Leveraging AI For Enhanced Retail Customer Experiences
Leveraging AI For Enhanced Retail Customer Experiences
Leveraging AI For Enhanced Retail Customer Experiences
Jigar Gupta
Jul 1, 2024




In today's retail world, Artificial Intelligence (AI) is more than just a buzzword—it's a game-changer. Imagine being able to offer your customers personalized experiences that make them feel valued and understood while also running your operations smoothly and efficiently. That's the power of Retail AI.
It doesn't just improve customer service; it transforms it, turning every interaction into an opportunity to delight your customers. With Retail AI, you can tailor your offerings to meet individual preferences, predict trends before they happen, and streamline your back-end processes. This means happier customers and a more profitable business.
Let’s look at how AI can be your secret weapon for enhanced retail customer experiences.
Personalization and Customer Engagement
In the evolving retail landscape, personalization has become crucial for effectively engaging customers. Retail AI plays a pivotal role in this transformation, using advanced data analysis to understand and meet customer needs.
But you might ask: Is personalization necessary? Will it change the retail landscape? So, let’s discuss its importance in light of current user expectations.
Importance of Personalization
In today's competitive market, customers expect interactions tailored to their preferences and behaviors. However, many retailers need help in achieving true personalization, such as handling vast amounts of data, integrating disparate systems, and maintaining customer privacy.
For example, a retailer might struggle to analyze data from both online and in-store purchases to create a cohesive customer profile. Retail AI offers effective solutions to these challenges by analyzing customer data in real time, predicting trends, and automating personalized recommendations.
For instance, AI can suggest products based on past purchases or browsing history, and in-store digital signage can display personalized offers as customers walk by. This technology ensures that every customer interaction is relevant and engaging, ultimately overcoming the hurdles and transforming the shopping experience into a personalized journey.
Retail AI handles personalization and customer engagement with a two-part solution:
Understanding and Meeting Customer Needs
Retail AI analyzes vast amounts of customer data to uncover patterns and preferences. This deep understanding allows you to anticipate what your customers want and tailor your offerings to meet their specific needs, creating a more personalized shopping experience. This can include data from web interactions, surveys, feedback, past purchases, etc.
However, you should also remember that having a robust AI testing platform to maintain this accuracy and integrity is important. Tools like Raga AI offer a one-stop solution for testing, adding guardrails, and AI governance.
Creating Personalized Shopping Experiences
By leveraging AI technologies such as digital signage and POS systems, you can create highly personalized shopping experiences. Digital signage can display tailored promotions and product recommendations, while AI-powered POS systems can offer customized discounts and loyalty rewards at checkout.
You can also use AI-driven websites to give each customer a personalized experience. This experience mainly has two factors:
Dynamic Pricing: Real-time changes to prices on demand, supply, and competition changes.
Targeted Promotions and Discounts: Personalized discounts based on past purchases, promotional images, videos, product suggestions, etc.
Benefits of Personalization for Retailers
Personalization through Retail AI not only enhances customer satisfaction but also drives brand loyalty and revenue growth. When customers feel understood and valued, they are more likely to return, leading to increased sales and long-term profitability for your business.
Product data can be overwhelming, especially for online retail stores. Users often need more time to search for the right product. A store serving products on old data frames will push its users away. However, analyzing data and predicting user behavior can help you serve personalized products to your customers.
Now, let’s shift gears and talk about how Retail AI can streamline your operations for maximum efficiency.
Streamlining Retail Operations
In the fast-paced world of retail, operational efficiency is key to maintaining a competitive edge. Retail AI offers innovative solutions to make decision-making more informed and processes more efficient. It streamlines the following aspects of operations:
Operational Efficiency and Decision Making
Retail AI enhances operational efficiency by providing real-time insights and predictive analytics, helping you make data-driven decisions. From optimizing staffing levels to forecasting demand (which we will discuss in detail), AI enables you to run your operations smoothly and effectively.
It can accelerate repetitive customer care tasks, like helping with common issues via automated chats. You can also use AI assistants to help employees handle their tasks effectively. Similarly, voice assistants can free a customer of web application ordering hassles. All of these methods help in the operational and decision-making process.
Improving Inventory Management through AI Technologies
Managing inventory is a critical challenge for retailers. AI technologies like smart shelves and inventory robots revolutionize this process. Smart shelves can automatically track stock levels and alert you when it's time to restock. At the same time, inventory robots can perform regular stock checks and ensure accurate inventory counts, reducing the risk of overstocking or stockouts. (We will also discuss Inventory optimization later)
AI can help track inventory across warehouses and alert retailers if a product is available in another warehouse. It can also be automated to check if a customer has received a faulty product and send a replacement with the touch of a button. Thus, managing inventory becomes a cake with the help of Retail AI.
Enhancing Loss Prevention Strategies
Retail shrinkage, caused by theft and errors, is a significant concern for retailers. AI can enhance loss prevention strategies by analyzing surveillance footage, identifying suspicious behavior, and alerting staff in real-time.
Additionally, AI can help detect and reduce fraud at the checkout, ensuring a more secure retail environment. By integrating these AI solutions, you can significantly reduce shrinkage and protect your bottom line.
Next, let's dive into how AI can help you keep your shelves stocked perfectly with accurate demand forecasting and inventory optimization.
Like Retail AI can prevent retailers’ losses, Ragai AI can cut your losses in AI development. This article explains The costs of errors in application development.
Demand Forecasting and Inventory Optimization
Accurately forecasting demand and optimizing inventory is critical to meeting customer needs and maximizing profitability. Retail AI, particularly machine learning and predictive analytics, plays a crucial role in achieving these goals on two levels:
Predictive Analytics for Effective Inventory Management
Machine learning algorithms analyze customer behaviors and purchasing patterns to predict future demand. Their capacity to process vast data on millions of data points makes their predictions reliable. You can use their help to understand future demand in specific months, festivals, seasons, regions, etc.
By understanding these trends, you can ensure optimal stock levels, avoiding both overstocking and stockouts. This leads to a more efficient inventory system that aligns with customer demand.
Real-Time Data Analysis for Fast and Informed Decision-Making
Real-time data analysis allows you to make quick, informed decisions about your inventory. By continuously monitoring sales and stock levels, Retail AI provides immediate insights that help you respond to market changes swiftly. This ensures that your inventory is always aligned with current demand, improving overall operational efficiency and customer satisfaction.
Moving on, let's explore how conversational AI is changing the face of customer service for the better.
Conversational AI and Customer Service
Conversational AI, including chatbots, is revolutionizing how retailers interact with their customers, offering real-time assistance and enhancing overall satisfaction.
Conversational AI can engage customers in personalized interactions, guiding them through product selections, answering queries, and even assisting with purchases. Advanced AI can understand and respond to customers using:
Sentiment Analysis - AI tools analyze real-time customer interactions to gauge emotional states, allowing for customized responses that improve satisfaction and reduce churn.
Predictive Analysis - Machine learning enables predictive analytics, allowing you to anticipate customer needs and behaviors. By analyzing historical data, machine learning models can forecast customer churn, upsell opportunities, and even predict support ticket volume, enabling proactive customer service.
Voice Analysis - AI tools listen to conversations and find patterns like delight, frustration, etc., helping agents improve their performance and make customers happier.
Using these metrics, chatbots can elevate customer experience in many ways, including:
Tailored Recommendations - Chatbots analyze customer preferences and purchase history to offer personalized recommendations, including special promotions and discounts, increasing the chances of conversion.
Immediate Assistance - Available 24/7, chatbots provide instant help to customers, guiding them through the purchase process and informing them of ongoing promotions or discounts.
Automated Alerts - Chatbots proactively inform customers about upcoming sales events, limited-time offers, and exclusive discounts, creating a sense of urgency and encouraging quick action.
Order Tracking and Updates - In addition to promotional activities, chatbots enhance post-purchase interactions by providing real-time order tracking and updates, ensuring a smooth customer experience.
Feedback Gathering - Chatbots collect feedback from customers about their shopping experience, preferences, and satisfaction levels, allowing retailers to improve their promotional strategies and offerings.
Thus, conversational AI and chatbots help customers have a seamless experience. Speaking of seamless experiences, let's see how Retail AI is removing every possible barrier to make shopping hassle-free for your customers.
Learn more on a practical guide on deploying LLMs (Large Language Models) for your Retail AI.
Creating Frictionless Shopping Experiences
Retail AI is at the forefront of creating frictionless shopping experiences.
Customers today demand quick, hassle-free shopping experiences. They expect minimal wait times, easy navigation, and personalized service that makes their shopping journey enjoyable and efficient.
AI-powered solutions like smart carts, automated checkouts, and personalized mobile apps are transforming the shopping experience. Smart carts can track items as customers shop, while automated checkout systems allow for quick, contactless payments, eliminating the need for long queues.
Technologies like Intel® RealSense™ cameras are pushing the boundaries of autonomous store development. These advanced cameras can monitor inventory in real time, track customer movements, and enable seamless transactions without traditional checkout processes. This technology helps create a truly frictionless shopping environment where customers can simply pick up items and walk out, with their purchases automatically billed to their accounts.
Are you curious about how all this works in the real world? Let's check out some impressive case studies.
Case Studies
Retail AI has proven its worth for many businesses and companies. Here are a few examples:
Nordstrom Department Store Company, USA
Their objective was to use search keywords to boost products to the top of search results. They passed all of their images to Google and created the product-side images. At runtime, once they detected the object, they sent the object to their search API and then to Google Vision Product Search. This new design simplified their architectural design, cut down on service costs, and increased accuracy to over 95%.
Pitney Bowes, USA
They focused on cross-border business for retailers, which is a significant market opportunity as many consumers shop internationally. However, entering this market involved complexities that Pitney Bowes addressed through data, AI, and ML. One key area is fraud detection.
They used AutoML Tables from Google to enhance their fraud detection capabilities. This technology helped identify fraudulent orders while minimizing false positives to ensure a smooth consumer experience. By integrating ML models, particularly XGBoost, they reduced fraud loss rates by 49% and review efforts by 14%.
Walt Disney Company
Disney’s old recommendation system, based on product metadata, often gave irrelevant suggestions. By using Google's deep learning models training on user interactions, they achieved more accurate and personalized recommendations, leading to increased engagement and revenue.
Key takeaways include the importance of quality data, effective testing, and proper placement of recommendations. Working with Google also ensured scalability and high accuracy. Disney continues to use these models, enhancing customer experiences and boosting sales.
Others
Apart from these, many others have benefited from Retail AI. Here are some examples of companies taking advantage of AI chatbots:
Unilever - 14x higher sales. 6,335 unique user interactions in the first 12 hours
Tesco - 4X increase in digital catalog views. 13% decrease in general inquiries to the customer service team
Decathlon - 41% conversion rate (ad click to membership). 346% increase in member acquisition. 875% return on their Facebook ad spend.
Ben & Jerry’s - Sales over delivered by 20%.
Michael Kors - Retain users with a containment rate of 98.32%. 45,000 new users per month. It generated more than 375,000 active users.
Lego - 25% of all in-season online sales, with a 6x return on ad spend within certain markets. 8.4x higher conversion rate.
Alibaba - Responded 95% of the customer services on Alibaba e-commerce platforms. We generated $31 billion in gross merchandise volume.
L'Oréal - 27X higher engagement than email.
These studies show the viability and outreach of Retail AI in the current market. But it's not all sunshine and rainbows; let’s tackle some of the challenges you might face with Retail AI.
Read this article to learn more about various case studies on AI adoption.
Challenges of Retail AI
While Retail AI offers numerous benefits, it also presents certain challenges that retailers must navigate to fully harness its potential.
Data Privacy and Security
One of the primary challenges of Retail AI is ensuring the privacy and security of customer data. Retailers must comply with stringent data protection regulations and implement robust security measures to protect sensitive information from breaches and unauthorized access.
With the aim of building the first automated AI testing platform, Raga AI Governance Hub can help you with AI governance, risk management, and regulatory compliance needs.
Integration with Existing Systems
Integrating AI solutions with existing retail systems can be complex and resource-intensive. Retailers often need help in seamlessly merging new AI technologies with their current infrastructure, requiring significant investment in time and resources to achieve smooth integration.
Cost and ROI Considerations
Implementing AI technologies can be expensive, and retailers need to carefully consider the cost versus the potential return on investment (ROI). While AI can drive significant efficiencies and revenue growth, the initial investment and ongoing maintenance costs can be a barrier, especially for smaller retailers.
Skill Gaps and Workforce Adaptation
The adoption of AI in retail requires a workforce skilled in AI and data analytics. However, there is often a gap between the required skills and the workforce's current capabilities. Retailers must invest in training and development to equip their employees with the necessary skills to work effectively with AI technologies.
Ethical and Bias Concerns
AI systems can inadvertently perpetuate biases present in the data they are trained on, leading to unfair or discriminatory outcomes. Retailers must be vigilant in ensuring their AI solutions are ethical and unbiased, which involves continuous monitoring and adjustments to the algorithms.
Addressing these challenges is crucial for retailers to leverage AI effectively and sustainably, ensuring they can fully benefit from its transformative potential. However, even with its challenges, Retail AI shows good promise as it eases retail business with many advantages.
Future Prospects
The future of AI in retail holds immense promise as technology continues to evolve, driving innovations that redefine how retailers engage and personalize customer experiences:
Emerging Trends in AI and Retail
Conversational AI and Chatbots are set to revolutionize customer service and engagement, offering hyper-personalized recommendations and seamless voice-based transactions. According to Juniper Research, retail chatbots are projected to save over $8 billion in customer service costs globally by 2026.
AI-powered Personalization Engines will become more sophisticated, integrating diverse data points such as weather conditions and social media sentiment to deliver real-time, personalized product suggestions. Accenture highlights that 75% of consumers now expect tailored offers based on their preferences.
The Integration of AI with Augmented Reality (AR) is poised to enhance the retail experience with immersive features like virtual try-ons and interactive product visualization. Technavio forecasts the AR market in retail to reach $84.67 billion by 2025.
The Future of AI in Retail Personalization
These trends forecast significant advancements in AI-driven retail personalization:
Hyper-localized Marketing strategies will enable retailers to tailor marketing campaigns based on local trends and consumer preferences, enhancing relevance and engagement.
Predictive Inventory Management powered by AI will optimize stock levels by analyzing customer data, predicting demand patterns, and minimizing stockouts and overstocking.
Personalized Pricing and Promotions will leverage AI algorithms to determine optimal pricing strategies based on individual customer behaviors and market dynamics.
Read this article to learn more about the future of AI Testing.
Strategies for Staying Ahead
To capitalize on these opportunities, retailers should adopt proactive strategies:
Invest in AI Expertise by cultivating internal AI capabilities or collaborating with specialized retail AI providers.
Prioritize Data Security and Privacy to build trust and compliance with stringent data protection regulations.
Embrace an Experimental Culture to innovate and refine AI-powered solutions for enhanced customer experiences continuously.
This proactive approach ensures retailers are well-positioned to harness AI's transformative potential and shape the future of retail personalization.
Conclusion
In conclusion, AI represents a transformative force in the retail industry, poised to elevate customer experiences to new heights. From personalized recommendations and seamless transactions facilitated by chatbots to immersive augmented reality applications, AI is reshaping how retailers engage with their customers. Embracing AI technologies is no longer just an option but a strategic imperative for retailers aiming to maintain a competitive edge in a rapidly evolving market.
However, integrating AI into a retail business model is cumbersome and attracts errors. Raga AI offers a one-stop shop for all your testing needs. You can also leverage tools like Raga AI LLM Hub, Testing Platform, and Governance Hub to ensure the quality of your LLM. Raga’s tools perform comprehensive testing for RAG applications and add guardrails to prevent adversarial attacks.
Using Raga AI, you can be sure that you get the best quality context, AI governance, risk management, and regulatory compliance. So give Raga AI a try, or Book a Demo today.
In today's retail world, Artificial Intelligence (AI) is more than just a buzzword—it's a game-changer. Imagine being able to offer your customers personalized experiences that make them feel valued and understood while also running your operations smoothly and efficiently. That's the power of Retail AI.
It doesn't just improve customer service; it transforms it, turning every interaction into an opportunity to delight your customers. With Retail AI, you can tailor your offerings to meet individual preferences, predict trends before they happen, and streamline your back-end processes. This means happier customers and a more profitable business.
Let’s look at how AI can be your secret weapon for enhanced retail customer experiences.
Personalization and Customer Engagement
In the evolving retail landscape, personalization has become crucial for effectively engaging customers. Retail AI plays a pivotal role in this transformation, using advanced data analysis to understand and meet customer needs.
But you might ask: Is personalization necessary? Will it change the retail landscape? So, let’s discuss its importance in light of current user expectations.
Importance of Personalization
In today's competitive market, customers expect interactions tailored to their preferences and behaviors. However, many retailers need help in achieving true personalization, such as handling vast amounts of data, integrating disparate systems, and maintaining customer privacy.
For example, a retailer might struggle to analyze data from both online and in-store purchases to create a cohesive customer profile. Retail AI offers effective solutions to these challenges by analyzing customer data in real time, predicting trends, and automating personalized recommendations.
For instance, AI can suggest products based on past purchases or browsing history, and in-store digital signage can display personalized offers as customers walk by. This technology ensures that every customer interaction is relevant and engaging, ultimately overcoming the hurdles and transforming the shopping experience into a personalized journey.
Retail AI handles personalization and customer engagement with a two-part solution:
Understanding and Meeting Customer Needs
Retail AI analyzes vast amounts of customer data to uncover patterns and preferences. This deep understanding allows you to anticipate what your customers want and tailor your offerings to meet their specific needs, creating a more personalized shopping experience. This can include data from web interactions, surveys, feedback, past purchases, etc.
However, you should also remember that having a robust AI testing platform to maintain this accuracy and integrity is important. Tools like Raga AI offer a one-stop solution for testing, adding guardrails, and AI governance.
Creating Personalized Shopping Experiences
By leveraging AI technologies such as digital signage and POS systems, you can create highly personalized shopping experiences. Digital signage can display tailored promotions and product recommendations, while AI-powered POS systems can offer customized discounts and loyalty rewards at checkout.
You can also use AI-driven websites to give each customer a personalized experience. This experience mainly has two factors:
Dynamic Pricing: Real-time changes to prices on demand, supply, and competition changes.
Targeted Promotions and Discounts: Personalized discounts based on past purchases, promotional images, videos, product suggestions, etc.
Benefits of Personalization for Retailers
Personalization through Retail AI not only enhances customer satisfaction but also drives brand loyalty and revenue growth. When customers feel understood and valued, they are more likely to return, leading to increased sales and long-term profitability for your business.
Product data can be overwhelming, especially for online retail stores. Users often need more time to search for the right product. A store serving products on old data frames will push its users away. However, analyzing data and predicting user behavior can help you serve personalized products to your customers.
Now, let’s shift gears and talk about how Retail AI can streamline your operations for maximum efficiency.
Streamlining Retail Operations
In the fast-paced world of retail, operational efficiency is key to maintaining a competitive edge. Retail AI offers innovative solutions to make decision-making more informed and processes more efficient. It streamlines the following aspects of operations:
Operational Efficiency and Decision Making
Retail AI enhances operational efficiency by providing real-time insights and predictive analytics, helping you make data-driven decisions. From optimizing staffing levels to forecasting demand (which we will discuss in detail), AI enables you to run your operations smoothly and effectively.
It can accelerate repetitive customer care tasks, like helping with common issues via automated chats. You can also use AI assistants to help employees handle their tasks effectively. Similarly, voice assistants can free a customer of web application ordering hassles. All of these methods help in the operational and decision-making process.
Improving Inventory Management through AI Technologies
Managing inventory is a critical challenge for retailers. AI technologies like smart shelves and inventory robots revolutionize this process. Smart shelves can automatically track stock levels and alert you when it's time to restock. At the same time, inventory robots can perform regular stock checks and ensure accurate inventory counts, reducing the risk of overstocking or stockouts. (We will also discuss Inventory optimization later)
AI can help track inventory across warehouses and alert retailers if a product is available in another warehouse. It can also be automated to check if a customer has received a faulty product and send a replacement with the touch of a button. Thus, managing inventory becomes a cake with the help of Retail AI.
Enhancing Loss Prevention Strategies
Retail shrinkage, caused by theft and errors, is a significant concern for retailers. AI can enhance loss prevention strategies by analyzing surveillance footage, identifying suspicious behavior, and alerting staff in real-time.
Additionally, AI can help detect and reduce fraud at the checkout, ensuring a more secure retail environment. By integrating these AI solutions, you can significantly reduce shrinkage and protect your bottom line.
Next, let's dive into how AI can help you keep your shelves stocked perfectly with accurate demand forecasting and inventory optimization.
Like Retail AI can prevent retailers’ losses, Ragai AI can cut your losses in AI development. This article explains The costs of errors in application development.
Demand Forecasting and Inventory Optimization
Accurately forecasting demand and optimizing inventory is critical to meeting customer needs and maximizing profitability. Retail AI, particularly machine learning and predictive analytics, plays a crucial role in achieving these goals on two levels:
Predictive Analytics for Effective Inventory Management
Machine learning algorithms analyze customer behaviors and purchasing patterns to predict future demand. Their capacity to process vast data on millions of data points makes their predictions reliable. You can use their help to understand future demand in specific months, festivals, seasons, regions, etc.
By understanding these trends, you can ensure optimal stock levels, avoiding both overstocking and stockouts. This leads to a more efficient inventory system that aligns with customer demand.
Real-Time Data Analysis for Fast and Informed Decision-Making
Real-time data analysis allows you to make quick, informed decisions about your inventory. By continuously monitoring sales and stock levels, Retail AI provides immediate insights that help you respond to market changes swiftly. This ensures that your inventory is always aligned with current demand, improving overall operational efficiency and customer satisfaction.
Moving on, let's explore how conversational AI is changing the face of customer service for the better.
Conversational AI and Customer Service
Conversational AI, including chatbots, is revolutionizing how retailers interact with their customers, offering real-time assistance and enhancing overall satisfaction.
Conversational AI can engage customers in personalized interactions, guiding them through product selections, answering queries, and even assisting with purchases. Advanced AI can understand and respond to customers using:
Sentiment Analysis - AI tools analyze real-time customer interactions to gauge emotional states, allowing for customized responses that improve satisfaction and reduce churn.
Predictive Analysis - Machine learning enables predictive analytics, allowing you to anticipate customer needs and behaviors. By analyzing historical data, machine learning models can forecast customer churn, upsell opportunities, and even predict support ticket volume, enabling proactive customer service.
Voice Analysis - AI tools listen to conversations and find patterns like delight, frustration, etc., helping agents improve their performance and make customers happier.
Using these metrics, chatbots can elevate customer experience in many ways, including:
Tailored Recommendations - Chatbots analyze customer preferences and purchase history to offer personalized recommendations, including special promotions and discounts, increasing the chances of conversion.
Immediate Assistance - Available 24/7, chatbots provide instant help to customers, guiding them through the purchase process and informing them of ongoing promotions or discounts.
Automated Alerts - Chatbots proactively inform customers about upcoming sales events, limited-time offers, and exclusive discounts, creating a sense of urgency and encouraging quick action.
Order Tracking and Updates - In addition to promotional activities, chatbots enhance post-purchase interactions by providing real-time order tracking and updates, ensuring a smooth customer experience.
Feedback Gathering - Chatbots collect feedback from customers about their shopping experience, preferences, and satisfaction levels, allowing retailers to improve their promotional strategies and offerings.
Thus, conversational AI and chatbots help customers have a seamless experience. Speaking of seamless experiences, let's see how Retail AI is removing every possible barrier to make shopping hassle-free for your customers.
Learn more on a practical guide on deploying LLMs (Large Language Models) for your Retail AI.
Creating Frictionless Shopping Experiences
Retail AI is at the forefront of creating frictionless shopping experiences.
Customers today demand quick, hassle-free shopping experiences. They expect minimal wait times, easy navigation, and personalized service that makes their shopping journey enjoyable and efficient.
AI-powered solutions like smart carts, automated checkouts, and personalized mobile apps are transforming the shopping experience. Smart carts can track items as customers shop, while automated checkout systems allow for quick, contactless payments, eliminating the need for long queues.
Technologies like Intel® RealSense™ cameras are pushing the boundaries of autonomous store development. These advanced cameras can monitor inventory in real time, track customer movements, and enable seamless transactions without traditional checkout processes. This technology helps create a truly frictionless shopping environment where customers can simply pick up items and walk out, with their purchases automatically billed to their accounts.
Are you curious about how all this works in the real world? Let's check out some impressive case studies.
Case Studies
Retail AI has proven its worth for many businesses and companies. Here are a few examples:
Nordstrom Department Store Company, USA
Their objective was to use search keywords to boost products to the top of search results. They passed all of their images to Google and created the product-side images. At runtime, once they detected the object, they sent the object to their search API and then to Google Vision Product Search. This new design simplified their architectural design, cut down on service costs, and increased accuracy to over 95%.
Pitney Bowes, USA
They focused on cross-border business for retailers, which is a significant market opportunity as many consumers shop internationally. However, entering this market involved complexities that Pitney Bowes addressed through data, AI, and ML. One key area is fraud detection.
They used AutoML Tables from Google to enhance their fraud detection capabilities. This technology helped identify fraudulent orders while minimizing false positives to ensure a smooth consumer experience. By integrating ML models, particularly XGBoost, they reduced fraud loss rates by 49% and review efforts by 14%.
Walt Disney Company
Disney’s old recommendation system, based on product metadata, often gave irrelevant suggestions. By using Google's deep learning models training on user interactions, they achieved more accurate and personalized recommendations, leading to increased engagement and revenue.
Key takeaways include the importance of quality data, effective testing, and proper placement of recommendations. Working with Google also ensured scalability and high accuracy. Disney continues to use these models, enhancing customer experiences and boosting sales.
Others
Apart from these, many others have benefited from Retail AI. Here are some examples of companies taking advantage of AI chatbots:
Unilever - 14x higher sales. 6,335 unique user interactions in the first 12 hours
Tesco - 4X increase in digital catalog views. 13% decrease in general inquiries to the customer service team
Decathlon - 41% conversion rate (ad click to membership). 346% increase in member acquisition. 875% return on their Facebook ad spend.
Ben & Jerry’s - Sales over delivered by 20%.
Michael Kors - Retain users with a containment rate of 98.32%. 45,000 new users per month. It generated more than 375,000 active users.
Lego - 25% of all in-season online sales, with a 6x return on ad spend within certain markets. 8.4x higher conversion rate.
Alibaba - Responded 95% of the customer services on Alibaba e-commerce platforms. We generated $31 billion in gross merchandise volume.
L'Oréal - 27X higher engagement than email.
These studies show the viability and outreach of Retail AI in the current market. But it's not all sunshine and rainbows; let’s tackle some of the challenges you might face with Retail AI.
Read this article to learn more about various case studies on AI adoption.
Challenges of Retail AI
While Retail AI offers numerous benefits, it also presents certain challenges that retailers must navigate to fully harness its potential.
Data Privacy and Security
One of the primary challenges of Retail AI is ensuring the privacy and security of customer data. Retailers must comply with stringent data protection regulations and implement robust security measures to protect sensitive information from breaches and unauthorized access.
With the aim of building the first automated AI testing platform, Raga AI Governance Hub can help you with AI governance, risk management, and regulatory compliance needs.
Integration with Existing Systems
Integrating AI solutions with existing retail systems can be complex and resource-intensive. Retailers often need help in seamlessly merging new AI technologies with their current infrastructure, requiring significant investment in time and resources to achieve smooth integration.
Cost and ROI Considerations
Implementing AI technologies can be expensive, and retailers need to carefully consider the cost versus the potential return on investment (ROI). While AI can drive significant efficiencies and revenue growth, the initial investment and ongoing maintenance costs can be a barrier, especially for smaller retailers.
Skill Gaps and Workforce Adaptation
The adoption of AI in retail requires a workforce skilled in AI and data analytics. However, there is often a gap between the required skills and the workforce's current capabilities. Retailers must invest in training and development to equip their employees with the necessary skills to work effectively with AI technologies.
Ethical and Bias Concerns
AI systems can inadvertently perpetuate biases present in the data they are trained on, leading to unfair or discriminatory outcomes. Retailers must be vigilant in ensuring their AI solutions are ethical and unbiased, which involves continuous monitoring and adjustments to the algorithms.
Addressing these challenges is crucial for retailers to leverage AI effectively and sustainably, ensuring they can fully benefit from its transformative potential. However, even with its challenges, Retail AI shows good promise as it eases retail business with many advantages.
Future Prospects
The future of AI in retail holds immense promise as technology continues to evolve, driving innovations that redefine how retailers engage and personalize customer experiences:
Emerging Trends in AI and Retail
Conversational AI and Chatbots are set to revolutionize customer service and engagement, offering hyper-personalized recommendations and seamless voice-based transactions. According to Juniper Research, retail chatbots are projected to save over $8 billion in customer service costs globally by 2026.
AI-powered Personalization Engines will become more sophisticated, integrating diverse data points such as weather conditions and social media sentiment to deliver real-time, personalized product suggestions. Accenture highlights that 75% of consumers now expect tailored offers based on their preferences.
The Integration of AI with Augmented Reality (AR) is poised to enhance the retail experience with immersive features like virtual try-ons and interactive product visualization. Technavio forecasts the AR market in retail to reach $84.67 billion by 2025.
The Future of AI in Retail Personalization
These trends forecast significant advancements in AI-driven retail personalization:
Hyper-localized Marketing strategies will enable retailers to tailor marketing campaigns based on local trends and consumer preferences, enhancing relevance and engagement.
Predictive Inventory Management powered by AI will optimize stock levels by analyzing customer data, predicting demand patterns, and minimizing stockouts and overstocking.
Personalized Pricing and Promotions will leverage AI algorithms to determine optimal pricing strategies based on individual customer behaviors and market dynamics.
Read this article to learn more about the future of AI Testing.
Strategies for Staying Ahead
To capitalize on these opportunities, retailers should adopt proactive strategies:
Invest in AI Expertise by cultivating internal AI capabilities or collaborating with specialized retail AI providers.
Prioritize Data Security and Privacy to build trust and compliance with stringent data protection regulations.
Embrace an Experimental Culture to innovate and refine AI-powered solutions for enhanced customer experiences continuously.
This proactive approach ensures retailers are well-positioned to harness AI's transformative potential and shape the future of retail personalization.
Conclusion
In conclusion, AI represents a transformative force in the retail industry, poised to elevate customer experiences to new heights. From personalized recommendations and seamless transactions facilitated by chatbots to immersive augmented reality applications, AI is reshaping how retailers engage with their customers. Embracing AI technologies is no longer just an option but a strategic imperative for retailers aiming to maintain a competitive edge in a rapidly evolving market.
However, integrating AI into a retail business model is cumbersome and attracts errors. Raga AI offers a one-stop shop for all your testing needs. You can also leverage tools like Raga AI LLM Hub, Testing Platform, and Governance Hub to ensure the quality of your LLM. Raga’s tools perform comprehensive testing for RAG applications and add guardrails to prevent adversarial attacks.
Using Raga AI, you can be sure that you get the best quality context, AI governance, risk management, and regulatory compliance. So give Raga AI a try, or Book a Demo today.
In today's retail world, Artificial Intelligence (AI) is more than just a buzzword—it's a game-changer. Imagine being able to offer your customers personalized experiences that make them feel valued and understood while also running your operations smoothly and efficiently. That's the power of Retail AI.
It doesn't just improve customer service; it transforms it, turning every interaction into an opportunity to delight your customers. With Retail AI, you can tailor your offerings to meet individual preferences, predict trends before they happen, and streamline your back-end processes. This means happier customers and a more profitable business.
Let’s look at how AI can be your secret weapon for enhanced retail customer experiences.
Personalization and Customer Engagement
In the evolving retail landscape, personalization has become crucial for effectively engaging customers. Retail AI plays a pivotal role in this transformation, using advanced data analysis to understand and meet customer needs.
But you might ask: Is personalization necessary? Will it change the retail landscape? So, let’s discuss its importance in light of current user expectations.
Importance of Personalization
In today's competitive market, customers expect interactions tailored to their preferences and behaviors. However, many retailers need help in achieving true personalization, such as handling vast amounts of data, integrating disparate systems, and maintaining customer privacy.
For example, a retailer might struggle to analyze data from both online and in-store purchases to create a cohesive customer profile. Retail AI offers effective solutions to these challenges by analyzing customer data in real time, predicting trends, and automating personalized recommendations.
For instance, AI can suggest products based on past purchases or browsing history, and in-store digital signage can display personalized offers as customers walk by. This technology ensures that every customer interaction is relevant and engaging, ultimately overcoming the hurdles and transforming the shopping experience into a personalized journey.
Retail AI handles personalization and customer engagement with a two-part solution:
Understanding and Meeting Customer Needs
Retail AI analyzes vast amounts of customer data to uncover patterns and preferences. This deep understanding allows you to anticipate what your customers want and tailor your offerings to meet their specific needs, creating a more personalized shopping experience. This can include data from web interactions, surveys, feedback, past purchases, etc.
However, you should also remember that having a robust AI testing platform to maintain this accuracy and integrity is important. Tools like Raga AI offer a one-stop solution for testing, adding guardrails, and AI governance.
Creating Personalized Shopping Experiences
By leveraging AI technologies such as digital signage and POS systems, you can create highly personalized shopping experiences. Digital signage can display tailored promotions and product recommendations, while AI-powered POS systems can offer customized discounts and loyalty rewards at checkout.
You can also use AI-driven websites to give each customer a personalized experience. This experience mainly has two factors:
Dynamic Pricing: Real-time changes to prices on demand, supply, and competition changes.
Targeted Promotions and Discounts: Personalized discounts based on past purchases, promotional images, videos, product suggestions, etc.
Benefits of Personalization for Retailers
Personalization through Retail AI not only enhances customer satisfaction but also drives brand loyalty and revenue growth. When customers feel understood and valued, they are more likely to return, leading to increased sales and long-term profitability for your business.
Product data can be overwhelming, especially for online retail stores. Users often need more time to search for the right product. A store serving products on old data frames will push its users away. However, analyzing data and predicting user behavior can help you serve personalized products to your customers.
Now, let’s shift gears and talk about how Retail AI can streamline your operations for maximum efficiency.
Streamlining Retail Operations
In the fast-paced world of retail, operational efficiency is key to maintaining a competitive edge. Retail AI offers innovative solutions to make decision-making more informed and processes more efficient. It streamlines the following aspects of operations:
Operational Efficiency and Decision Making
Retail AI enhances operational efficiency by providing real-time insights and predictive analytics, helping you make data-driven decisions. From optimizing staffing levels to forecasting demand (which we will discuss in detail), AI enables you to run your operations smoothly and effectively.
It can accelerate repetitive customer care tasks, like helping with common issues via automated chats. You can also use AI assistants to help employees handle their tasks effectively. Similarly, voice assistants can free a customer of web application ordering hassles. All of these methods help in the operational and decision-making process.
Improving Inventory Management through AI Technologies
Managing inventory is a critical challenge for retailers. AI technologies like smart shelves and inventory robots revolutionize this process. Smart shelves can automatically track stock levels and alert you when it's time to restock. At the same time, inventory robots can perform regular stock checks and ensure accurate inventory counts, reducing the risk of overstocking or stockouts. (We will also discuss Inventory optimization later)
AI can help track inventory across warehouses and alert retailers if a product is available in another warehouse. It can also be automated to check if a customer has received a faulty product and send a replacement with the touch of a button. Thus, managing inventory becomes a cake with the help of Retail AI.
Enhancing Loss Prevention Strategies
Retail shrinkage, caused by theft and errors, is a significant concern for retailers. AI can enhance loss prevention strategies by analyzing surveillance footage, identifying suspicious behavior, and alerting staff in real-time.
Additionally, AI can help detect and reduce fraud at the checkout, ensuring a more secure retail environment. By integrating these AI solutions, you can significantly reduce shrinkage and protect your bottom line.
Next, let's dive into how AI can help you keep your shelves stocked perfectly with accurate demand forecasting and inventory optimization.
Like Retail AI can prevent retailers’ losses, Ragai AI can cut your losses in AI development. This article explains The costs of errors in application development.
Demand Forecasting and Inventory Optimization
Accurately forecasting demand and optimizing inventory is critical to meeting customer needs and maximizing profitability. Retail AI, particularly machine learning and predictive analytics, plays a crucial role in achieving these goals on two levels:
Predictive Analytics for Effective Inventory Management
Machine learning algorithms analyze customer behaviors and purchasing patterns to predict future demand. Their capacity to process vast data on millions of data points makes their predictions reliable. You can use their help to understand future demand in specific months, festivals, seasons, regions, etc.
By understanding these trends, you can ensure optimal stock levels, avoiding both overstocking and stockouts. This leads to a more efficient inventory system that aligns with customer demand.
Real-Time Data Analysis for Fast and Informed Decision-Making
Real-time data analysis allows you to make quick, informed decisions about your inventory. By continuously monitoring sales and stock levels, Retail AI provides immediate insights that help you respond to market changes swiftly. This ensures that your inventory is always aligned with current demand, improving overall operational efficiency and customer satisfaction.
Moving on, let's explore how conversational AI is changing the face of customer service for the better.
Conversational AI and Customer Service
Conversational AI, including chatbots, is revolutionizing how retailers interact with their customers, offering real-time assistance and enhancing overall satisfaction.
Conversational AI can engage customers in personalized interactions, guiding them through product selections, answering queries, and even assisting with purchases. Advanced AI can understand and respond to customers using:
Sentiment Analysis - AI tools analyze real-time customer interactions to gauge emotional states, allowing for customized responses that improve satisfaction and reduce churn.
Predictive Analysis - Machine learning enables predictive analytics, allowing you to anticipate customer needs and behaviors. By analyzing historical data, machine learning models can forecast customer churn, upsell opportunities, and even predict support ticket volume, enabling proactive customer service.
Voice Analysis - AI tools listen to conversations and find patterns like delight, frustration, etc., helping agents improve their performance and make customers happier.
Using these metrics, chatbots can elevate customer experience in many ways, including:
Tailored Recommendations - Chatbots analyze customer preferences and purchase history to offer personalized recommendations, including special promotions and discounts, increasing the chances of conversion.
Immediate Assistance - Available 24/7, chatbots provide instant help to customers, guiding them through the purchase process and informing them of ongoing promotions or discounts.
Automated Alerts - Chatbots proactively inform customers about upcoming sales events, limited-time offers, and exclusive discounts, creating a sense of urgency and encouraging quick action.
Order Tracking and Updates - In addition to promotional activities, chatbots enhance post-purchase interactions by providing real-time order tracking and updates, ensuring a smooth customer experience.
Feedback Gathering - Chatbots collect feedback from customers about their shopping experience, preferences, and satisfaction levels, allowing retailers to improve their promotional strategies and offerings.
Thus, conversational AI and chatbots help customers have a seamless experience. Speaking of seamless experiences, let's see how Retail AI is removing every possible barrier to make shopping hassle-free for your customers.
Learn more on a practical guide on deploying LLMs (Large Language Models) for your Retail AI.
Creating Frictionless Shopping Experiences
Retail AI is at the forefront of creating frictionless shopping experiences.
Customers today demand quick, hassle-free shopping experiences. They expect minimal wait times, easy navigation, and personalized service that makes their shopping journey enjoyable and efficient.
AI-powered solutions like smart carts, automated checkouts, and personalized mobile apps are transforming the shopping experience. Smart carts can track items as customers shop, while automated checkout systems allow for quick, contactless payments, eliminating the need for long queues.
Technologies like Intel® RealSense™ cameras are pushing the boundaries of autonomous store development. These advanced cameras can monitor inventory in real time, track customer movements, and enable seamless transactions without traditional checkout processes. This technology helps create a truly frictionless shopping environment where customers can simply pick up items and walk out, with their purchases automatically billed to their accounts.
Are you curious about how all this works in the real world? Let's check out some impressive case studies.
Case Studies
Retail AI has proven its worth for many businesses and companies. Here are a few examples:
Nordstrom Department Store Company, USA
Their objective was to use search keywords to boost products to the top of search results. They passed all of their images to Google and created the product-side images. At runtime, once they detected the object, they sent the object to their search API and then to Google Vision Product Search. This new design simplified their architectural design, cut down on service costs, and increased accuracy to over 95%.
Pitney Bowes, USA
They focused on cross-border business for retailers, which is a significant market opportunity as many consumers shop internationally. However, entering this market involved complexities that Pitney Bowes addressed through data, AI, and ML. One key area is fraud detection.
They used AutoML Tables from Google to enhance their fraud detection capabilities. This technology helped identify fraudulent orders while minimizing false positives to ensure a smooth consumer experience. By integrating ML models, particularly XGBoost, they reduced fraud loss rates by 49% and review efforts by 14%.
Walt Disney Company
Disney’s old recommendation system, based on product metadata, often gave irrelevant suggestions. By using Google's deep learning models training on user interactions, they achieved more accurate and personalized recommendations, leading to increased engagement and revenue.
Key takeaways include the importance of quality data, effective testing, and proper placement of recommendations. Working with Google also ensured scalability and high accuracy. Disney continues to use these models, enhancing customer experiences and boosting sales.
Others
Apart from these, many others have benefited from Retail AI. Here are some examples of companies taking advantage of AI chatbots:
Unilever - 14x higher sales. 6,335 unique user interactions in the first 12 hours
Tesco - 4X increase in digital catalog views. 13% decrease in general inquiries to the customer service team
Decathlon - 41% conversion rate (ad click to membership). 346% increase in member acquisition. 875% return on their Facebook ad spend.
Ben & Jerry’s - Sales over delivered by 20%.
Michael Kors - Retain users with a containment rate of 98.32%. 45,000 new users per month. It generated more than 375,000 active users.
Lego - 25% of all in-season online sales, with a 6x return on ad spend within certain markets. 8.4x higher conversion rate.
Alibaba - Responded 95% of the customer services on Alibaba e-commerce platforms. We generated $31 billion in gross merchandise volume.
L'Oréal - 27X higher engagement than email.
These studies show the viability and outreach of Retail AI in the current market. But it's not all sunshine and rainbows; let’s tackle some of the challenges you might face with Retail AI.
Read this article to learn more about various case studies on AI adoption.
Challenges of Retail AI
While Retail AI offers numerous benefits, it also presents certain challenges that retailers must navigate to fully harness its potential.
Data Privacy and Security
One of the primary challenges of Retail AI is ensuring the privacy and security of customer data. Retailers must comply with stringent data protection regulations and implement robust security measures to protect sensitive information from breaches and unauthorized access.
With the aim of building the first automated AI testing platform, Raga AI Governance Hub can help you with AI governance, risk management, and regulatory compliance needs.
Integration with Existing Systems
Integrating AI solutions with existing retail systems can be complex and resource-intensive. Retailers often need help in seamlessly merging new AI technologies with their current infrastructure, requiring significant investment in time and resources to achieve smooth integration.
Cost and ROI Considerations
Implementing AI technologies can be expensive, and retailers need to carefully consider the cost versus the potential return on investment (ROI). While AI can drive significant efficiencies and revenue growth, the initial investment and ongoing maintenance costs can be a barrier, especially for smaller retailers.
Skill Gaps and Workforce Adaptation
The adoption of AI in retail requires a workforce skilled in AI and data analytics. However, there is often a gap between the required skills and the workforce's current capabilities. Retailers must invest in training and development to equip their employees with the necessary skills to work effectively with AI technologies.
Ethical and Bias Concerns
AI systems can inadvertently perpetuate biases present in the data they are trained on, leading to unfair or discriminatory outcomes. Retailers must be vigilant in ensuring their AI solutions are ethical and unbiased, which involves continuous monitoring and adjustments to the algorithms.
Addressing these challenges is crucial for retailers to leverage AI effectively and sustainably, ensuring they can fully benefit from its transformative potential. However, even with its challenges, Retail AI shows good promise as it eases retail business with many advantages.
Future Prospects
The future of AI in retail holds immense promise as technology continues to evolve, driving innovations that redefine how retailers engage and personalize customer experiences:
Emerging Trends in AI and Retail
Conversational AI and Chatbots are set to revolutionize customer service and engagement, offering hyper-personalized recommendations and seamless voice-based transactions. According to Juniper Research, retail chatbots are projected to save over $8 billion in customer service costs globally by 2026.
AI-powered Personalization Engines will become more sophisticated, integrating diverse data points such as weather conditions and social media sentiment to deliver real-time, personalized product suggestions. Accenture highlights that 75% of consumers now expect tailored offers based on their preferences.
The Integration of AI with Augmented Reality (AR) is poised to enhance the retail experience with immersive features like virtual try-ons and interactive product visualization. Technavio forecasts the AR market in retail to reach $84.67 billion by 2025.
The Future of AI in Retail Personalization
These trends forecast significant advancements in AI-driven retail personalization:
Hyper-localized Marketing strategies will enable retailers to tailor marketing campaigns based on local trends and consumer preferences, enhancing relevance and engagement.
Predictive Inventory Management powered by AI will optimize stock levels by analyzing customer data, predicting demand patterns, and minimizing stockouts and overstocking.
Personalized Pricing and Promotions will leverage AI algorithms to determine optimal pricing strategies based on individual customer behaviors and market dynamics.
Read this article to learn more about the future of AI Testing.
Strategies for Staying Ahead
To capitalize on these opportunities, retailers should adopt proactive strategies:
Invest in AI Expertise by cultivating internal AI capabilities or collaborating with specialized retail AI providers.
Prioritize Data Security and Privacy to build trust and compliance with stringent data protection regulations.
Embrace an Experimental Culture to innovate and refine AI-powered solutions for enhanced customer experiences continuously.
This proactive approach ensures retailers are well-positioned to harness AI's transformative potential and shape the future of retail personalization.
Conclusion
In conclusion, AI represents a transformative force in the retail industry, poised to elevate customer experiences to new heights. From personalized recommendations and seamless transactions facilitated by chatbots to immersive augmented reality applications, AI is reshaping how retailers engage with their customers. Embracing AI technologies is no longer just an option but a strategic imperative for retailers aiming to maintain a competitive edge in a rapidly evolving market.
However, integrating AI into a retail business model is cumbersome and attracts errors. Raga AI offers a one-stop shop for all your testing needs. You can also leverage tools like Raga AI LLM Hub, Testing Platform, and Governance Hub to ensure the quality of your LLM. Raga’s tools perform comprehensive testing for RAG applications and add guardrails to prevent adversarial attacks.
Using Raga AI, you can be sure that you get the best quality context, AI governance, risk management, and regulatory compliance. So give Raga AI a try, or Book a Demo today.
In today's retail world, Artificial Intelligence (AI) is more than just a buzzword—it's a game-changer. Imagine being able to offer your customers personalized experiences that make them feel valued and understood while also running your operations smoothly and efficiently. That's the power of Retail AI.
It doesn't just improve customer service; it transforms it, turning every interaction into an opportunity to delight your customers. With Retail AI, you can tailor your offerings to meet individual preferences, predict trends before they happen, and streamline your back-end processes. This means happier customers and a more profitable business.
Let’s look at how AI can be your secret weapon for enhanced retail customer experiences.
Personalization and Customer Engagement
In the evolving retail landscape, personalization has become crucial for effectively engaging customers. Retail AI plays a pivotal role in this transformation, using advanced data analysis to understand and meet customer needs.
But you might ask: Is personalization necessary? Will it change the retail landscape? So, let’s discuss its importance in light of current user expectations.
Importance of Personalization
In today's competitive market, customers expect interactions tailored to their preferences and behaviors. However, many retailers need help in achieving true personalization, such as handling vast amounts of data, integrating disparate systems, and maintaining customer privacy.
For example, a retailer might struggle to analyze data from both online and in-store purchases to create a cohesive customer profile. Retail AI offers effective solutions to these challenges by analyzing customer data in real time, predicting trends, and automating personalized recommendations.
For instance, AI can suggest products based on past purchases or browsing history, and in-store digital signage can display personalized offers as customers walk by. This technology ensures that every customer interaction is relevant and engaging, ultimately overcoming the hurdles and transforming the shopping experience into a personalized journey.
Retail AI handles personalization and customer engagement with a two-part solution:
Understanding and Meeting Customer Needs
Retail AI analyzes vast amounts of customer data to uncover patterns and preferences. This deep understanding allows you to anticipate what your customers want and tailor your offerings to meet their specific needs, creating a more personalized shopping experience. This can include data from web interactions, surveys, feedback, past purchases, etc.
However, you should also remember that having a robust AI testing platform to maintain this accuracy and integrity is important. Tools like Raga AI offer a one-stop solution for testing, adding guardrails, and AI governance.
Creating Personalized Shopping Experiences
By leveraging AI technologies such as digital signage and POS systems, you can create highly personalized shopping experiences. Digital signage can display tailored promotions and product recommendations, while AI-powered POS systems can offer customized discounts and loyalty rewards at checkout.
You can also use AI-driven websites to give each customer a personalized experience. This experience mainly has two factors:
Dynamic Pricing: Real-time changes to prices on demand, supply, and competition changes.
Targeted Promotions and Discounts: Personalized discounts based on past purchases, promotional images, videos, product suggestions, etc.
Benefits of Personalization for Retailers
Personalization through Retail AI not only enhances customer satisfaction but also drives brand loyalty and revenue growth. When customers feel understood and valued, they are more likely to return, leading to increased sales and long-term profitability for your business.
Product data can be overwhelming, especially for online retail stores. Users often need more time to search for the right product. A store serving products on old data frames will push its users away. However, analyzing data and predicting user behavior can help you serve personalized products to your customers.
Now, let’s shift gears and talk about how Retail AI can streamline your operations for maximum efficiency.
Streamlining Retail Operations
In the fast-paced world of retail, operational efficiency is key to maintaining a competitive edge. Retail AI offers innovative solutions to make decision-making more informed and processes more efficient. It streamlines the following aspects of operations:
Operational Efficiency and Decision Making
Retail AI enhances operational efficiency by providing real-time insights and predictive analytics, helping you make data-driven decisions. From optimizing staffing levels to forecasting demand (which we will discuss in detail), AI enables you to run your operations smoothly and effectively.
It can accelerate repetitive customer care tasks, like helping with common issues via automated chats. You can also use AI assistants to help employees handle their tasks effectively. Similarly, voice assistants can free a customer of web application ordering hassles. All of these methods help in the operational and decision-making process.
Improving Inventory Management through AI Technologies
Managing inventory is a critical challenge for retailers. AI technologies like smart shelves and inventory robots revolutionize this process. Smart shelves can automatically track stock levels and alert you when it's time to restock. At the same time, inventory robots can perform regular stock checks and ensure accurate inventory counts, reducing the risk of overstocking or stockouts. (We will also discuss Inventory optimization later)
AI can help track inventory across warehouses and alert retailers if a product is available in another warehouse. It can also be automated to check if a customer has received a faulty product and send a replacement with the touch of a button. Thus, managing inventory becomes a cake with the help of Retail AI.
Enhancing Loss Prevention Strategies
Retail shrinkage, caused by theft and errors, is a significant concern for retailers. AI can enhance loss prevention strategies by analyzing surveillance footage, identifying suspicious behavior, and alerting staff in real-time.
Additionally, AI can help detect and reduce fraud at the checkout, ensuring a more secure retail environment. By integrating these AI solutions, you can significantly reduce shrinkage and protect your bottom line.
Next, let's dive into how AI can help you keep your shelves stocked perfectly with accurate demand forecasting and inventory optimization.
Like Retail AI can prevent retailers’ losses, Ragai AI can cut your losses in AI development. This article explains The costs of errors in application development.
Demand Forecasting and Inventory Optimization
Accurately forecasting demand and optimizing inventory is critical to meeting customer needs and maximizing profitability. Retail AI, particularly machine learning and predictive analytics, plays a crucial role in achieving these goals on two levels:
Predictive Analytics for Effective Inventory Management
Machine learning algorithms analyze customer behaviors and purchasing patterns to predict future demand. Their capacity to process vast data on millions of data points makes their predictions reliable. You can use their help to understand future demand in specific months, festivals, seasons, regions, etc.
By understanding these trends, you can ensure optimal stock levels, avoiding both overstocking and stockouts. This leads to a more efficient inventory system that aligns with customer demand.
Real-Time Data Analysis for Fast and Informed Decision-Making
Real-time data analysis allows you to make quick, informed decisions about your inventory. By continuously monitoring sales and stock levels, Retail AI provides immediate insights that help you respond to market changes swiftly. This ensures that your inventory is always aligned with current demand, improving overall operational efficiency and customer satisfaction.
Moving on, let's explore how conversational AI is changing the face of customer service for the better.
Conversational AI and Customer Service
Conversational AI, including chatbots, is revolutionizing how retailers interact with their customers, offering real-time assistance and enhancing overall satisfaction.
Conversational AI can engage customers in personalized interactions, guiding them through product selections, answering queries, and even assisting with purchases. Advanced AI can understand and respond to customers using:
Sentiment Analysis - AI tools analyze real-time customer interactions to gauge emotional states, allowing for customized responses that improve satisfaction and reduce churn.
Predictive Analysis - Machine learning enables predictive analytics, allowing you to anticipate customer needs and behaviors. By analyzing historical data, machine learning models can forecast customer churn, upsell opportunities, and even predict support ticket volume, enabling proactive customer service.
Voice Analysis - AI tools listen to conversations and find patterns like delight, frustration, etc., helping agents improve their performance and make customers happier.
Using these metrics, chatbots can elevate customer experience in many ways, including:
Tailored Recommendations - Chatbots analyze customer preferences and purchase history to offer personalized recommendations, including special promotions and discounts, increasing the chances of conversion.
Immediate Assistance - Available 24/7, chatbots provide instant help to customers, guiding them through the purchase process and informing them of ongoing promotions or discounts.
Automated Alerts - Chatbots proactively inform customers about upcoming sales events, limited-time offers, and exclusive discounts, creating a sense of urgency and encouraging quick action.
Order Tracking and Updates - In addition to promotional activities, chatbots enhance post-purchase interactions by providing real-time order tracking and updates, ensuring a smooth customer experience.
Feedback Gathering - Chatbots collect feedback from customers about their shopping experience, preferences, and satisfaction levels, allowing retailers to improve their promotional strategies and offerings.
Thus, conversational AI and chatbots help customers have a seamless experience. Speaking of seamless experiences, let's see how Retail AI is removing every possible barrier to make shopping hassle-free for your customers.
Learn more on a practical guide on deploying LLMs (Large Language Models) for your Retail AI.
Creating Frictionless Shopping Experiences
Retail AI is at the forefront of creating frictionless shopping experiences.
Customers today demand quick, hassle-free shopping experiences. They expect minimal wait times, easy navigation, and personalized service that makes their shopping journey enjoyable and efficient.
AI-powered solutions like smart carts, automated checkouts, and personalized mobile apps are transforming the shopping experience. Smart carts can track items as customers shop, while automated checkout systems allow for quick, contactless payments, eliminating the need for long queues.
Technologies like Intel® RealSense™ cameras are pushing the boundaries of autonomous store development. These advanced cameras can monitor inventory in real time, track customer movements, and enable seamless transactions without traditional checkout processes. This technology helps create a truly frictionless shopping environment where customers can simply pick up items and walk out, with their purchases automatically billed to their accounts.
Are you curious about how all this works in the real world? Let's check out some impressive case studies.
Case Studies
Retail AI has proven its worth for many businesses and companies. Here are a few examples:
Nordstrom Department Store Company, USA
Their objective was to use search keywords to boost products to the top of search results. They passed all of their images to Google and created the product-side images. At runtime, once they detected the object, they sent the object to their search API and then to Google Vision Product Search. This new design simplified their architectural design, cut down on service costs, and increased accuracy to over 95%.
Pitney Bowes, USA
They focused on cross-border business for retailers, which is a significant market opportunity as many consumers shop internationally. However, entering this market involved complexities that Pitney Bowes addressed through data, AI, and ML. One key area is fraud detection.
They used AutoML Tables from Google to enhance their fraud detection capabilities. This technology helped identify fraudulent orders while minimizing false positives to ensure a smooth consumer experience. By integrating ML models, particularly XGBoost, they reduced fraud loss rates by 49% and review efforts by 14%.
Walt Disney Company
Disney’s old recommendation system, based on product metadata, often gave irrelevant suggestions. By using Google's deep learning models training on user interactions, they achieved more accurate and personalized recommendations, leading to increased engagement and revenue.
Key takeaways include the importance of quality data, effective testing, and proper placement of recommendations. Working with Google also ensured scalability and high accuracy. Disney continues to use these models, enhancing customer experiences and boosting sales.
Others
Apart from these, many others have benefited from Retail AI. Here are some examples of companies taking advantage of AI chatbots:
Unilever - 14x higher sales. 6,335 unique user interactions in the first 12 hours
Tesco - 4X increase in digital catalog views. 13% decrease in general inquiries to the customer service team
Decathlon - 41% conversion rate (ad click to membership). 346% increase in member acquisition. 875% return on their Facebook ad spend.
Ben & Jerry’s - Sales over delivered by 20%.
Michael Kors - Retain users with a containment rate of 98.32%. 45,000 new users per month. It generated more than 375,000 active users.
Lego - 25% of all in-season online sales, with a 6x return on ad spend within certain markets. 8.4x higher conversion rate.
Alibaba - Responded 95% of the customer services on Alibaba e-commerce platforms. We generated $31 billion in gross merchandise volume.
L'Oréal - 27X higher engagement than email.
These studies show the viability and outreach of Retail AI in the current market. But it's not all sunshine and rainbows; let’s tackle some of the challenges you might face with Retail AI.
Read this article to learn more about various case studies on AI adoption.
Challenges of Retail AI
While Retail AI offers numerous benefits, it also presents certain challenges that retailers must navigate to fully harness its potential.
Data Privacy and Security
One of the primary challenges of Retail AI is ensuring the privacy and security of customer data. Retailers must comply with stringent data protection regulations and implement robust security measures to protect sensitive information from breaches and unauthorized access.
With the aim of building the first automated AI testing platform, Raga AI Governance Hub can help you with AI governance, risk management, and regulatory compliance needs.
Integration with Existing Systems
Integrating AI solutions with existing retail systems can be complex and resource-intensive. Retailers often need help in seamlessly merging new AI technologies with their current infrastructure, requiring significant investment in time and resources to achieve smooth integration.
Cost and ROI Considerations
Implementing AI technologies can be expensive, and retailers need to carefully consider the cost versus the potential return on investment (ROI). While AI can drive significant efficiencies and revenue growth, the initial investment and ongoing maintenance costs can be a barrier, especially for smaller retailers.
Skill Gaps and Workforce Adaptation
The adoption of AI in retail requires a workforce skilled in AI and data analytics. However, there is often a gap between the required skills and the workforce's current capabilities. Retailers must invest in training and development to equip their employees with the necessary skills to work effectively with AI technologies.
Ethical and Bias Concerns
AI systems can inadvertently perpetuate biases present in the data they are trained on, leading to unfair or discriminatory outcomes. Retailers must be vigilant in ensuring their AI solutions are ethical and unbiased, which involves continuous monitoring and adjustments to the algorithms.
Addressing these challenges is crucial for retailers to leverage AI effectively and sustainably, ensuring they can fully benefit from its transformative potential. However, even with its challenges, Retail AI shows good promise as it eases retail business with many advantages.
Future Prospects
The future of AI in retail holds immense promise as technology continues to evolve, driving innovations that redefine how retailers engage and personalize customer experiences:
Emerging Trends in AI and Retail
Conversational AI and Chatbots are set to revolutionize customer service and engagement, offering hyper-personalized recommendations and seamless voice-based transactions. According to Juniper Research, retail chatbots are projected to save over $8 billion in customer service costs globally by 2026.
AI-powered Personalization Engines will become more sophisticated, integrating diverse data points such as weather conditions and social media sentiment to deliver real-time, personalized product suggestions. Accenture highlights that 75% of consumers now expect tailored offers based on their preferences.
The Integration of AI with Augmented Reality (AR) is poised to enhance the retail experience with immersive features like virtual try-ons and interactive product visualization. Technavio forecasts the AR market in retail to reach $84.67 billion by 2025.
The Future of AI in Retail Personalization
These trends forecast significant advancements in AI-driven retail personalization:
Hyper-localized Marketing strategies will enable retailers to tailor marketing campaigns based on local trends and consumer preferences, enhancing relevance and engagement.
Predictive Inventory Management powered by AI will optimize stock levels by analyzing customer data, predicting demand patterns, and minimizing stockouts and overstocking.
Personalized Pricing and Promotions will leverage AI algorithms to determine optimal pricing strategies based on individual customer behaviors and market dynamics.
Read this article to learn more about the future of AI Testing.
Strategies for Staying Ahead
To capitalize on these opportunities, retailers should adopt proactive strategies:
Invest in AI Expertise by cultivating internal AI capabilities or collaborating with specialized retail AI providers.
Prioritize Data Security and Privacy to build trust and compliance with stringent data protection regulations.
Embrace an Experimental Culture to innovate and refine AI-powered solutions for enhanced customer experiences continuously.
This proactive approach ensures retailers are well-positioned to harness AI's transformative potential and shape the future of retail personalization.
Conclusion
In conclusion, AI represents a transformative force in the retail industry, poised to elevate customer experiences to new heights. From personalized recommendations and seamless transactions facilitated by chatbots to immersive augmented reality applications, AI is reshaping how retailers engage with their customers. Embracing AI technologies is no longer just an option but a strategic imperative for retailers aiming to maintain a competitive edge in a rapidly evolving market.
However, integrating AI into a retail business model is cumbersome and attracts errors. Raga AI offers a one-stop shop for all your testing needs. You can also leverage tools like Raga AI LLM Hub, Testing Platform, and Governance Hub to ensure the quality of your LLM. Raga’s tools perform comprehensive testing for RAG applications and add guardrails to prevent adversarial attacks.
Using Raga AI, you can be sure that you get the best quality context, AI governance, risk management, and regulatory compliance. So give Raga AI a try, or Book a Demo today.
In today's retail world, Artificial Intelligence (AI) is more than just a buzzword—it's a game-changer. Imagine being able to offer your customers personalized experiences that make them feel valued and understood while also running your operations smoothly and efficiently. That's the power of Retail AI.
It doesn't just improve customer service; it transforms it, turning every interaction into an opportunity to delight your customers. With Retail AI, you can tailor your offerings to meet individual preferences, predict trends before they happen, and streamline your back-end processes. This means happier customers and a more profitable business.
Let’s look at how AI can be your secret weapon for enhanced retail customer experiences.
Personalization and Customer Engagement
In the evolving retail landscape, personalization has become crucial for effectively engaging customers. Retail AI plays a pivotal role in this transformation, using advanced data analysis to understand and meet customer needs.
But you might ask: Is personalization necessary? Will it change the retail landscape? So, let’s discuss its importance in light of current user expectations.
Importance of Personalization
In today's competitive market, customers expect interactions tailored to their preferences and behaviors. However, many retailers need help in achieving true personalization, such as handling vast amounts of data, integrating disparate systems, and maintaining customer privacy.
For example, a retailer might struggle to analyze data from both online and in-store purchases to create a cohesive customer profile. Retail AI offers effective solutions to these challenges by analyzing customer data in real time, predicting trends, and automating personalized recommendations.
For instance, AI can suggest products based on past purchases or browsing history, and in-store digital signage can display personalized offers as customers walk by. This technology ensures that every customer interaction is relevant and engaging, ultimately overcoming the hurdles and transforming the shopping experience into a personalized journey.
Retail AI handles personalization and customer engagement with a two-part solution:
Understanding and Meeting Customer Needs
Retail AI analyzes vast amounts of customer data to uncover patterns and preferences. This deep understanding allows you to anticipate what your customers want and tailor your offerings to meet their specific needs, creating a more personalized shopping experience. This can include data from web interactions, surveys, feedback, past purchases, etc.
However, you should also remember that having a robust AI testing platform to maintain this accuracy and integrity is important. Tools like Raga AI offer a one-stop solution for testing, adding guardrails, and AI governance.
Creating Personalized Shopping Experiences
By leveraging AI technologies such as digital signage and POS systems, you can create highly personalized shopping experiences. Digital signage can display tailored promotions and product recommendations, while AI-powered POS systems can offer customized discounts and loyalty rewards at checkout.
You can also use AI-driven websites to give each customer a personalized experience. This experience mainly has two factors:
Dynamic Pricing: Real-time changes to prices on demand, supply, and competition changes.
Targeted Promotions and Discounts: Personalized discounts based on past purchases, promotional images, videos, product suggestions, etc.
Benefits of Personalization for Retailers
Personalization through Retail AI not only enhances customer satisfaction but also drives brand loyalty and revenue growth. When customers feel understood and valued, they are more likely to return, leading to increased sales and long-term profitability for your business.
Product data can be overwhelming, especially for online retail stores. Users often need more time to search for the right product. A store serving products on old data frames will push its users away. However, analyzing data and predicting user behavior can help you serve personalized products to your customers.
Now, let’s shift gears and talk about how Retail AI can streamline your operations for maximum efficiency.
Streamlining Retail Operations
In the fast-paced world of retail, operational efficiency is key to maintaining a competitive edge. Retail AI offers innovative solutions to make decision-making more informed and processes more efficient. It streamlines the following aspects of operations:
Operational Efficiency and Decision Making
Retail AI enhances operational efficiency by providing real-time insights and predictive analytics, helping you make data-driven decisions. From optimizing staffing levels to forecasting demand (which we will discuss in detail), AI enables you to run your operations smoothly and effectively.
It can accelerate repetitive customer care tasks, like helping with common issues via automated chats. You can also use AI assistants to help employees handle their tasks effectively. Similarly, voice assistants can free a customer of web application ordering hassles. All of these methods help in the operational and decision-making process.
Improving Inventory Management through AI Technologies
Managing inventory is a critical challenge for retailers. AI technologies like smart shelves and inventory robots revolutionize this process. Smart shelves can automatically track stock levels and alert you when it's time to restock. At the same time, inventory robots can perform regular stock checks and ensure accurate inventory counts, reducing the risk of overstocking or stockouts. (We will also discuss Inventory optimization later)
AI can help track inventory across warehouses and alert retailers if a product is available in another warehouse. It can also be automated to check if a customer has received a faulty product and send a replacement with the touch of a button. Thus, managing inventory becomes a cake with the help of Retail AI.
Enhancing Loss Prevention Strategies
Retail shrinkage, caused by theft and errors, is a significant concern for retailers. AI can enhance loss prevention strategies by analyzing surveillance footage, identifying suspicious behavior, and alerting staff in real-time.
Additionally, AI can help detect and reduce fraud at the checkout, ensuring a more secure retail environment. By integrating these AI solutions, you can significantly reduce shrinkage and protect your bottom line.
Next, let's dive into how AI can help you keep your shelves stocked perfectly with accurate demand forecasting and inventory optimization.
Like Retail AI can prevent retailers’ losses, Ragai AI can cut your losses in AI development. This article explains The costs of errors in application development.
Demand Forecasting and Inventory Optimization
Accurately forecasting demand and optimizing inventory is critical to meeting customer needs and maximizing profitability. Retail AI, particularly machine learning and predictive analytics, plays a crucial role in achieving these goals on two levels:
Predictive Analytics for Effective Inventory Management
Machine learning algorithms analyze customer behaviors and purchasing patterns to predict future demand. Their capacity to process vast data on millions of data points makes their predictions reliable. You can use their help to understand future demand in specific months, festivals, seasons, regions, etc.
By understanding these trends, you can ensure optimal stock levels, avoiding both overstocking and stockouts. This leads to a more efficient inventory system that aligns with customer demand.
Real-Time Data Analysis for Fast and Informed Decision-Making
Real-time data analysis allows you to make quick, informed decisions about your inventory. By continuously monitoring sales and stock levels, Retail AI provides immediate insights that help you respond to market changes swiftly. This ensures that your inventory is always aligned with current demand, improving overall operational efficiency and customer satisfaction.
Moving on, let's explore how conversational AI is changing the face of customer service for the better.
Conversational AI and Customer Service
Conversational AI, including chatbots, is revolutionizing how retailers interact with their customers, offering real-time assistance and enhancing overall satisfaction.
Conversational AI can engage customers in personalized interactions, guiding them through product selections, answering queries, and even assisting with purchases. Advanced AI can understand and respond to customers using:
Sentiment Analysis - AI tools analyze real-time customer interactions to gauge emotional states, allowing for customized responses that improve satisfaction and reduce churn.
Predictive Analysis - Machine learning enables predictive analytics, allowing you to anticipate customer needs and behaviors. By analyzing historical data, machine learning models can forecast customer churn, upsell opportunities, and even predict support ticket volume, enabling proactive customer service.
Voice Analysis - AI tools listen to conversations and find patterns like delight, frustration, etc., helping agents improve their performance and make customers happier.
Using these metrics, chatbots can elevate customer experience in many ways, including:
Tailored Recommendations - Chatbots analyze customer preferences and purchase history to offer personalized recommendations, including special promotions and discounts, increasing the chances of conversion.
Immediate Assistance - Available 24/7, chatbots provide instant help to customers, guiding them through the purchase process and informing them of ongoing promotions or discounts.
Automated Alerts - Chatbots proactively inform customers about upcoming sales events, limited-time offers, and exclusive discounts, creating a sense of urgency and encouraging quick action.
Order Tracking and Updates - In addition to promotional activities, chatbots enhance post-purchase interactions by providing real-time order tracking and updates, ensuring a smooth customer experience.
Feedback Gathering - Chatbots collect feedback from customers about their shopping experience, preferences, and satisfaction levels, allowing retailers to improve their promotional strategies and offerings.
Thus, conversational AI and chatbots help customers have a seamless experience. Speaking of seamless experiences, let's see how Retail AI is removing every possible barrier to make shopping hassle-free for your customers.
Learn more on a practical guide on deploying LLMs (Large Language Models) for your Retail AI.
Creating Frictionless Shopping Experiences
Retail AI is at the forefront of creating frictionless shopping experiences.
Customers today demand quick, hassle-free shopping experiences. They expect minimal wait times, easy navigation, and personalized service that makes their shopping journey enjoyable and efficient.
AI-powered solutions like smart carts, automated checkouts, and personalized mobile apps are transforming the shopping experience. Smart carts can track items as customers shop, while automated checkout systems allow for quick, contactless payments, eliminating the need for long queues.
Technologies like Intel® RealSense™ cameras are pushing the boundaries of autonomous store development. These advanced cameras can monitor inventory in real time, track customer movements, and enable seamless transactions without traditional checkout processes. This technology helps create a truly frictionless shopping environment where customers can simply pick up items and walk out, with their purchases automatically billed to their accounts.
Are you curious about how all this works in the real world? Let's check out some impressive case studies.
Case Studies
Retail AI has proven its worth for many businesses and companies. Here are a few examples:
Nordstrom Department Store Company, USA
Their objective was to use search keywords to boost products to the top of search results. They passed all of their images to Google and created the product-side images. At runtime, once they detected the object, they sent the object to their search API and then to Google Vision Product Search. This new design simplified their architectural design, cut down on service costs, and increased accuracy to over 95%.
Pitney Bowes, USA
They focused on cross-border business for retailers, which is a significant market opportunity as many consumers shop internationally. However, entering this market involved complexities that Pitney Bowes addressed through data, AI, and ML. One key area is fraud detection.
They used AutoML Tables from Google to enhance their fraud detection capabilities. This technology helped identify fraudulent orders while minimizing false positives to ensure a smooth consumer experience. By integrating ML models, particularly XGBoost, they reduced fraud loss rates by 49% and review efforts by 14%.
Walt Disney Company
Disney’s old recommendation system, based on product metadata, often gave irrelevant suggestions. By using Google's deep learning models training on user interactions, they achieved more accurate and personalized recommendations, leading to increased engagement and revenue.
Key takeaways include the importance of quality data, effective testing, and proper placement of recommendations. Working with Google also ensured scalability and high accuracy. Disney continues to use these models, enhancing customer experiences and boosting sales.
Others
Apart from these, many others have benefited from Retail AI. Here are some examples of companies taking advantage of AI chatbots:
Unilever - 14x higher sales. 6,335 unique user interactions in the first 12 hours
Tesco - 4X increase in digital catalog views. 13% decrease in general inquiries to the customer service team
Decathlon - 41% conversion rate (ad click to membership). 346% increase in member acquisition. 875% return on their Facebook ad spend.
Ben & Jerry’s - Sales over delivered by 20%.
Michael Kors - Retain users with a containment rate of 98.32%. 45,000 new users per month. It generated more than 375,000 active users.
Lego - 25% of all in-season online sales, with a 6x return on ad spend within certain markets. 8.4x higher conversion rate.
Alibaba - Responded 95% of the customer services on Alibaba e-commerce platforms. We generated $31 billion in gross merchandise volume.
L'Oréal - 27X higher engagement than email.
These studies show the viability and outreach of Retail AI in the current market. But it's not all sunshine and rainbows; let’s tackle some of the challenges you might face with Retail AI.
Read this article to learn more about various case studies on AI adoption.
Challenges of Retail AI
While Retail AI offers numerous benefits, it also presents certain challenges that retailers must navigate to fully harness its potential.
Data Privacy and Security
One of the primary challenges of Retail AI is ensuring the privacy and security of customer data. Retailers must comply with stringent data protection regulations and implement robust security measures to protect sensitive information from breaches and unauthorized access.
With the aim of building the first automated AI testing platform, Raga AI Governance Hub can help you with AI governance, risk management, and regulatory compliance needs.
Integration with Existing Systems
Integrating AI solutions with existing retail systems can be complex and resource-intensive. Retailers often need help in seamlessly merging new AI technologies with their current infrastructure, requiring significant investment in time and resources to achieve smooth integration.
Cost and ROI Considerations
Implementing AI technologies can be expensive, and retailers need to carefully consider the cost versus the potential return on investment (ROI). While AI can drive significant efficiencies and revenue growth, the initial investment and ongoing maintenance costs can be a barrier, especially for smaller retailers.
Skill Gaps and Workforce Adaptation
The adoption of AI in retail requires a workforce skilled in AI and data analytics. However, there is often a gap between the required skills and the workforce's current capabilities. Retailers must invest in training and development to equip their employees with the necessary skills to work effectively with AI technologies.
Ethical and Bias Concerns
AI systems can inadvertently perpetuate biases present in the data they are trained on, leading to unfair or discriminatory outcomes. Retailers must be vigilant in ensuring their AI solutions are ethical and unbiased, which involves continuous monitoring and adjustments to the algorithms.
Addressing these challenges is crucial for retailers to leverage AI effectively and sustainably, ensuring they can fully benefit from its transformative potential. However, even with its challenges, Retail AI shows good promise as it eases retail business with many advantages.
Future Prospects
The future of AI in retail holds immense promise as technology continues to evolve, driving innovations that redefine how retailers engage and personalize customer experiences:
Emerging Trends in AI and Retail
Conversational AI and Chatbots are set to revolutionize customer service and engagement, offering hyper-personalized recommendations and seamless voice-based transactions. According to Juniper Research, retail chatbots are projected to save over $8 billion in customer service costs globally by 2026.
AI-powered Personalization Engines will become more sophisticated, integrating diverse data points such as weather conditions and social media sentiment to deliver real-time, personalized product suggestions. Accenture highlights that 75% of consumers now expect tailored offers based on their preferences.
The Integration of AI with Augmented Reality (AR) is poised to enhance the retail experience with immersive features like virtual try-ons and interactive product visualization. Technavio forecasts the AR market in retail to reach $84.67 billion by 2025.
The Future of AI in Retail Personalization
These trends forecast significant advancements in AI-driven retail personalization:
Hyper-localized Marketing strategies will enable retailers to tailor marketing campaigns based on local trends and consumer preferences, enhancing relevance and engagement.
Predictive Inventory Management powered by AI will optimize stock levels by analyzing customer data, predicting demand patterns, and minimizing stockouts and overstocking.
Personalized Pricing and Promotions will leverage AI algorithms to determine optimal pricing strategies based on individual customer behaviors and market dynamics.
Read this article to learn more about the future of AI Testing.
Strategies for Staying Ahead
To capitalize on these opportunities, retailers should adopt proactive strategies:
Invest in AI Expertise by cultivating internal AI capabilities or collaborating with specialized retail AI providers.
Prioritize Data Security and Privacy to build trust and compliance with stringent data protection regulations.
Embrace an Experimental Culture to innovate and refine AI-powered solutions for enhanced customer experiences continuously.
This proactive approach ensures retailers are well-positioned to harness AI's transformative potential and shape the future of retail personalization.
Conclusion
In conclusion, AI represents a transformative force in the retail industry, poised to elevate customer experiences to new heights. From personalized recommendations and seamless transactions facilitated by chatbots to immersive augmented reality applications, AI is reshaping how retailers engage with their customers. Embracing AI technologies is no longer just an option but a strategic imperative for retailers aiming to maintain a competitive edge in a rapidly evolving market.
However, integrating AI into a retail business model is cumbersome and attracts errors. Raga AI offers a one-stop shop for all your testing needs. You can also leverage tools like Raga AI LLM Hub, Testing Platform, and Governance Hub to ensure the quality of your LLM. Raga’s tools perform comprehensive testing for RAG applications and add guardrails to prevent adversarial attacks.
Using Raga AI, you can be sure that you get the best quality context, AI governance, risk management, and regulatory compliance. So give Raga AI a try, or Book a Demo today.
Subscribe to our newsletter to never miss an update
Other articles


Agents Talk, Models Think: A2A + MCP for Enterprise Agentic AI

Riya Parikh, Nitai Agarwal
Apr 27, 2025
Read the article
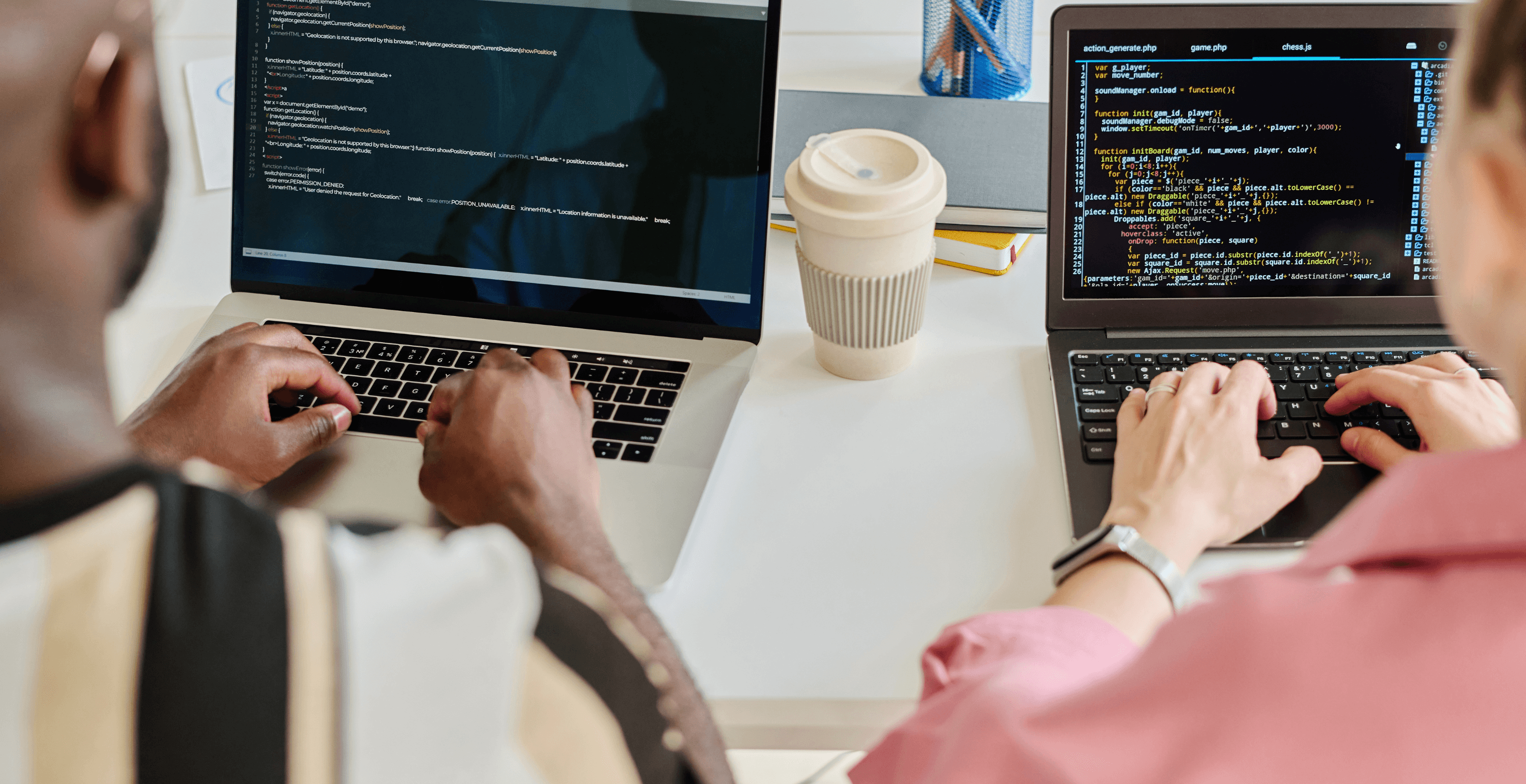
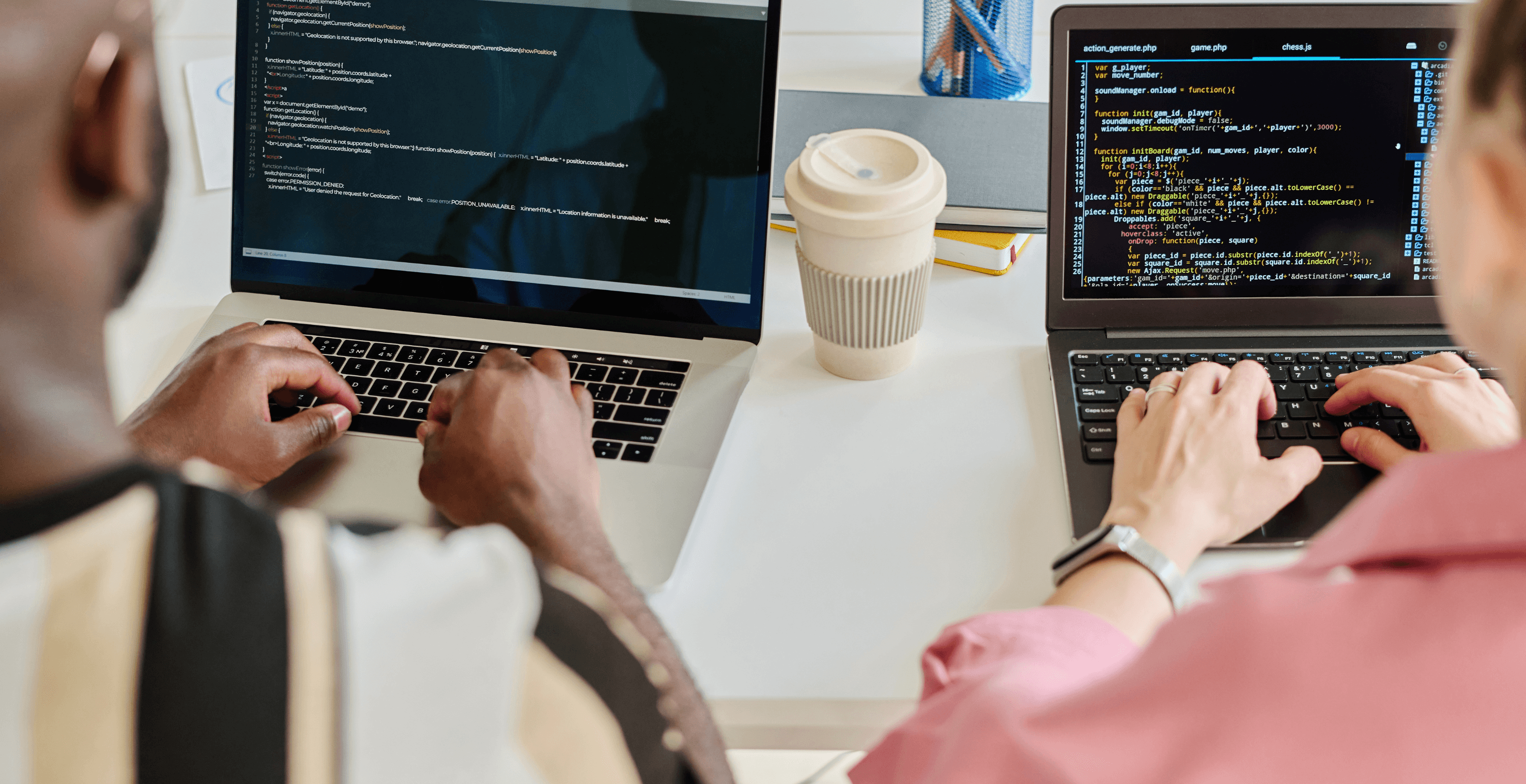
Understanding NeMo Guardrails: A Toolkit for LLM Security

Rehan Asif
Dec 24, 2024
Read the article


Understanding Differences in Large vs Small Language Models (LLM vs SLM)

Rehan Asif
Dec 21, 2024
Read the article