements in a conversational context. This involves several key methods:
Supervised Learning: Training the model on a labeled conversational dataset where inputs (user queries) and correct outputs (bot responses) are clearly defined.
Reinforcement Learning from Human Feedback (RLHF): A method where the model is fine-tuned based on feedback from human interactions, refining its responses to be more aligned with user expectations.
Data Requirement and Preparation for Fine-tuning
Need for High-quality Data
The foundation of effective fine-tuning lies in the dataset used. High-quality data that is clean, relevant, and representative of the task is crucial. This ensures the model learns the correct patterns and nuances of the specific domain or application. The following aspects are essential when considering data quality:
Relevance: The data should closely align with the task or domain the model is fine-tuning for.
Diversity: A varied dataset helps generalize the model's understanding and reduces biases.
Label Accuracy: For supervised learning tasks, the accuracy of labels directly impacts the model's performance.
Strategies for Addressing Challenges
Data Augmentation: Increase the size and diversity of your dataset through techniques such as paraphrasing, back-translation, or leveraging generative models.
Efficient Computing Resources: Utilise cloud computing services that offer scalable GPU resources to manage costs effectively. Additionally, it explores techniques like quantization and pruning to reduce model size and computational requirements.
Automated Hyperparameter Tuning: Employ tools and frameworks that support automated hyperparameter search to streamline the optimization process.
Incremental Learning: Regularly update your model with new data in smaller batches to keep it current and reduce the need for extensive retraining.
Conclusion
Fine-tuning LLMs with custom datasets is a robust process that significantly enhances the model's relevance, accuracy, and efficiency for specific tasks.
Despite its challenges, the benefits of fine-tuning—such as increased specificity and improved user interactions—make it an essential practice for anyone looking to leverage the full capabilities of Large Language Models.
As the field of NLP continues to evolve, fine-tuning remains crucial in adapting general-purpose models to meet the ever-changing needs of applications and users.
Ready to elevate your AI applications to new heights of efficiency and reliability? Join the forefront of AI innovation with RagaAI.
Whether you're working on LLMs, Computer Vision, or Tabular Data, RagaAI is designed to streamline your AI development, ensuring your projects are production-ready 3X faster. With RagaAI, you'll experience a remarkable 90% reduction in AI failures and a 50% reduction in operational costs. Try Raga today.
FAQ:
How do I choose the suitable pre-trained model for fine-tuning?
Consider the model's architecture, the dataset's size, and your application's specific requirements to select the most suitable pre-trained model.
What are the main challenges of fine-tuning LLMs?
The main challenges include acquiring high-quality, representative datasets, managing the costs of computational resources, and the need for continuous adjustments and updates to the model.
The advent of Large Language Models (LLMs) like GPT (Generative Pre-trained Transformer), Mistral, Llama, etc. has revolutionized the field of natural language processing (NLP). By understanding and generating human-like text, these models have become indispensable for various applications, from chatbots to content generation.
Understanding Pre-trained Large Language Models
LLMs utilize the Transformers architecture to comprehend and generate text, and they are trained on extensive datasets. Models like GPT have simplified the integration of NLP features into various applications.
Popular Datasets for Fine-tuning
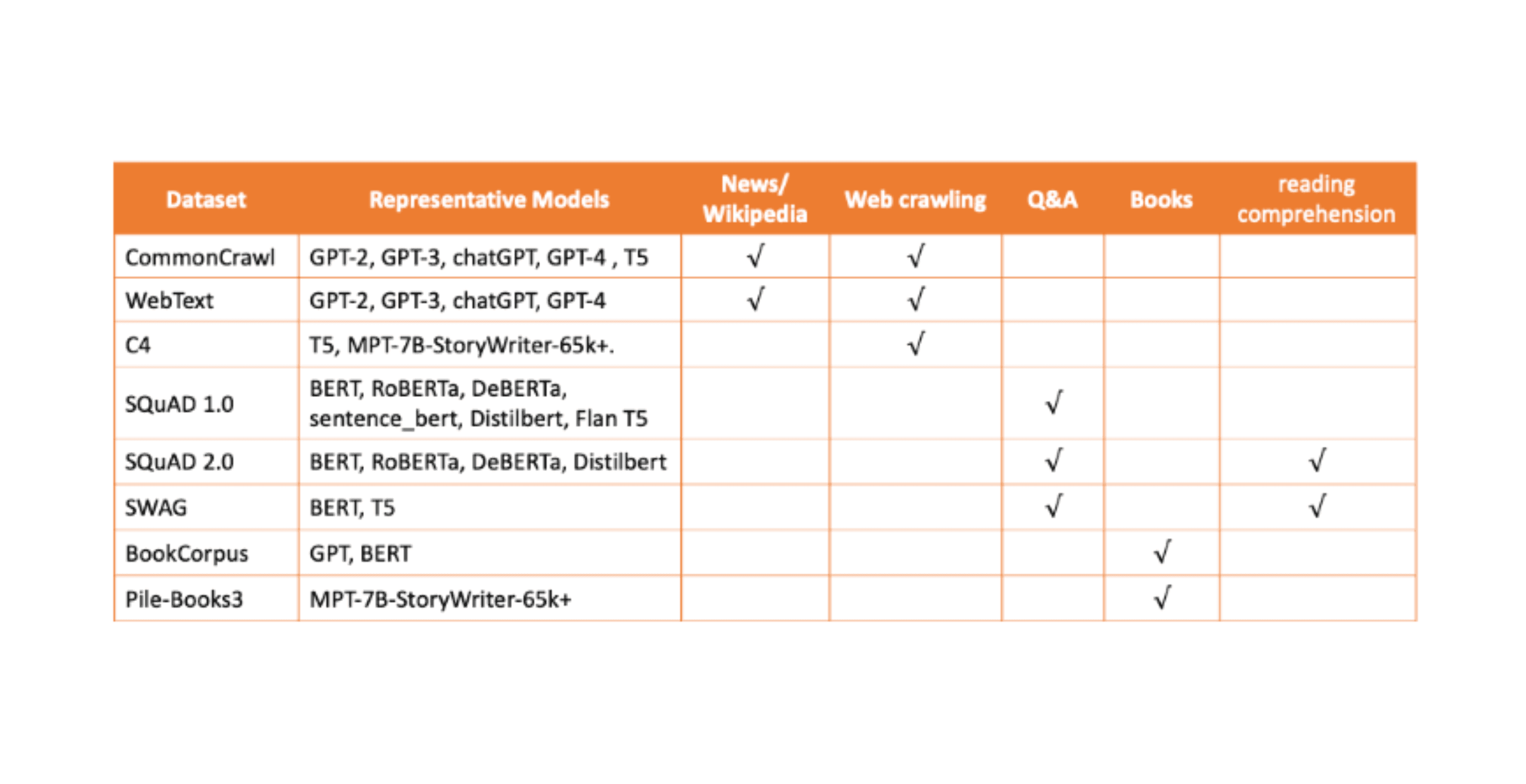
Source: Medium
GLUE: A collection of nine NLP tasks designed to evaluate model performance across various tasks.
SQUAD: A reading comprehension dataset consisting of questions posed on Wikipedia articles.
Common Crawl: A massive collection of web-crawled data that is highly diverse and useful for general domain fine-tuning.
More datasets for fine-tuning can be found here
GPT and similar models are at the forefront of NLP, offering simple text generation capabilities to complex dialogue systems. Pre-trained models are readily available through APIs, allowing developers to integrate advanced NLP features into their applications with minimal setup.
Loading A Pre-Trained Model
# Initialize tokenizer and model
From transformers import GPT2Model, GPT2Tokenizer
tokenizer = GPT2Tokenizer.from_pretrained('gpt2')
model = GPT2Model.from_pretrained('gpt2')
# Tokenize input text
inputs = tokenizer("Hello, world!", return_tensors="pt")
# Generate model output
outputs = model(**inputs)
Critical Steps in the Fine-tuning Process
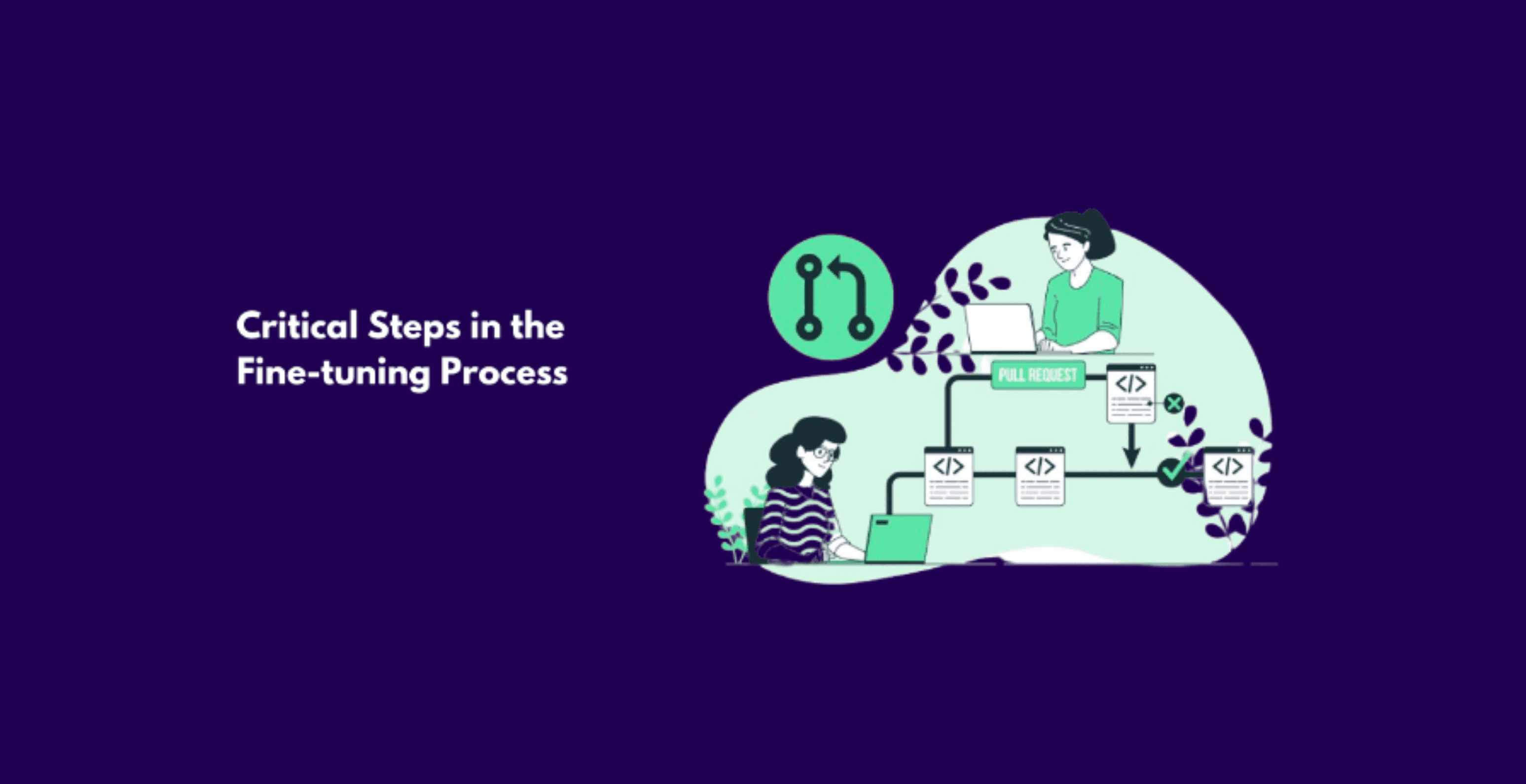
Selecting an Appropriate Model
The first step in fine-tuning involves selecting a suitable pre-trained model. This decision is pivotal because the chosen model's architecture, size, and initial training data significantly influence its adaptability to your specific task.
Architecture Consideration: Select a model architecture that is conducive to your task. For instance, GPT-3 is preferred for generative tasks, while BERT is better for classification tasks.
Size and Capacity: Larger models can achieve higher accuracy but require more computational resources for fine-tuning and inference.
Initial Pre-training Data: Consider what data the model was initially trained on; models pre-trained on domain-similar data might require less fine-tuning.
Preparing the Dataset
Proper dataset preparation is essential. It involves collecting, cleaning, and formatting your data so that it's suitable for training the model.
Dataset Collection: Compile a dataset that closely represents the task you're fine-tuning the model.
Cleaning and Preprocessing: Remove noise from the data and perform necessary preprocessing steps, including tokenization, truncation, and padding.
Splitting Data: Divide your dataset into training, validation, and test sets to ensure your model can be trained and evaluated effectively.
Executing the Fine-tuning Procedure
After preparing your dataset and selecting the appropriate model, you can proceed to fine-tune your model. This step adjusts the model's weights based on your custom dataset, improving its performance for your specific task.
Training Configuration: Define your training parameters, including learning rate, number of epochs, and batch size. These parameters significantly influence the fine-tuning outcome.
Model Training: Utilize the Trainer API or a custom training loop to fine-tune your model on the prepared dataset.
Evaluation and Adjustment: Regularly evaluate the model's performance on a validation set to adjust hyperparameters and prevent overfitting.
from transformers import GPT2LMHeadModel, GPT2Tokenizer, Trainer, TrainingArguments
model = GPT2LMHeadModel.from_pretrained('gpt2')
tokenizer = GPT2Tokenizer.from_pretrained('gpt2')# Prepare dataset
train_dataset = # Your custom dataset loading and processing logic here
# Training arguments
training_args = TrainingArguments(
output_dir='./results', # output directory
num_train_epochs=3, # total number of training epochs
per_device_train_batch_size=4, # batch size per device during training
warmup_steps=500, # number of warmup steps for learning rate scheduler
weight_decay=0.01, # strength of weight decay
)
# Initialize Trainer
trainer = Trainer(
model=model,
args=training_args,
train_dataset=train_dataset,
)
# Start training
trainer.train()
Best Practices for Successful Fine-tuning Hyperparameter Tuning: Experiment with different hyperparameters to find the best combination for your specific task. Tools like Hugging Face's Trainer offer hyperparameter search capabilities.
Monitoring and Evaluation: Use the validation set to monitor the model's performance during training. Adjust training parameters as necessary to improve results and avoid overfitting.
Incremental Training: Start with a small amount of data and gradually add more data or increase model complexity as needed. This approach can save computational resources and help identify the best training strategy.
Fine-tuning Methods and Approaches
Supervised Fine-tuning (SFT): This approach uses task-specific labeled data to guide the model's learning process.
Instruction Fine-tuning and Full Fine-tuning: These methods involve adjusting all model weights to align with the new task's requirements.
Parameter-efficient Fine-tuning (PEFT) and Quantized LoRA: These techniques aim for efficient adaptation, minimizing the resources required for fine-tuning.
Packages for Fine-tuning
Transformers by Hugging Face: A comprehensive library offering pre-trained models and utilities for NLP tasks.
Datasets by Hugging Face: A tool for loading and preparing datasets, making it easier to manage data for fine-tuning.
from datasets import load_dataset
# Load your dataset
dataset = load_dataset('path_to_your_dataset', split='train')
# Preprocess the dataset
def preprocess_function(examples):
return tokenizer(examples['text'], truncation=True, padding='max_length', max_length=512)
tokenized_dataset = dataset.map(preprocess_function, batched=True)
Selecting Datasets for Chatbot Fine-tuning
Fine-tuning a chatbot involves training the pre-existing LLM on a dataset that reflects the specific conversational context and user intents it will encounter. Here are some criteria and sources for selecting such datasets:
Task-Specific Data: Collect dialogues or conversational datasets closely aligned with the chatbot’s intended function.
Domain-Specific Interactions: For chatbots serving specific industries (e.g., healthcare, finance), incorporating industry-specific dialogue can drastically improve relevance and accuracy.
Fine-tuning Methods for Chatbots
Fine-tuning for chatbots focuses on adjusting the LLM to better understand and respond to user queries and statements in a conversational context. This involves several key methods:
Supervised Learning: Training the model on a labeled conversational dataset where inputs (user queries) and correct outputs (bot responses) are clearly defined.
Reinforcement Learning from Human Feedback (RLHF): A method where the model is fine-tuned based on feedback from human interactions, refining its responses to be more aligned with user expectations.
Data Requirement and Preparation for Fine-tuning
Need for High-quality Data
The foundation of effective fine-tuning lies in the dataset used. High-quality data that is clean, relevant, and representative of the task is crucial. This ensures the model learns the correct patterns and nuances of the specific domain or application. The following aspects are essential when considering data quality:
Relevance: The data should closely align with the task or domain the model is fine-tuning for.
Diversity: A varied dataset helps generalize the model's understanding and reduces biases.
Label Accuracy: For supervised learning tasks, the accuracy of labels directly impacts the model's performance.
Strategies for Addressing Challenges
Data Augmentation: Increase the size and diversity of your dataset through techniques such as paraphrasing, back-translation, or leveraging generative models.
Efficient Computing Resources: Utilise cloud computing services that offer scalable GPU resources to manage costs effectively. Additionally, it explores techniques like quantization and pruning to reduce model size and computational requirements.
Automated Hyperparameter Tuning: Employ tools and frameworks that support automated hyperparameter search to streamline the optimization process.
Incremental Learning: Regularly update your model with new data in smaller batches to keep it current and reduce the need for extensive retraining.
Conclusion
Fine-tuning LLMs with custom datasets is a robust process that significantly enhances the model's relevance, accuracy, and efficiency for specific tasks.
Despite its challenges, the benefits of fine-tuning—such as increased specificity and improved user interactions—make it an essential practice for anyone looking to leverage the full capabilities of Large Language Models.
As the field of NLP continues to evolve, fine-tuning remains crucial in adapting general-purpose models to meet the ever-changing needs of applications and users.
Ready to elevate your AI applications to new heights of efficiency and reliability? Join the forefront of AI innovation with RagaAI.
Whether you're working on LLMs, Computer Vision, or Tabular Data, RagaAI is designed to streamline your AI development, ensuring your projects are production-ready 3X faster. With RagaAI, you'll experience a remarkable 90% reduction in AI failures and a 50% reduction in operational costs. Try Raga today.
FAQ:
How do I choose the suitable pre-trained model for fine-tuning?
Consider the model's architecture, the dataset's size, and your application's specific requirements to select the most suitable pre-trained model.
What are the main challenges of fine-tuning LLMs?
The main challenges include acquiring high-quality, representative datasets, managing the costs of computational resources, and the need for continuous adjustments and updates to the model.
The advent of Large Language Models (LLMs) like GPT (Generative Pre-trained Transformer), Mistral, Llama, etc. has revolutionized the field of natural language processing (NLP). By understanding and generating human-like text, these models have become indispensable for various applications, from chatbots to content generation.
Understanding Pre-trained Large Language Models
LLMs utilize the Transformers architecture to comprehend and generate text, and they are trained on extensive datasets. Models like GPT have simplified the integration of NLP features into various applications.
Popular Datasets for Fine-tuning
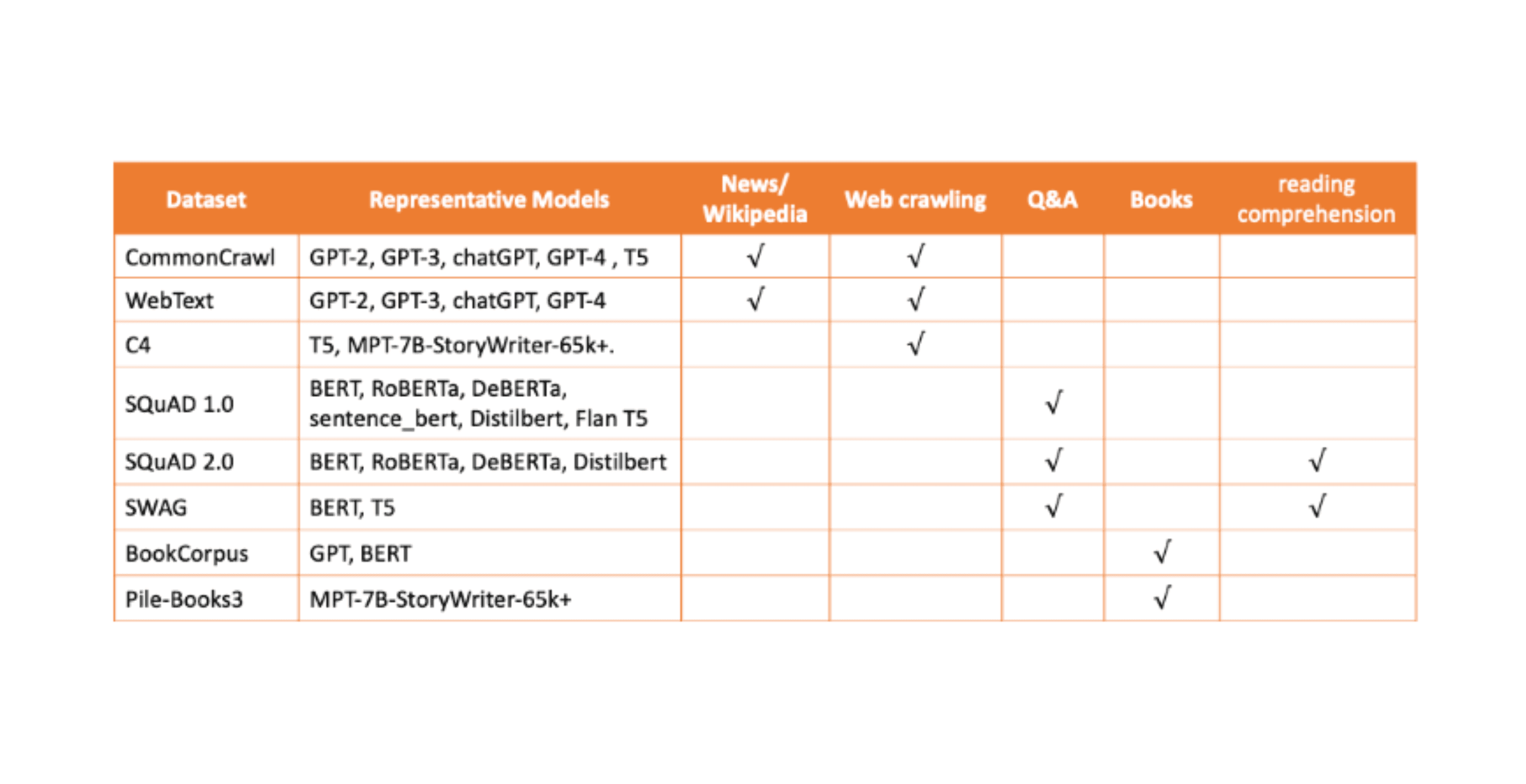
Source: Medium
GLUE: A collection of nine NLP tasks designed to evaluate model performance across various tasks.
SQUAD: A reading comprehension dataset consisting of questions posed on Wikipedia articles.
Common Crawl: A massive collection of web-crawled data that is highly diverse and useful for general domain fine-tuning.
More datasets for fine-tuning can be found here
GPT and similar models are at the forefront of NLP, offering simple text generation capabilities to complex dialogue systems. Pre-trained models are readily available through APIs, allowing developers to integrate advanced NLP features into their applications with minimal setup.
Loading A Pre-Trained Model
# Initialize tokenizer and model
From transformers import GPT2Model, GPT2Tokenizer
tokenizer = GPT2Tokenizer.from_pretrained('gpt2')
model = GPT2Model.from_pretrained('gpt2')
# Tokenize input text
inputs = tokenizer("Hello, world!", return_tensors="pt")
# Generate model output
outputs = model(**inputs)
Critical Steps in the Fine-tuning Process
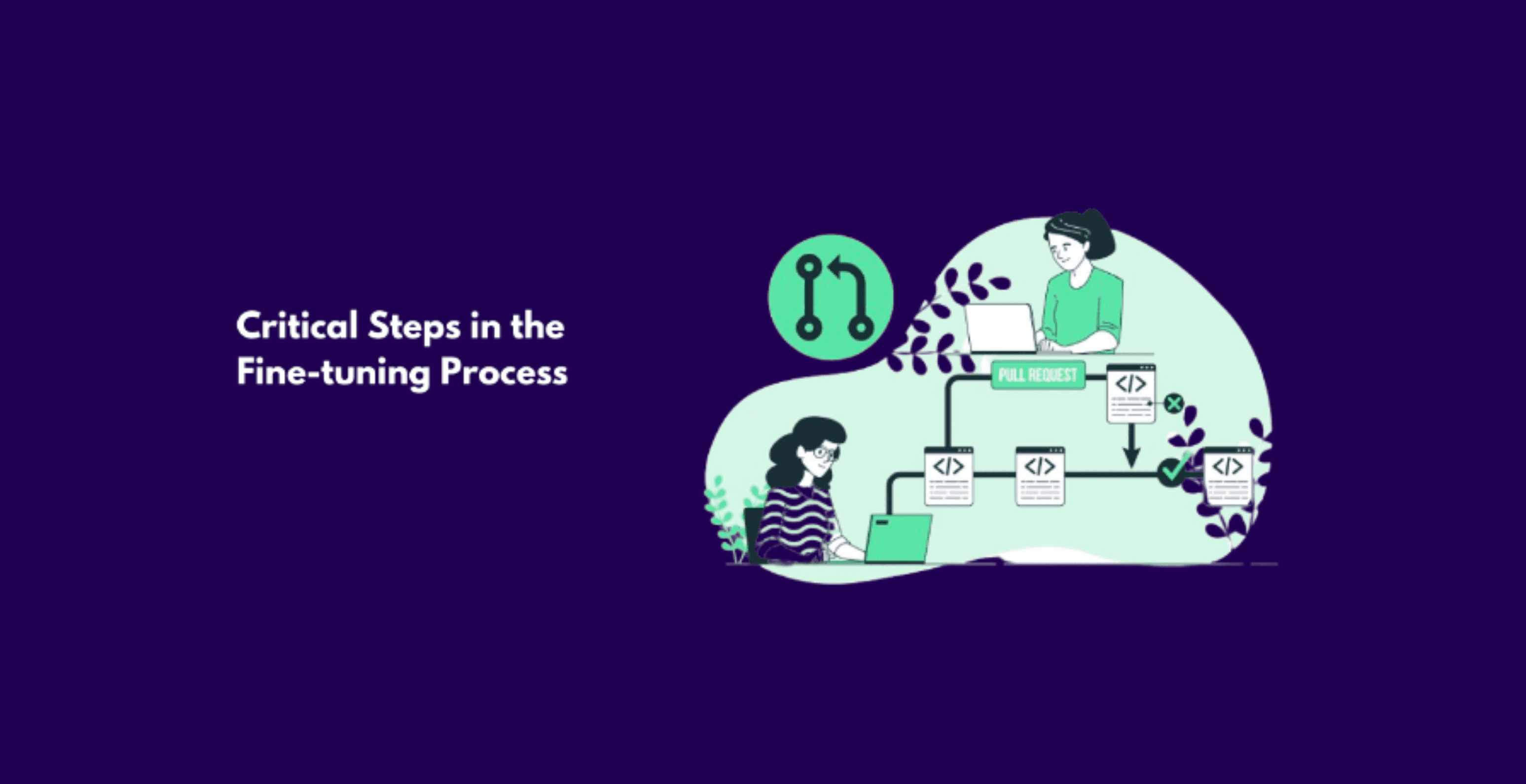
Selecting an Appropriate Model
The first step in fine-tuning involves selecting a suitable pre-trained model. This decision is pivotal because the chosen model's architecture, size, and initial training data significantly influence its adaptability to your specific task.
Architecture Consideration: Select a model architecture that is conducive to your task. For instance, GPT-3 is preferred for generative tasks, while BERT is better for classification tasks.
Size and Capacity: Larger models can achieve higher accuracy but require more computational resources for fine-tuning and inference.
Initial Pre-training Data: Consider what data the model was initially trained on; models pre-trained on domain-similar data might require less fine-tuning.
Preparing the Dataset
Proper dataset preparation is essential. It involves collecting, cleaning, and formatting your data so that it's suitable for training the model.
Dataset Collection: Compile a dataset that closely represents the task you're fine-tuning the model.
Cleaning and Preprocessing: Remove noise from the data and perform necessary preprocessing steps, including tokenization, truncation, and padding.
Splitting Data: Divide your dataset into training, validation, and test sets to ensure your model can be trained and evaluated effectively.
Executing the Fine-tuning Procedure
After preparing your dataset and selecting the appropriate model, you can proceed to fine-tune your model. This step adjusts the model's weights based on your custom dataset, improving its performance for your specific task.
Training Configuration: Define your training parameters, including learning rate, number of epochs, and batch size. These parameters significantly influence the fine-tuning outcome.
Model Training: Utilize the Trainer API or a custom training loop to fine-tune your model on the prepared dataset.
Evaluation and Adjustment: Regularly evaluate the model's performance on a validation set to adjust hyperparameters and prevent overfitting.
from transformers import GPT2LMHeadModel, GPT2Tokenizer, Trainer, TrainingArguments
model = GPT2LMHeadModel.from_pretrained('gpt2')
tokenizer = GPT2Tokenizer.from_pretrained('gpt2')# Prepare dataset
train_dataset = # Your custom dataset loading and processing logic here
# Training arguments
training_args = TrainingArguments(
output_dir='./results', # output directory
num_train_epochs=3, # total number of training epochs
per_device_train_batch_size=4, # batch size per device during training
warmup_steps=500, # number of warmup steps for learning rate scheduler
weight_decay=0.01, # strength of weight decay
)
# Initialize Trainer
trainer = Trainer(
model=model,
args=training_args,
train_dataset=train_dataset,
)
# Start training
trainer.train()
Best Practices for Successful Fine-tuning Hyperparameter Tuning: Experiment with different hyperparameters to find the best combination for your specific task. Tools like Hugging Face's Trainer offer hyperparameter search capabilities.
Monitoring and Evaluation: Use the validation set to monitor the model's performance during training. Adjust training parameters as necessary to improve results and avoid overfitting.
Incremental Training: Start with a small amount of data and gradually add more data or increase model complexity as needed. This approach can save computational resources and help identify the best training strategy.
Fine-tuning Methods and Approaches
Supervised Fine-tuning (SFT): This approach uses task-specific labeled data to guide the model's learning process.
Instruction Fine-tuning and Full Fine-tuning: These methods involve adjusting all model weights to align with the new task's requirements.
Parameter-efficient Fine-tuning (PEFT) and Quantized LoRA: These techniques aim for efficient adaptation, minimizing the resources required for fine-tuning.
Packages for Fine-tuning
Transformers by Hugging Face: A comprehensive library offering pre-trained models and utilities for NLP tasks.
Datasets by Hugging Face: A tool for loading and preparing datasets, making it easier to manage data for fine-tuning.
from datasets import load_dataset
# Load your dataset
dataset = load_dataset('path_to_your_dataset', split='train')
# Preprocess the dataset
def preprocess_function(examples):
return tokenizer(examples['text'], truncation=True, padding='max_length', max_length=512)
tokenized_dataset = dataset.map(preprocess_function, batched=True)
Selecting Datasets for Chatbot Fine-tuning
Fine-tuning a chatbot involves training the pre-existing LLM on a dataset that reflects the specific conversational context and user intents it will encounter. Here are some criteria and sources for selecting such datasets:
Task-Specific Data: Collect dialogues or conversational datasets closely aligned with the chatbot’s intended function.
Domain-Specific Interactions: For chatbots serving specific industries (e.g., healthcare, finance), incorporating industry-specific dialogue can drastically improve relevance and accuracy.
Fine-tuning Methods for Chatbots
Fine-tuning for chatbots focuses on adjusting the LLM to better understand and respond to user queries and statements in a conversational context. This involves several key methods:
Supervised Learning: Training the model on a labeled conversational dataset where inputs (user queries) and correct outputs (bot responses) are clearly defined.
Reinforcement Learning from Human Feedback (RLHF): A method where the model is fine-tuned based on feedback from human interactions, refining its responses to be more aligned with user expectations.
Data Requirement and Preparation for Fine-tuning
Need for High-quality Data
The foundation of effective fine-tuning lies in the dataset used. High-quality data that is clean, relevant, and representative of the task is crucial. This ensures the model learns the correct patterns and nuances of the specific domain or application. The following aspects are essential when considering data quality:
Relevance: The data should closely align with the task or domain the model is fine-tuning for.
Diversity: A varied dataset helps generalize the model's understanding and reduces biases.
Label Accuracy: For supervised learning tasks, the accuracy of labels directly impacts the model's performance.
Strategies for Addressing Challenges
Data Augmentation: Increase the size and diversity of your dataset through techniques such as paraphrasing, back-translation, or leveraging generative models.
Efficient Computing Resources: Utilise cloud computing services that offer scalable GPU resources to manage costs effectively. Additionally, it explores techniques like quantization and pruning to reduce model size and computational requirements.
Automated Hyperparameter Tuning: Employ tools and frameworks that support automated hyperparameter search to streamline the optimization process.
Incremental Learning: Regularly update your model with new data in smaller batches to keep it current and reduce the need for extensive retraining.
Conclusion
Fine-tuning LLMs with custom datasets is a robust process that significantly enhances the model's relevance, accuracy, and efficiency for specific tasks.
Despite its challenges, the benefits of fine-tuning—such as increased specificity and improved user interactions—make it an essential practice for anyone looking to leverage the full capabilities of Large Language Models.
As the field of NLP continues to evolve, fine-tuning remains crucial in adapting general-purpose models to meet the ever-changing needs of applications and users.
Ready to elevate your AI applications to new heights of efficiency and reliability? Join the forefront of AI innovation with RagaAI.
Whether you're working on LLMs, Computer Vision, or Tabular Data, RagaAI is designed to streamline your AI development, ensuring your projects are production-ready 3X faster. With RagaAI, you'll experience a remarkable 90% reduction in AI failures and a 50% reduction in operational costs. Try Raga today.
FAQ:
How do I choose the suitable pre-trained model for fine-tuning?
Consider the model's architecture, the dataset's size, and your application's specific requirements to select the most suitable pre-trained model.
What are the main challenges of fine-tuning LLMs?
The main challenges include acquiring high-quality, representative datasets, managing the costs of computational resources, and the need for continuous adjustments and updates to the model.
Subscribe to our newsletter to never miss an update
Other articles


Agents Talk, Models Think: A2A + MCP for Enterprise Agentic AI

Riya Parikh, Nitai Agarwal
Apr 27, 2025
Read the article
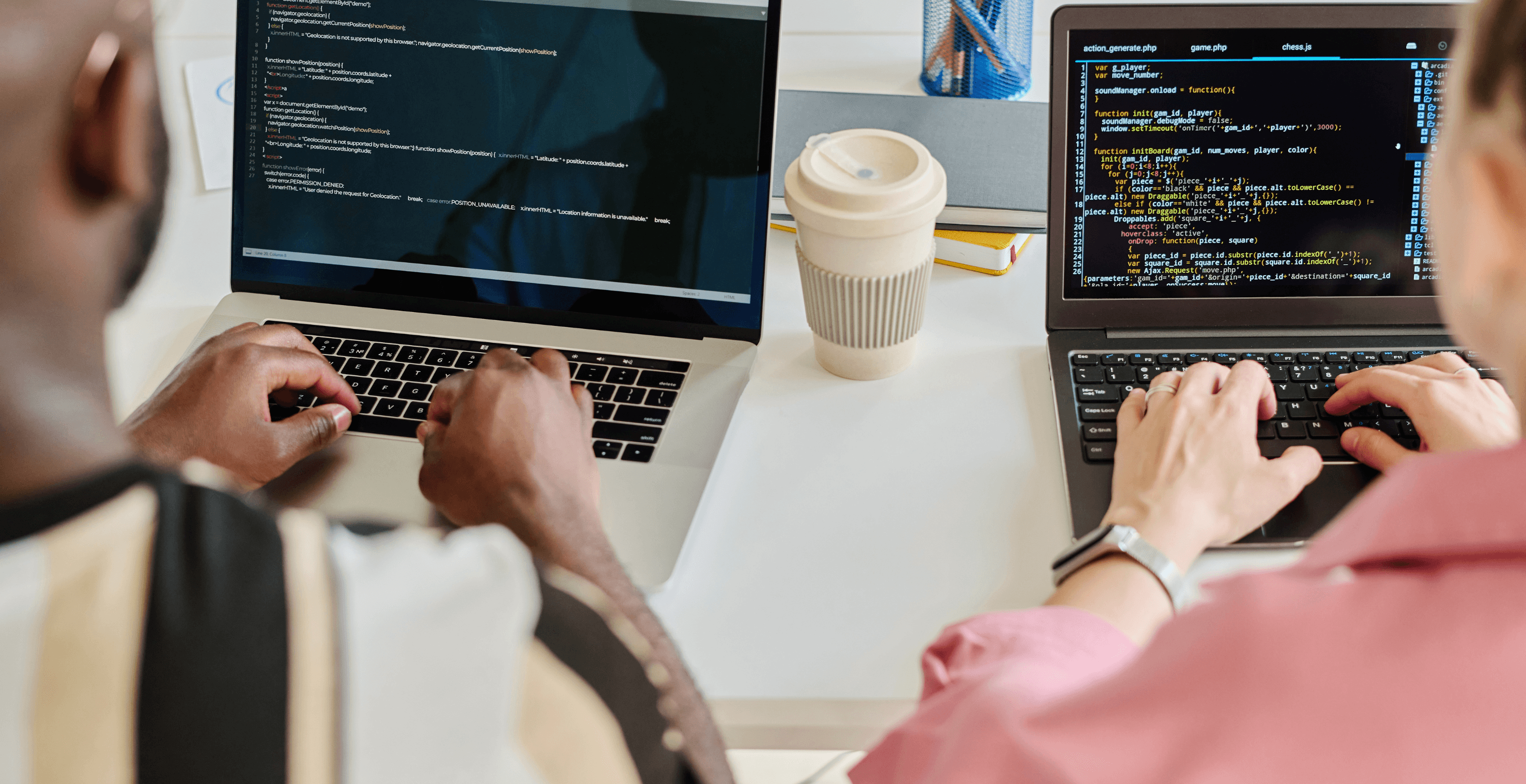
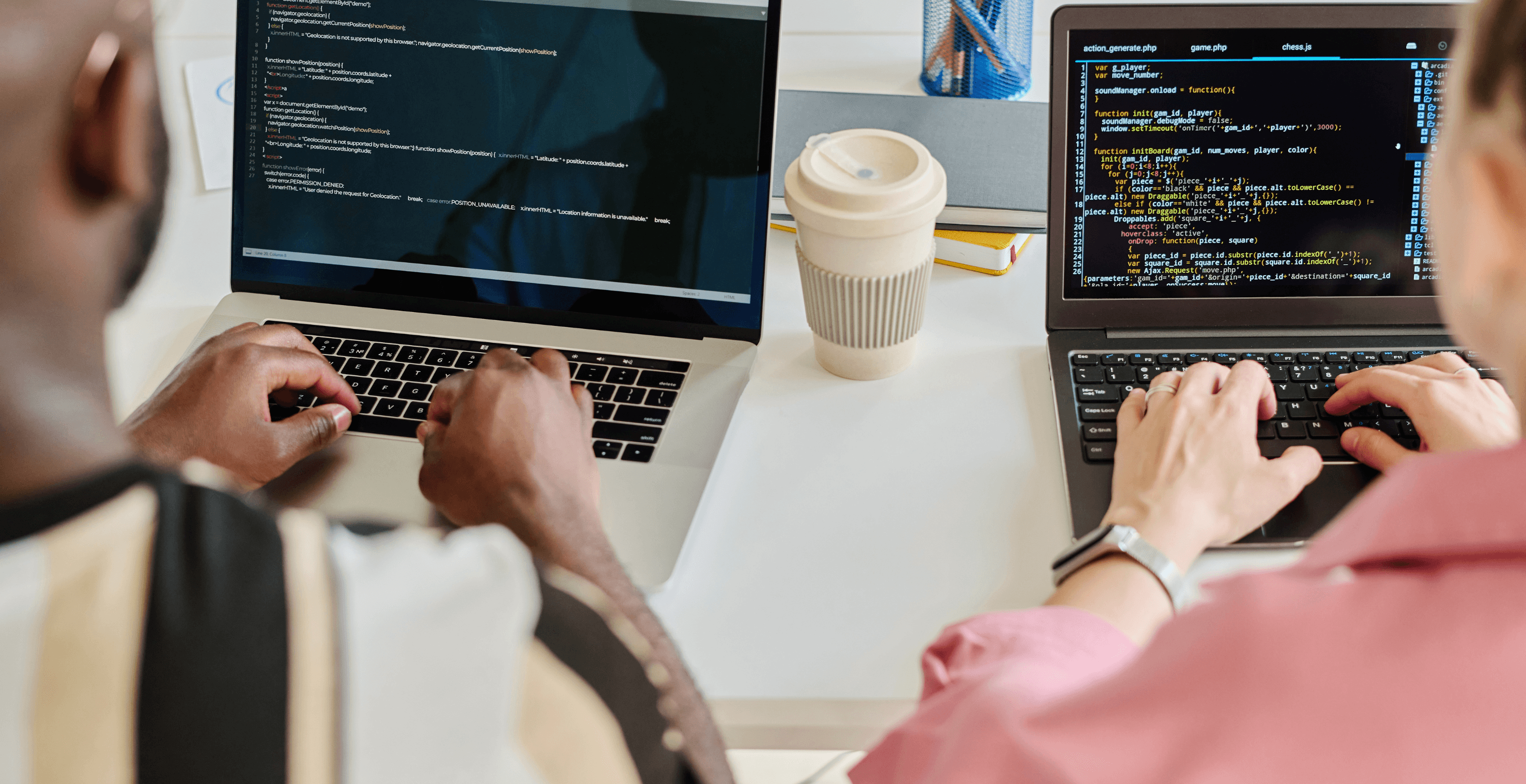
Understanding NeMo Guardrails: A Toolkit for LLM Security

Rehan Asif
Dec 24, 2024
Read the article


Understanding Differences in Large vs Small Language Models (LLM vs SLM)

Rehan Asif
Dec 21, 2024
Read the article