Developing AI Agent Strategies Using GPT
Developing AI Agent Strategies Using GPT
Developing AI Agent Strategies Using GPT
Jigar Gupta
Jul 24, 2024




Ever wondered how AI agent GPT AI revolutionized technology? Comprehending AI agent strategies and the crucial role of GPT in their expansion is critical. In this article, you'll delve into the strategies powering AI agents and find why GPT plays a crucial role in shaping their capabilities. Whether you're new to AI or looking to deepen your knowledge, you're about to uncover the captivating world of AI agents and their dependence on GPT technology. Let's dive in!
AI Agents and Their Capabilities
Want to know about AI Agents and what they are capable of? Let’s know in detail:
Definition and Key Concepts of AI Agents
AI agents are software programs designed to perform literal tasks separately, using artificial intelligence to make decisions and take actions. These agents dissect information, learn from interactions, and adjust their behavior over time, emulating human-like intelligence.
Difference between ChatGPT and AI agents
ChatGPT, like Agent GPT AI here, is a type of AI agent concentrated on natural language processing and conversation. While ChatGPT shines in producing text based on input, AI agents can vary extensively in their functionalities—from managing schedules and tasks to driving cars or diagnosing diseases.
Examples of Existing AI Agent Projects
Some notorious AI agent projects include virtual assistants like Siri and Alexa, which help users with mundane tasks. Autonomous vehicles, guided by AI, represent another frontier. Moreover, AI agents in healthcare dissect medical data to recommend treatments, exhibiting the broad applications of AI in various fields.
Now that we've seen some of the fantastic AI projects out there let's explore how different workflows impact the performance of these AI agents.
Discover how advancements in LLM and generative AI infrastructure are shaping the future of technology. Read our detailed guide on Exploring the Future of LLM and Generative AI Infrastructure.
Iterative Agent Workflows vs. Zero-Shot Mode
Welcome to the future of AI, where your apprehension of iterative agent workflows and zero-shot modes can revolutionize your approach to AI enforcement. Let's dive into these concepts and see how they can transform your use of Agent GPT AI.
Current Zero-Shot Usage in LLMs
When you think about zero-shot learning, you're noticing the cutting edge of language model capabilities. AI executes tasks in zero-shot mode without any prior precise training on those tasks. Envision asks an AI to translate a sentence into a language it has never confronted before or to craft a creative story based on an ambiguous prompt. Sounds like magic, right? In the synopsis of Large Language Models (LLMs) like GPT-3, zero-shot learning is used considerably for numerous applications, from responding to questions to creating content on the fly.
You benefit from zero-shot learning because it substantially reduces the time and effort needed to train models on new tasks. It's like having a Swiss army knife of AI, ready to tackle any challenge you throw at it with minimal preparation.
Alright, so we've understood the basics of zero-shot usage, but what's the flip side?
Challenges and Efficiency of Zero-Shot Generation
However, zero-shot learning isn't without its obstacles. One of the biggest challenges is precision. Because the AI hasn't been concretely trained on your particular task, its answers can sometimes be off the mark. You might ask it to draft a venture proposal, and instead, it gives you something more suited to a casual blog post. Thwarting, right?
Another problem is efficiency. Zero-shot generation can be computationally profound because the model is drawing on a vast array of comprehension to perform tasks it wasn't clearly trained to do. This means more refining power and longer response times. Plus, there's the ambiguity factor – the AI might demonstrate your prompt in unanticipated ways, leading to outputs that don't quite hit the mark.
Intrigued by zero-shot learning? Wait until you see how iterative workflows can make it even better.
Benefits of Iterative Agent Workflows
Enter iterative agent workflows, your AI's best buddy. Unlike zero-shot mode, iterative workflows involve continuous feedback loops where the AI's output is processed over numerous iterations. This approach can fiercely improve the quality and pertinence of the produced content.
Iterative agent workflows use the power of fine-tuning. You start with a foremost output, provide feedback, and the AI adapts its ensuing responses accordingly. This method reduces errors and improves the precision of the outcome. Envision carving a statue: with each sculpt, the form becomes explicit and more defined. That's exactly what iterative workflows do for your AI-generated content.
Moreover, iterative workflows can substantially enhance efficiency over time. As the AI grasps from each iteration, it becomes better at comprehending and implementing your literal needs, reducing the need for comprehensive rework. This not only saves time but also upgrades resource usage, making your AI deployment more cost-efficient.
By mastering both approaches, you can use the full potential of Agent GPT AI, revolutionizing your workflows and unleashing new levels of workflow and innovation.
Curious yet? Let's break down the step-by-step process to make the most out of your AI agent.
If you're intrigued in using AI for improved software development, don't miss our guide on Integrating AI Models with Continuous Integration Systems.
Key Steps in an Iterative Workflow
Agent GPT AI sleek your projects. To make the most out of this robust tool, you need to follow a planned, iterative workflow. Here’s how you can accomplish success:
Planning an Outline and Tasks
Commence by mapping out your project. Break down the project into tractable tasks. Think of this as your roadmap. It’s vital to have a clear abstract to guide you through each phase. This foremost strategy saves time and sets you up for streamlined implementation.
Executing the Top Task First
Now, tackle the most vital task first. This might be the core element of your project. By concentrating on the top priority, you ensure that the most crucial features are acknowledged early on. This sets a robust foundation for ensuing tasks.
Review and Revise Based on Feedback
Once you’ve implemented a task, pause for feedback. Team members, stakeholders, or even AI can provide feedback. Use this feedback to make essential revisions. Constant enhancement is the name of the game.
Reprioritize Tasks for Efficiency
After amending, reassess your remaining tasks. Adapt priorities based on what you’ve learned. Some tasks may shift in significance, and that’s okay. The aim is to stay dexterous and effective.
Iterate Until Goal Completion
Eventually, iterate this cycle. Implement, retrospect, revise, and reprioritize. Keep repeating until you accomplish your project goals. This recurring approach ensures that you’re continuously enhancing and adjusting to new data.
By adhering to these steps, you use Agent GPT AI’s full potential, making your workflow sleek and more efficient.
After nailing down the iterative workflow, it's time to see how these practices can elevate your performance metrics.
Explore our latest insights on using AI for improved retail customer experiences to discover how artificial intelligence can revolutionize your retail strategy. Read our detailed guide on Using AI For Enhanced Retail Customer Experiences.
Enhancing Performance Metrics with Agent Workflow
Agent GPT AI is a robust tool which can transform how you handle your performance metrics, ensuring you get the most out of your systems. Let’s learn how Agent GPT AI improves your performance through human assessment, a comparison between GPT-3.5 and GPT-4, and the effect of agent workflows.
HumanEval Coding Benchmark Analysis
When it comes to measuring performance, the HumanEval coding benchmark is a vital tool. It gives a thorough inspection of how AI models execute coding tasks, giving you clear perceptions of their capabilities. With Agent GPT AI, you can sleek this process, making it more effective and precise. You'll find that using Agent GPT AI for HumanEval coding benchmarks helps you determine strengths and weaknesses swiftly, permitting tormented enhancements and better overall performance.
Now that you've got the basics, let's dive deeper into a direct comparison between GPT-3.5 and GPT-4 – the reigning kings of AI models.
GPT-3.5 and GPT-4 Performance Comparison
Comprehending the distinctions between GPT-3.5 and GPT-4 is key to upgrading your AI productivity. GPT-4 has made substantial strides over its predecessor, providing improved language apprehension, better contextual awareness, and enhanced response precision. By using Agent GPT AI, you can smoothly incorporate these progressions into your system. This incorporation not only elevates performance but also ensures you're using the most expanded technology attainable, giving you a fierce edge.
Impact of Agent Workflows on Performance Improvements
Agent workflows are groundbreakers when it comes to enhancing performance metrics. They permit automated, intelligent task management, reducing the time and effort needed for manual oversight. With Agent GPT AI, this productivity becomes even more robust. You'll see enhancements in efficacy, precision, and overall workflow. Agent workflows help in breaking down intricate tasks into tractable segments, ensuring that each part of your system executes at peak performance.
Integrating Agent GPT AI into your performance metrics plan is a smart move. It improves the HumanEval coding benchmark inspection, provides a clear comparison between GPT-3.5 and GPT-4, and substantially enhances performance through intelligent agent workflows. By clasping these advancements, you're setting yourself up for success, ensuring your systems are always running at their best.
Great, you now understand performance metrics, but what about the core of agent design that makes it all possible?
Explore more with our article on Innovations In AI For Healthcare. Read our guide and learn various ways to improve the workflow in the healthcare sector with the help of AI!
Framework for Effective Agent Design Patterns
Ever wondered what makes an Agent GPT AI tick? Let’s learn about the core design patterns that revolutionize a simple agent into a dominator of workflow and intelligence.
Reflection: LLMs Improving Their Work
What if you have a personal assistant that gets better every time it helps you? That's what reflection is all about. Agent GPT AI uses large language models (LLMs) to retrospect and improve their outputs. These agents reflect on past performance, learn from their mistakes, upgrade their responses, and provide gradually more precise and pertinent data. It’s like having an assistant that constantly teaches itself to serve you better.
Tool Use: Utilizing Web Search and Other Tools
Knowledge is power, and attaining the right tools can make all the distinctions. Agent GPT AI shines by smoothly incorporating web searches and other digital tools. Need the up-to-date information? The agent can clean the web in seconds. Looking for explicit software usefulness? The AI taps into its digital toolbox to find the best solution. You get a thorough response swiftly and effectively without having to lift a finger.
Planning: Creating and Executing Multi-Step Plans
Intricate tasks often need pragmatic strategizing and accurate implementation. Agent GPT AI excels by designing and adhering to multistep plans. Whether you're managing a project, planning a marketing campaign, or simply arranging your week, the AI generates a step-by-step blueprint to accomplish your goals. With its diligent planning capabilities, you can rest easy knowing every aspect of your task is covered.
By getting skilled in these key design patterns, Agent GPT AI becomes more than just a tool—it’s a revolutionary force in your digital armaments. Use the power of reflection, tool use, strategic planning, and collaboration to unleash new levels of efficacy and innovation.
Do you have a handle on the design patterns? Let's now get into the nitty-gritty of developing and deploying your AI agents.
For a deeper comprehension of applicable applications, check out our article on In-Depth Case Studies in AI Model Testing: Exploring Real-World Applications and Insights.
Developing and Deploying AI Agents
Let’s learn how you can smoothly evolve and deploy your very own AI agents:
Automatic Setup Processes
Automatic setup processes sleek the initial configuration to ensure you can get started with minimal hassle. You’ll find that the automation not only saves time but also reduces the potential for human error. Your concentration can stay on what matters most: using AI for potent outcomes.
Prerequisites for Deployment
Before you hit the ground running, there are a few prerequisites you need to tick off:
Data Readiness: Ensure you have immaculate, well-organized data. The quality of input data directly impacts the performance of your AI agent.
Infrastructure: Validate that your hardware and software infrastructure meets the demands. This includes adequate computational power and congenial software versions.
Access Permissions: Accord the essential access permissions to pertinent team members and systems. Proper access management averts future obstacles.
Training Resources: Equip your team with the right resources. Training materials and documentation are crucial for streamlined functioning.
Step-by-Step Setup Instructions
Ready to set up your Agent GPT AI? Follow these direct steps to get your AI agent up and running:
Download the AI Software:
Visit the official website and download the latest version of Agent GPT AI.
Ensure you choose the correct version congenial to your operating system.
Install the Software:
Run the installation file and follow the on-screen guidelines.
Choose the default installation path or choose a custom location if preferred.
Configure Initial Settings:
Launch the software and go to the settings menu.
Input your license key or activation code.
Configure basic settings such as language preference, default data directories, and logging options.
Connect to Data Sources:
Go to the data incorporation section and add your data sources.
Provide significant credentials and ensure successful connections.
Set Up User Accounts:
Create user accounts for your team members.
Assign apt roles and attain levels based on their responsibilities.
Train Your AI Agent:
Load your initial data set and start the training process.
Monitor the training progress and make adaptations as required to enhance precision.
Deploy the AI Agent:
Once training is complete, deploy the AI agent to your desired environment.
Conduct a series of tests to ensure it operates as expected.
Monitor and Optimize:
Frequently check the performance metrics.
Make constant enhancements based on feedback and performance data.
By adhering to these steps, you’ll have your Agent GPT AI functional in no time, ready to transform your functioning.
If you're intrigued by optimizing data management and ensuring compliance in AI projects, make sure to check out our detailed guide on the Best Practices in Data Governance for AI.
Conclusion
To conclude the guide, mastering AI agent strategies using GPT opens doors to unmatched efficacy and performance enhancements. Explore these strategies to innovate and lead in your field.
Ready to take your AI agent strategies to the next level? Sign up with Raga AI and unleash the full potential of GPT in your projects.
Ever wondered how AI agent GPT AI revolutionized technology? Comprehending AI agent strategies and the crucial role of GPT in their expansion is critical. In this article, you'll delve into the strategies powering AI agents and find why GPT plays a crucial role in shaping their capabilities. Whether you're new to AI or looking to deepen your knowledge, you're about to uncover the captivating world of AI agents and their dependence on GPT technology. Let's dive in!
AI Agents and Their Capabilities
Want to know about AI Agents and what they are capable of? Let’s know in detail:
Definition and Key Concepts of AI Agents
AI agents are software programs designed to perform literal tasks separately, using artificial intelligence to make decisions and take actions. These agents dissect information, learn from interactions, and adjust their behavior over time, emulating human-like intelligence.
Difference between ChatGPT and AI agents
ChatGPT, like Agent GPT AI here, is a type of AI agent concentrated on natural language processing and conversation. While ChatGPT shines in producing text based on input, AI agents can vary extensively in their functionalities—from managing schedules and tasks to driving cars or diagnosing diseases.
Examples of Existing AI Agent Projects
Some notorious AI agent projects include virtual assistants like Siri and Alexa, which help users with mundane tasks. Autonomous vehicles, guided by AI, represent another frontier. Moreover, AI agents in healthcare dissect medical data to recommend treatments, exhibiting the broad applications of AI in various fields.
Now that we've seen some of the fantastic AI projects out there let's explore how different workflows impact the performance of these AI agents.
Discover how advancements in LLM and generative AI infrastructure are shaping the future of technology. Read our detailed guide on Exploring the Future of LLM and Generative AI Infrastructure.
Iterative Agent Workflows vs. Zero-Shot Mode
Welcome to the future of AI, where your apprehension of iterative agent workflows and zero-shot modes can revolutionize your approach to AI enforcement. Let's dive into these concepts and see how they can transform your use of Agent GPT AI.
Current Zero-Shot Usage in LLMs
When you think about zero-shot learning, you're noticing the cutting edge of language model capabilities. AI executes tasks in zero-shot mode without any prior precise training on those tasks. Envision asks an AI to translate a sentence into a language it has never confronted before or to craft a creative story based on an ambiguous prompt. Sounds like magic, right? In the synopsis of Large Language Models (LLMs) like GPT-3, zero-shot learning is used considerably for numerous applications, from responding to questions to creating content on the fly.
You benefit from zero-shot learning because it substantially reduces the time and effort needed to train models on new tasks. It's like having a Swiss army knife of AI, ready to tackle any challenge you throw at it with minimal preparation.
Alright, so we've understood the basics of zero-shot usage, but what's the flip side?
Challenges and Efficiency of Zero-Shot Generation
However, zero-shot learning isn't without its obstacles. One of the biggest challenges is precision. Because the AI hasn't been concretely trained on your particular task, its answers can sometimes be off the mark. You might ask it to draft a venture proposal, and instead, it gives you something more suited to a casual blog post. Thwarting, right?
Another problem is efficiency. Zero-shot generation can be computationally profound because the model is drawing on a vast array of comprehension to perform tasks it wasn't clearly trained to do. This means more refining power and longer response times. Plus, there's the ambiguity factor – the AI might demonstrate your prompt in unanticipated ways, leading to outputs that don't quite hit the mark.
Intrigued by zero-shot learning? Wait until you see how iterative workflows can make it even better.
Benefits of Iterative Agent Workflows
Enter iterative agent workflows, your AI's best buddy. Unlike zero-shot mode, iterative workflows involve continuous feedback loops where the AI's output is processed over numerous iterations. This approach can fiercely improve the quality and pertinence of the produced content.
Iterative agent workflows use the power of fine-tuning. You start with a foremost output, provide feedback, and the AI adapts its ensuing responses accordingly. This method reduces errors and improves the precision of the outcome. Envision carving a statue: with each sculpt, the form becomes explicit and more defined. That's exactly what iterative workflows do for your AI-generated content.
Moreover, iterative workflows can substantially enhance efficiency over time. As the AI grasps from each iteration, it becomes better at comprehending and implementing your literal needs, reducing the need for comprehensive rework. This not only saves time but also upgrades resource usage, making your AI deployment more cost-efficient.
By mastering both approaches, you can use the full potential of Agent GPT AI, revolutionizing your workflows and unleashing new levels of workflow and innovation.
Curious yet? Let's break down the step-by-step process to make the most out of your AI agent.
If you're intrigued in using AI for improved software development, don't miss our guide on Integrating AI Models with Continuous Integration Systems.
Key Steps in an Iterative Workflow
Agent GPT AI sleek your projects. To make the most out of this robust tool, you need to follow a planned, iterative workflow. Here’s how you can accomplish success:
Planning an Outline and Tasks
Commence by mapping out your project. Break down the project into tractable tasks. Think of this as your roadmap. It’s vital to have a clear abstract to guide you through each phase. This foremost strategy saves time and sets you up for streamlined implementation.
Executing the Top Task First
Now, tackle the most vital task first. This might be the core element of your project. By concentrating on the top priority, you ensure that the most crucial features are acknowledged early on. This sets a robust foundation for ensuing tasks.
Review and Revise Based on Feedback
Once you’ve implemented a task, pause for feedback. Team members, stakeholders, or even AI can provide feedback. Use this feedback to make essential revisions. Constant enhancement is the name of the game.
Reprioritize Tasks for Efficiency
After amending, reassess your remaining tasks. Adapt priorities based on what you’ve learned. Some tasks may shift in significance, and that’s okay. The aim is to stay dexterous and effective.
Iterate Until Goal Completion
Eventually, iterate this cycle. Implement, retrospect, revise, and reprioritize. Keep repeating until you accomplish your project goals. This recurring approach ensures that you’re continuously enhancing and adjusting to new data.
By adhering to these steps, you use Agent GPT AI’s full potential, making your workflow sleek and more efficient.
After nailing down the iterative workflow, it's time to see how these practices can elevate your performance metrics.
Explore our latest insights on using AI for improved retail customer experiences to discover how artificial intelligence can revolutionize your retail strategy. Read our detailed guide on Using AI For Enhanced Retail Customer Experiences.
Enhancing Performance Metrics with Agent Workflow
Agent GPT AI is a robust tool which can transform how you handle your performance metrics, ensuring you get the most out of your systems. Let’s learn how Agent GPT AI improves your performance through human assessment, a comparison between GPT-3.5 and GPT-4, and the effect of agent workflows.
HumanEval Coding Benchmark Analysis
When it comes to measuring performance, the HumanEval coding benchmark is a vital tool. It gives a thorough inspection of how AI models execute coding tasks, giving you clear perceptions of their capabilities. With Agent GPT AI, you can sleek this process, making it more effective and precise. You'll find that using Agent GPT AI for HumanEval coding benchmarks helps you determine strengths and weaknesses swiftly, permitting tormented enhancements and better overall performance.
Now that you've got the basics, let's dive deeper into a direct comparison between GPT-3.5 and GPT-4 – the reigning kings of AI models.
GPT-3.5 and GPT-4 Performance Comparison
Comprehending the distinctions between GPT-3.5 and GPT-4 is key to upgrading your AI productivity. GPT-4 has made substantial strides over its predecessor, providing improved language apprehension, better contextual awareness, and enhanced response precision. By using Agent GPT AI, you can smoothly incorporate these progressions into your system. This incorporation not only elevates performance but also ensures you're using the most expanded technology attainable, giving you a fierce edge.
Impact of Agent Workflows on Performance Improvements
Agent workflows are groundbreakers when it comes to enhancing performance metrics. They permit automated, intelligent task management, reducing the time and effort needed for manual oversight. With Agent GPT AI, this productivity becomes even more robust. You'll see enhancements in efficacy, precision, and overall workflow. Agent workflows help in breaking down intricate tasks into tractable segments, ensuring that each part of your system executes at peak performance.
Integrating Agent GPT AI into your performance metrics plan is a smart move. It improves the HumanEval coding benchmark inspection, provides a clear comparison between GPT-3.5 and GPT-4, and substantially enhances performance through intelligent agent workflows. By clasping these advancements, you're setting yourself up for success, ensuring your systems are always running at their best.
Great, you now understand performance metrics, but what about the core of agent design that makes it all possible?
Explore more with our article on Innovations In AI For Healthcare. Read our guide and learn various ways to improve the workflow in the healthcare sector with the help of AI!
Framework for Effective Agent Design Patterns
Ever wondered what makes an Agent GPT AI tick? Let’s learn about the core design patterns that revolutionize a simple agent into a dominator of workflow and intelligence.
Reflection: LLMs Improving Their Work
What if you have a personal assistant that gets better every time it helps you? That's what reflection is all about. Agent GPT AI uses large language models (LLMs) to retrospect and improve their outputs. These agents reflect on past performance, learn from their mistakes, upgrade their responses, and provide gradually more precise and pertinent data. It’s like having an assistant that constantly teaches itself to serve you better.
Tool Use: Utilizing Web Search and Other Tools
Knowledge is power, and attaining the right tools can make all the distinctions. Agent GPT AI shines by smoothly incorporating web searches and other digital tools. Need the up-to-date information? The agent can clean the web in seconds. Looking for explicit software usefulness? The AI taps into its digital toolbox to find the best solution. You get a thorough response swiftly and effectively without having to lift a finger.
Planning: Creating and Executing Multi-Step Plans
Intricate tasks often need pragmatic strategizing and accurate implementation. Agent GPT AI excels by designing and adhering to multistep plans. Whether you're managing a project, planning a marketing campaign, or simply arranging your week, the AI generates a step-by-step blueprint to accomplish your goals. With its diligent planning capabilities, you can rest easy knowing every aspect of your task is covered.
By getting skilled in these key design patterns, Agent GPT AI becomes more than just a tool—it’s a revolutionary force in your digital armaments. Use the power of reflection, tool use, strategic planning, and collaboration to unleash new levels of efficacy and innovation.
Do you have a handle on the design patterns? Let's now get into the nitty-gritty of developing and deploying your AI agents.
For a deeper comprehension of applicable applications, check out our article on In-Depth Case Studies in AI Model Testing: Exploring Real-World Applications and Insights.
Developing and Deploying AI Agents
Let’s learn how you can smoothly evolve and deploy your very own AI agents:
Automatic Setup Processes
Automatic setup processes sleek the initial configuration to ensure you can get started with minimal hassle. You’ll find that the automation not only saves time but also reduces the potential for human error. Your concentration can stay on what matters most: using AI for potent outcomes.
Prerequisites for Deployment
Before you hit the ground running, there are a few prerequisites you need to tick off:
Data Readiness: Ensure you have immaculate, well-organized data. The quality of input data directly impacts the performance of your AI agent.
Infrastructure: Validate that your hardware and software infrastructure meets the demands. This includes adequate computational power and congenial software versions.
Access Permissions: Accord the essential access permissions to pertinent team members and systems. Proper access management averts future obstacles.
Training Resources: Equip your team with the right resources. Training materials and documentation are crucial for streamlined functioning.
Step-by-Step Setup Instructions
Ready to set up your Agent GPT AI? Follow these direct steps to get your AI agent up and running:
Download the AI Software:
Visit the official website and download the latest version of Agent GPT AI.
Ensure you choose the correct version congenial to your operating system.
Install the Software:
Run the installation file and follow the on-screen guidelines.
Choose the default installation path or choose a custom location if preferred.
Configure Initial Settings:
Launch the software and go to the settings menu.
Input your license key or activation code.
Configure basic settings such as language preference, default data directories, and logging options.
Connect to Data Sources:
Go to the data incorporation section and add your data sources.
Provide significant credentials and ensure successful connections.
Set Up User Accounts:
Create user accounts for your team members.
Assign apt roles and attain levels based on their responsibilities.
Train Your AI Agent:
Load your initial data set and start the training process.
Monitor the training progress and make adaptations as required to enhance precision.
Deploy the AI Agent:
Once training is complete, deploy the AI agent to your desired environment.
Conduct a series of tests to ensure it operates as expected.
Monitor and Optimize:
Frequently check the performance metrics.
Make constant enhancements based on feedback and performance data.
By adhering to these steps, you’ll have your Agent GPT AI functional in no time, ready to transform your functioning.
If you're intrigued by optimizing data management and ensuring compliance in AI projects, make sure to check out our detailed guide on the Best Practices in Data Governance for AI.
Conclusion
To conclude the guide, mastering AI agent strategies using GPT opens doors to unmatched efficacy and performance enhancements. Explore these strategies to innovate and lead in your field.
Ready to take your AI agent strategies to the next level? Sign up with Raga AI and unleash the full potential of GPT in your projects.
Ever wondered how AI agent GPT AI revolutionized technology? Comprehending AI agent strategies and the crucial role of GPT in their expansion is critical. In this article, you'll delve into the strategies powering AI agents and find why GPT plays a crucial role in shaping their capabilities. Whether you're new to AI or looking to deepen your knowledge, you're about to uncover the captivating world of AI agents and their dependence on GPT technology. Let's dive in!
AI Agents and Their Capabilities
Want to know about AI Agents and what they are capable of? Let’s know in detail:
Definition and Key Concepts of AI Agents
AI agents are software programs designed to perform literal tasks separately, using artificial intelligence to make decisions and take actions. These agents dissect information, learn from interactions, and adjust their behavior over time, emulating human-like intelligence.
Difference between ChatGPT and AI agents
ChatGPT, like Agent GPT AI here, is a type of AI agent concentrated on natural language processing and conversation. While ChatGPT shines in producing text based on input, AI agents can vary extensively in their functionalities—from managing schedules and tasks to driving cars or diagnosing diseases.
Examples of Existing AI Agent Projects
Some notorious AI agent projects include virtual assistants like Siri and Alexa, which help users with mundane tasks. Autonomous vehicles, guided by AI, represent another frontier. Moreover, AI agents in healthcare dissect medical data to recommend treatments, exhibiting the broad applications of AI in various fields.
Now that we've seen some of the fantastic AI projects out there let's explore how different workflows impact the performance of these AI agents.
Discover how advancements in LLM and generative AI infrastructure are shaping the future of technology. Read our detailed guide on Exploring the Future of LLM and Generative AI Infrastructure.
Iterative Agent Workflows vs. Zero-Shot Mode
Welcome to the future of AI, where your apprehension of iterative agent workflows and zero-shot modes can revolutionize your approach to AI enforcement. Let's dive into these concepts and see how they can transform your use of Agent GPT AI.
Current Zero-Shot Usage in LLMs
When you think about zero-shot learning, you're noticing the cutting edge of language model capabilities. AI executes tasks in zero-shot mode without any prior precise training on those tasks. Envision asks an AI to translate a sentence into a language it has never confronted before or to craft a creative story based on an ambiguous prompt. Sounds like magic, right? In the synopsis of Large Language Models (LLMs) like GPT-3, zero-shot learning is used considerably for numerous applications, from responding to questions to creating content on the fly.
You benefit from zero-shot learning because it substantially reduces the time and effort needed to train models on new tasks. It's like having a Swiss army knife of AI, ready to tackle any challenge you throw at it with minimal preparation.
Alright, so we've understood the basics of zero-shot usage, but what's the flip side?
Challenges and Efficiency of Zero-Shot Generation
However, zero-shot learning isn't without its obstacles. One of the biggest challenges is precision. Because the AI hasn't been concretely trained on your particular task, its answers can sometimes be off the mark. You might ask it to draft a venture proposal, and instead, it gives you something more suited to a casual blog post. Thwarting, right?
Another problem is efficiency. Zero-shot generation can be computationally profound because the model is drawing on a vast array of comprehension to perform tasks it wasn't clearly trained to do. This means more refining power and longer response times. Plus, there's the ambiguity factor – the AI might demonstrate your prompt in unanticipated ways, leading to outputs that don't quite hit the mark.
Intrigued by zero-shot learning? Wait until you see how iterative workflows can make it even better.
Benefits of Iterative Agent Workflows
Enter iterative agent workflows, your AI's best buddy. Unlike zero-shot mode, iterative workflows involve continuous feedback loops where the AI's output is processed over numerous iterations. This approach can fiercely improve the quality and pertinence of the produced content.
Iterative agent workflows use the power of fine-tuning. You start with a foremost output, provide feedback, and the AI adapts its ensuing responses accordingly. This method reduces errors and improves the precision of the outcome. Envision carving a statue: with each sculpt, the form becomes explicit and more defined. That's exactly what iterative workflows do for your AI-generated content.
Moreover, iterative workflows can substantially enhance efficiency over time. As the AI grasps from each iteration, it becomes better at comprehending and implementing your literal needs, reducing the need for comprehensive rework. This not only saves time but also upgrades resource usage, making your AI deployment more cost-efficient.
By mastering both approaches, you can use the full potential of Agent GPT AI, revolutionizing your workflows and unleashing new levels of workflow and innovation.
Curious yet? Let's break down the step-by-step process to make the most out of your AI agent.
If you're intrigued in using AI for improved software development, don't miss our guide on Integrating AI Models with Continuous Integration Systems.
Key Steps in an Iterative Workflow
Agent GPT AI sleek your projects. To make the most out of this robust tool, you need to follow a planned, iterative workflow. Here’s how you can accomplish success:
Planning an Outline and Tasks
Commence by mapping out your project. Break down the project into tractable tasks. Think of this as your roadmap. It’s vital to have a clear abstract to guide you through each phase. This foremost strategy saves time and sets you up for streamlined implementation.
Executing the Top Task First
Now, tackle the most vital task first. This might be the core element of your project. By concentrating on the top priority, you ensure that the most crucial features are acknowledged early on. This sets a robust foundation for ensuing tasks.
Review and Revise Based on Feedback
Once you’ve implemented a task, pause for feedback. Team members, stakeholders, or even AI can provide feedback. Use this feedback to make essential revisions. Constant enhancement is the name of the game.
Reprioritize Tasks for Efficiency
After amending, reassess your remaining tasks. Adapt priorities based on what you’ve learned. Some tasks may shift in significance, and that’s okay. The aim is to stay dexterous and effective.
Iterate Until Goal Completion
Eventually, iterate this cycle. Implement, retrospect, revise, and reprioritize. Keep repeating until you accomplish your project goals. This recurring approach ensures that you’re continuously enhancing and adjusting to new data.
By adhering to these steps, you use Agent GPT AI’s full potential, making your workflow sleek and more efficient.
After nailing down the iterative workflow, it's time to see how these practices can elevate your performance metrics.
Explore our latest insights on using AI for improved retail customer experiences to discover how artificial intelligence can revolutionize your retail strategy. Read our detailed guide on Using AI For Enhanced Retail Customer Experiences.
Enhancing Performance Metrics with Agent Workflow
Agent GPT AI is a robust tool which can transform how you handle your performance metrics, ensuring you get the most out of your systems. Let’s learn how Agent GPT AI improves your performance through human assessment, a comparison between GPT-3.5 and GPT-4, and the effect of agent workflows.
HumanEval Coding Benchmark Analysis
When it comes to measuring performance, the HumanEval coding benchmark is a vital tool. It gives a thorough inspection of how AI models execute coding tasks, giving you clear perceptions of their capabilities. With Agent GPT AI, you can sleek this process, making it more effective and precise. You'll find that using Agent GPT AI for HumanEval coding benchmarks helps you determine strengths and weaknesses swiftly, permitting tormented enhancements and better overall performance.
Now that you've got the basics, let's dive deeper into a direct comparison between GPT-3.5 and GPT-4 – the reigning kings of AI models.
GPT-3.5 and GPT-4 Performance Comparison
Comprehending the distinctions between GPT-3.5 and GPT-4 is key to upgrading your AI productivity. GPT-4 has made substantial strides over its predecessor, providing improved language apprehension, better contextual awareness, and enhanced response precision. By using Agent GPT AI, you can smoothly incorporate these progressions into your system. This incorporation not only elevates performance but also ensures you're using the most expanded technology attainable, giving you a fierce edge.
Impact of Agent Workflows on Performance Improvements
Agent workflows are groundbreakers when it comes to enhancing performance metrics. They permit automated, intelligent task management, reducing the time and effort needed for manual oversight. With Agent GPT AI, this productivity becomes even more robust. You'll see enhancements in efficacy, precision, and overall workflow. Agent workflows help in breaking down intricate tasks into tractable segments, ensuring that each part of your system executes at peak performance.
Integrating Agent GPT AI into your performance metrics plan is a smart move. It improves the HumanEval coding benchmark inspection, provides a clear comparison between GPT-3.5 and GPT-4, and substantially enhances performance through intelligent agent workflows. By clasping these advancements, you're setting yourself up for success, ensuring your systems are always running at their best.
Great, you now understand performance metrics, but what about the core of agent design that makes it all possible?
Explore more with our article on Innovations In AI For Healthcare. Read our guide and learn various ways to improve the workflow in the healthcare sector with the help of AI!
Framework for Effective Agent Design Patterns
Ever wondered what makes an Agent GPT AI tick? Let’s learn about the core design patterns that revolutionize a simple agent into a dominator of workflow and intelligence.
Reflection: LLMs Improving Their Work
What if you have a personal assistant that gets better every time it helps you? That's what reflection is all about. Agent GPT AI uses large language models (LLMs) to retrospect and improve their outputs. These agents reflect on past performance, learn from their mistakes, upgrade their responses, and provide gradually more precise and pertinent data. It’s like having an assistant that constantly teaches itself to serve you better.
Tool Use: Utilizing Web Search and Other Tools
Knowledge is power, and attaining the right tools can make all the distinctions. Agent GPT AI shines by smoothly incorporating web searches and other digital tools. Need the up-to-date information? The agent can clean the web in seconds. Looking for explicit software usefulness? The AI taps into its digital toolbox to find the best solution. You get a thorough response swiftly and effectively without having to lift a finger.
Planning: Creating and Executing Multi-Step Plans
Intricate tasks often need pragmatic strategizing and accurate implementation. Agent GPT AI excels by designing and adhering to multistep plans. Whether you're managing a project, planning a marketing campaign, or simply arranging your week, the AI generates a step-by-step blueprint to accomplish your goals. With its diligent planning capabilities, you can rest easy knowing every aspect of your task is covered.
By getting skilled in these key design patterns, Agent GPT AI becomes more than just a tool—it’s a revolutionary force in your digital armaments. Use the power of reflection, tool use, strategic planning, and collaboration to unleash new levels of efficacy and innovation.
Do you have a handle on the design patterns? Let's now get into the nitty-gritty of developing and deploying your AI agents.
For a deeper comprehension of applicable applications, check out our article on In-Depth Case Studies in AI Model Testing: Exploring Real-World Applications and Insights.
Developing and Deploying AI Agents
Let’s learn how you can smoothly evolve and deploy your very own AI agents:
Automatic Setup Processes
Automatic setup processes sleek the initial configuration to ensure you can get started with minimal hassle. You’ll find that the automation not only saves time but also reduces the potential for human error. Your concentration can stay on what matters most: using AI for potent outcomes.
Prerequisites for Deployment
Before you hit the ground running, there are a few prerequisites you need to tick off:
Data Readiness: Ensure you have immaculate, well-organized data. The quality of input data directly impacts the performance of your AI agent.
Infrastructure: Validate that your hardware and software infrastructure meets the demands. This includes adequate computational power and congenial software versions.
Access Permissions: Accord the essential access permissions to pertinent team members and systems. Proper access management averts future obstacles.
Training Resources: Equip your team with the right resources. Training materials and documentation are crucial for streamlined functioning.
Step-by-Step Setup Instructions
Ready to set up your Agent GPT AI? Follow these direct steps to get your AI agent up and running:
Download the AI Software:
Visit the official website and download the latest version of Agent GPT AI.
Ensure you choose the correct version congenial to your operating system.
Install the Software:
Run the installation file and follow the on-screen guidelines.
Choose the default installation path or choose a custom location if preferred.
Configure Initial Settings:
Launch the software and go to the settings menu.
Input your license key or activation code.
Configure basic settings such as language preference, default data directories, and logging options.
Connect to Data Sources:
Go to the data incorporation section and add your data sources.
Provide significant credentials and ensure successful connections.
Set Up User Accounts:
Create user accounts for your team members.
Assign apt roles and attain levels based on their responsibilities.
Train Your AI Agent:
Load your initial data set and start the training process.
Monitor the training progress and make adaptations as required to enhance precision.
Deploy the AI Agent:
Once training is complete, deploy the AI agent to your desired environment.
Conduct a series of tests to ensure it operates as expected.
Monitor and Optimize:
Frequently check the performance metrics.
Make constant enhancements based on feedback and performance data.
By adhering to these steps, you’ll have your Agent GPT AI functional in no time, ready to transform your functioning.
If you're intrigued by optimizing data management and ensuring compliance in AI projects, make sure to check out our detailed guide on the Best Practices in Data Governance for AI.
Conclusion
To conclude the guide, mastering AI agent strategies using GPT opens doors to unmatched efficacy and performance enhancements. Explore these strategies to innovate and lead in your field.
Ready to take your AI agent strategies to the next level? Sign up with Raga AI and unleash the full potential of GPT in your projects.
Ever wondered how AI agent GPT AI revolutionized technology? Comprehending AI agent strategies and the crucial role of GPT in their expansion is critical. In this article, you'll delve into the strategies powering AI agents and find why GPT plays a crucial role in shaping their capabilities. Whether you're new to AI or looking to deepen your knowledge, you're about to uncover the captivating world of AI agents and their dependence on GPT technology. Let's dive in!
AI Agents and Their Capabilities
Want to know about AI Agents and what they are capable of? Let’s know in detail:
Definition and Key Concepts of AI Agents
AI agents are software programs designed to perform literal tasks separately, using artificial intelligence to make decisions and take actions. These agents dissect information, learn from interactions, and adjust their behavior over time, emulating human-like intelligence.
Difference between ChatGPT and AI agents
ChatGPT, like Agent GPT AI here, is a type of AI agent concentrated on natural language processing and conversation. While ChatGPT shines in producing text based on input, AI agents can vary extensively in their functionalities—from managing schedules and tasks to driving cars or diagnosing diseases.
Examples of Existing AI Agent Projects
Some notorious AI agent projects include virtual assistants like Siri and Alexa, which help users with mundane tasks. Autonomous vehicles, guided by AI, represent another frontier. Moreover, AI agents in healthcare dissect medical data to recommend treatments, exhibiting the broad applications of AI in various fields.
Now that we've seen some of the fantastic AI projects out there let's explore how different workflows impact the performance of these AI agents.
Discover how advancements in LLM and generative AI infrastructure are shaping the future of technology. Read our detailed guide on Exploring the Future of LLM and Generative AI Infrastructure.
Iterative Agent Workflows vs. Zero-Shot Mode
Welcome to the future of AI, where your apprehension of iterative agent workflows and zero-shot modes can revolutionize your approach to AI enforcement. Let's dive into these concepts and see how they can transform your use of Agent GPT AI.
Current Zero-Shot Usage in LLMs
When you think about zero-shot learning, you're noticing the cutting edge of language model capabilities. AI executes tasks in zero-shot mode without any prior precise training on those tasks. Envision asks an AI to translate a sentence into a language it has never confronted before or to craft a creative story based on an ambiguous prompt. Sounds like magic, right? In the synopsis of Large Language Models (LLMs) like GPT-3, zero-shot learning is used considerably for numerous applications, from responding to questions to creating content on the fly.
You benefit from zero-shot learning because it substantially reduces the time and effort needed to train models on new tasks. It's like having a Swiss army knife of AI, ready to tackle any challenge you throw at it with minimal preparation.
Alright, so we've understood the basics of zero-shot usage, but what's the flip side?
Challenges and Efficiency of Zero-Shot Generation
However, zero-shot learning isn't without its obstacles. One of the biggest challenges is precision. Because the AI hasn't been concretely trained on your particular task, its answers can sometimes be off the mark. You might ask it to draft a venture proposal, and instead, it gives you something more suited to a casual blog post. Thwarting, right?
Another problem is efficiency. Zero-shot generation can be computationally profound because the model is drawing on a vast array of comprehension to perform tasks it wasn't clearly trained to do. This means more refining power and longer response times. Plus, there's the ambiguity factor – the AI might demonstrate your prompt in unanticipated ways, leading to outputs that don't quite hit the mark.
Intrigued by zero-shot learning? Wait until you see how iterative workflows can make it even better.
Benefits of Iterative Agent Workflows
Enter iterative agent workflows, your AI's best buddy. Unlike zero-shot mode, iterative workflows involve continuous feedback loops where the AI's output is processed over numerous iterations. This approach can fiercely improve the quality and pertinence of the produced content.
Iterative agent workflows use the power of fine-tuning. You start with a foremost output, provide feedback, and the AI adapts its ensuing responses accordingly. This method reduces errors and improves the precision of the outcome. Envision carving a statue: with each sculpt, the form becomes explicit and more defined. That's exactly what iterative workflows do for your AI-generated content.
Moreover, iterative workflows can substantially enhance efficiency over time. As the AI grasps from each iteration, it becomes better at comprehending and implementing your literal needs, reducing the need for comprehensive rework. This not only saves time but also upgrades resource usage, making your AI deployment more cost-efficient.
By mastering both approaches, you can use the full potential of Agent GPT AI, revolutionizing your workflows and unleashing new levels of workflow and innovation.
Curious yet? Let's break down the step-by-step process to make the most out of your AI agent.
If you're intrigued in using AI for improved software development, don't miss our guide on Integrating AI Models with Continuous Integration Systems.
Key Steps in an Iterative Workflow
Agent GPT AI sleek your projects. To make the most out of this robust tool, you need to follow a planned, iterative workflow. Here’s how you can accomplish success:
Planning an Outline and Tasks
Commence by mapping out your project. Break down the project into tractable tasks. Think of this as your roadmap. It’s vital to have a clear abstract to guide you through each phase. This foremost strategy saves time and sets you up for streamlined implementation.
Executing the Top Task First
Now, tackle the most vital task first. This might be the core element of your project. By concentrating on the top priority, you ensure that the most crucial features are acknowledged early on. This sets a robust foundation for ensuing tasks.
Review and Revise Based on Feedback
Once you’ve implemented a task, pause for feedback. Team members, stakeholders, or even AI can provide feedback. Use this feedback to make essential revisions. Constant enhancement is the name of the game.
Reprioritize Tasks for Efficiency
After amending, reassess your remaining tasks. Adapt priorities based on what you’ve learned. Some tasks may shift in significance, and that’s okay. The aim is to stay dexterous and effective.
Iterate Until Goal Completion
Eventually, iterate this cycle. Implement, retrospect, revise, and reprioritize. Keep repeating until you accomplish your project goals. This recurring approach ensures that you’re continuously enhancing and adjusting to new data.
By adhering to these steps, you use Agent GPT AI’s full potential, making your workflow sleek and more efficient.
After nailing down the iterative workflow, it's time to see how these practices can elevate your performance metrics.
Explore our latest insights on using AI for improved retail customer experiences to discover how artificial intelligence can revolutionize your retail strategy. Read our detailed guide on Using AI For Enhanced Retail Customer Experiences.
Enhancing Performance Metrics with Agent Workflow
Agent GPT AI is a robust tool which can transform how you handle your performance metrics, ensuring you get the most out of your systems. Let’s learn how Agent GPT AI improves your performance through human assessment, a comparison between GPT-3.5 and GPT-4, and the effect of agent workflows.
HumanEval Coding Benchmark Analysis
When it comes to measuring performance, the HumanEval coding benchmark is a vital tool. It gives a thorough inspection of how AI models execute coding tasks, giving you clear perceptions of their capabilities. With Agent GPT AI, you can sleek this process, making it more effective and precise. You'll find that using Agent GPT AI for HumanEval coding benchmarks helps you determine strengths and weaknesses swiftly, permitting tormented enhancements and better overall performance.
Now that you've got the basics, let's dive deeper into a direct comparison between GPT-3.5 and GPT-4 – the reigning kings of AI models.
GPT-3.5 and GPT-4 Performance Comparison
Comprehending the distinctions between GPT-3.5 and GPT-4 is key to upgrading your AI productivity. GPT-4 has made substantial strides over its predecessor, providing improved language apprehension, better contextual awareness, and enhanced response precision. By using Agent GPT AI, you can smoothly incorporate these progressions into your system. This incorporation not only elevates performance but also ensures you're using the most expanded technology attainable, giving you a fierce edge.
Impact of Agent Workflows on Performance Improvements
Agent workflows are groundbreakers when it comes to enhancing performance metrics. They permit automated, intelligent task management, reducing the time and effort needed for manual oversight. With Agent GPT AI, this productivity becomes even more robust. You'll see enhancements in efficacy, precision, and overall workflow. Agent workflows help in breaking down intricate tasks into tractable segments, ensuring that each part of your system executes at peak performance.
Integrating Agent GPT AI into your performance metrics plan is a smart move. It improves the HumanEval coding benchmark inspection, provides a clear comparison between GPT-3.5 and GPT-4, and substantially enhances performance through intelligent agent workflows. By clasping these advancements, you're setting yourself up for success, ensuring your systems are always running at their best.
Great, you now understand performance metrics, but what about the core of agent design that makes it all possible?
Explore more with our article on Innovations In AI For Healthcare. Read our guide and learn various ways to improve the workflow in the healthcare sector with the help of AI!
Framework for Effective Agent Design Patterns
Ever wondered what makes an Agent GPT AI tick? Let’s learn about the core design patterns that revolutionize a simple agent into a dominator of workflow and intelligence.
Reflection: LLMs Improving Their Work
What if you have a personal assistant that gets better every time it helps you? That's what reflection is all about. Agent GPT AI uses large language models (LLMs) to retrospect and improve their outputs. These agents reflect on past performance, learn from their mistakes, upgrade their responses, and provide gradually more precise and pertinent data. It’s like having an assistant that constantly teaches itself to serve you better.
Tool Use: Utilizing Web Search and Other Tools
Knowledge is power, and attaining the right tools can make all the distinctions. Agent GPT AI shines by smoothly incorporating web searches and other digital tools. Need the up-to-date information? The agent can clean the web in seconds. Looking for explicit software usefulness? The AI taps into its digital toolbox to find the best solution. You get a thorough response swiftly and effectively without having to lift a finger.
Planning: Creating and Executing Multi-Step Plans
Intricate tasks often need pragmatic strategizing and accurate implementation. Agent GPT AI excels by designing and adhering to multistep plans. Whether you're managing a project, planning a marketing campaign, or simply arranging your week, the AI generates a step-by-step blueprint to accomplish your goals. With its diligent planning capabilities, you can rest easy knowing every aspect of your task is covered.
By getting skilled in these key design patterns, Agent GPT AI becomes more than just a tool—it’s a revolutionary force in your digital armaments. Use the power of reflection, tool use, strategic planning, and collaboration to unleash new levels of efficacy and innovation.
Do you have a handle on the design patterns? Let's now get into the nitty-gritty of developing and deploying your AI agents.
For a deeper comprehension of applicable applications, check out our article on In-Depth Case Studies in AI Model Testing: Exploring Real-World Applications and Insights.
Developing and Deploying AI Agents
Let’s learn how you can smoothly evolve and deploy your very own AI agents:
Automatic Setup Processes
Automatic setup processes sleek the initial configuration to ensure you can get started with minimal hassle. You’ll find that the automation not only saves time but also reduces the potential for human error. Your concentration can stay on what matters most: using AI for potent outcomes.
Prerequisites for Deployment
Before you hit the ground running, there are a few prerequisites you need to tick off:
Data Readiness: Ensure you have immaculate, well-organized data. The quality of input data directly impacts the performance of your AI agent.
Infrastructure: Validate that your hardware and software infrastructure meets the demands. This includes adequate computational power and congenial software versions.
Access Permissions: Accord the essential access permissions to pertinent team members and systems. Proper access management averts future obstacles.
Training Resources: Equip your team with the right resources. Training materials and documentation are crucial for streamlined functioning.
Step-by-Step Setup Instructions
Ready to set up your Agent GPT AI? Follow these direct steps to get your AI agent up and running:
Download the AI Software:
Visit the official website and download the latest version of Agent GPT AI.
Ensure you choose the correct version congenial to your operating system.
Install the Software:
Run the installation file and follow the on-screen guidelines.
Choose the default installation path or choose a custom location if preferred.
Configure Initial Settings:
Launch the software and go to the settings menu.
Input your license key or activation code.
Configure basic settings such as language preference, default data directories, and logging options.
Connect to Data Sources:
Go to the data incorporation section and add your data sources.
Provide significant credentials and ensure successful connections.
Set Up User Accounts:
Create user accounts for your team members.
Assign apt roles and attain levels based on their responsibilities.
Train Your AI Agent:
Load your initial data set and start the training process.
Monitor the training progress and make adaptations as required to enhance precision.
Deploy the AI Agent:
Once training is complete, deploy the AI agent to your desired environment.
Conduct a series of tests to ensure it operates as expected.
Monitor and Optimize:
Frequently check the performance metrics.
Make constant enhancements based on feedback and performance data.
By adhering to these steps, you’ll have your Agent GPT AI functional in no time, ready to transform your functioning.
If you're intrigued by optimizing data management and ensuring compliance in AI projects, make sure to check out our detailed guide on the Best Practices in Data Governance for AI.
Conclusion
To conclude the guide, mastering AI agent strategies using GPT opens doors to unmatched efficacy and performance enhancements. Explore these strategies to innovate and lead in your field.
Ready to take your AI agent strategies to the next level? Sign up with Raga AI and unleash the full potential of GPT in your projects.
Ever wondered how AI agent GPT AI revolutionized technology? Comprehending AI agent strategies and the crucial role of GPT in their expansion is critical. In this article, you'll delve into the strategies powering AI agents and find why GPT plays a crucial role in shaping their capabilities. Whether you're new to AI or looking to deepen your knowledge, you're about to uncover the captivating world of AI agents and their dependence on GPT technology. Let's dive in!
AI Agents and Their Capabilities
Want to know about AI Agents and what they are capable of? Let’s know in detail:
Definition and Key Concepts of AI Agents
AI agents are software programs designed to perform literal tasks separately, using artificial intelligence to make decisions and take actions. These agents dissect information, learn from interactions, and adjust their behavior over time, emulating human-like intelligence.
Difference between ChatGPT and AI agents
ChatGPT, like Agent GPT AI here, is a type of AI agent concentrated on natural language processing and conversation. While ChatGPT shines in producing text based on input, AI agents can vary extensively in their functionalities—from managing schedules and tasks to driving cars or diagnosing diseases.
Examples of Existing AI Agent Projects
Some notorious AI agent projects include virtual assistants like Siri and Alexa, which help users with mundane tasks. Autonomous vehicles, guided by AI, represent another frontier. Moreover, AI agents in healthcare dissect medical data to recommend treatments, exhibiting the broad applications of AI in various fields.
Now that we've seen some of the fantastic AI projects out there let's explore how different workflows impact the performance of these AI agents.
Discover how advancements in LLM and generative AI infrastructure are shaping the future of technology. Read our detailed guide on Exploring the Future of LLM and Generative AI Infrastructure.
Iterative Agent Workflows vs. Zero-Shot Mode
Welcome to the future of AI, where your apprehension of iterative agent workflows and zero-shot modes can revolutionize your approach to AI enforcement. Let's dive into these concepts and see how they can transform your use of Agent GPT AI.
Current Zero-Shot Usage in LLMs
When you think about zero-shot learning, you're noticing the cutting edge of language model capabilities. AI executes tasks in zero-shot mode without any prior precise training on those tasks. Envision asks an AI to translate a sentence into a language it has never confronted before or to craft a creative story based on an ambiguous prompt. Sounds like magic, right? In the synopsis of Large Language Models (LLMs) like GPT-3, zero-shot learning is used considerably for numerous applications, from responding to questions to creating content on the fly.
You benefit from zero-shot learning because it substantially reduces the time and effort needed to train models on new tasks. It's like having a Swiss army knife of AI, ready to tackle any challenge you throw at it with minimal preparation.
Alright, so we've understood the basics of zero-shot usage, but what's the flip side?
Challenges and Efficiency of Zero-Shot Generation
However, zero-shot learning isn't without its obstacles. One of the biggest challenges is precision. Because the AI hasn't been concretely trained on your particular task, its answers can sometimes be off the mark. You might ask it to draft a venture proposal, and instead, it gives you something more suited to a casual blog post. Thwarting, right?
Another problem is efficiency. Zero-shot generation can be computationally profound because the model is drawing on a vast array of comprehension to perform tasks it wasn't clearly trained to do. This means more refining power and longer response times. Plus, there's the ambiguity factor – the AI might demonstrate your prompt in unanticipated ways, leading to outputs that don't quite hit the mark.
Intrigued by zero-shot learning? Wait until you see how iterative workflows can make it even better.
Benefits of Iterative Agent Workflows
Enter iterative agent workflows, your AI's best buddy. Unlike zero-shot mode, iterative workflows involve continuous feedback loops where the AI's output is processed over numerous iterations. This approach can fiercely improve the quality and pertinence of the produced content.
Iterative agent workflows use the power of fine-tuning. You start with a foremost output, provide feedback, and the AI adapts its ensuing responses accordingly. This method reduces errors and improves the precision of the outcome. Envision carving a statue: with each sculpt, the form becomes explicit and more defined. That's exactly what iterative workflows do for your AI-generated content.
Moreover, iterative workflows can substantially enhance efficiency over time. As the AI grasps from each iteration, it becomes better at comprehending and implementing your literal needs, reducing the need for comprehensive rework. This not only saves time but also upgrades resource usage, making your AI deployment more cost-efficient.
By mastering both approaches, you can use the full potential of Agent GPT AI, revolutionizing your workflows and unleashing new levels of workflow and innovation.
Curious yet? Let's break down the step-by-step process to make the most out of your AI agent.
If you're intrigued in using AI for improved software development, don't miss our guide on Integrating AI Models with Continuous Integration Systems.
Key Steps in an Iterative Workflow
Agent GPT AI sleek your projects. To make the most out of this robust tool, you need to follow a planned, iterative workflow. Here’s how you can accomplish success:
Planning an Outline and Tasks
Commence by mapping out your project. Break down the project into tractable tasks. Think of this as your roadmap. It’s vital to have a clear abstract to guide you through each phase. This foremost strategy saves time and sets you up for streamlined implementation.
Executing the Top Task First
Now, tackle the most vital task first. This might be the core element of your project. By concentrating on the top priority, you ensure that the most crucial features are acknowledged early on. This sets a robust foundation for ensuing tasks.
Review and Revise Based on Feedback
Once you’ve implemented a task, pause for feedback. Team members, stakeholders, or even AI can provide feedback. Use this feedback to make essential revisions. Constant enhancement is the name of the game.
Reprioritize Tasks for Efficiency
After amending, reassess your remaining tasks. Adapt priorities based on what you’ve learned. Some tasks may shift in significance, and that’s okay. The aim is to stay dexterous and effective.
Iterate Until Goal Completion
Eventually, iterate this cycle. Implement, retrospect, revise, and reprioritize. Keep repeating until you accomplish your project goals. This recurring approach ensures that you’re continuously enhancing and adjusting to new data.
By adhering to these steps, you use Agent GPT AI’s full potential, making your workflow sleek and more efficient.
After nailing down the iterative workflow, it's time to see how these practices can elevate your performance metrics.
Explore our latest insights on using AI for improved retail customer experiences to discover how artificial intelligence can revolutionize your retail strategy. Read our detailed guide on Using AI For Enhanced Retail Customer Experiences.
Enhancing Performance Metrics with Agent Workflow
Agent GPT AI is a robust tool which can transform how you handle your performance metrics, ensuring you get the most out of your systems. Let’s learn how Agent GPT AI improves your performance through human assessment, a comparison between GPT-3.5 and GPT-4, and the effect of agent workflows.
HumanEval Coding Benchmark Analysis
When it comes to measuring performance, the HumanEval coding benchmark is a vital tool. It gives a thorough inspection of how AI models execute coding tasks, giving you clear perceptions of their capabilities. With Agent GPT AI, you can sleek this process, making it more effective and precise. You'll find that using Agent GPT AI for HumanEval coding benchmarks helps you determine strengths and weaknesses swiftly, permitting tormented enhancements and better overall performance.
Now that you've got the basics, let's dive deeper into a direct comparison between GPT-3.5 and GPT-4 – the reigning kings of AI models.
GPT-3.5 and GPT-4 Performance Comparison
Comprehending the distinctions between GPT-3.5 and GPT-4 is key to upgrading your AI productivity. GPT-4 has made substantial strides over its predecessor, providing improved language apprehension, better contextual awareness, and enhanced response precision. By using Agent GPT AI, you can smoothly incorporate these progressions into your system. This incorporation not only elevates performance but also ensures you're using the most expanded technology attainable, giving you a fierce edge.
Impact of Agent Workflows on Performance Improvements
Agent workflows are groundbreakers when it comes to enhancing performance metrics. They permit automated, intelligent task management, reducing the time and effort needed for manual oversight. With Agent GPT AI, this productivity becomes even more robust. You'll see enhancements in efficacy, precision, and overall workflow. Agent workflows help in breaking down intricate tasks into tractable segments, ensuring that each part of your system executes at peak performance.
Integrating Agent GPT AI into your performance metrics plan is a smart move. It improves the HumanEval coding benchmark inspection, provides a clear comparison between GPT-3.5 and GPT-4, and substantially enhances performance through intelligent agent workflows. By clasping these advancements, you're setting yourself up for success, ensuring your systems are always running at their best.
Great, you now understand performance metrics, but what about the core of agent design that makes it all possible?
Explore more with our article on Innovations In AI For Healthcare. Read our guide and learn various ways to improve the workflow in the healthcare sector with the help of AI!
Framework for Effective Agent Design Patterns
Ever wondered what makes an Agent GPT AI tick? Let’s learn about the core design patterns that revolutionize a simple agent into a dominator of workflow and intelligence.
Reflection: LLMs Improving Their Work
What if you have a personal assistant that gets better every time it helps you? That's what reflection is all about. Agent GPT AI uses large language models (LLMs) to retrospect and improve their outputs. These agents reflect on past performance, learn from their mistakes, upgrade their responses, and provide gradually more precise and pertinent data. It’s like having an assistant that constantly teaches itself to serve you better.
Tool Use: Utilizing Web Search and Other Tools
Knowledge is power, and attaining the right tools can make all the distinctions. Agent GPT AI shines by smoothly incorporating web searches and other digital tools. Need the up-to-date information? The agent can clean the web in seconds. Looking for explicit software usefulness? The AI taps into its digital toolbox to find the best solution. You get a thorough response swiftly and effectively without having to lift a finger.
Planning: Creating and Executing Multi-Step Plans
Intricate tasks often need pragmatic strategizing and accurate implementation. Agent GPT AI excels by designing and adhering to multistep plans. Whether you're managing a project, planning a marketing campaign, or simply arranging your week, the AI generates a step-by-step blueprint to accomplish your goals. With its diligent planning capabilities, you can rest easy knowing every aspect of your task is covered.
By getting skilled in these key design patterns, Agent GPT AI becomes more than just a tool—it’s a revolutionary force in your digital armaments. Use the power of reflection, tool use, strategic planning, and collaboration to unleash new levels of efficacy and innovation.
Do you have a handle on the design patterns? Let's now get into the nitty-gritty of developing and deploying your AI agents.
For a deeper comprehension of applicable applications, check out our article on In-Depth Case Studies in AI Model Testing: Exploring Real-World Applications and Insights.
Developing and Deploying AI Agents
Let’s learn how you can smoothly evolve and deploy your very own AI agents:
Automatic Setup Processes
Automatic setup processes sleek the initial configuration to ensure you can get started with minimal hassle. You’ll find that the automation not only saves time but also reduces the potential for human error. Your concentration can stay on what matters most: using AI for potent outcomes.
Prerequisites for Deployment
Before you hit the ground running, there are a few prerequisites you need to tick off:
Data Readiness: Ensure you have immaculate, well-organized data. The quality of input data directly impacts the performance of your AI agent.
Infrastructure: Validate that your hardware and software infrastructure meets the demands. This includes adequate computational power and congenial software versions.
Access Permissions: Accord the essential access permissions to pertinent team members and systems. Proper access management averts future obstacles.
Training Resources: Equip your team with the right resources. Training materials and documentation are crucial for streamlined functioning.
Step-by-Step Setup Instructions
Ready to set up your Agent GPT AI? Follow these direct steps to get your AI agent up and running:
Download the AI Software:
Visit the official website and download the latest version of Agent GPT AI.
Ensure you choose the correct version congenial to your operating system.
Install the Software:
Run the installation file and follow the on-screen guidelines.
Choose the default installation path or choose a custom location if preferred.
Configure Initial Settings:
Launch the software and go to the settings menu.
Input your license key or activation code.
Configure basic settings such as language preference, default data directories, and logging options.
Connect to Data Sources:
Go to the data incorporation section and add your data sources.
Provide significant credentials and ensure successful connections.
Set Up User Accounts:
Create user accounts for your team members.
Assign apt roles and attain levels based on their responsibilities.
Train Your AI Agent:
Load your initial data set and start the training process.
Monitor the training progress and make adaptations as required to enhance precision.
Deploy the AI Agent:
Once training is complete, deploy the AI agent to your desired environment.
Conduct a series of tests to ensure it operates as expected.
Monitor and Optimize:
Frequently check the performance metrics.
Make constant enhancements based on feedback and performance data.
By adhering to these steps, you’ll have your Agent GPT AI functional in no time, ready to transform your functioning.
If you're intrigued by optimizing data management and ensuring compliance in AI projects, make sure to check out our detailed guide on the Best Practices in Data Governance for AI.
Conclusion
To conclude the guide, mastering AI agent strategies using GPT opens doors to unmatched efficacy and performance enhancements. Explore these strategies to innovate and lead in your field.
Ready to take your AI agent strategies to the next level? Sign up with Raga AI and unleash the full potential of GPT in your projects.
Subscribe to our newsletter to never miss an update
Other articles


Agents Talk, Models Think: A2A + MCP for Enterprise Agentic AI

Riya Parikh, Nitai Agarwal
Apr 27, 2025
Read the article
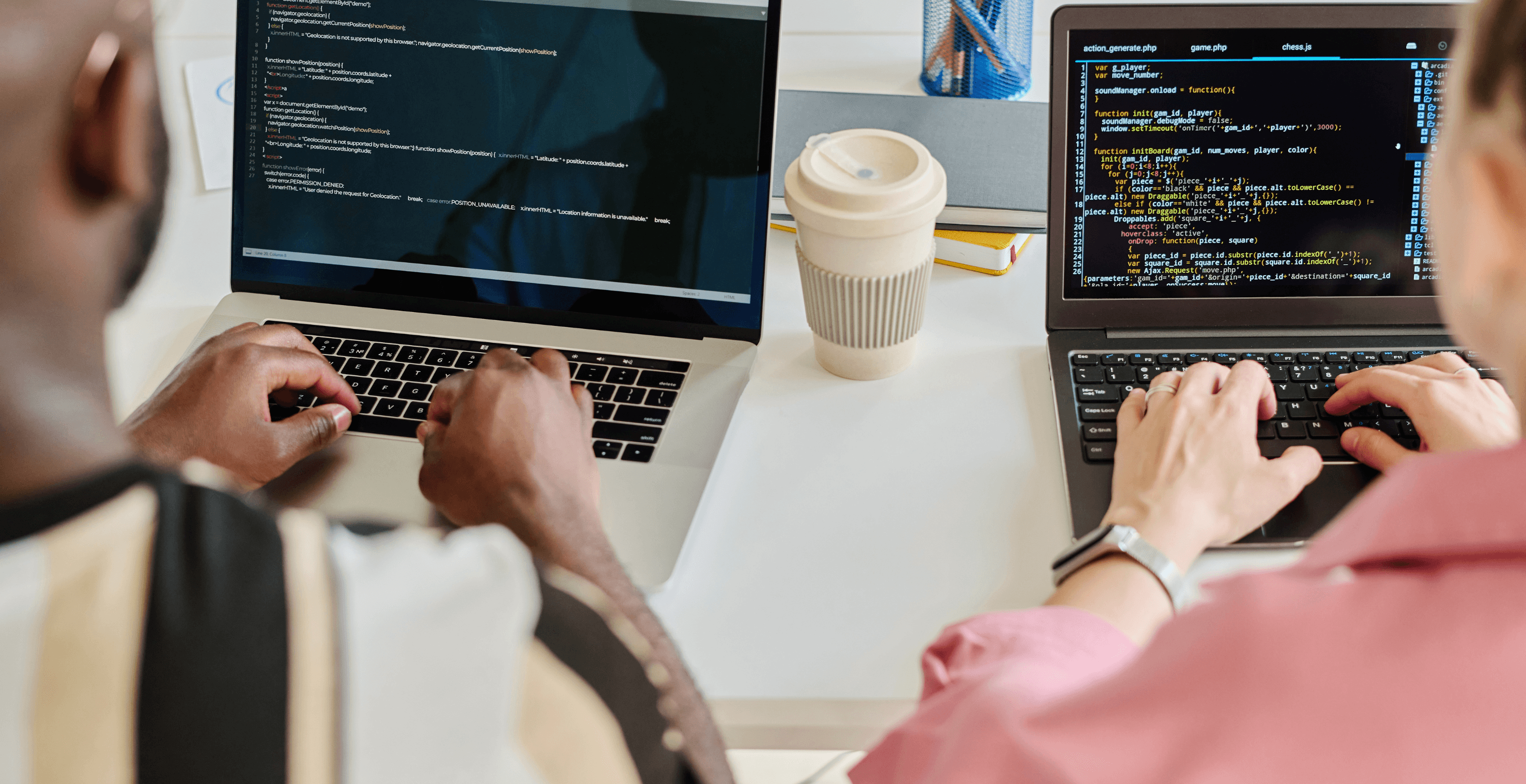
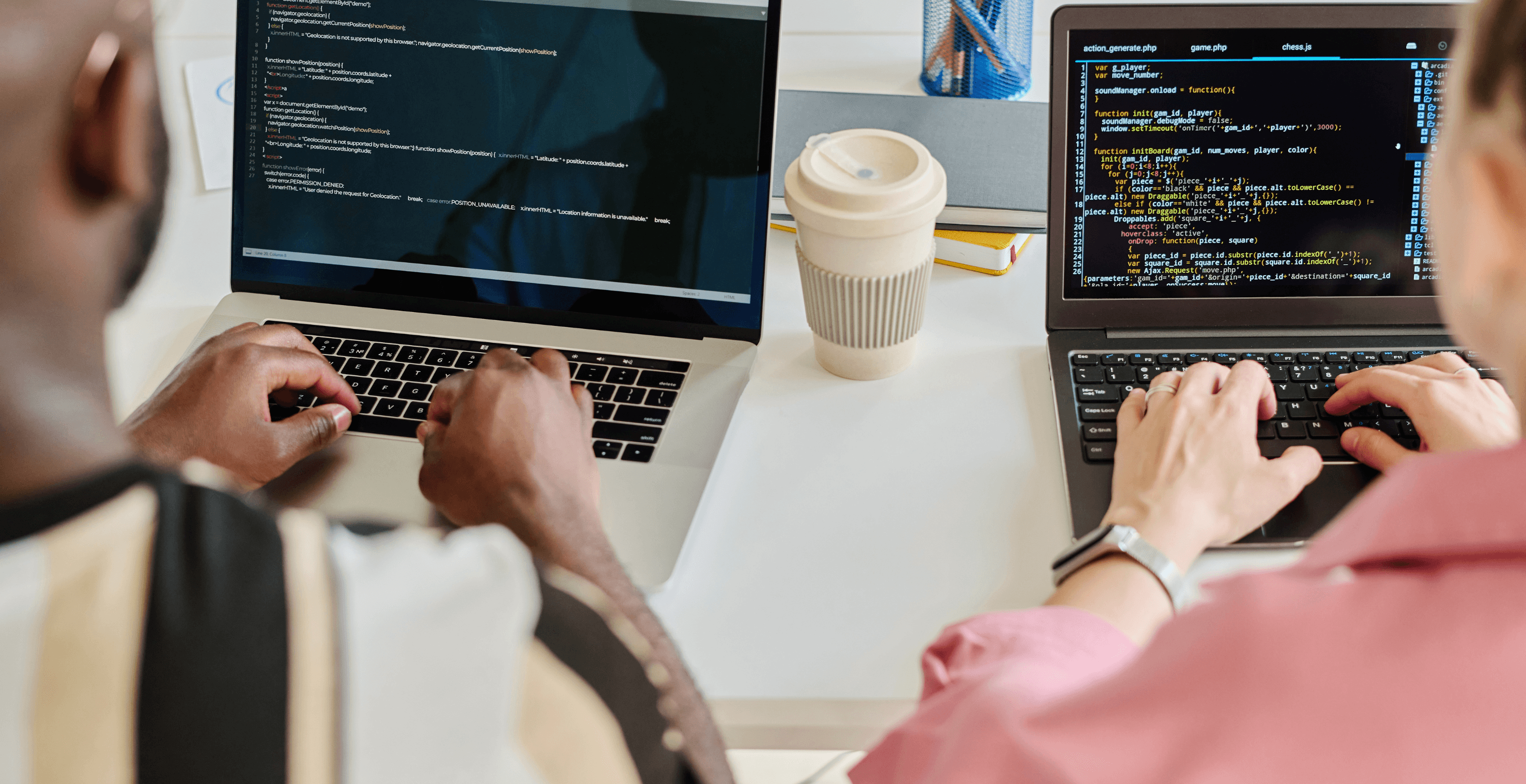
Understanding NeMo Guardrails: A Toolkit for LLM Security

Rehan Asif
Dec 24, 2024
Read the article


Understanding Differences in Large vs Small Language Models (LLM vs SLM)

Rehan Asif
Dec 21, 2024
Read the article