Exploring the Future of LLM and Generative AI Infrastructure
Rehan Asif
Jul 13, 2024
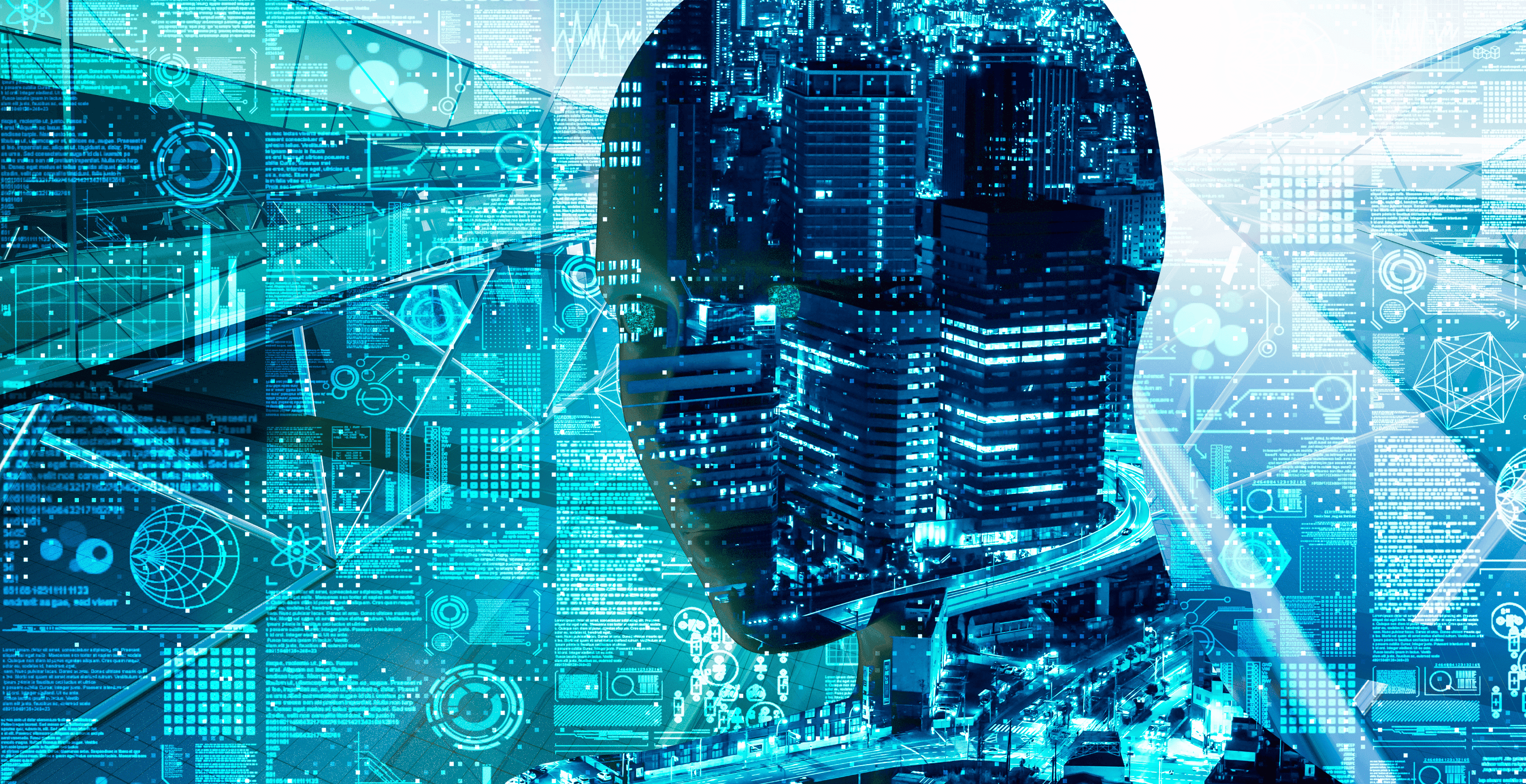
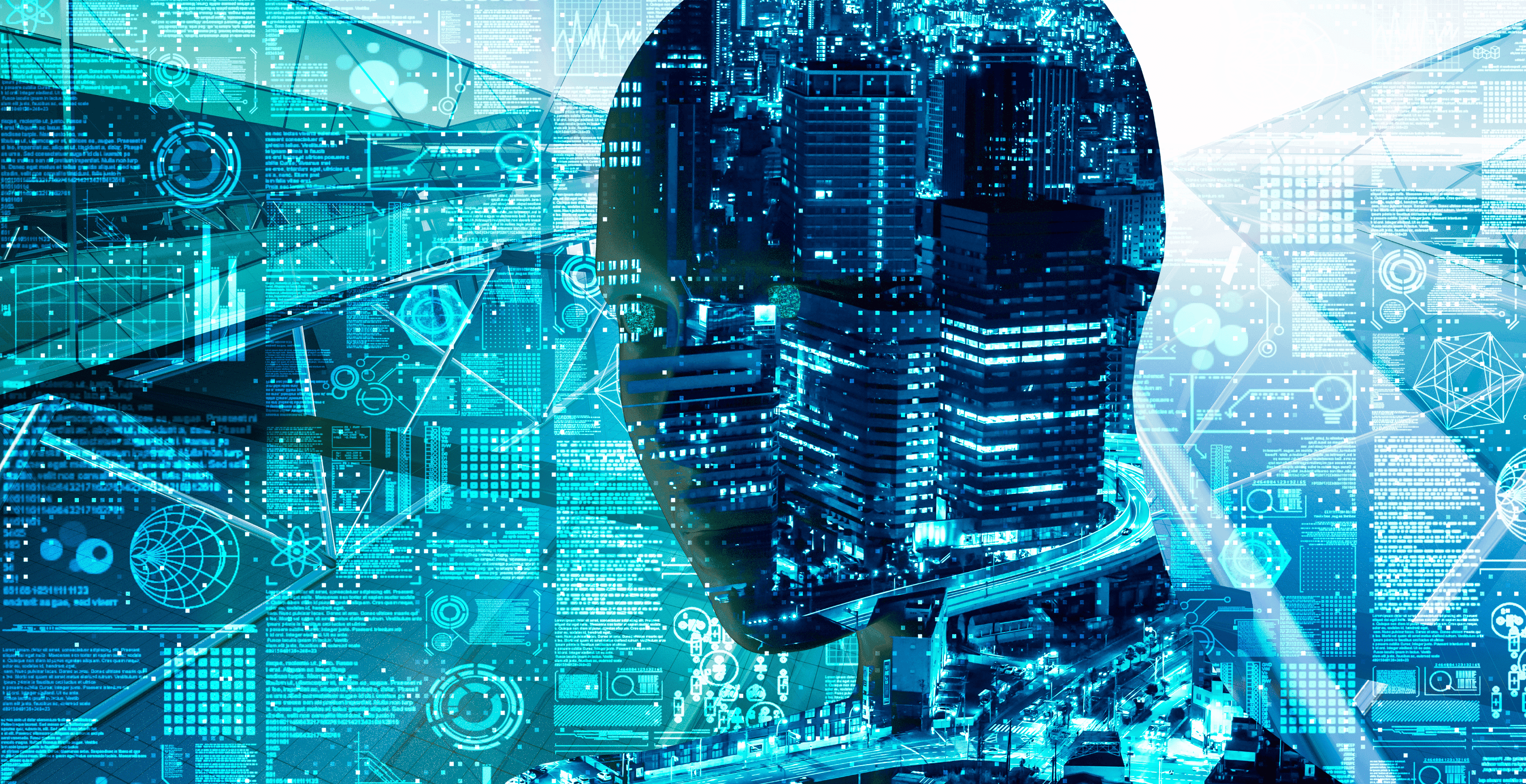
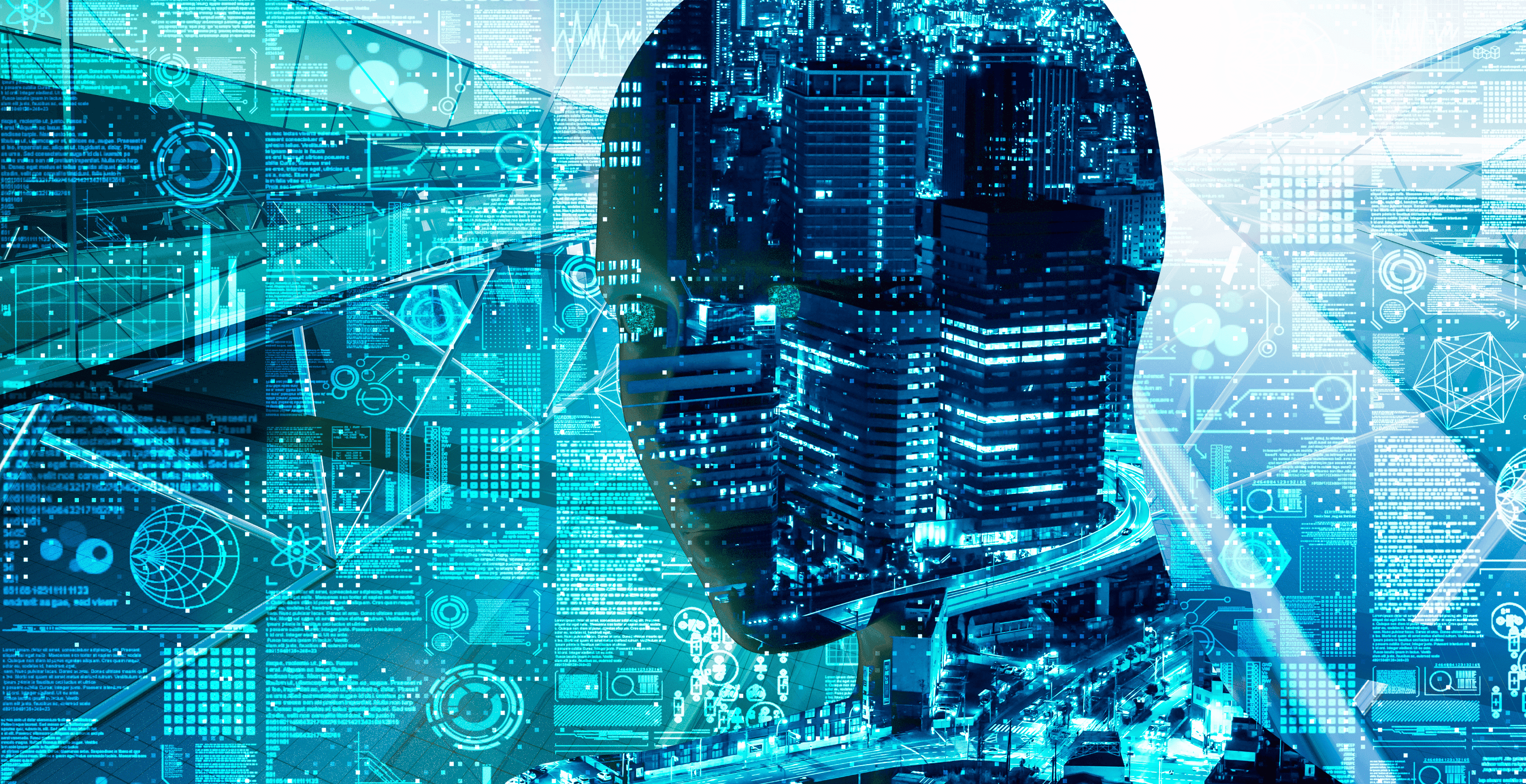
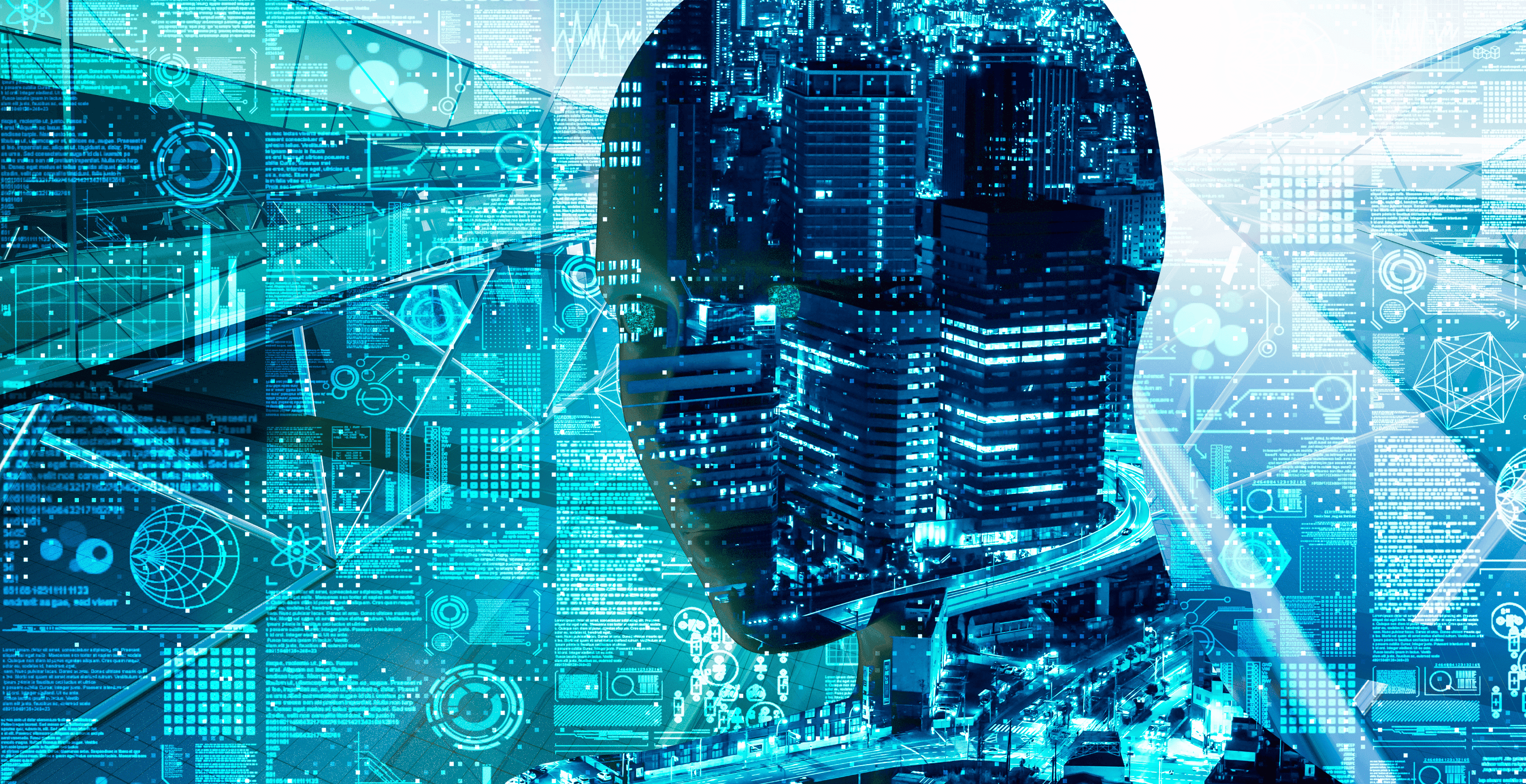
Who needs superheroes when you have Large Language Models (LLMs) and Generative AI reshaping the world?
To truly employ the power of Large Language Models (LLMs) and Generative AI, you need a sophisticated infrastructure. This infrastructure not only upholds the profound computational stipulations but also ensures smooth workflow and manageability. Over the years, experts have customized and transformed the infrastructure for these applications, playing a crucial role in intensifying AI technology.
The Role of Large Language Models in Modern Technology
Understanding and Replicating Human Speech with Large Language Models
Large Language Models (LLMs) are a type of artificial intelligence designed to comprehend and produce human language. Think of them as acutely advanced text mainframes that can comprehend context, pick up on nuances, and even produce text that sounds like it was written by a person. Researchers instruct these models on huge amounts of data, captivating the complexities of human speech motifs.
At their fundamental, LLMs operate by forecasting the next word in a sentence based on the context offered by the previous words. This might sound simple, but it’s an intricate task that involves comprehending grammar, context and even cultural references. The more information they are instructed on, the better they become at imitating human language, making them indispensable tools for numerous applications in modern technology.
Examples of LLM Applications in the Industry
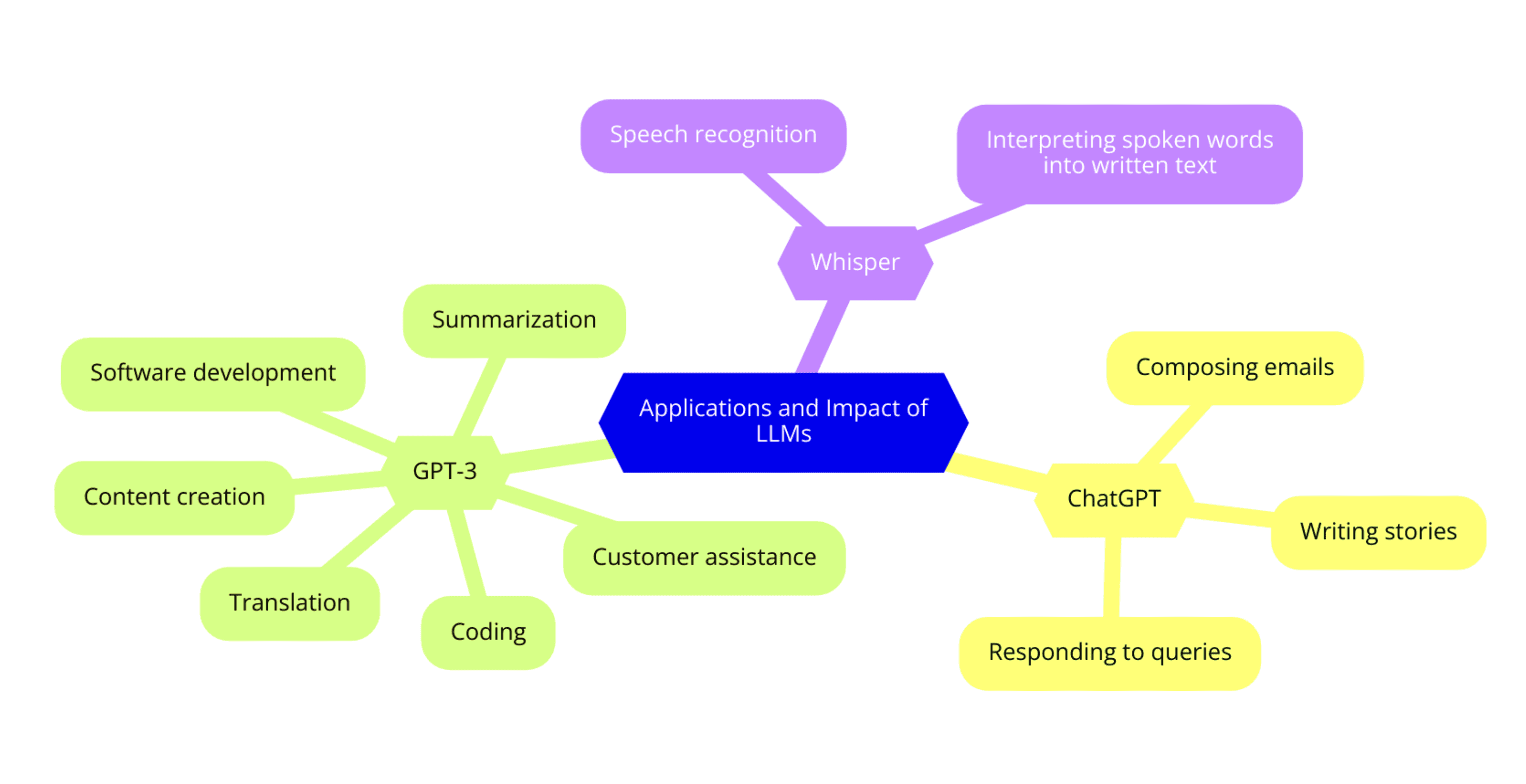
LLMs have made substantial progress in numerous industries, exhibiting their ingenuity and potential.
ChatGPT: A noticeable instance is OpenAI developed ChatGPT, an AI designed to help in producing human-like text based on the input it derives. Whether you need help composing an email, writing a story, or responding to queries, ChatGPT can handle it with imposing precision and smoothness.
GPT-3: Another renowned LLM is GPT-3, also by OpenAI. It’s one of most advanced language models accessible, able to execute enormous tasks like summarization, translation, and even coding. Its capability to produce coherent and contextually pertinent text has made it a groundbreaker in fields such as customer assistance, content creation and even software development.
Whisper: OpenAI’s Whisper is yet another intriguing application of LLM technology. Unlike ChatGPT and GPT-3, which concentrate on text, Developers created Whisper for speech recognition. It can interpret spoken words into written text with outstanding precision, making it certainly effective for applications like translation services, voice-activated assistants and more.
These instances emphasize how LLMs are transforming the way we communicate with technology, making tasks simpler, swifter and more effective. The probable applications are colossal, and we’re just starting to scratch the surface of what these significant models can achieve.
Want to know how to efficiently measure and assess the performance of large language models? Check out our comprehensive guide on Evaluating Large Language Models: Methods And Metrics.
Now, let's break down what makes this infrastructure so robust.
Generative AI and Its Infrastructure Needs
How Generative AI Uses LLM Outputs to Create Original Content
Generative AI uses the power of Large Language Models (LLMs) to create eccentric and original content. When you think about it, these models are like the brains behind Artificial Intelligence, refining enormous amounts of data to comprehend language motifs and nuances. Envision you are writing an article. Instead of you doing all the hefty lifting, an LLM dissects relative content, grasps from it, and produces a draft that imitates human writing.
This ability is critical because it permits AI to generate high-quality content, from blogs and fictions to even code and design components. The more information an LLM has, the better it becomes at producing pertinent and appealing content. So, when you see an Artificial Intelligence tool drizzling-out a near immaculate piece of writing, it’s all thanks to the broad training and information fed into these LLMs. It’s like having a private support who’s read every book, blog and article on the topic at hand.
The Generative AI Flywheel and the Necessity for Robust, Scalable Infrastructure
Now, let’s delve into the Generative AI Flywheel. This notion revolves around constant enhancement and innovation in Artificial Intelligence. Think of it as a cycle where Artificial Intelligence models grasp, produce, and receive feedback, and then process their yields based on that response. It’s an endless loop of improvement. You require a sturdy and ductile infrastructure for this flywheel to spin sleekly.
Why is this infrastructure so important? Envisage trying to run a marathon without apt training or apparatus. You wouldn’t get far, right? Likewise, generative AI requires significant hardware and software to refine vast amounts of information swiftly and effectively. Flexible infrastructure ensures that as your AI demands grow, your systems can handle the load without a hindrance.
When you handle generative AI, precision and innovation become unchangeable. Sturdy infrastructure supports these by giving the computational power required for deep learning and intricate data refining. Without it, your AI models would scuffle, resulting in slower refining times and less precise yields.
The success of generative AI articulates the sleek incorporation of LLM yields and the support of a strong infrastructure. By ensuring you have both, you’re setting the stage for AI that’s not only innovative but also dependable and effective.
Core Components of LLM and Generative AI Infrastructure
To truly comprehend LLM infrastructure, delve into its core components. These components are the foundation of any successful enforcement of LLM and generative AI technologies.
High-Performance Computing, Scalable Cloud Solutions, and Efficient Data Storage
Foremost, high-performance computing (HPC) is significant. You can’t run intricate models without significant computing resources. Think of HPC as the engine of your Artificial Intelligence system, handling all those profound computations at a rapid speed. This ensures that your models run sleekly and effectively.
Next, adaptable cloud solutions come into play. As you data cultivates, you need the adaptability to scale your operations without any gimmicks. Cloud solutions offer this scalability, permitting you to handle augmenting workloads sleekly. Plus, they provide the ease of sustaining your infrastructure distantly, saving you the hassle of physical maintenance.
Effective data storage and management are equally critical. You need a sturdy system to store enormous amounts of information safely and access it swiftly whenever needed. Apt data management ensures that your data is not only secure but also easily recoverable, which is crucial for training and fine-tuning your models.
Specialized Infrastructure Considerations
When you get into the particulars of LLM infrastructure, certain esoteric contemplations become pivotal. GPU-intensive workloads are one such aspect. GPUs (Graphics Processing Units) are specifically attired for the parallel refining tasks that AI model requires. They substantially boost the training process, permitting you to handle more information and intricate computations efficiently.
Containerization is another key element. Using containers allows you to bundle your applications and their reliabilities together, ensuring they run constantly across distinct computing environments. This makes deployment and gauging much easier and more dependable, as containers provide a structured unit of software.
An adaptable LLM infrastructure uses high-performance assessing, ductile cloud solutions, and effective data storage, adorned by specialized contemplations such as GPU workloads and containerization. By concentrating on these elements, you can build a sturdy foundation for your generative AI projects.
To delve deeper into how LLM parameters can be refined and upgraded, check out our comprehensive guide "A Brief Guide To LLM Parameters: Tuning and Optimization."
Evolving Trends in LLM and Generative AI Infrastructure
Innovations in Cloud Infrastructure Solutions
Cloud infrastructure is the backbone of LLM and Generative AI. Innovations in this space are groundbreakers. For example, instance startup optimizations are making a substantial distinction. You’re seeing swifter boot times and more effective resource allotment, which means your AI models can be up and running in no time.
Compute attainability is also enhanced. Cloud providers are rolling out more prominent and adaptable options, permitting you to scale your functioning smoothly. This adaptability ensures that you have the right amount of compute power precisely when you require it, improving the effectiveness and pace of your AI tasks.
Balancing Cost, Performance, and Reliability
In the scenario of LLM and Generative AI, locating the sweet spot between price, performance, and dependability is critical. You need to sustain expenditures without yielding on the performance of your models.
Arising solutions are helping accomplish this balance. For instance, new pricing models and resource management tools let you upgrade costs while maintaining high performance. You can now run profound AI tasks without breaking the bank, ensuring that your models perform steadfastly under several circumstances.
By concentrating on these developing trends, you’re setting a stage for the future where LLM and Generative AI can prosper, delivering incredible outcomes with optimal effectiveness.
Want to make your LLM development 3X faster? Comprehend how using the RagaAI LLM Hub can make your LLM development 3X faster, saving you time and resources.
Speaking of trends, let’s dive into the specific platforms that are addressing some of the biggest challenges in the field.
Addressing the Challenges Through Dedicated Platforms
Ray’s Contributions to Resolving Scalability and Complexity Challenges
When it comes to deploying foundation models, expandability and intricacy are two of the biggest obstacles. Ray has appeared as a groundbreaker in this arena. The designers created it to streamline the process of building and scaling AI applications. Imagine you're trying to fetch multiple tasks concurrently; Ray acts like an extra pair of hands, making it much simpler to sustain everything.
Ray’s dispensation enforcement structure allows you to break down intricate tasks into smaller, tractable units. This approach substantially reduces the intricacy of large-scale AI operations. Furthermore, it sustains a variety of machine learning libraries and structures, making it highly adaptable. By using Ray, you can effectively scale your AI applications across multiple tumors, ensuring they run sleekly even as they grow in size and heterogeneity.
Anyscale’s Seamless Integration for LLM APIs
Anyscale takes things a step further by providing a sleek incorporation of LLM APIs. Imagine you have a splendid kitchen but no chef; Anyscale steps in as the skilled chef, managing all the complex information so you can enjoy the final product. It concentrates on excluding the frustrations of infrastructure management, permitting you to focus on developing and deploying your models.
With Anyscale, you don’t have to worry about the fundamental infrastructure. Anyscale offers an instinctive platform that sustains everything from resource allotment to scaling. This means you can deploy your LLM APIs smoothly, without getting tangled down by the technical intricacies. By streamlining the infrastructure management process, Anyscale ensures that your concentration stays on innovation and development, rather than on sustaining and scaling the infrastructure.
Platforms such as Ray and Anyscale are important in conquering the difficulties linked with LLM and generative AI. They offer substantial tools and support to handle scalability and intricacy, enabling you to deploy sturdy AI models with ease.
So, how do all these components fit together to create a modern LLM infrastructure stack?
The Modern LLM Infrastructure Stack
Comprehending the components of the modern LLM infrastructure stack is significant for using the power of Generative AI. You need to contemplate numerous elements, from data management to deployment and monitoring, to build a sturdy system.
Components: Data Management, Deployment, and Monitoring
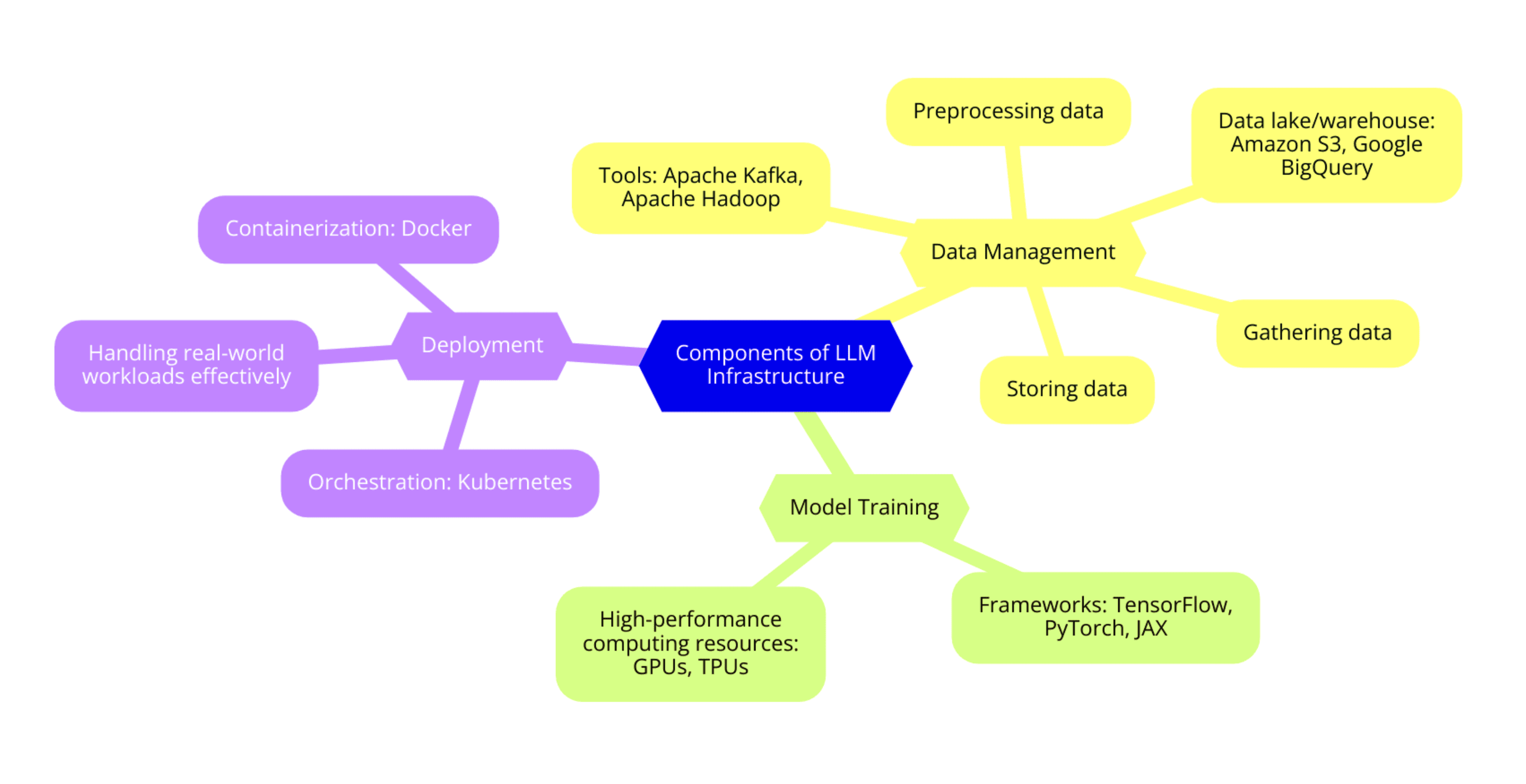
Data Management: The backbone of any LLM infrastructure is effective data management. This process involves gathering, storing, or preprocessing enormous amounts of information. Tools such as Apache Kafka and Apache Hadoop play pivotal roles here, enabling smooth data devouring and refining. You also need a sturdy data lake or warehouse, like Amazon S3 or Google BigQuery, to handle the scale of data needed for training LLMs.
Model Training: Once your information is in place, the next step is model training. You’ll use structures such as TensorFlow, PyTorch, or JAX to establish and instruct your models. These structures provide the adaptability and power significant to build intricate auditory networks. High-execution computing resources, indulging GPUs and TPUs, are also necessary for boosting the training process.
Deployment: Deploying your instructed models need a distinct set of tools and practices. Containerization technologies such as Docker and orchestration platforms such as Kubernetes are indispensable. They help in sustaining and gauging your applications, ensuring that your models can handle real-world workloads effectively.
But what about keeping your data safe and compliant while using these advanced tools?
Emerging Tools and Platforms
Staying ahead in the field of LLM infrastructure means keeping an eye on emerging tools and platforms that support distinct layers of the heap.
Data Management Tools
New platforms such as Delta Lake and Apache Iceberg are transforming data management. They offer ACID transactions and enable ductile data refining, making them ideal for handling the enormous datasets needed by LLMs.
Model Training Platforms
Platforms like Google AI Platform, Amazon SageMaker, and Microsoft Azure ML are simplifying the model training process. These sustained services provide everything from data preprocessing to model deployment, reducing the intricate and duration required to get your models into production.
Deployment and Monitoring Solutions
For deployment, solutions such as Kubernetes-based Kubeflow and TensorFlow Serving are obtaining eminence. They streamline the deployment process and integrate smoothly with other cloud-native tools. For monitoring, innovative tools such as New Relic and Datadog provide thorough luminosity helping you sustain maximum performance.
Security and Governance in LLM and Generative AI Applications
Security and Governance are uppermost when dealing with LLMs and Generative AI applications. Ensuring data seclusion, governance and compliance is an important challenge, but numerous solutions are arising. Those challenges are:
Data Privacy and Governance
To acknowledge data seclusion, you must enforce strict data governance strategies. Tools such as Apache Ranger and Privacera can help execute these policies, ensuring that sensitive information is handled precisely. In addition, compliance with regulations like GDPR and CCPA is significant, and solutions such as TrustArc and OneTrust can assist in accomplishing this docility.
Innovative Security Approaches
Innovative approaches to LLM applications security include using Private IPs and advanced cloud security contraptions. Private IPs limit access to your models, adding an auxiliary layer of security. Cloud providers such as AWS, Azure and Google Cloud provide numerous security attributes, including identity and access management (IAM), encryption, and network security which are important for safeguarding your AI applications.
Now that we've covered security and compliance, let's wrap up by looking at the future of LLM and Generative AI infrastructure.
Looking to delve deeper into AI technologies? Check out our pragmatic article on Building Low-Code LLM Apps with Visual Programming to sleek your development process.
Conclusion
Advanced infrastructure is critical to unleashing the full potential of LLM and Generative AI technologies. As these technologies progresses, so too must the infrastructure that supports them. Constant innovation and adjustment are key to staying ahead and meeting future requirements.
Who needs superheroes when you have Large Language Models (LLMs) and Generative AI reshaping the world?
To truly employ the power of Large Language Models (LLMs) and Generative AI, you need a sophisticated infrastructure. This infrastructure not only upholds the profound computational stipulations but also ensures smooth workflow and manageability. Over the years, experts have customized and transformed the infrastructure for these applications, playing a crucial role in intensifying AI technology.
The Role of Large Language Models in Modern Technology
Understanding and Replicating Human Speech with Large Language Models
Large Language Models (LLMs) are a type of artificial intelligence designed to comprehend and produce human language. Think of them as acutely advanced text mainframes that can comprehend context, pick up on nuances, and even produce text that sounds like it was written by a person. Researchers instruct these models on huge amounts of data, captivating the complexities of human speech motifs.
At their fundamental, LLMs operate by forecasting the next word in a sentence based on the context offered by the previous words. This might sound simple, but it’s an intricate task that involves comprehending grammar, context and even cultural references. The more information they are instructed on, the better they become at imitating human language, making them indispensable tools for numerous applications in modern technology.
Examples of LLM Applications in the Industry
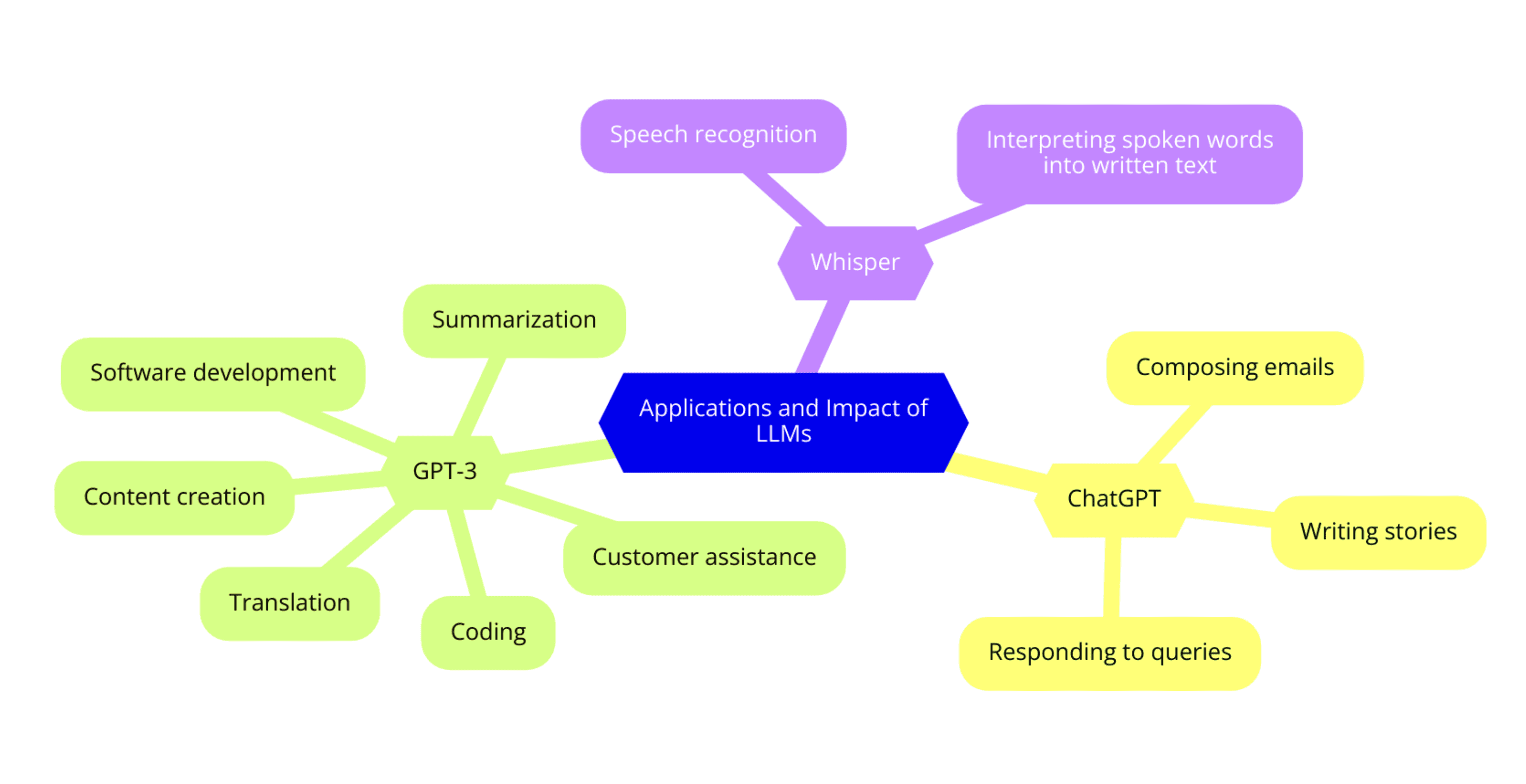
LLMs have made substantial progress in numerous industries, exhibiting their ingenuity and potential.
ChatGPT: A noticeable instance is OpenAI developed ChatGPT, an AI designed to help in producing human-like text based on the input it derives. Whether you need help composing an email, writing a story, or responding to queries, ChatGPT can handle it with imposing precision and smoothness.
GPT-3: Another renowned LLM is GPT-3, also by OpenAI. It’s one of most advanced language models accessible, able to execute enormous tasks like summarization, translation, and even coding. Its capability to produce coherent and contextually pertinent text has made it a groundbreaker in fields such as customer assistance, content creation and even software development.
Whisper: OpenAI’s Whisper is yet another intriguing application of LLM technology. Unlike ChatGPT and GPT-3, which concentrate on text, Developers created Whisper for speech recognition. It can interpret spoken words into written text with outstanding precision, making it certainly effective for applications like translation services, voice-activated assistants and more.
These instances emphasize how LLMs are transforming the way we communicate with technology, making tasks simpler, swifter and more effective. The probable applications are colossal, and we’re just starting to scratch the surface of what these significant models can achieve.
Want to know how to efficiently measure and assess the performance of large language models? Check out our comprehensive guide on Evaluating Large Language Models: Methods And Metrics.
Now, let's break down what makes this infrastructure so robust.
Generative AI and Its Infrastructure Needs
How Generative AI Uses LLM Outputs to Create Original Content
Generative AI uses the power of Large Language Models (LLMs) to create eccentric and original content. When you think about it, these models are like the brains behind Artificial Intelligence, refining enormous amounts of data to comprehend language motifs and nuances. Envision you are writing an article. Instead of you doing all the hefty lifting, an LLM dissects relative content, grasps from it, and produces a draft that imitates human writing.
This ability is critical because it permits AI to generate high-quality content, from blogs and fictions to even code and design components. The more information an LLM has, the better it becomes at producing pertinent and appealing content. So, when you see an Artificial Intelligence tool drizzling-out a near immaculate piece of writing, it’s all thanks to the broad training and information fed into these LLMs. It’s like having a private support who’s read every book, blog and article on the topic at hand.
The Generative AI Flywheel and the Necessity for Robust, Scalable Infrastructure
Now, let’s delve into the Generative AI Flywheel. This notion revolves around constant enhancement and innovation in Artificial Intelligence. Think of it as a cycle where Artificial Intelligence models grasp, produce, and receive feedback, and then process their yields based on that response. It’s an endless loop of improvement. You require a sturdy and ductile infrastructure for this flywheel to spin sleekly.
Why is this infrastructure so important? Envisage trying to run a marathon without apt training or apparatus. You wouldn’t get far, right? Likewise, generative AI requires significant hardware and software to refine vast amounts of information swiftly and effectively. Flexible infrastructure ensures that as your AI demands grow, your systems can handle the load without a hindrance.
When you handle generative AI, precision and innovation become unchangeable. Sturdy infrastructure supports these by giving the computational power required for deep learning and intricate data refining. Without it, your AI models would scuffle, resulting in slower refining times and less precise yields.
The success of generative AI articulates the sleek incorporation of LLM yields and the support of a strong infrastructure. By ensuring you have both, you’re setting the stage for AI that’s not only innovative but also dependable and effective.
Core Components of LLM and Generative AI Infrastructure
To truly comprehend LLM infrastructure, delve into its core components. These components are the foundation of any successful enforcement of LLM and generative AI technologies.
High-Performance Computing, Scalable Cloud Solutions, and Efficient Data Storage
Foremost, high-performance computing (HPC) is significant. You can’t run intricate models without significant computing resources. Think of HPC as the engine of your Artificial Intelligence system, handling all those profound computations at a rapid speed. This ensures that your models run sleekly and effectively.
Next, adaptable cloud solutions come into play. As you data cultivates, you need the adaptability to scale your operations without any gimmicks. Cloud solutions offer this scalability, permitting you to handle augmenting workloads sleekly. Plus, they provide the ease of sustaining your infrastructure distantly, saving you the hassle of physical maintenance.
Effective data storage and management are equally critical. You need a sturdy system to store enormous amounts of information safely and access it swiftly whenever needed. Apt data management ensures that your data is not only secure but also easily recoverable, which is crucial for training and fine-tuning your models.
Specialized Infrastructure Considerations
When you get into the particulars of LLM infrastructure, certain esoteric contemplations become pivotal. GPU-intensive workloads are one such aspect. GPUs (Graphics Processing Units) are specifically attired for the parallel refining tasks that AI model requires. They substantially boost the training process, permitting you to handle more information and intricate computations efficiently.
Containerization is another key element. Using containers allows you to bundle your applications and their reliabilities together, ensuring they run constantly across distinct computing environments. This makes deployment and gauging much easier and more dependable, as containers provide a structured unit of software.
An adaptable LLM infrastructure uses high-performance assessing, ductile cloud solutions, and effective data storage, adorned by specialized contemplations such as GPU workloads and containerization. By concentrating on these elements, you can build a sturdy foundation for your generative AI projects.
To delve deeper into how LLM parameters can be refined and upgraded, check out our comprehensive guide "A Brief Guide To LLM Parameters: Tuning and Optimization."
Evolving Trends in LLM and Generative AI Infrastructure
Innovations in Cloud Infrastructure Solutions
Cloud infrastructure is the backbone of LLM and Generative AI. Innovations in this space are groundbreakers. For example, instance startup optimizations are making a substantial distinction. You’re seeing swifter boot times and more effective resource allotment, which means your AI models can be up and running in no time.
Compute attainability is also enhanced. Cloud providers are rolling out more prominent and adaptable options, permitting you to scale your functioning smoothly. This adaptability ensures that you have the right amount of compute power precisely when you require it, improving the effectiveness and pace of your AI tasks.
Balancing Cost, Performance, and Reliability
In the scenario of LLM and Generative AI, locating the sweet spot between price, performance, and dependability is critical. You need to sustain expenditures without yielding on the performance of your models.
Arising solutions are helping accomplish this balance. For instance, new pricing models and resource management tools let you upgrade costs while maintaining high performance. You can now run profound AI tasks without breaking the bank, ensuring that your models perform steadfastly under several circumstances.
By concentrating on these developing trends, you’re setting a stage for the future where LLM and Generative AI can prosper, delivering incredible outcomes with optimal effectiveness.
Want to make your LLM development 3X faster? Comprehend how using the RagaAI LLM Hub can make your LLM development 3X faster, saving you time and resources.
Speaking of trends, let’s dive into the specific platforms that are addressing some of the biggest challenges in the field.
Addressing the Challenges Through Dedicated Platforms
Ray’s Contributions to Resolving Scalability and Complexity Challenges
When it comes to deploying foundation models, expandability and intricacy are two of the biggest obstacles. Ray has appeared as a groundbreaker in this arena. The designers created it to streamline the process of building and scaling AI applications. Imagine you're trying to fetch multiple tasks concurrently; Ray acts like an extra pair of hands, making it much simpler to sustain everything.
Ray’s dispensation enforcement structure allows you to break down intricate tasks into smaller, tractable units. This approach substantially reduces the intricacy of large-scale AI operations. Furthermore, it sustains a variety of machine learning libraries and structures, making it highly adaptable. By using Ray, you can effectively scale your AI applications across multiple tumors, ensuring they run sleekly even as they grow in size and heterogeneity.
Anyscale’s Seamless Integration for LLM APIs
Anyscale takes things a step further by providing a sleek incorporation of LLM APIs. Imagine you have a splendid kitchen but no chef; Anyscale steps in as the skilled chef, managing all the complex information so you can enjoy the final product. It concentrates on excluding the frustrations of infrastructure management, permitting you to focus on developing and deploying your models.
With Anyscale, you don’t have to worry about the fundamental infrastructure. Anyscale offers an instinctive platform that sustains everything from resource allotment to scaling. This means you can deploy your LLM APIs smoothly, without getting tangled down by the technical intricacies. By streamlining the infrastructure management process, Anyscale ensures that your concentration stays on innovation and development, rather than on sustaining and scaling the infrastructure.
Platforms such as Ray and Anyscale are important in conquering the difficulties linked with LLM and generative AI. They offer substantial tools and support to handle scalability and intricacy, enabling you to deploy sturdy AI models with ease.
So, how do all these components fit together to create a modern LLM infrastructure stack?
The Modern LLM Infrastructure Stack
Comprehending the components of the modern LLM infrastructure stack is significant for using the power of Generative AI. You need to contemplate numerous elements, from data management to deployment and monitoring, to build a sturdy system.
Components: Data Management, Deployment, and Monitoring
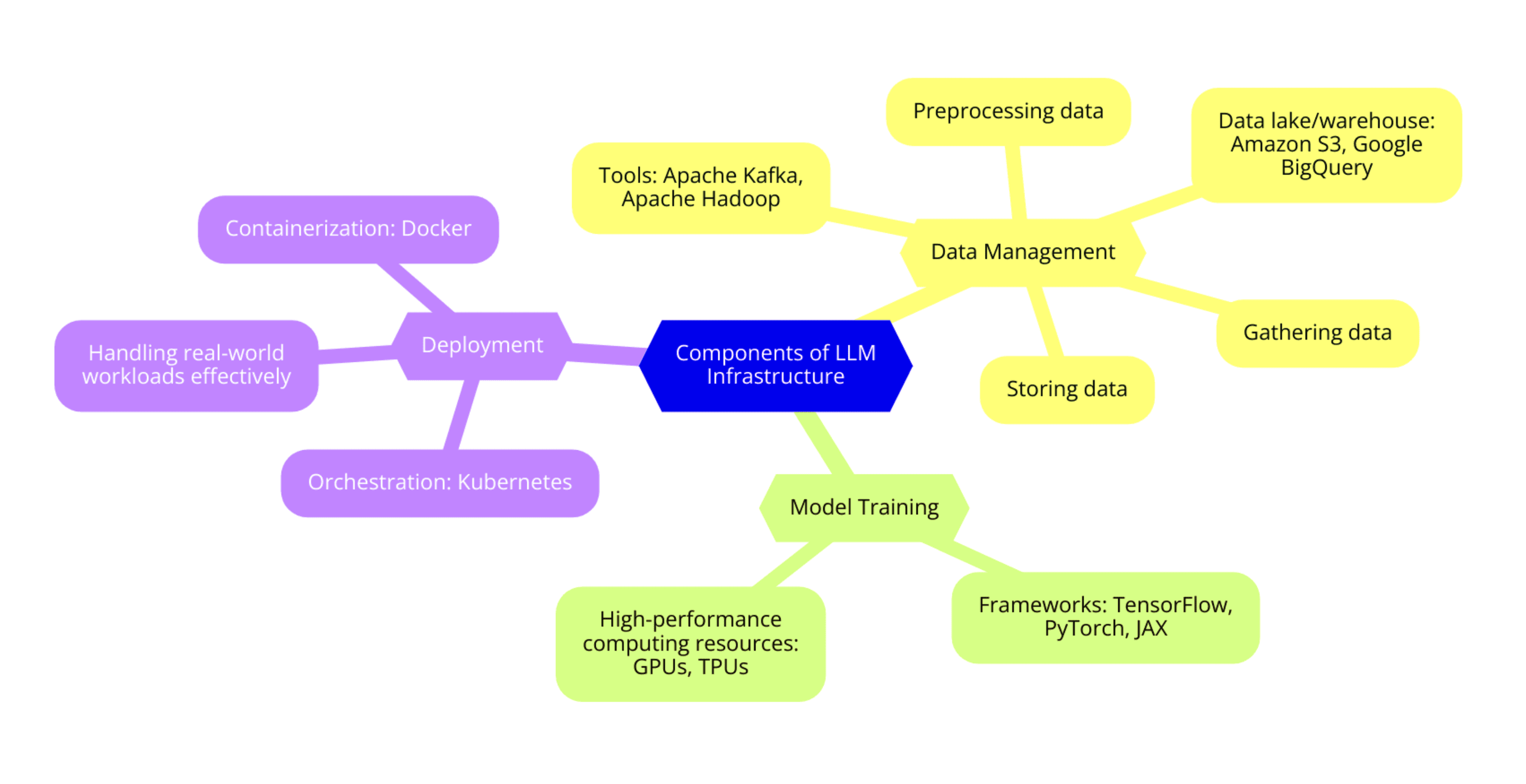
Data Management: The backbone of any LLM infrastructure is effective data management. This process involves gathering, storing, or preprocessing enormous amounts of information. Tools such as Apache Kafka and Apache Hadoop play pivotal roles here, enabling smooth data devouring and refining. You also need a sturdy data lake or warehouse, like Amazon S3 or Google BigQuery, to handle the scale of data needed for training LLMs.
Model Training: Once your information is in place, the next step is model training. You’ll use structures such as TensorFlow, PyTorch, or JAX to establish and instruct your models. These structures provide the adaptability and power significant to build intricate auditory networks. High-execution computing resources, indulging GPUs and TPUs, are also necessary for boosting the training process.
Deployment: Deploying your instructed models need a distinct set of tools and practices. Containerization technologies such as Docker and orchestration platforms such as Kubernetes are indispensable. They help in sustaining and gauging your applications, ensuring that your models can handle real-world workloads effectively.
But what about keeping your data safe and compliant while using these advanced tools?
Emerging Tools and Platforms
Staying ahead in the field of LLM infrastructure means keeping an eye on emerging tools and platforms that support distinct layers of the heap.
Data Management Tools
New platforms such as Delta Lake and Apache Iceberg are transforming data management. They offer ACID transactions and enable ductile data refining, making them ideal for handling the enormous datasets needed by LLMs.
Model Training Platforms
Platforms like Google AI Platform, Amazon SageMaker, and Microsoft Azure ML are simplifying the model training process. These sustained services provide everything from data preprocessing to model deployment, reducing the intricate and duration required to get your models into production.
Deployment and Monitoring Solutions
For deployment, solutions such as Kubernetes-based Kubeflow and TensorFlow Serving are obtaining eminence. They streamline the deployment process and integrate smoothly with other cloud-native tools. For monitoring, innovative tools such as New Relic and Datadog provide thorough luminosity helping you sustain maximum performance.
Security and Governance in LLM and Generative AI Applications
Security and Governance are uppermost when dealing with LLMs and Generative AI applications. Ensuring data seclusion, governance and compliance is an important challenge, but numerous solutions are arising. Those challenges are:
Data Privacy and Governance
To acknowledge data seclusion, you must enforce strict data governance strategies. Tools such as Apache Ranger and Privacera can help execute these policies, ensuring that sensitive information is handled precisely. In addition, compliance with regulations like GDPR and CCPA is significant, and solutions such as TrustArc and OneTrust can assist in accomplishing this docility.
Innovative Security Approaches
Innovative approaches to LLM applications security include using Private IPs and advanced cloud security contraptions. Private IPs limit access to your models, adding an auxiliary layer of security. Cloud providers such as AWS, Azure and Google Cloud provide numerous security attributes, including identity and access management (IAM), encryption, and network security which are important for safeguarding your AI applications.
Now that we've covered security and compliance, let's wrap up by looking at the future of LLM and Generative AI infrastructure.
Looking to delve deeper into AI technologies? Check out our pragmatic article on Building Low-Code LLM Apps with Visual Programming to sleek your development process.
Conclusion
Advanced infrastructure is critical to unleashing the full potential of LLM and Generative AI technologies. As these technologies progresses, so too must the infrastructure that supports them. Constant innovation and adjustment are key to staying ahead and meeting future requirements.
Who needs superheroes when you have Large Language Models (LLMs) and Generative AI reshaping the world?
To truly employ the power of Large Language Models (LLMs) and Generative AI, you need a sophisticated infrastructure. This infrastructure not only upholds the profound computational stipulations but also ensures smooth workflow and manageability. Over the years, experts have customized and transformed the infrastructure for these applications, playing a crucial role in intensifying AI technology.
The Role of Large Language Models in Modern Technology
Understanding and Replicating Human Speech with Large Language Models
Large Language Models (LLMs) are a type of artificial intelligence designed to comprehend and produce human language. Think of them as acutely advanced text mainframes that can comprehend context, pick up on nuances, and even produce text that sounds like it was written by a person. Researchers instruct these models on huge amounts of data, captivating the complexities of human speech motifs.
At their fundamental, LLMs operate by forecasting the next word in a sentence based on the context offered by the previous words. This might sound simple, but it’s an intricate task that involves comprehending grammar, context and even cultural references. The more information they are instructed on, the better they become at imitating human language, making them indispensable tools for numerous applications in modern technology.
Examples of LLM Applications in the Industry
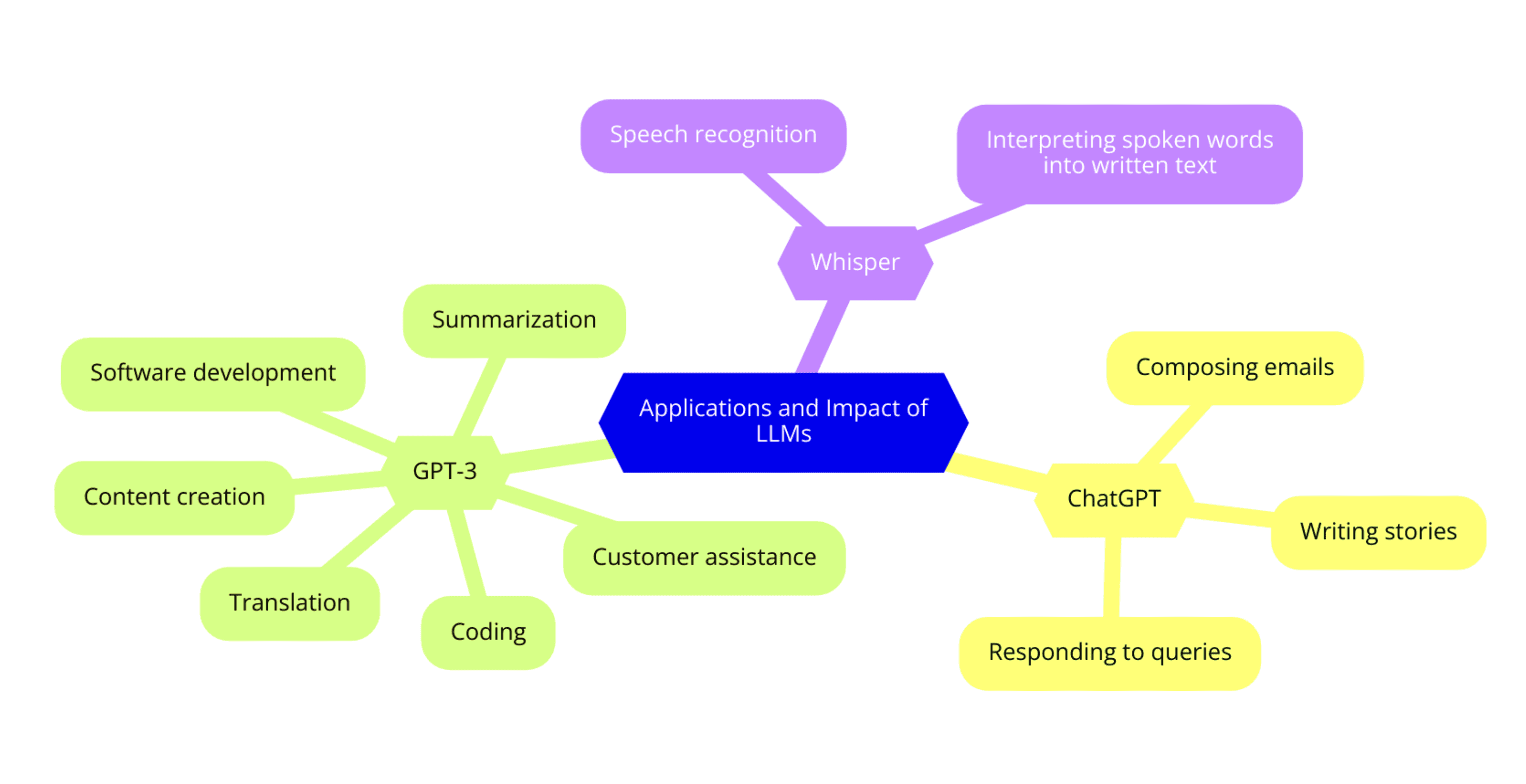
LLMs have made substantial progress in numerous industries, exhibiting their ingenuity and potential.
ChatGPT: A noticeable instance is OpenAI developed ChatGPT, an AI designed to help in producing human-like text based on the input it derives. Whether you need help composing an email, writing a story, or responding to queries, ChatGPT can handle it with imposing precision and smoothness.
GPT-3: Another renowned LLM is GPT-3, also by OpenAI. It’s one of most advanced language models accessible, able to execute enormous tasks like summarization, translation, and even coding. Its capability to produce coherent and contextually pertinent text has made it a groundbreaker in fields such as customer assistance, content creation and even software development.
Whisper: OpenAI’s Whisper is yet another intriguing application of LLM technology. Unlike ChatGPT and GPT-3, which concentrate on text, Developers created Whisper for speech recognition. It can interpret spoken words into written text with outstanding precision, making it certainly effective for applications like translation services, voice-activated assistants and more.
These instances emphasize how LLMs are transforming the way we communicate with technology, making tasks simpler, swifter and more effective. The probable applications are colossal, and we’re just starting to scratch the surface of what these significant models can achieve.
Want to know how to efficiently measure and assess the performance of large language models? Check out our comprehensive guide on Evaluating Large Language Models: Methods And Metrics.
Now, let's break down what makes this infrastructure so robust.
Generative AI and Its Infrastructure Needs
How Generative AI Uses LLM Outputs to Create Original Content
Generative AI uses the power of Large Language Models (LLMs) to create eccentric and original content. When you think about it, these models are like the brains behind Artificial Intelligence, refining enormous amounts of data to comprehend language motifs and nuances. Envision you are writing an article. Instead of you doing all the hefty lifting, an LLM dissects relative content, grasps from it, and produces a draft that imitates human writing.
This ability is critical because it permits AI to generate high-quality content, from blogs and fictions to even code and design components. The more information an LLM has, the better it becomes at producing pertinent and appealing content. So, when you see an Artificial Intelligence tool drizzling-out a near immaculate piece of writing, it’s all thanks to the broad training and information fed into these LLMs. It’s like having a private support who’s read every book, blog and article on the topic at hand.
The Generative AI Flywheel and the Necessity for Robust, Scalable Infrastructure
Now, let’s delve into the Generative AI Flywheel. This notion revolves around constant enhancement and innovation in Artificial Intelligence. Think of it as a cycle where Artificial Intelligence models grasp, produce, and receive feedback, and then process their yields based on that response. It’s an endless loop of improvement. You require a sturdy and ductile infrastructure for this flywheel to spin sleekly.
Why is this infrastructure so important? Envisage trying to run a marathon without apt training or apparatus. You wouldn’t get far, right? Likewise, generative AI requires significant hardware and software to refine vast amounts of information swiftly and effectively. Flexible infrastructure ensures that as your AI demands grow, your systems can handle the load without a hindrance.
When you handle generative AI, precision and innovation become unchangeable. Sturdy infrastructure supports these by giving the computational power required for deep learning and intricate data refining. Without it, your AI models would scuffle, resulting in slower refining times and less precise yields.
The success of generative AI articulates the sleek incorporation of LLM yields and the support of a strong infrastructure. By ensuring you have both, you’re setting the stage for AI that’s not only innovative but also dependable and effective.
Core Components of LLM and Generative AI Infrastructure
To truly comprehend LLM infrastructure, delve into its core components. These components are the foundation of any successful enforcement of LLM and generative AI technologies.
High-Performance Computing, Scalable Cloud Solutions, and Efficient Data Storage
Foremost, high-performance computing (HPC) is significant. You can’t run intricate models without significant computing resources. Think of HPC as the engine of your Artificial Intelligence system, handling all those profound computations at a rapid speed. This ensures that your models run sleekly and effectively.
Next, adaptable cloud solutions come into play. As you data cultivates, you need the adaptability to scale your operations without any gimmicks. Cloud solutions offer this scalability, permitting you to handle augmenting workloads sleekly. Plus, they provide the ease of sustaining your infrastructure distantly, saving you the hassle of physical maintenance.
Effective data storage and management are equally critical. You need a sturdy system to store enormous amounts of information safely and access it swiftly whenever needed. Apt data management ensures that your data is not only secure but also easily recoverable, which is crucial for training and fine-tuning your models.
Specialized Infrastructure Considerations
When you get into the particulars of LLM infrastructure, certain esoteric contemplations become pivotal. GPU-intensive workloads are one such aspect. GPUs (Graphics Processing Units) are specifically attired for the parallel refining tasks that AI model requires. They substantially boost the training process, permitting you to handle more information and intricate computations efficiently.
Containerization is another key element. Using containers allows you to bundle your applications and their reliabilities together, ensuring they run constantly across distinct computing environments. This makes deployment and gauging much easier and more dependable, as containers provide a structured unit of software.
An adaptable LLM infrastructure uses high-performance assessing, ductile cloud solutions, and effective data storage, adorned by specialized contemplations such as GPU workloads and containerization. By concentrating on these elements, you can build a sturdy foundation for your generative AI projects.
To delve deeper into how LLM parameters can be refined and upgraded, check out our comprehensive guide "A Brief Guide To LLM Parameters: Tuning and Optimization."
Evolving Trends in LLM and Generative AI Infrastructure
Innovations in Cloud Infrastructure Solutions
Cloud infrastructure is the backbone of LLM and Generative AI. Innovations in this space are groundbreakers. For example, instance startup optimizations are making a substantial distinction. You’re seeing swifter boot times and more effective resource allotment, which means your AI models can be up and running in no time.
Compute attainability is also enhanced. Cloud providers are rolling out more prominent and adaptable options, permitting you to scale your functioning smoothly. This adaptability ensures that you have the right amount of compute power precisely when you require it, improving the effectiveness and pace of your AI tasks.
Balancing Cost, Performance, and Reliability
In the scenario of LLM and Generative AI, locating the sweet spot between price, performance, and dependability is critical. You need to sustain expenditures without yielding on the performance of your models.
Arising solutions are helping accomplish this balance. For instance, new pricing models and resource management tools let you upgrade costs while maintaining high performance. You can now run profound AI tasks without breaking the bank, ensuring that your models perform steadfastly under several circumstances.
By concentrating on these developing trends, you’re setting a stage for the future where LLM and Generative AI can prosper, delivering incredible outcomes with optimal effectiveness.
Want to make your LLM development 3X faster? Comprehend how using the RagaAI LLM Hub can make your LLM development 3X faster, saving you time and resources.
Speaking of trends, let’s dive into the specific platforms that are addressing some of the biggest challenges in the field.
Addressing the Challenges Through Dedicated Platforms
Ray’s Contributions to Resolving Scalability and Complexity Challenges
When it comes to deploying foundation models, expandability and intricacy are two of the biggest obstacles. Ray has appeared as a groundbreaker in this arena. The designers created it to streamline the process of building and scaling AI applications. Imagine you're trying to fetch multiple tasks concurrently; Ray acts like an extra pair of hands, making it much simpler to sustain everything.
Ray’s dispensation enforcement structure allows you to break down intricate tasks into smaller, tractable units. This approach substantially reduces the intricacy of large-scale AI operations. Furthermore, it sustains a variety of machine learning libraries and structures, making it highly adaptable. By using Ray, you can effectively scale your AI applications across multiple tumors, ensuring they run sleekly even as they grow in size and heterogeneity.
Anyscale’s Seamless Integration for LLM APIs
Anyscale takes things a step further by providing a sleek incorporation of LLM APIs. Imagine you have a splendid kitchen but no chef; Anyscale steps in as the skilled chef, managing all the complex information so you can enjoy the final product. It concentrates on excluding the frustrations of infrastructure management, permitting you to focus on developing and deploying your models.
With Anyscale, you don’t have to worry about the fundamental infrastructure. Anyscale offers an instinctive platform that sustains everything from resource allotment to scaling. This means you can deploy your LLM APIs smoothly, without getting tangled down by the technical intricacies. By streamlining the infrastructure management process, Anyscale ensures that your concentration stays on innovation and development, rather than on sustaining and scaling the infrastructure.
Platforms such as Ray and Anyscale are important in conquering the difficulties linked with LLM and generative AI. They offer substantial tools and support to handle scalability and intricacy, enabling you to deploy sturdy AI models with ease.
So, how do all these components fit together to create a modern LLM infrastructure stack?
The Modern LLM Infrastructure Stack
Comprehending the components of the modern LLM infrastructure stack is significant for using the power of Generative AI. You need to contemplate numerous elements, from data management to deployment and monitoring, to build a sturdy system.
Components: Data Management, Deployment, and Monitoring
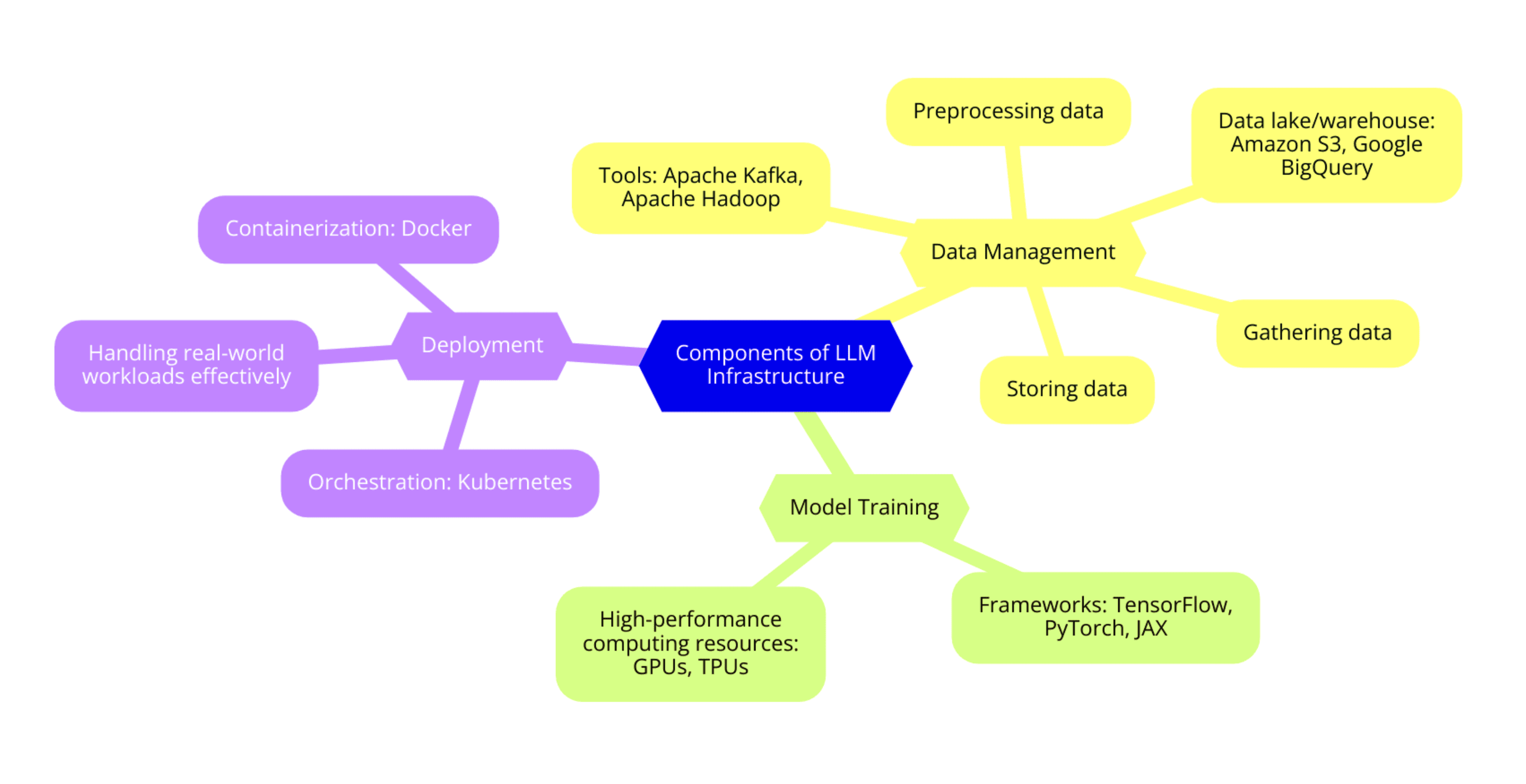
Data Management: The backbone of any LLM infrastructure is effective data management. This process involves gathering, storing, or preprocessing enormous amounts of information. Tools such as Apache Kafka and Apache Hadoop play pivotal roles here, enabling smooth data devouring and refining. You also need a sturdy data lake or warehouse, like Amazon S3 or Google BigQuery, to handle the scale of data needed for training LLMs.
Model Training: Once your information is in place, the next step is model training. You’ll use structures such as TensorFlow, PyTorch, or JAX to establish and instruct your models. These structures provide the adaptability and power significant to build intricate auditory networks. High-execution computing resources, indulging GPUs and TPUs, are also necessary for boosting the training process.
Deployment: Deploying your instructed models need a distinct set of tools and practices. Containerization technologies such as Docker and orchestration platforms such as Kubernetes are indispensable. They help in sustaining and gauging your applications, ensuring that your models can handle real-world workloads effectively.
But what about keeping your data safe and compliant while using these advanced tools?
Emerging Tools and Platforms
Staying ahead in the field of LLM infrastructure means keeping an eye on emerging tools and platforms that support distinct layers of the heap.
Data Management Tools
New platforms such as Delta Lake and Apache Iceberg are transforming data management. They offer ACID transactions and enable ductile data refining, making them ideal for handling the enormous datasets needed by LLMs.
Model Training Platforms
Platforms like Google AI Platform, Amazon SageMaker, and Microsoft Azure ML are simplifying the model training process. These sustained services provide everything from data preprocessing to model deployment, reducing the intricate and duration required to get your models into production.
Deployment and Monitoring Solutions
For deployment, solutions such as Kubernetes-based Kubeflow and TensorFlow Serving are obtaining eminence. They streamline the deployment process and integrate smoothly with other cloud-native tools. For monitoring, innovative tools such as New Relic and Datadog provide thorough luminosity helping you sustain maximum performance.
Security and Governance in LLM and Generative AI Applications
Security and Governance are uppermost when dealing with LLMs and Generative AI applications. Ensuring data seclusion, governance and compliance is an important challenge, but numerous solutions are arising. Those challenges are:
Data Privacy and Governance
To acknowledge data seclusion, you must enforce strict data governance strategies. Tools such as Apache Ranger and Privacera can help execute these policies, ensuring that sensitive information is handled precisely. In addition, compliance with regulations like GDPR and CCPA is significant, and solutions such as TrustArc and OneTrust can assist in accomplishing this docility.
Innovative Security Approaches
Innovative approaches to LLM applications security include using Private IPs and advanced cloud security contraptions. Private IPs limit access to your models, adding an auxiliary layer of security. Cloud providers such as AWS, Azure and Google Cloud provide numerous security attributes, including identity and access management (IAM), encryption, and network security which are important for safeguarding your AI applications.
Now that we've covered security and compliance, let's wrap up by looking at the future of LLM and Generative AI infrastructure.
Looking to delve deeper into AI technologies? Check out our pragmatic article on Building Low-Code LLM Apps with Visual Programming to sleek your development process.
Conclusion
Advanced infrastructure is critical to unleashing the full potential of LLM and Generative AI technologies. As these technologies progresses, so too must the infrastructure that supports them. Constant innovation and adjustment are key to staying ahead and meeting future requirements.
Who needs superheroes when you have Large Language Models (LLMs) and Generative AI reshaping the world?
To truly employ the power of Large Language Models (LLMs) and Generative AI, you need a sophisticated infrastructure. This infrastructure not only upholds the profound computational stipulations but also ensures smooth workflow and manageability. Over the years, experts have customized and transformed the infrastructure for these applications, playing a crucial role in intensifying AI technology.
The Role of Large Language Models in Modern Technology
Understanding and Replicating Human Speech with Large Language Models
Large Language Models (LLMs) are a type of artificial intelligence designed to comprehend and produce human language. Think of them as acutely advanced text mainframes that can comprehend context, pick up on nuances, and even produce text that sounds like it was written by a person. Researchers instruct these models on huge amounts of data, captivating the complexities of human speech motifs.
At their fundamental, LLMs operate by forecasting the next word in a sentence based on the context offered by the previous words. This might sound simple, but it’s an intricate task that involves comprehending grammar, context and even cultural references. The more information they are instructed on, the better they become at imitating human language, making them indispensable tools for numerous applications in modern technology.
Examples of LLM Applications in the Industry
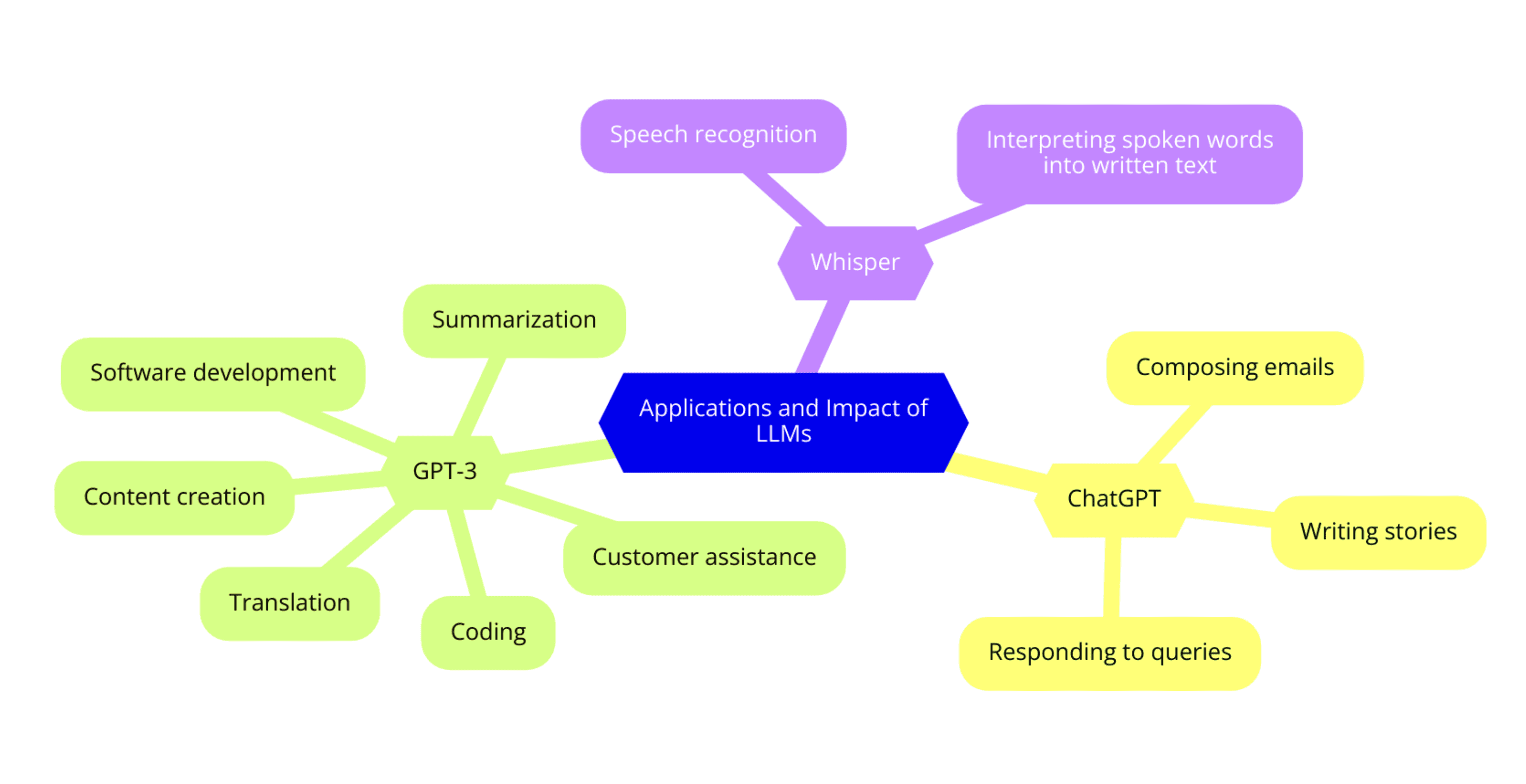
LLMs have made substantial progress in numerous industries, exhibiting their ingenuity and potential.
ChatGPT: A noticeable instance is OpenAI developed ChatGPT, an AI designed to help in producing human-like text based on the input it derives. Whether you need help composing an email, writing a story, or responding to queries, ChatGPT can handle it with imposing precision and smoothness.
GPT-3: Another renowned LLM is GPT-3, also by OpenAI. It’s one of most advanced language models accessible, able to execute enormous tasks like summarization, translation, and even coding. Its capability to produce coherent and contextually pertinent text has made it a groundbreaker in fields such as customer assistance, content creation and even software development.
Whisper: OpenAI’s Whisper is yet another intriguing application of LLM technology. Unlike ChatGPT and GPT-3, which concentrate on text, Developers created Whisper for speech recognition. It can interpret spoken words into written text with outstanding precision, making it certainly effective for applications like translation services, voice-activated assistants and more.
These instances emphasize how LLMs are transforming the way we communicate with technology, making tasks simpler, swifter and more effective. The probable applications are colossal, and we’re just starting to scratch the surface of what these significant models can achieve.
Want to know how to efficiently measure and assess the performance of large language models? Check out our comprehensive guide on Evaluating Large Language Models: Methods And Metrics.
Now, let's break down what makes this infrastructure so robust.
Generative AI and Its Infrastructure Needs
How Generative AI Uses LLM Outputs to Create Original Content
Generative AI uses the power of Large Language Models (LLMs) to create eccentric and original content. When you think about it, these models are like the brains behind Artificial Intelligence, refining enormous amounts of data to comprehend language motifs and nuances. Envision you are writing an article. Instead of you doing all the hefty lifting, an LLM dissects relative content, grasps from it, and produces a draft that imitates human writing.
This ability is critical because it permits AI to generate high-quality content, from blogs and fictions to even code and design components. The more information an LLM has, the better it becomes at producing pertinent and appealing content. So, when you see an Artificial Intelligence tool drizzling-out a near immaculate piece of writing, it’s all thanks to the broad training and information fed into these LLMs. It’s like having a private support who’s read every book, blog and article on the topic at hand.
The Generative AI Flywheel and the Necessity for Robust, Scalable Infrastructure
Now, let’s delve into the Generative AI Flywheel. This notion revolves around constant enhancement and innovation in Artificial Intelligence. Think of it as a cycle where Artificial Intelligence models grasp, produce, and receive feedback, and then process their yields based on that response. It’s an endless loop of improvement. You require a sturdy and ductile infrastructure for this flywheel to spin sleekly.
Why is this infrastructure so important? Envisage trying to run a marathon without apt training or apparatus. You wouldn’t get far, right? Likewise, generative AI requires significant hardware and software to refine vast amounts of information swiftly and effectively. Flexible infrastructure ensures that as your AI demands grow, your systems can handle the load without a hindrance.
When you handle generative AI, precision and innovation become unchangeable. Sturdy infrastructure supports these by giving the computational power required for deep learning and intricate data refining. Without it, your AI models would scuffle, resulting in slower refining times and less precise yields.
The success of generative AI articulates the sleek incorporation of LLM yields and the support of a strong infrastructure. By ensuring you have both, you’re setting the stage for AI that’s not only innovative but also dependable and effective.
Core Components of LLM and Generative AI Infrastructure
To truly comprehend LLM infrastructure, delve into its core components. These components are the foundation of any successful enforcement of LLM and generative AI technologies.
High-Performance Computing, Scalable Cloud Solutions, and Efficient Data Storage
Foremost, high-performance computing (HPC) is significant. You can’t run intricate models without significant computing resources. Think of HPC as the engine of your Artificial Intelligence system, handling all those profound computations at a rapid speed. This ensures that your models run sleekly and effectively.
Next, adaptable cloud solutions come into play. As you data cultivates, you need the adaptability to scale your operations without any gimmicks. Cloud solutions offer this scalability, permitting you to handle augmenting workloads sleekly. Plus, they provide the ease of sustaining your infrastructure distantly, saving you the hassle of physical maintenance.
Effective data storage and management are equally critical. You need a sturdy system to store enormous amounts of information safely and access it swiftly whenever needed. Apt data management ensures that your data is not only secure but also easily recoverable, which is crucial for training and fine-tuning your models.
Specialized Infrastructure Considerations
When you get into the particulars of LLM infrastructure, certain esoteric contemplations become pivotal. GPU-intensive workloads are one such aspect. GPUs (Graphics Processing Units) are specifically attired for the parallel refining tasks that AI model requires. They substantially boost the training process, permitting you to handle more information and intricate computations efficiently.
Containerization is another key element. Using containers allows you to bundle your applications and their reliabilities together, ensuring they run constantly across distinct computing environments. This makes deployment and gauging much easier and more dependable, as containers provide a structured unit of software.
An adaptable LLM infrastructure uses high-performance assessing, ductile cloud solutions, and effective data storage, adorned by specialized contemplations such as GPU workloads and containerization. By concentrating on these elements, you can build a sturdy foundation for your generative AI projects.
To delve deeper into how LLM parameters can be refined and upgraded, check out our comprehensive guide "A Brief Guide To LLM Parameters: Tuning and Optimization."
Evolving Trends in LLM and Generative AI Infrastructure
Innovations in Cloud Infrastructure Solutions
Cloud infrastructure is the backbone of LLM and Generative AI. Innovations in this space are groundbreakers. For example, instance startup optimizations are making a substantial distinction. You’re seeing swifter boot times and more effective resource allotment, which means your AI models can be up and running in no time.
Compute attainability is also enhanced. Cloud providers are rolling out more prominent and adaptable options, permitting you to scale your functioning smoothly. This adaptability ensures that you have the right amount of compute power precisely when you require it, improving the effectiveness and pace of your AI tasks.
Balancing Cost, Performance, and Reliability
In the scenario of LLM and Generative AI, locating the sweet spot between price, performance, and dependability is critical. You need to sustain expenditures without yielding on the performance of your models.
Arising solutions are helping accomplish this balance. For instance, new pricing models and resource management tools let you upgrade costs while maintaining high performance. You can now run profound AI tasks without breaking the bank, ensuring that your models perform steadfastly under several circumstances.
By concentrating on these developing trends, you’re setting a stage for the future where LLM and Generative AI can prosper, delivering incredible outcomes with optimal effectiveness.
Want to make your LLM development 3X faster? Comprehend how using the RagaAI LLM Hub can make your LLM development 3X faster, saving you time and resources.
Speaking of trends, let’s dive into the specific platforms that are addressing some of the biggest challenges in the field.
Addressing the Challenges Through Dedicated Platforms
Ray’s Contributions to Resolving Scalability and Complexity Challenges
When it comes to deploying foundation models, expandability and intricacy are two of the biggest obstacles. Ray has appeared as a groundbreaker in this arena. The designers created it to streamline the process of building and scaling AI applications. Imagine you're trying to fetch multiple tasks concurrently; Ray acts like an extra pair of hands, making it much simpler to sustain everything.
Ray’s dispensation enforcement structure allows you to break down intricate tasks into smaller, tractable units. This approach substantially reduces the intricacy of large-scale AI operations. Furthermore, it sustains a variety of machine learning libraries and structures, making it highly adaptable. By using Ray, you can effectively scale your AI applications across multiple tumors, ensuring they run sleekly even as they grow in size and heterogeneity.
Anyscale’s Seamless Integration for LLM APIs
Anyscale takes things a step further by providing a sleek incorporation of LLM APIs. Imagine you have a splendid kitchen but no chef; Anyscale steps in as the skilled chef, managing all the complex information so you can enjoy the final product. It concentrates on excluding the frustrations of infrastructure management, permitting you to focus on developing and deploying your models.
With Anyscale, you don’t have to worry about the fundamental infrastructure. Anyscale offers an instinctive platform that sustains everything from resource allotment to scaling. This means you can deploy your LLM APIs smoothly, without getting tangled down by the technical intricacies. By streamlining the infrastructure management process, Anyscale ensures that your concentration stays on innovation and development, rather than on sustaining and scaling the infrastructure.
Platforms such as Ray and Anyscale are important in conquering the difficulties linked with LLM and generative AI. They offer substantial tools and support to handle scalability and intricacy, enabling you to deploy sturdy AI models with ease.
So, how do all these components fit together to create a modern LLM infrastructure stack?
The Modern LLM Infrastructure Stack
Comprehending the components of the modern LLM infrastructure stack is significant for using the power of Generative AI. You need to contemplate numerous elements, from data management to deployment and monitoring, to build a sturdy system.
Components: Data Management, Deployment, and Monitoring
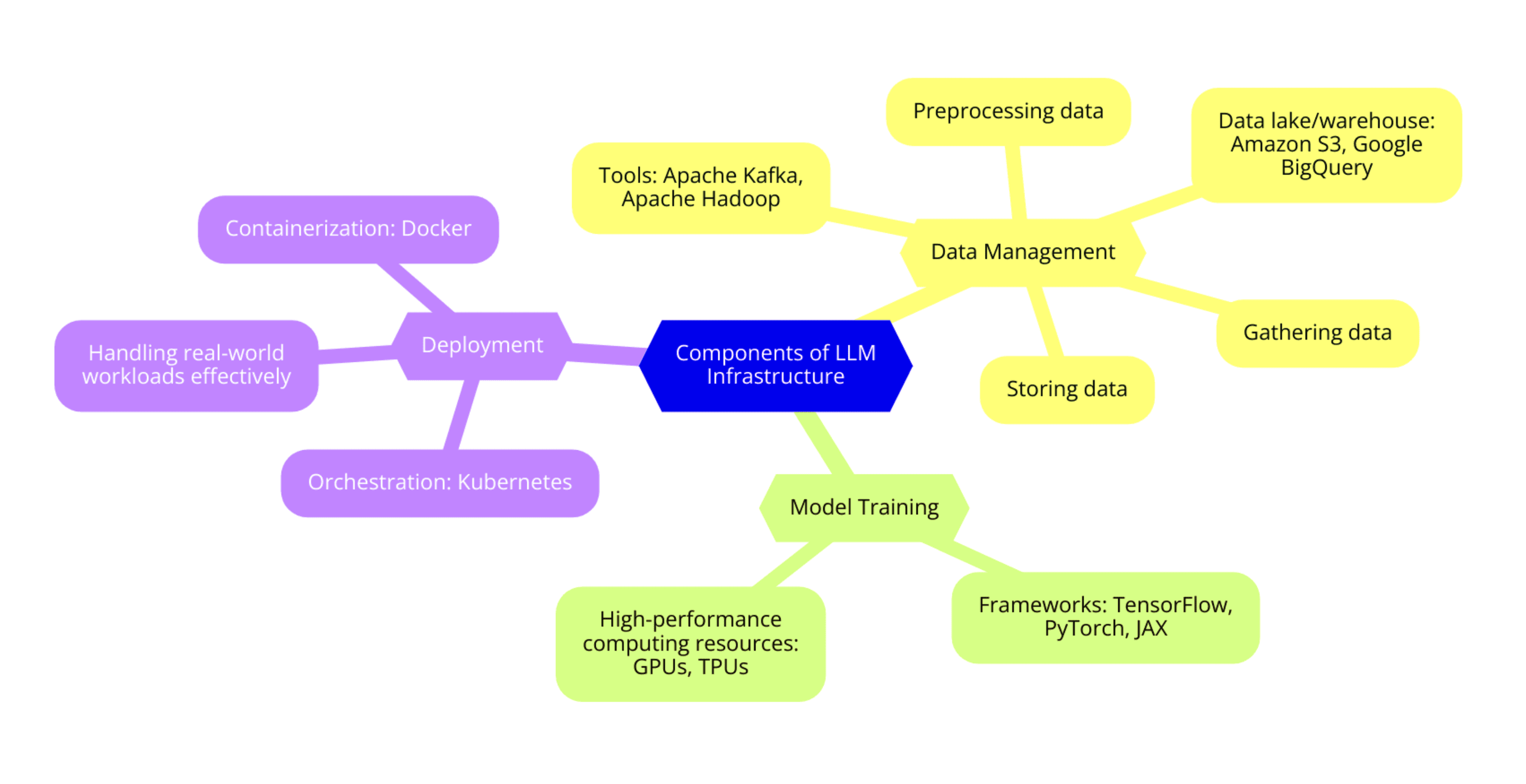
Data Management: The backbone of any LLM infrastructure is effective data management. This process involves gathering, storing, or preprocessing enormous amounts of information. Tools such as Apache Kafka and Apache Hadoop play pivotal roles here, enabling smooth data devouring and refining. You also need a sturdy data lake or warehouse, like Amazon S3 or Google BigQuery, to handle the scale of data needed for training LLMs.
Model Training: Once your information is in place, the next step is model training. You’ll use structures such as TensorFlow, PyTorch, or JAX to establish and instruct your models. These structures provide the adaptability and power significant to build intricate auditory networks. High-execution computing resources, indulging GPUs and TPUs, are also necessary for boosting the training process.
Deployment: Deploying your instructed models need a distinct set of tools and practices. Containerization technologies such as Docker and orchestration platforms such as Kubernetes are indispensable. They help in sustaining and gauging your applications, ensuring that your models can handle real-world workloads effectively.
But what about keeping your data safe and compliant while using these advanced tools?
Emerging Tools and Platforms
Staying ahead in the field of LLM infrastructure means keeping an eye on emerging tools and platforms that support distinct layers of the heap.
Data Management Tools
New platforms such as Delta Lake and Apache Iceberg are transforming data management. They offer ACID transactions and enable ductile data refining, making them ideal for handling the enormous datasets needed by LLMs.
Model Training Platforms
Platforms like Google AI Platform, Amazon SageMaker, and Microsoft Azure ML are simplifying the model training process. These sustained services provide everything from data preprocessing to model deployment, reducing the intricate and duration required to get your models into production.
Deployment and Monitoring Solutions
For deployment, solutions such as Kubernetes-based Kubeflow and TensorFlow Serving are obtaining eminence. They streamline the deployment process and integrate smoothly with other cloud-native tools. For monitoring, innovative tools such as New Relic and Datadog provide thorough luminosity helping you sustain maximum performance.
Security and Governance in LLM and Generative AI Applications
Security and Governance are uppermost when dealing with LLMs and Generative AI applications. Ensuring data seclusion, governance and compliance is an important challenge, but numerous solutions are arising. Those challenges are:
Data Privacy and Governance
To acknowledge data seclusion, you must enforce strict data governance strategies. Tools such as Apache Ranger and Privacera can help execute these policies, ensuring that sensitive information is handled precisely. In addition, compliance with regulations like GDPR and CCPA is significant, and solutions such as TrustArc and OneTrust can assist in accomplishing this docility.
Innovative Security Approaches
Innovative approaches to LLM applications security include using Private IPs and advanced cloud security contraptions. Private IPs limit access to your models, adding an auxiliary layer of security. Cloud providers such as AWS, Azure and Google Cloud provide numerous security attributes, including identity and access management (IAM), encryption, and network security which are important for safeguarding your AI applications.
Now that we've covered security and compliance, let's wrap up by looking at the future of LLM and Generative AI infrastructure.
Looking to delve deeper into AI technologies? Check out our pragmatic article on Building Low-Code LLM Apps with Visual Programming to sleek your development process.
Conclusion
Advanced infrastructure is critical to unleashing the full potential of LLM and Generative AI technologies. As these technologies progresses, so too must the infrastructure that supports them. Constant innovation and adjustment are key to staying ahead and meeting future requirements.
Who needs superheroes when you have Large Language Models (LLMs) and Generative AI reshaping the world?
To truly employ the power of Large Language Models (LLMs) and Generative AI, you need a sophisticated infrastructure. This infrastructure not only upholds the profound computational stipulations but also ensures smooth workflow and manageability. Over the years, experts have customized and transformed the infrastructure for these applications, playing a crucial role in intensifying AI technology.
The Role of Large Language Models in Modern Technology
Understanding and Replicating Human Speech with Large Language Models
Large Language Models (LLMs) are a type of artificial intelligence designed to comprehend and produce human language. Think of them as acutely advanced text mainframes that can comprehend context, pick up on nuances, and even produce text that sounds like it was written by a person. Researchers instruct these models on huge amounts of data, captivating the complexities of human speech motifs.
At their fundamental, LLMs operate by forecasting the next word in a sentence based on the context offered by the previous words. This might sound simple, but it’s an intricate task that involves comprehending grammar, context and even cultural references. The more information they are instructed on, the better they become at imitating human language, making them indispensable tools for numerous applications in modern technology.
Examples of LLM Applications in the Industry
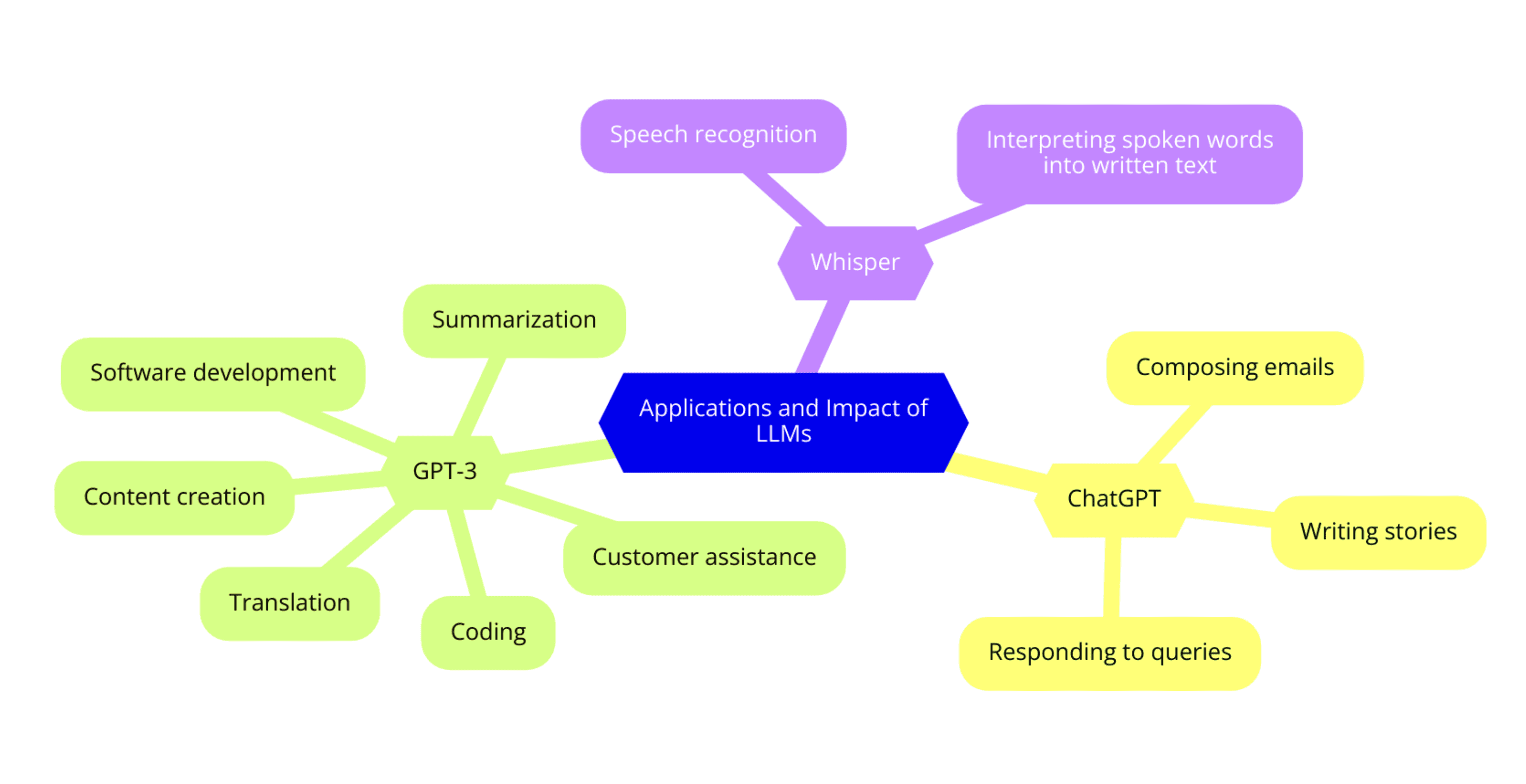
LLMs have made substantial progress in numerous industries, exhibiting their ingenuity and potential.
ChatGPT: A noticeable instance is OpenAI developed ChatGPT, an AI designed to help in producing human-like text based on the input it derives. Whether you need help composing an email, writing a story, or responding to queries, ChatGPT can handle it with imposing precision and smoothness.
GPT-3: Another renowned LLM is GPT-3, also by OpenAI. It’s one of most advanced language models accessible, able to execute enormous tasks like summarization, translation, and even coding. Its capability to produce coherent and contextually pertinent text has made it a groundbreaker in fields such as customer assistance, content creation and even software development.
Whisper: OpenAI’s Whisper is yet another intriguing application of LLM technology. Unlike ChatGPT and GPT-3, which concentrate on text, Developers created Whisper for speech recognition. It can interpret spoken words into written text with outstanding precision, making it certainly effective for applications like translation services, voice-activated assistants and more.
These instances emphasize how LLMs are transforming the way we communicate with technology, making tasks simpler, swifter and more effective. The probable applications are colossal, and we’re just starting to scratch the surface of what these significant models can achieve.
Want to know how to efficiently measure and assess the performance of large language models? Check out our comprehensive guide on Evaluating Large Language Models: Methods And Metrics.
Now, let's break down what makes this infrastructure so robust.
Generative AI and Its Infrastructure Needs
How Generative AI Uses LLM Outputs to Create Original Content
Generative AI uses the power of Large Language Models (LLMs) to create eccentric and original content. When you think about it, these models are like the brains behind Artificial Intelligence, refining enormous amounts of data to comprehend language motifs and nuances. Envision you are writing an article. Instead of you doing all the hefty lifting, an LLM dissects relative content, grasps from it, and produces a draft that imitates human writing.
This ability is critical because it permits AI to generate high-quality content, from blogs and fictions to even code and design components. The more information an LLM has, the better it becomes at producing pertinent and appealing content. So, when you see an Artificial Intelligence tool drizzling-out a near immaculate piece of writing, it’s all thanks to the broad training and information fed into these LLMs. It’s like having a private support who’s read every book, blog and article on the topic at hand.
The Generative AI Flywheel and the Necessity for Robust, Scalable Infrastructure
Now, let’s delve into the Generative AI Flywheel. This notion revolves around constant enhancement and innovation in Artificial Intelligence. Think of it as a cycle where Artificial Intelligence models grasp, produce, and receive feedback, and then process their yields based on that response. It’s an endless loop of improvement. You require a sturdy and ductile infrastructure for this flywheel to spin sleekly.
Why is this infrastructure so important? Envisage trying to run a marathon without apt training or apparatus. You wouldn’t get far, right? Likewise, generative AI requires significant hardware and software to refine vast amounts of information swiftly and effectively. Flexible infrastructure ensures that as your AI demands grow, your systems can handle the load without a hindrance.
When you handle generative AI, precision and innovation become unchangeable. Sturdy infrastructure supports these by giving the computational power required for deep learning and intricate data refining. Without it, your AI models would scuffle, resulting in slower refining times and less precise yields.
The success of generative AI articulates the sleek incorporation of LLM yields and the support of a strong infrastructure. By ensuring you have both, you’re setting the stage for AI that’s not only innovative but also dependable and effective.
Core Components of LLM and Generative AI Infrastructure
To truly comprehend LLM infrastructure, delve into its core components. These components are the foundation of any successful enforcement of LLM and generative AI technologies.
High-Performance Computing, Scalable Cloud Solutions, and Efficient Data Storage
Foremost, high-performance computing (HPC) is significant. You can’t run intricate models without significant computing resources. Think of HPC as the engine of your Artificial Intelligence system, handling all those profound computations at a rapid speed. This ensures that your models run sleekly and effectively.
Next, adaptable cloud solutions come into play. As you data cultivates, you need the adaptability to scale your operations without any gimmicks. Cloud solutions offer this scalability, permitting you to handle augmenting workloads sleekly. Plus, they provide the ease of sustaining your infrastructure distantly, saving you the hassle of physical maintenance.
Effective data storage and management are equally critical. You need a sturdy system to store enormous amounts of information safely and access it swiftly whenever needed. Apt data management ensures that your data is not only secure but also easily recoverable, which is crucial for training and fine-tuning your models.
Specialized Infrastructure Considerations
When you get into the particulars of LLM infrastructure, certain esoteric contemplations become pivotal. GPU-intensive workloads are one such aspect. GPUs (Graphics Processing Units) are specifically attired for the parallel refining tasks that AI model requires. They substantially boost the training process, permitting you to handle more information and intricate computations efficiently.
Containerization is another key element. Using containers allows you to bundle your applications and their reliabilities together, ensuring they run constantly across distinct computing environments. This makes deployment and gauging much easier and more dependable, as containers provide a structured unit of software.
An adaptable LLM infrastructure uses high-performance assessing, ductile cloud solutions, and effective data storage, adorned by specialized contemplations such as GPU workloads and containerization. By concentrating on these elements, you can build a sturdy foundation for your generative AI projects.
To delve deeper into how LLM parameters can be refined and upgraded, check out our comprehensive guide "A Brief Guide To LLM Parameters: Tuning and Optimization."
Evolving Trends in LLM and Generative AI Infrastructure
Innovations in Cloud Infrastructure Solutions
Cloud infrastructure is the backbone of LLM and Generative AI. Innovations in this space are groundbreakers. For example, instance startup optimizations are making a substantial distinction. You’re seeing swifter boot times and more effective resource allotment, which means your AI models can be up and running in no time.
Compute attainability is also enhanced. Cloud providers are rolling out more prominent and adaptable options, permitting you to scale your functioning smoothly. This adaptability ensures that you have the right amount of compute power precisely when you require it, improving the effectiveness and pace of your AI tasks.
Balancing Cost, Performance, and Reliability
In the scenario of LLM and Generative AI, locating the sweet spot between price, performance, and dependability is critical. You need to sustain expenditures without yielding on the performance of your models.
Arising solutions are helping accomplish this balance. For instance, new pricing models and resource management tools let you upgrade costs while maintaining high performance. You can now run profound AI tasks without breaking the bank, ensuring that your models perform steadfastly under several circumstances.
By concentrating on these developing trends, you’re setting a stage for the future where LLM and Generative AI can prosper, delivering incredible outcomes with optimal effectiveness.
Want to make your LLM development 3X faster? Comprehend how using the RagaAI LLM Hub can make your LLM development 3X faster, saving you time and resources.
Speaking of trends, let’s dive into the specific platforms that are addressing some of the biggest challenges in the field.
Addressing the Challenges Through Dedicated Platforms
Ray’s Contributions to Resolving Scalability and Complexity Challenges
When it comes to deploying foundation models, expandability and intricacy are two of the biggest obstacles. Ray has appeared as a groundbreaker in this arena. The designers created it to streamline the process of building and scaling AI applications. Imagine you're trying to fetch multiple tasks concurrently; Ray acts like an extra pair of hands, making it much simpler to sustain everything.
Ray’s dispensation enforcement structure allows you to break down intricate tasks into smaller, tractable units. This approach substantially reduces the intricacy of large-scale AI operations. Furthermore, it sustains a variety of machine learning libraries and structures, making it highly adaptable. By using Ray, you can effectively scale your AI applications across multiple tumors, ensuring they run sleekly even as they grow in size and heterogeneity.
Anyscale’s Seamless Integration for LLM APIs
Anyscale takes things a step further by providing a sleek incorporation of LLM APIs. Imagine you have a splendid kitchen but no chef; Anyscale steps in as the skilled chef, managing all the complex information so you can enjoy the final product. It concentrates on excluding the frustrations of infrastructure management, permitting you to focus on developing and deploying your models.
With Anyscale, you don’t have to worry about the fundamental infrastructure. Anyscale offers an instinctive platform that sustains everything from resource allotment to scaling. This means you can deploy your LLM APIs smoothly, without getting tangled down by the technical intricacies. By streamlining the infrastructure management process, Anyscale ensures that your concentration stays on innovation and development, rather than on sustaining and scaling the infrastructure.
Platforms such as Ray and Anyscale are important in conquering the difficulties linked with LLM and generative AI. They offer substantial tools and support to handle scalability and intricacy, enabling you to deploy sturdy AI models with ease.
So, how do all these components fit together to create a modern LLM infrastructure stack?
The Modern LLM Infrastructure Stack
Comprehending the components of the modern LLM infrastructure stack is significant for using the power of Generative AI. You need to contemplate numerous elements, from data management to deployment and monitoring, to build a sturdy system.
Components: Data Management, Deployment, and Monitoring
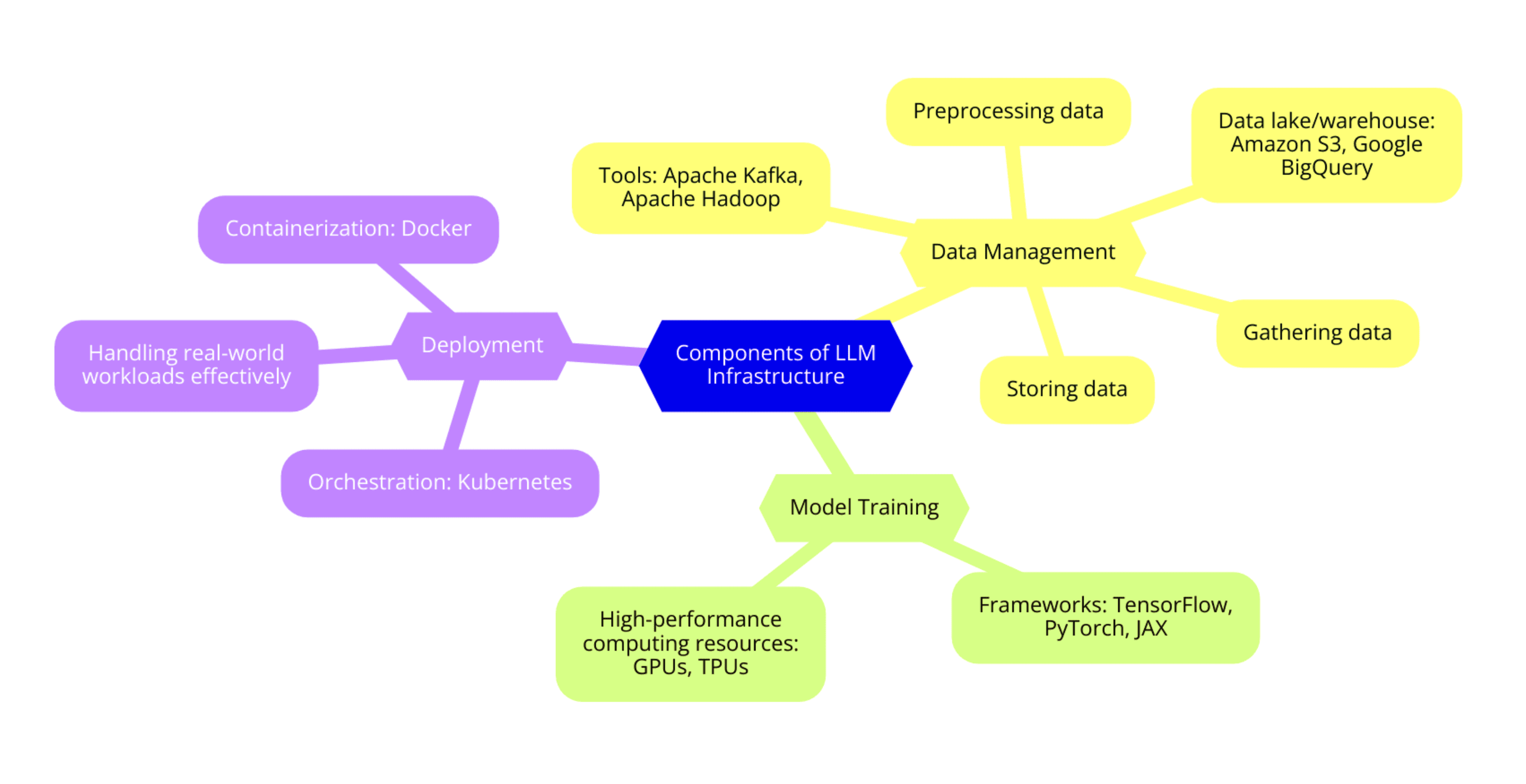
Data Management: The backbone of any LLM infrastructure is effective data management. This process involves gathering, storing, or preprocessing enormous amounts of information. Tools such as Apache Kafka and Apache Hadoop play pivotal roles here, enabling smooth data devouring and refining. You also need a sturdy data lake or warehouse, like Amazon S3 or Google BigQuery, to handle the scale of data needed for training LLMs.
Model Training: Once your information is in place, the next step is model training. You’ll use structures such as TensorFlow, PyTorch, or JAX to establish and instruct your models. These structures provide the adaptability and power significant to build intricate auditory networks. High-execution computing resources, indulging GPUs and TPUs, are also necessary for boosting the training process.
Deployment: Deploying your instructed models need a distinct set of tools and practices. Containerization technologies such as Docker and orchestration platforms such as Kubernetes are indispensable. They help in sustaining and gauging your applications, ensuring that your models can handle real-world workloads effectively.
But what about keeping your data safe and compliant while using these advanced tools?
Emerging Tools and Platforms
Staying ahead in the field of LLM infrastructure means keeping an eye on emerging tools and platforms that support distinct layers of the heap.
Data Management Tools
New platforms such as Delta Lake and Apache Iceberg are transforming data management. They offer ACID transactions and enable ductile data refining, making them ideal for handling the enormous datasets needed by LLMs.
Model Training Platforms
Platforms like Google AI Platform, Amazon SageMaker, and Microsoft Azure ML are simplifying the model training process. These sustained services provide everything from data preprocessing to model deployment, reducing the intricate and duration required to get your models into production.
Deployment and Monitoring Solutions
For deployment, solutions such as Kubernetes-based Kubeflow and TensorFlow Serving are obtaining eminence. They streamline the deployment process and integrate smoothly with other cloud-native tools. For monitoring, innovative tools such as New Relic and Datadog provide thorough luminosity helping you sustain maximum performance.
Security and Governance in LLM and Generative AI Applications
Security and Governance are uppermost when dealing with LLMs and Generative AI applications. Ensuring data seclusion, governance and compliance is an important challenge, but numerous solutions are arising. Those challenges are:
Data Privacy and Governance
To acknowledge data seclusion, you must enforce strict data governance strategies. Tools such as Apache Ranger and Privacera can help execute these policies, ensuring that sensitive information is handled precisely. In addition, compliance with regulations like GDPR and CCPA is significant, and solutions such as TrustArc and OneTrust can assist in accomplishing this docility.
Innovative Security Approaches
Innovative approaches to LLM applications security include using Private IPs and advanced cloud security contraptions. Private IPs limit access to your models, adding an auxiliary layer of security. Cloud providers such as AWS, Azure and Google Cloud provide numerous security attributes, including identity and access management (IAM), encryption, and network security which are important for safeguarding your AI applications.
Now that we've covered security and compliance, let's wrap up by looking at the future of LLM and Generative AI infrastructure.
Looking to delve deeper into AI technologies? Check out our pragmatic article on Building Low-Code LLM Apps with Visual Programming to sleek your development process.
Conclusion
Advanced infrastructure is critical to unleashing the full potential of LLM and Generative AI technologies. As these technologies progresses, so too must the infrastructure that supports them. Constant innovation and adjustment are key to staying ahead and meeting future requirements.
Subscribe to our newsletter to never miss an update
Subscribe to our newsletter to never miss an update
Other articles
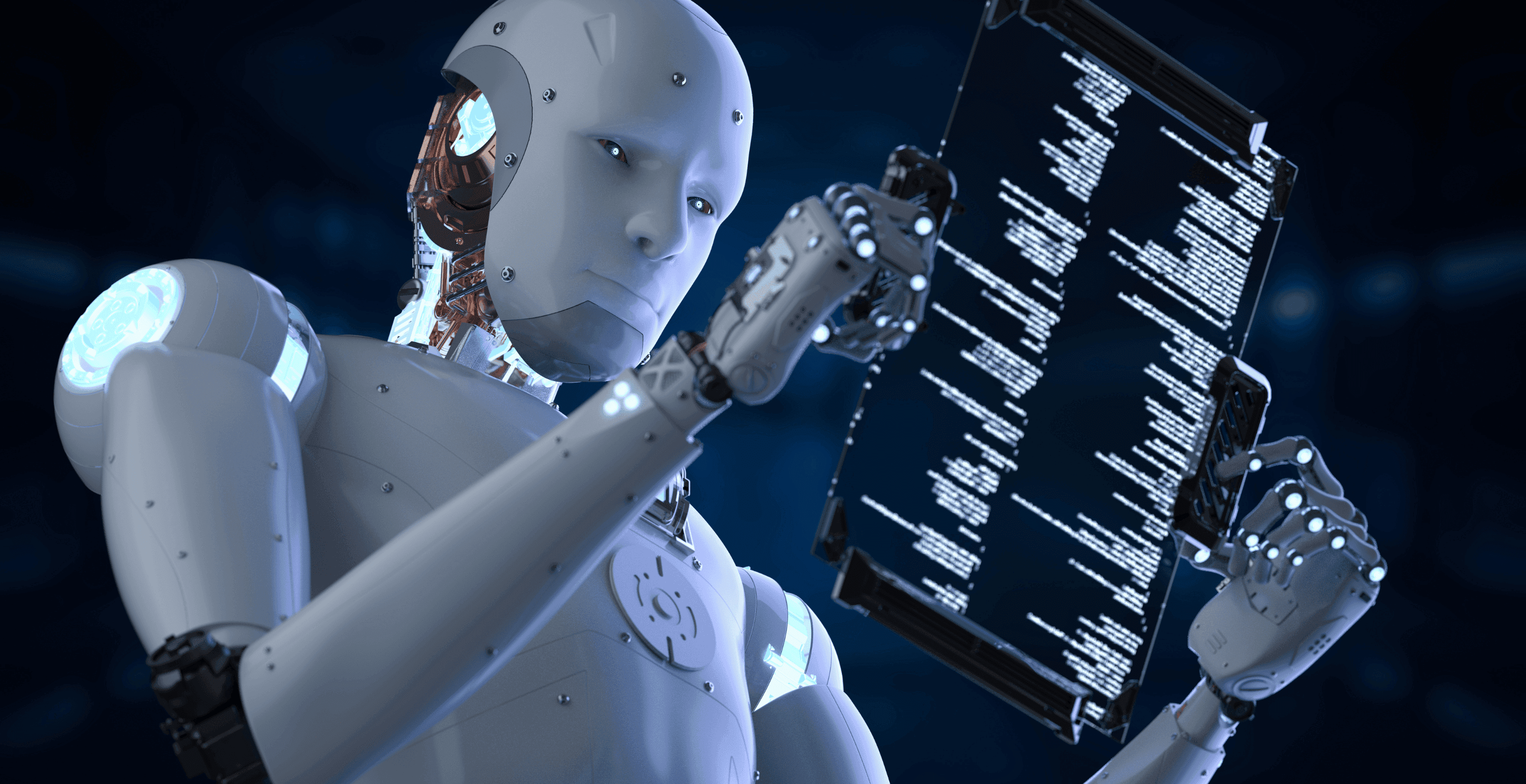
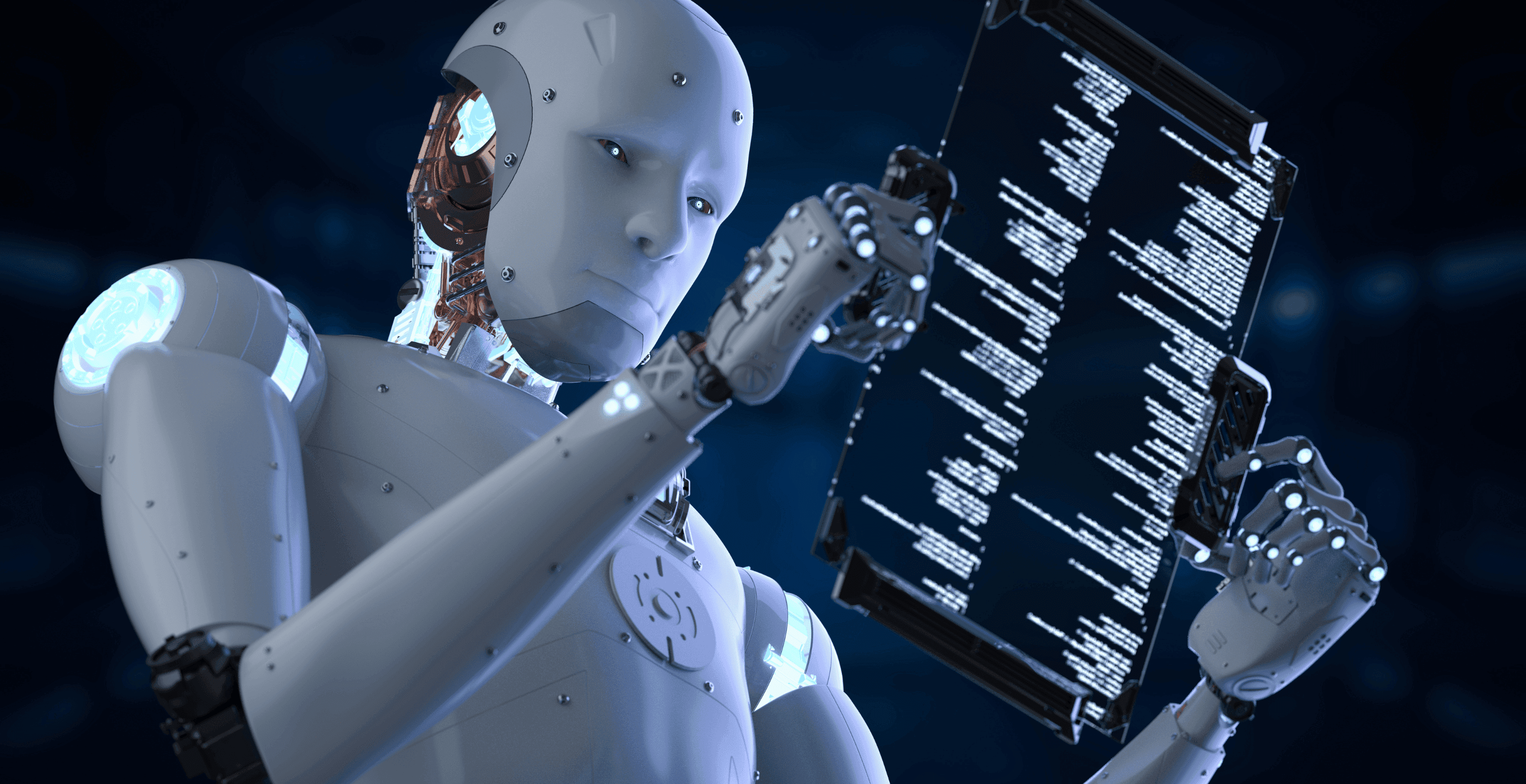
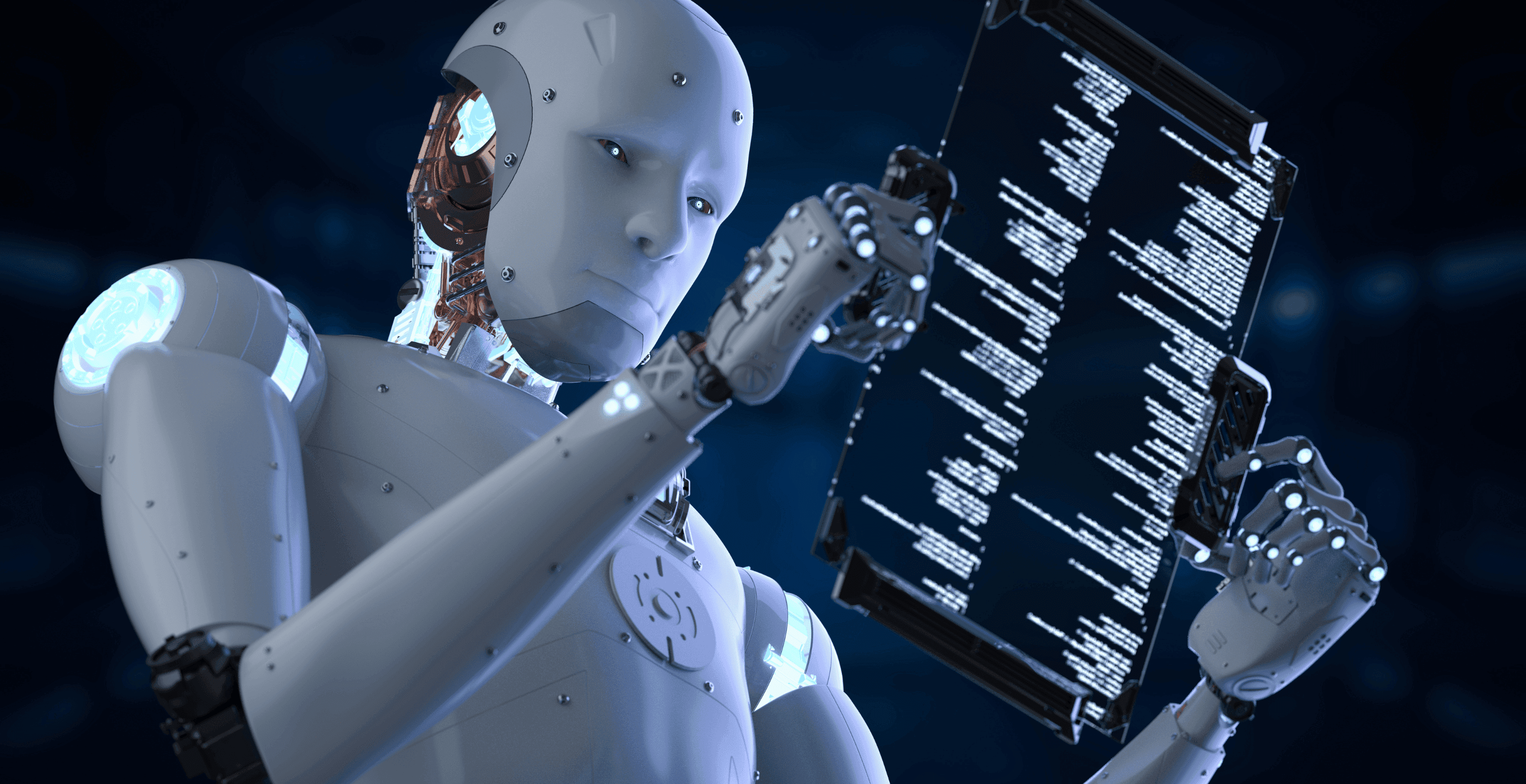
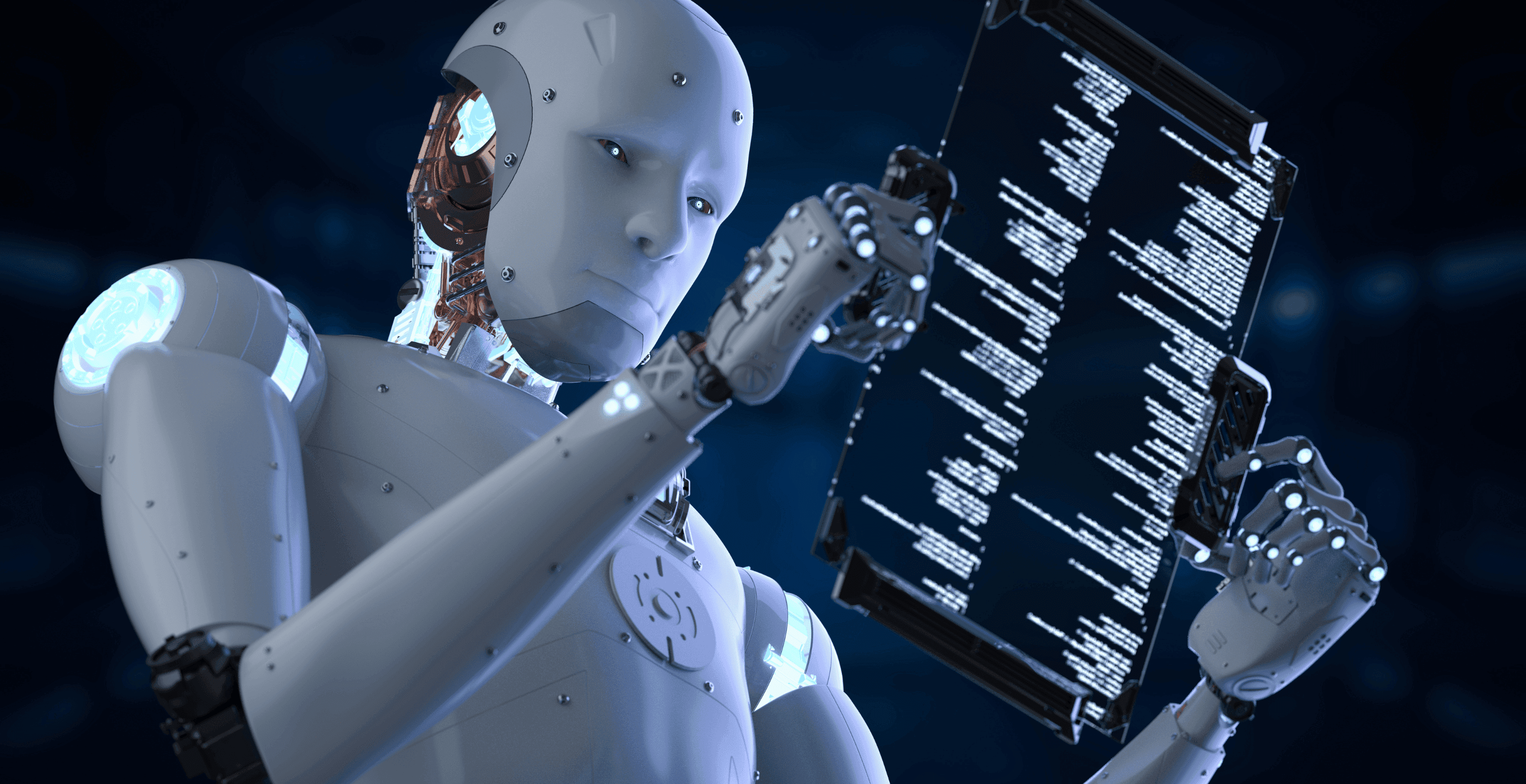
Exploring Intelligent Agents in AI

Rehan Asif
Jan 3, 2025
Read the article
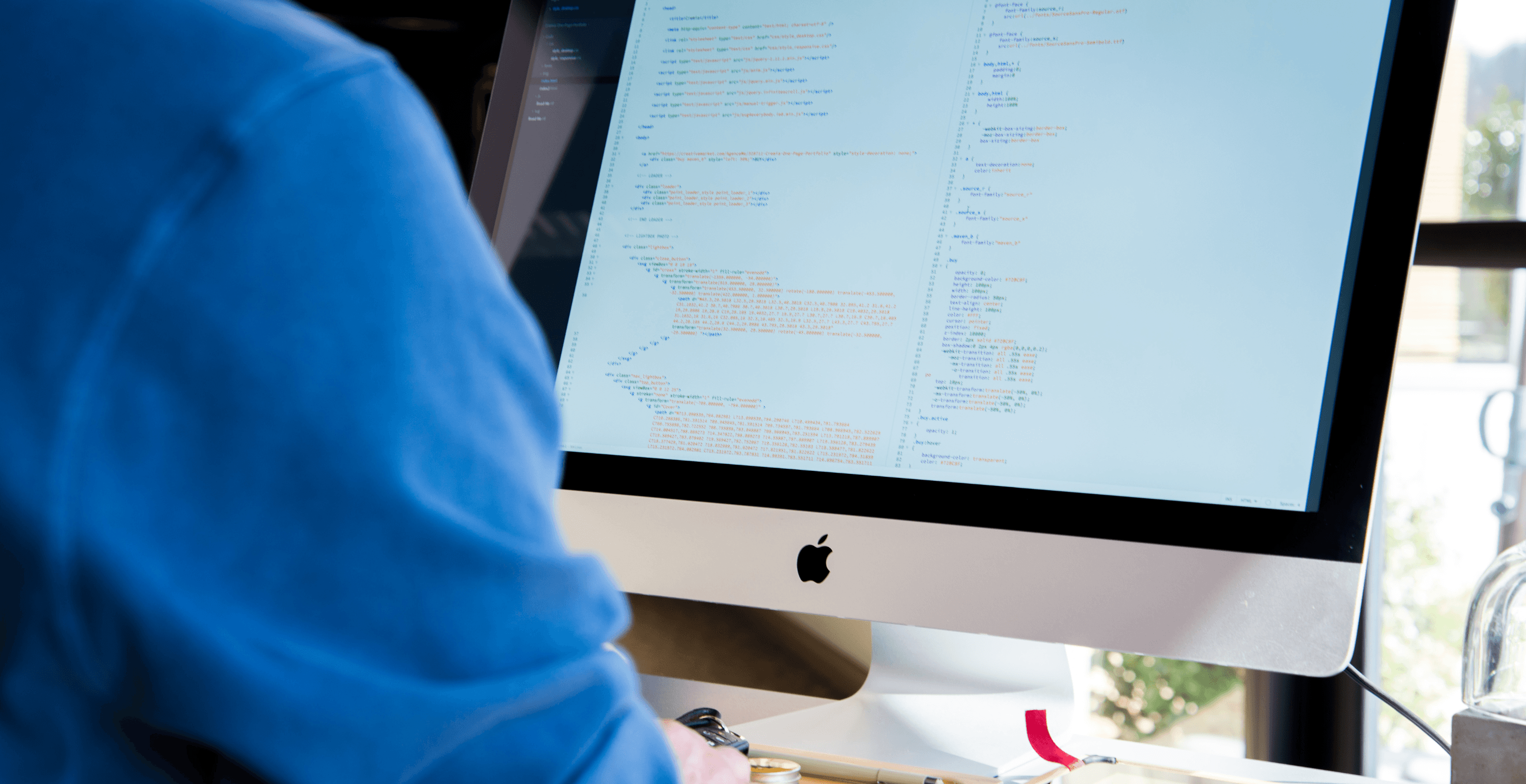
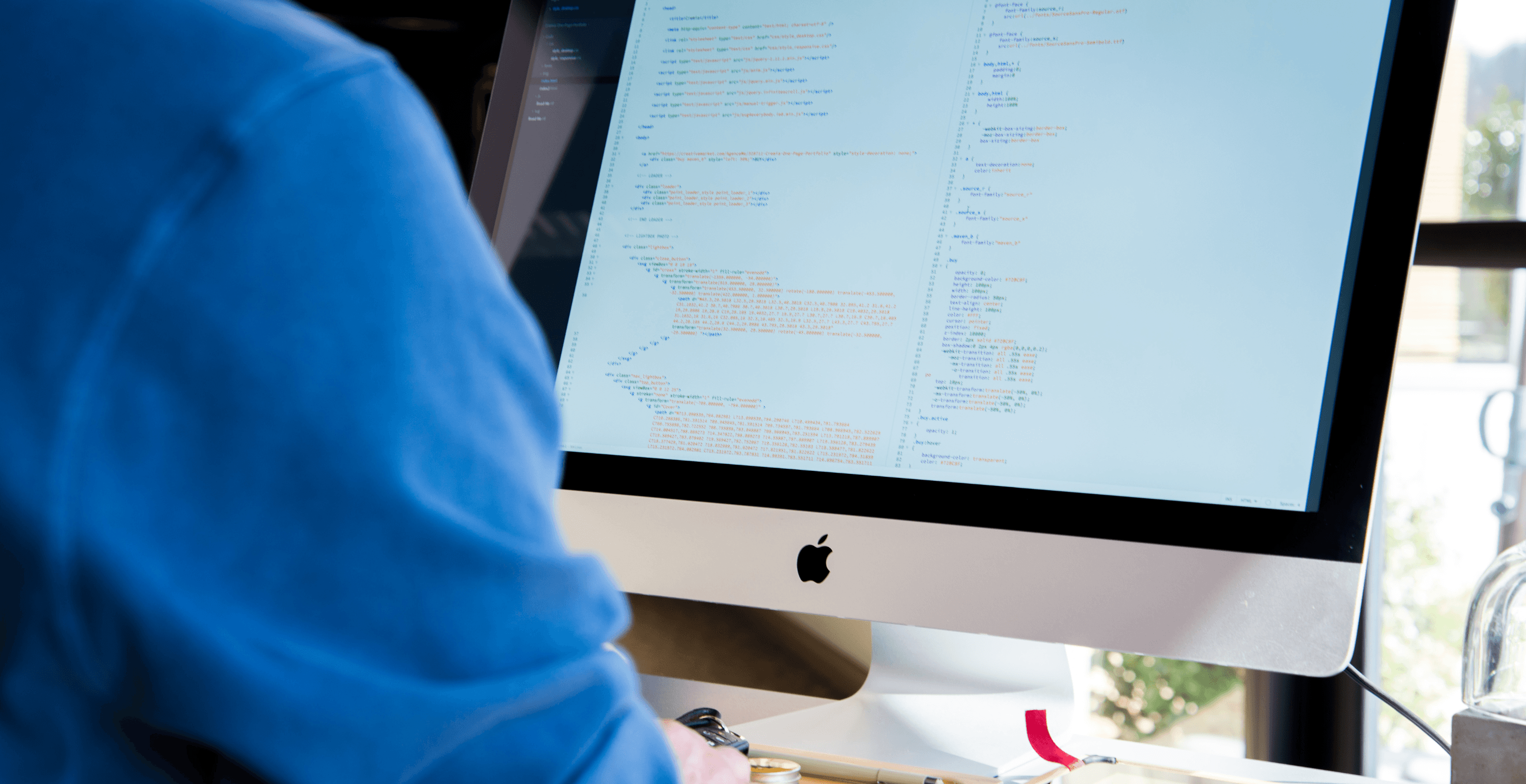
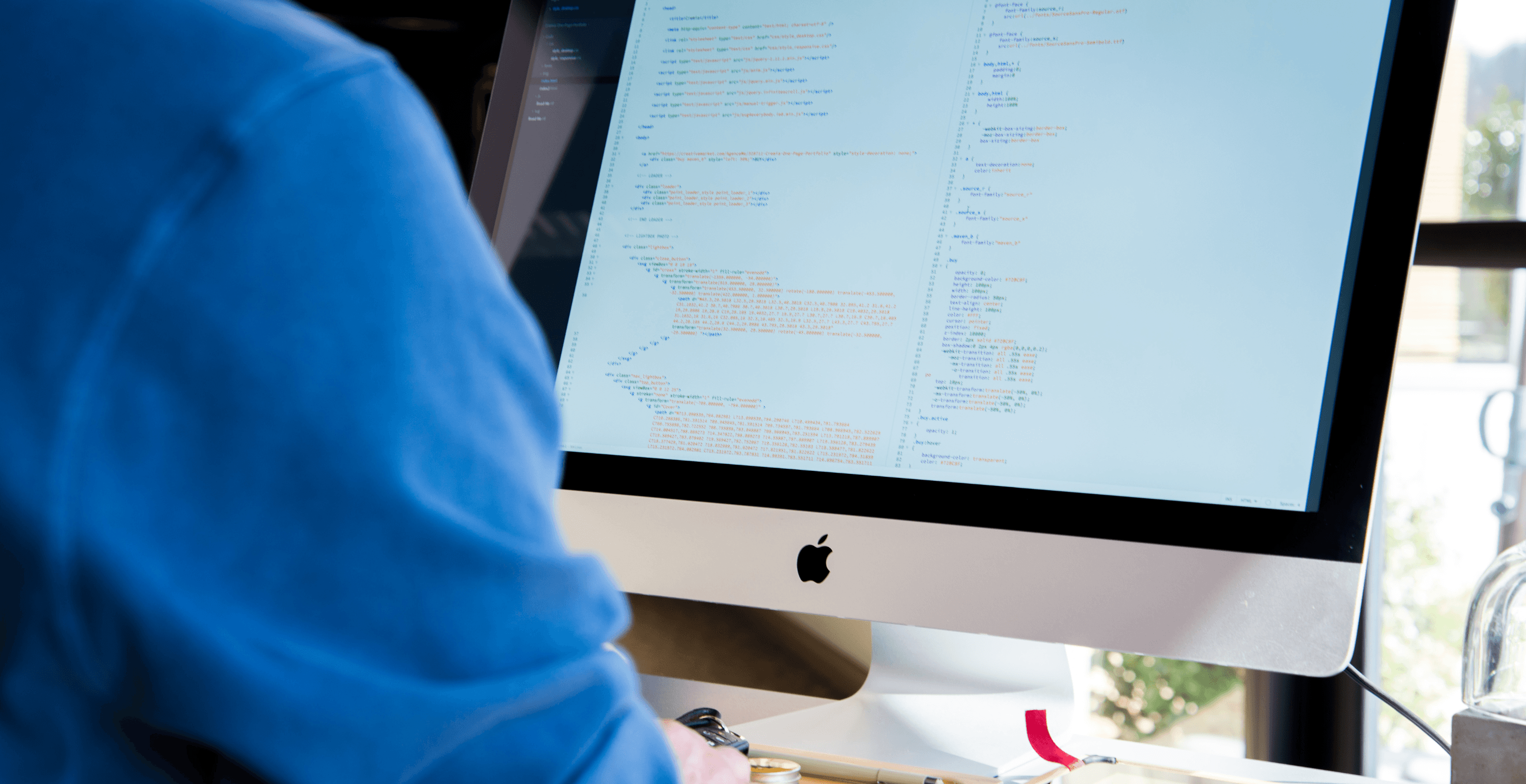
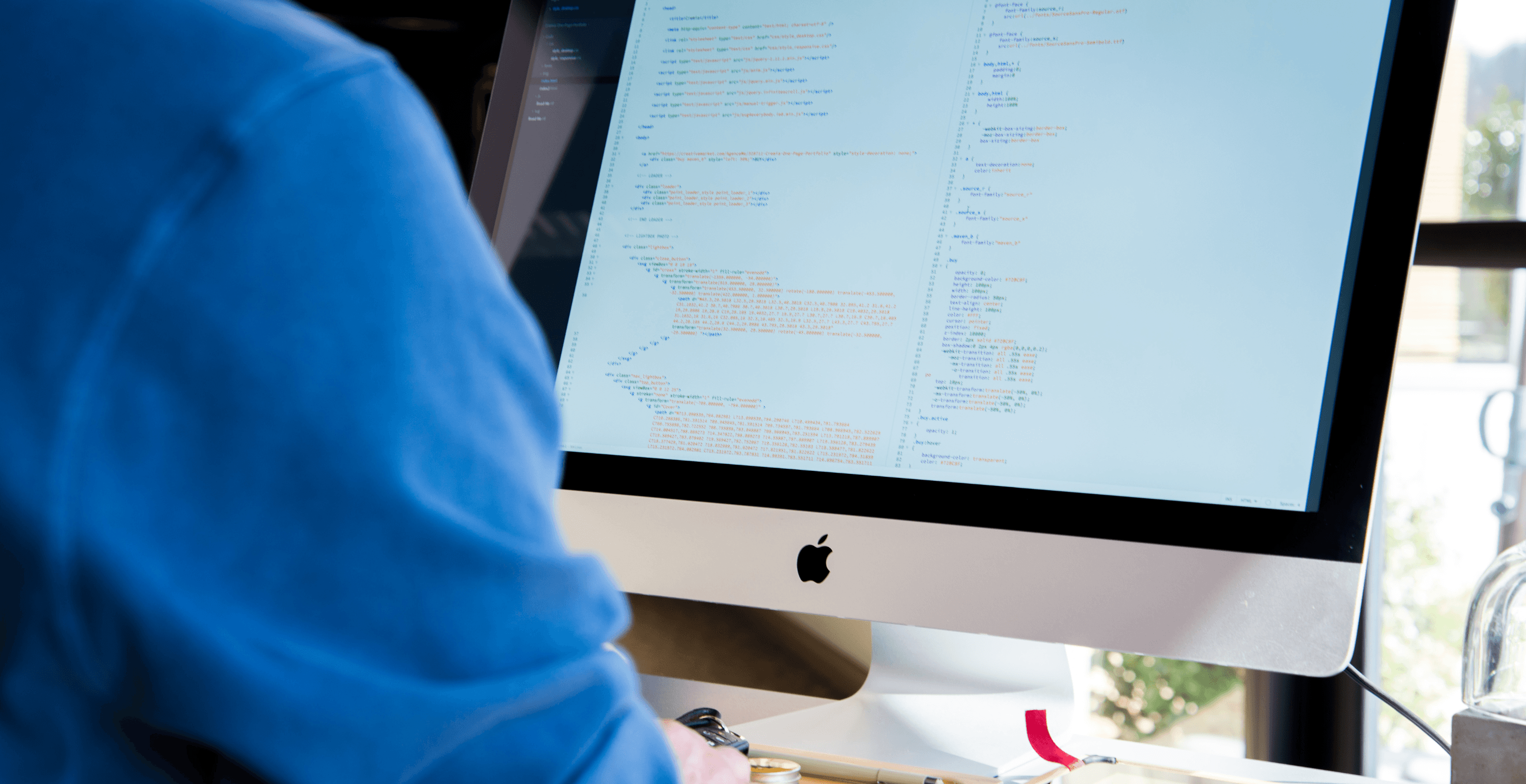
Understanding What AI Red Teaming Means for Generative Models
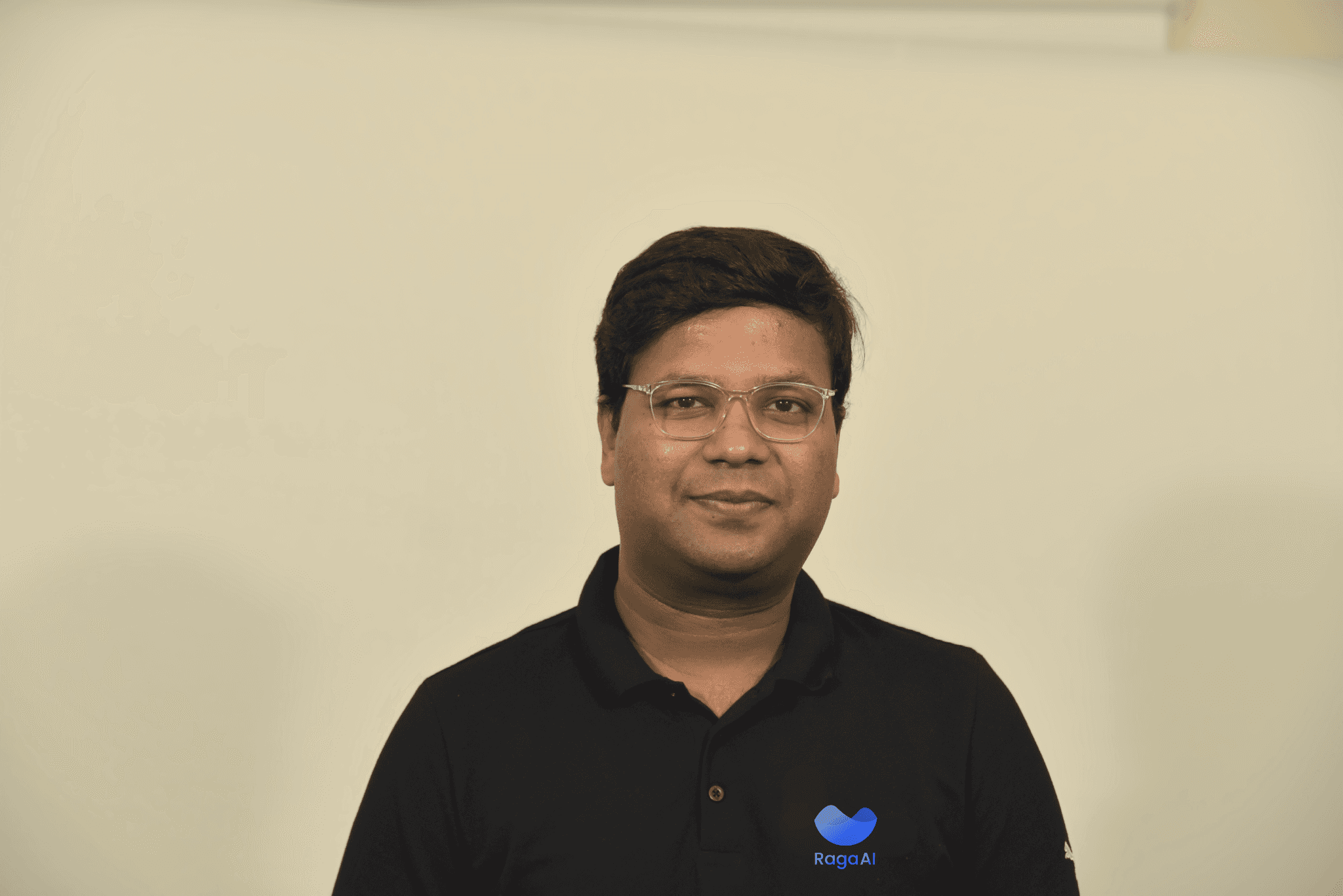
Jigar Gupta
Dec 30, 2024
Read the article
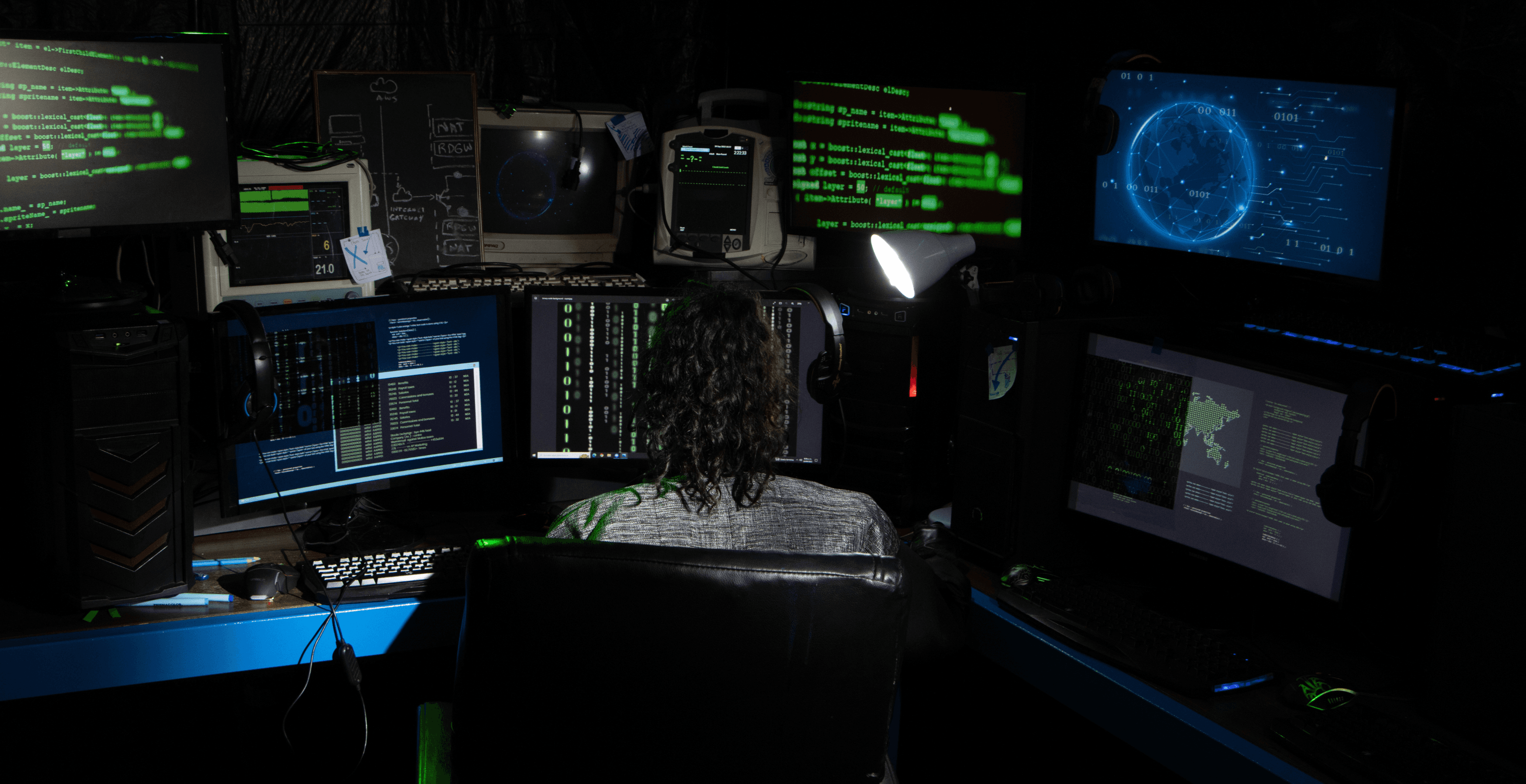
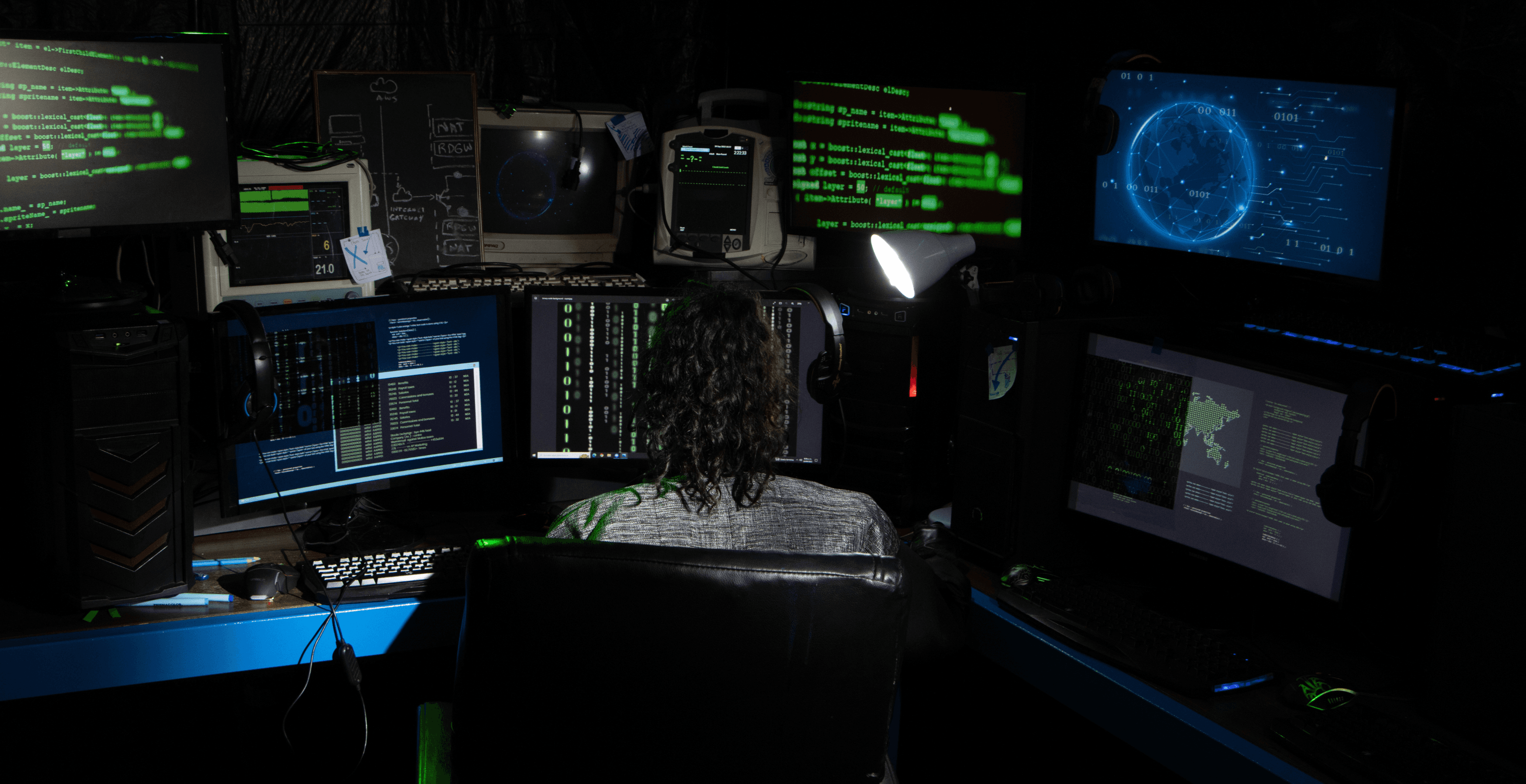
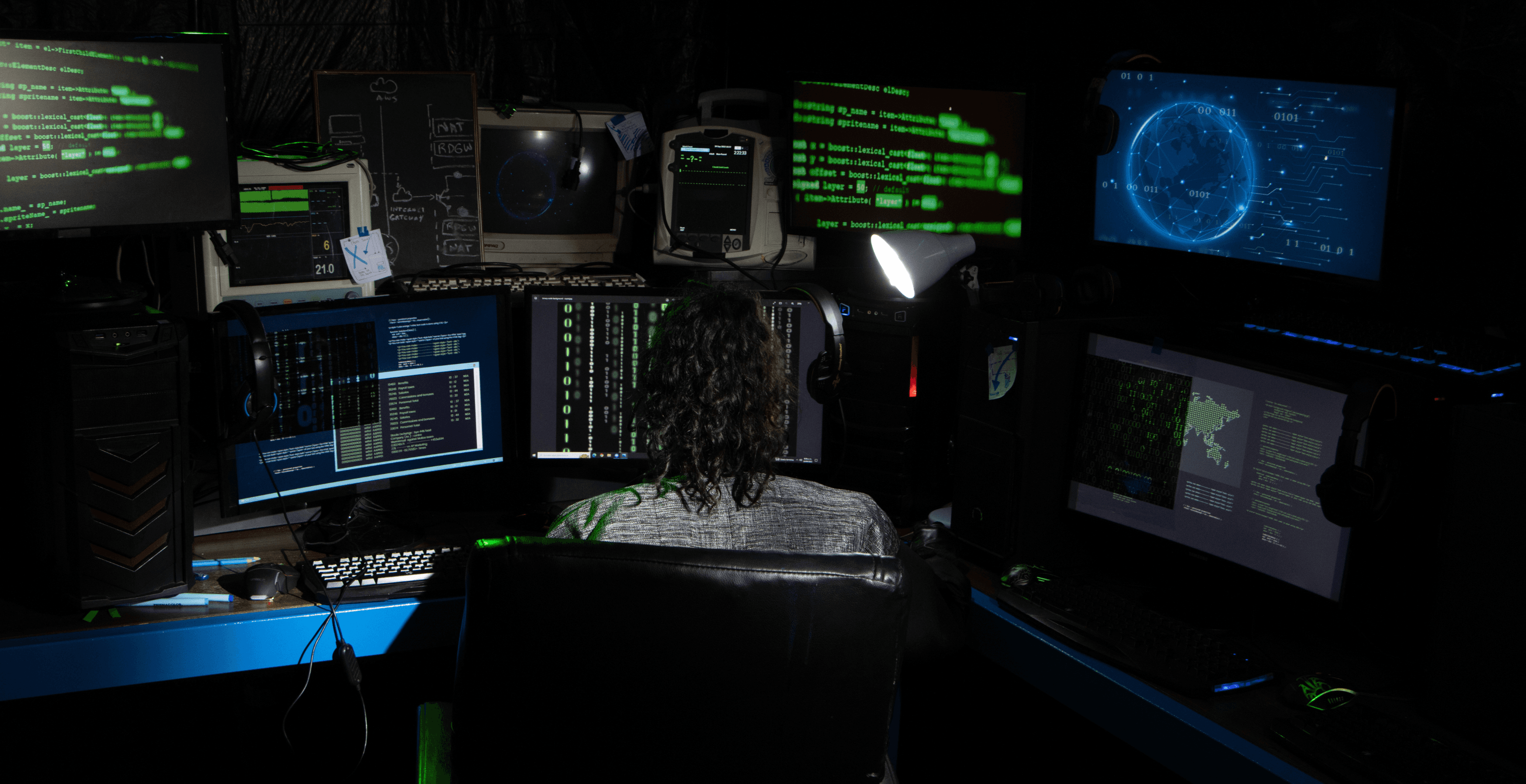
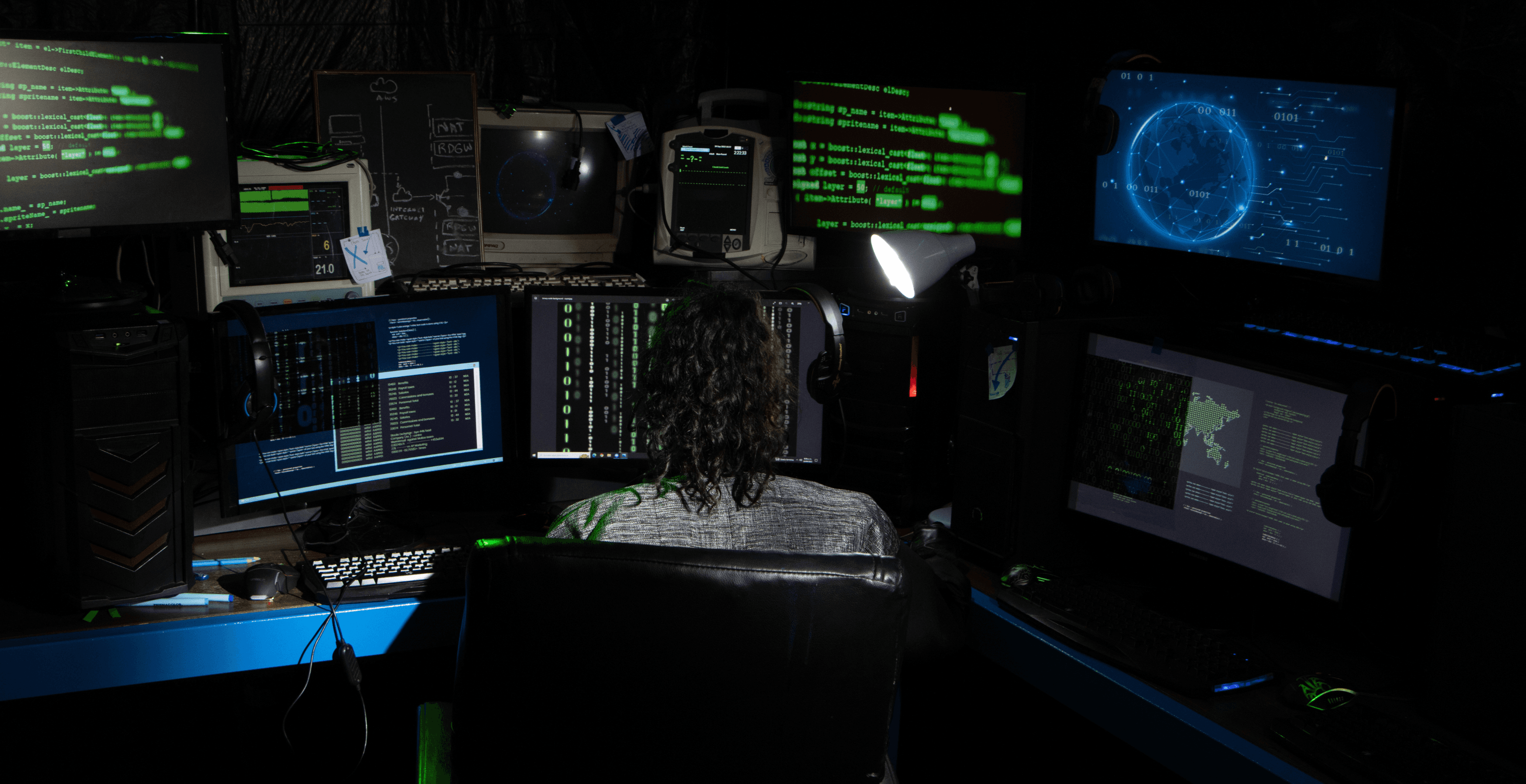
RAG vs Fine-Tuning: Choosing the Best AI Learning Technique
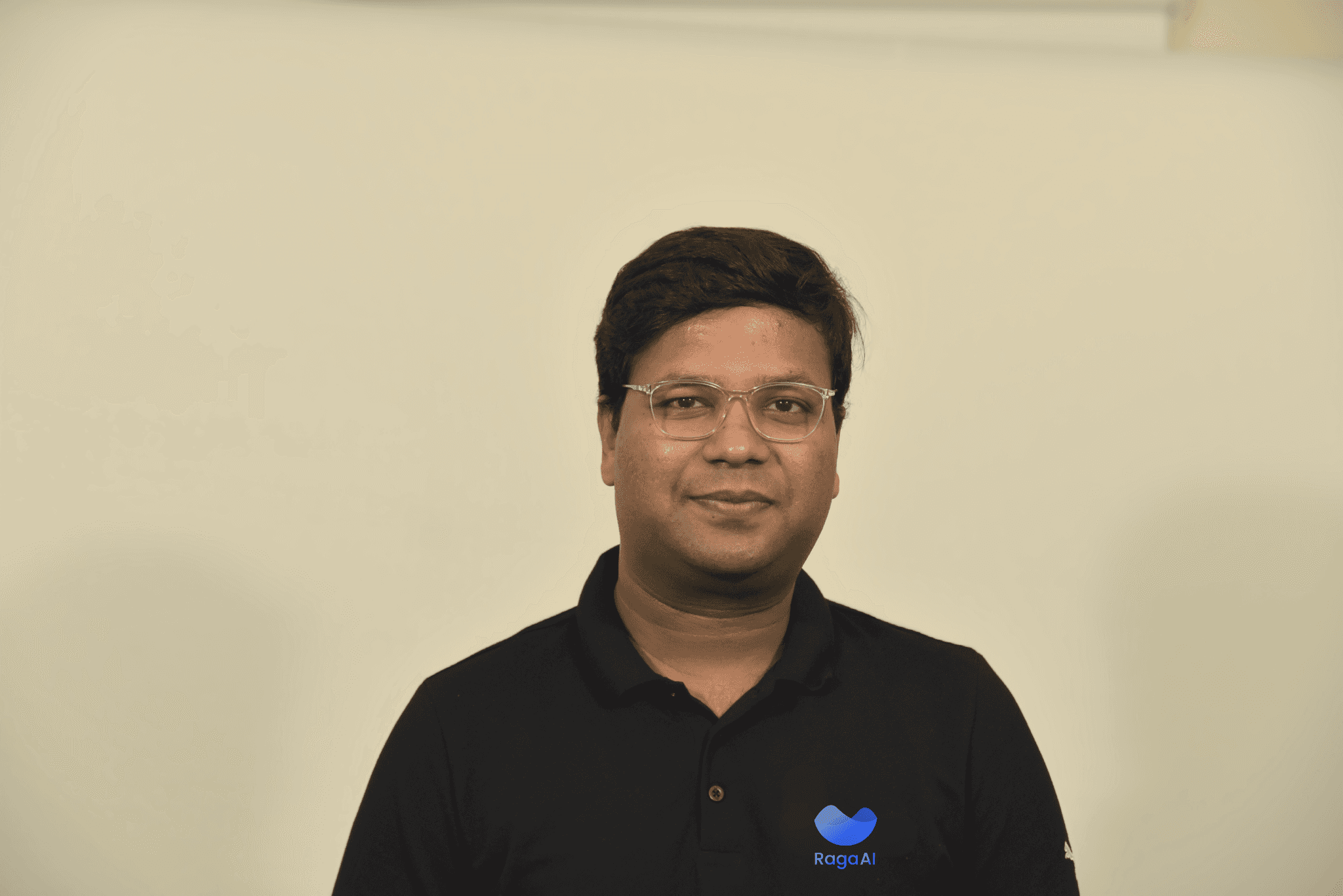
Jigar Gupta
Dec 27, 2024
Read the article
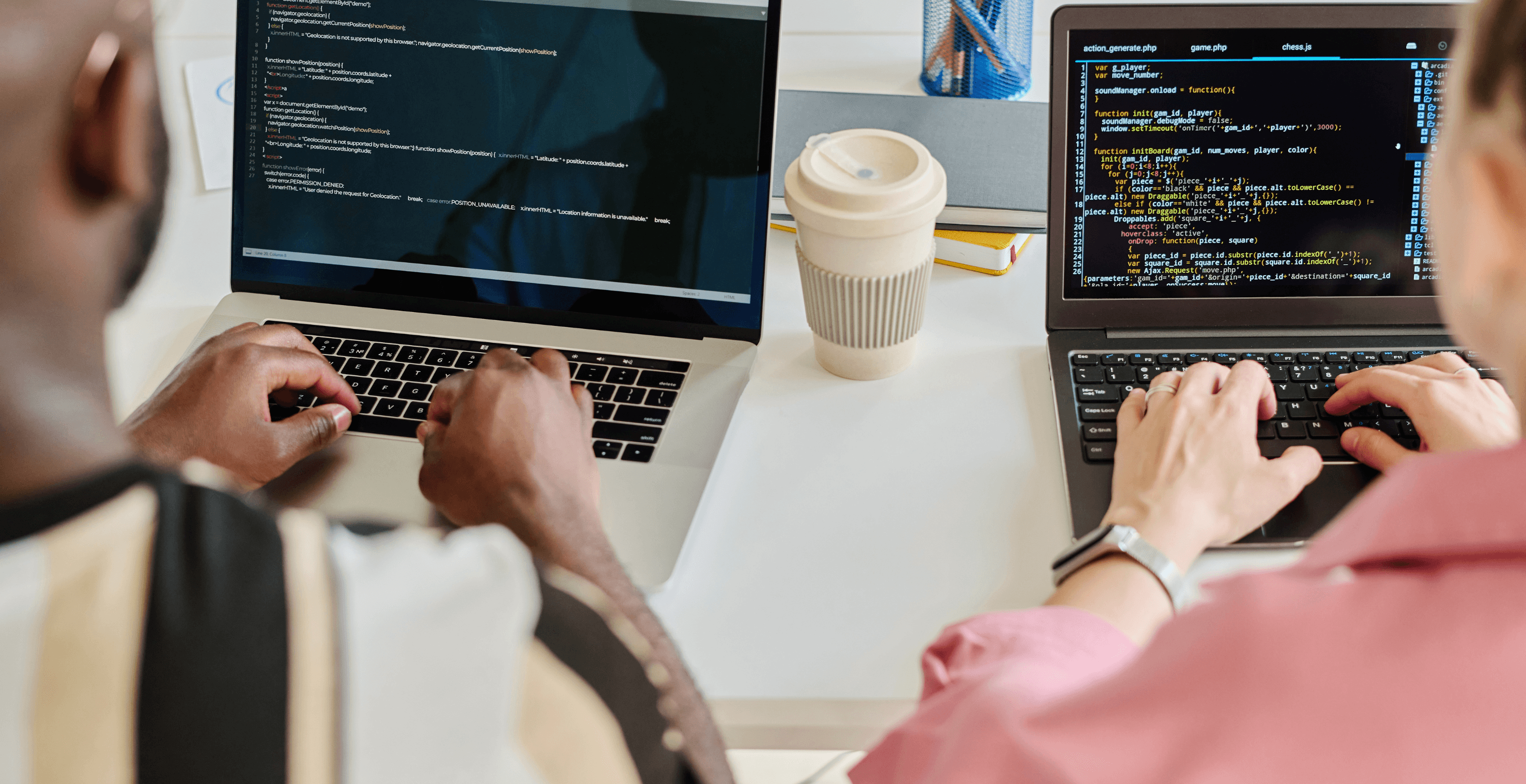
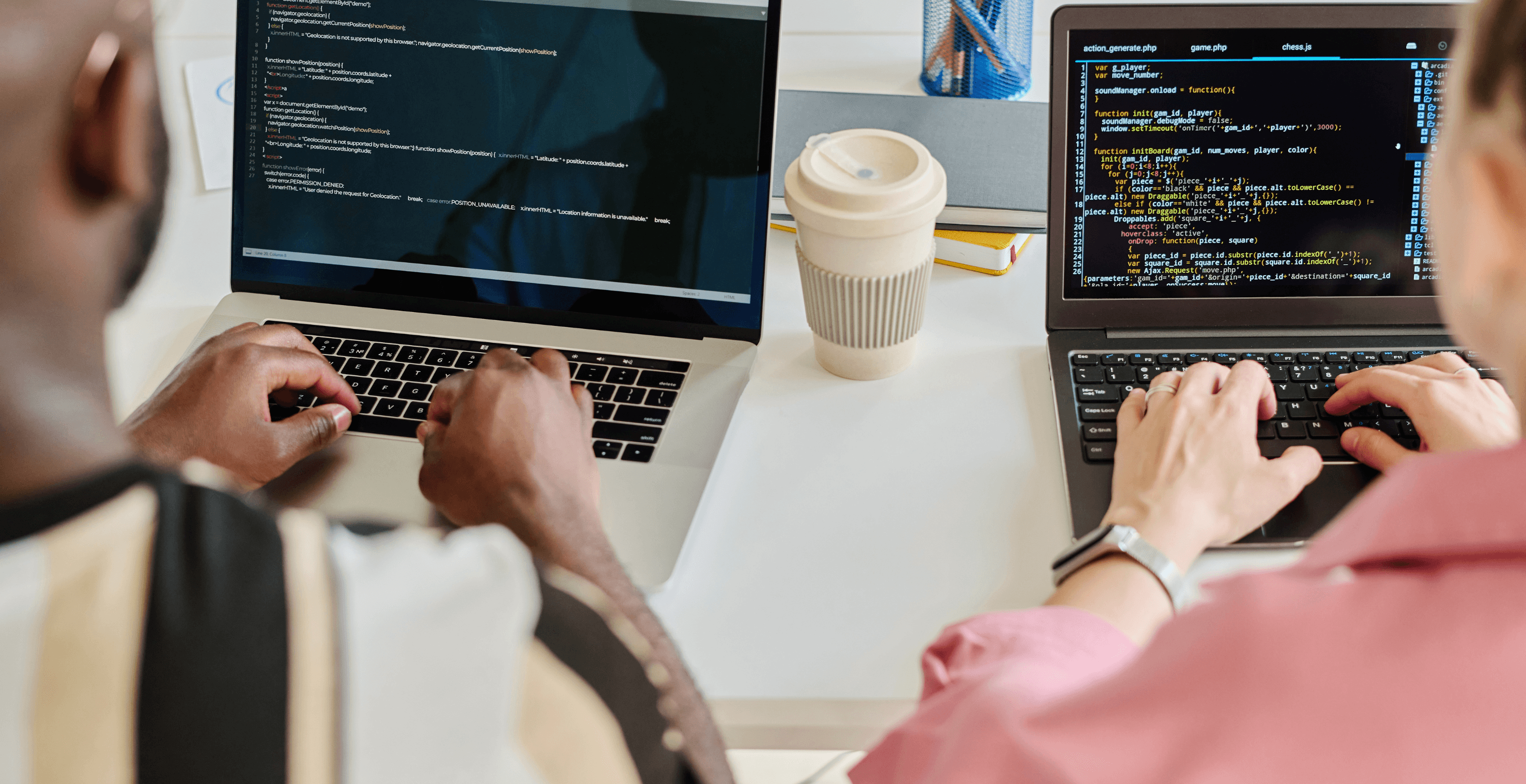
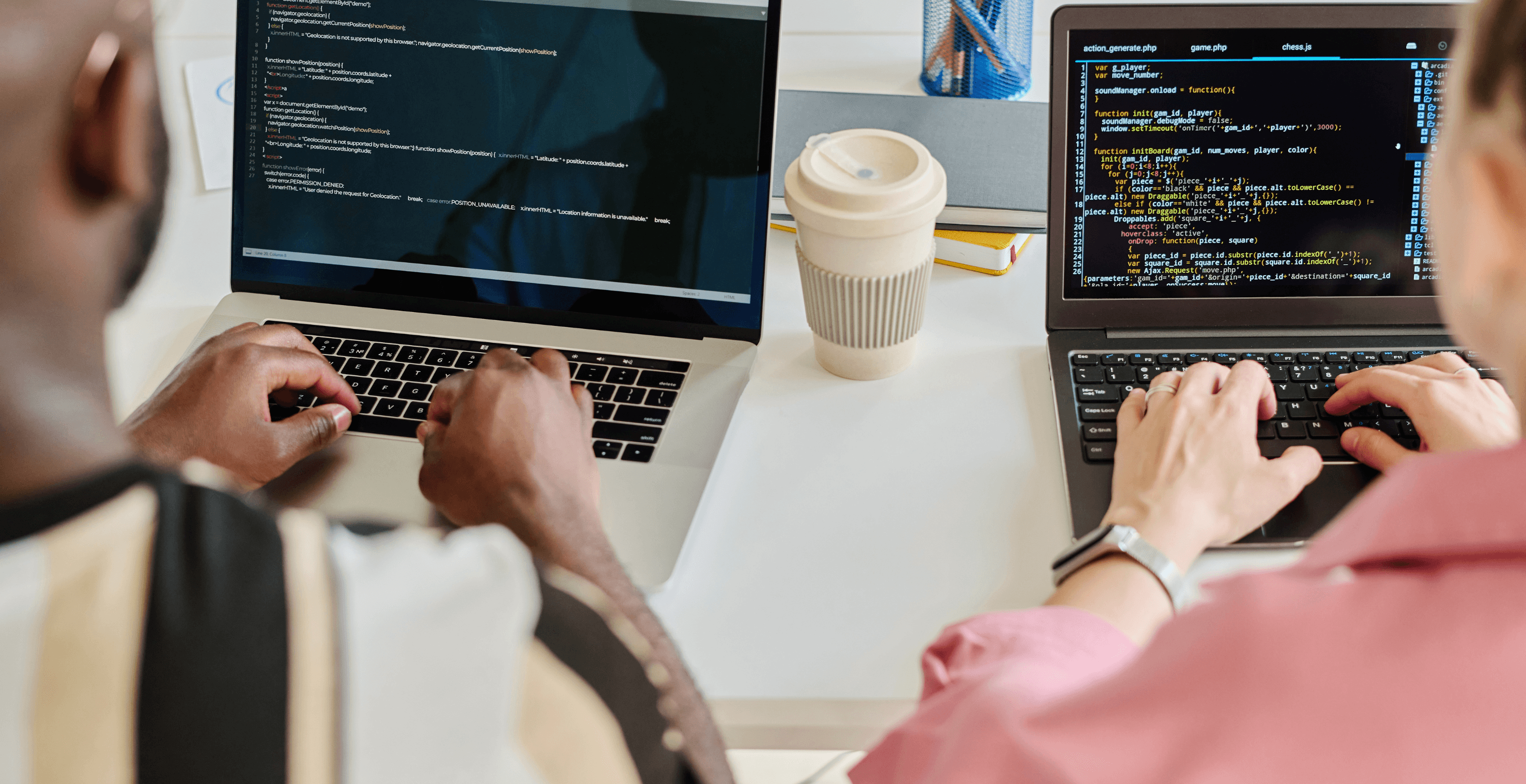
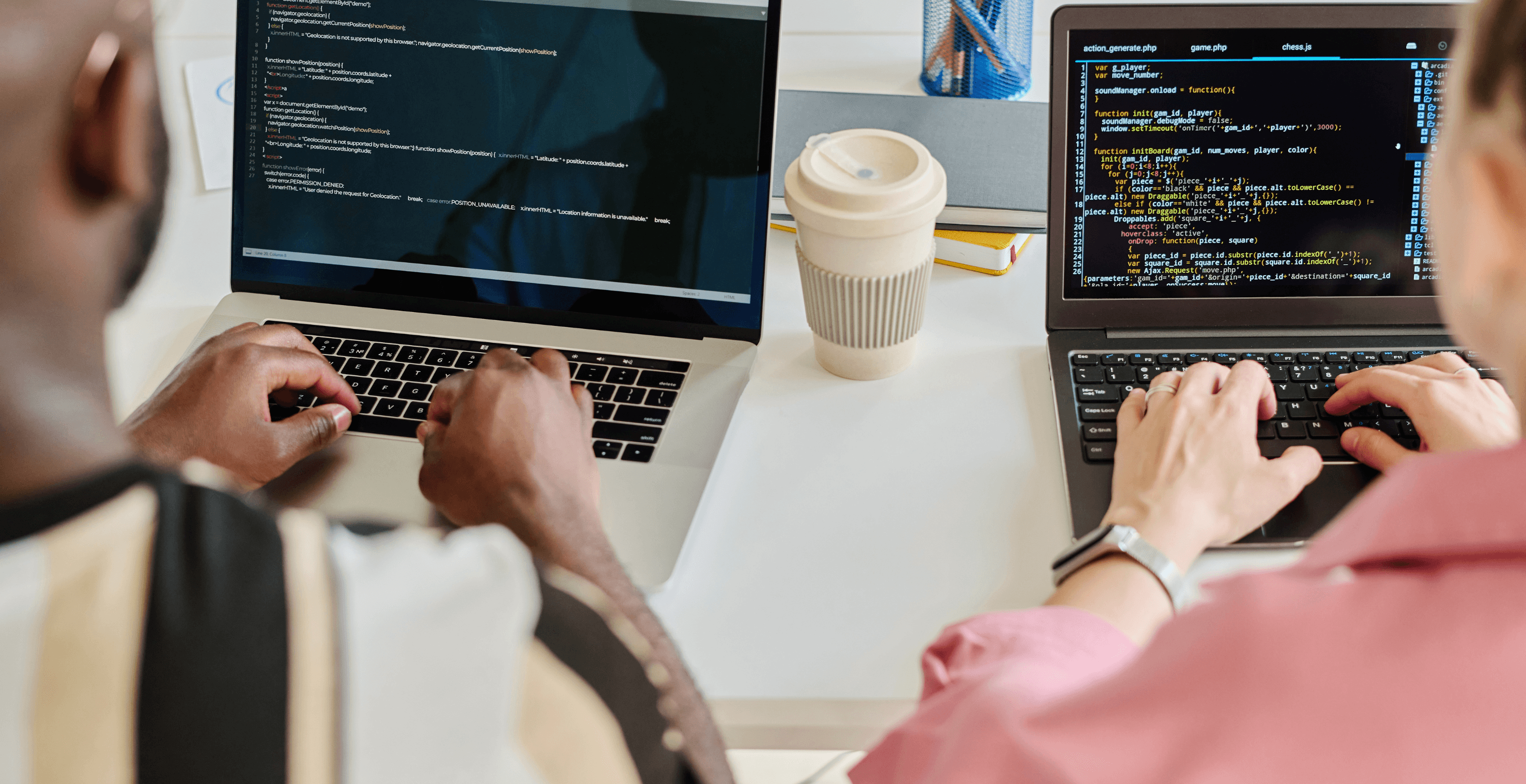
Understanding NeMo Guardrails: A Toolkit for LLM Security

Rehan Asif
Dec 24, 2024
Read the article




Understanding Differences in Large vs Small Language Models (LLM vs SLM)

Rehan Asif
Dec 21, 2024
Read the article




Understanding What an AI Agent is: Key Applications and Examples
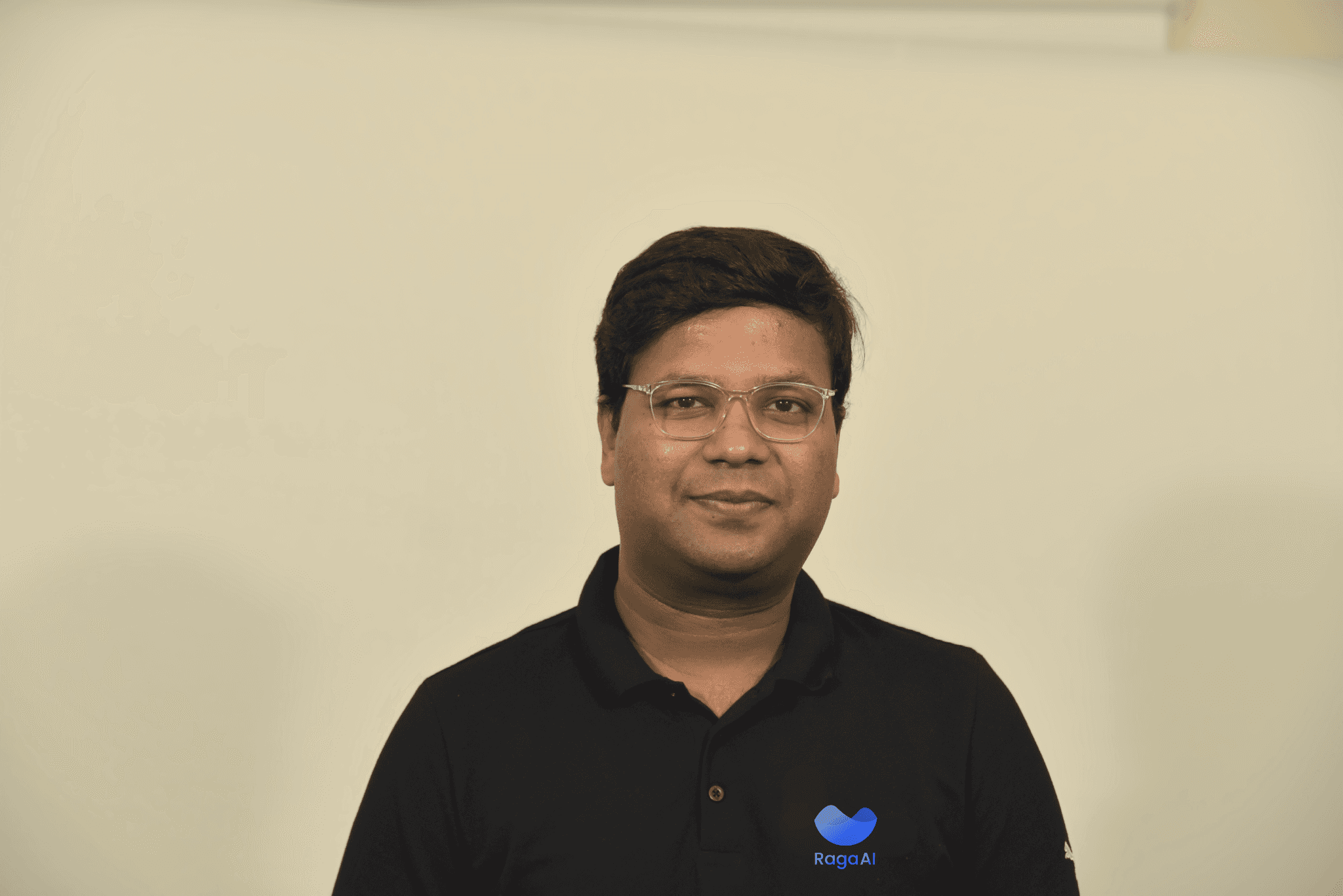
Jigar Gupta
Dec 17, 2024
Read the article




Prompt Engineering and Retrieval Augmented Generation (RAG)
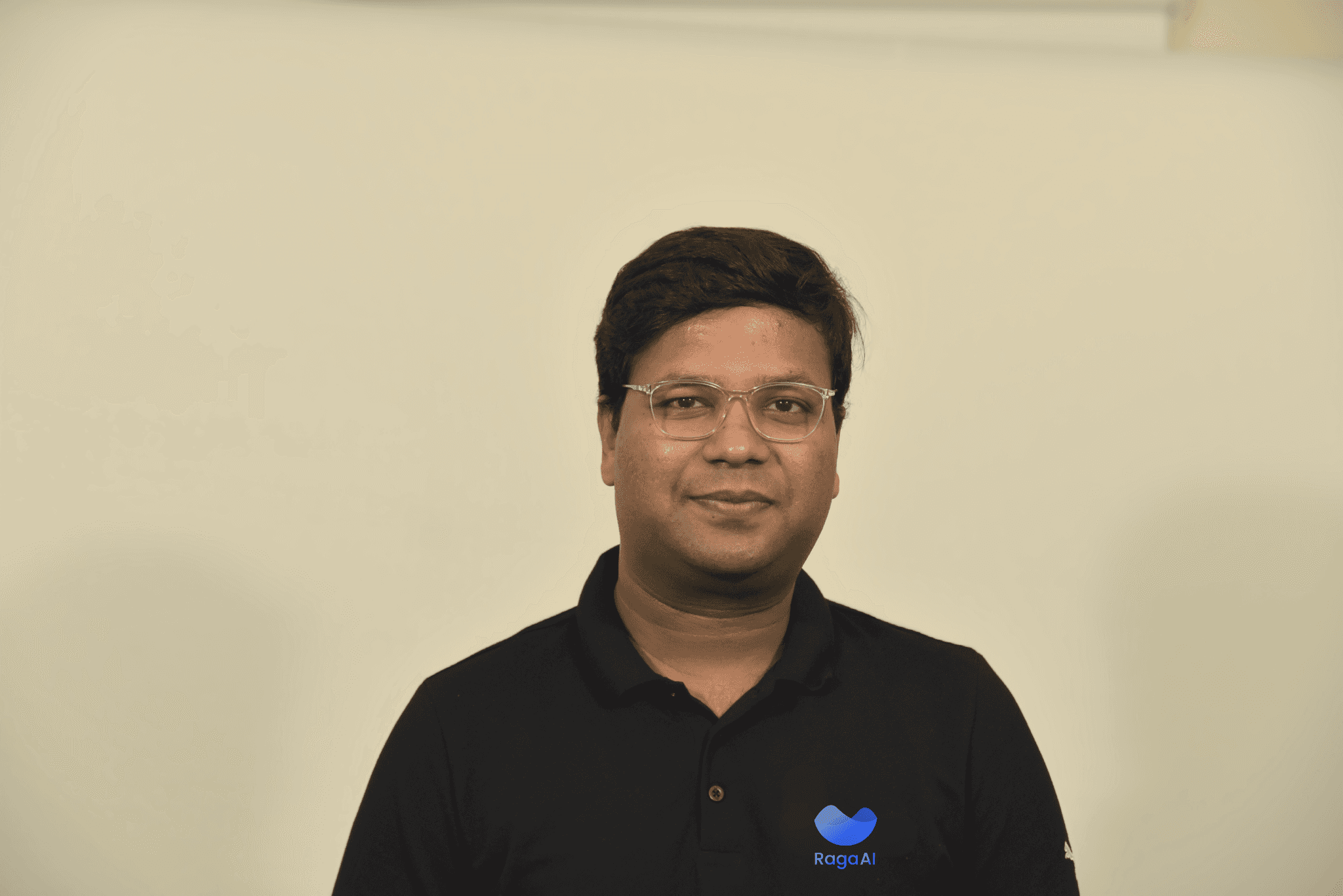
Jigar Gupta
Dec 12, 2024
Read the article




Exploring How Multimodal Large Language Models Work

Rehan Asif
Dec 9, 2024
Read the article
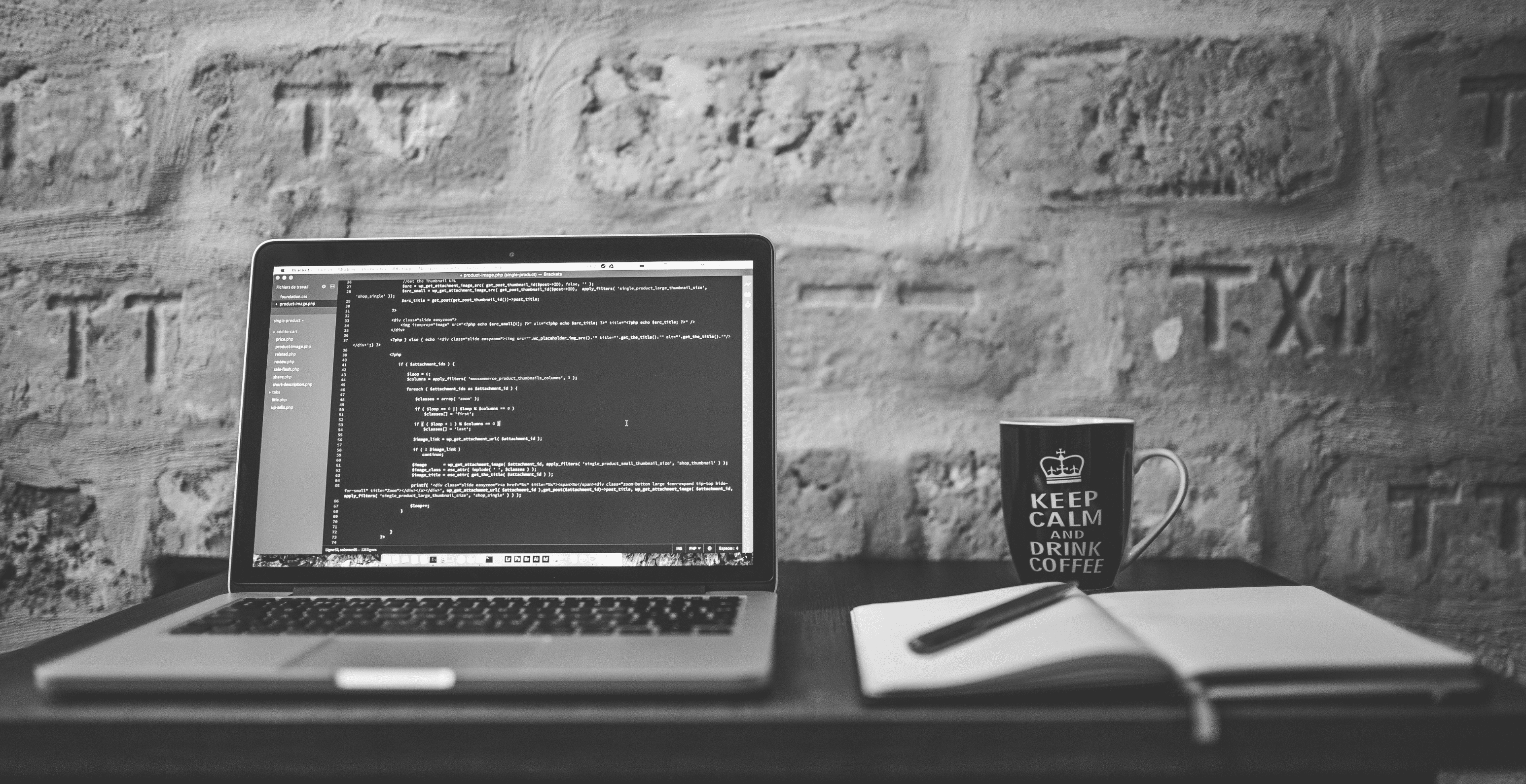
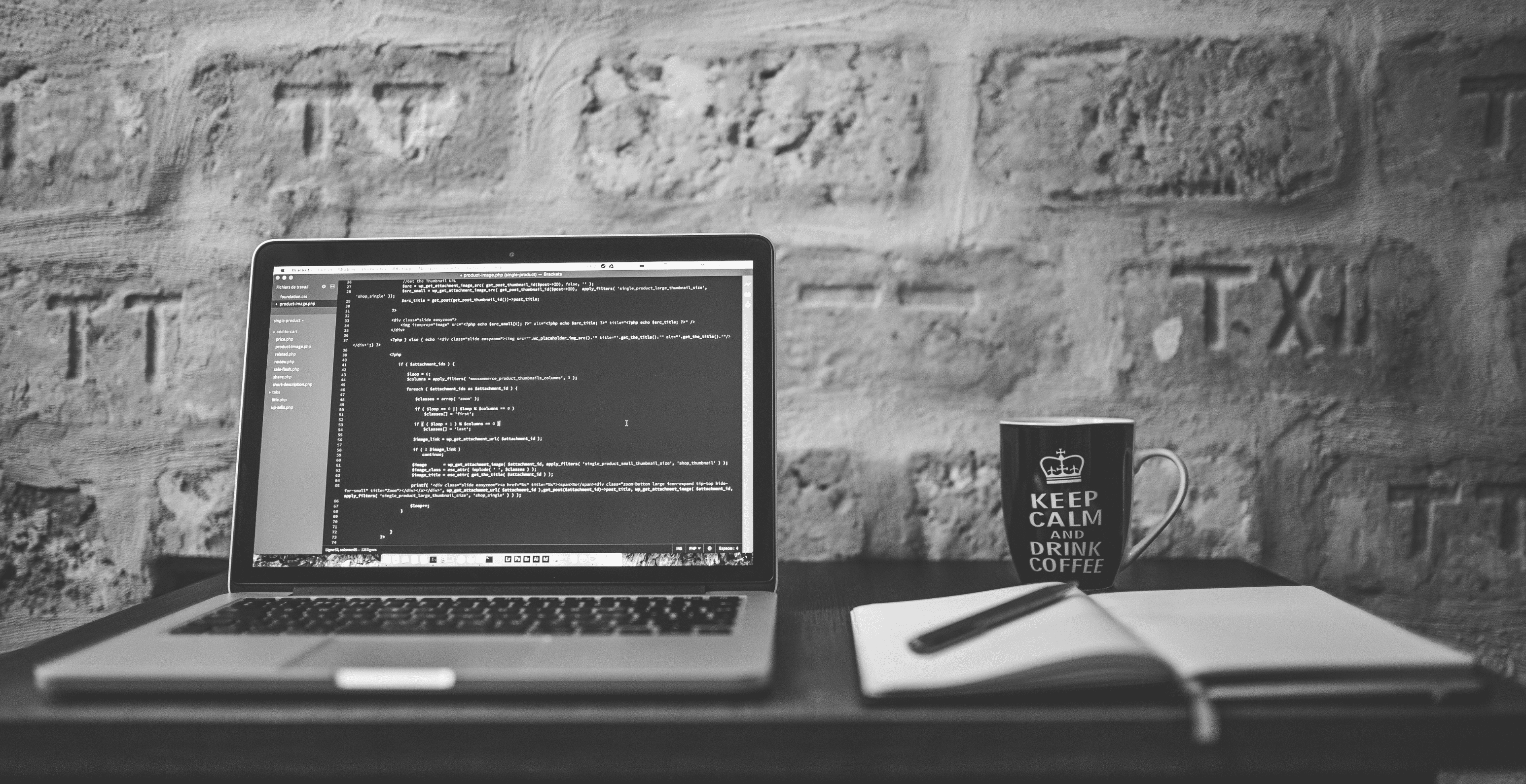
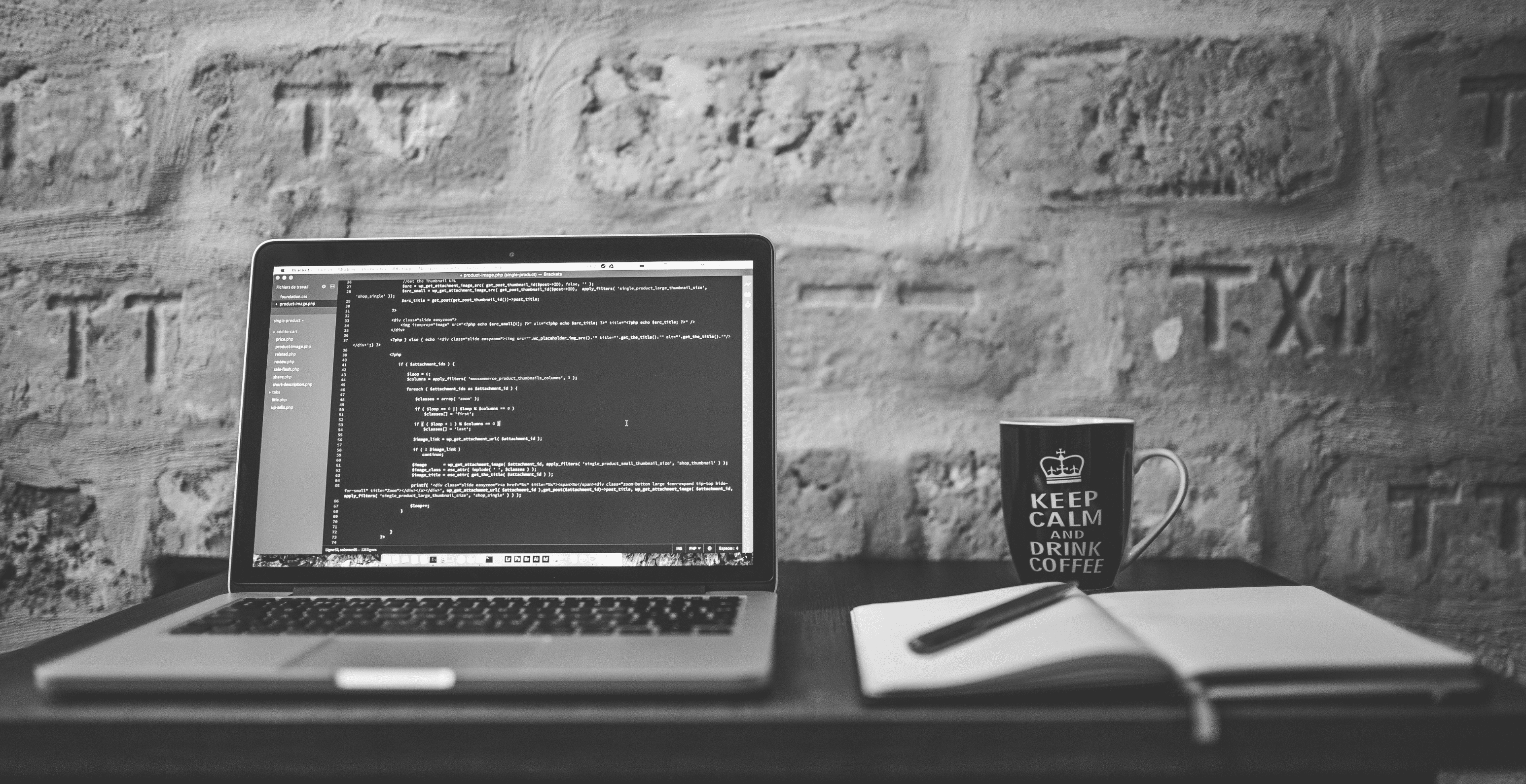
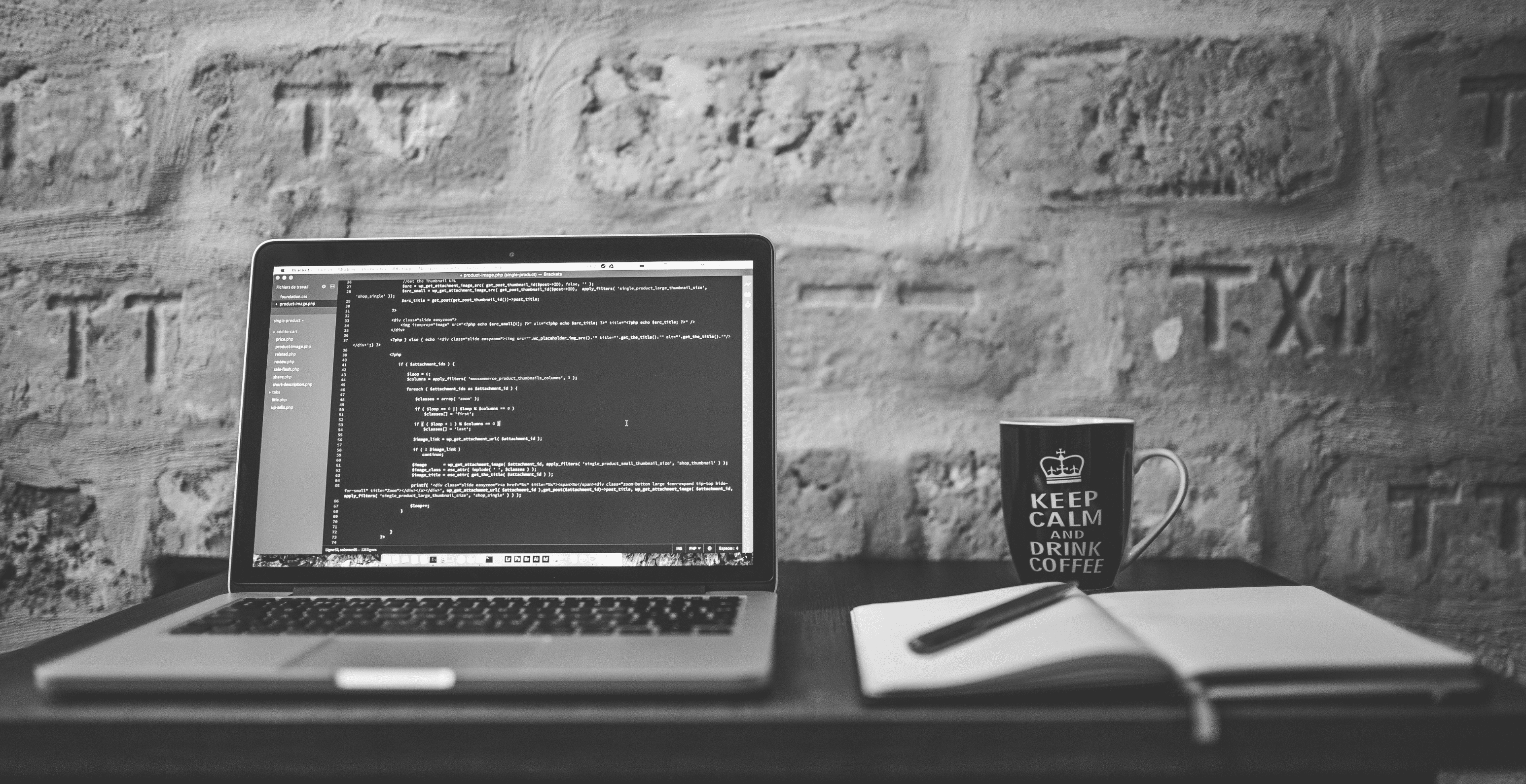
Evaluating and Enhancing LLM-as-a-Judge with Automated Tools

Rehan Asif
Dec 6, 2024
Read the article




Optimizing Performance and Cost by Caching LLM Queries

Rehan Asif
Dec 3, 2024
Read the article




LoRA vs RAG: Full Model Fine-Tuning in Large Language Models
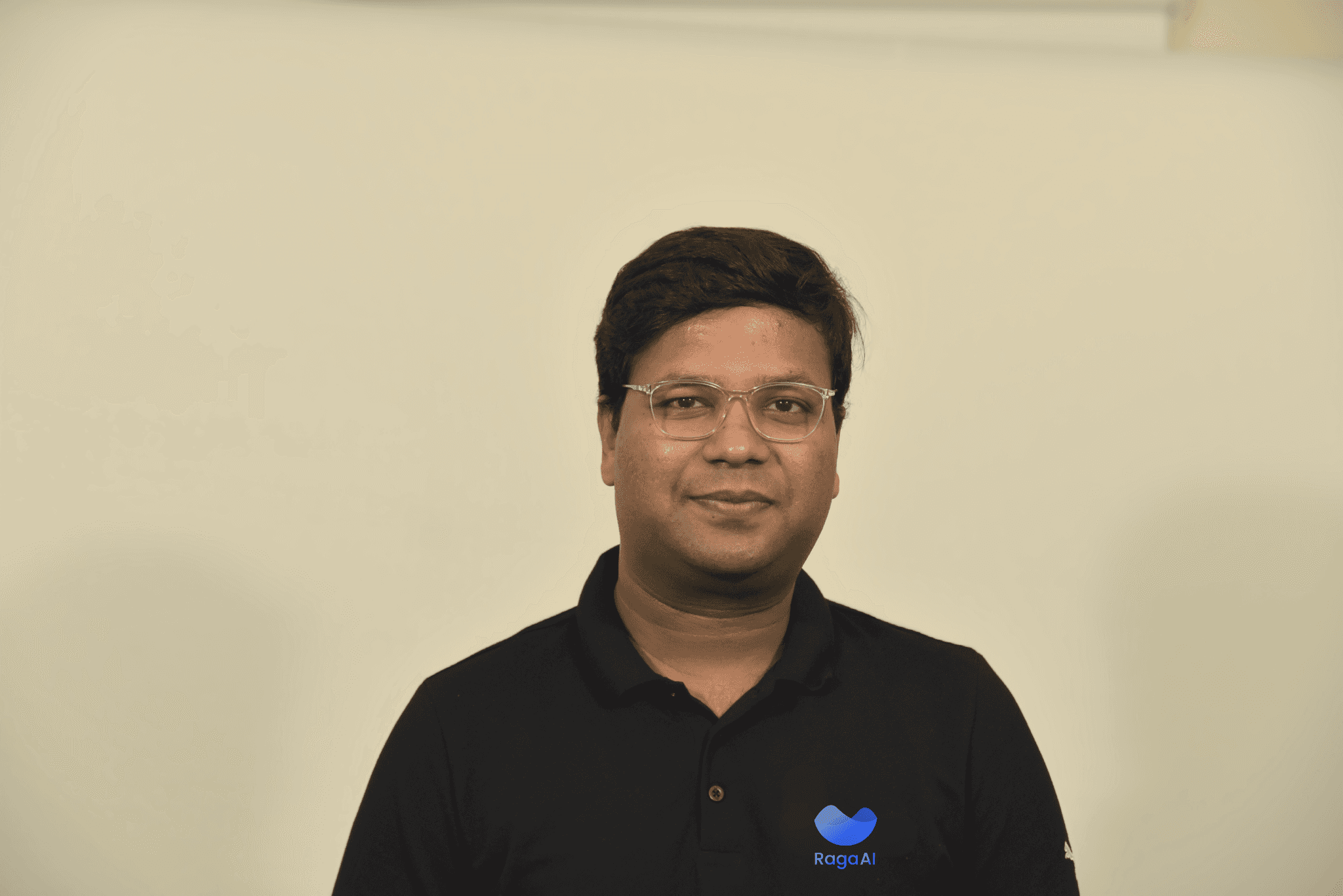
Jigar Gupta
Nov 30, 2024
Read the article
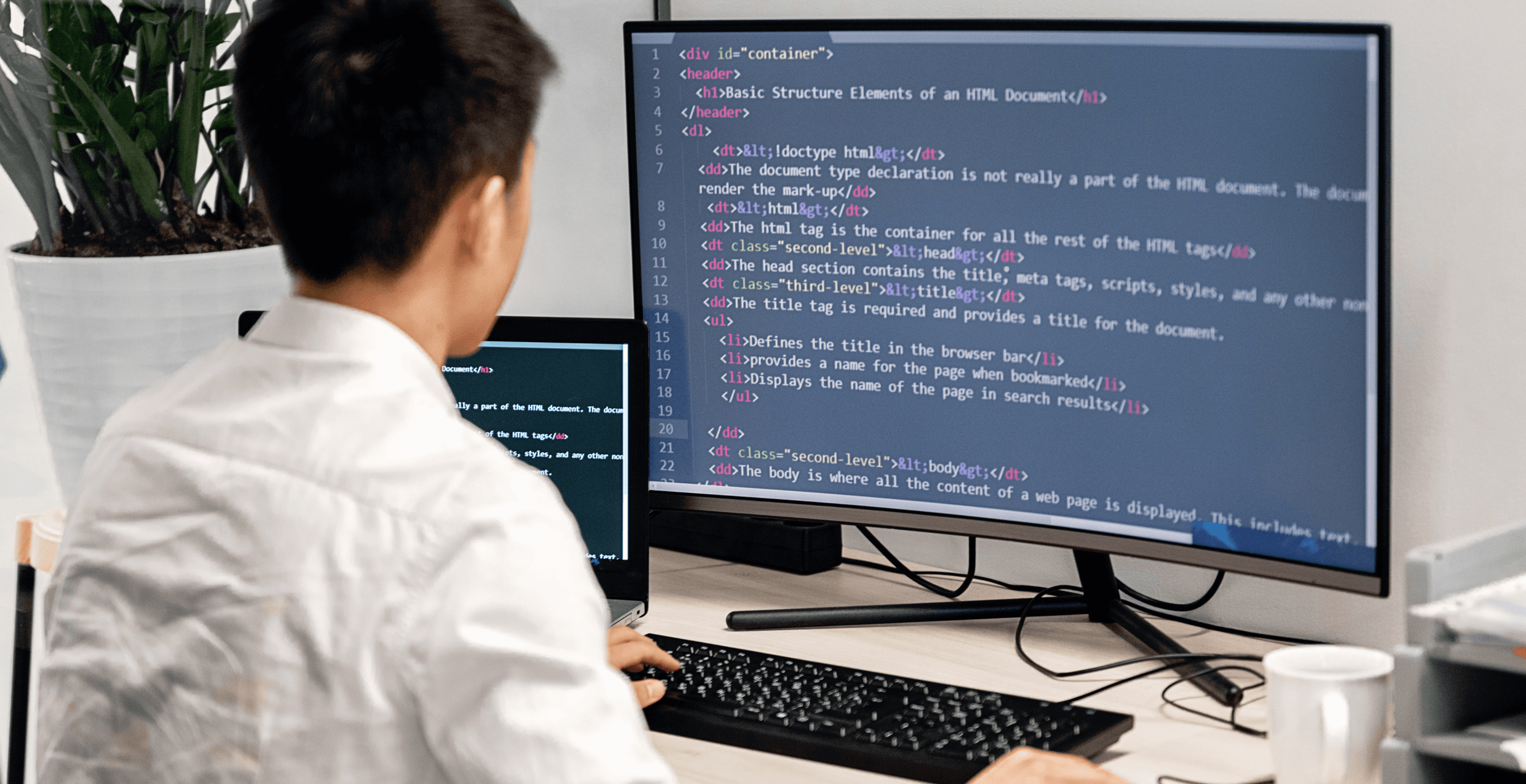
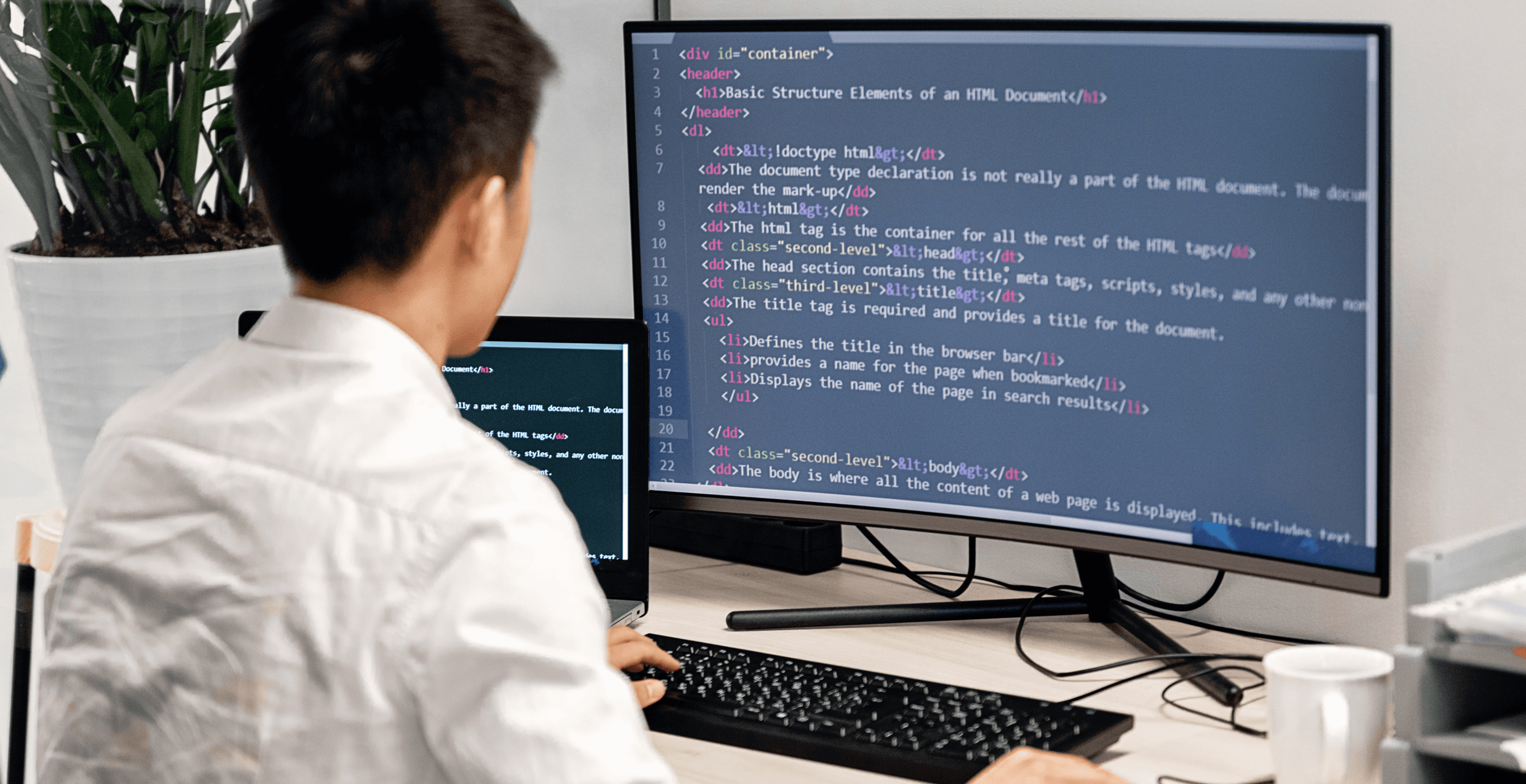
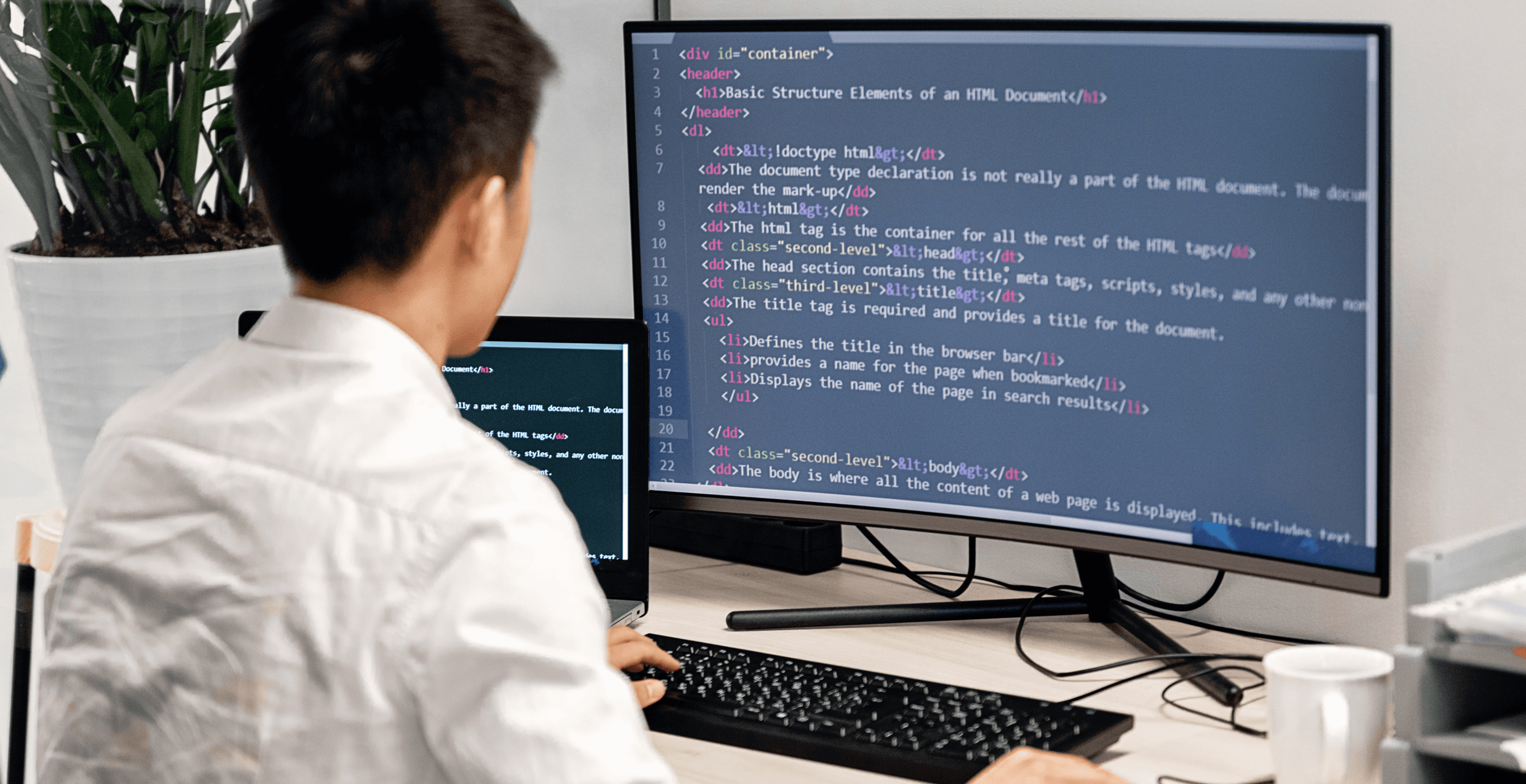
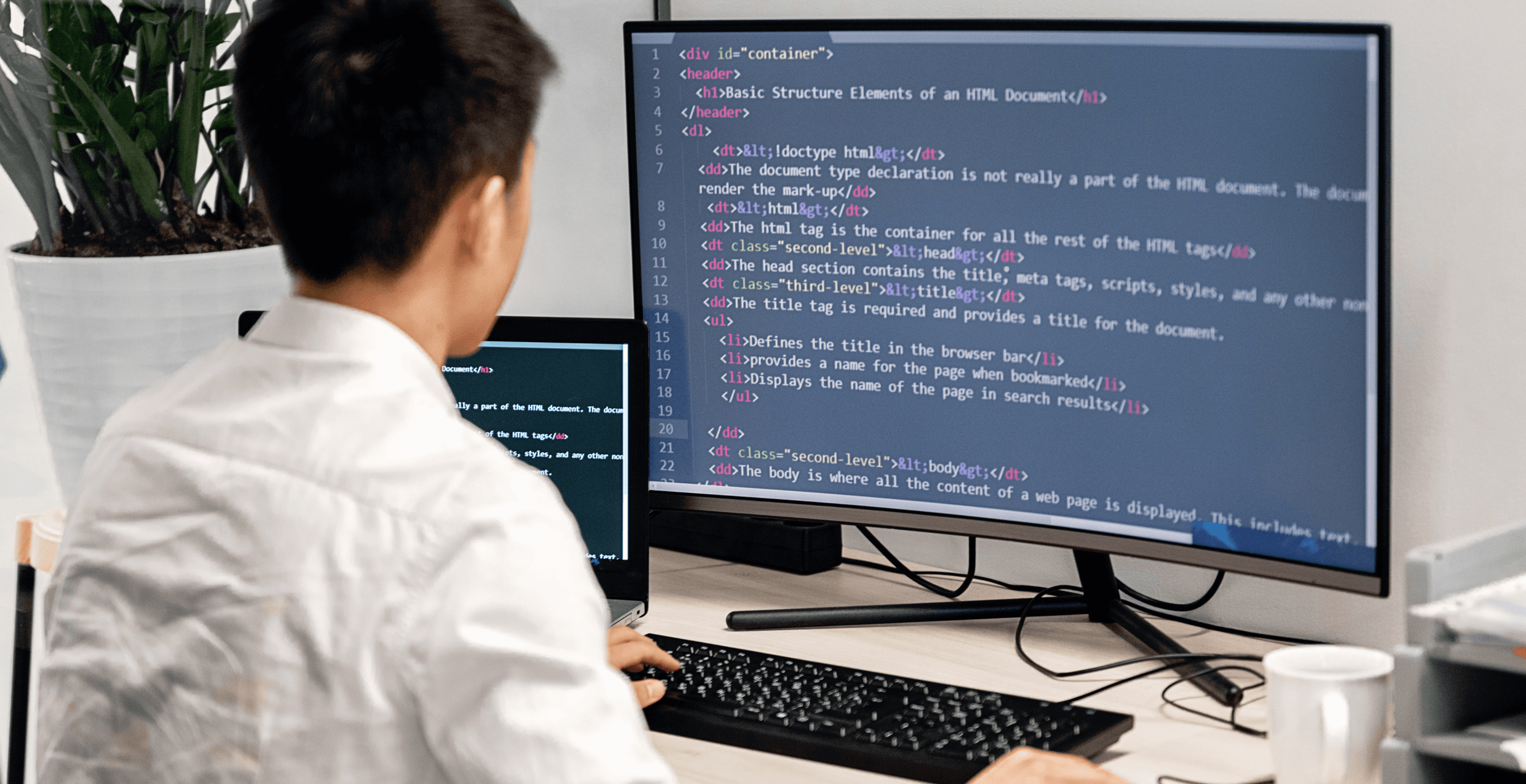
Steps to Train LLM on Personal Data

Rehan Asif
Nov 28, 2024
Read the article




Step by Step Guide to Building RAG-based LLM Applications with Examples

Rehan Asif
Nov 27, 2024
Read the article
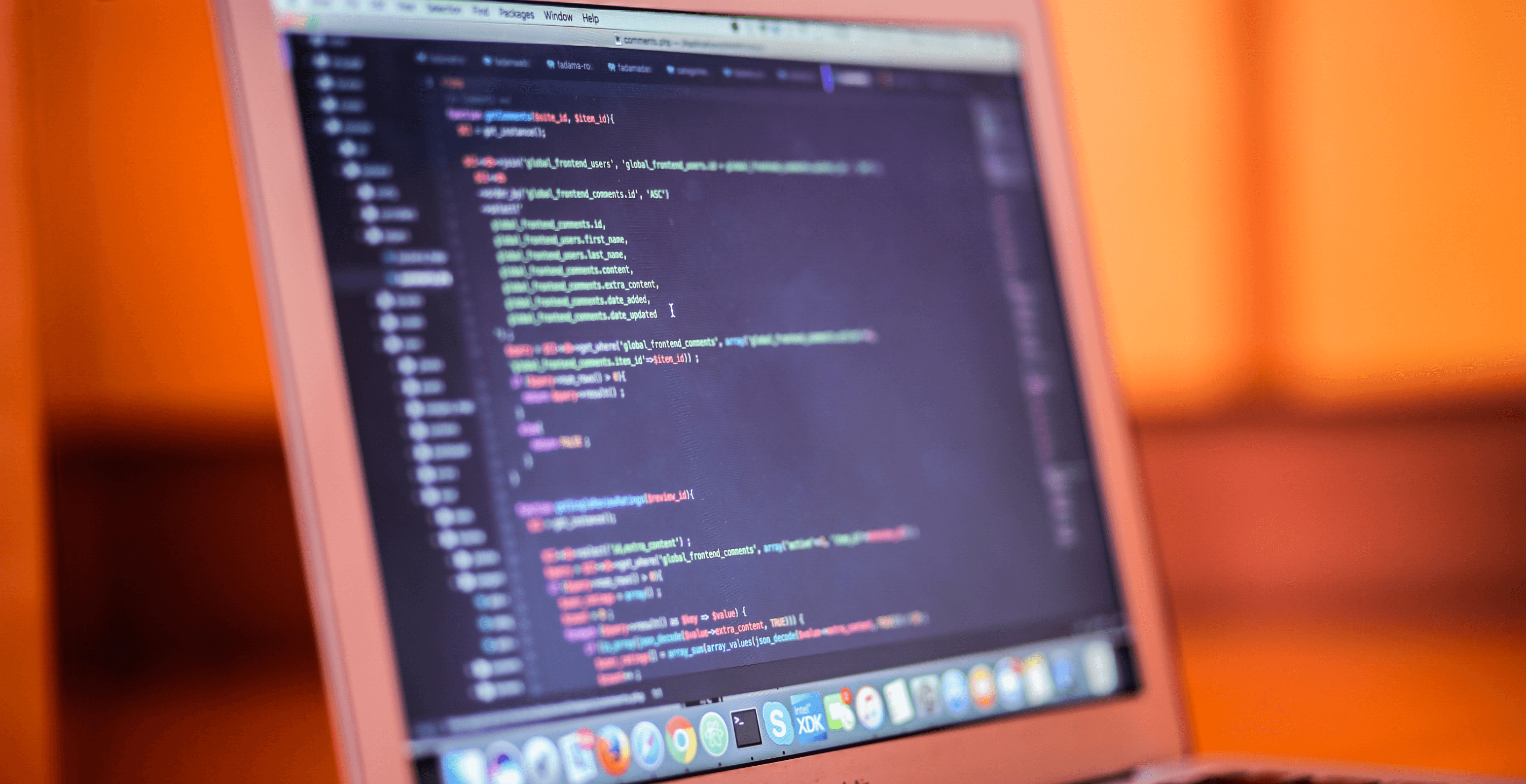
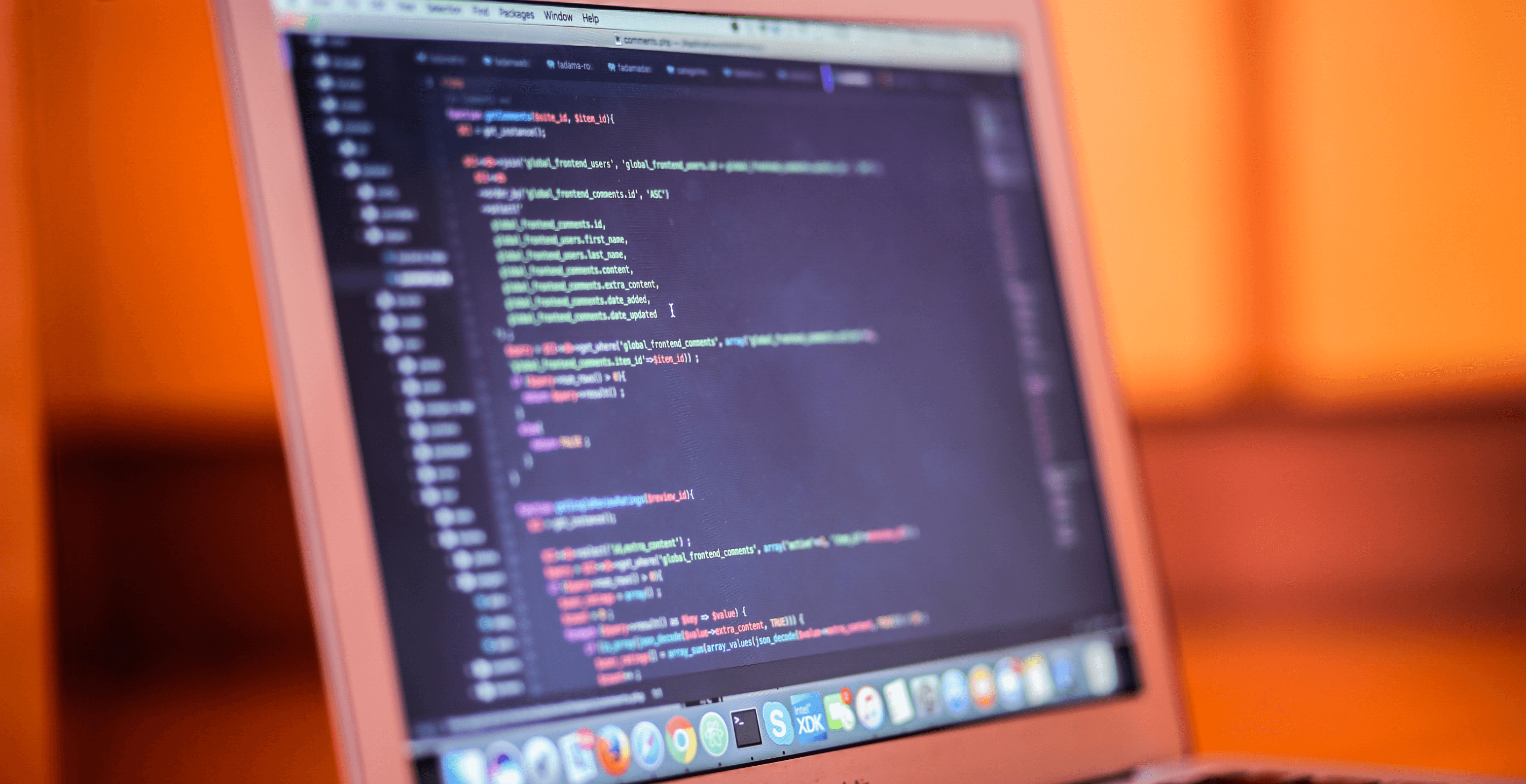
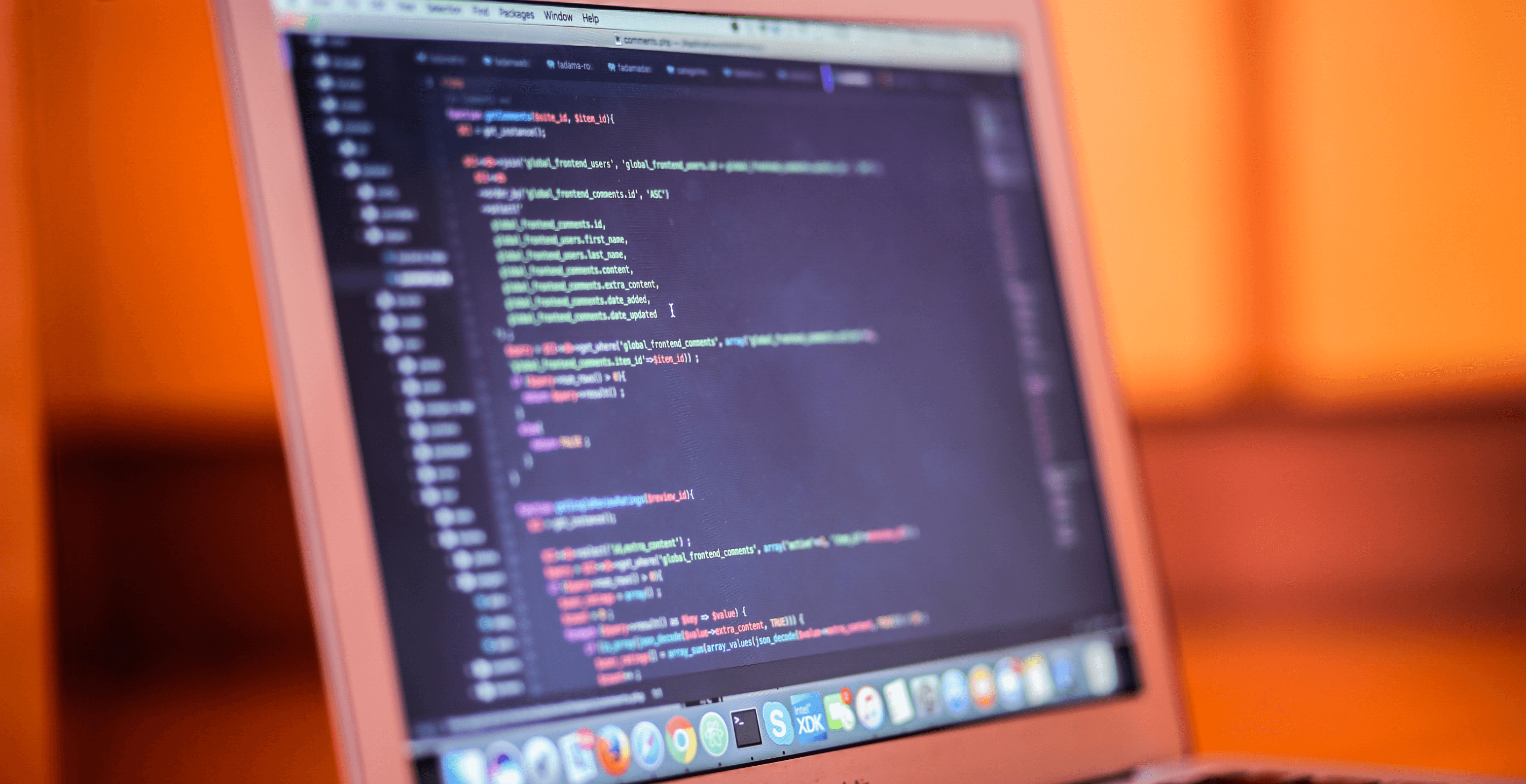
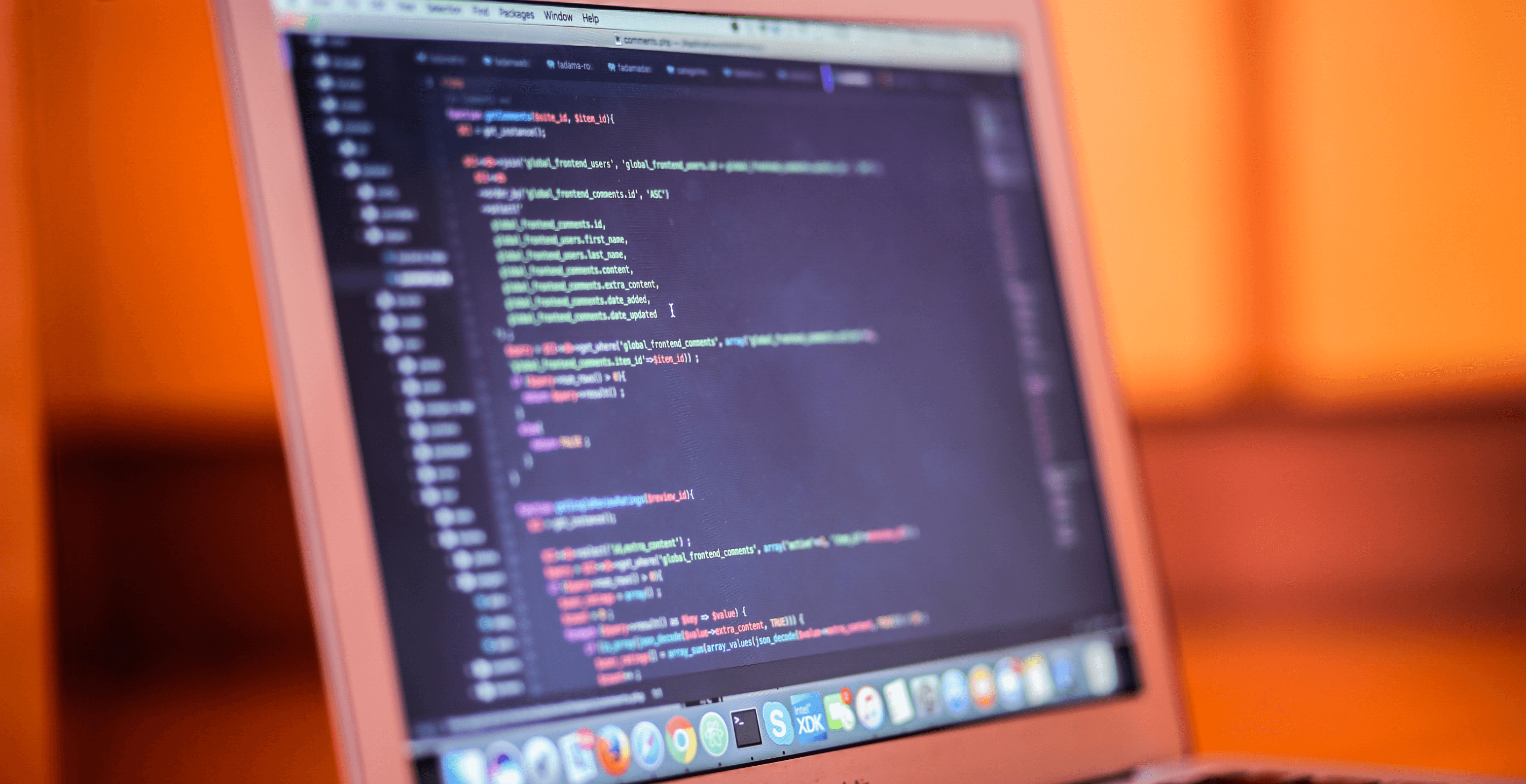
Building AI Agentic Workflows with Multi-Agent Collaboration
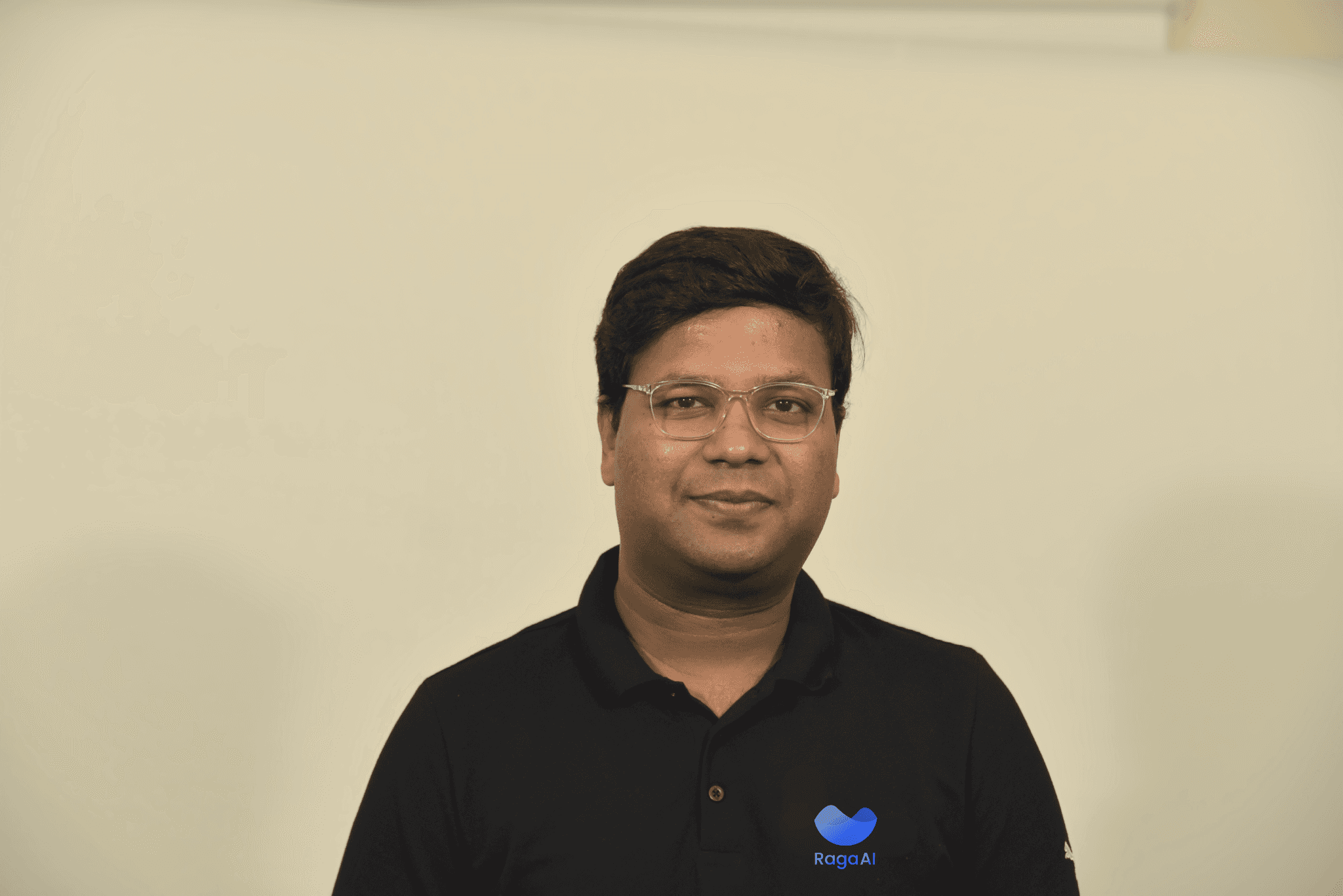
Jigar Gupta
Nov 25, 2024
Read the article




Top Large Language Models (LLMs) in 2024

Rehan Asif
Nov 22, 2024
Read the article
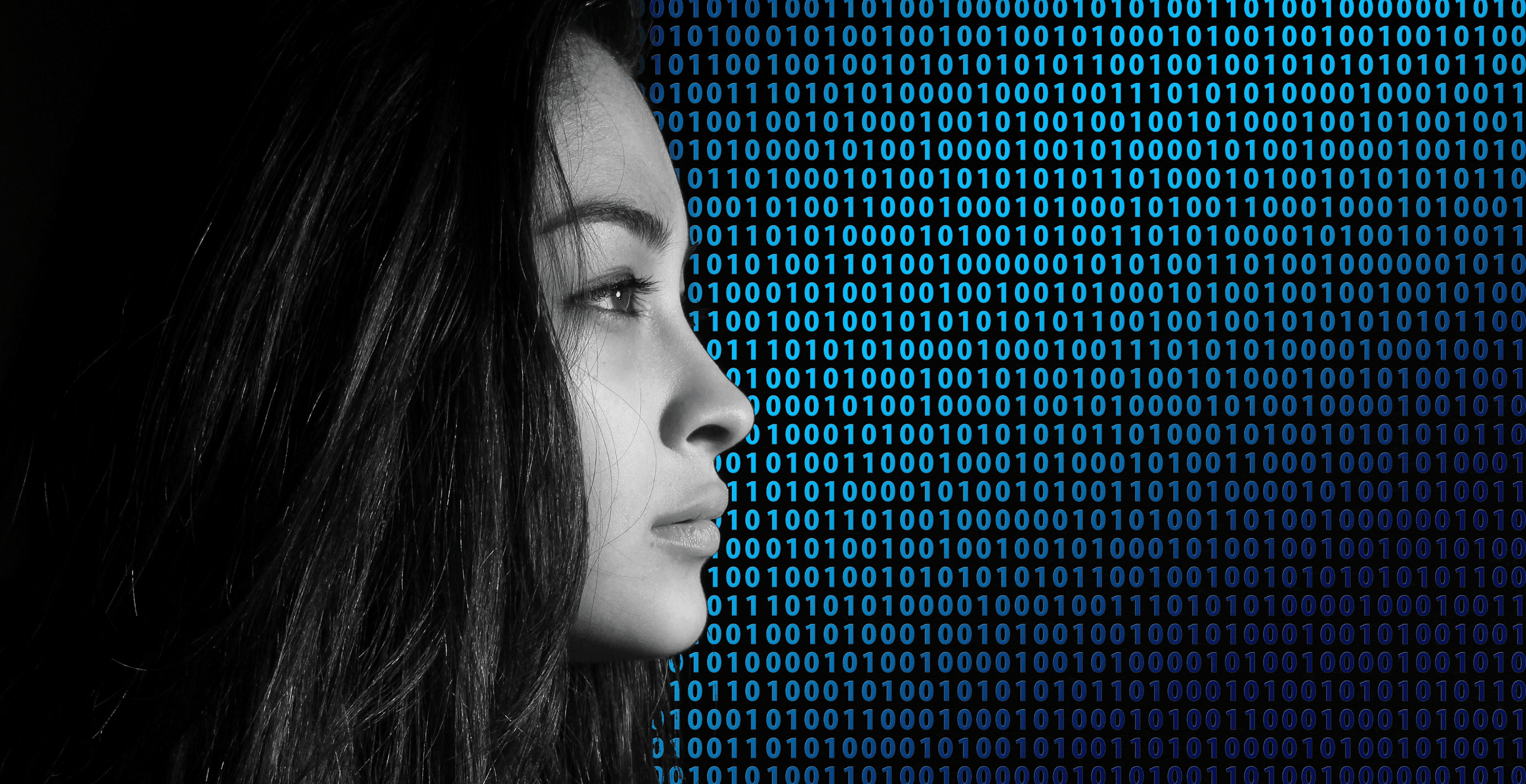
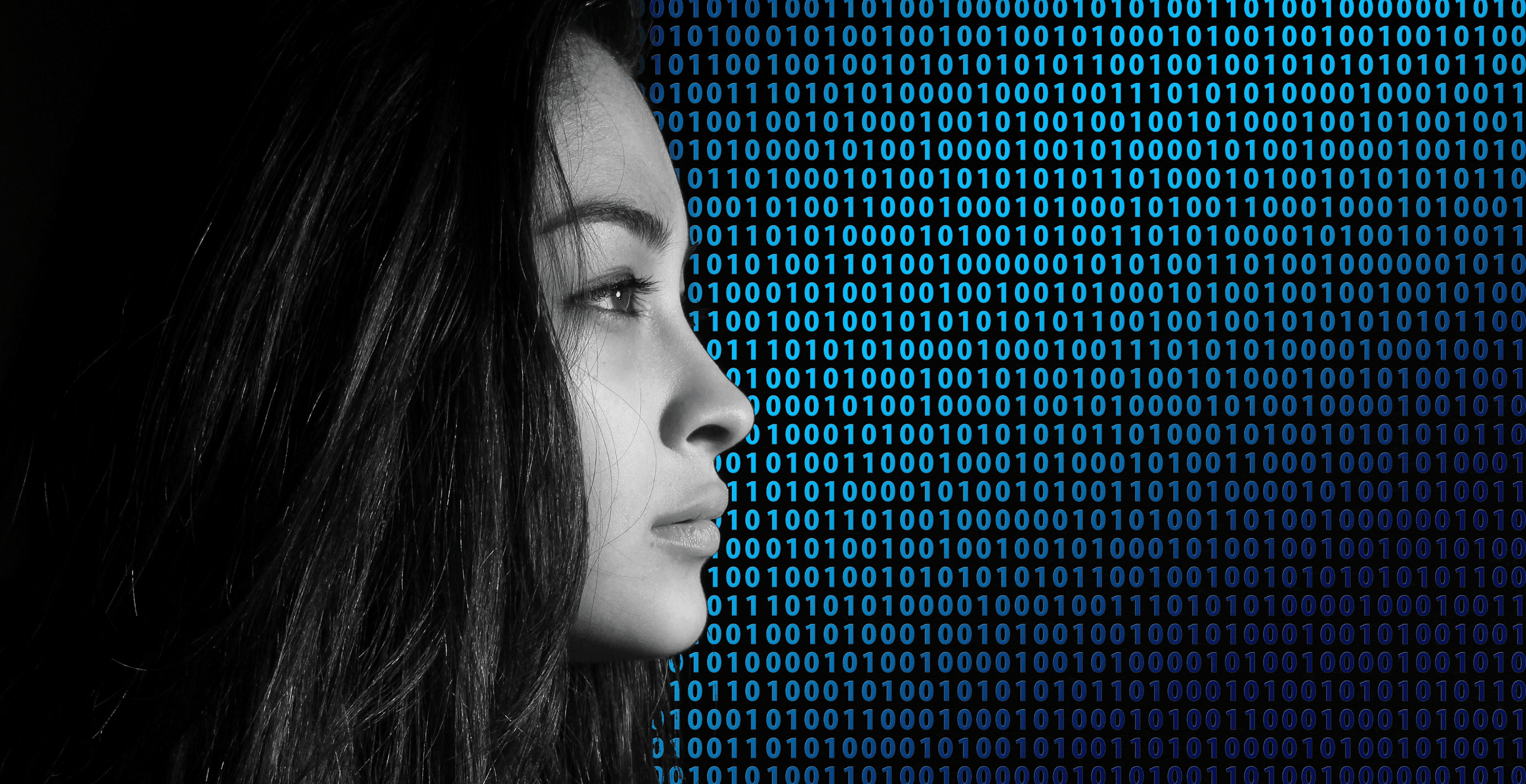
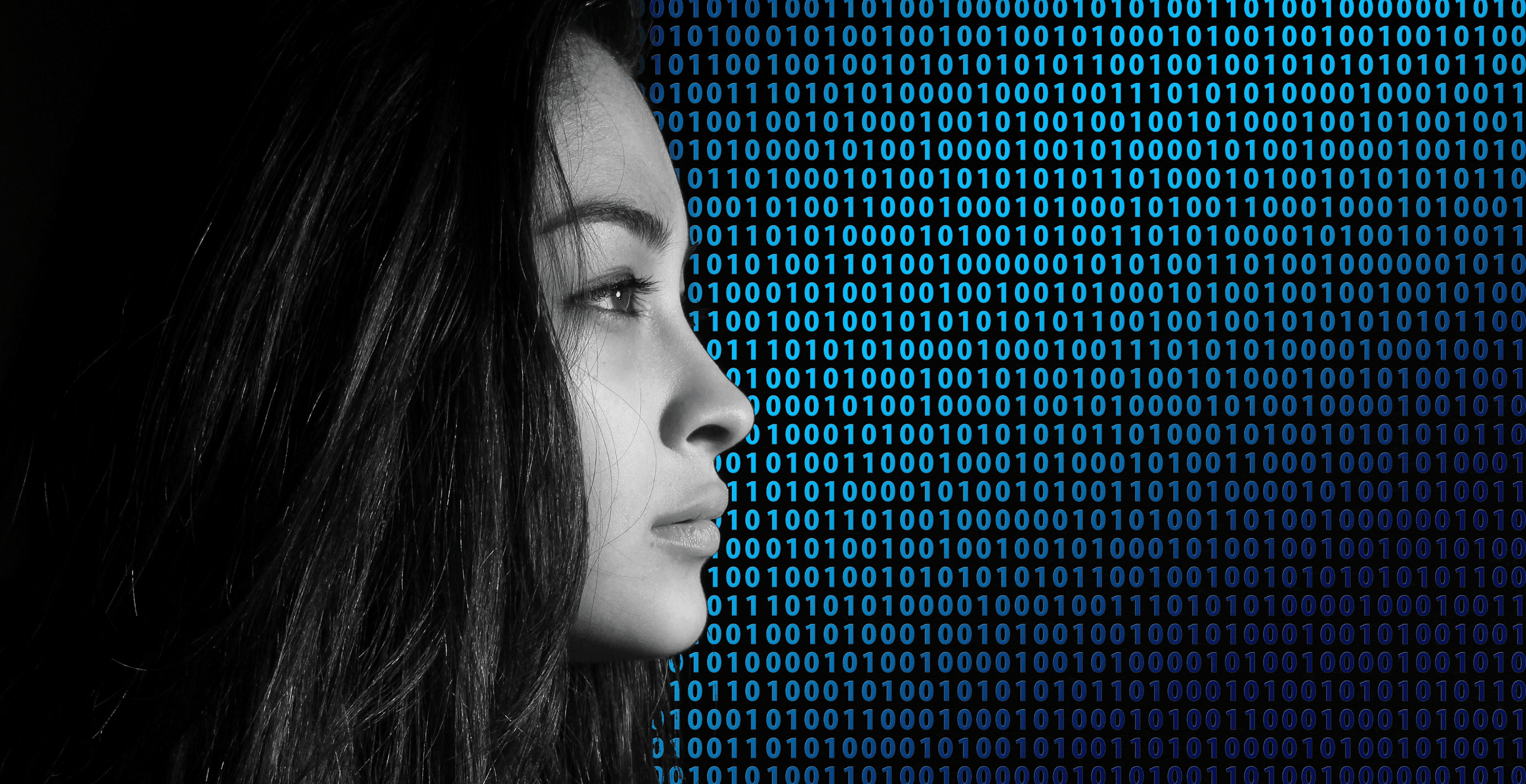
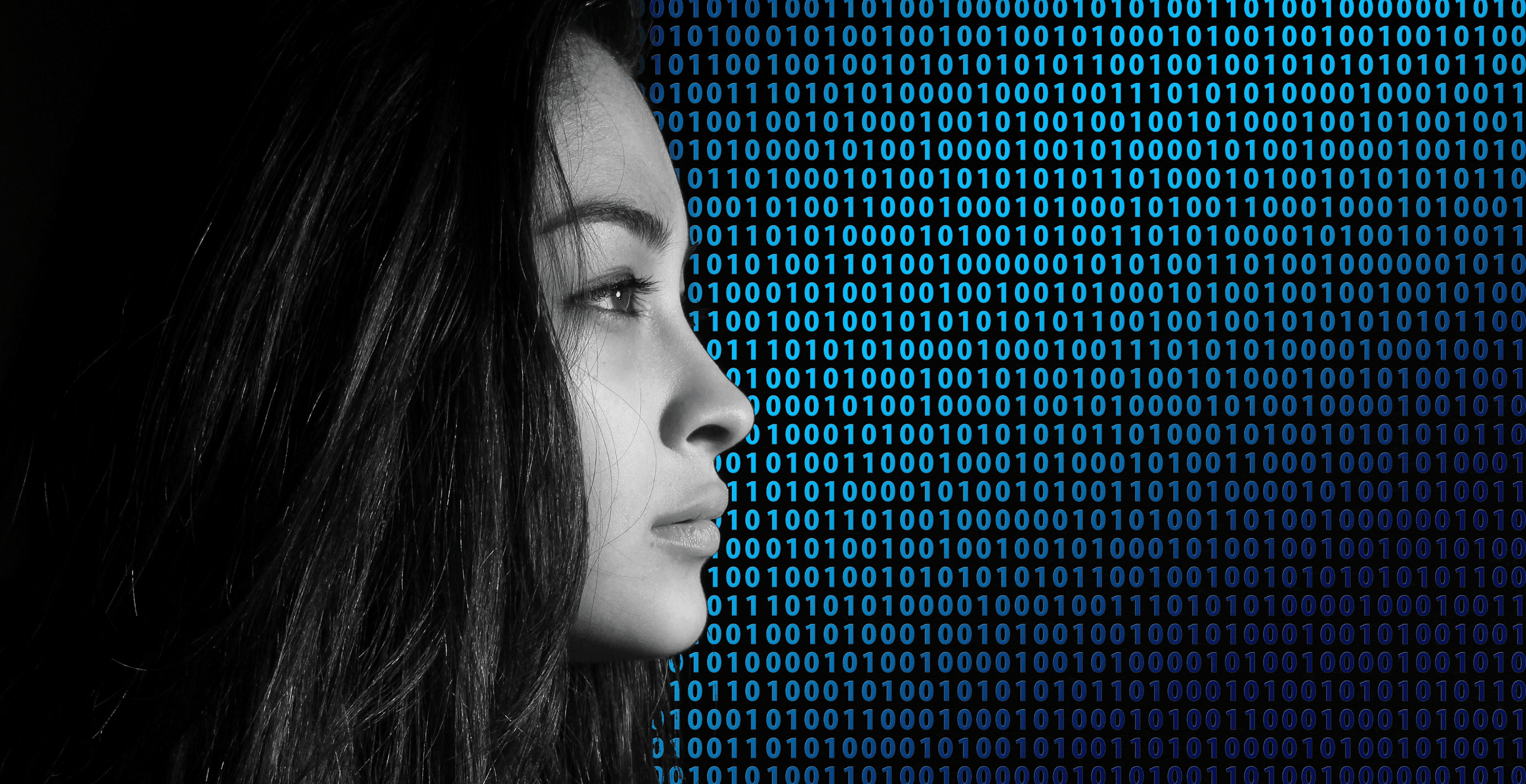
Creating Apps with Large Language Models

Rehan Asif
Nov 21, 2024
Read the article
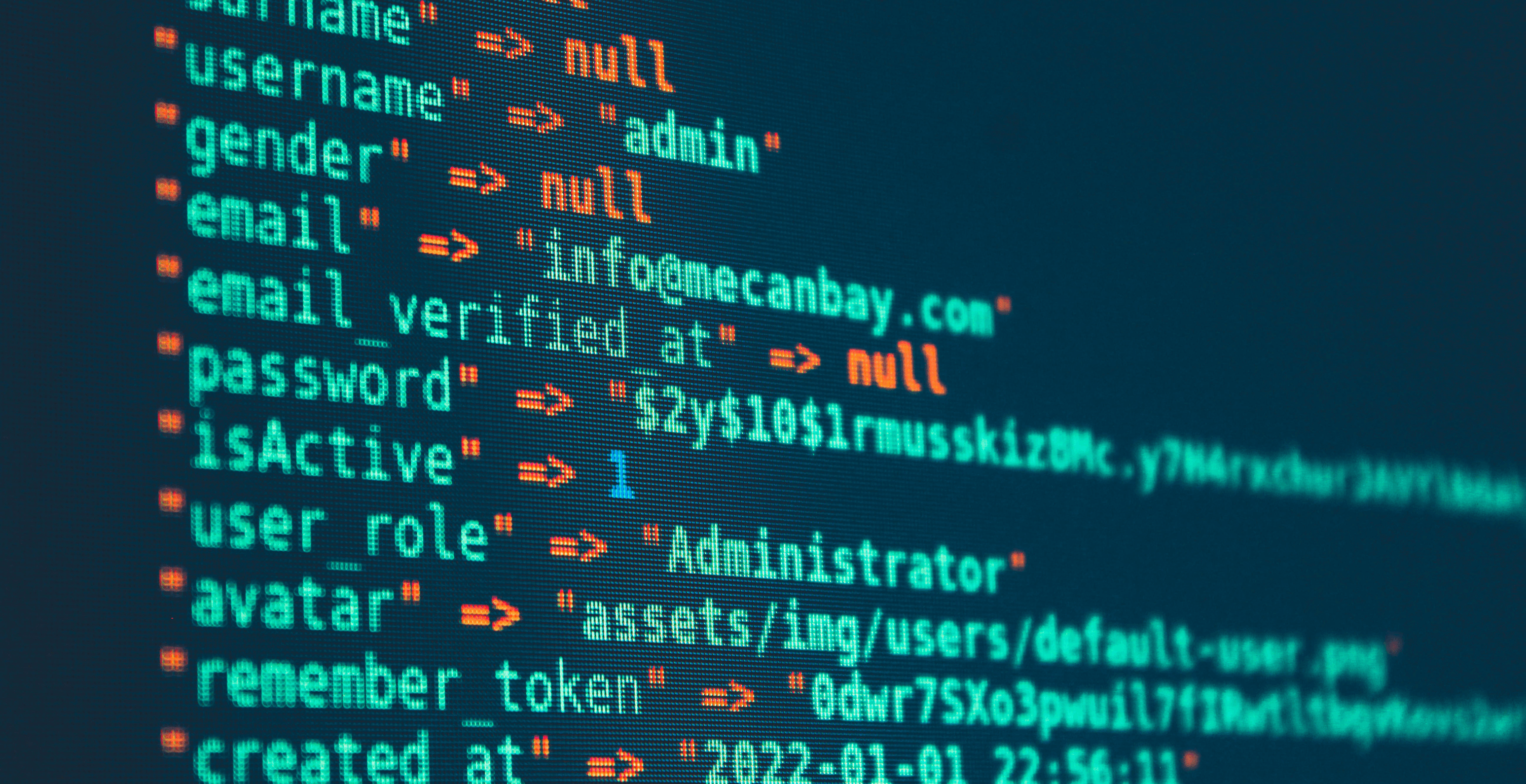
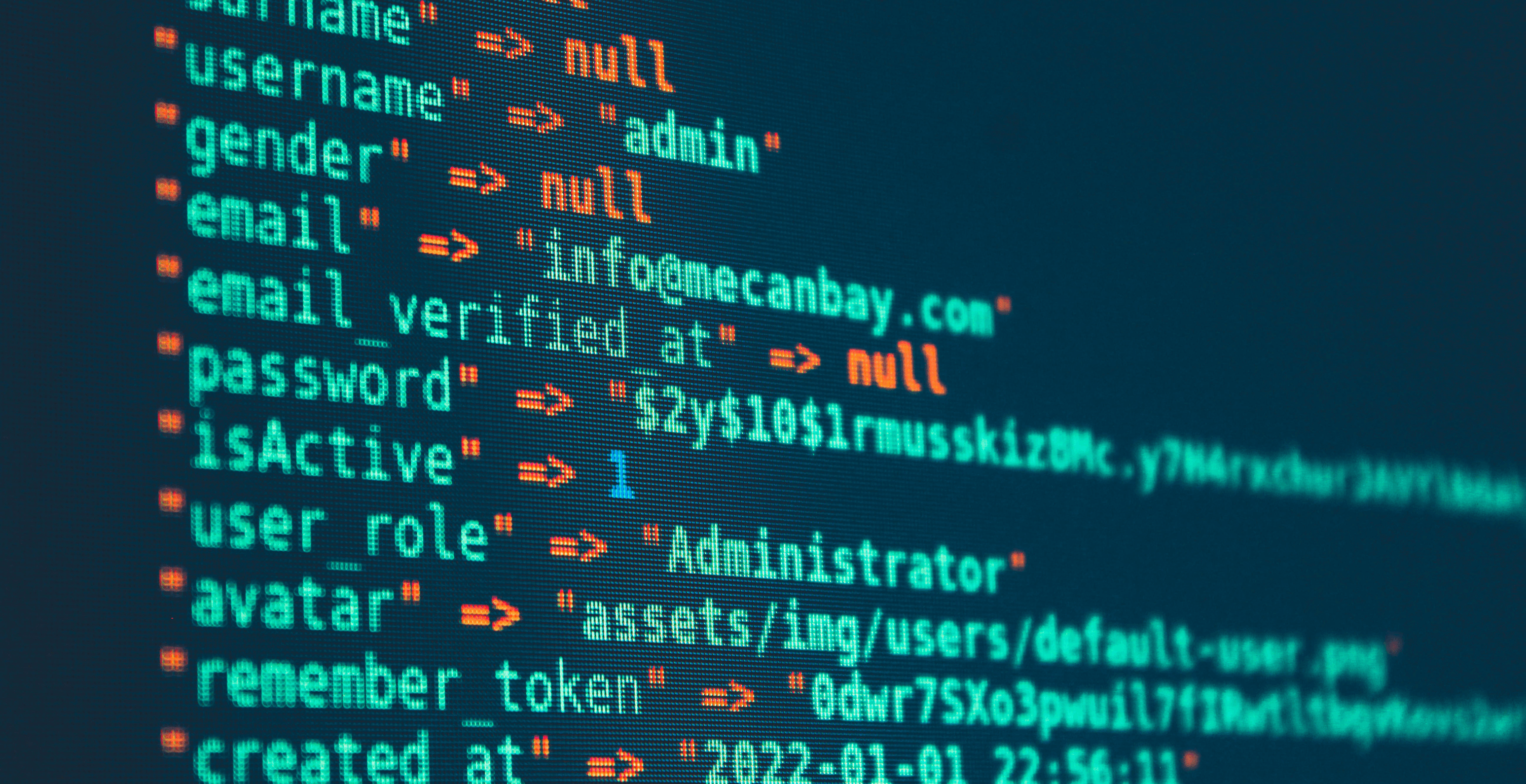
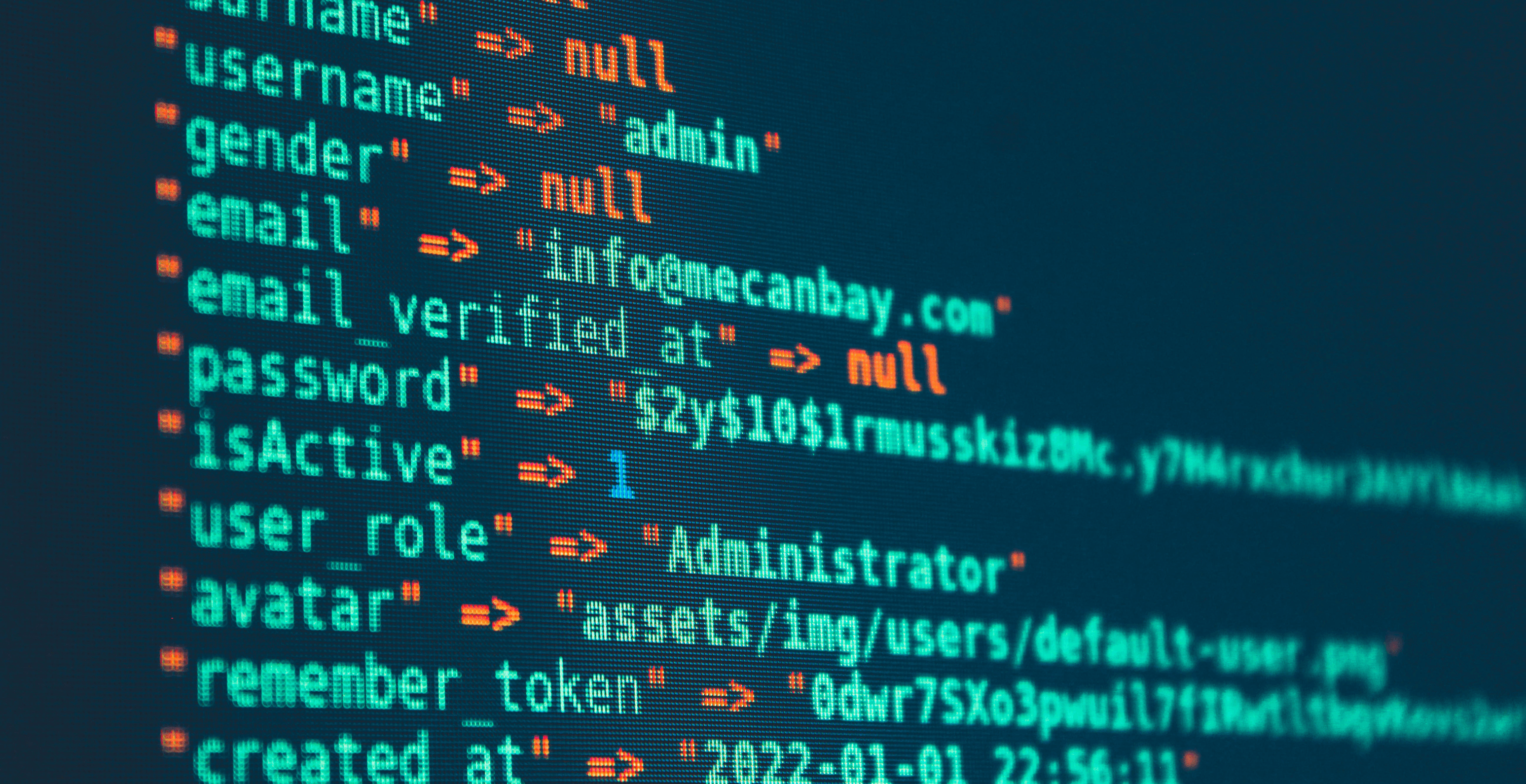
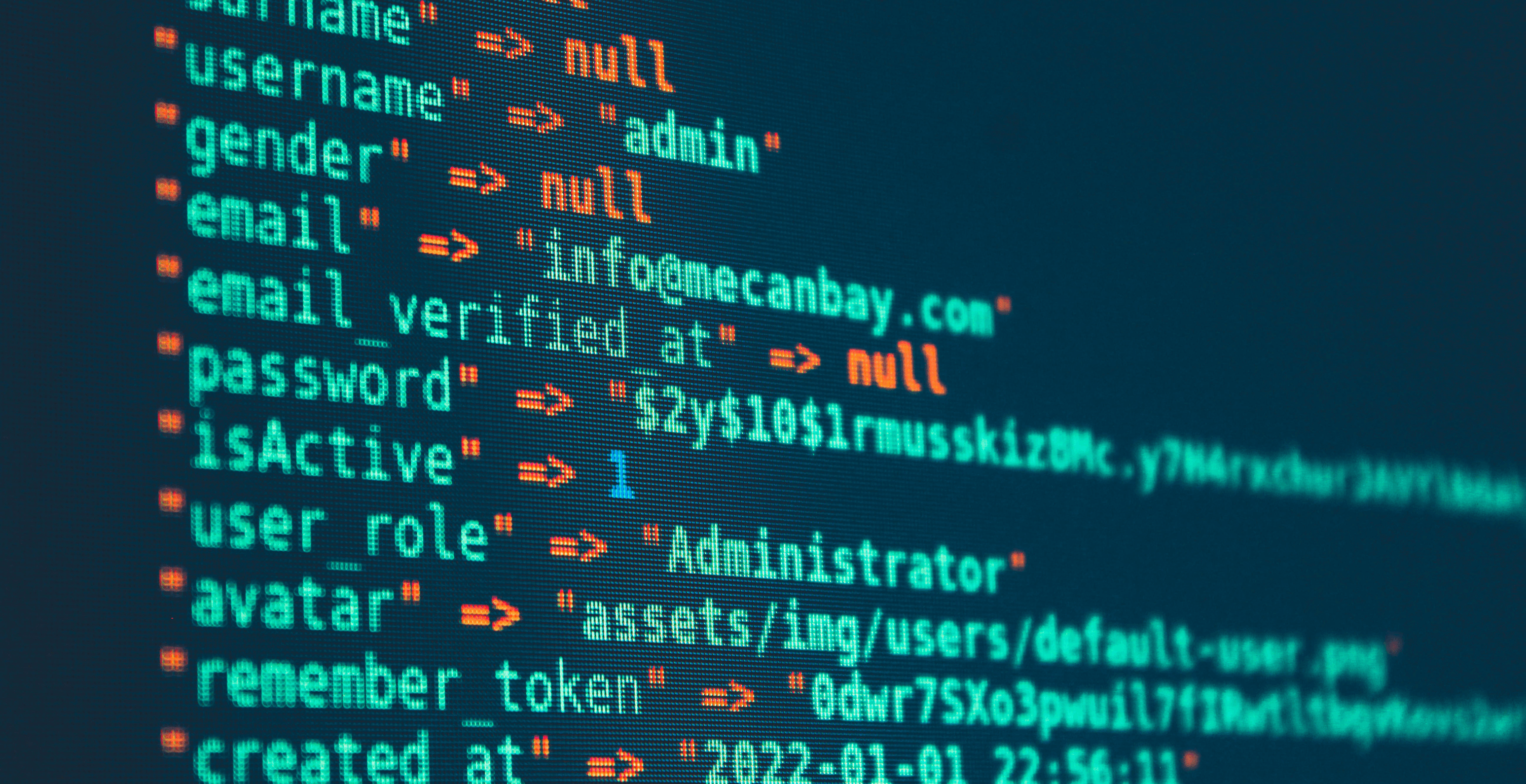
Best Practices In Data Governance For AI
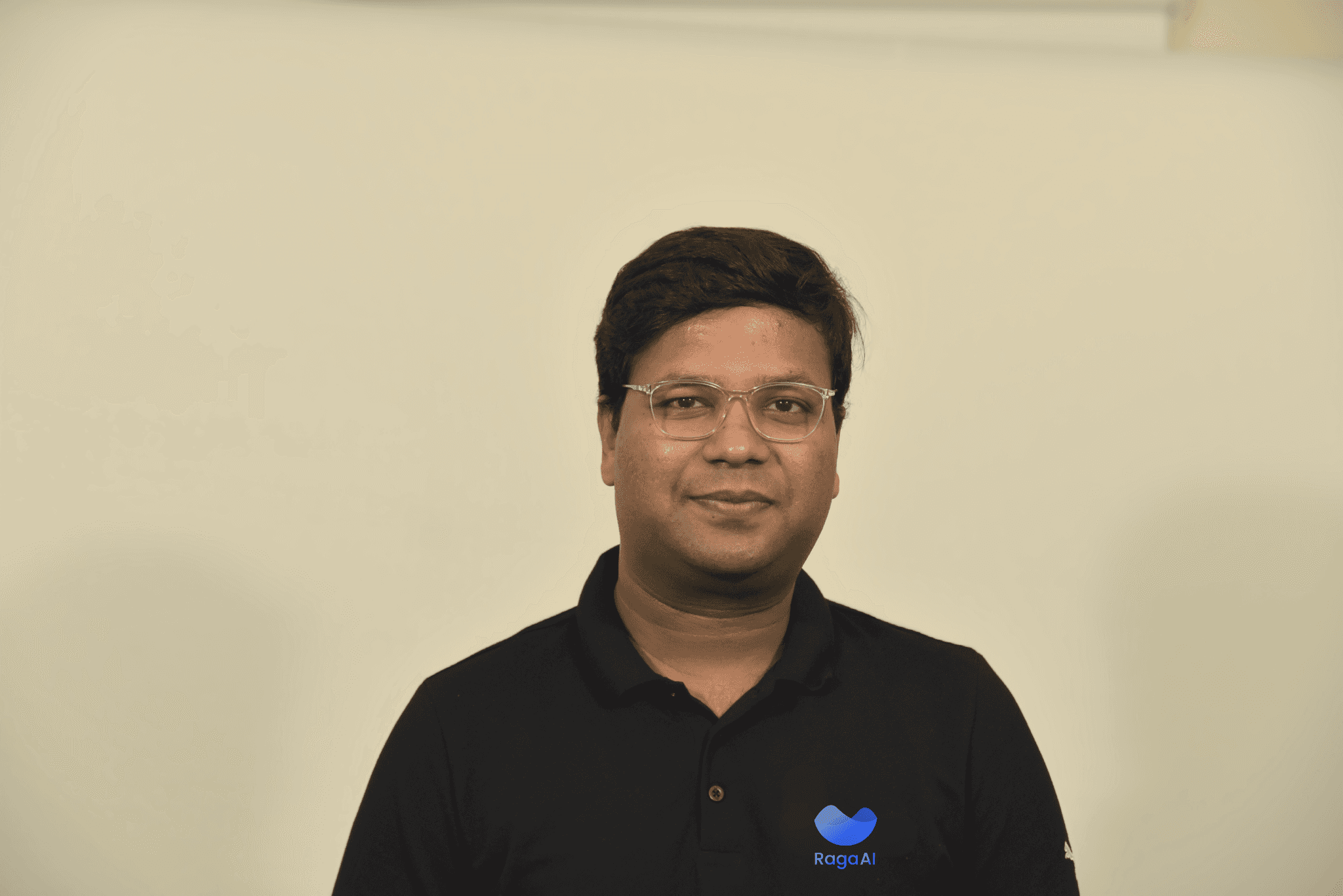
Jigar Gupta
Nov 17, 2024
Read the article
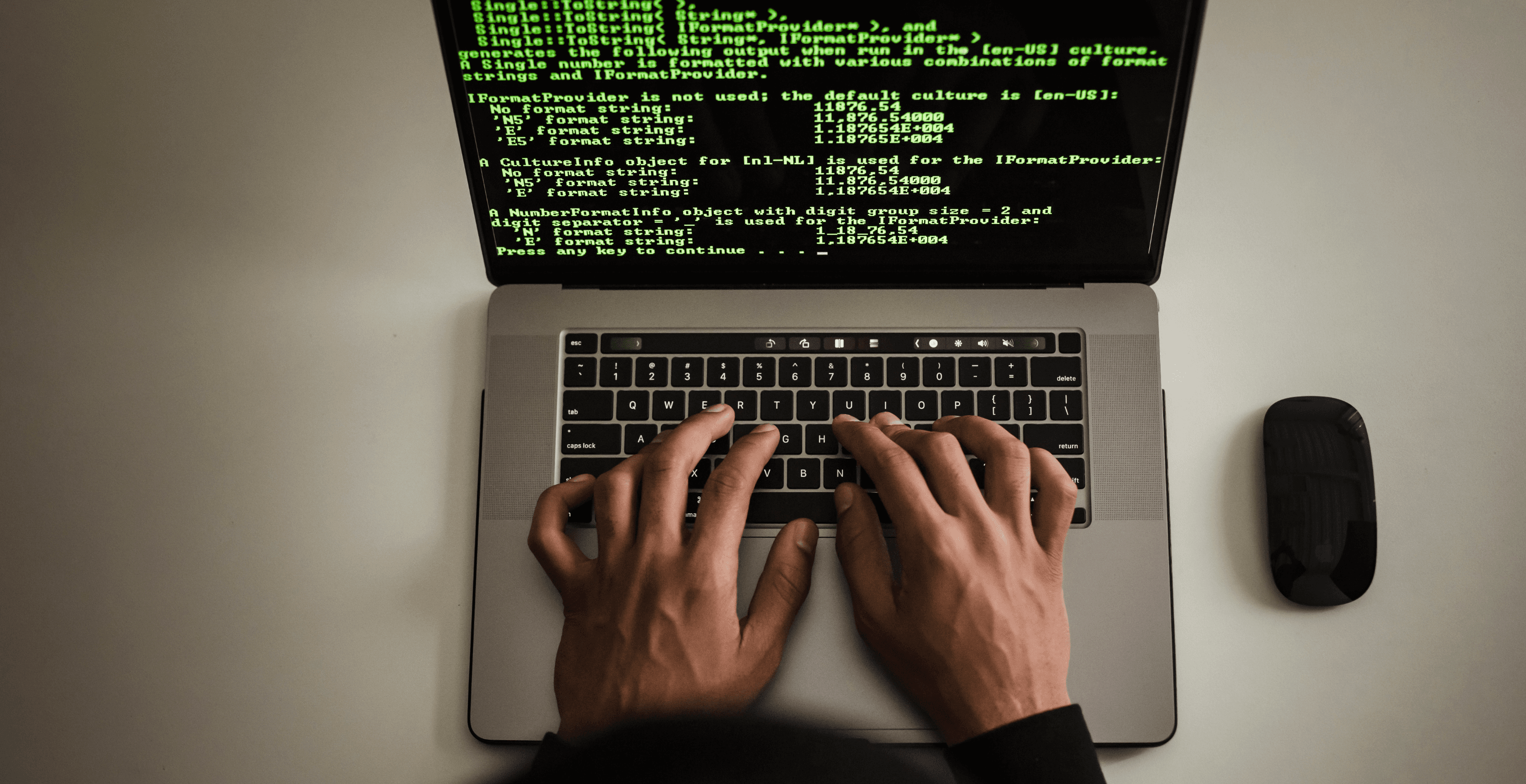
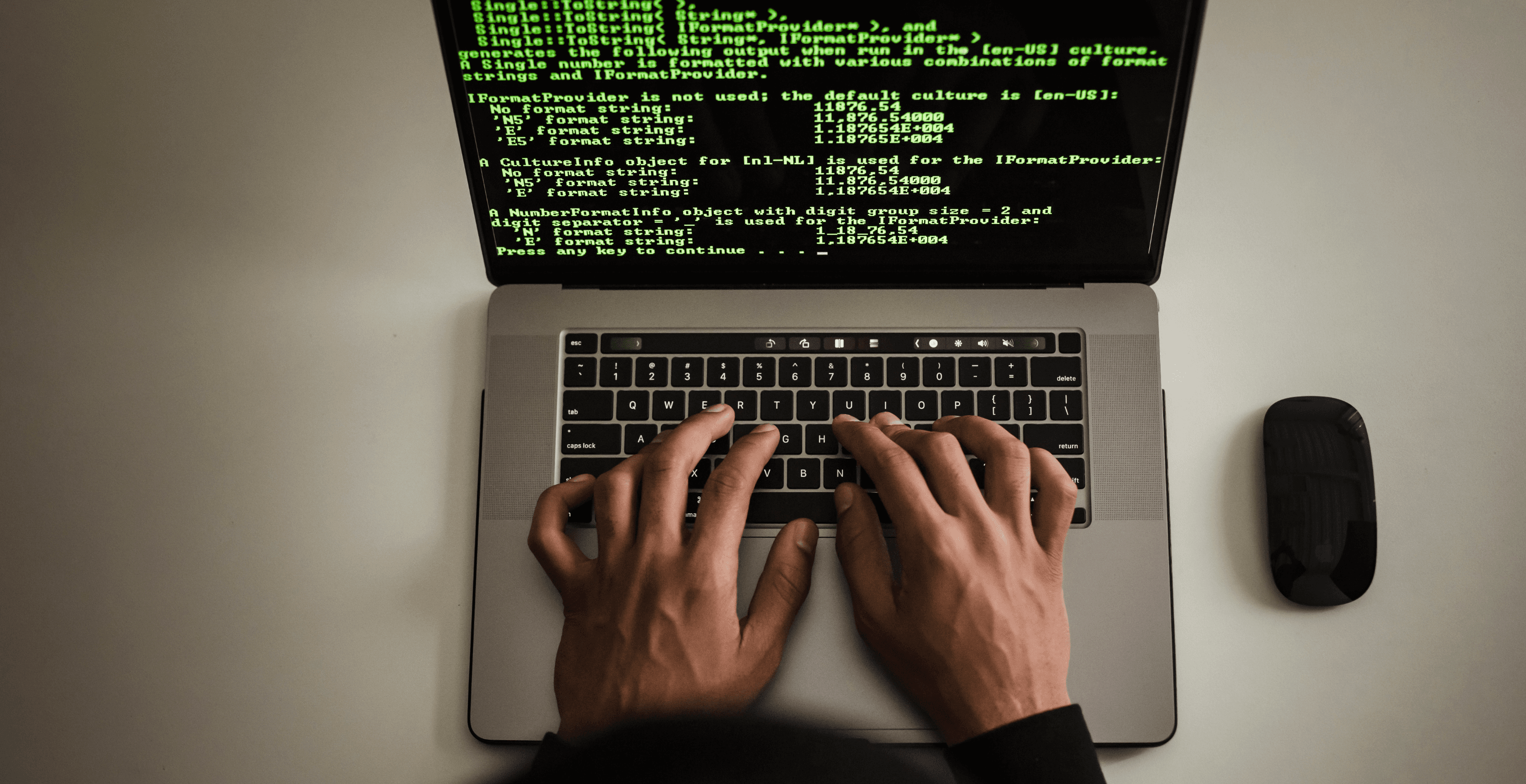
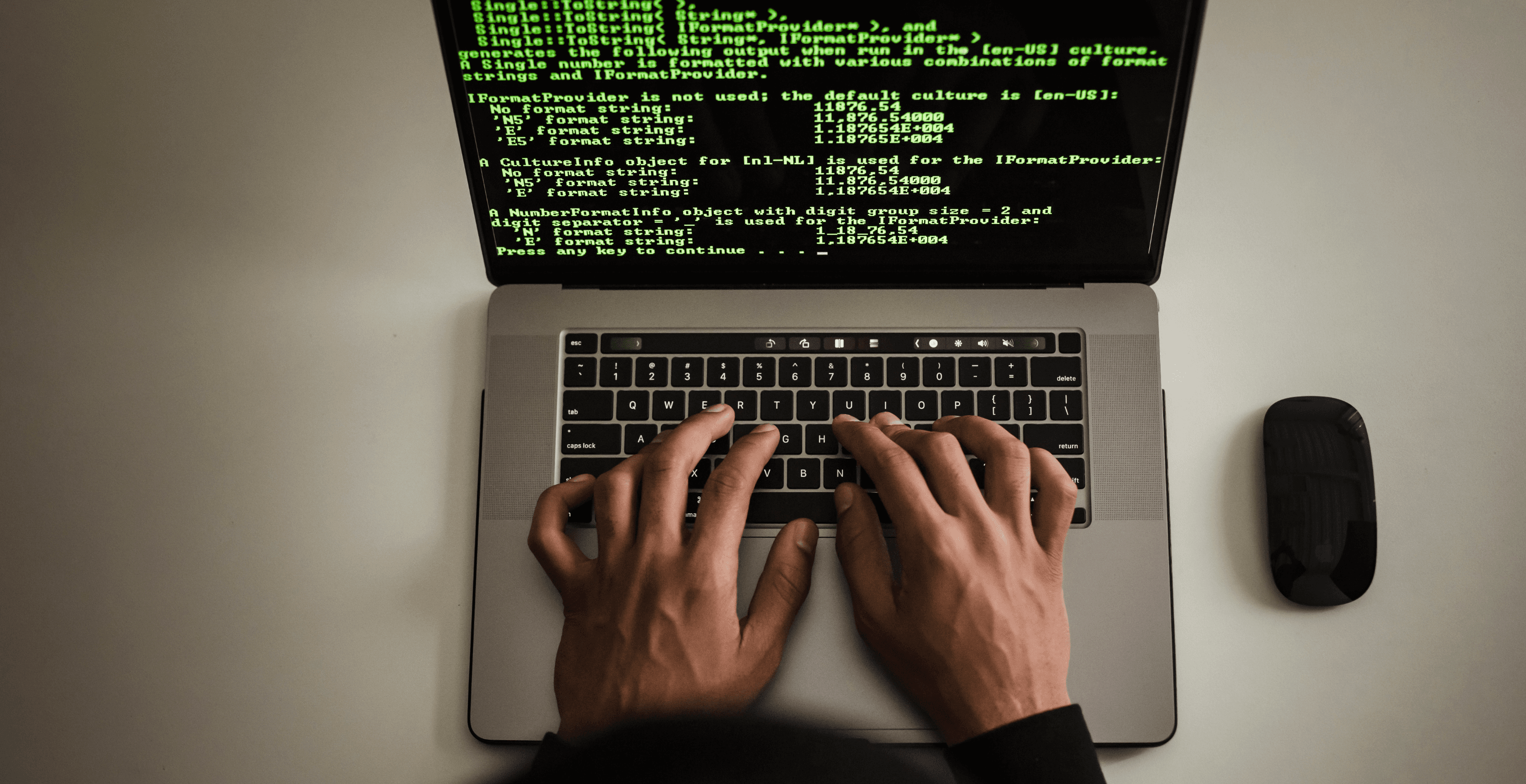
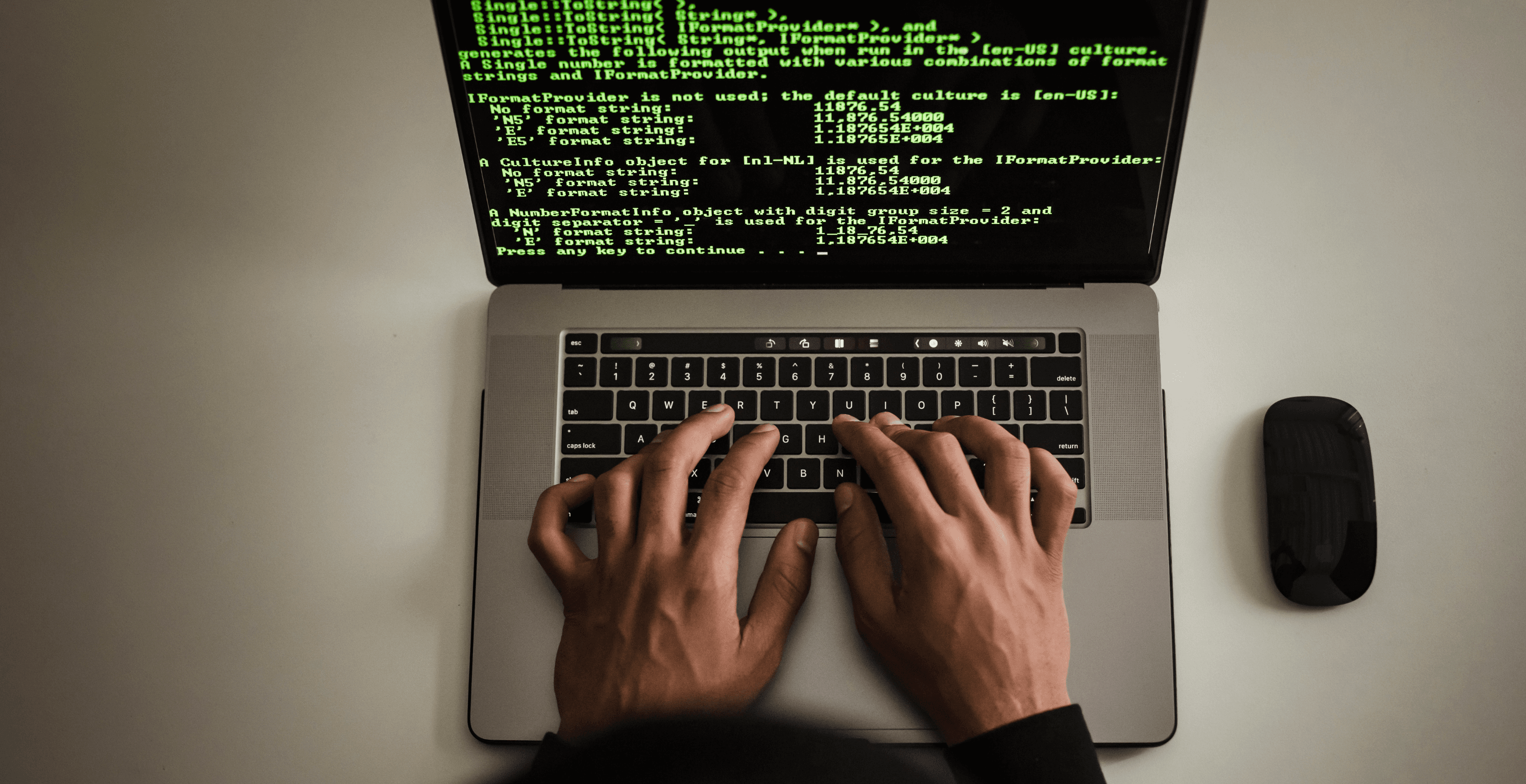
Transforming Conversational AI with Large Language Models

Rehan Asif
Nov 15, 2024
Read the article
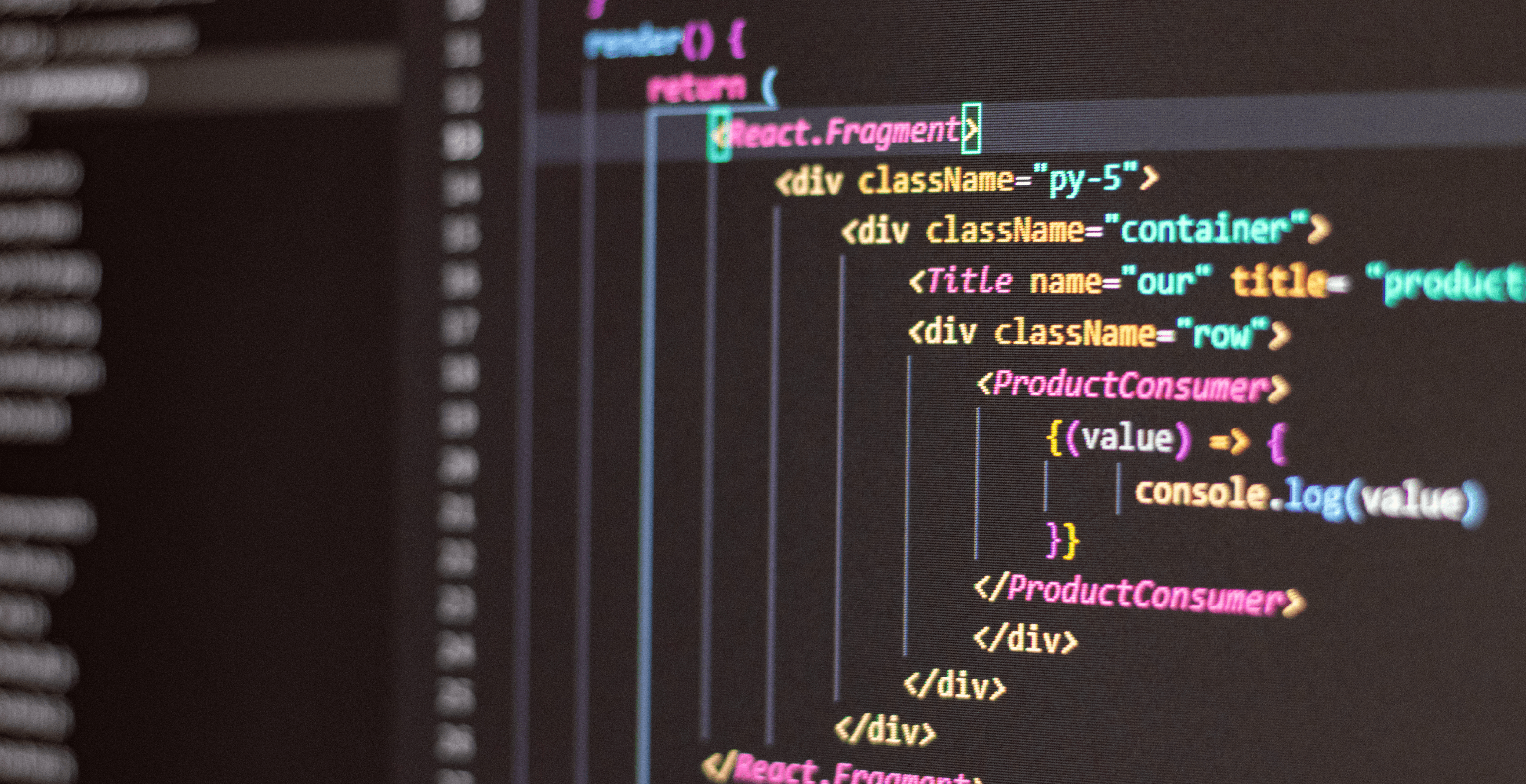
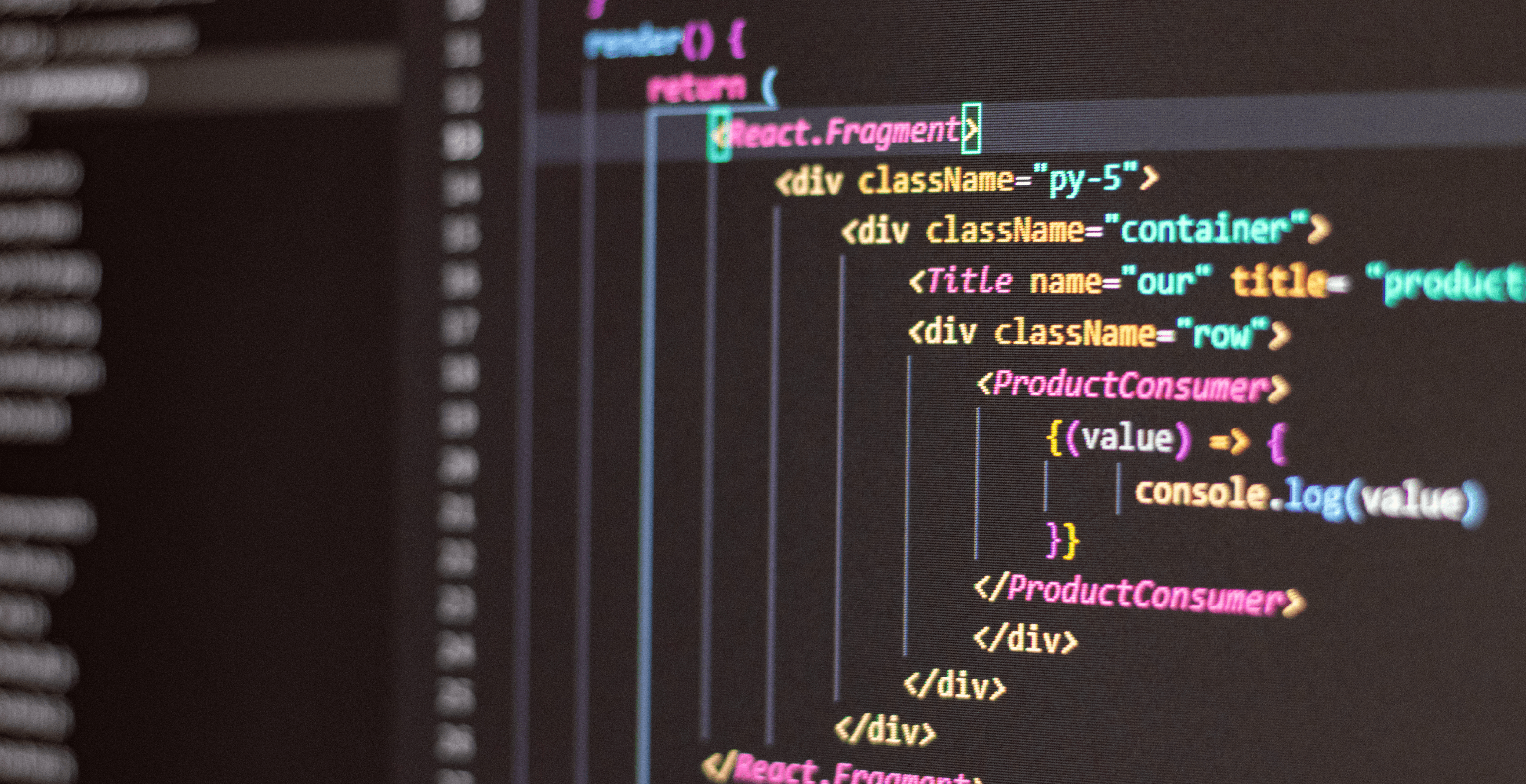
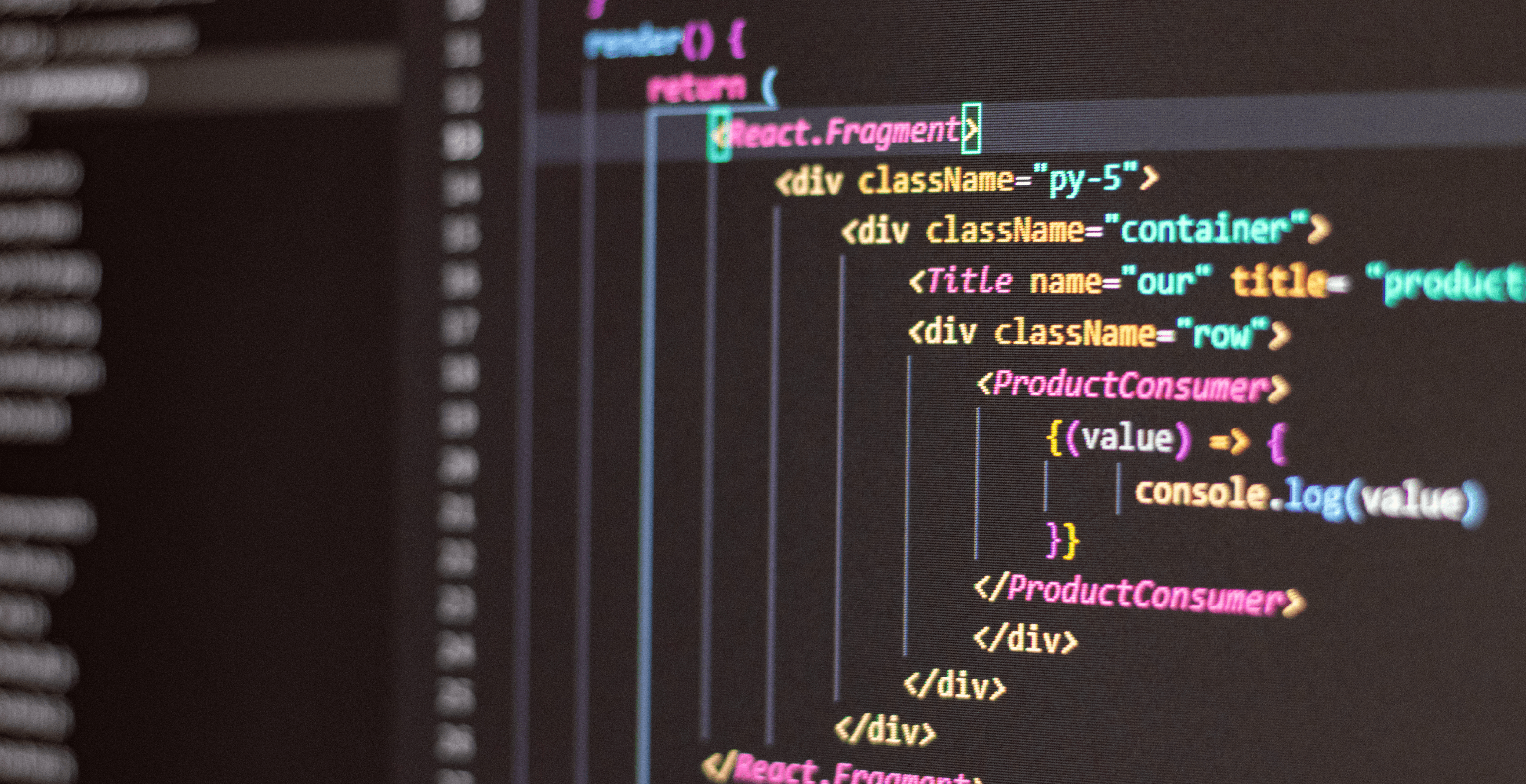
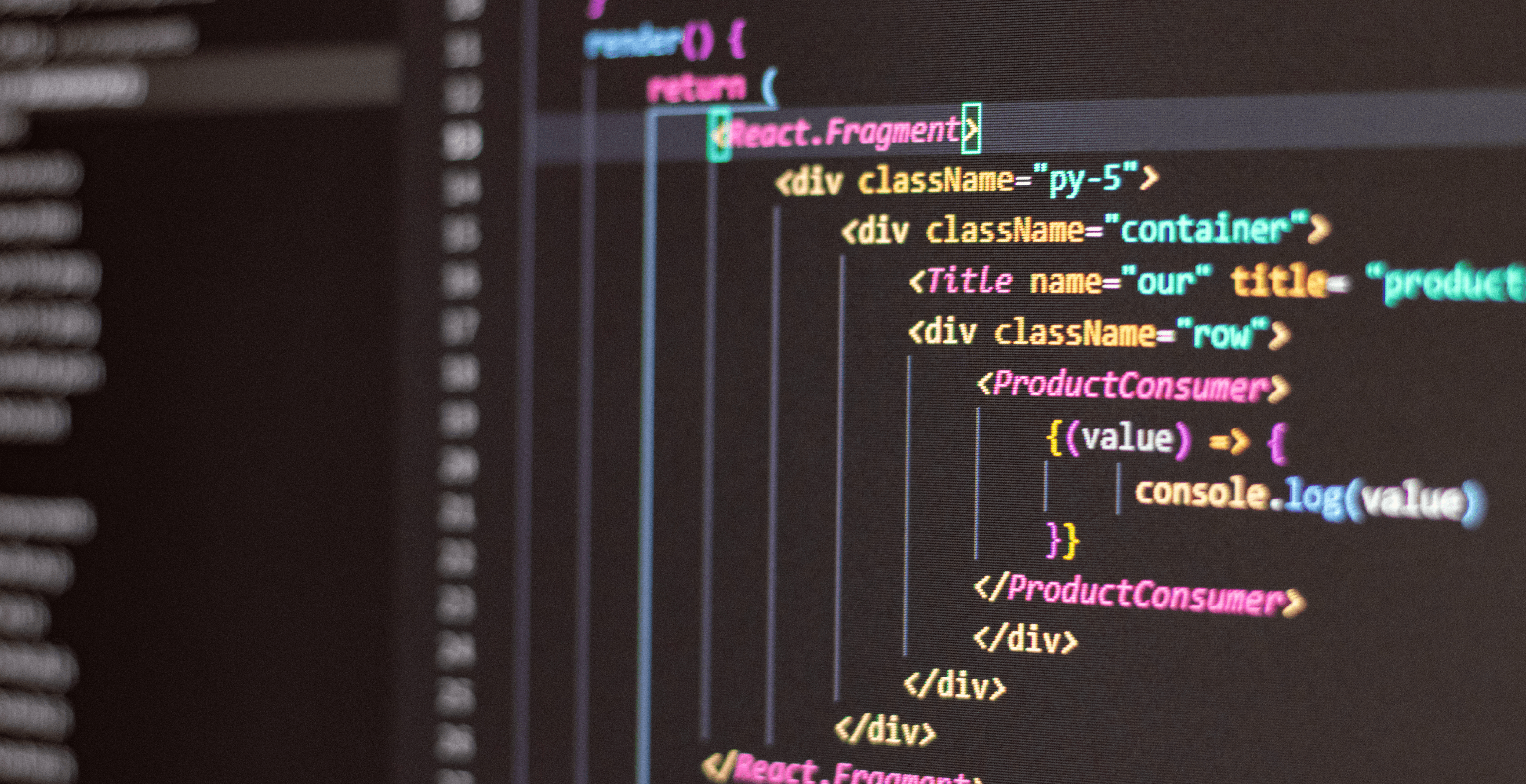
Deploying Generative AI Agents with Local LLMs

Rehan Asif
Nov 13, 2024
Read the article




Exploring Different Types of AI Agents with Key Examples
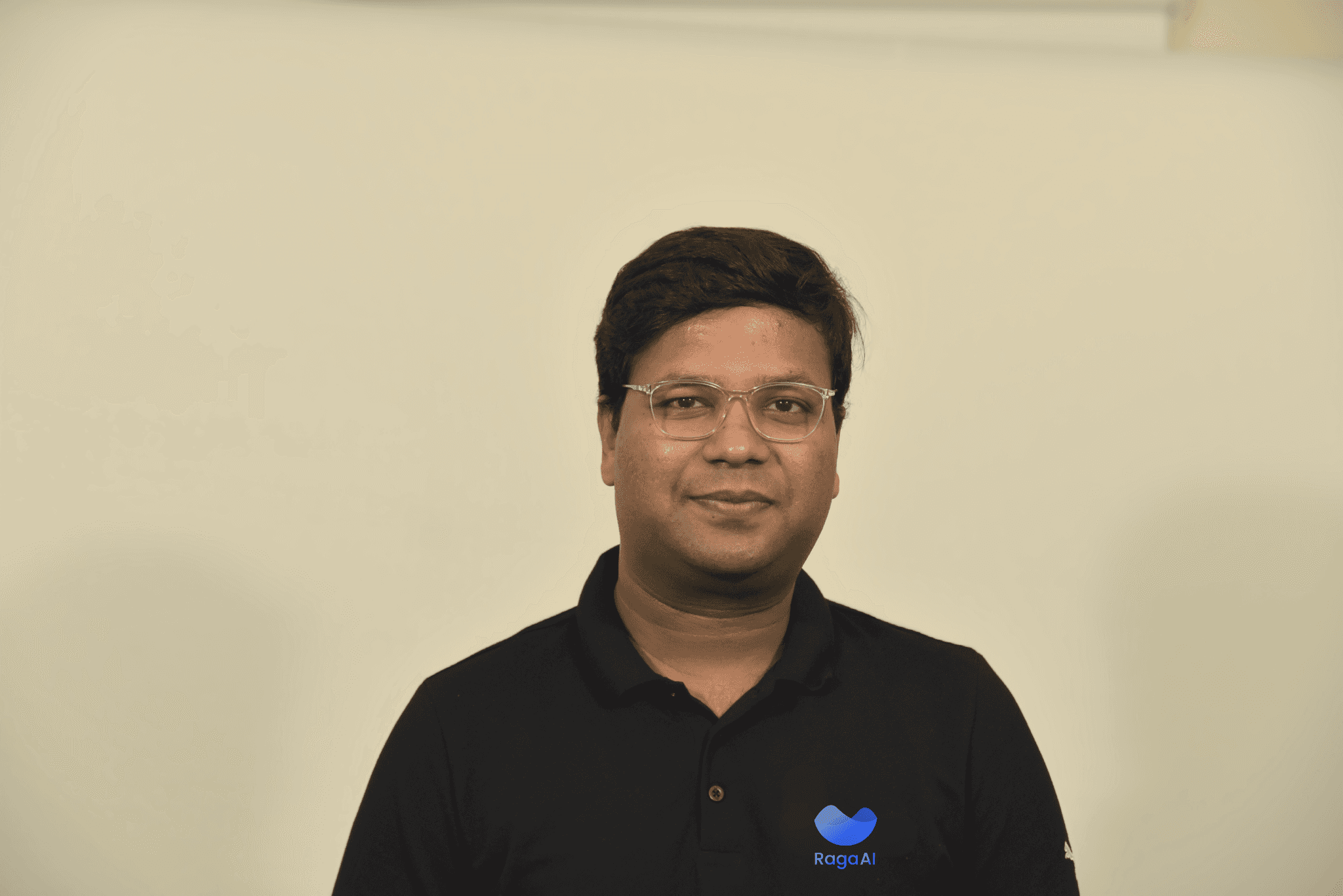
Jigar Gupta
Nov 11, 2024
Read the article




Creating Your Own Personal LLM Agents: Introduction to Implementation

Rehan Asif
Nov 8, 2024
Read the article




Exploring Agentic AI Architecture and Design Patterns
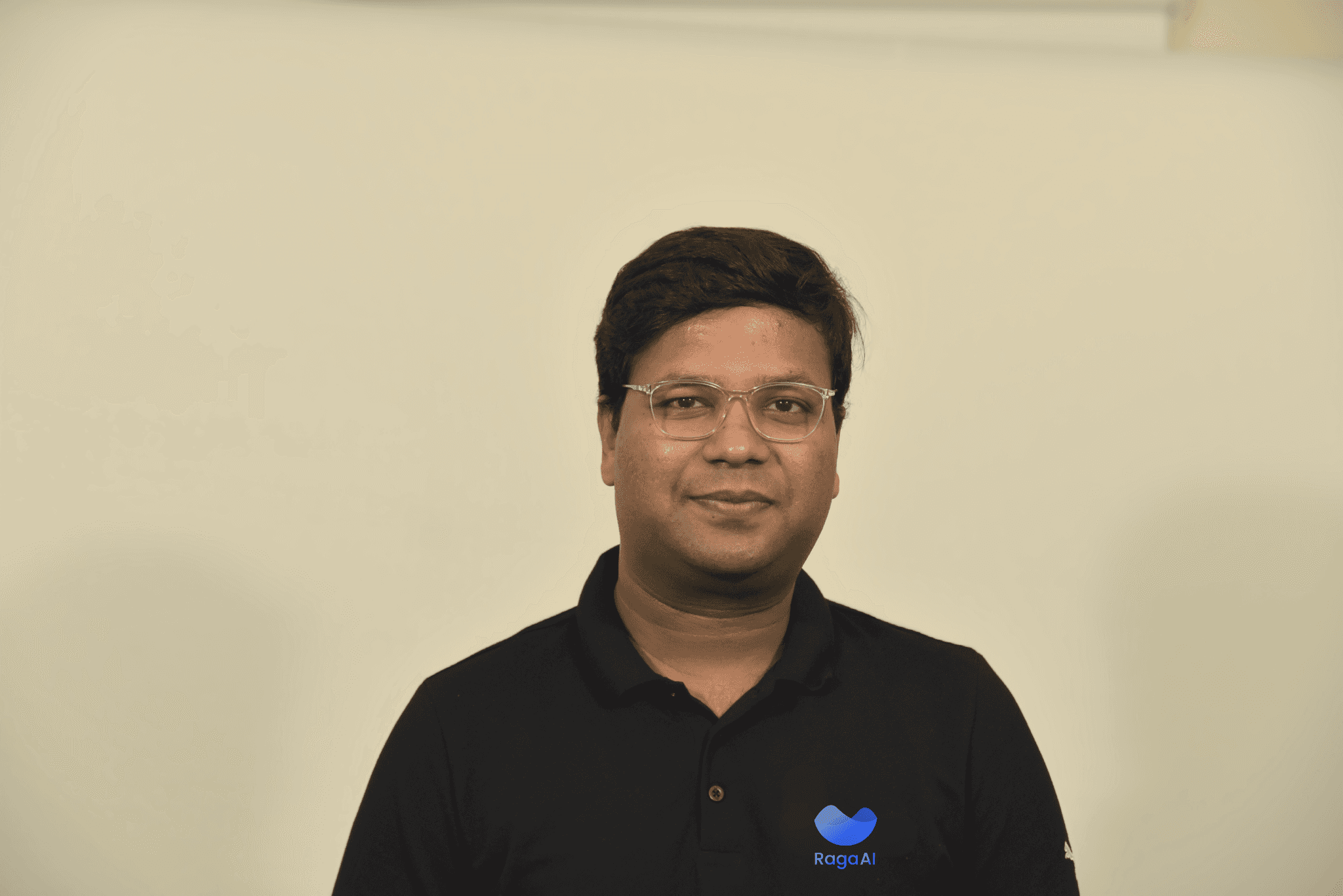
Jigar Gupta
Nov 6, 2024
Read the article
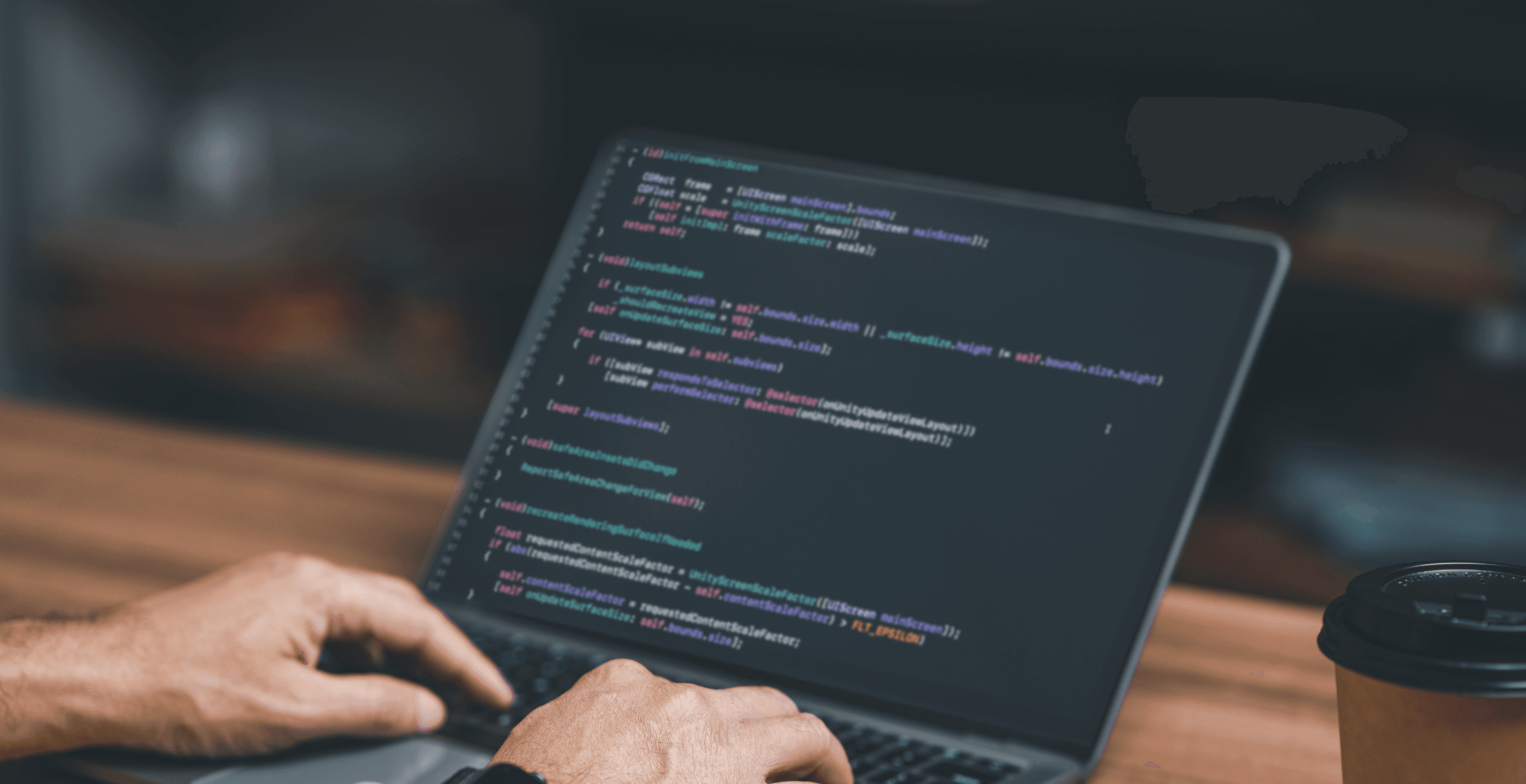
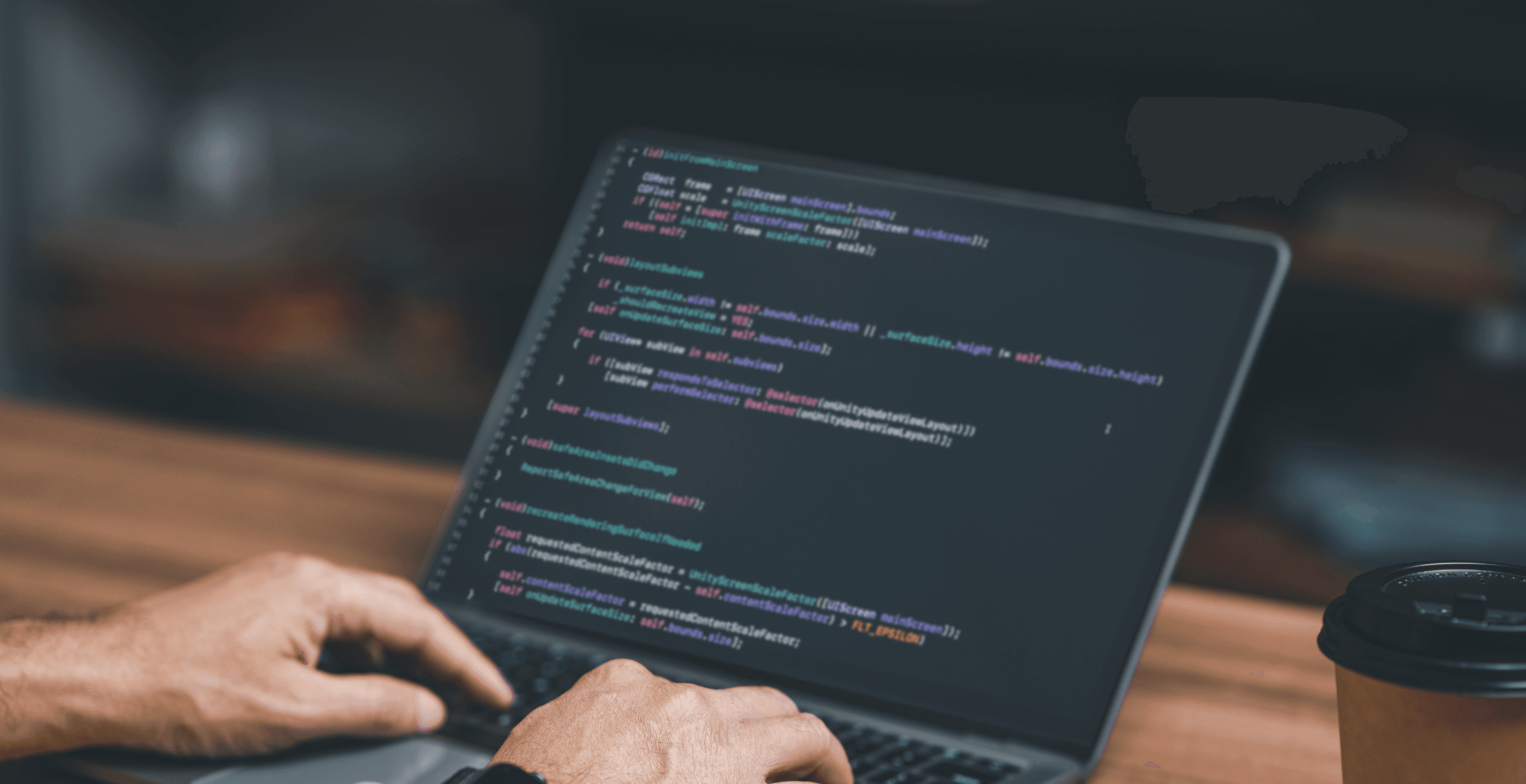
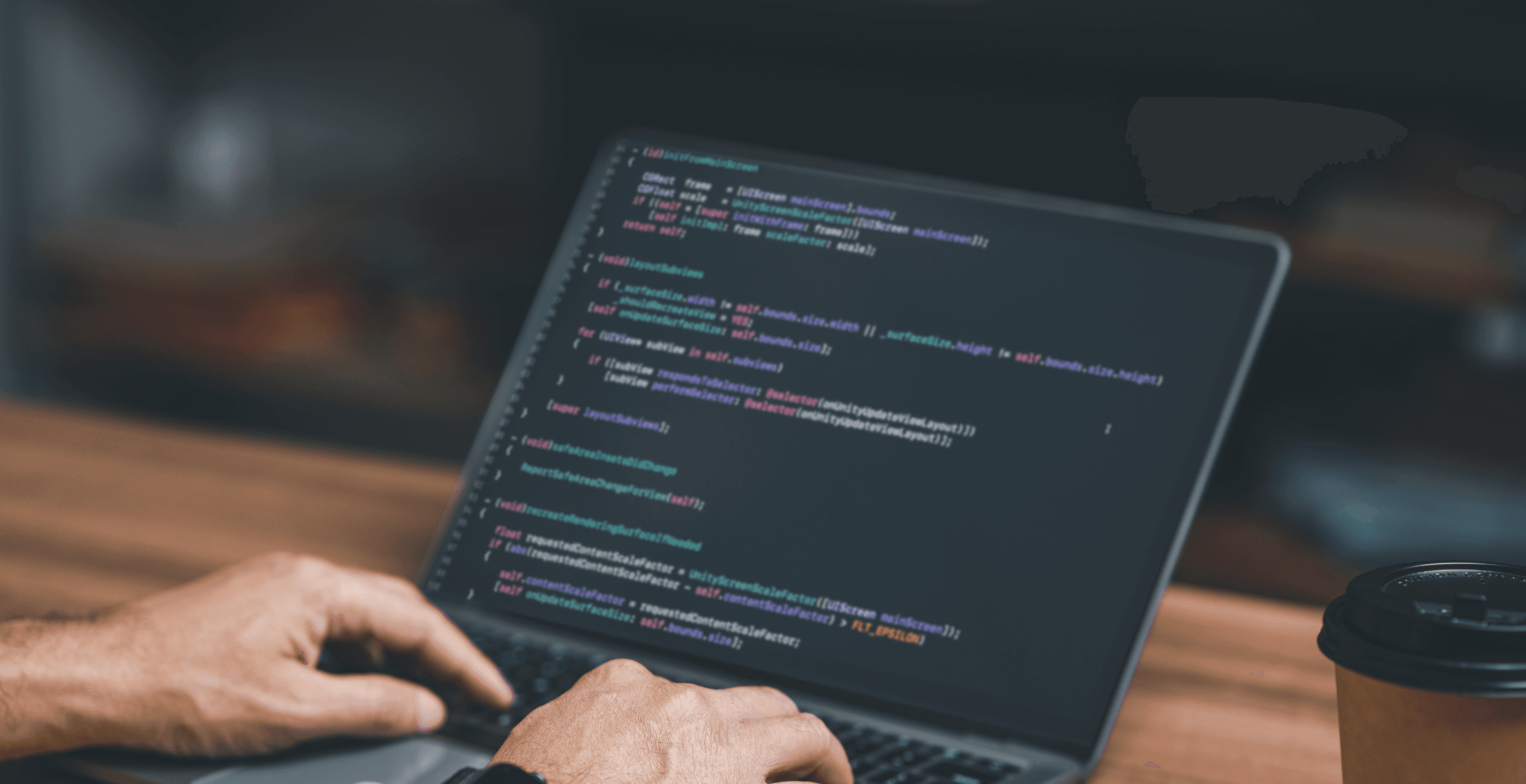
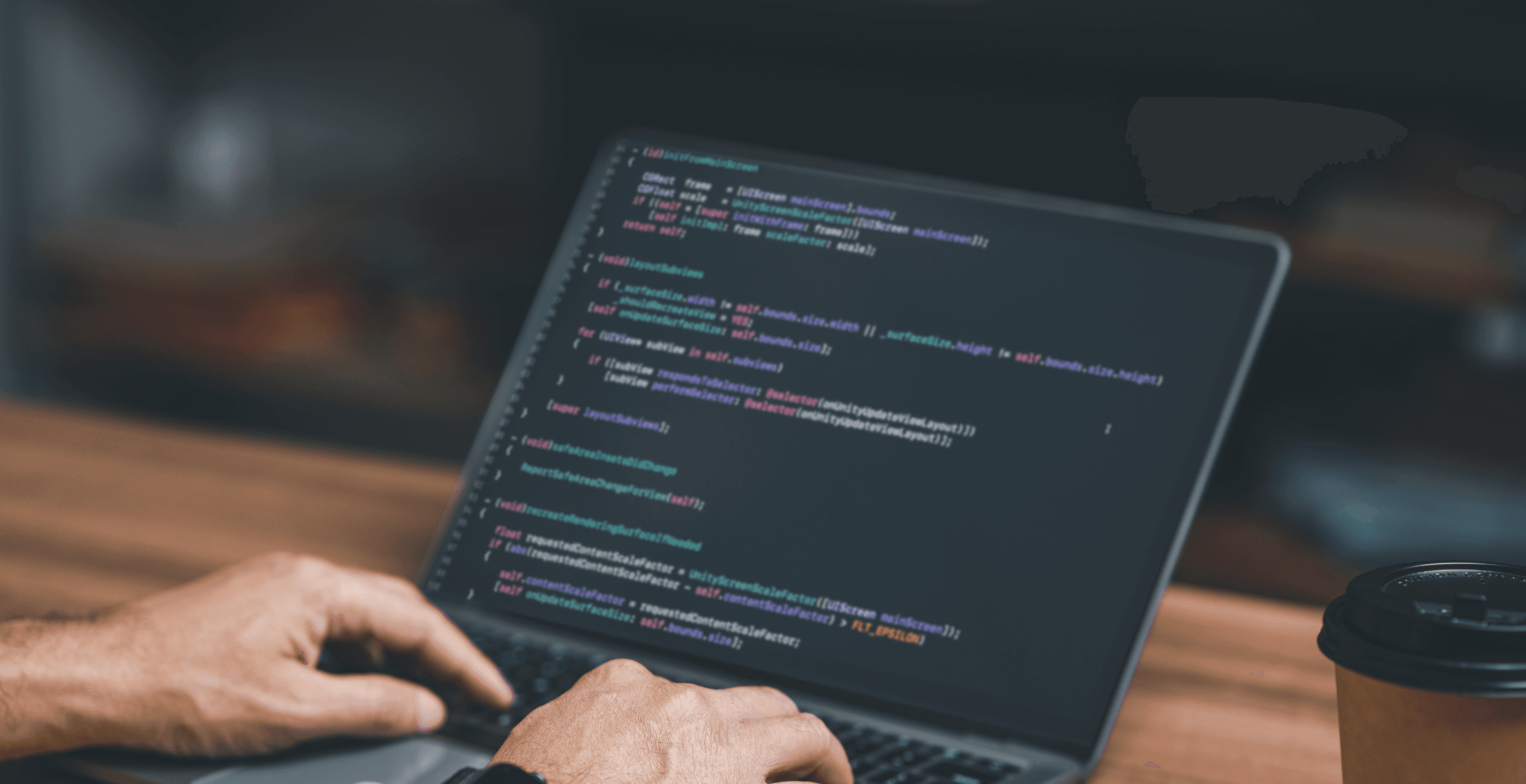
Building Your First LLM Agent Framework Application

Rehan Asif
Nov 4, 2024
Read the article




Multi-Agent Design and Collaboration Patterns

Rehan Asif
Nov 1, 2024
Read the article




Creating Your Own LLM Agent Application from Scratch

Rehan Asif
Oct 30, 2024
Read the article




Solving LLM Token Limit Issues: Understanding and Approaches

Rehan Asif
Oct 27, 2024
Read the article
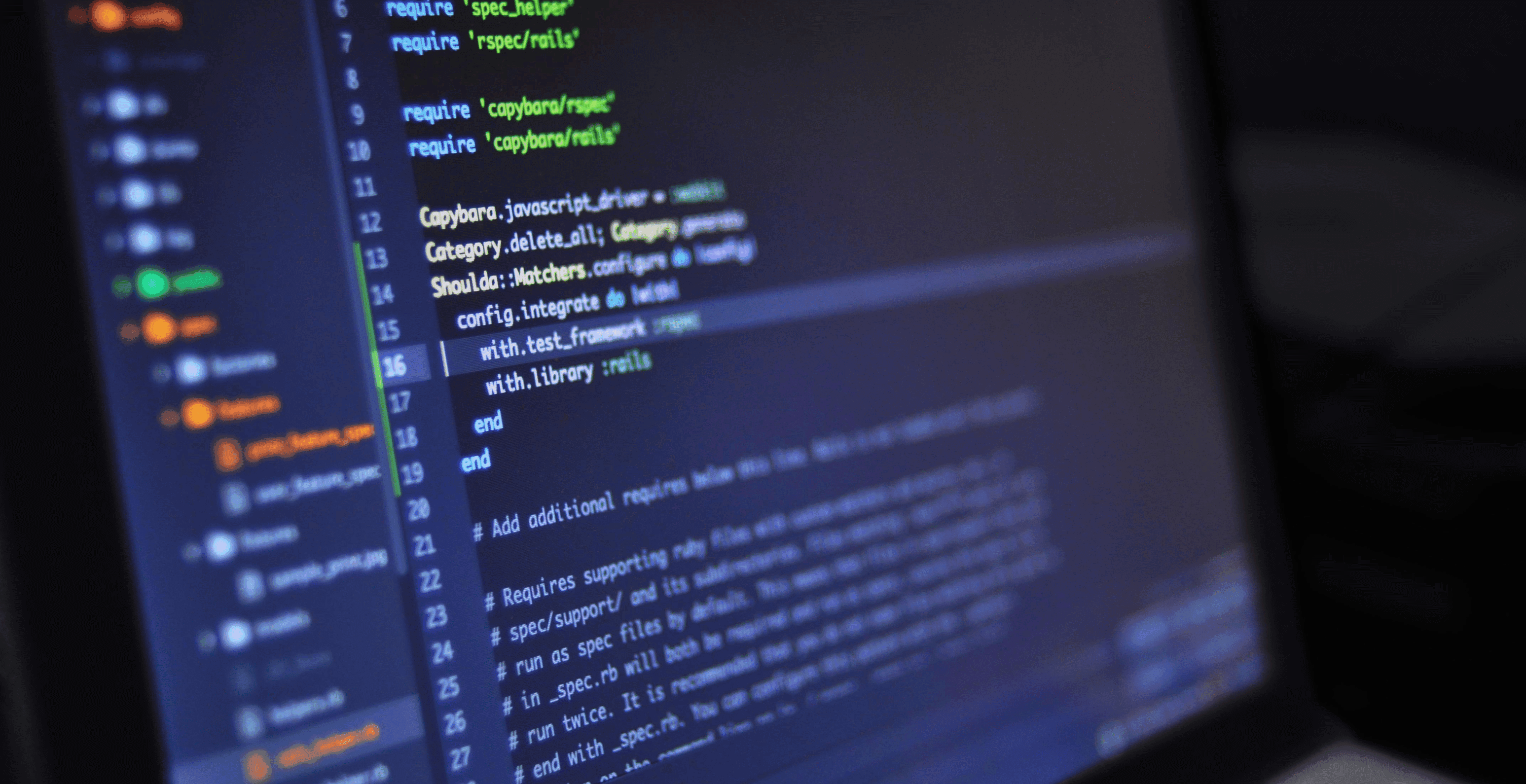
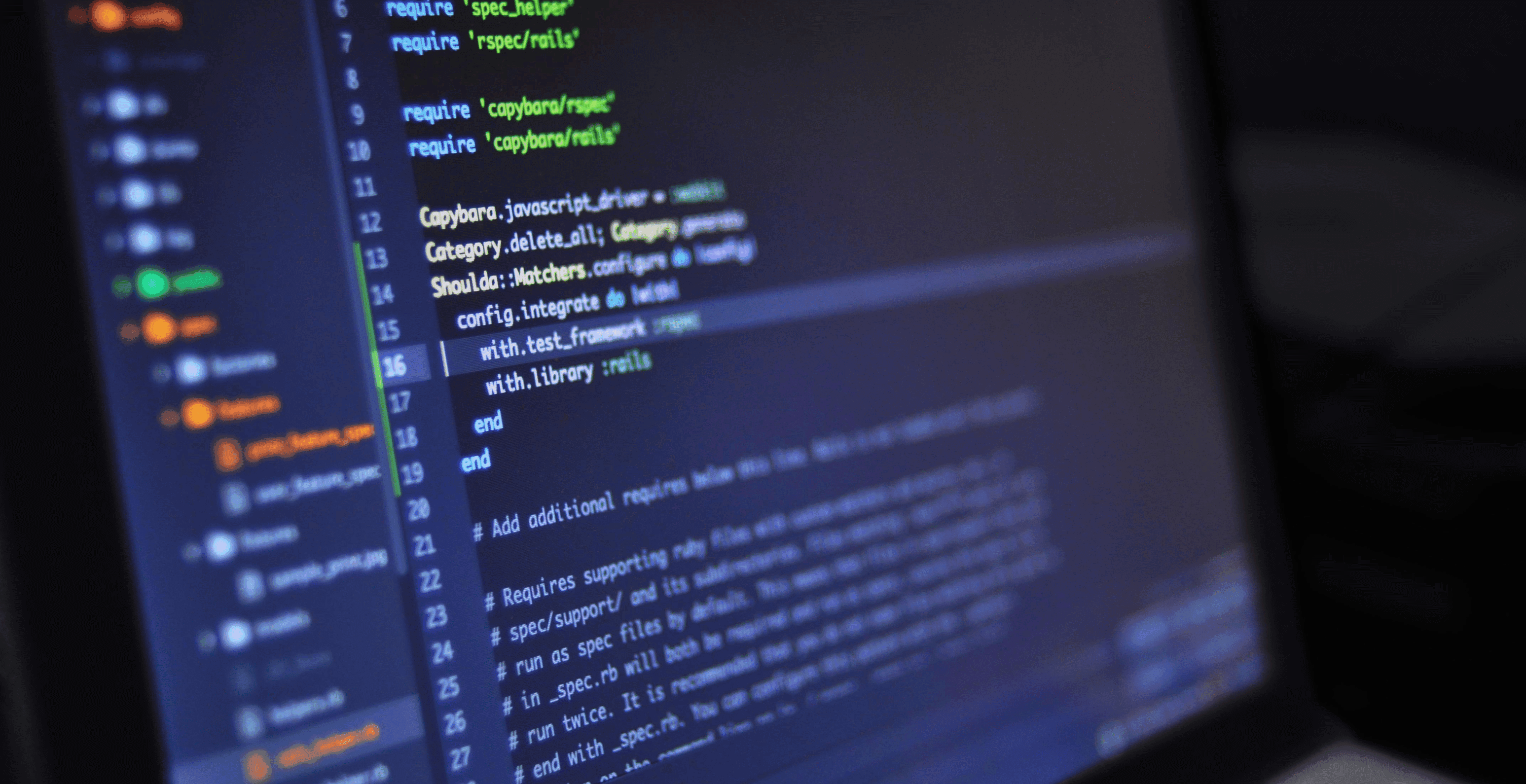
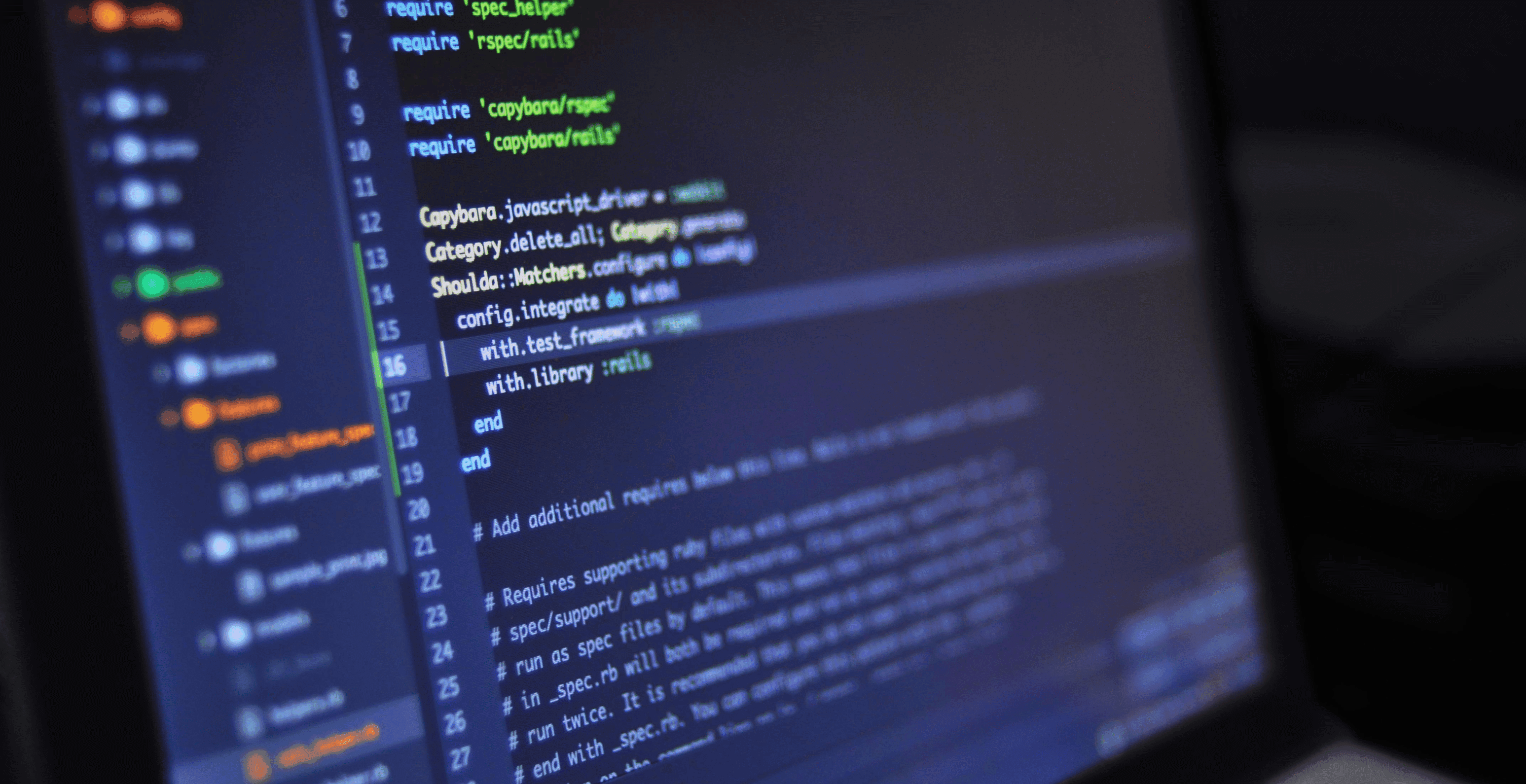
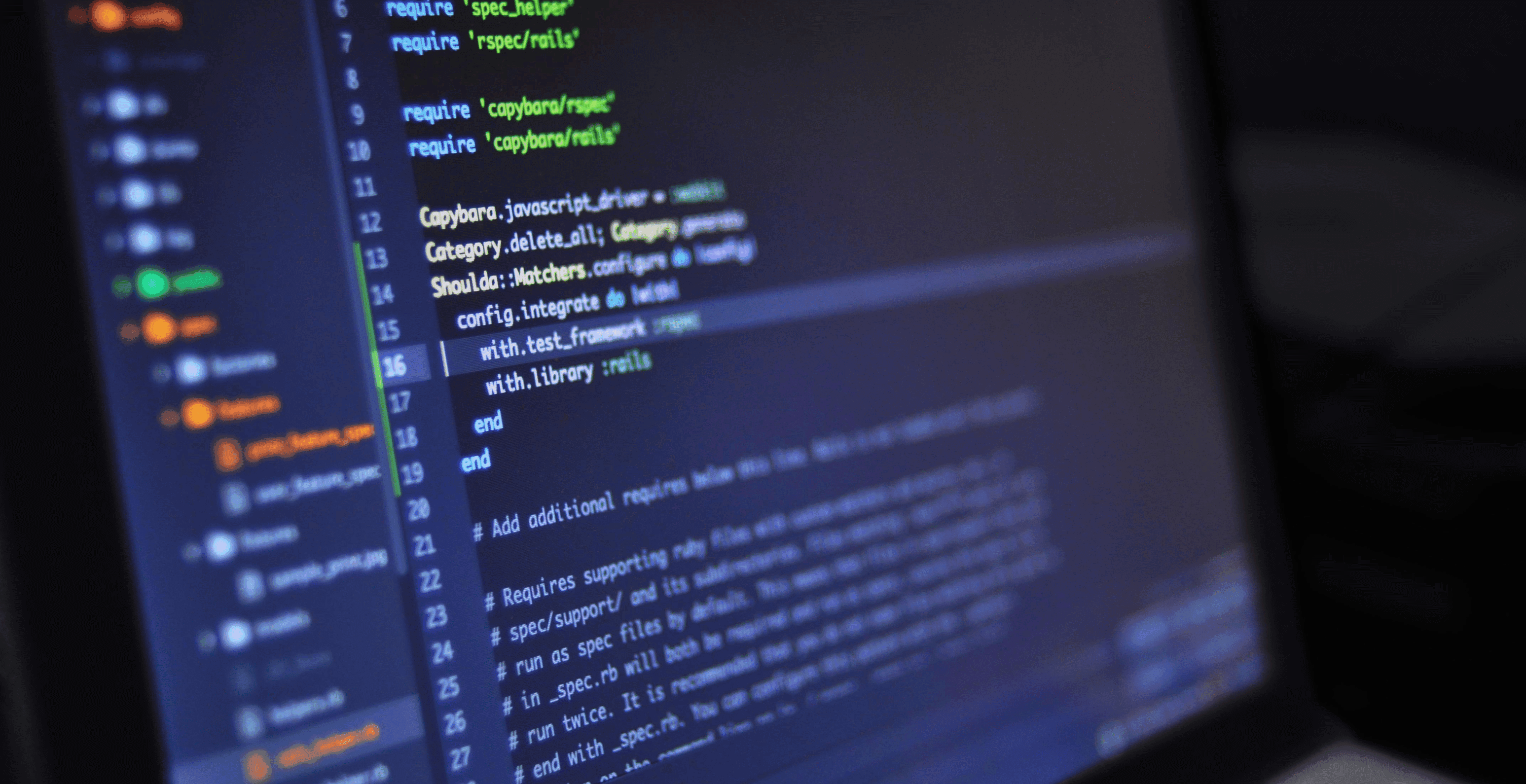
Understanding the Impact of Inference Cost on Generative AI Adoption
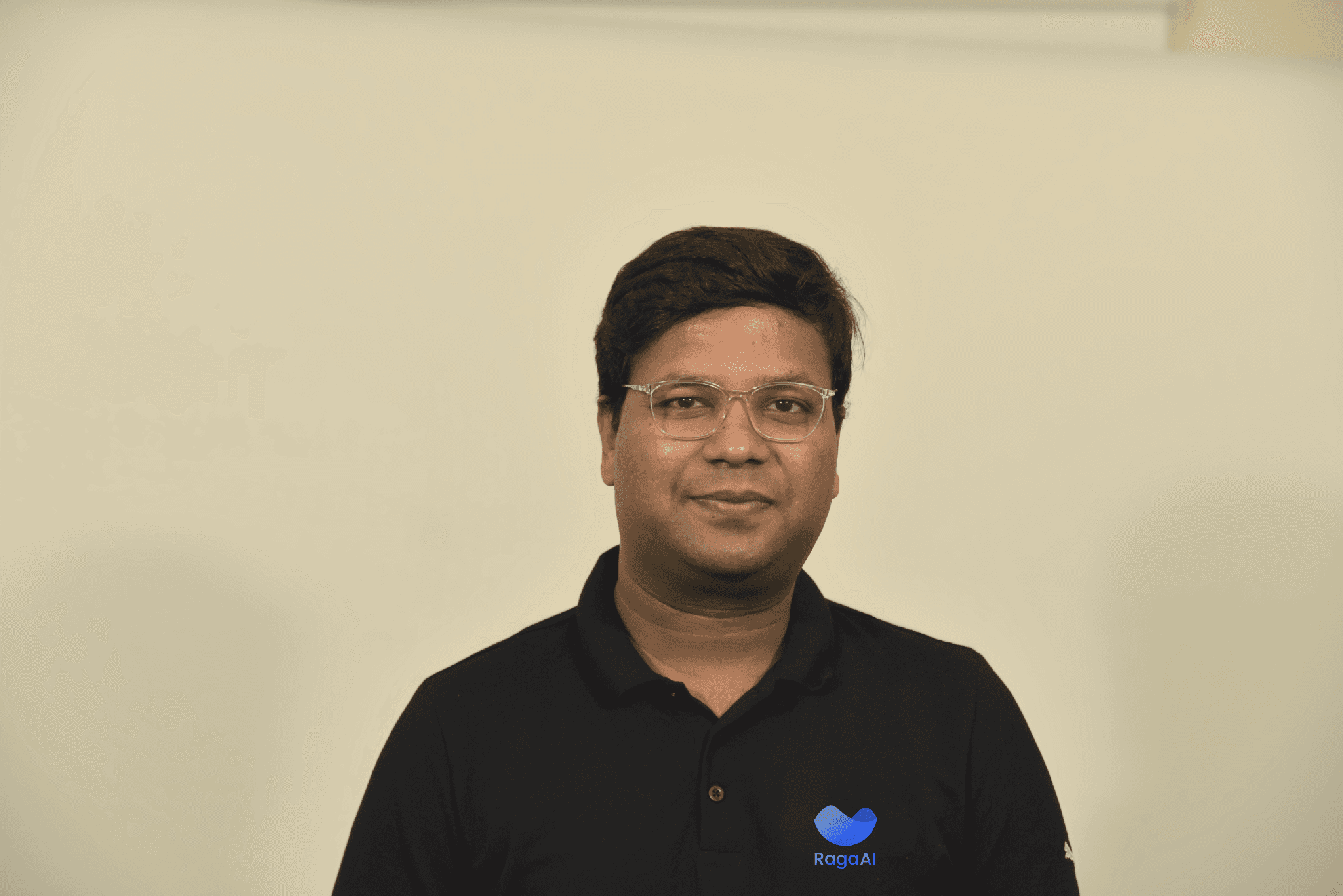
Jigar Gupta
Oct 24, 2024
Read the article




Data Security: Risks, Solutions, Types and Best Practices
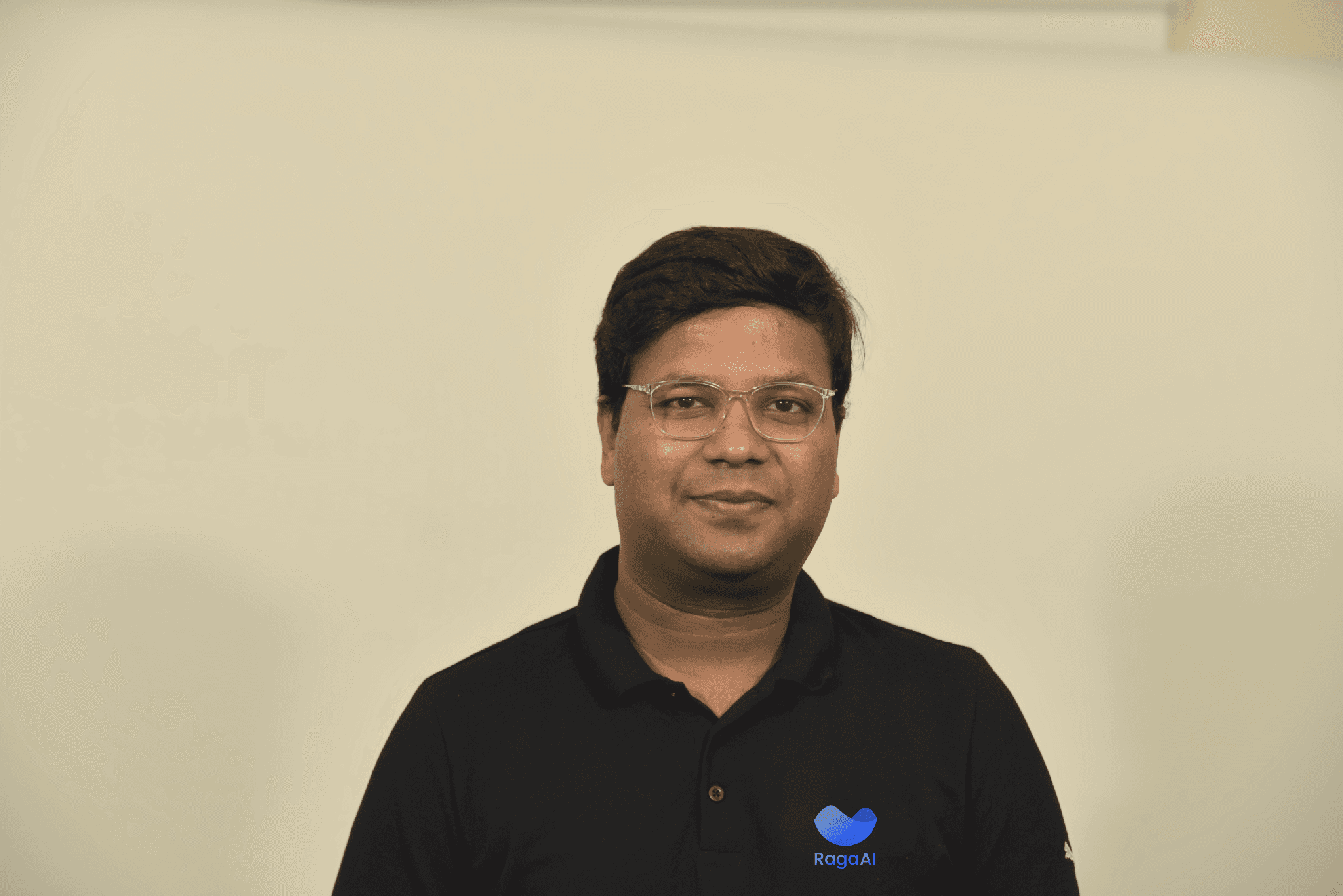
Jigar Gupta
Oct 21, 2024
Read the article
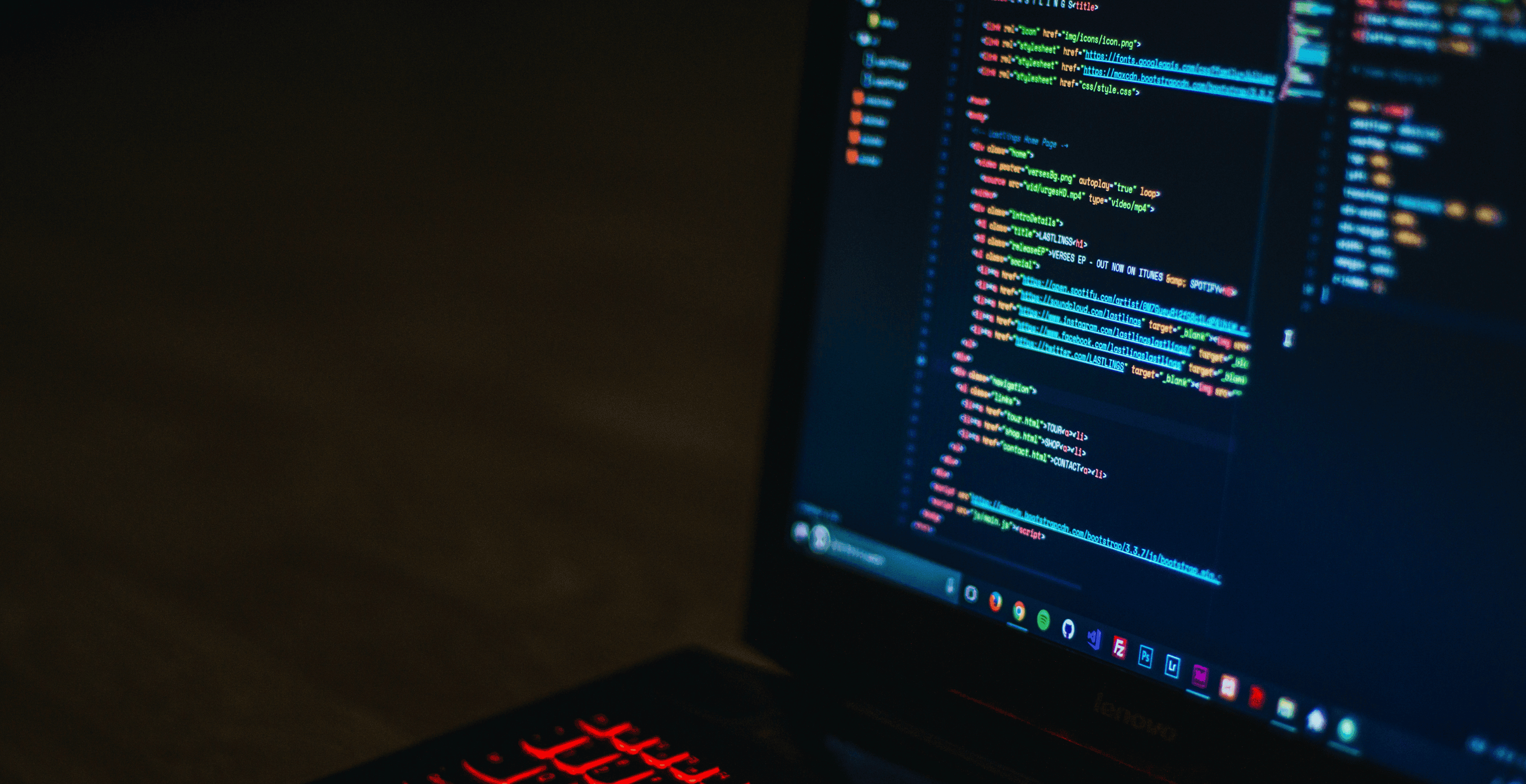
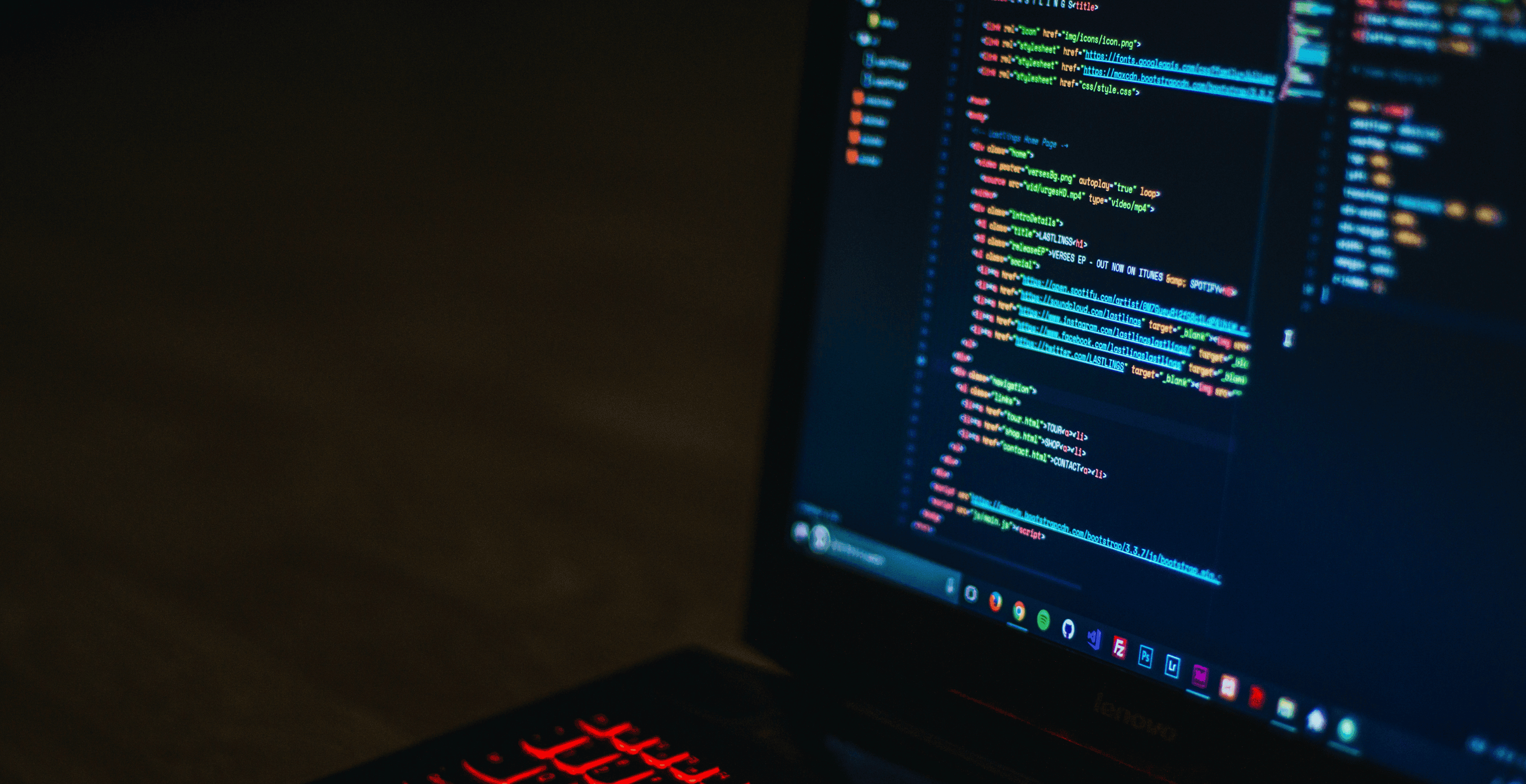
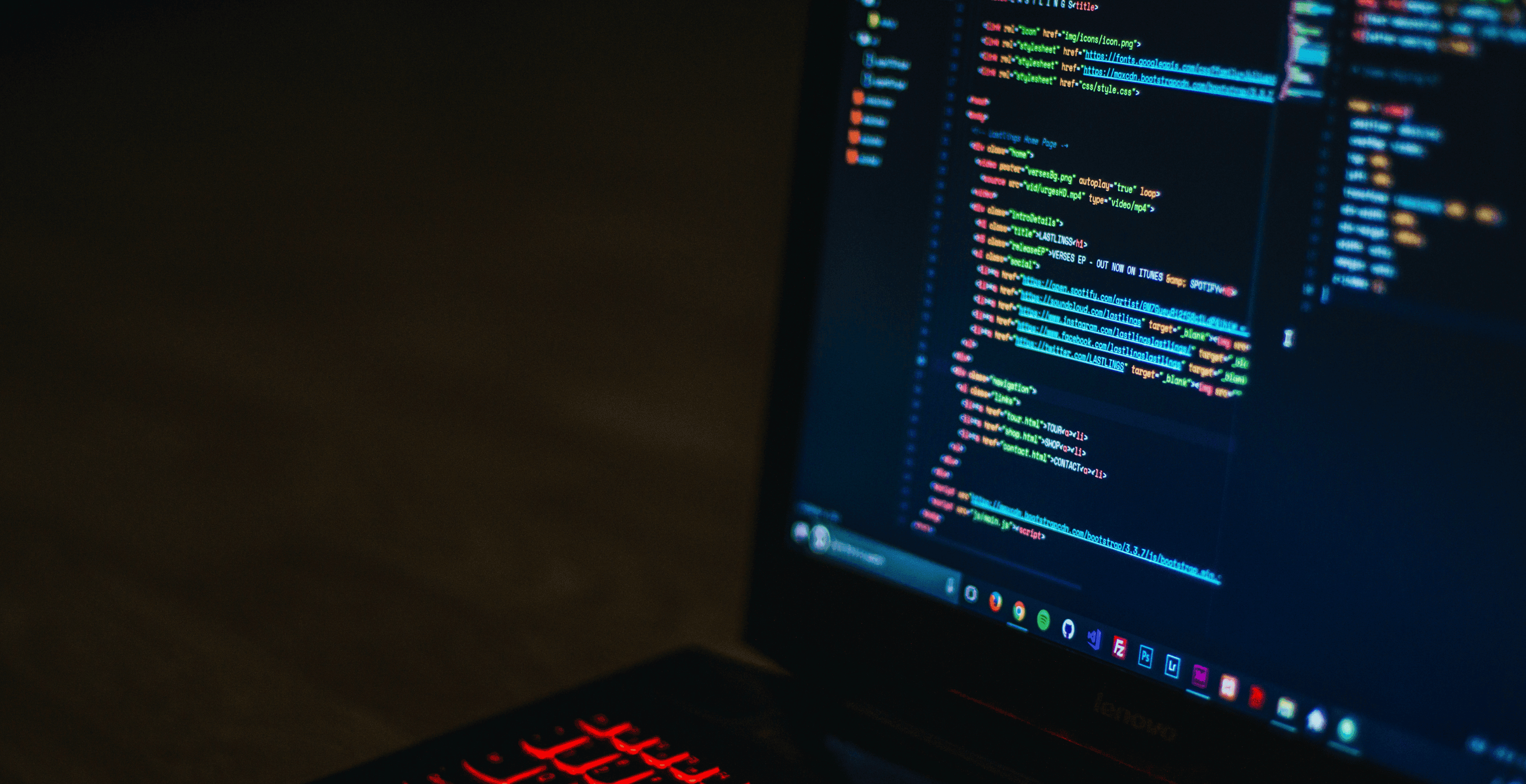
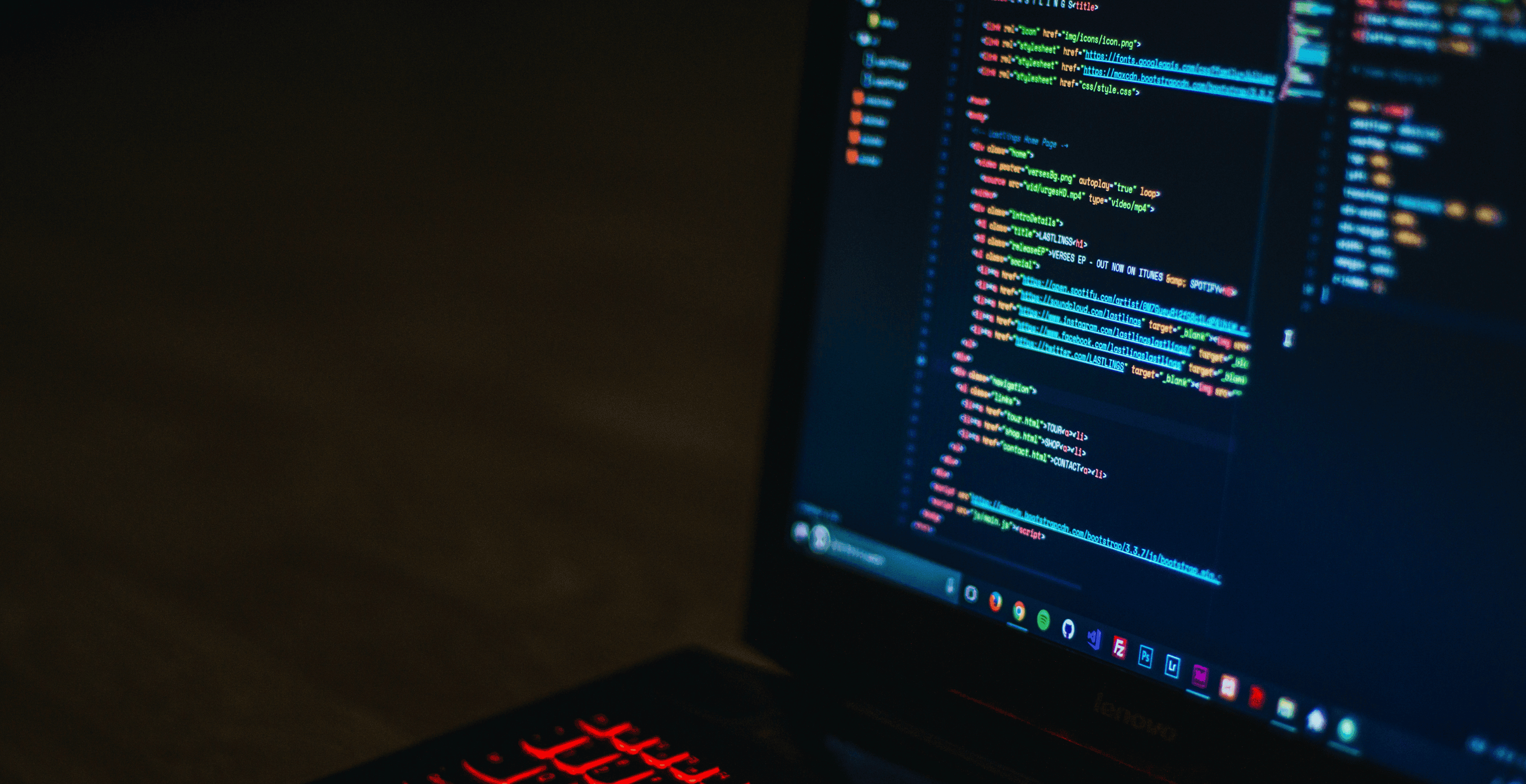
Getting Contextual Understanding Right for RAG Applications
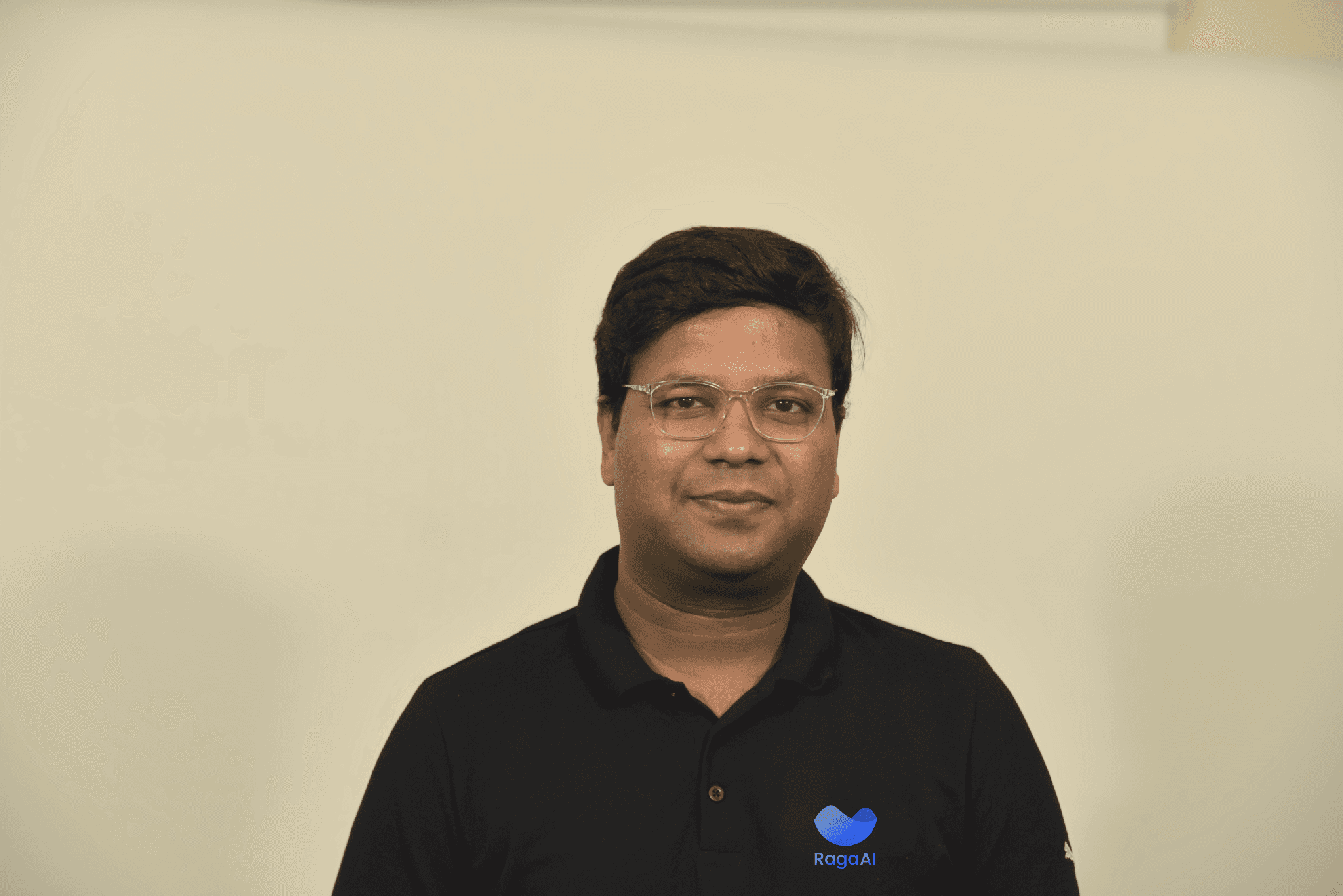
Jigar Gupta
Oct 19, 2024
Read the article
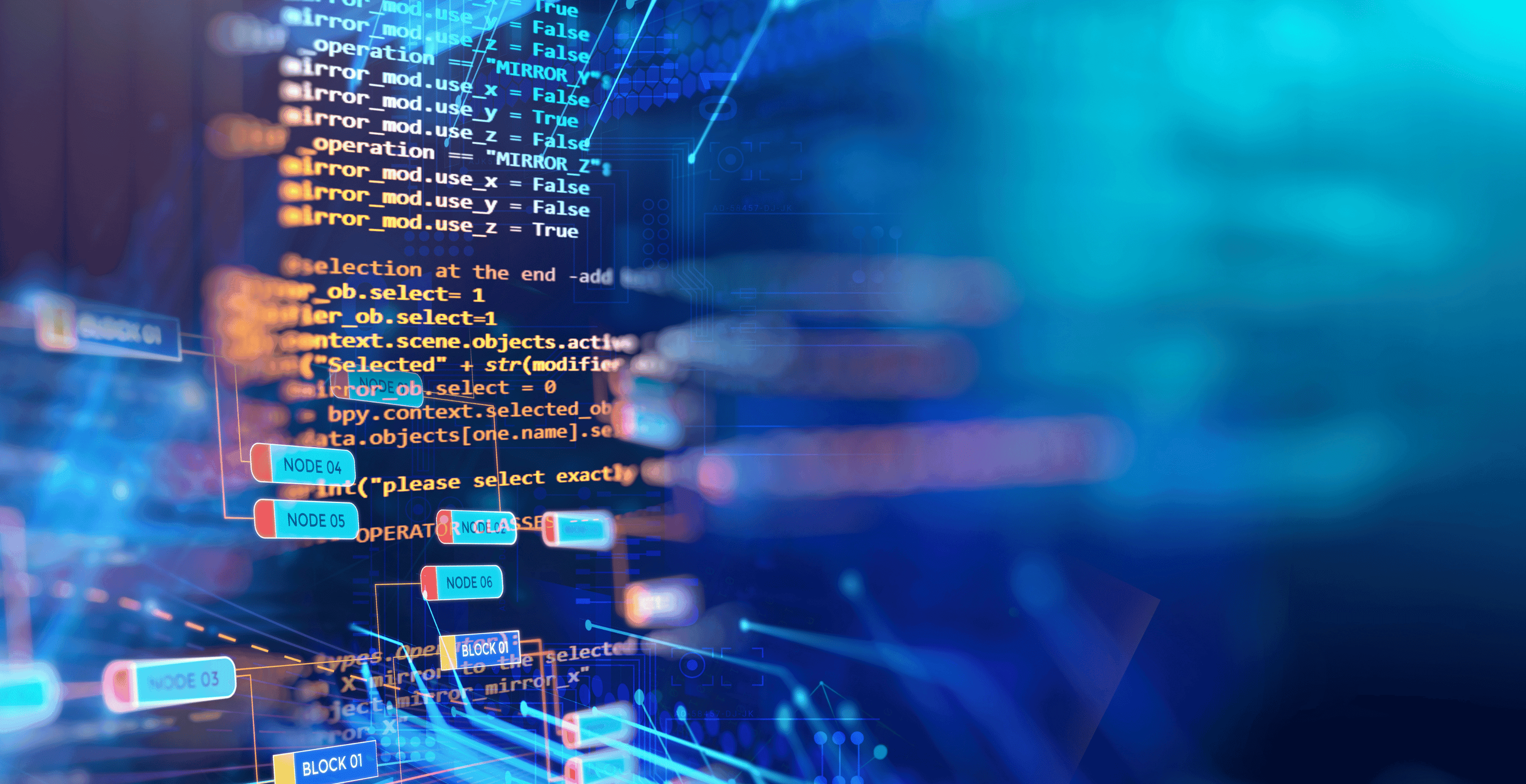
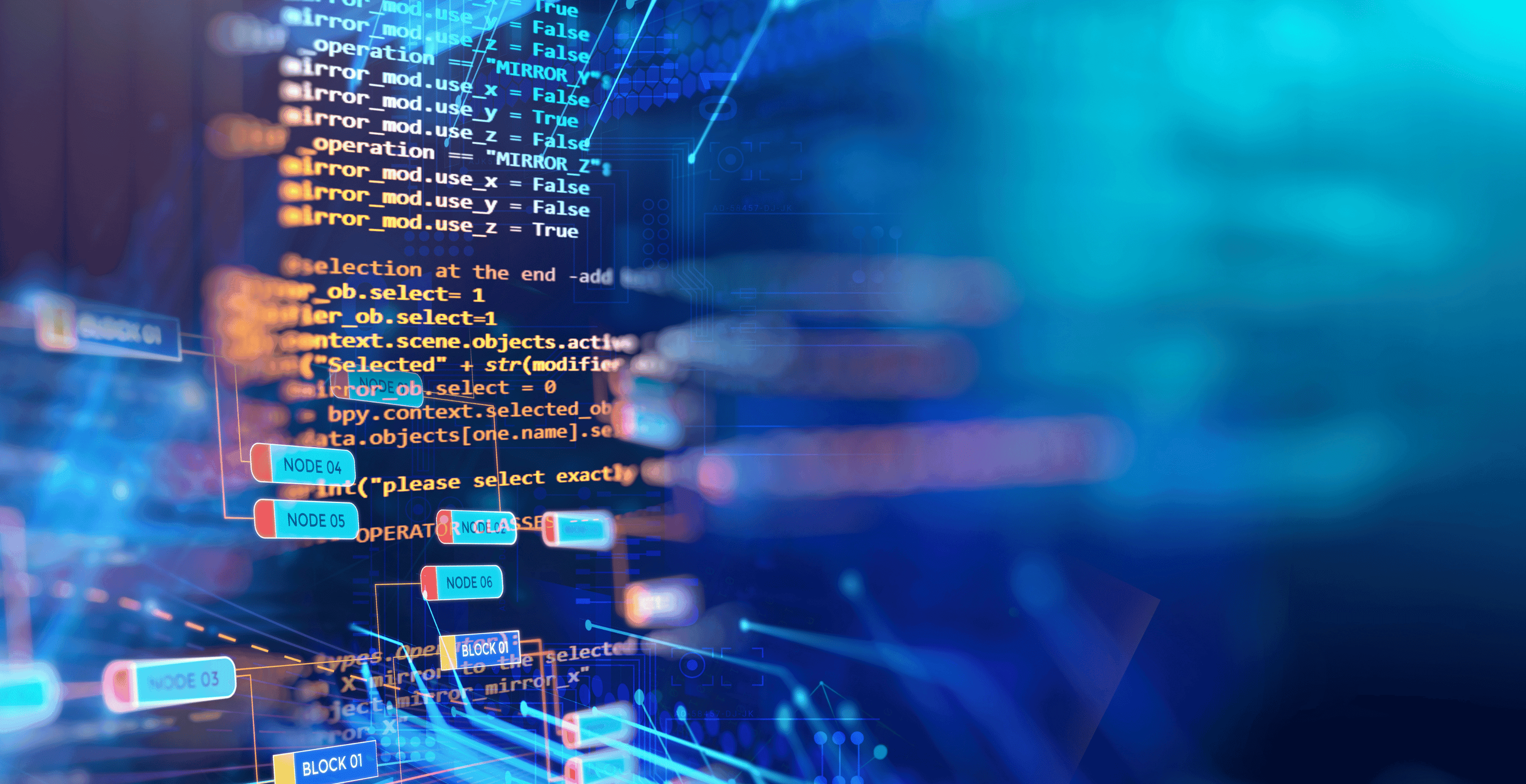
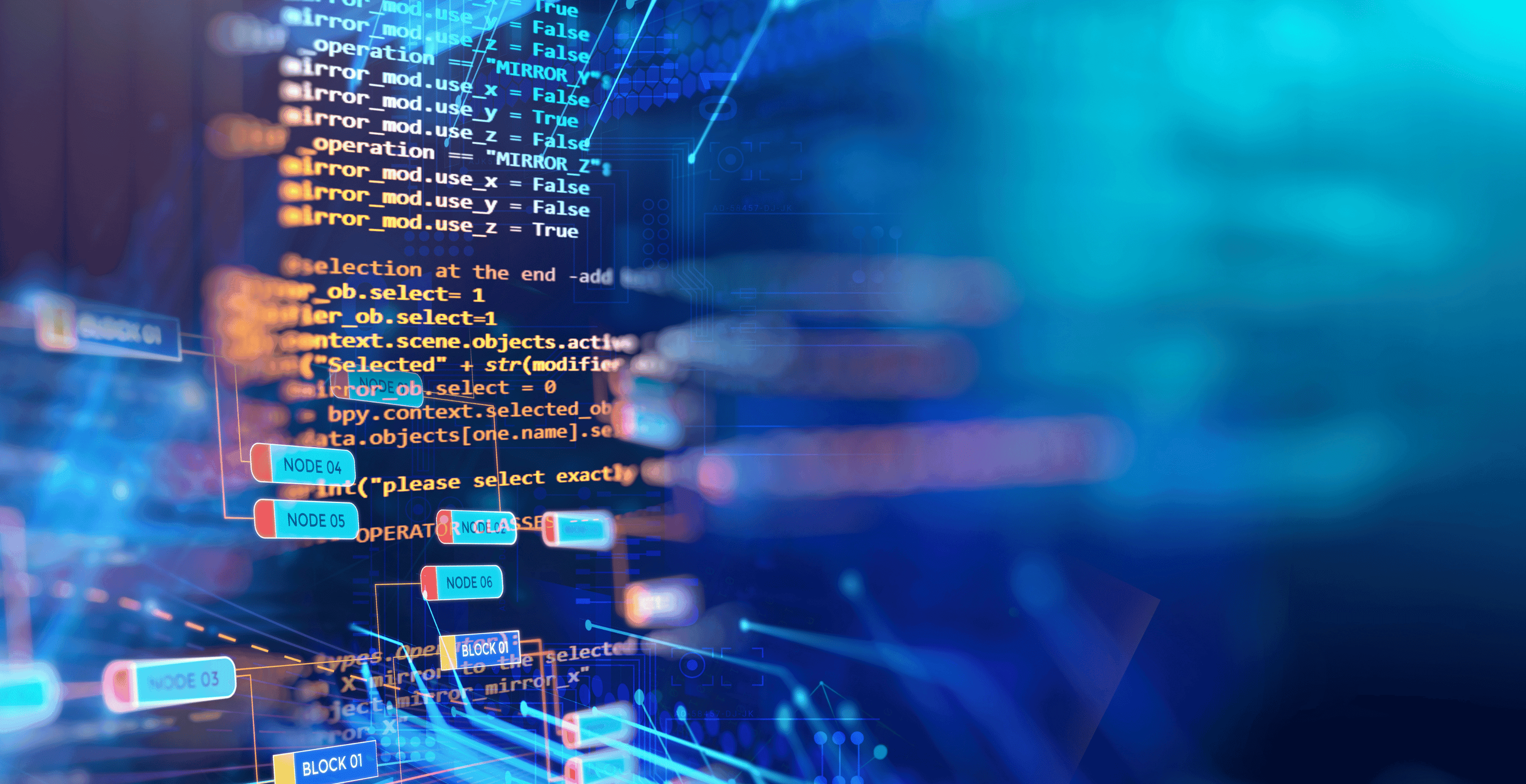
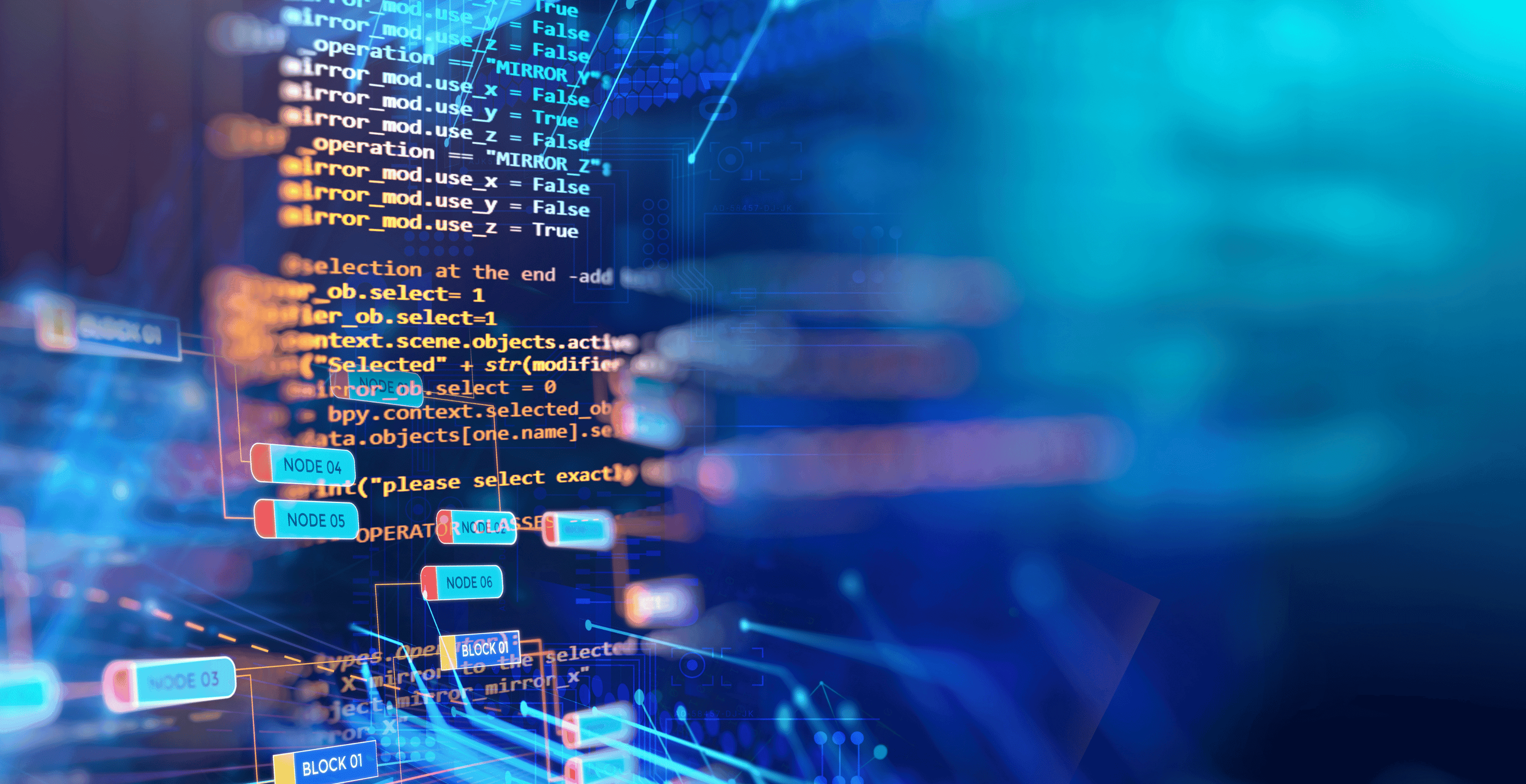
Understanding Data Fragmentation and Strategies to Overcome It
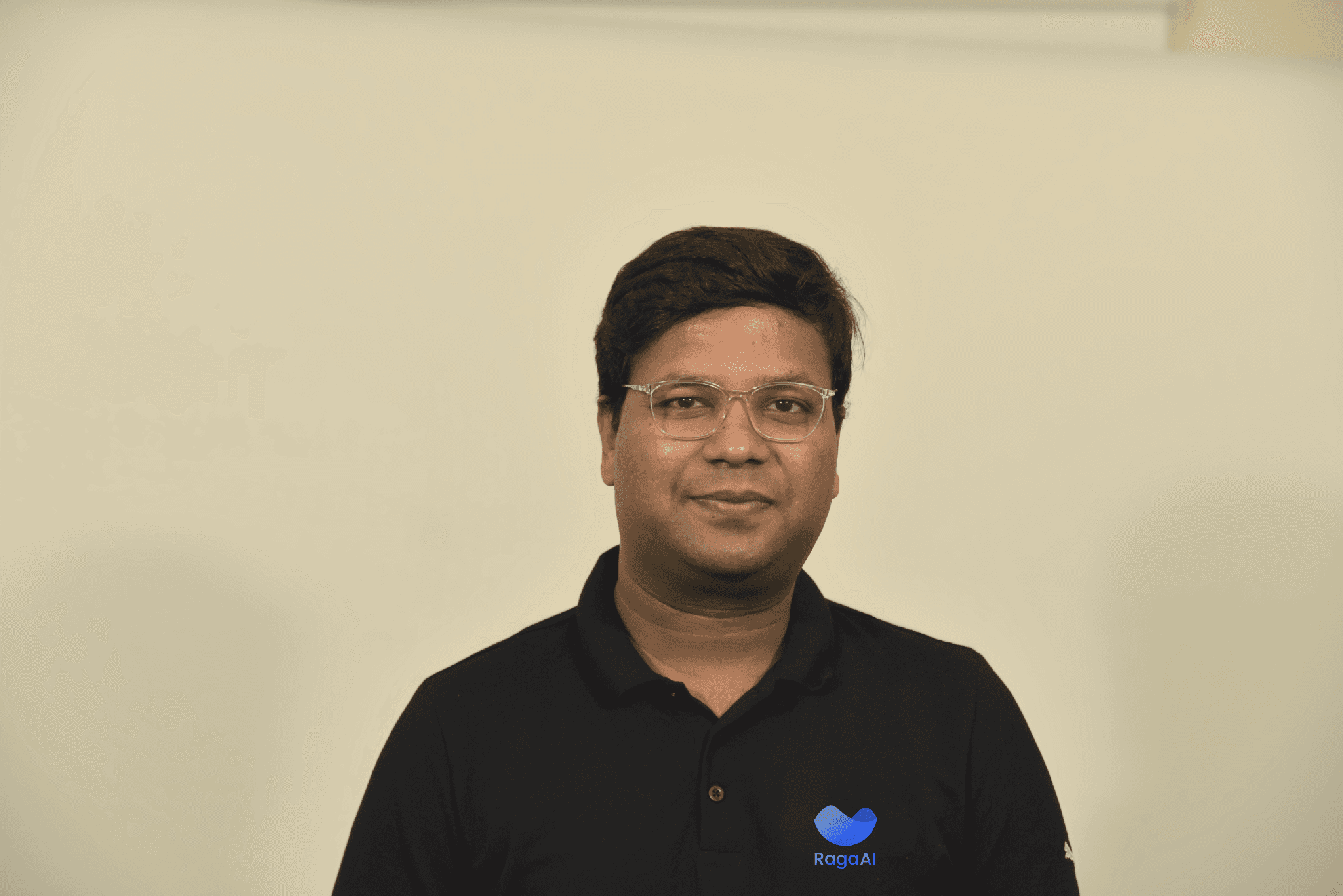
Jigar Gupta
Oct 16, 2024
Read the article
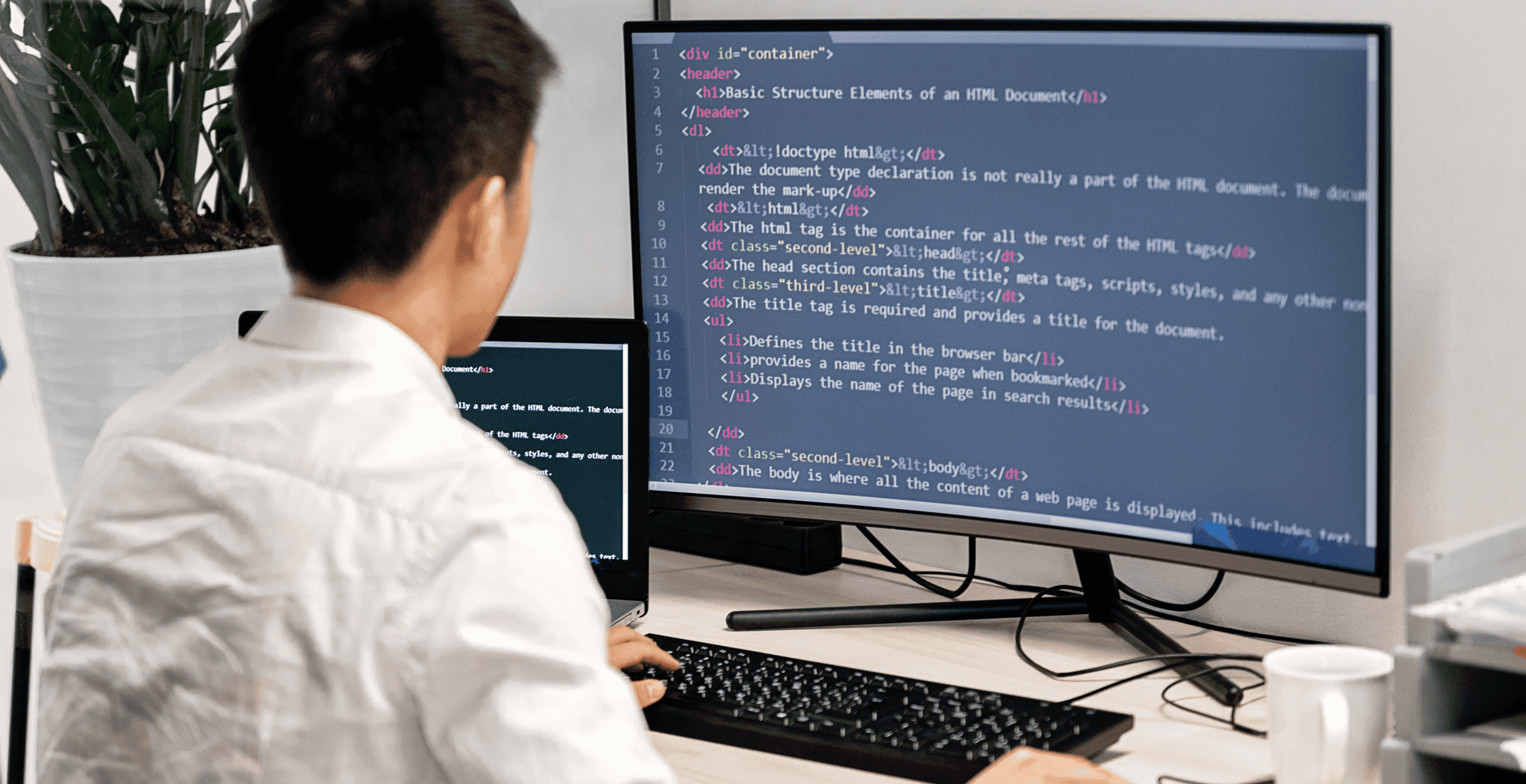
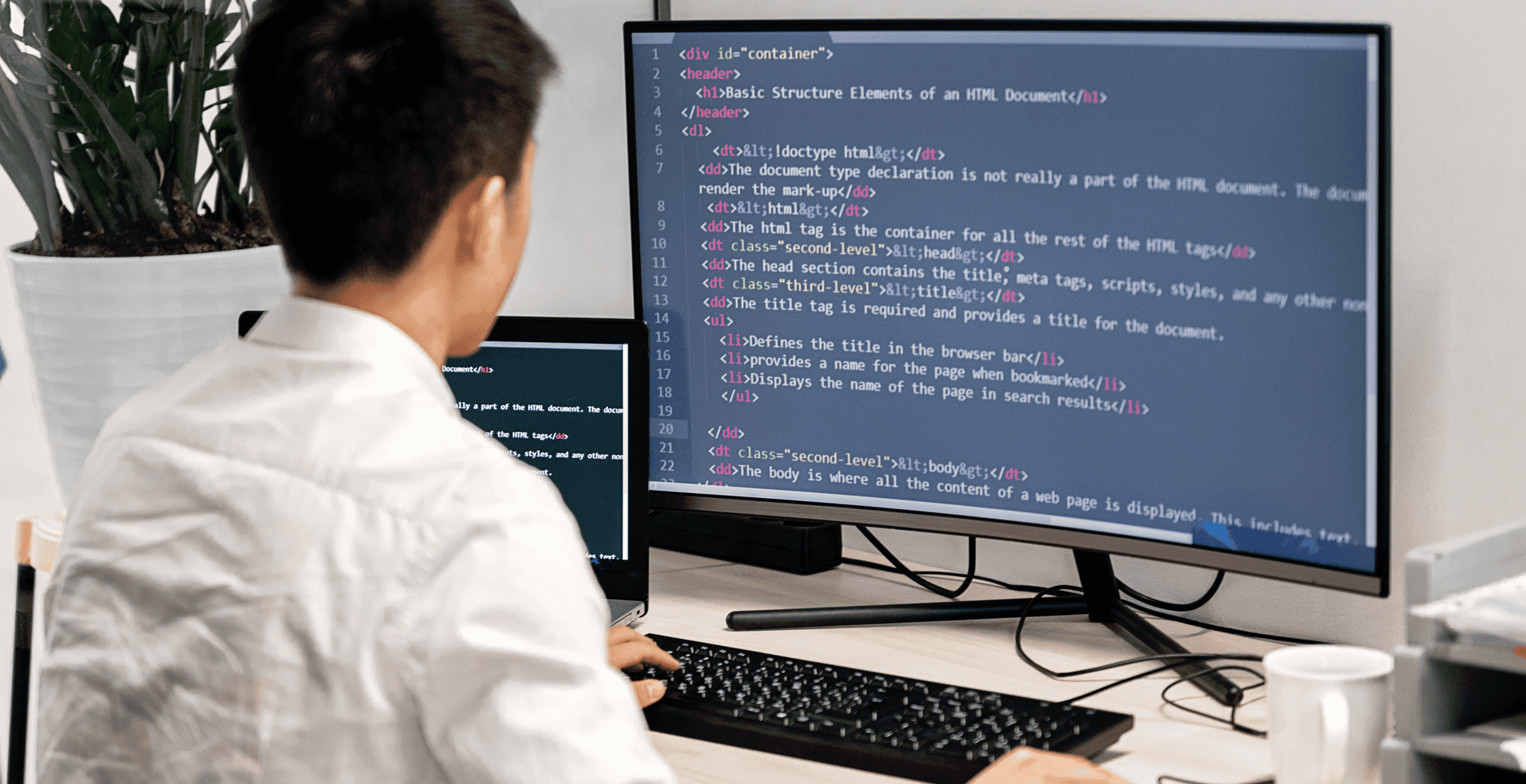
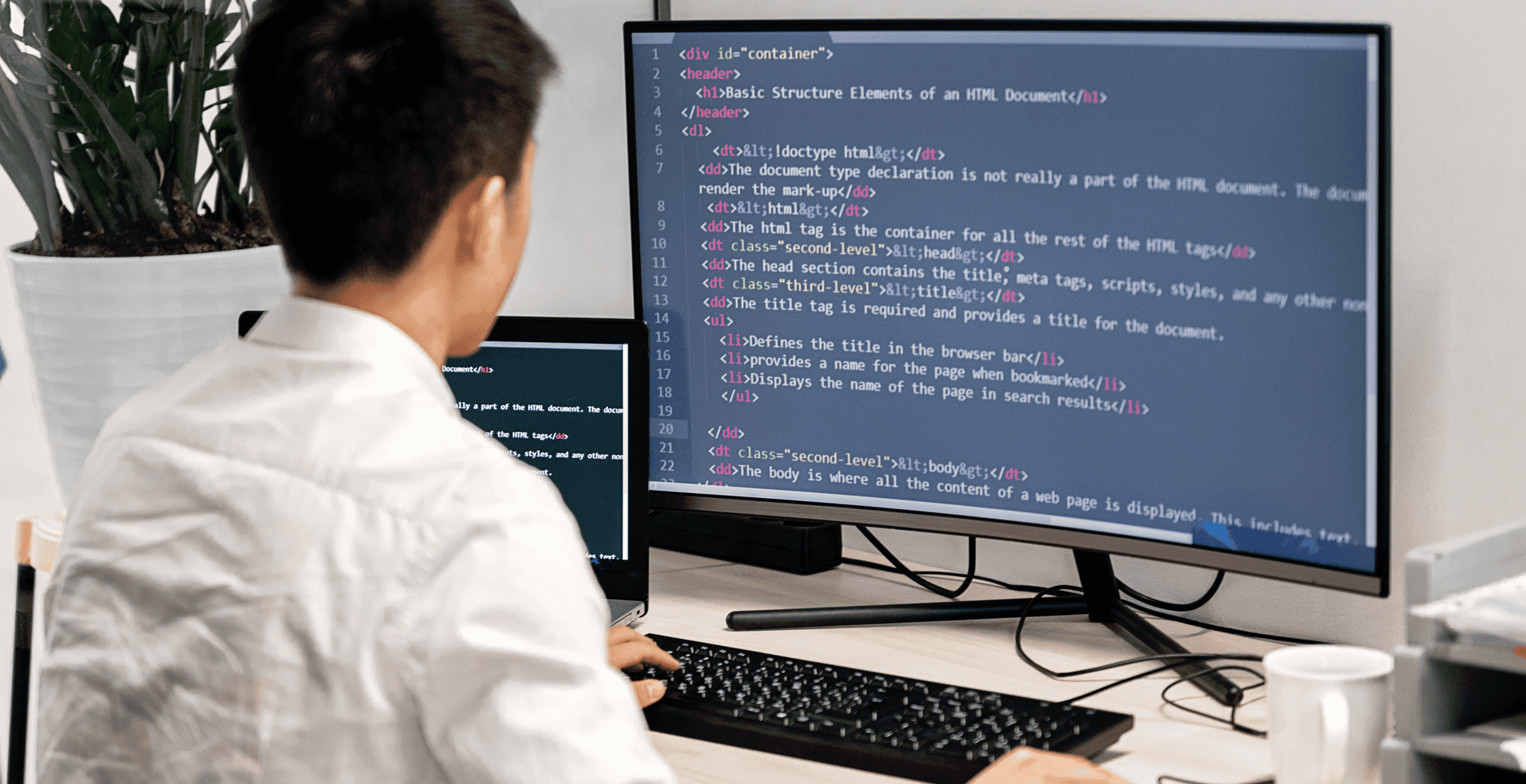
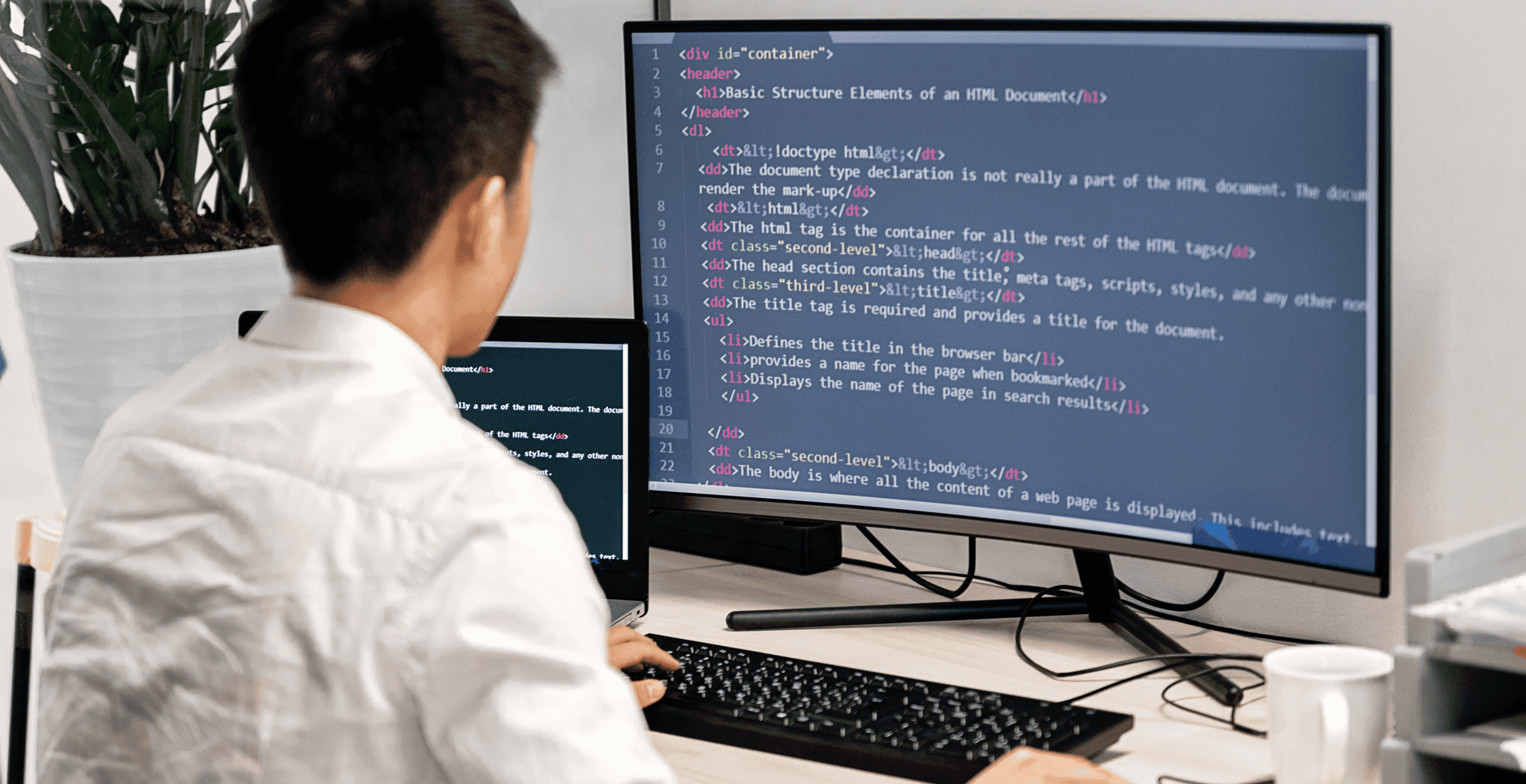
Understanding Techniques and Applications for Grounding LLMs in Data

Rehan Asif
Oct 13, 2024
Read the article




Advantages Of Using LLMs For Rapid Application Development

Rehan Asif
Oct 10, 2024
Read the article
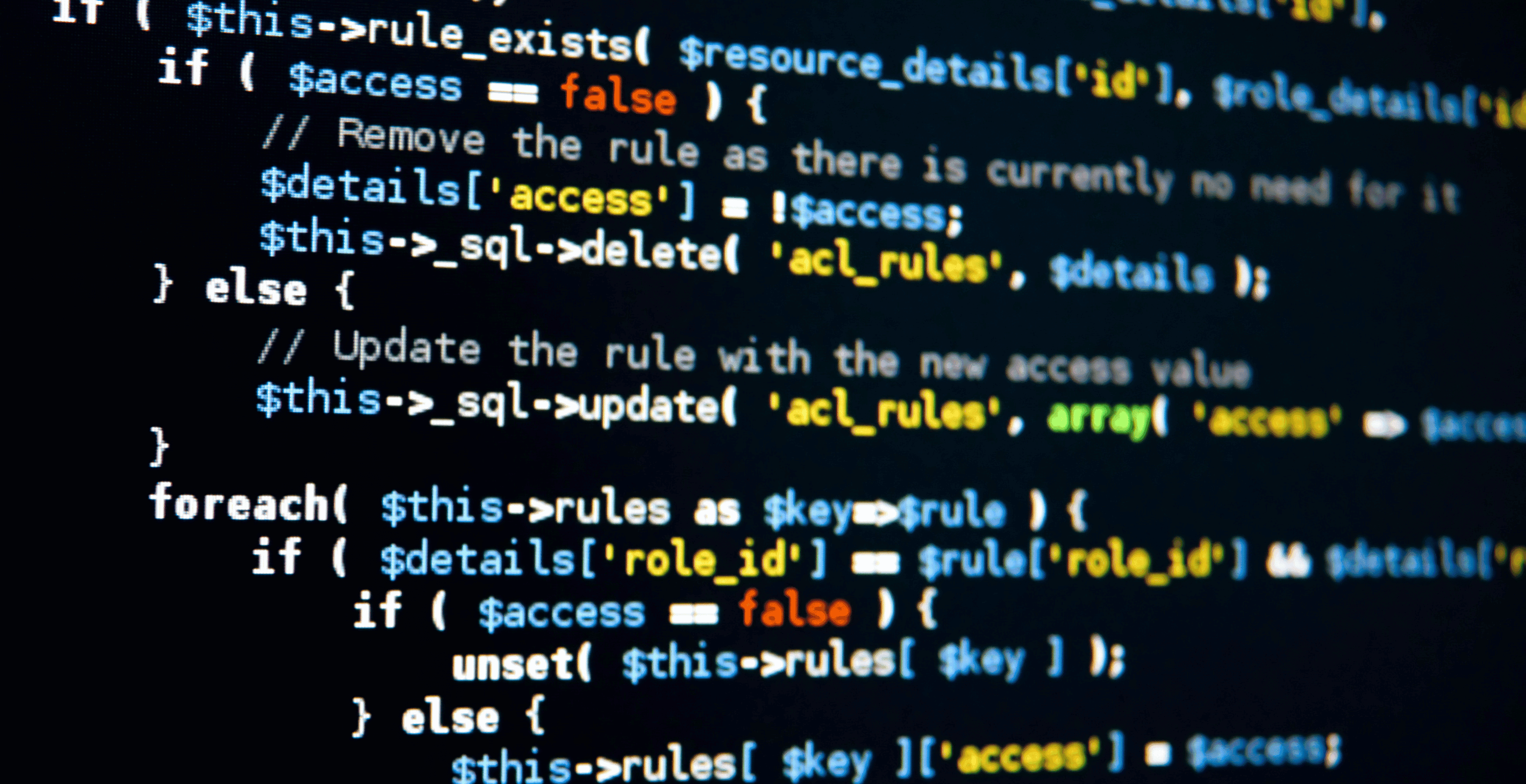
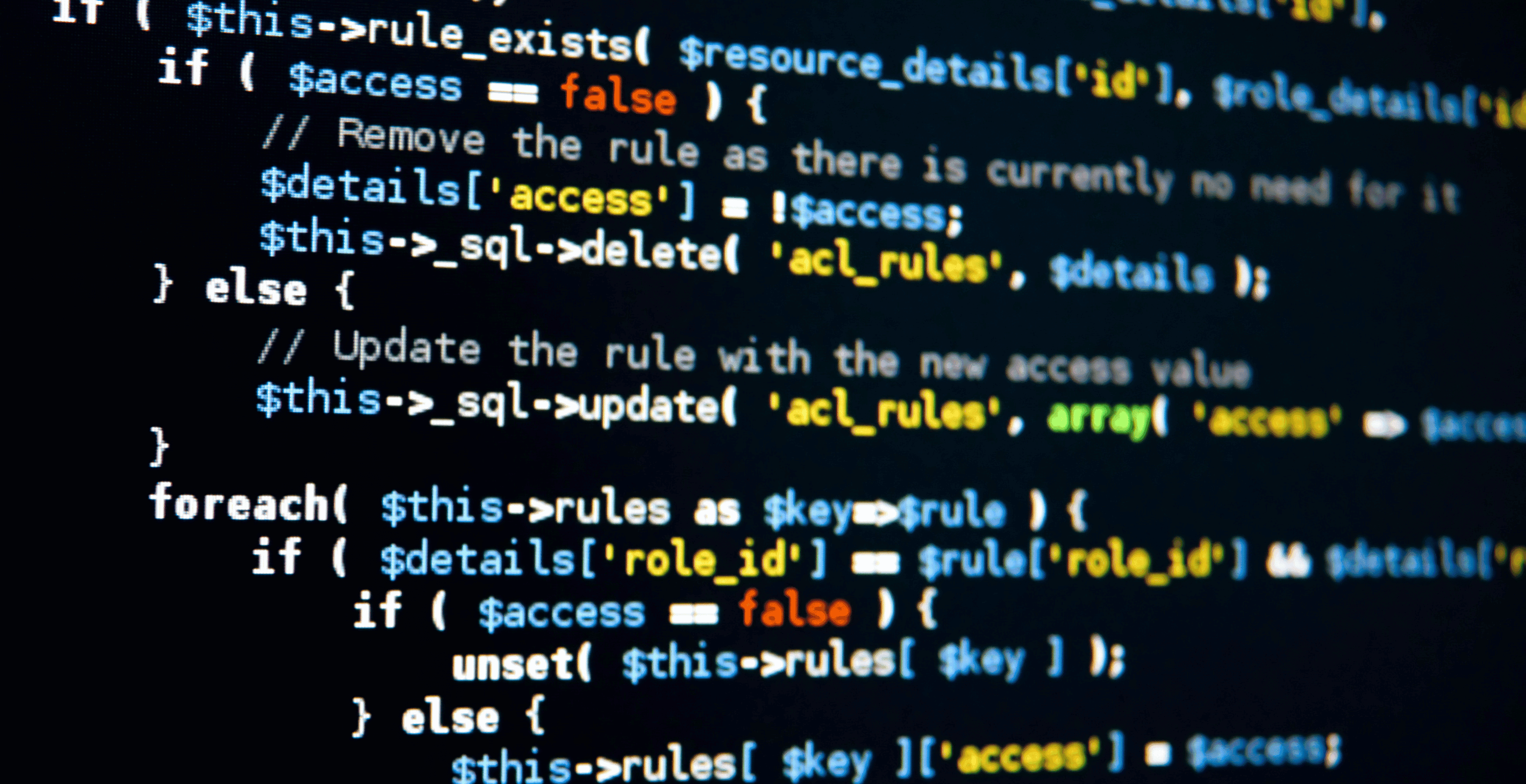
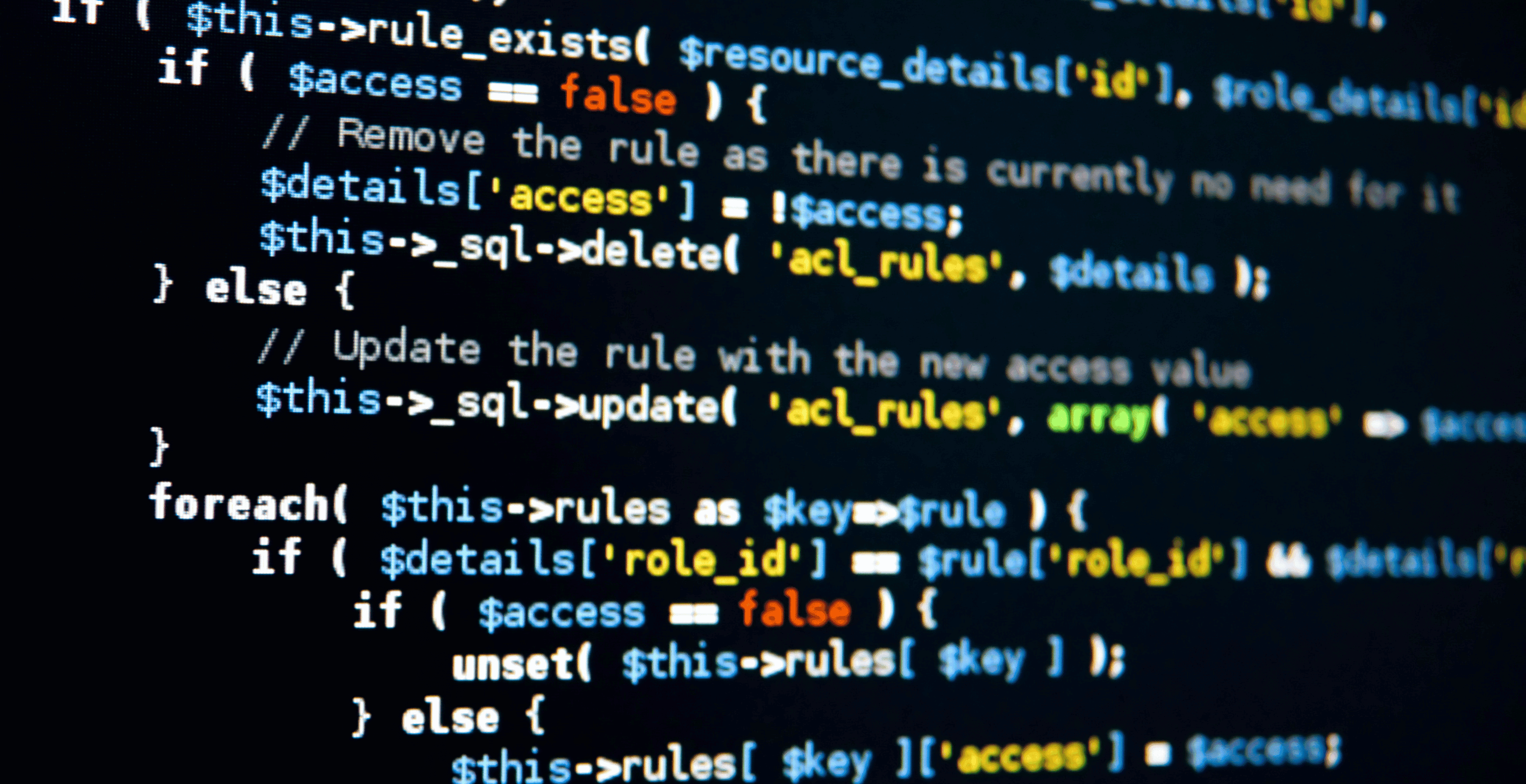
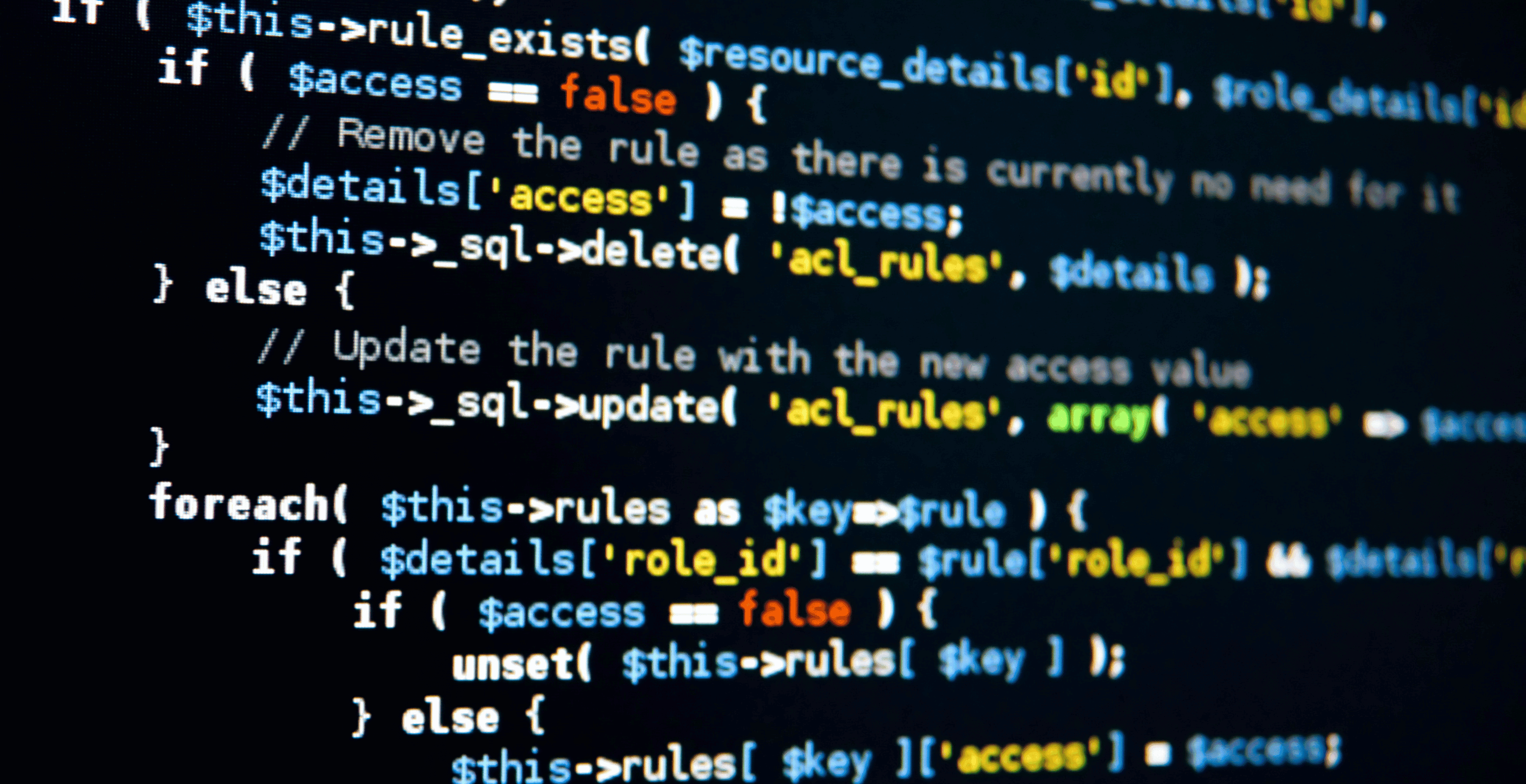
Understanding React Agent in LangChain Engineering

Rehan Asif
Oct 7, 2024
Read the article
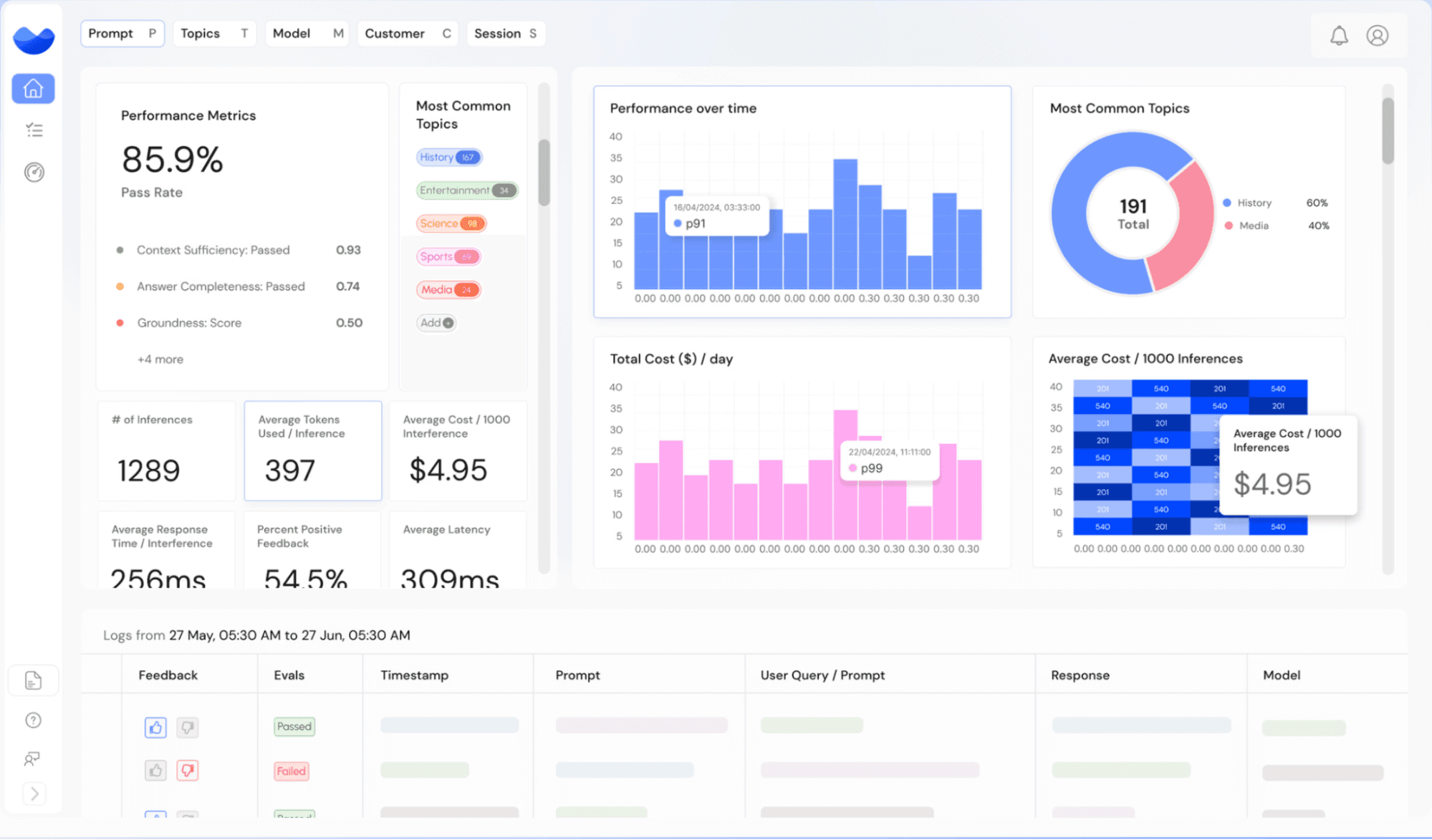
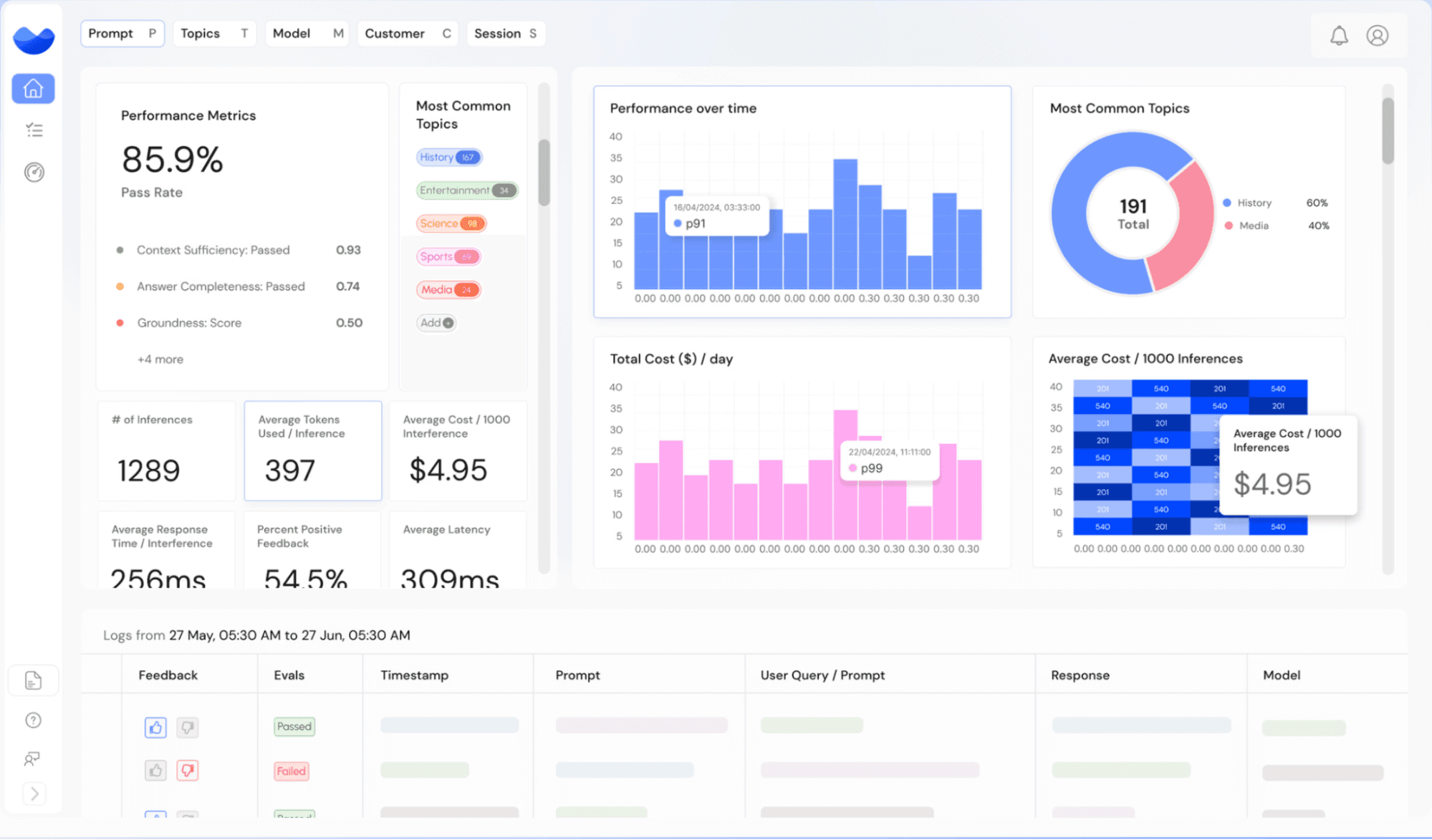
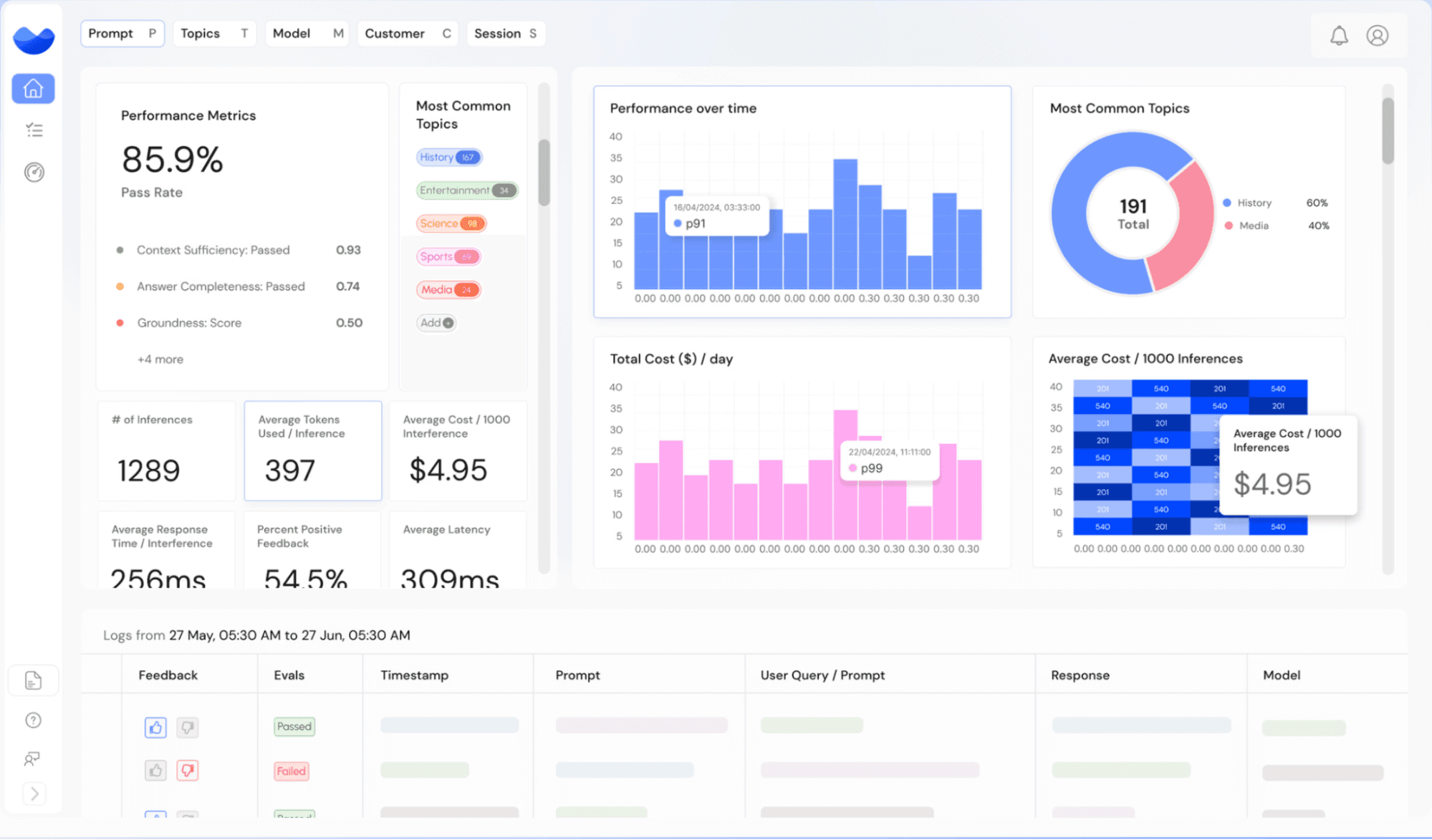
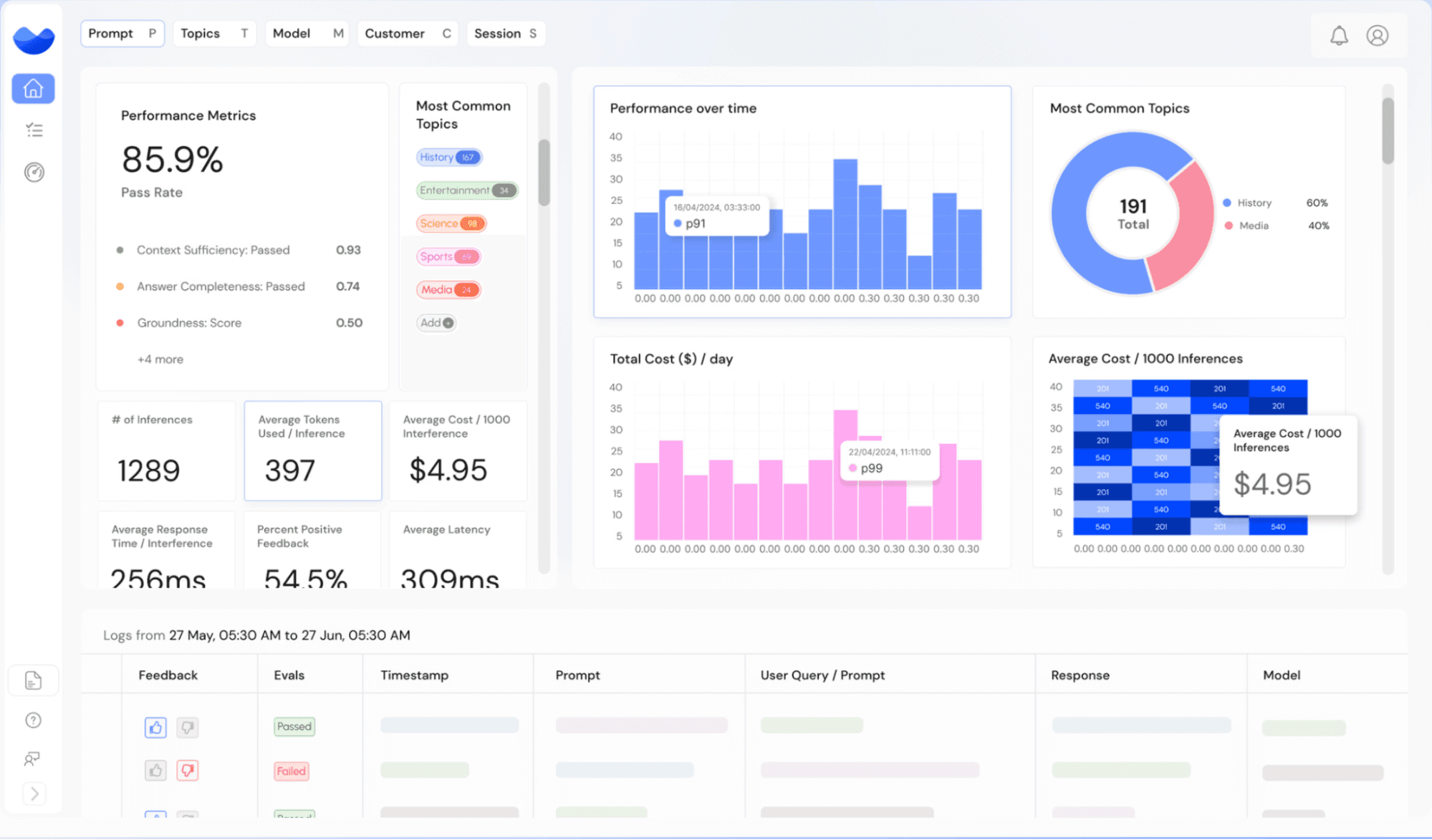
Using RagaAI Catalyst to Evaluate LLM Applications

Gaurav Agarwal
Oct 4, 2024
Read the article




Step-by-Step Guide on Training Large Language Models

Rehan Asif
Oct 1, 2024
Read the article
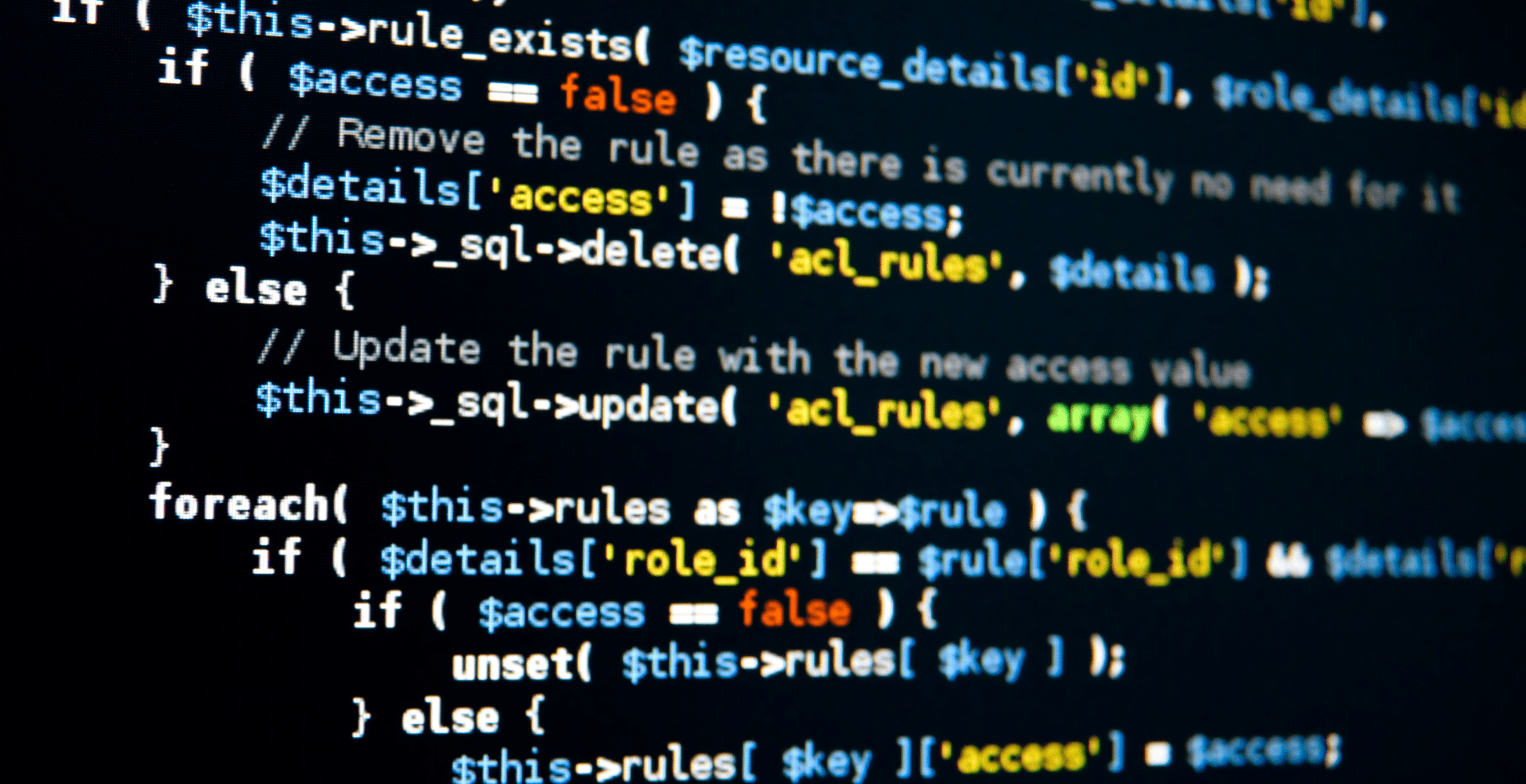
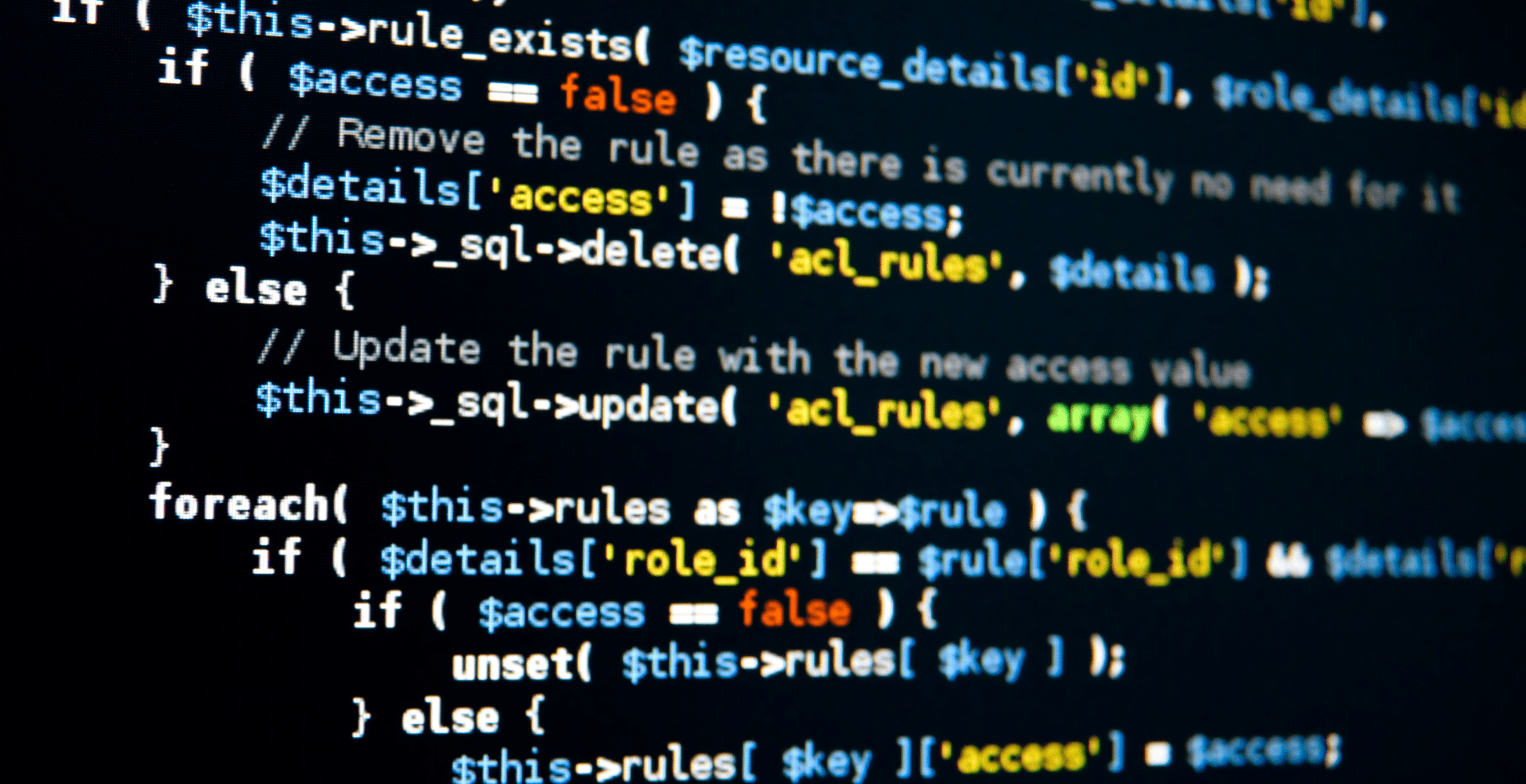
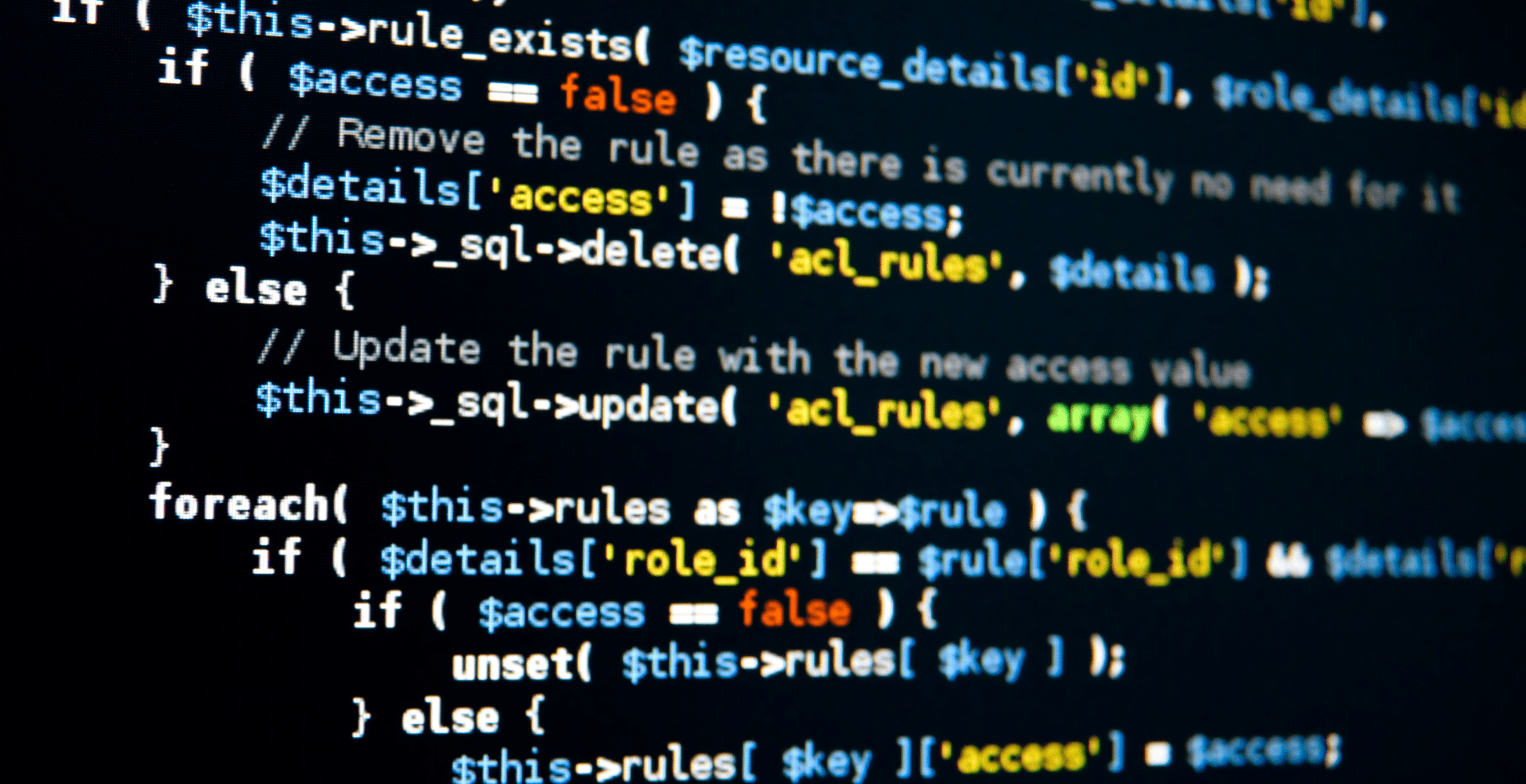
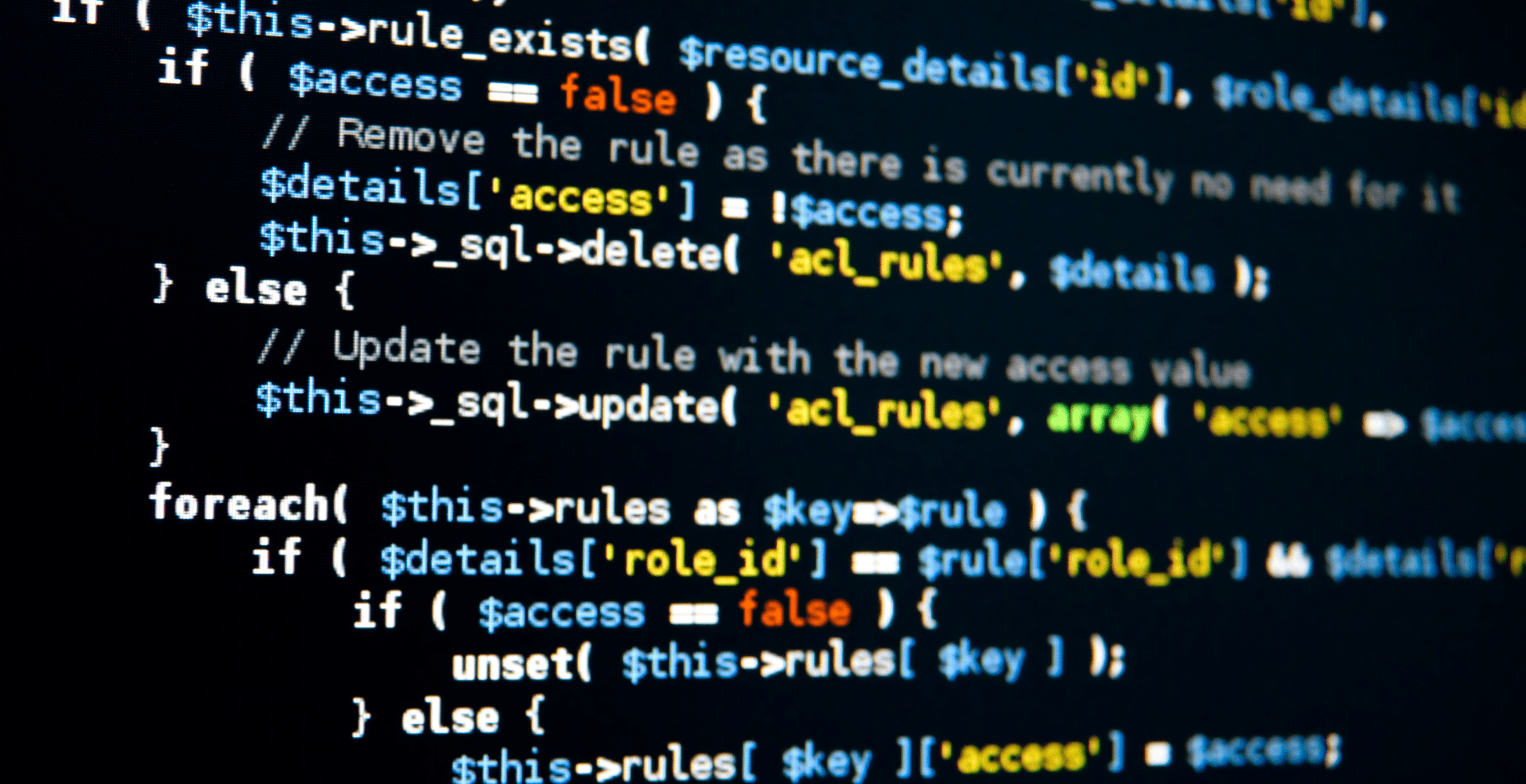
Understanding LLM Agent Architecture

Rehan Asif
Aug 19, 2024
Read the article




Understanding the Need and Possibilities of AI Guardrails Today
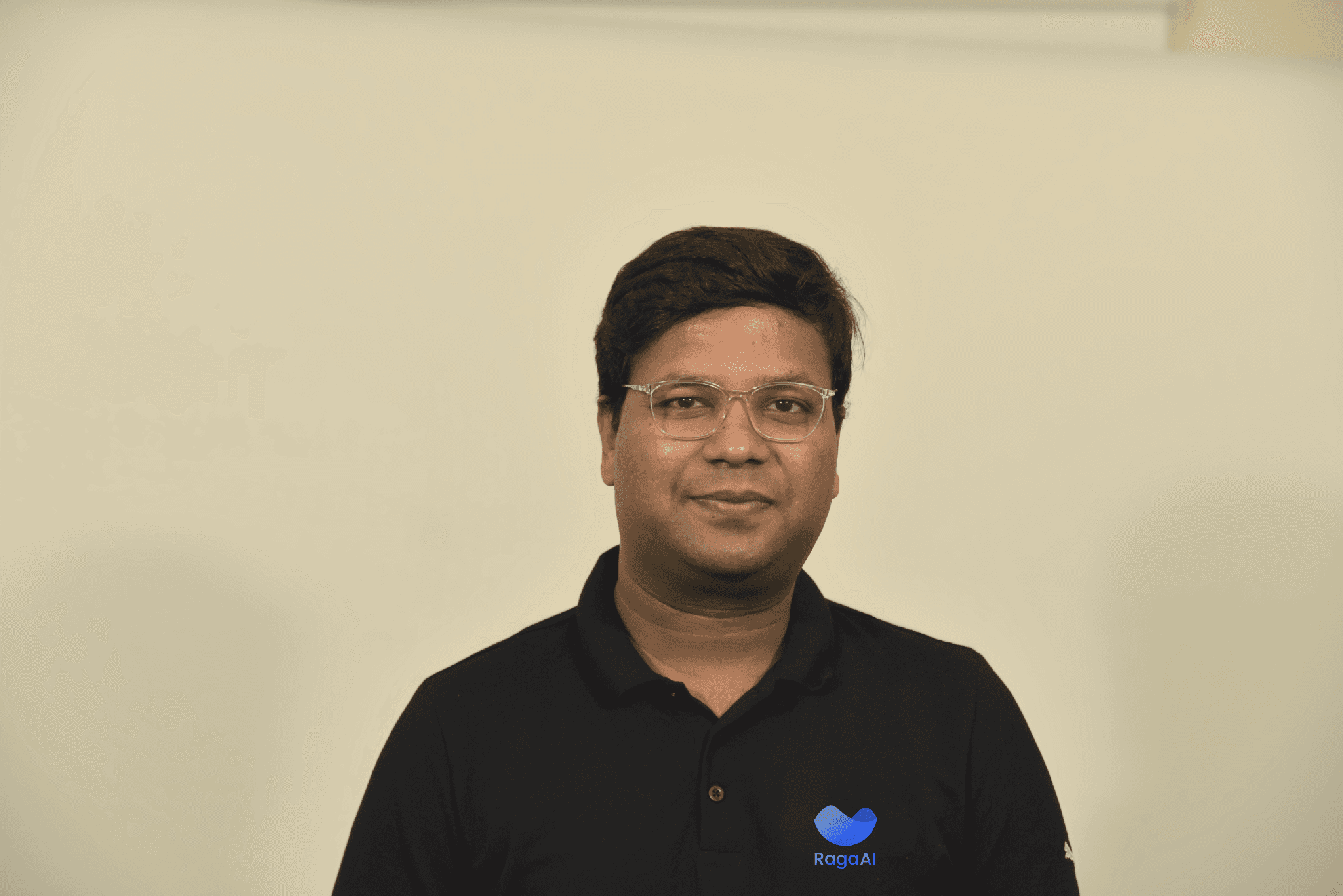
Jigar Gupta
Aug 19, 2024
Read the article




How to Prepare Quality Dataset for LLM Training

Rehan Asif
Aug 14, 2024
Read the article




Understanding Multi-Agent LLM Framework and Its Performance Scaling

Rehan Asif
Aug 15, 2024
Read the article
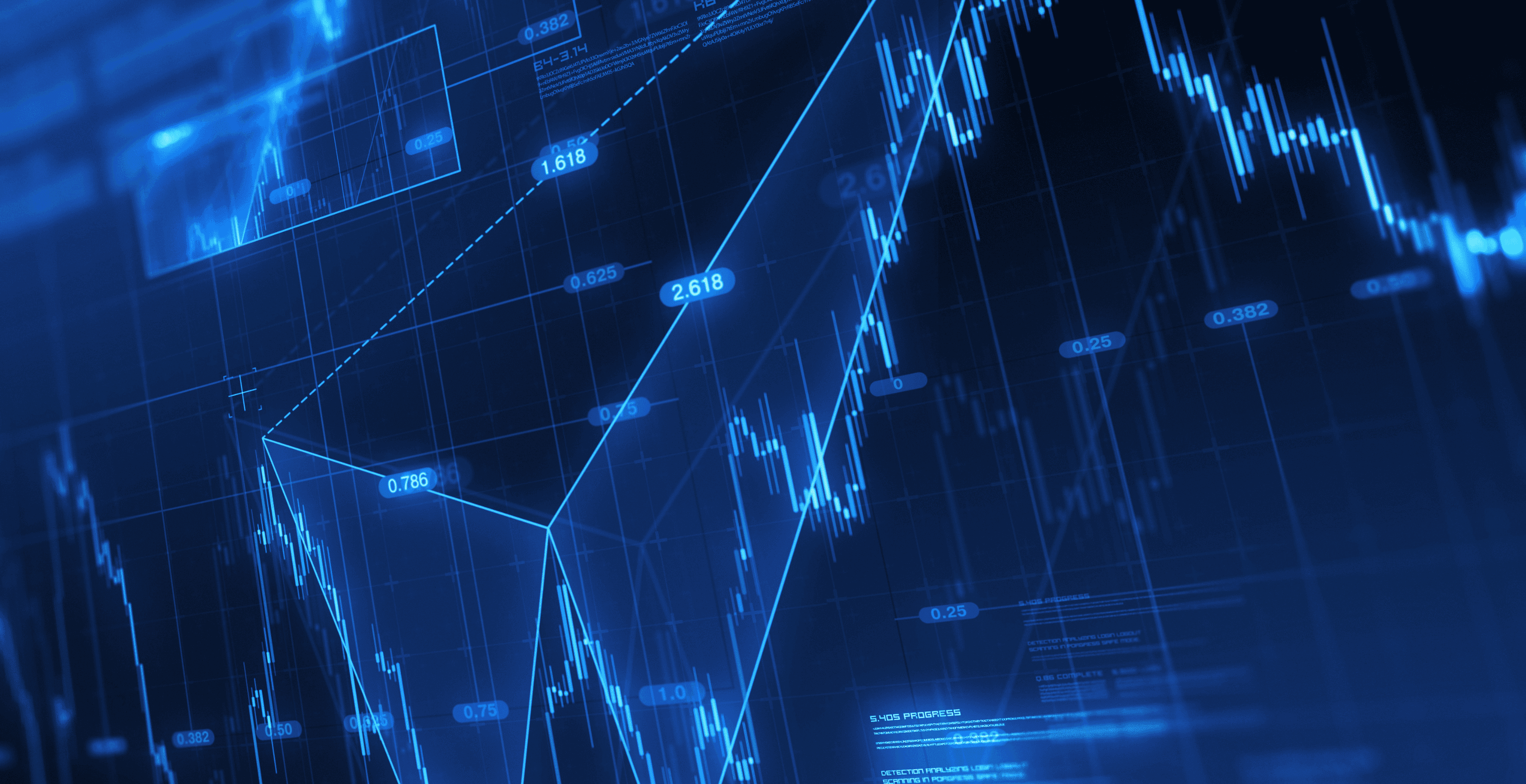
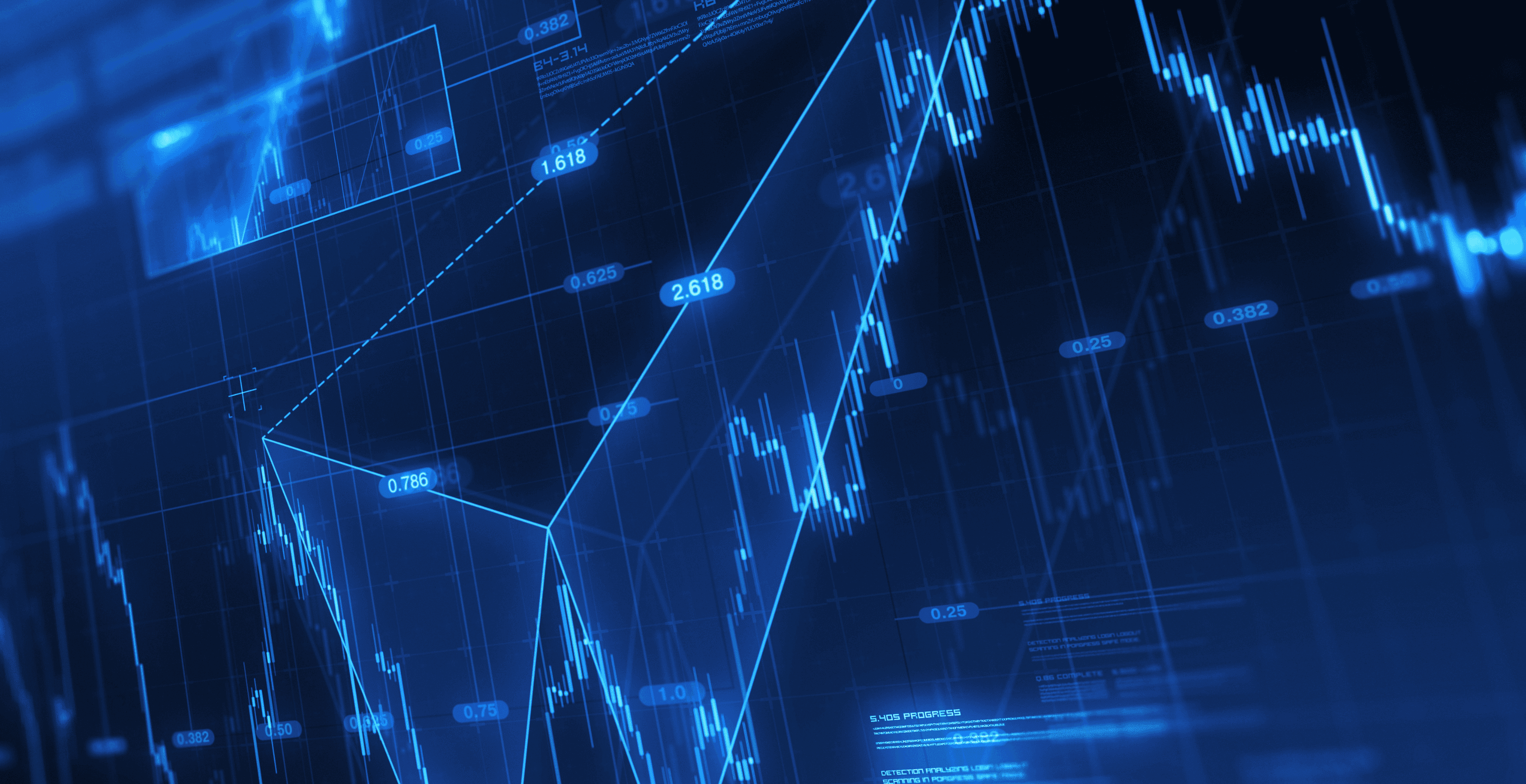
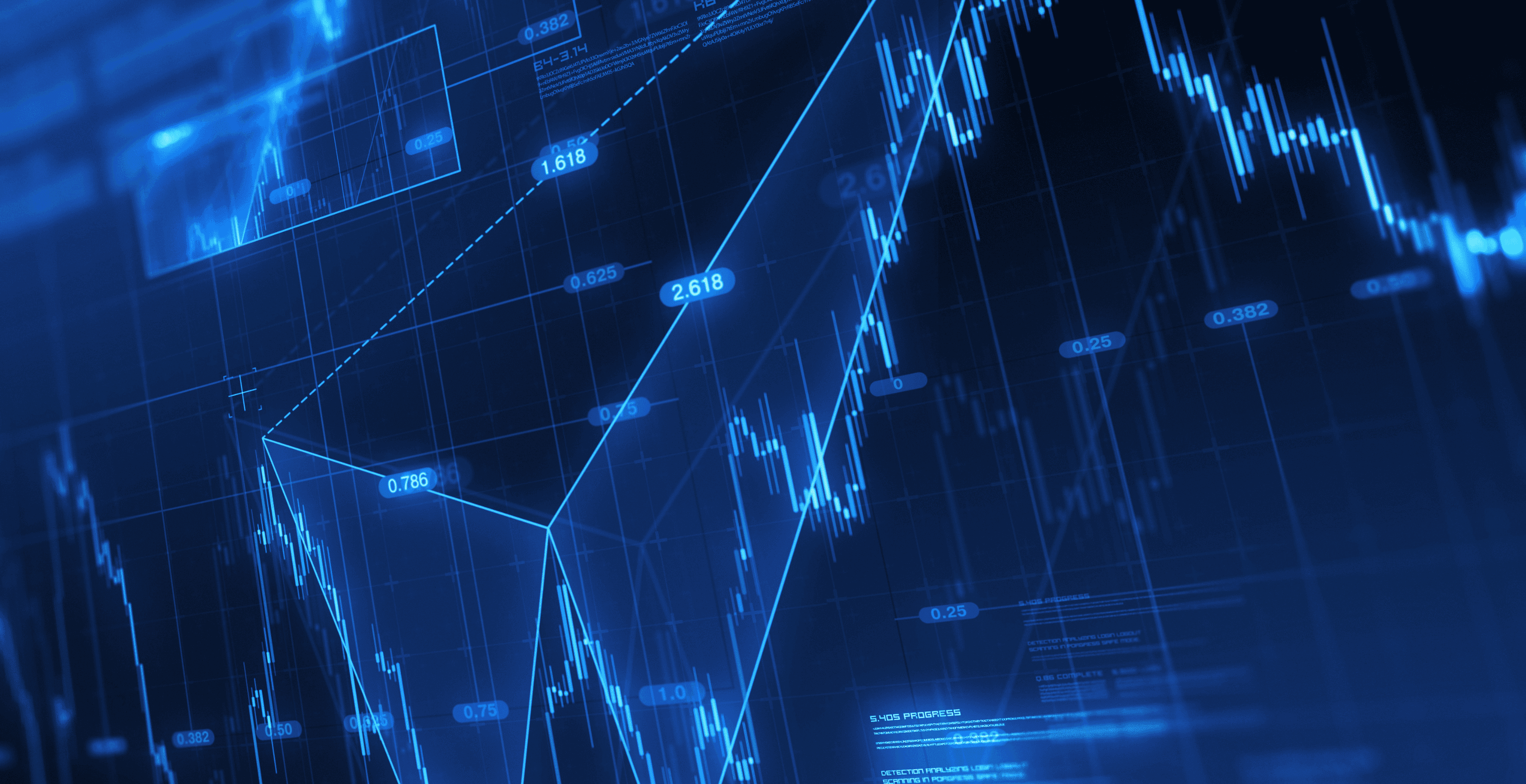
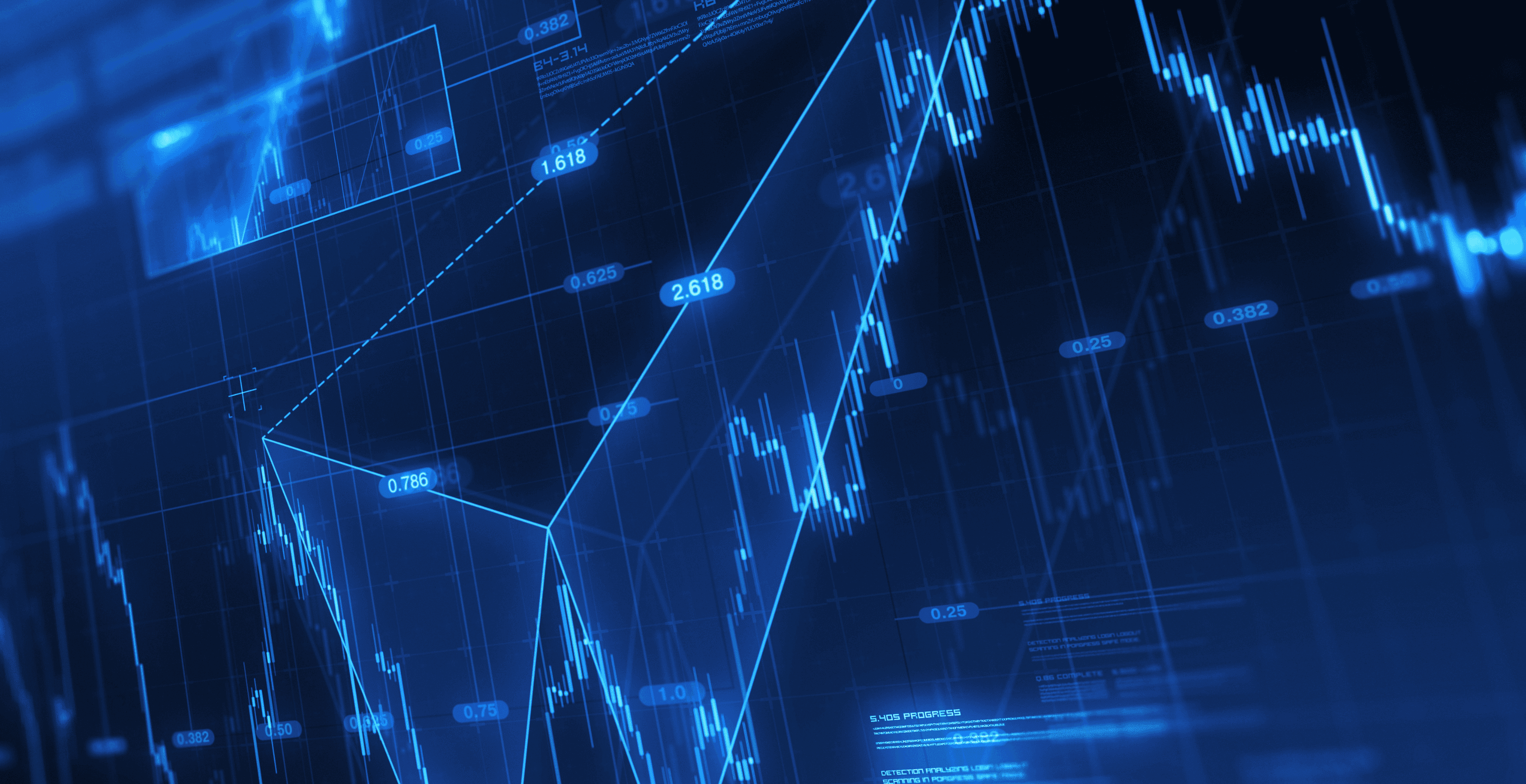
Understanding and Tackling Data Drift: Causes, Impact, and Automation Strategies
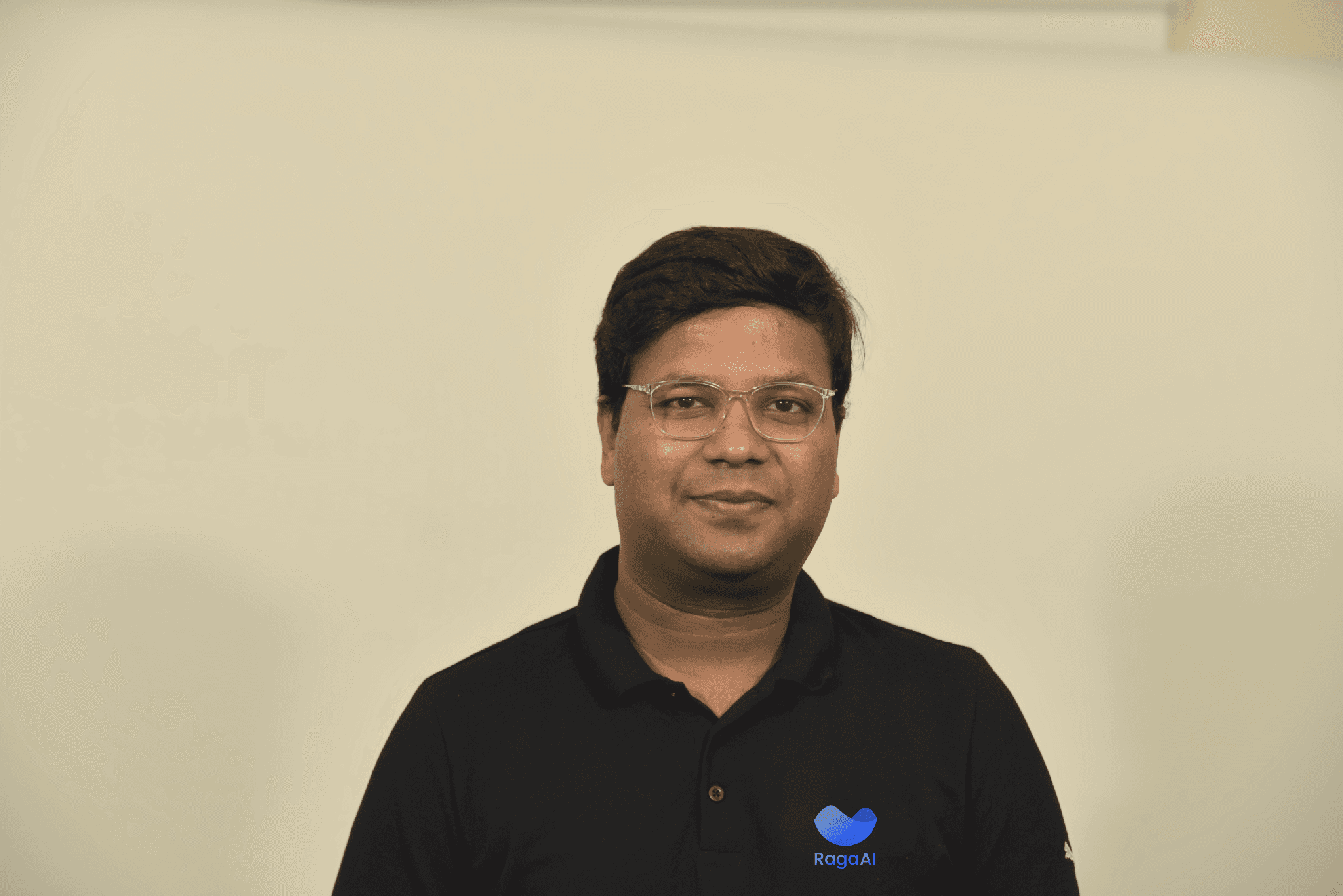
Jigar Gupta
Aug 14, 2024
Read the article




Introducing RagaAI Catalyst: Best in class automated LLM evaluation with 93% Human Alignment
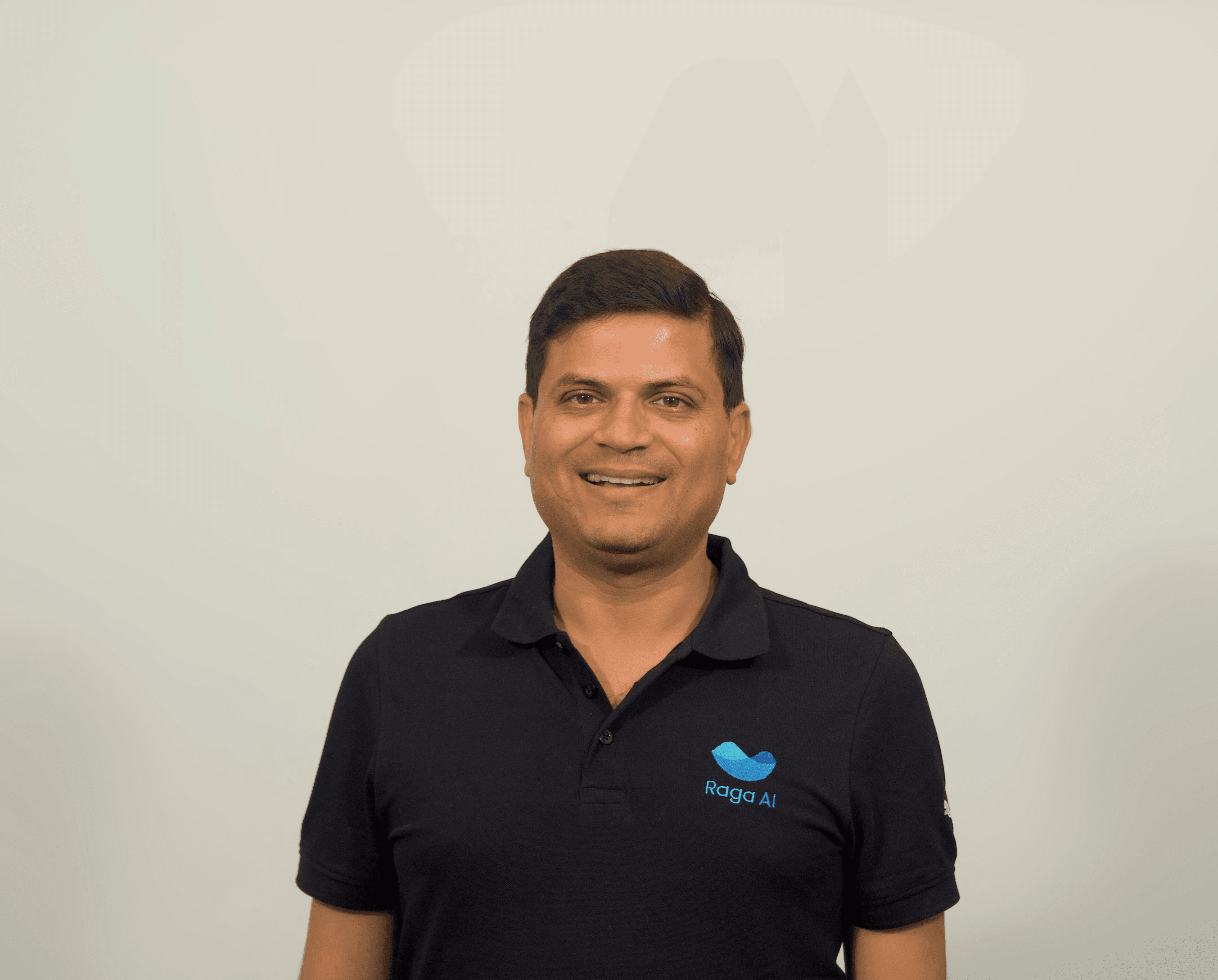
Gaurav Agarwal
Jul 15, 2024
Read the article




Key Pillars and Techniques for LLM Observability and Monitoring

Rehan Asif
Jul 24, 2024
Read the article
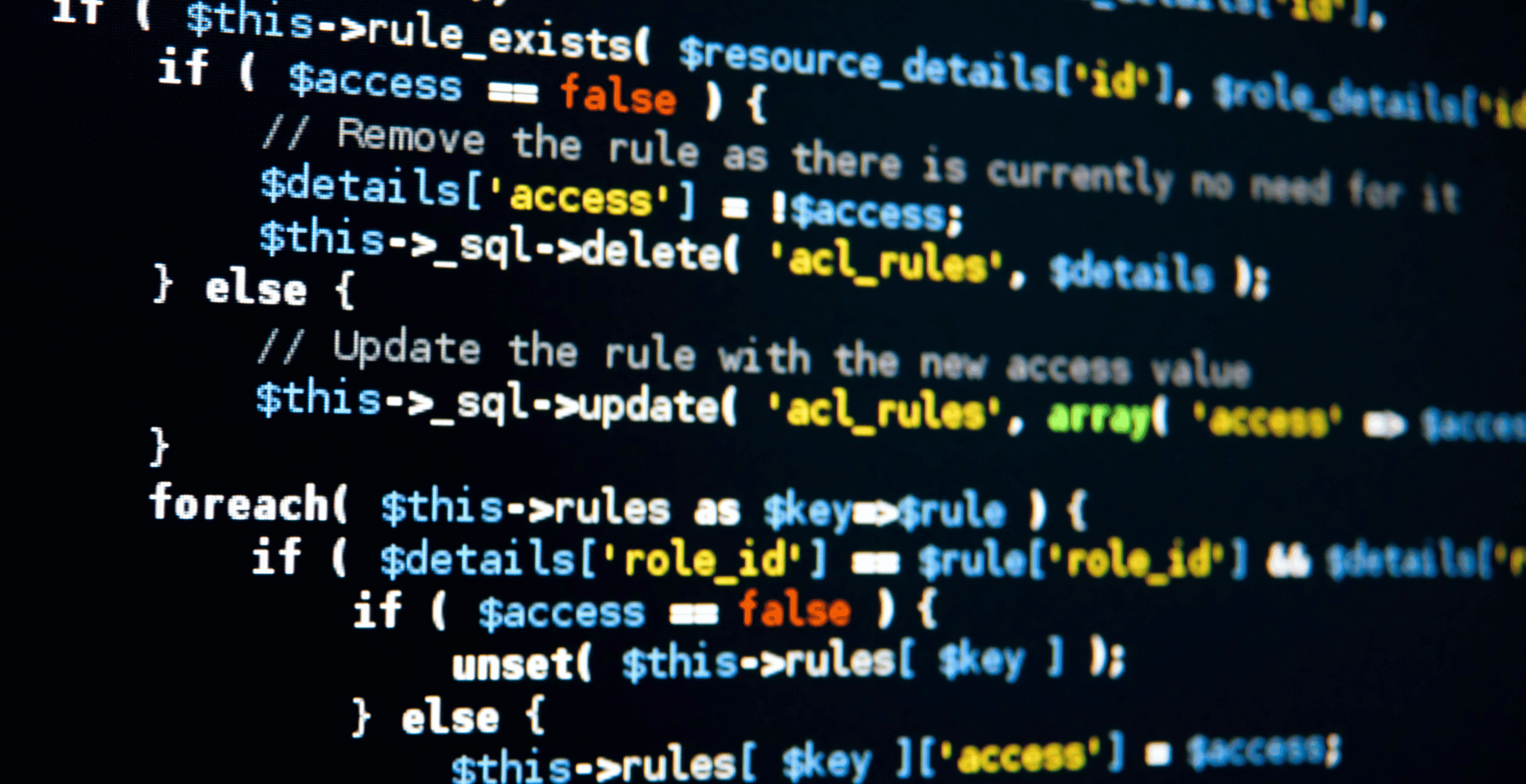
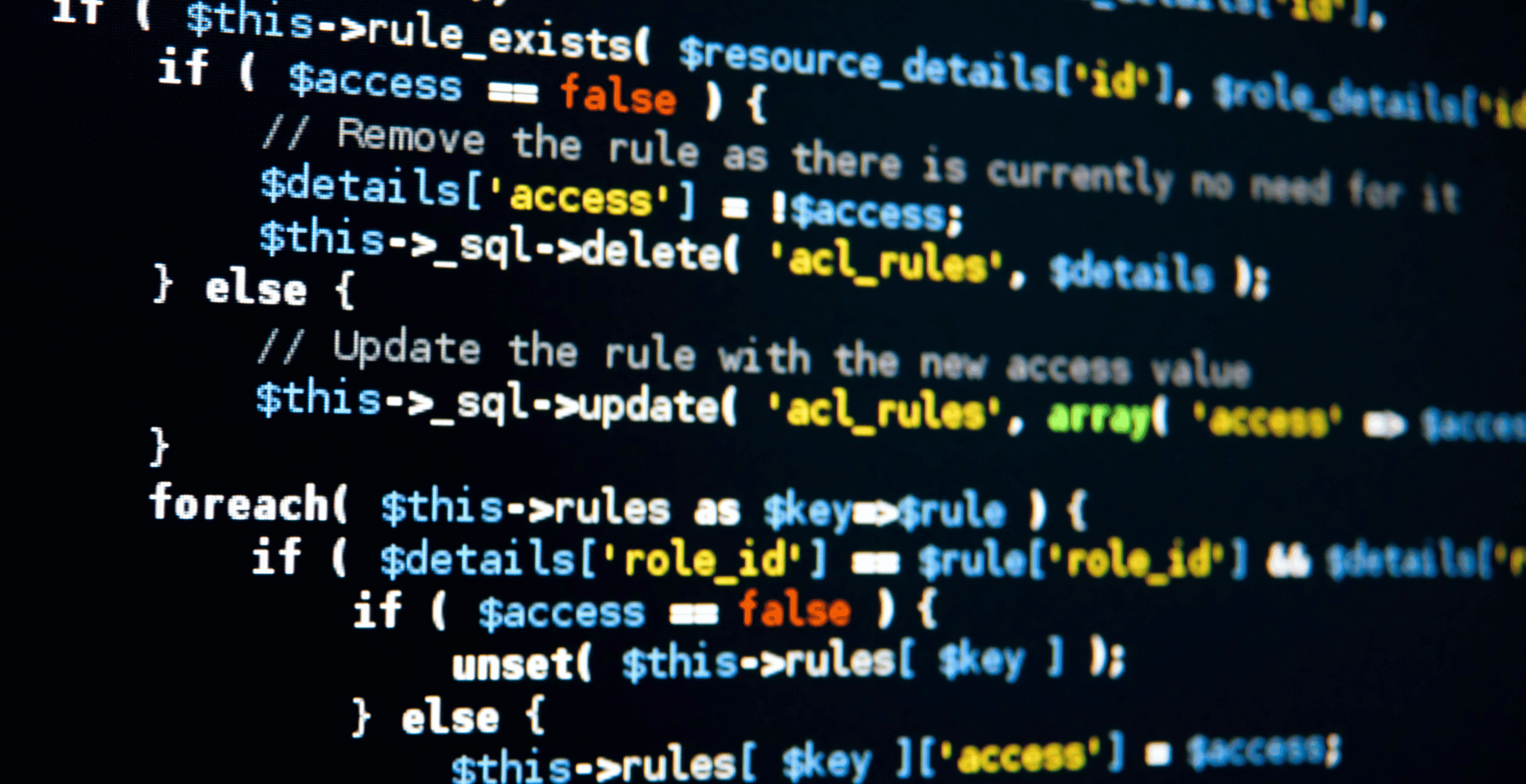
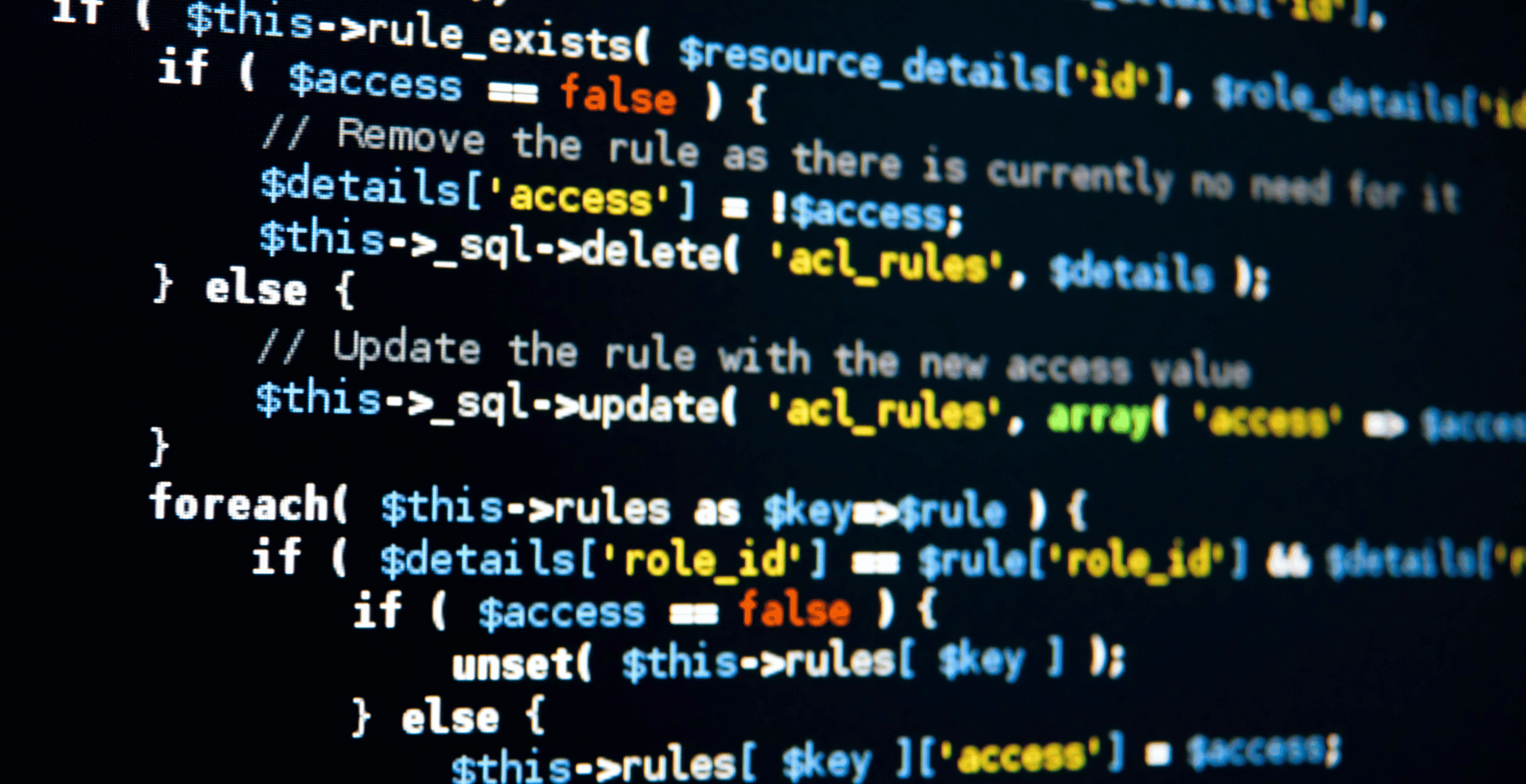
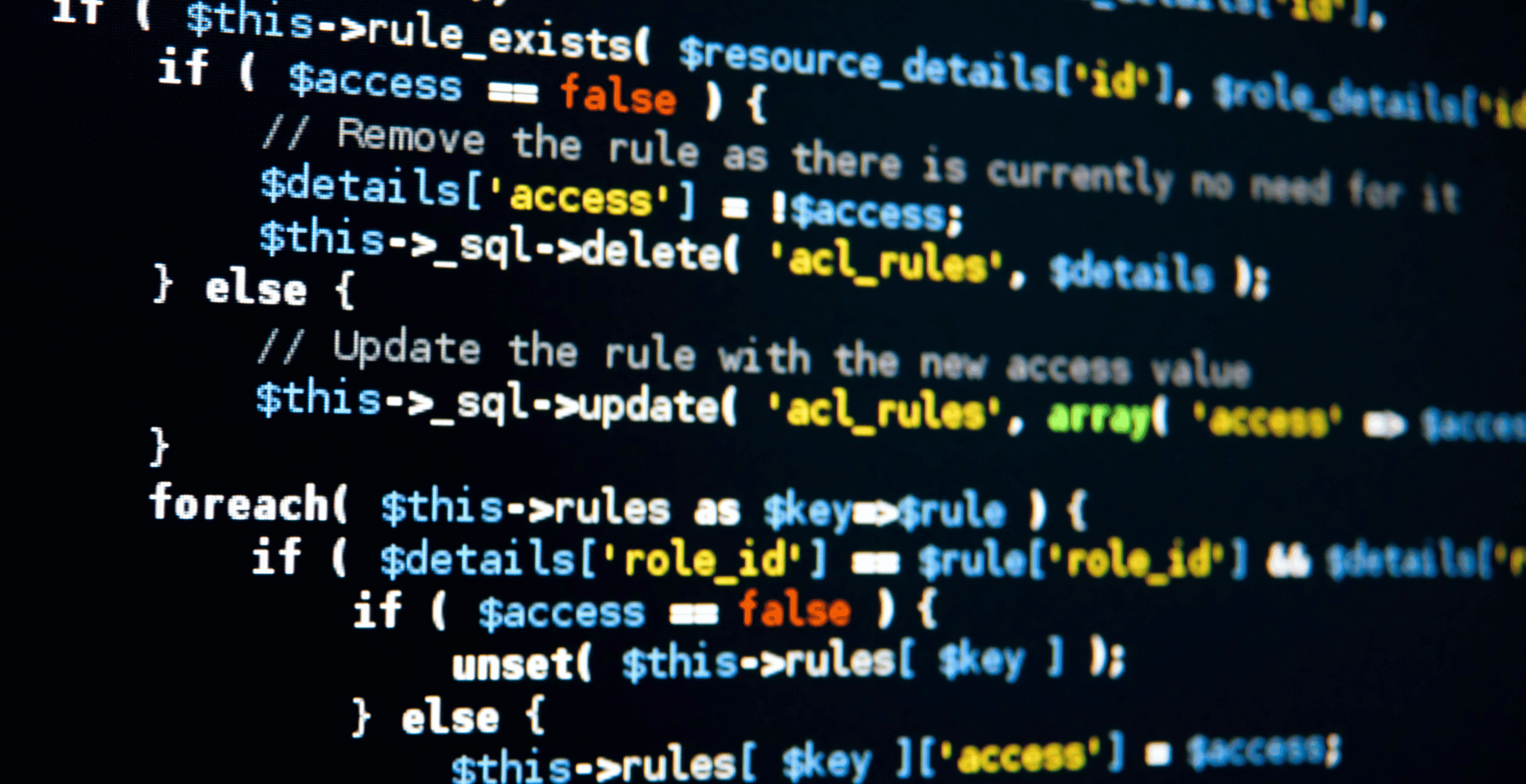
Introduction to What is LLM Agents and How They Work?

Rehan Asif
Jul 24, 2024
Read the article




Analysis of the Large Language Model Landscape Evolution

Rehan Asif
Jul 24, 2024
Read the article




Marketing Success With Retrieval Augmented Generation (RAG) Platforms
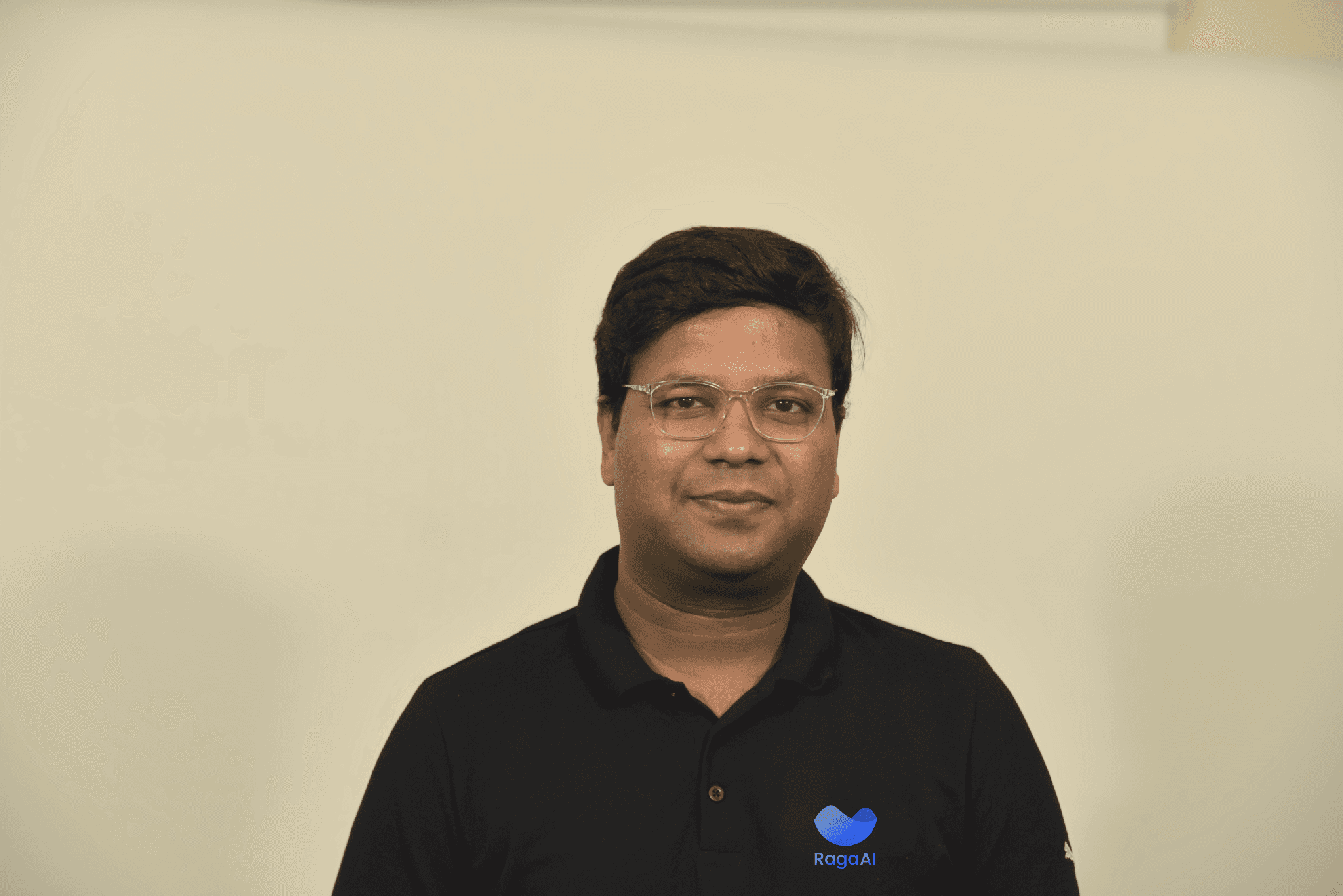
Jigar Gupta
Jul 24, 2024
Read the article




Developing AI Agent Strategies Using GPT
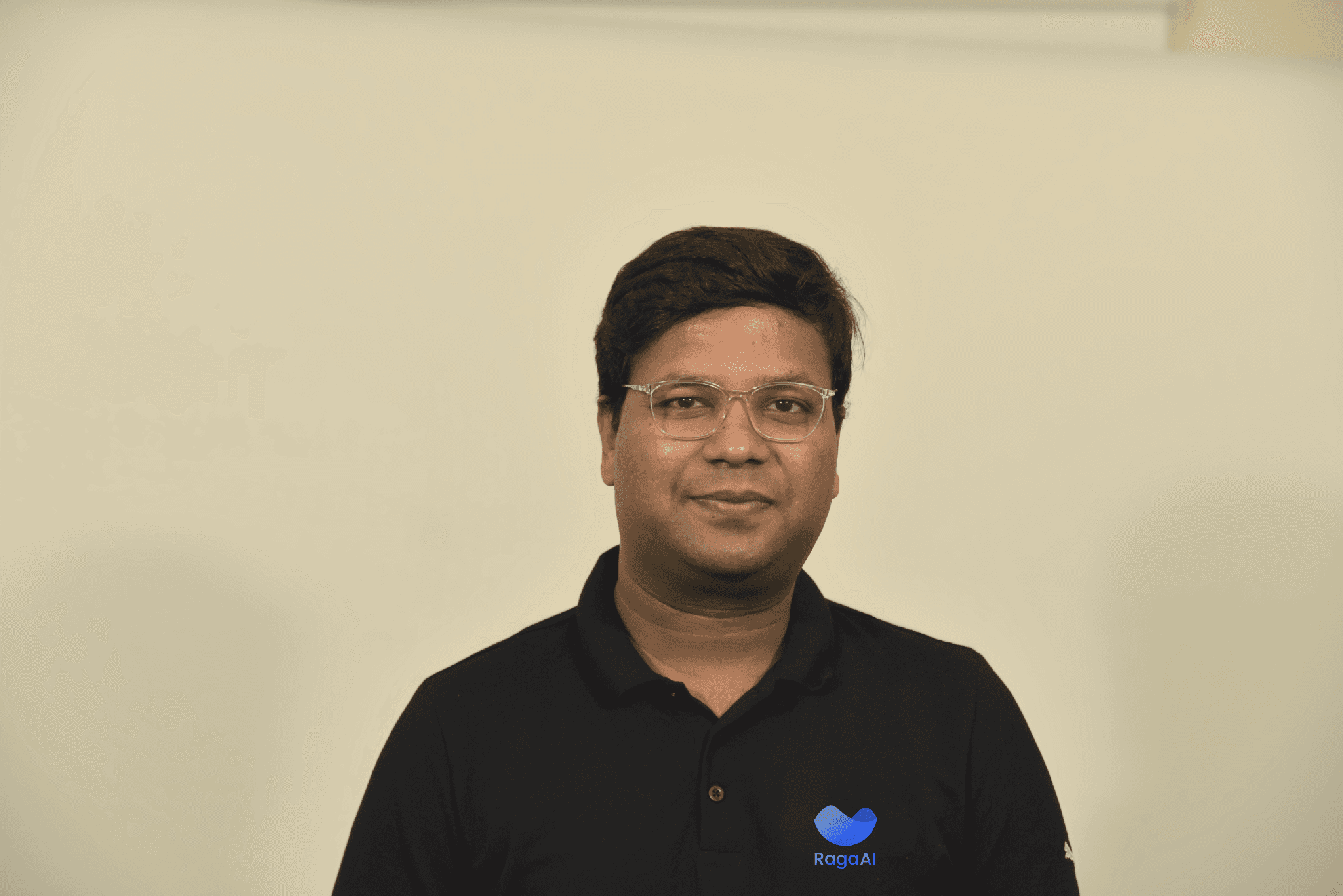
Jigar Gupta
Jul 24, 2024
Read the article




Identifying Triggers for Retraining AI Models to Maintain Performance
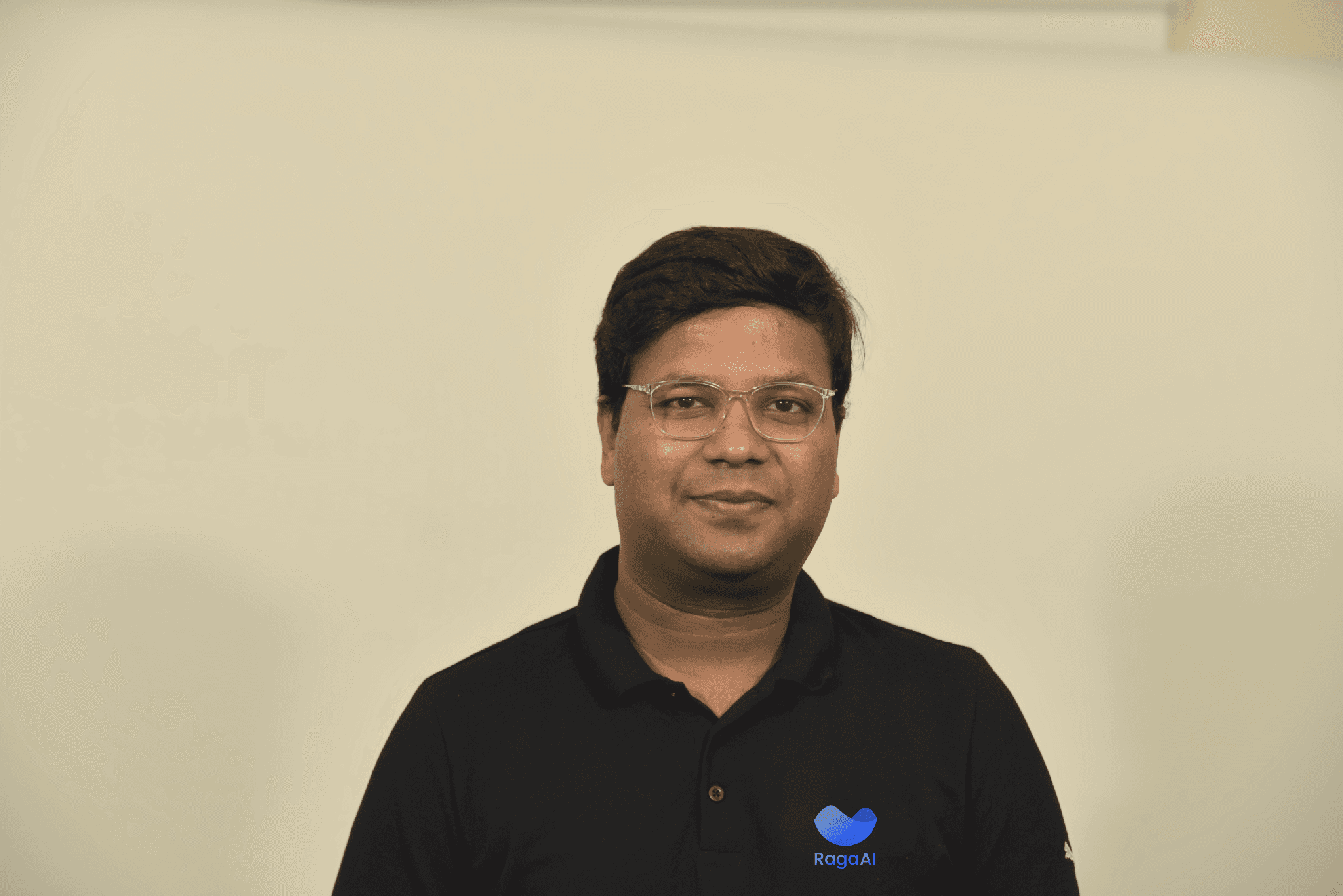
Jigar Gupta
Jul 16, 2024
Read the article
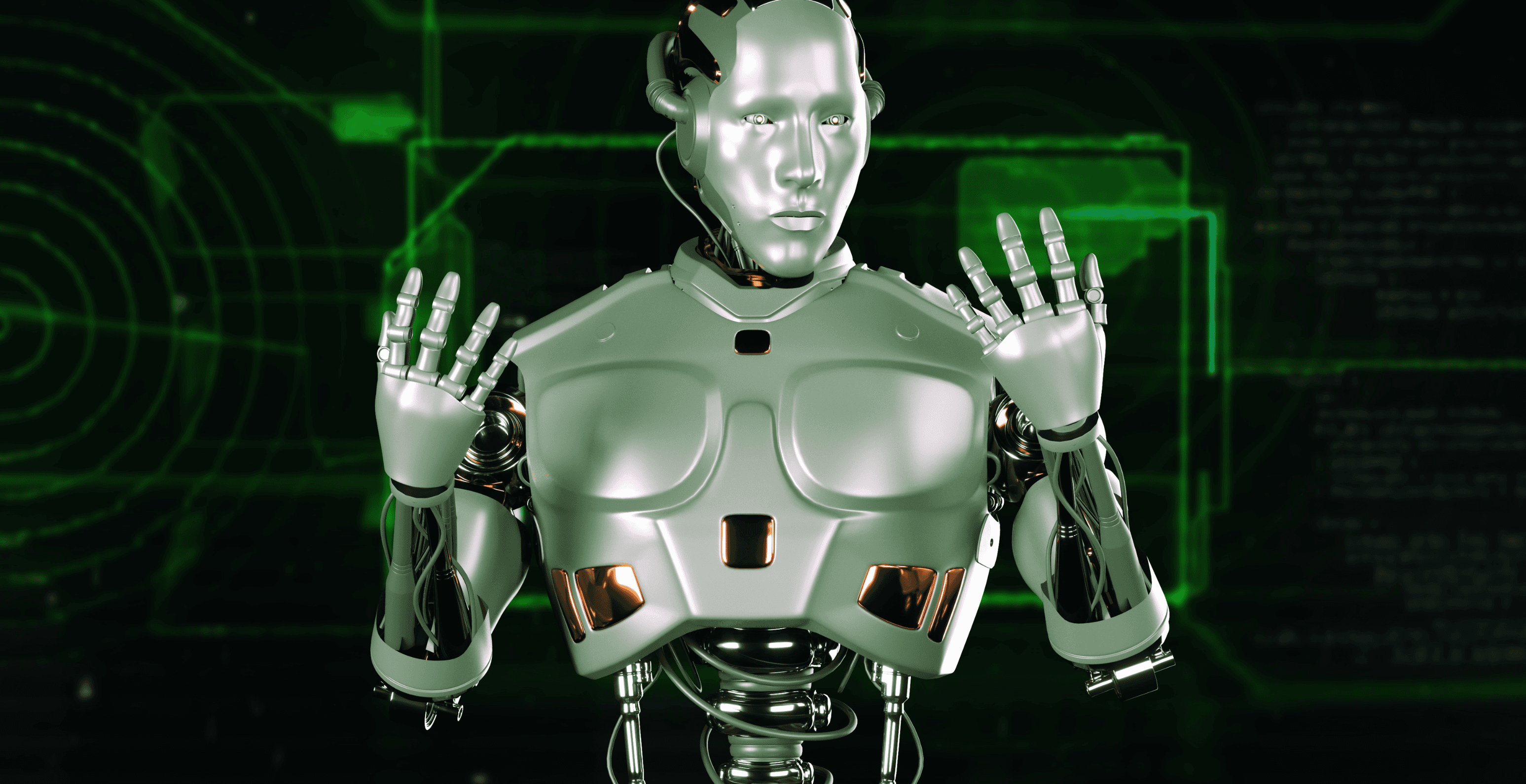
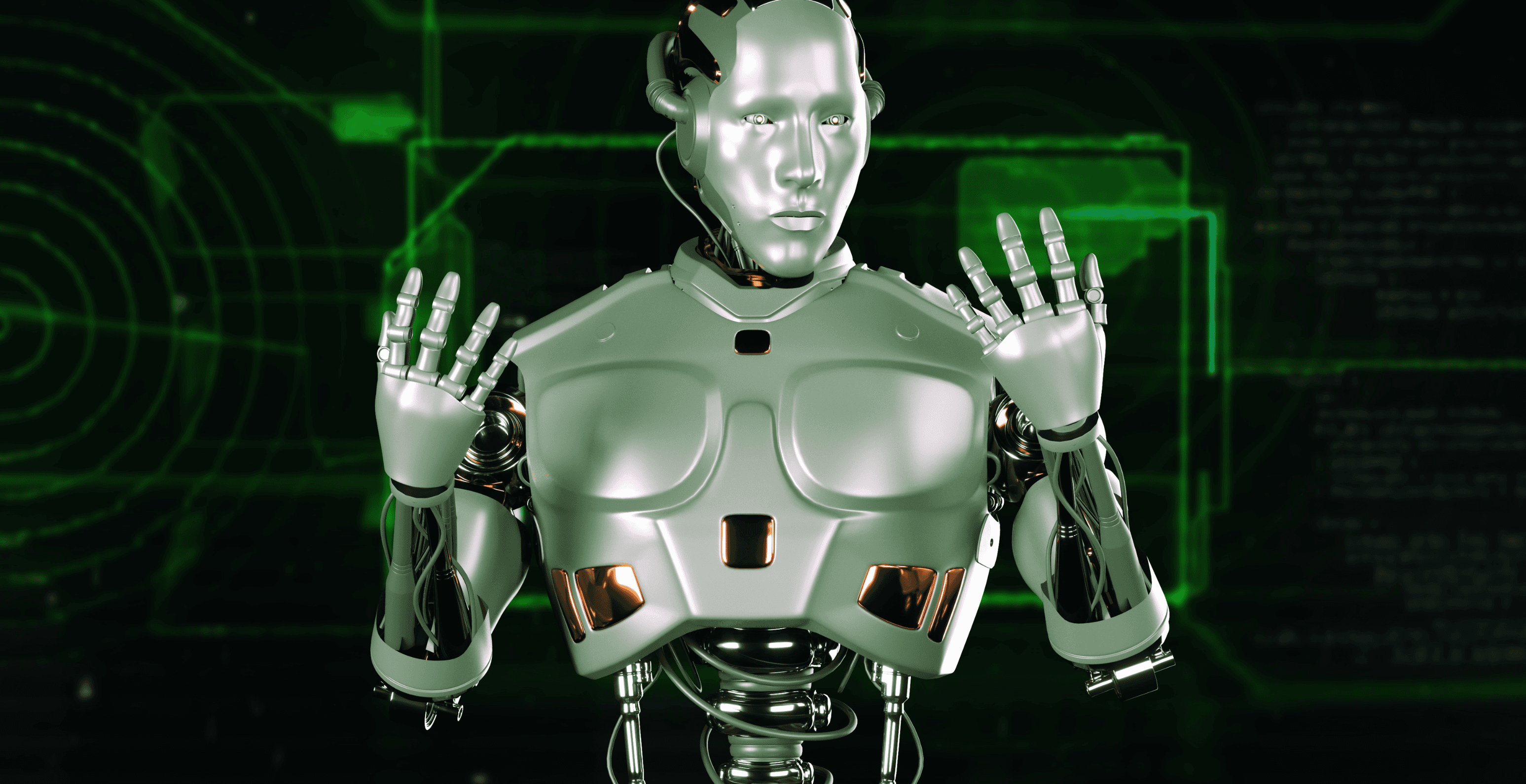
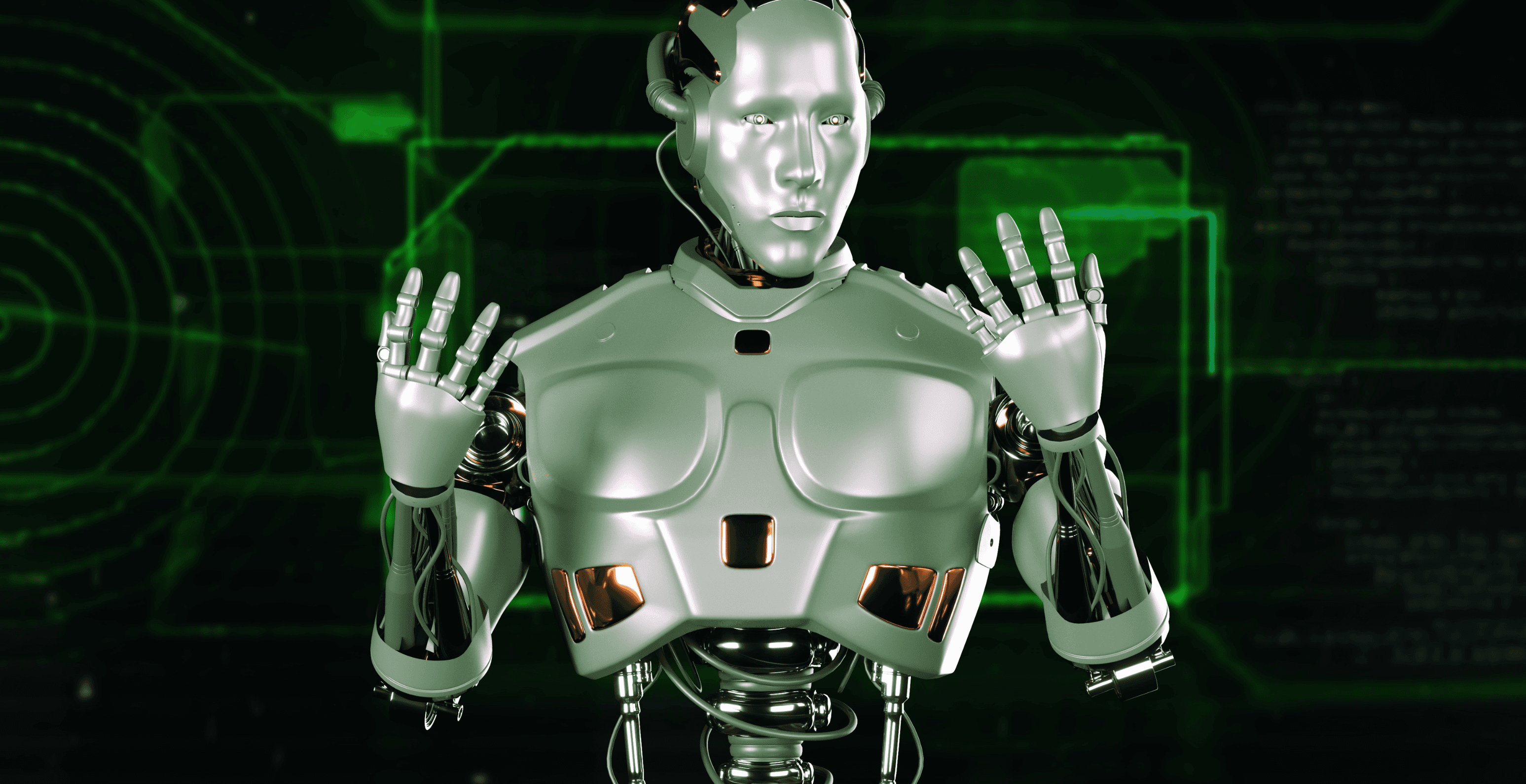
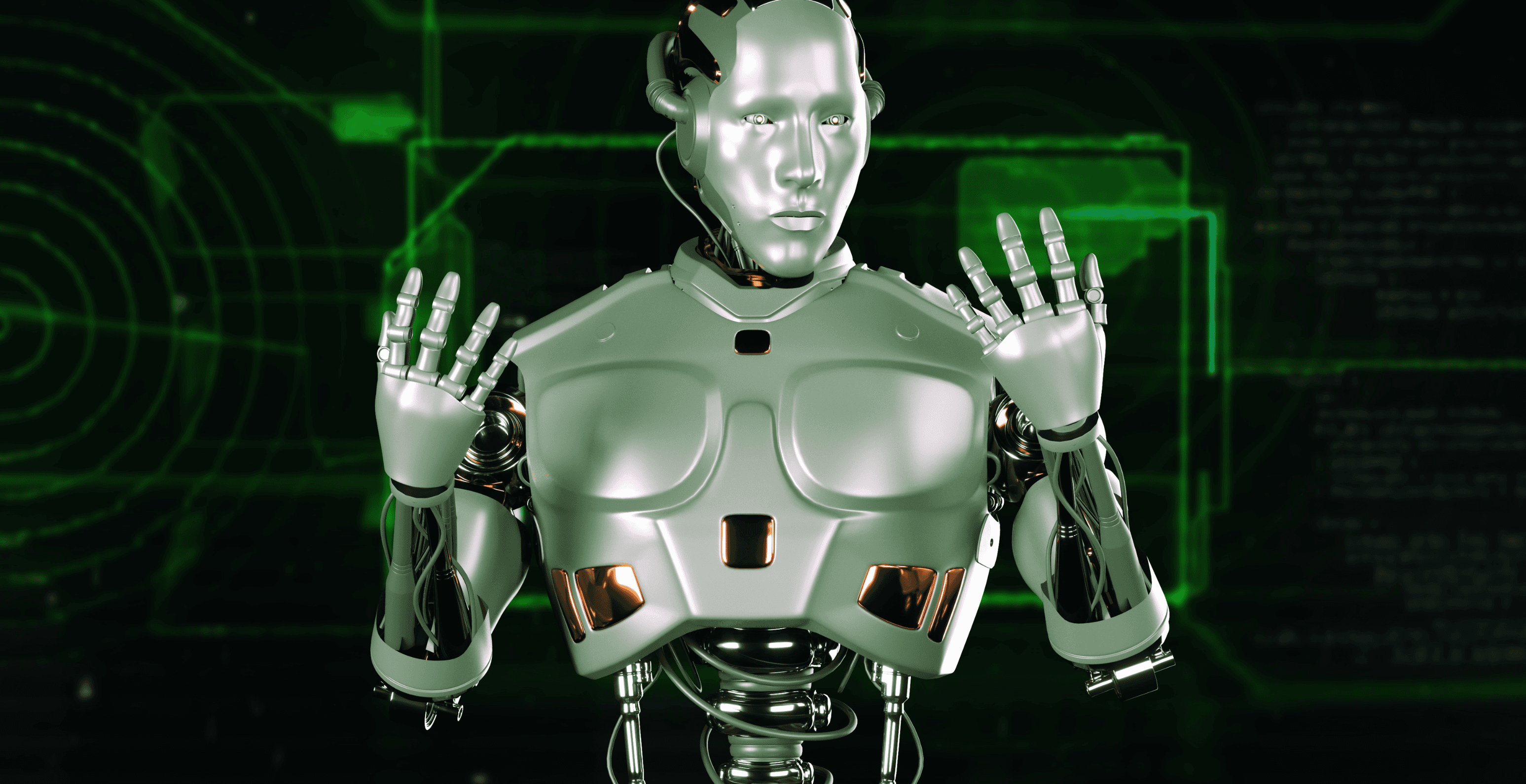
Agentic Design Patterns In LLM-Based Applications

Rehan Asif
Jul 16, 2024
Read the article
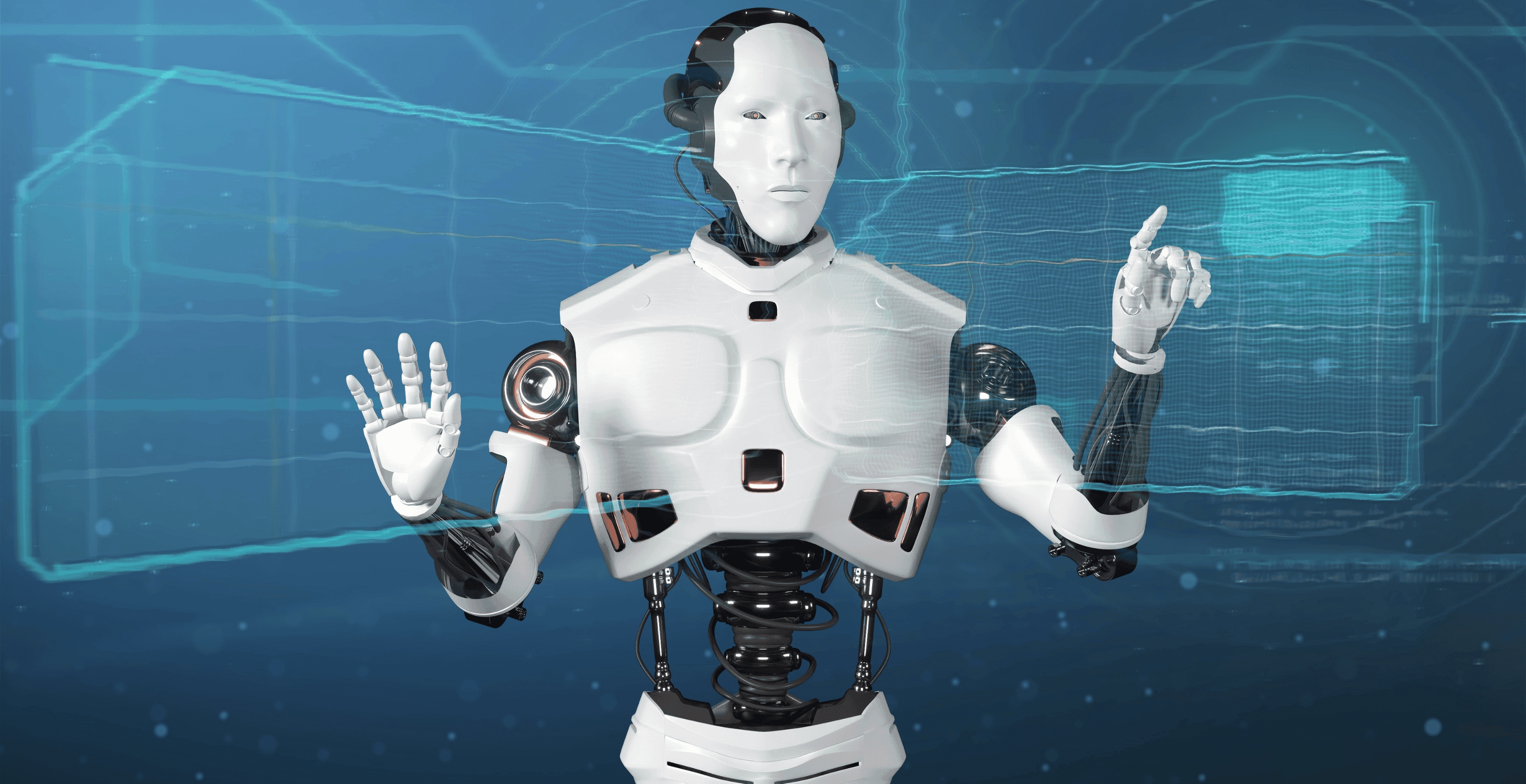
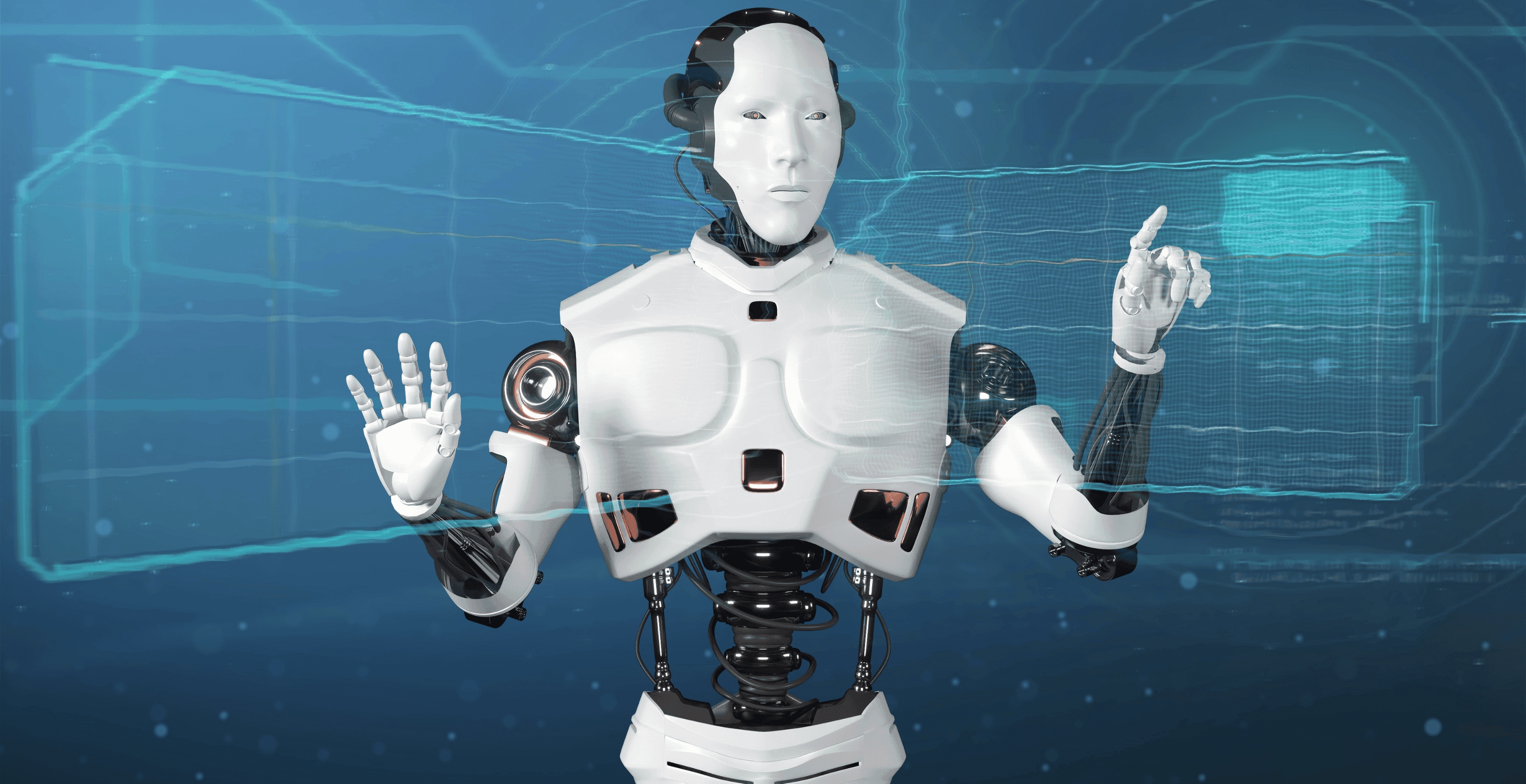
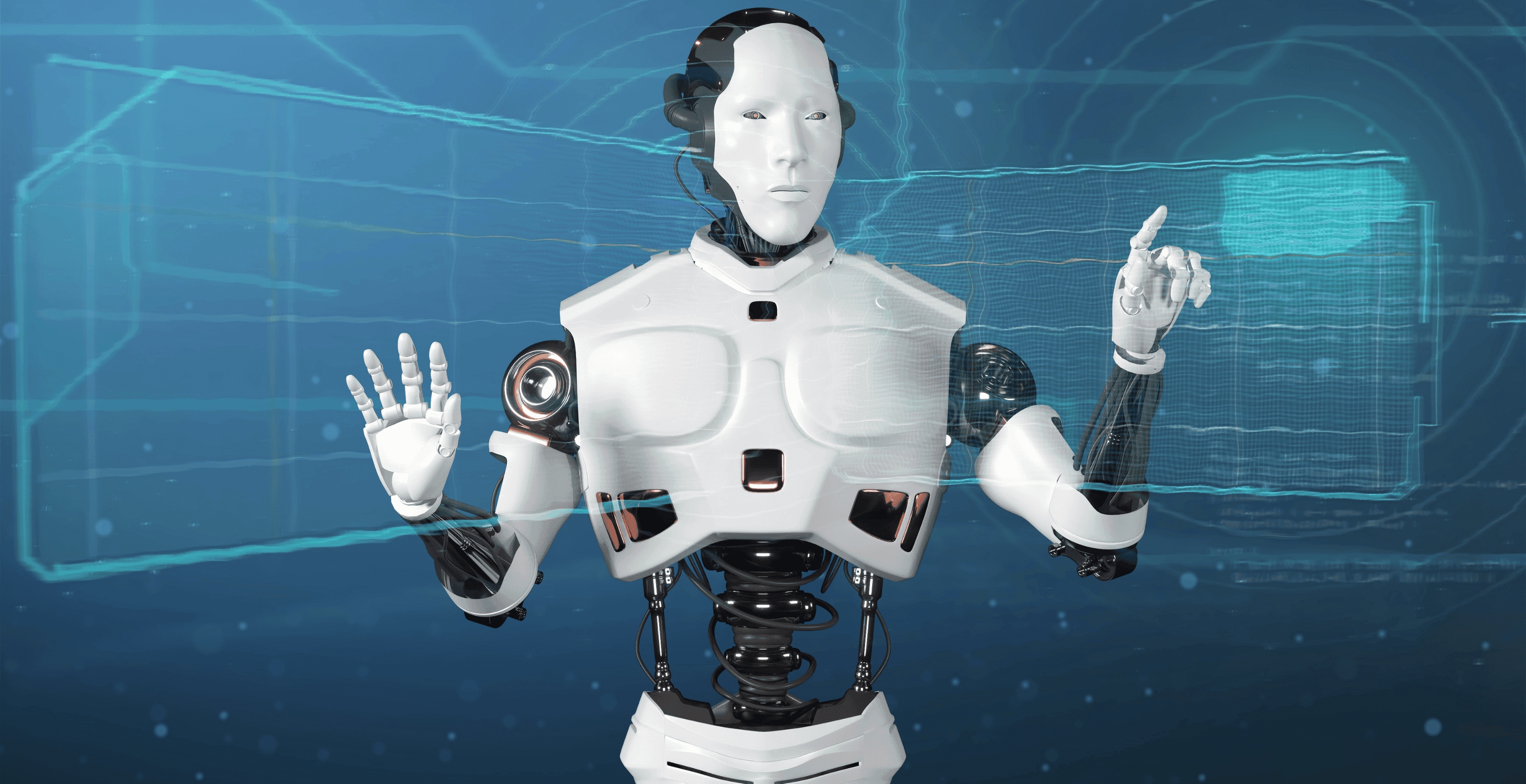
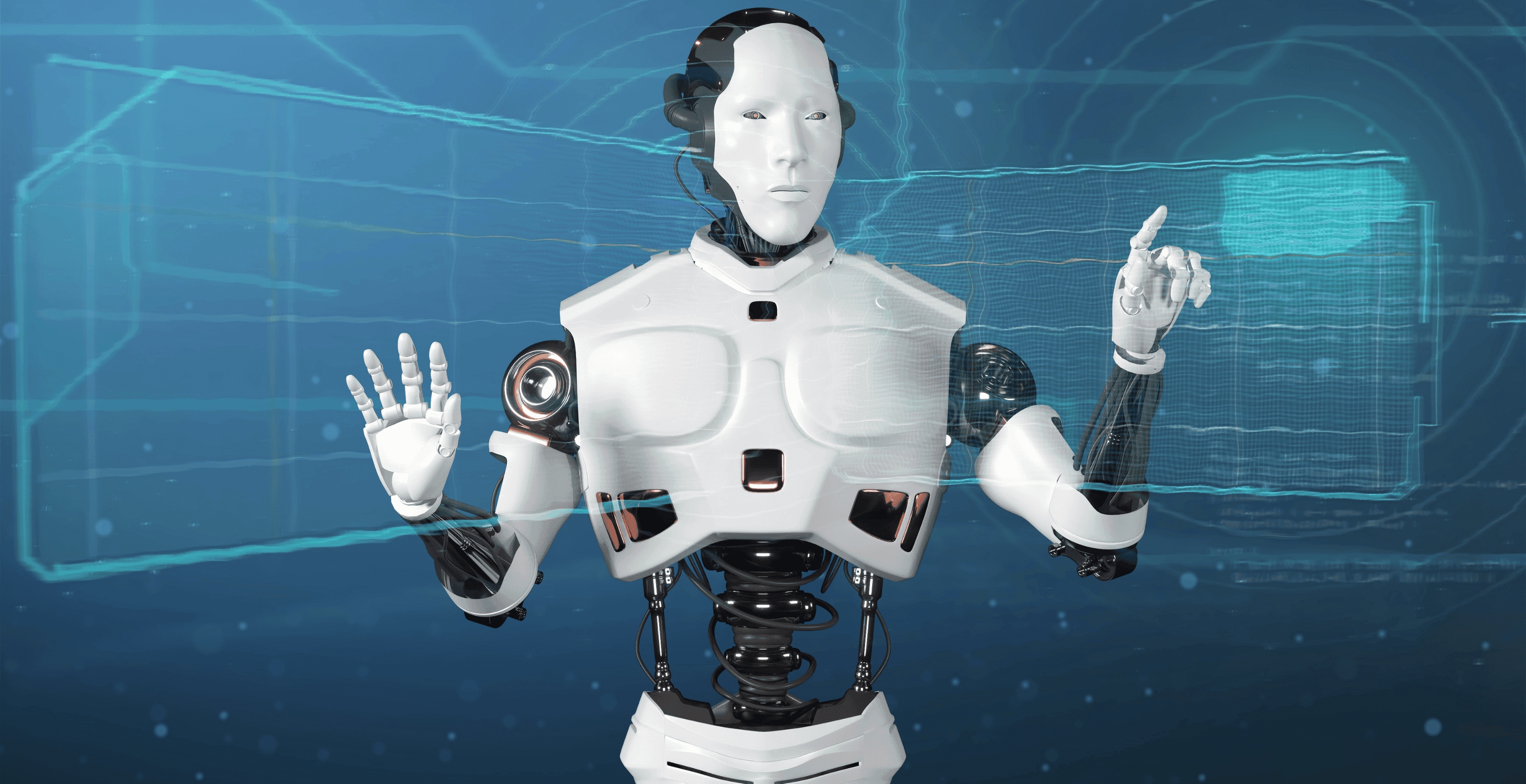
Generative AI And Document Question Answering With LLMs
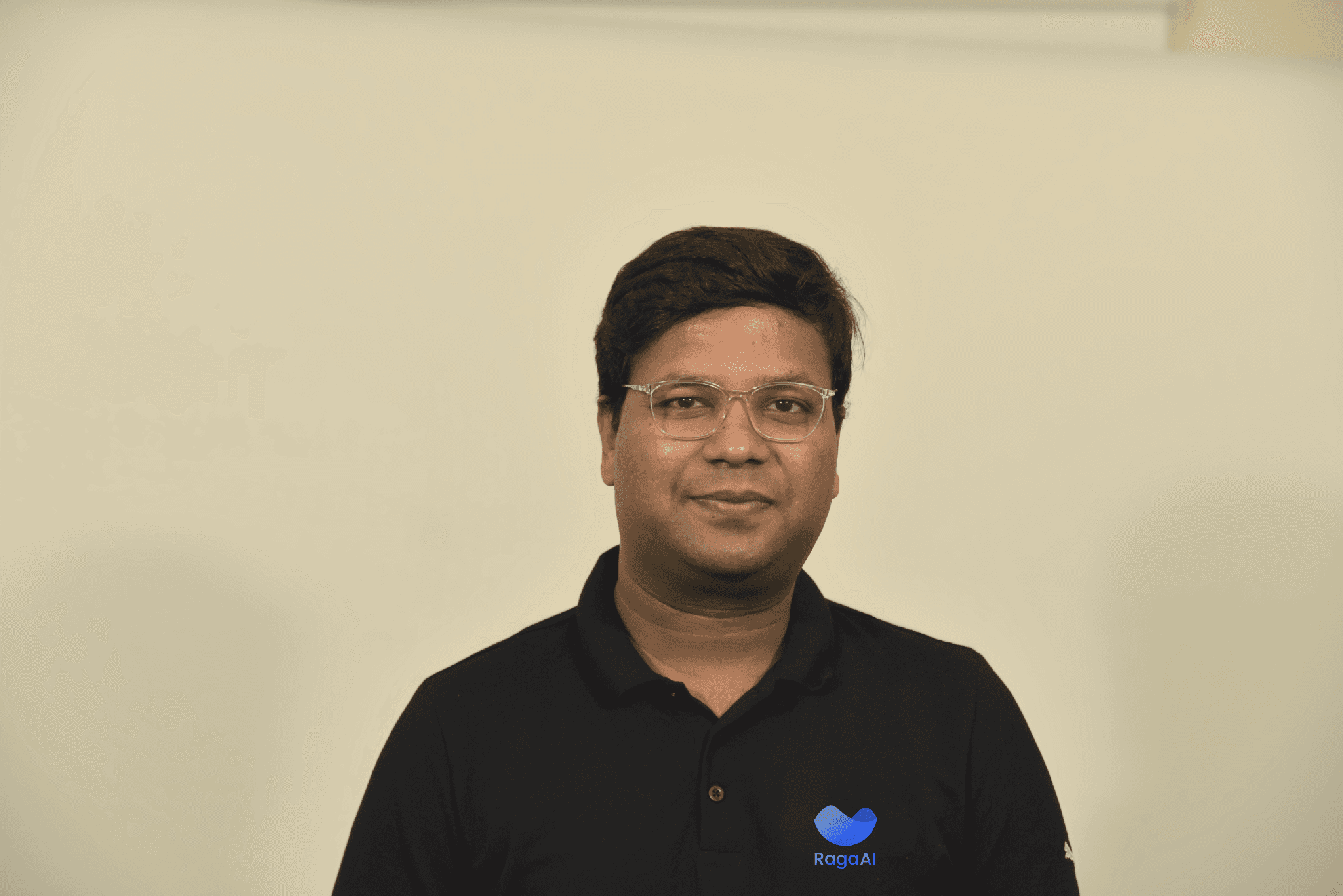
Jigar Gupta
Jul 15, 2024
Read the article




How to Fine-Tune ChatGPT for Your Use Case - Step by Step Guide
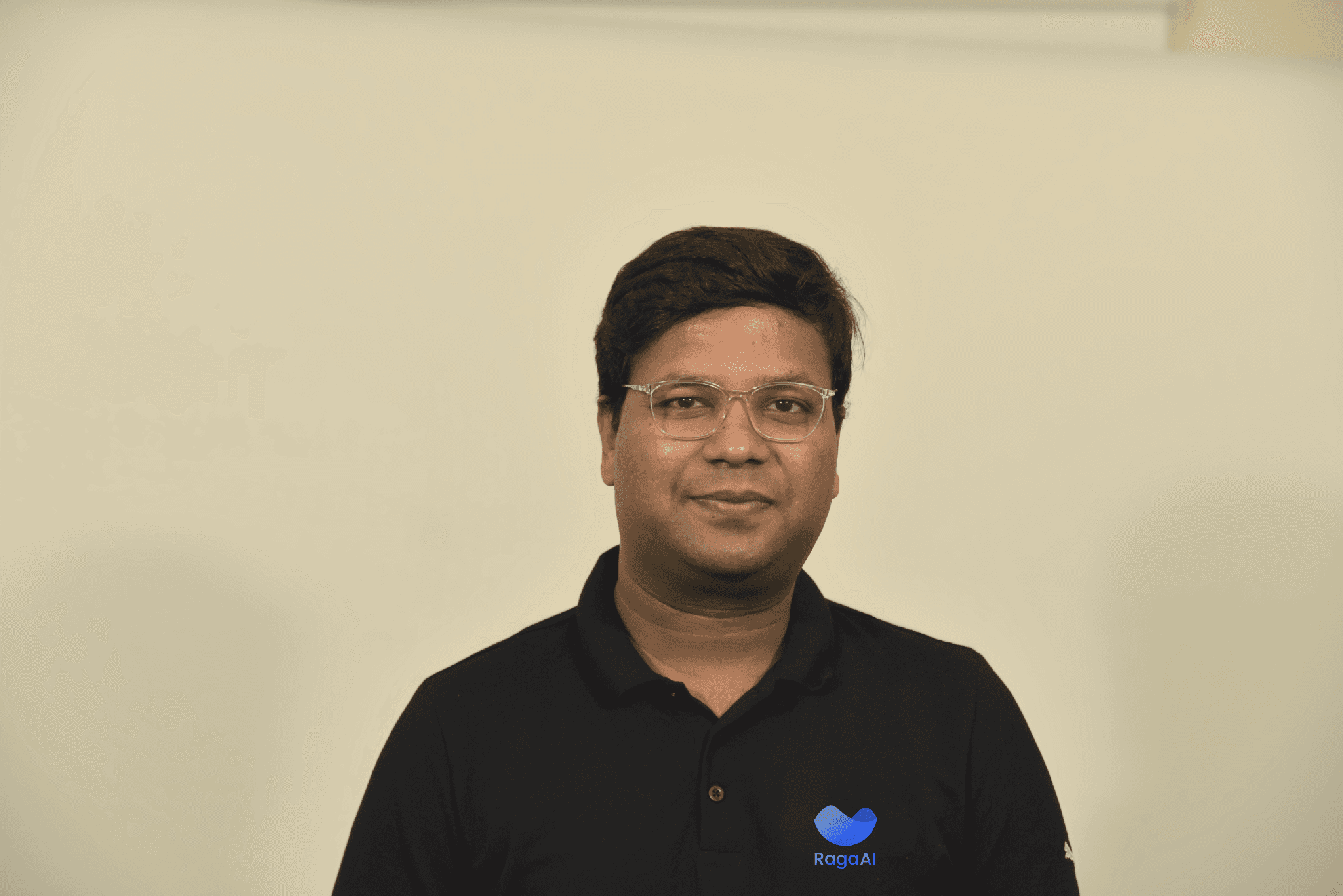
Jigar Gupta
Jul 15, 2024
Read the article




Security and LLM Firewall Controls

Rehan Asif
Jul 15, 2024
Read the article
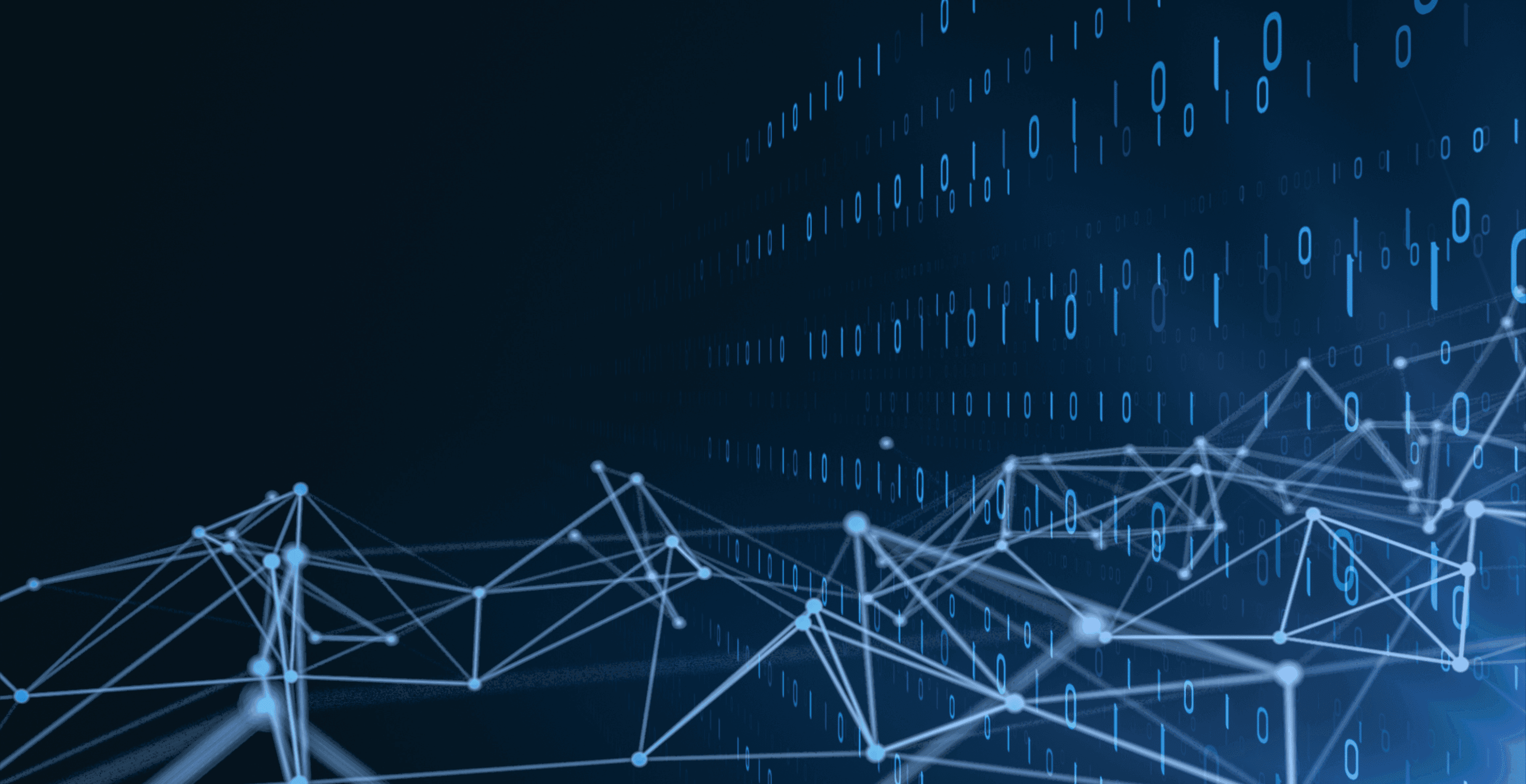
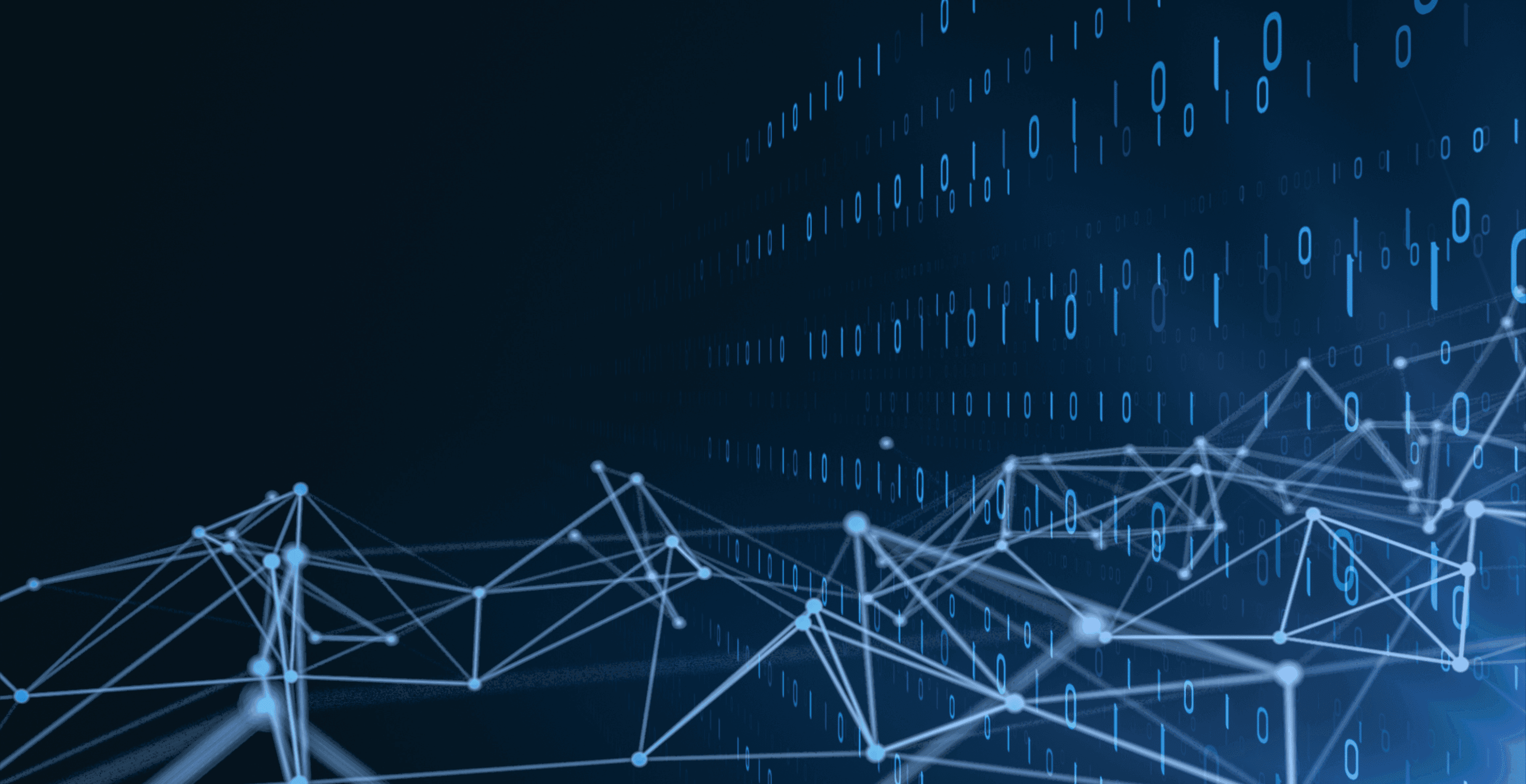
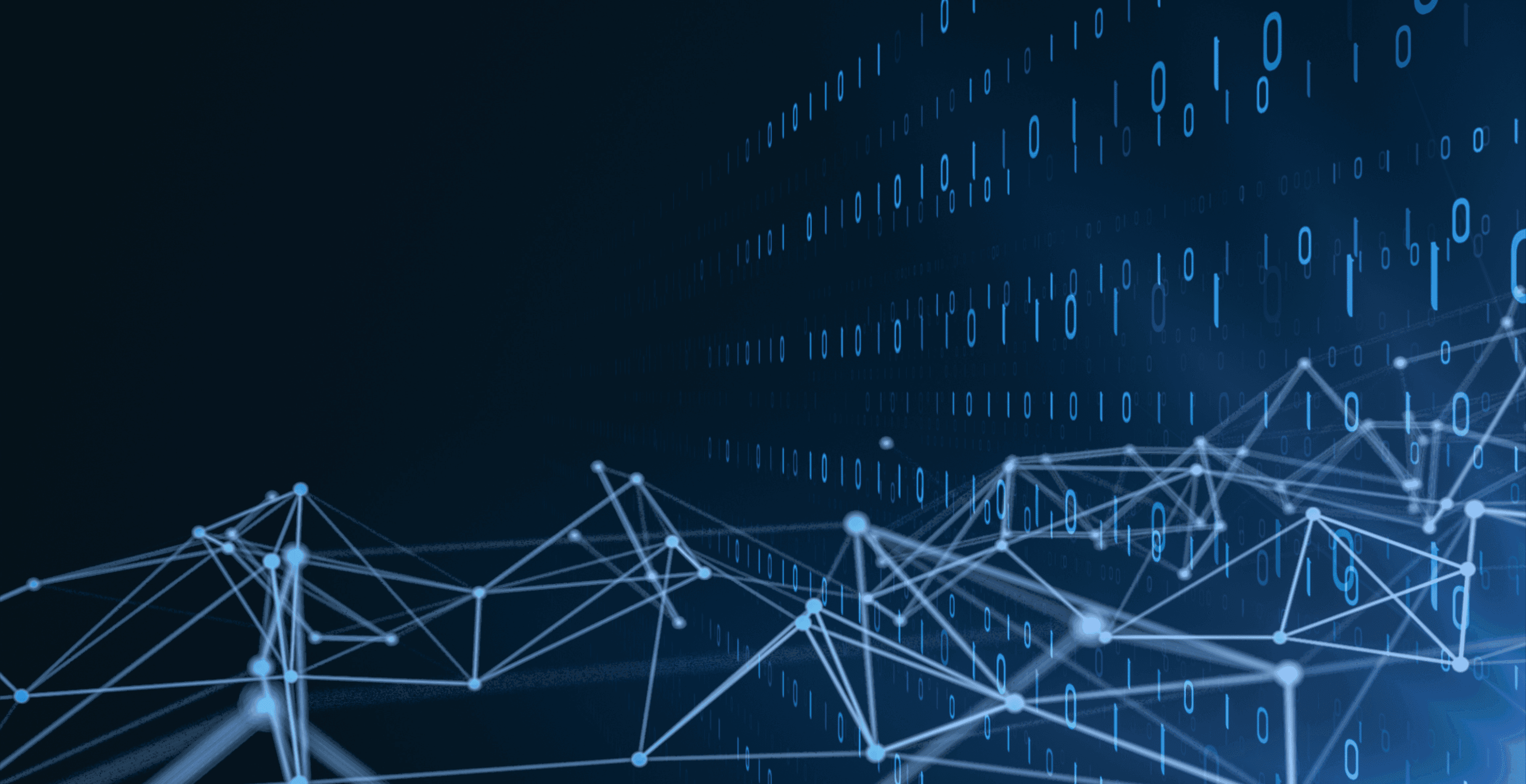
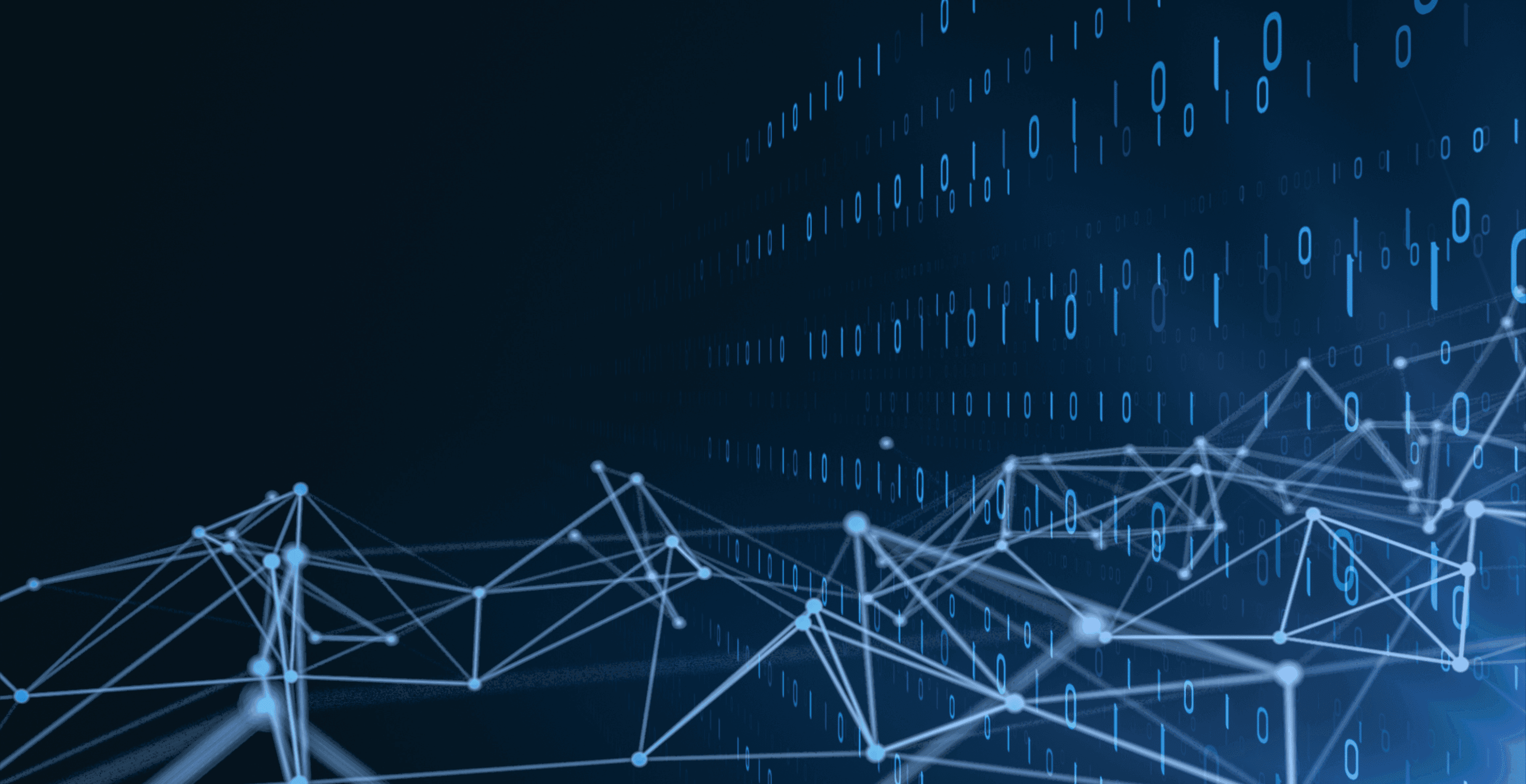
Understanding the Use of Guardrail Metrics in Ensuring LLM Safety

Rehan Asif
Jul 13, 2024
Read the article
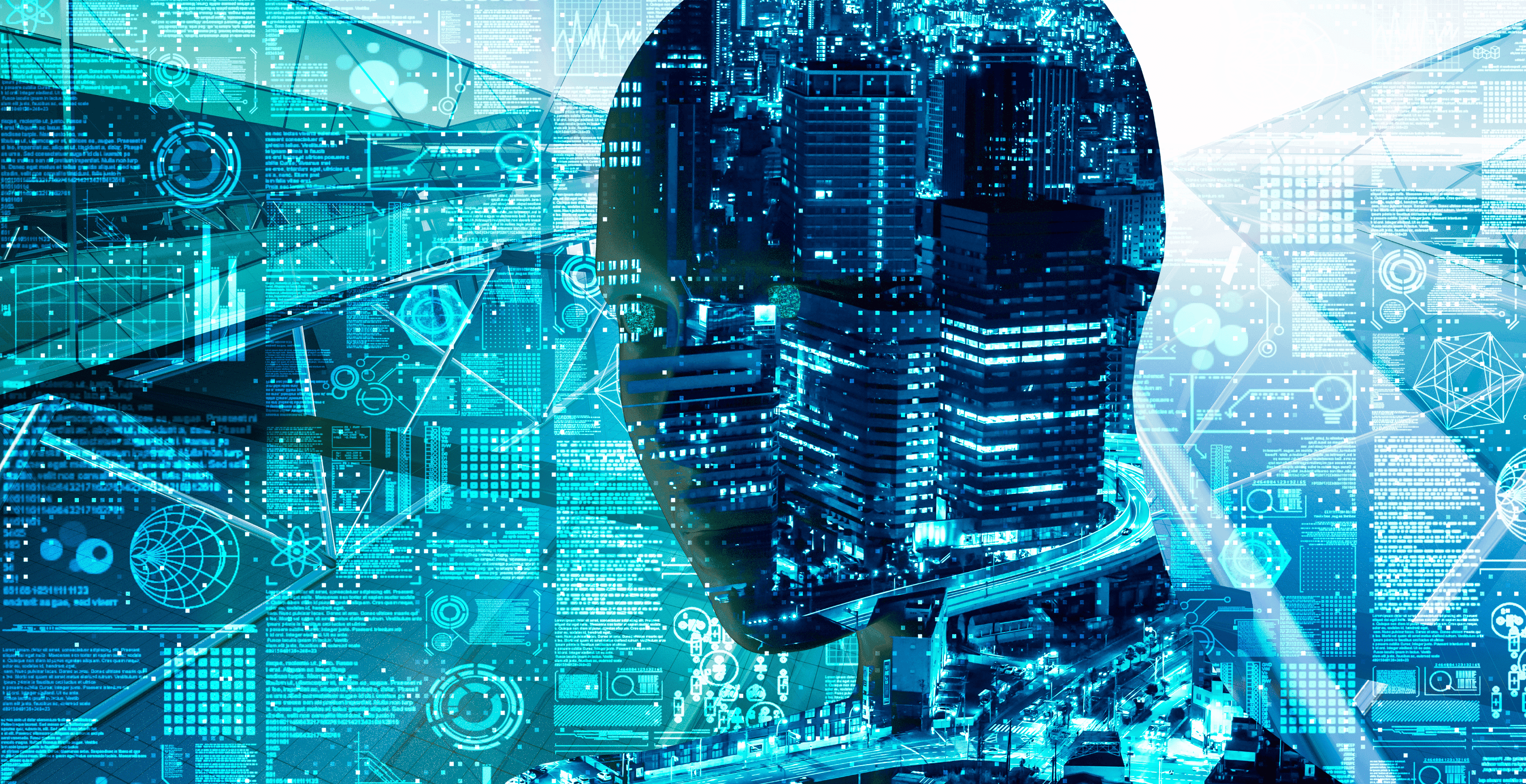
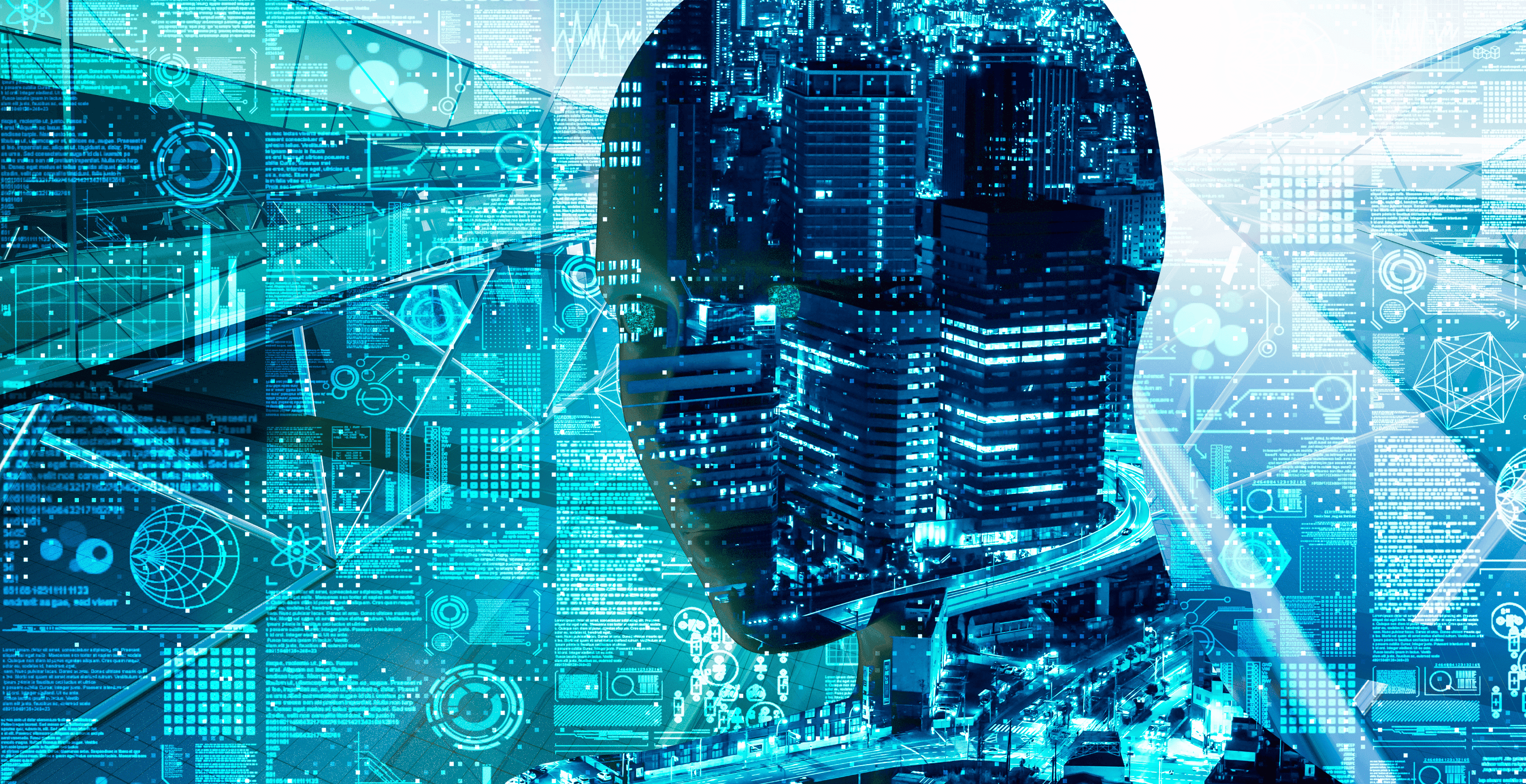
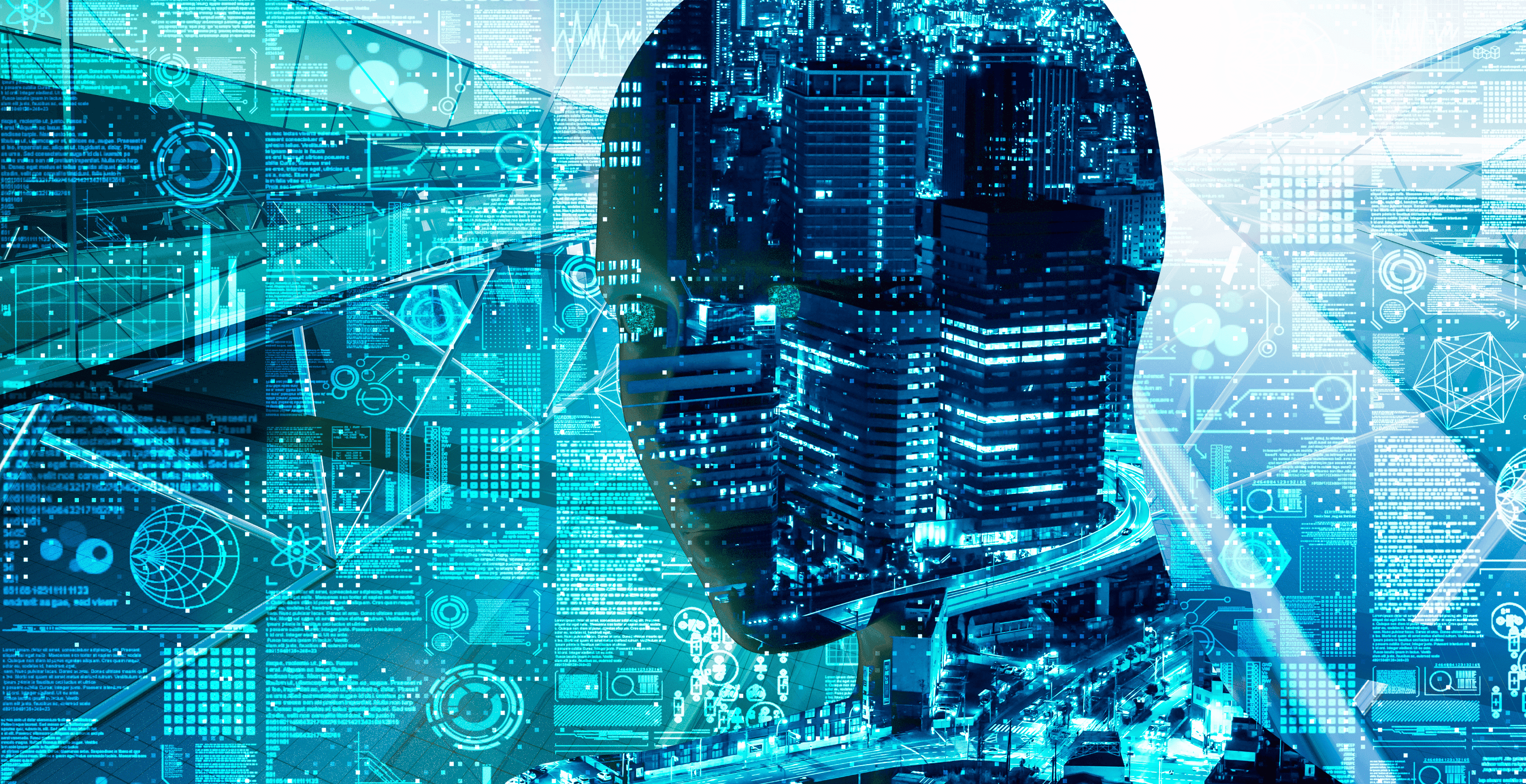
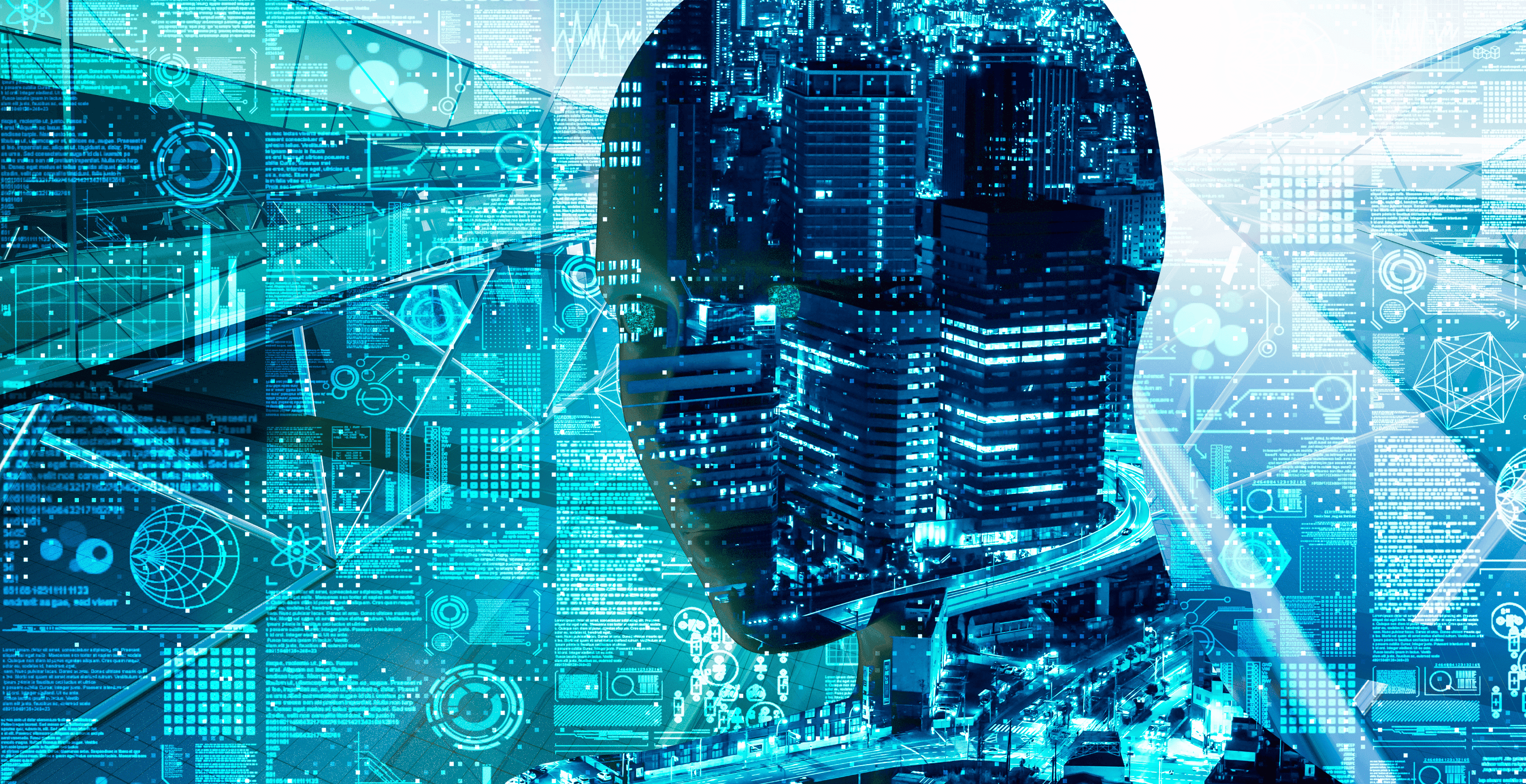
Exploring the Future of LLM and Generative AI Infrastructure

Rehan Asif
Jul 13, 2024
Read the article




Comprehensive Guide to RLHF and Fine Tuning LLMs from Scratch

Rehan Asif
Jul 13, 2024
Read the article
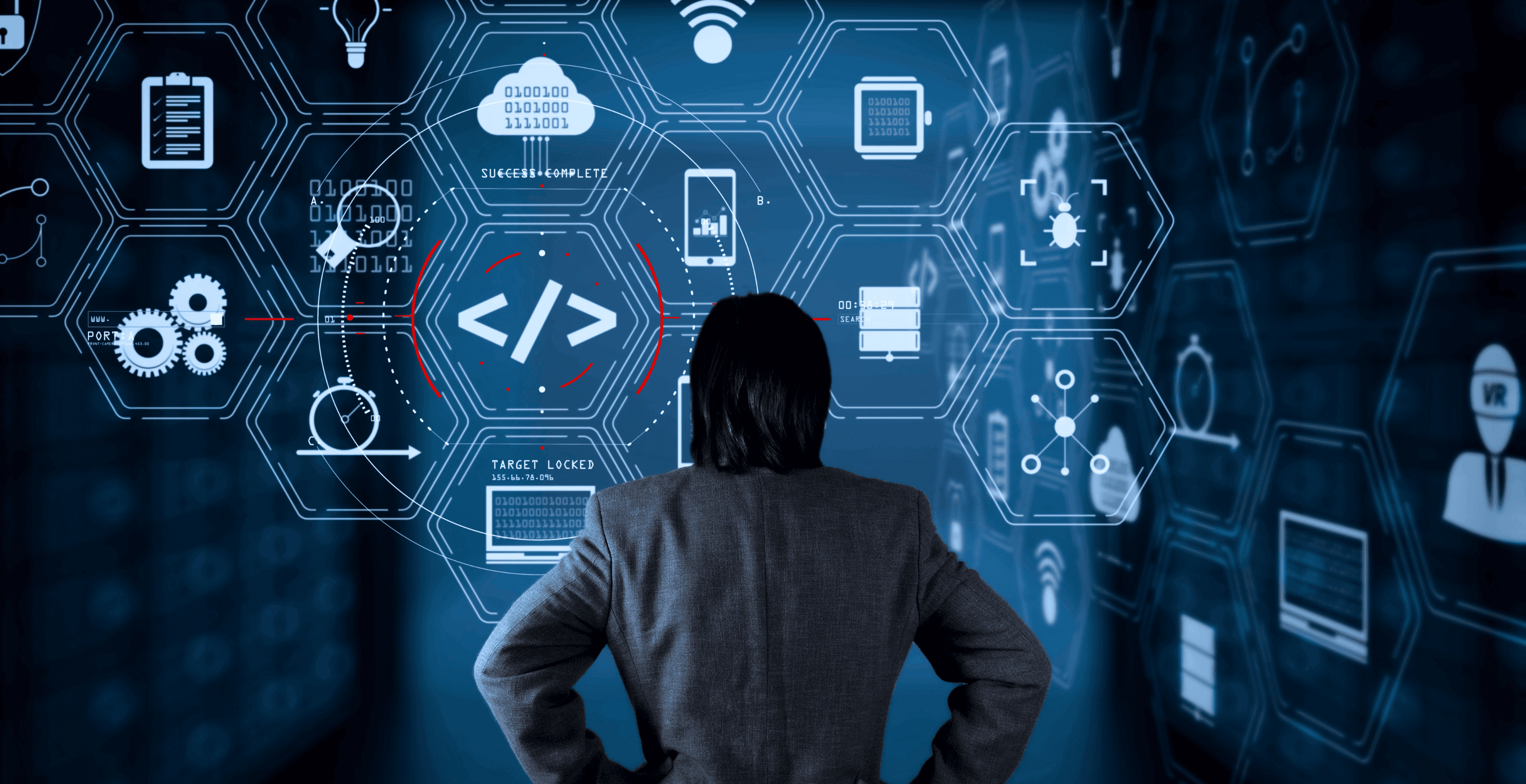
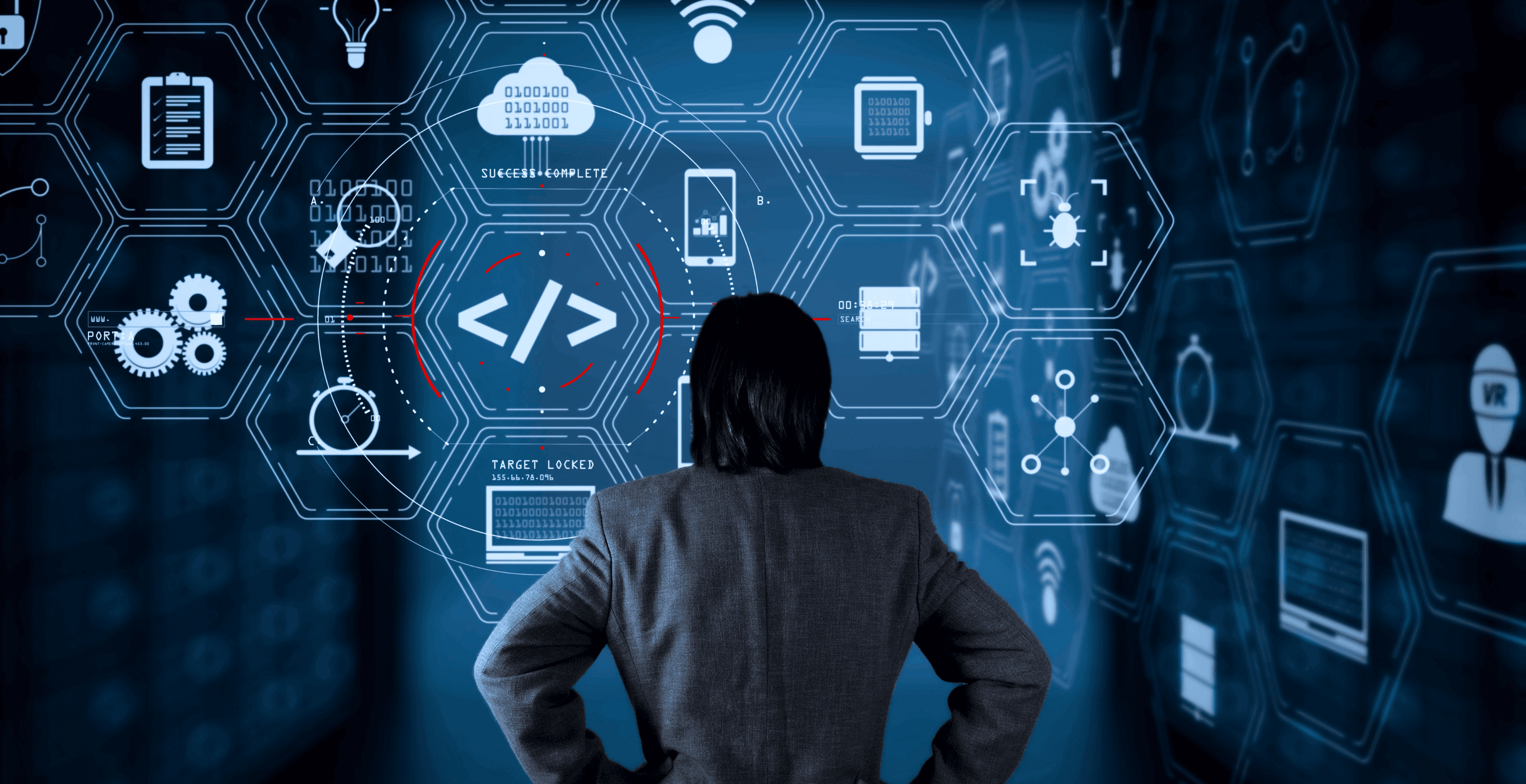
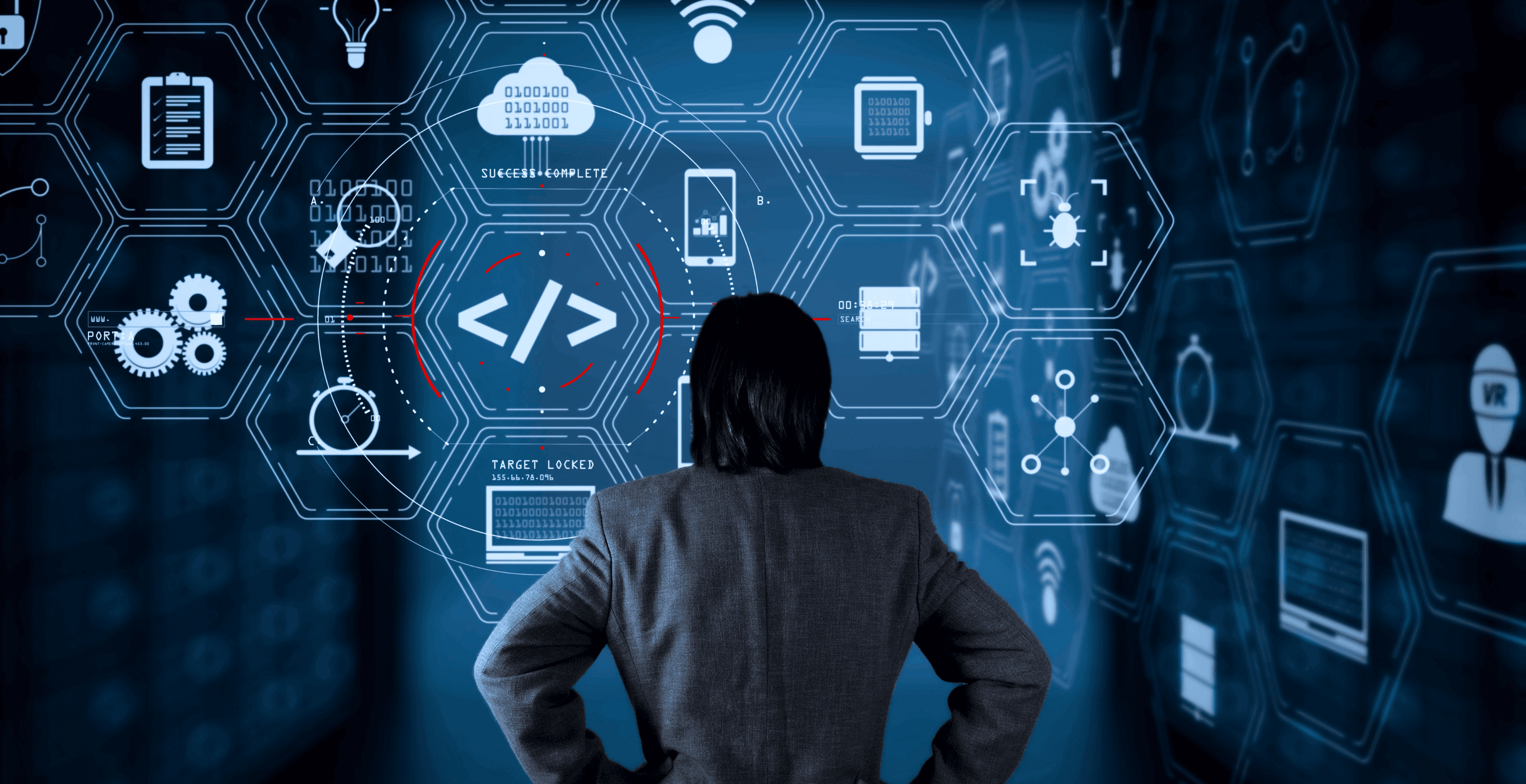
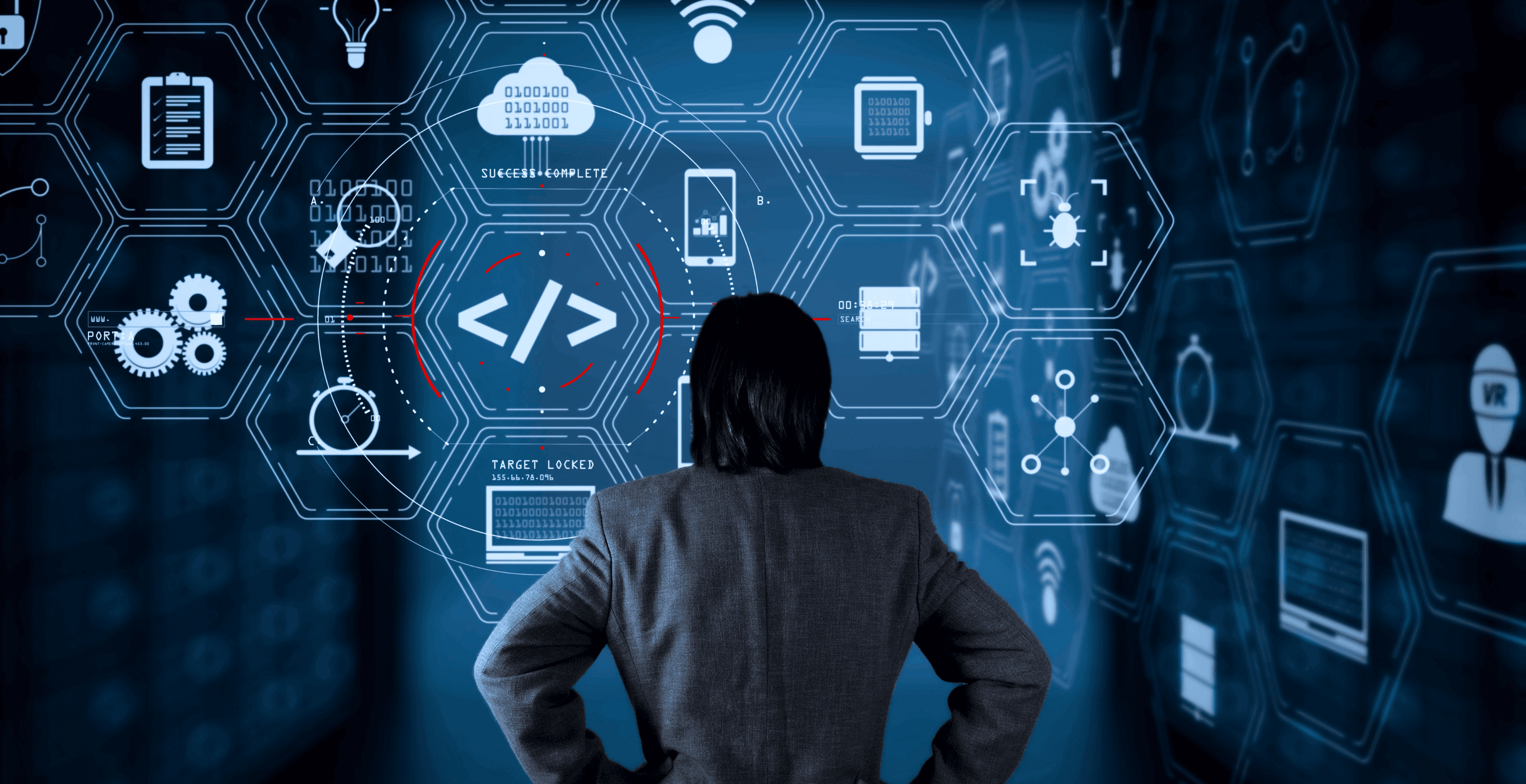
Using Synthetic Data To Enrich RAG Applications
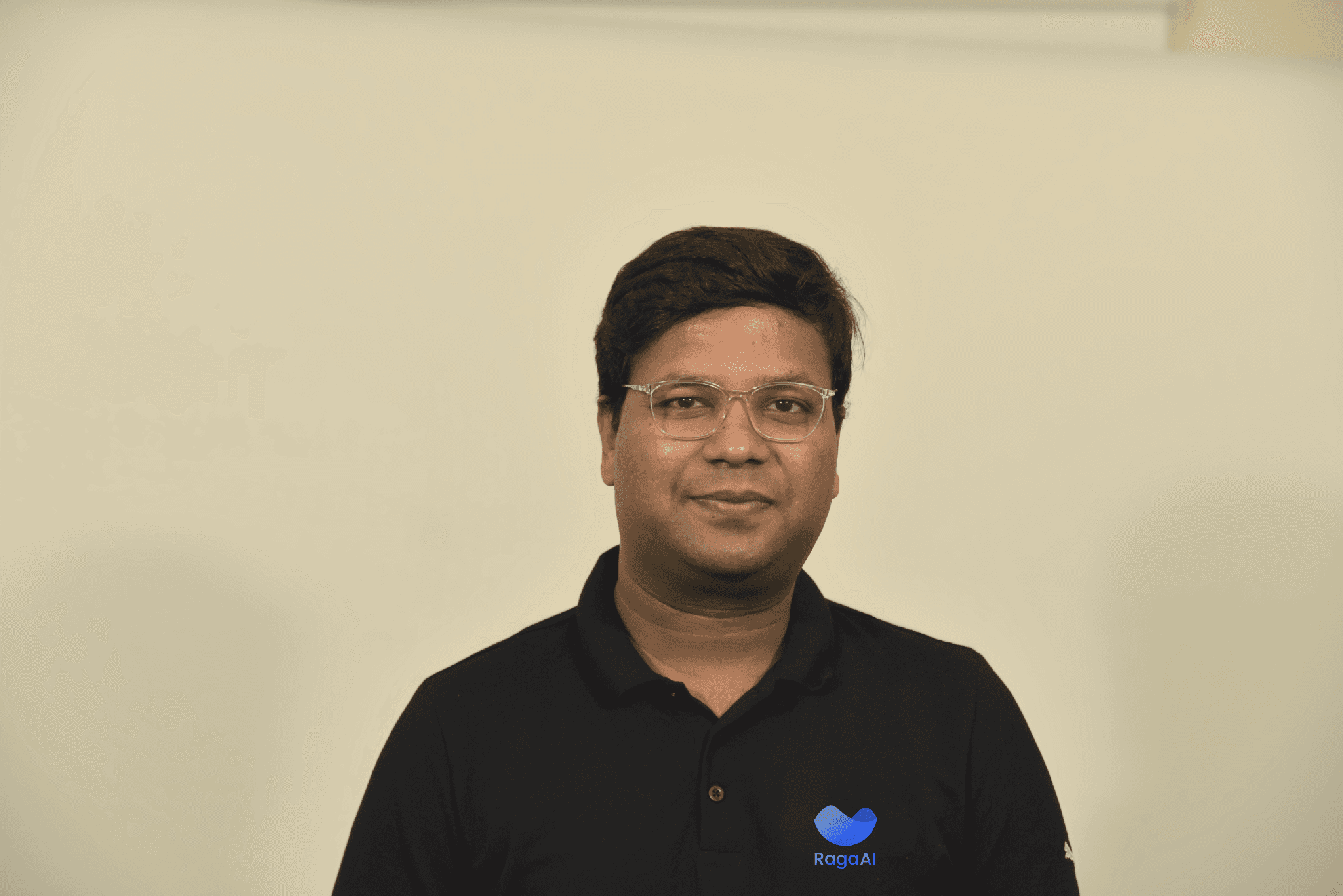
Jigar Gupta
Jul 13, 2024
Read the article




Comparing Different Large Language Model (LLM) Frameworks

Rehan Asif
Jul 12, 2024
Read the article
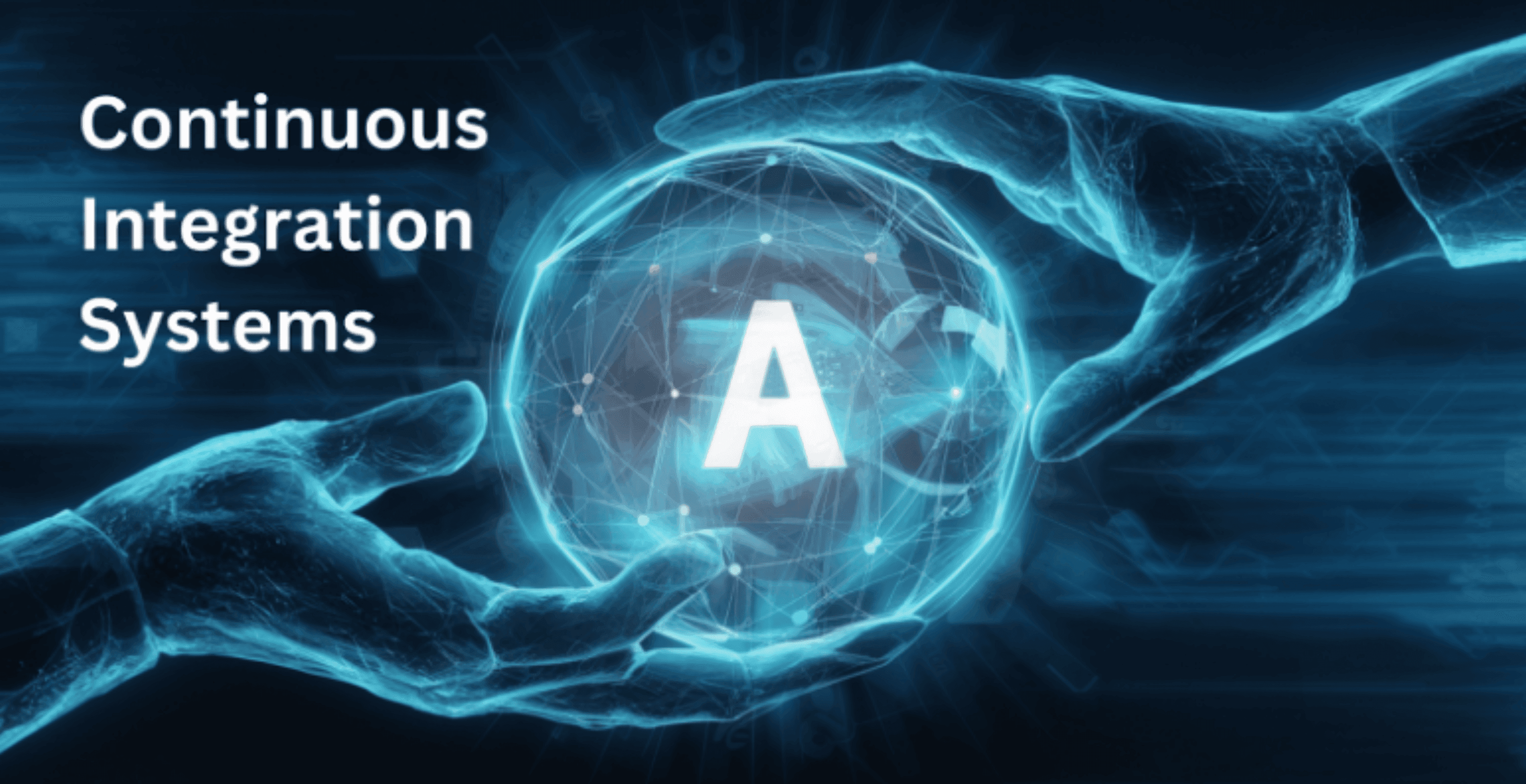
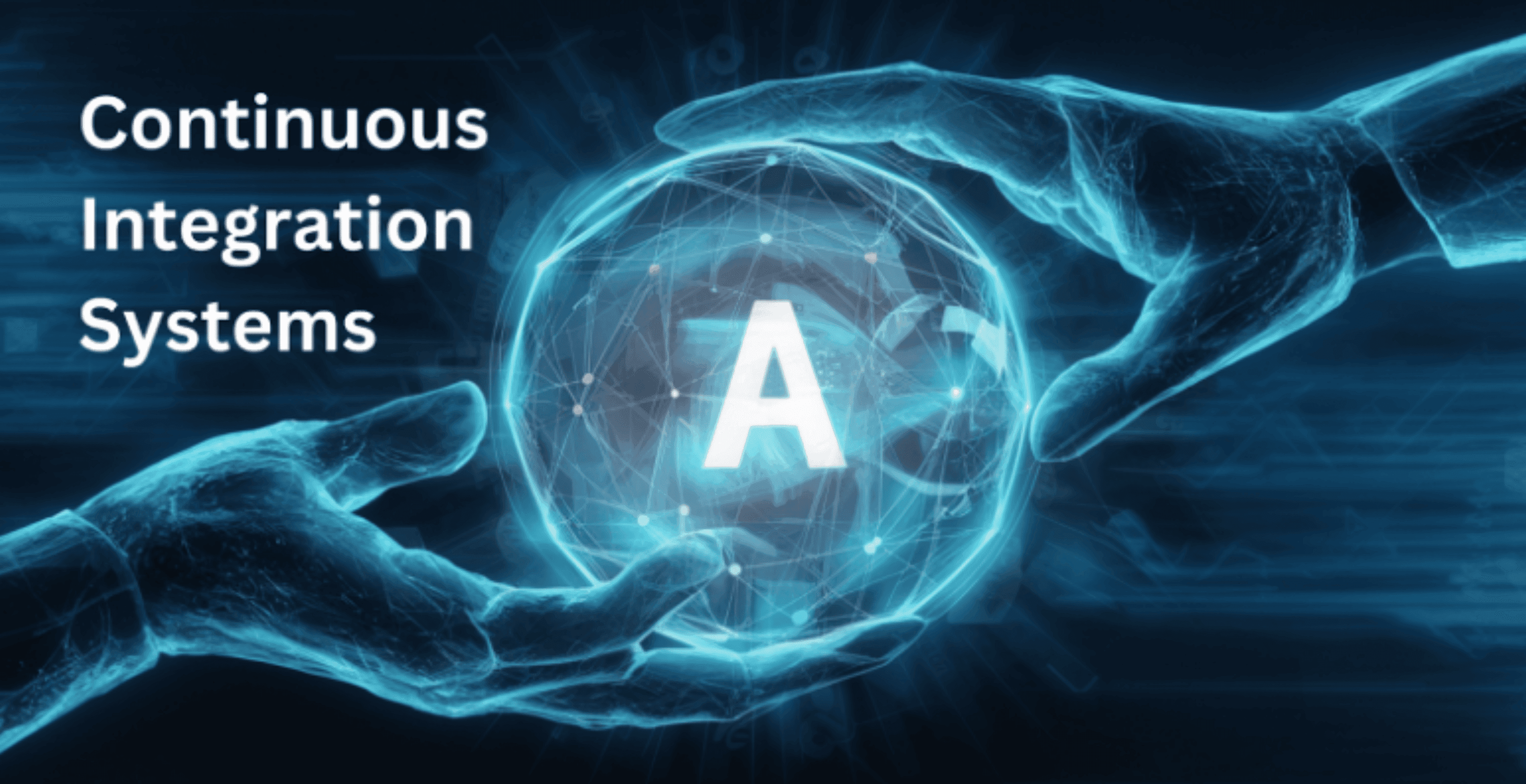
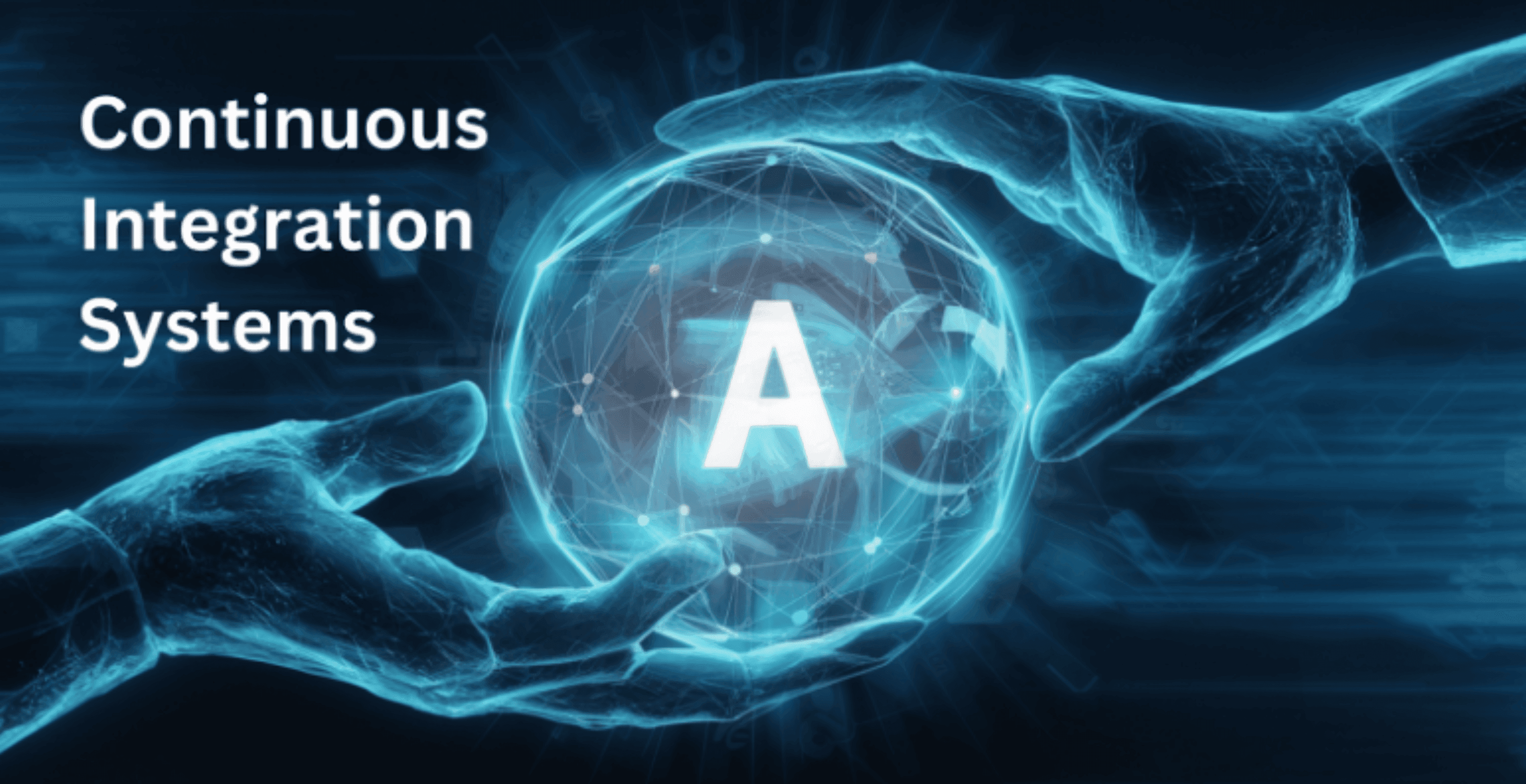
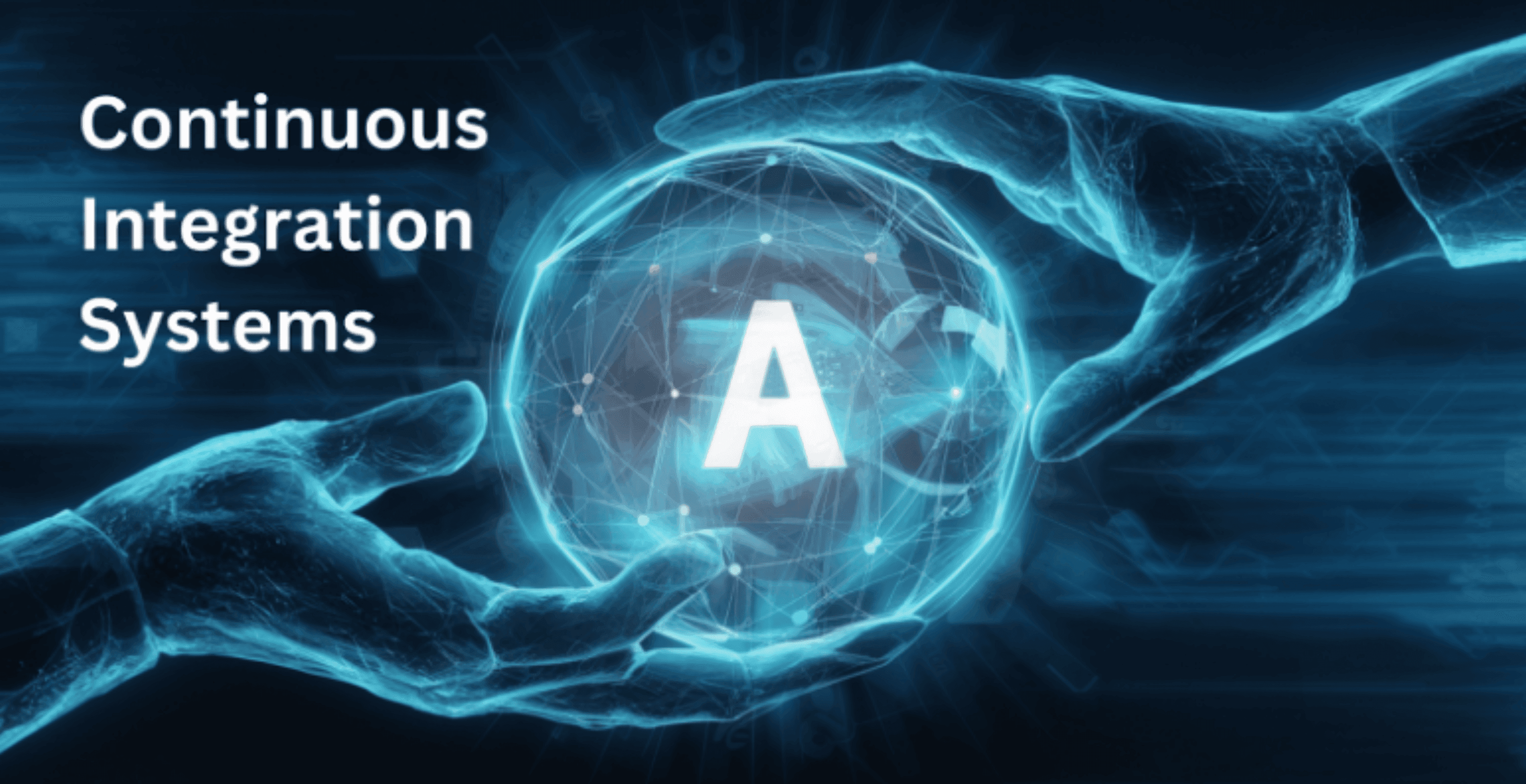
Integrating AI Models with Continuous Integration Systems
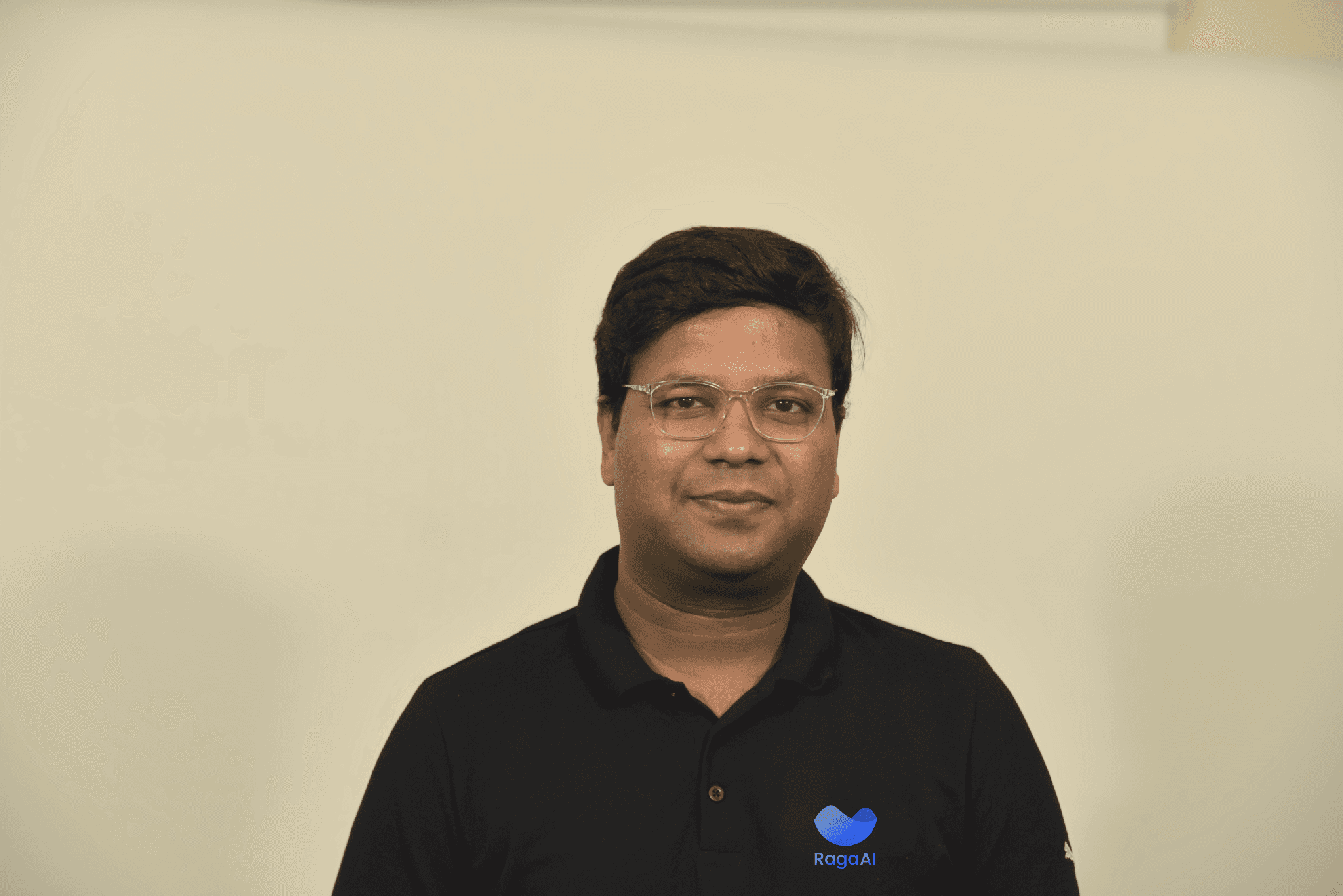
Jigar Gupta
Jul 12, 2024
Read the article




Understanding Retrieval Augmented Generation for Large Language Models: A Survey
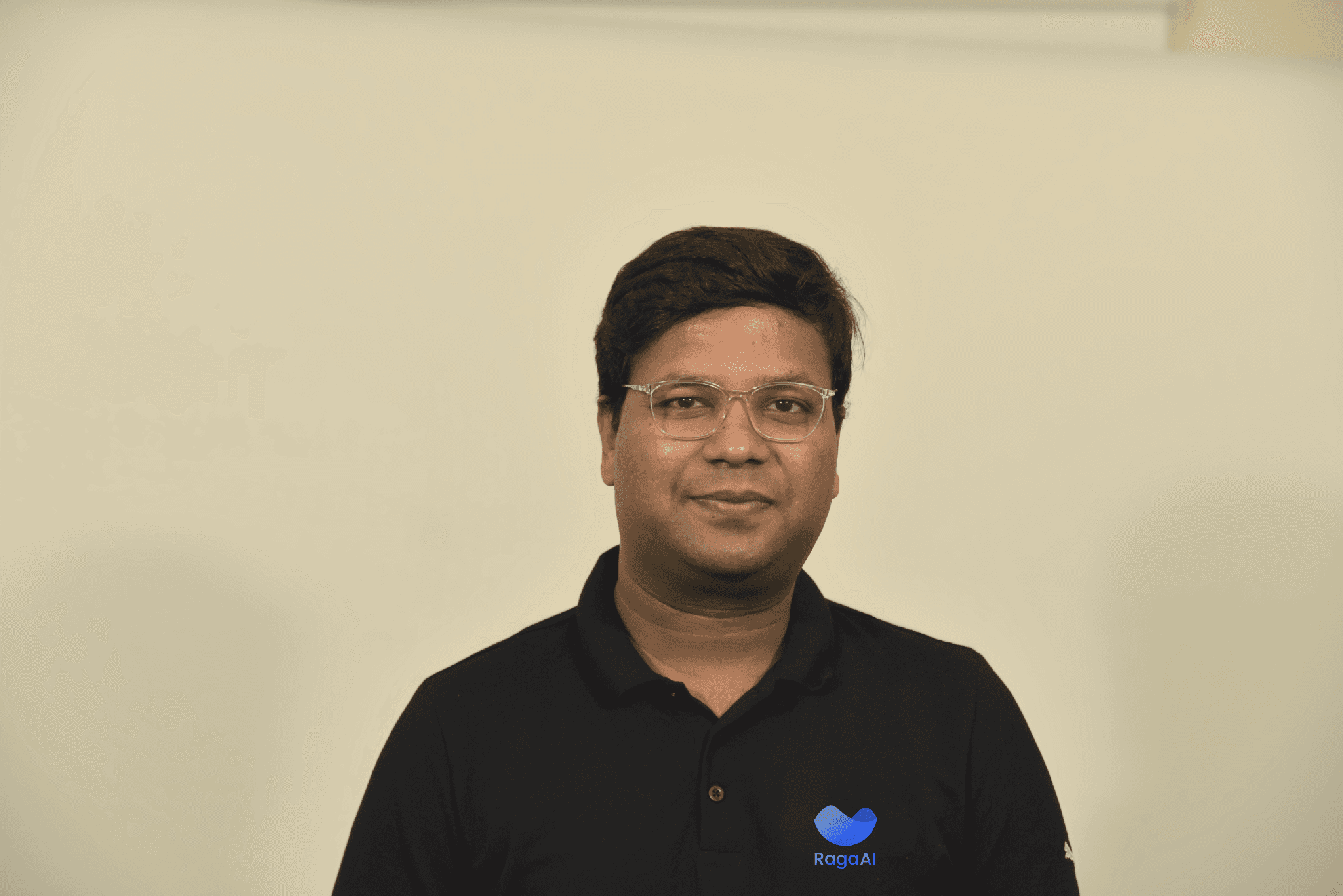
Jigar Gupta
Jul 12, 2024
Read the article




Leveraging AI For Enhanced Retail Customer Experiences
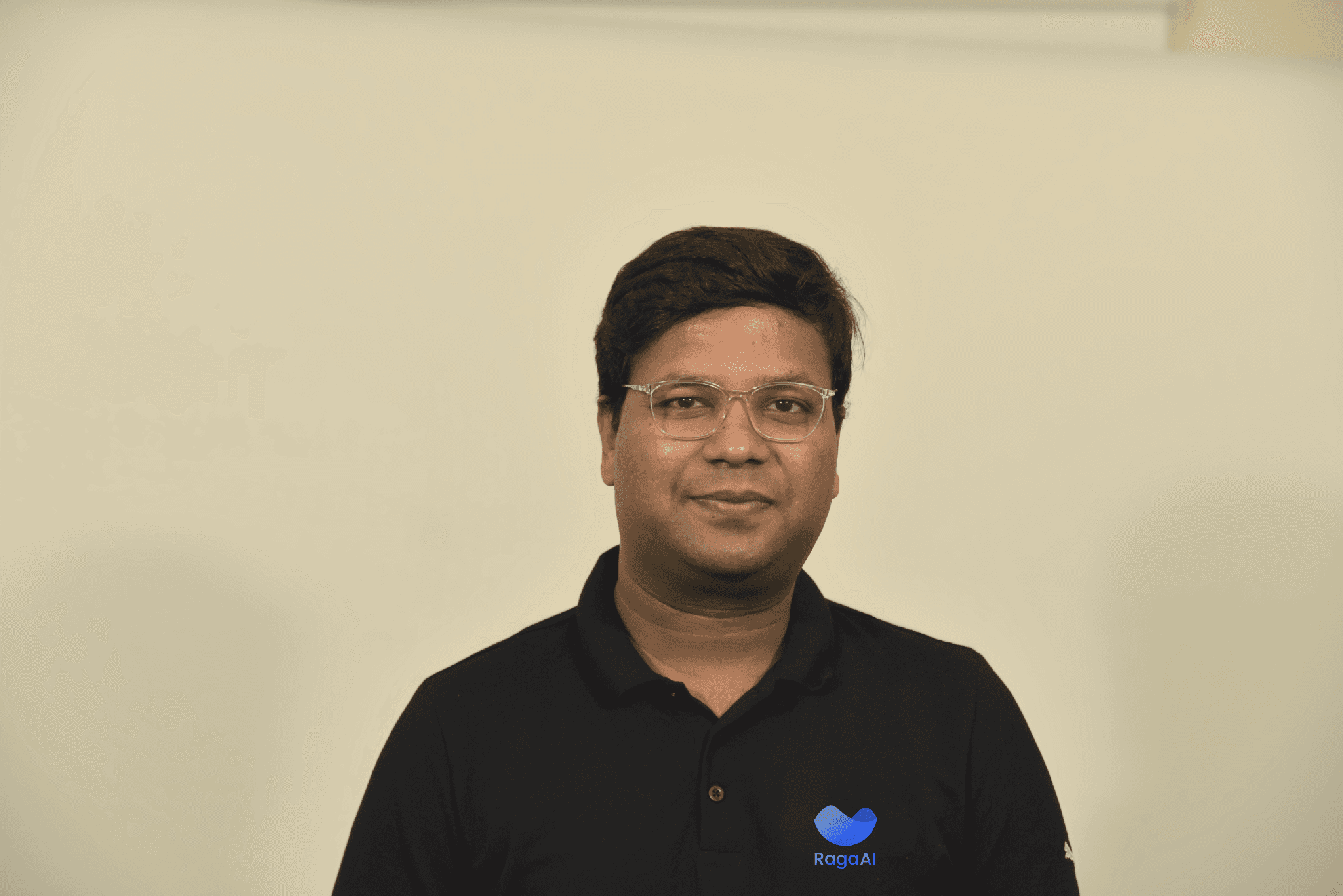
Jigar Gupta
Jul 1, 2024
Read the article
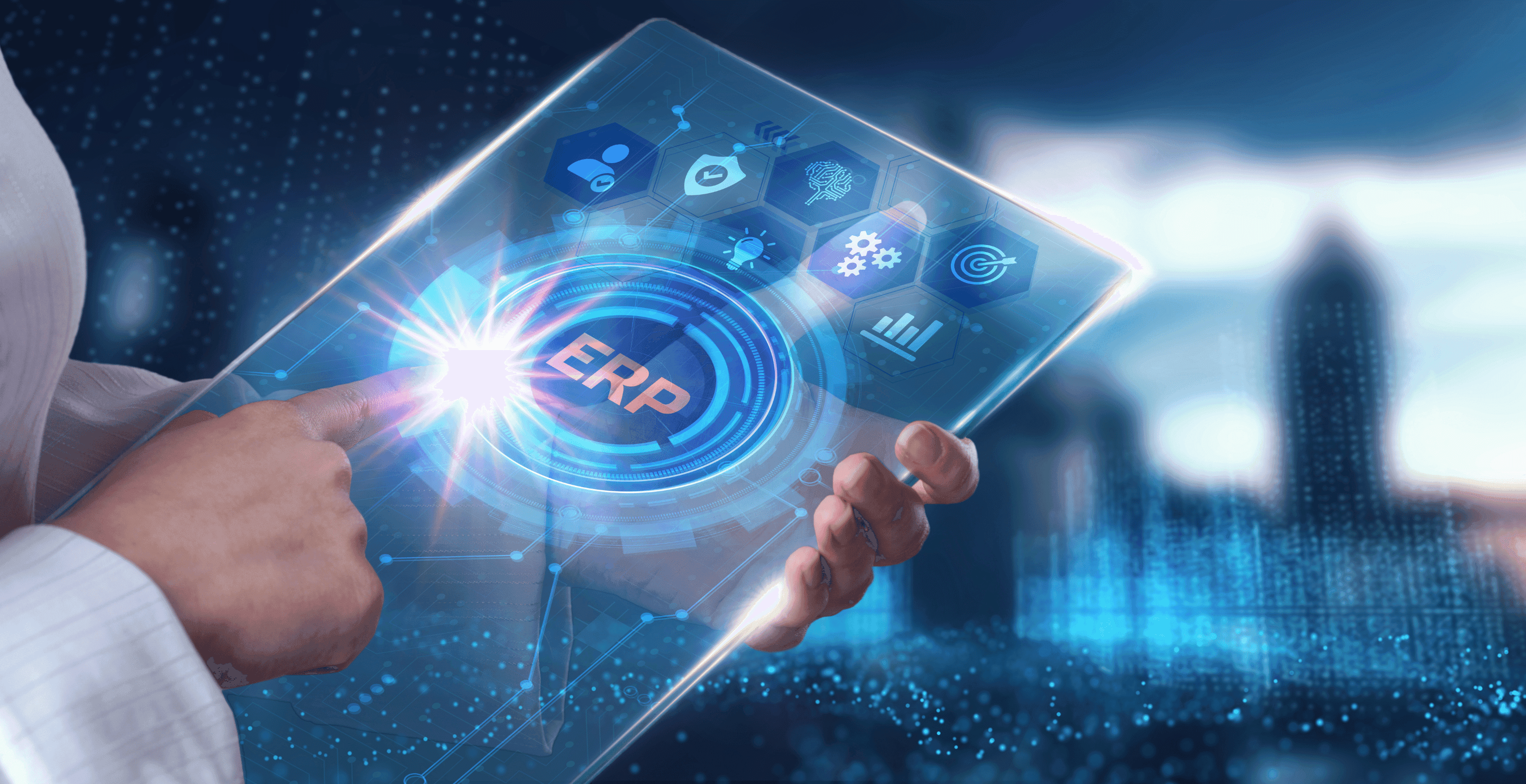
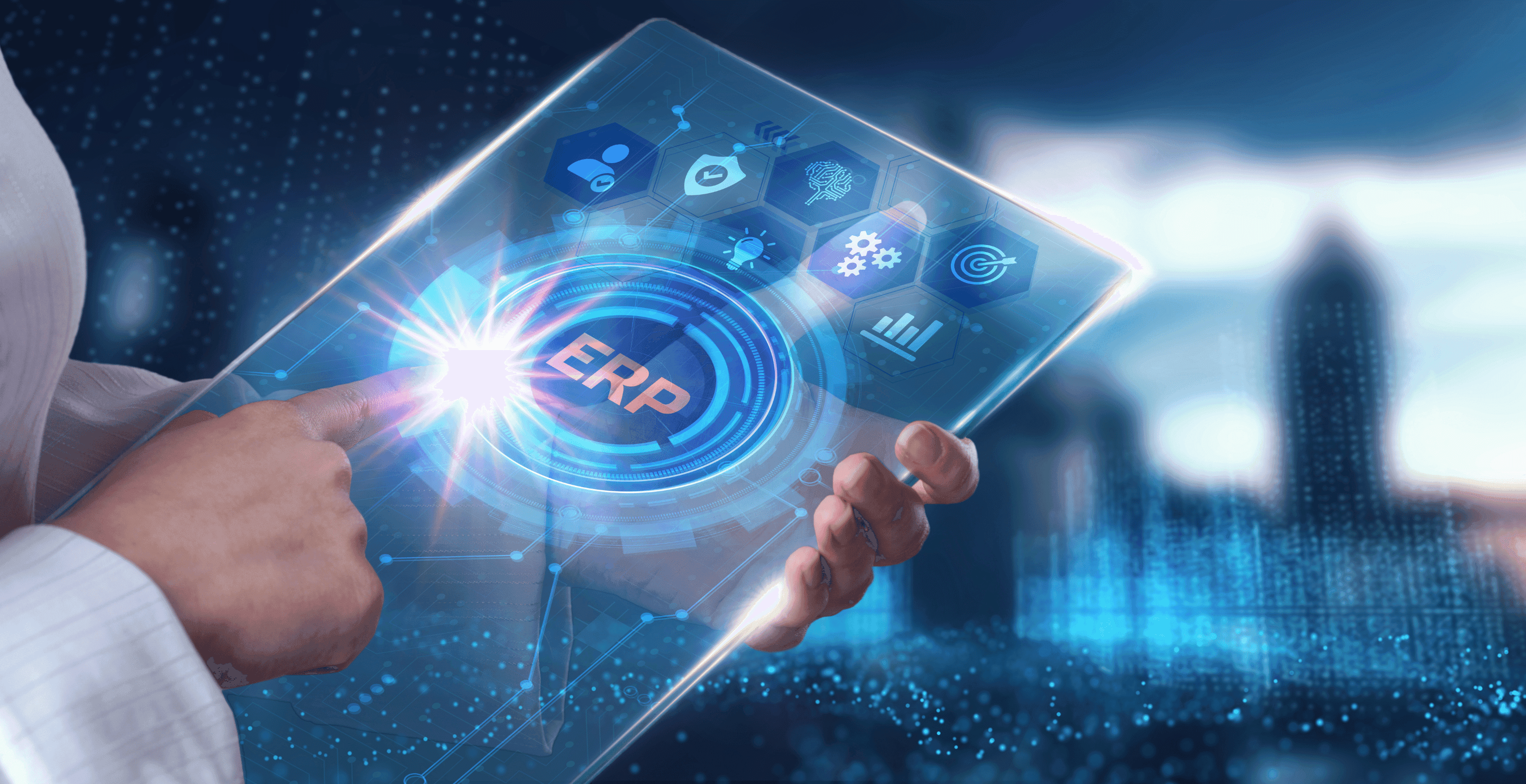
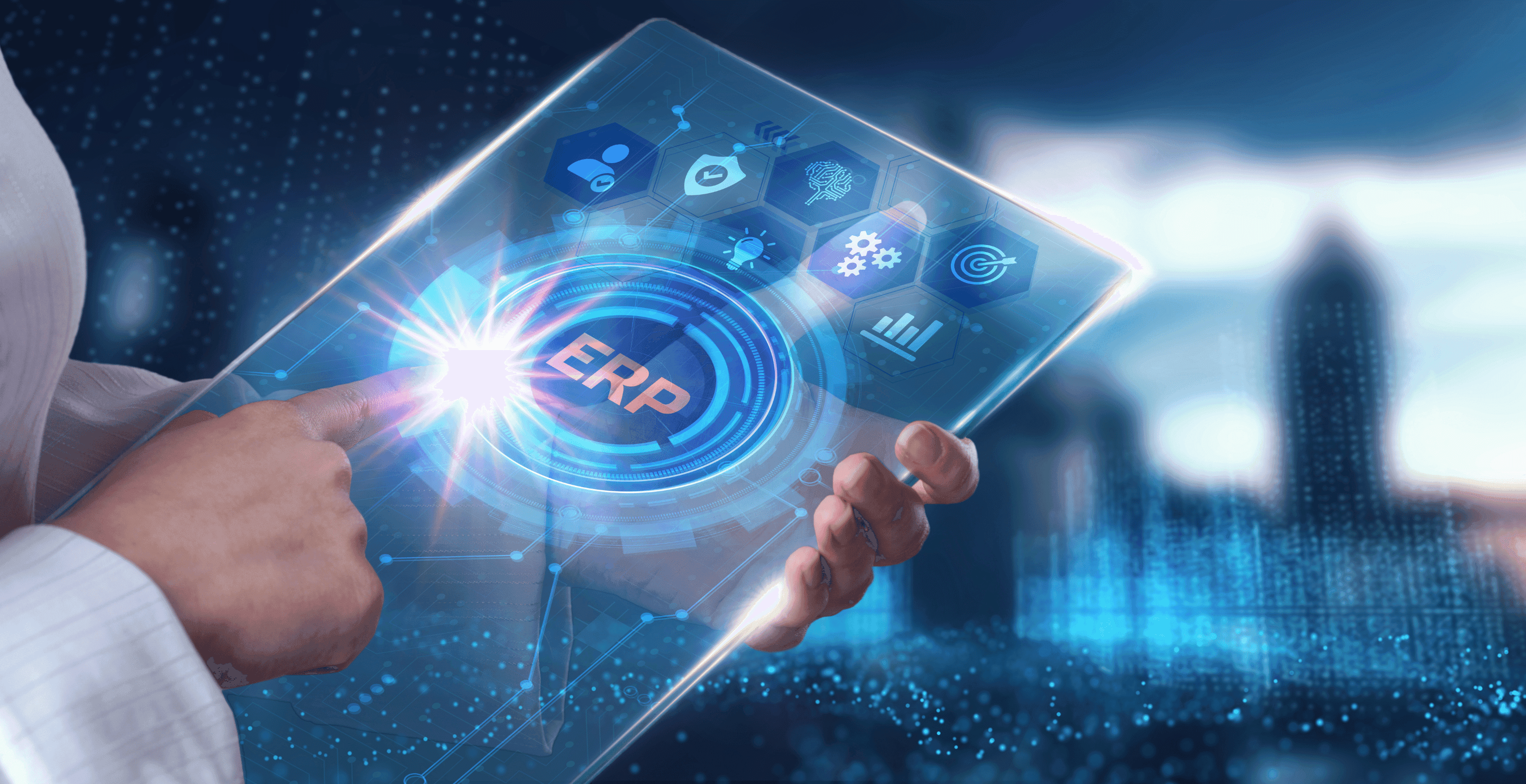
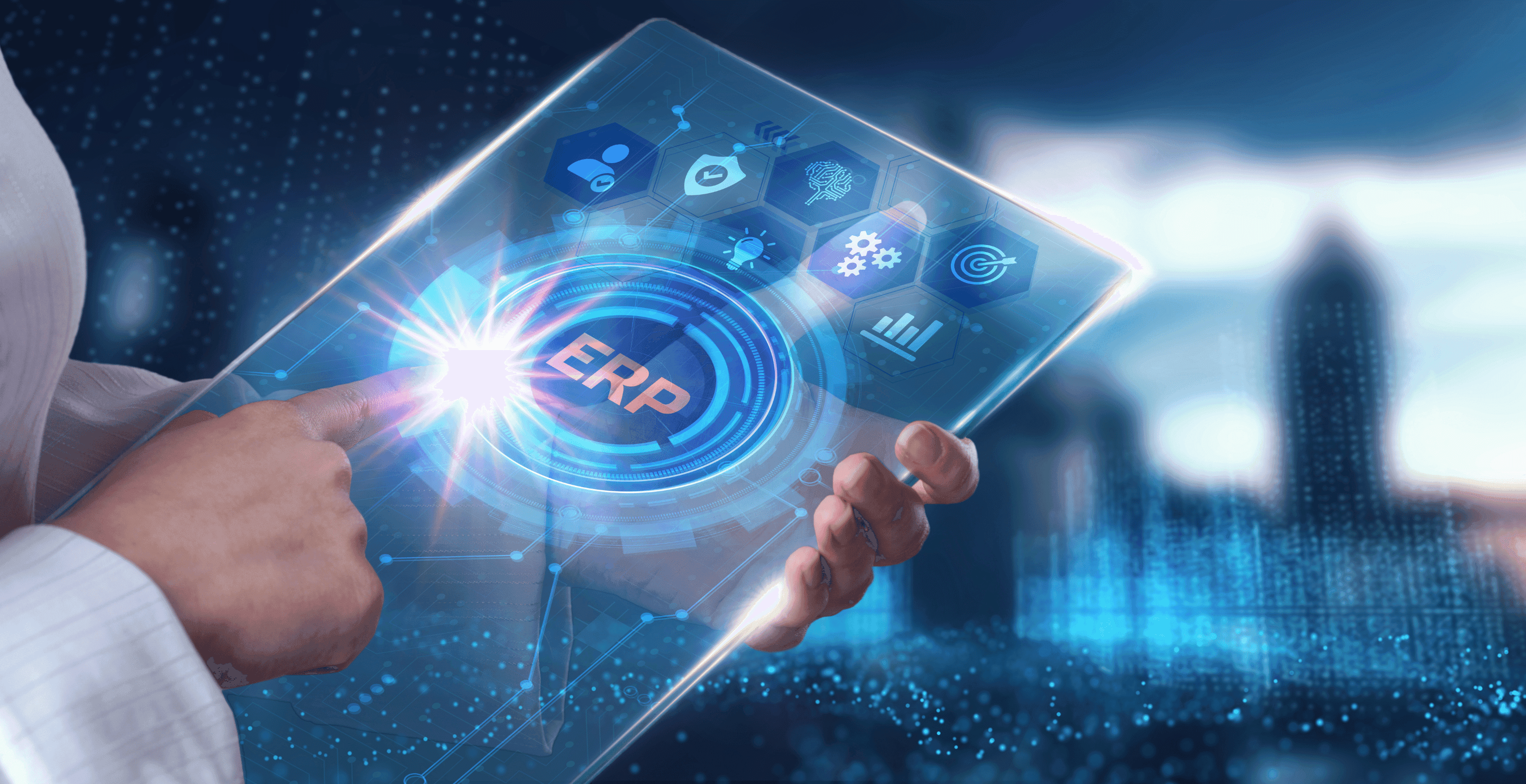
Enhancing Enterprise Search Using RAG and LLMs

Rehan Asif
Jul 1, 2024
Read the article
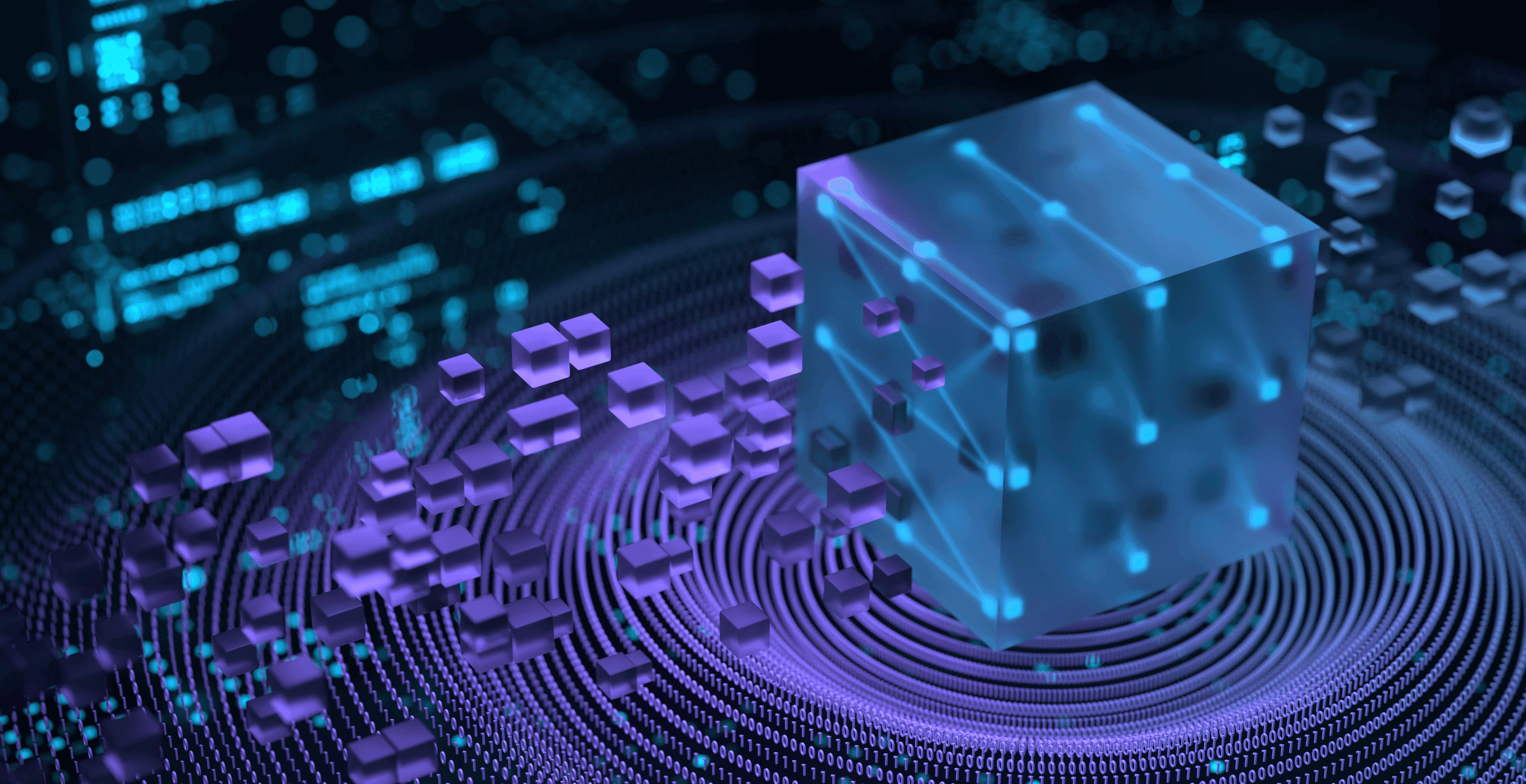
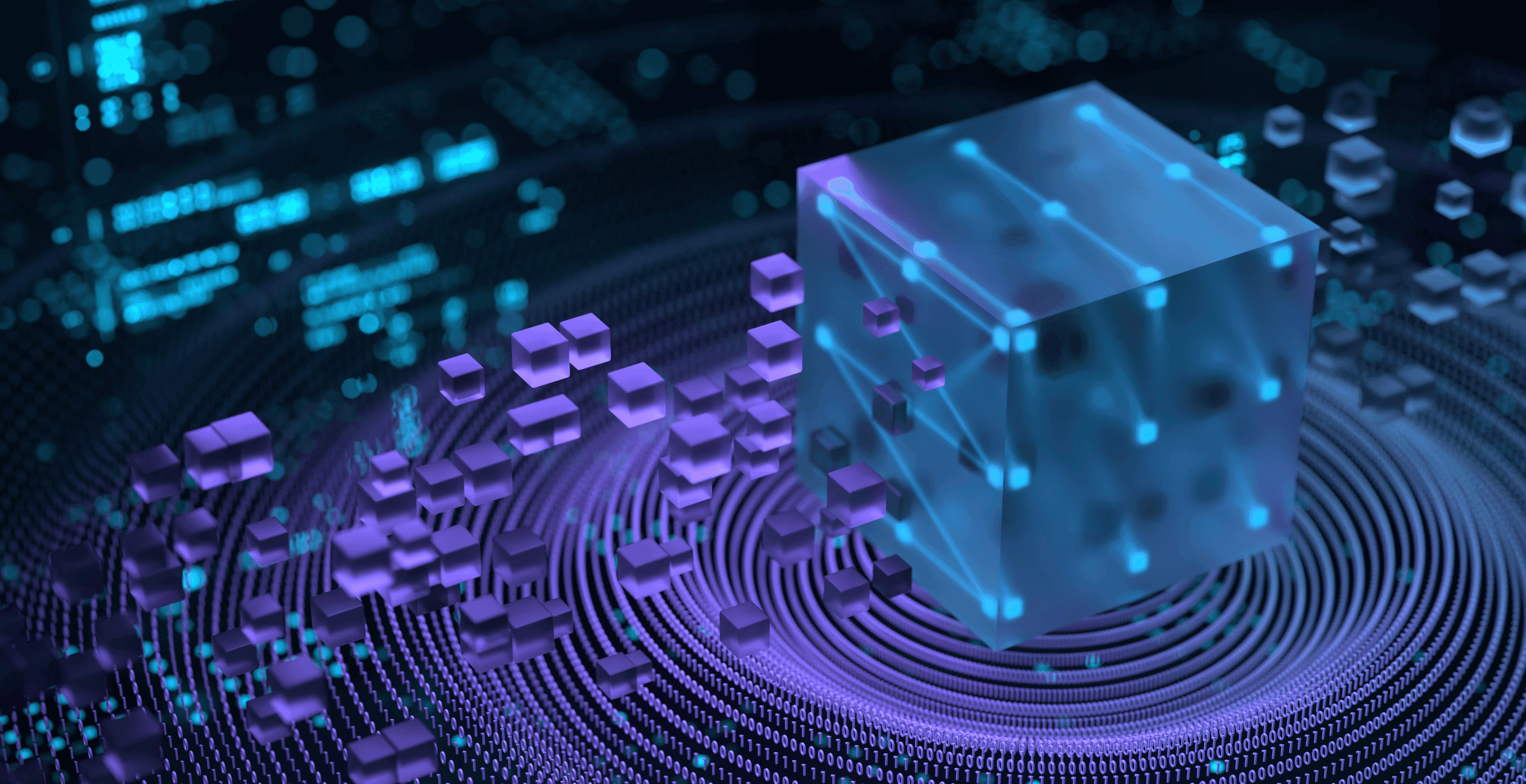
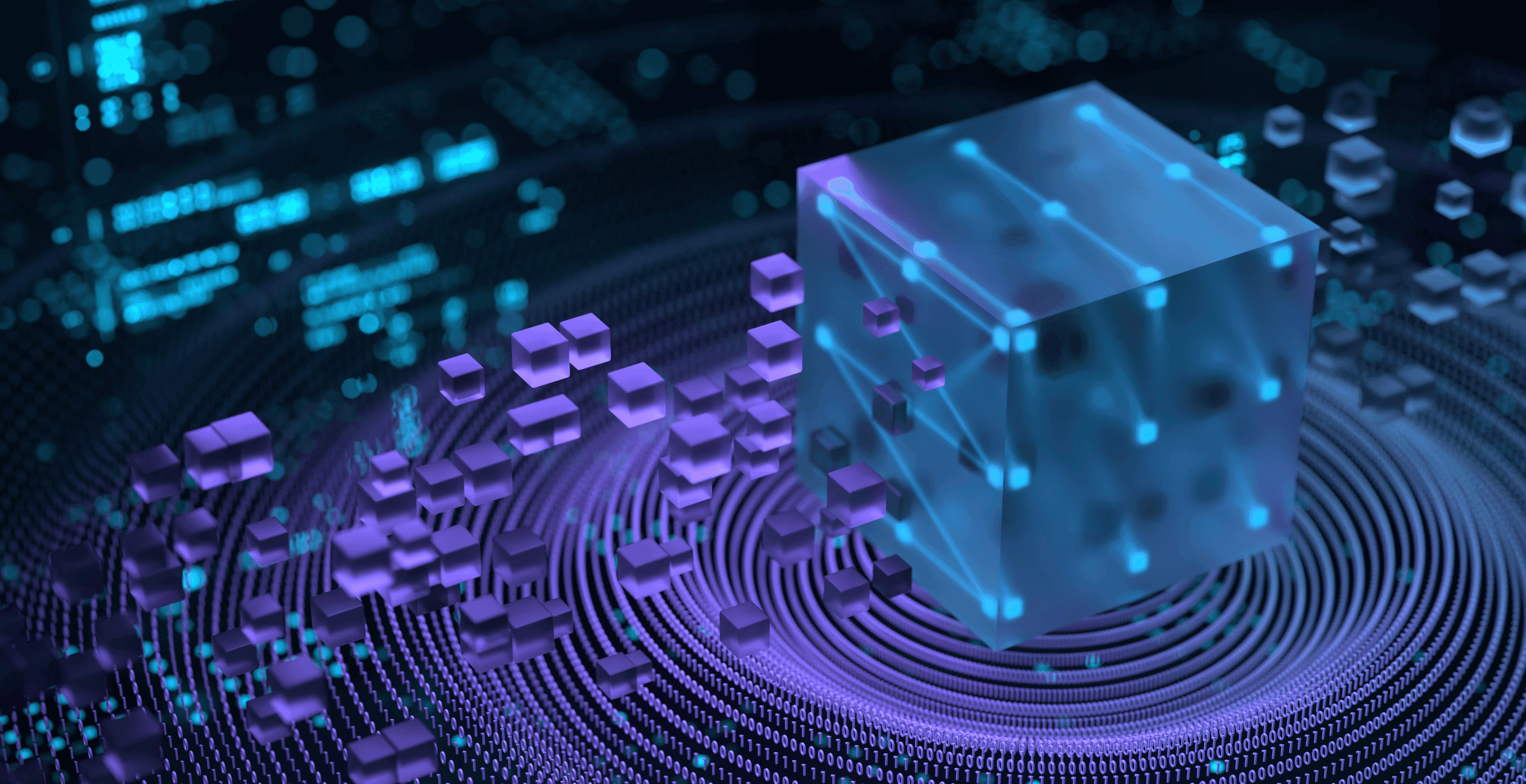
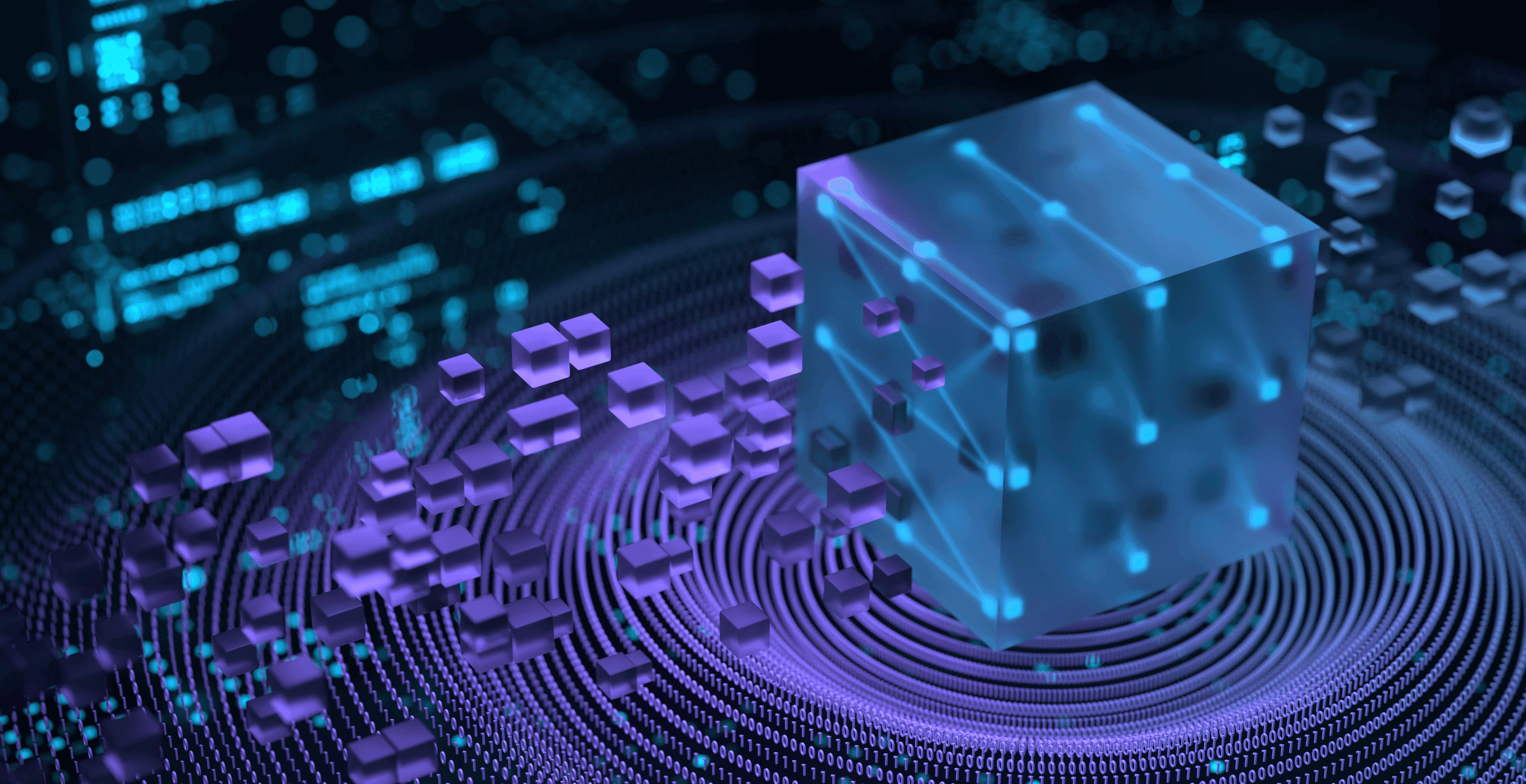
Importance of Accuracy and Reliability in Tabular Data Models
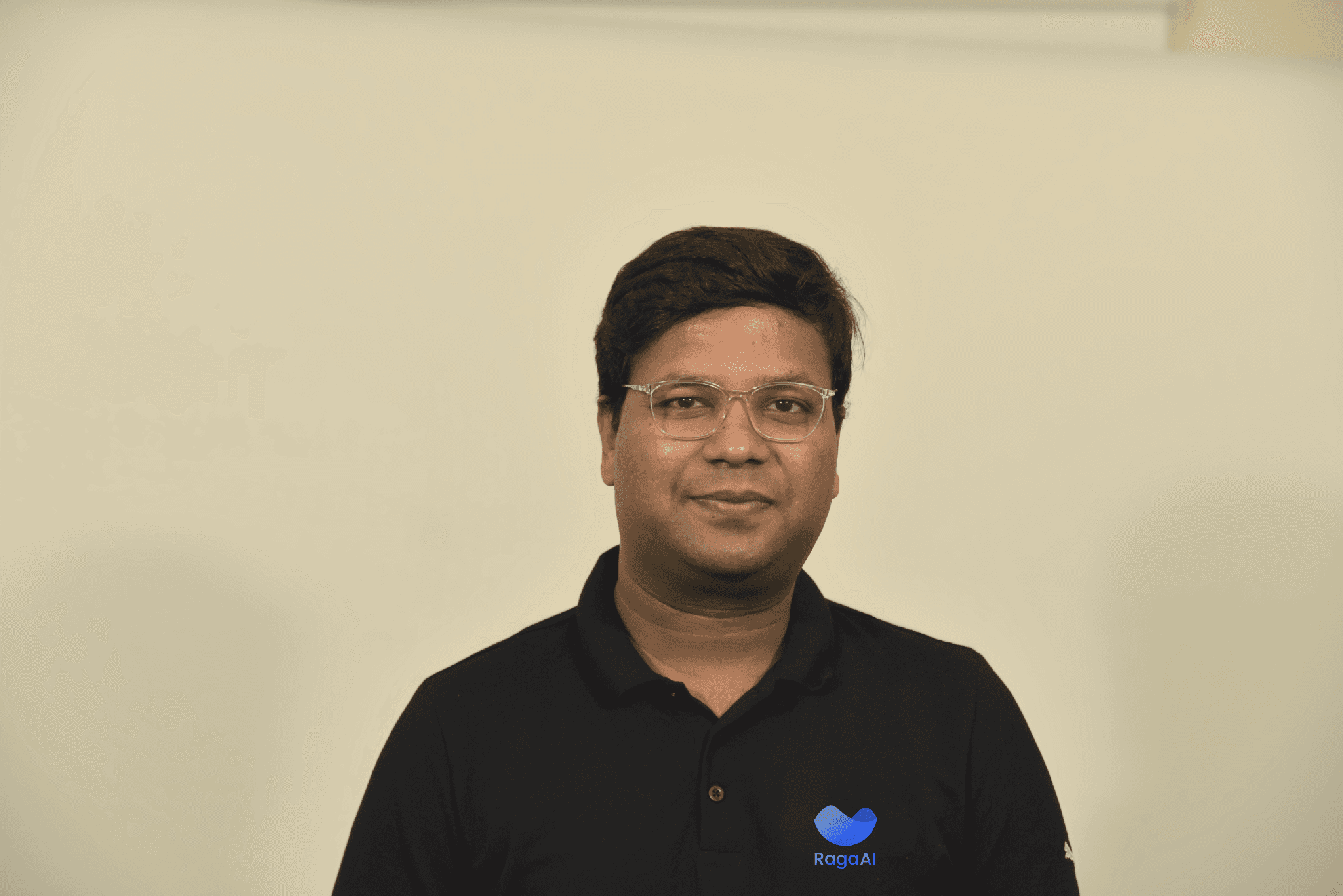
Jigar Gupta
Jul 1, 2024
Read the article
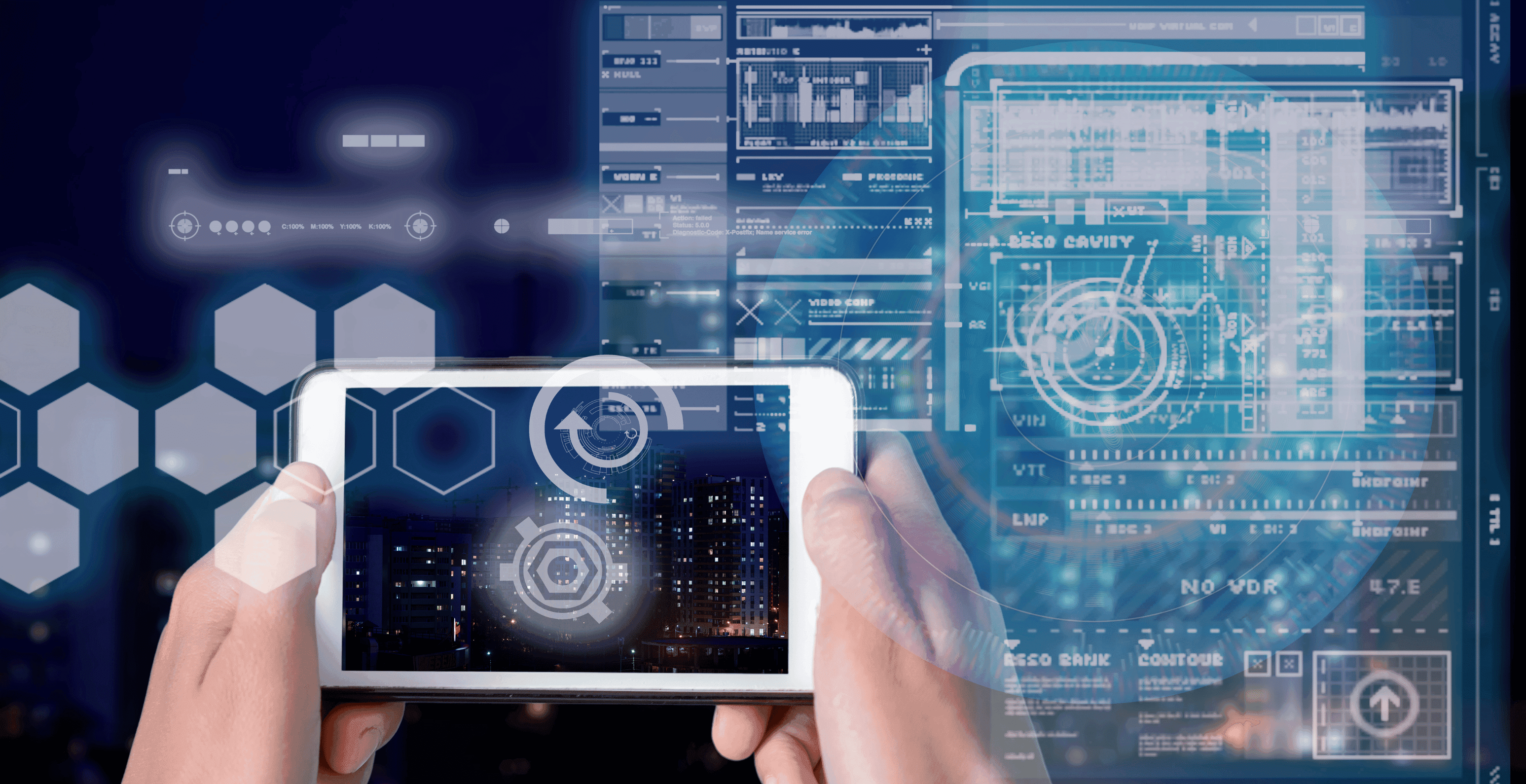
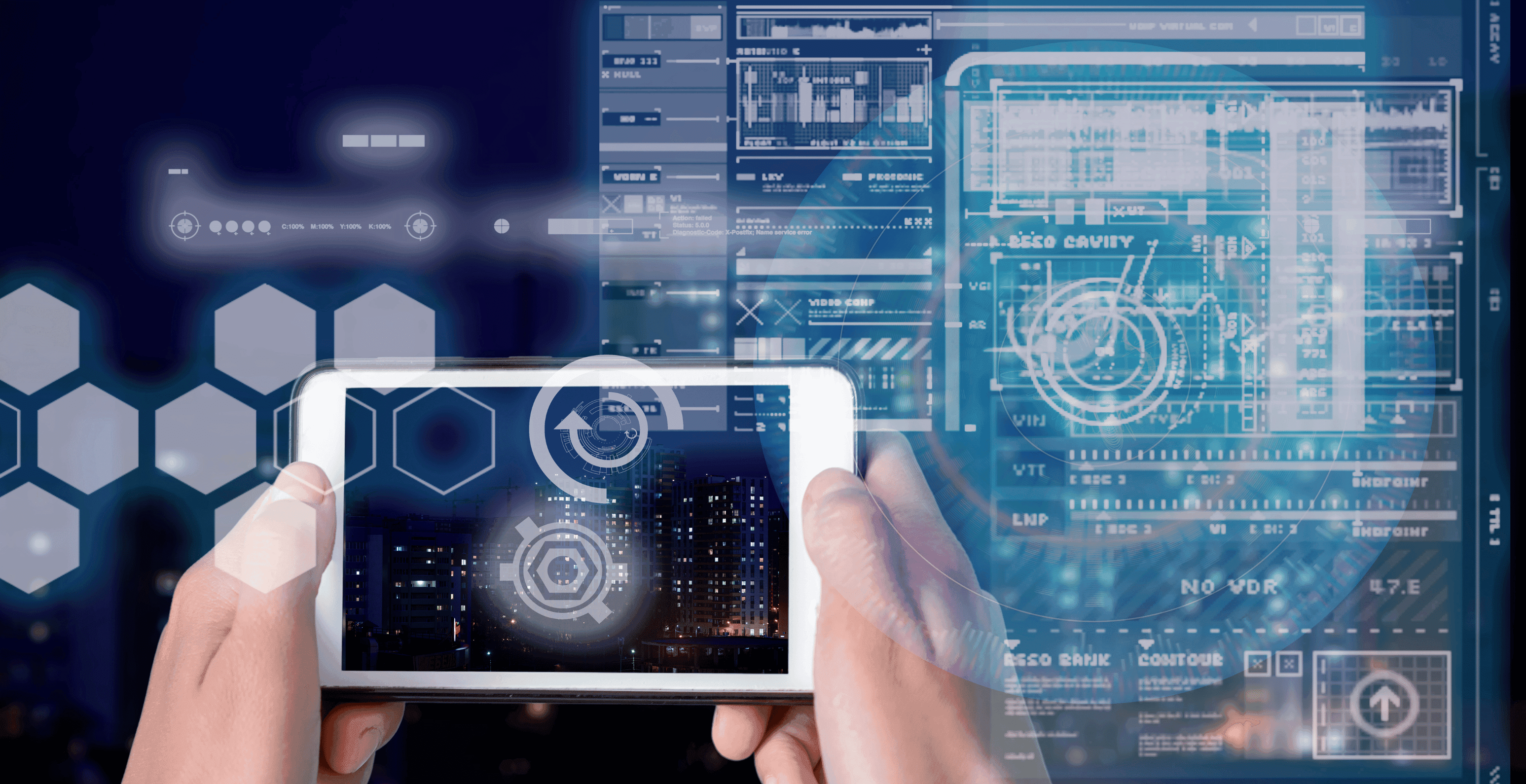
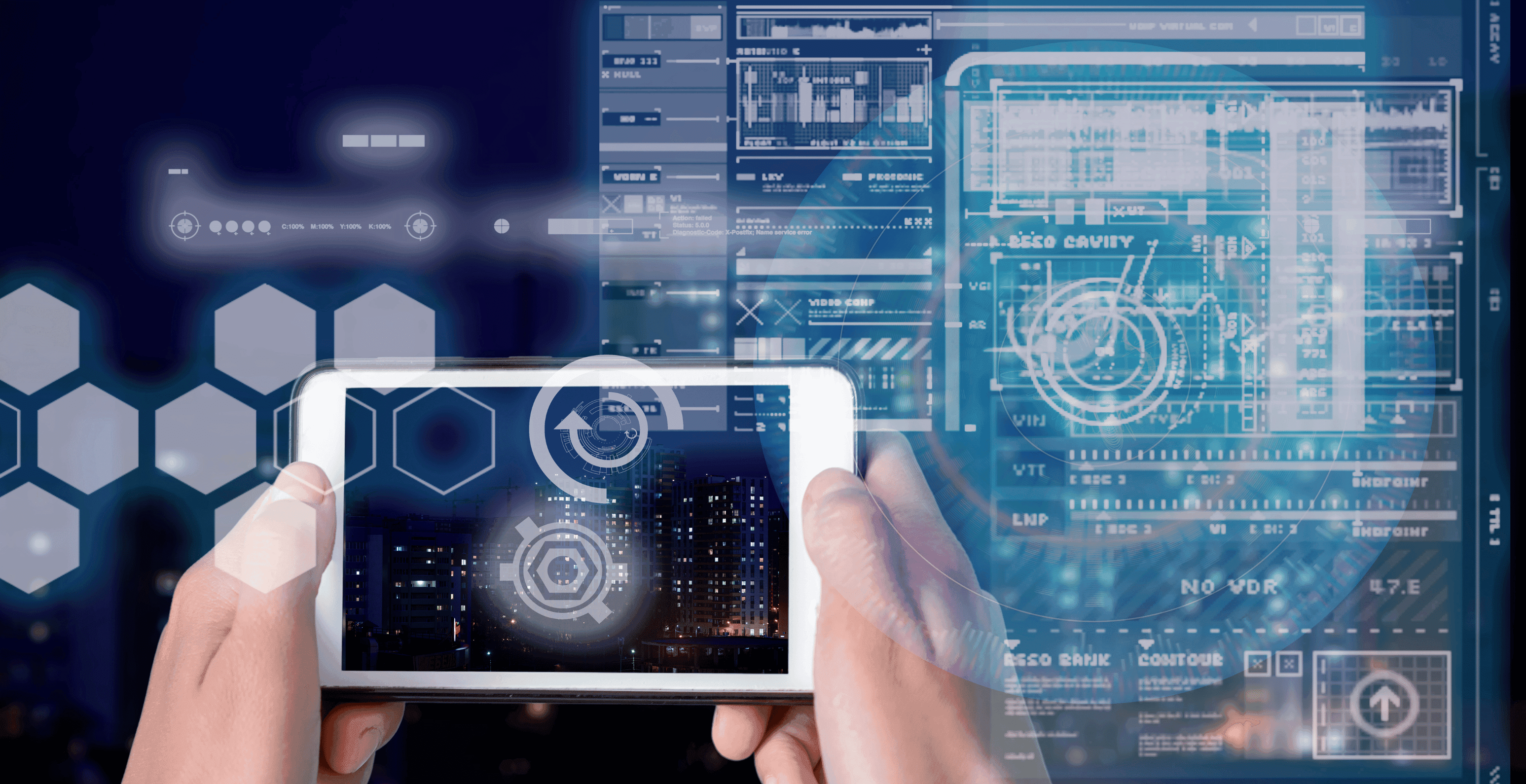
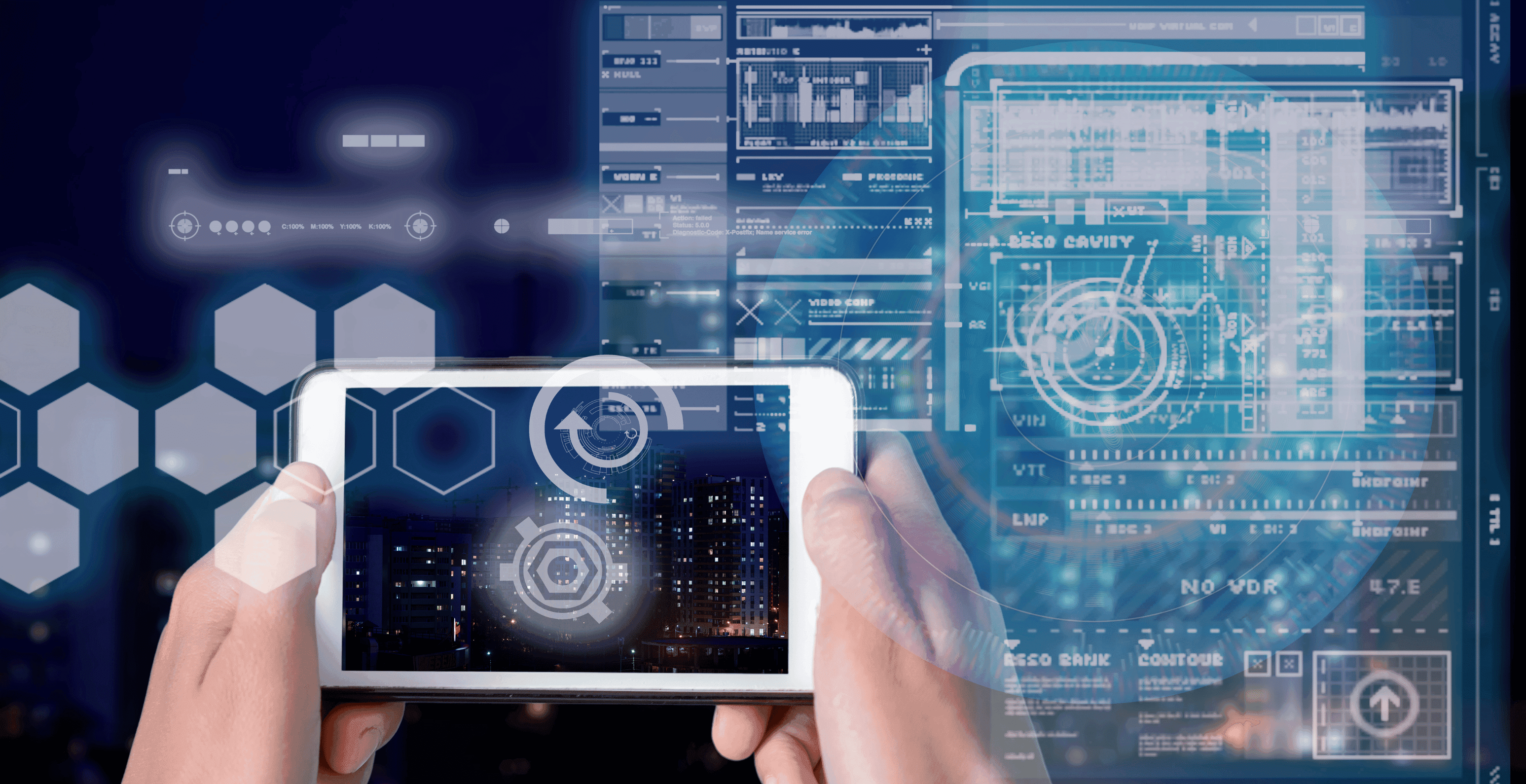
Information Retrieval And LLMs: RAG Explained

Rehan Asif
Jul 1, 2024
Read the article




Introduction to LLM Powered Autonomous Agents

Rehan Asif
Jul 1, 2024
Read the article
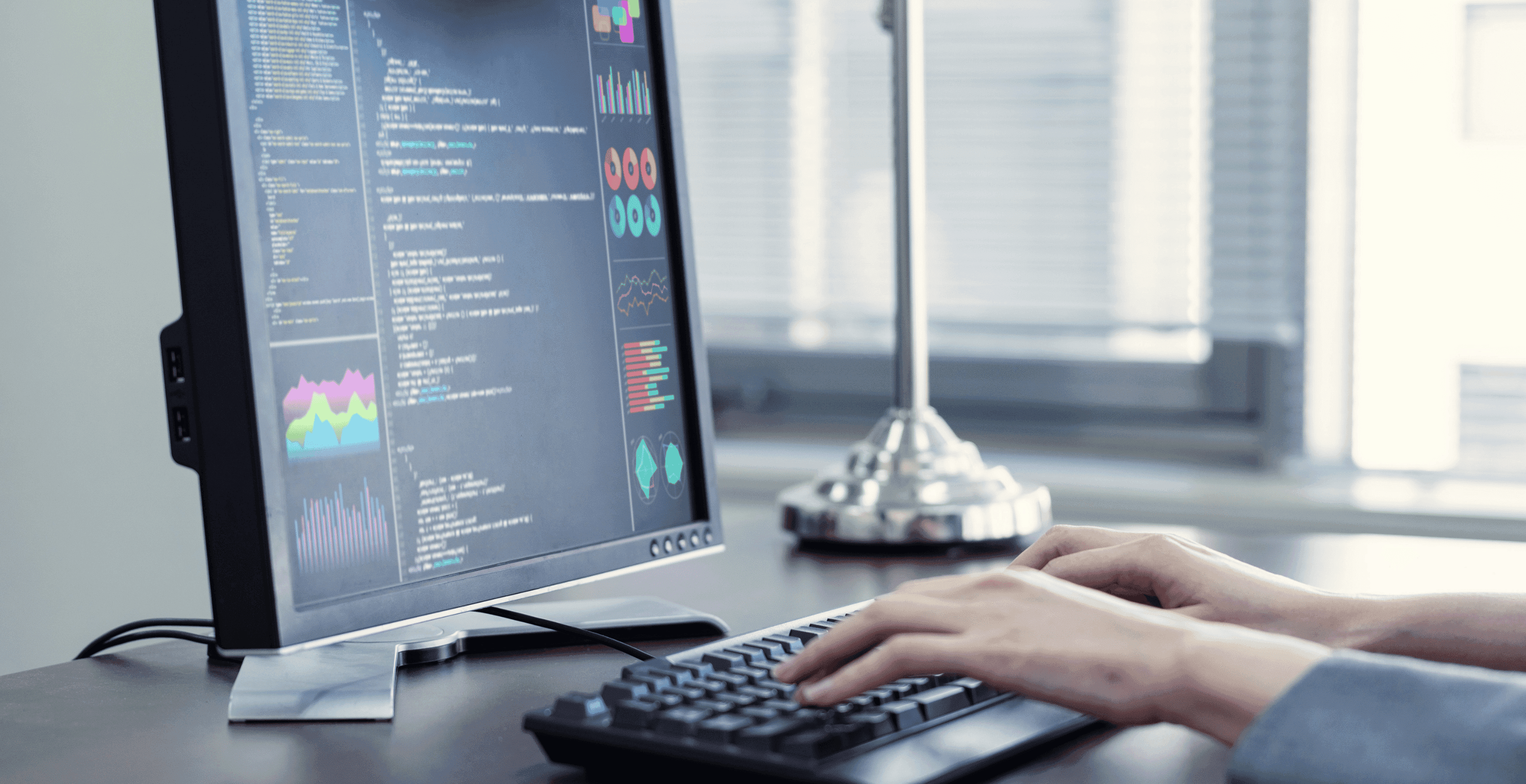
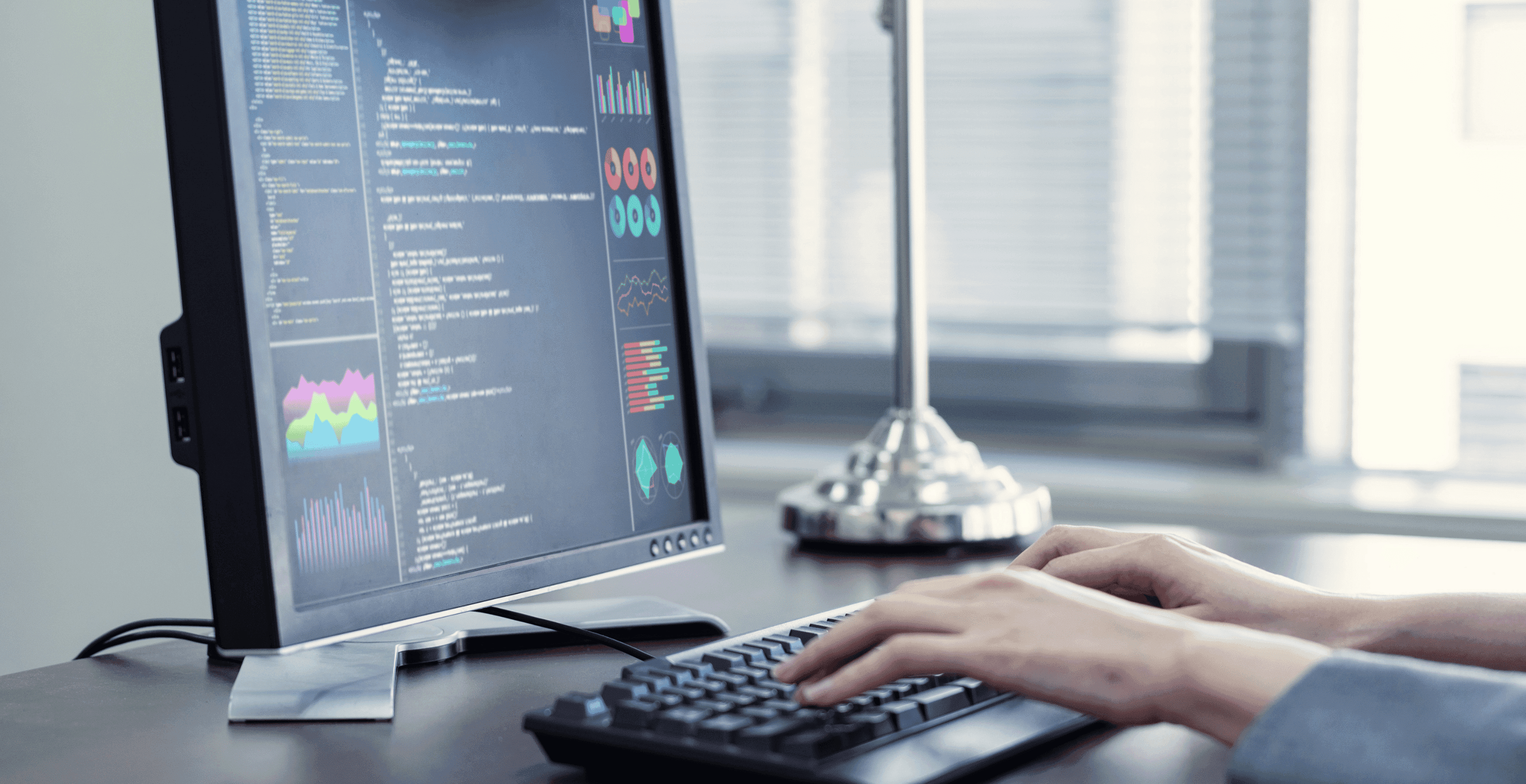
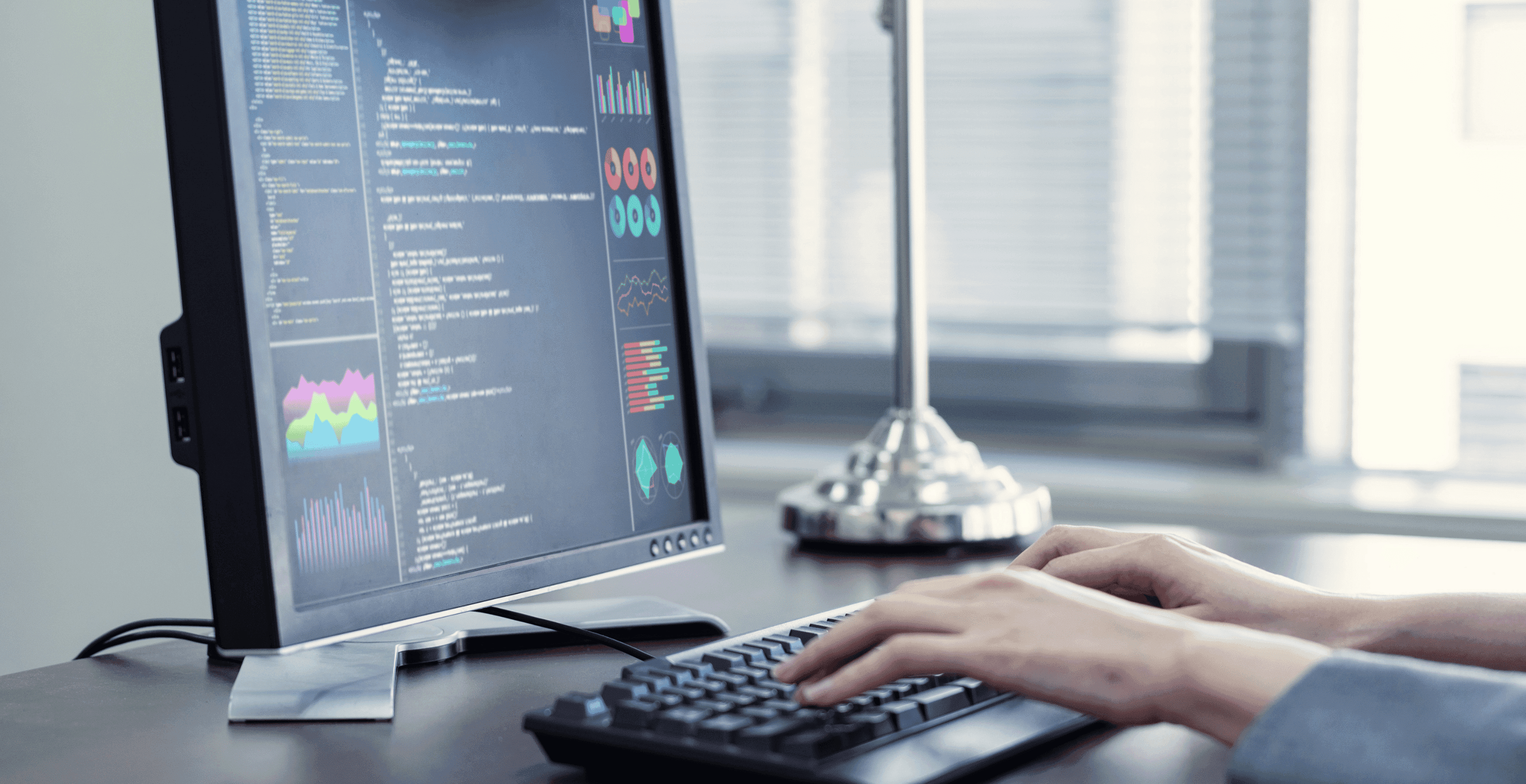
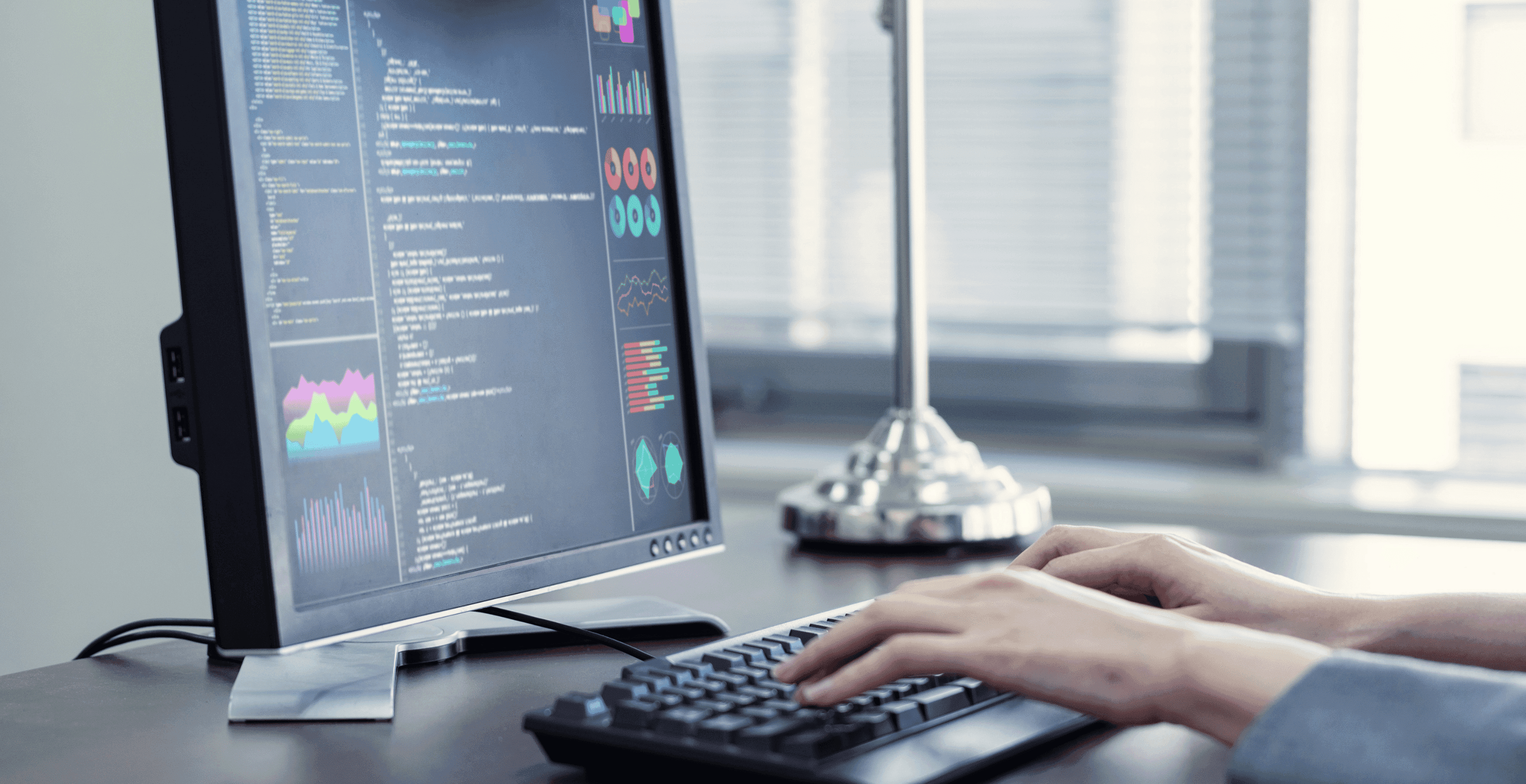
Guide on Unified Multi-Dimensional LLM Evaluation and Benchmark Metrics

Rehan Asif
Jul 1, 2024
Read the article




Innovations In AI For Healthcare
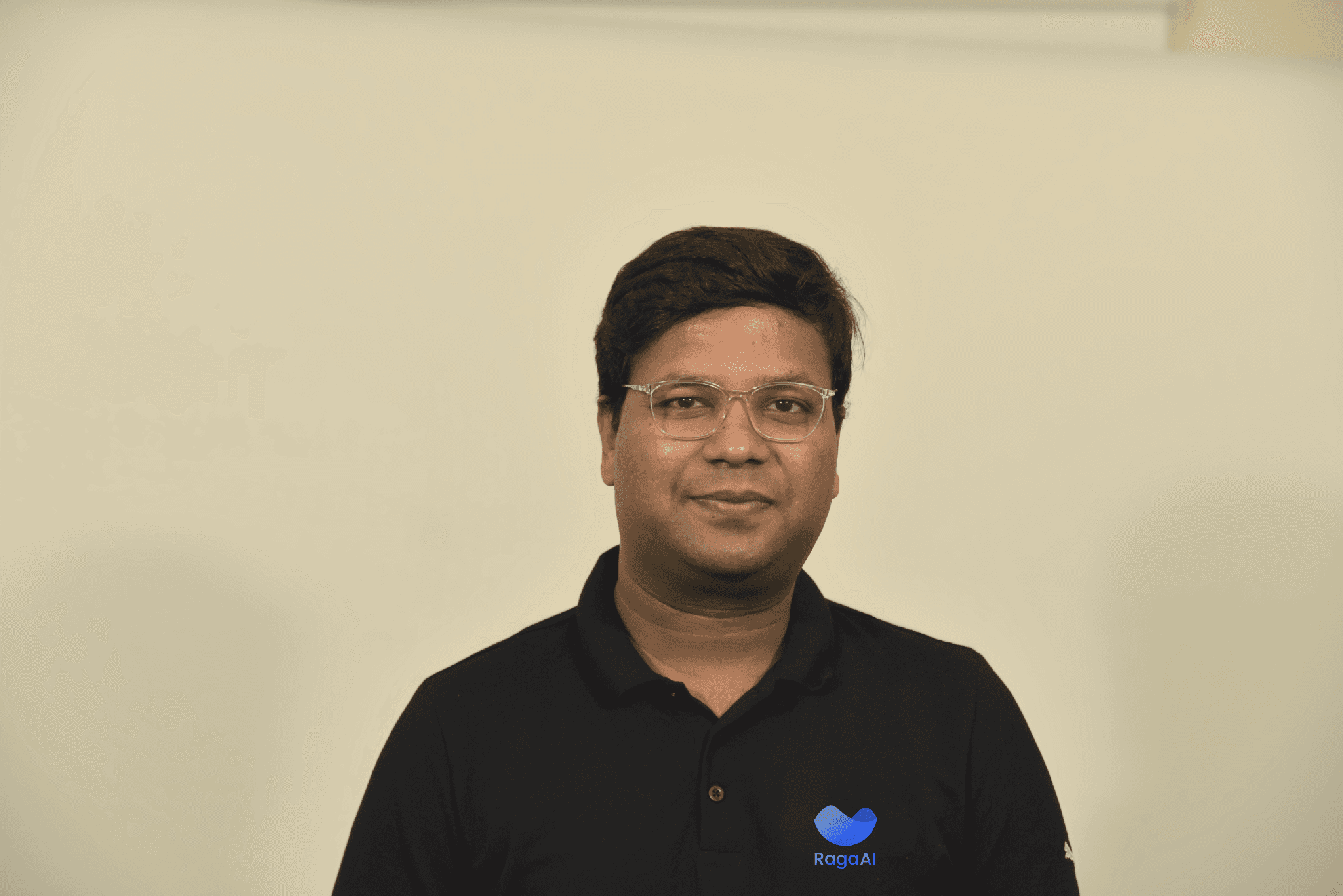
Jigar Gupta
Jun 24, 2024
Read the article




Implementing AI-Driven Inventory Management For The Retail Industry
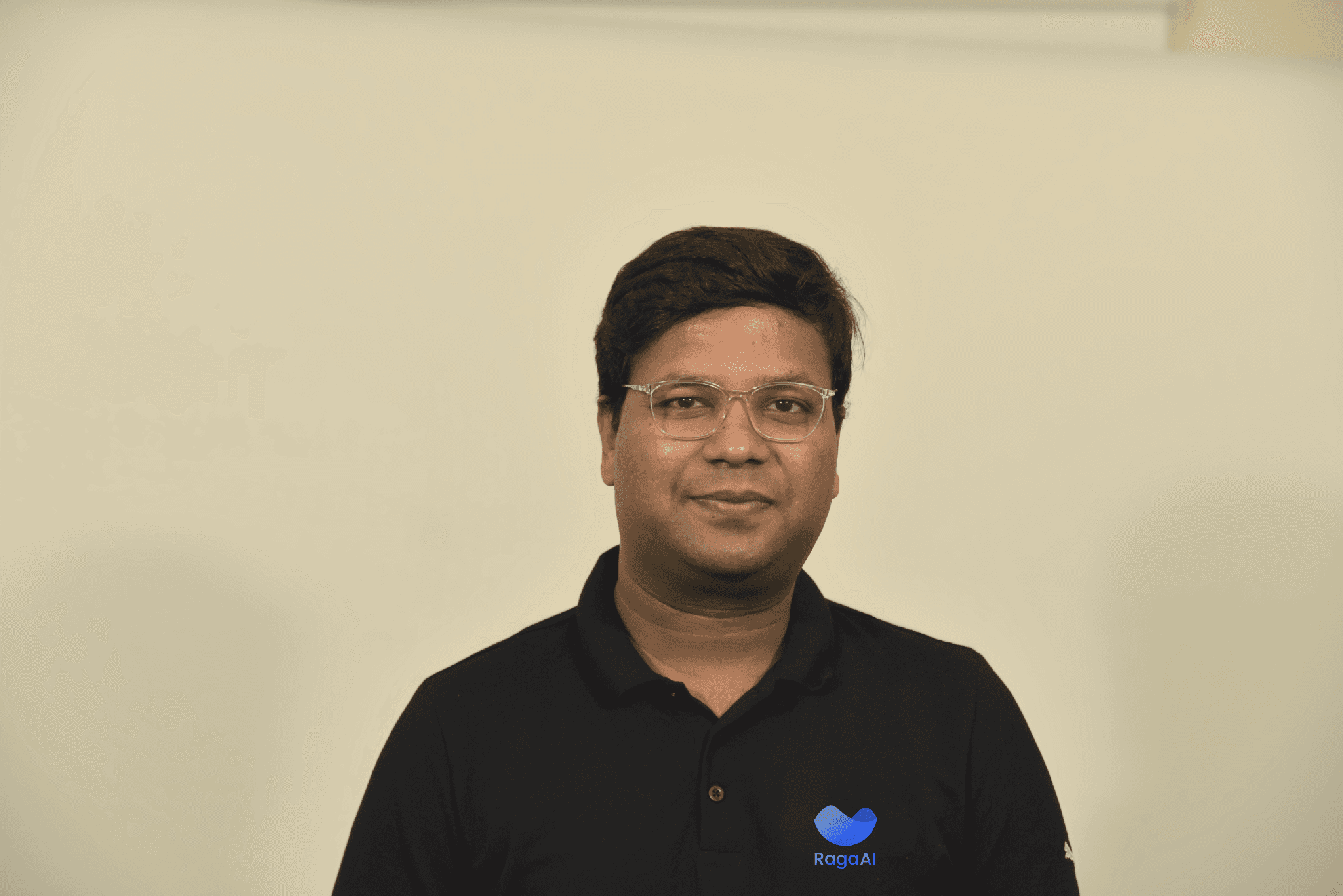
Jigar Gupta
Jun 24, 2024
Read the article




Practical Retrieval Augmented Generation: Use Cases And Impact
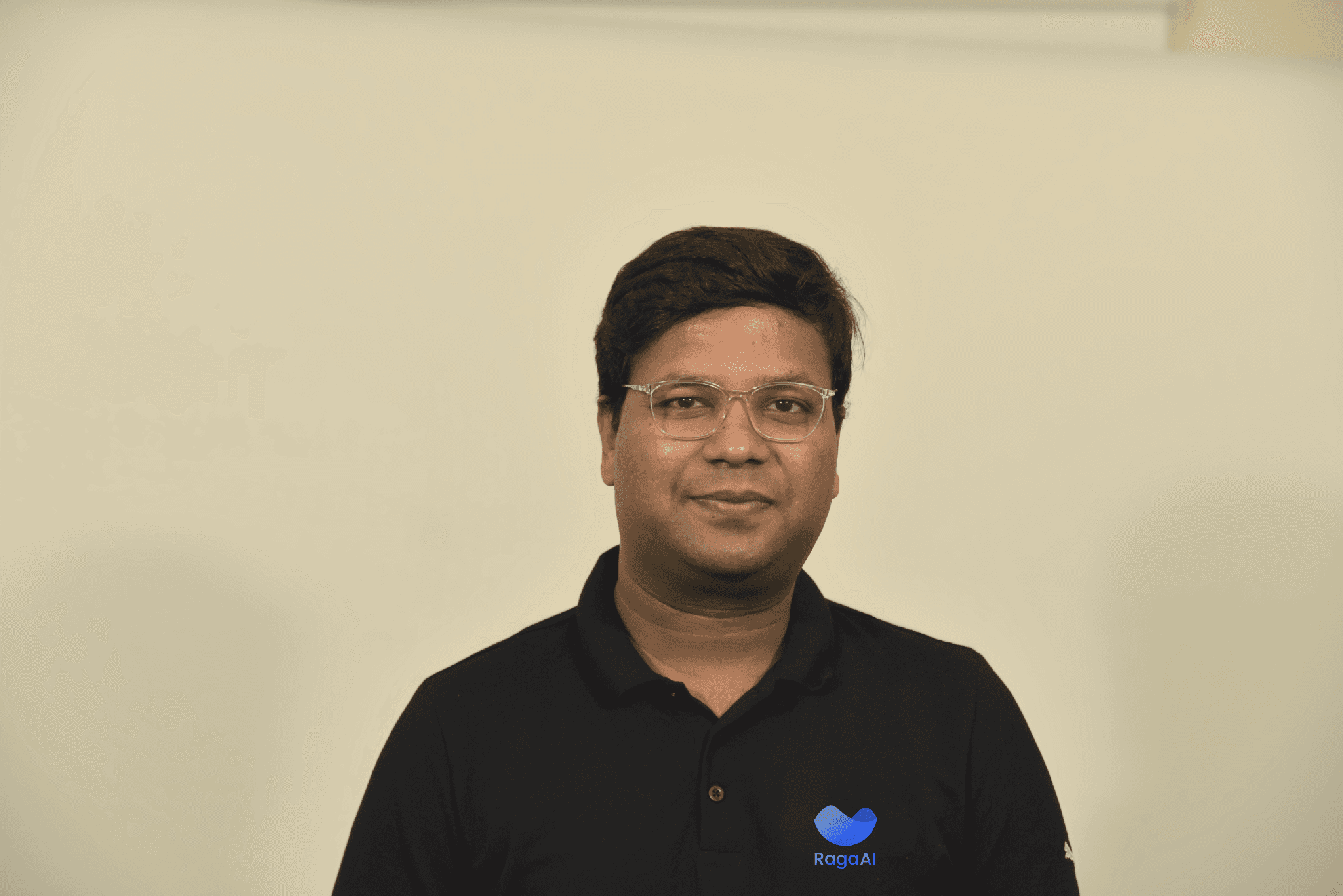
Jigar Gupta
Jun 24, 2024
Read the article




LLM Pre-Training and Fine-Tuning Differences

Rehan Asif
Jun 23, 2024
Read the article




20 LLM Project Ideas For Beginners Using Large Language Models

Rehan Asif
Jun 23, 2024
Read the article




Understanding LLM Parameters: Tuning Top-P, Temperature And Tokens

Rehan Asif
Jun 23, 2024
Read the article




Understanding Large Action Models In AI

Rehan Asif
Jun 23, 2024
Read the article




Building And Implementing Custom LLM Guardrails

Rehan Asif
Jun 12, 2024
Read the article




Understanding LLM Alignment: A Simple Guide

Rehan Asif
Jun 12, 2024
Read the article
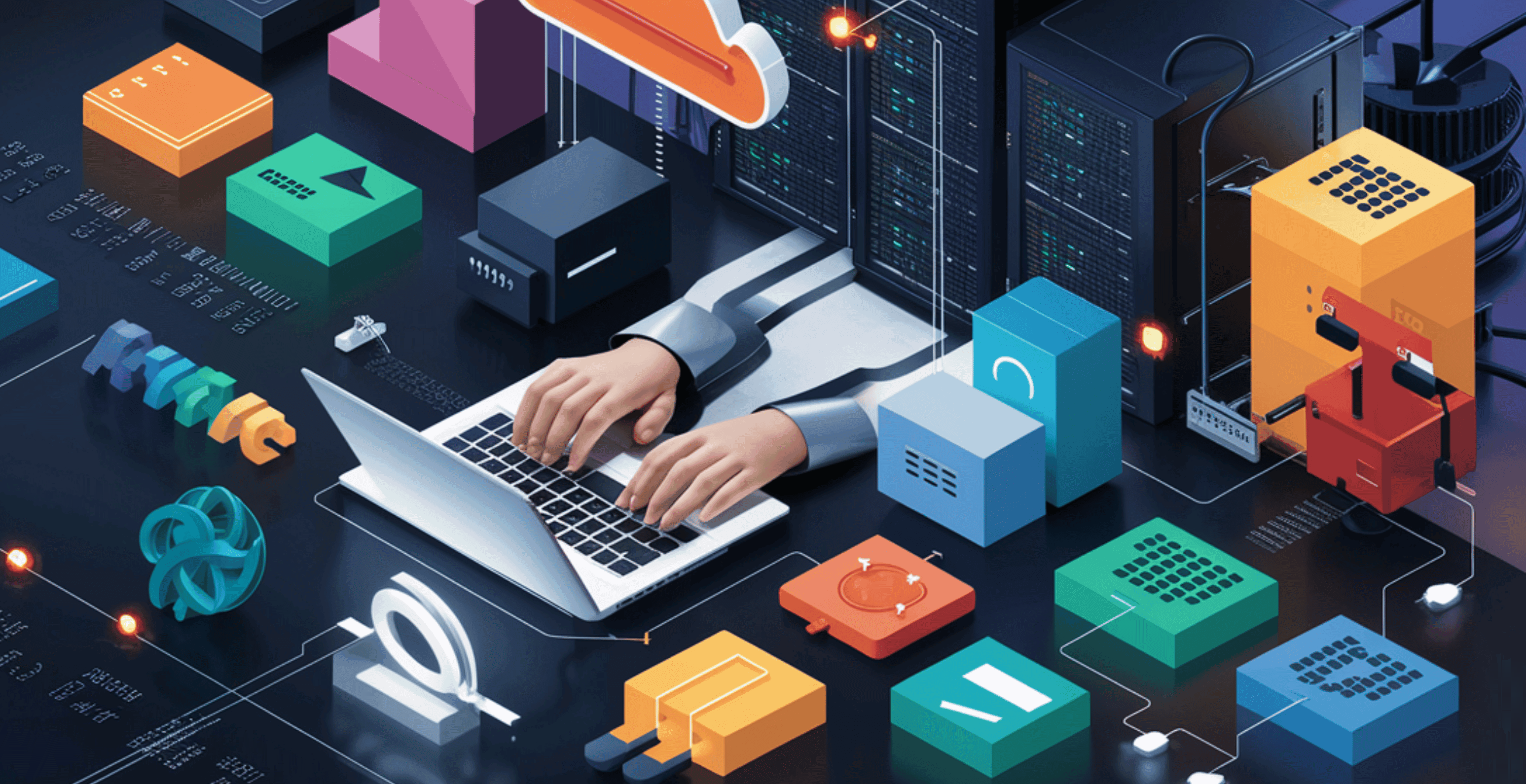
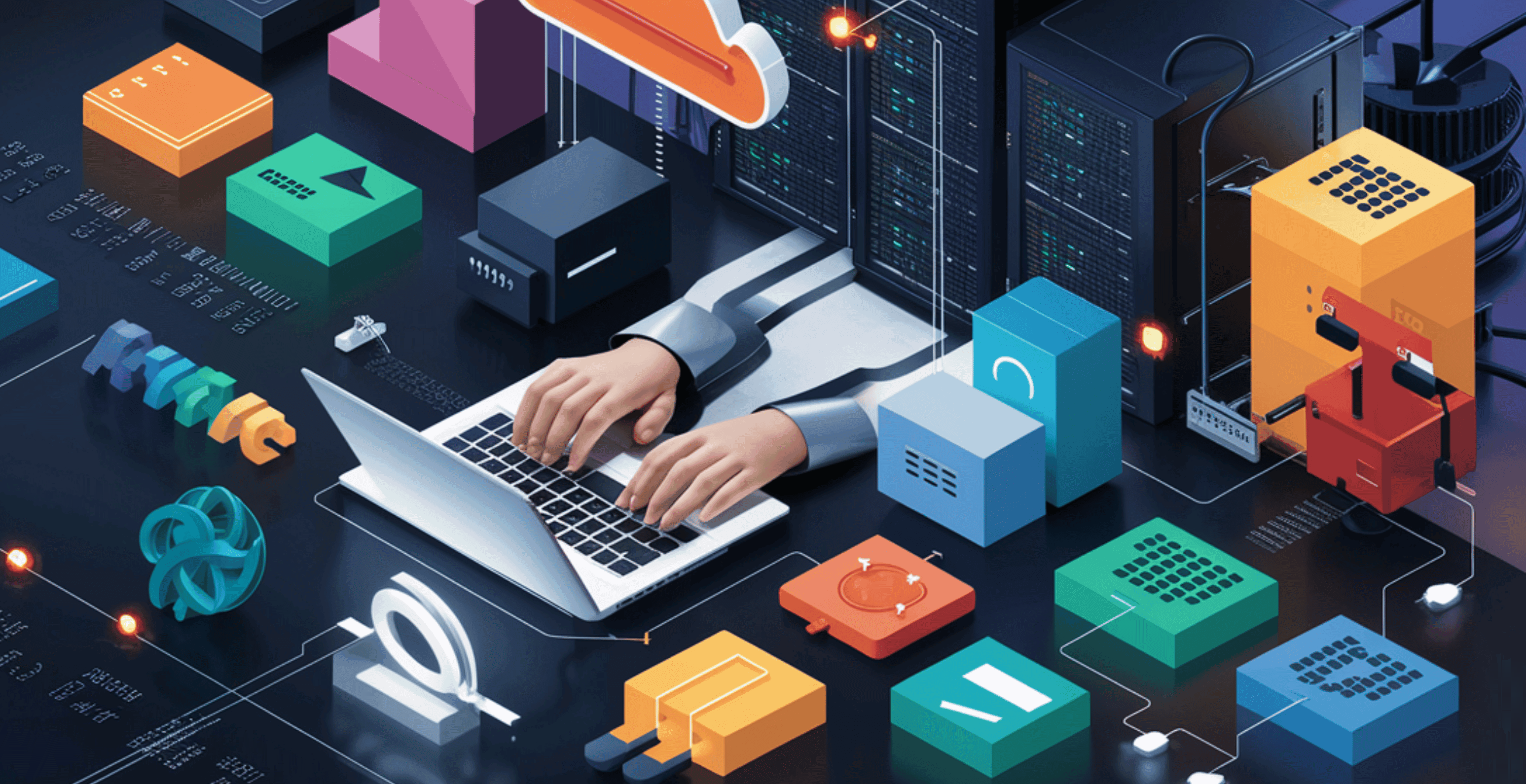
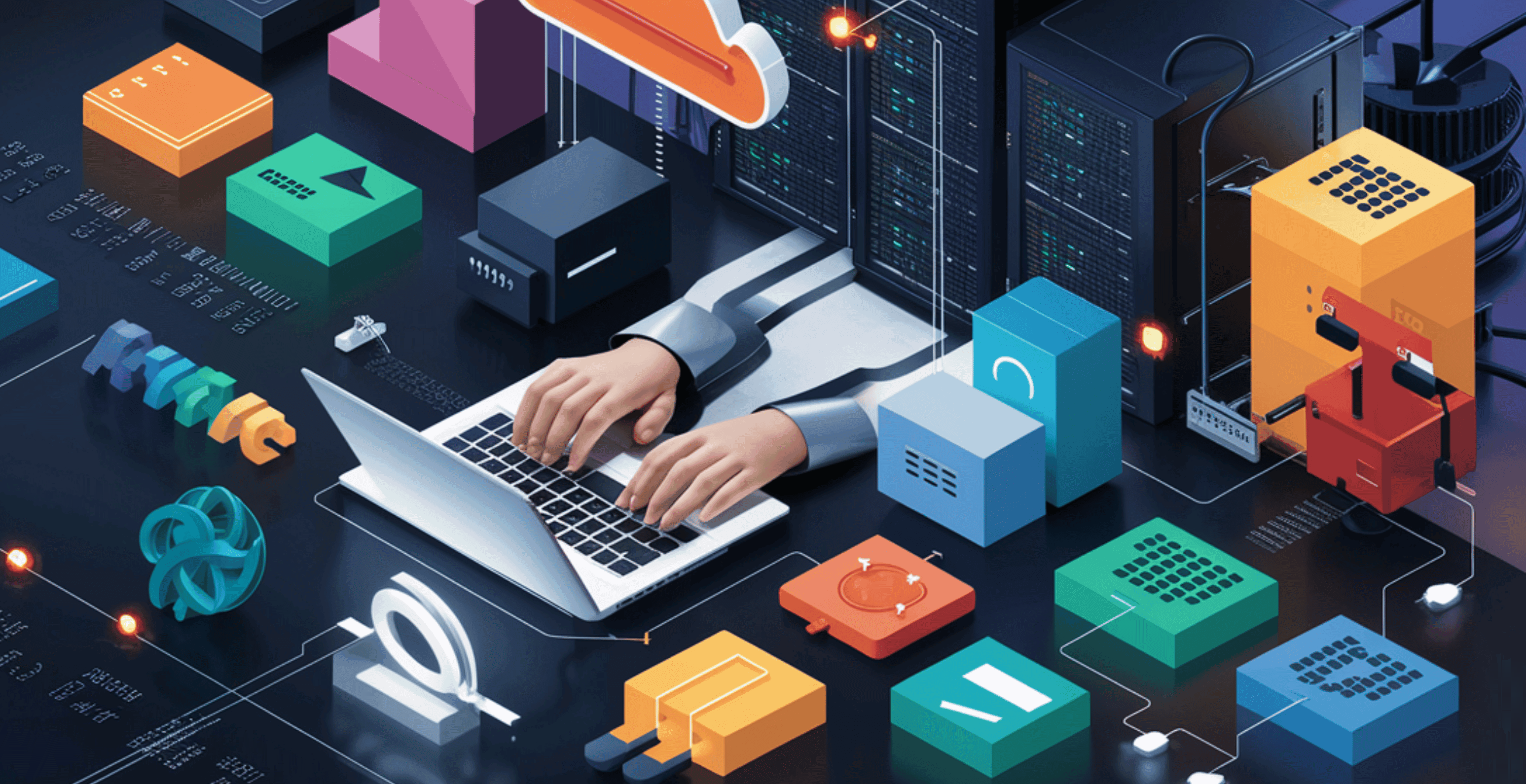
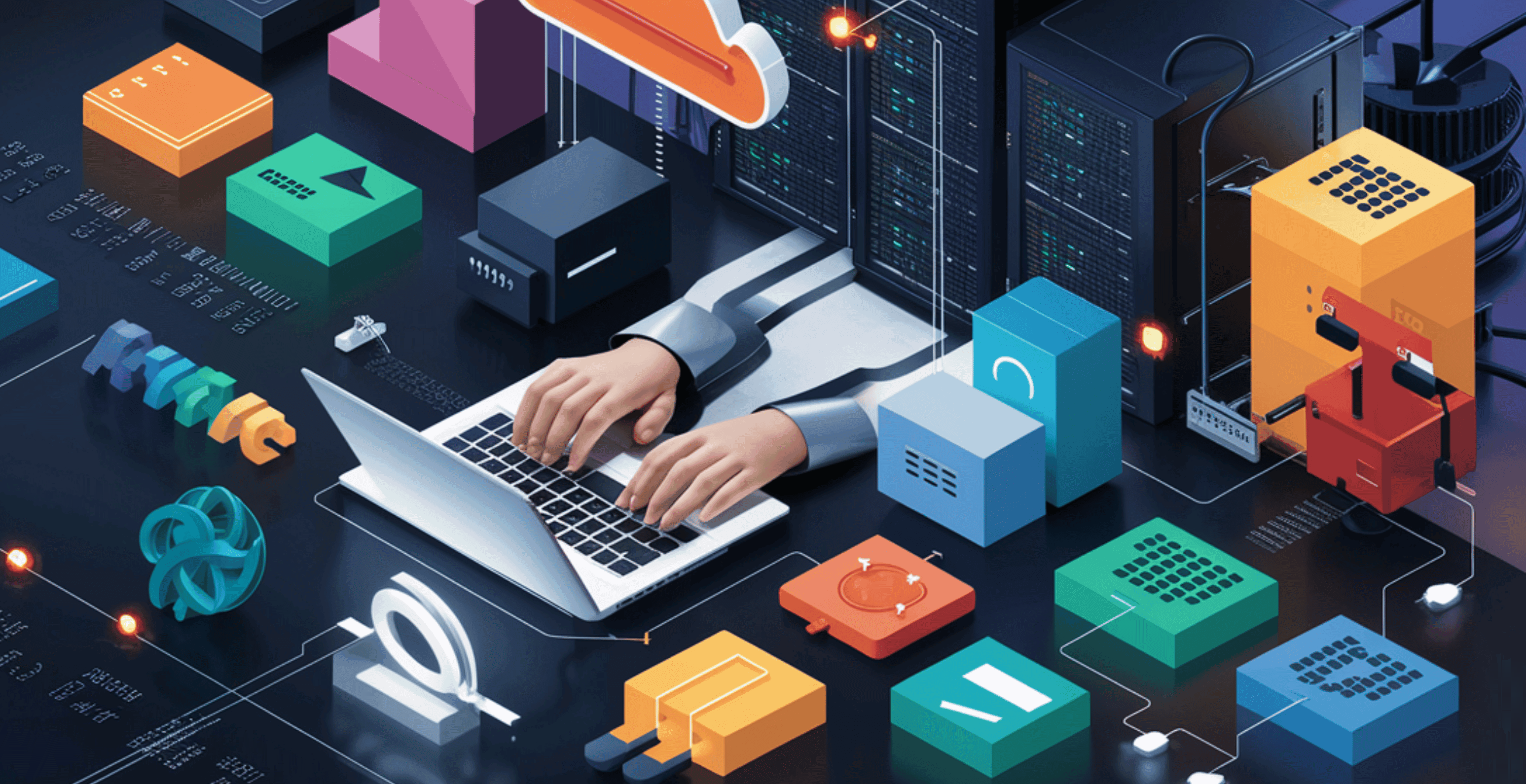
Practical Strategies For Self-Hosting Large Language Models

Rehan Asif
Jun 12, 2024
Read the article
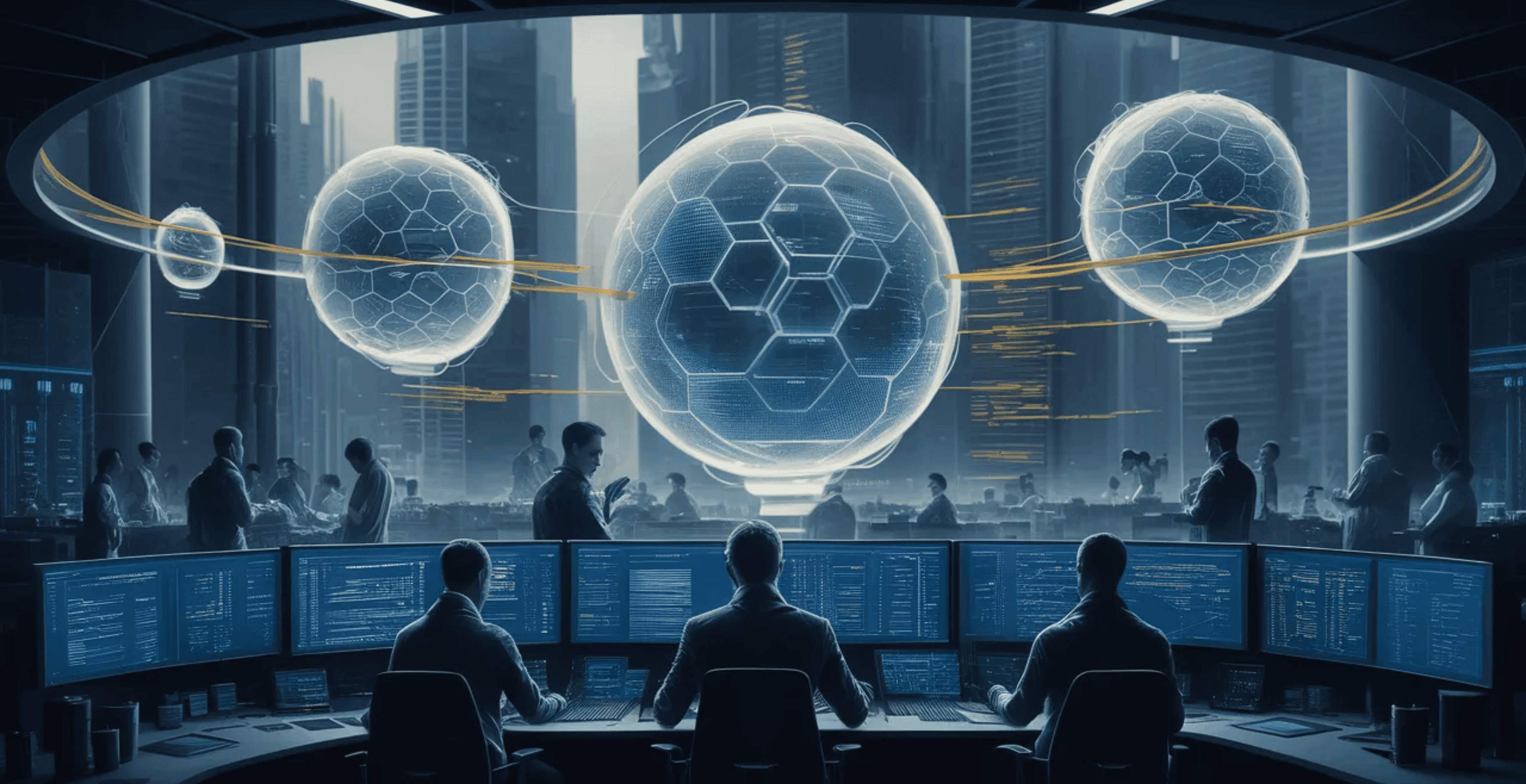
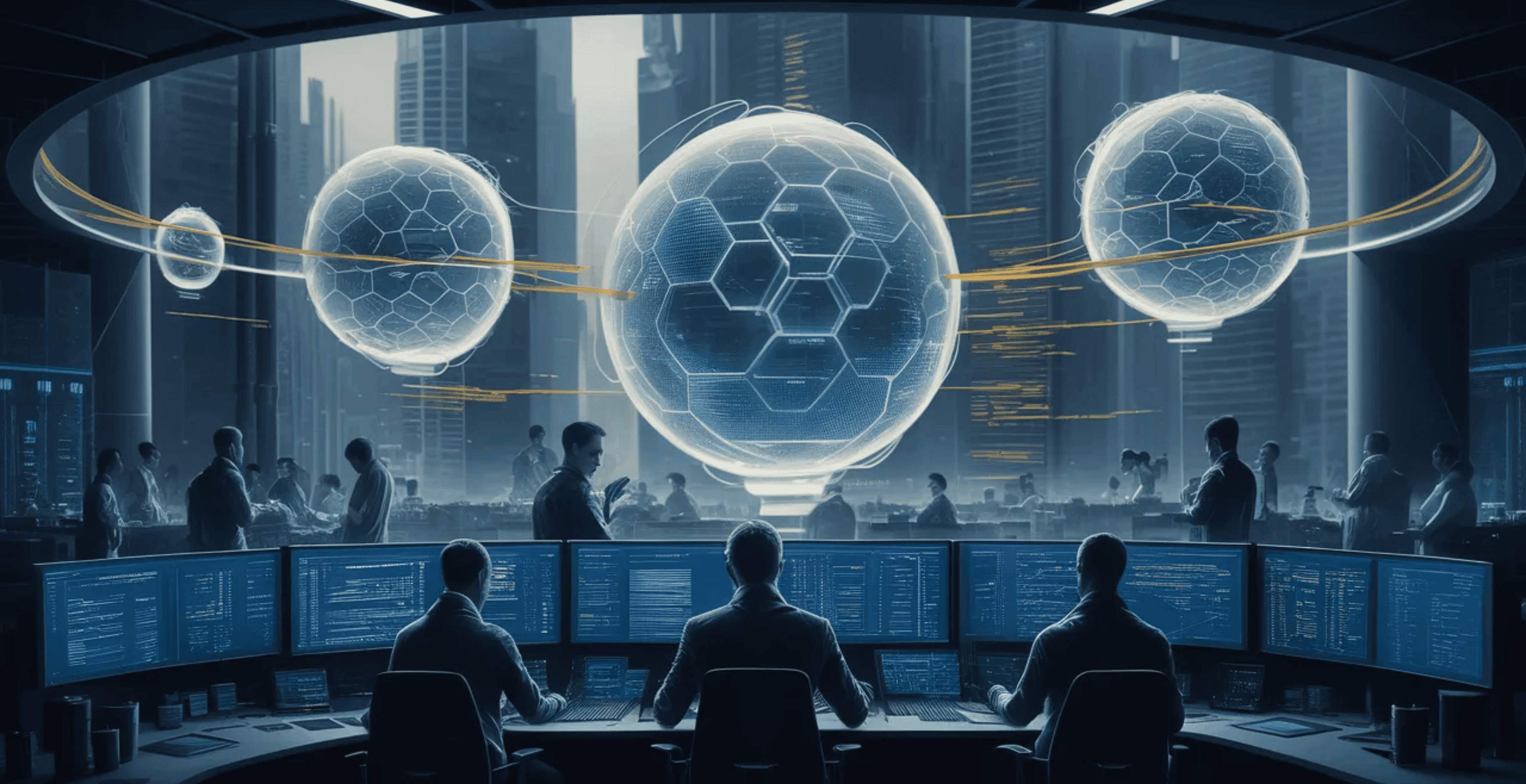
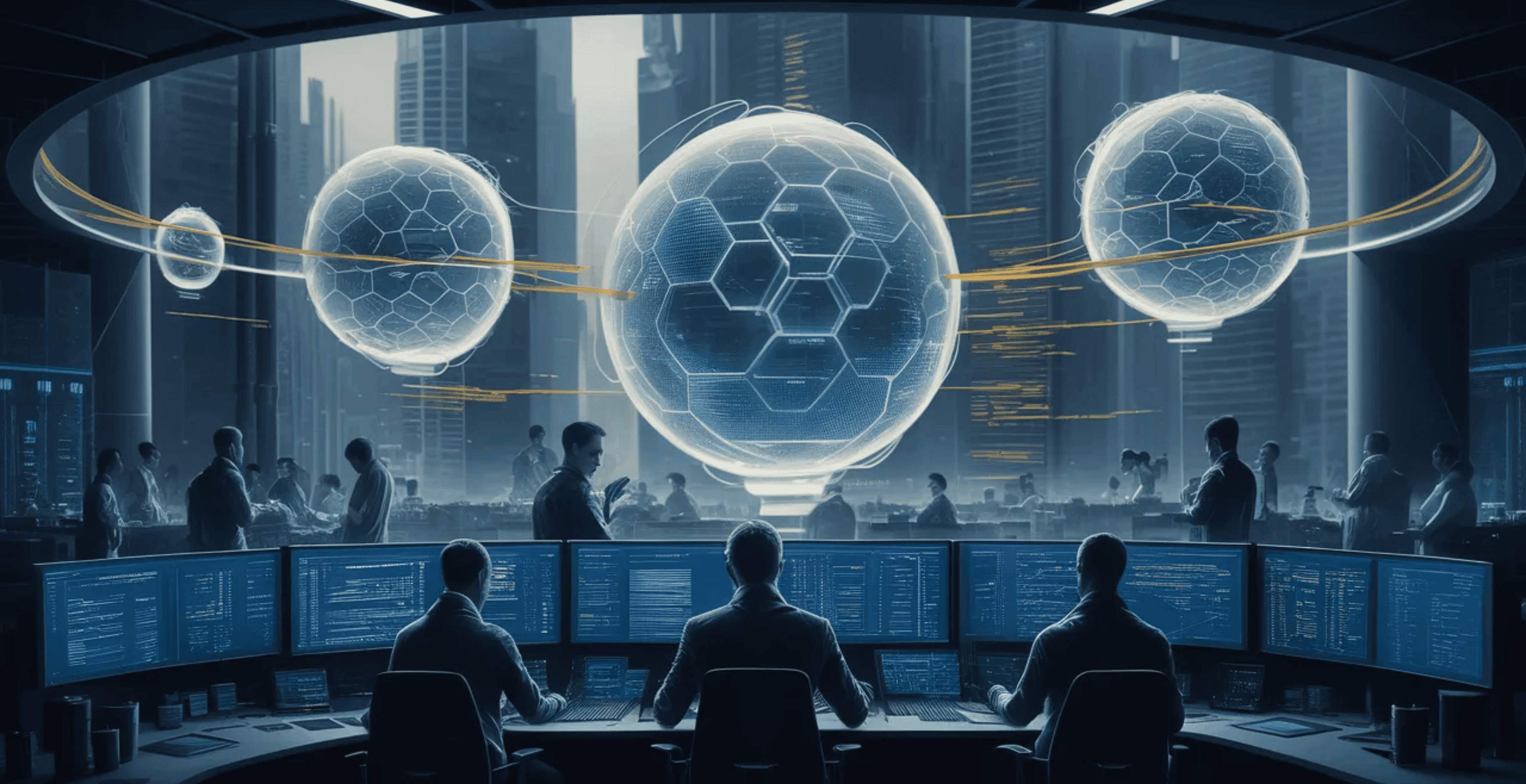
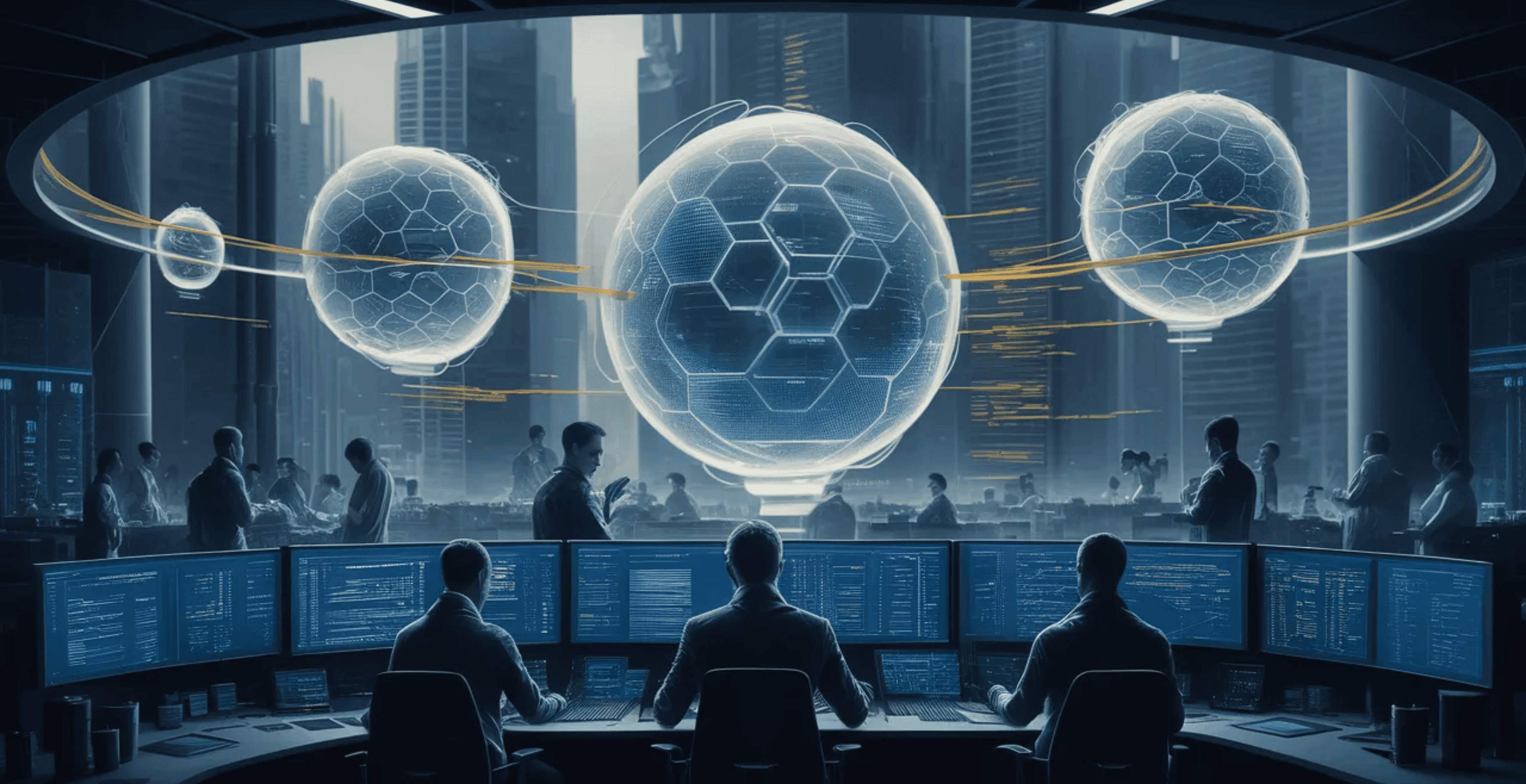
Practical Guide For Deploying LLMs In Production

Rehan Asif
Jun 12, 2024
Read the article
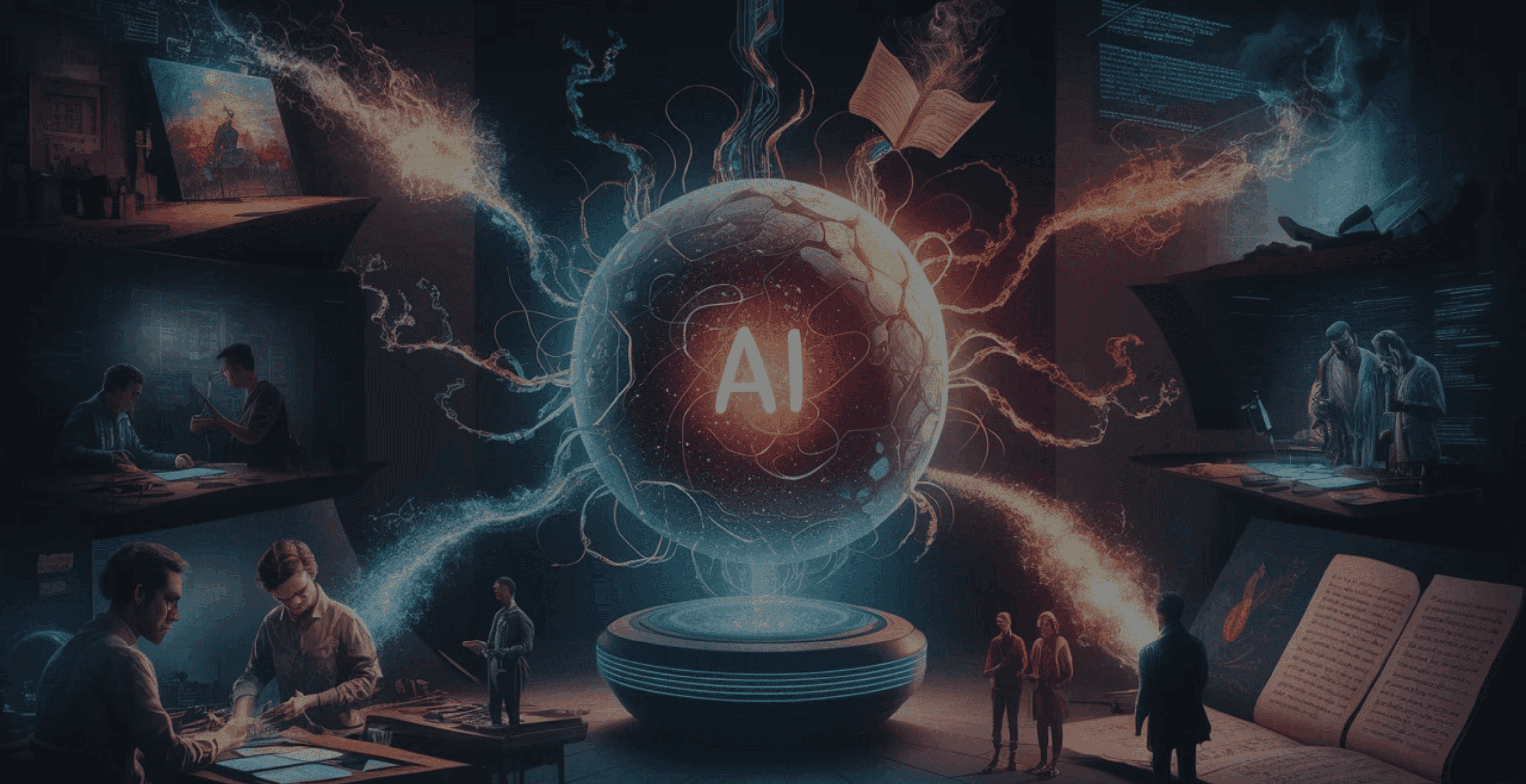
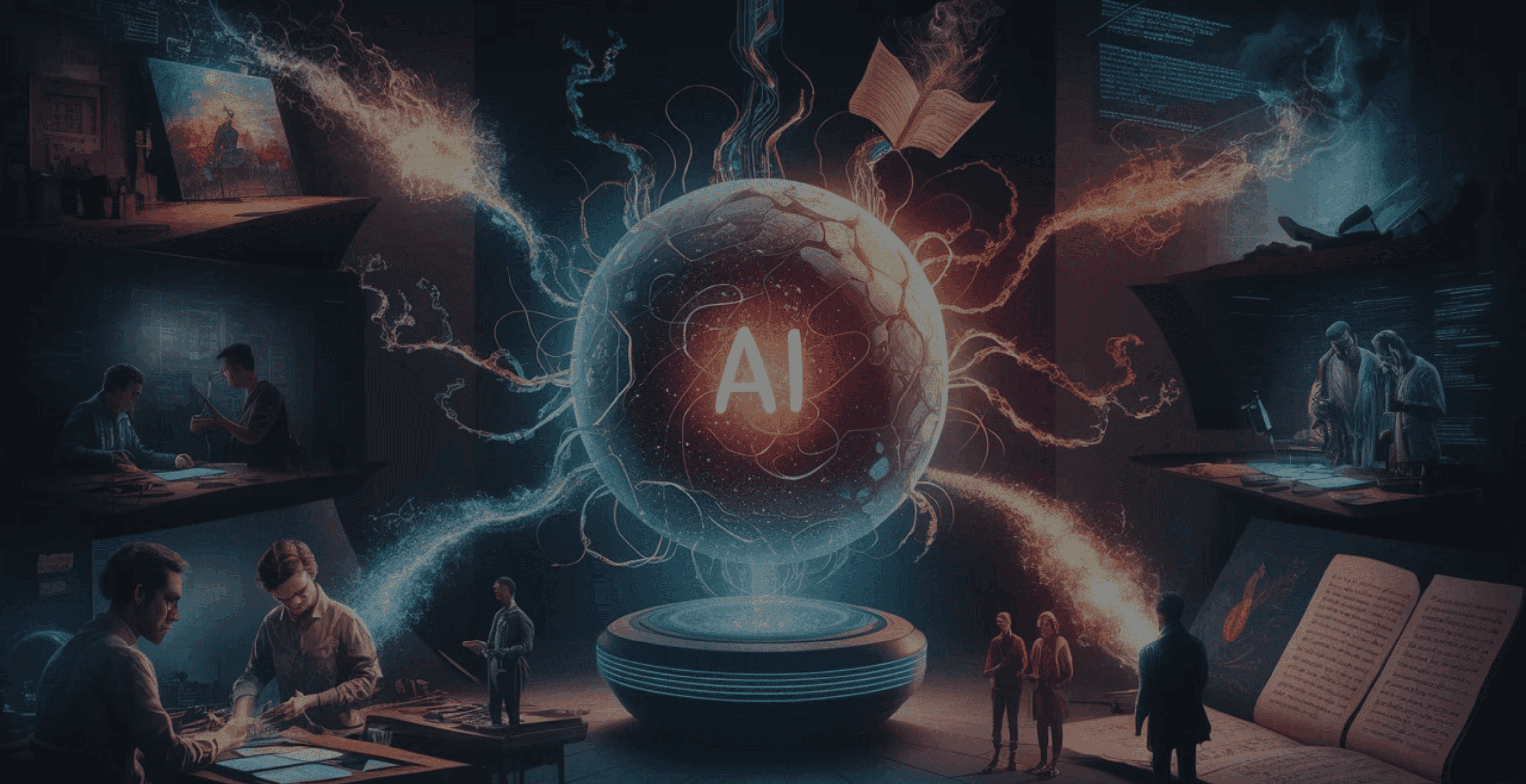
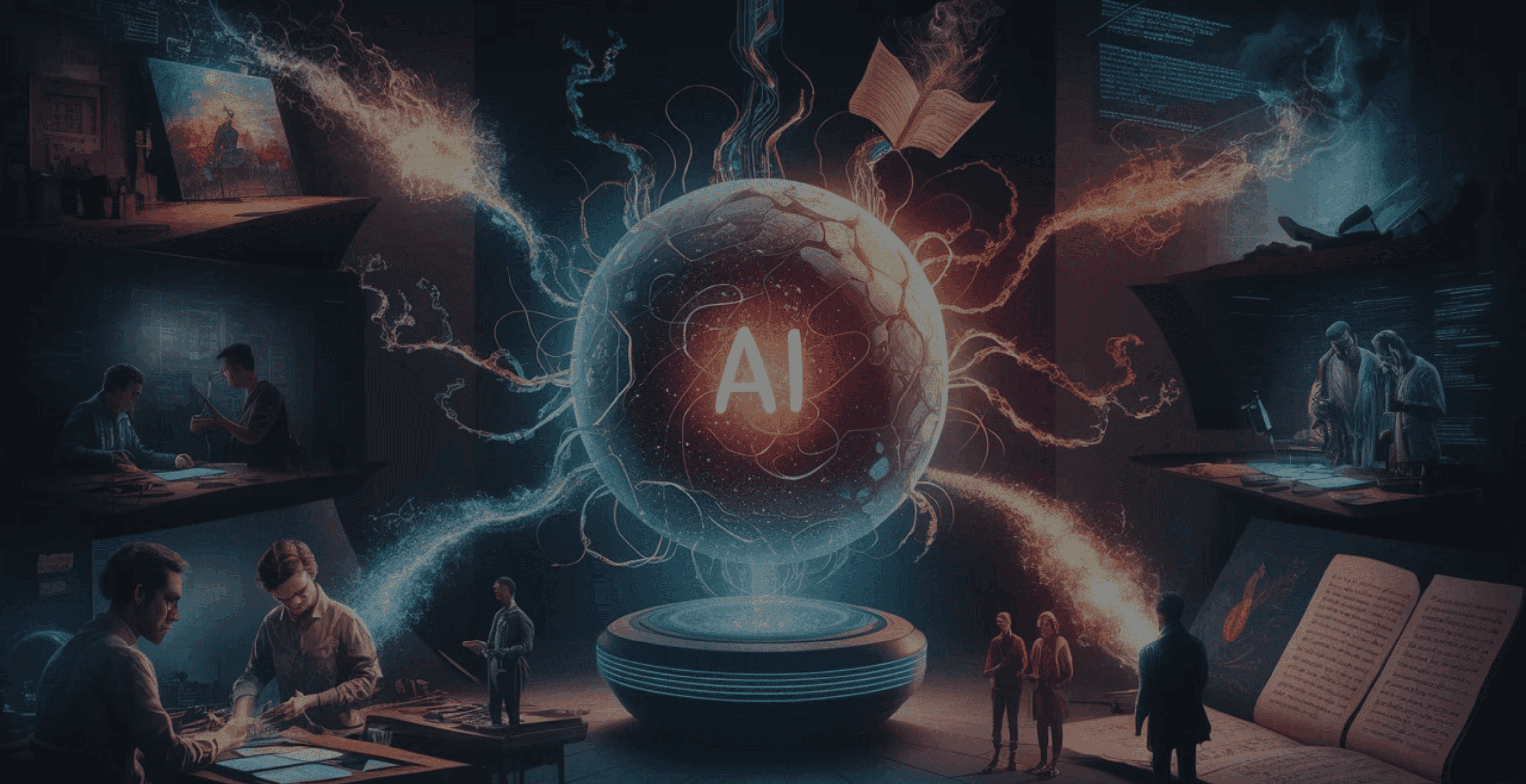
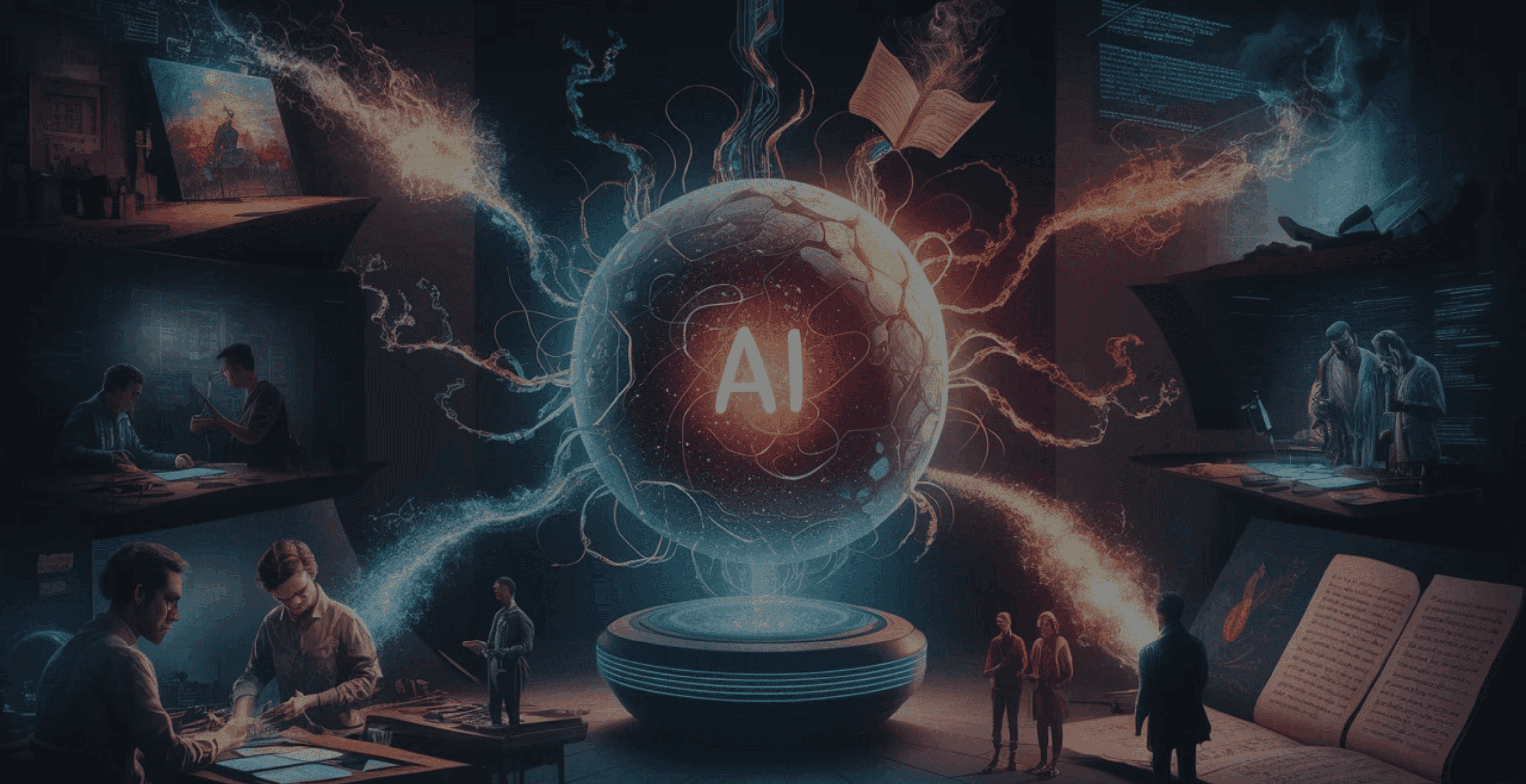
The Impact Of Generative Models On Content Creation
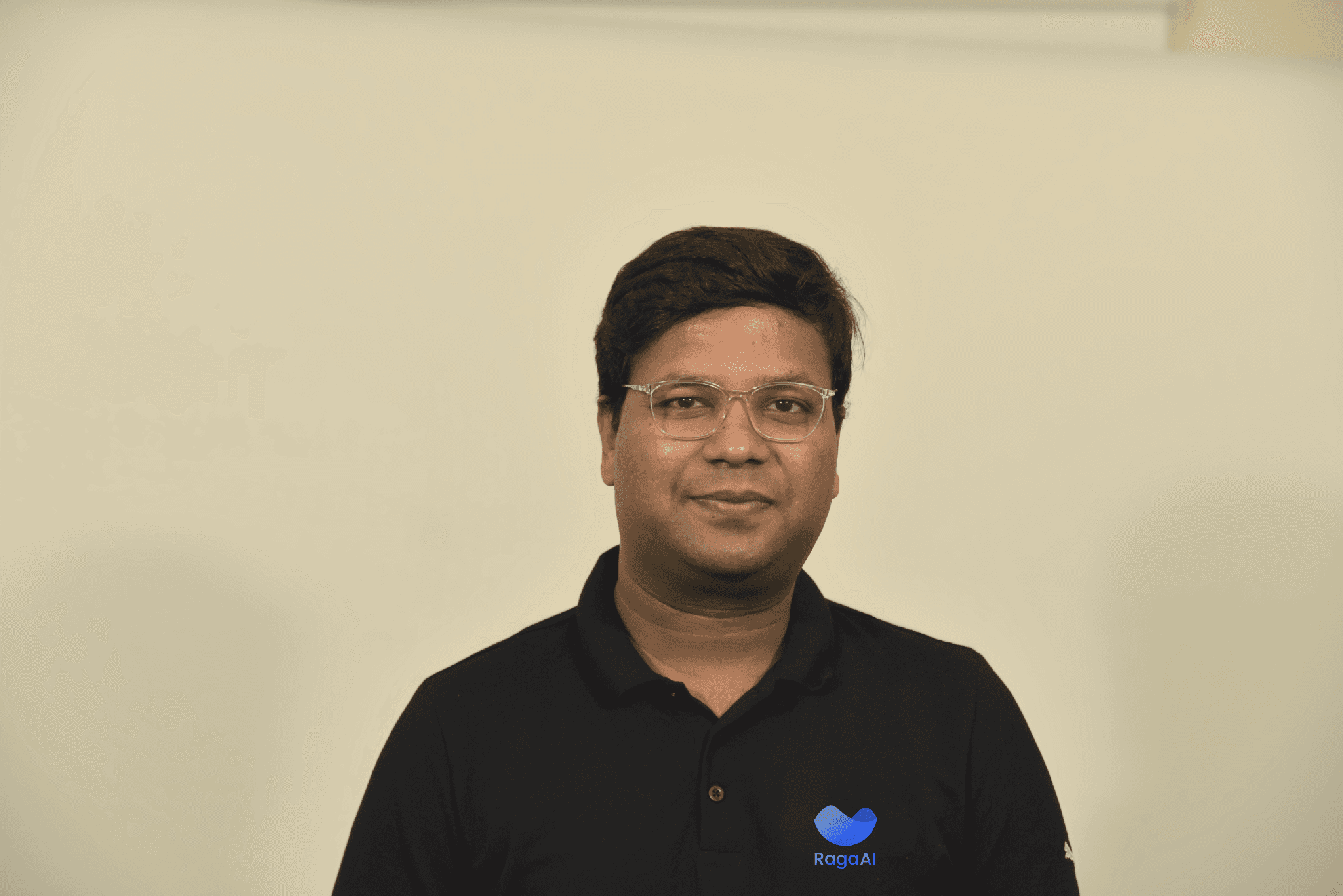
Jigar Gupta
Jun 12, 2024
Read the article




Implementing Regression Tests In AI Development
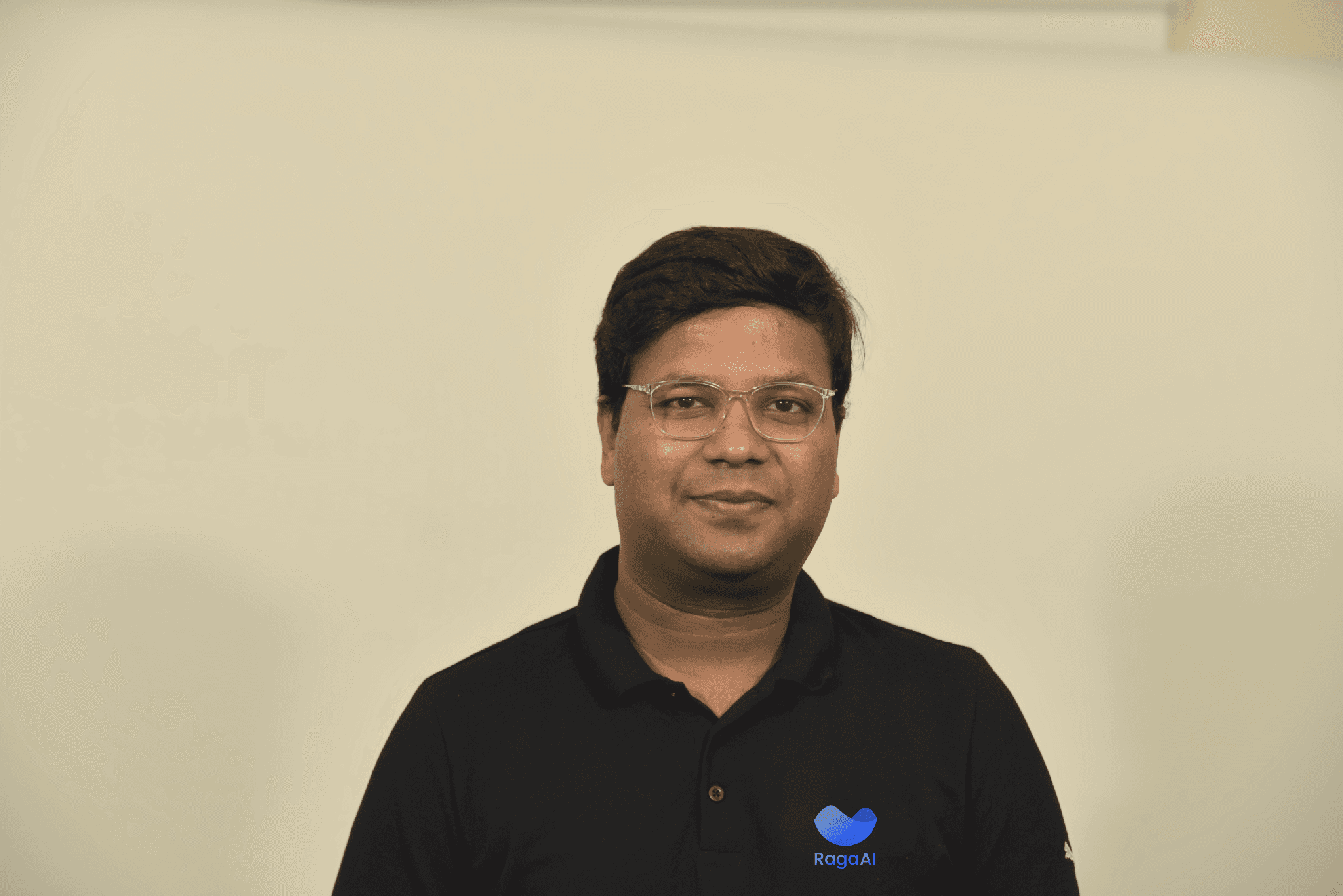
Jigar Gupta
Jun 12, 2024
Read the article
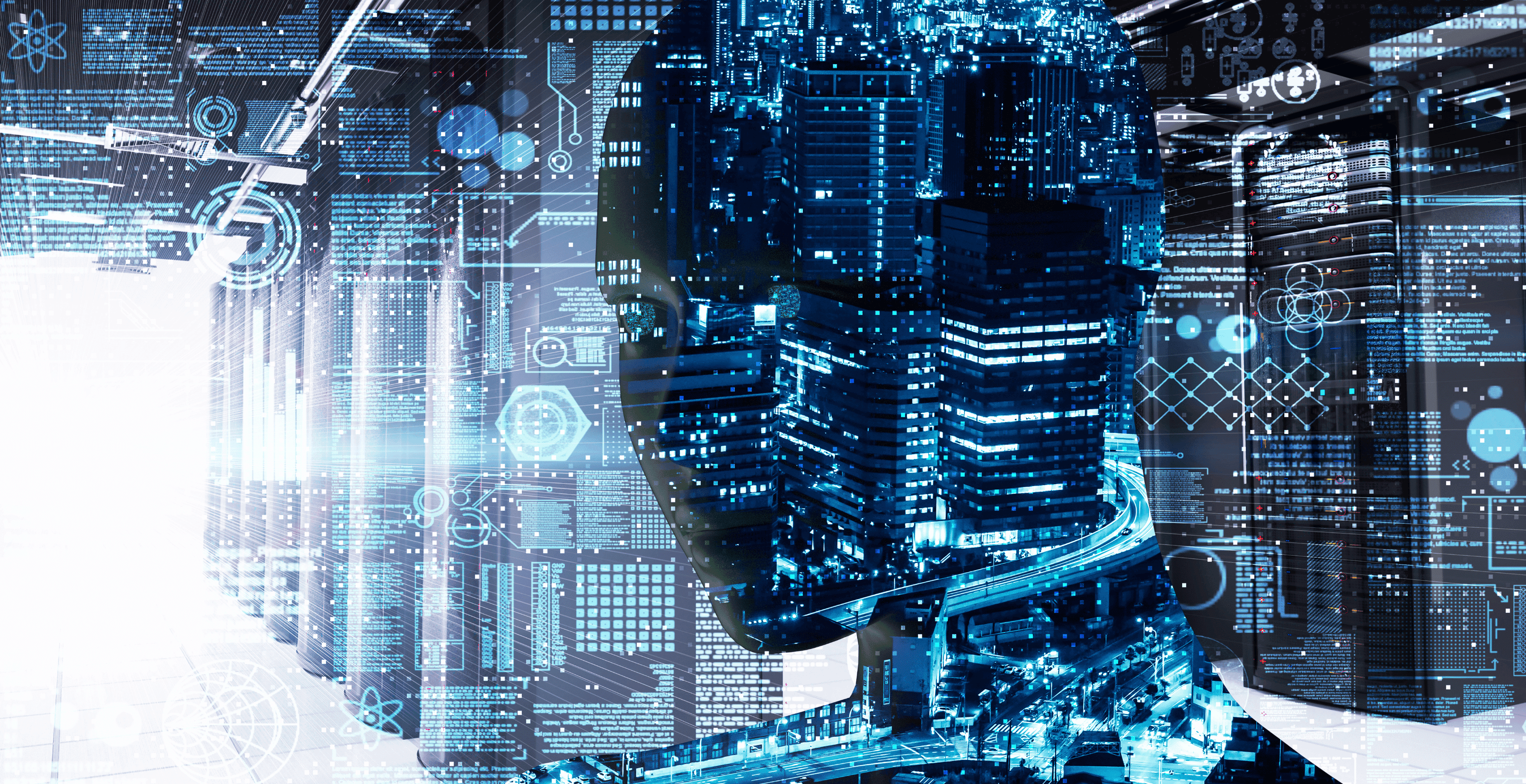
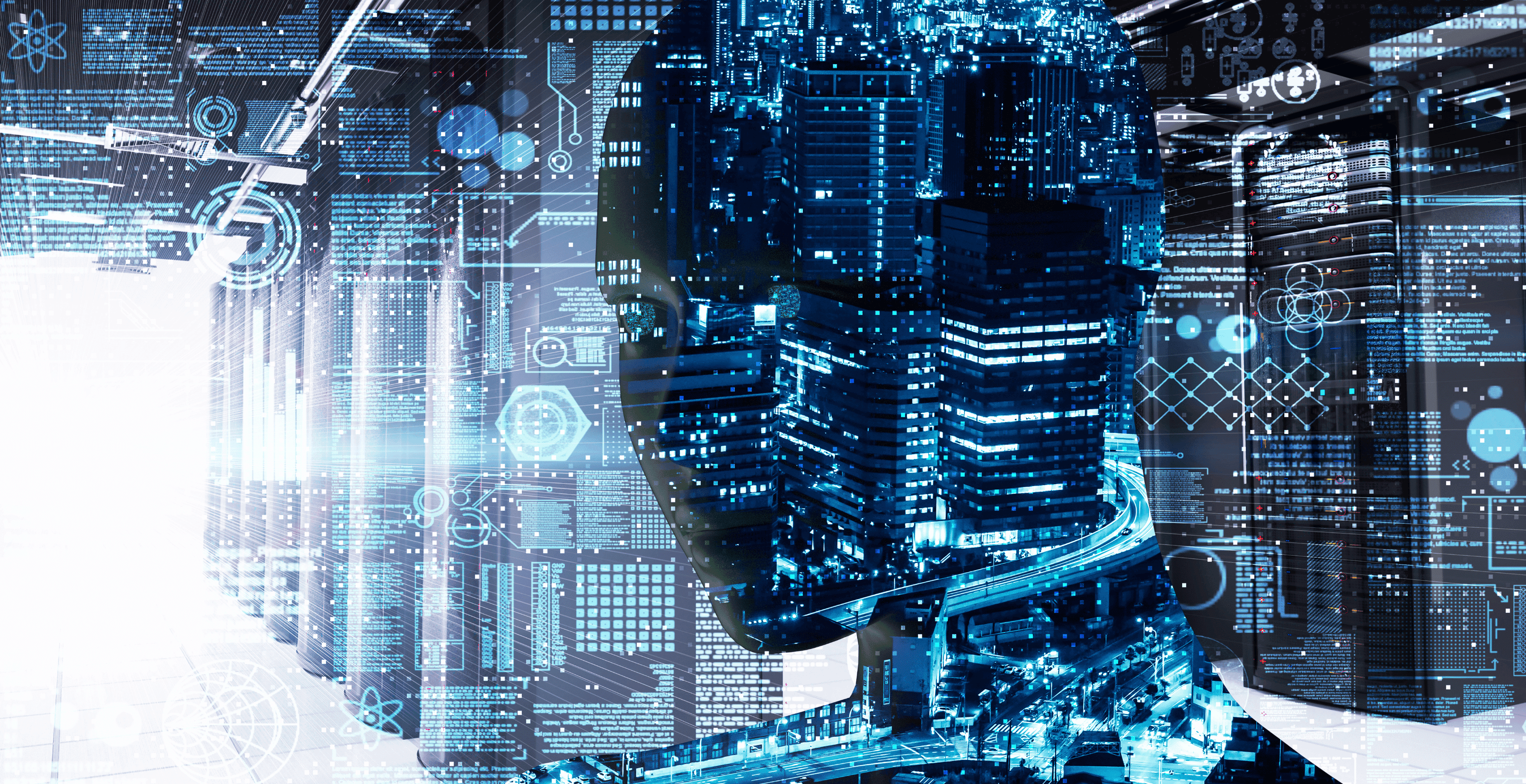
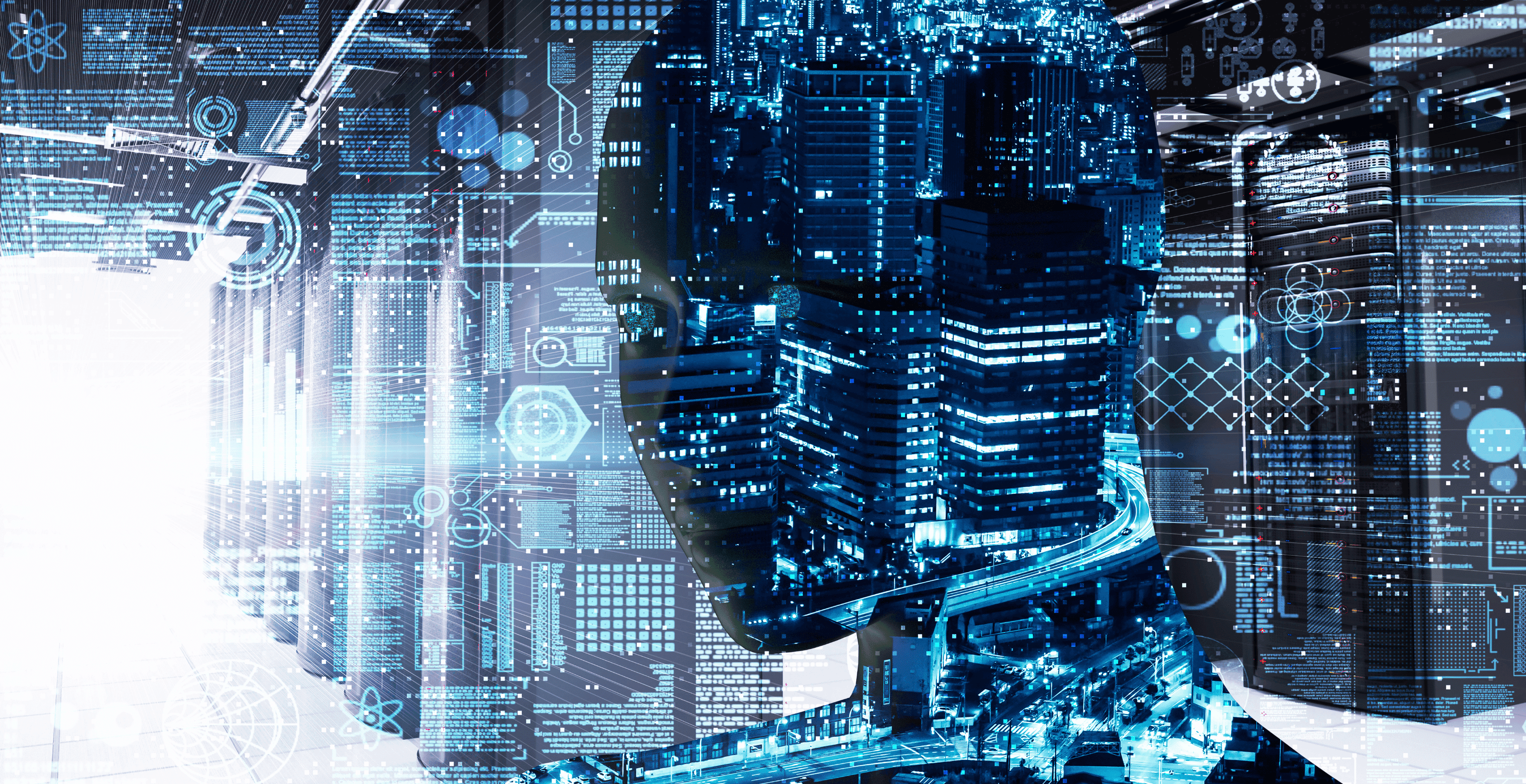
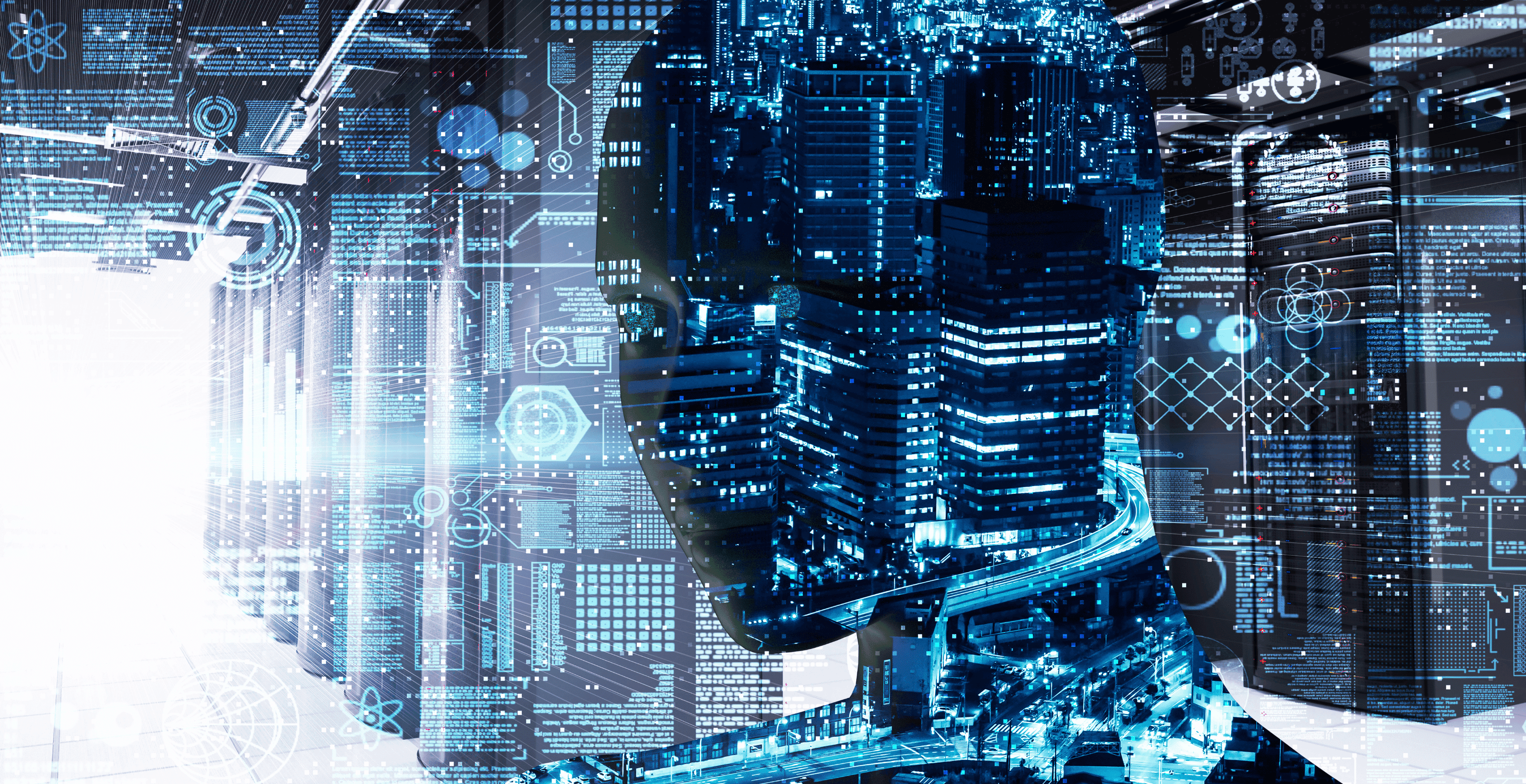
In-Depth Case Studies in AI Model Testing: Exploring Real-World Applications and Insights
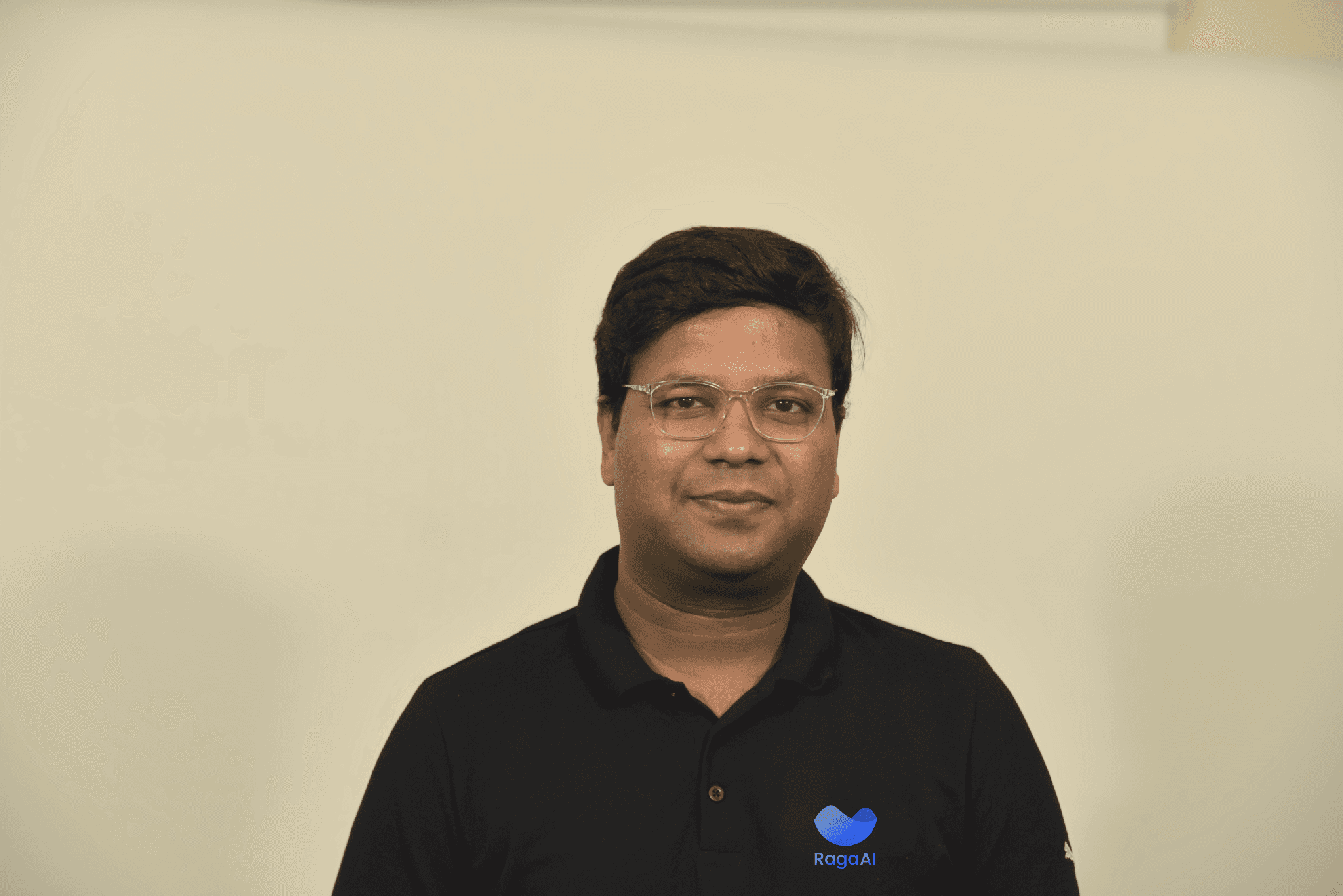
Jigar Gupta
Jun 11, 2024
Read the article




Techniques and Importance of Stress Testing AI Systems
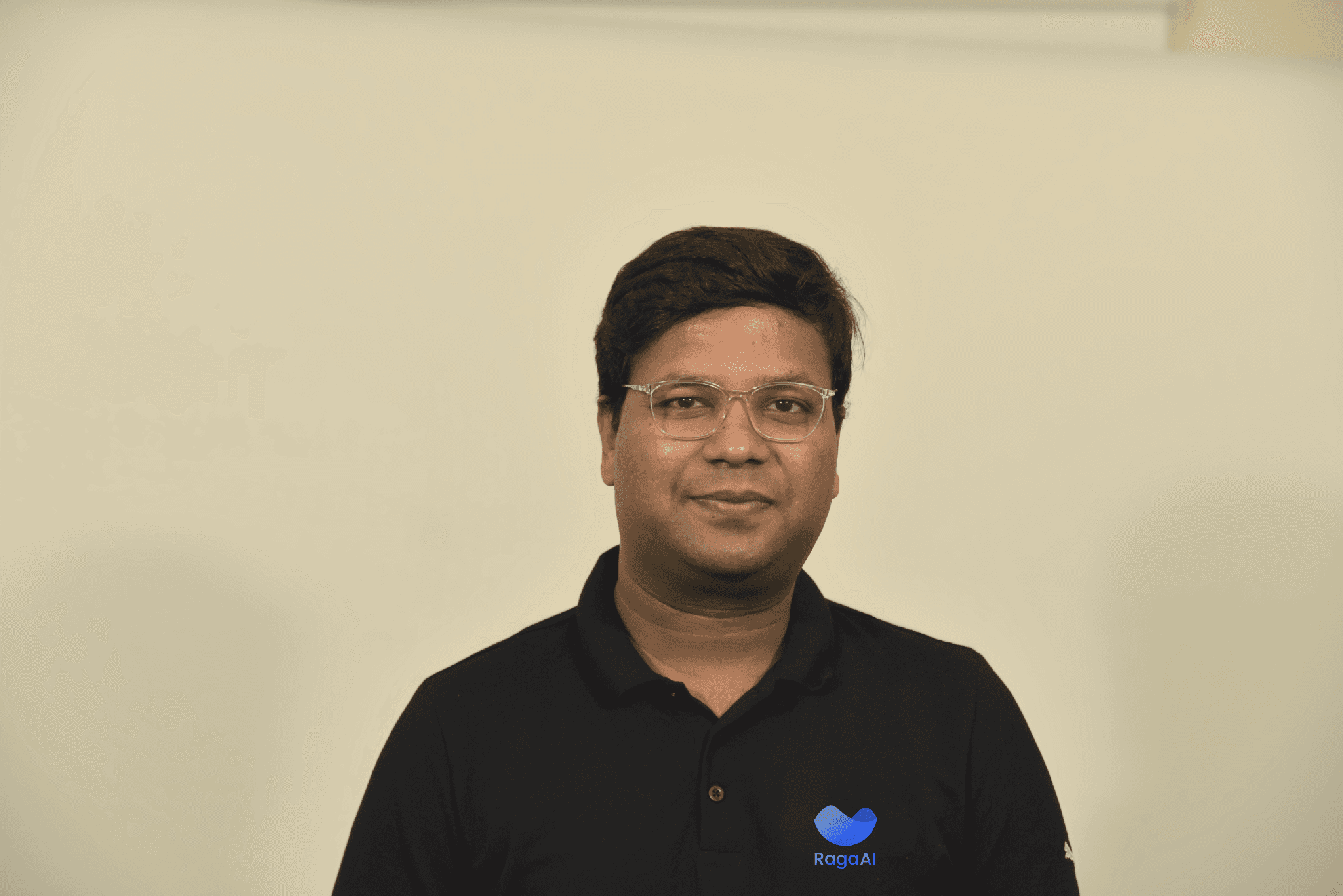
Jigar Gupta
Jun 11, 2024
Read the article
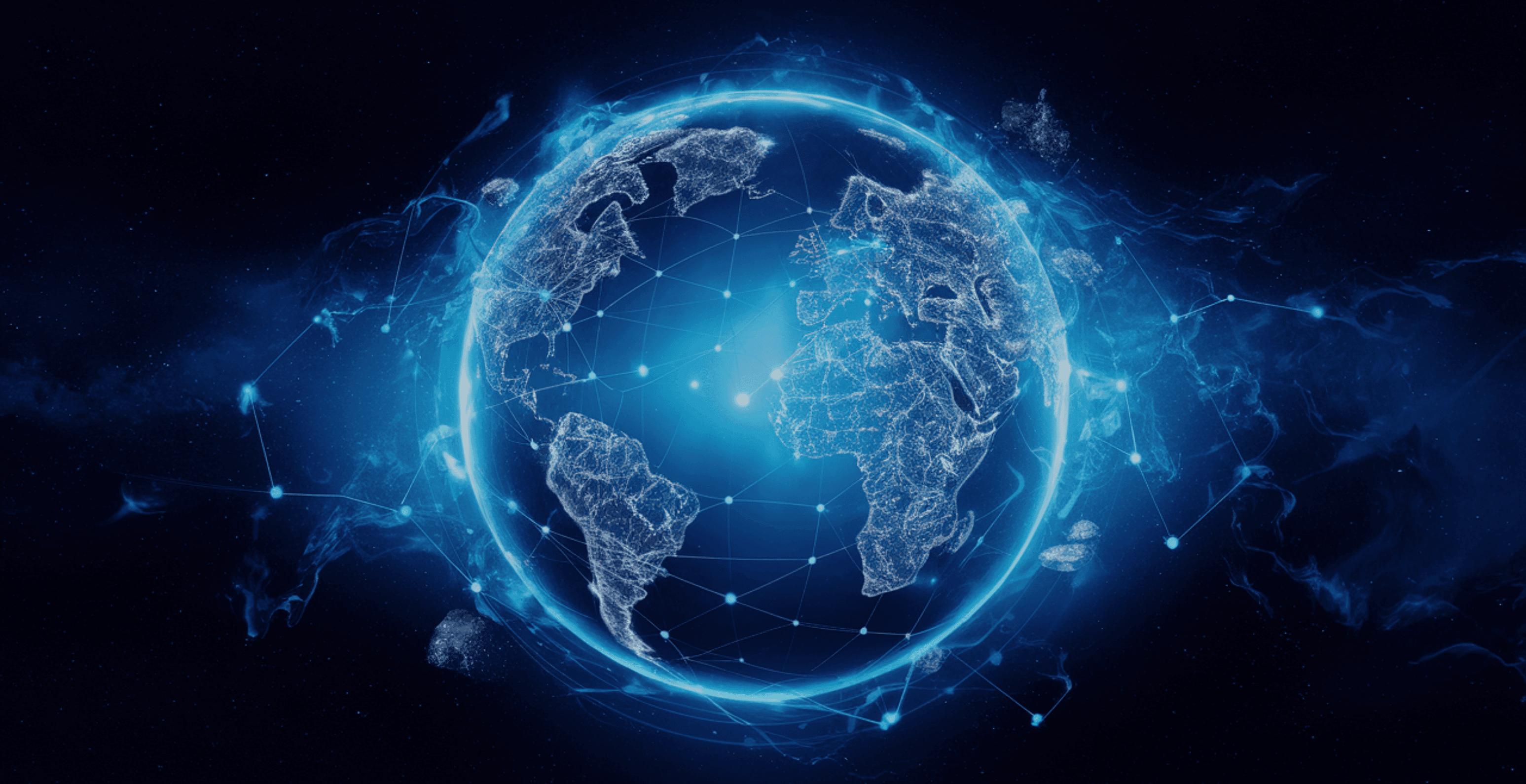
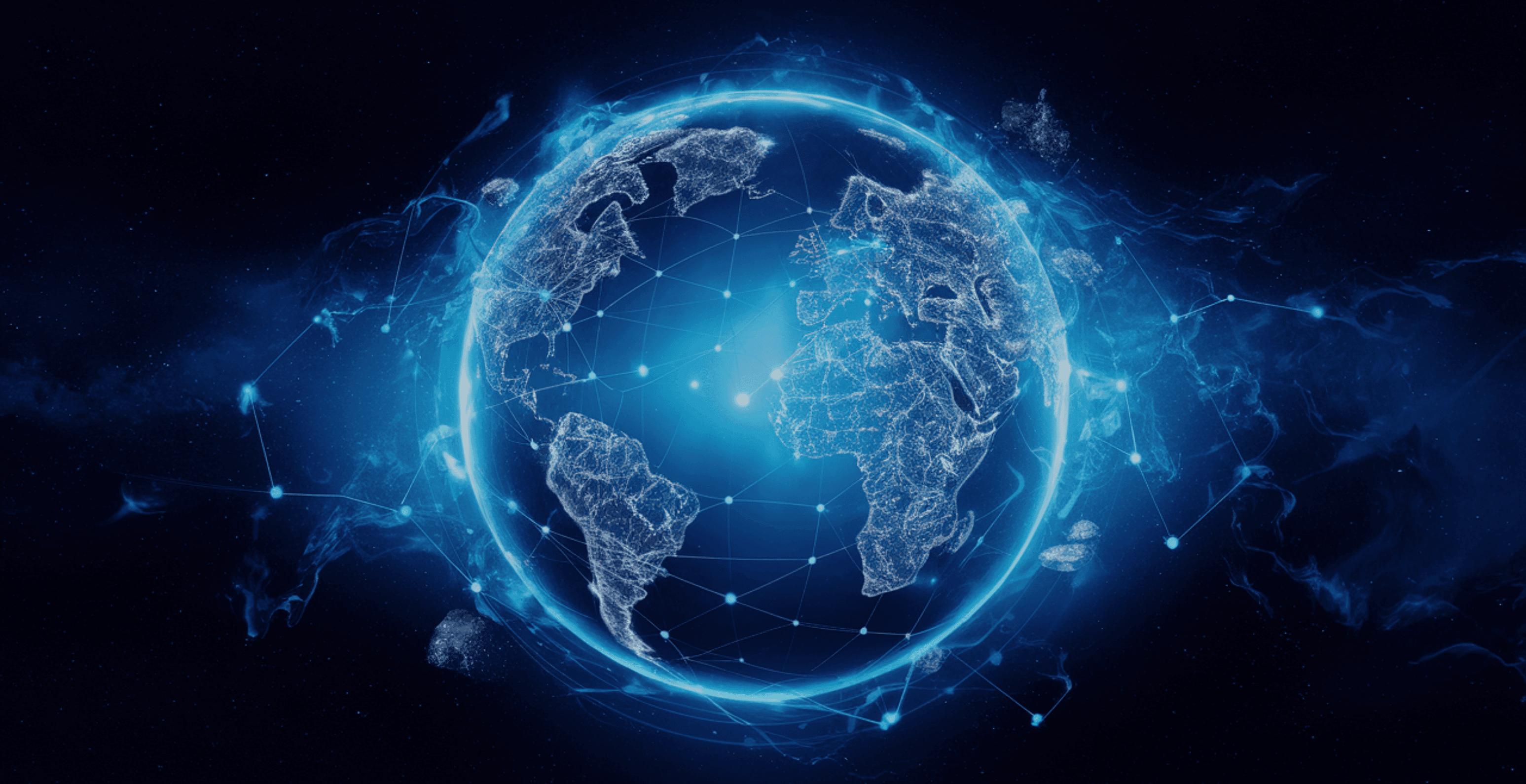
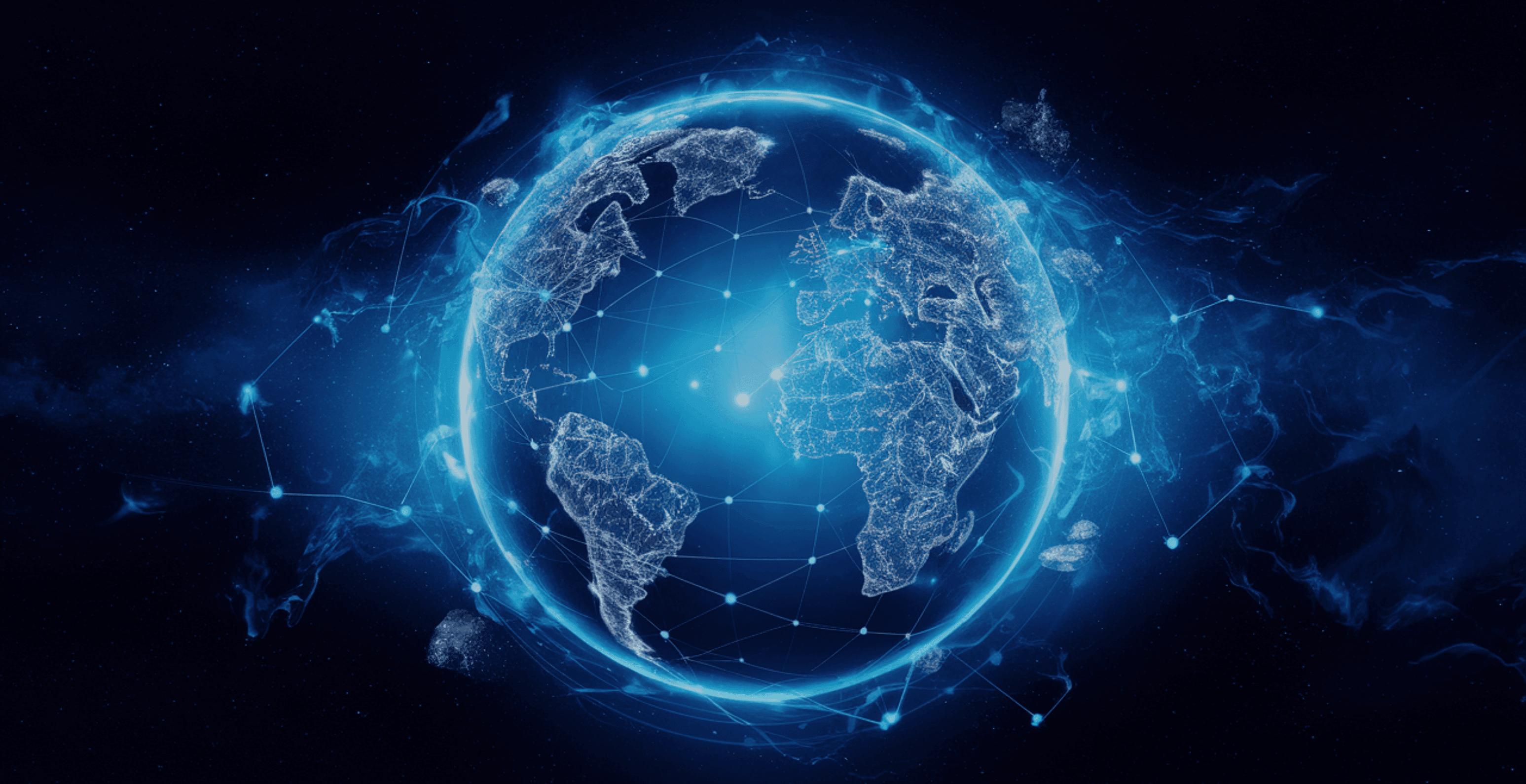
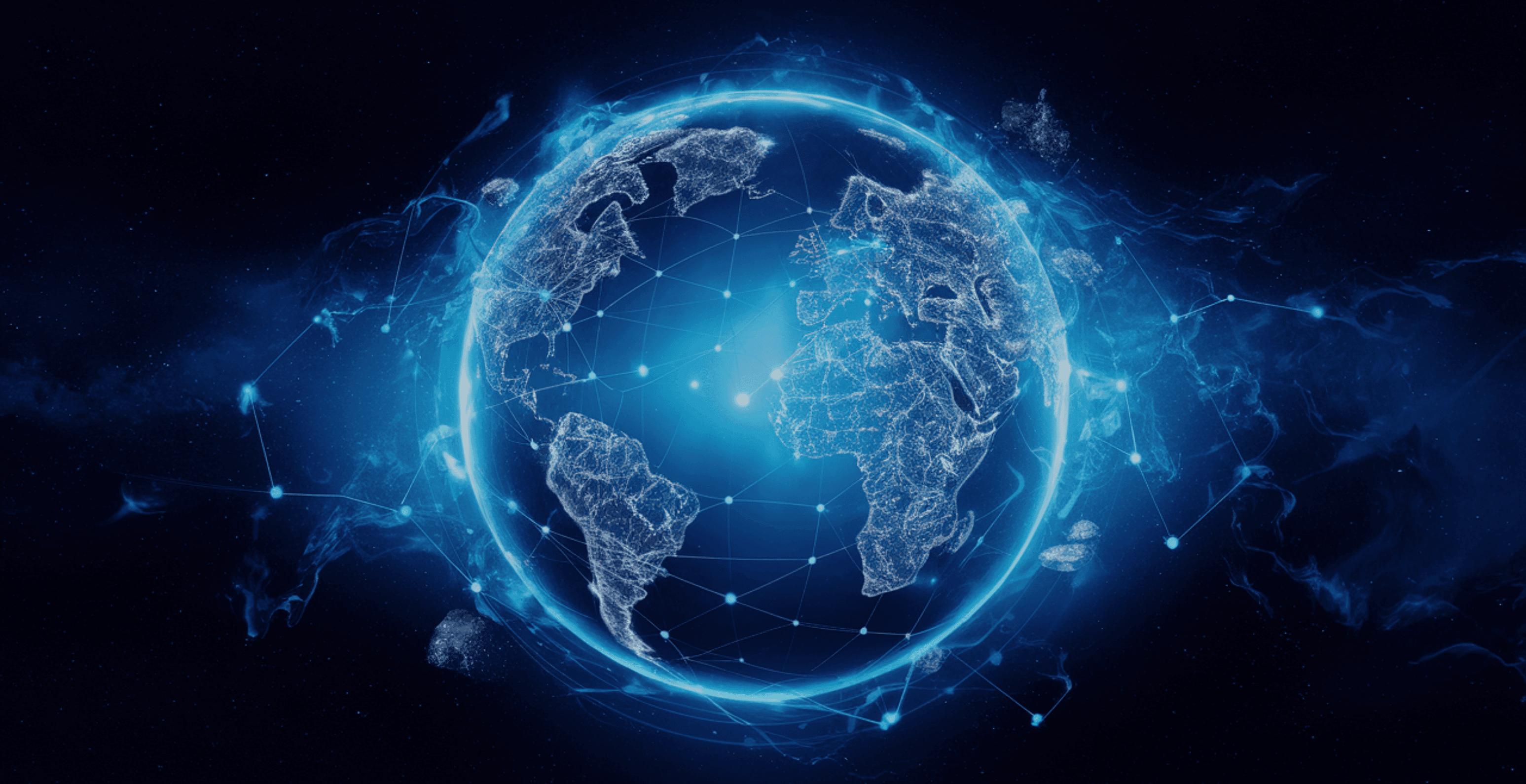
Navigating Global AI Regulations and Standards

Rehan Asif
Jun 10, 2024
Read the article




The Cost of Errors In AI Application Development

Rehan Asif
Jun 10, 2024
Read the article
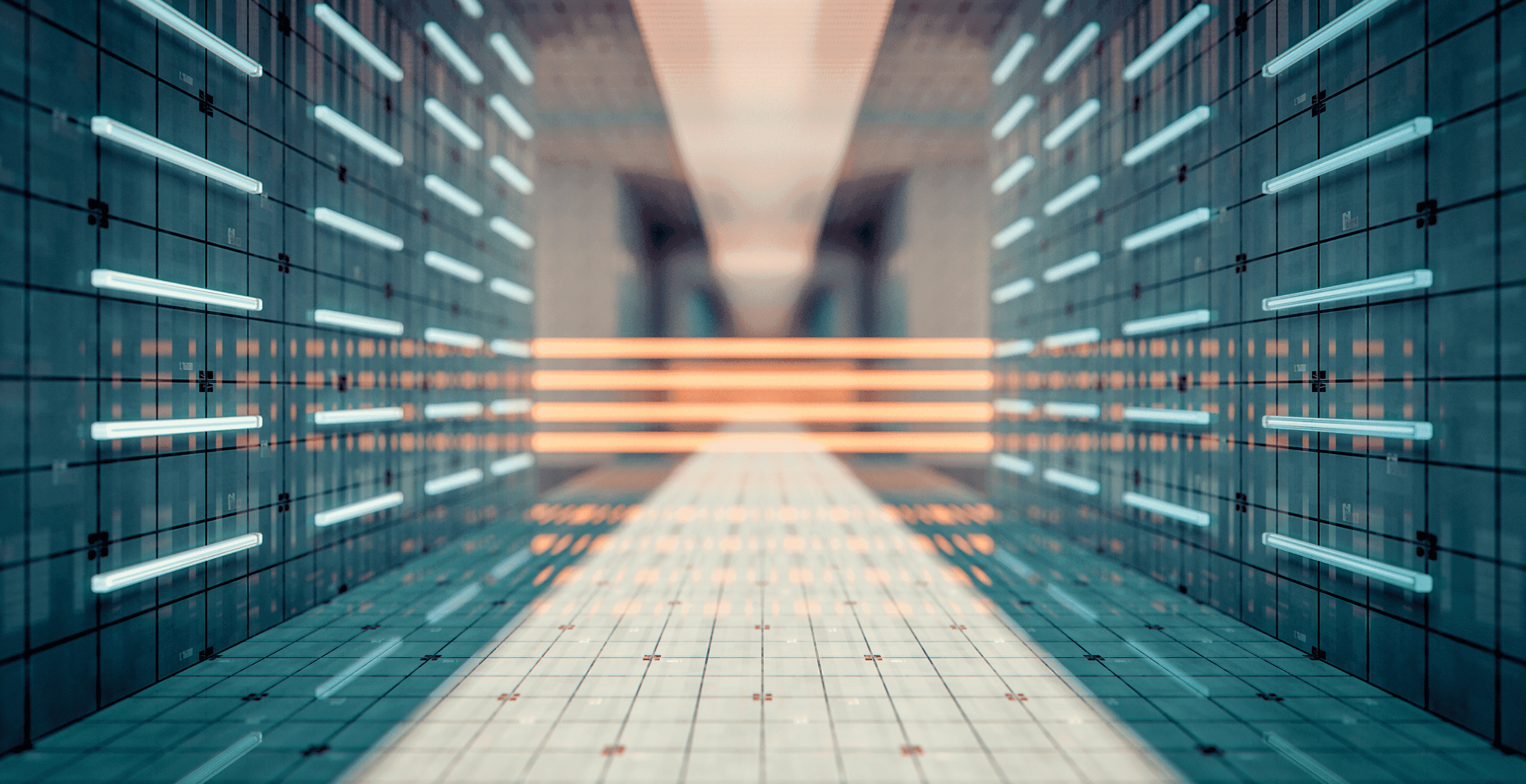
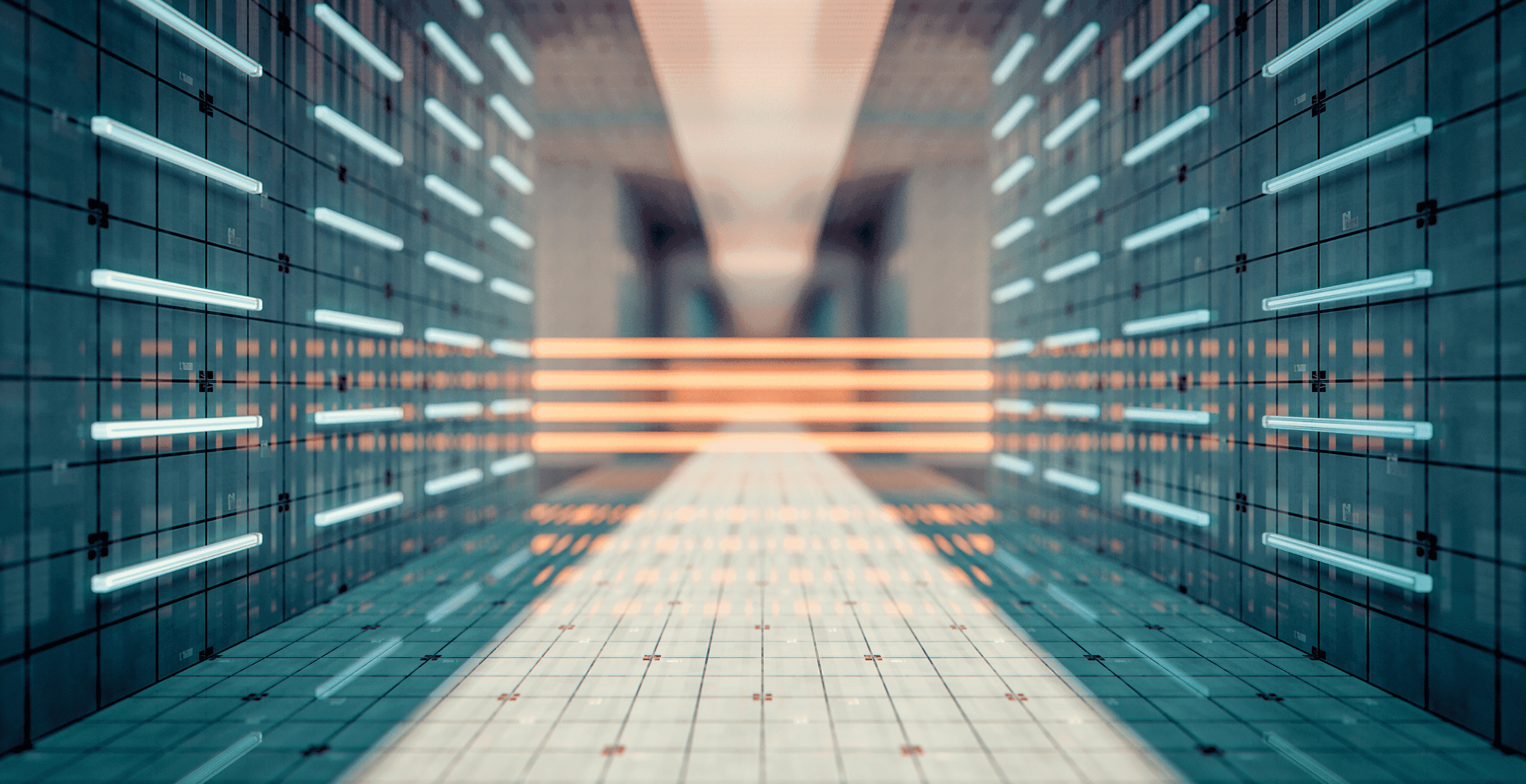
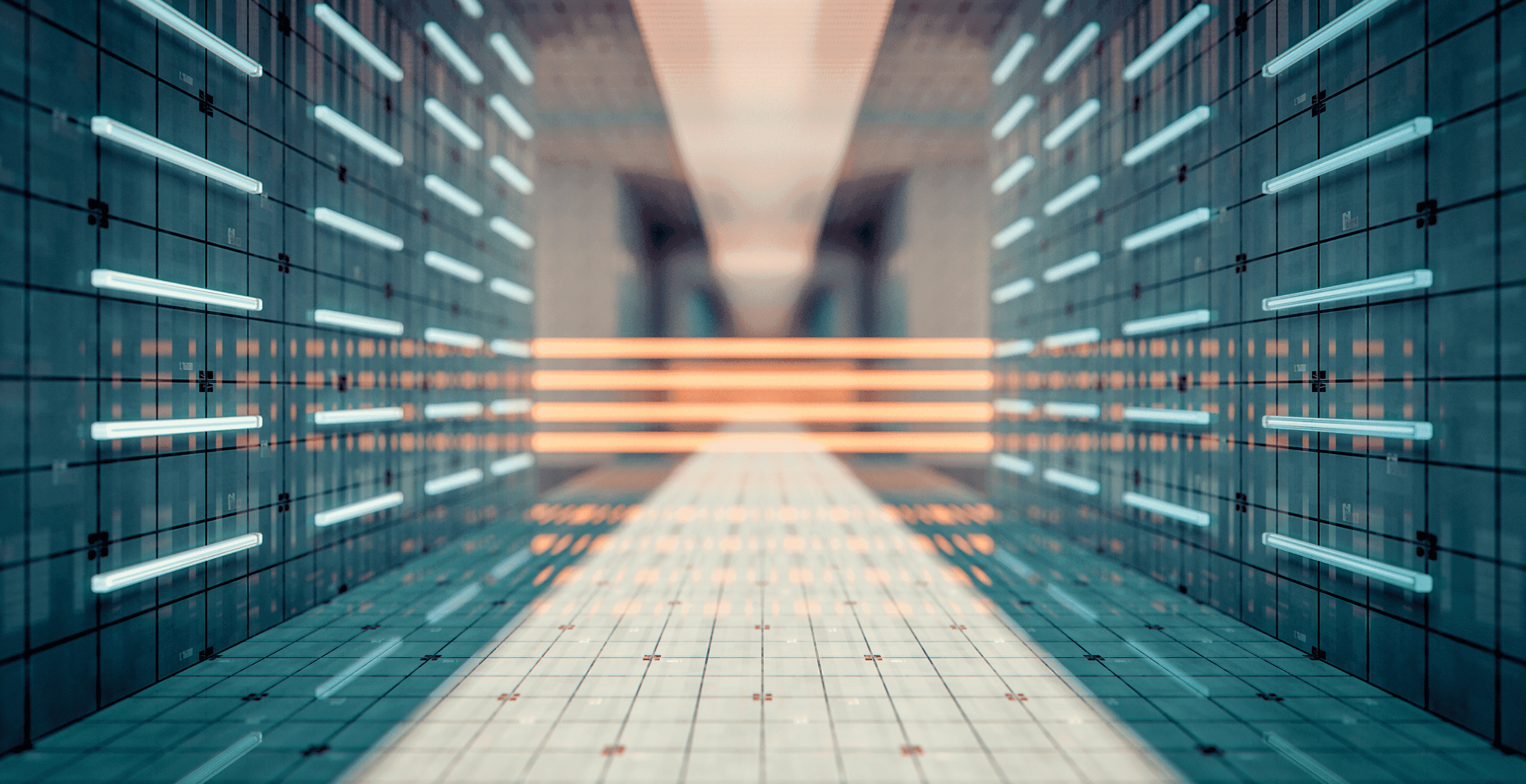
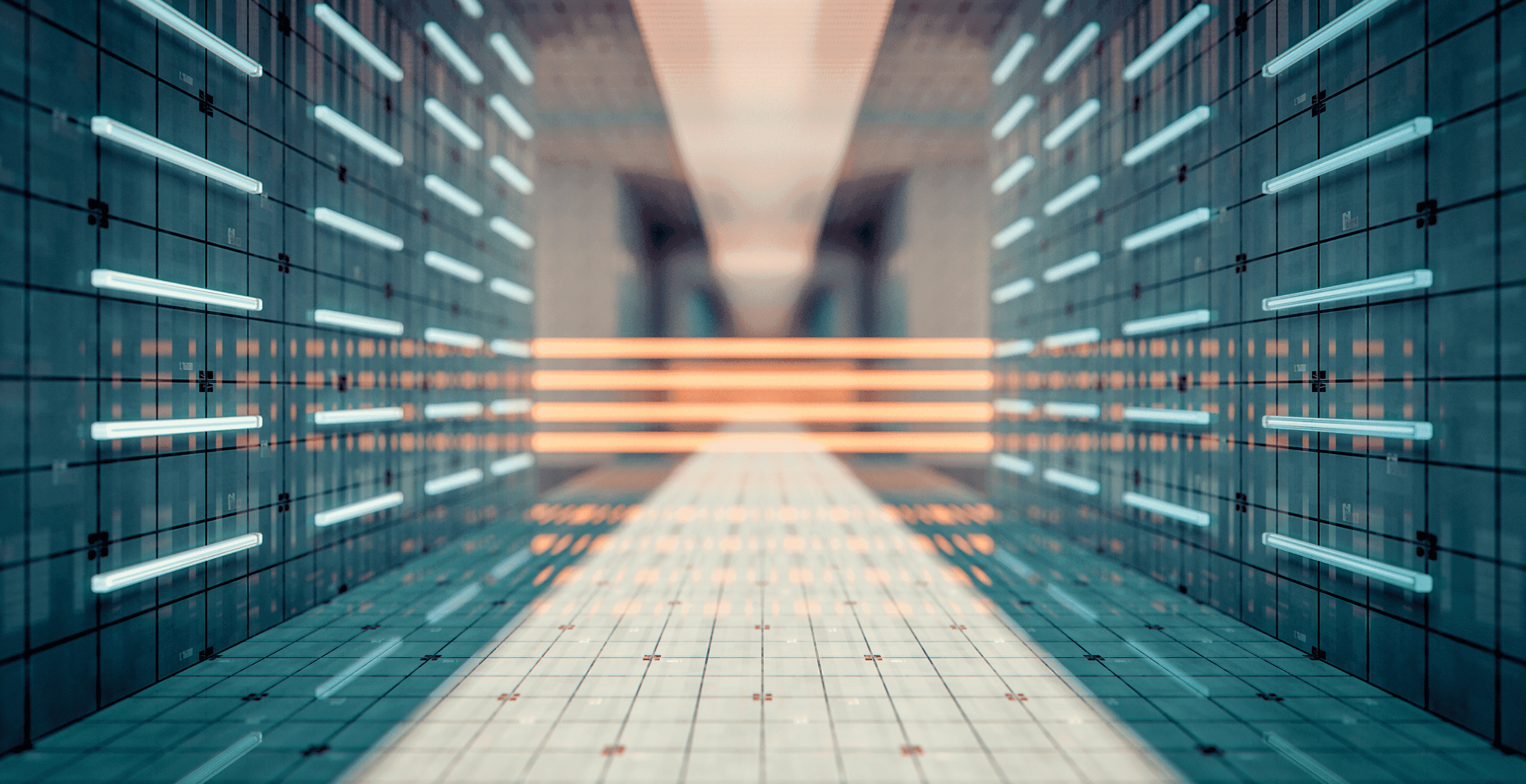
Best Practices In Data Governance For AI

Rehan Asif
Jun 10, 2024
Read the article




Success Stories And Case Studies Of AI Adoption Across Industries
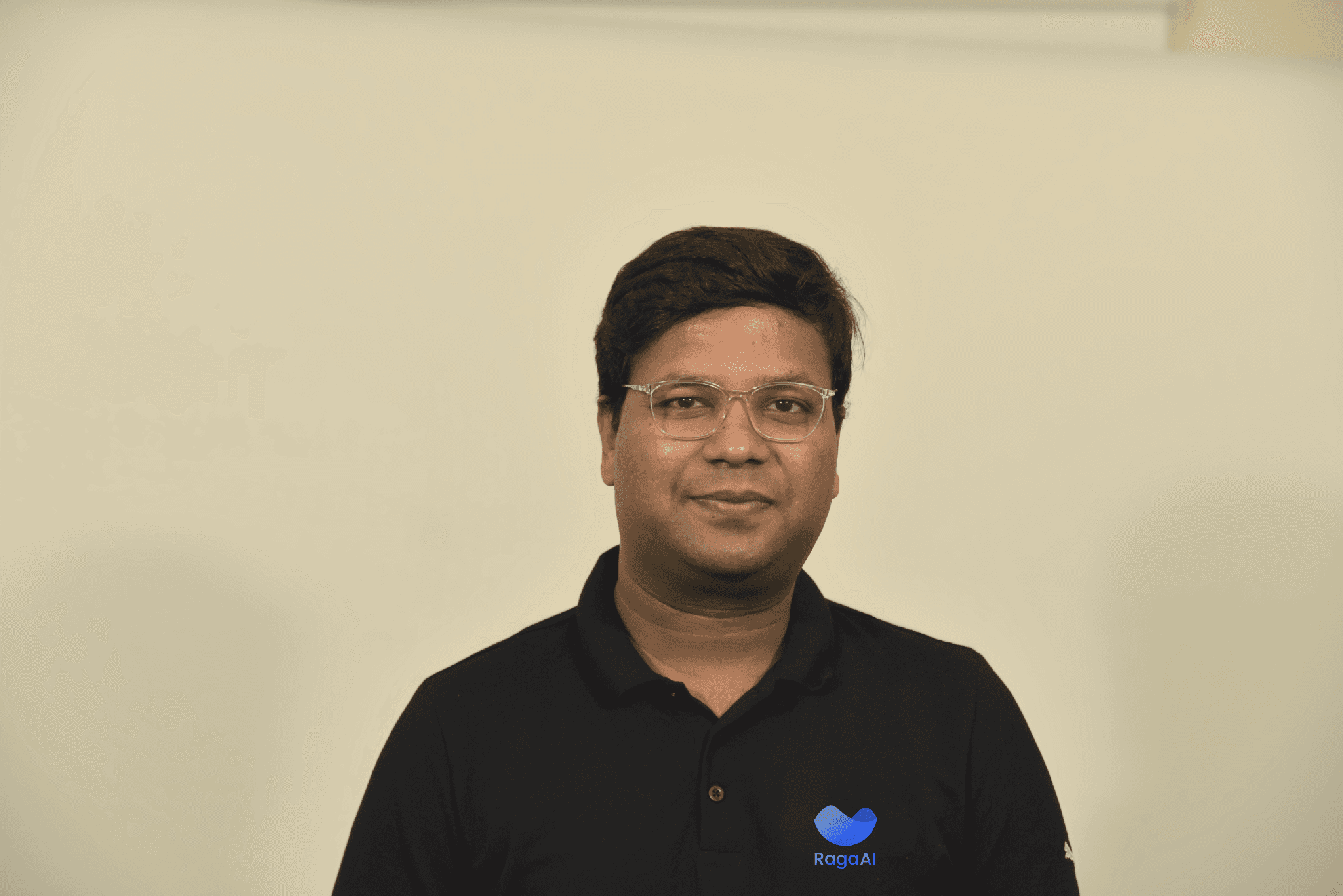
Jigar Gupta
May 1, 2024
Read the article
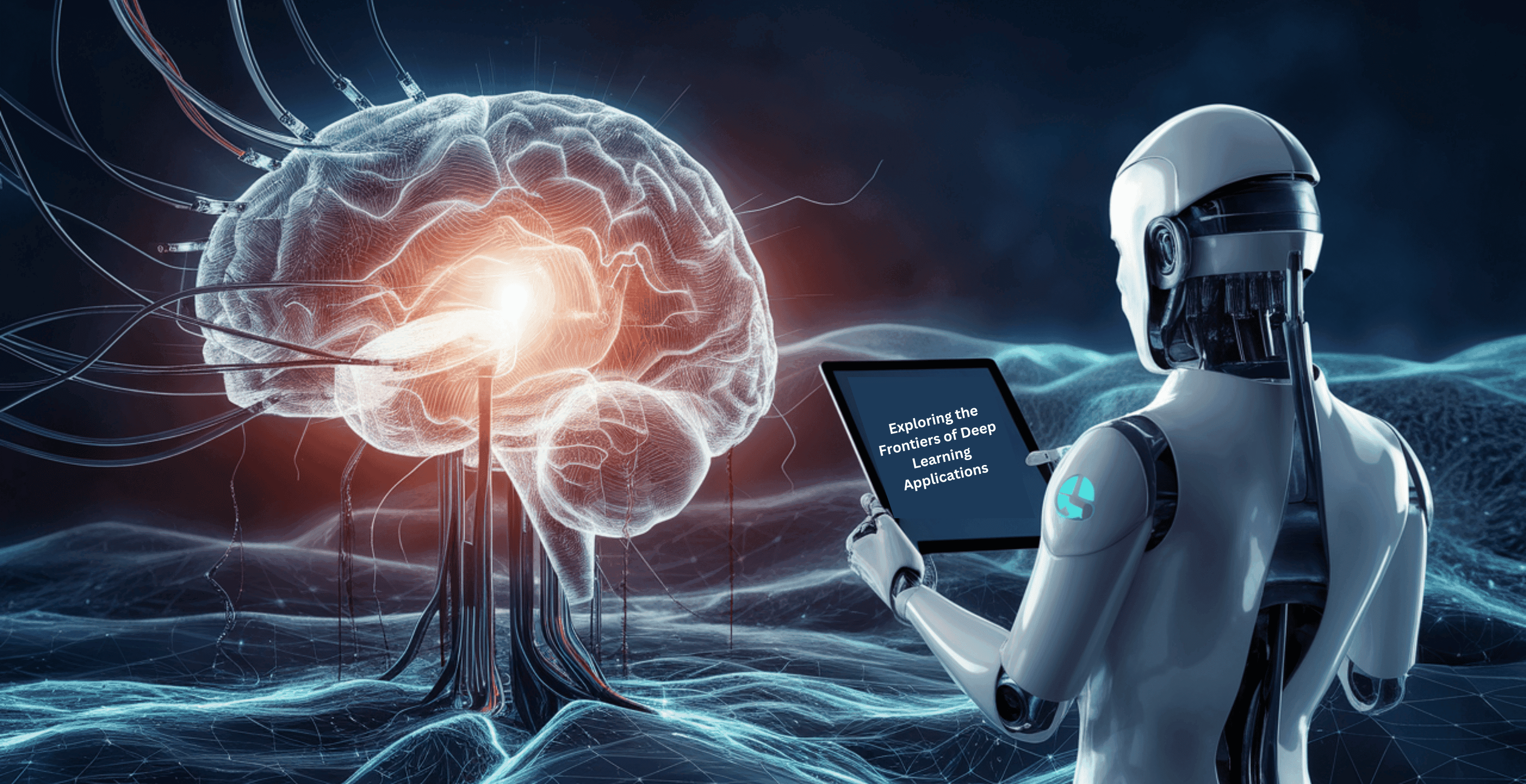
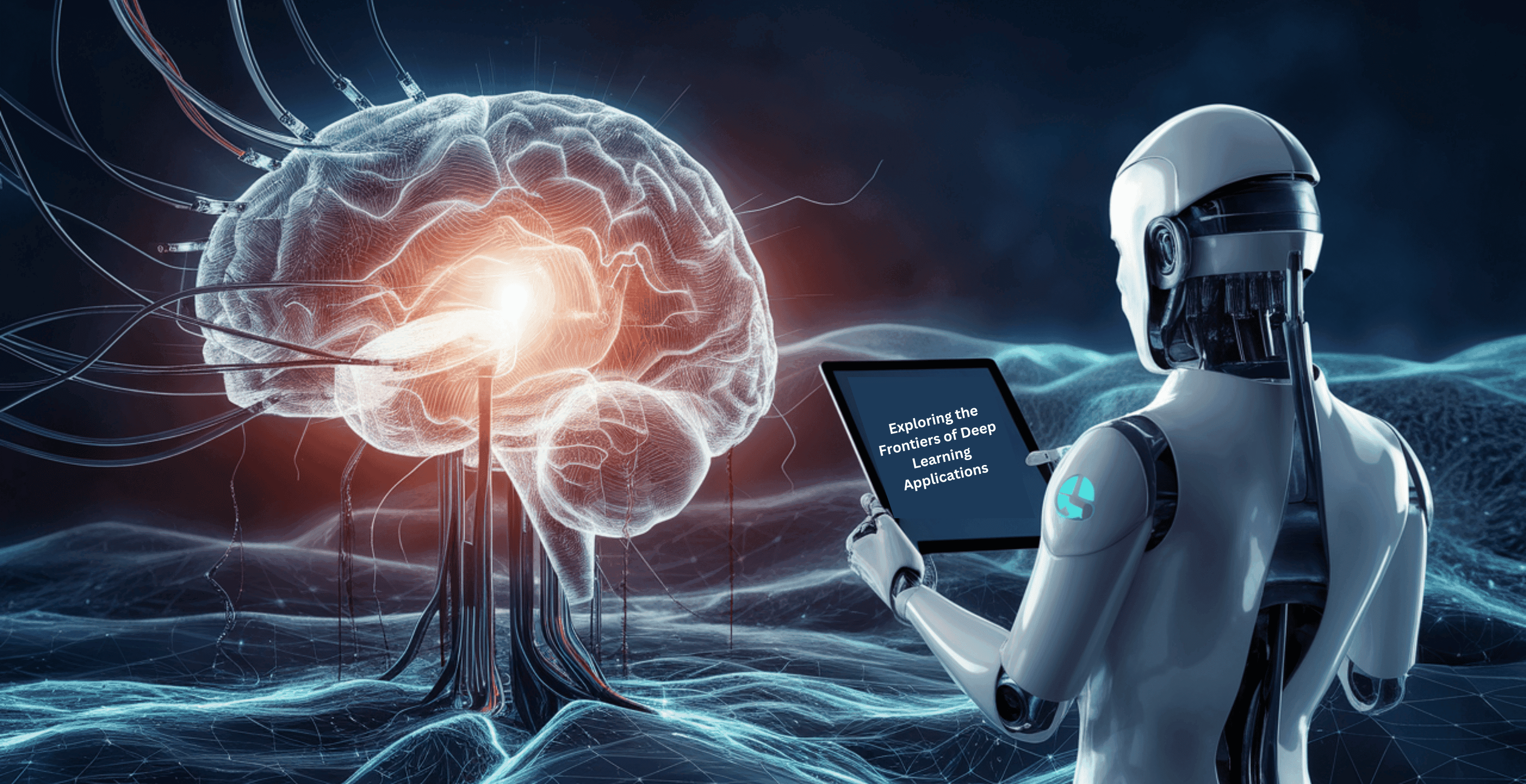
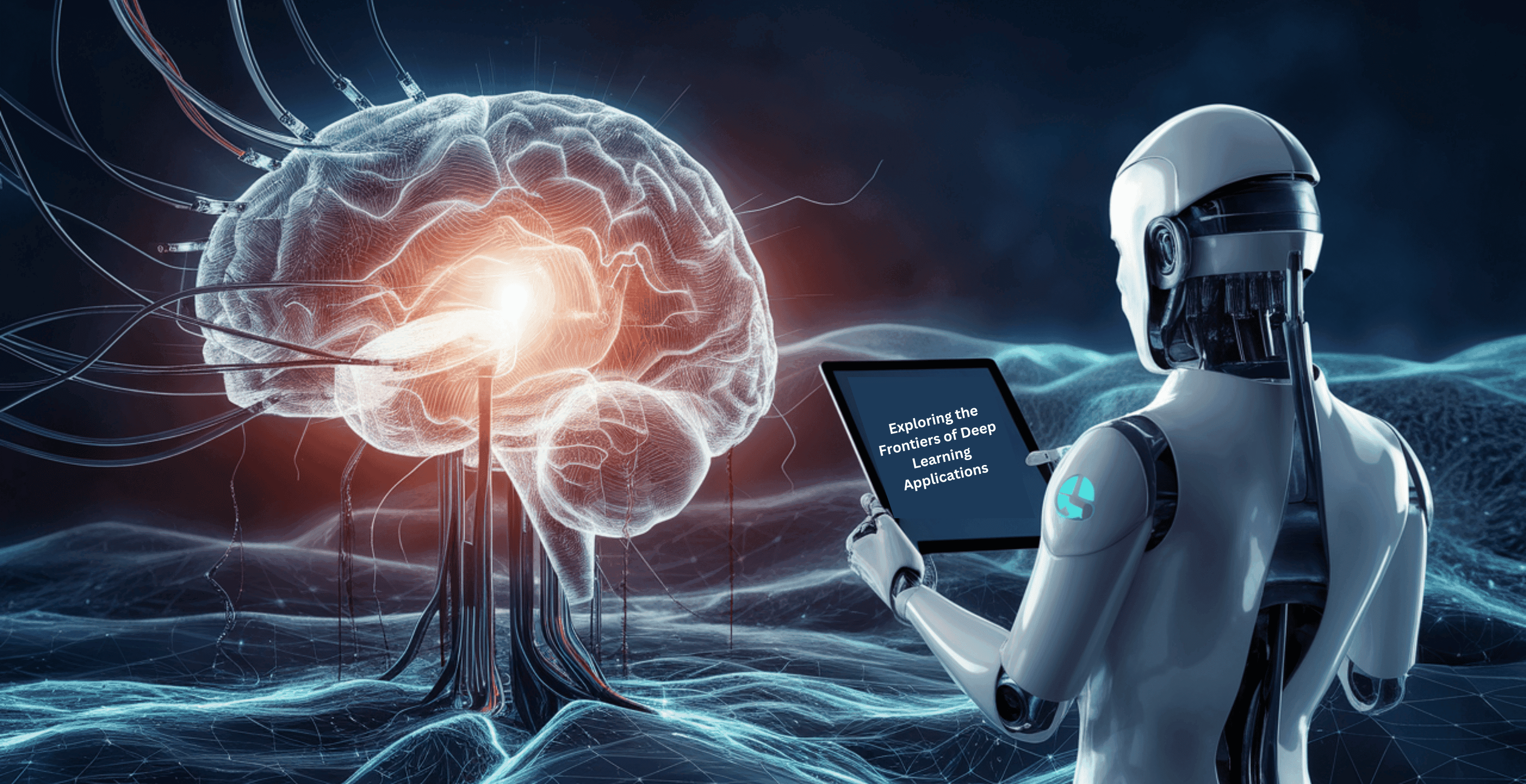
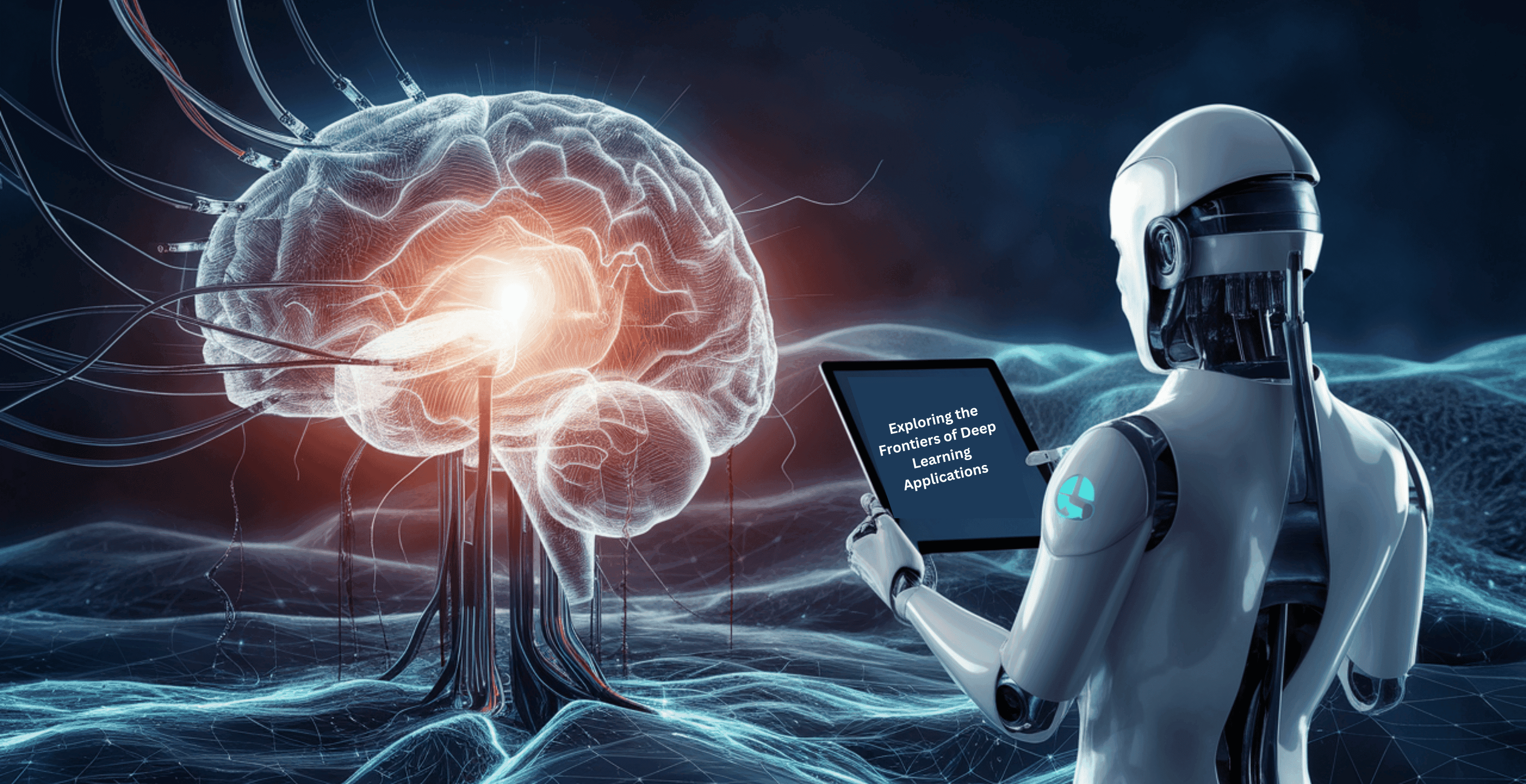
Exploring The Frontiers Of Deep Learning Applications
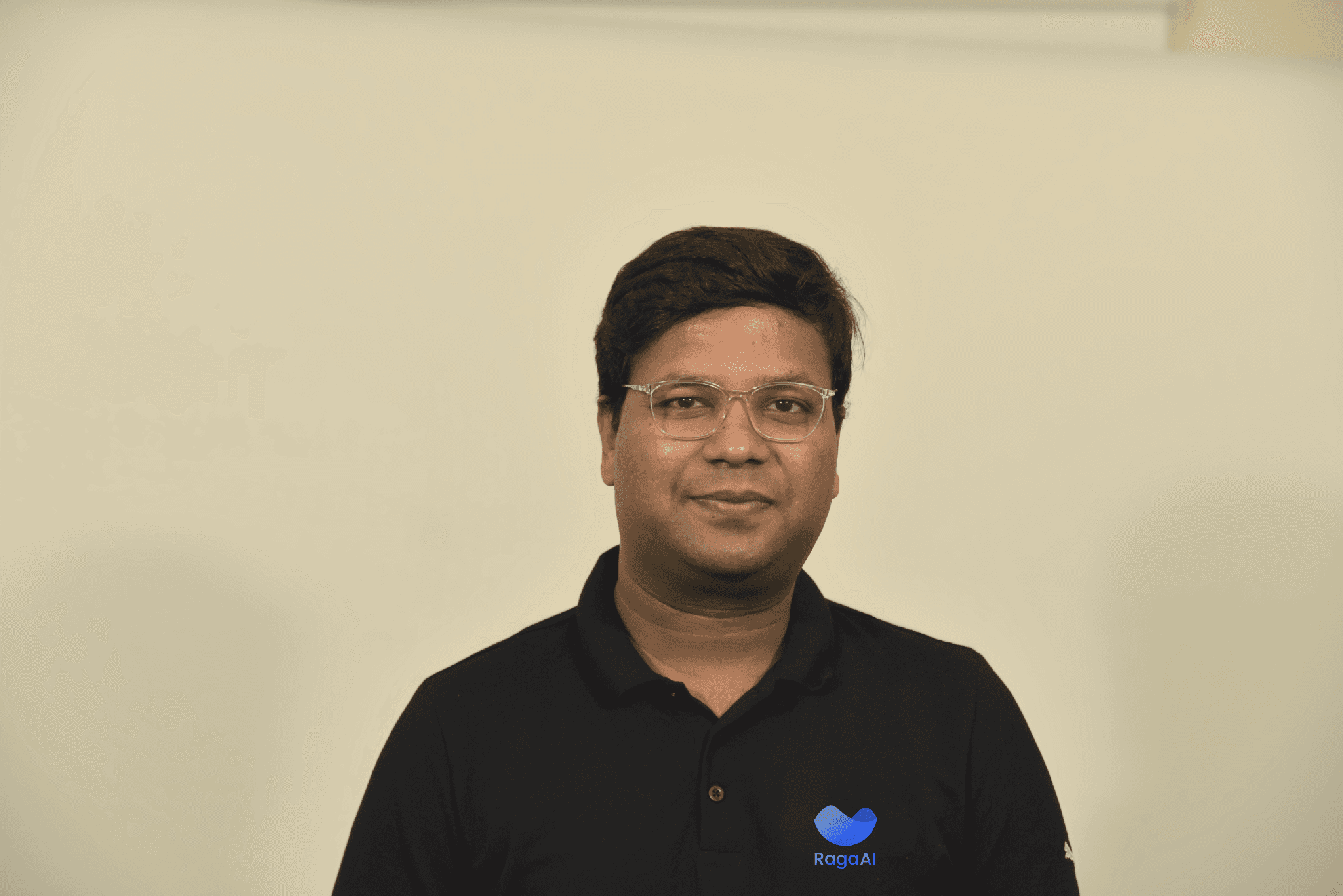
Jigar Gupta
May 1, 2024
Read the article




Integration Of RAG Platforms With Existing Enterprise Systems
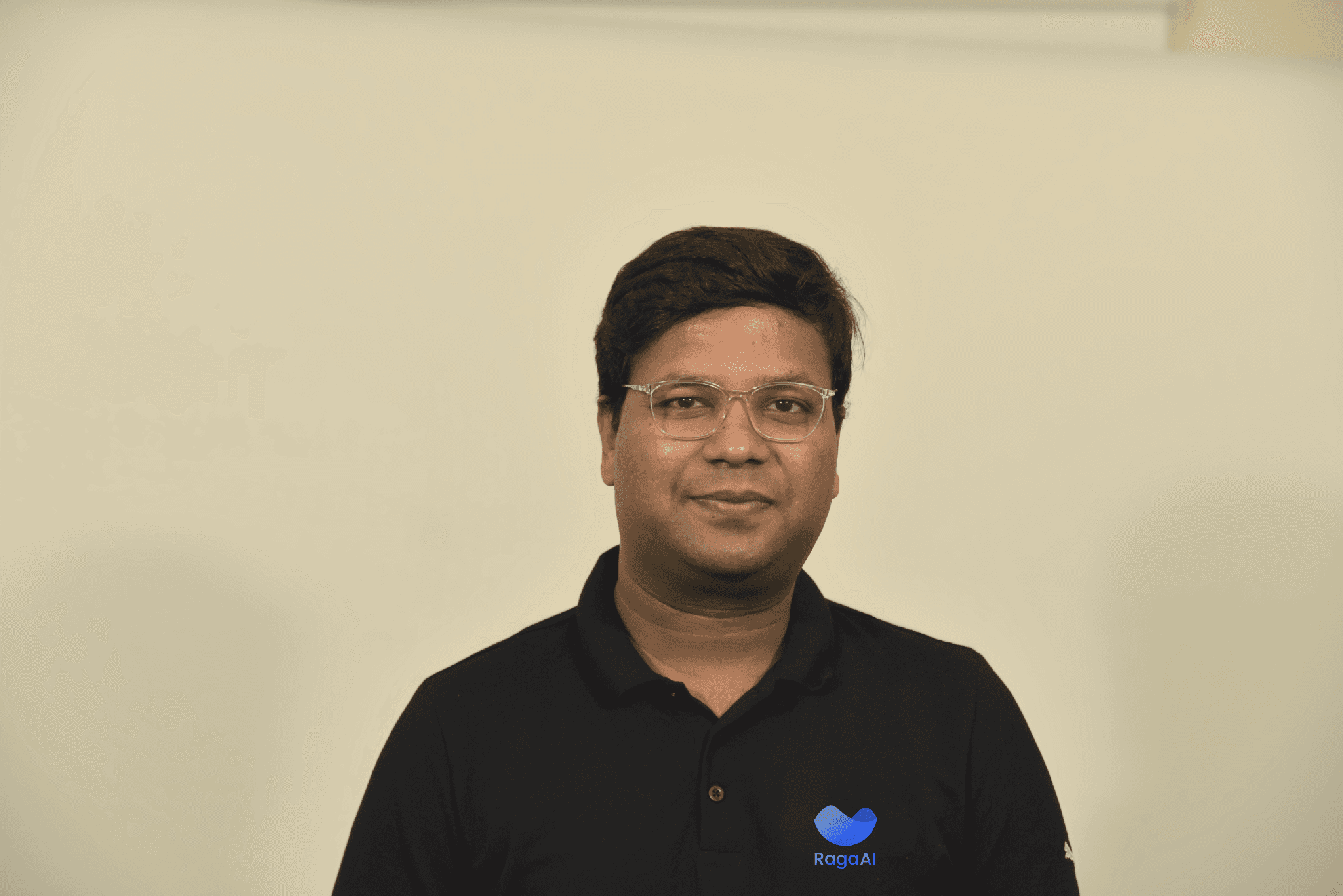
Jigar Gupta
Apr 30, 2024
Read the article




Multimodal LLMS Using Image And Text

Rehan Asif
Apr 30, 2024
Read the article




Understanding ML Model Monitoring In Production

Rehan Asif
Apr 30, 2024
Read the article
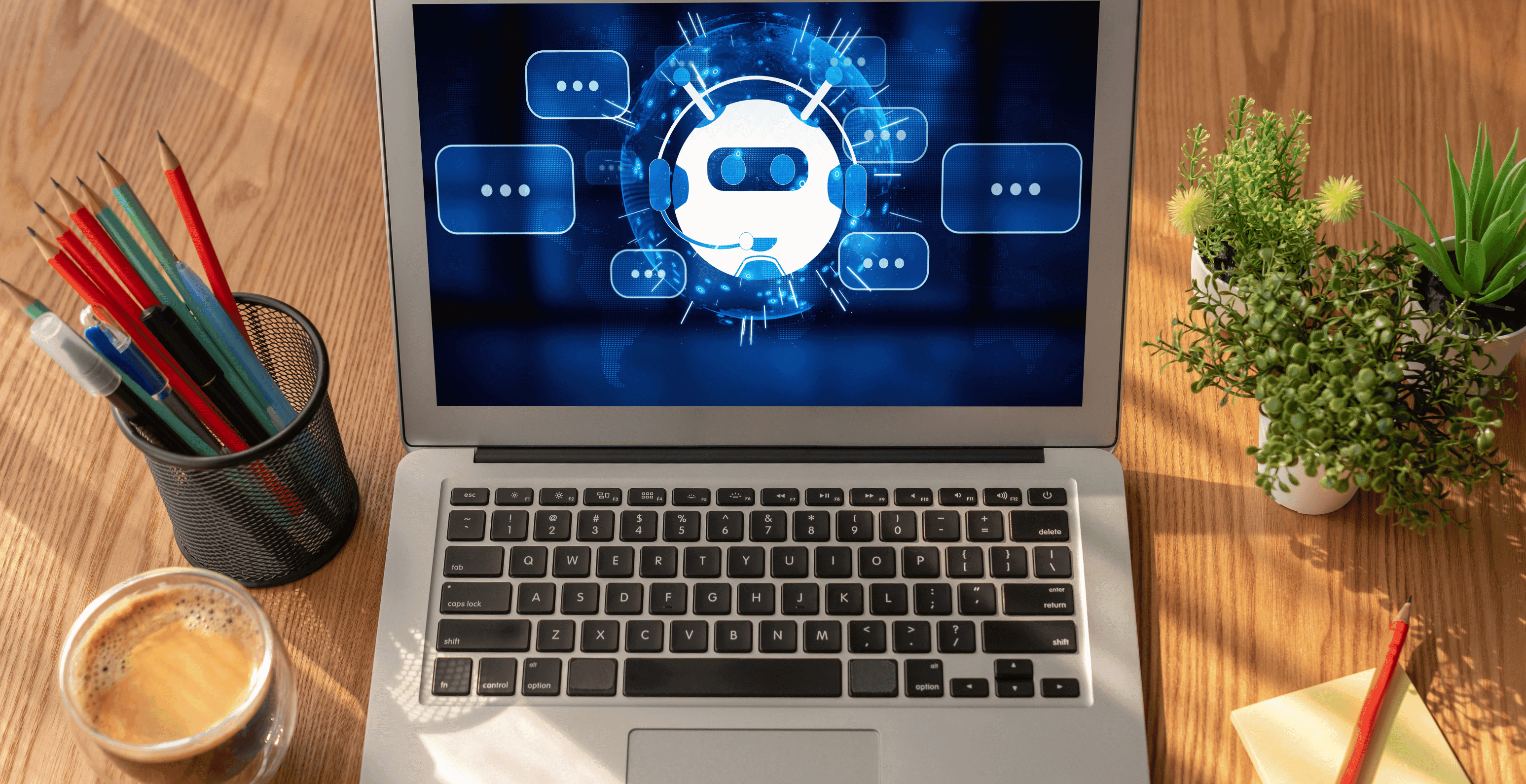
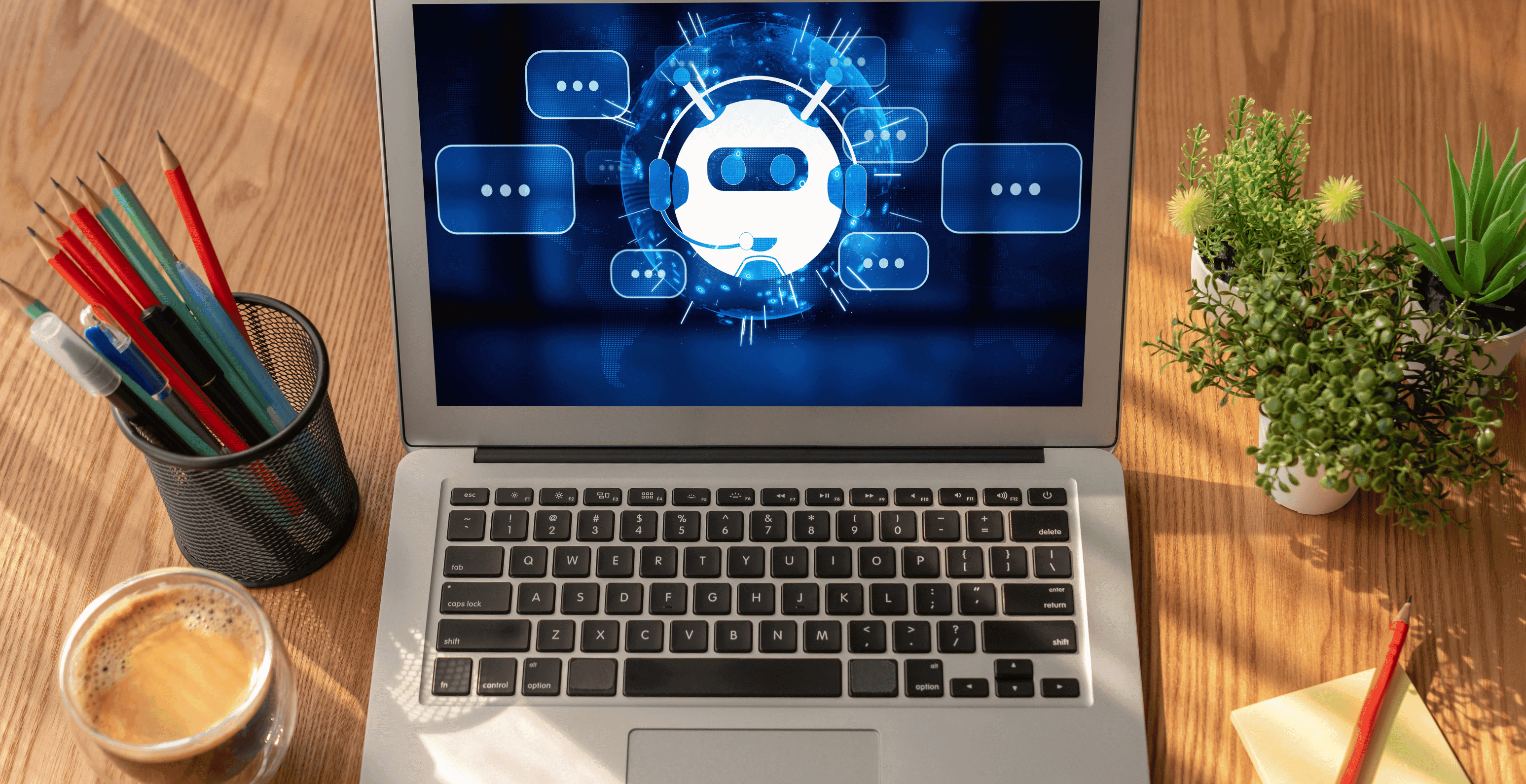
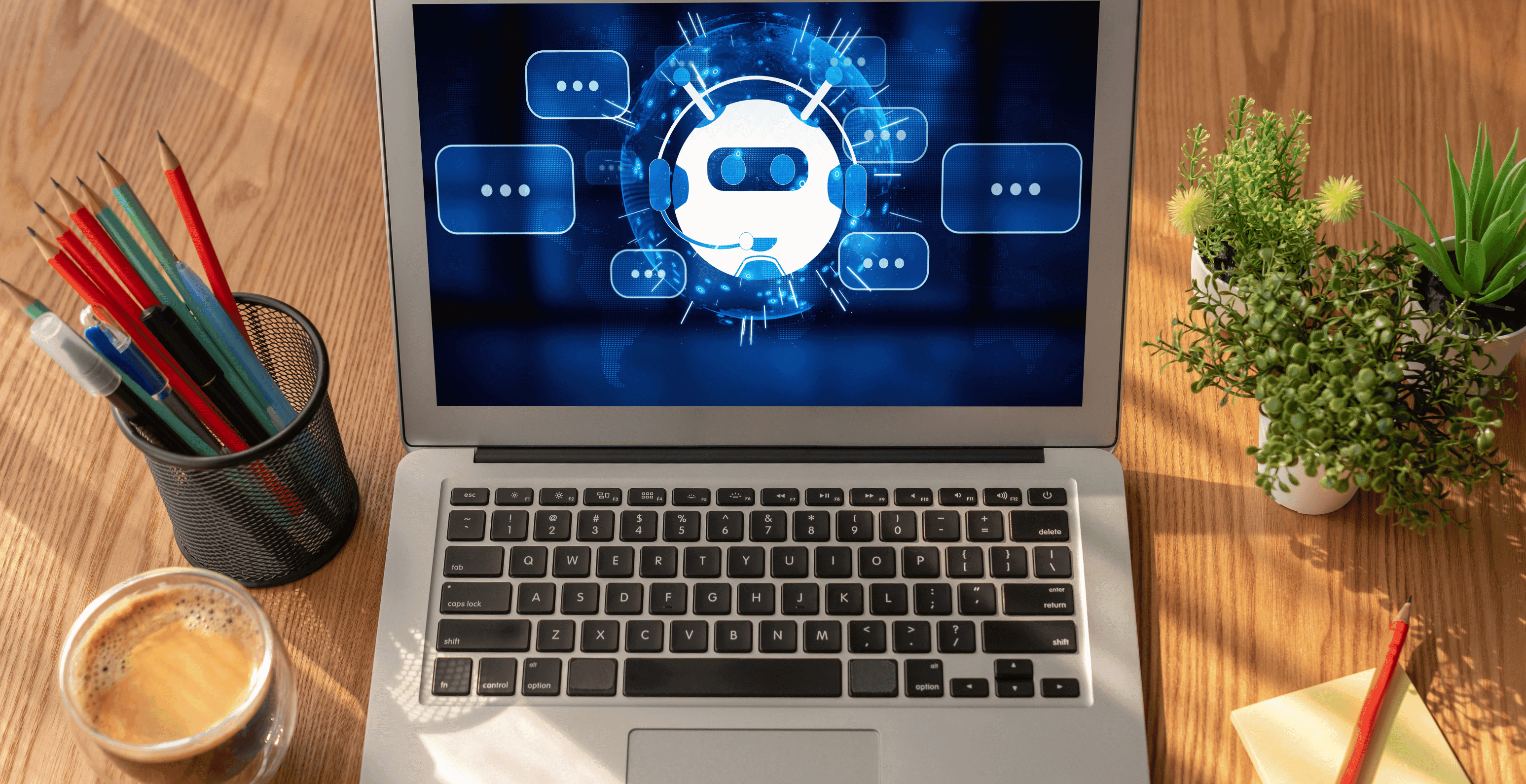
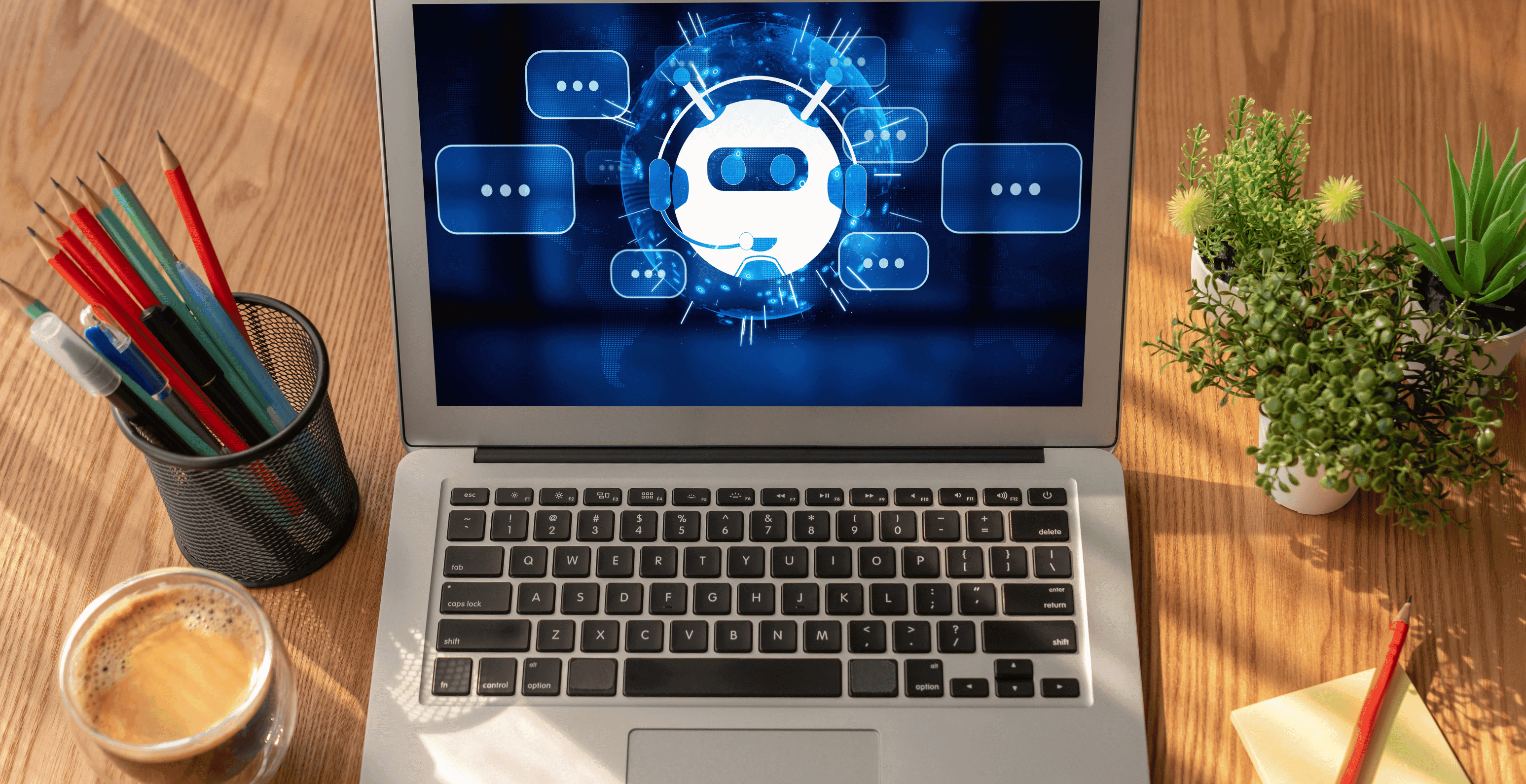
Strategic Approach To Testing AI-Powered Applications And Systems

Rehan Asif
Apr 30, 2024
Read the article




Navigating GDPR Compliance for AI Applications

Rehan Asif
Apr 26, 2024
Read the article
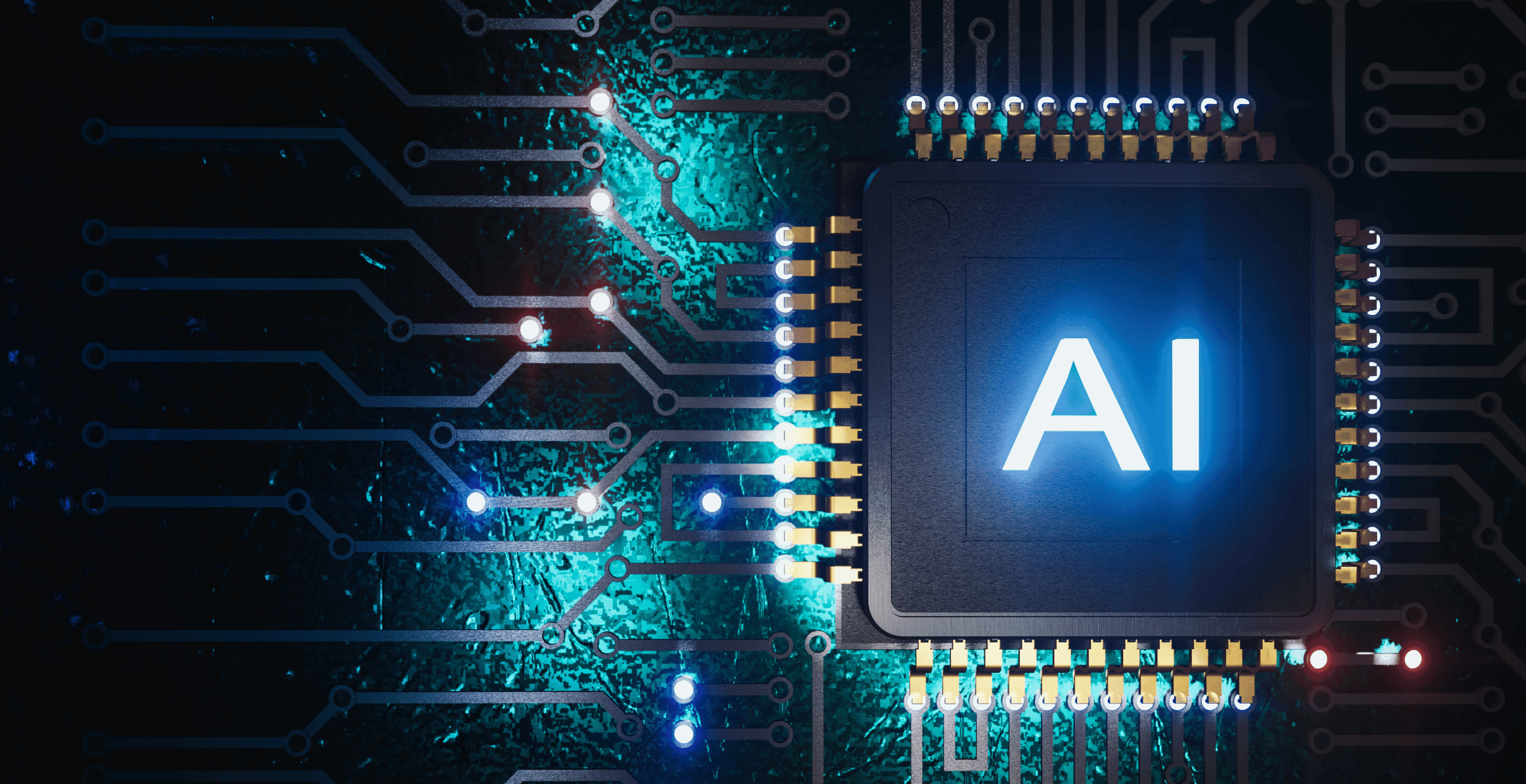
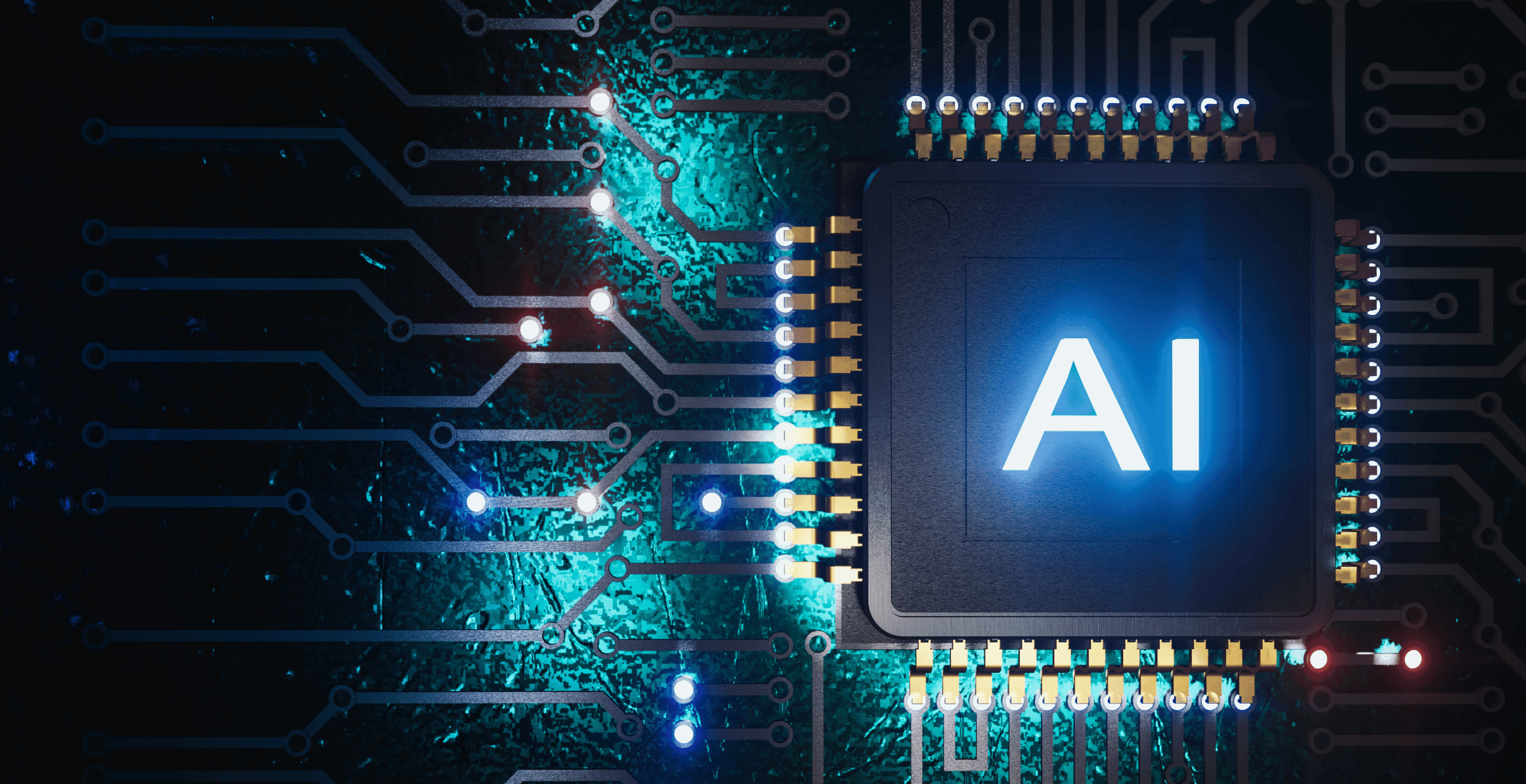
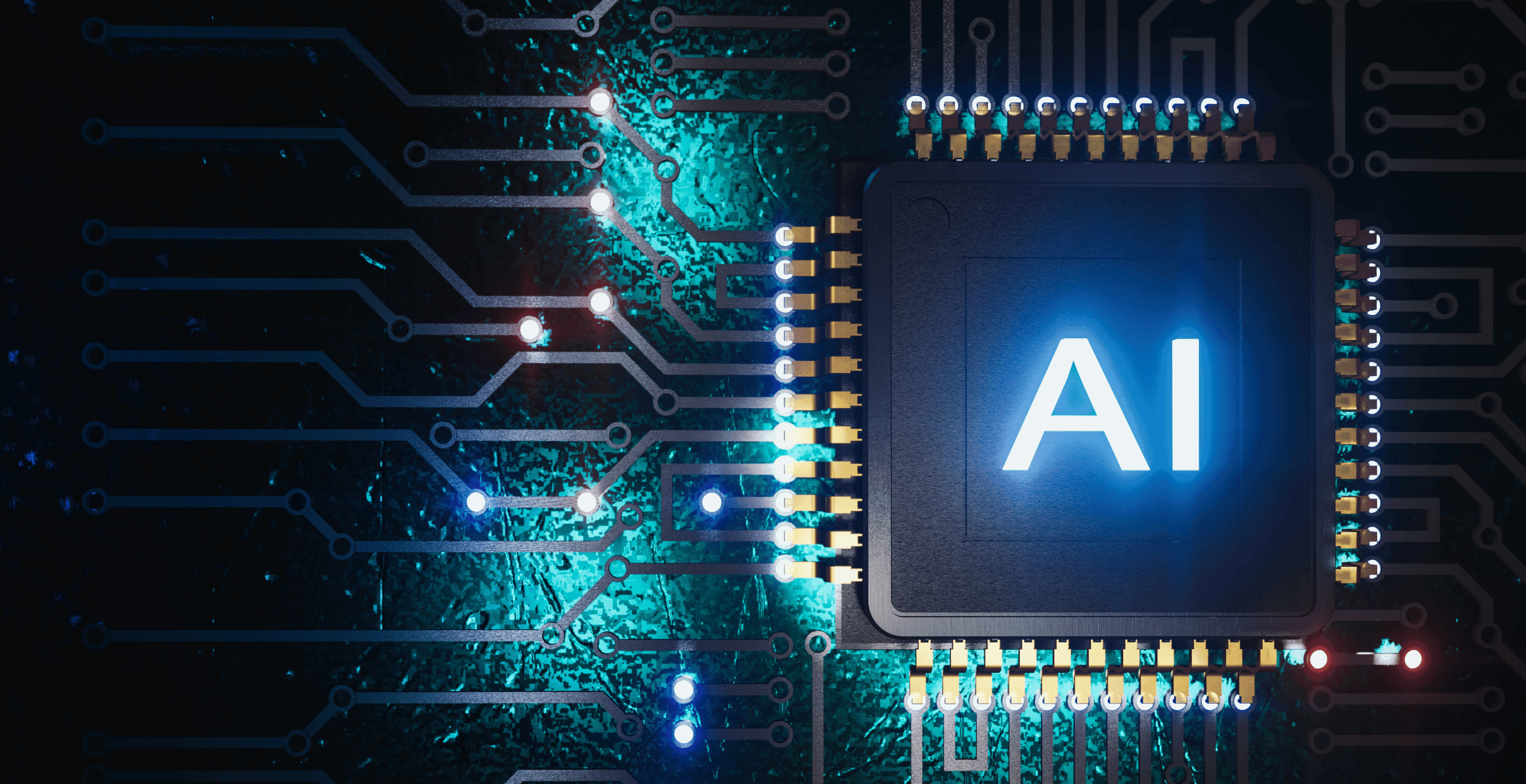
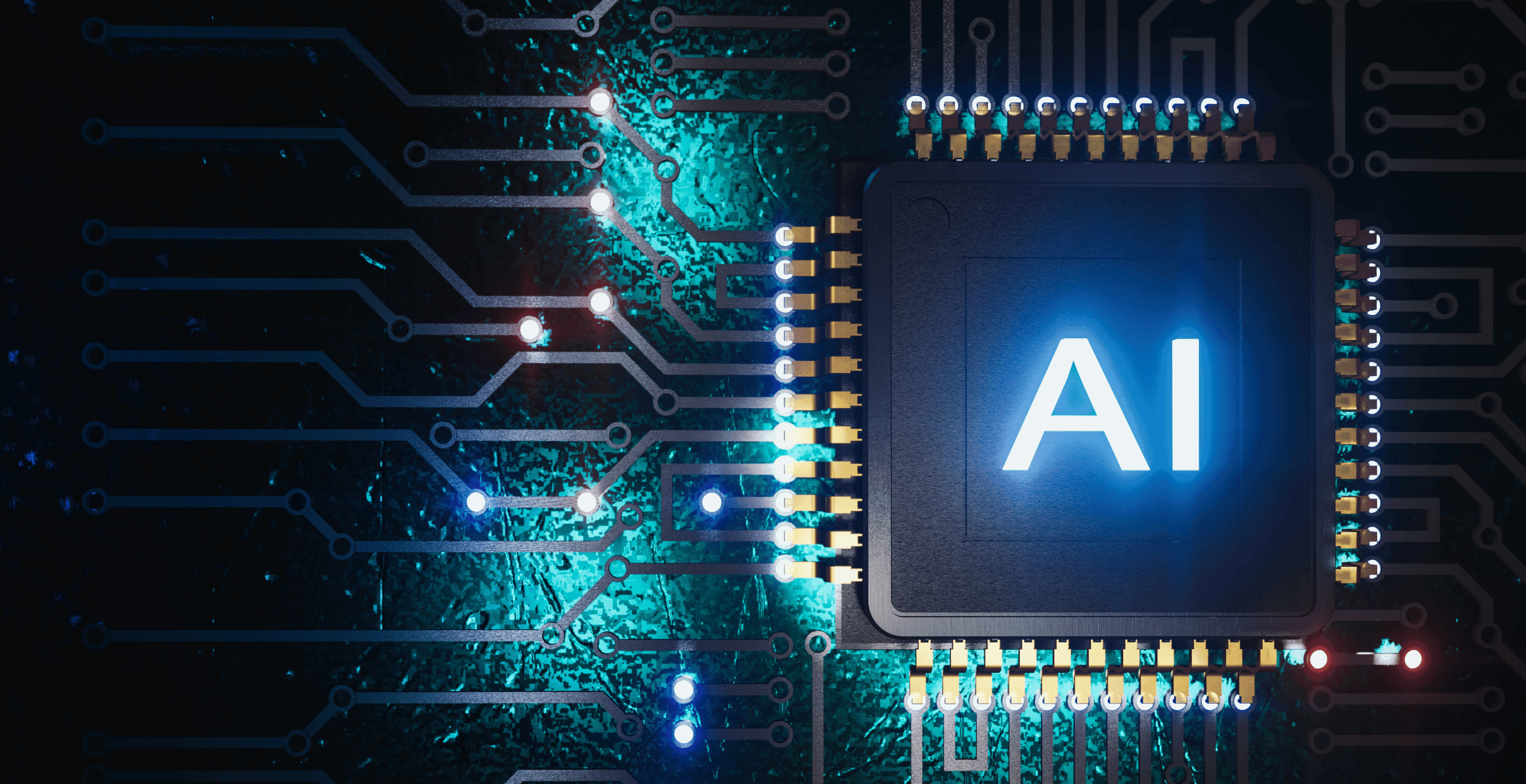
The Impact of AI Governance on Innovation and Development Speed

Rehan Asif
Apr 26, 2024
Read the article
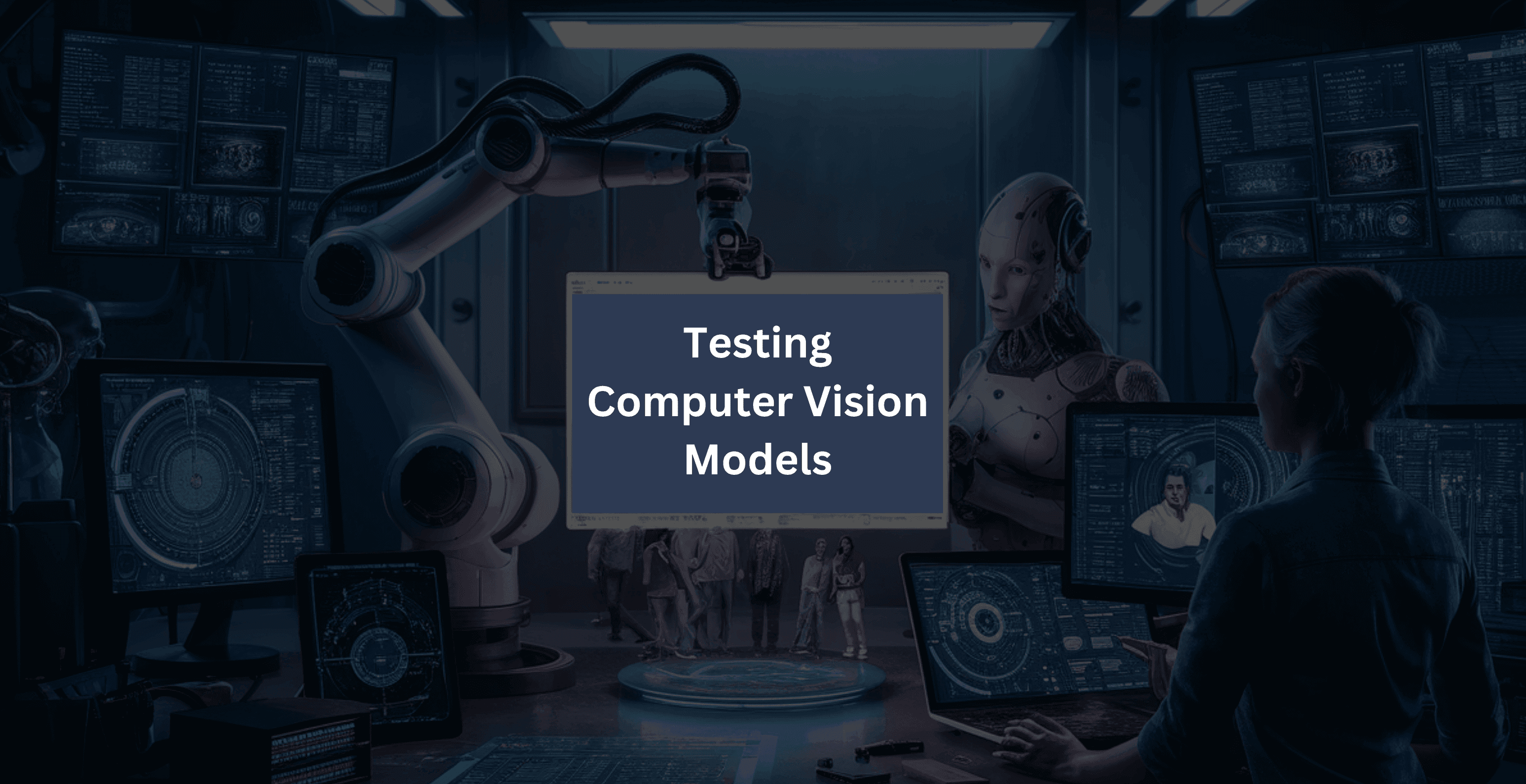
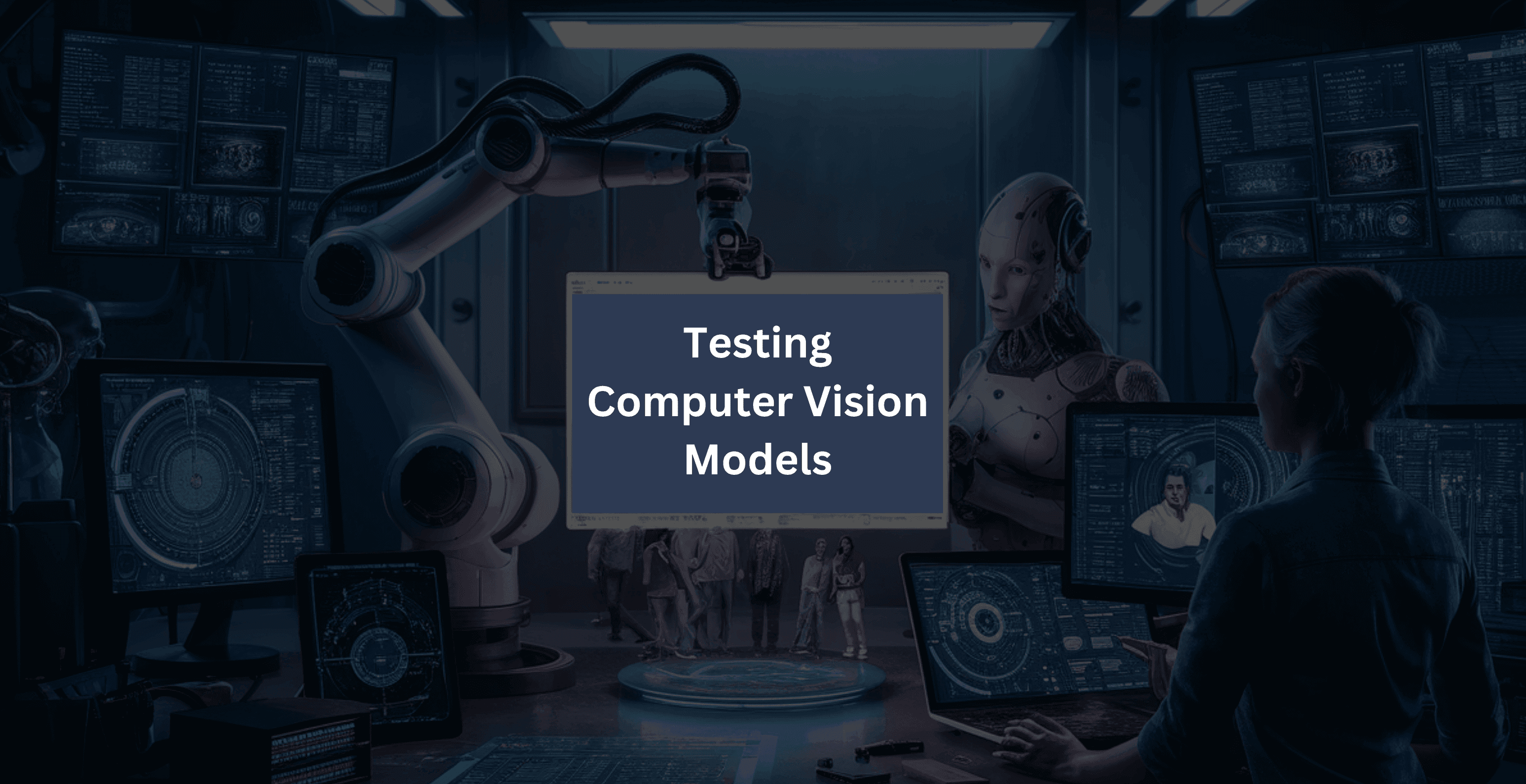
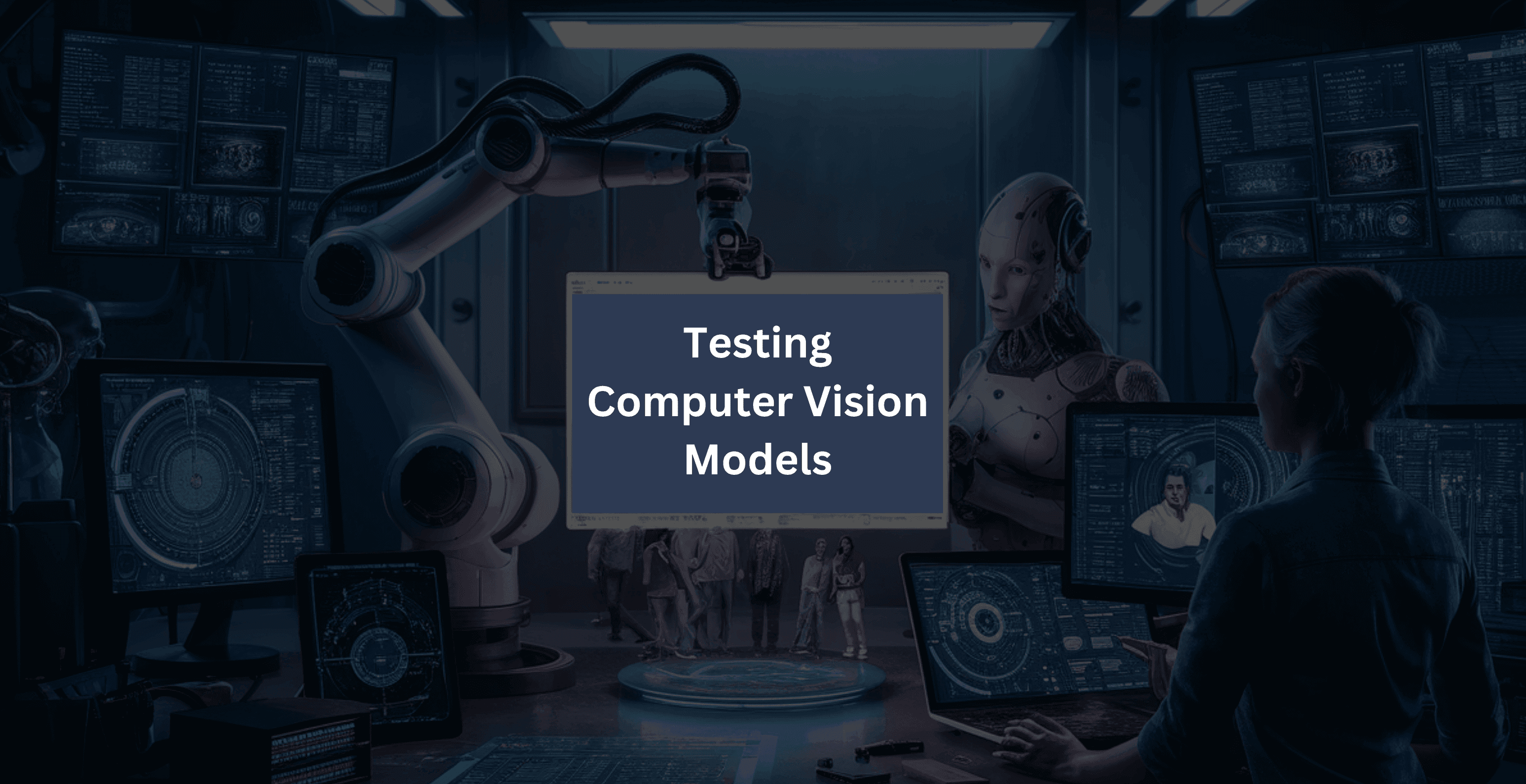
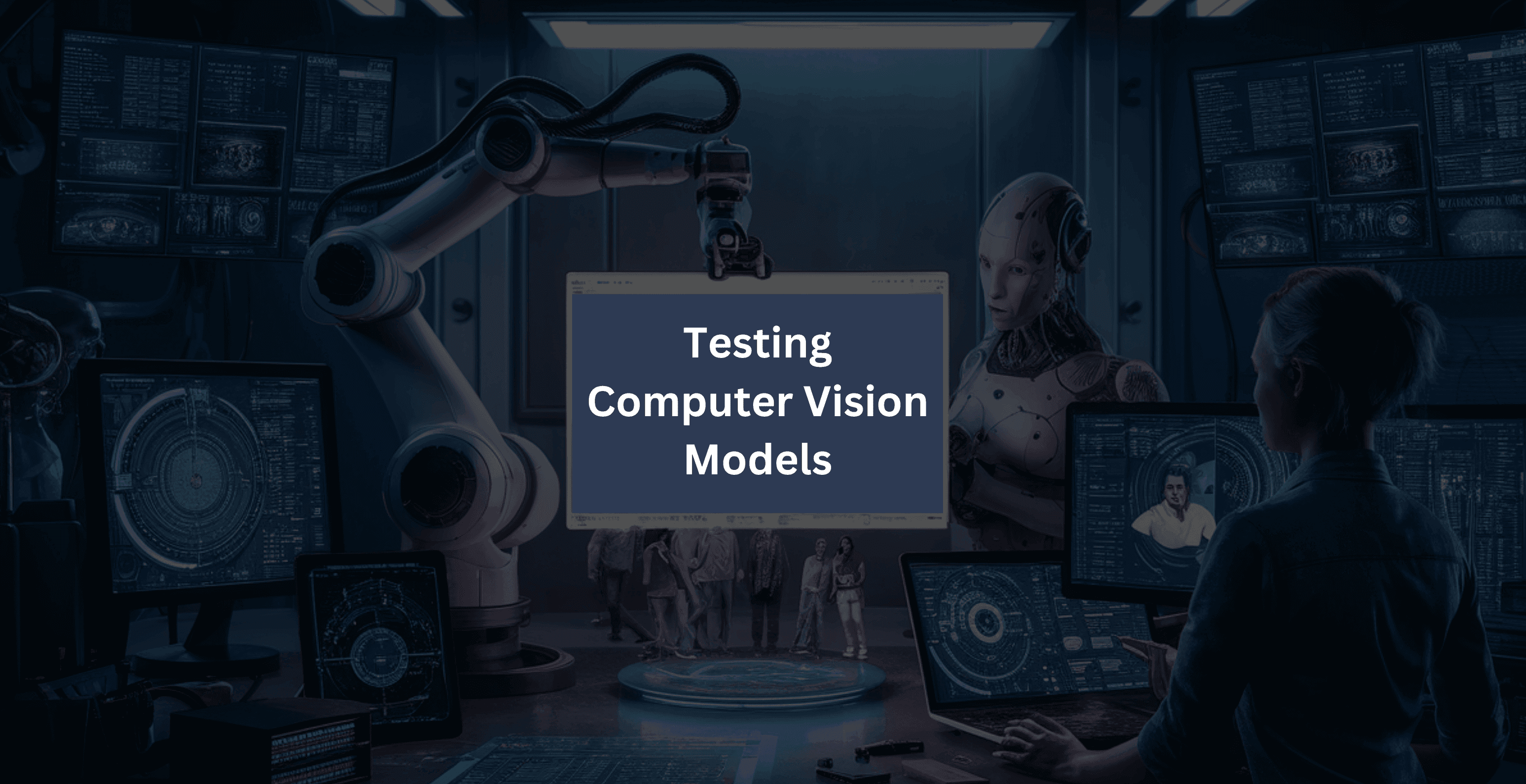
Best Practices For Testing Computer Vision Models
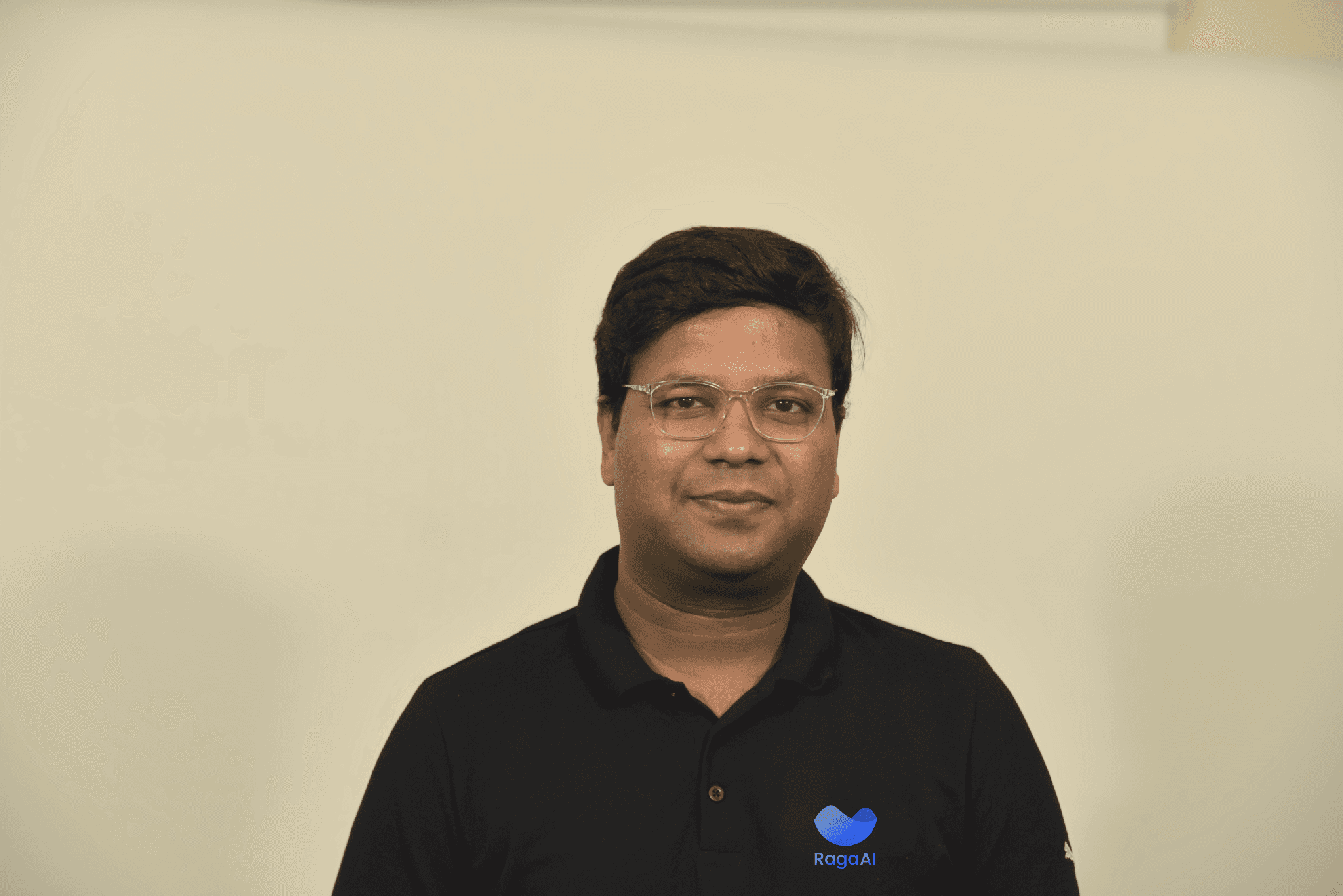
Jigar Gupta
Apr 25, 2024
Read the article
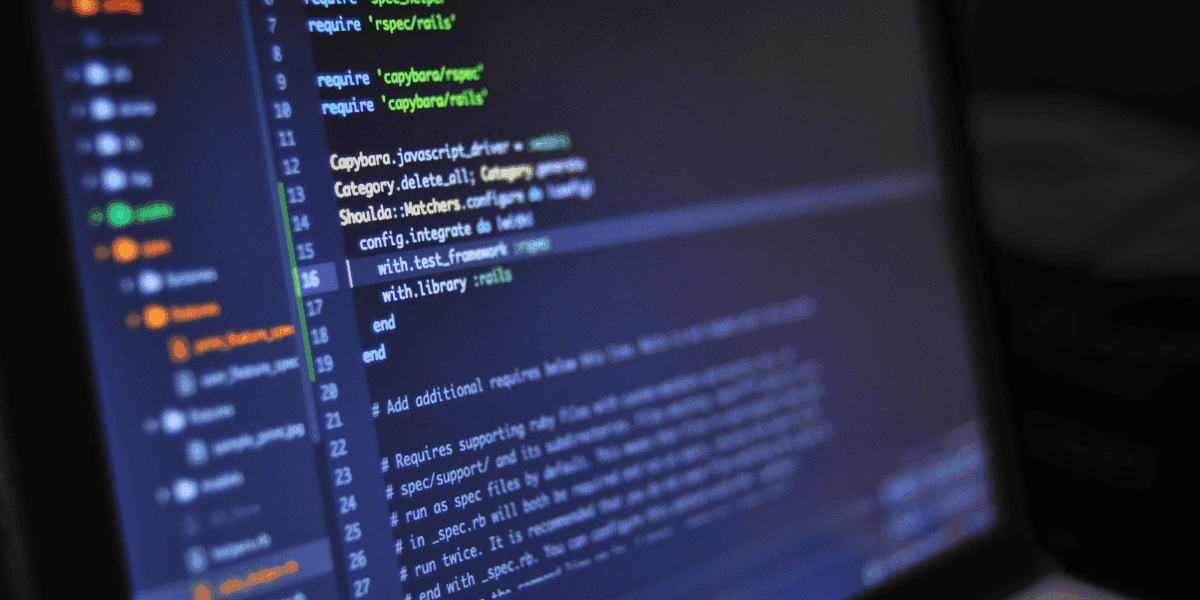
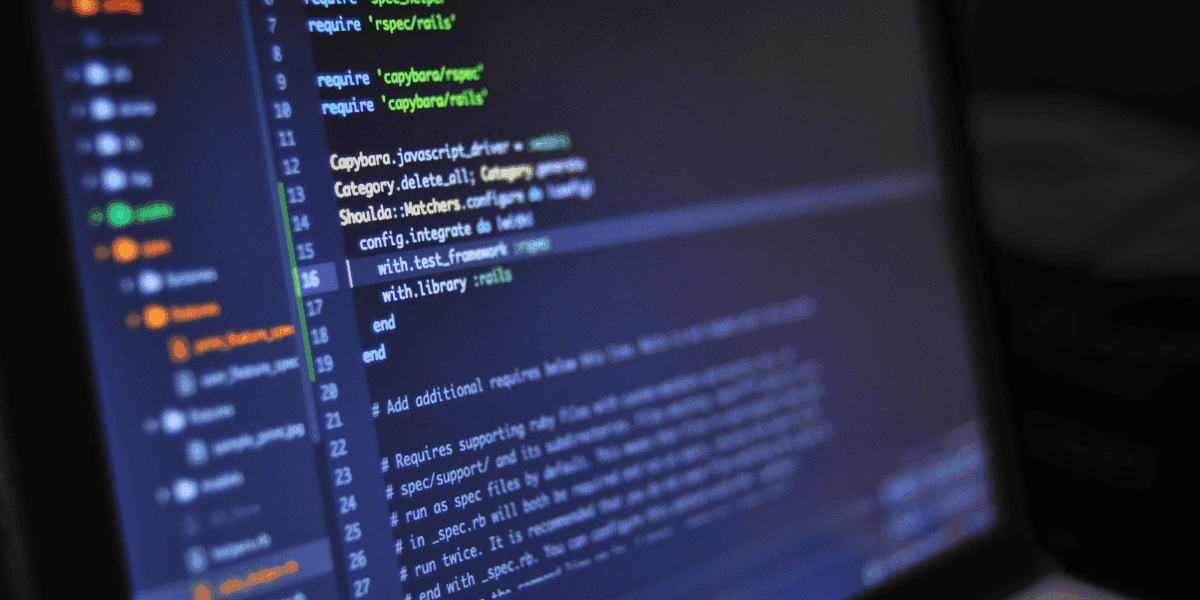
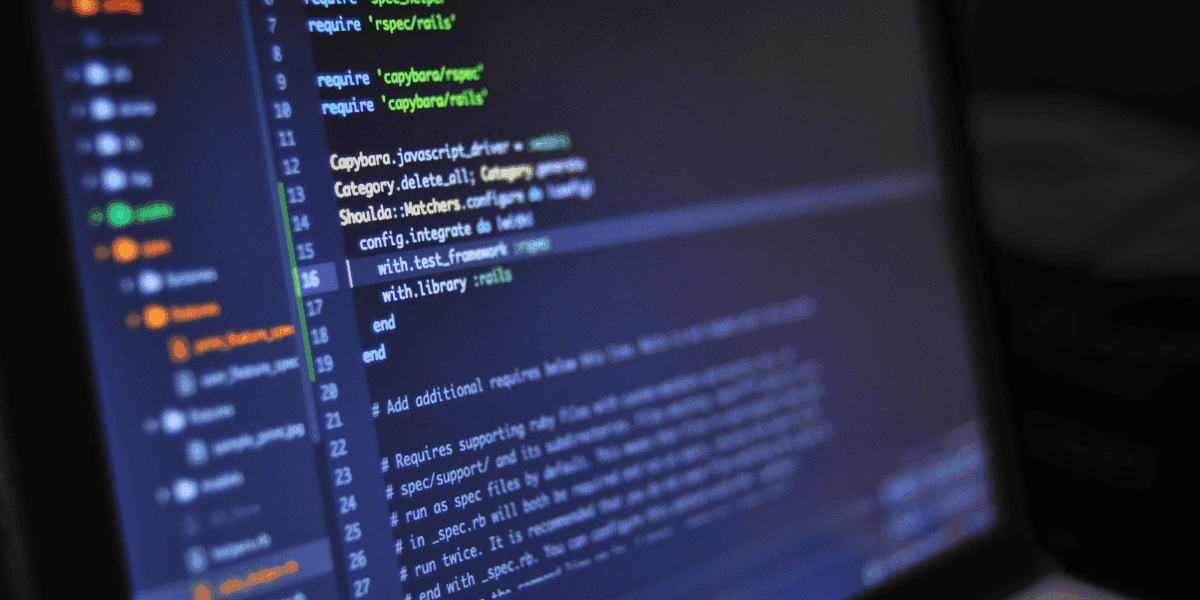
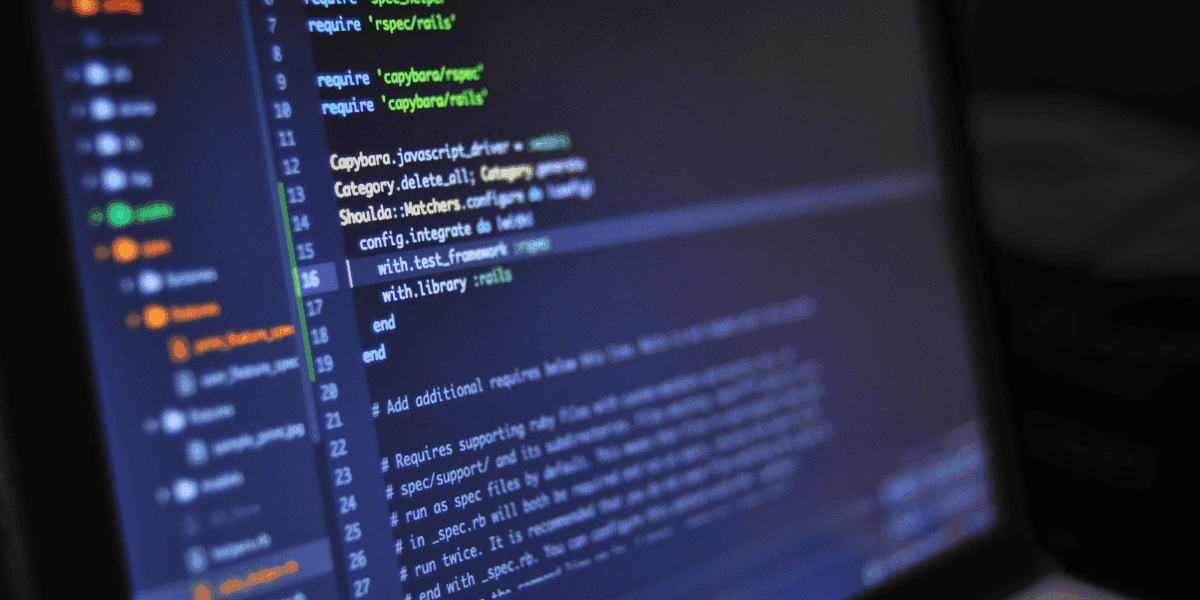
Building Low-Code LLM Apps with Visual Programming

Rehan Asif
Apr 26, 2024
Read the article




Understanding AI regulations In Finance

Akshat Gupta
Apr 26, 2024
Read the article




Compliance Automation: Getting Started with Regulatory Management

Akshat Gupta
Apr 25, 2024
Read the article
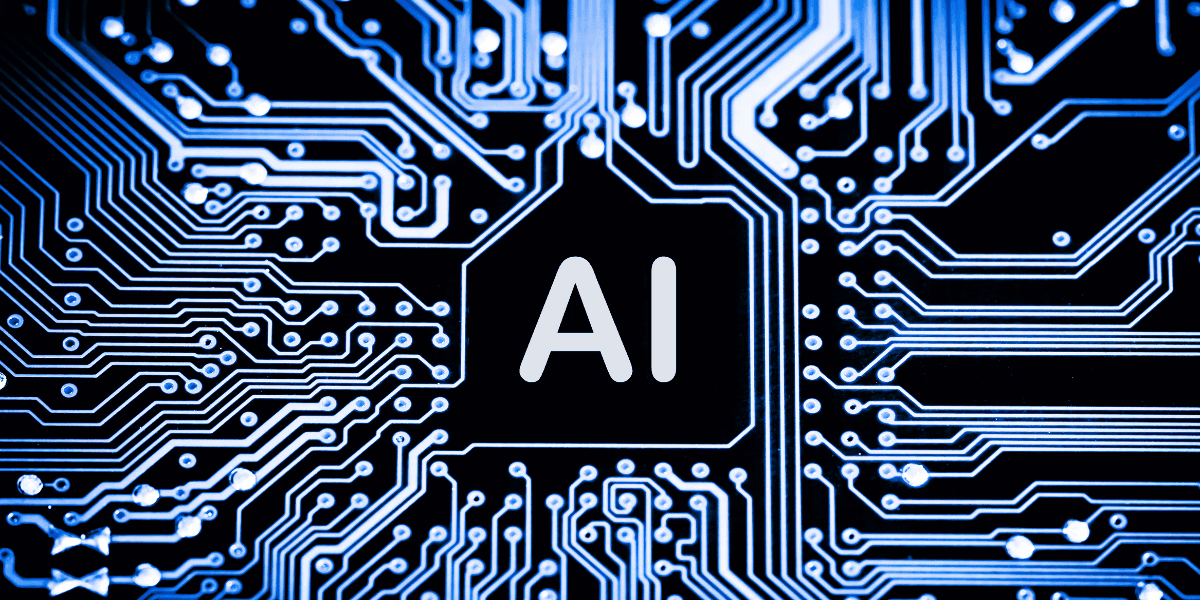
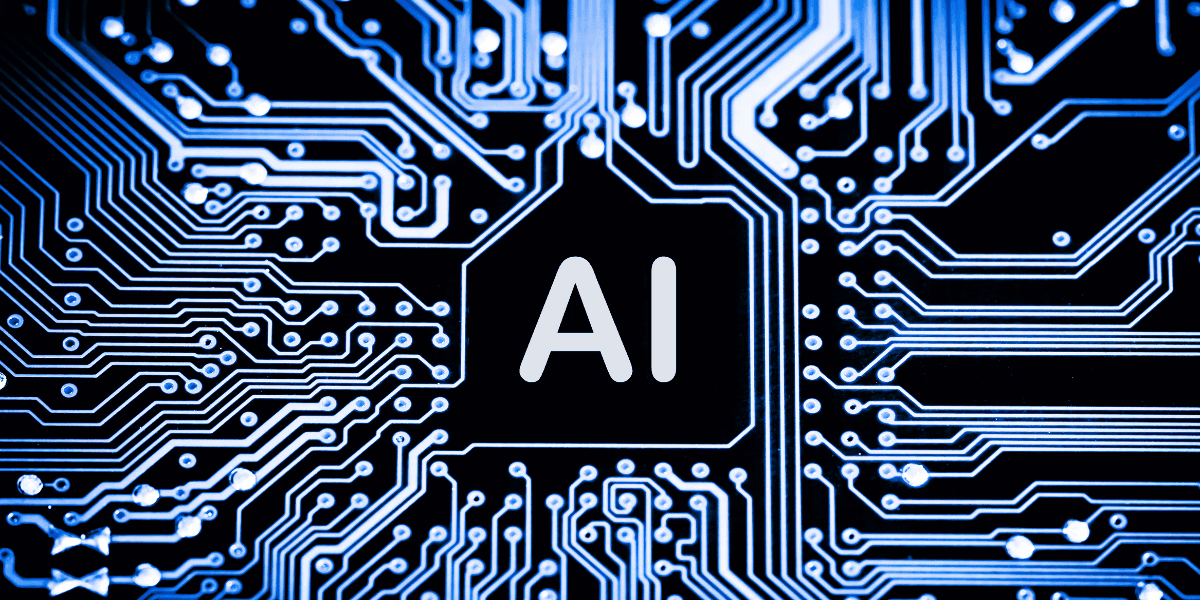
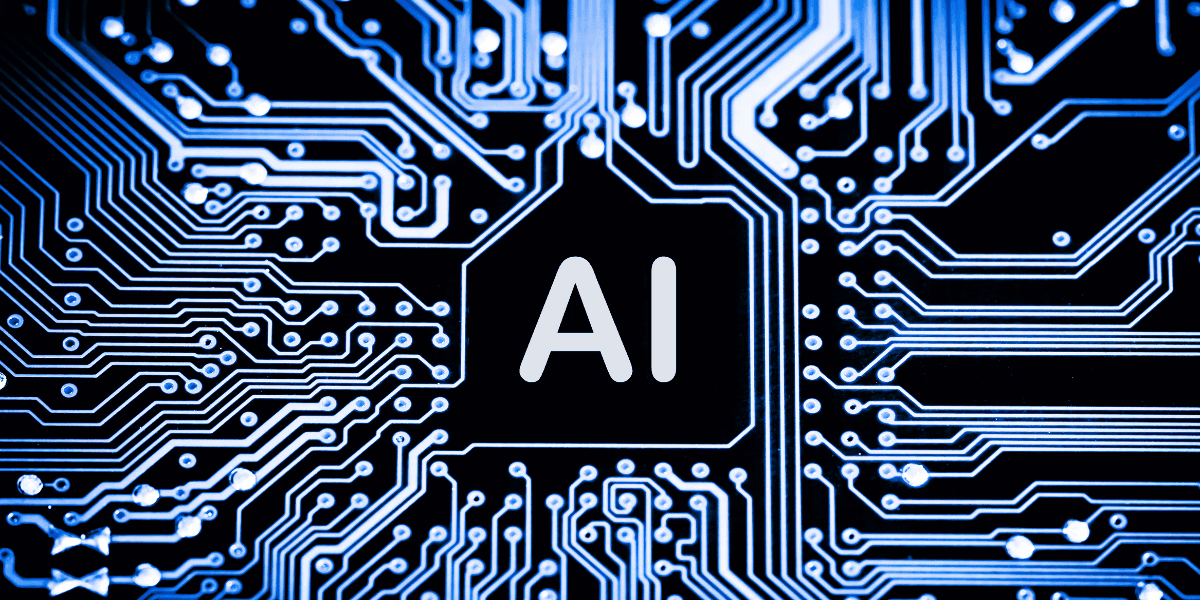
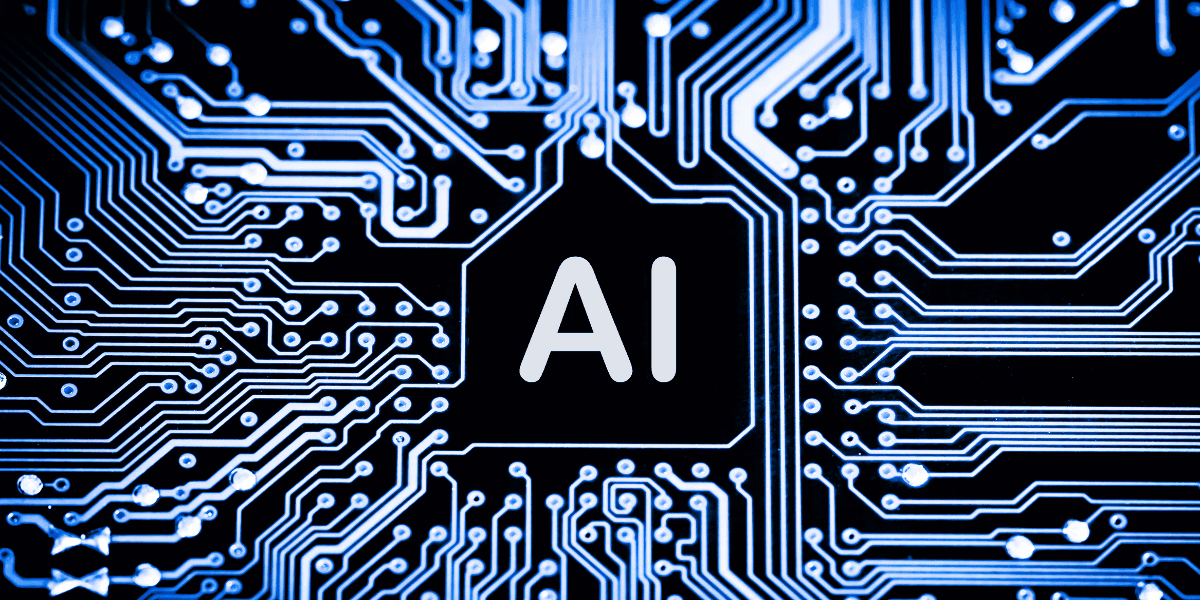
Practical Guide to Fine-Tuning OpenAI GPT Models Using Python

Rehan Asif
Apr 24, 2024
Read the article




Comparing Different Large Language Models (LLM)

Rehan Asif
Apr 23, 2024
Read the article




Evaluating Large Language Models: Methods And Metrics

Rehan Asif
Apr 22, 2024
Read the article




Significant AI Errors, Mistakes, Failures, and Flaws Companies Encounter

Akshat Gupta
Apr 21, 2024
Read the article




Challenges and Strategies for Implementing Enterprise LLM

Rehan Asif
Apr 20, 2024
Read the article




Enhancing Computer Vision with Synthetic Data: Advantages and Generation Techniques
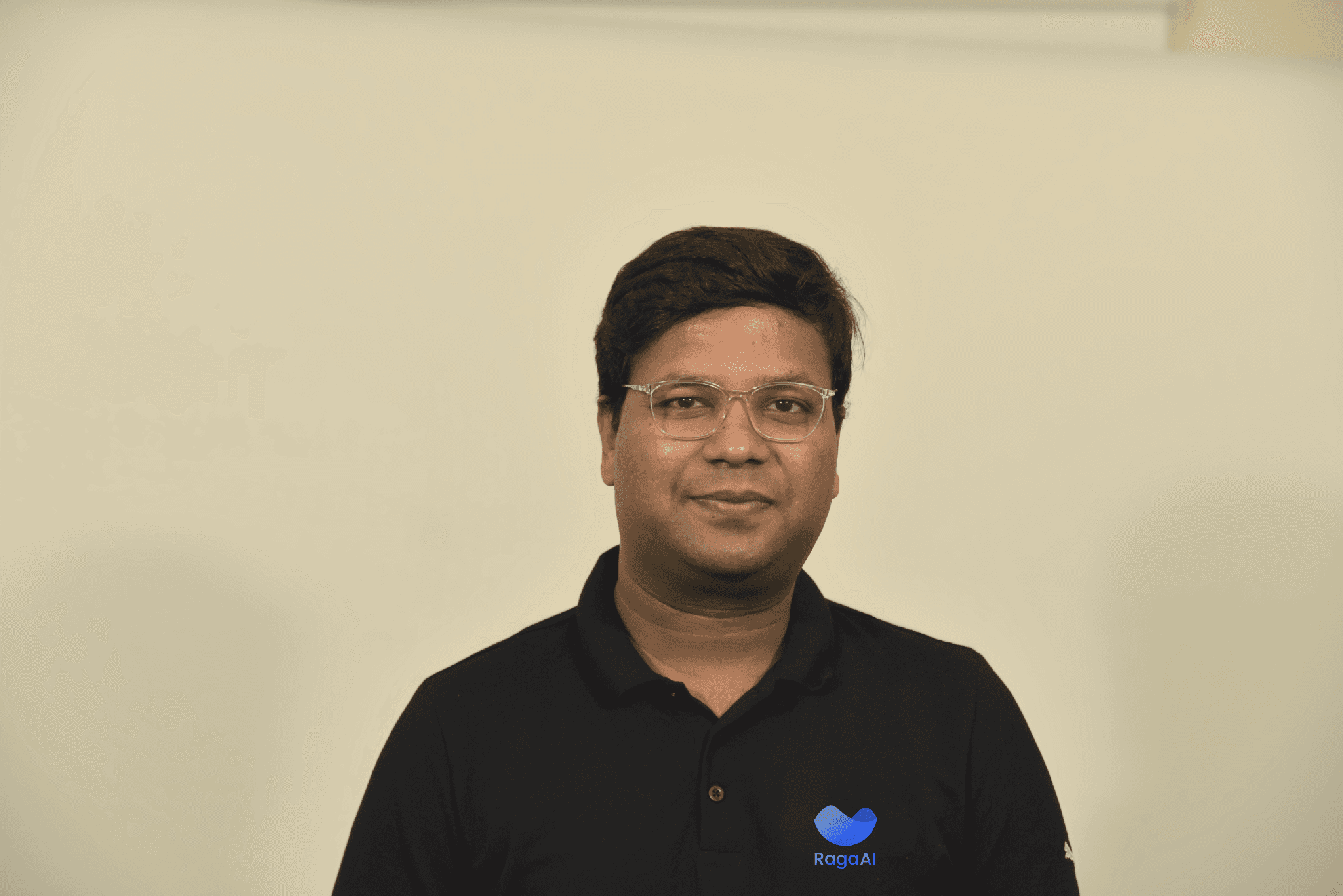
Jigar Gupta
Apr 20, 2024
Read the article
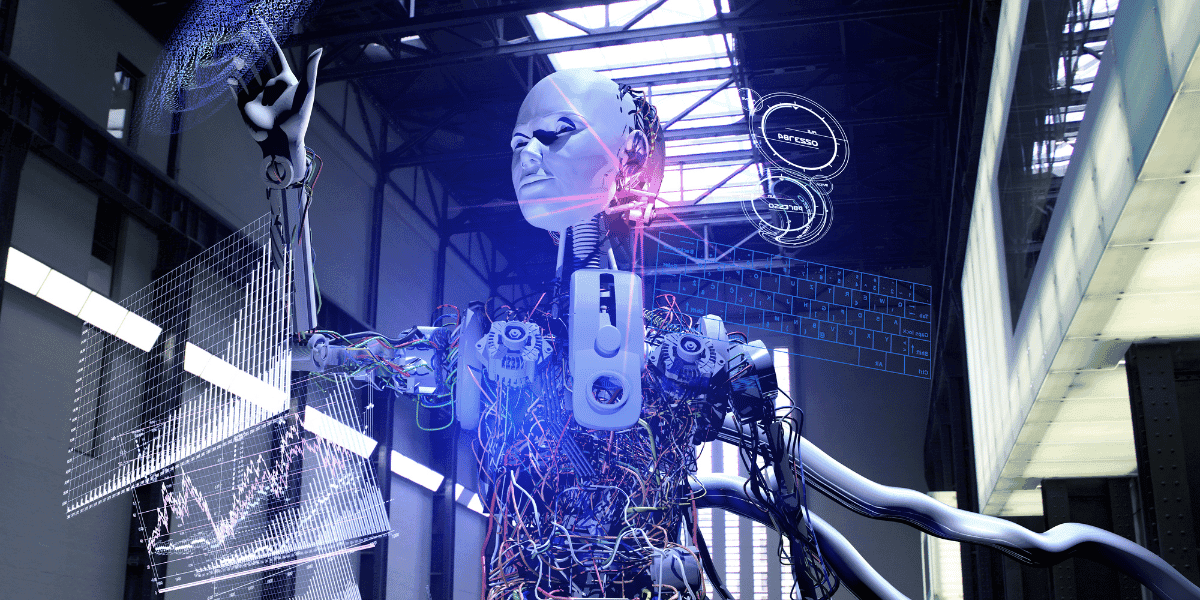
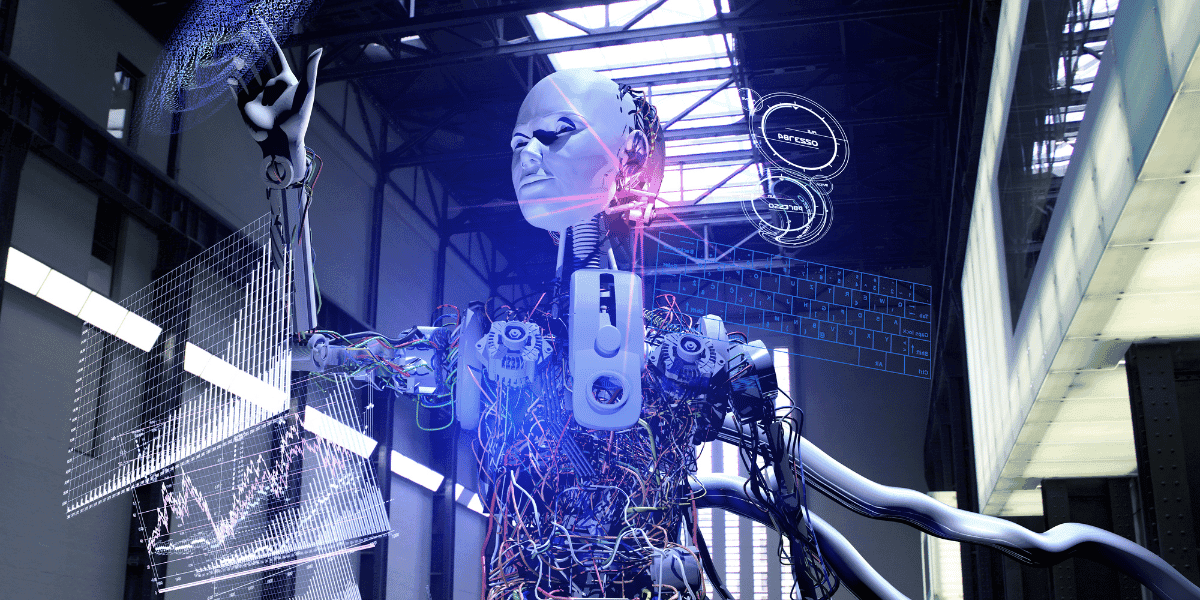
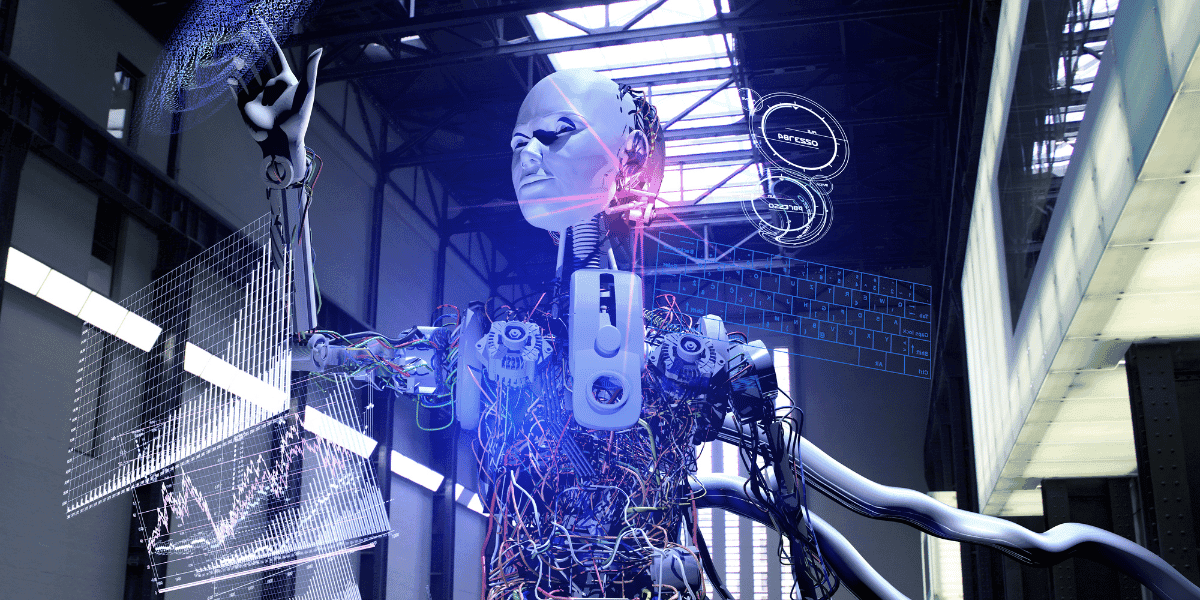
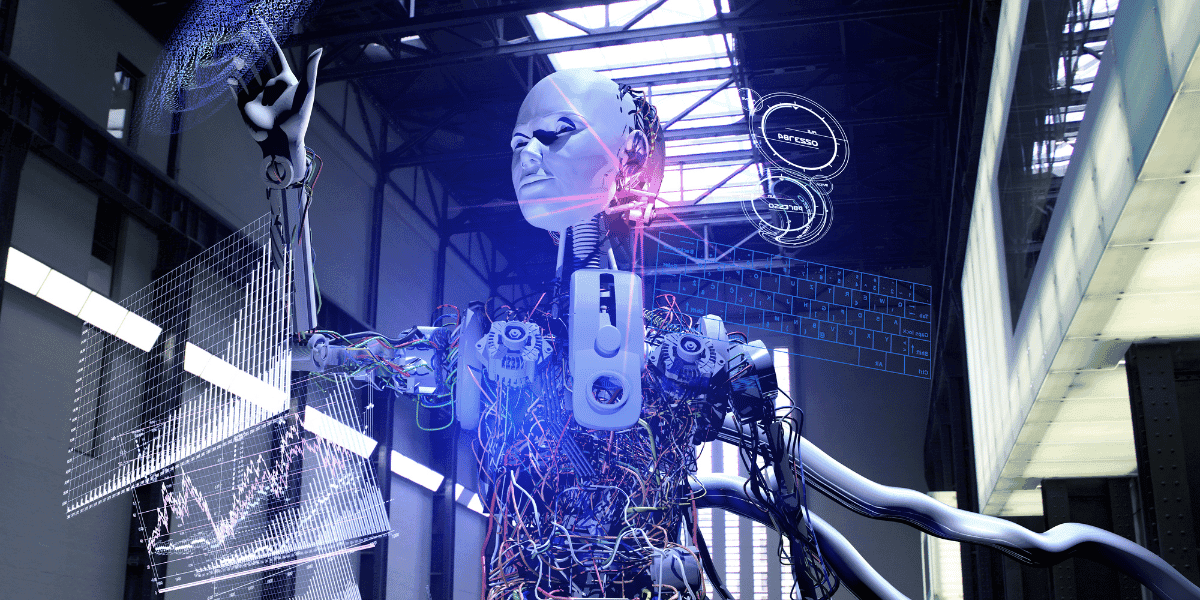
Building Trust In Artificial Intelligence Systems

Akshat Gupta
Apr 19, 2024
Read the article




A Brief Guide To LLM Parameters: Tuning and Optimization

Rehan Asif
Apr 18, 2024
Read the article




Unlocking The Potential Of Computer Vision Testing: Key Techniques And Tools
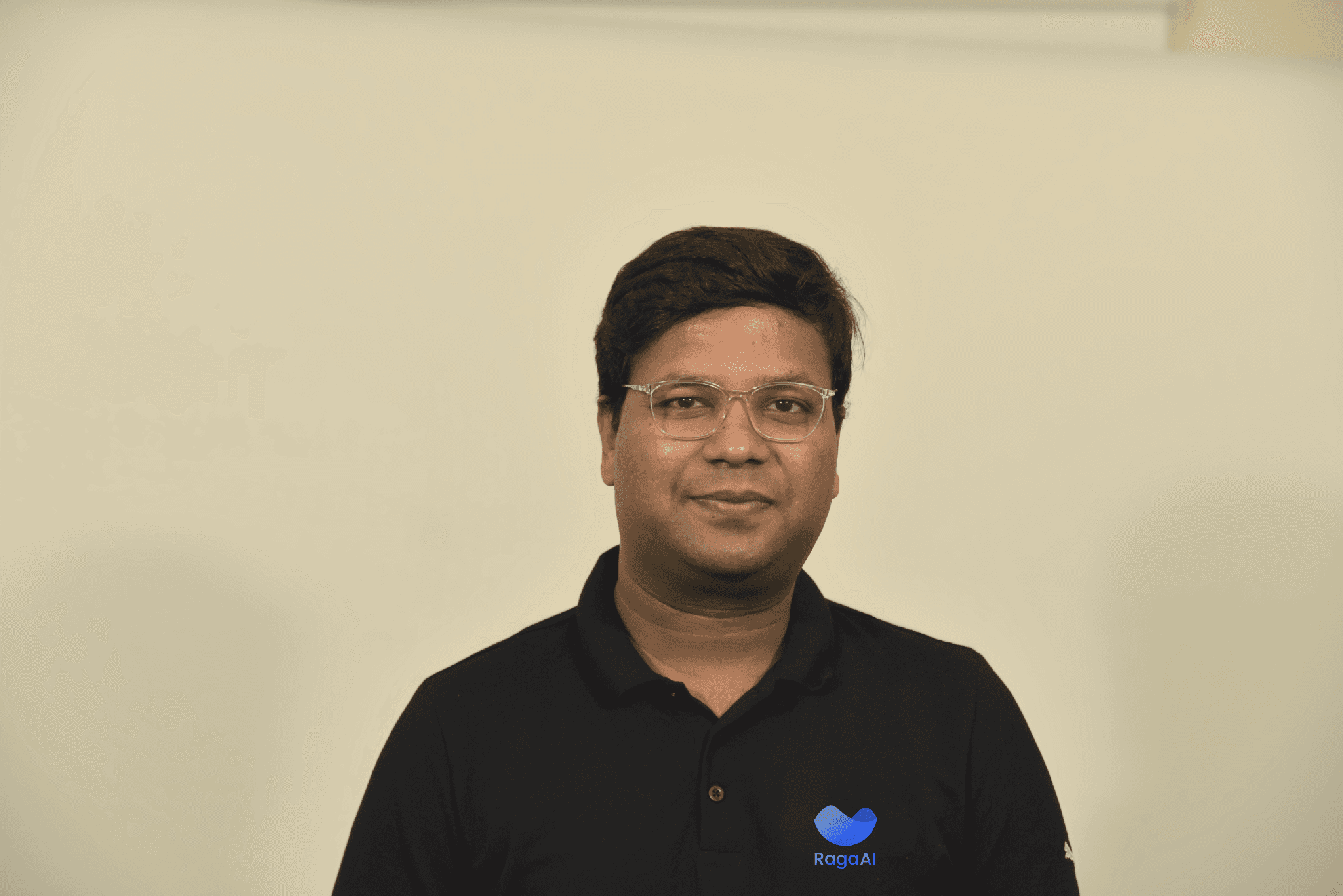
Jigar Gupta
Apr 17, 2024
Read the article




Understanding AI Regulatory Compliance And Its Importance

Akshat Gupta
Apr 16, 2024
Read the article
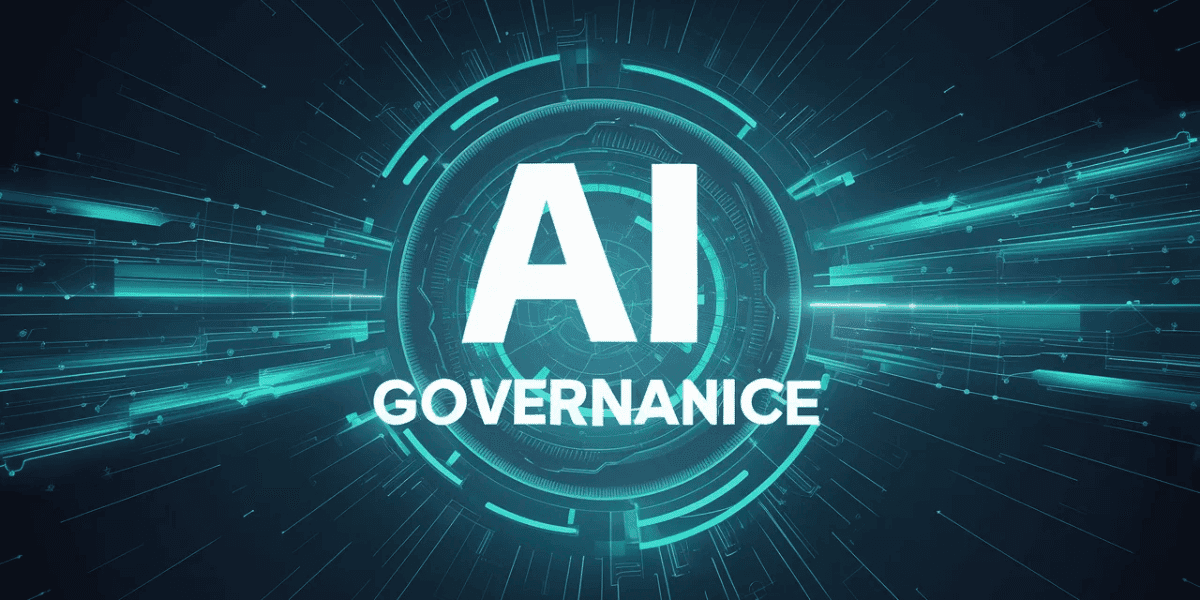
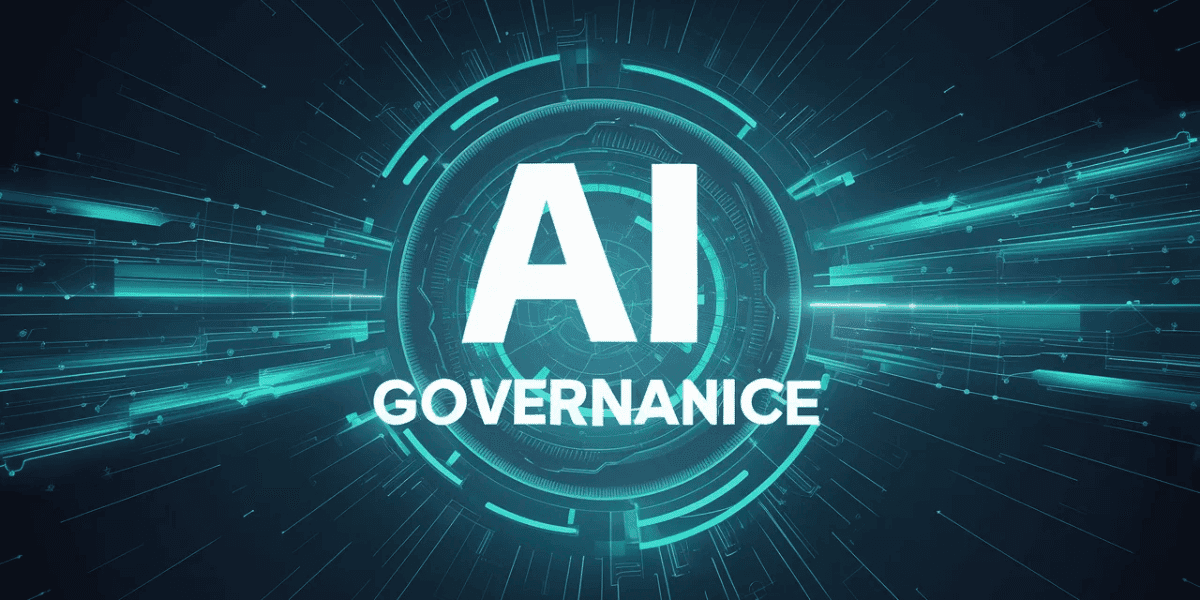
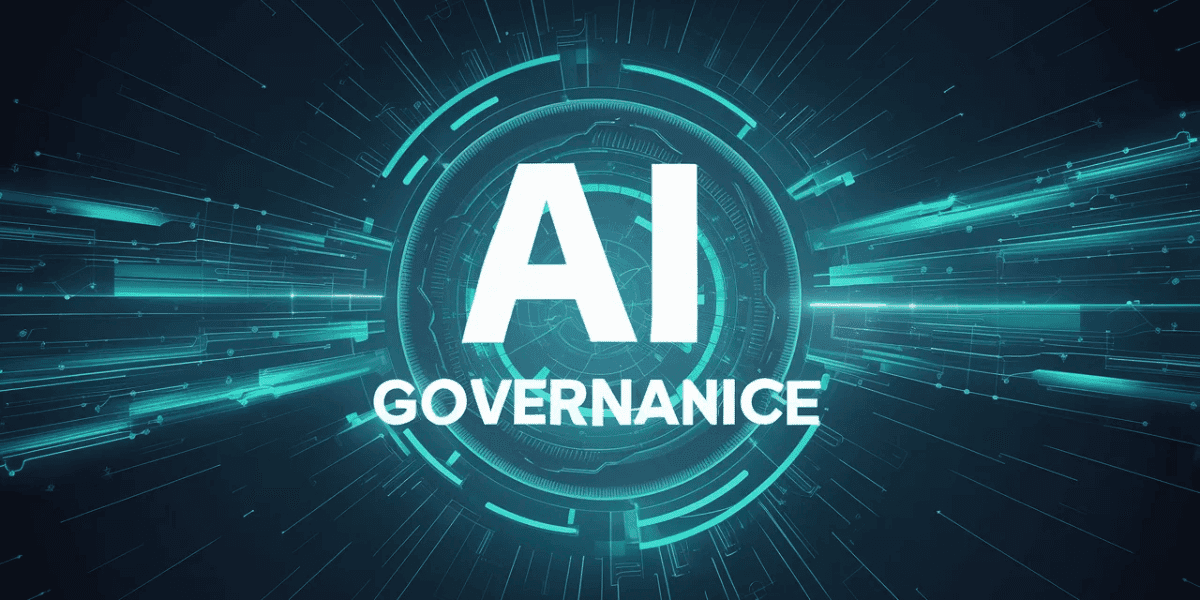
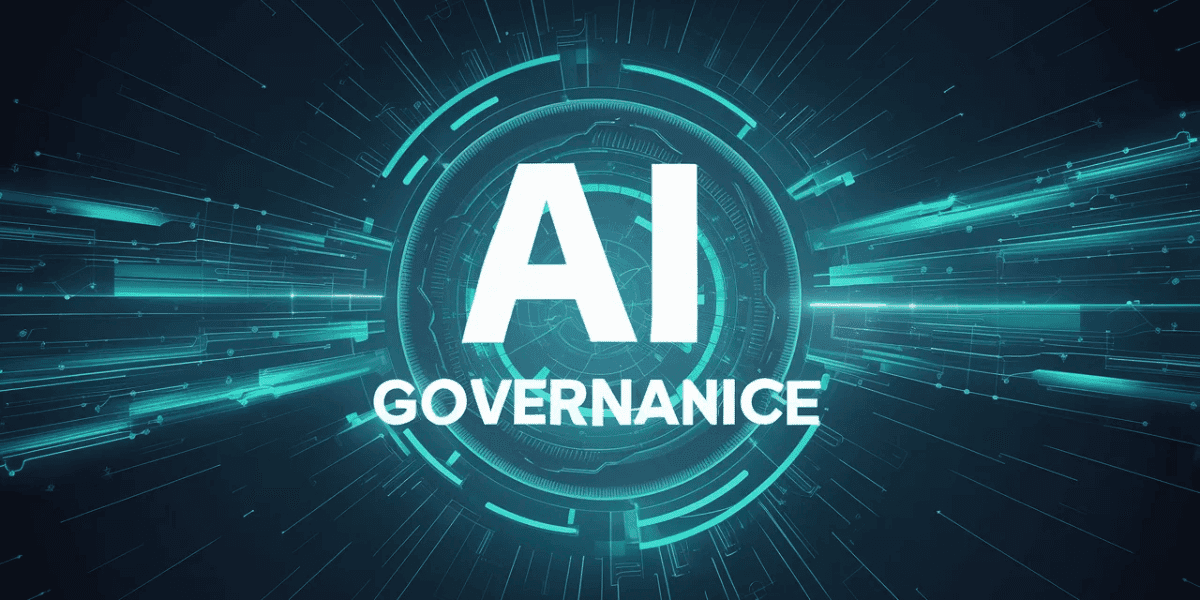
Understanding The Basics Of AI Governance

Akshat Gupta
Apr 15, 2024
Read the article




Understanding Prompt Engineering: A Guide

Rehan Asif
Apr 15, 2024
Read the article
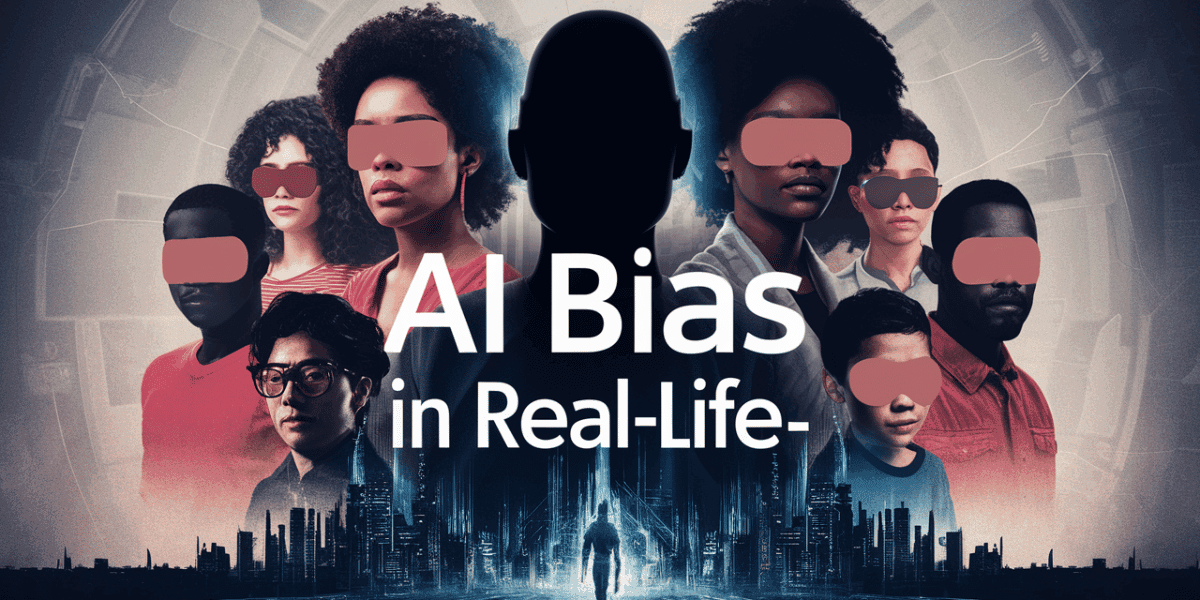
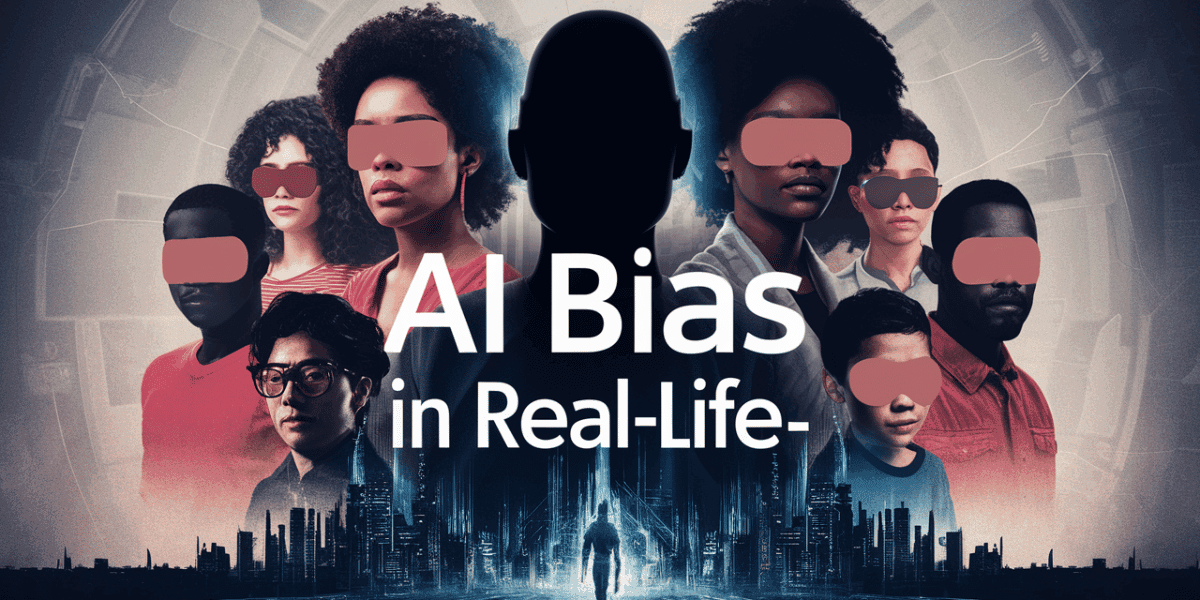
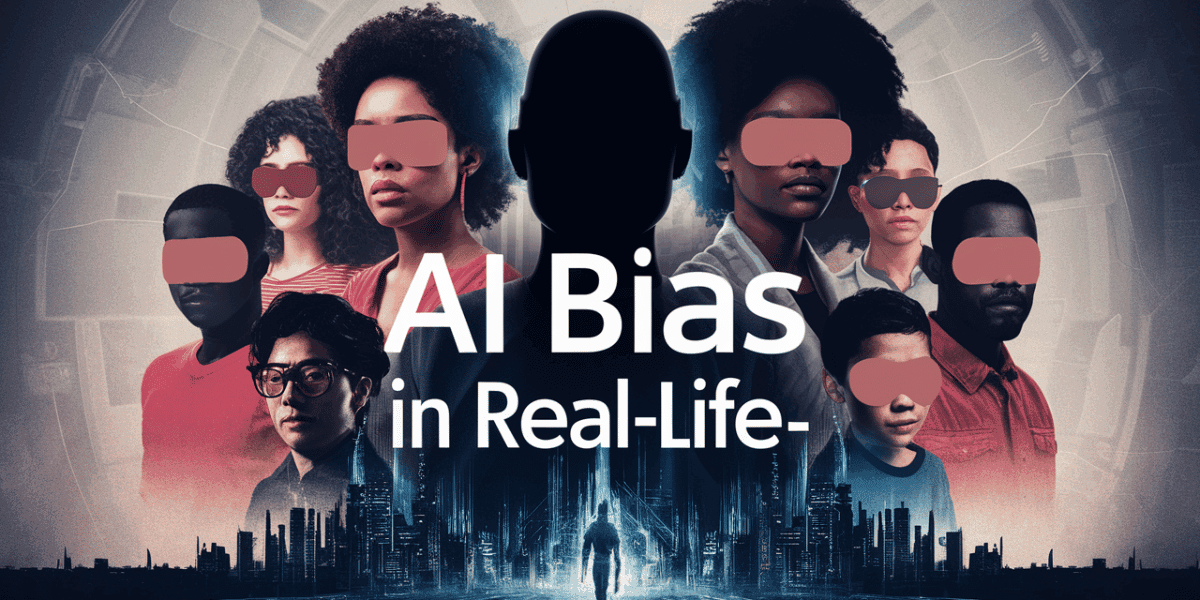
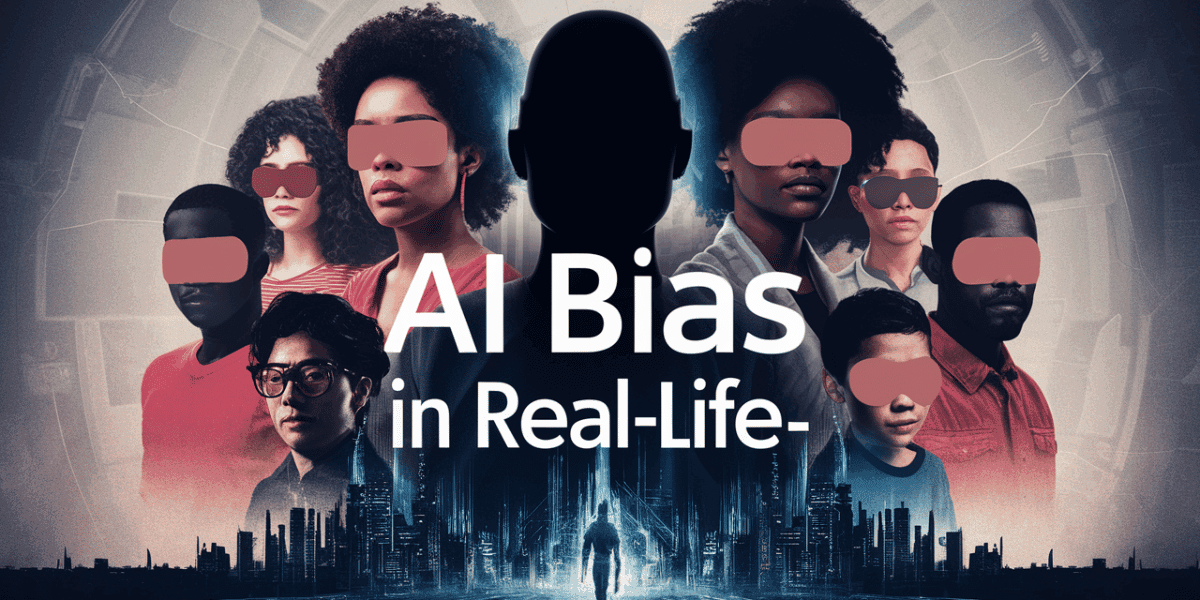
Examples And Strategies To Mitigate AI Bias In Real-Life

Akshat Gupta
Apr 14, 2024
Read the article




Understanding The Basics Of LLM Fine-tuning With Custom Data

Rehan Asif
Apr 13, 2024
Read the article
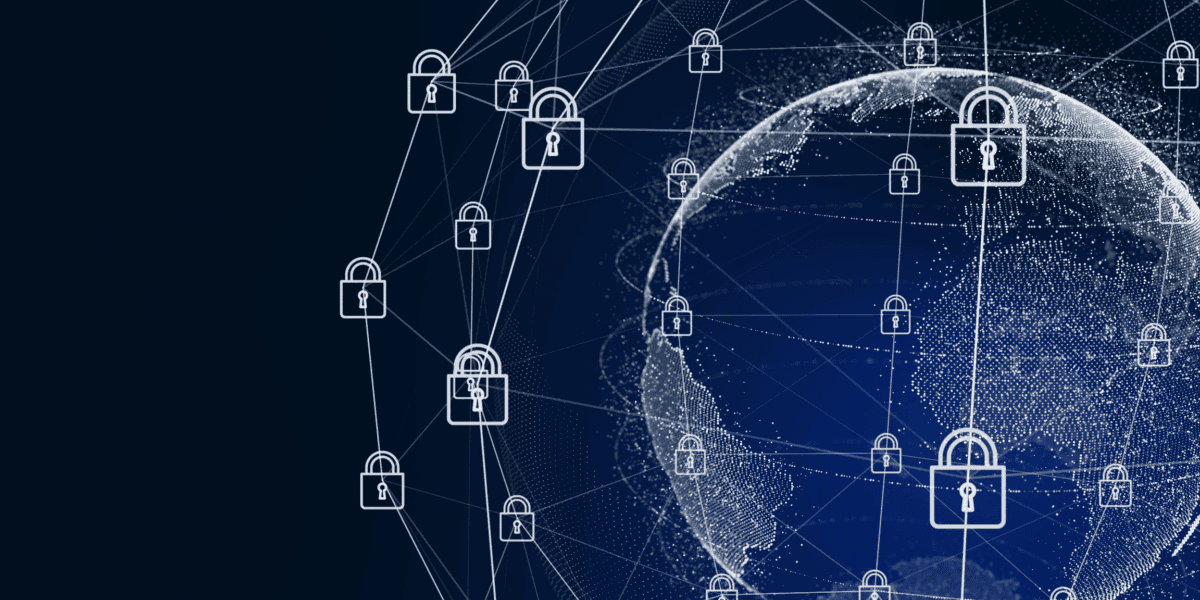
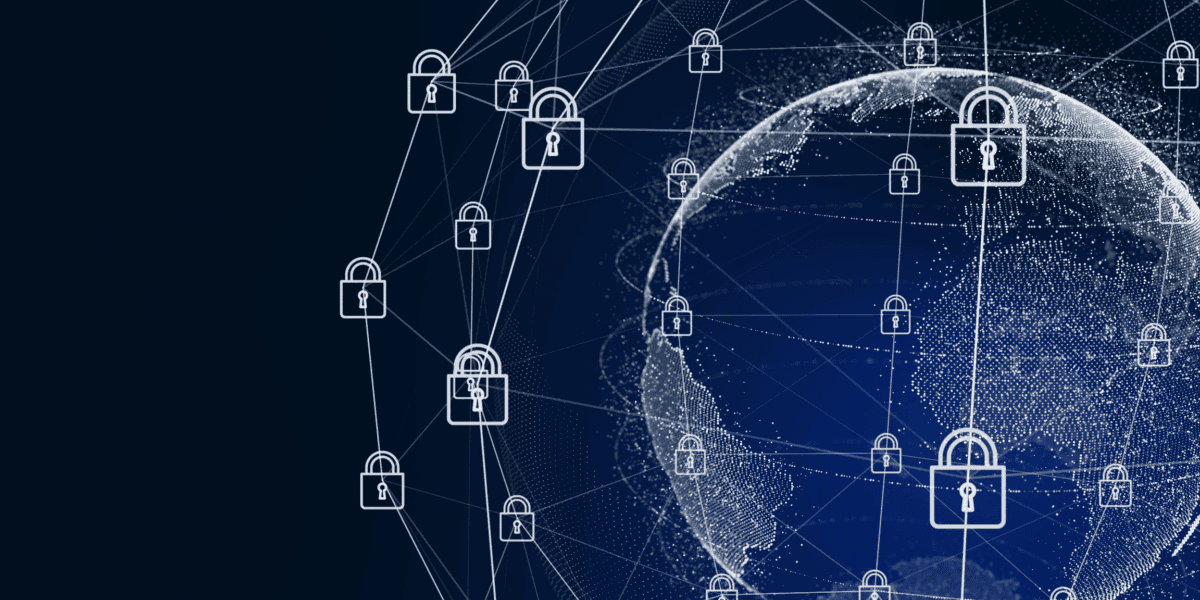
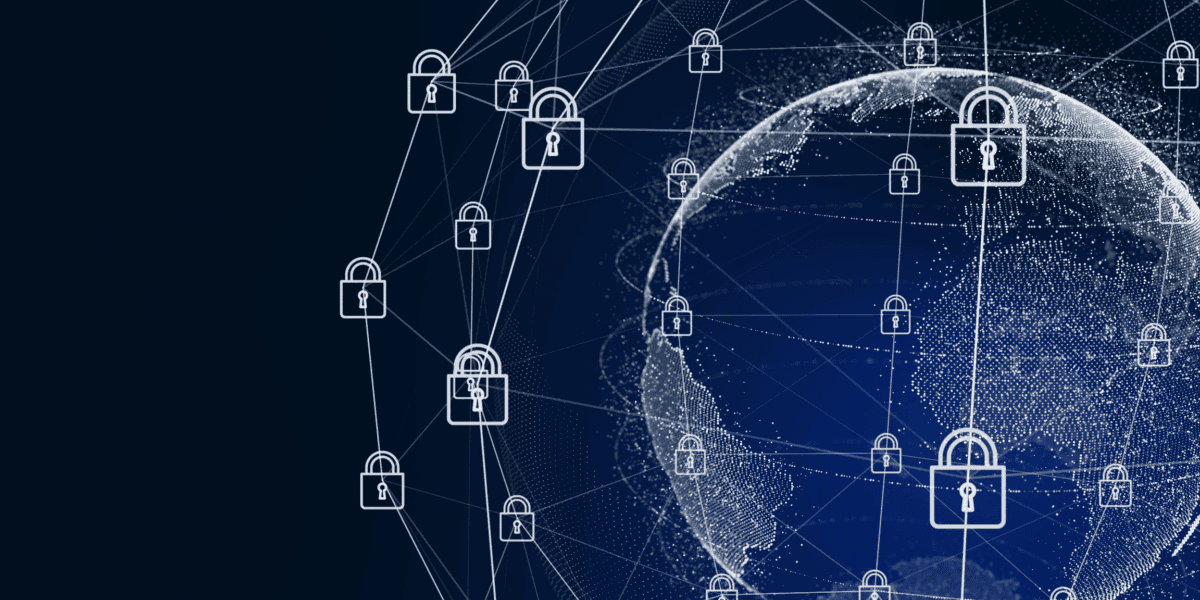
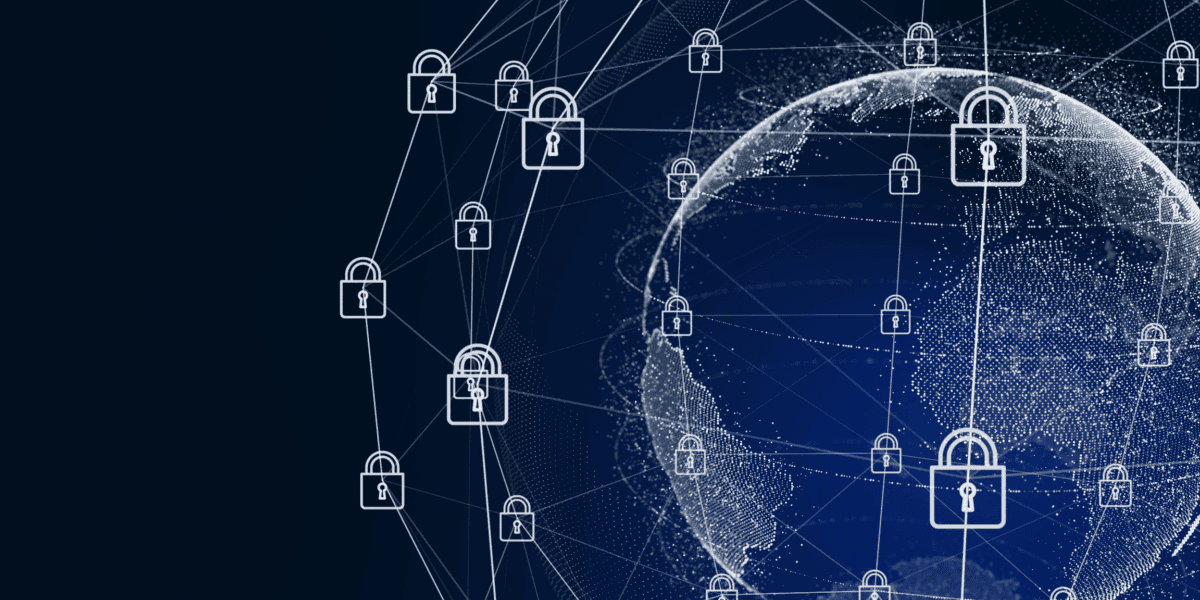
Overview Of Key Concepts In AI Safety And Security
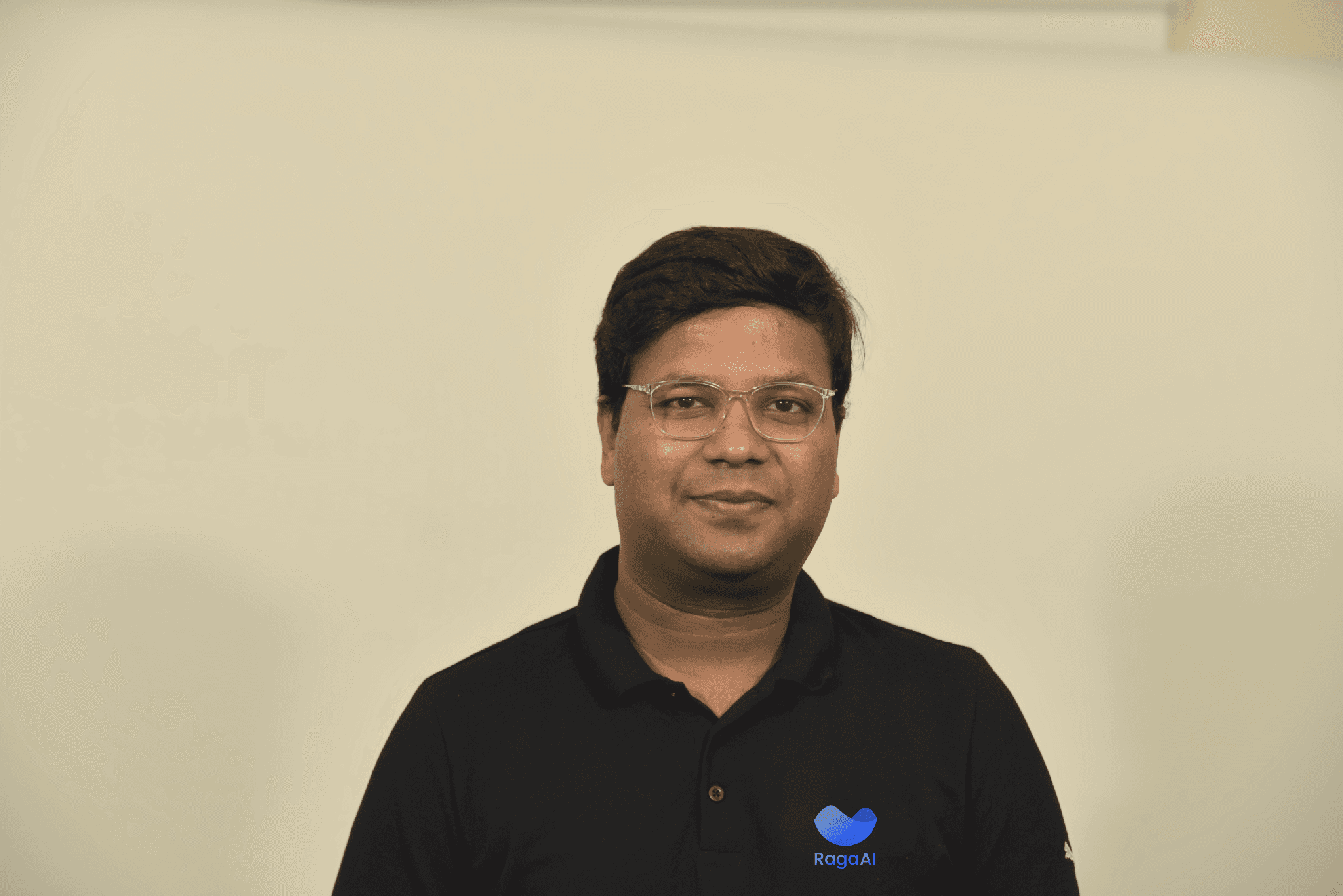
Jigar Gupta
Apr 12, 2024
Read the article




Understanding Hallucinations In LLMs

Rehan Asif
Apr 7, 2024
Read the article




Demystifying FDA's Approach to AI/ML in Healthcare: Your Ultimate Guide
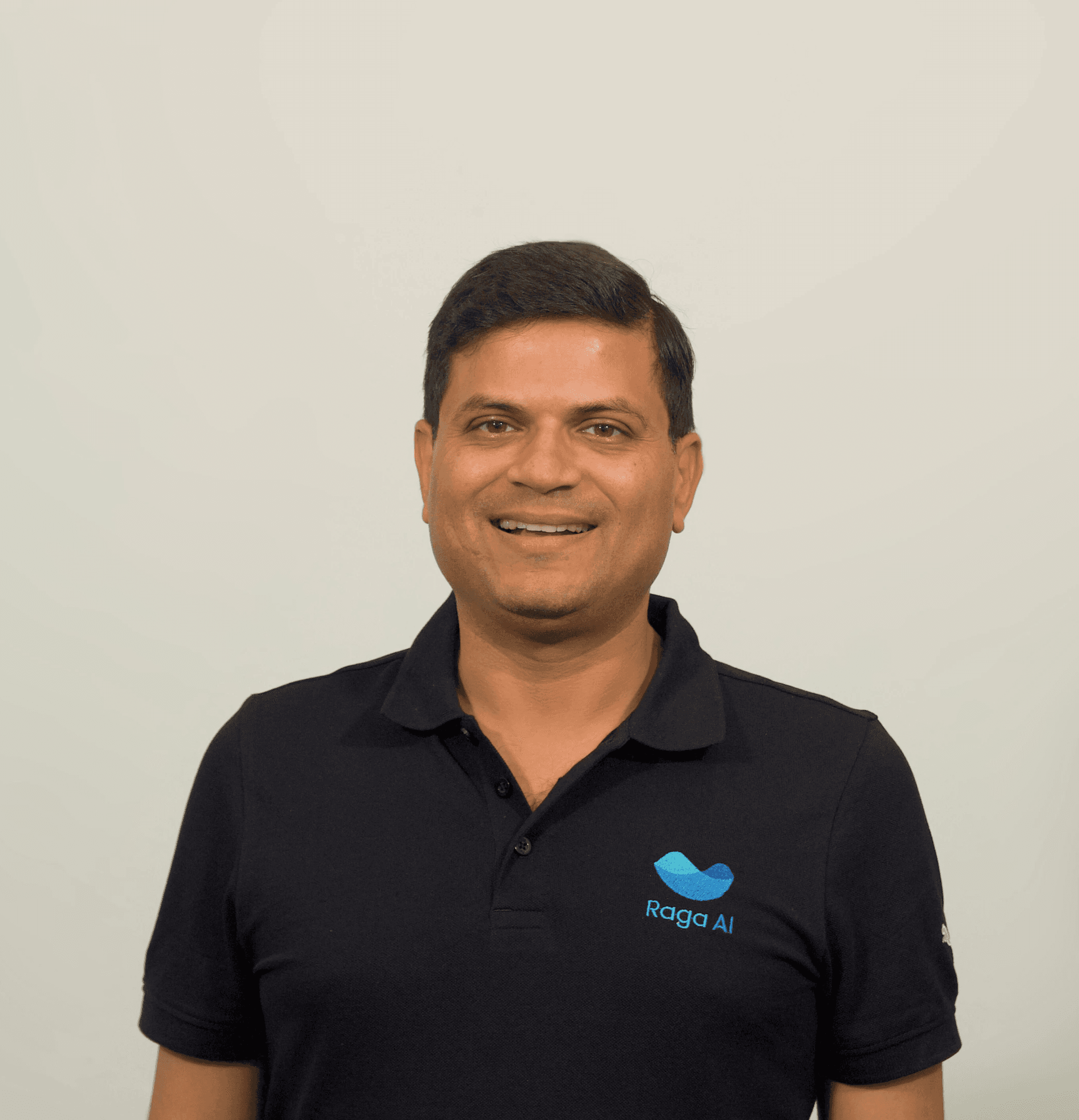
Gaurav Agarwal
Apr 4, 2024
Read the article




Navigating AI Governance in Aerospace Industry

Akshat Gupta
Apr 3, 2024
Read the article




The White House Executive Order on Safe and Trustworthy AI

Jigar Gupta
Mar 29, 2024
Read the article




The EU AI Act - All you need to know
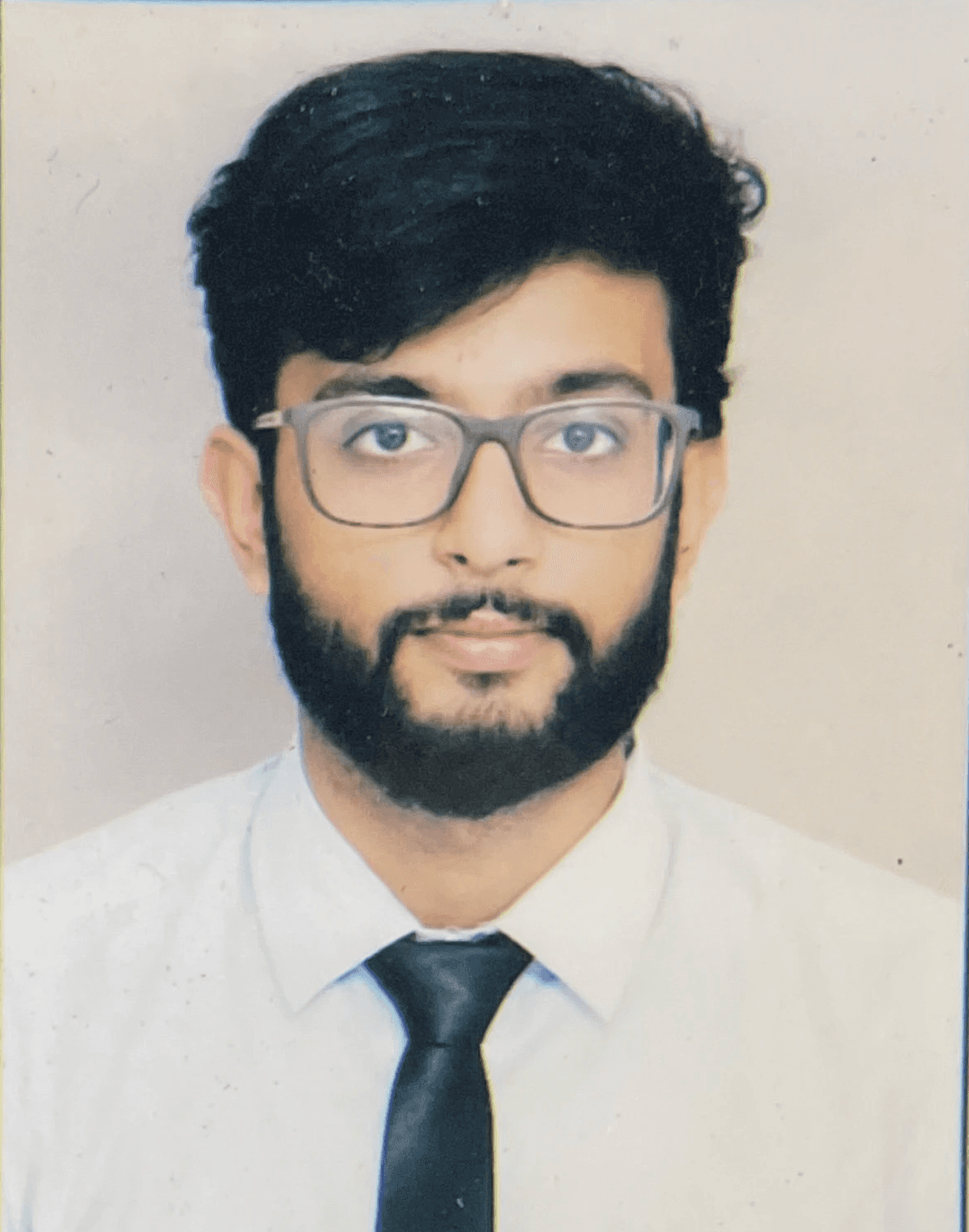
Akshat Gupta
Mar 27, 2024
Read the article
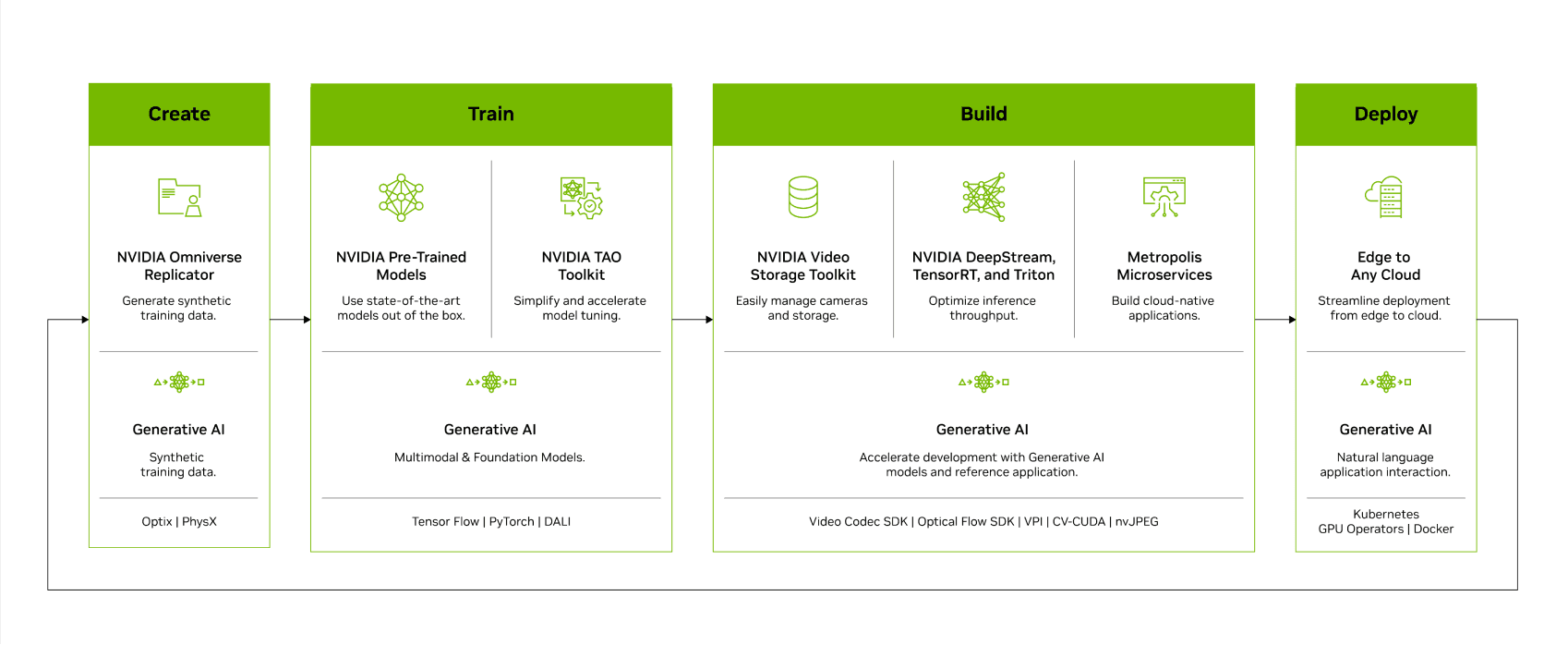
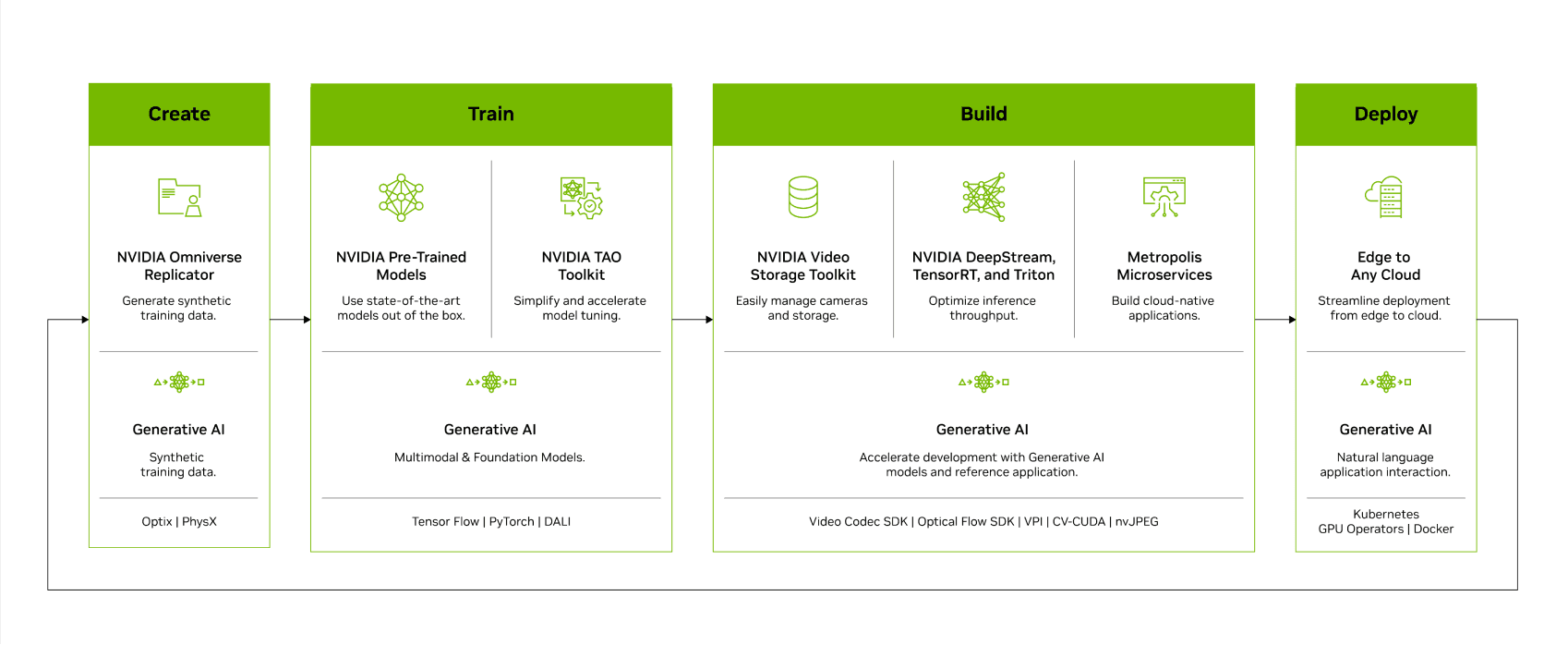
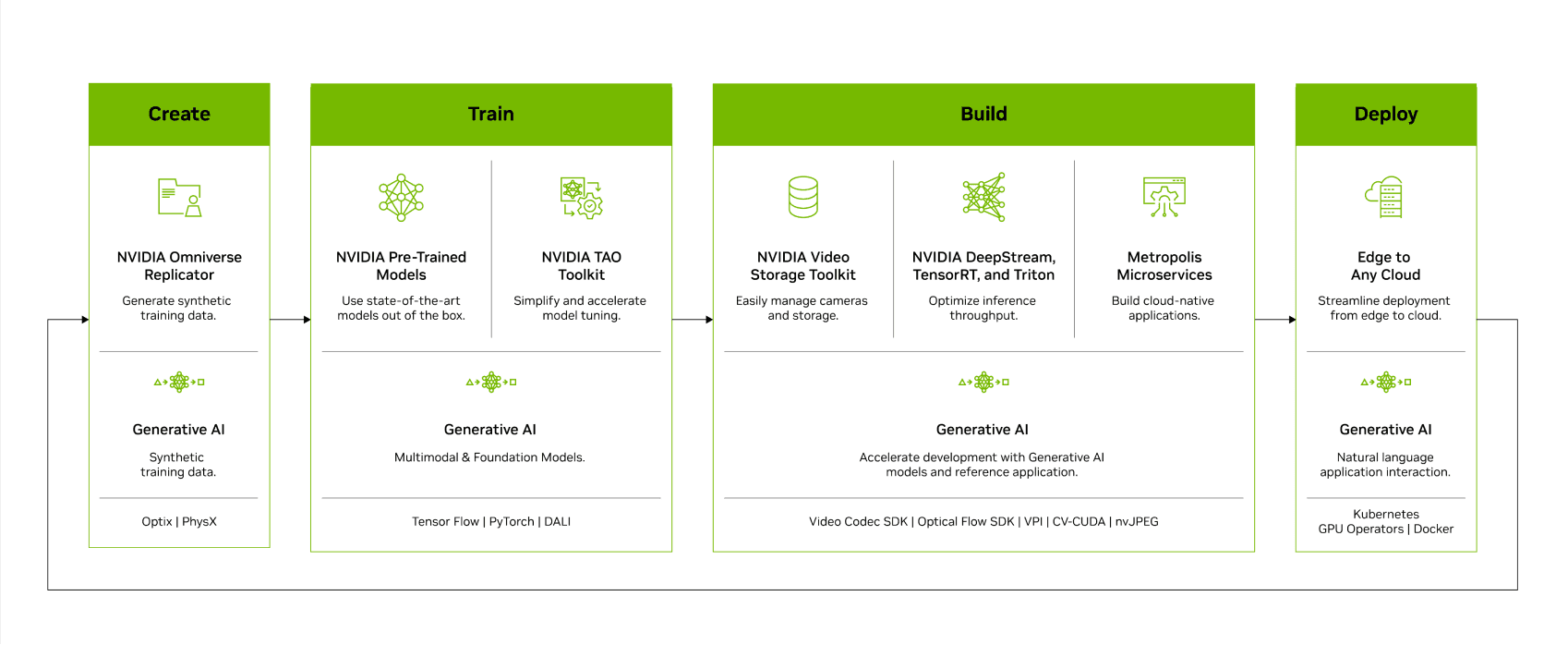
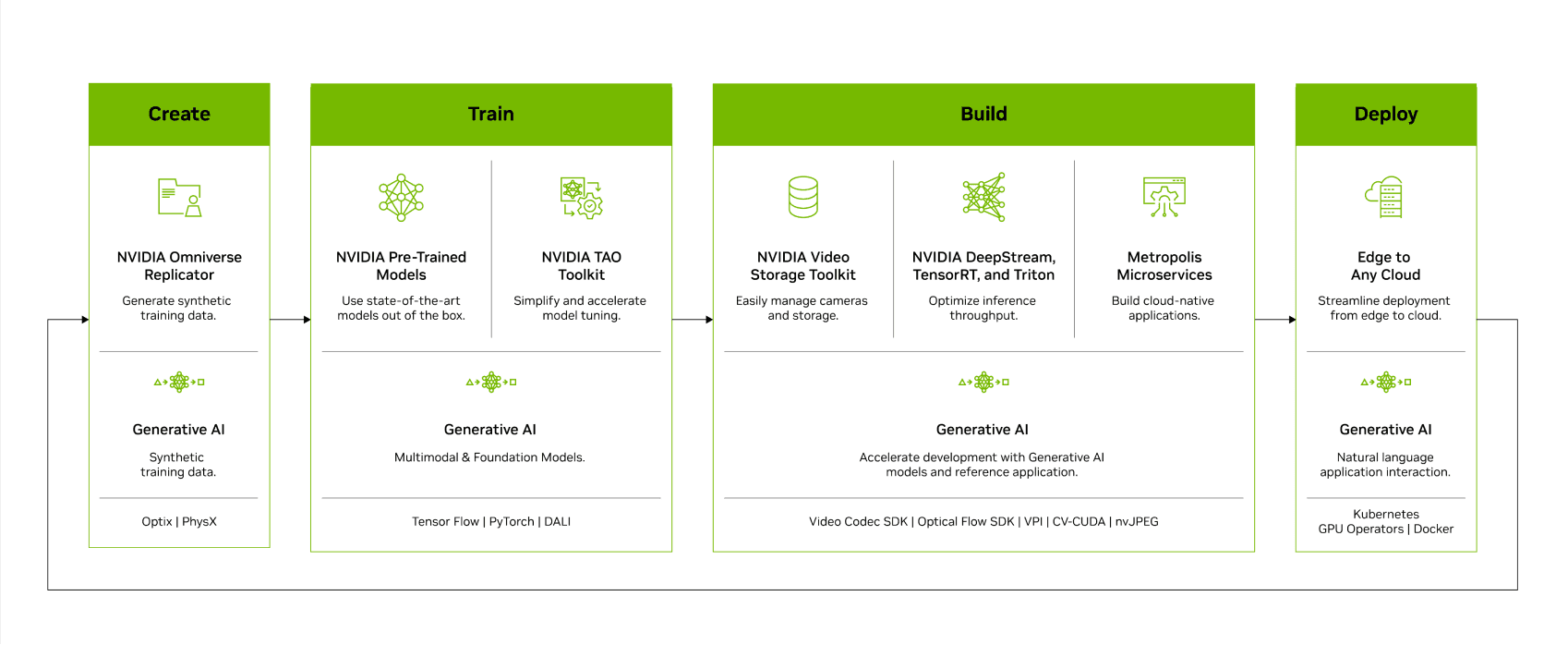
Enhancing Edge AI with RagaAI Integration on NVIDIA Metropolis

Siddharth Jain
Mar 15, 2024
Read the article




RagaAI releases the most comprehensive open-source LLM Evaluation and Guardrails package

Gaurav Agarwal
Mar 7, 2024
Read the article
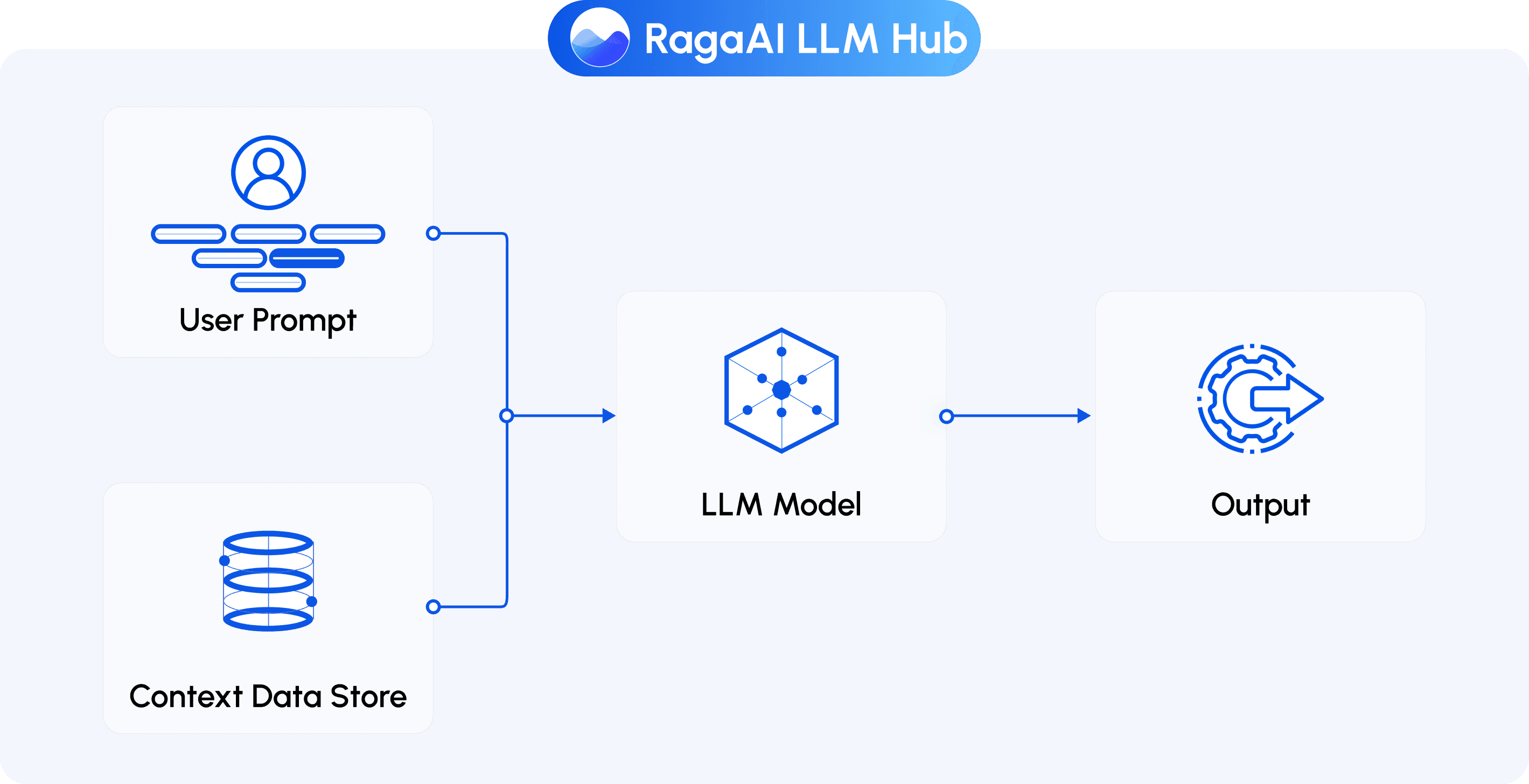
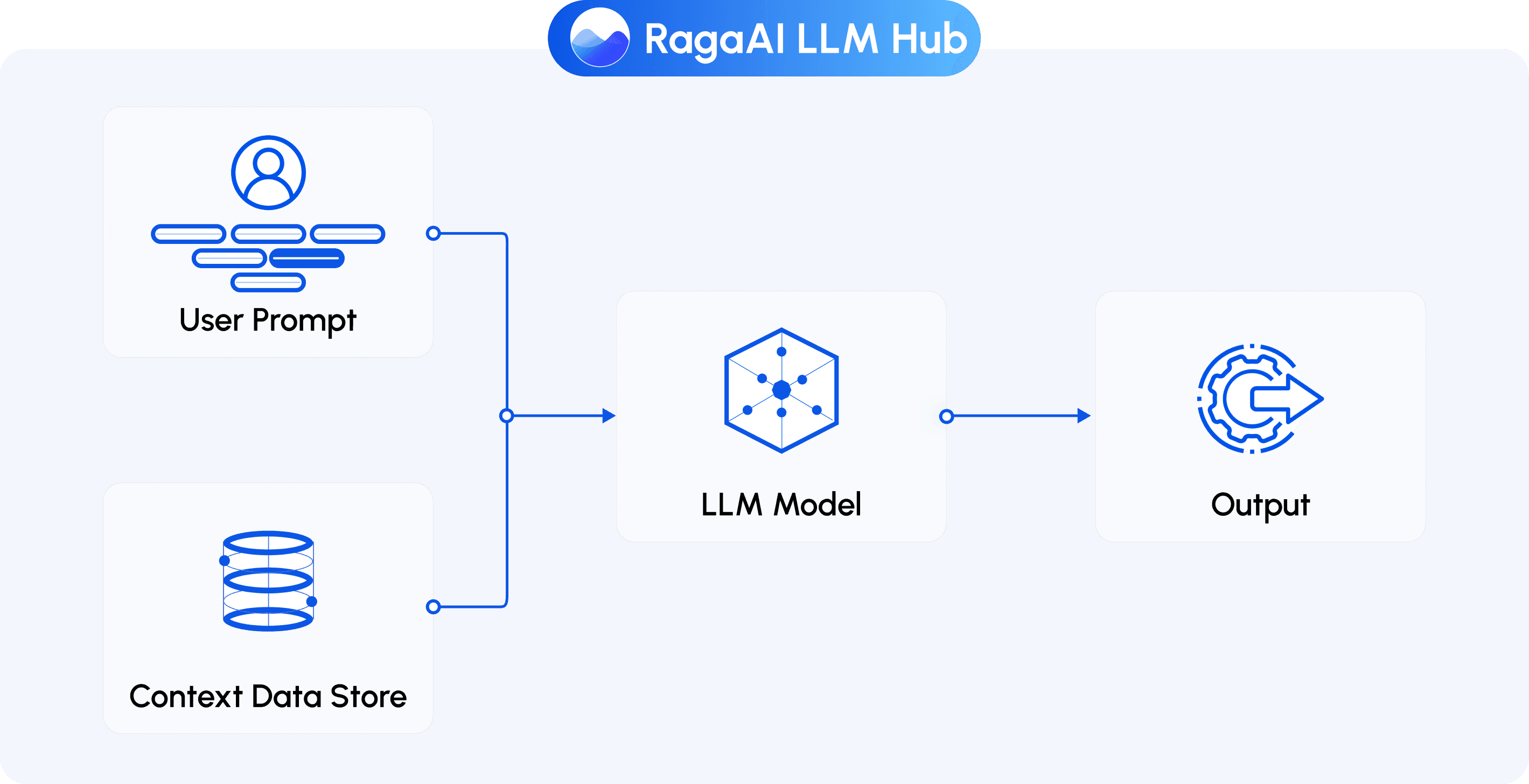
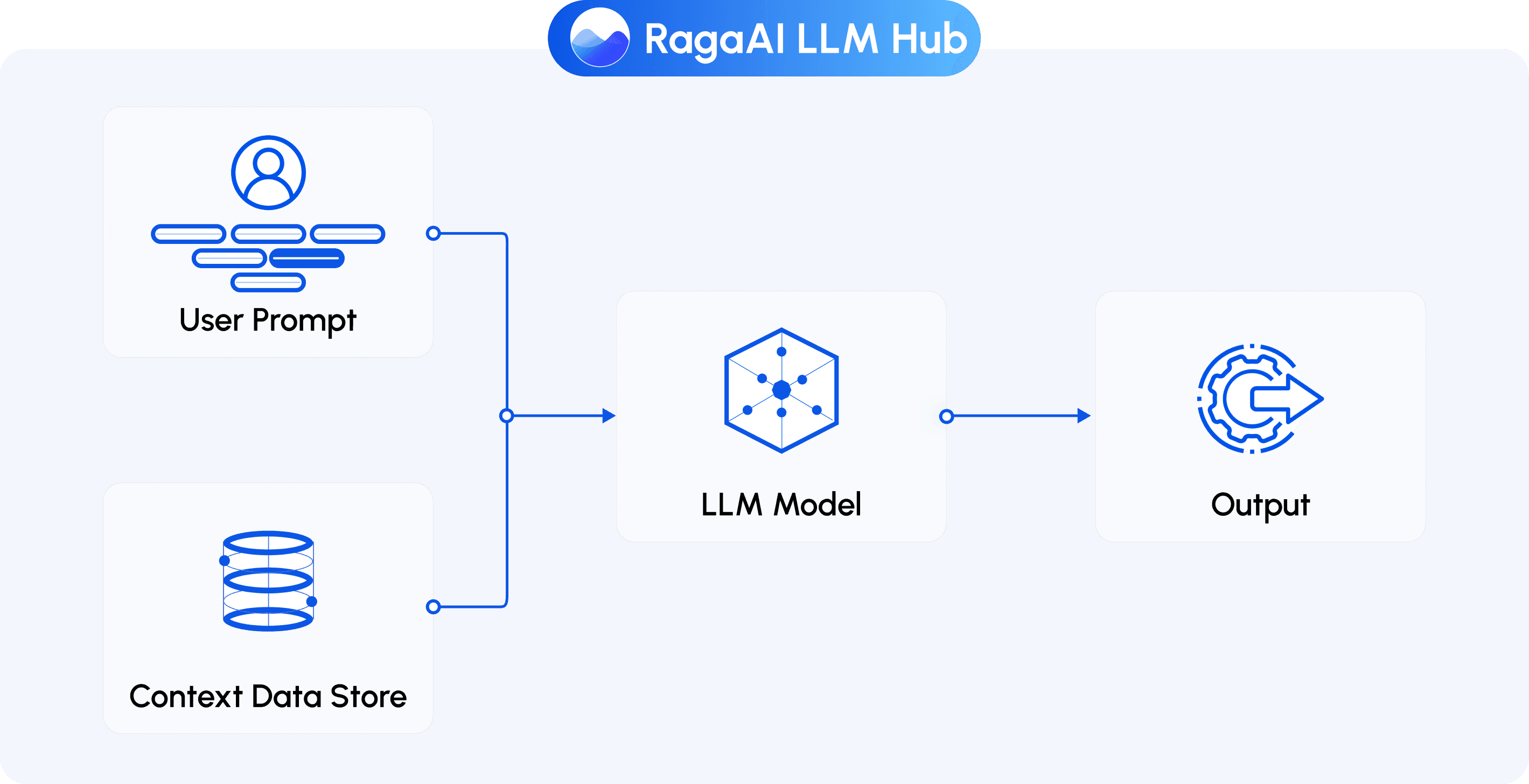
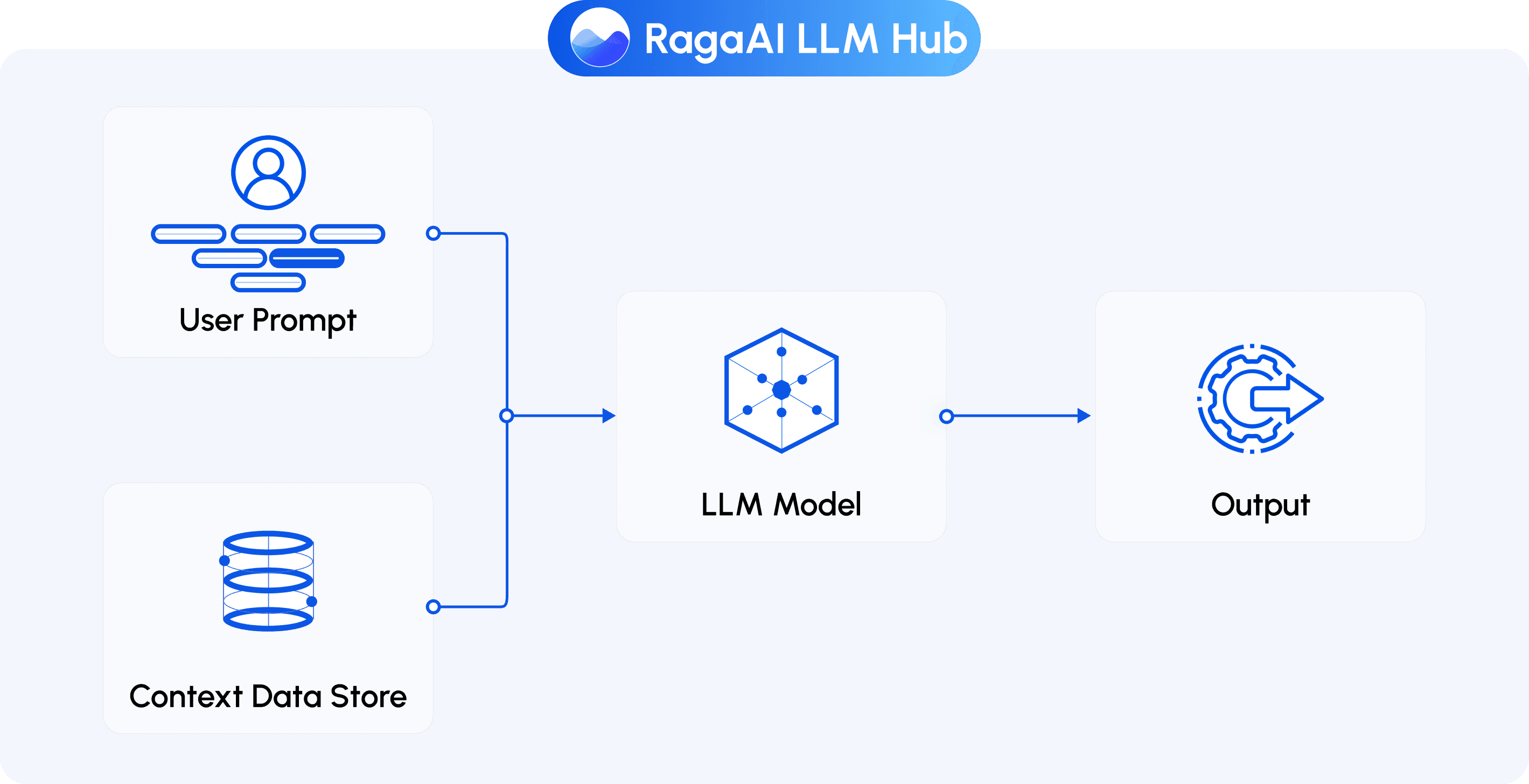
A Guide to Evaluating LLM Applications and enabling Guardrails using Raga-LLM-Hub

Rehan Asif
Mar 7, 2024
Read the article




Identifying edge cases within CelebA Dataset using RagaAI testing Platform

Rehan Asif
Feb 15, 2024
Read the article
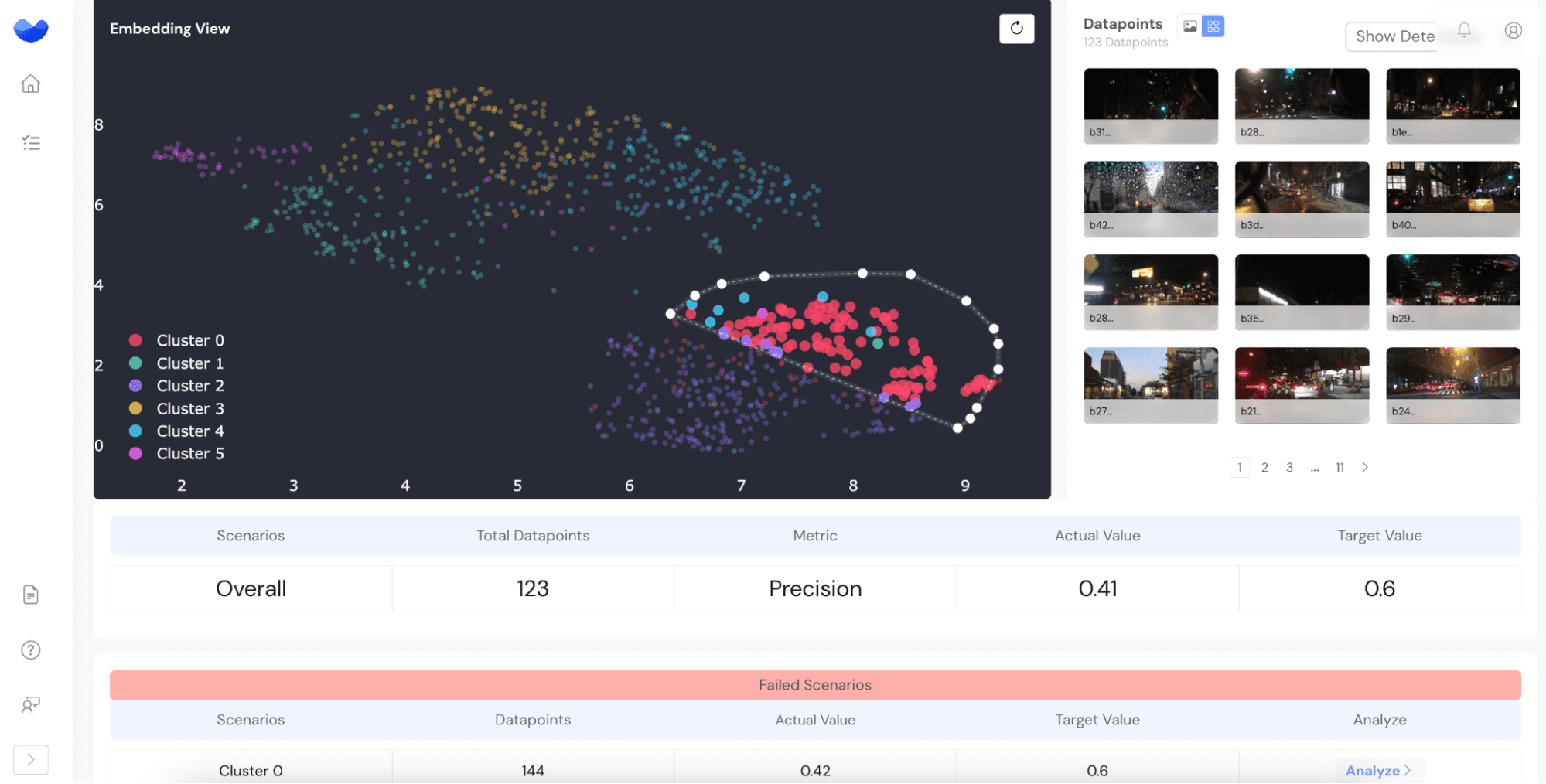
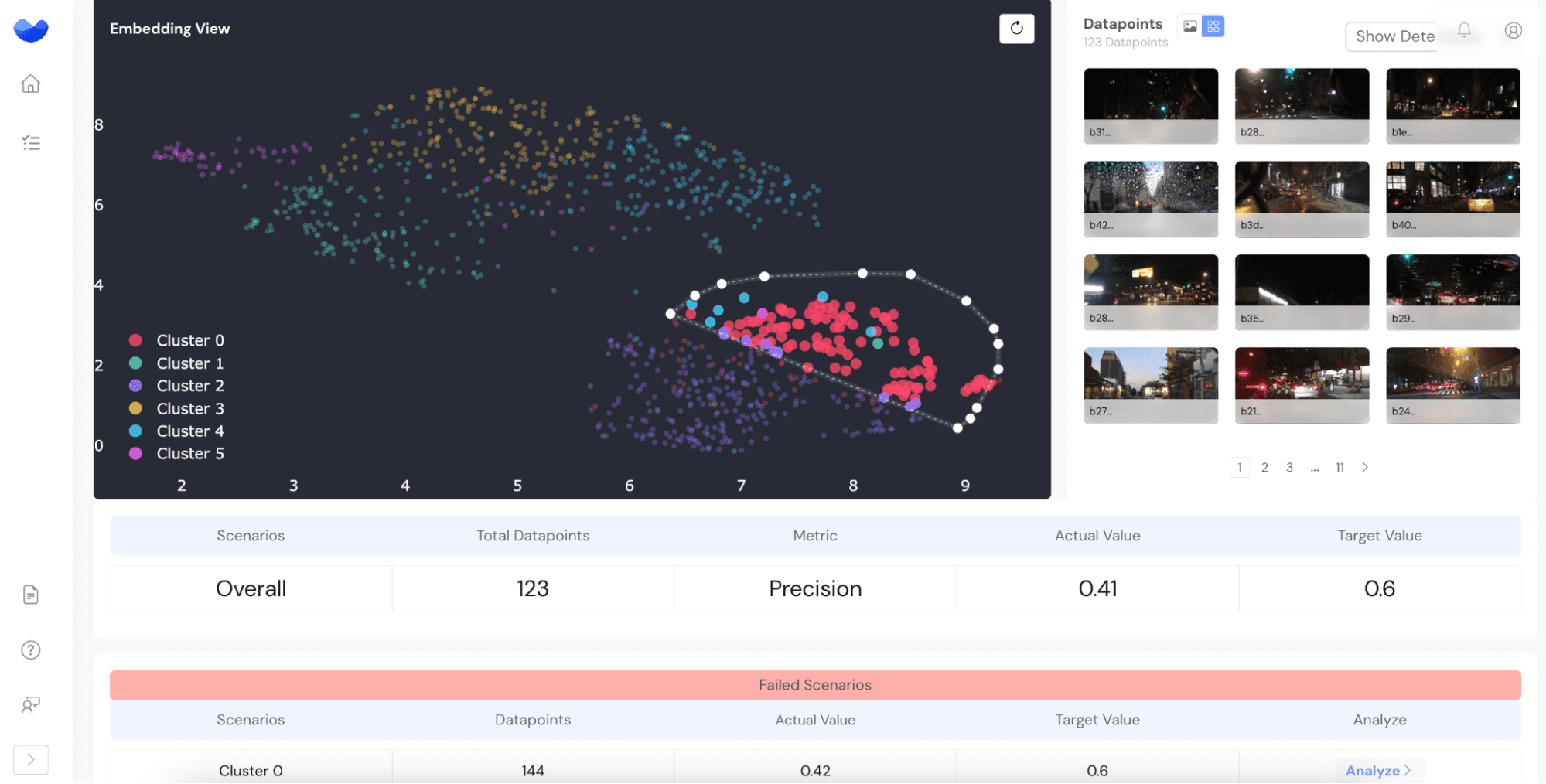
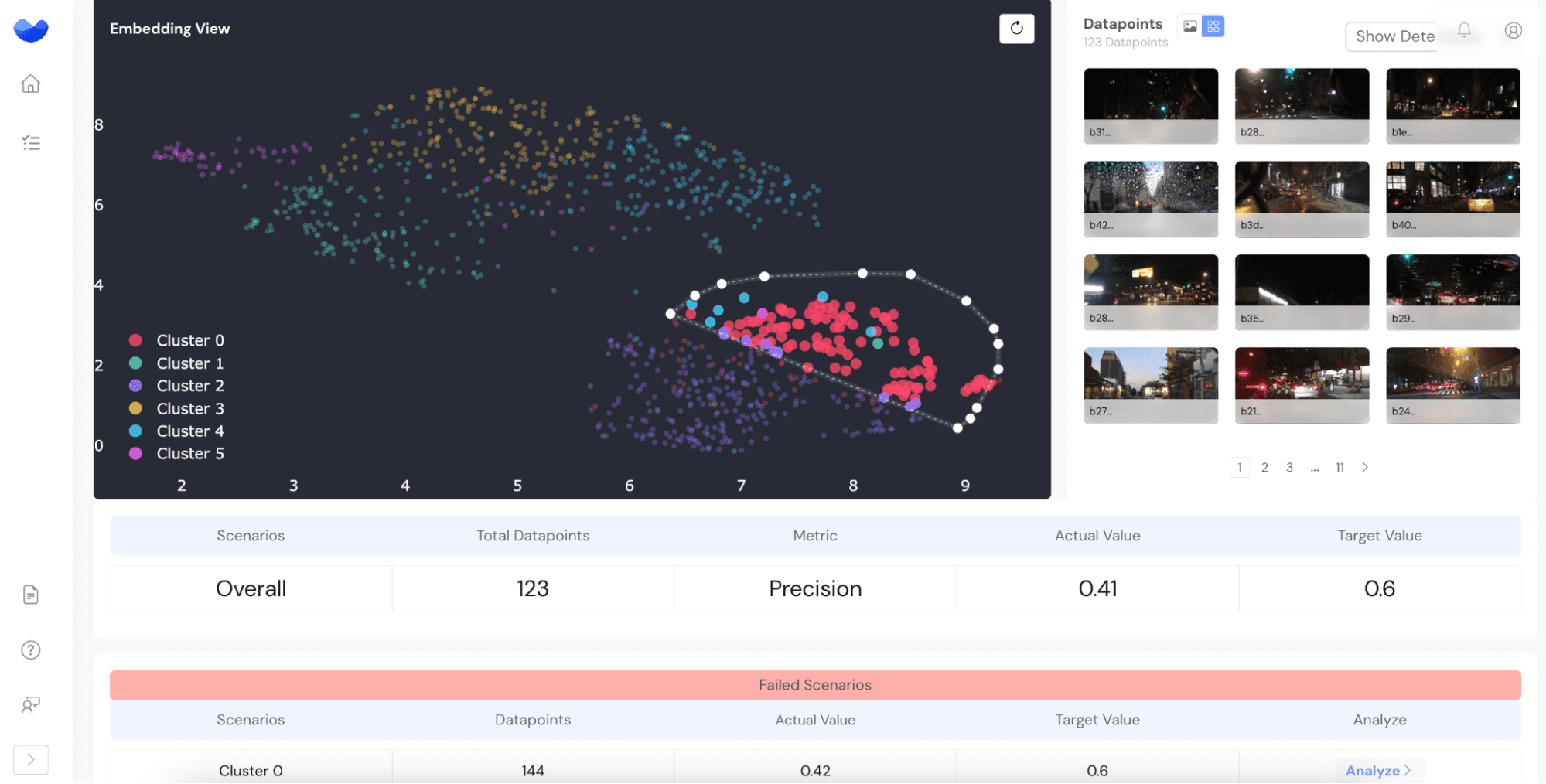
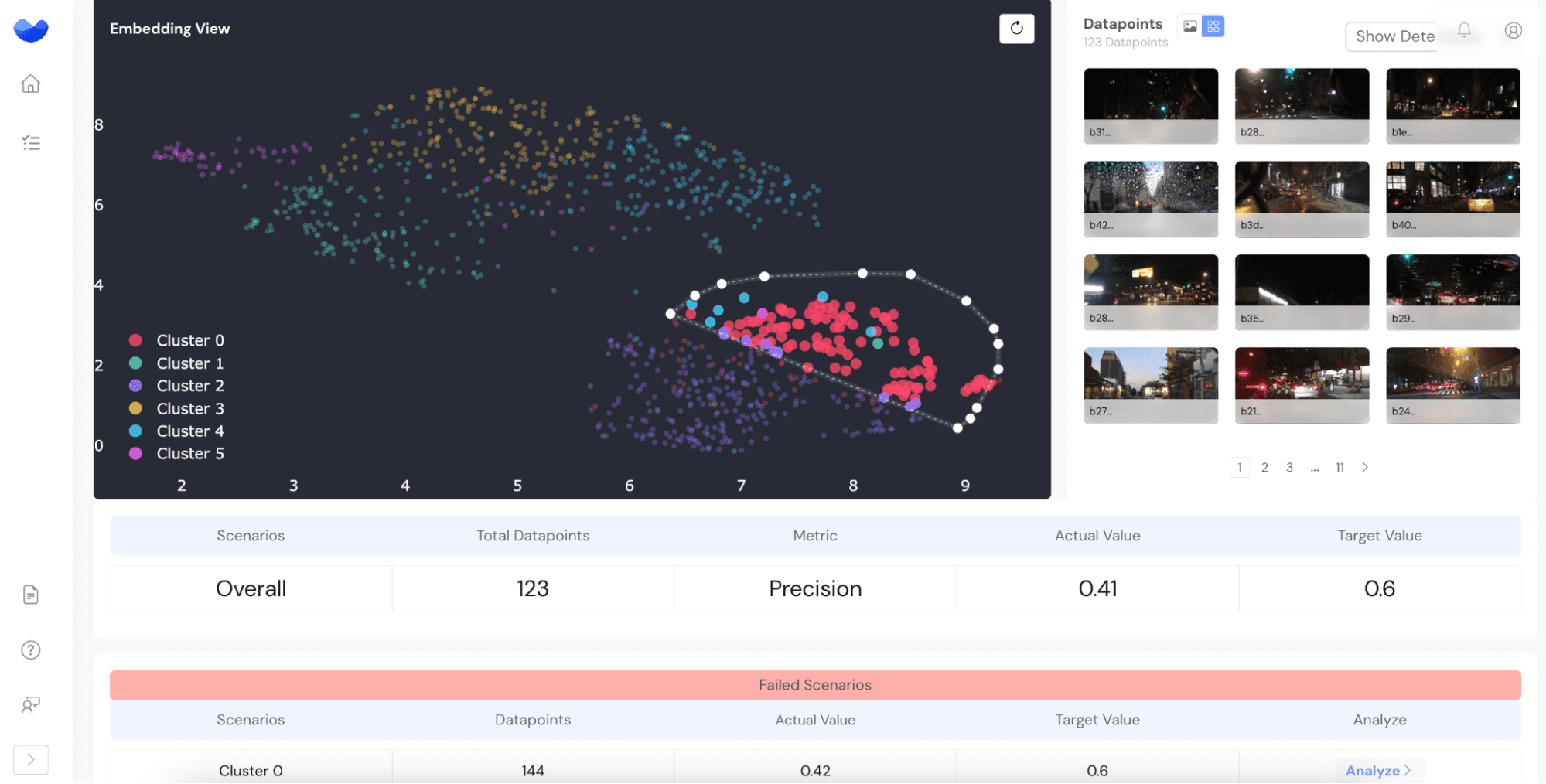
How to Detect and Fix AI Issues with RagaAI
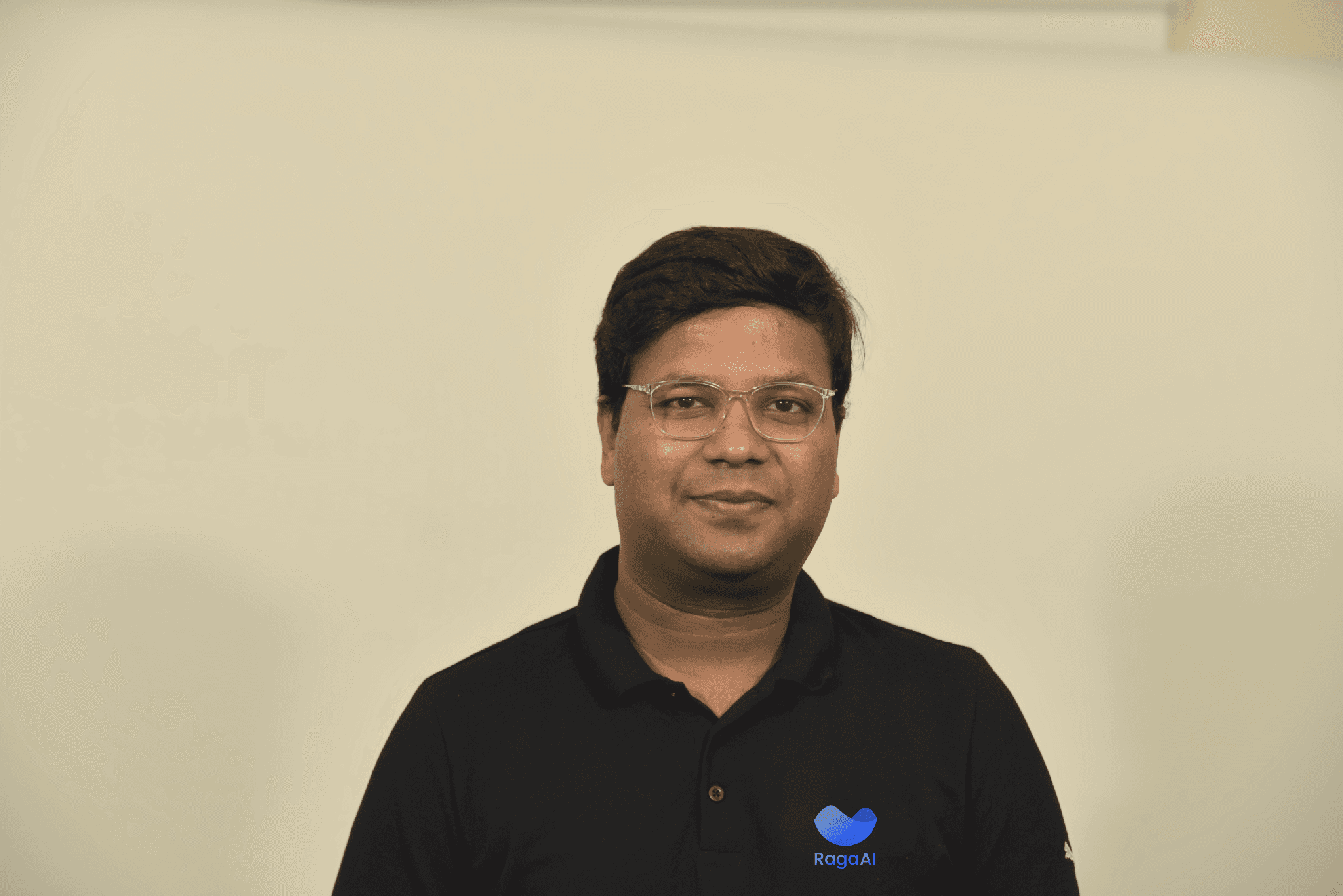
Jigar Gupta
Feb 16, 2024
Read the article
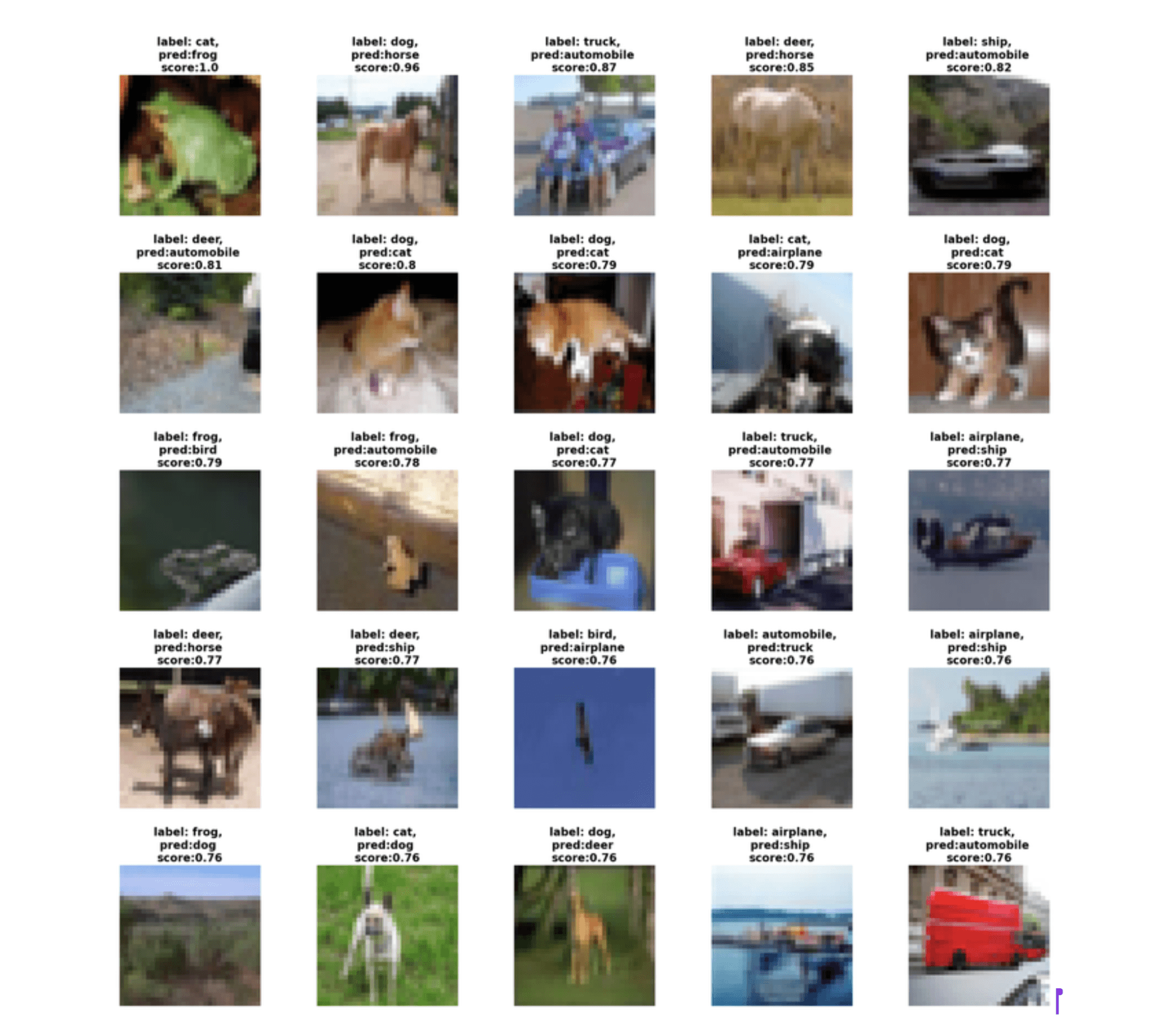
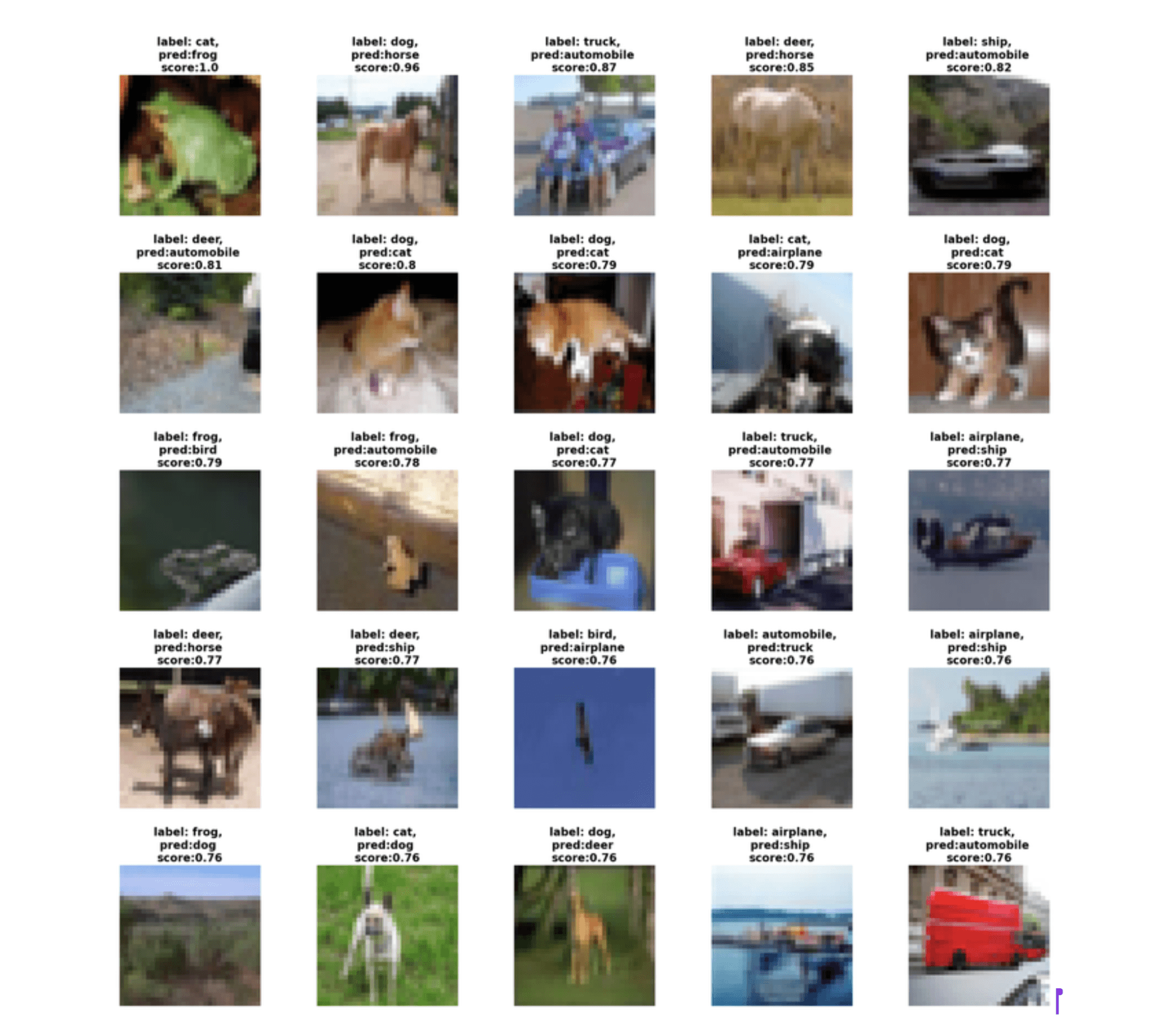
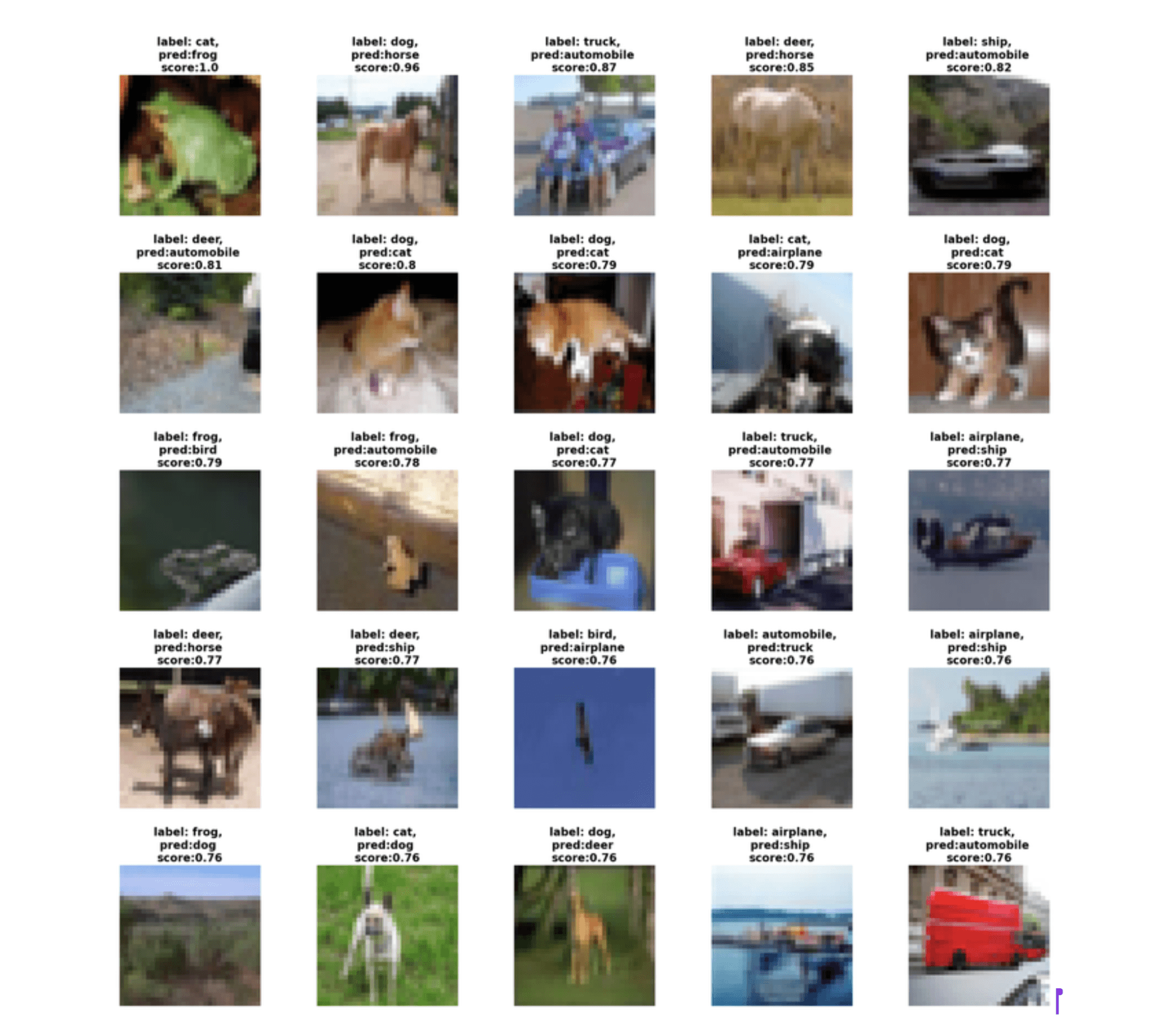
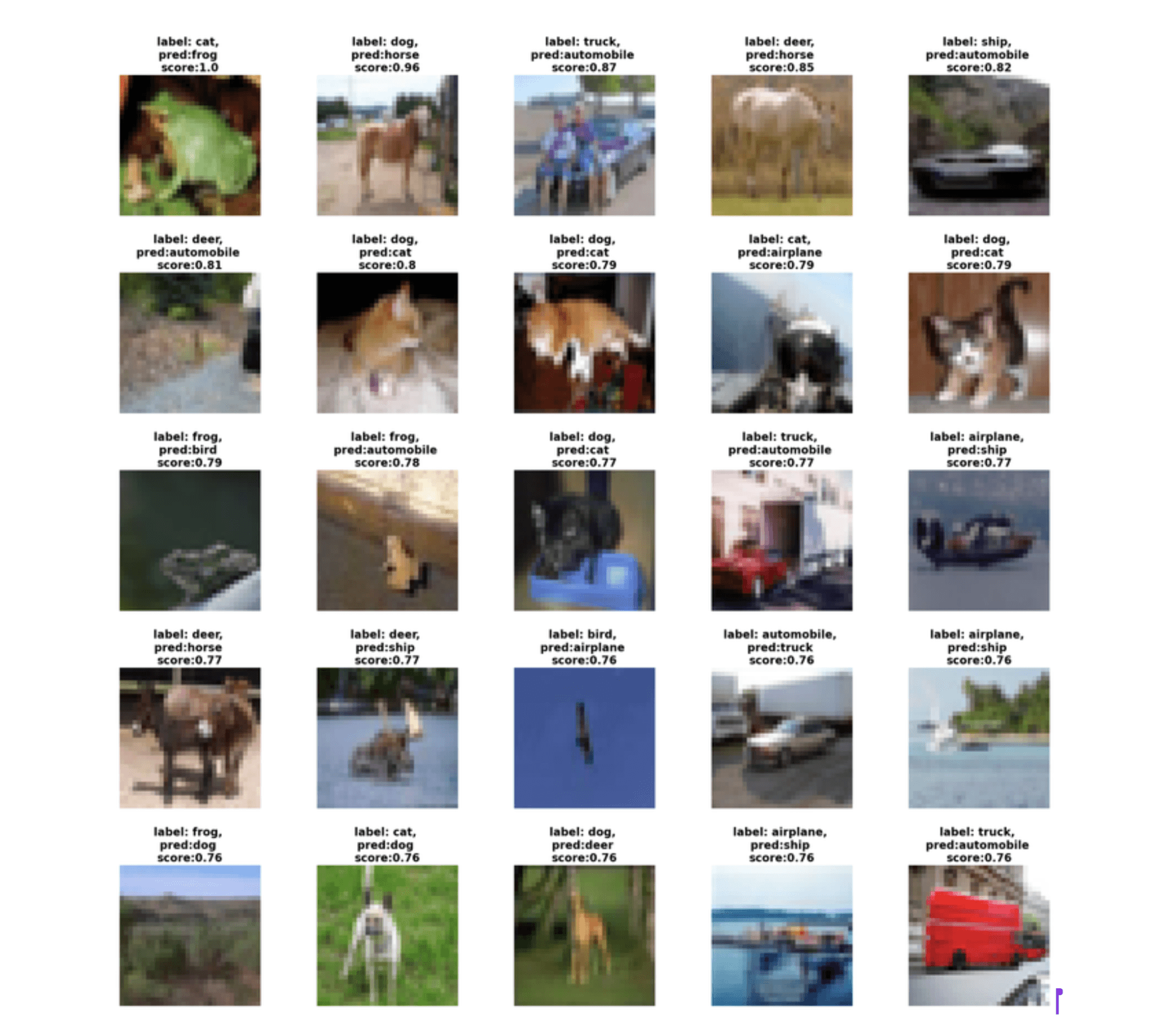
Detection of Labelling Issue in CIFAR-10 Dataset using RagaAI Platform
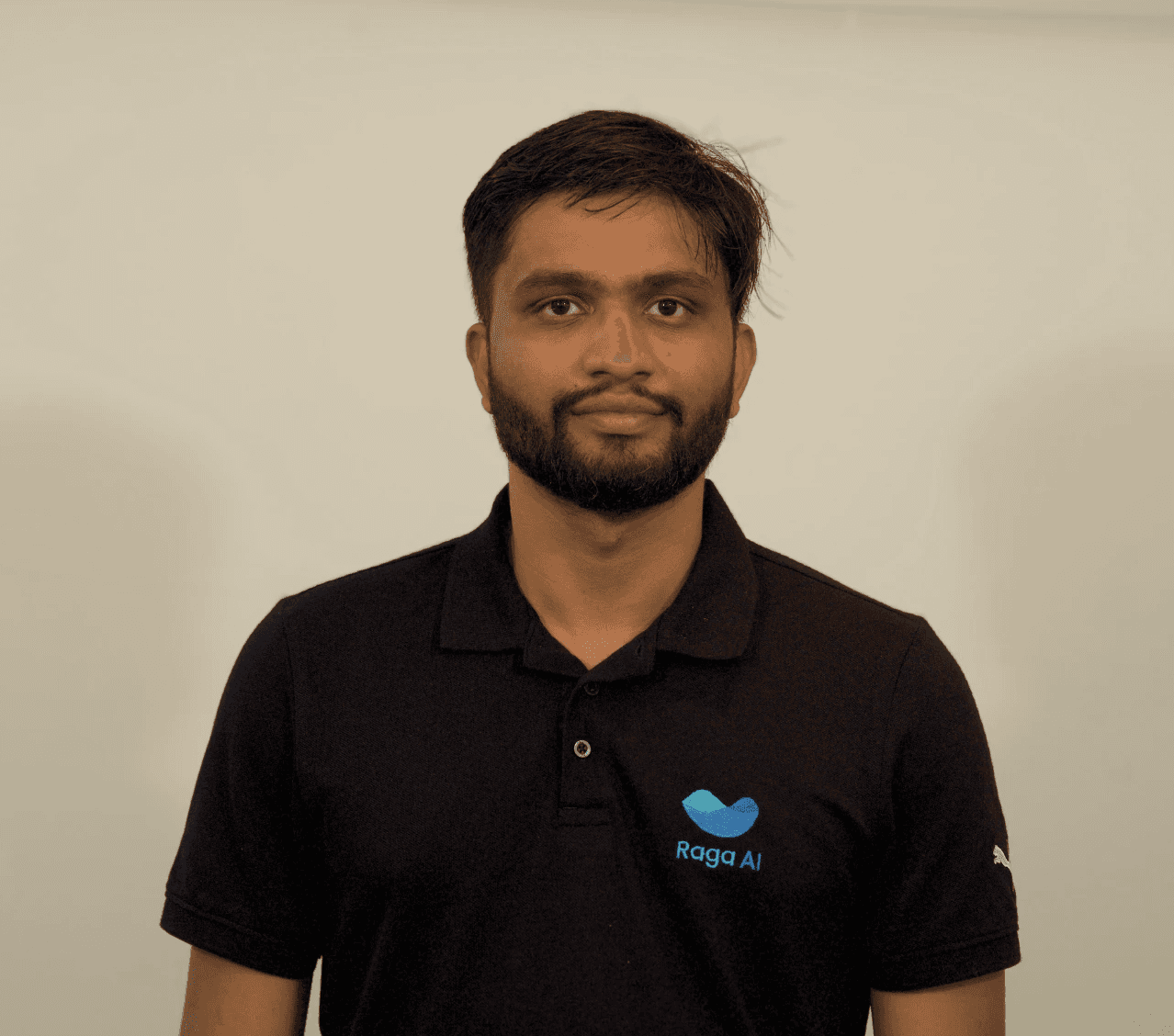
Rehan Asif
Feb 5, 2024
Read the article
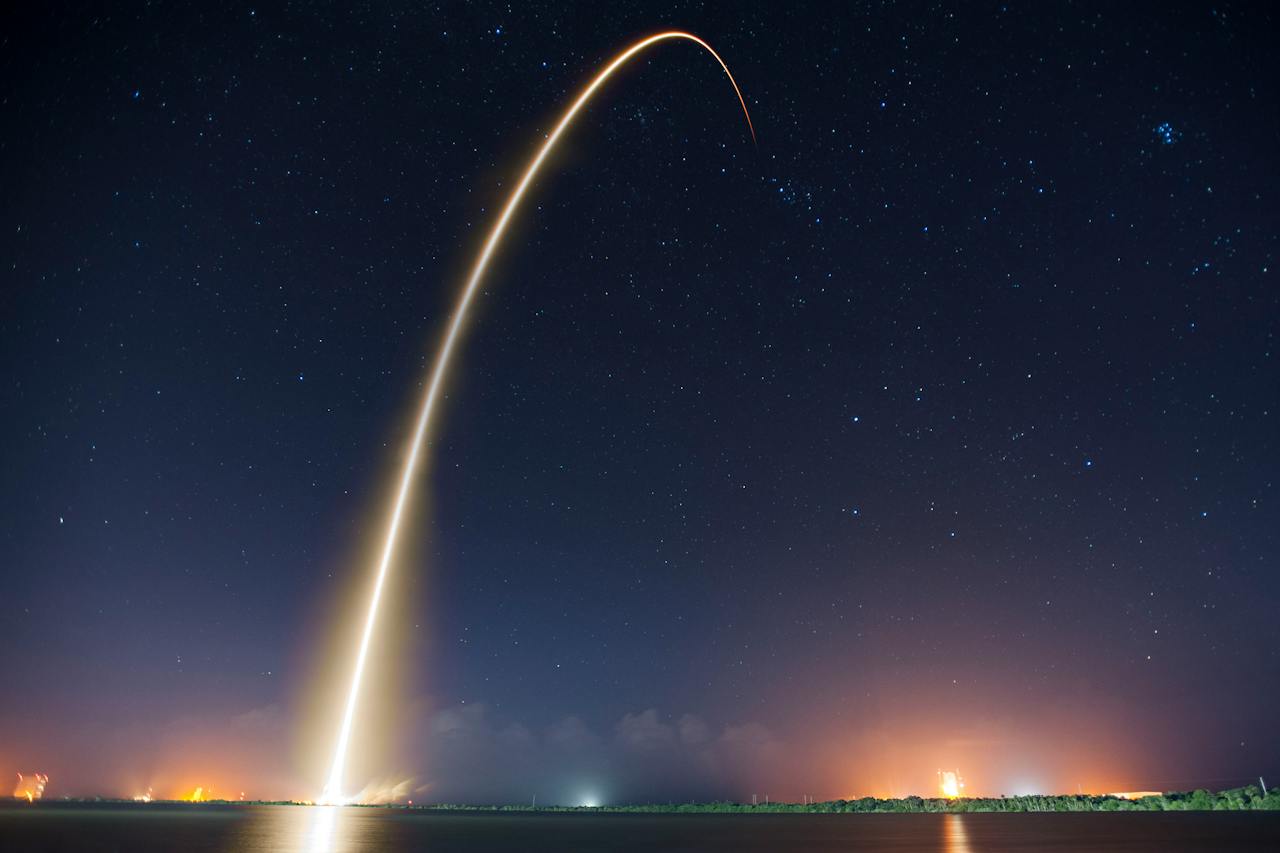
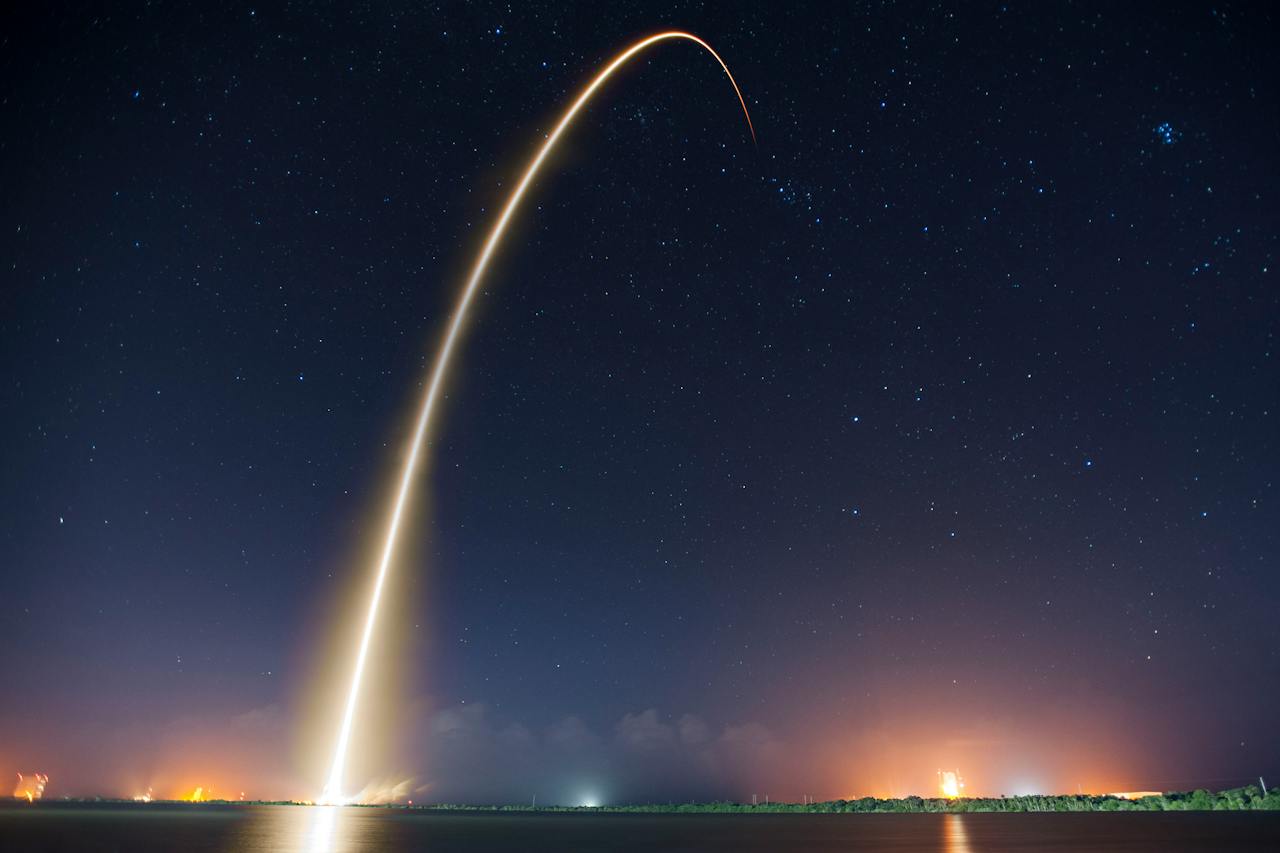
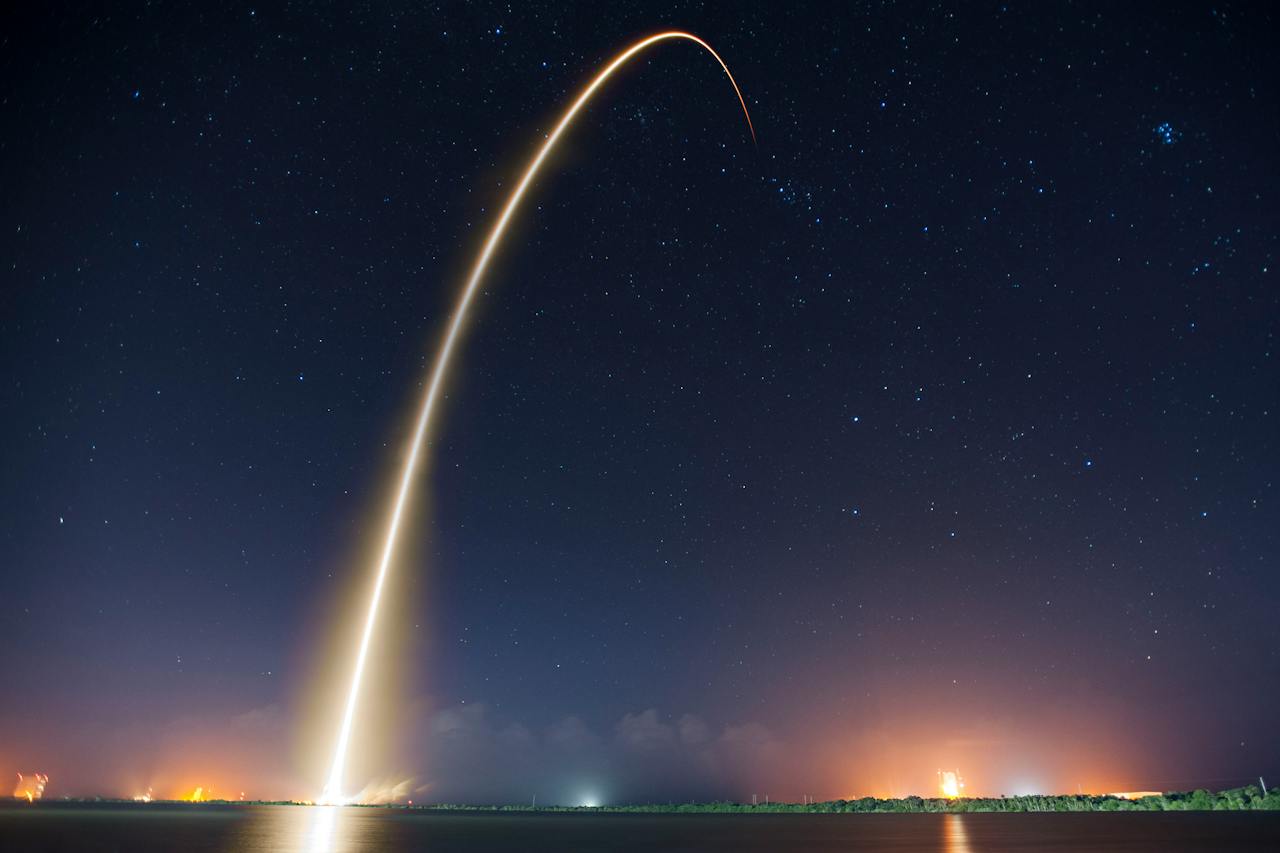
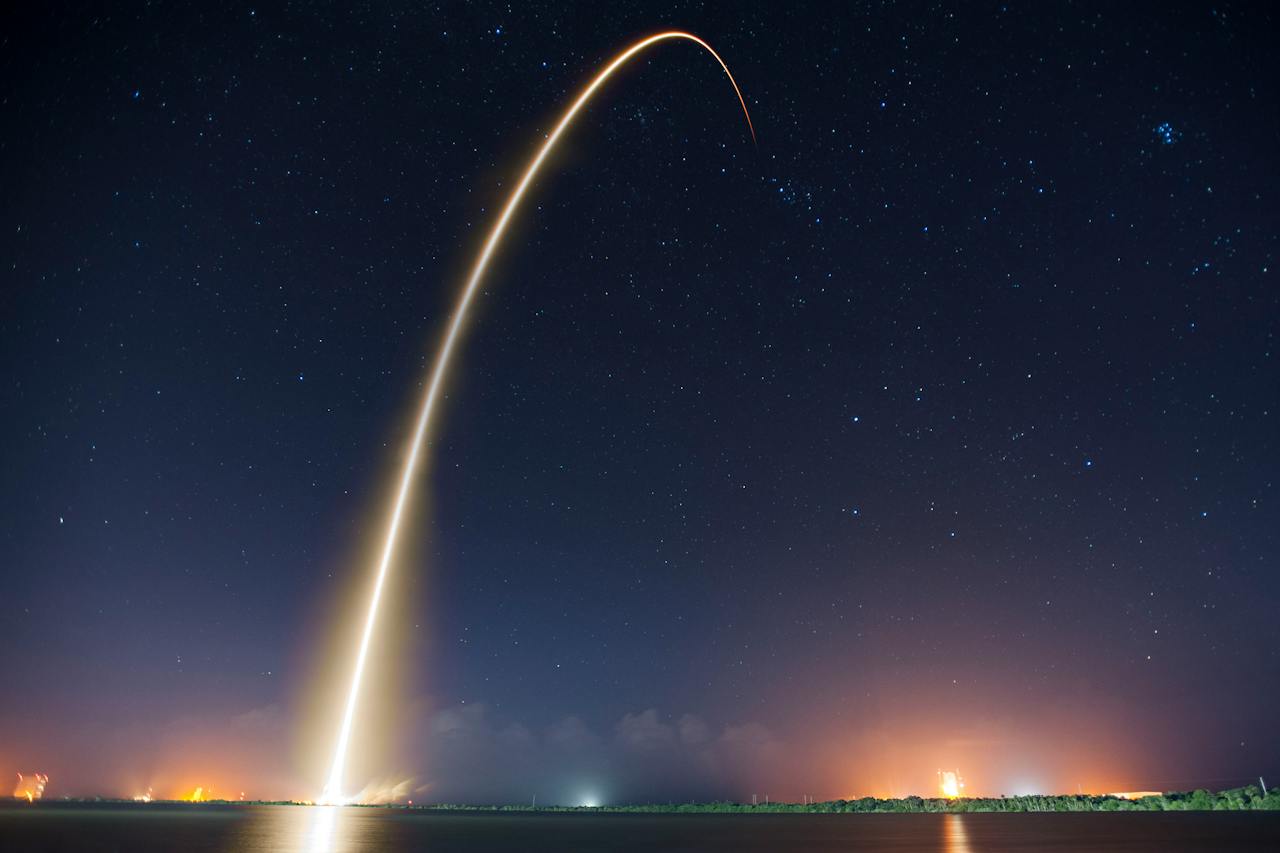
RagaAI emerges from Stealth with the most Comprehensive Testing Platform for AI
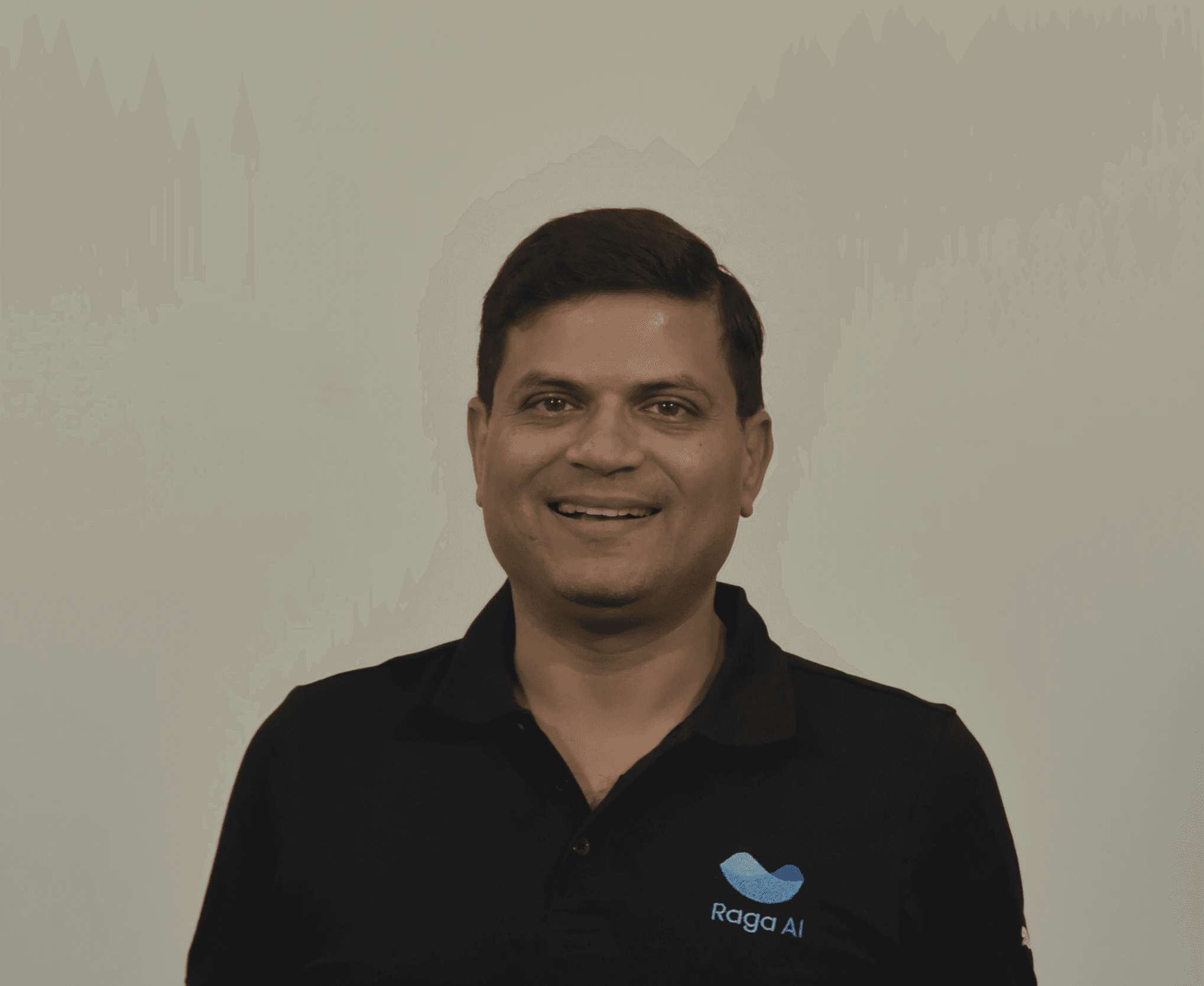
Gaurav Agarwal
Jan 23, 2024
Read the article




AI’s Missing Piece: Comprehensive AI Testing

Gaurav Agarwal
Jan 11, 2024
Read the article




Introducing RagaAI - The Future of AI Testing
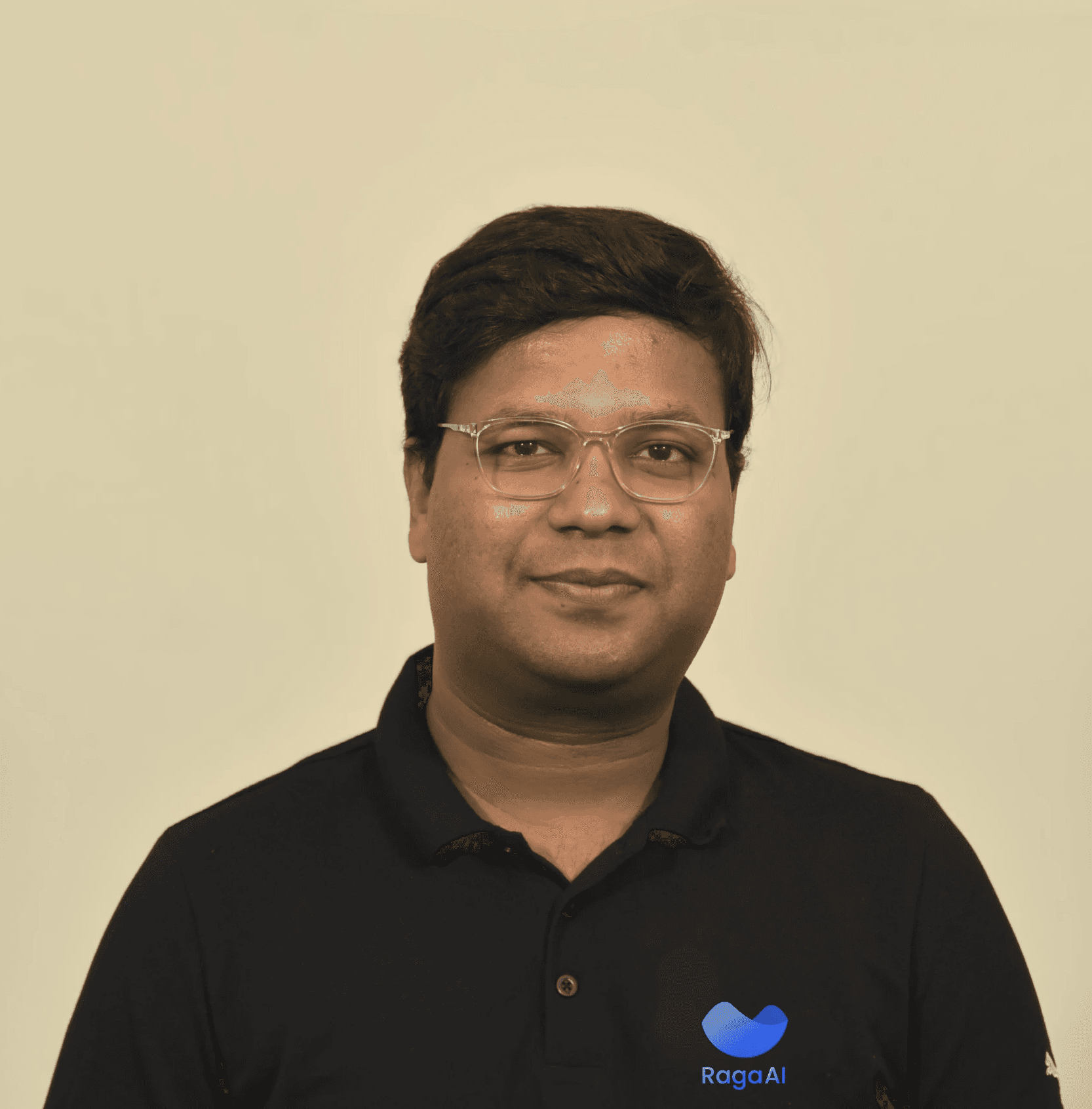
Jigar Gupta
Jan 14, 2024
Read the article




Introducing RagaAI DNA: The Multi-modal Foundation Model for AI Testing
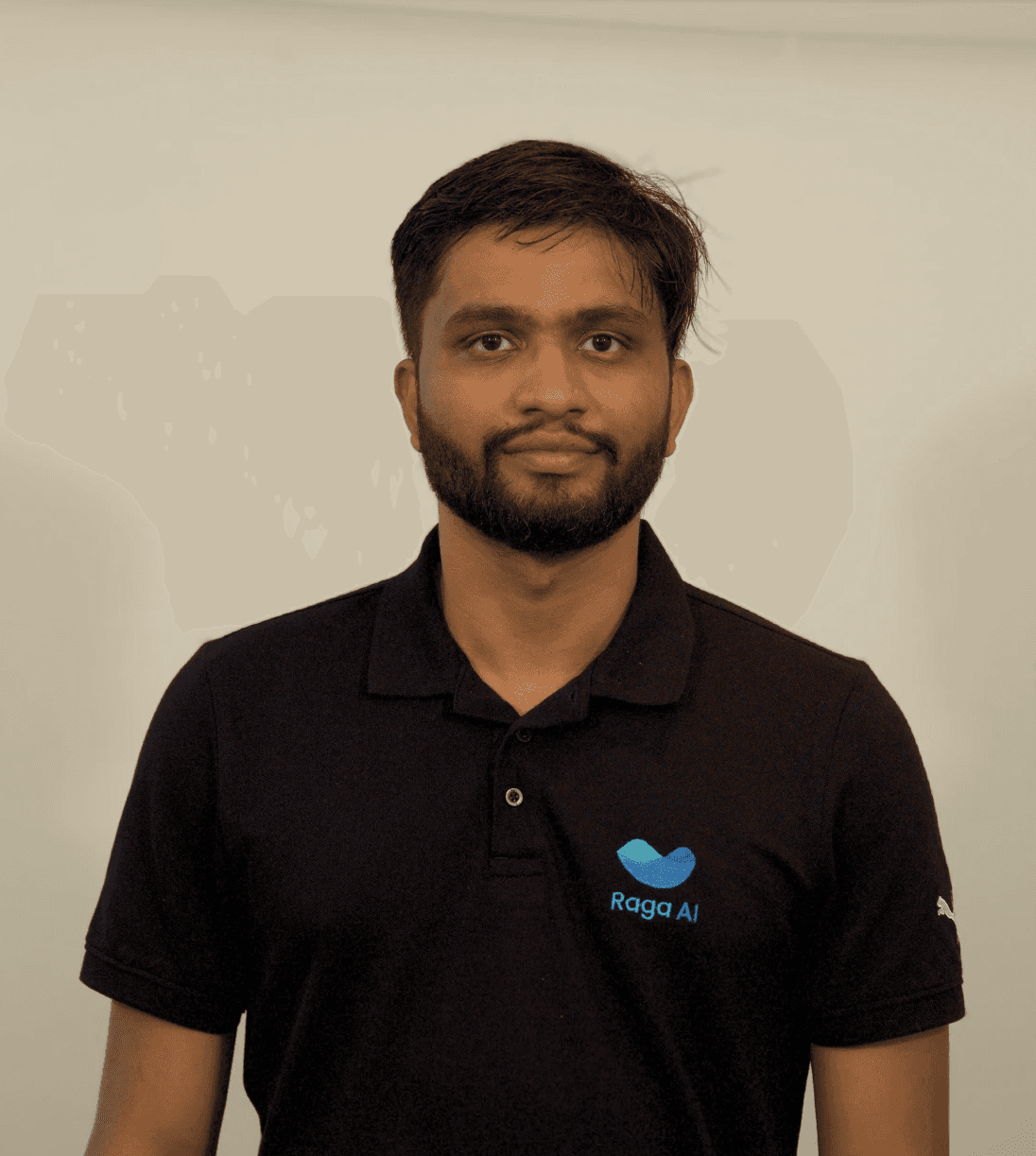
Rehan Asif
Jan 13, 2024
Read the article
Get Started With RagaAI®
Book a Demo
Schedule a call with AI Testing Experts

Get Started With RagaAI®
Book a Demo
Schedule a call with AI Testing Experts
