Integrating AI Models with Continuous Integration Systems
Integrating AI Models with Continuous Integration Systems
Integrating AI Models with Continuous Integration Systems
Jigar Gupta
Jul 12, 2024
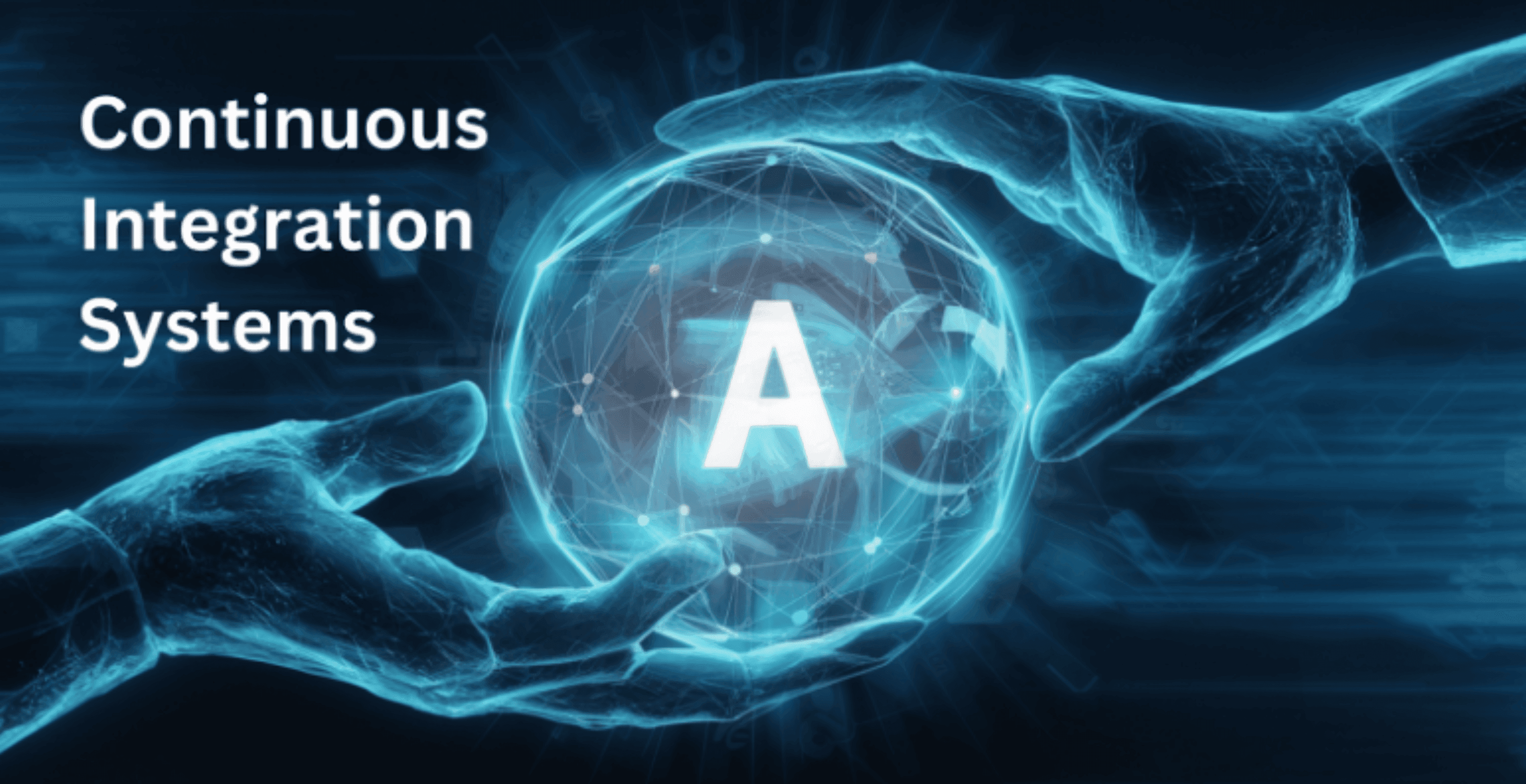
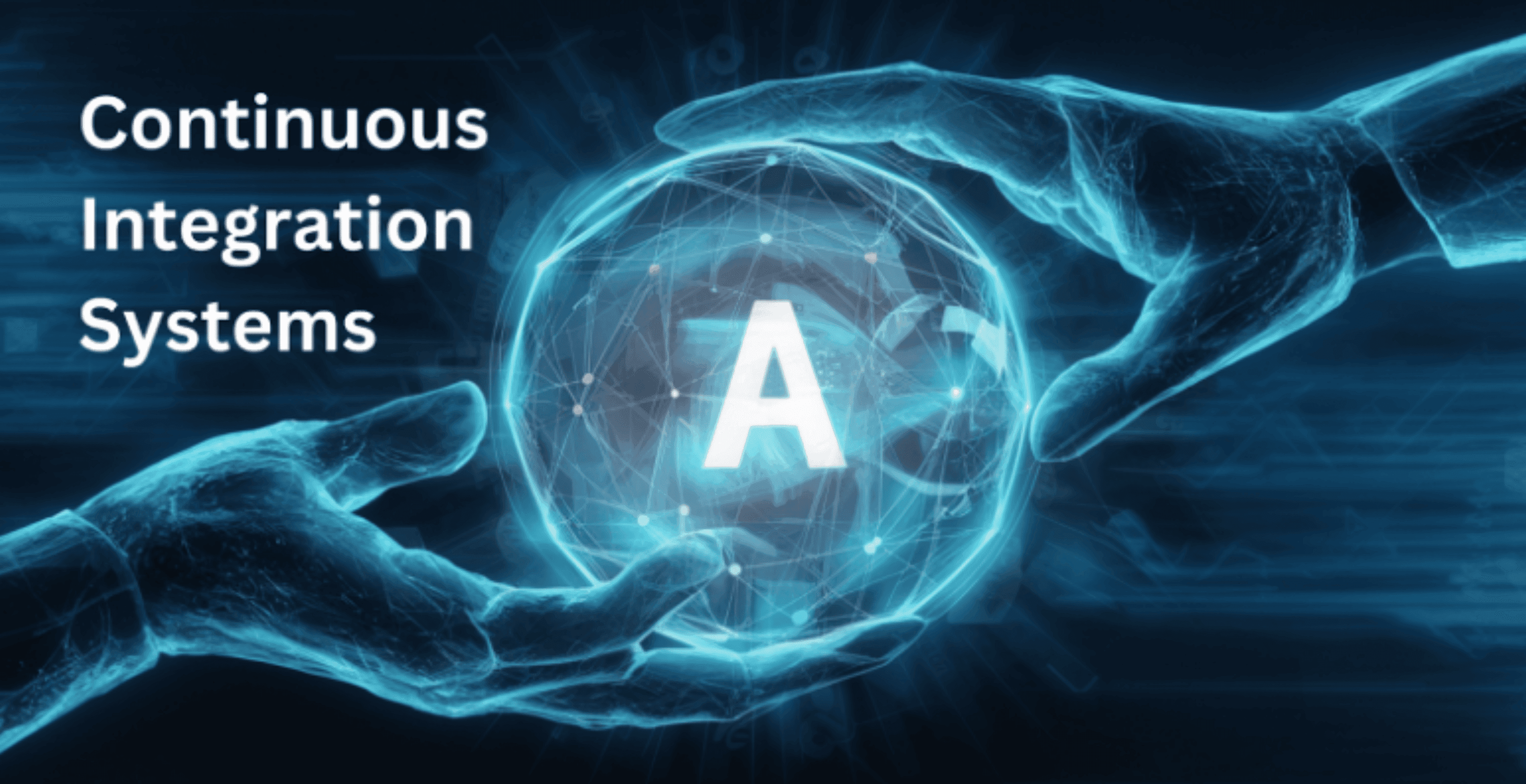
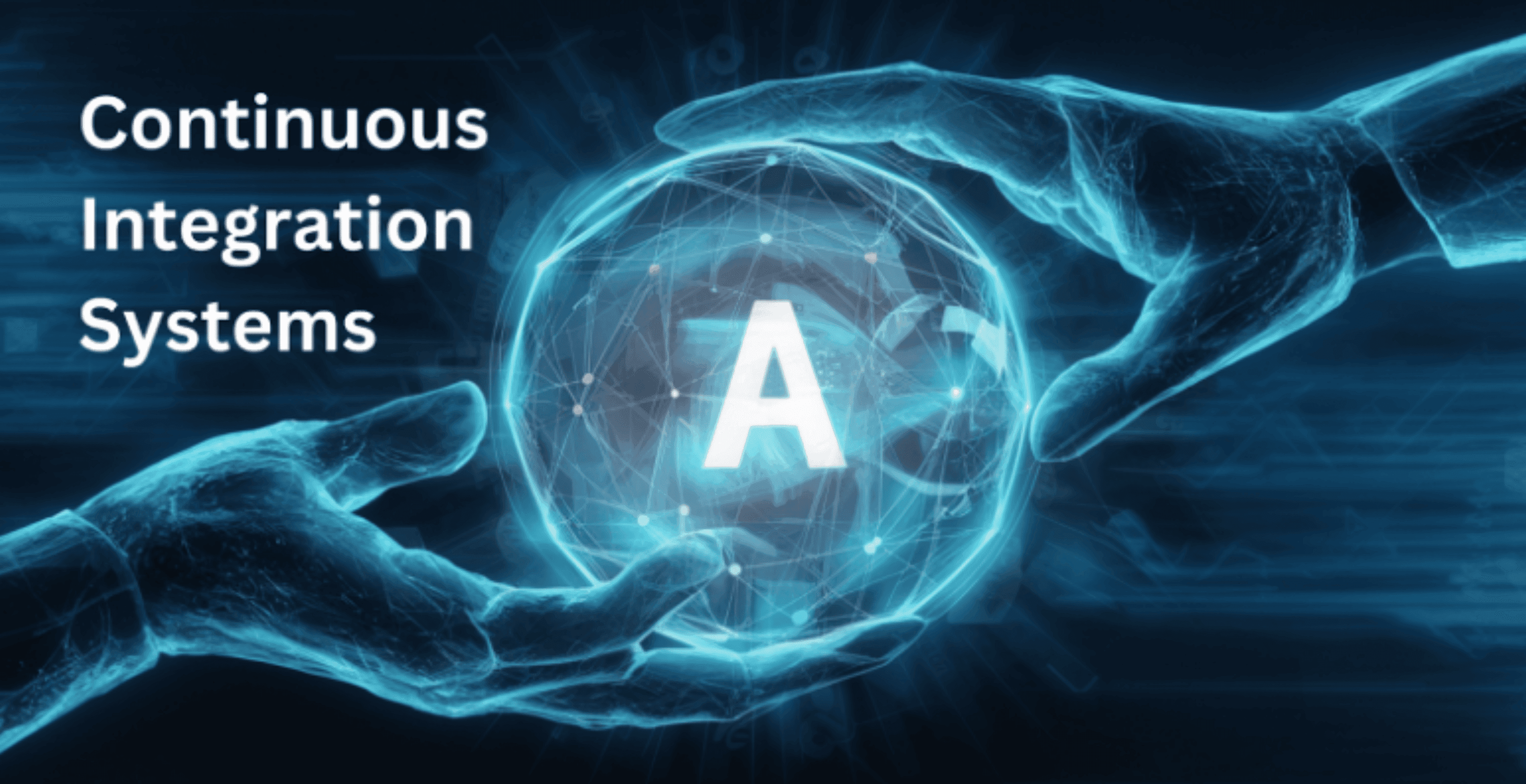
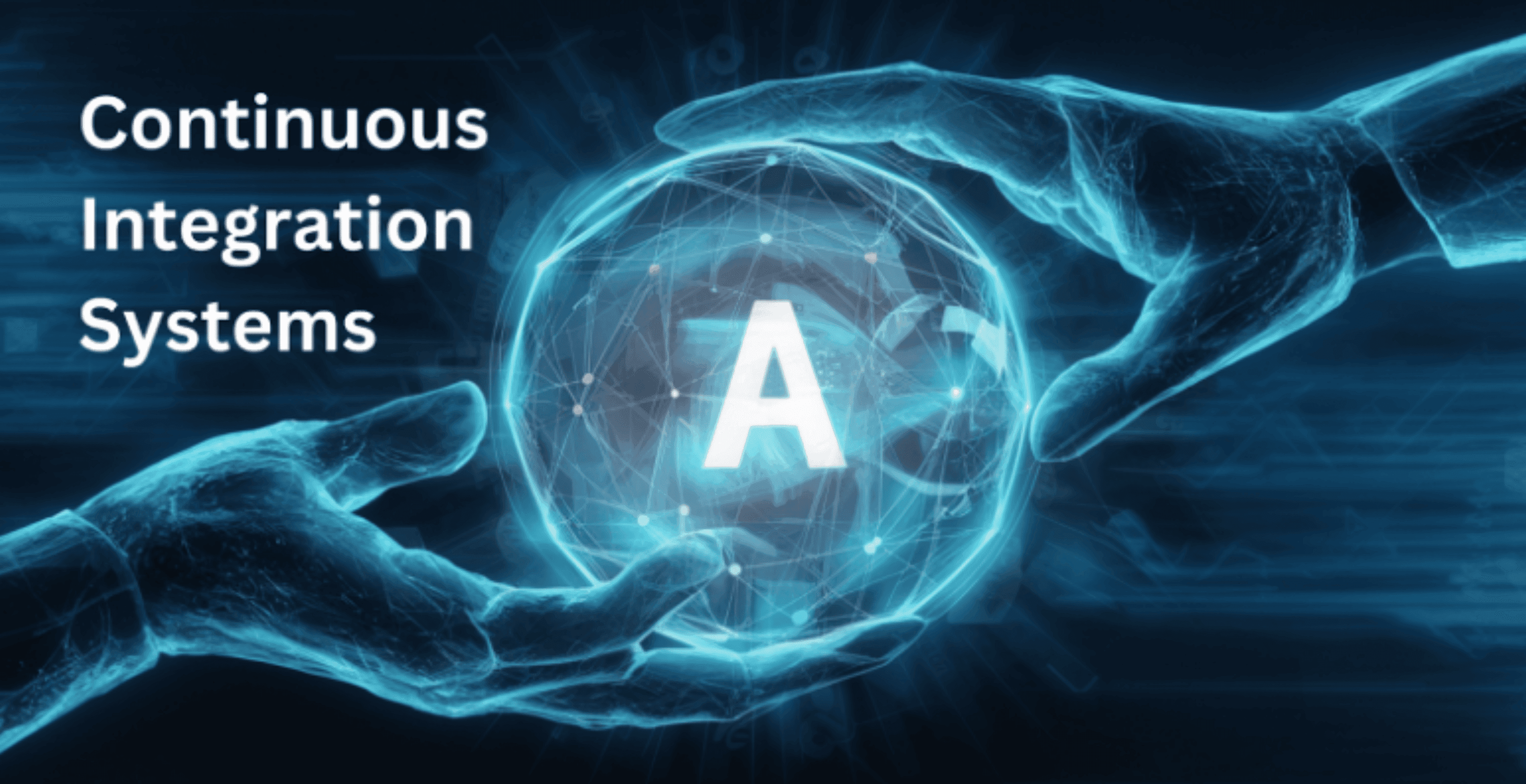
Continuous Integration (CI) is a software development practice where developers frequently merge their code changes into a central repository, after which automated builds and tests are run. The primary goals of CI are to improve software quality and to reduce the time taken to deliver it by replacing the traditional practice of developers working in isolation for an extended period.
Integrating AI models into CI systems brings significant benefits, such as ensuring the consistency of AI models with the application code, automating the model training and deployment processes, and continuously evaluating the model against real-world data and metrics.
Integrating AI Models with CI Systems
Automating Model Training and Evaluation
Integrating AI models into CI systems involves automating the model training and evaluation process, which is crucial for maintaining model accuracy and performance over time.
Triggering Model Training on Code Changes
Automating the training process begins with setting up triggers in the CI system so that any changes to model code or data automatically initiate a new training cycle. This ensures that the model adapts continuously to new data or changes in business requirements.
Automated Testing and Evaluation
After training, the model is automatically evaluated to ensure it meets predefined performance criteria. This might involve accuracy checks, performance metrics, or other validation techniques to ensure the model's effectiveness before it moves to deployment.
Continuous Delivery of AI Models
Continuous Delivery (CD) extends the concept of CI by ensuring that, in addition to automated testing, the model can be released to production reliably at any time.
Packaging and Versioning Models
AI models are packaged with all necessary components (including data preprocessing and post-processing stages) and versioned. This versioning is crucial for rollback scenarios and understanding model evolution over time.
Deploying Models to Production Environments
Automated scripts or workflows ensure models are deployed into production environments without requiring manual intervention. This can include updates to live systems or staging environments for further acceptance testing.
Monitoring and Maintenance
Once deployed, it's vital to continuously monitor the model's performance to catch any issues that might arise due to changing data patterns or other external factors.
Monitoring Model Performance
Continuous monitoring tools are used to track how well the model is performing in real-time. This can help detect performance degradation or failures, triggering alerts for human intervention or automated retraining workflows.
Retraining and Updating Models
AI models can drift from their trained state due to changes in input data over time. CI systems can automate the process of retraining and updating models, ensuring they remain accurate and relevant.
Best Practices
When integrating AI models with CI systems, certain best practices ensure the process is efficient and effective:
Reproducibility and Versioning
Versioning Data, Code, and Models
Maintain rigorous version control of not only the code but also the datasets and model versions. This aids in tracking changes, ensuring reproducibility, and facilitating audits.
Reproducible Training Environments
Use containerization technologies like Docker to create reproducible training environments. This minimizes the "it works on my machine" problem and ensures consistency across development, testing, and production environments.
Efficient Resource Management
Scaling Compute Resources for Training
Utilize dynamic resource allocation strategies to scale up resources for model training and scale down when not in use, optimizing cost and efficiency.
Optimizing Model Serving
Depending on the model complexity and inference speed requirements, choose the appropriate serving architecture, whether it's on-premises, in the cloud, or at the edge.
Testing and Validation
Unit and Integration Testing for AI Components
Develop comprehensive test suites for AI components to ensure they function correctly as standalone units and when integrated with other system components.
Validating Model Outputs
Regularly validate model outputs against expected outcomes to catch any anomalies or deviations that could indicate problems with the model.
Security and Compliance
Secure Model Deployment and Serving
Implement robust security protocols for deploying and serving models, including authentication, encryption, and secure access controls.
Compliance with Regulations
Ensure that model training, deployment, and operations comply with relevant regulations like GDPR for data privacy or HIPAA for healthcare information.
Next, we'll explore some of the challenges and future directions in integrating AI with CI systems, shedding light on areas like handling large models, ensuring explainability, and maintaining ethical standards.
Let's explore some of the key challenges faced when integrating AI models with Continuous Integration systems, as well as future directions and potential advancements in this field.
Challenges and Future Directions

Handling Large and Complex Models
The complexity and size of AI models, especially deep learning models, pose significant challenges in terms of computational resources and integration complexity.
Challenge: Managing the computational load and memory requirements of large models can be challenging, especially when trying to integrate these models into existing CI pipelines that are not originally designed to handle such loads.
Future Direction: Development of more efficient model architectures and compression techniques to reduce the size and computational demands of AI models without sacrificing performance.
Explainable AI and Interpretability
As AI models become more prevalent in critical applications, the need for explainability and interpretability grows. Stakeholders not only need to trust the model's outputs but also understand how decisions are made.
Challenge: Deep learning models, in particular, are often seen as "black boxes" due to their complex nature, making it difficult to interpret their decision-making processes.
Future Direction: Increased focus on developing techniques and tools for Explainable AI (XAI) that can make these models more transparent and understandable to users.
Ethical Considerations and Responsible AI
Integrating AI into CI systems must also consider ethical implications, ensuring that AI systems operate within accepted moral and ethical boundaries.
Challenge: Ensuring AI systems do not perpetuate biases or make unfair decisions, especially in sectors like recruitment, healthcare, and law enforcement.
Future Direction: Implementing and enforcing ethical guidelines and standards for AI development and deployment. This includes continuous monitoring and testing to detect and mitigate biases in AI models.
Case Studies and Examples
For incorporating AI models with continuous integration (CI) systems, it’s advantageous to see a concrete instance to comprehend how this can sleek your evolution process. Let’s know through a comprehensive case study using Jenkins, a popular CI/CD framework, to exhibit how you can effectively incorporate your AI models into a CI pipeline.
Setting the Stage: Your Project
Suppose you’re working on an AI project that indulges developing a model to forecast customer behavior based on their previous interactions with your website. Your aim is to constantly enhance the model based on new information and deploy updates without disturbing the user experience.
Integrating AI with Jenkins
Setting Up Jenkins: Initially, set up Jenkins on your server. You will need to install the significant plugins for your project, like Git for source control and Machine Learning plugin if you’re dealing with precise ML tasks.
Creating a Pipeline: Create a Jenkins pipeline that triggers every time you push new code to your Git storage. This pipeline should include steps such as data preprocessing, model training, assessment, and deployment.
Data Management: Ensure your information is attainable and correctly formatted for training. Jenkins can automatically pull the newest information from your repository solution (like AWS S3) before each training session.
Training the Model: Use Jenkins to enforce your training scripts. Configure your pipeline to use virtual environments or Docker containers to sustain reliability and ensure your training environment is constant.
Evaluating the Model: After training, assess your model’s performance using verification data. Set up norms for performance metrics (like precision or FI- score) that must be met before proceeding with deployment.
Automating Testing and Deployment: If the model meets the performance norms, automatically deploy it to your production environment. Jenkins can handle rolling updates to ensure there’s no interruption for your users.
Monitoring and Feedback: Once deployed, monitor the model’s performance in real-time. Jenkins can be configured to alert you if the model’s performance deteriorates, triggering a retraining cycle with new data.
Why Jenkins?
Using Jenkins for CI/CD in AI projects provides numerous benefits:
Automation: Automates the whole lifecycle of your model from data preprocessing to deployment.
Scalability: Easily scales with your project as it evolves in intricacy and information volume.
Consistency: Ensures that every version of the model is examined and deployed in the same manner.
By incorporating AI models with Jenkins, you sleek your productivity, reduce the threat of mistakes, and ensure that your models are always upgraded and ready for production. This approach not only saves time but also substantially enhances the dependability of your AI applications.
Real-world Examples of AI Model Integration with CI Systems
Tech Companies
Google: Google uses AI extensively in its CI/CD pipelines to enhance various products. For instance, Google Photos uses machine learning models to power features like image recognition and auto-tagging. These models are continuously updated and deployed using Google's robust CI/CD systems that handle billions of images.
Netflix: Netflix utilizes AI to refine and improve its recommendation algorithms continuously. The integration of these AI models into their CI/CD pipeline allows for rapid iteration and deployment, which helps Netflix tailor content to user preferences more effectively and maintain its competitive edge in streaming.
Facebook: At Facebook, AI models are integrated into a CI/CD framework to manage and improve the performance of various features like news feed algorithms and ad targeting. The company uses AI to analyze user interactions and automatically tests and rolls out updates to enhance user engagement.
Healthcare
PathAI: This company is using AI to assist pathologists in diagnosing and researching diseases more accurately and efficiently. PathAI integrates AI models into its CI/CD pipelines to ensure that the latest medical research and data can be continuously incorporated into their models, improving diagnostic accuracy.
Tempus: Tempus applies AI in precision medicine to personalize cancer treatments. Their CI/CD system ensures that AI models are frequently updated with the latest clinical data, helping to tailor treatments to individual patients based on genetic data and disease markers.
Lessons Learned and Best Practices from Industry
Continuous Monitoring: Companies like Google and Netflix have robust monitoring systems that continuously assess the performance of deployed AI models. This practice helps in quickly identifying and addressing issues that could affect user experience or system performance.
Staged Deployment: Facebook and other tech giants often use staged deployment strategies. By gradually rolling out updates, they can monitor the impact and effectiveness of new models under real-world conditions without fully committing all resources. This method helps mitigate risks and allows for adjustments before wider release.
These examples demonstrate the effectiveness of integrating AI models with CI/CD systems across various sectors. By adopting best practices such as continuous monitoring and staged deployment, companies can ensure that their AI-driven innovations are delivered efficiently and reliably, providing them with a strategic advantage in their respective fields.
As we've explored the integration of AI models into Continuous Integration (CI) systems, let's now focus on some key resources and further readings that can provide deeper insights and practical guidance for those interested in further exploring this field.
Conclusion
Integrating AI models into CI systems is a dynamic and evolving field that bridges the gap between traditional software development practices and the innovative capabilities of AI. As this technology continues to evolve, staying informed through credible resources, continuous learning, and community engagement will be key to leveraging its full potential.
The integration of AI and CI is not just about enhancing software development workflows but also about transforming how businesses operate and compete in an increasingly data-driven world. As we look forward, the potential for AI to revolutionize industries by making software development faster, more efficient, and increasingly automated is immense.
Whether you're a developer, a data scientist, or a business leader, understanding and implementing AI within CI systems can provide a competitive edge and drive significant advancements in your projects and products.
Continuous Integration (CI) is a software development practice where developers frequently merge their code changes into a central repository, after which automated builds and tests are run. The primary goals of CI are to improve software quality and to reduce the time taken to deliver it by replacing the traditional practice of developers working in isolation for an extended period.
Integrating AI models into CI systems brings significant benefits, such as ensuring the consistency of AI models with the application code, automating the model training and deployment processes, and continuously evaluating the model against real-world data and metrics.
Integrating AI Models with CI Systems
Automating Model Training and Evaluation
Integrating AI models into CI systems involves automating the model training and evaluation process, which is crucial for maintaining model accuracy and performance over time.
Triggering Model Training on Code Changes
Automating the training process begins with setting up triggers in the CI system so that any changes to model code or data automatically initiate a new training cycle. This ensures that the model adapts continuously to new data or changes in business requirements.
Automated Testing and Evaluation
After training, the model is automatically evaluated to ensure it meets predefined performance criteria. This might involve accuracy checks, performance metrics, or other validation techniques to ensure the model's effectiveness before it moves to deployment.
Continuous Delivery of AI Models
Continuous Delivery (CD) extends the concept of CI by ensuring that, in addition to automated testing, the model can be released to production reliably at any time.
Packaging and Versioning Models
AI models are packaged with all necessary components (including data preprocessing and post-processing stages) and versioned. This versioning is crucial for rollback scenarios and understanding model evolution over time.
Deploying Models to Production Environments
Automated scripts or workflows ensure models are deployed into production environments without requiring manual intervention. This can include updates to live systems or staging environments for further acceptance testing.
Monitoring and Maintenance
Once deployed, it's vital to continuously monitor the model's performance to catch any issues that might arise due to changing data patterns or other external factors.
Monitoring Model Performance
Continuous monitoring tools are used to track how well the model is performing in real-time. This can help detect performance degradation or failures, triggering alerts for human intervention or automated retraining workflows.
Retraining and Updating Models
AI models can drift from their trained state due to changes in input data over time. CI systems can automate the process of retraining and updating models, ensuring they remain accurate and relevant.
Best Practices
When integrating AI models with CI systems, certain best practices ensure the process is efficient and effective:
Reproducibility and Versioning
Versioning Data, Code, and Models
Maintain rigorous version control of not only the code but also the datasets and model versions. This aids in tracking changes, ensuring reproducibility, and facilitating audits.
Reproducible Training Environments
Use containerization technologies like Docker to create reproducible training environments. This minimizes the "it works on my machine" problem and ensures consistency across development, testing, and production environments.
Efficient Resource Management
Scaling Compute Resources for Training
Utilize dynamic resource allocation strategies to scale up resources for model training and scale down when not in use, optimizing cost and efficiency.
Optimizing Model Serving
Depending on the model complexity and inference speed requirements, choose the appropriate serving architecture, whether it's on-premises, in the cloud, or at the edge.
Testing and Validation
Unit and Integration Testing for AI Components
Develop comprehensive test suites for AI components to ensure they function correctly as standalone units and when integrated with other system components.
Validating Model Outputs
Regularly validate model outputs against expected outcomes to catch any anomalies or deviations that could indicate problems with the model.
Security and Compliance
Secure Model Deployment and Serving
Implement robust security protocols for deploying and serving models, including authentication, encryption, and secure access controls.
Compliance with Regulations
Ensure that model training, deployment, and operations comply with relevant regulations like GDPR for data privacy or HIPAA for healthcare information.
Next, we'll explore some of the challenges and future directions in integrating AI with CI systems, shedding light on areas like handling large models, ensuring explainability, and maintaining ethical standards.
Let's explore some of the key challenges faced when integrating AI models with Continuous Integration systems, as well as future directions and potential advancements in this field.
Challenges and Future Directions

Handling Large and Complex Models
The complexity and size of AI models, especially deep learning models, pose significant challenges in terms of computational resources and integration complexity.
Challenge: Managing the computational load and memory requirements of large models can be challenging, especially when trying to integrate these models into existing CI pipelines that are not originally designed to handle such loads.
Future Direction: Development of more efficient model architectures and compression techniques to reduce the size and computational demands of AI models without sacrificing performance.
Explainable AI and Interpretability
As AI models become more prevalent in critical applications, the need for explainability and interpretability grows. Stakeholders not only need to trust the model's outputs but also understand how decisions are made.
Challenge: Deep learning models, in particular, are often seen as "black boxes" due to their complex nature, making it difficult to interpret their decision-making processes.
Future Direction: Increased focus on developing techniques and tools for Explainable AI (XAI) that can make these models more transparent and understandable to users.
Ethical Considerations and Responsible AI
Integrating AI into CI systems must also consider ethical implications, ensuring that AI systems operate within accepted moral and ethical boundaries.
Challenge: Ensuring AI systems do not perpetuate biases or make unfair decisions, especially in sectors like recruitment, healthcare, and law enforcement.
Future Direction: Implementing and enforcing ethical guidelines and standards for AI development and deployment. This includes continuous monitoring and testing to detect and mitigate biases in AI models.
Case Studies and Examples
For incorporating AI models with continuous integration (CI) systems, it’s advantageous to see a concrete instance to comprehend how this can sleek your evolution process. Let’s know through a comprehensive case study using Jenkins, a popular CI/CD framework, to exhibit how you can effectively incorporate your AI models into a CI pipeline.
Setting the Stage: Your Project
Suppose you’re working on an AI project that indulges developing a model to forecast customer behavior based on their previous interactions with your website. Your aim is to constantly enhance the model based on new information and deploy updates without disturbing the user experience.
Integrating AI with Jenkins
Setting Up Jenkins: Initially, set up Jenkins on your server. You will need to install the significant plugins for your project, like Git for source control and Machine Learning plugin if you’re dealing with precise ML tasks.
Creating a Pipeline: Create a Jenkins pipeline that triggers every time you push new code to your Git storage. This pipeline should include steps such as data preprocessing, model training, assessment, and deployment.
Data Management: Ensure your information is attainable and correctly formatted for training. Jenkins can automatically pull the newest information from your repository solution (like AWS S3) before each training session.
Training the Model: Use Jenkins to enforce your training scripts. Configure your pipeline to use virtual environments or Docker containers to sustain reliability and ensure your training environment is constant.
Evaluating the Model: After training, assess your model’s performance using verification data. Set up norms for performance metrics (like precision or FI- score) that must be met before proceeding with deployment.
Automating Testing and Deployment: If the model meets the performance norms, automatically deploy it to your production environment. Jenkins can handle rolling updates to ensure there’s no interruption for your users.
Monitoring and Feedback: Once deployed, monitor the model’s performance in real-time. Jenkins can be configured to alert you if the model’s performance deteriorates, triggering a retraining cycle with new data.
Why Jenkins?
Using Jenkins for CI/CD in AI projects provides numerous benefits:
Automation: Automates the whole lifecycle of your model from data preprocessing to deployment.
Scalability: Easily scales with your project as it evolves in intricacy and information volume.
Consistency: Ensures that every version of the model is examined and deployed in the same manner.
By incorporating AI models with Jenkins, you sleek your productivity, reduce the threat of mistakes, and ensure that your models are always upgraded and ready for production. This approach not only saves time but also substantially enhances the dependability of your AI applications.
Real-world Examples of AI Model Integration with CI Systems
Tech Companies
Google: Google uses AI extensively in its CI/CD pipelines to enhance various products. For instance, Google Photos uses machine learning models to power features like image recognition and auto-tagging. These models are continuously updated and deployed using Google's robust CI/CD systems that handle billions of images.
Netflix: Netflix utilizes AI to refine and improve its recommendation algorithms continuously. The integration of these AI models into their CI/CD pipeline allows for rapid iteration and deployment, which helps Netflix tailor content to user preferences more effectively and maintain its competitive edge in streaming.
Facebook: At Facebook, AI models are integrated into a CI/CD framework to manage and improve the performance of various features like news feed algorithms and ad targeting. The company uses AI to analyze user interactions and automatically tests and rolls out updates to enhance user engagement.
Healthcare
PathAI: This company is using AI to assist pathologists in diagnosing and researching diseases more accurately and efficiently. PathAI integrates AI models into its CI/CD pipelines to ensure that the latest medical research and data can be continuously incorporated into their models, improving diagnostic accuracy.
Tempus: Tempus applies AI in precision medicine to personalize cancer treatments. Their CI/CD system ensures that AI models are frequently updated with the latest clinical data, helping to tailor treatments to individual patients based on genetic data and disease markers.
Lessons Learned and Best Practices from Industry
Continuous Monitoring: Companies like Google and Netflix have robust monitoring systems that continuously assess the performance of deployed AI models. This practice helps in quickly identifying and addressing issues that could affect user experience or system performance.
Staged Deployment: Facebook and other tech giants often use staged deployment strategies. By gradually rolling out updates, they can monitor the impact and effectiveness of new models under real-world conditions without fully committing all resources. This method helps mitigate risks and allows for adjustments before wider release.
These examples demonstrate the effectiveness of integrating AI models with CI/CD systems across various sectors. By adopting best practices such as continuous monitoring and staged deployment, companies can ensure that their AI-driven innovations are delivered efficiently and reliably, providing them with a strategic advantage in their respective fields.
As we've explored the integration of AI models into Continuous Integration (CI) systems, let's now focus on some key resources and further readings that can provide deeper insights and practical guidance for those interested in further exploring this field.
Conclusion
Integrating AI models into CI systems is a dynamic and evolving field that bridges the gap between traditional software development practices and the innovative capabilities of AI. As this technology continues to evolve, staying informed through credible resources, continuous learning, and community engagement will be key to leveraging its full potential.
The integration of AI and CI is not just about enhancing software development workflows but also about transforming how businesses operate and compete in an increasingly data-driven world. As we look forward, the potential for AI to revolutionize industries by making software development faster, more efficient, and increasingly automated is immense.
Whether you're a developer, a data scientist, or a business leader, understanding and implementing AI within CI systems can provide a competitive edge and drive significant advancements in your projects and products.
Continuous Integration (CI) is a software development practice where developers frequently merge their code changes into a central repository, after which automated builds and tests are run. The primary goals of CI are to improve software quality and to reduce the time taken to deliver it by replacing the traditional practice of developers working in isolation for an extended period.
Integrating AI models into CI systems brings significant benefits, such as ensuring the consistency of AI models with the application code, automating the model training and deployment processes, and continuously evaluating the model against real-world data and metrics.
Integrating AI Models with CI Systems
Automating Model Training and Evaluation
Integrating AI models into CI systems involves automating the model training and evaluation process, which is crucial for maintaining model accuracy and performance over time.
Triggering Model Training on Code Changes
Automating the training process begins with setting up triggers in the CI system so that any changes to model code or data automatically initiate a new training cycle. This ensures that the model adapts continuously to new data or changes in business requirements.
Automated Testing and Evaluation
After training, the model is automatically evaluated to ensure it meets predefined performance criteria. This might involve accuracy checks, performance metrics, or other validation techniques to ensure the model's effectiveness before it moves to deployment.
Continuous Delivery of AI Models
Continuous Delivery (CD) extends the concept of CI by ensuring that, in addition to automated testing, the model can be released to production reliably at any time.
Packaging and Versioning Models
AI models are packaged with all necessary components (including data preprocessing and post-processing stages) and versioned. This versioning is crucial for rollback scenarios and understanding model evolution over time.
Deploying Models to Production Environments
Automated scripts or workflows ensure models are deployed into production environments without requiring manual intervention. This can include updates to live systems or staging environments for further acceptance testing.
Monitoring and Maintenance
Once deployed, it's vital to continuously monitor the model's performance to catch any issues that might arise due to changing data patterns or other external factors.
Monitoring Model Performance
Continuous monitoring tools are used to track how well the model is performing in real-time. This can help detect performance degradation or failures, triggering alerts for human intervention or automated retraining workflows.
Retraining and Updating Models
AI models can drift from their trained state due to changes in input data over time. CI systems can automate the process of retraining and updating models, ensuring they remain accurate and relevant.
Best Practices
When integrating AI models with CI systems, certain best practices ensure the process is efficient and effective:
Reproducibility and Versioning
Versioning Data, Code, and Models
Maintain rigorous version control of not only the code but also the datasets and model versions. This aids in tracking changes, ensuring reproducibility, and facilitating audits.
Reproducible Training Environments
Use containerization technologies like Docker to create reproducible training environments. This minimizes the "it works on my machine" problem and ensures consistency across development, testing, and production environments.
Efficient Resource Management
Scaling Compute Resources for Training
Utilize dynamic resource allocation strategies to scale up resources for model training and scale down when not in use, optimizing cost and efficiency.
Optimizing Model Serving
Depending on the model complexity and inference speed requirements, choose the appropriate serving architecture, whether it's on-premises, in the cloud, or at the edge.
Testing and Validation
Unit and Integration Testing for AI Components
Develop comprehensive test suites for AI components to ensure they function correctly as standalone units and when integrated with other system components.
Validating Model Outputs
Regularly validate model outputs against expected outcomes to catch any anomalies or deviations that could indicate problems with the model.
Security and Compliance
Secure Model Deployment and Serving
Implement robust security protocols for deploying and serving models, including authentication, encryption, and secure access controls.
Compliance with Regulations
Ensure that model training, deployment, and operations comply with relevant regulations like GDPR for data privacy or HIPAA for healthcare information.
Next, we'll explore some of the challenges and future directions in integrating AI with CI systems, shedding light on areas like handling large models, ensuring explainability, and maintaining ethical standards.
Let's explore some of the key challenges faced when integrating AI models with Continuous Integration systems, as well as future directions and potential advancements in this field.
Challenges and Future Directions

Handling Large and Complex Models
The complexity and size of AI models, especially deep learning models, pose significant challenges in terms of computational resources and integration complexity.
Challenge: Managing the computational load and memory requirements of large models can be challenging, especially when trying to integrate these models into existing CI pipelines that are not originally designed to handle such loads.
Future Direction: Development of more efficient model architectures and compression techniques to reduce the size and computational demands of AI models without sacrificing performance.
Explainable AI and Interpretability
As AI models become more prevalent in critical applications, the need for explainability and interpretability grows. Stakeholders not only need to trust the model's outputs but also understand how decisions are made.
Challenge: Deep learning models, in particular, are often seen as "black boxes" due to their complex nature, making it difficult to interpret their decision-making processes.
Future Direction: Increased focus on developing techniques and tools for Explainable AI (XAI) that can make these models more transparent and understandable to users.
Ethical Considerations and Responsible AI
Integrating AI into CI systems must also consider ethical implications, ensuring that AI systems operate within accepted moral and ethical boundaries.
Challenge: Ensuring AI systems do not perpetuate biases or make unfair decisions, especially in sectors like recruitment, healthcare, and law enforcement.
Future Direction: Implementing and enforcing ethical guidelines and standards for AI development and deployment. This includes continuous monitoring and testing to detect and mitigate biases in AI models.
Case Studies and Examples
For incorporating AI models with continuous integration (CI) systems, it’s advantageous to see a concrete instance to comprehend how this can sleek your evolution process. Let’s know through a comprehensive case study using Jenkins, a popular CI/CD framework, to exhibit how you can effectively incorporate your AI models into a CI pipeline.
Setting the Stage: Your Project
Suppose you’re working on an AI project that indulges developing a model to forecast customer behavior based on their previous interactions with your website. Your aim is to constantly enhance the model based on new information and deploy updates without disturbing the user experience.
Integrating AI with Jenkins
Setting Up Jenkins: Initially, set up Jenkins on your server. You will need to install the significant plugins for your project, like Git for source control and Machine Learning plugin if you’re dealing with precise ML tasks.
Creating a Pipeline: Create a Jenkins pipeline that triggers every time you push new code to your Git storage. This pipeline should include steps such as data preprocessing, model training, assessment, and deployment.
Data Management: Ensure your information is attainable and correctly formatted for training. Jenkins can automatically pull the newest information from your repository solution (like AWS S3) before each training session.
Training the Model: Use Jenkins to enforce your training scripts. Configure your pipeline to use virtual environments or Docker containers to sustain reliability and ensure your training environment is constant.
Evaluating the Model: After training, assess your model’s performance using verification data. Set up norms for performance metrics (like precision or FI- score) that must be met before proceeding with deployment.
Automating Testing and Deployment: If the model meets the performance norms, automatically deploy it to your production environment. Jenkins can handle rolling updates to ensure there’s no interruption for your users.
Monitoring and Feedback: Once deployed, monitor the model’s performance in real-time. Jenkins can be configured to alert you if the model’s performance deteriorates, triggering a retraining cycle with new data.
Why Jenkins?
Using Jenkins for CI/CD in AI projects provides numerous benefits:
Automation: Automates the whole lifecycle of your model from data preprocessing to deployment.
Scalability: Easily scales with your project as it evolves in intricacy and information volume.
Consistency: Ensures that every version of the model is examined and deployed in the same manner.
By incorporating AI models with Jenkins, you sleek your productivity, reduce the threat of mistakes, and ensure that your models are always upgraded and ready for production. This approach not only saves time but also substantially enhances the dependability of your AI applications.
Real-world Examples of AI Model Integration with CI Systems
Tech Companies
Google: Google uses AI extensively in its CI/CD pipelines to enhance various products. For instance, Google Photos uses machine learning models to power features like image recognition and auto-tagging. These models are continuously updated and deployed using Google's robust CI/CD systems that handle billions of images.
Netflix: Netflix utilizes AI to refine and improve its recommendation algorithms continuously. The integration of these AI models into their CI/CD pipeline allows for rapid iteration and deployment, which helps Netflix tailor content to user preferences more effectively and maintain its competitive edge in streaming.
Facebook: At Facebook, AI models are integrated into a CI/CD framework to manage and improve the performance of various features like news feed algorithms and ad targeting. The company uses AI to analyze user interactions and automatically tests and rolls out updates to enhance user engagement.
Healthcare
PathAI: This company is using AI to assist pathologists in diagnosing and researching diseases more accurately and efficiently. PathAI integrates AI models into its CI/CD pipelines to ensure that the latest medical research and data can be continuously incorporated into their models, improving diagnostic accuracy.
Tempus: Tempus applies AI in precision medicine to personalize cancer treatments. Their CI/CD system ensures that AI models are frequently updated with the latest clinical data, helping to tailor treatments to individual patients based on genetic data and disease markers.
Lessons Learned and Best Practices from Industry
Continuous Monitoring: Companies like Google and Netflix have robust monitoring systems that continuously assess the performance of deployed AI models. This practice helps in quickly identifying and addressing issues that could affect user experience or system performance.
Staged Deployment: Facebook and other tech giants often use staged deployment strategies. By gradually rolling out updates, they can monitor the impact and effectiveness of new models under real-world conditions without fully committing all resources. This method helps mitigate risks and allows for adjustments before wider release.
These examples demonstrate the effectiveness of integrating AI models with CI/CD systems across various sectors. By adopting best practices such as continuous monitoring and staged deployment, companies can ensure that their AI-driven innovations are delivered efficiently and reliably, providing them with a strategic advantage in their respective fields.
As we've explored the integration of AI models into Continuous Integration (CI) systems, let's now focus on some key resources and further readings that can provide deeper insights and practical guidance for those interested in further exploring this field.
Conclusion
Integrating AI models into CI systems is a dynamic and evolving field that bridges the gap between traditional software development practices and the innovative capabilities of AI. As this technology continues to evolve, staying informed through credible resources, continuous learning, and community engagement will be key to leveraging its full potential.
The integration of AI and CI is not just about enhancing software development workflows but also about transforming how businesses operate and compete in an increasingly data-driven world. As we look forward, the potential for AI to revolutionize industries by making software development faster, more efficient, and increasingly automated is immense.
Whether you're a developer, a data scientist, or a business leader, understanding and implementing AI within CI systems can provide a competitive edge and drive significant advancements in your projects and products.
Continuous Integration (CI) is a software development practice where developers frequently merge their code changes into a central repository, after which automated builds and tests are run. The primary goals of CI are to improve software quality and to reduce the time taken to deliver it by replacing the traditional practice of developers working in isolation for an extended period.
Integrating AI models into CI systems brings significant benefits, such as ensuring the consistency of AI models with the application code, automating the model training and deployment processes, and continuously evaluating the model against real-world data and metrics.
Integrating AI Models with CI Systems
Automating Model Training and Evaluation
Integrating AI models into CI systems involves automating the model training and evaluation process, which is crucial for maintaining model accuracy and performance over time.
Triggering Model Training on Code Changes
Automating the training process begins with setting up triggers in the CI system so that any changes to model code or data automatically initiate a new training cycle. This ensures that the model adapts continuously to new data or changes in business requirements.
Automated Testing and Evaluation
After training, the model is automatically evaluated to ensure it meets predefined performance criteria. This might involve accuracy checks, performance metrics, or other validation techniques to ensure the model's effectiveness before it moves to deployment.
Continuous Delivery of AI Models
Continuous Delivery (CD) extends the concept of CI by ensuring that, in addition to automated testing, the model can be released to production reliably at any time.
Packaging and Versioning Models
AI models are packaged with all necessary components (including data preprocessing and post-processing stages) and versioned. This versioning is crucial for rollback scenarios and understanding model evolution over time.
Deploying Models to Production Environments
Automated scripts or workflows ensure models are deployed into production environments without requiring manual intervention. This can include updates to live systems or staging environments for further acceptance testing.
Monitoring and Maintenance
Once deployed, it's vital to continuously monitor the model's performance to catch any issues that might arise due to changing data patterns or other external factors.
Monitoring Model Performance
Continuous monitoring tools are used to track how well the model is performing in real-time. This can help detect performance degradation or failures, triggering alerts for human intervention or automated retraining workflows.
Retraining and Updating Models
AI models can drift from their trained state due to changes in input data over time. CI systems can automate the process of retraining and updating models, ensuring they remain accurate and relevant.
Best Practices
When integrating AI models with CI systems, certain best practices ensure the process is efficient and effective:
Reproducibility and Versioning
Versioning Data, Code, and Models
Maintain rigorous version control of not only the code but also the datasets and model versions. This aids in tracking changes, ensuring reproducibility, and facilitating audits.
Reproducible Training Environments
Use containerization technologies like Docker to create reproducible training environments. This minimizes the "it works on my machine" problem and ensures consistency across development, testing, and production environments.
Efficient Resource Management
Scaling Compute Resources for Training
Utilize dynamic resource allocation strategies to scale up resources for model training and scale down when not in use, optimizing cost and efficiency.
Optimizing Model Serving
Depending on the model complexity and inference speed requirements, choose the appropriate serving architecture, whether it's on-premises, in the cloud, or at the edge.
Testing and Validation
Unit and Integration Testing for AI Components
Develop comprehensive test suites for AI components to ensure they function correctly as standalone units and when integrated with other system components.
Validating Model Outputs
Regularly validate model outputs against expected outcomes to catch any anomalies or deviations that could indicate problems with the model.
Security and Compliance
Secure Model Deployment and Serving
Implement robust security protocols for deploying and serving models, including authentication, encryption, and secure access controls.
Compliance with Regulations
Ensure that model training, deployment, and operations comply with relevant regulations like GDPR for data privacy or HIPAA for healthcare information.
Next, we'll explore some of the challenges and future directions in integrating AI with CI systems, shedding light on areas like handling large models, ensuring explainability, and maintaining ethical standards.
Let's explore some of the key challenges faced when integrating AI models with Continuous Integration systems, as well as future directions and potential advancements in this field.
Challenges and Future Directions

Handling Large and Complex Models
The complexity and size of AI models, especially deep learning models, pose significant challenges in terms of computational resources and integration complexity.
Challenge: Managing the computational load and memory requirements of large models can be challenging, especially when trying to integrate these models into existing CI pipelines that are not originally designed to handle such loads.
Future Direction: Development of more efficient model architectures and compression techniques to reduce the size and computational demands of AI models without sacrificing performance.
Explainable AI and Interpretability
As AI models become more prevalent in critical applications, the need for explainability and interpretability grows. Stakeholders not only need to trust the model's outputs but also understand how decisions are made.
Challenge: Deep learning models, in particular, are often seen as "black boxes" due to their complex nature, making it difficult to interpret their decision-making processes.
Future Direction: Increased focus on developing techniques and tools for Explainable AI (XAI) that can make these models more transparent and understandable to users.
Ethical Considerations and Responsible AI
Integrating AI into CI systems must also consider ethical implications, ensuring that AI systems operate within accepted moral and ethical boundaries.
Challenge: Ensuring AI systems do not perpetuate biases or make unfair decisions, especially in sectors like recruitment, healthcare, and law enforcement.
Future Direction: Implementing and enforcing ethical guidelines and standards for AI development and deployment. This includes continuous monitoring and testing to detect and mitigate biases in AI models.
Case Studies and Examples
For incorporating AI models with continuous integration (CI) systems, it’s advantageous to see a concrete instance to comprehend how this can sleek your evolution process. Let’s know through a comprehensive case study using Jenkins, a popular CI/CD framework, to exhibit how you can effectively incorporate your AI models into a CI pipeline.
Setting the Stage: Your Project
Suppose you’re working on an AI project that indulges developing a model to forecast customer behavior based on their previous interactions with your website. Your aim is to constantly enhance the model based on new information and deploy updates without disturbing the user experience.
Integrating AI with Jenkins
Setting Up Jenkins: Initially, set up Jenkins on your server. You will need to install the significant plugins for your project, like Git for source control and Machine Learning plugin if you’re dealing with precise ML tasks.
Creating a Pipeline: Create a Jenkins pipeline that triggers every time you push new code to your Git storage. This pipeline should include steps such as data preprocessing, model training, assessment, and deployment.
Data Management: Ensure your information is attainable and correctly formatted for training. Jenkins can automatically pull the newest information from your repository solution (like AWS S3) before each training session.
Training the Model: Use Jenkins to enforce your training scripts. Configure your pipeline to use virtual environments or Docker containers to sustain reliability and ensure your training environment is constant.
Evaluating the Model: After training, assess your model’s performance using verification data. Set up norms for performance metrics (like precision or FI- score) that must be met before proceeding with deployment.
Automating Testing and Deployment: If the model meets the performance norms, automatically deploy it to your production environment. Jenkins can handle rolling updates to ensure there’s no interruption for your users.
Monitoring and Feedback: Once deployed, monitor the model’s performance in real-time. Jenkins can be configured to alert you if the model’s performance deteriorates, triggering a retraining cycle with new data.
Why Jenkins?
Using Jenkins for CI/CD in AI projects provides numerous benefits:
Automation: Automates the whole lifecycle of your model from data preprocessing to deployment.
Scalability: Easily scales with your project as it evolves in intricacy and information volume.
Consistency: Ensures that every version of the model is examined and deployed in the same manner.
By incorporating AI models with Jenkins, you sleek your productivity, reduce the threat of mistakes, and ensure that your models are always upgraded and ready for production. This approach not only saves time but also substantially enhances the dependability of your AI applications.
Real-world Examples of AI Model Integration with CI Systems
Tech Companies
Google: Google uses AI extensively in its CI/CD pipelines to enhance various products. For instance, Google Photos uses machine learning models to power features like image recognition and auto-tagging. These models are continuously updated and deployed using Google's robust CI/CD systems that handle billions of images.
Netflix: Netflix utilizes AI to refine and improve its recommendation algorithms continuously. The integration of these AI models into their CI/CD pipeline allows for rapid iteration and deployment, which helps Netflix tailor content to user preferences more effectively and maintain its competitive edge in streaming.
Facebook: At Facebook, AI models are integrated into a CI/CD framework to manage and improve the performance of various features like news feed algorithms and ad targeting. The company uses AI to analyze user interactions and automatically tests and rolls out updates to enhance user engagement.
Healthcare
PathAI: This company is using AI to assist pathologists in diagnosing and researching diseases more accurately and efficiently. PathAI integrates AI models into its CI/CD pipelines to ensure that the latest medical research and data can be continuously incorporated into their models, improving diagnostic accuracy.
Tempus: Tempus applies AI in precision medicine to personalize cancer treatments. Their CI/CD system ensures that AI models are frequently updated with the latest clinical data, helping to tailor treatments to individual patients based on genetic data and disease markers.
Lessons Learned and Best Practices from Industry
Continuous Monitoring: Companies like Google and Netflix have robust monitoring systems that continuously assess the performance of deployed AI models. This practice helps in quickly identifying and addressing issues that could affect user experience or system performance.
Staged Deployment: Facebook and other tech giants often use staged deployment strategies. By gradually rolling out updates, they can monitor the impact and effectiveness of new models under real-world conditions without fully committing all resources. This method helps mitigate risks and allows for adjustments before wider release.
These examples demonstrate the effectiveness of integrating AI models with CI/CD systems across various sectors. By adopting best practices such as continuous monitoring and staged deployment, companies can ensure that their AI-driven innovations are delivered efficiently and reliably, providing them with a strategic advantage in their respective fields.
As we've explored the integration of AI models into Continuous Integration (CI) systems, let's now focus on some key resources and further readings that can provide deeper insights and practical guidance for those interested in further exploring this field.
Conclusion
Integrating AI models into CI systems is a dynamic and evolving field that bridges the gap between traditional software development practices and the innovative capabilities of AI. As this technology continues to evolve, staying informed through credible resources, continuous learning, and community engagement will be key to leveraging its full potential.
The integration of AI and CI is not just about enhancing software development workflows but also about transforming how businesses operate and compete in an increasingly data-driven world. As we look forward, the potential for AI to revolutionize industries by making software development faster, more efficient, and increasingly automated is immense.
Whether you're a developer, a data scientist, or a business leader, understanding and implementing AI within CI systems can provide a competitive edge and drive significant advancements in your projects and products.
Continuous Integration (CI) is a software development practice where developers frequently merge their code changes into a central repository, after which automated builds and tests are run. The primary goals of CI are to improve software quality and to reduce the time taken to deliver it by replacing the traditional practice of developers working in isolation for an extended period.
Integrating AI models into CI systems brings significant benefits, such as ensuring the consistency of AI models with the application code, automating the model training and deployment processes, and continuously evaluating the model against real-world data and metrics.
Integrating AI Models with CI Systems
Automating Model Training and Evaluation
Integrating AI models into CI systems involves automating the model training and evaluation process, which is crucial for maintaining model accuracy and performance over time.
Triggering Model Training on Code Changes
Automating the training process begins with setting up triggers in the CI system so that any changes to model code or data automatically initiate a new training cycle. This ensures that the model adapts continuously to new data or changes in business requirements.
Automated Testing and Evaluation
After training, the model is automatically evaluated to ensure it meets predefined performance criteria. This might involve accuracy checks, performance metrics, or other validation techniques to ensure the model's effectiveness before it moves to deployment.
Continuous Delivery of AI Models
Continuous Delivery (CD) extends the concept of CI by ensuring that, in addition to automated testing, the model can be released to production reliably at any time.
Packaging and Versioning Models
AI models are packaged with all necessary components (including data preprocessing and post-processing stages) and versioned. This versioning is crucial for rollback scenarios and understanding model evolution over time.
Deploying Models to Production Environments
Automated scripts or workflows ensure models are deployed into production environments without requiring manual intervention. This can include updates to live systems or staging environments for further acceptance testing.
Monitoring and Maintenance
Once deployed, it's vital to continuously monitor the model's performance to catch any issues that might arise due to changing data patterns or other external factors.
Monitoring Model Performance
Continuous monitoring tools are used to track how well the model is performing in real-time. This can help detect performance degradation or failures, triggering alerts for human intervention or automated retraining workflows.
Retraining and Updating Models
AI models can drift from their trained state due to changes in input data over time. CI systems can automate the process of retraining and updating models, ensuring they remain accurate and relevant.
Best Practices
When integrating AI models with CI systems, certain best practices ensure the process is efficient and effective:
Reproducibility and Versioning
Versioning Data, Code, and Models
Maintain rigorous version control of not only the code but also the datasets and model versions. This aids in tracking changes, ensuring reproducibility, and facilitating audits.
Reproducible Training Environments
Use containerization technologies like Docker to create reproducible training environments. This minimizes the "it works on my machine" problem and ensures consistency across development, testing, and production environments.
Efficient Resource Management
Scaling Compute Resources for Training
Utilize dynamic resource allocation strategies to scale up resources for model training and scale down when not in use, optimizing cost and efficiency.
Optimizing Model Serving
Depending on the model complexity and inference speed requirements, choose the appropriate serving architecture, whether it's on-premises, in the cloud, or at the edge.
Testing and Validation
Unit and Integration Testing for AI Components
Develop comprehensive test suites for AI components to ensure they function correctly as standalone units and when integrated with other system components.
Validating Model Outputs
Regularly validate model outputs against expected outcomes to catch any anomalies or deviations that could indicate problems with the model.
Security and Compliance
Secure Model Deployment and Serving
Implement robust security protocols for deploying and serving models, including authentication, encryption, and secure access controls.
Compliance with Regulations
Ensure that model training, deployment, and operations comply with relevant regulations like GDPR for data privacy or HIPAA for healthcare information.
Next, we'll explore some of the challenges and future directions in integrating AI with CI systems, shedding light on areas like handling large models, ensuring explainability, and maintaining ethical standards.
Let's explore some of the key challenges faced when integrating AI models with Continuous Integration systems, as well as future directions and potential advancements in this field.
Challenges and Future Directions

Handling Large and Complex Models
The complexity and size of AI models, especially deep learning models, pose significant challenges in terms of computational resources and integration complexity.
Challenge: Managing the computational load and memory requirements of large models can be challenging, especially when trying to integrate these models into existing CI pipelines that are not originally designed to handle such loads.
Future Direction: Development of more efficient model architectures and compression techniques to reduce the size and computational demands of AI models without sacrificing performance.
Explainable AI and Interpretability
As AI models become more prevalent in critical applications, the need for explainability and interpretability grows. Stakeholders not only need to trust the model's outputs but also understand how decisions are made.
Challenge: Deep learning models, in particular, are often seen as "black boxes" due to their complex nature, making it difficult to interpret their decision-making processes.
Future Direction: Increased focus on developing techniques and tools for Explainable AI (XAI) that can make these models more transparent and understandable to users.
Ethical Considerations and Responsible AI
Integrating AI into CI systems must also consider ethical implications, ensuring that AI systems operate within accepted moral and ethical boundaries.
Challenge: Ensuring AI systems do not perpetuate biases or make unfair decisions, especially in sectors like recruitment, healthcare, and law enforcement.
Future Direction: Implementing and enforcing ethical guidelines and standards for AI development and deployment. This includes continuous monitoring and testing to detect and mitigate biases in AI models.
Case Studies and Examples
For incorporating AI models with continuous integration (CI) systems, it’s advantageous to see a concrete instance to comprehend how this can sleek your evolution process. Let’s know through a comprehensive case study using Jenkins, a popular CI/CD framework, to exhibit how you can effectively incorporate your AI models into a CI pipeline.
Setting the Stage: Your Project
Suppose you’re working on an AI project that indulges developing a model to forecast customer behavior based on their previous interactions with your website. Your aim is to constantly enhance the model based on new information and deploy updates without disturbing the user experience.
Integrating AI with Jenkins
Setting Up Jenkins: Initially, set up Jenkins on your server. You will need to install the significant plugins for your project, like Git for source control and Machine Learning plugin if you’re dealing with precise ML tasks.
Creating a Pipeline: Create a Jenkins pipeline that triggers every time you push new code to your Git storage. This pipeline should include steps such as data preprocessing, model training, assessment, and deployment.
Data Management: Ensure your information is attainable and correctly formatted for training. Jenkins can automatically pull the newest information from your repository solution (like AWS S3) before each training session.
Training the Model: Use Jenkins to enforce your training scripts. Configure your pipeline to use virtual environments or Docker containers to sustain reliability and ensure your training environment is constant.
Evaluating the Model: After training, assess your model’s performance using verification data. Set up norms for performance metrics (like precision or FI- score) that must be met before proceeding with deployment.
Automating Testing and Deployment: If the model meets the performance norms, automatically deploy it to your production environment. Jenkins can handle rolling updates to ensure there’s no interruption for your users.
Monitoring and Feedback: Once deployed, monitor the model’s performance in real-time. Jenkins can be configured to alert you if the model’s performance deteriorates, triggering a retraining cycle with new data.
Why Jenkins?
Using Jenkins for CI/CD in AI projects provides numerous benefits:
Automation: Automates the whole lifecycle of your model from data preprocessing to deployment.
Scalability: Easily scales with your project as it evolves in intricacy and information volume.
Consistency: Ensures that every version of the model is examined and deployed in the same manner.
By incorporating AI models with Jenkins, you sleek your productivity, reduce the threat of mistakes, and ensure that your models are always upgraded and ready for production. This approach not only saves time but also substantially enhances the dependability of your AI applications.
Real-world Examples of AI Model Integration with CI Systems
Tech Companies
Google: Google uses AI extensively in its CI/CD pipelines to enhance various products. For instance, Google Photos uses machine learning models to power features like image recognition and auto-tagging. These models are continuously updated and deployed using Google's robust CI/CD systems that handle billions of images.
Netflix: Netflix utilizes AI to refine and improve its recommendation algorithms continuously. The integration of these AI models into their CI/CD pipeline allows for rapid iteration and deployment, which helps Netflix tailor content to user preferences more effectively and maintain its competitive edge in streaming.
Facebook: At Facebook, AI models are integrated into a CI/CD framework to manage and improve the performance of various features like news feed algorithms and ad targeting. The company uses AI to analyze user interactions and automatically tests and rolls out updates to enhance user engagement.
Healthcare
PathAI: This company is using AI to assist pathologists in diagnosing and researching diseases more accurately and efficiently. PathAI integrates AI models into its CI/CD pipelines to ensure that the latest medical research and data can be continuously incorporated into their models, improving diagnostic accuracy.
Tempus: Tempus applies AI in precision medicine to personalize cancer treatments. Their CI/CD system ensures that AI models are frequently updated with the latest clinical data, helping to tailor treatments to individual patients based on genetic data and disease markers.
Lessons Learned and Best Practices from Industry
Continuous Monitoring: Companies like Google and Netflix have robust monitoring systems that continuously assess the performance of deployed AI models. This practice helps in quickly identifying and addressing issues that could affect user experience or system performance.
Staged Deployment: Facebook and other tech giants often use staged deployment strategies. By gradually rolling out updates, they can monitor the impact and effectiveness of new models under real-world conditions without fully committing all resources. This method helps mitigate risks and allows for adjustments before wider release.
These examples demonstrate the effectiveness of integrating AI models with CI/CD systems across various sectors. By adopting best practices such as continuous monitoring and staged deployment, companies can ensure that their AI-driven innovations are delivered efficiently and reliably, providing them with a strategic advantage in their respective fields.
As we've explored the integration of AI models into Continuous Integration (CI) systems, let's now focus on some key resources and further readings that can provide deeper insights and practical guidance for those interested in further exploring this field.
Conclusion
Integrating AI models into CI systems is a dynamic and evolving field that bridges the gap between traditional software development practices and the innovative capabilities of AI. As this technology continues to evolve, staying informed through credible resources, continuous learning, and community engagement will be key to leveraging its full potential.
The integration of AI and CI is not just about enhancing software development workflows but also about transforming how businesses operate and compete in an increasingly data-driven world. As we look forward, the potential for AI to revolutionize industries by making software development faster, more efficient, and increasingly automated is immense.
Whether you're a developer, a data scientist, or a business leader, understanding and implementing AI within CI systems can provide a competitive edge and drive significant advancements in your projects and products.
Subscribe to our newsletter to never miss an update
Other articles


Agents Talk, Models Think: A2A + MCP for Enterprise Agentic AI

Riya Parikh, Nitai Agarwal
Apr 27, 2025
Read the article
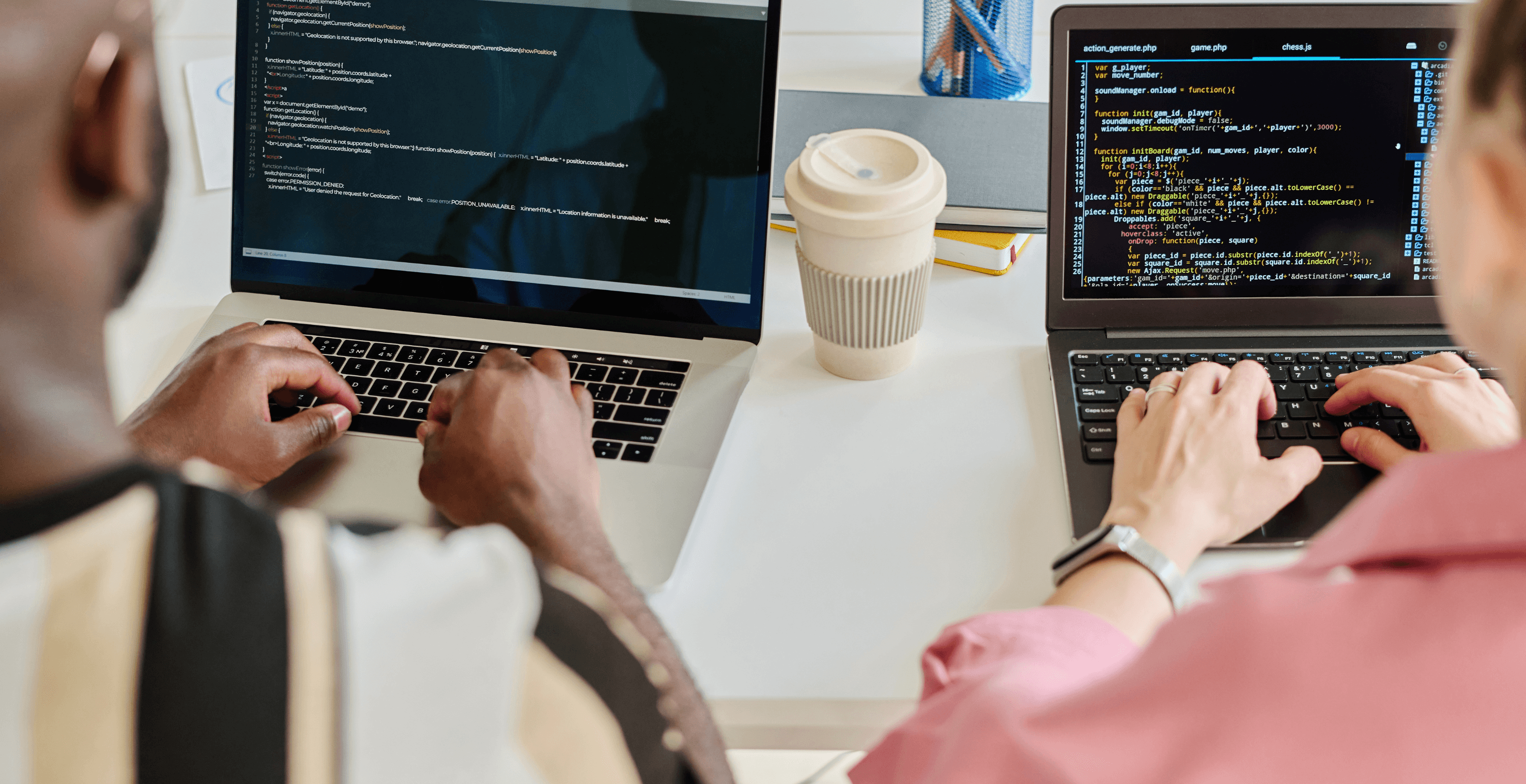
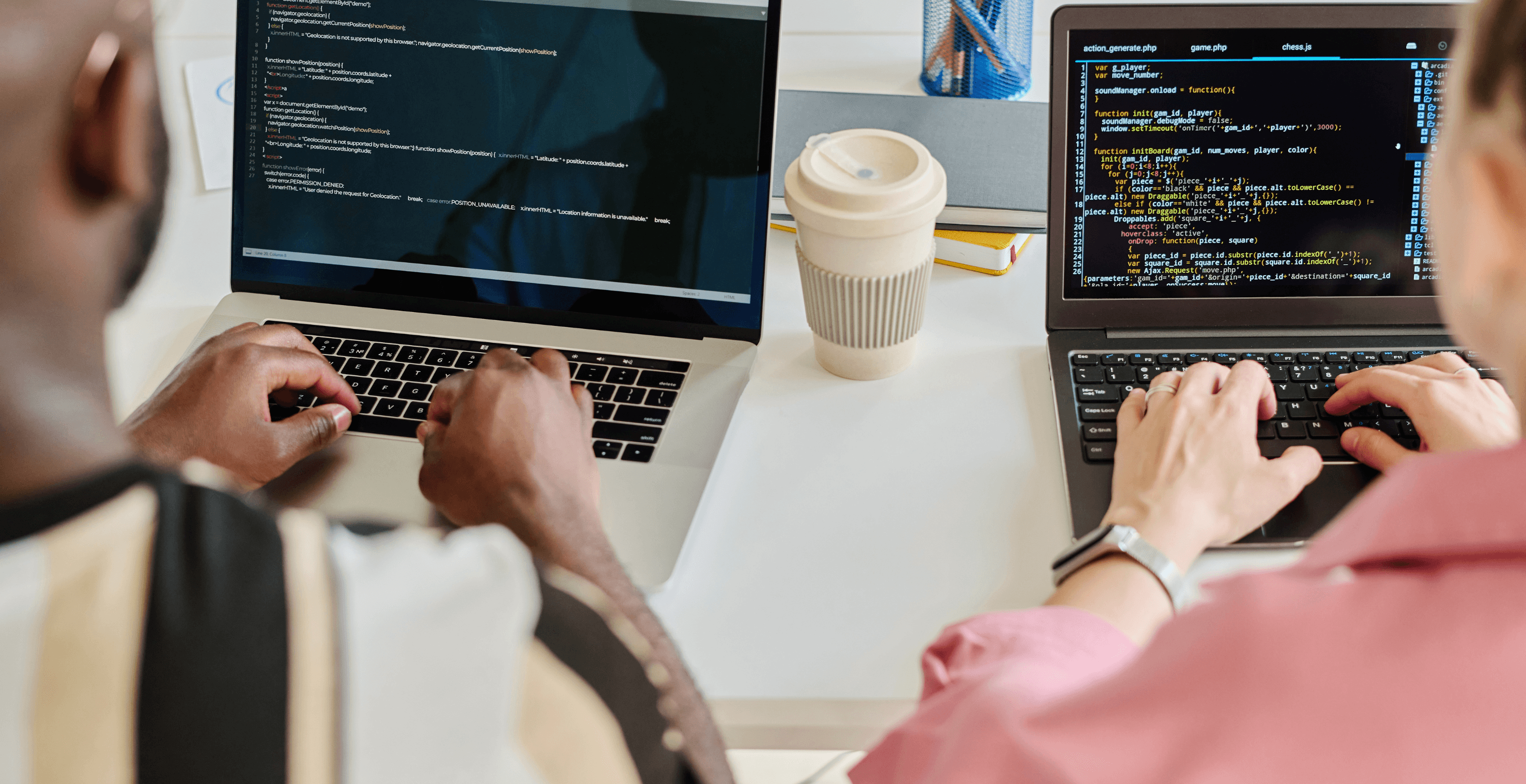
Understanding NeMo Guardrails: A Toolkit for LLM Security

Rehan Asif
Dec 24, 2024
Read the article


Understanding Differences in Large vs Small Language Models (LLM vs SLM)

Rehan Asif
Dec 21, 2024
Read the article