Exploring The Frontiers Of Deep Learning Applications
Exploring The Frontiers Of Deep Learning Applications
Exploring The Frontiers Of Deep Learning Applications
Jigar Gupta
May 1, 2024
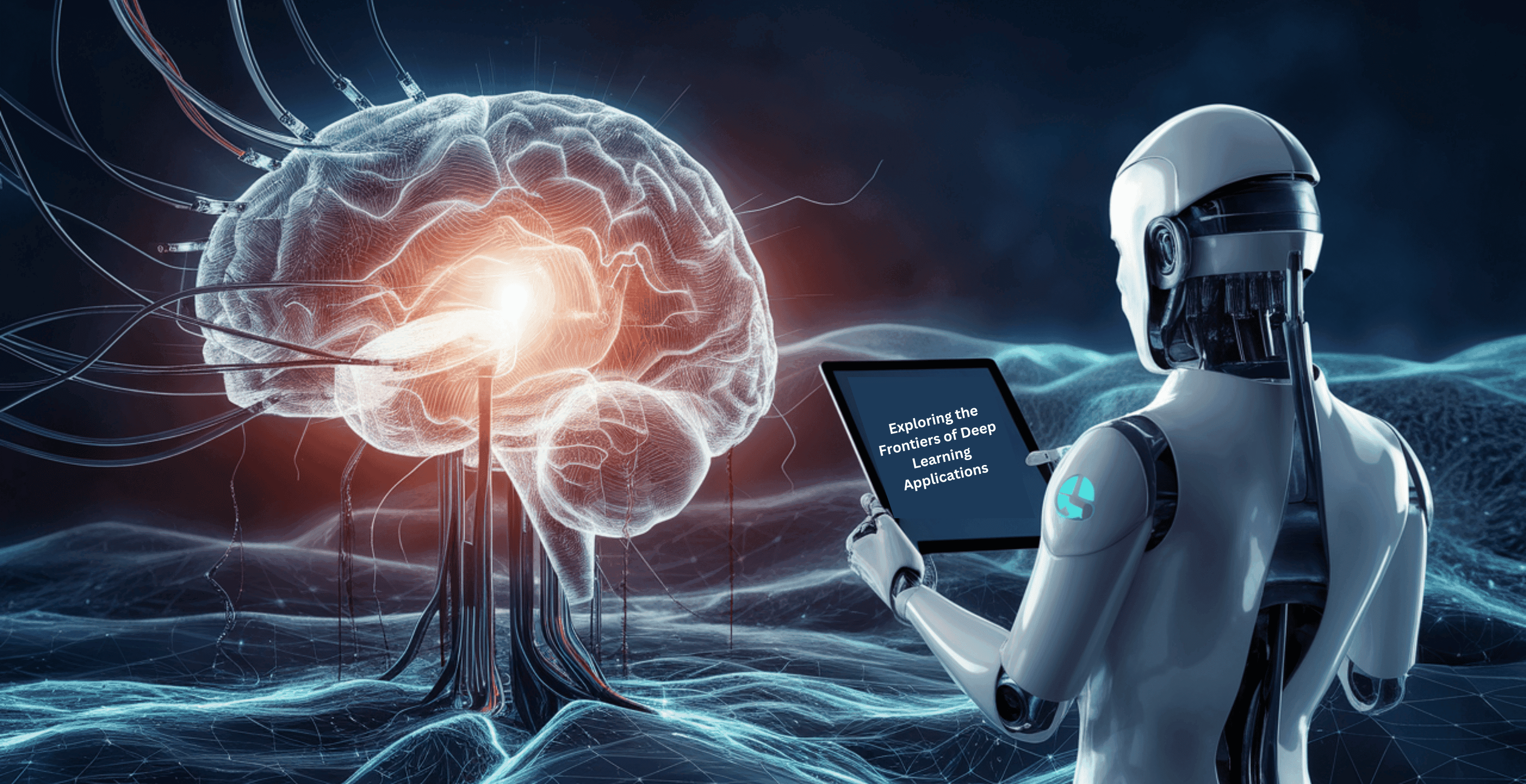
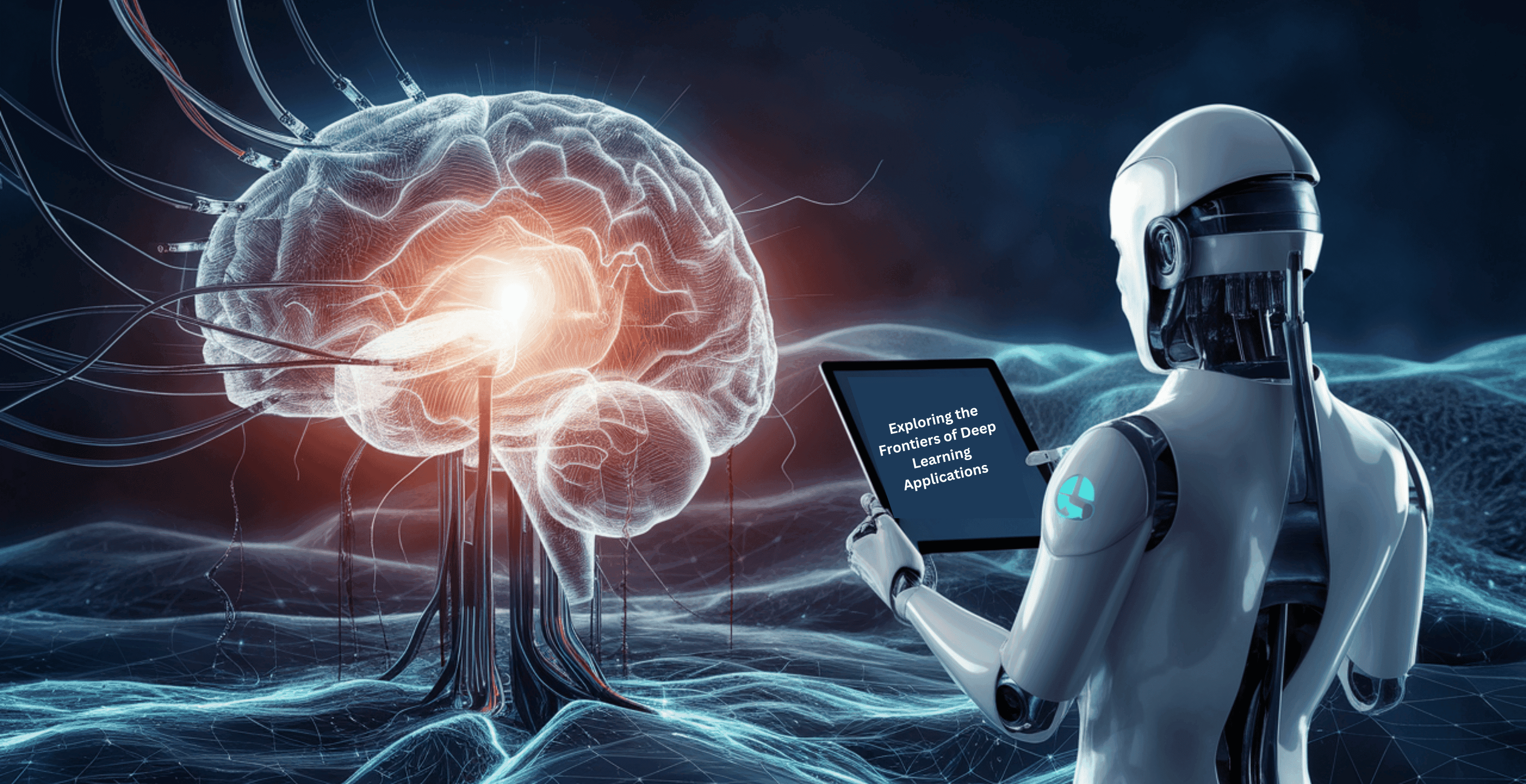
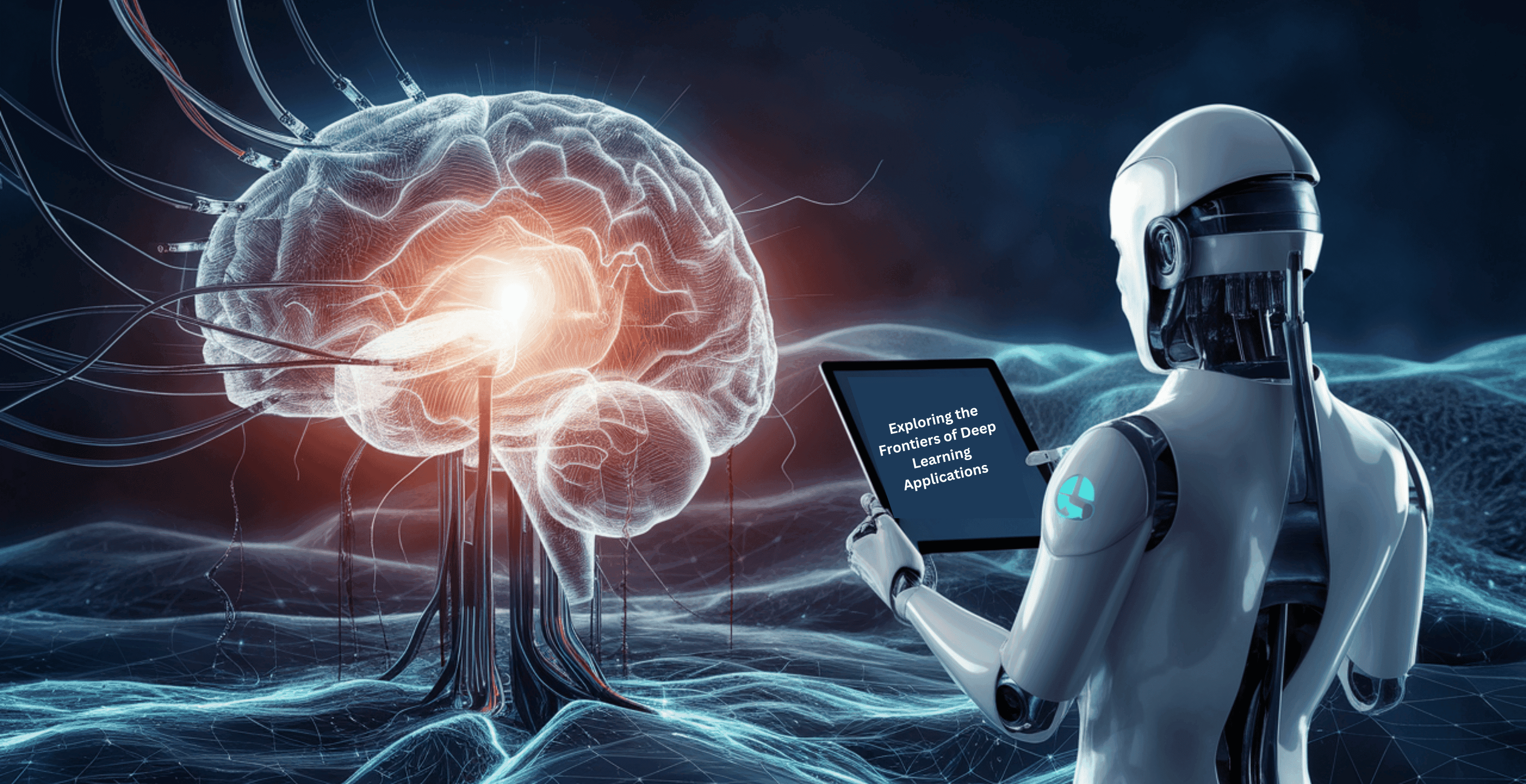
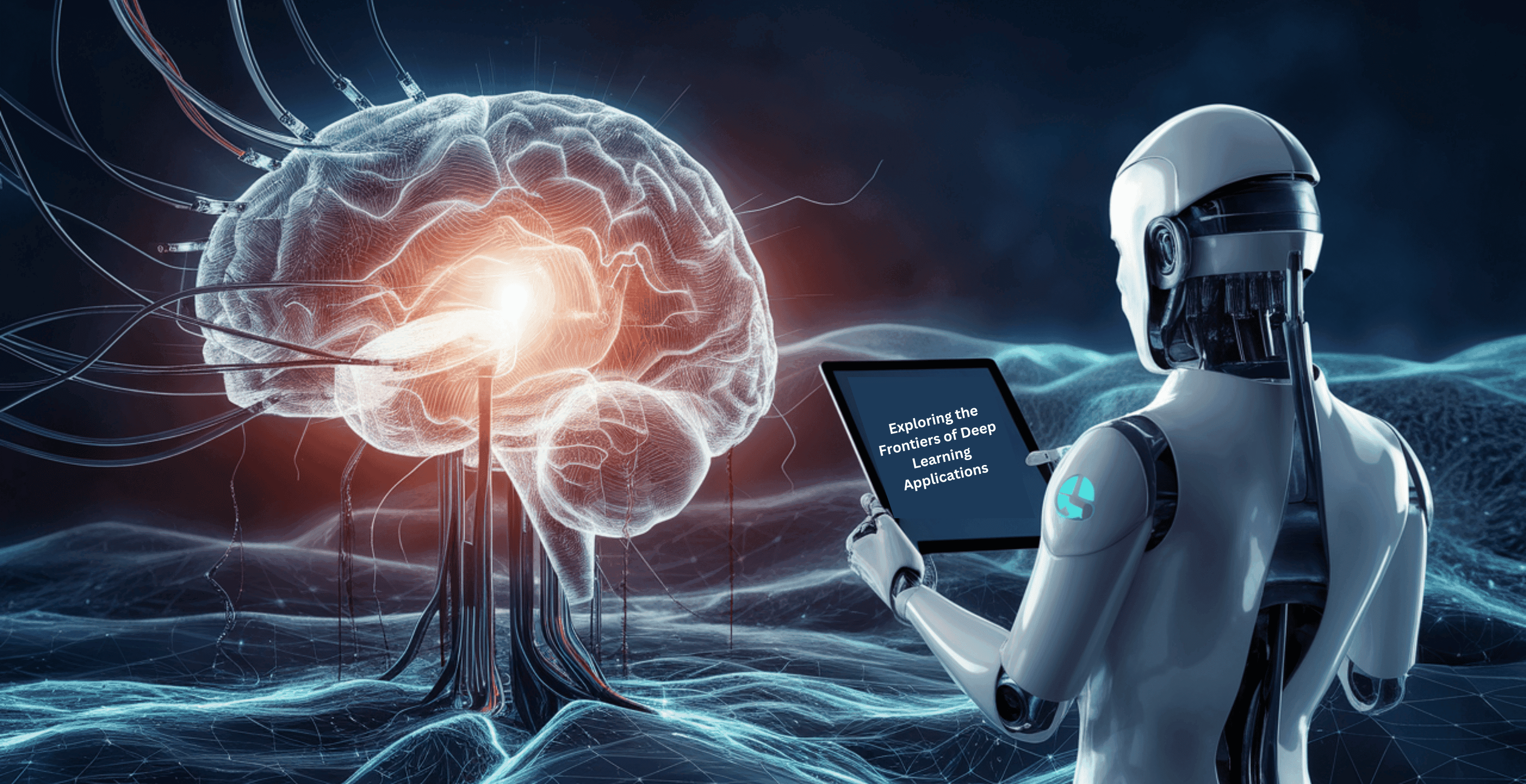
Deep learning is an advanced form of artificial intelligence that mimics the workings of the human brain in processing data and creating patterns for use in decision making. It is a key technology behind many innovative applications that enhance our daily lives and transform industries.
From speech recognition in virtual assistants to recommendation systems on streaming platforms, deep learning powers a range of technologies that enhance user experiences and increase automation. Its ability to learn from vast amounts of data allows it to make highly accurate predictions and automate complex tasks.
With a clear understanding of what deep learning is and where we encounter it in our daily lives, let's delve deeper into how deep learning powers these applications.
How Deep Learning Powers Applications?
The Structure of Deep Learning Neural Networks: Input Layer, Hidden Layers, and Output Layer
Deep learning models are structured in layers, each designed to recognize different aspects of data:
Input Layer: Takes raw data and prepares it for processing.
Hidden Layers: Perform computations and feature extractions. These layers are where most of the learning happens.
Output Layer: Produces the final output, making sense of the processed data.
Comparison with the Human Brain's Neural Networks
Much like neurons in the human brain, nodes in these layers are interconnected and transmit information. Each connection's strength can change as the system learns, similar to how our brains strengthen or weaken synaptic connections based on new experiences.
The Learning Process of Deep Learning Systems, Including Supervised and Unsupervised Learning
Deep learning systems can learn through:
Supervised Learning: Where models learn from labeled data, making it easier for them to predict outcomes based on past examples.
Unsupervised Learning: Where models identify patterns and relationships in data without any explicit instructions, adapting to the data’s inherent structures.
Now, to further clarify the distinction between deep learning and its broader category, let's compare it with general machine learning.
Deep Learning vs. Machine Learning
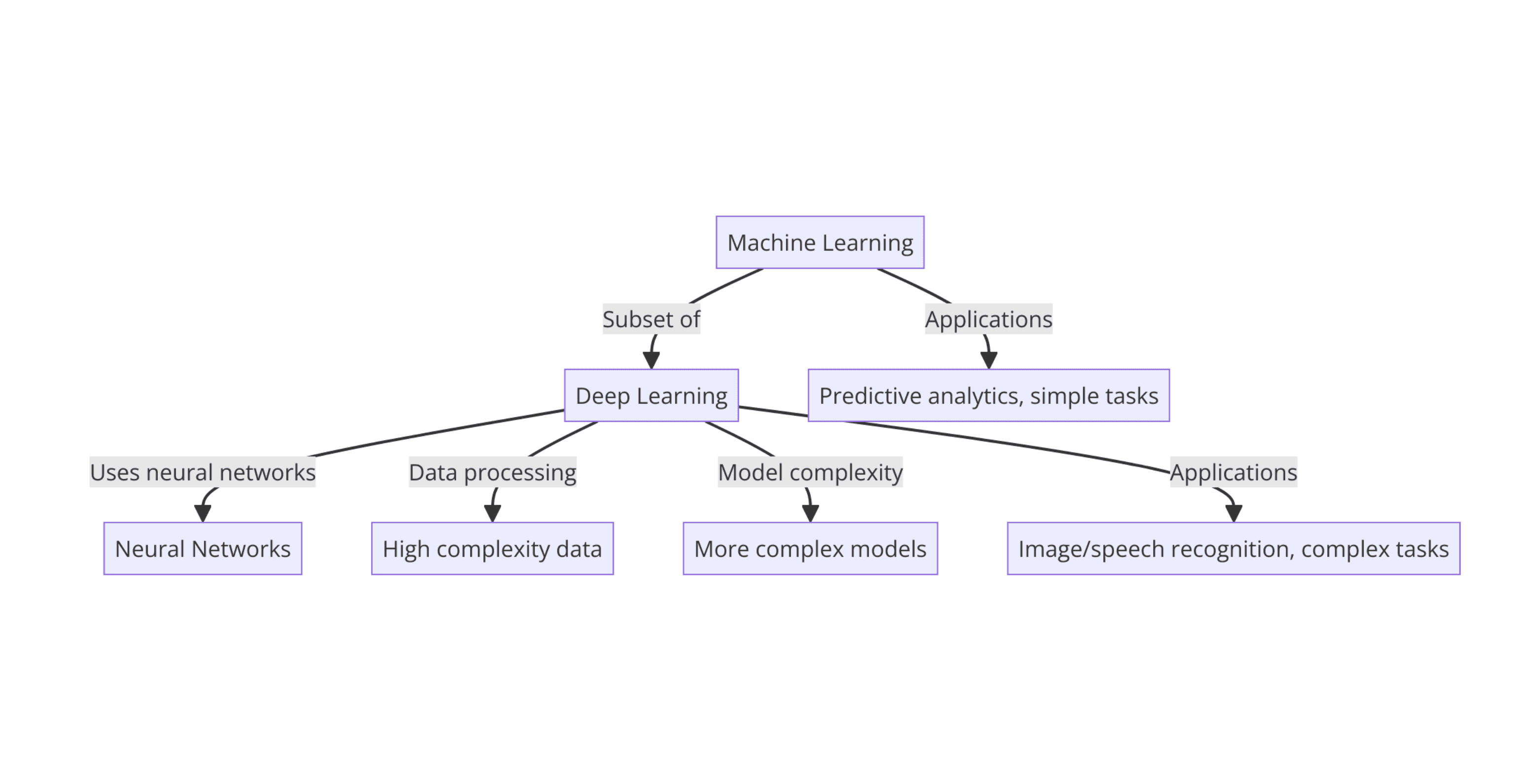
Deep Learning as a Subset of Machine Learning
Deep learning is a subset of machine learning that uses multi-layered neural networks to analyze various factors of data. It specializes in learning from data that is unstructured or unlabeled.
Data Processing: Deep learning is capable of processing and learning from data with much higher complexity than traditional machine learning.
Complexity: Deep learning models are typically more complex, capable of automatically discovering the representations needed for feature detection or classification from raw data.
Applications: While machine learning is often used for predictive analytics and simpler classification tasks, deep learning excels in areas where human-like cognition and complexity are required, such as image and speech recognition.
Having established how deep learning differs from and extends traditional machine learning, we'll next explore its broad applications across various industries, highlighting its impact on each sector.
Let's explore the broad applications of deep learning across various sectors, illustrating how this technology not only enhances current systems but also pioneers new innovations.
Broad Applications of Deep Learning
Automotive: Improving Vehicle Autonomy and Safety Features
In the automotive industry, deep learning is pivotal in developing advanced driver-assistance systems (ADAS) and autonomous vehicles. By analyzing real-time data from vehicle sensors and cameras, deep learning algorithms improve decision-making on the road, enhancing safety and driving efficiency.
Aerospace: Enhancing Navigation Systems and Flight Simulations
Deep learning algorithms are employed in aerospace for optimizing flight paths, improving navigation systems, and conducting sophisticated simulations that help in training pilots and testing aircraft under various conditions. These applications ensure higher safety and cost efficiency in aerospace operations.
Manufacturing: Optimizing Production Lines and Predictive Maintenance
In manufacturing, deep learning drives automation of complex production processes and predictive maintenance of equipment. By predicting failures before they occur and optimizing manufacturing workflows, deep learning significantly reduces downtime and increases productivity.
Electronics: Advancing Smart Devices and Personal Assistants
Deep learning transforms the electronics sector by enhancing the functionality of smart devices and personal assistants. It enables devices to understand and predict user preferences and respond to commands more accurately, making everyday interactions with technology smoother and more intuitive.
Medical Research: Revolutionizing Diagnostics and Patient Care
Perhaps one of the most impactful applications of deep learning is in medical research and healthcare, where it assists in diagnosing diseases with high accuracy through imaging data analysis and supports personalized medicine by adapting treatments to individual patient profiles.
Revolutionary Impact of Deep Learning Across Sectors
Computer Vision: Transforming Face Recognition and Image Processing
Deep learning has dramatically advanced computer vision technologies, enabling applications from security enhancements through facial recognition to new levels of interaction between computers and the visual world, such as real-time video analysis.
Speech Recognition: Elevating Voice Assistants and Natural Language Interfaces
Deep learning improves speech recognition systems, allowing for more accurate and natural interactions with voice-activated assistants and making these technologies accessible in more languages and dialects.
Natural Language Processing (NLP): Innovating Translation Services and Content Analysis
In NLP, deep learning models handle complex language data and enable more accurate translations, sentiment analysis, and content personalization, significantly enhancing communication across language barriers.
Recommendation Engines: Personalizing Online Consumer Experiences
Online platforms use deep learning to power recommendation engines that predict user preferences with unprecedented accuracy. This personalization improves user engagement and satisfaction, driving sales and content consumption.
With deep learning creating waves across various sectors, it's crucial to acknowledge the challenges this technology faces and the advancements that address these issues.
Let's delve into the challenges associated with deep learning, and the recent advancements that aim to overcome these hurdles, paving the way for more efficient and widespread applications of this transformative technology.
Challenges and Advances in Deep Learning
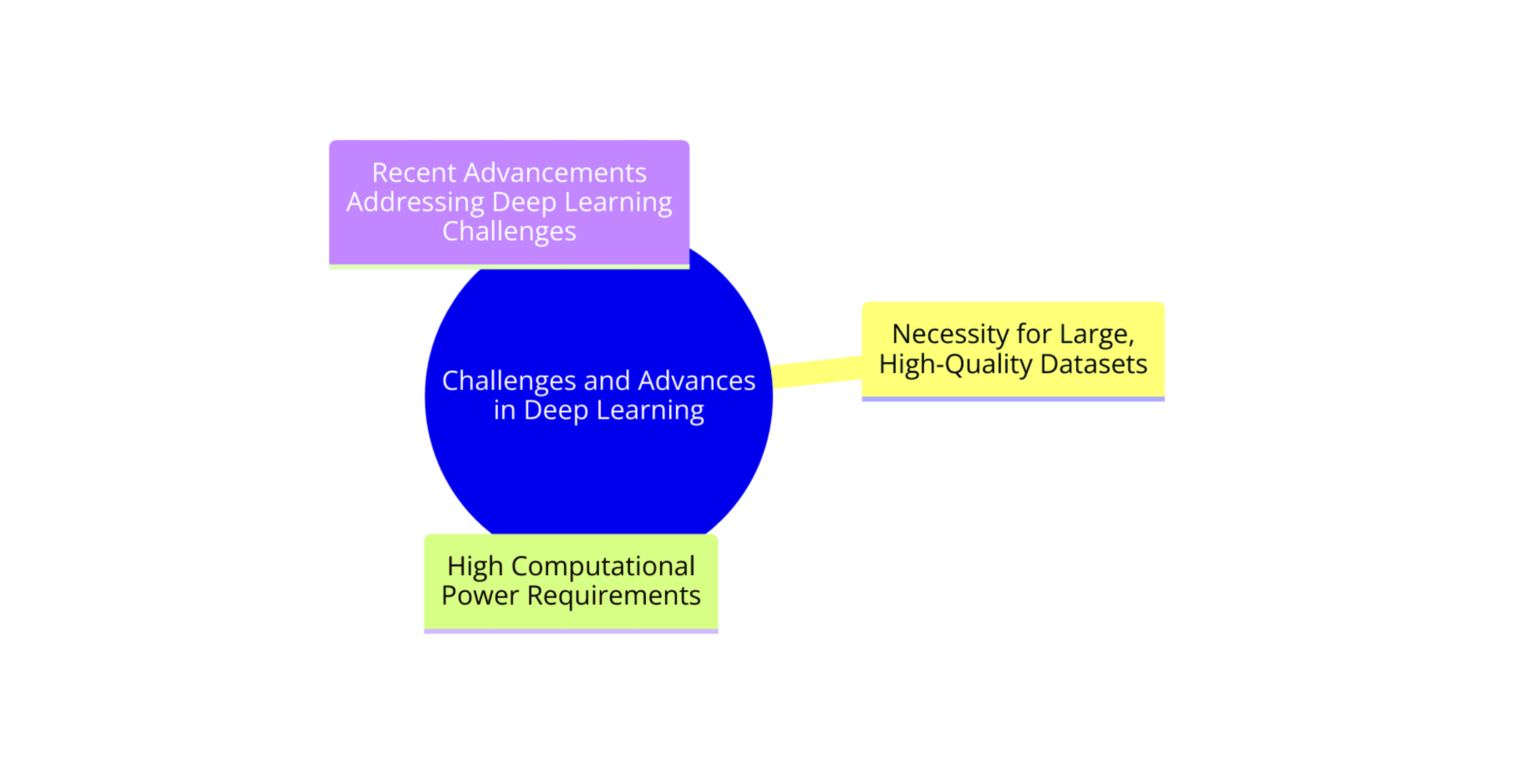
Necessity for Large, High-Quality Datasets
One of the primary challenges in deep learning is the necessity for vast amounts of high-quality data to train models effectively. Large datasets are crucial for achieving the accuracy and reliability expected from deep learning applications.
Solution: Advances in data synthesis and augmentation techniques allow for the expansion of dataset sizes without compromising quality. Additionally, transfer learning techniques enable models to learn from one domain and apply knowledge to another, reducing the total amount of data required.
High Computational Power Requirements
Deep learning models, particularly those involved in processing complex data like images and videos, require substantial computational resources, which can be cost-prohibitive.
Solution: Recent developments in hardware, such as GPUs and specialized processors like TPUs (Tensor Processing Units), have significantly reduced the computational load. Cloud-based solutions also offer scalable resources, making deep learning more accessible.
Recent Advancements Addressing Deep Learning Challenges
Technological advancements continue to address the inherent challenges of deep learning:
Efficient Network Architectures: Innovations like network pruning and the development of more efficient neural network architectures reduce the computational and memory overhead of training and deploying deep learning models.
Enhanced Learning Algorithms: Improvements in learning algorithms have enhanced the speed and efficiency of model training. Techniques like few-shot learning and reinforcement learning are breaking new ground in how models can be trained with less data and fewer resources.
Federated Learning: This technique allows for the training of algorithms across multiple decentralized devices or servers holding local data samples, without exchanging them. This method helps address privacy concerns, reduces the need for centralized data storage, and decreases bandwidth.
Future of Deep Learning
The Ongoing Evolution of Deep Learning Technologies
The field of deep learning is continuously evolving, with research pushing the boundaries of what's possible. This includes more sophisticated models that can handle complex tasks with greater accuracy and efficiency.
Potential Future Applications and the Impact on Various Industries
As deep learning technologies mature, their potential applications expand. Future developments could revolutionize sectors such as robotics, quantum computing, and even arts and creativity, significantly altering industry landscapes and operational protocols.
Innovations in Hardware and Algorithms Supporting Deep Learning
The hardware and algorithms that support deep learning are also advancing. Innovations in processor technology and algorithmic efficiency are making it feasible to deploy complex models on mobile devices and edge computing platforms, broadening the scope and accessibility of deep learning applications.
Learning Resources and Career Prospects
Information on Courses and Frameworks for Learning Deep Learning
For anyone looking to start or advance their knowledge in deep learning, numerous educational resources and frameworks are available to help:
Courses: Many online platforms offer specialized courses in deep learning. Coursera partners with top universities and companies to offer courses that cover both theoretical foundations and practical applications. Udacity and edX also provide comprehensive nanodegree programs and professional certifications.
Frameworks: TensorFlow, developed by Google, and PyTorch, developed by Facebook, are among the leading frameworks used for deep learning applications. These frameworks are supported by extensive documentation, tutorials, and active community forums that help new learners and seasoned professionals alike.
The Growing Demand for Professionals Skilled in Deep Learning
The demand for deep learning skills is surging across various industries including technology, healthcare, finance, and automotive.
This demand is driven by the need to extract more complex insights from data and automate tasks that traditionally require human intelligence.
Discussion on Career Paths and Salary Expectations in the Field
Careers in deep learning are both diverse and rewarding:
Data Scientist: Specializing in deep learning, focusing on developing algorithms that can learn from and make predictions on data.
AI Research Scientist: Working on the cutting edge of artificial intelligence, improving the techniques used to build deep learning models.
Machine Learning Engineer: Implementing models in practical applications, optimizing them for performance and scalability.
Salaries in these fields are highly competitive, reflecting both the demand for these skills and the value these professionals bring to businesses. In the U.S., salaries can range from $100,000 to over $150,000 annually, depending on experience, location, and specific industry.
FAQs on Deep Learning Applications
To round off our exploration, let's address some frequently asked questions about deep learning applications, which might help clarify common queries for those new to the field.
What are the most common applications of deep learning today?
Deep learning is commonly used in applications like image and speech recognition, autonomous vehicles, and personalized recommendation systems.
How does deep learning differ from traditional machine learning in application?
Deep learning typically requires more data and computational power but excels in handling very complex patterns and predictions, making it ideal for applications like natural language processing and computer vision.
Tips for Beginners Looking to Enter the Field of Deep Learning
Start Small: Begin with understanding the basics of neural networks and gradually move to more complex structures like convolutional and recurrent neural networks.
Hands-On Practice: Utilize open-source datasets to practice building and training models. Platforms like Kaggle provide a good starting point with competitions designed to challenge and enhance your skills.
Community and Networking: Engaging with the community through forums, local meetups, and conferences can provide invaluable learning opportunities and connections in the industry.
This comprehensive look into deep learning not only highlights its importance across various sectors but also provides a roadmap for those interested in diving into this exciting field. Whether you're just starting out or looking to refine your skills, the resources and career opportunities in deep learning are vast and growing.
Deep learning is an advanced form of artificial intelligence that mimics the workings of the human brain in processing data and creating patterns for use in decision making. It is a key technology behind many innovative applications that enhance our daily lives and transform industries.
From speech recognition in virtual assistants to recommendation systems on streaming platforms, deep learning powers a range of technologies that enhance user experiences and increase automation. Its ability to learn from vast amounts of data allows it to make highly accurate predictions and automate complex tasks.
With a clear understanding of what deep learning is and where we encounter it in our daily lives, let's delve deeper into how deep learning powers these applications.
How Deep Learning Powers Applications?
The Structure of Deep Learning Neural Networks: Input Layer, Hidden Layers, and Output Layer
Deep learning models are structured in layers, each designed to recognize different aspects of data:
Input Layer: Takes raw data and prepares it for processing.
Hidden Layers: Perform computations and feature extractions. These layers are where most of the learning happens.
Output Layer: Produces the final output, making sense of the processed data.
Comparison with the Human Brain's Neural Networks
Much like neurons in the human brain, nodes in these layers are interconnected and transmit information. Each connection's strength can change as the system learns, similar to how our brains strengthen or weaken synaptic connections based on new experiences.
The Learning Process of Deep Learning Systems, Including Supervised and Unsupervised Learning
Deep learning systems can learn through:
Supervised Learning: Where models learn from labeled data, making it easier for them to predict outcomes based on past examples.
Unsupervised Learning: Where models identify patterns and relationships in data without any explicit instructions, adapting to the data’s inherent structures.
Now, to further clarify the distinction between deep learning and its broader category, let's compare it with general machine learning.
Deep Learning vs. Machine Learning
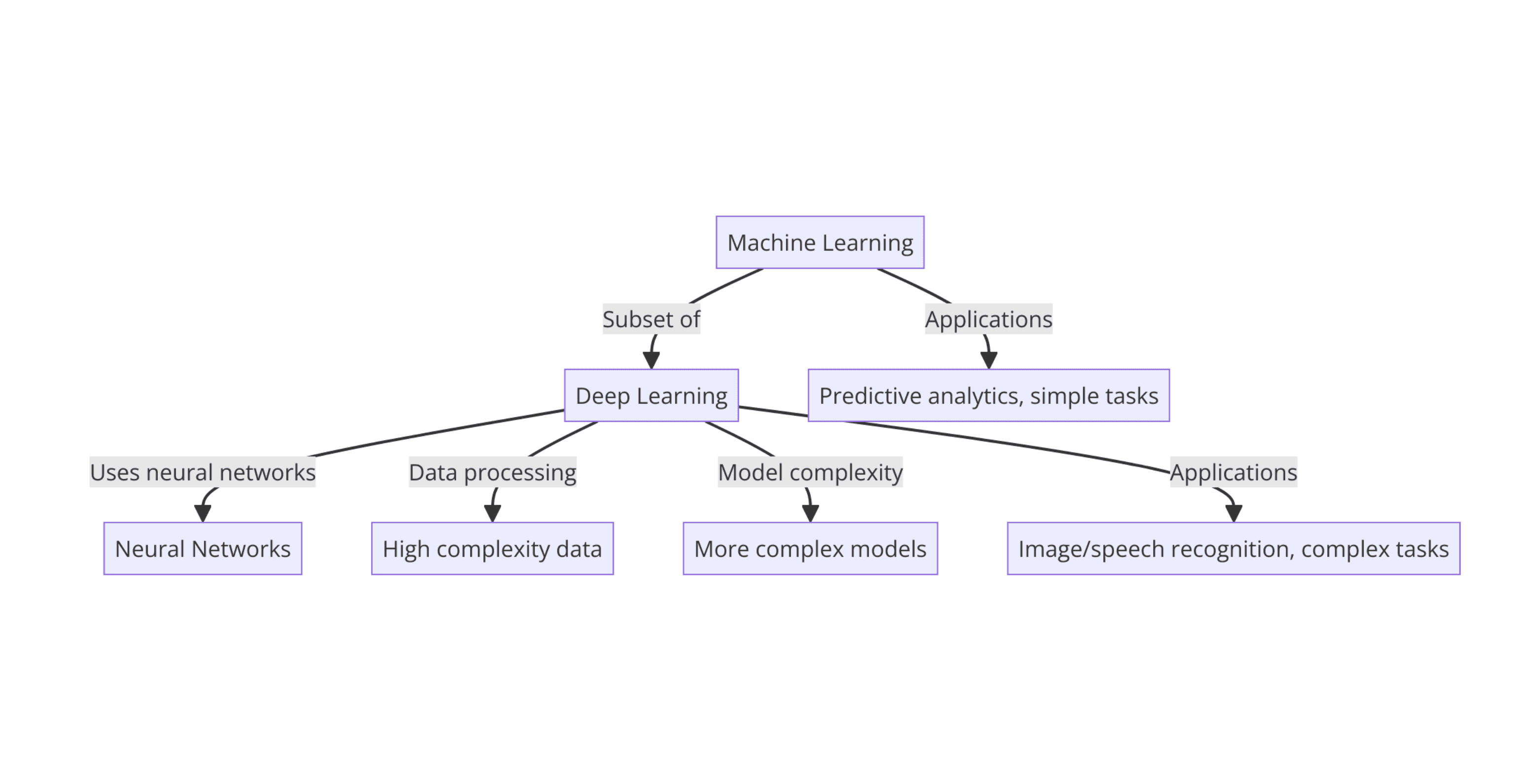
Deep Learning as a Subset of Machine Learning
Deep learning is a subset of machine learning that uses multi-layered neural networks to analyze various factors of data. It specializes in learning from data that is unstructured or unlabeled.
Data Processing: Deep learning is capable of processing and learning from data with much higher complexity than traditional machine learning.
Complexity: Deep learning models are typically more complex, capable of automatically discovering the representations needed for feature detection or classification from raw data.
Applications: While machine learning is often used for predictive analytics and simpler classification tasks, deep learning excels in areas where human-like cognition and complexity are required, such as image and speech recognition.
Having established how deep learning differs from and extends traditional machine learning, we'll next explore its broad applications across various industries, highlighting its impact on each sector.
Let's explore the broad applications of deep learning across various sectors, illustrating how this technology not only enhances current systems but also pioneers new innovations.
Broad Applications of Deep Learning
Automotive: Improving Vehicle Autonomy and Safety Features
In the automotive industry, deep learning is pivotal in developing advanced driver-assistance systems (ADAS) and autonomous vehicles. By analyzing real-time data from vehicle sensors and cameras, deep learning algorithms improve decision-making on the road, enhancing safety and driving efficiency.
Aerospace: Enhancing Navigation Systems and Flight Simulations
Deep learning algorithms are employed in aerospace for optimizing flight paths, improving navigation systems, and conducting sophisticated simulations that help in training pilots and testing aircraft under various conditions. These applications ensure higher safety and cost efficiency in aerospace operations.
Manufacturing: Optimizing Production Lines and Predictive Maintenance
In manufacturing, deep learning drives automation of complex production processes and predictive maintenance of equipment. By predicting failures before they occur and optimizing manufacturing workflows, deep learning significantly reduces downtime and increases productivity.
Electronics: Advancing Smart Devices and Personal Assistants
Deep learning transforms the electronics sector by enhancing the functionality of smart devices and personal assistants. It enables devices to understand and predict user preferences and respond to commands more accurately, making everyday interactions with technology smoother and more intuitive.
Medical Research: Revolutionizing Diagnostics and Patient Care
Perhaps one of the most impactful applications of deep learning is in medical research and healthcare, where it assists in diagnosing diseases with high accuracy through imaging data analysis and supports personalized medicine by adapting treatments to individual patient profiles.
Revolutionary Impact of Deep Learning Across Sectors
Computer Vision: Transforming Face Recognition and Image Processing
Deep learning has dramatically advanced computer vision technologies, enabling applications from security enhancements through facial recognition to new levels of interaction between computers and the visual world, such as real-time video analysis.
Speech Recognition: Elevating Voice Assistants and Natural Language Interfaces
Deep learning improves speech recognition systems, allowing for more accurate and natural interactions with voice-activated assistants and making these technologies accessible in more languages and dialects.
Natural Language Processing (NLP): Innovating Translation Services and Content Analysis
In NLP, deep learning models handle complex language data and enable more accurate translations, sentiment analysis, and content personalization, significantly enhancing communication across language barriers.
Recommendation Engines: Personalizing Online Consumer Experiences
Online platforms use deep learning to power recommendation engines that predict user preferences with unprecedented accuracy. This personalization improves user engagement and satisfaction, driving sales and content consumption.
With deep learning creating waves across various sectors, it's crucial to acknowledge the challenges this technology faces and the advancements that address these issues.
Let's delve into the challenges associated with deep learning, and the recent advancements that aim to overcome these hurdles, paving the way for more efficient and widespread applications of this transformative technology.
Challenges and Advances in Deep Learning
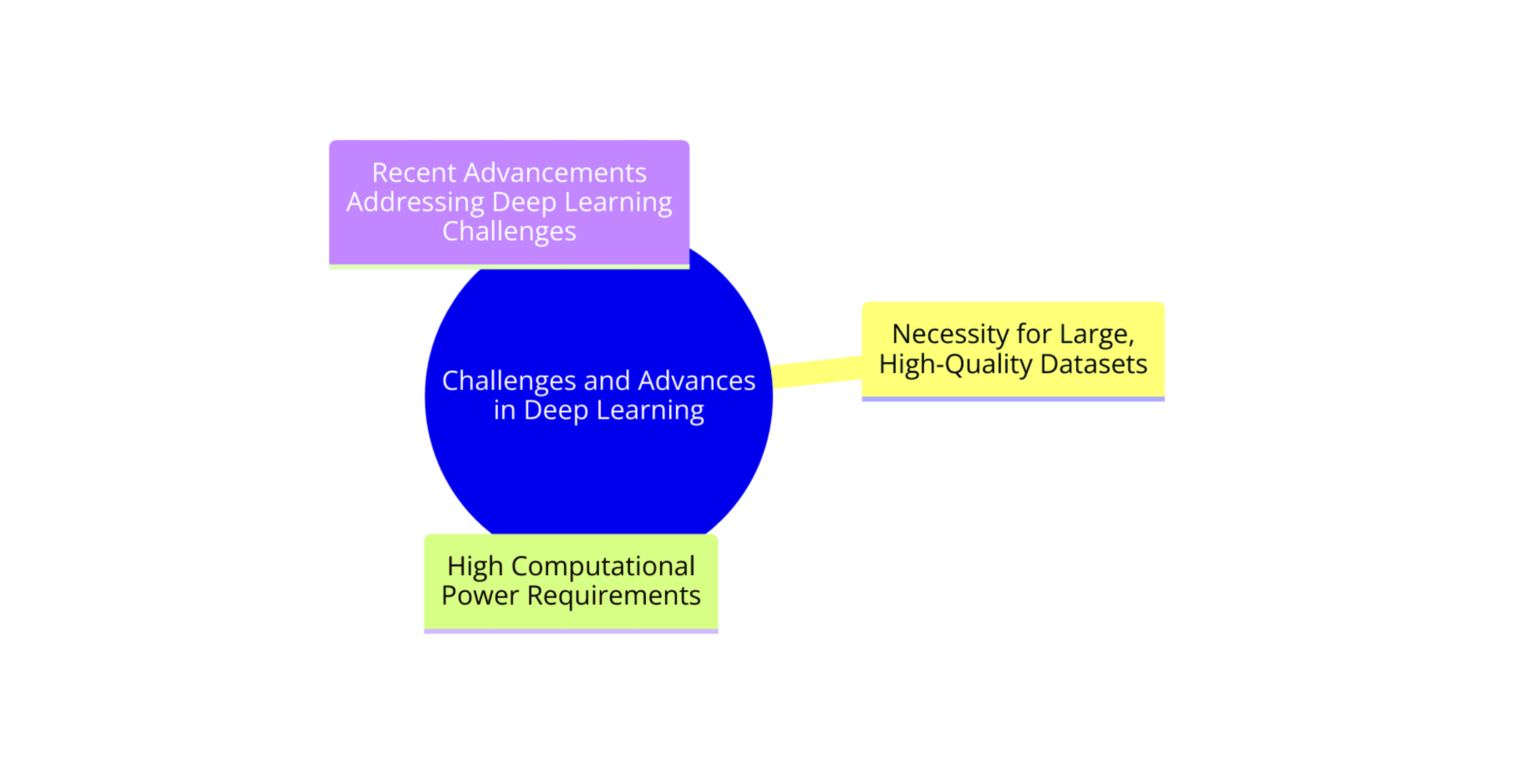
Necessity for Large, High-Quality Datasets
One of the primary challenges in deep learning is the necessity for vast amounts of high-quality data to train models effectively. Large datasets are crucial for achieving the accuracy and reliability expected from deep learning applications.
Solution: Advances in data synthesis and augmentation techniques allow for the expansion of dataset sizes without compromising quality. Additionally, transfer learning techniques enable models to learn from one domain and apply knowledge to another, reducing the total amount of data required.
High Computational Power Requirements
Deep learning models, particularly those involved in processing complex data like images and videos, require substantial computational resources, which can be cost-prohibitive.
Solution: Recent developments in hardware, such as GPUs and specialized processors like TPUs (Tensor Processing Units), have significantly reduced the computational load. Cloud-based solutions also offer scalable resources, making deep learning more accessible.
Recent Advancements Addressing Deep Learning Challenges
Technological advancements continue to address the inherent challenges of deep learning:
Efficient Network Architectures: Innovations like network pruning and the development of more efficient neural network architectures reduce the computational and memory overhead of training and deploying deep learning models.
Enhanced Learning Algorithms: Improvements in learning algorithms have enhanced the speed and efficiency of model training. Techniques like few-shot learning and reinforcement learning are breaking new ground in how models can be trained with less data and fewer resources.
Federated Learning: This technique allows for the training of algorithms across multiple decentralized devices or servers holding local data samples, without exchanging them. This method helps address privacy concerns, reduces the need for centralized data storage, and decreases bandwidth.
Future of Deep Learning
The Ongoing Evolution of Deep Learning Technologies
The field of deep learning is continuously evolving, with research pushing the boundaries of what's possible. This includes more sophisticated models that can handle complex tasks with greater accuracy and efficiency.
Potential Future Applications and the Impact on Various Industries
As deep learning technologies mature, their potential applications expand. Future developments could revolutionize sectors such as robotics, quantum computing, and even arts and creativity, significantly altering industry landscapes and operational protocols.
Innovations in Hardware and Algorithms Supporting Deep Learning
The hardware and algorithms that support deep learning are also advancing. Innovations in processor technology and algorithmic efficiency are making it feasible to deploy complex models on mobile devices and edge computing platforms, broadening the scope and accessibility of deep learning applications.
Learning Resources and Career Prospects
Information on Courses and Frameworks for Learning Deep Learning
For anyone looking to start or advance their knowledge in deep learning, numerous educational resources and frameworks are available to help:
Courses: Many online platforms offer specialized courses in deep learning. Coursera partners with top universities and companies to offer courses that cover both theoretical foundations and practical applications. Udacity and edX also provide comprehensive nanodegree programs and professional certifications.
Frameworks: TensorFlow, developed by Google, and PyTorch, developed by Facebook, are among the leading frameworks used for deep learning applications. These frameworks are supported by extensive documentation, tutorials, and active community forums that help new learners and seasoned professionals alike.
The Growing Demand for Professionals Skilled in Deep Learning
The demand for deep learning skills is surging across various industries including technology, healthcare, finance, and automotive.
This demand is driven by the need to extract more complex insights from data and automate tasks that traditionally require human intelligence.
Discussion on Career Paths and Salary Expectations in the Field
Careers in deep learning are both diverse and rewarding:
Data Scientist: Specializing in deep learning, focusing on developing algorithms that can learn from and make predictions on data.
AI Research Scientist: Working on the cutting edge of artificial intelligence, improving the techniques used to build deep learning models.
Machine Learning Engineer: Implementing models in practical applications, optimizing them for performance and scalability.
Salaries in these fields are highly competitive, reflecting both the demand for these skills and the value these professionals bring to businesses. In the U.S., salaries can range from $100,000 to over $150,000 annually, depending on experience, location, and specific industry.
FAQs on Deep Learning Applications
To round off our exploration, let's address some frequently asked questions about deep learning applications, which might help clarify common queries for those new to the field.
What are the most common applications of deep learning today?
Deep learning is commonly used in applications like image and speech recognition, autonomous vehicles, and personalized recommendation systems.
How does deep learning differ from traditional machine learning in application?
Deep learning typically requires more data and computational power but excels in handling very complex patterns and predictions, making it ideal for applications like natural language processing and computer vision.
Tips for Beginners Looking to Enter the Field of Deep Learning
Start Small: Begin with understanding the basics of neural networks and gradually move to more complex structures like convolutional and recurrent neural networks.
Hands-On Practice: Utilize open-source datasets to practice building and training models. Platforms like Kaggle provide a good starting point with competitions designed to challenge and enhance your skills.
Community and Networking: Engaging with the community through forums, local meetups, and conferences can provide invaluable learning opportunities and connections in the industry.
This comprehensive look into deep learning not only highlights its importance across various sectors but also provides a roadmap for those interested in diving into this exciting field. Whether you're just starting out or looking to refine your skills, the resources and career opportunities in deep learning are vast and growing.
Deep learning is an advanced form of artificial intelligence that mimics the workings of the human brain in processing data and creating patterns for use in decision making. It is a key technology behind many innovative applications that enhance our daily lives and transform industries.
From speech recognition in virtual assistants to recommendation systems on streaming platforms, deep learning powers a range of technologies that enhance user experiences and increase automation. Its ability to learn from vast amounts of data allows it to make highly accurate predictions and automate complex tasks.
With a clear understanding of what deep learning is and where we encounter it in our daily lives, let's delve deeper into how deep learning powers these applications.
How Deep Learning Powers Applications?
The Structure of Deep Learning Neural Networks: Input Layer, Hidden Layers, and Output Layer
Deep learning models are structured in layers, each designed to recognize different aspects of data:
Input Layer: Takes raw data and prepares it for processing.
Hidden Layers: Perform computations and feature extractions. These layers are where most of the learning happens.
Output Layer: Produces the final output, making sense of the processed data.
Comparison with the Human Brain's Neural Networks
Much like neurons in the human brain, nodes in these layers are interconnected and transmit information. Each connection's strength can change as the system learns, similar to how our brains strengthen or weaken synaptic connections based on new experiences.
The Learning Process of Deep Learning Systems, Including Supervised and Unsupervised Learning
Deep learning systems can learn through:
Supervised Learning: Where models learn from labeled data, making it easier for them to predict outcomes based on past examples.
Unsupervised Learning: Where models identify patterns and relationships in data without any explicit instructions, adapting to the data’s inherent structures.
Now, to further clarify the distinction between deep learning and its broader category, let's compare it with general machine learning.
Deep Learning vs. Machine Learning
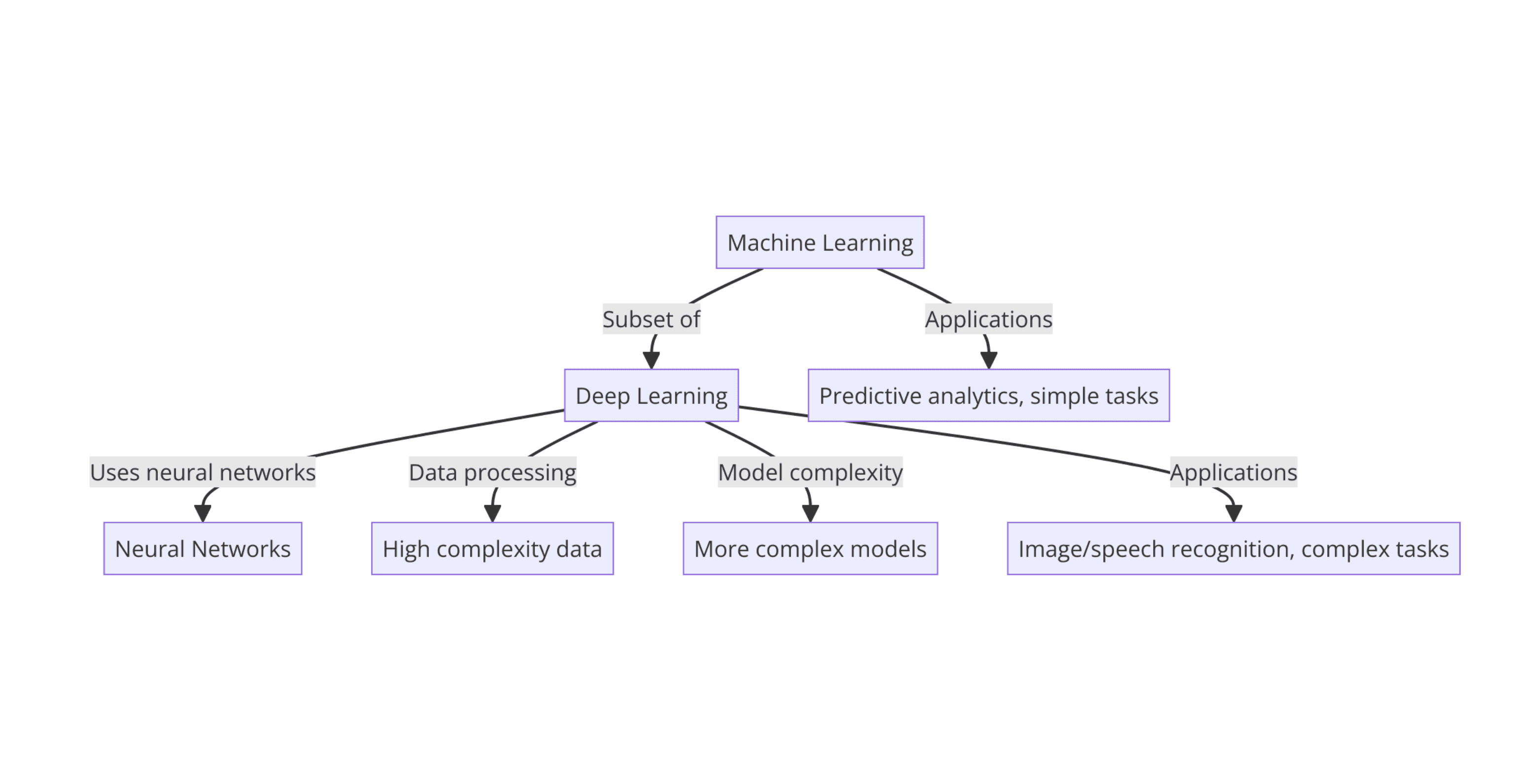
Deep Learning as a Subset of Machine Learning
Deep learning is a subset of machine learning that uses multi-layered neural networks to analyze various factors of data. It specializes in learning from data that is unstructured or unlabeled.
Data Processing: Deep learning is capable of processing and learning from data with much higher complexity than traditional machine learning.
Complexity: Deep learning models are typically more complex, capable of automatically discovering the representations needed for feature detection or classification from raw data.
Applications: While machine learning is often used for predictive analytics and simpler classification tasks, deep learning excels in areas where human-like cognition and complexity are required, such as image and speech recognition.
Having established how deep learning differs from and extends traditional machine learning, we'll next explore its broad applications across various industries, highlighting its impact on each sector.
Let's explore the broad applications of deep learning across various sectors, illustrating how this technology not only enhances current systems but also pioneers new innovations.
Broad Applications of Deep Learning
Automotive: Improving Vehicle Autonomy and Safety Features
In the automotive industry, deep learning is pivotal in developing advanced driver-assistance systems (ADAS) and autonomous vehicles. By analyzing real-time data from vehicle sensors and cameras, deep learning algorithms improve decision-making on the road, enhancing safety and driving efficiency.
Aerospace: Enhancing Navigation Systems and Flight Simulations
Deep learning algorithms are employed in aerospace for optimizing flight paths, improving navigation systems, and conducting sophisticated simulations that help in training pilots and testing aircraft under various conditions. These applications ensure higher safety and cost efficiency in aerospace operations.
Manufacturing: Optimizing Production Lines and Predictive Maintenance
In manufacturing, deep learning drives automation of complex production processes and predictive maintenance of equipment. By predicting failures before they occur and optimizing manufacturing workflows, deep learning significantly reduces downtime and increases productivity.
Electronics: Advancing Smart Devices and Personal Assistants
Deep learning transforms the electronics sector by enhancing the functionality of smart devices and personal assistants. It enables devices to understand and predict user preferences and respond to commands more accurately, making everyday interactions with technology smoother and more intuitive.
Medical Research: Revolutionizing Diagnostics and Patient Care
Perhaps one of the most impactful applications of deep learning is in medical research and healthcare, where it assists in diagnosing diseases with high accuracy through imaging data analysis and supports personalized medicine by adapting treatments to individual patient profiles.
Revolutionary Impact of Deep Learning Across Sectors
Computer Vision: Transforming Face Recognition and Image Processing
Deep learning has dramatically advanced computer vision technologies, enabling applications from security enhancements through facial recognition to new levels of interaction between computers and the visual world, such as real-time video analysis.
Speech Recognition: Elevating Voice Assistants and Natural Language Interfaces
Deep learning improves speech recognition systems, allowing for more accurate and natural interactions with voice-activated assistants and making these technologies accessible in more languages and dialects.
Natural Language Processing (NLP): Innovating Translation Services and Content Analysis
In NLP, deep learning models handle complex language data and enable more accurate translations, sentiment analysis, and content personalization, significantly enhancing communication across language barriers.
Recommendation Engines: Personalizing Online Consumer Experiences
Online platforms use deep learning to power recommendation engines that predict user preferences with unprecedented accuracy. This personalization improves user engagement and satisfaction, driving sales and content consumption.
With deep learning creating waves across various sectors, it's crucial to acknowledge the challenges this technology faces and the advancements that address these issues.
Let's delve into the challenges associated with deep learning, and the recent advancements that aim to overcome these hurdles, paving the way for more efficient and widespread applications of this transformative technology.
Challenges and Advances in Deep Learning
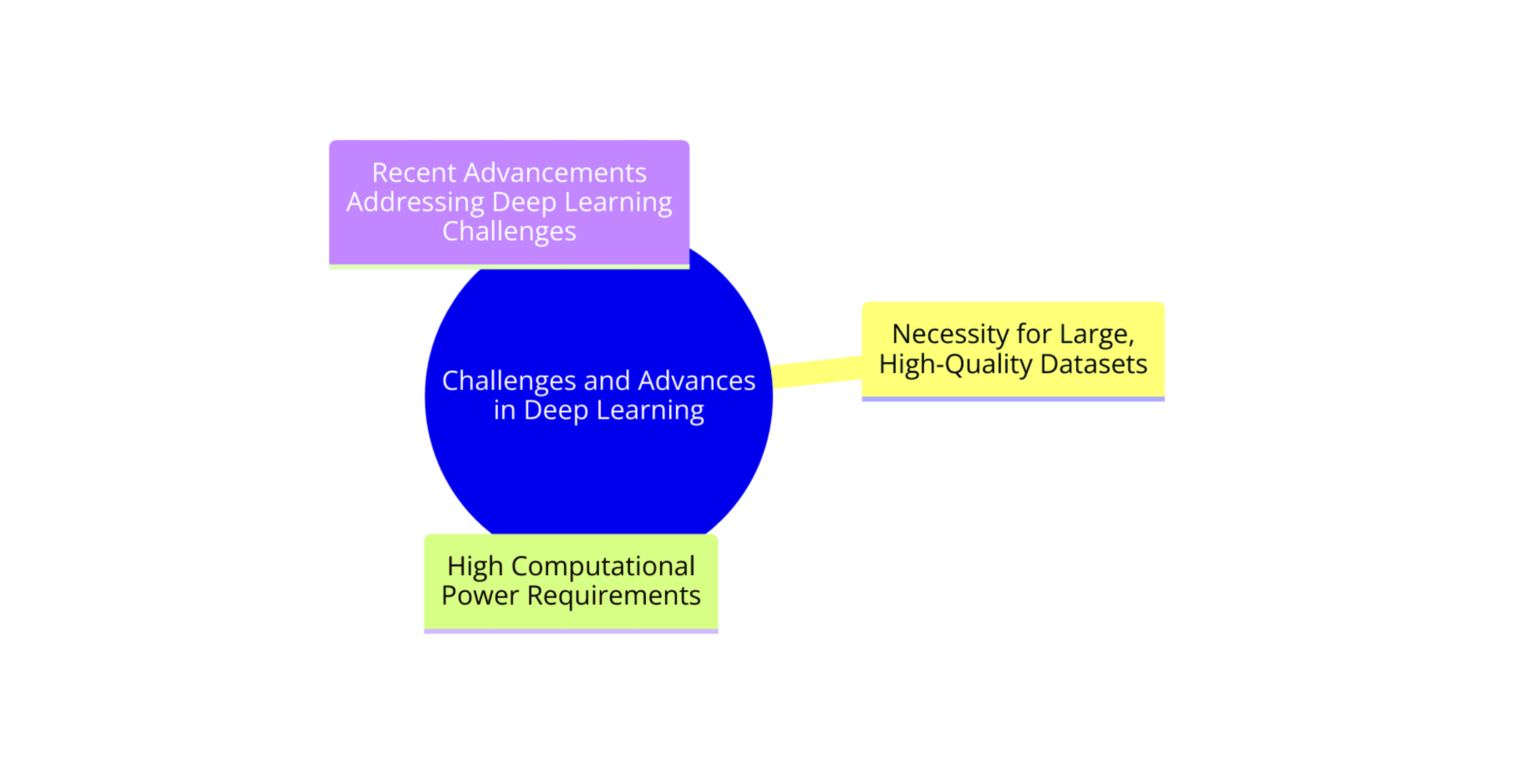
Necessity for Large, High-Quality Datasets
One of the primary challenges in deep learning is the necessity for vast amounts of high-quality data to train models effectively. Large datasets are crucial for achieving the accuracy and reliability expected from deep learning applications.
Solution: Advances in data synthesis and augmentation techniques allow for the expansion of dataset sizes without compromising quality. Additionally, transfer learning techniques enable models to learn from one domain and apply knowledge to another, reducing the total amount of data required.
High Computational Power Requirements
Deep learning models, particularly those involved in processing complex data like images and videos, require substantial computational resources, which can be cost-prohibitive.
Solution: Recent developments in hardware, such as GPUs and specialized processors like TPUs (Tensor Processing Units), have significantly reduced the computational load. Cloud-based solutions also offer scalable resources, making deep learning more accessible.
Recent Advancements Addressing Deep Learning Challenges
Technological advancements continue to address the inherent challenges of deep learning:
Efficient Network Architectures: Innovations like network pruning and the development of more efficient neural network architectures reduce the computational and memory overhead of training and deploying deep learning models.
Enhanced Learning Algorithms: Improvements in learning algorithms have enhanced the speed and efficiency of model training. Techniques like few-shot learning and reinforcement learning are breaking new ground in how models can be trained with less data and fewer resources.
Federated Learning: This technique allows for the training of algorithms across multiple decentralized devices or servers holding local data samples, without exchanging them. This method helps address privacy concerns, reduces the need for centralized data storage, and decreases bandwidth.
Future of Deep Learning
The Ongoing Evolution of Deep Learning Technologies
The field of deep learning is continuously evolving, with research pushing the boundaries of what's possible. This includes more sophisticated models that can handle complex tasks with greater accuracy and efficiency.
Potential Future Applications and the Impact on Various Industries
As deep learning technologies mature, their potential applications expand. Future developments could revolutionize sectors such as robotics, quantum computing, and even arts and creativity, significantly altering industry landscapes and operational protocols.
Innovations in Hardware and Algorithms Supporting Deep Learning
The hardware and algorithms that support deep learning are also advancing. Innovations in processor technology and algorithmic efficiency are making it feasible to deploy complex models on mobile devices and edge computing platforms, broadening the scope and accessibility of deep learning applications.
Learning Resources and Career Prospects
Information on Courses and Frameworks for Learning Deep Learning
For anyone looking to start or advance their knowledge in deep learning, numerous educational resources and frameworks are available to help:
Courses: Many online platforms offer specialized courses in deep learning. Coursera partners with top universities and companies to offer courses that cover both theoretical foundations and practical applications. Udacity and edX also provide comprehensive nanodegree programs and professional certifications.
Frameworks: TensorFlow, developed by Google, and PyTorch, developed by Facebook, are among the leading frameworks used for deep learning applications. These frameworks are supported by extensive documentation, tutorials, and active community forums that help new learners and seasoned professionals alike.
The Growing Demand for Professionals Skilled in Deep Learning
The demand for deep learning skills is surging across various industries including technology, healthcare, finance, and automotive.
This demand is driven by the need to extract more complex insights from data and automate tasks that traditionally require human intelligence.
Discussion on Career Paths and Salary Expectations in the Field
Careers in deep learning are both diverse and rewarding:
Data Scientist: Specializing in deep learning, focusing on developing algorithms that can learn from and make predictions on data.
AI Research Scientist: Working on the cutting edge of artificial intelligence, improving the techniques used to build deep learning models.
Machine Learning Engineer: Implementing models in practical applications, optimizing them for performance and scalability.
Salaries in these fields are highly competitive, reflecting both the demand for these skills and the value these professionals bring to businesses. In the U.S., salaries can range from $100,000 to over $150,000 annually, depending on experience, location, and specific industry.
FAQs on Deep Learning Applications
To round off our exploration, let's address some frequently asked questions about deep learning applications, which might help clarify common queries for those new to the field.
What are the most common applications of deep learning today?
Deep learning is commonly used in applications like image and speech recognition, autonomous vehicles, and personalized recommendation systems.
How does deep learning differ from traditional machine learning in application?
Deep learning typically requires more data and computational power but excels in handling very complex patterns and predictions, making it ideal for applications like natural language processing and computer vision.
Tips for Beginners Looking to Enter the Field of Deep Learning
Start Small: Begin with understanding the basics of neural networks and gradually move to more complex structures like convolutional and recurrent neural networks.
Hands-On Practice: Utilize open-source datasets to practice building and training models. Platforms like Kaggle provide a good starting point with competitions designed to challenge and enhance your skills.
Community and Networking: Engaging with the community through forums, local meetups, and conferences can provide invaluable learning opportunities and connections in the industry.
This comprehensive look into deep learning not only highlights its importance across various sectors but also provides a roadmap for those interested in diving into this exciting field. Whether you're just starting out or looking to refine your skills, the resources and career opportunities in deep learning are vast and growing.
Deep learning is an advanced form of artificial intelligence that mimics the workings of the human brain in processing data and creating patterns for use in decision making. It is a key technology behind many innovative applications that enhance our daily lives and transform industries.
From speech recognition in virtual assistants to recommendation systems on streaming platforms, deep learning powers a range of technologies that enhance user experiences and increase automation. Its ability to learn from vast amounts of data allows it to make highly accurate predictions and automate complex tasks.
With a clear understanding of what deep learning is and where we encounter it in our daily lives, let's delve deeper into how deep learning powers these applications.
How Deep Learning Powers Applications?
The Structure of Deep Learning Neural Networks: Input Layer, Hidden Layers, and Output Layer
Deep learning models are structured in layers, each designed to recognize different aspects of data:
Input Layer: Takes raw data and prepares it for processing.
Hidden Layers: Perform computations and feature extractions. These layers are where most of the learning happens.
Output Layer: Produces the final output, making sense of the processed data.
Comparison with the Human Brain's Neural Networks
Much like neurons in the human brain, nodes in these layers are interconnected and transmit information. Each connection's strength can change as the system learns, similar to how our brains strengthen or weaken synaptic connections based on new experiences.
The Learning Process of Deep Learning Systems, Including Supervised and Unsupervised Learning
Deep learning systems can learn through:
Supervised Learning: Where models learn from labeled data, making it easier for them to predict outcomes based on past examples.
Unsupervised Learning: Where models identify patterns and relationships in data without any explicit instructions, adapting to the data’s inherent structures.
Now, to further clarify the distinction between deep learning and its broader category, let's compare it with general machine learning.
Deep Learning vs. Machine Learning
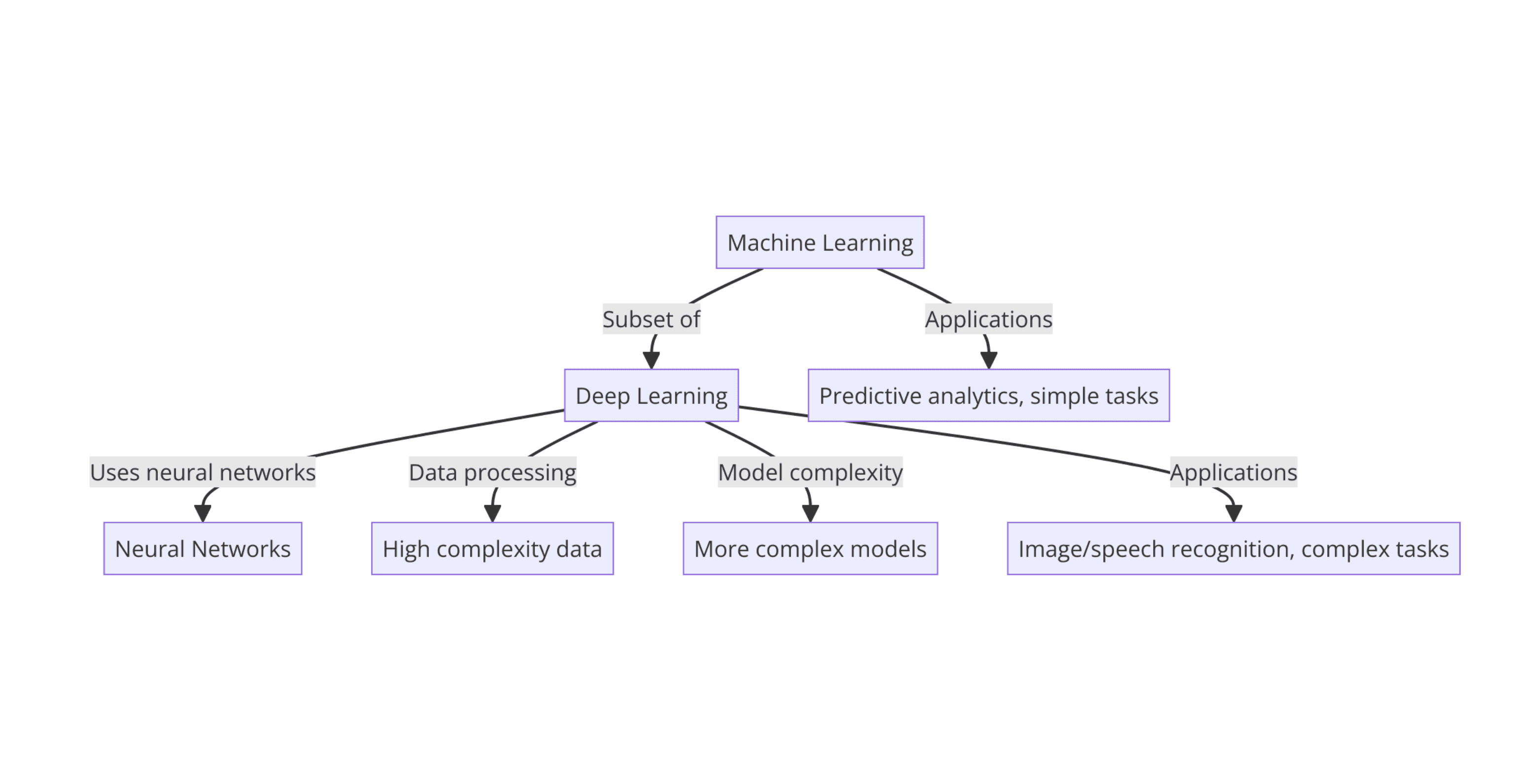
Deep Learning as a Subset of Machine Learning
Deep learning is a subset of machine learning that uses multi-layered neural networks to analyze various factors of data. It specializes in learning from data that is unstructured or unlabeled.
Data Processing: Deep learning is capable of processing and learning from data with much higher complexity than traditional machine learning.
Complexity: Deep learning models are typically more complex, capable of automatically discovering the representations needed for feature detection or classification from raw data.
Applications: While machine learning is often used for predictive analytics and simpler classification tasks, deep learning excels in areas where human-like cognition and complexity are required, such as image and speech recognition.
Having established how deep learning differs from and extends traditional machine learning, we'll next explore its broad applications across various industries, highlighting its impact on each sector.
Let's explore the broad applications of deep learning across various sectors, illustrating how this technology not only enhances current systems but also pioneers new innovations.
Broad Applications of Deep Learning
Automotive: Improving Vehicle Autonomy and Safety Features
In the automotive industry, deep learning is pivotal in developing advanced driver-assistance systems (ADAS) and autonomous vehicles. By analyzing real-time data from vehicle sensors and cameras, deep learning algorithms improve decision-making on the road, enhancing safety and driving efficiency.
Aerospace: Enhancing Navigation Systems and Flight Simulations
Deep learning algorithms are employed in aerospace for optimizing flight paths, improving navigation systems, and conducting sophisticated simulations that help in training pilots and testing aircraft under various conditions. These applications ensure higher safety and cost efficiency in aerospace operations.
Manufacturing: Optimizing Production Lines and Predictive Maintenance
In manufacturing, deep learning drives automation of complex production processes and predictive maintenance of equipment. By predicting failures before they occur and optimizing manufacturing workflows, deep learning significantly reduces downtime and increases productivity.
Electronics: Advancing Smart Devices and Personal Assistants
Deep learning transforms the electronics sector by enhancing the functionality of smart devices and personal assistants. It enables devices to understand and predict user preferences and respond to commands more accurately, making everyday interactions with technology smoother and more intuitive.
Medical Research: Revolutionizing Diagnostics and Patient Care
Perhaps one of the most impactful applications of deep learning is in medical research and healthcare, where it assists in diagnosing diseases with high accuracy through imaging data analysis and supports personalized medicine by adapting treatments to individual patient profiles.
Revolutionary Impact of Deep Learning Across Sectors
Computer Vision: Transforming Face Recognition and Image Processing
Deep learning has dramatically advanced computer vision technologies, enabling applications from security enhancements through facial recognition to new levels of interaction between computers and the visual world, such as real-time video analysis.
Speech Recognition: Elevating Voice Assistants and Natural Language Interfaces
Deep learning improves speech recognition systems, allowing for more accurate and natural interactions with voice-activated assistants and making these technologies accessible in more languages and dialects.
Natural Language Processing (NLP): Innovating Translation Services and Content Analysis
In NLP, deep learning models handle complex language data and enable more accurate translations, sentiment analysis, and content personalization, significantly enhancing communication across language barriers.
Recommendation Engines: Personalizing Online Consumer Experiences
Online platforms use deep learning to power recommendation engines that predict user preferences with unprecedented accuracy. This personalization improves user engagement and satisfaction, driving sales and content consumption.
With deep learning creating waves across various sectors, it's crucial to acknowledge the challenges this technology faces and the advancements that address these issues.
Let's delve into the challenges associated with deep learning, and the recent advancements that aim to overcome these hurdles, paving the way for more efficient and widespread applications of this transformative technology.
Challenges and Advances in Deep Learning
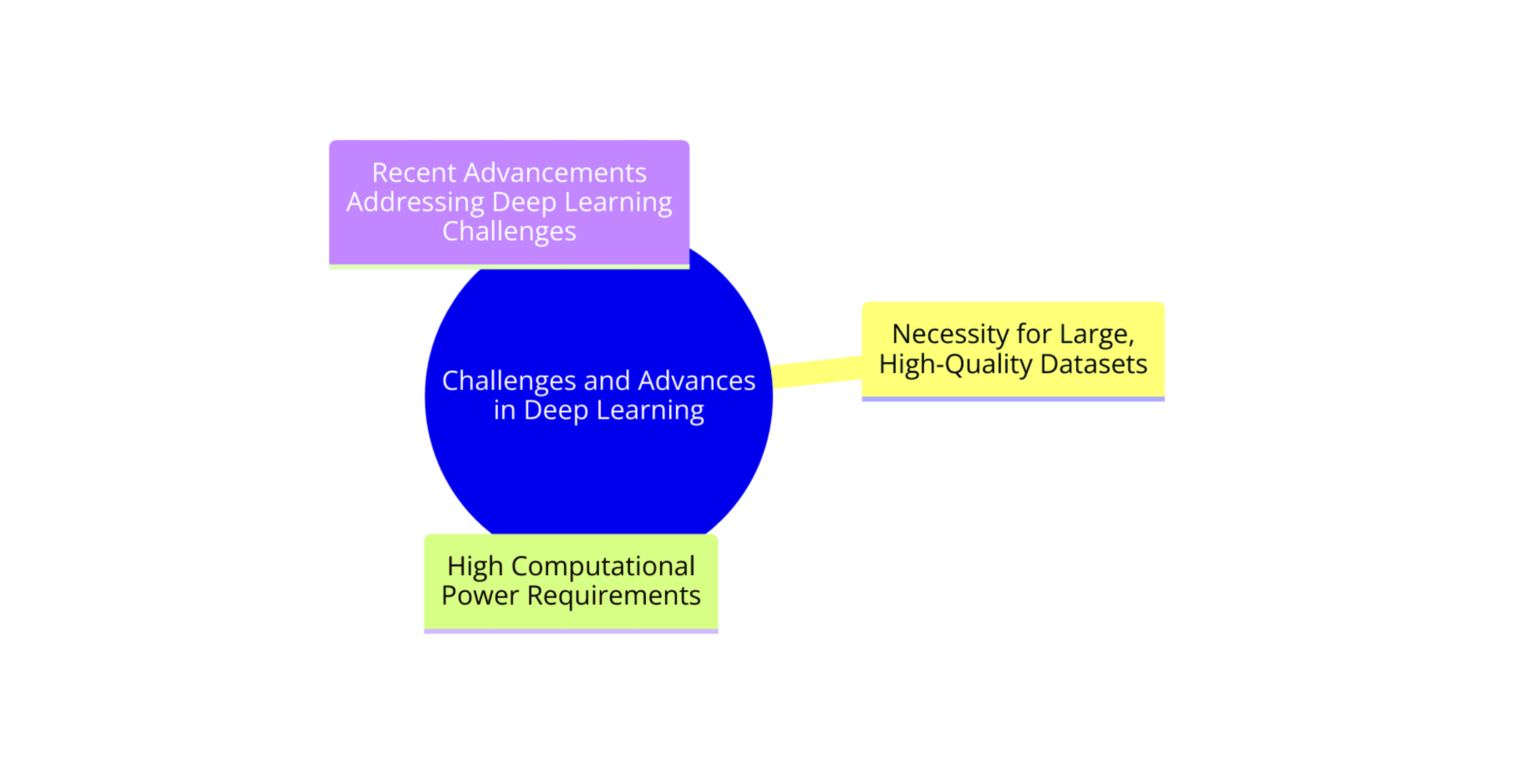
Necessity for Large, High-Quality Datasets
One of the primary challenges in deep learning is the necessity for vast amounts of high-quality data to train models effectively. Large datasets are crucial for achieving the accuracy and reliability expected from deep learning applications.
Solution: Advances in data synthesis and augmentation techniques allow for the expansion of dataset sizes without compromising quality. Additionally, transfer learning techniques enable models to learn from one domain and apply knowledge to another, reducing the total amount of data required.
High Computational Power Requirements
Deep learning models, particularly those involved in processing complex data like images and videos, require substantial computational resources, which can be cost-prohibitive.
Solution: Recent developments in hardware, such as GPUs and specialized processors like TPUs (Tensor Processing Units), have significantly reduced the computational load. Cloud-based solutions also offer scalable resources, making deep learning more accessible.
Recent Advancements Addressing Deep Learning Challenges
Technological advancements continue to address the inherent challenges of deep learning:
Efficient Network Architectures: Innovations like network pruning and the development of more efficient neural network architectures reduce the computational and memory overhead of training and deploying deep learning models.
Enhanced Learning Algorithms: Improvements in learning algorithms have enhanced the speed and efficiency of model training. Techniques like few-shot learning and reinforcement learning are breaking new ground in how models can be trained with less data and fewer resources.
Federated Learning: This technique allows for the training of algorithms across multiple decentralized devices or servers holding local data samples, without exchanging them. This method helps address privacy concerns, reduces the need for centralized data storage, and decreases bandwidth.
Future of Deep Learning
The Ongoing Evolution of Deep Learning Technologies
The field of deep learning is continuously evolving, with research pushing the boundaries of what's possible. This includes more sophisticated models that can handle complex tasks with greater accuracy and efficiency.
Potential Future Applications and the Impact on Various Industries
As deep learning technologies mature, their potential applications expand. Future developments could revolutionize sectors such as robotics, quantum computing, and even arts and creativity, significantly altering industry landscapes and operational protocols.
Innovations in Hardware and Algorithms Supporting Deep Learning
The hardware and algorithms that support deep learning are also advancing. Innovations in processor technology and algorithmic efficiency are making it feasible to deploy complex models on mobile devices and edge computing platforms, broadening the scope and accessibility of deep learning applications.
Learning Resources and Career Prospects
Information on Courses and Frameworks for Learning Deep Learning
For anyone looking to start or advance their knowledge in deep learning, numerous educational resources and frameworks are available to help:
Courses: Many online platforms offer specialized courses in deep learning. Coursera partners with top universities and companies to offer courses that cover both theoretical foundations and practical applications. Udacity and edX also provide comprehensive nanodegree programs and professional certifications.
Frameworks: TensorFlow, developed by Google, and PyTorch, developed by Facebook, are among the leading frameworks used for deep learning applications. These frameworks are supported by extensive documentation, tutorials, and active community forums that help new learners and seasoned professionals alike.
The Growing Demand for Professionals Skilled in Deep Learning
The demand for deep learning skills is surging across various industries including technology, healthcare, finance, and automotive.
This demand is driven by the need to extract more complex insights from data and automate tasks that traditionally require human intelligence.
Discussion on Career Paths and Salary Expectations in the Field
Careers in deep learning are both diverse and rewarding:
Data Scientist: Specializing in deep learning, focusing on developing algorithms that can learn from and make predictions on data.
AI Research Scientist: Working on the cutting edge of artificial intelligence, improving the techniques used to build deep learning models.
Machine Learning Engineer: Implementing models in practical applications, optimizing them for performance and scalability.
Salaries in these fields are highly competitive, reflecting both the demand for these skills and the value these professionals bring to businesses. In the U.S., salaries can range from $100,000 to over $150,000 annually, depending on experience, location, and specific industry.
FAQs on Deep Learning Applications
To round off our exploration, let's address some frequently asked questions about deep learning applications, which might help clarify common queries for those new to the field.
What are the most common applications of deep learning today?
Deep learning is commonly used in applications like image and speech recognition, autonomous vehicles, and personalized recommendation systems.
How does deep learning differ from traditional machine learning in application?
Deep learning typically requires more data and computational power but excels in handling very complex patterns and predictions, making it ideal for applications like natural language processing and computer vision.
Tips for Beginners Looking to Enter the Field of Deep Learning
Start Small: Begin with understanding the basics of neural networks and gradually move to more complex structures like convolutional and recurrent neural networks.
Hands-On Practice: Utilize open-source datasets to practice building and training models. Platforms like Kaggle provide a good starting point with competitions designed to challenge and enhance your skills.
Community and Networking: Engaging with the community through forums, local meetups, and conferences can provide invaluable learning opportunities and connections in the industry.
This comprehensive look into deep learning not only highlights its importance across various sectors but also provides a roadmap for those interested in diving into this exciting field. Whether you're just starting out or looking to refine your skills, the resources and career opportunities in deep learning are vast and growing.
Deep learning is an advanced form of artificial intelligence that mimics the workings of the human brain in processing data and creating patterns for use in decision making. It is a key technology behind many innovative applications that enhance our daily lives and transform industries.
From speech recognition in virtual assistants to recommendation systems on streaming platforms, deep learning powers a range of technologies that enhance user experiences and increase automation. Its ability to learn from vast amounts of data allows it to make highly accurate predictions and automate complex tasks.
With a clear understanding of what deep learning is and where we encounter it in our daily lives, let's delve deeper into how deep learning powers these applications.
How Deep Learning Powers Applications?
The Structure of Deep Learning Neural Networks: Input Layer, Hidden Layers, and Output Layer
Deep learning models are structured in layers, each designed to recognize different aspects of data:
Input Layer: Takes raw data and prepares it for processing.
Hidden Layers: Perform computations and feature extractions. These layers are where most of the learning happens.
Output Layer: Produces the final output, making sense of the processed data.
Comparison with the Human Brain's Neural Networks
Much like neurons in the human brain, nodes in these layers are interconnected and transmit information. Each connection's strength can change as the system learns, similar to how our brains strengthen or weaken synaptic connections based on new experiences.
The Learning Process of Deep Learning Systems, Including Supervised and Unsupervised Learning
Deep learning systems can learn through:
Supervised Learning: Where models learn from labeled data, making it easier for them to predict outcomes based on past examples.
Unsupervised Learning: Where models identify patterns and relationships in data without any explicit instructions, adapting to the data’s inherent structures.
Now, to further clarify the distinction between deep learning and its broader category, let's compare it with general machine learning.
Deep Learning vs. Machine Learning
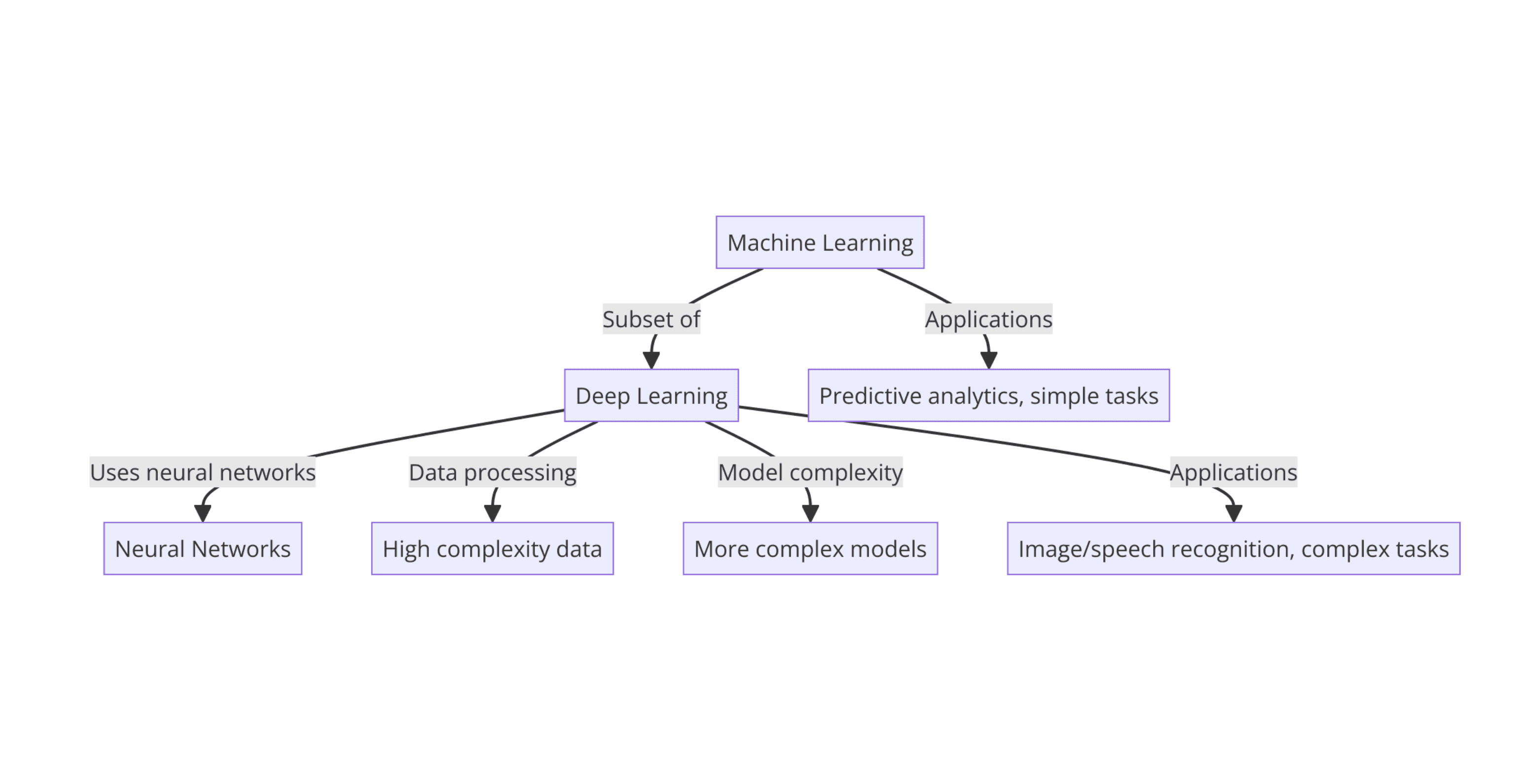
Deep Learning as a Subset of Machine Learning
Deep learning is a subset of machine learning that uses multi-layered neural networks to analyze various factors of data. It specializes in learning from data that is unstructured or unlabeled.
Data Processing: Deep learning is capable of processing and learning from data with much higher complexity than traditional machine learning.
Complexity: Deep learning models are typically more complex, capable of automatically discovering the representations needed for feature detection or classification from raw data.
Applications: While machine learning is often used for predictive analytics and simpler classification tasks, deep learning excels in areas where human-like cognition and complexity are required, such as image and speech recognition.
Having established how deep learning differs from and extends traditional machine learning, we'll next explore its broad applications across various industries, highlighting its impact on each sector.
Let's explore the broad applications of deep learning across various sectors, illustrating how this technology not only enhances current systems but also pioneers new innovations.
Broad Applications of Deep Learning
Automotive: Improving Vehicle Autonomy and Safety Features
In the automotive industry, deep learning is pivotal in developing advanced driver-assistance systems (ADAS) and autonomous vehicles. By analyzing real-time data from vehicle sensors and cameras, deep learning algorithms improve decision-making on the road, enhancing safety and driving efficiency.
Aerospace: Enhancing Navigation Systems and Flight Simulations
Deep learning algorithms are employed in aerospace for optimizing flight paths, improving navigation systems, and conducting sophisticated simulations that help in training pilots and testing aircraft under various conditions. These applications ensure higher safety and cost efficiency in aerospace operations.
Manufacturing: Optimizing Production Lines and Predictive Maintenance
In manufacturing, deep learning drives automation of complex production processes and predictive maintenance of equipment. By predicting failures before they occur and optimizing manufacturing workflows, deep learning significantly reduces downtime and increases productivity.
Electronics: Advancing Smart Devices and Personal Assistants
Deep learning transforms the electronics sector by enhancing the functionality of smart devices and personal assistants. It enables devices to understand and predict user preferences and respond to commands more accurately, making everyday interactions with technology smoother and more intuitive.
Medical Research: Revolutionizing Diagnostics and Patient Care
Perhaps one of the most impactful applications of deep learning is in medical research and healthcare, where it assists in diagnosing diseases with high accuracy through imaging data analysis and supports personalized medicine by adapting treatments to individual patient profiles.
Revolutionary Impact of Deep Learning Across Sectors
Computer Vision: Transforming Face Recognition and Image Processing
Deep learning has dramatically advanced computer vision technologies, enabling applications from security enhancements through facial recognition to new levels of interaction between computers and the visual world, such as real-time video analysis.
Speech Recognition: Elevating Voice Assistants and Natural Language Interfaces
Deep learning improves speech recognition systems, allowing for more accurate and natural interactions with voice-activated assistants and making these technologies accessible in more languages and dialects.
Natural Language Processing (NLP): Innovating Translation Services and Content Analysis
In NLP, deep learning models handle complex language data and enable more accurate translations, sentiment analysis, and content personalization, significantly enhancing communication across language barriers.
Recommendation Engines: Personalizing Online Consumer Experiences
Online platforms use deep learning to power recommendation engines that predict user preferences with unprecedented accuracy. This personalization improves user engagement and satisfaction, driving sales and content consumption.
With deep learning creating waves across various sectors, it's crucial to acknowledge the challenges this technology faces and the advancements that address these issues.
Let's delve into the challenges associated with deep learning, and the recent advancements that aim to overcome these hurdles, paving the way for more efficient and widespread applications of this transformative technology.
Challenges and Advances in Deep Learning
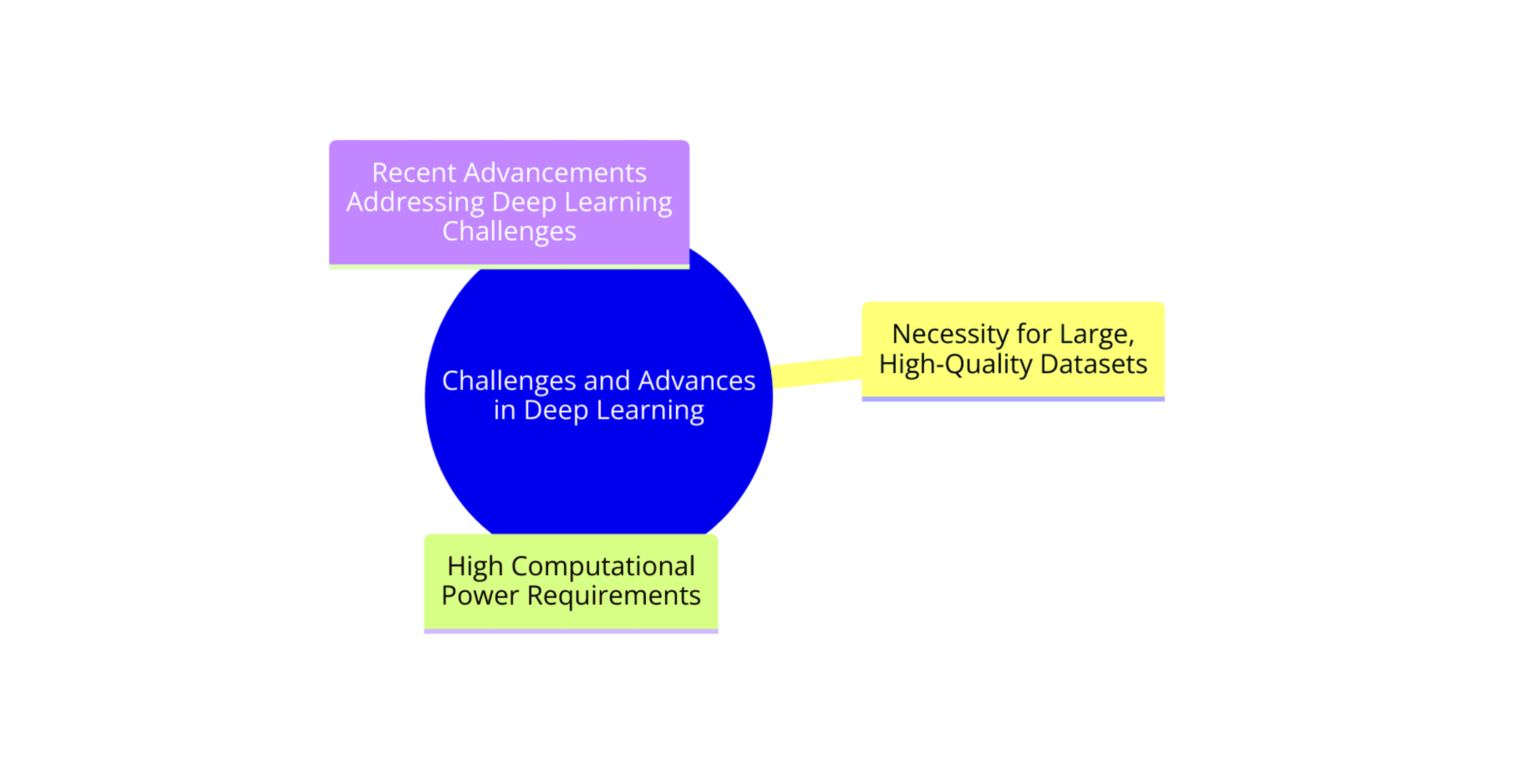
Necessity for Large, High-Quality Datasets
One of the primary challenges in deep learning is the necessity for vast amounts of high-quality data to train models effectively. Large datasets are crucial for achieving the accuracy and reliability expected from deep learning applications.
Solution: Advances in data synthesis and augmentation techniques allow for the expansion of dataset sizes without compromising quality. Additionally, transfer learning techniques enable models to learn from one domain and apply knowledge to another, reducing the total amount of data required.
High Computational Power Requirements
Deep learning models, particularly those involved in processing complex data like images and videos, require substantial computational resources, which can be cost-prohibitive.
Solution: Recent developments in hardware, such as GPUs and specialized processors like TPUs (Tensor Processing Units), have significantly reduced the computational load. Cloud-based solutions also offer scalable resources, making deep learning more accessible.
Recent Advancements Addressing Deep Learning Challenges
Technological advancements continue to address the inherent challenges of deep learning:
Efficient Network Architectures: Innovations like network pruning and the development of more efficient neural network architectures reduce the computational and memory overhead of training and deploying deep learning models.
Enhanced Learning Algorithms: Improvements in learning algorithms have enhanced the speed and efficiency of model training. Techniques like few-shot learning and reinforcement learning are breaking new ground in how models can be trained with less data and fewer resources.
Federated Learning: This technique allows for the training of algorithms across multiple decentralized devices or servers holding local data samples, without exchanging them. This method helps address privacy concerns, reduces the need for centralized data storage, and decreases bandwidth.
Future of Deep Learning
The Ongoing Evolution of Deep Learning Technologies
The field of deep learning is continuously evolving, with research pushing the boundaries of what's possible. This includes more sophisticated models that can handle complex tasks with greater accuracy and efficiency.
Potential Future Applications and the Impact on Various Industries
As deep learning technologies mature, their potential applications expand. Future developments could revolutionize sectors such as robotics, quantum computing, and even arts and creativity, significantly altering industry landscapes and operational protocols.
Innovations in Hardware and Algorithms Supporting Deep Learning
The hardware and algorithms that support deep learning are also advancing. Innovations in processor technology and algorithmic efficiency are making it feasible to deploy complex models on mobile devices and edge computing platforms, broadening the scope and accessibility of deep learning applications.
Learning Resources and Career Prospects
Information on Courses and Frameworks for Learning Deep Learning
For anyone looking to start or advance their knowledge in deep learning, numerous educational resources and frameworks are available to help:
Courses: Many online platforms offer specialized courses in deep learning. Coursera partners with top universities and companies to offer courses that cover both theoretical foundations and practical applications. Udacity and edX also provide comprehensive nanodegree programs and professional certifications.
Frameworks: TensorFlow, developed by Google, and PyTorch, developed by Facebook, are among the leading frameworks used for deep learning applications. These frameworks are supported by extensive documentation, tutorials, and active community forums that help new learners and seasoned professionals alike.
The Growing Demand for Professionals Skilled in Deep Learning
The demand for deep learning skills is surging across various industries including technology, healthcare, finance, and automotive.
This demand is driven by the need to extract more complex insights from data and automate tasks that traditionally require human intelligence.
Discussion on Career Paths and Salary Expectations in the Field
Careers in deep learning are both diverse and rewarding:
Data Scientist: Specializing in deep learning, focusing on developing algorithms that can learn from and make predictions on data.
AI Research Scientist: Working on the cutting edge of artificial intelligence, improving the techniques used to build deep learning models.
Machine Learning Engineer: Implementing models in practical applications, optimizing them for performance and scalability.
Salaries in these fields are highly competitive, reflecting both the demand for these skills and the value these professionals bring to businesses. In the U.S., salaries can range from $100,000 to over $150,000 annually, depending on experience, location, and specific industry.
FAQs on Deep Learning Applications
To round off our exploration, let's address some frequently asked questions about deep learning applications, which might help clarify common queries for those new to the field.
What are the most common applications of deep learning today?
Deep learning is commonly used in applications like image and speech recognition, autonomous vehicles, and personalized recommendation systems.
How does deep learning differ from traditional machine learning in application?
Deep learning typically requires more data and computational power but excels in handling very complex patterns and predictions, making it ideal for applications like natural language processing and computer vision.
Tips for Beginners Looking to Enter the Field of Deep Learning
Start Small: Begin with understanding the basics of neural networks and gradually move to more complex structures like convolutional and recurrent neural networks.
Hands-On Practice: Utilize open-source datasets to practice building and training models. Platforms like Kaggle provide a good starting point with competitions designed to challenge and enhance your skills.
Community and Networking: Engaging with the community through forums, local meetups, and conferences can provide invaluable learning opportunities and connections in the industry.
This comprehensive look into deep learning not only highlights its importance across various sectors but also provides a roadmap for those interested in diving into this exciting field. Whether you're just starting out or looking to refine your skills, the resources and career opportunities in deep learning are vast and growing.