Practical Guide For Deploying LLMs In Production
Practical Guide For Deploying LLMs In Production
Practical Guide For Deploying LLMs In Production
Rehan Asif
Jun 12, 2024
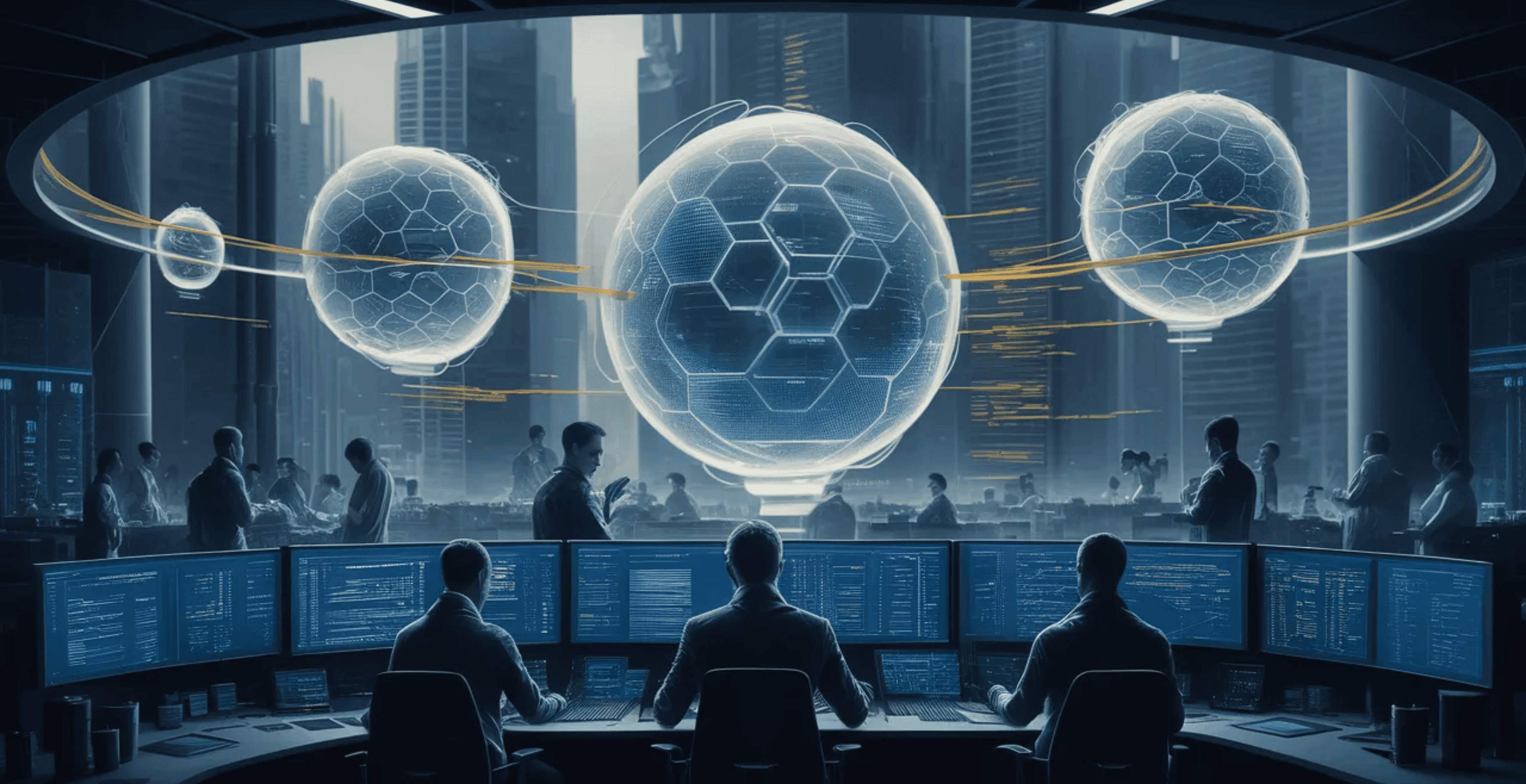
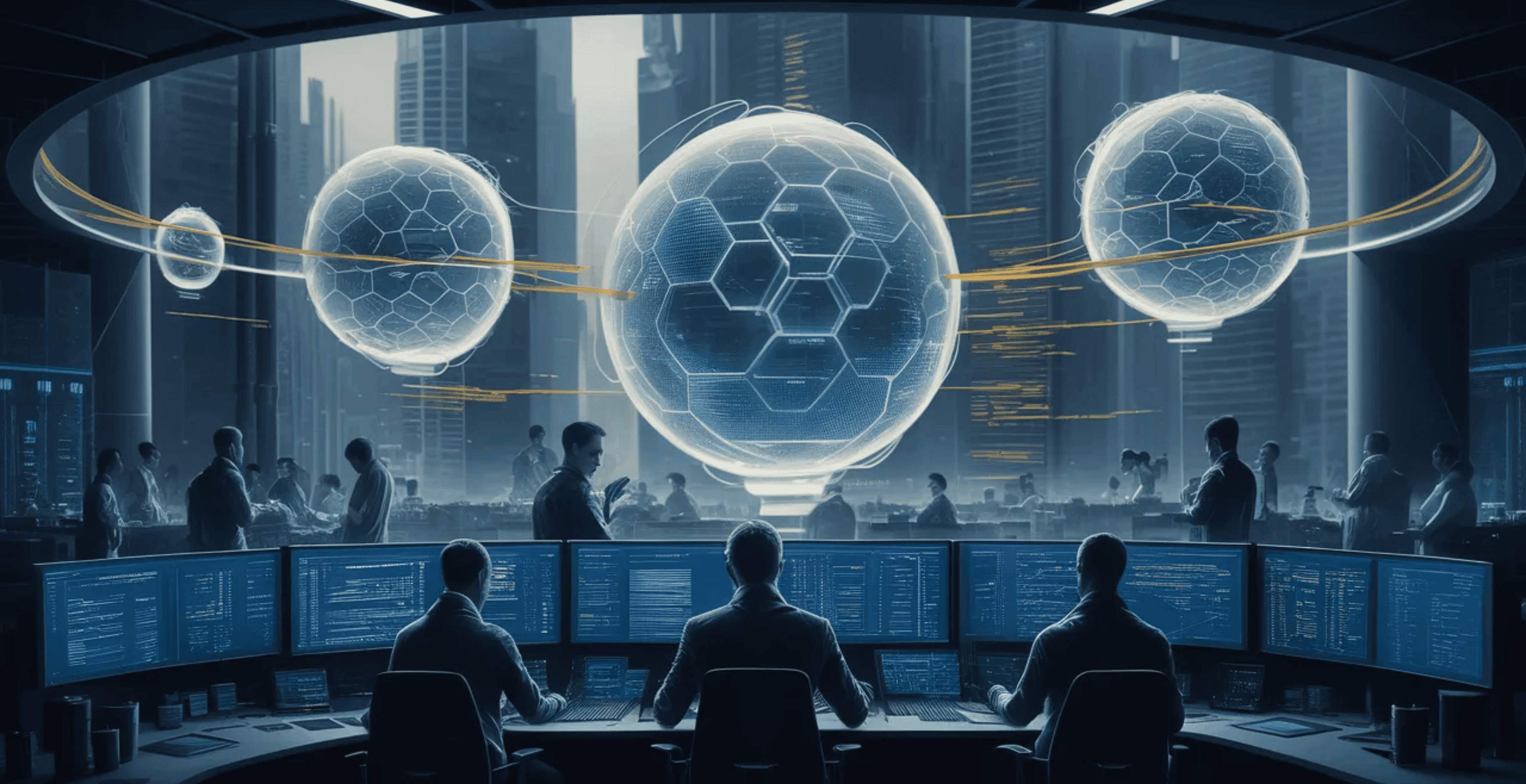
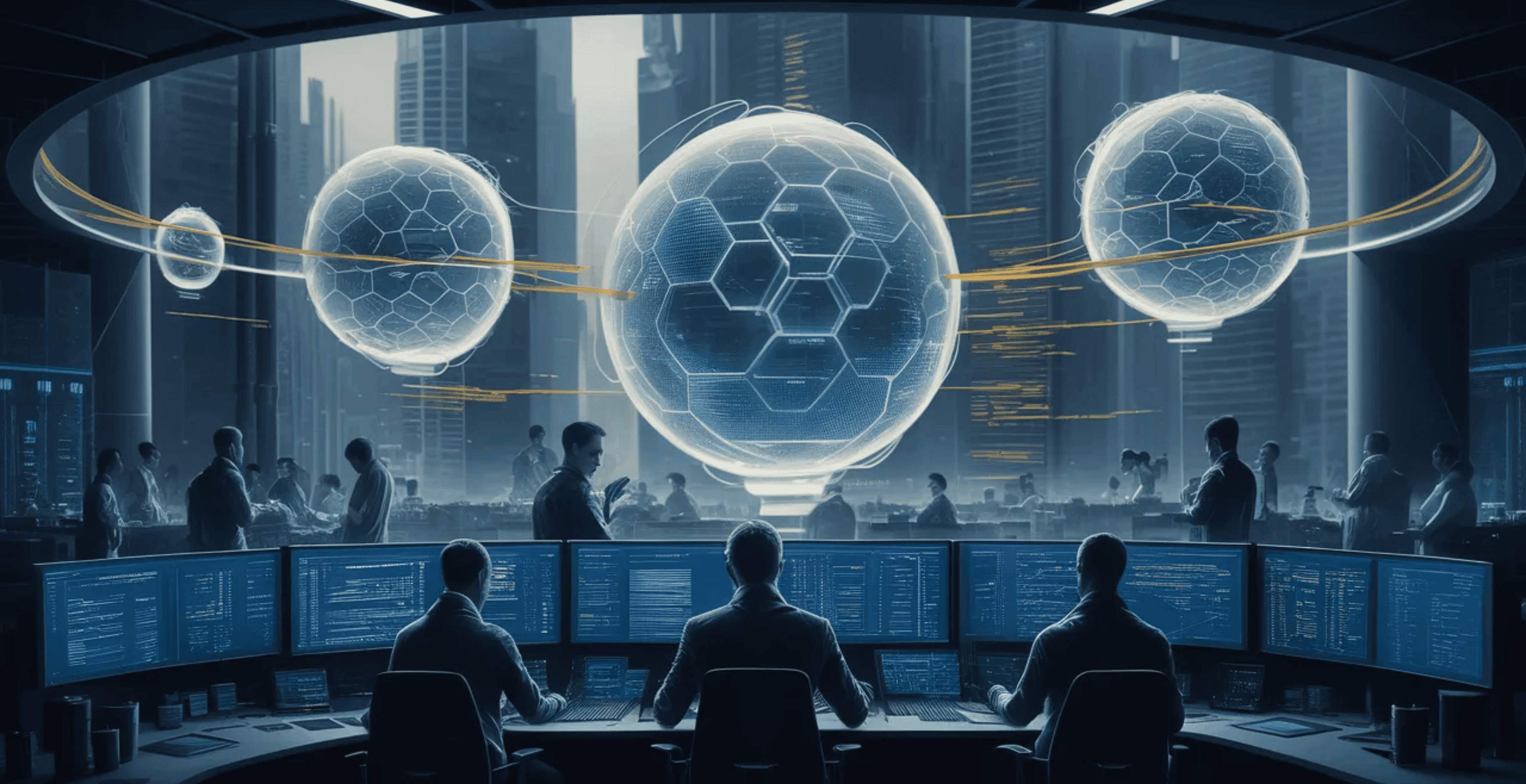
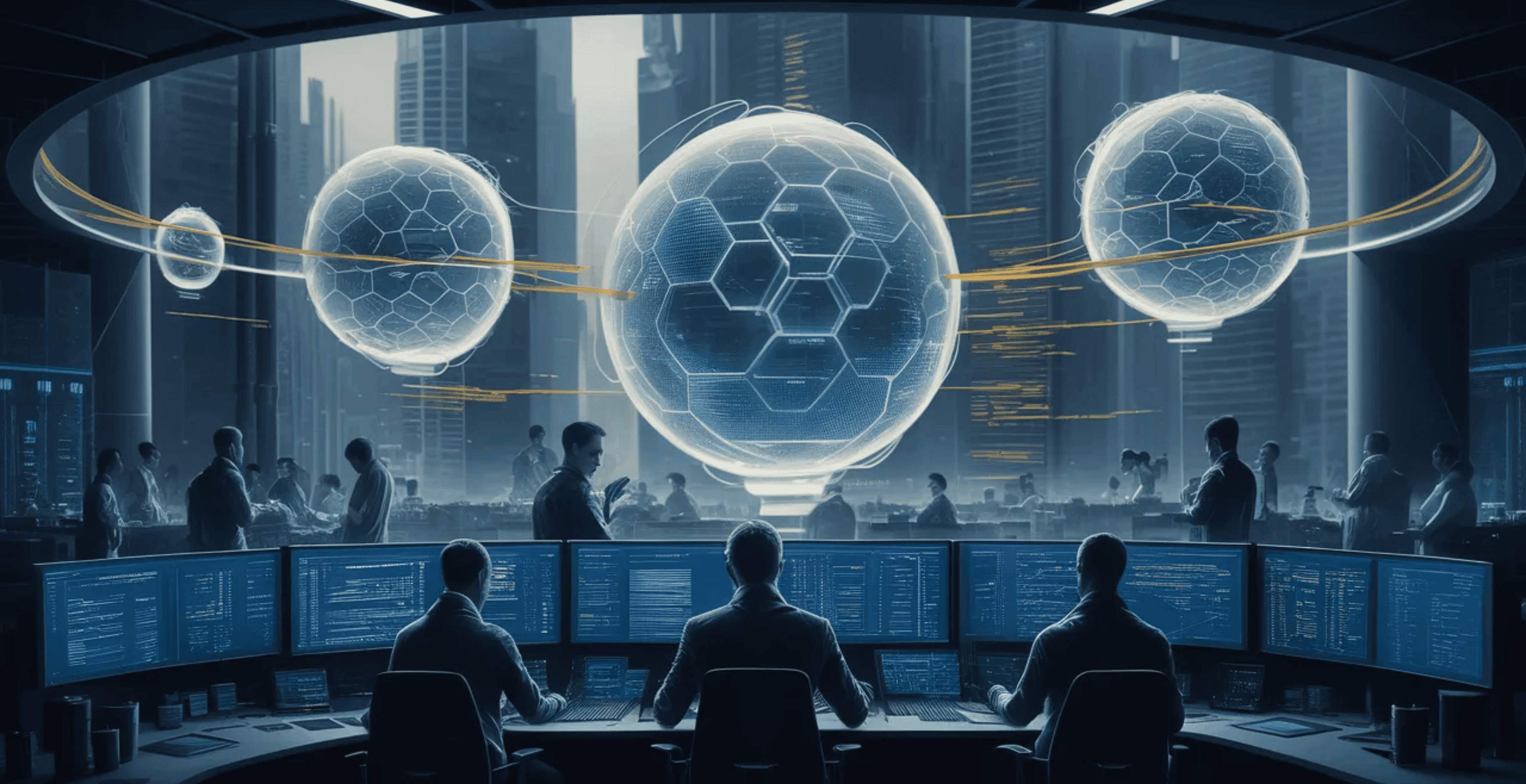
The prospect of machine learning is swiftly emerging, and at the vanguard of this revolution are large language models (LLMs). These prominent tools are revisiting what’s feasible with Natural Language Processing (NLP), from producing human-like text to providing intuitive customer service communications. But while the potential of LLMs is enormous, transforming from a demonstration of the concept of a deployable application is a formidable challenge.
Deploying LLMs in production involves a quirky set of obstacles, equating a requirement for efficient augmentation techniques such as prompting v/s fine-tuning, handling delayed data transfer, and ensuring data integrity and seclusion. This guide will cover sturdy plans and practical tips to make the journey sleeker and more effective.
Challenges of Deploying LLMs in Production
Ambiguity and Inconsistency in Outputs
Natural Language is fundamentally vague, leading to inconsistent yields from LLMs. This inconsistency makes immediate assessment and upgradation critical. You need to give time to processing prompts and examining numerous synopses to ensure steady performance. Frequently updating and repeating your prompts can help alleviate unanticipated or incorrect responses.
Cost and Latency Considerations
Cost and Latency are substantial components prompting deployment decisions. The length of prompts and yields directly affect these elements. You must balance these distinct approaches, such as prompting, fine-tuning, or both. Fine-tuning can offer more congruous outcomes but might indulge higher upfront costs. Prompt engineering can be economical initially but may need ongoing adaptations to maintain performance.
High Latency and Inference Costs
High latency and inference costs present challenges for full-stack engineering teams. LLMs need considerable arithmetic resources, resulting in slower reply times and increased functional expenses. You should traverse upgrade methods like model distillation, thresholding, or using more effective architectures to curtail these loads. Enforcing cache plans for regularly demanding outputs can also help enhance latency.
Significance of Data Quality and Privacy
Data Quality substantially affects the performance of LLMs. High-quality, disparate datasets lead to better model productivity. You need to pioneer sturdy data gathering and cleaning procedures to ensure the training data is delegated and precise. Model selection and assessing plans are equally significant. Frequently gauge your models against industry standards and constantly observe their performance in production.
Privacy deliberations are paramount when handling user data. To protect sensitive data, comply with data protection regulations and enforce powerful security measures. Methods such as differential privacy and consolidated learning can help improve privacy while maintaining the model's performance.
Addressing these challenges can help you effectively deploy LLMs in production, utilize their full potential, and maintain effectiveness and dependability.
Best Practices for LLM Deployment
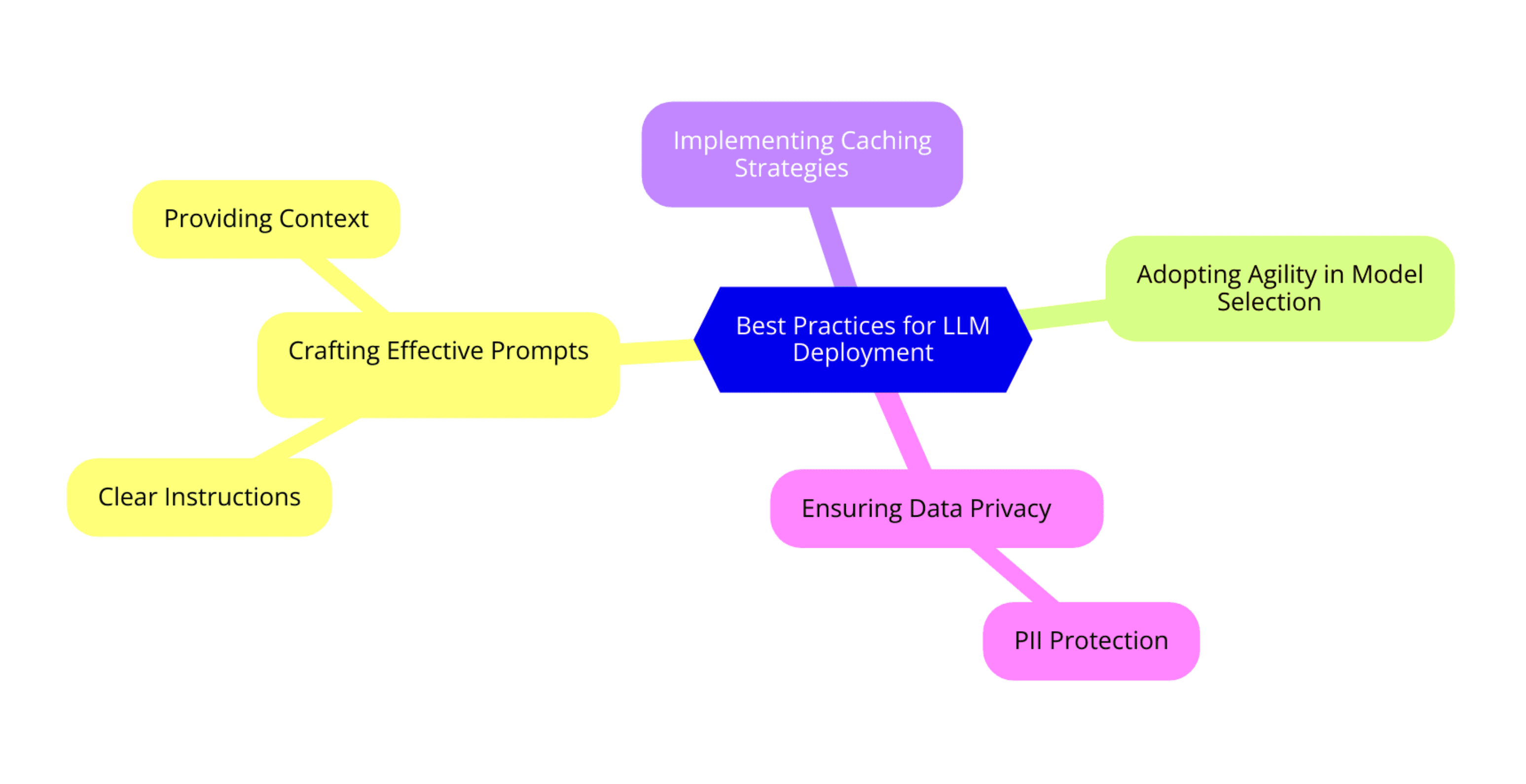
Crafting Effective Prompts with Clear Instructions and Context
When deploying a large language model (LLM), focus on giving transparent directions and pertinent context in your prompts. This substantially improves the precision and pertinence of the model’s responses. Clear directions guide the model toward the desired output, while context ensures it comprehends the nuances and particulars of the request. For instance, instead of asking, “Tell me about Python, “you could state, “Give an outline of Python programming language attributes, indulging its syntax and prevalent utilization cases.”
Adopting Agility in Model Selection
Maintain adaptability in selecting the apt LLM for your application. Evaluate LLM based on performance metrics and cost deliberations, and be ready to switch between distinct models as required. This flexibility permits you to upgrade for precise tasks, balancing elements such as response quality, dormancy and functional expenses. For example, you might utilize a more significant model for intricate queries and a cost-effective one for easier tasks.
Implementing Caching Strategies
Implement cache mechanisms to reserve and reutilize verified answers. This approach minimizes excess computations, improves response times and curtailing computational fixed costs. For FAQs or common questions, caching antecedently produced responses ensures constant and rapid delivery, enhancing user experience and system effectiveness.
Ensuring Data Privacy and PII Protection
Prioritize user data privacy by integrating sturdy gauges to determine and remove Personally Identifiable Information (PII) from communications. Use mechanical tools and algorithms to determine and unname sensitive data, protecting user privacy and following data safeguarding regulations. Implementing these measures not only to safeguard users but also to build trust in your LLM application.
Augmentation Techniques for Optimizing LLM Performance
Introduction to Prompting and Fine-Tuning
When it comes to getting the most out of Large Language Models (LLMs), two predominant methods excels: prompting and fine-tuning. Envision prompting as having a comprehensive conversation with your model–giving it precise directions to shape its reactions. On the other hand, fine-tuning is like instructing an athlete, where you process the model’s capabilities by feeding it a considered dataset to contemplate in implicit tasks.
In the industry, there is a sustained debate about which technique is better. Some dispute that prompting is more adaptable and brisk to enforce, while others believe fine-tuning leads to domineering, task-precise performance. Eventually, both techniques have their merits and can be utilized independently to upgrade your LLMs output.
Overview of Retrieval Augmented Generation (RAG)
You might have observed that sometimes LLMs, despite their enormous comprehension, can generate responses that are off the mark. That’s where Retrieval-Augmented Generation (RAG) steps in. RAG enhances the standard and pertinence of responses by incorporating external information retrieval systems with the production abilities of the LLM.
Think of RAG as giving your model ingress to a library of knowledge that it can discuss while producing responses. This hybrid approach ensures that the data your model gives is not only coherent but also precise and up-to-date.
Prompt Tuning and Distillation
Now, let’s discuss prompt tuning and distillation–two methods that help minor models punch above their weight. Immediate tuning involves adapting the model’s prompts to enhance performance on precise tasks without modifying the model’s fundamental architecture. It’s like fine-tuning but at the level of the directions to give.
Distillation, on the other hand, is a bit more intricate. It involves instructing a smaller
Model to mock the behavior of a greater, more stronger one. This procedure helps balance performance and expense, enabling you to deploy effective models without yielding too much on quality.
By utilizing immediate tuning, you can improve the abilities of smaller models without the hefty computational expenses allied with training large models. And with distillation, you accomplish similar results by transferring the knowledge from a larger model to a smaller one.
Building Scalable LLM Applications
Building scalable LLM applications need a planned approach to ensure effectiveness, dependability and safety. Here’s how you can accomplish that:
Emphasize AI Governance
AI Governance is critical when deploying LLM applications. You need to enforce human-in-the-loop systems for verification, ensuring that yields are precise and pertinent. Automated toxicity detection systems are equally significant to maintain the standard and applicability of the produced content. This dual approach helps in alleviating threats and enhancing overall performance of your LLM applications.
Key Elements for LLM Deployment at Scale
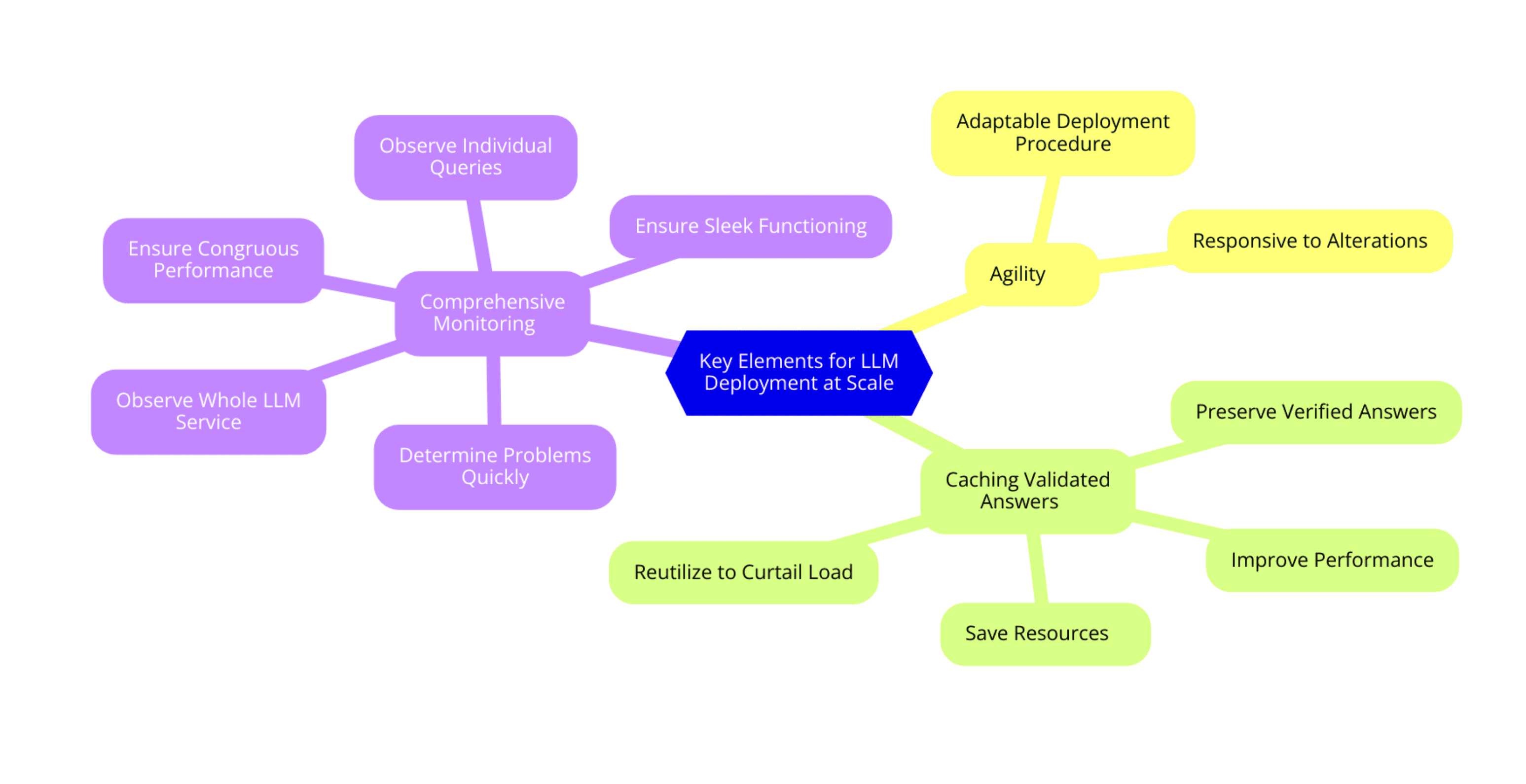
To deploy LLMs at scale efficiently, concentrate on these chief components:
Agility: Ensure your deployment procedure is adaptable and receptive to alterations. This permits you to adjust rapidly to new needs and problems.
Caching Validated Answers: Preserve and reutilize previously verified answers to curtail computational load and response times. This not only improves performance but also saves resources.
Comprehensive Monitoring: Observe both individual queries and the whole LLM service constantly. This helps in determining and figuring problems immediately, ensuring sleek functioning and congruous performance.
Introducing the LLM Mesh
The LLM Mesh is an innovative solution for accessing Generative AI models and services in a ductile and secure manner. It offers a combined structure that streamlines the incorporation and handling of numerous AI elements, ensuring sleek communication and function across distinct systems. By enforcing an LLM mesh, you improve the expandability and security of your AI applications, making it easier to deploy and handle large-scale LLM solutions.
By concentrating on these strategies, you can build sturdy and scalable LLM applications that meet your requirements proficiently and effectively.
Also Read:- Evaluating Large Language Models: Methods and Metrics
Task Composability and Control Flows in LLM Applications
Executing Tasks Sequentially, Parallelly, or Based on Conditions
When you are operating with LLM agents for multi-task apps, comprehending task enforcement is critical. You might enforce tasks successively, where one task adheres another, ideal for synopsis requiring dependent steps. On the other hand, you can run tasks in correspondence, elevating effectiveness by managing multiple functionality concurrently. Contingent enforcement (using "if" or "for" loops) lets you manage more intricate productivity, provoking precise tasks based on implicit circumstances.
Differentiating Between Tools and Plugins
It’s significant to differentiate between tools and plugins when constructing dependable LLM apps. Tools are separate utilities that offer precise usefulness, while plugins are extensions that can improve existing structures. Both are critical, but pragmatic testing of LLM agents ensures dependability. Knowing this discrepancy helps you select the right elements for your requirements, improving the soundness and efficiency of your applications.
Exploring Distinct Control Flow Types
Choosing the right control flow type can substantially affect your LLM applications performance. Sequential control flows are effortless and immaculate for tasks that adhere to a rigid order. Parallel control flows, on the other hand, permit synchronous task enforcement, saving time and resources. Contingent flows, such as “if” and “for” loops, provides the adaptability to enforce tasks based on assured circumstances or repetitions, making your apps more robust and responsive.
By comprehending and efficiently using these methods, you are better adapted to child sophisticated and effective LLM applications that meet your precise needs.
Promising Use Cases for LLMs
AI Assistant, Chatbot for Customer Service and Engagement
Envision having an AI assistant that’s attainable 24/7 to acknowledge consumer doubts and problems. LLMs can improve chatbots to provide precise and compassionate responses, enhancing customer satisfaction and engagement. Latest progressions show that AI-driven chatbots can manage up to 80% of everyday queries, extricating human support staff to intercept more intricate issues.
Code Generation: Enhancing Programming and Development
If you are a designer, you have likely desired for rapid coding solutions. LLMs such as OpenAI’s codex can comprehend natural language prompts and yields code snippets in multiple programming languages. This not only hastens the development process but also helps you grab bugs early. GitHub's Copilot, powered by Codex, has already become an indispensable tool for many developers.
Personalized Learning and Training
LLMs can act as tailored tutors, customizing lessons and study material based on your learning speed and approach. Whether you are a student or professional looking to upgrade, AI-driven platforms can offer you personalized educational content. Latest studies specify that customized learning techniques can enhance procurement rates by up to 30%.
'Talk-to-Data' for Intuitive Analysis
Envision simply asking queries or doubts about your data and receiving immediate, perceptive responses. LLMs are making this a reality by empowering ‘Talk-to-Data’ attributes in data inspection tools. Instead of writing intricate questions or doubts, you can now ask in plain English, and the AI explicates your intention, producing accurate outcomes. This inventiveness transforms how ventures and researchers engage with their information, making inspection more attainable and inherent. With such tools, regulating is time-saving, and valuable perceptions are more easily exposed.
Spotlight on RagaAI LLM Hub
If you are looking for a pragmatic platform to traverse and deploy LLMs, RagaAI LLM Hub provides a unified solution. It offers access to various generative AI models and services, ensuring you have the tools required to flawlessly incorporate LLM capabilities into your apps. With attributes designed for manageability and security, RagaAI LLM Hub is a magnificent resource for expert and experienced AI developers.
Conclusion
The world of LLM applications is revolutionizing at rapid speed, and staying ahead needs an accurate deployment plan. From composing efficient prompts to integrating progressed methods like RAG and fine-tuning, the expedition to deployable LLM applications can be intricate but extremely fruitful.
As you outlook that phase, remember that adaptability, sturdy governance, and constant learning are your best supporters. Whether you are improving customer assistance, generating advanced analytics, or developing inventive AI products, deploying LLMs efficiently will be a groundbreaker for your undertakings.
Stay interested, stay supple, and keep propelling the boundaries of what’s achievable with LLMs. Happy deploying!
The prospect of machine learning is swiftly emerging, and at the vanguard of this revolution are large language models (LLMs). These prominent tools are revisiting what’s feasible with Natural Language Processing (NLP), from producing human-like text to providing intuitive customer service communications. But while the potential of LLMs is enormous, transforming from a demonstration of the concept of a deployable application is a formidable challenge.
Deploying LLMs in production involves a quirky set of obstacles, equating a requirement for efficient augmentation techniques such as prompting v/s fine-tuning, handling delayed data transfer, and ensuring data integrity and seclusion. This guide will cover sturdy plans and practical tips to make the journey sleeker and more effective.
Challenges of Deploying LLMs in Production
Ambiguity and Inconsistency in Outputs
Natural Language is fundamentally vague, leading to inconsistent yields from LLMs. This inconsistency makes immediate assessment and upgradation critical. You need to give time to processing prompts and examining numerous synopses to ensure steady performance. Frequently updating and repeating your prompts can help alleviate unanticipated or incorrect responses.
Cost and Latency Considerations
Cost and Latency are substantial components prompting deployment decisions. The length of prompts and yields directly affect these elements. You must balance these distinct approaches, such as prompting, fine-tuning, or both. Fine-tuning can offer more congruous outcomes but might indulge higher upfront costs. Prompt engineering can be economical initially but may need ongoing adaptations to maintain performance.
High Latency and Inference Costs
High latency and inference costs present challenges for full-stack engineering teams. LLMs need considerable arithmetic resources, resulting in slower reply times and increased functional expenses. You should traverse upgrade methods like model distillation, thresholding, or using more effective architectures to curtail these loads. Enforcing cache plans for regularly demanding outputs can also help enhance latency.
Significance of Data Quality and Privacy
Data Quality substantially affects the performance of LLMs. High-quality, disparate datasets lead to better model productivity. You need to pioneer sturdy data gathering and cleaning procedures to ensure the training data is delegated and precise. Model selection and assessing plans are equally significant. Frequently gauge your models against industry standards and constantly observe their performance in production.
Privacy deliberations are paramount when handling user data. To protect sensitive data, comply with data protection regulations and enforce powerful security measures. Methods such as differential privacy and consolidated learning can help improve privacy while maintaining the model's performance.
Addressing these challenges can help you effectively deploy LLMs in production, utilize their full potential, and maintain effectiveness and dependability.
Best Practices for LLM Deployment
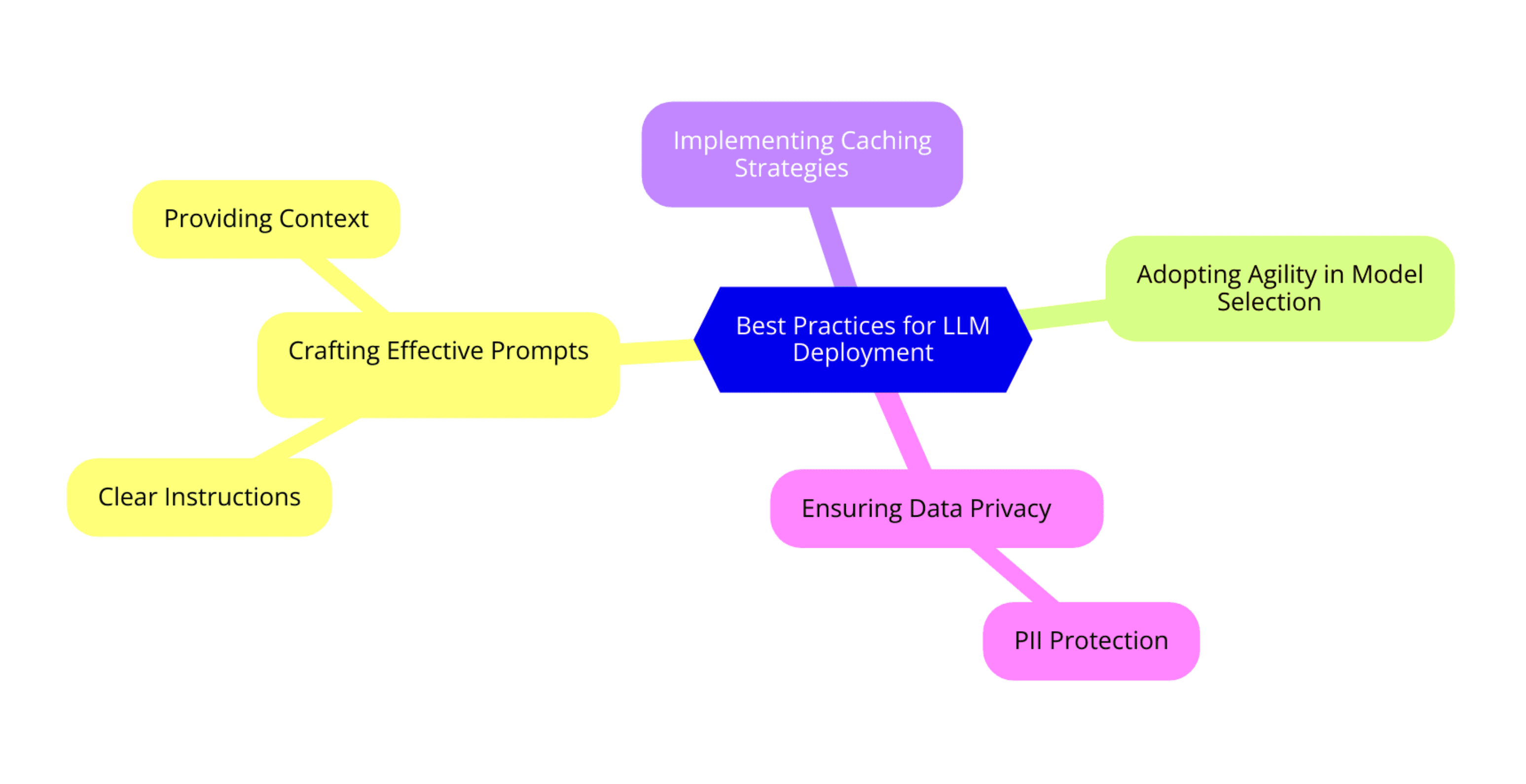
Crafting Effective Prompts with Clear Instructions and Context
When deploying a large language model (LLM), focus on giving transparent directions and pertinent context in your prompts. This substantially improves the precision and pertinence of the model’s responses. Clear directions guide the model toward the desired output, while context ensures it comprehends the nuances and particulars of the request. For instance, instead of asking, “Tell me about Python, “you could state, “Give an outline of Python programming language attributes, indulging its syntax and prevalent utilization cases.”
Adopting Agility in Model Selection
Maintain adaptability in selecting the apt LLM for your application. Evaluate LLM based on performance metrics and cost deliberations, and be ready to switch between distinct models as required. This flexibility permits you to upgrade for precise tasks, balancing elements such as response quality, dormancy and functional expenses. For example, you might utilize a more significant model for intricate queries and a cost-effective one for easier tasks.
Implementing Caching Strategies
Implement cache mechanisms to reserve and reutilize verified answers. This approach minimizes excess computations, improves response times and curtailing computational fixed costs. For FAQs or common questions, caching antecedently produced responses ensures constant and rapid delivery, enhancing user experience and system effectiveness.
Ensuring Data Privacy and PII Protection
Prioritize user data privacy by integrating sturdy gauges to determine and remove Personally Identifiable Information (PII) from communications. Use mechanical tools and algorithms to determine and unname sensitive data, protecting user privacy and following data safeguarding regulations. Implementing these measures not only to safeguard users but also to build trust in your LLM application.
Augmentation Techniques for Optimizing LLM Performance
Introduction to Prompting and Fine-Tuning
When it comes to getting the most out of Large Language Models (LLMs), two predominant methods excels: prompting and fine-tuning. Envision prompting as having a comprehensive conversation with your model–giving it precise directions to shape its reactions. On the other hand, fine-tuning is like instructing an athlete, where you process the model’s capabilities by feeding it a considered dataset to contemplate in implicit tasks.
In the industry, there is a sustained debate about which technique is better. Some dispute that prompting is more adaptable and brisk to enforce, while others believe fine-tuning leads to domineering, task-precise performance. Eventually, both techniques have their merits and can be utilized independently to upgrade your LLMs output.
Overview of Retrieval Augmented Generation (RAG)
You might have observed that sometimes LLMs, despite their enormous comprehension, can generate responses that are off the mark. That’s where Retrieval-Augmented Generation (RAG) steps in. RAG enhances the standard and pertinence of responses by incorporating external information retrieval systems with the production abilities of the LLM.
Think of RAG as giving your model ingress to a library of knowledge that it can discuss while producing responses. This hybrid approach ensures that the data your model gives is not only coherent but also precise and up-to-date.
Prompt Tuning and Distillation
Now, let’s discuss prompt tuning and distillation–two methods that help minor models punch above their weight. Immediate tuning involves adapting the model’s prompts to enhance performance on precise tasks without modifying the model’s fundamental architecture. It’s like fine-tuning but at the level of the directions to give.
Distillation, on the other hand, is a bit more intricate. It involves instructing a smaller
Model to mock the behavior of a greater, more stronger one. This procedure helps balance performance and expense, enabling you to deploy effective models without yielding too much on quality.
By utilizing immediate tuning, you can improve the abilities of smaller models without the hefty computational expenses allied with training large models. And with distillation, you accomplish similar results by transferring the knowledge from a larger model to a smaller one.
Building Scalable LLM Applications
Building scalable LLM applications need a planned approach to ensure effectiveness, dependability and safety. Here’s how you can accomplish that:
Emphasize AI Governance
AI Governance is critical when deploying LLM applications. You need to enforce human-in-the-loop systems for verification, ensuring that yields are precise and pertinent. Automated toxicity detection systems are equally significant to maintain the standard and applicability of the produced content. This dual approach helps in alleviating threats and enhancing overall performance of your LLM applications.
Key Elements for LLM Deployment at Scale
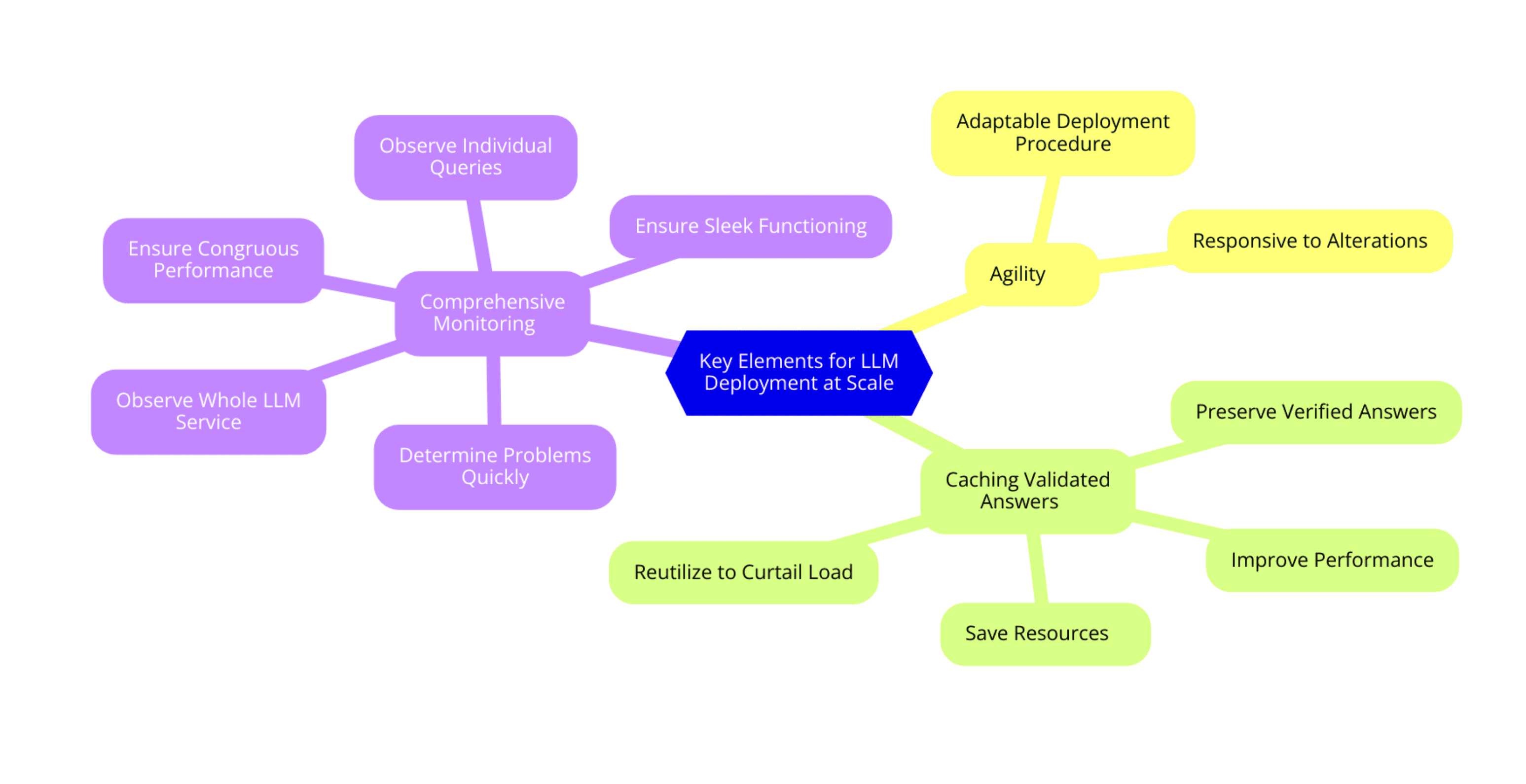
To deploy LLMs at scale efficiently, concentrate on these chief components:
Agility: Ensure your deployment procedure is adaptable and receptive to alterations. This permits you to adjust rapidly to new needs and problems.
Caching Validated Answers: Preserve and reutilize previously verified answers to curtail computational load and response times. This not only improves performance but also saves resources.
Comprehensive Monitoring: Observe both individual queries and the whole LLM service constantly. This helps in determining and figuring problems immediately, ensuring sleek functioning and congruous performance.
Introducing the LLM Mesh
The LLM Mesh is an innovative solution for accessing Generative AI models and services in a ductile and secure manner. It offers a combined structure that streamlines the incorporation and handling of numerous AI elements, ensuring sleek communication and function across distinct systems. By enforcing an LLM mesh, you improve the expandability and security of your AI applications, making it easier to deploy and handle large-scale LLM solutions.
By concentrating on these strategies, you can build sturdy and scalable LLM applications that meet your requirements proficiently and effectively.
Also Read:- Evaluating Large Language Models: Methods and Metrics
Task Composability and Control Flows in LLM Applications
Executing Tasks Sequentially, Parallelly, or Based on Conditions
When you are operating with LLM agents for multi-task apps, comprehending task enforcement is critical. You might enforce tasks successively, where one task adheres another, ideal for synopsis requiring dependent steps. On the other hand, you can run tasks in correspondence, elevating effectiveness by managing multiple functionality concurrently. Contingent enforcement (using "if" or "for" loops) lets you manage more intricate productivity, provoking precise tasks based on implicit circumstances.
Differentiating Between Tools and Plugins
It’s significant to differentiate between tools and plugins when constructing dependable LLM apps. Tools are separate utilities that offer precise usefulness, while plugins are extensions that can improve existing structures. Both are critical, but pragmatic testing of LLM agents ensures dependability. Knowing this discrepancy helps you select the right elements for your requirements, improving the soundness and efficiency of your applications.
Exploring Distinct Control Flow Types
Choosing the right control flow type can substantially affect your LLM applications performance. Sequential control flows are effortless and immaculate for tasks that adhere to a rigid order. Parallel control flows, on the other hand, permit synchronous task enforcement, saving time and resources. Contingent flows, such as “if” and “for” loops, provides the adaptability to enforce tasks based on assured circumstances or repetitions, making your apps more robust and responsive.
By comprehending and efficiently using these methods, you are better adapted to child sophisticated and effective LLM applications that meet your precise needs.
Promising Use Cases for LLMs
AI Assistant, Chatbot for Customer Service and Engagement
Envision having an AI assistant that’s attainable 24/7 to acknowledge consumer doubts and problems. LLMs can improve chatbots to provide precise and compassionate responses, enhancing customer satisfaction and engagement. Latest progressions show that AI-driven chatbots can manage up to 80% of everyday queries, extricating human support staff to intercept more intricate issues.
Code Generation: Enhancing Programming and Development
If you are a designer, you have likely desired for rapid coding solutions. LLMs such as OpenAI’s codex can comprehend natural language prompts and yields code snippets in multiple programming languages. This not only hastens the development process but also helps you grab bugs early. GitHub's Copilot, powered by Codex, has already become an indispensable tool for many developers.
Personalized Learning and Training
LLMs can act as tailored tutors, customizing lessons and study material based on your learning speed and approach. Whether you are a student or professional looking to upgrade, AI-driven platforms can offer you personalized educational content. Latest studies specify that customized learning techniques can enhance procurement rates by up to 30%.
'Talk-to-Data' for Intuitive Analysis
Envision simply asking queries or doubts about your data and receiving immediate, perceptive responses. LLMs are making this a reality by empowering ‘Talk-to-Data’ attributes in data inspection tools. Instead of writing intricate questions or doubts, you can now ask in plain English, and the AI explicates your intention, producing accurate outcomes. This inventiveness transforms how ventures and researchers engage with their information, making inspection more attainable and inherent. With such tools, regulating is time-saving, and valuable perceptions are more easily exposed.
Spotlight on RagaAI LLM Hub
If you are looking for a pragmatic platform to traverse and deploy LLMs, RagaAI LLM Hub provides a unified solution. It offers access to various generative AI models and services, ensuring you have the tools required to flawlessly incorporate LLM capabilities into your apps. With attributes designed for manageability and security, RagaAI LLM Hub is a magnificent resource for expert and experienced AI developers.
Conclusion
The world of LLM applications is revolutionizing at rapid speed, and staying ahead needs an accurate deployment plan. From composing efficient prompts to integrating progressed methods like RAG and fine-tuning, the expedition to deployable LLM applications can be intricate but extremely fruitful.
As you outlook that phase, remember that adaptability, sturdy governance, and constant learning are your best supporters. Whether you are improving customer assistance, generating advanced analytics, or developing inventive AI products, deploying LLMs efficiently will be a groundbreaker for your undertakings.
Stay interested, stay supple, and keep propelling the boundaries of what’s achievable with LLMs. Happy deploying!
The prospect of machine learning is swiftly emerging, and at the vanguard of this revolution are large language models (LLMs). These prominent tools are revisiting what’s feasible with Natural Language Processing (NLP), from producing human-like text to providing intuitive customer service communications. But while the potential of LLMs is enormous, transforming from a demonstration of the concept of a deployable application is a formidable challenge.
Deploying LLMs in production involves a quirky set of obstacles, equating a requirement for efficient augmentation techniques such as prompting v/s fine-tuning, handling delayed data transfer, and ensuring data integrity and seclusion. This guide will cover sturdy plans and practical tips to make the journey sleeker and more effective.
Challenges of Deploying LLMs in Production
Ambiguity and Inconsistency in Outputs
Natural Language is fundamentally vague, leading to inconsistent yields from LLMs. This inconsistency makes immediate assessment and upgradation critical. You need to give time to processing prompts and examining numerous synopses to ensure steady performance. Frequently updating and repeating your prompts can help alleviate unanticipated or incorrect responses.
Cost and Latency Considerations
Cost and Latency are substantial components prompting deployment decisions. The length of prompts and yields directly affect these elements. You must balance these distinct approaches, such as prompting, fine-tuning, or both. Fine-tuning can offer more congruous outcomes but might indulge higher upfront costs. Prompt engineering can be economical initially but may need ongoing adaptations to maintain performance.
High Latency and Inference Costs
High latency and inference costs present challenges for full-stack engineering teams. LLMs need considerable arithmetic resources, resulting in slower reply times and increased functional expenses. You should traverse upgrade methods like model distillation, thresholding, or using more effective architectures to curtail these loads. Enforcing cache plans for regularly demanding outputs can also help enhance latency.
Significance of Data Quality and Privacy
Data Quality substantially affects the performance of LLMs. High-quality, disparate datasets lead to better model productivity. You need to pioneer sturdy data gathering and cleaning procedures to ensure the training data is delegated and precise. Model selection and assessing plans are equally significant. Frequently gauge your models against industry standards and constantly observe their performance in production.
Privacy deliberations are paramount when handling user data. To protect sensitive data, comply with data protection regulations and enforce powerful security measures. Methods such as differential privacy and consolidated learning can help improve privacy while maintaining the model's performance.
Addressing these challenges can help you effectively deploy LLMs in production, utilize their full potential, and maintain effectiveness and dependability.
Best Practices for LLM Deployment
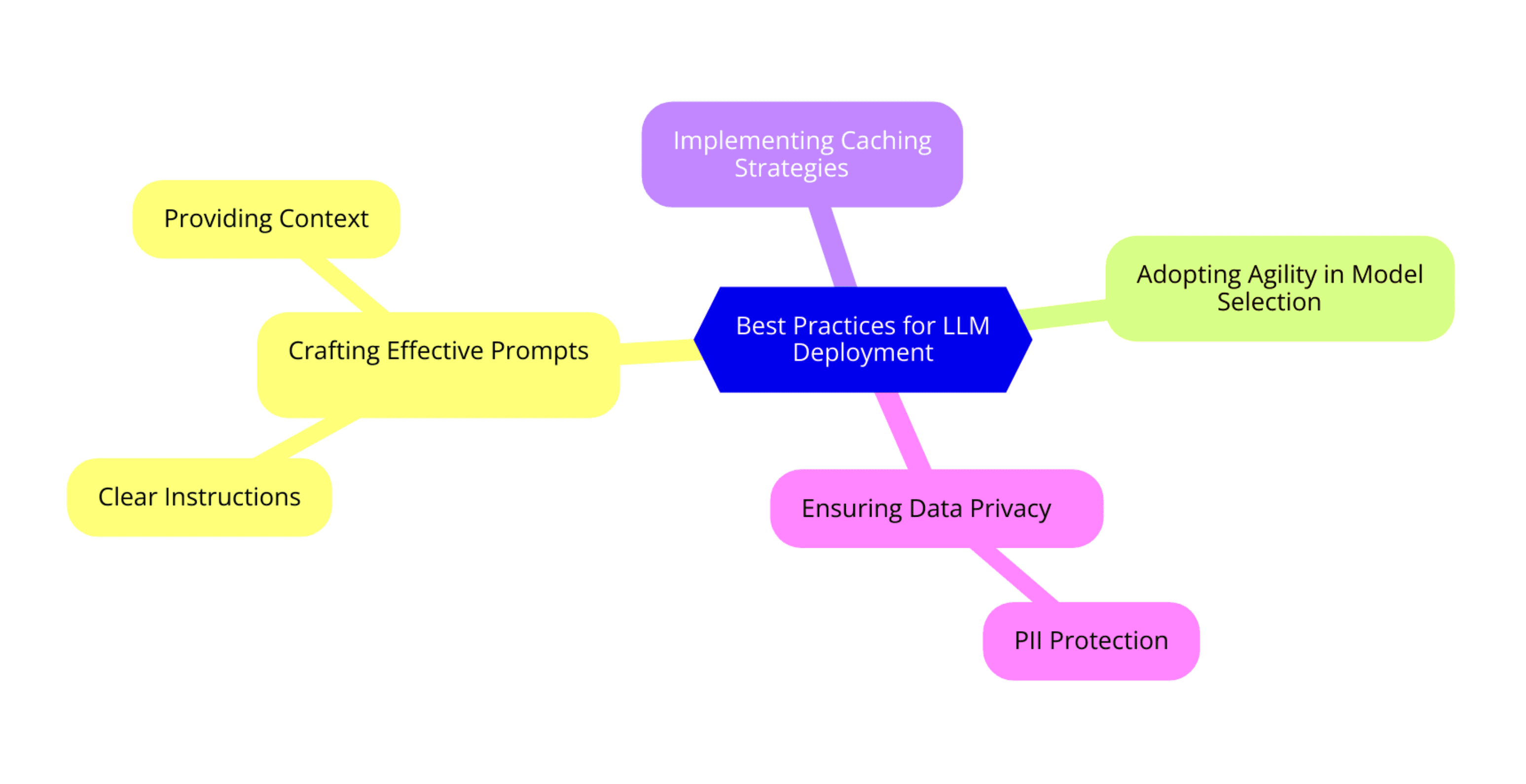
Crafting Effective Prompts with Clear Instructions and Context
When deploying a large language model (LLM), focus on giving transparent directions and pertinent context in your prompts. This substantially improves the precision and pertinence of the model’s responses. Clear directions guide the model toward the desired output, while context ensures it comprehends the nuances and particulars of the request. For instance, instead of asking, “Tell me about Python, “you could state, “Give an outline of Python programming language attributes, indulging its syntax and prevalent utilization cases.”
Adopting Agility in Model Selection
Maintain adaptability in selecting the apt LLM for your application. Evaluate LLM based on performance metrics and cost deliberations, and be ready to switch between distinct models as required. This flexibility permits you to upgrade for precise tasks, balancing elements such as response quality, dormancy and functional expenses. For example, you might utilize a more significant model for intricate queries and a cost-effective one for easier tasks.
Implementing Caching Strategies
Implement cache mechanisms to reserve and reutilize verified answers. This approach minimizes excess computations, improves response times and curtailing computational fixed costs. For FAQs or common questions, caching antecedently produced responses ensures constant and rapid delivery, enhancing user experience and system effectiveness.
Ensuring Data Privacy and PII Protection
Prioritize user data privacy by integrating sturdy gauges to determine and remove Personally Identifiable Information (PII) from communications. Use mechanical tools and algorithms to determine and unname sensitive data, protecting user privacy and following data safeguarding regulations. Implementing these measures not only to safeguard users but also to build trust in your LLM application.
Augmentation Techniques for Optimizing LLM Performance
Introduction to Prompting and Fine-Tuning
When it comes to getting the most out of Large Language Models (LLMs), two predominant methods excels: prompting and fine-tuning. Envision prompting as having a comprehensive conversation with your model–giving it precise directions to shape its reactions. On the other hand, fine-tuning is like instructing an athlete, where you process the model’s capabilities by feeding it a considered dataset to contemplate in implicit tasks.
In the industry, there is a sustained debate about which technique is better. Some dispute that prompting is more adaptable and brisk to enforce, while others believe fine-tuning leads to domineering, task-precise performance. Eventually, both techniques have their merits and can be utilized independently to upgrade your LLMs output.
Overview of Retrieval Augmented Generation (RAG)
You might have observed that sometimes LLMs, despite their enormous comprehension, can generate responses that are off the mark. That’s where Retrieval-Augmented Generation (RAG) steps in. RAG enhances the standard and pertinence of responses by incorporating external information retrieval systems with the production abilities of the LLM.
Think of RAG as giving your model ingress to a library of knowledge that it can discuss while producing responses. This hybrid approach ensures that the data your model gives is not only coherent but also precise and up-to-date.
Prompt Tuning and Distillation
Now, let’s discuss prompt tuning and distillation–two methods that help minor models punch above their weight. Immediate tuning involves adapting the model’s prompts to enhance performance on precise tasks without modifying the model’s fundamental architecture. It’s like fine-tuning but at the level of the directions to give.
Distillation, on the other hand, is a bit more intricate. It involves instructing a smaller
Model to mock the behavior of a greater, more stronger one. This procedure helps balance performance and expense, enabling you to deploy effective models without yielding too much on quality.
By utilizing immediate tuning, you can improve the abilities of smaller models without the hefty computational expenses allied with training large models. And with distillation, you accomplish similar results by transferring the knowledge from a larger model to a smaller one.
Building Scalable LLM Applications
Building scalable LLM applications need a planned approach to ensure effectiveness, dependability and safety. Here’s how you can accomplish that:
Emphasize AI Governance
AI Governance is critical when deploying LLM applications. You need to enforce human-in-the-loop systems for verification, ensuring that yields are precise and pertinent. Automated toxicity detection systems are equally significant to maintain the standard and applicability of the produced content. This dual approach helps in alleviating threats and enhancing overall performance of your LLM applications.
Key Elements for LLM Deployment at Scale
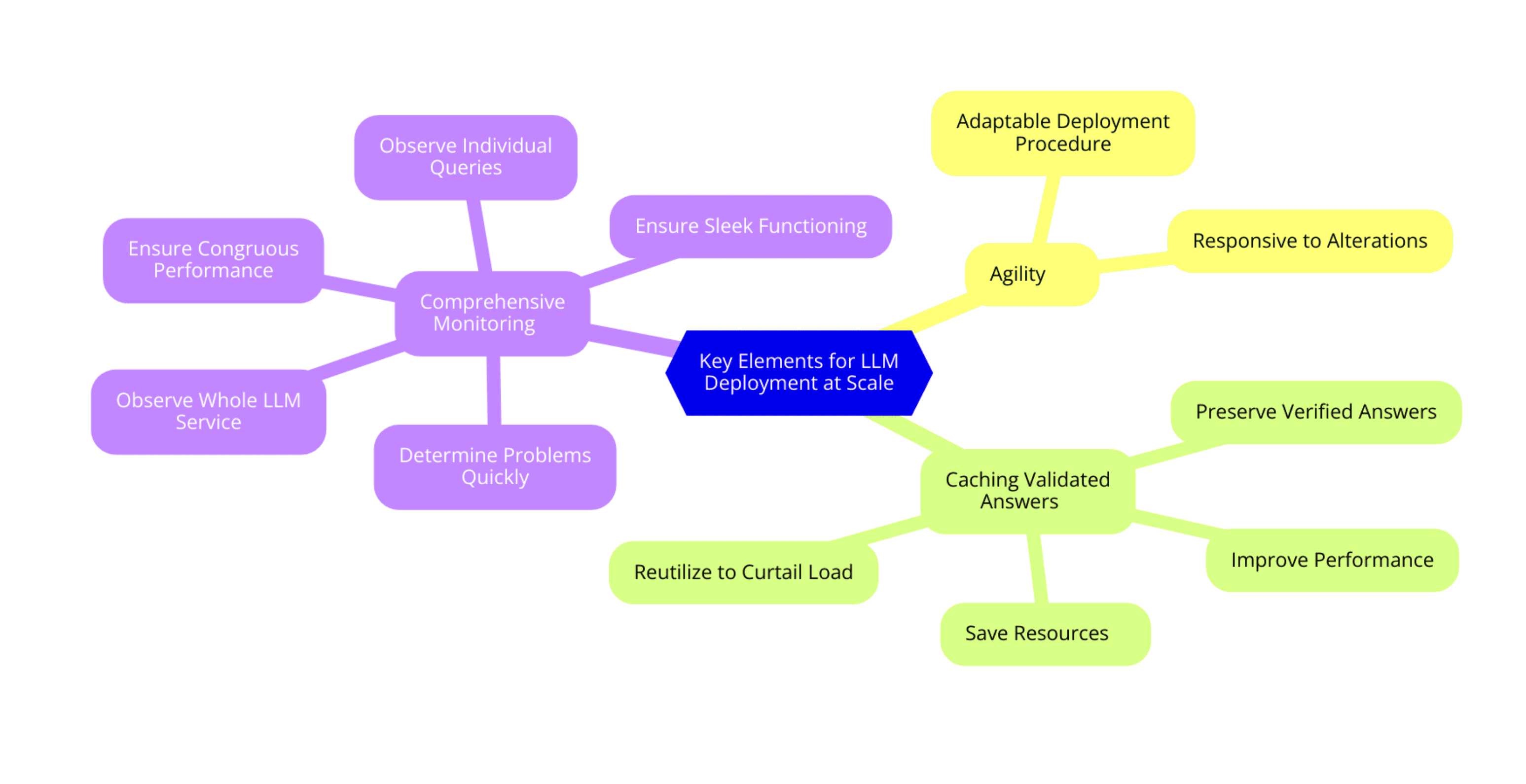
To deploy LLMs at scale efficiently, concentrate on these chief components:
Agility: Ensure your deployment procedure is adaptable and receptive to alterations. This permits you to adjust rapidly to new needs and problems.
Caching Validated Answers: Preserve and reutilize previously verified answers to curtail computational load and response times. This not only improves performance but also saves resources.
Comprehensive Monitoring: Observe both individual queries and the whole LLM service constantly. This helps in determining and figuring problems immediately, ensuring sleek functioning and congruous performance.
Introducing the LLM Mesh
The LLM Mesh is an innovative solution for accessing Generative AI models and services in a ductile and secure manner. It offers a combined structure that streamlines the incorporation and handling of numerous AI elements, ensuring sleek communication and function across distinct systems. By enforcing an LLM mesh, you improve the expandability and security of your AI applications, making it easier to deploy and handle large-scale LLM solutions.
By concentrating on these strategies, you can build sturdy and scalable LLM applications that meet your requirements proficiently and effectively.
Also Read:- Evaluating Large Language Models: Methods and Metrics
Task Composability and Control Flows in LLM Applications
Executing Tasks Sequentially, Parallelly, or Based on Conditions
When you are operating with LLM agents for multi-task apps, comprehending task enforcement is critical. You might enforce tasks successively, where one task adheres another, ideal for synopsis requiring dependent steps. On the other hand, you can run tasks in correspondence, elevating effectiveness by managing multiple functionality concurrently. Contingent enforcement (using "if" or "for" loops) lets you manage more intricate productivity, provoking precise tasks based on implicit circumstances.
Differentiating Between Tools and Plugins
It’s significant to differentiate between tools and plugins when constructing dependable LLM apps. Tools are separate utilities that offer precise usefulness, while plugins are extensions that can improve existing structures. Both are critical, but pragmatic testing of LLM agents ensures dependability. Knowing this discrepancy helps you select the right elements for your requirements, improving the soundness and efficiency of your applications.
Exploring Distinct Control Flow Types
Choosing the right control flow type can substantially affect your LLM applications performance. Sequential control flows are effortless and immaculate for tasks that adhere to a rigid order. Parallel control flows, on the other hand, permit synchronous task enforcement, saving time and resources. Contingent flows, such as “if” and “for” loops, provides the adaptability to enforce tasks based on assured circumstances or repetitions, making your apps more robust and responsive.
By comprehending and efficiently using these methods, you are better adapted to child sophisticated and effective LLM applications that meet your precise needs.
Promising Use Cases for LLMs
AI Assistant, Chatbot for Customer Service and Engagement
Envision having an AI assistant that’s attainable 24/7 to acknowledge consumer doubts and problems. LLMs can improve chatbots to provide precise and compassionate responses, enhancing customer satisfaction and engagement. Latest progressions show that AI-driven chatbots can manage up to 80% of everyday queries, extricating human support staff to intercept more intricate issues.
Code Generation: Enhancing Programming and Development
If you are a designer, you have likely desired for rapid coding solutions. LLMs such as OpenAI’s codex can comprehend natural language prompts and yields code snippets in multiple programming languages. This not only hastens the development process but also helps you grab bugs early. GitHub's Copilot, powered by Codex, has already become an indispensable tool for many developers.
Personalized Learning and Training
LLMs can act as tailored tutors, customizing lessons and study material based on your learning speed and approach. Whether you are a student or professional looking to upgrade, AI-driven platforms can offer you personalized educational content. Latest studies specify that customized learning techniques can enhance procurement rates by up to 30%.
'Talk-to-Data' for Intuitive Analysis
Envision simply asking queries or doubts about your data and receiving immediate, perceptive responses. LLMs are making this a reality by empowering ‘Talk-to-Data’ attributes in data inspection tools. Instead of writing intricate questions or doubts, you can now ask in plain English, and the AI explicates your intention, producing accurate outcomes. This inventiveness transforms how ventures and researchers engage with their information, making inspection more attainable and inherent. With such tools, regulating is time-saving, and valuable perceptions are more easily exposed.
Spotlight on RagaAI LLM Hub
If you are looking for a pragmatic platform to traverse and deploy LLMs, RagaAI LLM Hub provides a unified solution. It offers access to various generative AI models and services, ensuring you have the tools required to flawlessly incorporate LLM capabilities into your apps. With attributes designed for manageability and security, RagaAI LLM Hub is a magnificent resource for expert and experienced AI developers.
Conclusion
The world of LLM applications is revolutionizing at rapid speed, and staying ahead needs an accurate deployment plan. From composing efficient prompts to integrating progressed methods like RAG and fine-tuning, the expedition to deployable LLM applications can be intricate but extremely fruitful.
As you outlook that phase, remember that adaptability, sturdy governance, and constant learning are your best supporters. Whether you are improving customer assistance, generating advanced analytics, or developing inventive AI products, deploying LLMs efficiently will be a groundbreaker for your undertakings.
Stay interested, stay supple, and keep propelling the boundaries of what’s achievable with LLMs. Happy deploying!
The prospect of machine learning is swiftly emerging, and at the vanguard of this revolution are large language models (LLMs). These prominent tools are revisiting what’s feasible with Natural Language Processing (NLP), from producing human-like text to providing intuitive customer service communications. But while the potential of LLMs is enormous, transforming from a demonstration of the concept of a deployable application is a formidable challenge.
Deploying LLMs in production involves a quirky set of obstacles, equating a requirement for efficient augmentation techniques such as prompting v/s fine-tuning, handling delayed data transfer, and ensuring data integrity and seclusion. This guide will cover sturdy plans and practical tips to make the journey sleeker and more effective.
Challenges of Deploying LLMs in Production
Ambiguity and Inconsistency in Outputs
Natural Language is fundamentally vague, leading to inconsistent yields from LLMs. This inconsistency makes immediate assessment and upgradation critical. You need to give time to processing prompts and examining numerous synopses to ensure steady performance. Frequently updating and repeating your prompts can help alleviate unanticipated or incorrect responses.
Cost and Latency Considerations
Cost and Latency are substantial components prompting deployment decisions. The length of prompts and yields directly affect these elements. You must balance these distinct approaches, such as prompting, fine-tuning, or both. Fine-tuning can offer more congruous outcomes but might indulge higher upfront costs. Prompt engineering can be economical initially but may need ongoing adaptations to maintain performance.
High Latency and Inference Costs
High latency and inference costs present challenges for full-stack engineering teams. LLMs need considerable arithmetic resources, resulting in slower reply times and increased functional expenses. You should traverse upgrade methods like model distillation, thresholding, or using more effective architectures to curtail these loads. Enforcing cache plans for regularly demanding outputs can also help enhance latency.
Significance of Data Quality and Privacy
Data Quality substantially affects the performance of LLMs. High-quality, disparate datasets lead to better model productivity. You need to pioneer sturdy data gathering and cleaning procedures to ensure the training data is delegated and precise. Model selection and assessing plans are equally significant. Frequently gauge your models against industry standards and constantly observe their performance in production.
Privacy deliberations are paramount when handling user data. To protect sensitive data, comply with data protection regulations and enforce powerful security measures. Methods such as differential privacy and consolidated learning can help improve privacy while maintaining the model's performance.
Addressing these challenges can help you effectively deploy LLMs in production, utilize their full potential, and maintain effectiveness and dependability.
Best Practices for LLM Deployment
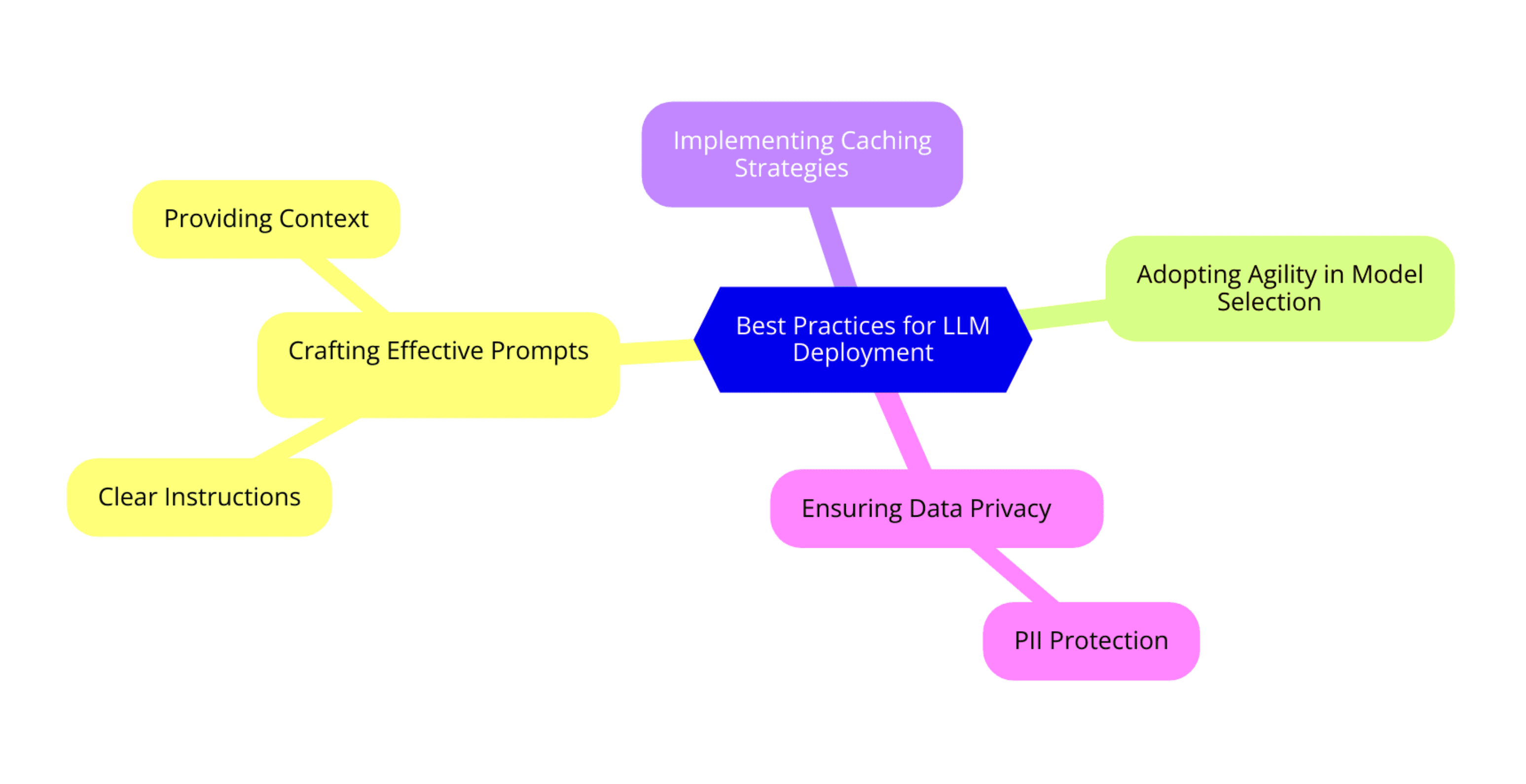
Crafting Effective Prompts with Clear Instructions and Context
When deploying a large language model (LLM), focus on giving transparent directions and pertinent context in your prompts. This substantially improves the precision and pertinence of the model’s responses. Clear directions guide the model toward the desired output, while context ensures it comprehends the nuances and particulars of the request. For instance, instead of asking, “Tell me about Python, “you could state, “Give an outline of Python programming language attributes, indulging its syntax and prevalent utilization cases.”
Adopting Agility in Model Selection
Maintain adaptability in selecting the apt LLM for your application. Evaluate LLM based on performance metrics and cost deliberations, and be ready to switch between distinct models as required. This flexibility permits you to upgrade for precise tasks, balancing elements such as response quality, dormancy and functional expenses. For example, you might utilize a more significant model for intricate queries and a cost-effective one for easier tasks.
Implementing Caching Strategies
Implement cache mechanisms to reserve and reutilize verified answers. This approach minimizes excess computations, improves response times and curtailing computational fixed costs. For FAQs or common questions, caching antecedently produced responses ensures constant and rapid delivery, enhancing user experience and system effectiveness.
Ensuring Data Privacy and PII Protection
Prioritize user data privacy by integrating sturdy gauges to determine and remove Personally Identifiable Information (PII) from communications. Use mechanical tools and algorithms to determine and unname sensitive data, protecting user privacy and following data safeguarding regulations. Implementing these measures not only to safeguard users but also to build trust in your LLM application.
Augmentation Techniques for Optimizing LLM Performance
Introduction to Prompting and Fine-Tuning
When it comes to getting the most out of Large Language Models (LLMs), two predominant methods excels: prompting and fine-tuning. Envision prompting as having a comprehensive conversation with your model–giving it precise directions to shape its reactions. On the other hand, fine-tuning is like instructing an athlete, where you process the model’s capabilities by feeding it a considered dataset to contemplate in implicit tasks.
In the industry, there is a sustained debate about which technique is better. Some dispute that prompting is more adaptable and brisk to enforce, while others believe fine-tuning leads to domineering, task-precise performance. Eventually, both techniques have their merits and can be utilized independently to upgrade your LLMs output.
Overview of Retrieval Augmented Generation (RAG)
You might have observed that sometimes LLMs, despite their enormous comprehension, can generate responses that are off the mark. That’s where Retrieval-Augmented Generation (RAG) steps in. RAG enhances the standard and pertinence of responses by incorporating external information retrieval systems with the production abilities of the LLM.
Think of RAG as giving your model ingress to a library of knowledge that it can discuss while producing responses. This hybrid approach ensures that the data your model gives is not only coherent but also precise and up-to-date.
Prompt Tuning and Distillation
Now, let’s discuss prompt tuning and distillation–two methods that help minor models punch above their weight. Immediate tuning involves adapting the model’s prompts to enhance performance on precise tasks without modifying the model’s fundamental architecture. It’s like fine-tuning but at the level of the directions to give.
Distillation, on the other hand, is a bit more intricate. It involves instructing a smaller
Model to mock the behavior of a greater, more stronger one. This procedure helps balance performance and expense, enabling you to deploy effective models without yielding too much on quality.
By utilizing immediate tuning, you can improve the abilities of smaller models without the hefty computational expenses allied with training large models. And with distillation, you accomplish similar results by transferring the knowledge from a larger model to a smaller one.
Building Scalable LLM Applications
Building scalable LLM applications need a planned approach to ensure effectiveness, dependability and safety. Here’s how you can accomplish that:
Emphasize AI Governance
AI Governance is critical when deploying LLM applications. You need to enforce human-in-the-loop systems for verification, ensuring that yields are precise and pertinent. Automated toxicity detection systems are equally significant to maintain the standard and applicability of the produced content. This dual approach helps in alleviating threats and enhancing overall performance of your LLM applications.
Key Elements for LLM Deployment at Scale
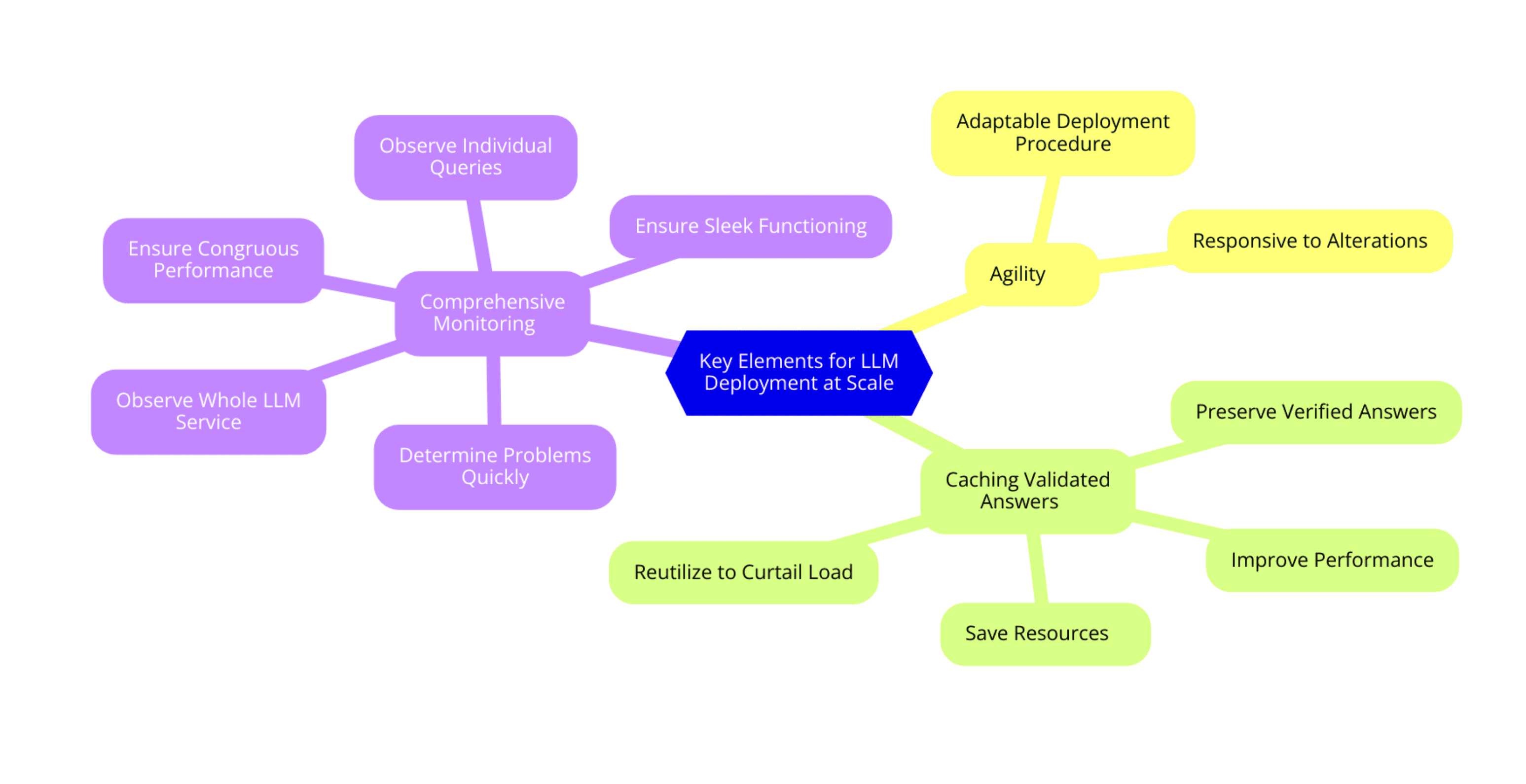
To deploy LLMs at scale efficiently, concentrate on these chief components:
Agility: Ensure your deployment procedure is adaptable and receptive to alterations. This permits you to adjust rapidly to new needs and problems.
Caching Validated Answers: Preserve and reutilize previously verified answers to curtail computational load and response times. This not only improves performance but also saves resources.
Comprehensive Monitoring: Observe both individual queries and the whole LLM service constantly. This helps in determining and figuring problems immediately, ensuring sleek functioning and congruous performance.
Introducing the LLM Mesh
The LLM Mesh is an innovative solution for accessing Generative AI models and services in a ductile and secure manner. It offers a combined structure that streamlines the incorporation and handling of numerous AI elements, ensuring sleek communication and function across distinct systems. By enforcing an LLM mesh, you improve the expandability and security of your AI applications, making it easier to deploy and handle large-scale LLM solutions.
By concentrating on these strategies, you can build sturdy and scalable LLM applications that meet your requirements proficiently and effectively.
Also Read:- Evaluating Large Language Models: Methods and Metrics
Task Composability and Control Flows in LLM Applications
Executing Tasks Sequentially, Parallelly, or Based on Conditions
When you are operating with LLM agents for multi-task apps, comprehending task enforcement is critical. You might enforce tasks successively, where one task adheres another, ideal for synopsis requiring dependent steps. On the other hand, you can run tasks in correspondence, elevating effectiveness by managing multiple functionality concurrently. Contingent enforcement (using "if" or "for" loops) lets you manage more intricate productivity, provoking precise tasks based on implicit circumstances.
Differentiating Between Tools and Plugins
It’s significant to differentiate between tools and plugins when constructing dependable LLM apps. Tools are separate utilities that offer precise usefulness, while plugins are extensions that can improve existing structures. Both are critical, but pragmatic testing of LLM agents ensures dependability. Knowing this discrepancy helps you select the right elements for your requirements, improving the soundness and efficiency of your applications.
Exploring Distinct Control Flow Types
Choosing the right control flow type can substantially affect your LLM applications performance. Sequential control flows are effortless and immaculate for tasks that adhere to a rigid order. Parallel control flows, on the other hand, permit synchronous task enforcement, saving time and resources. Contingent flows, such as “if” and “for” loops, provides the adaptability to enforce tasks based on assured circumstances or repetitions, making your apps more robust and responsive.
By comprehending and efficiently using these methods, you are better adapted to child sophisticated and effective LLM applications that meet your precise needs.
Promising Use Cases for LLMs
AI Assistant, Chatbot for Customer Service and Engagement
Envision having an AI assistant that’s attainable 24/7 to acknowledge consumer doubts and problems. LLMs can improve chatbots to provide precise and compassionate responses, enhancing customer satisfaction and engagement. Latest progressions show that AI-driven chatbots can manage up to 80% of everyday queries, extricating human support staff to intercept more intricate issues.
Code Generation: Enhancing Programming and Development
If you are a designer, you have likely desired for rapid coding solutions. LLMs such as OpenAI’s codex can comprehend natural language prompts and yields code snippets in multiple programming languages. This not only hastens the development process but also helps you grab bugs early. GitHub's Copilot, powered by Codex, has already become an indispensable tool for many developers.
Personalized Learning and Training
LLMs can act as tailored tutors, customizing lessons and study material based on your learning speed and approach. Whether you are a student or professional looking to upgrade, AI-driven platforms can offer you personalized educational content. Latest studies specify that customized learning techniques can enhance procurement rates by up to 30%.
'Talk-to-Data' for Intuitive Analysis
Envision simply asking queries or doubts about your data and receiving immediate, perceptive responses. LLMs are making this a reality by empowering ‘Talk-to-Data’ attributes in data inspection tools. Instead of writing intricate questions or doubts, you can now ask in plain English, and the AI explicates your intention, producing accurate outcomes. This inventiveness transforms how ventures and researchers engage with their information, making inspection more attainable and inherent. With such tools, regulating is time-saving, and valuable perceptions are more easily exposed.
Spotlight on RagaAI LLM Hub
If you are looking for a pragmatic platform to traverse and deploy LLMs, RagaAI LLM Hub provides a unified solution. It offers access to various generative AI models and services, ensuring you have the tools required to flawlessly incorporate LLM capabilities into your apps. With attributes designed for manageability and security, RagaAI LLM Hub is a magnificent resource for expert and experienced AI developers.
Conclusion
The world of LLM applications is revolutionizing at rapid speed, and staying ahead needs an accurate deployment plan. From composing efficient prompts to integrating progressed methods like RAG and fine-tuning, the expedition to deployable LLM applications can be intricate but extremely fruitful.
As you outlook that phase, remember that adaptability, sturdy governance, and constant learning are your best supporters. Whether you are improving customer assistance, generating advanced analytics, or developing inventive AI products, deploying LLMs efficiently will be a groundbreaker for your undertakings.
Stay interested, stay supple, and keep propelling the boundaries of what’s achievable with LLMs. Happy deploying!
The prospect of machine learning is swiftly emerging, and at the vanguard of this revolution are large language models (LLMs). These prominent tools are revisiting what’s feasible with Natural Language Processing (NLP), from producing human-like text to providing intuitive customer service communications. But while the potential of LLMs is enormous, transforming from a demonstration of the concept of a deployable application is a formidable challenge.
Deploying LLMs in production involves a quirky set of obstacles, equating a requirement for efficient augmentation techniques such as prompting v/s fine-tuning, handling delayed data transfer, and ensuring data integrity and seclusion. This guide will cover sturdy plans and practical tips to make the journey sleeker and more effective.
Challenges of Deploying LLMs in Production
Ambiguity and Inconsistency in Outputs
Natural Language is fundamentally vague, leading to inconsistent yields from LLMs. This inconsistency makes immediate assessment and upgradation critical. You need to give time to processing prompts and examining numerous synopses to ensure steady performance. Frequently updating and repeating your prompts can help alleviate unanticipated or incorrect responses.
Cost and Latency Considerations
Cost and Latency are substantial components prompting deployment decisions. The length of prompts and yields directly affect these elements. You must balance these distinct approaches, such as prompting, fine-tuning, or both. Fine-tuning can offer more congruous outcomes but might indulge higher upfront costs. Prompt engineering can be economical initially but may need ongoing adaptations to maintain performance.
High Latency and Inference Costs
High latency and inference costs present challenges for full-stack engineering teams. LLMs need considerable arithmetic resources, resulting in slower reply times and increased functional expenses. You should traverse upgrade methods like model distillation, thresholding, or using more effective architectures to curtail these loads. Enforcing cache plans for regularly demanding outputs can also help enhance latency.
Significance of Data Quality and Privacy
Data Quality substantially affects the performance of LLMs. High-quality, disparate datasets lead to better model productivity. You need to pioneer sturdy data gathering and cleaning procedures to ensure the training data is delegated and precise. Model selection and assessing plans are equally significant. Frequently gauge your models against industry standards and constantly observe their performance in production.
Privacy deliberations are paramount when handling user data. To protect sensitive data, comply with data protection regulations and enforce powerful security measures. Methods such as differential privacy and consolidated learning can help improve privacy while maintaining the model's performance.
Addressing these challenges can help you effectively deploy LLMs in production, utilize their full potential, and maintain effectiveness and dependability.
Best Practices for LLM Deployment
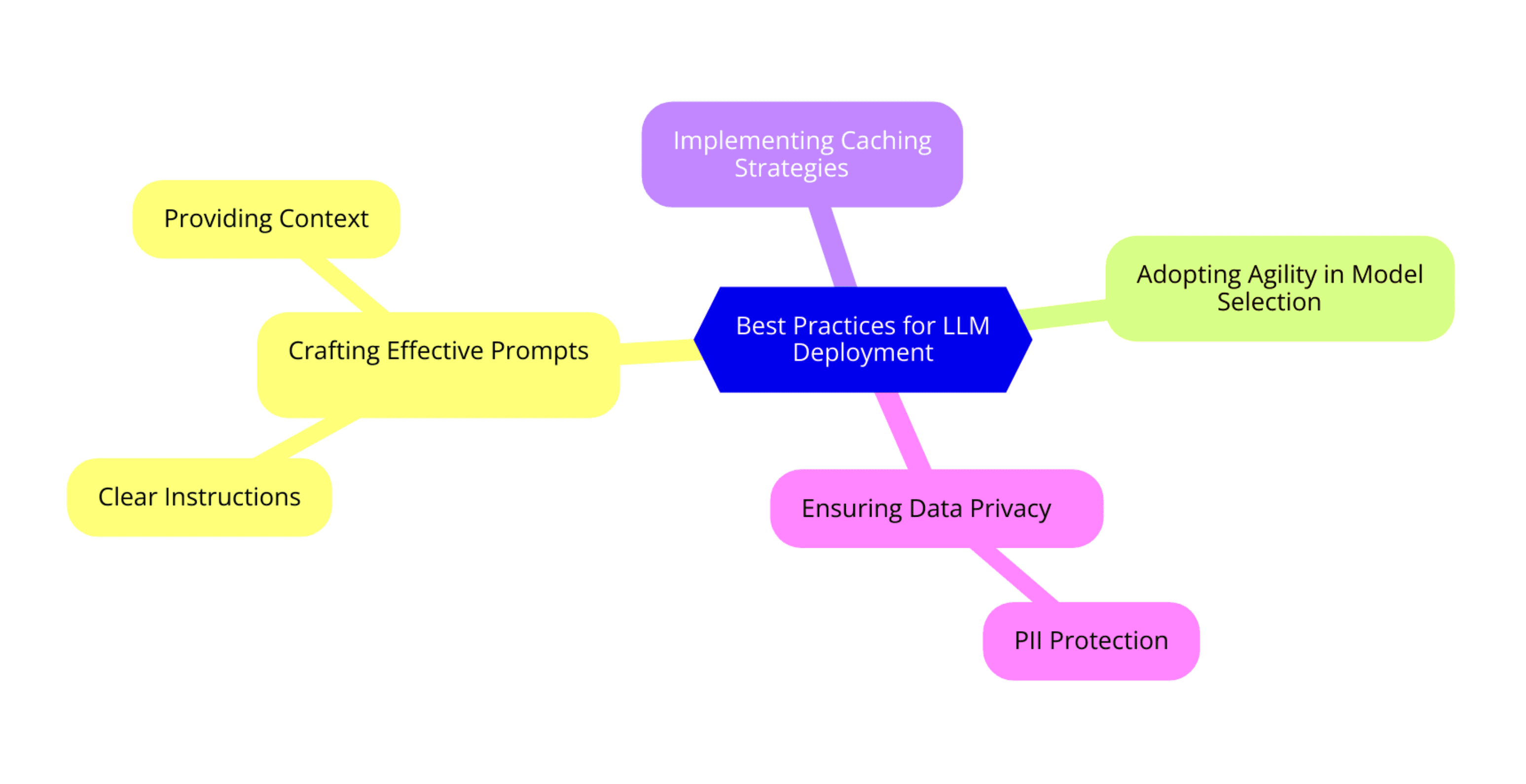
Crafting Effective Prompts with Clear Instructions and Context
When deploying a large language model (LLM), focus on giving transparent directions and pertinent context in your prompts. This substantially improves the precision and pertinence of the model’s responses. Clear directions guide the model toward the desired output, while context ensures it comprehends the nuances and particulars of the request. For instance, instead of asking, “Tell me about Python, “you could state, “Give an outline of Python programming language attributes, indulging its syntax and prevalent utilization cases.”
Adopting Agility in Model Selection
Maintain adaptability in selecting the apt LLM for your application. Evaluate LLM based on performance metrics and cost deliberations, and be ready to switch between distinct models as required. This flexibility permits you to upgrade for precise tasks, balancing elements such as response quality, dormancy and functional expenses. For example, you might utilize a more significant model for intricate queries and a cost-effective one for easier tasks.
Implementing Caching Strategies
Implement cache mechanisms to reserve and reutilize verified answers. This approach minimizes excess computations, improves response times and curtailing computational fixed costs. For FAQs or common questions, caching antecedently produced responses ensures constant and rapid delivery, enhancing user experience and system effectiveness.
Ensuring Data Privacy and PII Protection
Prioritize user data privacy by integrating sturdy gauges to determine and remove Personally Identifiable Information (PII) from communications. Use mechanical tools and algorithms to determine and unname sensitive data, protecting user privacy and following data safeguarding regulations. Implementing these measures not only to safeguard users but also to build trust in your LLM application.
Augmentation Techniques for Optimizing LLM Performance
Introduction to Prompting and Fine-Tuning
When it comes to getting the most out of Large Language Models (LLMs), two predominant methods excels: prompting and fine-tuning. Envision prompting as having a comprehensive conversation with your model–giving it precise directions to shape its reactions. On the other hand, fine-tuning is like instructing an athlete, where you process the model’s capabilities by feeding it a considered dataset to contemplate in implicit tasks.
In the industry, there is a sustained debate about which technique is better. Some dispute that prompting is more adaptable and brisk to enforce, while others believe fine-tuning leads to domineering, task-precise performance. Eventually, both techniques have their merits and can be utilized independently to upgrade your LLMs output.
Overview of Retrieval Augmented Generation (RAG)
You might have observed that sometimes LLMs, despite their enormous comprehension, can generate responses that are off the mark. That’s where Retrieval-Augmented Generation (RAG) steps in. RAG enhances the standard and pertinence of responses by incorporating external information retrieval systems with the production abilities of the LLM.
Think of RAG as giving your model ingress to a library of knowledge that it can discuss while producing responses. This hybrid approach ensures that the data your model gives is not only coherent but also precise and up-to-date.
Prompt Tuning and Distillation
Now, let’s discuss prompt tuning and distillation–two methods that help minor models punch above their weight. Immediate tuning involves adapting the model’s prompts to enhance performance on precise tasks without modifying the model’s fundamental architecture. It’s like fine-tuning but at the level of the directions to give.
Distillation, on the other hand, is a bit more intricate. It involves instructing a smaller
Model to mock the behavior of a greater, more stronger one. This procedure helps balance performance and expense, enabling you to deploy effective models without yielding too much on quality.
By utilizing immediate tuning, you can improve the abilities of smaller models without the hefty computational expenses allied with training large models. And with distillation, you accomplish similar results by transferring the knowledge from a larger model to a smaller one.
Building Scalable LLM Applications
Building scalable LLM applications need a planned approach to ensure effectiveness, dependability and safety. Here’s how you can accomplish that:
Emphasize AI Governance
AI Governance is critical when deploying LLM applications. You need to enforce human-in-the-loop systems for verification, ensuring that yields are precise and pertinent. Automated toxicity detection systems are equally significant to maintain the standard and applicability of the produced content. This dual approach helps in alleviating threats and enhancing overall performance of your LLM applications.
Key Elements for LLM Deployment at Scale
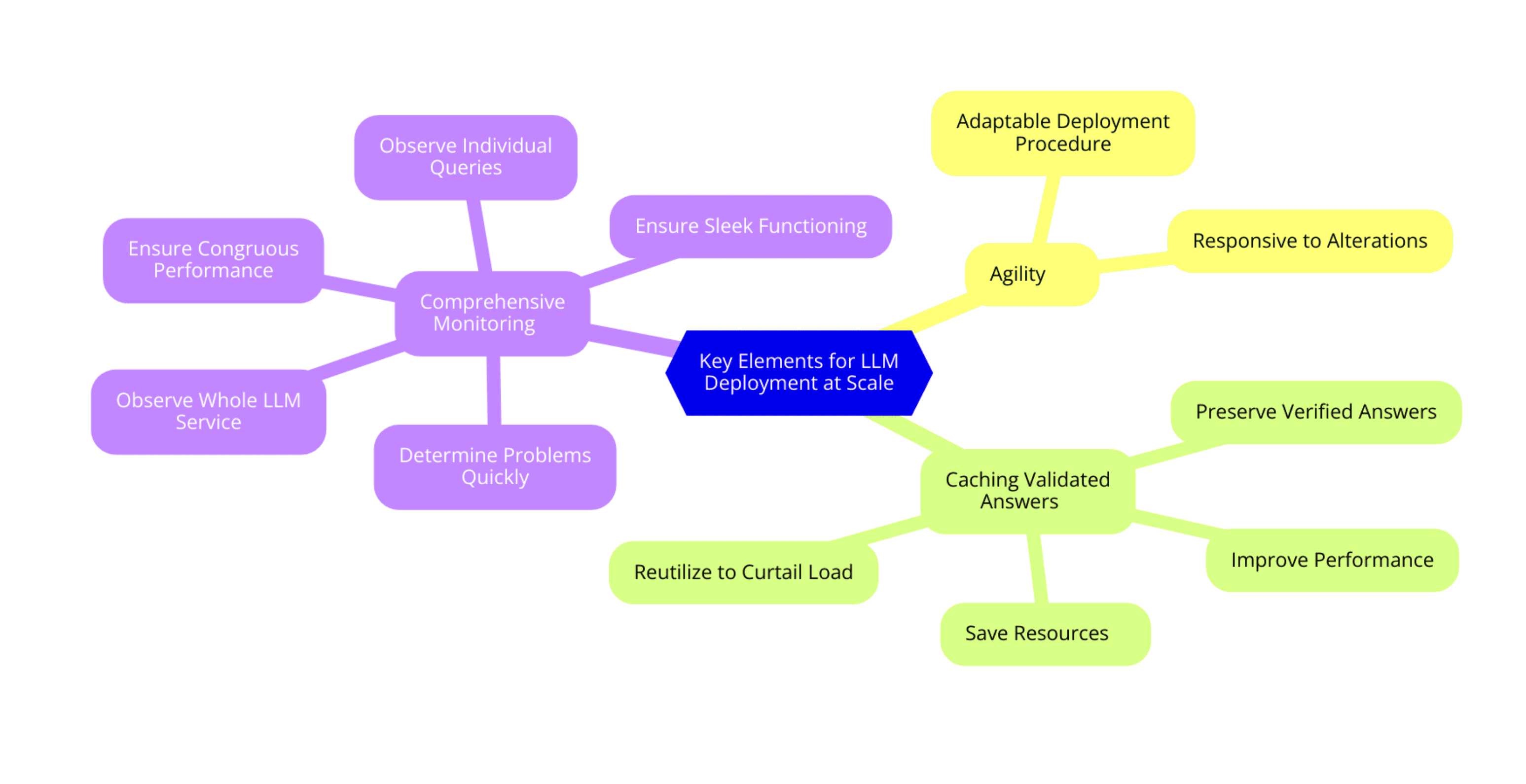
To deploy LLMs at scale efficiently, concentrate on these chief components:
Agility: Ensure your deployment procedure is adaptable and receptive to alterations. This permits you to adjust rapidly to new needs and problems.
Caching Validated Answers: Preserve and reutilize previously verified answers to curtail computational load and response times. This not only improves performance but also saves resources.
Comprehensive Monitoring: Observe both individual queries and the whole LLM service constantly. This helps in determining and figuring problems immediately, ensuring sleek functioning and congruous performance.
Introducing the LLM Mesh
The LLM Mesh is an innovative solution for accessing Generative AI models and services in a ductile and secure manner. It offers a combined structure that streamlines the incorporation and handling of numerous AI elements, ensuring sleek communication and function across distinct systems. By enforcing an LLM mesh, you improve the expandability and security of your AI applications, making it easier to deploy and handle large-scale LLM solutions.
By concentrating on these strategies, you can build sturdy and scalable LLM applications that meet your requirements proficiently and effectively.
Also Read:- Evaluating Large Language Models: Methods and Metrics
Task Composability and Control Flows in LLM Applications
Executing Tasks Sequentially, Parallelly, or Based on Conditions
When you are operating with LLM agents for multi-task apps, comprehending task enforcement is critical. You might enforce tasks successively, where one task adheres another, ideal for synopsis requiring dependent steps. On the other hand, you can run tasks in correspondence, elevating effectiveness by managing multiple functionality concurrently. Contingent enforcement (using "if" or "for" loops) lets you manage more intricate productivity, provoking precise tasks based on implicit circumstances.
Differentiating Between Tools and Plugins
It’s significant to differentiate between tools and plugins when constructing dependable LLM apps. Tools are separate utilities that offer precise usefulness, while plugins are extensions that can improve existing structures. Both are critical, but pragmatic testing of LLM agents ensures dependability. Knowing this discrepancy helps you select the right elements for your requirements, improving the soundness and efficiency of your applications.
Exploring Distinct Control Flow Types
Choosing the right control flow type can substantially affect your LLM applications performance. Sequential control flows are effortless and immaculate for tasks that adhere to a rigid order. Parallel control flows, on the other hand, permit synchronous task enforcement, saving time and resources. Contingent flows, such as “if” and “for” loops, provides the adaptability to enforce tasks based on assured circumstances or repetitions, making your apps more robust and responsive.
By comprehending and efficiently using these methods, you are better adapted to child sophisticated and effective LLM applications that meet your precise needs.
Promising Use Cases for LLMs
AI Assistant, Chatbot for Customer Service and Engagement
Envision having an AI assistant that’s attainable 24/7 to acknowledge consumer doubts and problems. LLMs can improve chatbots to provide precise and compassionate responses, enhancing customer satisfaction and engagement. Latest progressions show that AI-driven chatbots can manage up to 80% of everyday queries, extricating human support staff to intercept more intricate issues.
Code Generation: Enhancing Programming and Development
If you are a designer, you have likely desired for rapid coding solutions. LLMs such as OpenAI’s codex can comprehend natural language prompts and yields code snippets in multiple programming languages. This not only hastens the development process but also helps you grab bugs early. GitHub's Copilot, powered by Codex, has already become an indispensable tool for many developers.
Personalized Learning and Training
LLMs can act as tailored tutors, customizing lessons and study material based on your learning speed and approach. Whether you are a student or professional looking to upgrade, AI-driven platforms can offer you personalized educational content. Latest studies specify that customized learning techniques can enhance procurement rates by up to 30%.
'Talk-to-Data' for Intuitive Analysis
Envision simply asking queries or doubts about your data and receiving immediate, perceptive responses. LLMs are making this a reality by empowering ‘Talk-to-Data’ attributes in data inspection tools. Instead of writing intricate questions or doubts, you can now ask in plain English, and the AI explicates your intention, producing accurate outcomes. This inventiveness transforms how ventures and researchers engage with their information, making inspection more attainable and inherent. With such tools, regulating is time-saving, and valuable perceptions are more easily exposed.
Spotlight on RagaAI LLM Hub
If you are looking for a pragmatic platform to traverse and deploy LLMs, RagaAI LLM Hub provides a unified solution. It offers access to various generative AI models and services, ensuring you have the tools required to flawlessly incorporate LLM capabilities into your apps. With attributes designed for manageability and security, RagaAI LLM Hub is a magnificent resource for expert and experienced AI developers.
Conclusion
The world of LLM applications is revolutionizing at rapid speed, and staying ahead needs an accurate deployment plan. From composing efficient prompts to integrating progressed methods like RAG and fine-tuning, the expedition to deployable LLM applications can be intricate but extremely fruitful.
As you outlook that phase, remember that adaptability, sturdy governance, and constant learning are your best supporters. Whether you are improving customer assistance, generating advanced analytics, or developing inventive AI products, deploying LLMs efficiently will be a groundbreaker for your undertakings.
Stay interested, stay supple, and keep propelling the boundaries of what’s achievable with LLMs. Happy deploying!