Customer Case Study
Advancing Geospatial Semantic Segmentation through Automated Testing with RagaAI
Object Detection
Agriculture, Climate Change, Maps, Defence
Client Profile
RagaAI’s client, a prominent player in the geospatial industry, undertook the challenge of deploying cutting-edge semantic segmentation models to enhance their mapping and geospatial intelligence capabilities. Focused on delivering accurate results, they used the RagaAI Testing Platform to holistically evaluate and fix gaps in the AI development process.
Challenge
Our client, a leader in the geospatial industry, embarked on a mission to elevate its mapping and geospatial intelligence capabilities by deploying state-of-the-art semantic segmentation models. Focused on achieving unparalleled accuracy, the client faced challenges related to data annotations, model performance in edge cases, data drift in production environments, model explainability, and overall robustness. Seeking a comprehensive solution, they turned to RagaAI to address these multifaceted issues.
Solution
RagaAI's Testing Platform, tailored for geospatial applications, provided a holistic evaluation of the client's models. At the core of this solution was RagaAI DNA, a proprietary foundational model meticulously tuned for geospatial applications. This specialised model, developed as RagaAI's intellectual property, became the linchpin for resolving challenges unique to the geospatial domain.
Approaches
Failure Mode Analysis
Identifying clusters in the dataset where the model is performing poorly.
Improving model for the clusters by fine-tuning with more dataset for these clusters.
Data Labelling Tests
Identified issues such as wrong borders, wrong pixel annotations, incorrect labelling by using RagaAI automated labelling inconsistency tests.
Model improved by removing/correcting labelling issues by 13%.
Data Drift
Using RagaAI s post monitoring led to the identification of data drift in the field data set w.r.t training data sets.
Mitigated data drift, by ensuring re-labelling and retraining on the drifted data set to ensure performance of models over time.
Model A/B Testing
Improved models were regression tested against previous version of model to check for significant changes.
Ensuring that model updates do not degrade existing functionalities RagaAI runs regression testing very thoroughly.
Stress Testing Using Synthetic Data
Implemented stress testing with generating new scenarios using Generative AI techniques.
Stress Testing the models on these scenarios to ensure optimum model performance on these new scenarios.
At a Glance
Challenges
Enhance accuracy of geospatial semantic segmentation models.
Solutions
RagaAI Testing Platform for comprehensive model evaluation
RagaAI DNA, a specialised foundational model for geospatial applications.
Results
75% Initial Model Accuracy
91% Post-deployment Model Accuracy
Conclusion
Transformation:
RagaAI's solutions revolutionized geospatial AI testing.
Key Components:
Failure mode analysis, precise data labelling, adaptive data drift strategies, A/B testing, and synthetic data stress testing5.
Gains Achieved:
Marked improvement in accuracy, reliability, and overall model performance5
Cost-Effective:
RagaAI emerged as a cost-effective alternative to in-house development5
Excellence Commitment:
Reinforced RagaAI's commitment to setting new geospatial standards.
Other Case Studies
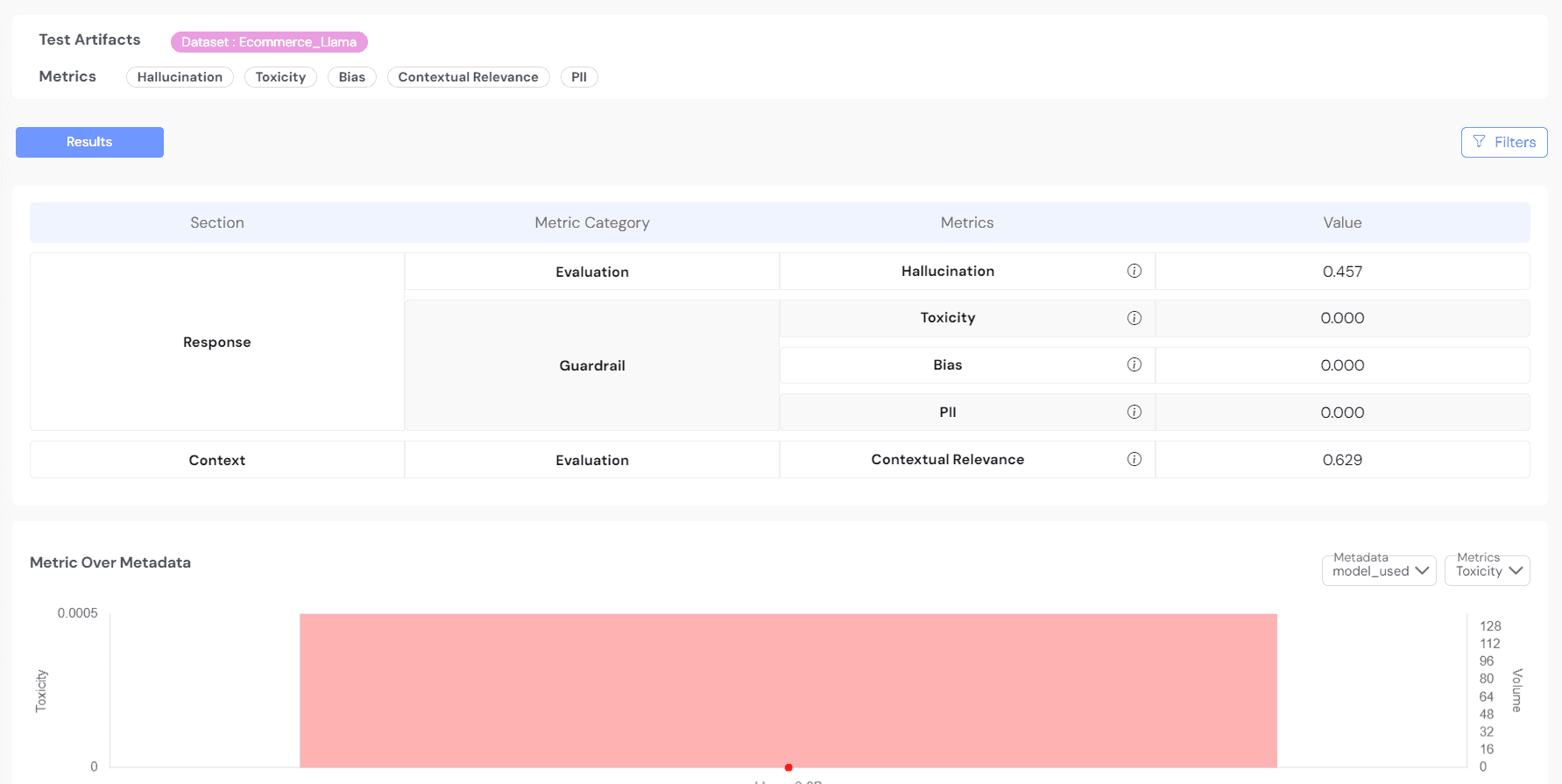
Safeguarding Enterprise LLM Applications: Enhancing Reliability with RagaAI's Guardrails
Safeguarding Enterprise LLM Applications: Enhancing Reliability with RagaAI's Guardrails
Read the article
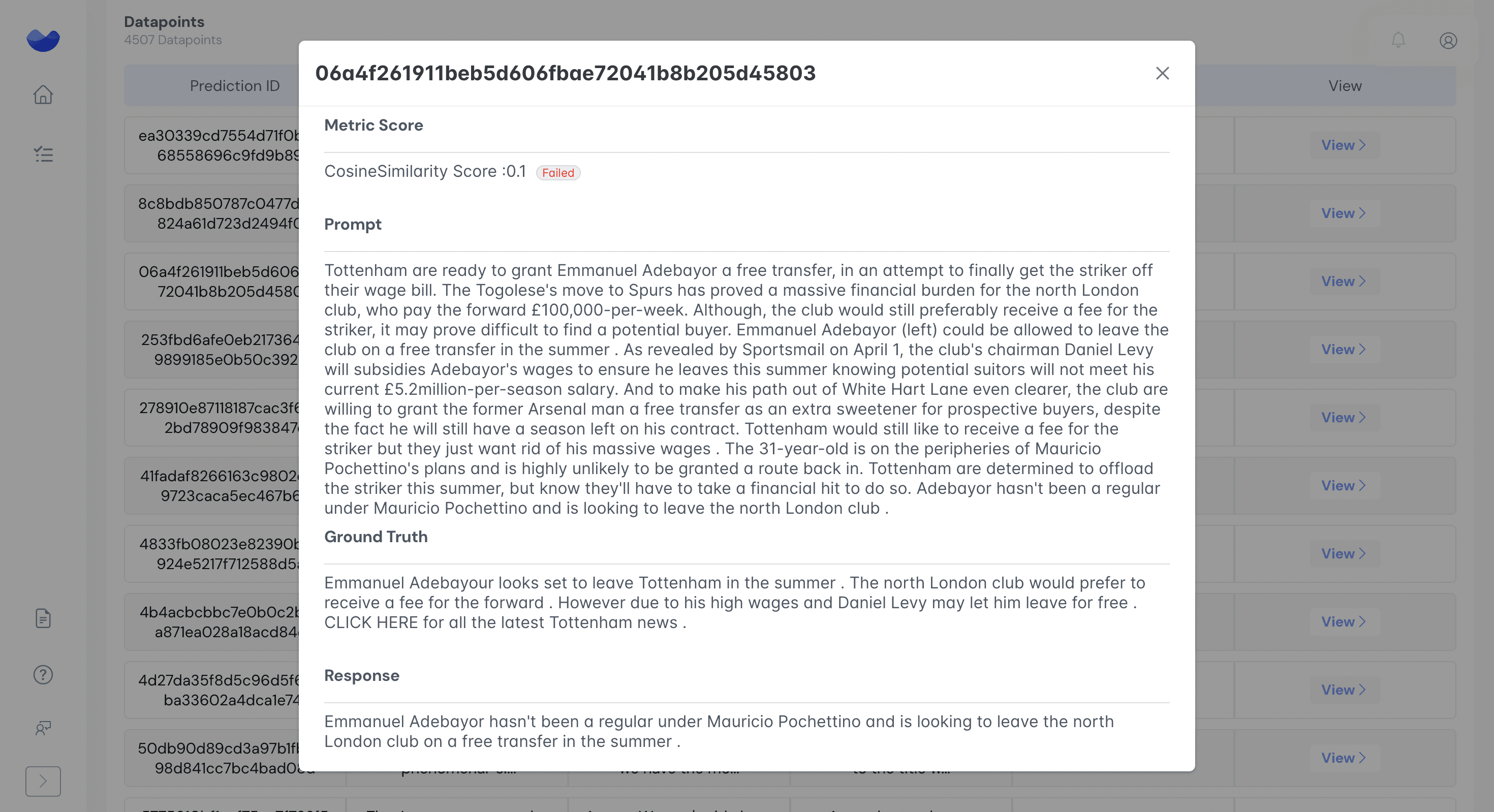
Evaluating and Monitoring an Enterprise LLM Application
Evaluating and Monitoring an Enterprise LLM Application
Read the article
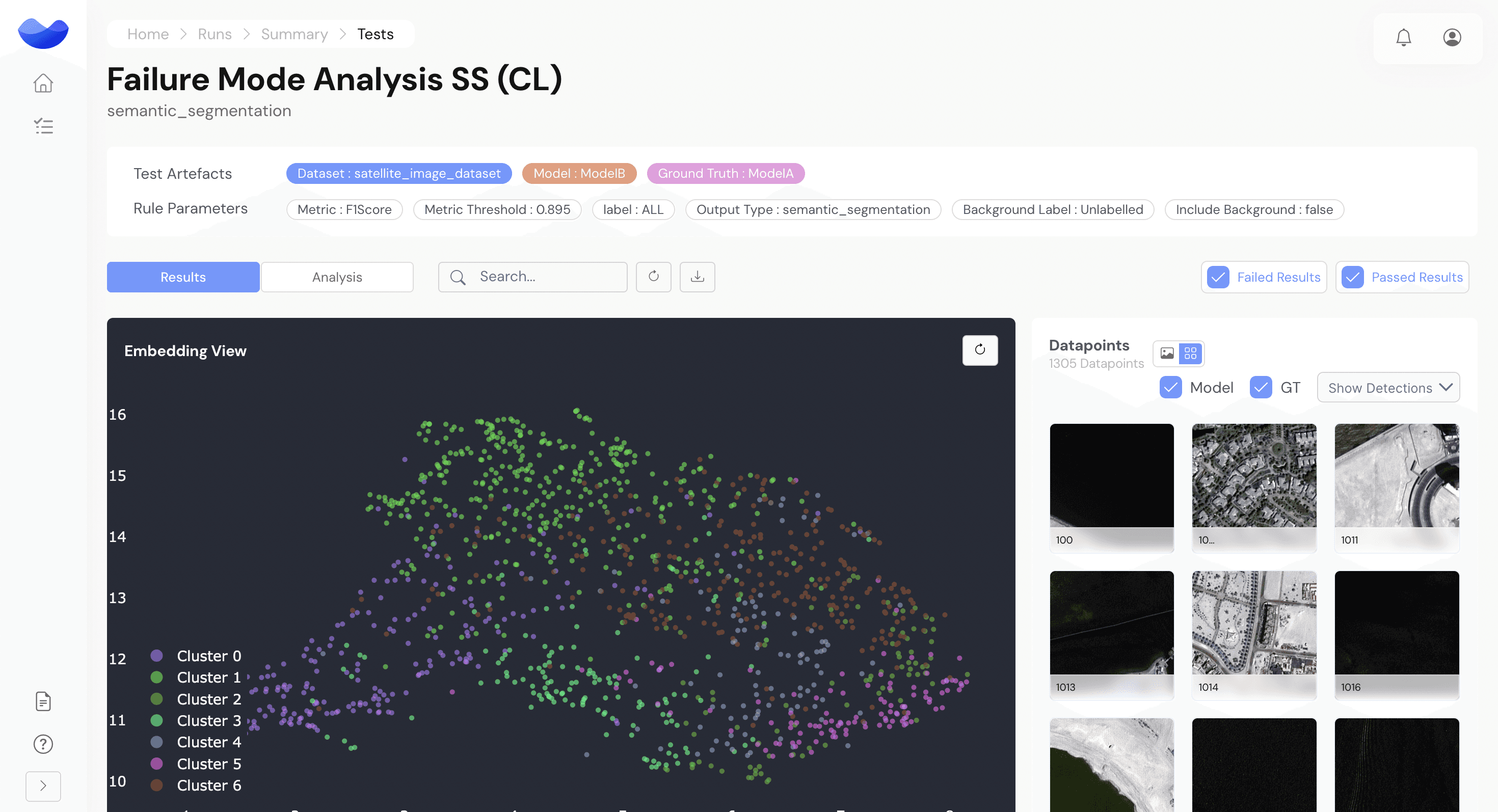
Advancing Geospatial Semantic Segmentation through Automated Testing with RagaAI
Advancing Geospatial Semantic Segmentation through Automated Testing with RagaAI
Read the article