In-Depth Case Studies in AI Model Testing: Exploring Real-World Applications and Insights
In-Depth Case Studies in AI Model Testing: Exploring Real-World Applications and Insights
In-Depth Case Studies in AI Model Testing: Exploring Real-World Applications and Insights
Jigar Gupta
Jun 11, 2024
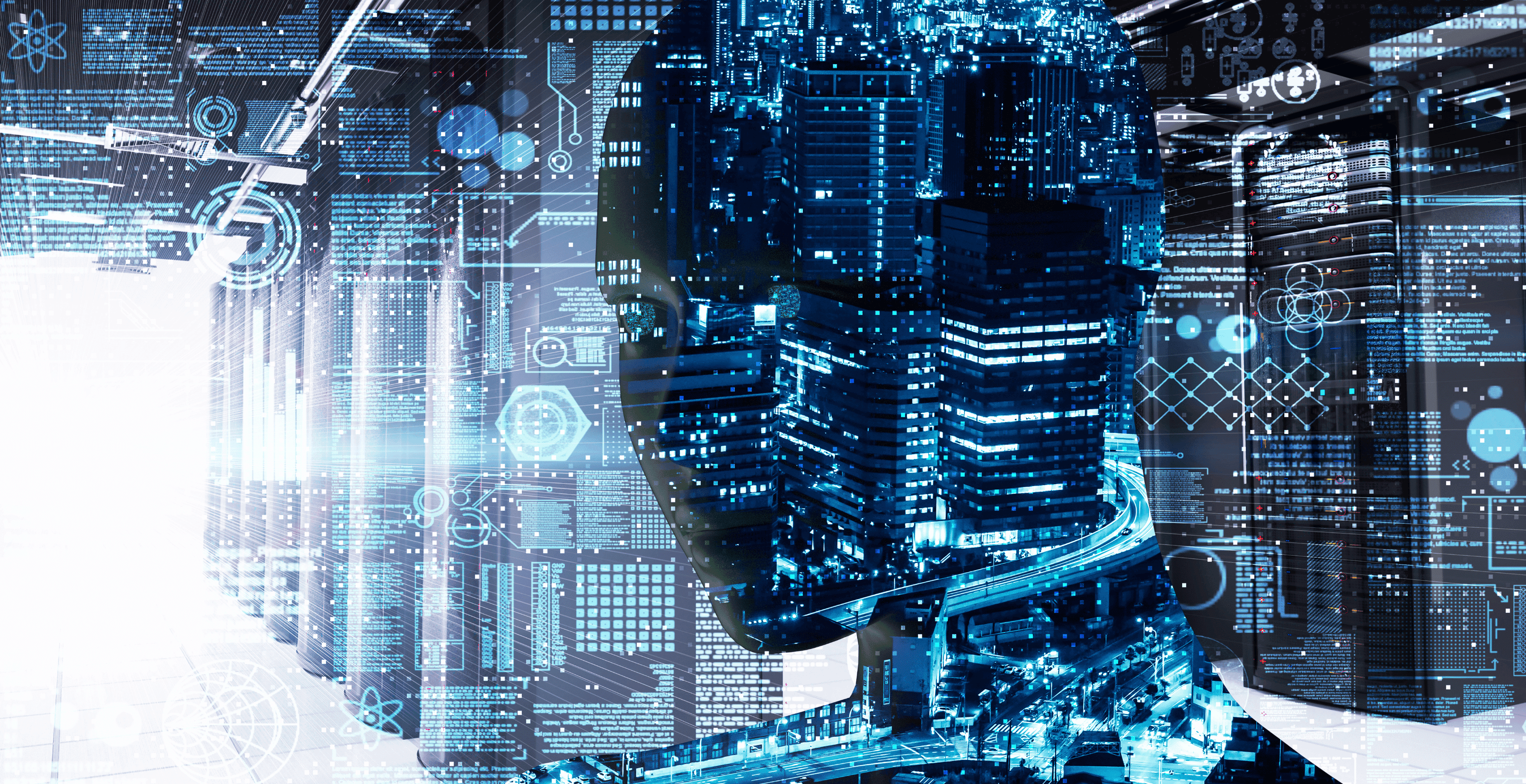
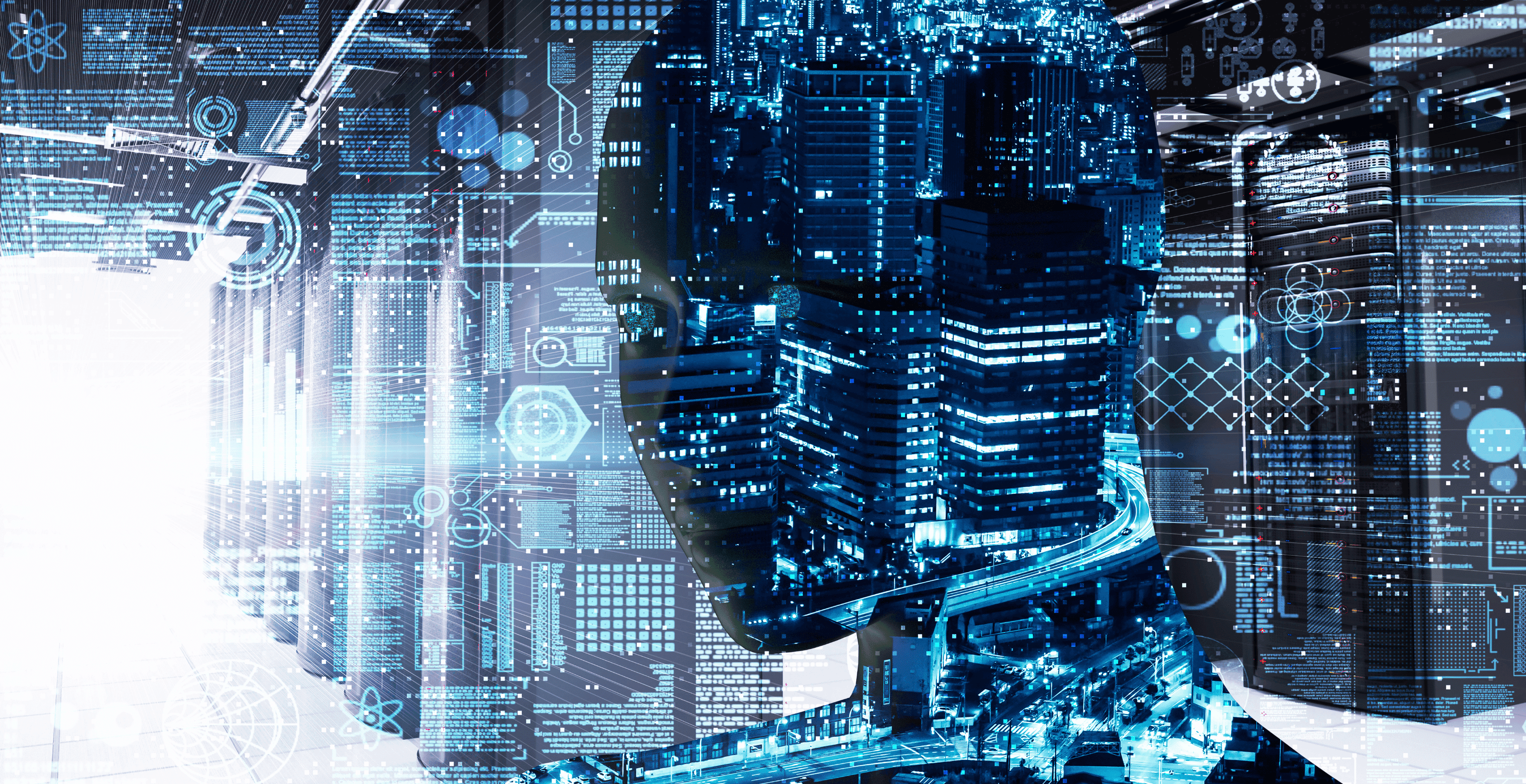
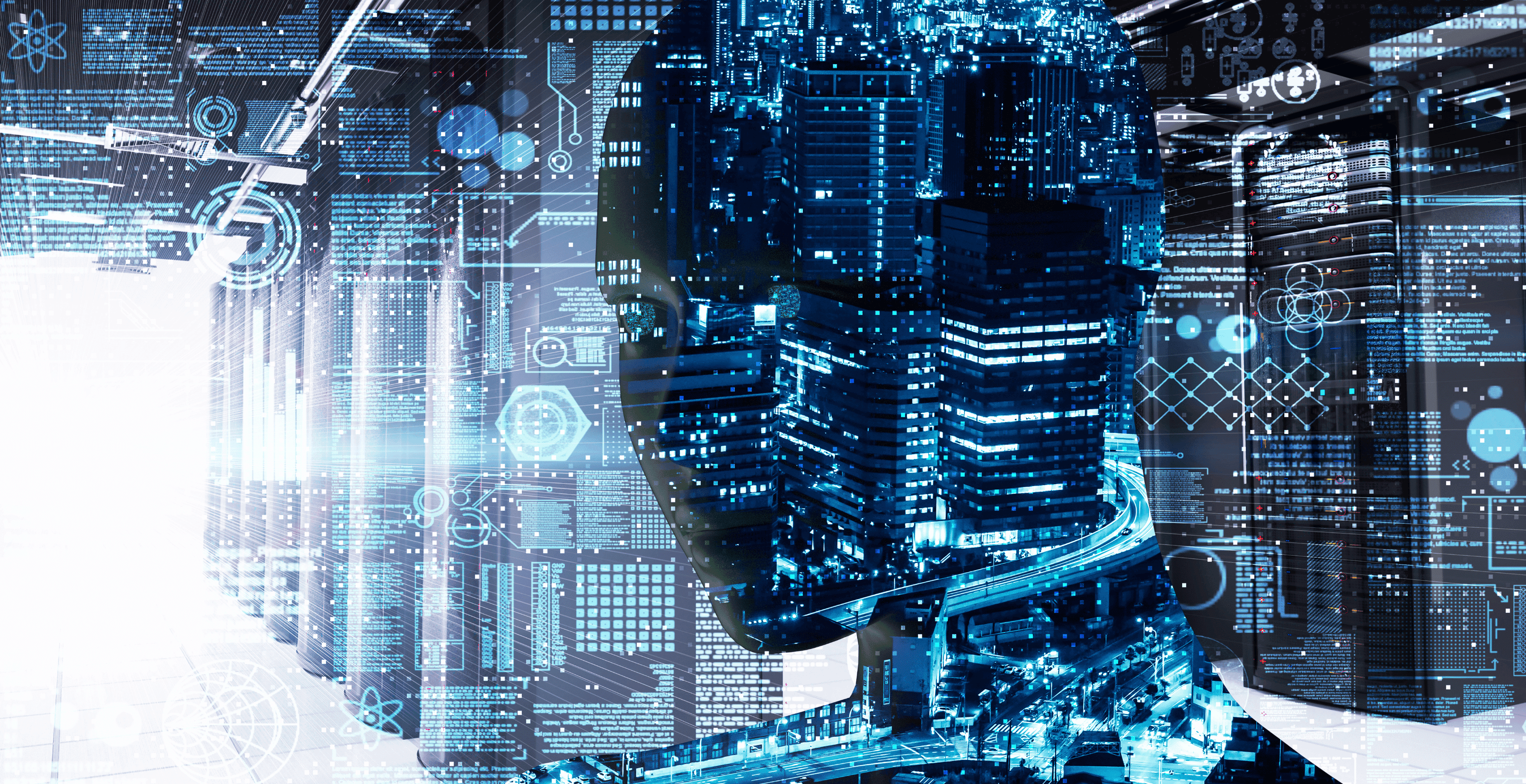
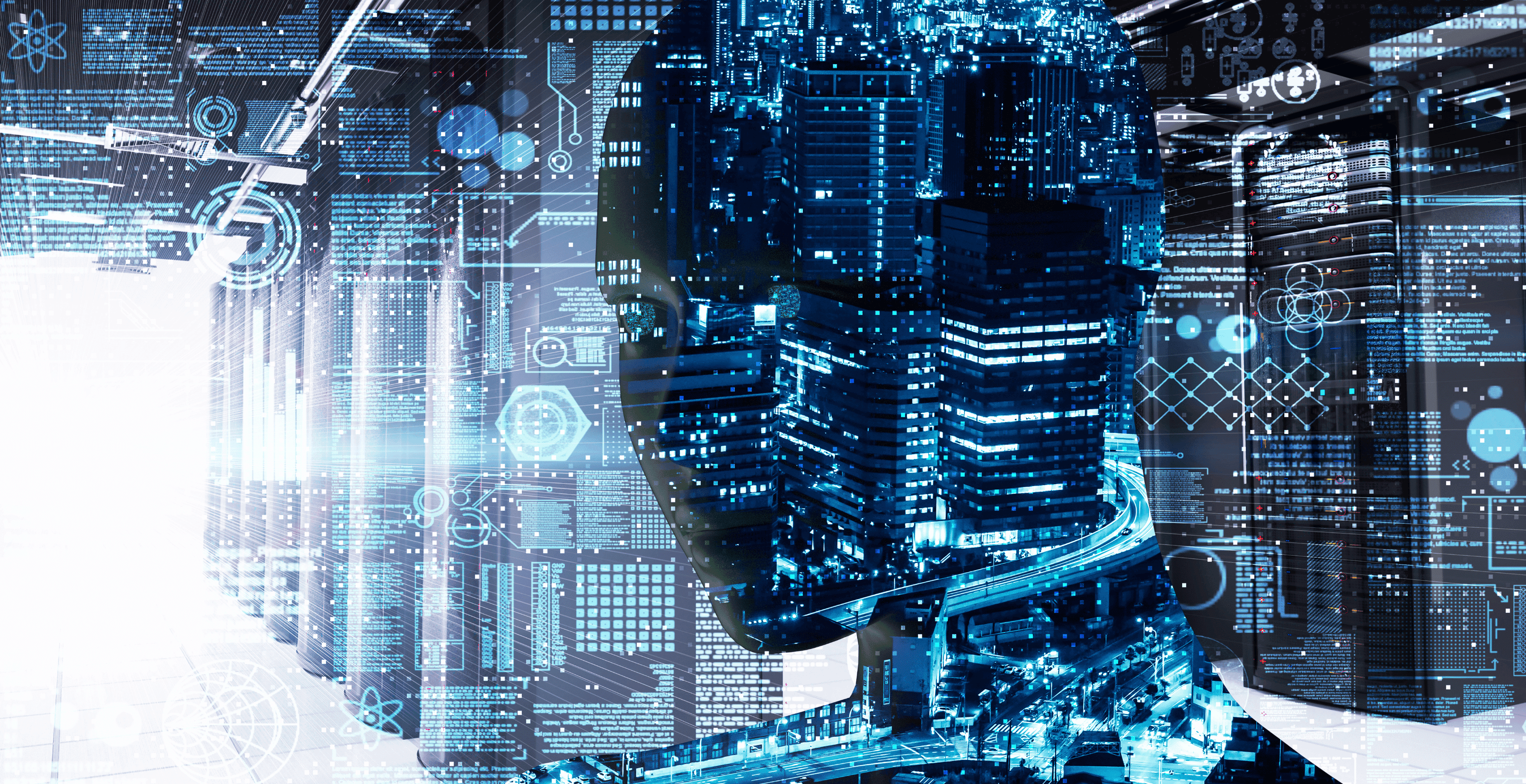
As we examine the quickly progressing globe of Artificial Intelligence, you'll see testing AI models isn't just a step in growth—it's a foundation for positioning dependable and efficient technology across varied sectors.
From healthcare to automotive industries, the strict testing of AI models ensures they perform cautiously and effectively in real-world applications.
In this guide, we delve deeper into exploring several AI model testing case studies that feature the unique challenges and techniques involved in AI model testing across distinct fields.
Case Study 1: Healthcare Industry
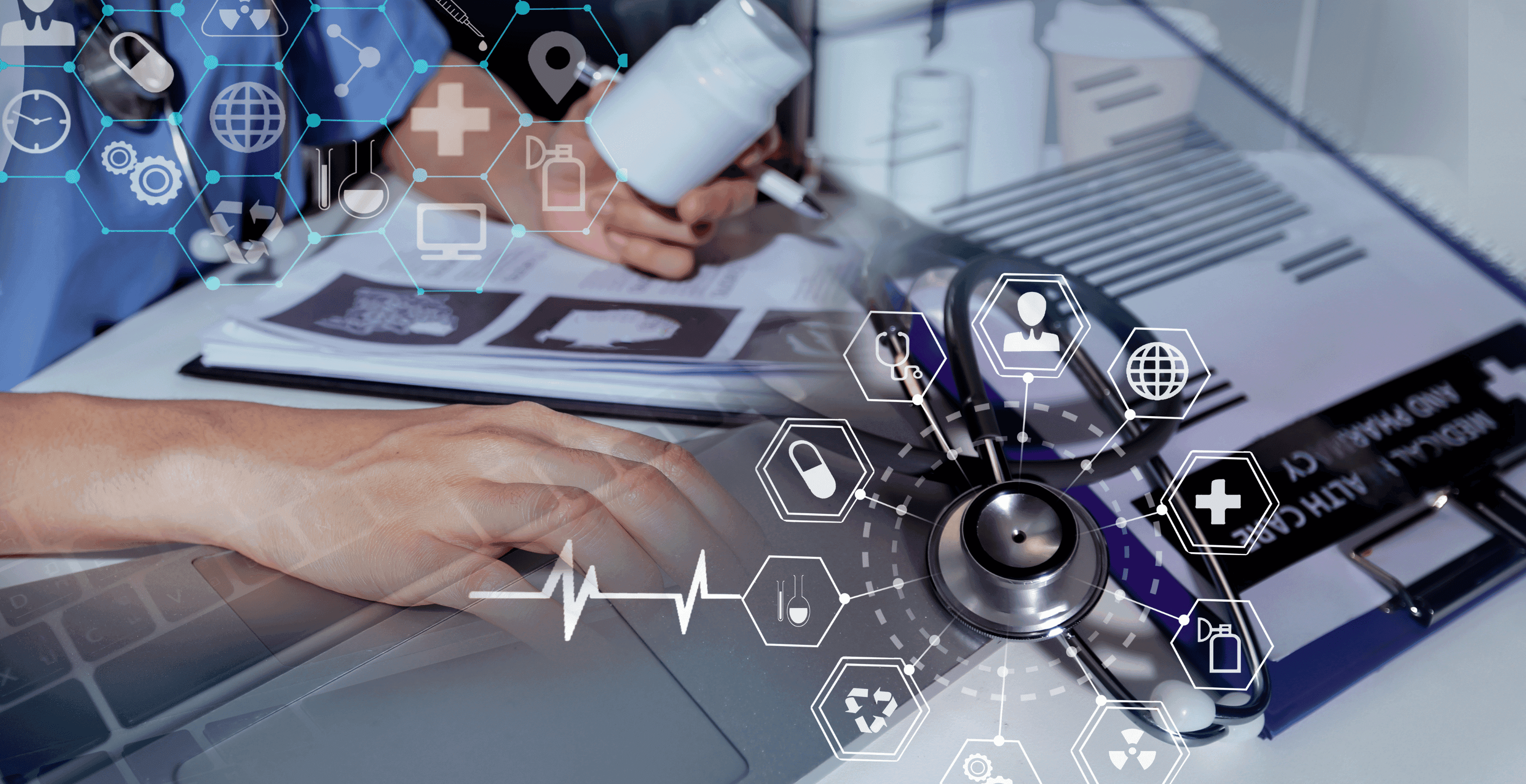
Project Description
The project revolves around using Artificial Intelligence (AI) to improve patient monitoring and enhance the fall discovery apparatus in a healthcare setting.
The AI system constantly monitors patients, especially those with mobility problems or those at high risk of stumbles, using sensors and machine learning algorithms. This technology intends to quickly alert the healthcare staff when a stumble is located or forecasted, enabling instant intervention.
AI Model Testing Challenges
Testing the AI model in this circumstance presents numerous challenges:
Ensuring Precision: This AI must precisely locate actual stumbles without misinterpreting other activities or movements as falls. High precision is critical to ensure that the staff can depend on the cautions to classify and reply to actual events.
Reducing False Alarms: Diminishing false positives is important to prevent alarm fatigue among the healthcare staff. Too many inaccurate alarms could result in desensitization, resulting in slower replies to actual stumbles or even ignoring the cautions.
Testing Methodologies
Below given are the ensuing methodologies that are engaged to address these difficulties:
Human Activity Recognition: This indulges utilizing machine learning algorithms to identify the data gathered from sensors worn by patients or incorporated into their environments. The algorithms are instructed to differentiate between distinct types of human movements and concretely recognize patterns reflective of a fall.
Data Augmentation: Improving the dataset with synthetic falls and non-fall activities to instruct the model more extensively.
Cross-Verification: Utilizing analytical approaches to verify the model’s efficacy across distinct patient enumerations and settings.
Real-Time Testing: Enforcing the system in a restrained environment where real-time observation and feedback can be utilized to fine-tune the model.
Outcome
Positioning this AI system in healthcare facilities has resulted in numerous positive results:
Improved Patient Security:- Instant discovery and notification of stumbles decrease the threat of serious injuries.
Increase Staff Efficiency: Staff can assign their time more efficiently, as the Artificial Intelligence supports observing and provides precise alerts, permitting them to concentrate more on direct patient care and other crucial tasks.
Enhanced Response Times: The system assures that healthcare facilitators can reply more instantly to actual falls, certainly decreasing the rigidity of fall-related injuries.
Overall, the utilization of AI for patient observation and stumble identification in the healthcare industry depicts a crucial progression in both patient care and functional efficacy.
Case Study 2: Automotive Industry

Project Description
In the automotive sector, AI incorporation concentrates on developing sophisticated independent vehicle maneuvering systems. These systems are made to illustrate and react to intricate driving environments without human input.
AI Model Testing Challenges
One of the predominant challenges in enforcing Artificial Intelligence in independent vehicles is assuring safety. Autonomous Vehicles must be reliable under a myriad of driving circumstances and synopsis, which requires broad testing to confirm that AI’s decision-making abilities are constantly safe. Key challenges include:
Intricacy of Real-World Synopsis: Simulating the myriad unpredictable changes that occur in real-world driving synopsis is absolutely challenging. This indulges everything from abrupt weather changes to odd behavior of other road users.
Safety Concerns: The stakes are absolutely high in independent driving. Any drawbacks in AI performance could lead to errors that may threaten human life.
Testing Methodologies
To acknowledge these challenges, an amalgamation of automated and manual testing techniques was enforced:
Automated Tests: These contain simulated environments where AI systems are subjected to a variety of driving circumstances. Automated testing tools generate various synopsis and changes, troubling the AI’s decision-making processes to ensure soundness and dependability.
Manual Verification: This indulges real-world experimentations and human oversight, where independent systems are observed by human drivers to take over if significant. This stage is important for verifying the AI’s performance in actual driving circumstances and for collecting data on any problems or inconsistencies that occur.
Outcome
The harsh and adaptable testing technique led to the progression of dependable and safe autonomous driving abilities. The AI models engaged in the navigation systems confirmed a high level of precision and correspondence, capable of managing varied and challenging driving circumstances. This triumph marks a significant advancement in the tracking of fully independent vehicles, promising improved security and efficacy on the roads.
Alright, now that we've seen how AI is revolutionizing sectors from health to automotive, let's shake things up and move the spotlight to shopping aisles and digital carts. Ready to see how AI is making our shopping smarter? Dive into Case Study 3 to find out.
Case Study 3: Retail and Consumer Goods
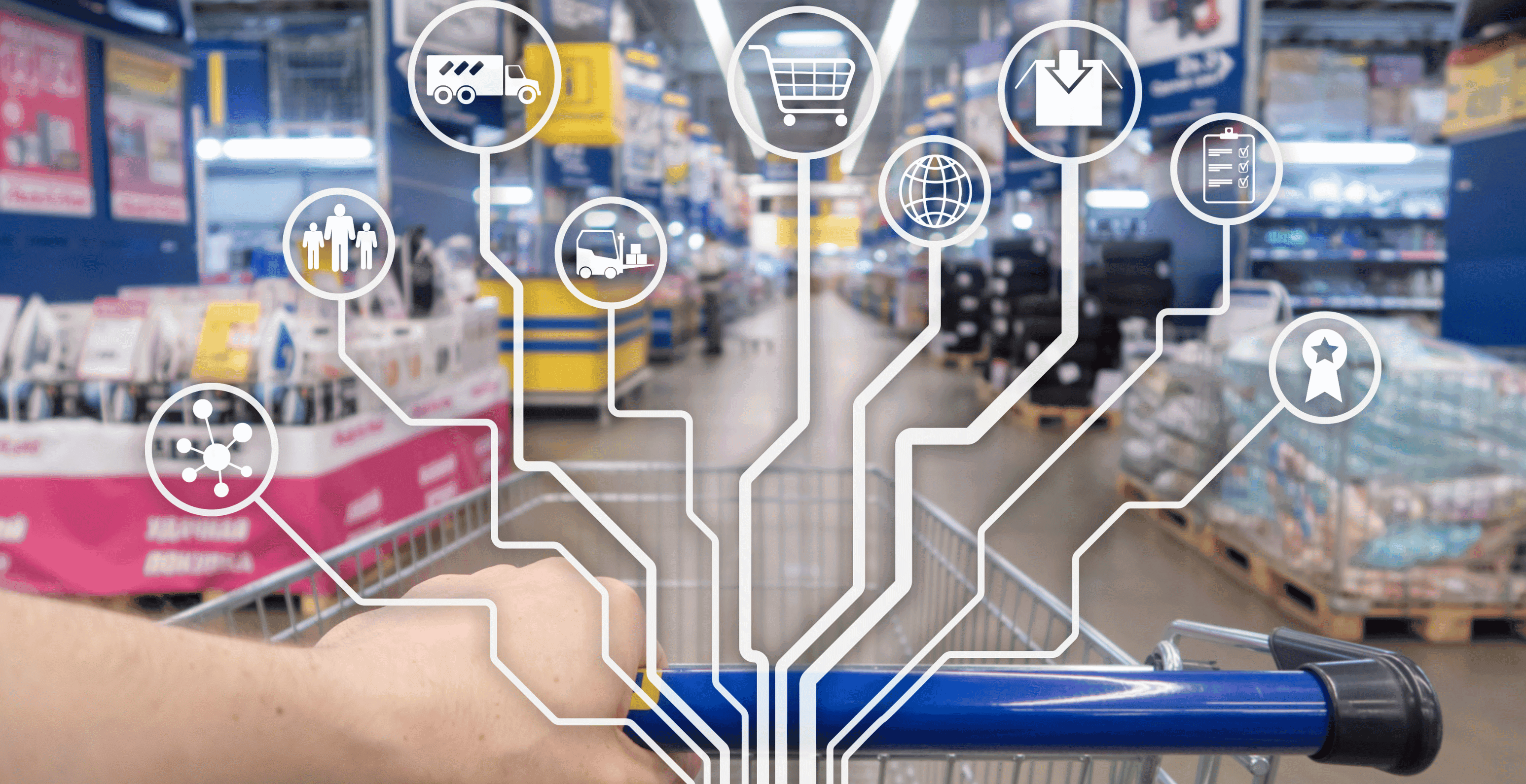
Project Description
Positioning AI in the retail sector substantially concentrates on improving personalized shopping experiences. This indulges using AI to tailor product advice, pricing plans, and marketing efforts, directly to individual customer choices and behaviors. The aim is to create a smooth, appealing and highly customized shopping journey that inspires customer loyalty and increases sales.
AI Model Testing Challenges
Examining AI models in the retail circumstances depicts numerous unique challenges:
Handling Data Security: Ensuring that the data of consumers is utilized morally and in compliance with global data protection and regulations (like GDPR) is supreme. The models must manage sensitive data safely while still being able to originate significant understanding.
Enhancing Recommendation Systems: The efficacy of AI-driven guidance systems is critical for customized experiences. These systems must be examined strictly to ensure they are not only precise but also adjustable to changing customer choices and behaviors.
Testing Methodologies
To acknowledge these challenges, the team applies particular testing techniques:
A/B Testing: This technique permits retailers to compare 2 versions of their Artificial Intelligence models to decide which one performs better in real-globe synopsis. For example, 2 distinct advice algorithms can be examined contemporaneously to see which gives more accurate suggestions to the user.
Stress Testing Machine Learning Models: Stress testing contains placing the Artificial Intelligence models through scenarios with drastic circumstances to ensure they can manage unforeseen surges in data or functioning demands. This is crucial for retail events such as Black Friday sales when system rigidness and manageability are examined to the limits.
Outcome
The planned application of these testing techniques results in crucial improvements in customer satisfaction and business progress. Customers get advantage from a more customized shopping experience that feels peculiarly tailored to their requirements, which in turn promotes greater allegiance and affiance. For ventures, this reiterates increased efficacy in inventory management, more efficient marketing, and eventually higher sales revenues.
These outcomes emphasize the significance of harsh AI model testing in creating strict, efficient AI applications in retail and consumer goods industries.
Now, buckle up because it's time to dive into the world of FinTech, where every decimal and digit is a game-changer. Let’s see how AI is crunching numbers to pave the way for smarter financial decisions. Ready? Here comes Case Study 4!
Case Study 4: Financial Technology (FinTech)

Project Description
The project concentrates on enforcing AI technologies to improve predictive analytics within the domain of market intelligence. This application of Artificial Intelligence aims to dissect vast amounts of financial data to predict the trends of the market, helping ventures and stakeholders make informed choices.
AI Model Testing Challenges
One of the main challenges faced during the execution was ensuring the precision and performance of the Artificial Intelligence models under diverse and often unpredictable market circumstances. Financial markets are affected by several elements, indulging economic indicators, political events, and social tendencies which all impact the constancy of the models.
Testing Methodologies
To acknowledge these challenges, the team engaged a blend of behavioral tests and A/B testing. Behavioral tests helped comprehend how the model demeanor under distinct market scenarios, especially during strange or drastic market circumstances. A/B testing, on the other hand, permits the team to test with distinct versions of the model to decide which provided the most dependable predictions.
Outcome
The rigid testing techniques contributed to the progression of rigid AI systems capable of delivering dependable predictive insights. This empowered financial choice makers with improved analytical tools, substantially assisting in strategy articulation and risk evaluation.
Conclusion
As these case studies illustrate, AI model testing plays a crucial role in ensuring functional success across several industries. The future direction of Artificial Intelligence model testing indulges in not only clarifying these methodologies but also incorporating new technologies that improves the precision and efficacy of AI applications.
As we look ahead, the continued focus on strict testing and quality conviction will undoubtedly drive the next wave of inventiveness in AI technology, making it an even more essential tool in our everyday lives.
FAQs
What is the use case of AI in the Automotive Industry?
AI has numerous dynamic use cases in the automotive industry, modifying how vehicles are designed, built and propelled. Below given are some key applications:
Autonomous Driving: AI is the backbone of self-driving technology. It proceeds data from vehicle sensors and cameras to steer roads, comprehend traffic circumstances, and make real-time choices, improving security and efficacy.
Predictive Maintenance: AI Algorithms dissects data from various vehicle detectors to predict when parts might fail or need maintenance. This helps in decreasing layoff and maintenance charges and enhances the life span of vehicles.
Manufacturing Automation: Robots and automation systems powered by Artificial Intelligence enhance the accuracy and efficacy of automobile assembly in manufacturing plants. These systems can adjust for distinct tasks without the requirement for reprogramming.
What is responsible AI in the automotive industry?
Responsible AI in the automotive industry refers to the ethical design, growth and execution of AI technologies in a manner that is ethical, safe, and advantageous to society. This indulges various key aspects:
Safety and Reliability: Artificial Intelligence systems utilized in vehicles, like independent driving systems and driver-hinder technologies, must be carefully examined and demonstrated to be secured under distinctive circumstances.
Transparency and Accountability: It's crucial for automotive firms to be transparent about how their AI systems work. This means giving clear details on how decisions are made by AI technologies and being liable for the outcomes.
Privacy and Data Security: With vehicles gathering vast amounts of information, indulging personal details about drivers and their practice, strong amounts must be in place to safeguard this data. Ensuring privacy and safeguarding against data violation are critical.
As we examine the quickly progressing globe of Artificial Intelligence, you'll see testing AI models isn't just a step in growth—it's a foundation for positioning dependable and efficient technology across varied sectors.
From healthcare to automotive industries, the strict testing of AI models ensures they perform cautiously and effectively in real-world applications.
In this guide, we delve deeper into exploring several AI model testing case studies that feature the unique challenges and techniques involved in AI model testing across distinct fields.
Case Study 1: Healthcare Industry
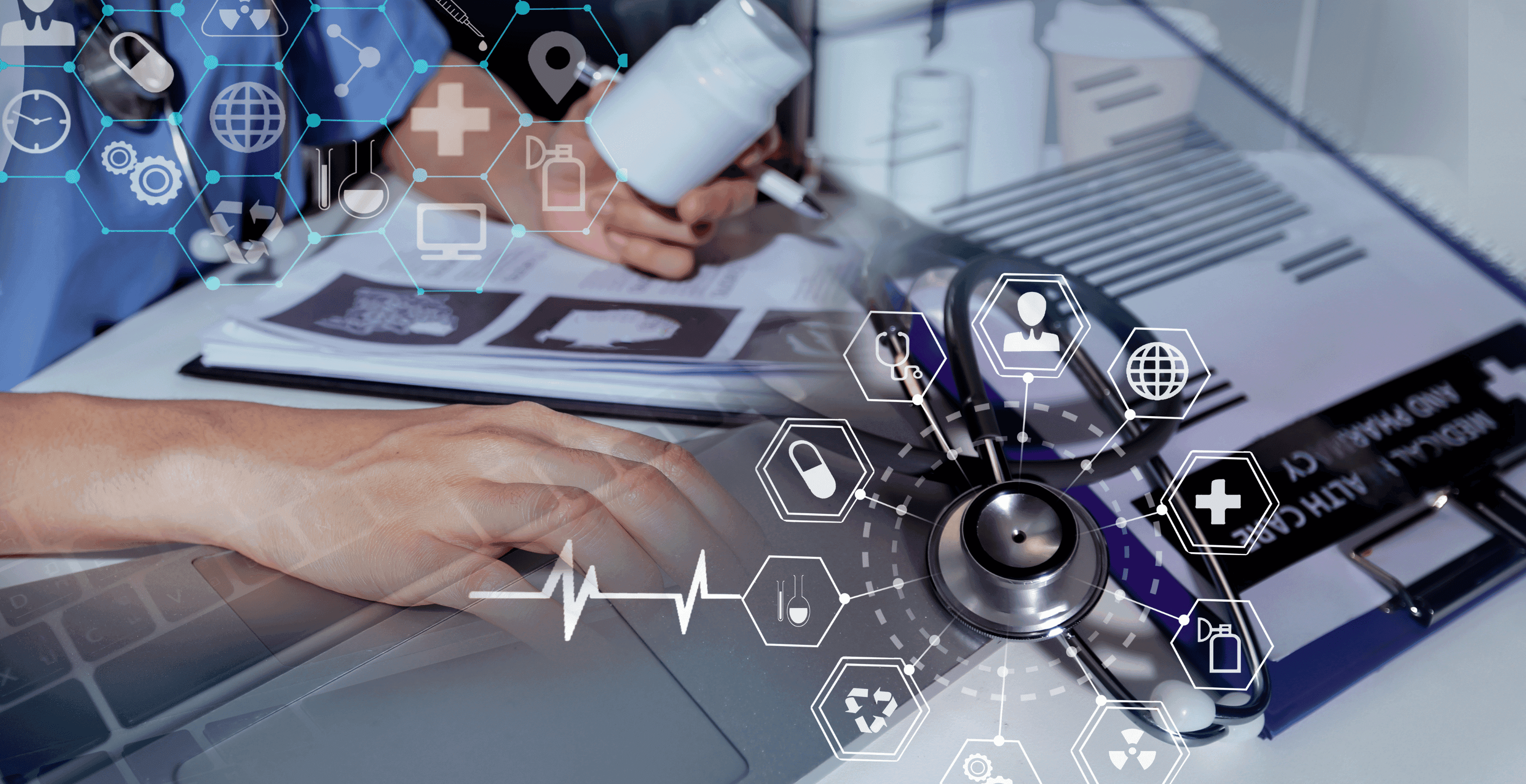
Project Description
The project revolves around using Artificial Intelligence (AI) to improve patient monitoring and enhance the fall discovery apparatus in a healthcare setting.
The AI system constantly monitors patients, especially those with mobility problems or those at high risk of stumbles, using sensors and machine learning algorithms. This technology intends to quickly alert the healthcare staff when a stumble is located or forecasted, enabling instant intervention.
AI Model Testing Challenges
Testing the AI model in this circumstance presents numerous challenges:
Ensuring Precision: This AI must precisely locate actual stumbles without misinterpreting other activities or movements as falls. High precision is critical to ensure that the staff can depend on the cautions to classify and reply to actual events.
Reducing False Alarms: Diminishing false positives is important to prevent alarm fatigue among the healthcare staff. Too many inaccurate alarms could result in desensitization, resulting in slower replies to actual stumbles or even ignoring the cautions.
Testing Methodologies
Below given are the ensuing methodologies that are engaged to address these difficulties:
Human Activity Recognition: This indulges utilizing machine learning algorithms to identify the data gathered from sensors worn by patients or incorporated into their environments. The algorithms are instructed to differentiate between distinct types of human movements and concretely recognize patterns reflective of a fall.
Data Augmentation: Improving the dataset with synthetic falls and non-fall activities to instruct the model more extensively.
Cross-Verification: Utilizing analytical approaches to verify the model’s efficacy across distinct patient enumerations and settings.
Real-Time Testing: Enforcing the system in a restrained environment where real-time observation and feedback can be utilized to fine-tune the model.
Outcome
Positioning this AI system in healthcare facilities has resulted in numerous positive results:
Improved Patient Security:- Instant discovery and notification of stumbles decrease the threat of serious injuries.
Increase Staff Efficiency: Staff can assign their time more efficiently, as the Artificial Intelligence supports observing and provides precise alerts, permitting them to concentrate more on direct patient care and other crucial tasks.
Enhanced Response Times: The system assures that healthcare facilitators can reply more instantly to actual falls, certainly decreasing the rigidity of fall-related injuries.
Overall, the utilization of AI for patient observation and stumble identification in the healthcare industry depicts a crucial progression in both patient care and functional efficacy.
Case Study 2: Automotive Industry

Project Description
In the automotive sector, AI incorporation concentrates on developing sophisticated independent vehicle maneuvering systems. These systems are made to illustrate and react to intricate driving environments without human input.
AI Model Testing Challenges
One of the predominant challenges in enforcing Artificial Intelligence in independent vehicles is assuring safety. Autonomous Vehicles must be reliable under a myriad of driving circumstances and synopsis, which requires broad testing to confirm that AI’s decision-making abilities are constantly safe. Key challenges include:
Intricacy of Real-World Synopsis: Simulating the myriad unpredictable changes that occur in real-world driving synopsis is absolutely challenging. This indulges everything from abrupt weather changes to odd behavior of other road users.
Safety Concerns: The stakes are absolutely high in independent driving. Any drawbacks in AI performance could lead to errors that may threaten human life.
Testing Methodologies
To acknowledge these challenges, an amalgamation of automated and manual testing techniques was enforced:
Automated Tests: These contain simulated environments where AI systems are subjected to a variety of driving circumstances. Automated testing tools generate various synopsis and changes, troubling the AI’s decision-making processes to ensure soundness and dependability.
Manual Verification: This indulges real-world experimentations and human oversight, where independent systems are observed by human drivers to take over if significant. This stage is important for verifying the AI’s performance in actual driving circumstances and for collecting data on any problems or inconsistencies that occur.
Outcome
The harsh and adaptable testing technique led to the progression of dependable and safe autonomous driving abilities. The AI models engaged in the navigation systems confirmed a high level of precision and correspondence, capable of managing varied and challenging driving circumstances. This triumph marks a significant advancement in the tracking of fully independent vehicles, promising improved security and efficacy on the roads.
Alright, now that we've seen how AI is revolutionizing sectors from health to automotive, let's shake things up and move the spotlight to shopping aisles and digital carts. Ready to see how AI is making our shopping smarter? Dive into Case Study 3 to find out.
Case Study 3: Retail and Consumer Goods
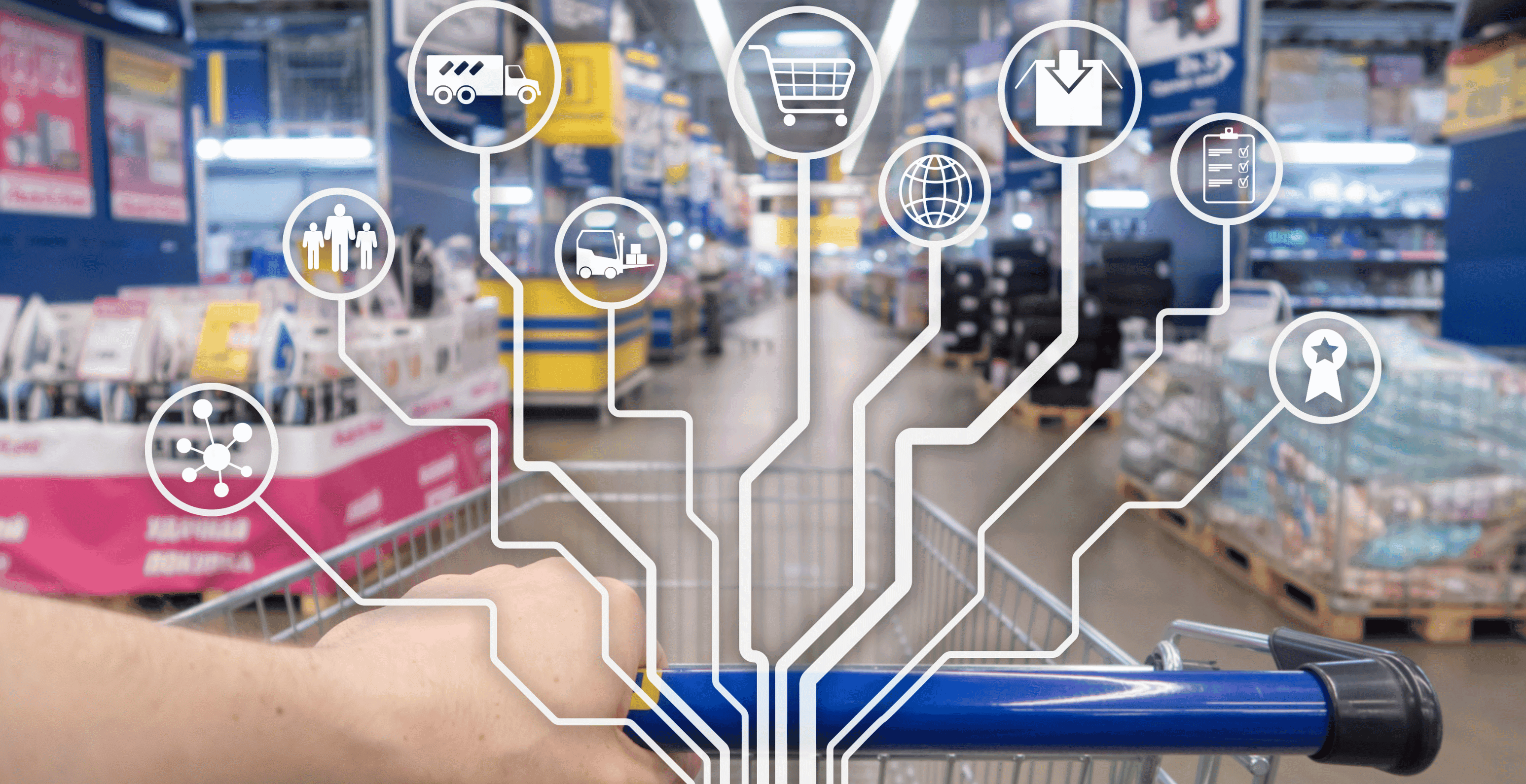
Project Description
Positioning AI in the retail sector substantially concentrates on improving personalized shopping experiences. This indulges using AI to tailor product advice, pricing plans, and marketing efforts, directly to individual customer choices and behaviors. The aim is to create a smooth, appealing and highly customized shopping journey that inspires customer loyalty and increases sales.
AI Model Testing Challenges
Examining AI models in the retail circumstances depicts numerous unique challenges:
Handling Data Security: Ensuring that the data of consumers is utilized morally and in compliance with global data protection and regulations (like GDPR) is supreme. The models must manage sensitive data safely while still being able to originate significant understanding.
Enhancing Recommendation Systems: The efficacy of AI-driven guidance systems is critical for customized experiences. These systems must be examined strictly to ensure they are not only precise but also adjustable to changing customer choices and behaviors.
Testing Methodologies
To acknowledge these challenges, the team applies particular testing techniques:
A/B Testing: This technique permits retailers to compare 2 versions of their Artificial Intelligence models to decide which one performs better in real-globe synopsis. For example, 2 distinct advice algorithms can be examined contemporaneously to see which gives more accurate suggestions to the user.
Stress Testing Machine Learning Models: Stress testing contains placing the Artificial Intelligence models through scenarios with drastic circumstances to ensure they can manage unforeseen surges in data or functioning demands. This is crucial for retail events such as Black Friday sales when system rigidness and manageability are examined to the limits.
Outcome
The planned application of these testing techniques results in crucial improvements in customer satisfaction and business progress. Customers get advantage from a more customized shopping experience that feels peculiarly tailored to their requirements, which in turn promotes greater allegiance and affiance. For ventures, this reiterates increased efficacy in inventory management, more efficient marketing, and eventually higher sales revenues.
These outcomes emphasize the significance of harsh AI model testing in creating strict, efficient AI applications in retail and consumer goods industries.
Now, buckle up because it's time to dive into the world of FinTech, where every decimal and digit is a game-changer. Let’s see how AI is crunching numbers to pave the way for smarter financial decisions. Ready? Here comes Case Study 4!
Case Study 4: Financial Technology (FinTech)

Project Description
The project concentrates on enforcing AI technologies to improve predictive analytics within the domain of market intelligence. This application of Artificial Intelligence aims to dissect vast amounts of financial data to predict the trends of the market, helping ventures and stakeholders make informed choices.
AI Model Testing Challenges
One of the main challenges faced during the execution was ensuring the precision and performance of the Artificial Intelligence models under diverse and often unpredictable market circumstances. Financial markets are affected by several elements, indulging economic indicators, political events, and social tendencies which all impact the constancy of the models.
Testing Methodologies
To acknowledge these challenges, the team engaged a blend of behavioral tests and A/B testing. Behavioral tests helped comprehend how the model demeanor under distinct market scenarios, especially during strange or drastic market circumstances. A/B testing, on the other hand, permits the team to test with distinct versions of the model to decide which provided the most dependable predictions.
Outcome
The rigid testing techniques contributed to the progression of rigid AI systems capable of delivering dependable predictive insights. This empowered financial choice makers with improved analytical tools, substantially assisting in strategy articulation and risk evaluation.
Conclusion
As these case studies illustrate, AI model testing plays a crucial role in ensuring functional success across several industries. The future direction of Artificial Intelligence model testing indulges in not only clarifying these methodologies but also incorporating new technologies that improves the precision and efficacy of AI applications.
As we look ahead, the continued focus on strict testing and quality conviction will undoubtedly drive the next wave of inventiveness in AI technology, making it an even more essential tool in our everyday lives.
FAQs
What is the use case of AI in the Automotive Industry?
AI has numerous dynamic use cases in the automotive industry, modifying how vehicles are designed, built and propelled. Below given are some key applications:
Autonomous Driving: AI is the backbone of self-driving technology. It proceeds data from vehicle sensors and cameras to steer roads, comprehend traffic circumstances, and make real-time choices, improving security and efficacy.
Predictive Maintenance: AI Algorithms dissects data from various vehicle detectors to predict when parts might fail or need maintenance. This helps in decreasing layoff and maintenance charges and enhances the life span of vehicles.
Manufacturing Automation: Robots and automation systems powered by Artificial Intelligence enhance the accuracy and efficacy of automobile assembly in manufacturing plants. These systems can adjust for distinct tasks without the requirement for reprogramming.
What is responsible AI in the automotive industry?
Responsible AI in the automotive industry refers to the ethical design, growth and execution of AI technologies in a manner that is ethical, safe, and advantageous to society. This indulges various key aspects:
Safety and Reliability: Artificial Intelligence systems utilized in vehicles, like independent driving systems and driver-hinder technologies, must be carefully examined and demonstrated to be secured under distinctive circumstances.
Transparency and Accountability: It's crucial for automotive firms to be transparent about how their AI systems work. This means giving clear details on how decisions are made by AI technologies and being liable for the outcomes.
Privacy and Data Security: With vehicles gathering vast amounts of information, indulging personal details about drivers and their practice, strong amounts must be in place to safeguard this data. Ensuring privacy and safeguarding against data violation are critical.
As we examine the quickly progressing globe of Artificial Intelligence, you'll see testing AI models isn't just a step in growth—it's a foundation for positioning dependable and efficient technology across varied sectors.
From healthcare to automotive industries, the strict testing of AI models ensures they perform cautiously and effectively in real-world applications.
In this guide, we delve deeper into exploring several AI model testing case studies that feature the unique challenges and techniques involved in AI model testing across distinct fields.
Case Study 1: Healthcare Industry
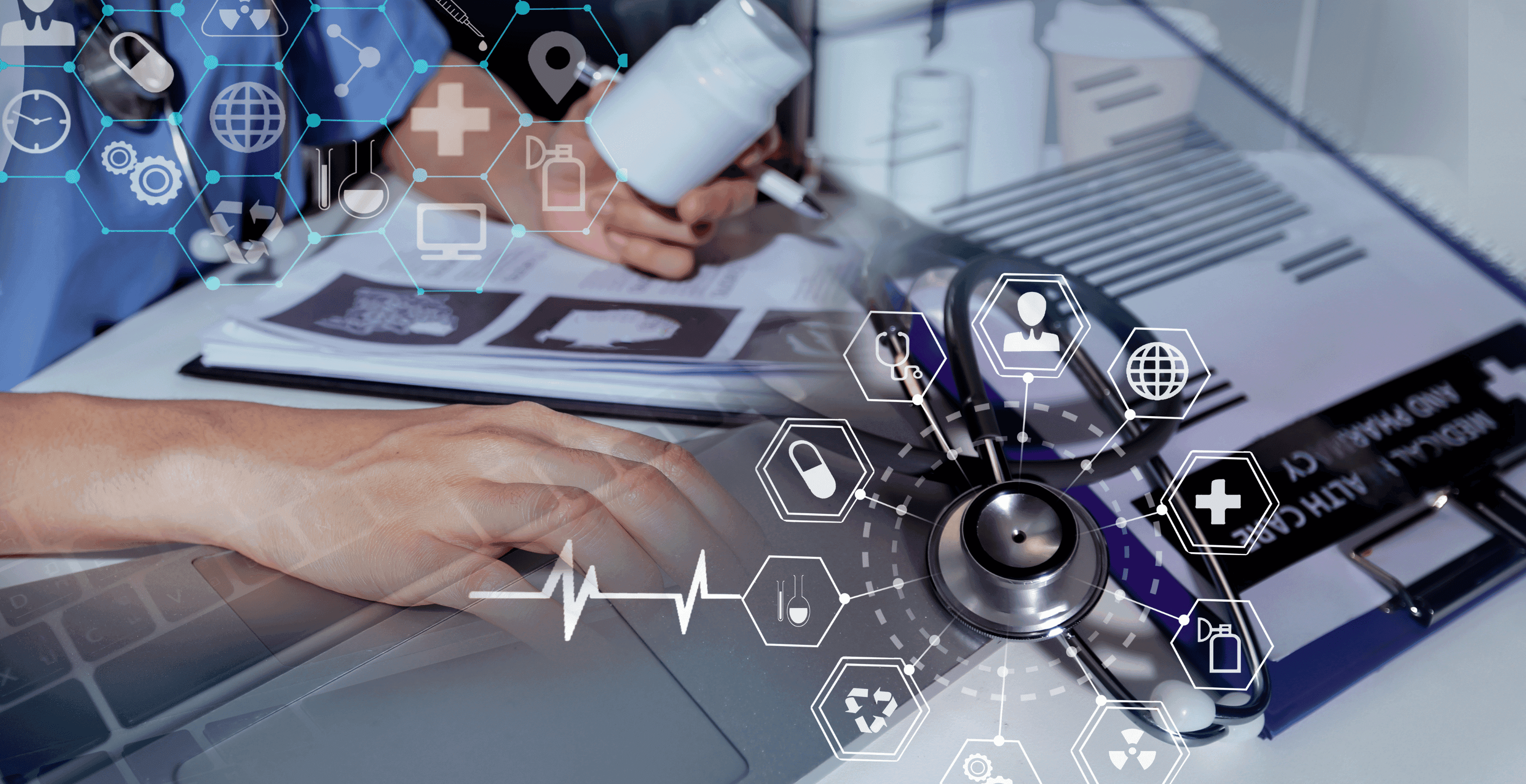
Project Description
The project revolves around using Artificial Intelligence (AI) to improve patient monitoring and enhance the fall discovery apparatus in a healthcare setting.
The AI system constantly monitors patients, especially those with mobility problems or those at high risk of stumbles, using sensors and machine learning algorithms. This technology intends to quickly alert the healthcare staff when a stumble is located or forecasted, enabling instant intervention.
AI Model Testing Challenges
Testing the AI model in this circumstance presents numerous challenges:
Ensuring Precision: This AI must precisely locate actual stumbles without misinterpreting other activities or movements as falls. High precision is critical to ensure that the staff can depend on the cautions to classify and reply to actual events.
Reducing False Alarms: Diminishing false positives is important to prevent alarm fatigue among the healthcare staff. Too many inaccurate alarms could result in desensitization, resulting in slower replies to actual stumbles or even ignoring the cautions.
Testing Methodologies
Below given are the ensuing methodologies that are engaged to address these difficulties:
Human Activity Recognition: This indulges utilizing machine learning algorithms to identify the data gathered from sensors worn by patients or incorporated into their environments. The algorithms are instructed to differentiate between distinct types of human movements and concretely recognize patterns reflective of a fall.
Data Augmentation: Improving the dataset with synthetic falls and non-fall activities to instruct the model more extensively.
Cross-Verification: Utilizing analytical approaches to verify the model’s efficacy across distinct patient enumerations and settings.
Real-Time Testing: Enforcing the system in a restrained environment where real-time observation and feedback can be utilized to fine-tune the model.
Outcome
Positioning this AI system in healthcare facilities has resulted in numerous positive results:
Improved Patient Security:- Instant discovery and notification of stumbles decrease the threat of serious injuries.
Increase Staff Efficiency: Staff can assign their time more efficiently, as the Artificial Intelligence supports observing and provides precise alerts, permitting them to concentrate more on direct patient care and other crucial tasks.
Enhanced Response Times: The system assures that healthcare facilitators can reply more instantly to actual falls, certainly decreasing the rigidity of fall-related injuries.
Overall, the utilization of AI for patient observation and stumble identification in the healthcare industry depicts a crucial progression in both patient care and functional efficacy.
Case Study 2: Automotive Industry

Project Description
In the automotive sector, AI incorporation concentrates on developing sophisticated independent vehicle maneuvering systems. These systems are made to illustrate and react to intricate driving environments without human input.
AI Model Testing Challenges
One of the predominant challenges in enforcing Artificial Intelligence in independent vehicles is assuring safety. Autonomous Vehicles must be reliable under a myriad of driving circumstances and synopsis, which requires broad testing to confirm that AI’s decision-making abilities are constantly safe. Key challenges include:
Intricacy of Real-World Synopsis: Simulating the myriad unpredictable changes that occur in real-world driving synopsis is absolutely challenging. This indulges everything from abrupt weather changes to odd behavior of other road users.
Safety Concerns: The stakes are absolutely high in independent driving. Any drawbacks in AI performance could lead to errors that may threaten human life.
Testing Methodologies
To acknowledge these challenges, an amalgamation of automated and manual testing techniques was enforced:
Automated Tests: These contain simulated environments where AI systems are subjected to a variety of driving circumstances. Automated testing tools generate various synopsis and changes, troubling the AI’s decision-making processes to ensure soundness and dependability.
Manual Verification: This indulges real-world experimentations and human oversight, where independent systems are observed by human drivers to take over if significant. This stage is important for verifying the AI’s performance in actual driving circumstances and for collecting data on any problems or inconsistencies that occur.
Outcome
The harsh and adaptable testing technique led to the progression of dependable and safe autonomous driving abilities. The AI models engaged in the navigation systems confirmed a high level of precision and correspondence, capable of managing varied and challenging driving circumstances. This triumph marks a significant advancement in the tracking of fully independent vehicles, promising improved security and efficacy on the roads.
Alright, now that we've seen how AI is revolutionizing sectors from health to automotive, let's shake things up and move the spotlight to shopping aisles and digital carts. Ready to see how AI is making our shopping smarter? Dive into Case Study 3 to find out.
Case Study 3: Retail and Consumer Goods
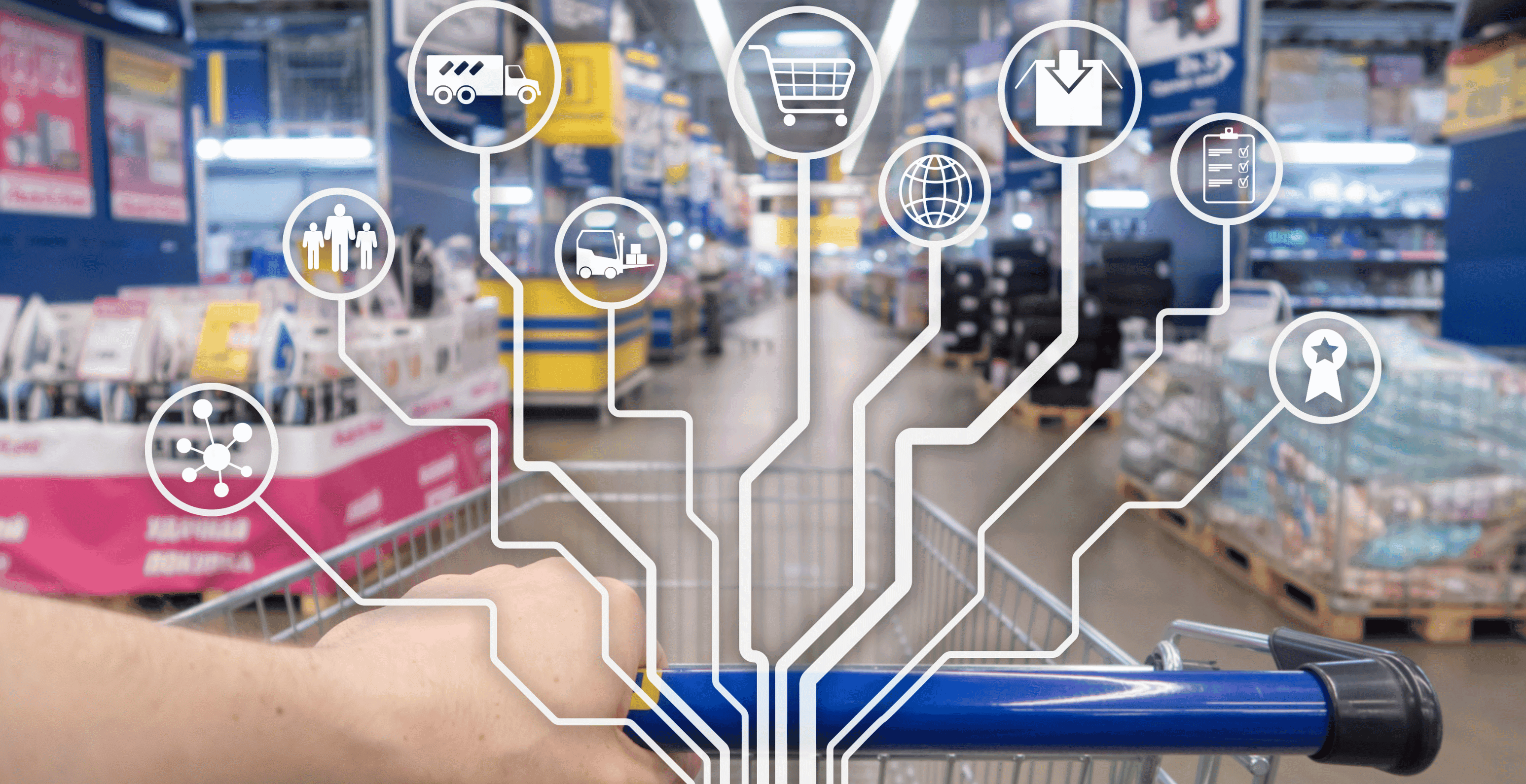
Project Description
Positioning AI in the retail sector substantially concentrates on improving personalized shopping experiences. This indulges using AI to tailor product advice, pricing plans, and marketing efforts, directly to individual customer choices and behaviors. The aim is to create a smooth, appealing and highly customized shopping journey that inspires customer loyalty and increases sales.
AI Model Testing Challenges
Examining AI models in the retail circumstances depicts numerous unique challenges:
Handling Data Security: Ensuring that the data of consumers is utilized morally and in compliance with global data protection and regulations (like GDPR) is supreme. The models must manage sensitive data safely while still being able to originate significant understanding.
Enhancing Recommendation Systems: The efficacy of AI-driven guidance systems is critical for customized experiences. These systems must be examined strictly to ensure they are not only precise but also adjustable to changing customer choices and behaviors.
Testing Methodologies
To acknowledge these challenges, the team applies particular testing techniques:
A/B Testing: This technique permits retailers to compare 2 versions of their Artificial Intelligence models to decide which one performs better in real-globe synopsis. For example, 2 distinct advice algorithms can be examined contemporaneously to see which gives more accurate suggestions to the user.
Stress Testing Machine Learning Models: Stress testing contains placing the Artificial Intelligence models through scenarios with drastic circumstances to ensure they can manage unforeseen surges in data or functioning demands. This is crucial for retail events such as Black Friday sales when system rigidness and manageability are examined to the limits.
Outcome
The planned application of these testing techniques results in crucial improvements in customer satisfaction and business progress. Customers get advantage from a more customized shopping experience that feels peculiarly tailored to their requirements, which in turn promotes greater allegiance and affiance. For ventures, this reiterates increased efficacy in inventory management, more efficient marketing, and eventually higher sales revenues.
These outcomes emphasize the significance of harsh AI model testing in creating strict, efficient AI applications in retail and consumer goods industries.
Now, buckle up because it's time to dive into the world of FinTech, where every decimal and digit is a game-changer. Let’s see how AI is crunching numbers to pave the way for smarter financial decisions. Ready? Here comes Case Study 4!
Case Study 4: Financial Technology (FinTech)

Project Description
The project concentrates on enforcing AI technologies to improve predictive analytics within the domain of market intelligence. This application of Artificial Intelligence aims to dissect vast amounts of financial data to predict the trends of the market, helping ventures and stakeholders make informed choices.
AI Model Testing Challenges
One of the main challenges faced during the execution was ensuring the precision and performance of the Artificial Intelligence models under diverse and often unpredictable market circumstances. Financial markets are affected by several elements, indulging economic indicators, political events, and social tendencies which all impact the constancy of the models.
Testing Methodologies
To acknowledge these challenges, the team engaged a blend of behavioral tests and A/B testing. Behavioral tests helped comprehend how the model demeanor under distinct market scenarios, especially during strange or drastic market circumstances. A/B testing, on the other hand, permits the team to test with distinct versions of the model to decide which provided the most dependable predictions.
Outcome
The rigid testing techniques contributed to the progression of rigid AI systems capable of delivering dependable predictive insights. This empowered financial choice makers with improved analytical tools, substantially assisting in strategy articulation and risk evaluation.
Conclusion
As these case studies illustrate, AI model testing plays a crucial role in ensuring functional success across several industries. The future direction of Artificial Intelligence model testing indulges in not only clarifying these methodologies but also incorporating new technologies that improves the precision and efficacy of AI applications.
As we look ahead, the continued focus on strict testing and quality conviction will undoubtedly drive the next wave of inventiveness in AI technology, making it an even more essential tool in our everyday lives.
FAQs
What is the use case of AI in the Automotive Industry?
AI has numerous dynamic use cases in the automotive industry, modifying how vehicles are designed, built and propelled. Below given are some key applications:
Autonomous Driving: AI is the backbone of self-driving technology. It proceeds data from vehicle sensors and cameras to steer roads, comprehend traffic circumstances, and make real-time choices, improving security and efficacy.
Predictive Maintenance: AI Algorithms dissects data from various vehicle detectors to predict when parts might fail or need maintenance. This helps in decreasing layoff and maintenance charges and enhances the life span of vehicles.
Manufacturing Automation: Robots and automation systems powered by Artificial Intelligence enhance the accuracy and efficacy of automobile assembly in manufacturing plants. These systems can adjust for distinct tasks without the requirement for reprogramming.
What is responsible AI in the automotive industry?
Responsible AI in the automotive industry refers to the ethical design, growth and execution of AI technologies in a manner that is ethical, safe, and advantageous to society. This indulges various key aspects:
Safety and Reliability: Artificial Intelligence systems utilized in vehicles, like independent driving systems and driver-hinder technologies, must be carefully examined and demonstrated to be secured under distinctive circumstances.
Transparency and Accountability: It's crucial for automotive firms to be transparent about how their AI systems work. This means giving clear details on how decisions are made by AI technologies and being liable for the outcomes.
Privacy and Data Security: With vehicles gathering vast amounts of information, indulging personal details about drivers and their practice, strong amounts must be in place to safeguard this data. Ensuring privacy and safeguarding against data violation are critical.
As we examine the quickly progressing globe of Artificial Intelligence, you'll see testing AI models isn't just a step in growth—it's a foundation for positioning dependable and efficient technology across varied sectors.
From healthcare to automotive industries, the strict testing of AI models ensures they perform cautiously and effectively in real-world applications.
In this guide, we delve deeper into exploring several AI model testing case studies that feature the unique challenges and techniques involved in AI model testing across distinct fields.
Case Study 1: Healthcare Industry
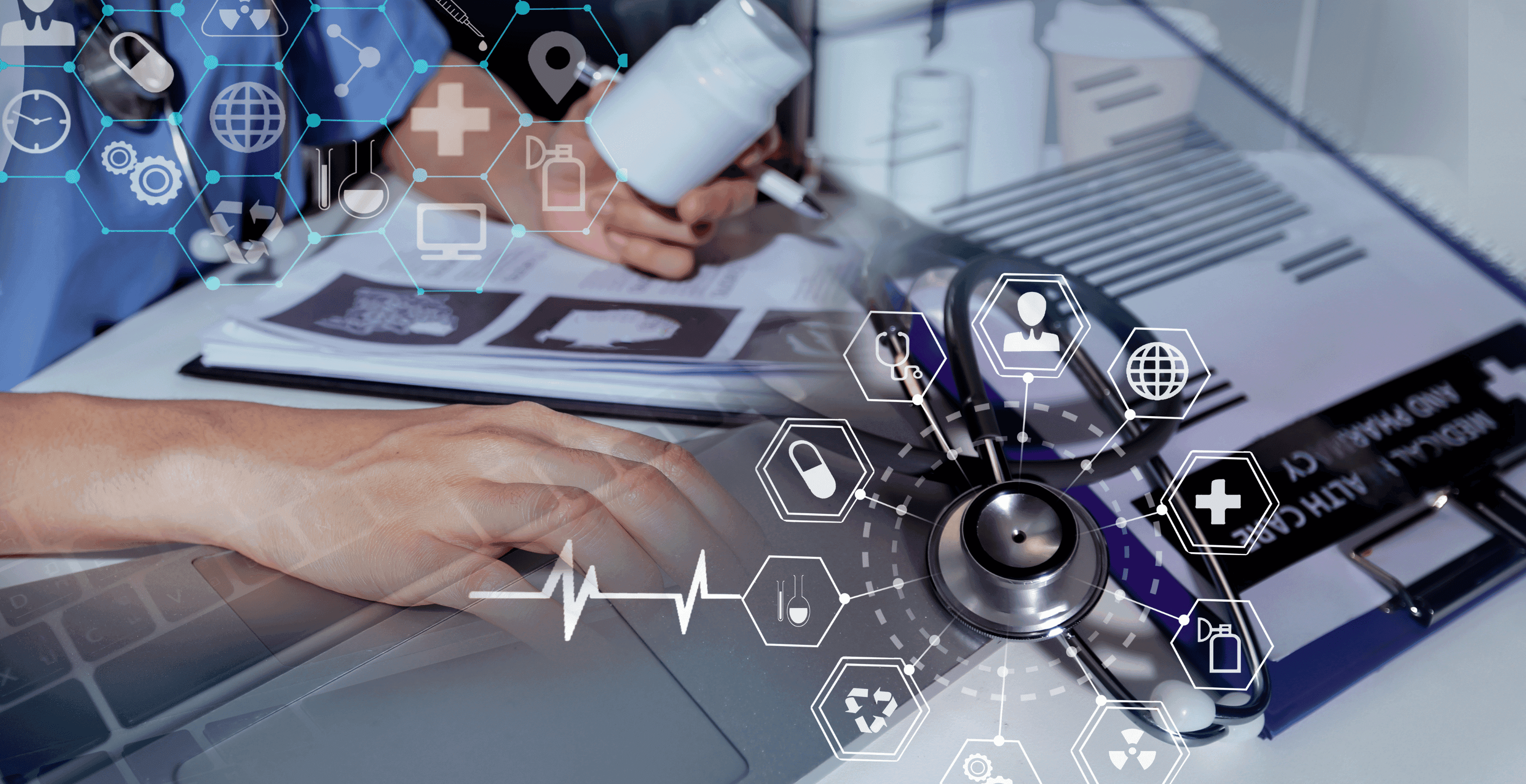
Project Description
The project revolves around using Artificial Intelligence (AI) to improve patient monitoring and enhance the fall discovery apparatus in a healthcare setting.
The AI system constantly monitors patients, especially those with mobility problems or those at high risk of stumbles, using sensors and machine learning algorithms. This technology intends to quickly alert the healthcare staff when a stumble is located or forecasted, enabling instant intervention.
AI Model Testing Challenges
Testing the AI model in this circumstance presents numerous challenges:
Ensuring Precision: This AI must precisely locate actual stumbles without misinterpreting other activities or movements as falls. High precision is critical to ensure that the staff can depend on the cautions to classify and reply to actual events.
Reducing False Alarms: Diminishing false positives is important to prevent alarm fatigue among the healthcare staff. Too many inaccurate alarms could result in desensitization, resulting in slower replies to actual stumbles or even ignoring the cautions.
Testing Methodologies
Below given are the ensuing methodologies that are engaged to address these difficulties:
Human Activity Recognition: This indulges utilizing machine learning algorithms to identify the data gathered from sensors worn by patients or incorporated into their environments. The algorithms are instructed to differentiate between distinct types of human movements and concretely recognize patterns reflective of a fall.
Data Augmentation: Improving the dataset with synthetic falls and non-fall activities to instruct the model more extensively.
Cross-Verification: Utilizing analytical approaches to verify the model’s efficacy across distinct patient enumerations and settings.
Real-Time Testing: Enforcing the system in a restrained environment where real-time observation and feedback can be utilized to fine-tune the model.
Outcome
Positioning this AI system in healthcare facilities has resulted in numerous positive results:
Improved Patient Security:- Instant discovery and notification of stumbles decrease the threat of serious injuries.
Increase Staff Efficiency: Staff can assign their time more efficiently, as the Artificial Intelligence supports observing and provides precise alerts, permitting them to concentrate more on direct patient care and other crucial tasks.
Enhanced Response Times: The system assures that healthcare facilitators can reply more instantly to actual falls, certainly decreasing the rigidity of fall-related injuries.
Overall, the utilization of AI for patient observation and stumble identification in the healthcare industry depicts a crucial progression in both patient care and functional efficacy.
Case Study 2: Automotive Industry

Project Description
In the automotive sector, AI incorporation concentrates on developing sophisticated independent vehicle maneuvering systems. These systems are made to illustrate and react to intricate driving environments without human input.
AI Model Testing Challenges
One of the predominant challenges in enforcing Artificial Intelligence in independent vehicles is assuring safety. Autonomous Vehicles must be reliable under a myriad of driving circumstances and synopsis, which requires broad testing to confirm that AI’s decision-making abilities are constantly safe. Key challenges include:
Intricacy of Real-World Synopsis: Simulating the myriad unpredictable changes that occur in real-world driving synopsis is absolutely challenging. This indulges everything from abrupt weather changes to odd behavior of other road users.
Safety Concerns: The stakes are absolutely high in independent driving. Any drawbacks in AI performance could lead to errors that may threaten human life.
Testing Methodologies
To acknowledge these challenges, an amalgamation of automated and manual testing techniques was enforced:
Automated Tests: These contain simulated environments where AI systems are subjected to a variety of driving circumstances. Automated testing tools generate various synopsis and changes, troubling the AI’s decision-making processes to ensure soundness and dependability.
Manual Verification: This indulges real-world experimentations and human oversight, where independent systems are observed by human drivers to take over if significant. This stage is important for verifying the AI’s performance in actual driving circumstances and for collecting data on any problems or inconsistencies that occur.
Outcome
The harsh and adaptable testing technique led to the progression of dependable and safe autonomous driving abilities. The AI models engaged in the navigation systems confirmed a high level of precision and correspondence, capable of managing varied and challenging driving circumstances. This triumph marks a significant advancement in the tracking of fully independent vehicles, promising improved security and efficacy on the roads.
Alright, now that we've seen how AI is revolutionizing sectors from health to automotive, let's shake things up and move the spotlight to shopping aisles and digital carts. Ready to see how AI is making our shopping smarter? Dive into Case Study 3 to find out.
Case Study 3: Retail and Consumer Goods
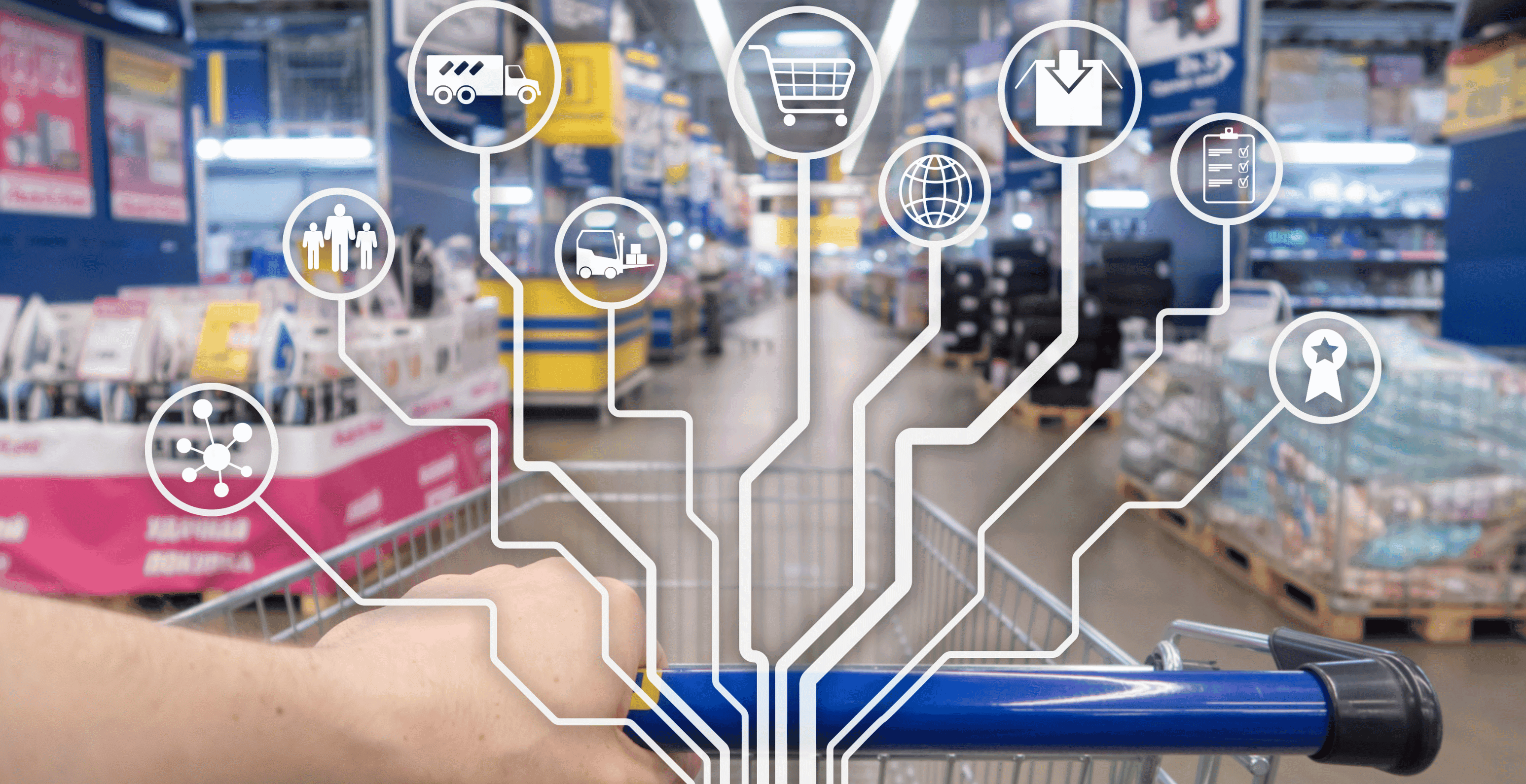
Project Description
Positioning AI in the retail sector substantially concentrates on improving personalized shopping experiences. This indulges using AI to tailor product advice, pricing plans, and marketing efforts, directly to individual customer choices and behaviors. The aim is to create a smooth, appealing and highly customized shopping journey that inspires customer loyalty and increases sales.
AI Model Testing Challenges
Examining AI models in the retail circumstances depicts numerous unique challenges:
Handling Data Security: Ensuring that the data of consumers is utilized morally and in compliance with global data protection and regulations (like GDPR) is supreme. The models must manage sensitive data safely while still being able to originate significant understanding.
Enhancing Recommendation Systems: The efficacy of AI-driven guidance systems is critical for customized experiences. These systems must be examined strictly to ensure they are not only precise but also adjustable to changing customer choices and behaviors.
Testing Methodologies
To acknowledge these challenges, the team applies particular testing techniques:
A/B Testing: This technique permits retailers to compare 2 versions of their Artificial Intelligence models to decide which one performs better in real-globe synopsis. For example, 2 distinct advice algorithms can be examined contemporaneously to see which gives more accurate suggestions to the user.
Stress Testing Machine Learning Models: Stress testing contains placing the Artificial Intelligence models through scenarios with drastic circumstances to ensure they can manage unforeseen surges in data or functioning demands. This is crucial for retail events such as Black Friday sales when system rigidness and manageability are examined to the limits.
Outcome
The planned application of these testing techniques results in crucial improvements in customer satisfaction and business progress. Customers get advantage from a more customized shopping experience that feels peculiarly tailored to their requirements, which in turn promotes greater allegiance and affiance. For ventures, this reiterates increased efficacy in inventory management, more efficient marketing, and eventually higher sales revenues.
These outcomes emphasize the significance of harsh AI model testing in creating strict, efficient AI applications in retail and consumer goods industries.
Now, buckle up because it's time to dive into the world of FinTech, where every decimal and digit is a game-changer. Let’s see how AI is crunching numbers to pave the way for smarter financial decisions. Ready? Here comes Case Study 4!
Case Study 4: Financial Technology (FinTech)

Project Description
The project concentrates on enforcing AI technologies to improve predictive analytics within the domain of market intelligence. This application of Artificial Intelligence aims to dissect vast amounts of financial data to predict the trends of the market, helping ventures and stakeholders make informed choices.
AI Model Testing Challenges
One of the main challenges faced during the execution was ensuring the precision and performance of the Artificial Intelligence models under diverse and often unpredictable market circumstances. Financial markets are affected by several elements, indulging economic indicators, political events, and social tendencies which all impact the constancy of the models.
Testing Methodologies
To acknowledge these challenges, the team engaged a blend of behavioral tests and A/B testing. Behavioral tests helped comprehend how the model demeanor under distinct market scenarios, especially during strange or drastic market circumstances. A/B testing, on the other hand, permits the team to test with distinct versions of the model to decide which provided the most dependable predictions.
Outcome
The rigid testing techniques contributed to the progression of rigid AI systems capable of delivering dependable predictive insights. This empowered financial choice makers with improved analytical tools, substantially assisting in strategy articulation and risk evaluation.
Conclusion
As these case studies illustrate, AI model testing plays a crucial role in ensuring functional success across several industries. The future direction of Artificial Intelligence model testing indulges in not only clarifying these methodologies but also incorporating new technologies that improves the precision and efficacy of AI applications.
As we look ahead, the continued focus on strict testing and quality conviction will undoubtedly drive the next wave of inventiveness in AI technology, making it an even more essential tool in our everyday lives.
FAQs
What is the use case of AI in the Automotive Industry?
AI has numerous dynamic use cases in the automotive industry, modifying how vehicles are designed, built and propelled. Below given are some key applications:
Autonomous Driving: AI is the backbone of self-driving technology. It proceeds data from vehicle sensors and cameras to steer roads, comprehend traffic circumstances, and make real-time choices, improving security and efficacy.
Predictive Maintenance: AI Algorithms dissects data from various vehicle detectors to predict when parts might fail or need maintenance. This helps in decreasing layoff and maintenance charges and enhances the life span of vehicles.
Manufacturing Automation: Robots and automation systems powered by Artificial Intelligence enhance the accuracy and efficacy of automobile assembly in manufacturing plants. These systems can adjust for distinct tasks without the requirement for reprogramming.
What is responsible AI in the automotive industry?
Responsible AI in the automotive industry refers to the ethical design, growth and execution of AI technologies in a manner that is ethical, safe, and advantageous to society. This indulges various key aspects:
Safety and Reliability: Artificial Intelligence systems utilized in vehicles, like independent driving systems and driver-hinder technologies, must be carefully examined and demonstrated to be secured under distinctive circumstances.
Transparency and Accountability: It's crucial for automotive firms to be transparent about how their AI systems work. This means giving clear details on how decisions are made by AI technologies and being liable for the outcomes.
Privacy and Data Security: With vehicles gathering vast amounts of information, indulging personal details about drivers and their practice, strong amounts must be in place to safeguard this data. Ensuring privacy and safeguarding against data violation are critical.
As we examine the quickly progressing globe of Artificial Intelligence, you'll see testing AI models isn't just a step in growth—it's a foundation for positioning dependable and efficient technology across varied sectors.
From healthcare to automotive industries, the strict testing of AI models ensures they perform cautiously and effectively in real-world applications.
In this guide, we delve deeper into exploring several AI model testing case studies that feature the unique challenges and techniques involved in AI model testing across distinct fields.
Case Study 1: Healthcare Industry
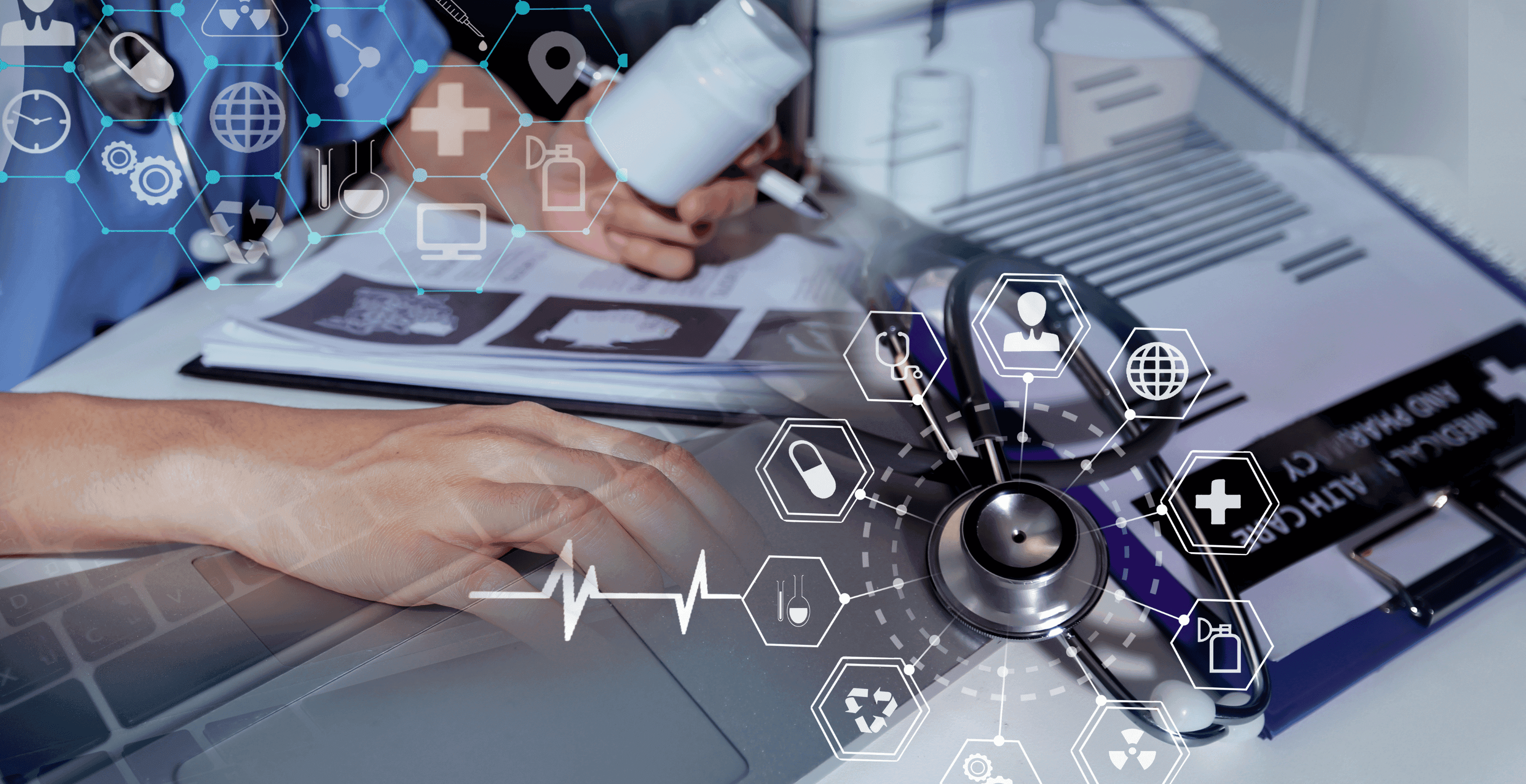
Project Description
The project revolves around using Artificial Intelligence (AI) to improve patient monitoring and enhance the fall discovery apparatus in a healthcare setting.
The AI system constantly monitors patients, especially those with mobility problems or those at high risk of stumbles, using sensors and machine learning algorithms. This technology intends to quickly alert the healthcare staff when a stumble is located or forecasted, enabling instant intervention.
AI Model Testing Challenges
Testing the AI model in this circumstance presents numerous challenges:
Ensuring Precision: This AI must precisely locate actual stumbles without misinterpreting other activities or movements as falls. High precision is critical to ensure that the staff can depend on the cautions to classify and reply to actual events.
Reducing False Alarms: Diminishing false positives is important to prevent alarm fatigue among the healthcare staff. Too many inaccurate alarms could result in desensitization, resulting in slower replies to actual stumbles or even ignoring the cautions.
Testing Methodologies
Below given are the ensuing methodologies that are engaged to address these difficulties:
Human Activity Recognition: This indulges utilizing machine learning algorithms to identify the data gathered from sensors worn by patients or incorporated into their environments. The algorithms are instructed to differentiate between distinct types of human movements and concretely recognize patterns reflective of a fall.
Data Augmentation: Improving the dataset with synthetic falls and non-fall activities to instruct the model more extensively.
Cross-Verification: Utilizing analytical approaches to verify the model’s efficacy across distinct patient enumerations and settings.
Real-Time Testing: Enforcing the system in a restrained environment where real-time observation and feedback can be utilized to fine-tune the model.
Outcome
Positioning this AI system in healthcare facilities has resulted in numerous positive results:
Improved Patient Security:- Instant discovery and notification of stumbles decrease the threat of serious injuries.
Increase Staff Efficiency: Staff can assign their time more efficiently, as the Artificial Intelligence supports observing and provides precise alerts, permitting them to concentrate more on direct patient care and other crucial tasks.
Enhanced Response Times: The system assures that healthcare facilitators can reply more instantly to actual falls, certainly decreasing the rigidity of fall-related injuries.
Overall, the utilization of AI for patient observation and stumble identification in the healthcare industry depicts a crucial progression in both patient care and functional efficacy.
Case Study 2: Automotive Industry

Project Description
In the automotive sector, AI incorporation concentrates on developing sophisticated independent vehicle maneuvering systems. These systems are made to illustrate and react to intricate driving environments without human input.
AI Model Testing Challenges
One of the predominant challenges in enforcing Artificial Intelligence in independent vehicles is assuring safety. Autonomous Vehicles must be reliable under a myriad of driving circumstances and synopsis, which requires broad testing to confirm that AI’s decision-making abilities are constantly safe. Key challenges include:
Intricacy of Real-World Synopsis: Simulating the myriad unpredictable changes that occur in real-world driving synopsis is absolutely challenging. This indulges everything from abrupt weather changes to odd behavior of other road users.
Safety Concerns: The stakes are absolutely high in independent driving. Any drawbacks in AI performance could lead to errors that may threaten human life.
Testing Methodologies
To acknowledge these challenges, an amalgamation of automated and manual testing techniques was enforced:
Automated Tests: These contain simulated environments where AI systems are subjected to a variety of driving circumstances. Automated testing tools generate various synopsis and changes, troubling the AI’s decision-making processes to ensure soundness and dependability.
Manual Verification: This indulges real-world experimentations and human oversight, where independent systems are observed by human drivers to take over if significant. This stage is important for verifying the AI’s performance in actual driving circumstances and for collecting data on any problems or inconsistencies that occur.
Outcome
The harsh and adaptable testing technique led to the progression of dependable and safe autonomous driving abilities. The AI models engaged in the navigation systems confirmed a high level of precision and correspondence, capable of managing varied and challenging driving circumstances. This triumph marks a significant advancement in the tracking of fully independent vehicles, promising improved security and efficacy on the roads.
Alright, now that we've seen how AI is revolutionizing sectors from health to automotive, let's shake things up and move the spotlight to shopping aisles and digital carts. Ready to see how AI is making our shopping smarter? Dive into Case Study 3 to find out.
Case Study 3: Retail and Consumer Goods
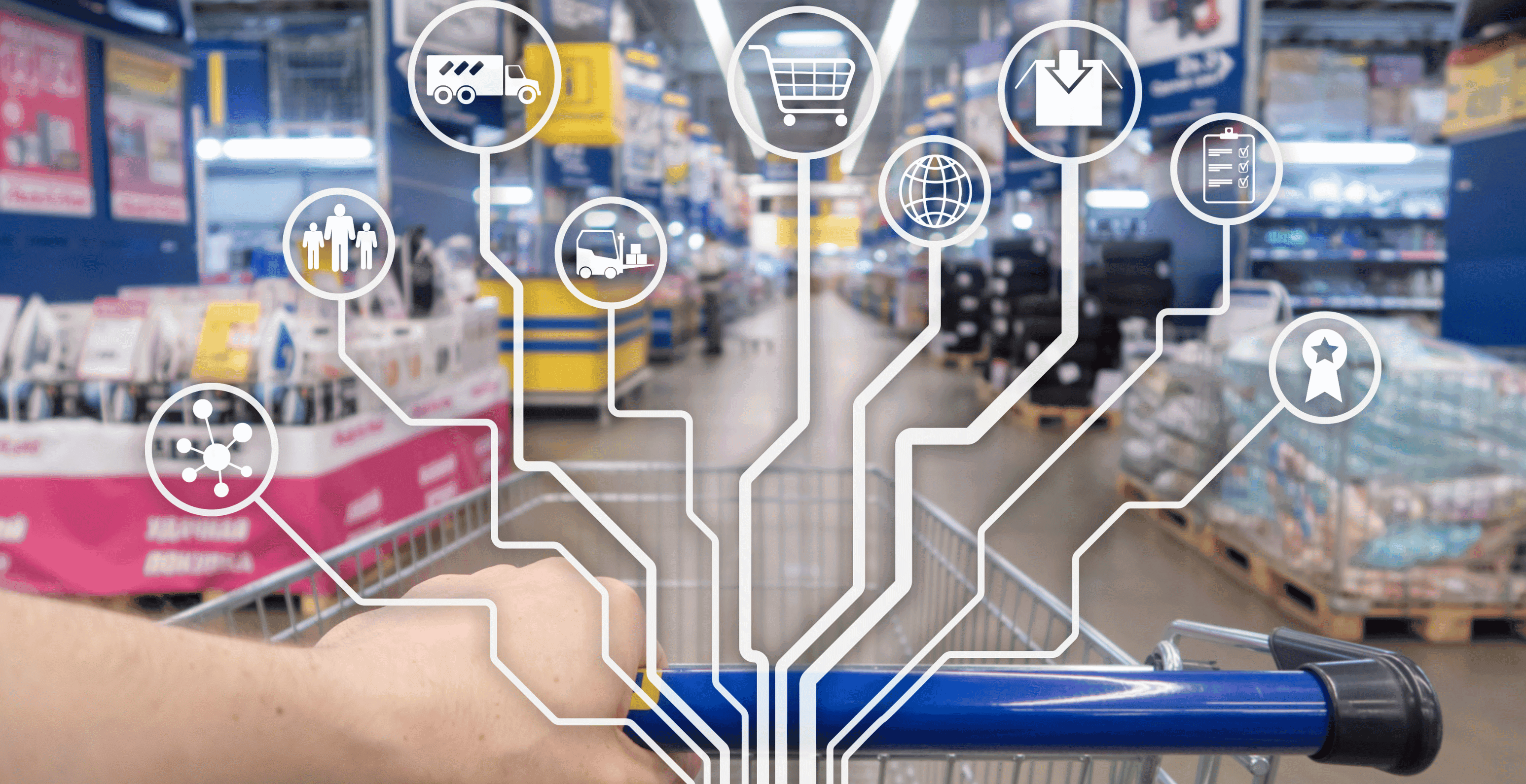
Project Description
Positioning AI in the retail sector substantially concentrates on improving personalized shopping experiences. This indulges using AI to tailor product advice, pricing plans, and marketing efforts, directly to individual customer choices and behaviors. The aim is to create a smooth, appealing and highly customized shopping journey that inspires customer loyalty and increases sales.
AI Model Testing Challenges
Examining AI models in the retail circumstances depicts numerous unique challenges:
Handling Data Security: Ensuring that the data of consumers is utilized morally and in compliance with global data protection and regulations (like GDPR) is supreme. The models must manage sensitive data safely while still being able to originate significant understanding.
Enhancing Recommendation Systems: The efficacy of AI-driven guidance systems is critical for customized experiences. These systems must be examined strictly to ensure they are not only precise but also adjustable to changing customer choices and behaviors.
Testing Methodologies
To acknowledge these challenges, the team applies particular testing techniques:
A/B Testing: This technique permits retailers to compare 2 versions of their Artificial Intelligence models to decide which one performs better in real-globe synopsis. For example, 2 distinct advice algorithms can be examined contemporaneously to see which gives more accurate suggestions to the user.
Stress Testing Machine Learning Models: Stress testing contains placing the Artificial Intelligence models through scenarios with drastic circumstances to ensure they can manage unforeseen surges in data or functioning demands. This is crucial for retail events such as Black Friday sales when system rigidness and manageability are examined to the limits.
Outcome
The planned application of these testing techniques results in crucial improvements in customer satisfaction and business progress. Customers get advantage from a more customized shopping experience that feels peculiarly tailored to their requirements, which in turn promotes greater allegiance and affiance. For ventures, this reiterates increased efficacy in inventory management, more efficient marketing, and eventually higher sales revenues.
These outcomes emphasize the significance of harsh AI model testing in creating strict, efficient AI applications in retail and consumer goods industries.
Now, buckle up because it's time to dive into the world of FinTech, where every decimal and digit is a game-changer. Let’s see how AI is crunching numbers to pave the way for smarter financial decisions. Ready? Here comes Case Study 4!
Case Study 4: Financial Technology (FinTech)

Project Description
The project concentrates on enforcing AI technologies to improve predictive analytics within the domain of market intelligence. This application of Artificial Intelligence aims to dissect vast amounts of financial data to predict the trends of the market, helping ventures and stakeholders make informed choices.
AI Model Testing Challenges
One of the main challenges faced during the execution was ensuring the precision and performance of the Artificial Intelligence models under diverse and often unpredictable market circumstances. Financial markets are affected by several elements, indulging economic indicators, political events, and social tendencies which all impact the constancy of the models.
Testing Methodologies
To acknowledge these challenges, the team engaged a blend of behavioral tests and A/B testing. Behavioral tests helped comprehend how the model demeanor under distinct market scenarios, especially during strange or drastic market circumstances. A/B testing, on the other hand, permits the team to test with distinct versions of the model to decide which provided the most dependable predictions.
Outcome
The rigid testing techniques contributed to the progression of rigid AI systems capable of delivering dependable predictive insights. This empowered financial choice makers with improved analytical tools, substantially assisting in strategy articulation and risk evaluation.
Conclusion
As these case studies illustrate, AI model testing plays a crucial role in ensuring functional success across several industries. The future direction of Artificial Intelligence model testing indulges in not only clarifying these methodologies but also incorporating new technologies that improves the precision and efficacy of AI applications.
As we look ahead, the continued focus on strict testing and quality conviction will undoubtedly drive the next wave of inventiveness in AI technology, making it an even more essential tool in our everyday lives.
FAQs
What is the use case of AI in the Automotive Industry?
AI has numerous dynamic use cases in the automotive industry, modifying how vehicles are designed, built and propelled. Below given are some key applications:
Autonomous Driving: AI is the backbone of self-driving technology. It proceeds data from vehicle sensors and cameras to steer roads, comprehend traffic circumstances, and make real-time choices, improving security and efficacy.
Predictive Maintenance: AI Algorithms dissects data from various vehicle detectors to predict when parts might fail or need maintenance. This helps in decreasing layoff and maintenance charges and enhances the life span of vehicles.
Manufacturing Automation: Robots and automation systems powered by Artificial Intelligence enhance the accuracy and efficacy of automobile assembly in manufacturing plants. These systems can adjust for distinct tasks without the requirement for reprogramming.
What is responsible AI in the automotive industry?
Responsible AI in the automotive industry refers to the ethical design, growth and execution of AI technologies in a manner that is ethical, safe, and advantageous to society. This indulges various key aspects:
Safety and Reliability: Artificial Intelligence systems utilized in vehicles, like independent driving systems and driver-hinder technologies, must be carefully examined and demonstrated to be secured under distinctive circumstances.
Transparency and Accountability: It's crucial for automotive firms to be transparent about how their AI systems work. This means giving clear details on how decisions are made by AI technologies and being liable for the outcomes.
Privacy and Data Security: With vehicles gathering vast amounts of information, indulging personal details about drivers and their practice, strong amounts must be in place to safeguard this data. Ensuring privacy and safeguarding against data violation are critical.
Subscribe to our newsletter to never miss an update
Other articles


Agents Talk, Models Think: A2A + MCP for Enterprise Agentic AI

Riya Parikh, Nitai Agarwal
Apr 27, 2025
Read the article
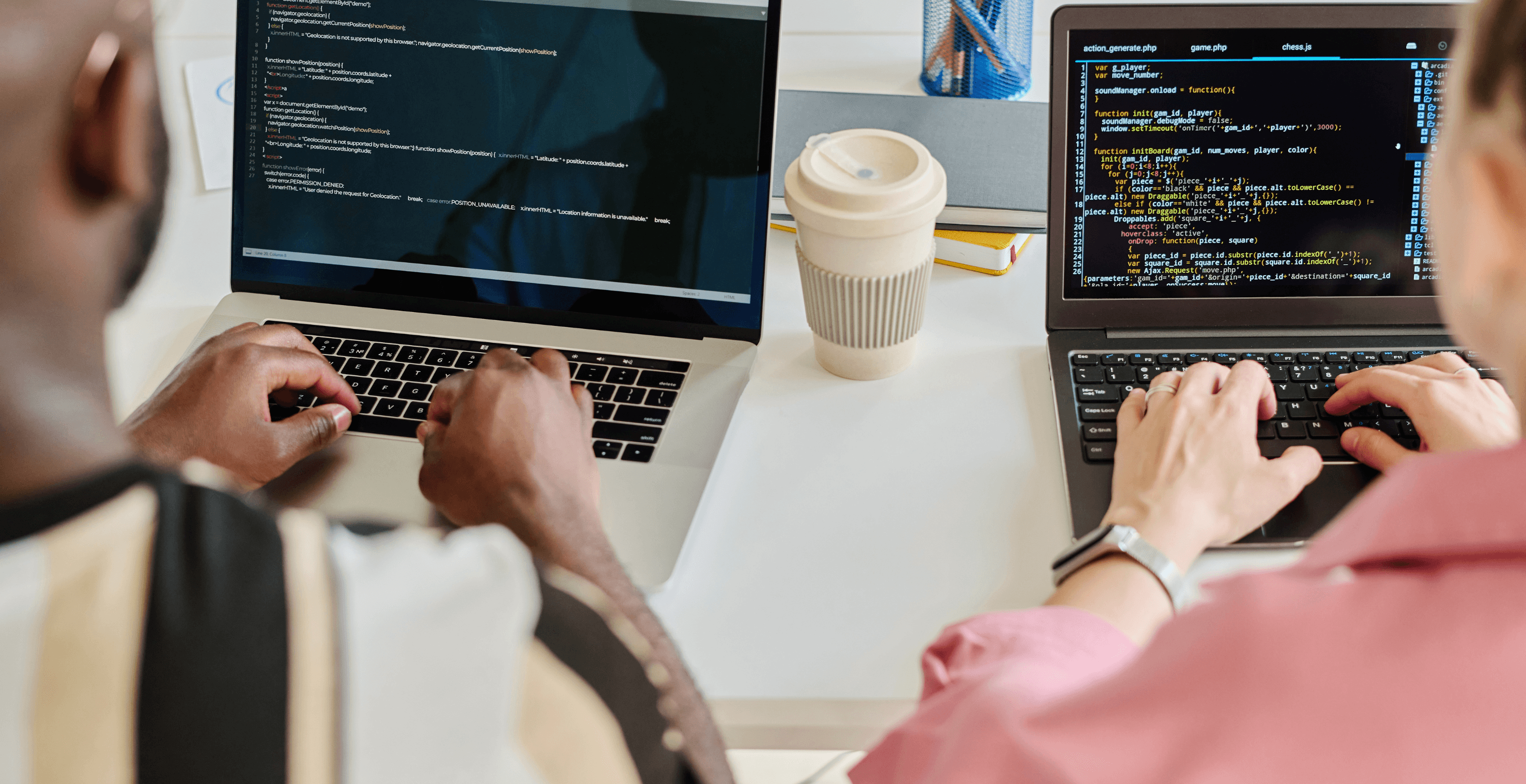
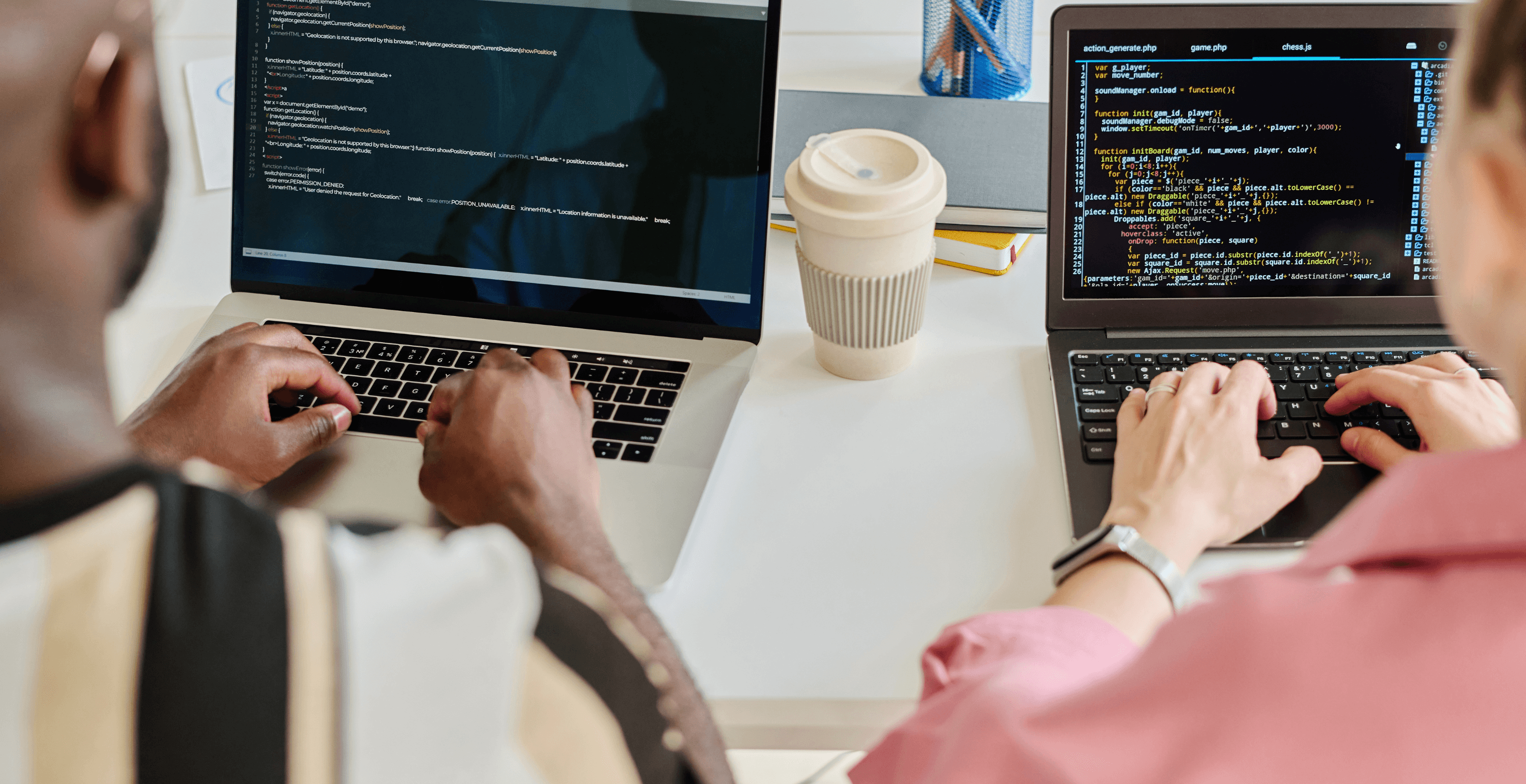
Understanding NeMo Guardrails: A Toolkit for LLM Security

Rehan Asif
Dec 24, 2024
Read the article


Understanding Differences in Large vs Small Language Models (LLM vs SLM)

Rehan Asif
Dec 21, 2024
Read the article