Success Stories And Case Studies Of AI Adoption Across Industries
Success Stories And Case Studies Of AI Adoption Across Industries
Success Stories And Case Studies Of AI Adoption Across Industries
Jigar Gupta
May 1, 2024




AI, a combination of machine learning, data analytics, and automation, is not just a futuristic concept but a present-day powerhouse driving innovation across various sectors.
From healthcare to finance, retail to manufacturing, and beyond, AI is at the forefront of revolutionizing how businesses operate, enhancing efficiency, and crafting personalized user experiences
The importance of AI adoption cannot be overstated. As we delve into this topic, we aim to shed light on the myriad ways AI technologies are being integrated into different industries, not just to streamline operations but to solve complex problems and create opportunities that were unimaginable just a few decades ago.
Our journey through this landscape will highlight significant success stories and insightful case studies that demonstrate the profound impact of AI.
Healthcare Industry
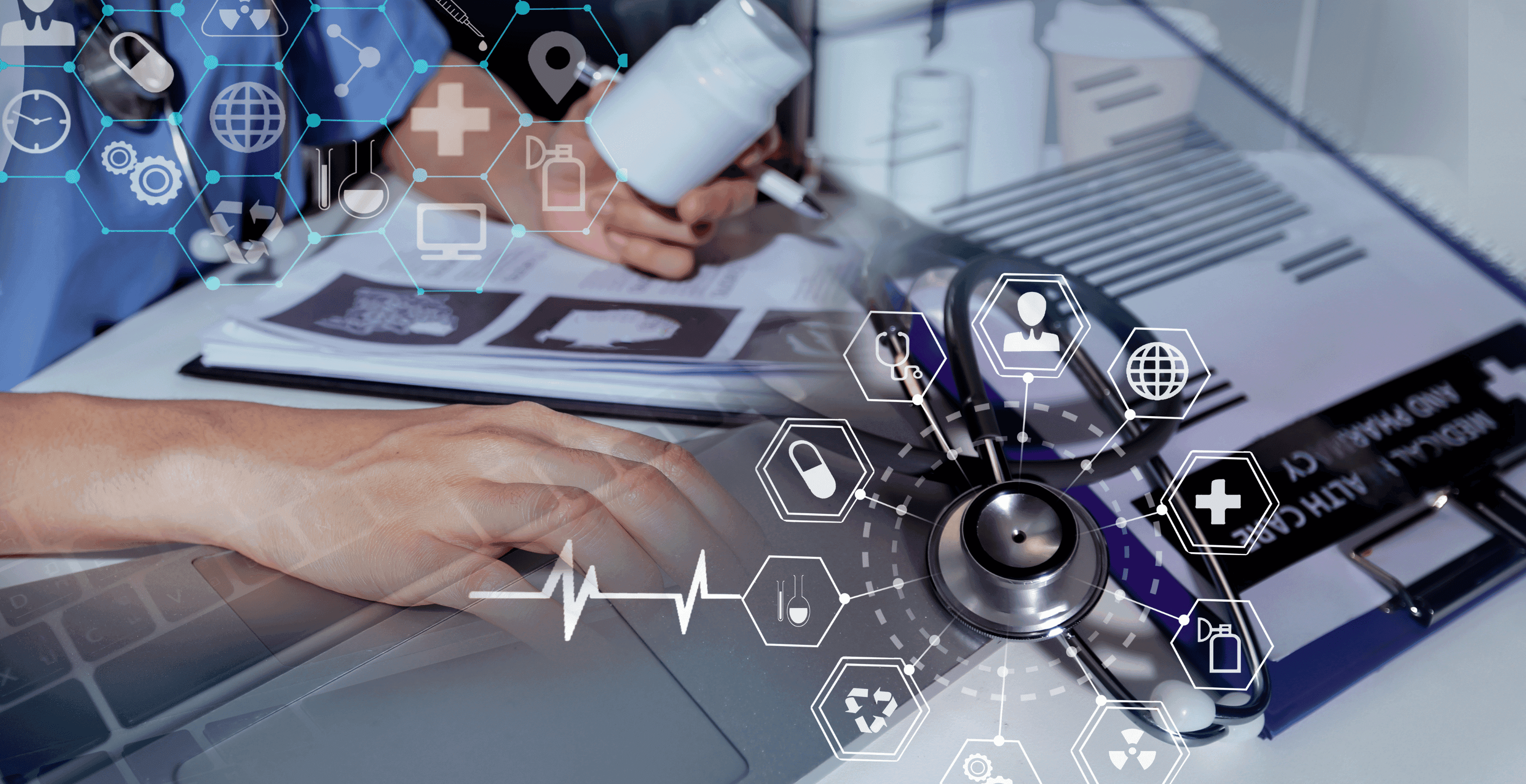
AI-Assisted Drug Discovery and Development
One notable example of AI's impact on drug discovery is the work of Baidu Research, which developed deep learning algorithms that outperform humans in identifying breast cancer metastasis.
These algorithms demonstrate AI's ability to analyze complex datasets, including genomic information, patient records, and drug compound libraries, to uncover insights that may not be immediately apparent to human researchers.
AI has significantly improved the efficiency of drug discovery at companies like Baidu Research in several key ways:
Accelerating lead compound discovery: AI-powered virtual screening tools can rapidly evaluate millions of chemical compounds to identify those with the highest potential for therapeutic activity, speeding up the lead compound discovery process.
Optimizing mRNA vaccine design: Baidu Research developed an AI algorithm called LinearDesign that can rapidly design highly stable COVID-19 mRNA vaccine sequences, achieving a 128-fold increase in antibody response compared to previous methods. This breakthrough can be applied to a wider range of therapeutic proteins.
Predicting drug properties: Baidu Research has developed novel machine learning-based computational biology algorithms for predicting drug properties, binding affinity, and designing new drug candidates, aiming to provide an AI-enhanced tool for facilitating drug discovery.
Leveraging knowledge graphs: Baidu's AI technologies, such as knowledge graphs, are being used to improve drug target discovery and validation, as well as small-molecule design. Knowledge graphs help connect disparate data sources to uncover new insights.
Optimizing workflows: By integrating AI into various stages of the drug discovery pipeline, including target identification, lead optimization, and preclinical testing, Baidu Research is able to streamline workflows and make the overall process more efficient.
These AI-driven advancements have the potential to significantly reduce the time and cost associated with drug development, which is typically a lengthy and expensive process. Baidu Research's work demonstrates the transformative impact AI can have on accelerating drug discovery and development.
Despite the promising results, it's important to note that AI is not a panacea for drug discovery challenges. Careful validation and integration with human expertise are crucial to ensure the reliability and safety of AI-generated insights.
Ongoing research and collaboration between AI experts and pharmaceutical researchers will be key to fully realizing the potential of AI in accelerating drug discovery and development.
AI for Fraud Detection and Risk Management
One notable success story in AI for fraud detection and risk management is the case of JPMorgan Chase. The bank implemented AI algorithms to enhance fraud detection capabilities, resulting in a significant reduction in false positives and improved detection accuracy.
By leveraging machine learning models, JPMorgan Chase was able to analyze vast amounts of transaction data in real-time, identifying suspicious patterns and anomalies that could indicate fraudulent activities.
JPMorgan Chase’s Use of AI:
Data Analysis: JPMorgan Chase's AI system processes large volumes of transaction data, looking for patterns indicative of fraudulent behavior.
Machine Learning Models: The bank uses sophisticated machine learning algorithms to detect anomalies and predict potential fraud.
Real-time Monitoring: The AI system operates in real-time, allowing for immediate detection and response to suspicious activities.
Continuous Learning: The system continuously learns from new data and feedback, improving its accuracy and staying ahead of evolving fraud tactics.
Reduced False Positives: By leveraging AI, JPMorgan Chase has significantly reduced false positives, minimizing disruptions to legitimate transactions.
Enhanced Detection Accuracy: The AI algorithms have improved the bank's ability to detect fraudulent activities early, preventing financial losses and protecting customers.
Impact:
Improved Fraud Prevention: JPMorgan Chase's AI implementation has strengthened its fraud prevention capabilities, safeguarding customer assets and financial transactions.
Cost Savings: By reducing false positives and improving detection accuracy, the bank has saved resources previously spent on investigating false alarms.
Enhanced Customer Trust: The proactive fraud detection enabled by AI has enhanced customer trust and confidence in the bank's security measures.
Industry Leadership: JPMorgan Chase's success in AI-driven fraud detection has positioned it as a leader in leveraging technology for risk management in the banking sector.
The success of JPMorgan Chase's AI implementation showcases the power of AI in combating financial fraud, enhancing risk management practices, and protecting both the institution and its customers from fraudulent activities.
Retail and E-commerce
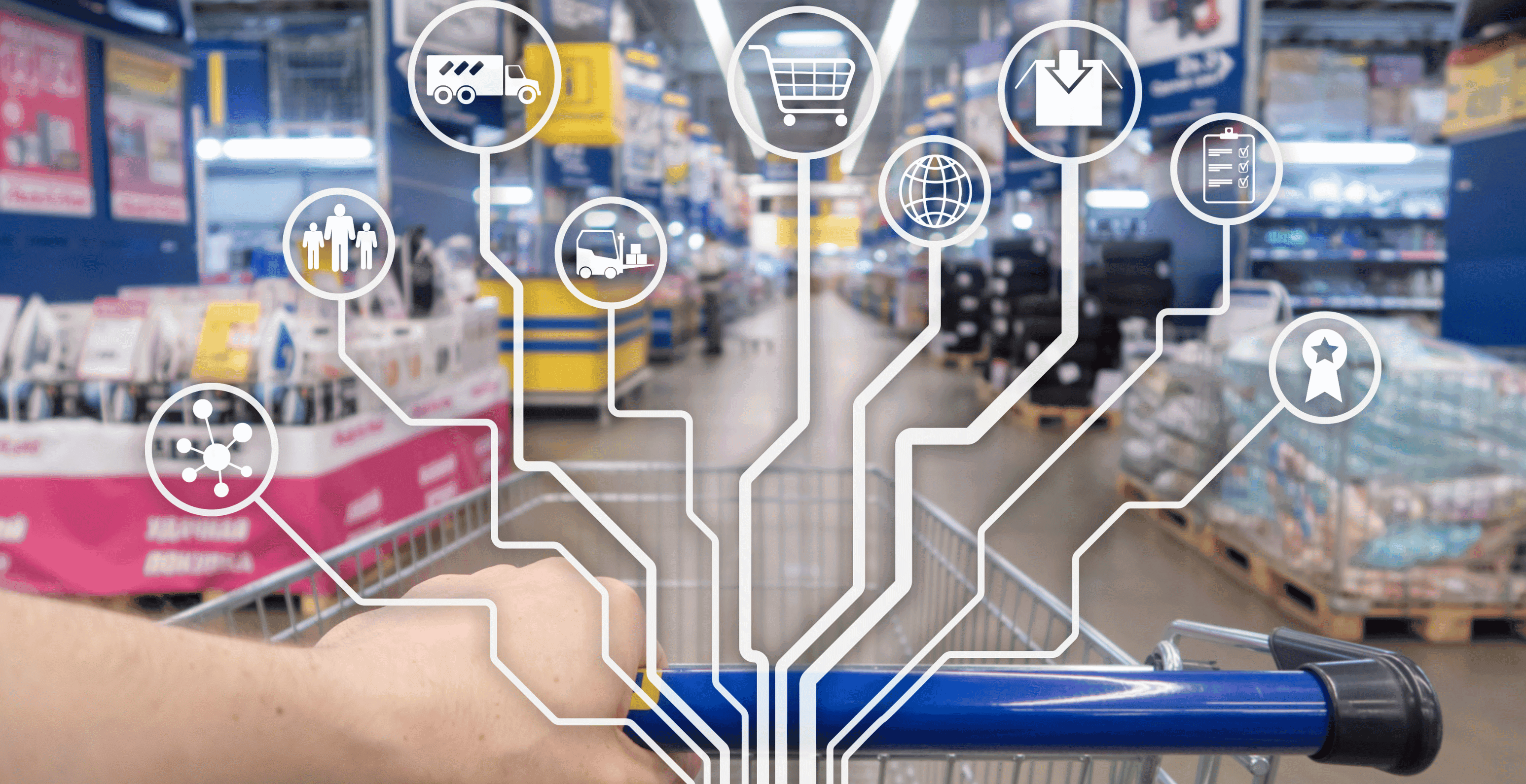
AI for Product Recommendation and Personalization
Amazon, the e-commerce giant, has been at the forefront of leveraging AI for product recommendation and personalization.
By analyzing customer browsing history, purchase patterns, and preferences, Amazon's AI algorithms generate highly accurate and personalized product recommendations123. This has significantly boosted customer engagement, increased sales, and improved the overall shopping experience.
How Amazon's AI-Powered Recommendations Work?
Amazon's recommendation system collects and analyzes vast amounts of customer data, including:
Browsing history: The products a customer has viewed or searched for
Purchase history: The products a customer has bought in the past
Ratings and reviews: The customer's ratings and reviews of products
Demographic data: The customer's age, gender, location, and other relevant information
Using this data, Amazon's AI algorithms identify patterns and correlations to predict what a customer might be interested in.
The system also takes into account the behavior of similar customers to provide even more personalized recommendations1.
Benefits of AI-Powered Recommendations
By implementing AI-driven product recommendations, Amazon has achieved several key benefits:
Increased sales: Personalized recommendations have been shown to drive a significant increase in sales, as customers are more likely to purchase products that are tailored to their preferences2.
Enhanced customer engagement: Relevant and engaging product recommendations keep customers on the site for longer, leading to increased browsing and exploration of Amazon's vast product catalog1.
Improved customer satisfaction: Customers appreciate the convenience and relevance of personalized recommendations, leading to higher satisfaction levels and increased loyalty to the Amazon brand3.
Competitive advantage: Amazon's advanced AI capabilities in product recommendation and personalization have given it a significant edge over competitors in the e-commerce space2.
Future Developments
As AI technology continues to advance, Amazon is likely to further refine and enhance its product recommendation system. Potential future developments may include:
Incorporating real-time data: Using AI to analyze customer behavior and preferences in real-time, allowing for even more dynamic and responsive recommendations4.
Expanding to new channels: Applying AI-powered recommendations to other channels, such as mobile apps and voice assistants, to provide a seamless and consistent experience across all touchpoints5.
Leveraging new data sources: Integrating additional data sources, such as social media activity and external market trends, to further improve the accuracy and relevance of recommendations3.
By continuously investing in and improving its AI capabilities, Amazon is well-positioned to maintain its leadership in the e-commerce industry and deliver exceptional customer experiences through personalized product recommendations.
Manufacturing and Industrial Automation
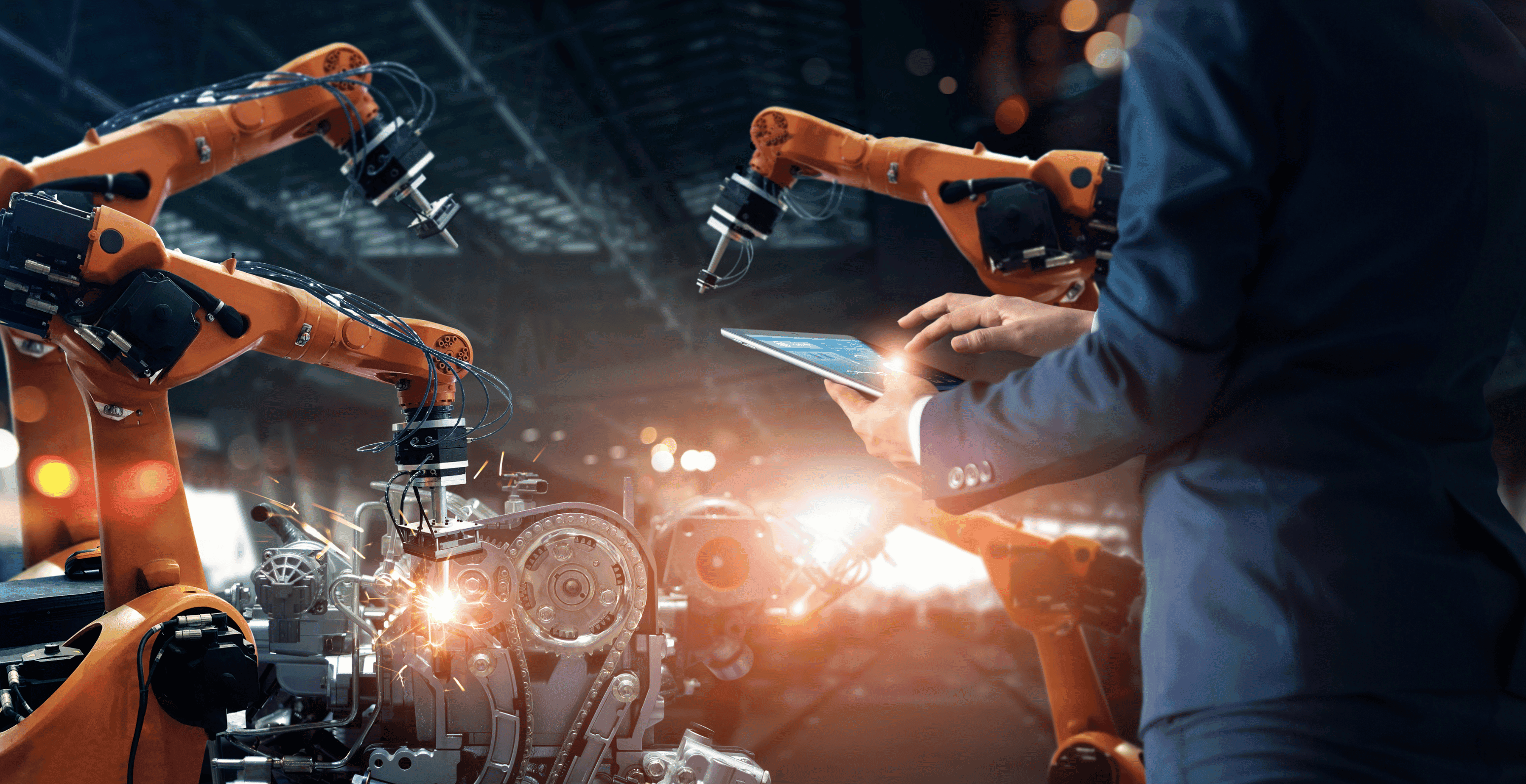
AI for Predictive Maintenance and Quality Control
A notable example of AI for predictive maintenance and quality control is the implementation by Siemens. The company leverages AI and machine learning to maximize productivity in the industrial and manufacturing sectors.
By exploring the capabilities of AI and machine learning, Siemens has developed technologies that reduce machinery costs, improve consistency with automation, and deliver instant data for quickly adjusting workflows 2.
Siemens' Approach to AI in Predictive Maintenance and Quality Control
Siemens utilizes AI and machine learning in the following ways to enhance predictive maintenance and quality control:
Predictive Maintenance: By analyzing real-time data from sensors embedded in machinery, Siemens' AI algorithms can predict potential equipment failures before they occur. This proactive approach allows for timely maintenance, reducing downtime and minimizing repair costs.
Quality Control: AI-driven quality control systems analyze production data to predict potential quality issues before they occur. By identifying anomalies and deviations in the manufacturing process, Siemens can take corrective actions to maintain product quality standards.
Automation and Consistency: AI technologies enable automation of manual processes, ensuring consistency in manufacturing operations. By leveraging machine learning algorithms, Siemens can optimize workflows, reduce errors, and improve operational efficiency.
Data-Driven Decision-Making: Siemens' AI systems provide instant data insights, allowing for quick adjustments in production processes. Real-time analytics enable agile decision-making, enhancing overall productivity and responsiveness to changing demands.
Impact and Benefits
The implementation of AI for predictive maintenance and quality control at Siemens has resulted in several key benefits:
Maximized Productivity: By leveraging AI technologies, Siemens has optimized machinery performance, reduced downtime, and maximized productivity in manufacturing operations.
Cost Reduction: Predictive maintenance has helped Siemens reduce machinery costs by proactively addressing maintenance needs and avoiding costly breakdowns.
Improved Quality: AI-driven quality control systems have enhanced product quality by identifying and addressing potential issues early in the manufacturing process.
Enhanced Automation: Automation through AI has improved consistency, reduced errors, and streamlined workflows, leading to increased operational efficiency.
Agile Decision-Making: Real-time data insights provided by AI systems enable Siemens to make quick and informed decisions, ensuring responsiveness to market demands and operational changes.
Siemens' successful integration of AI for predictive maintenance and quality control showcases the transformative potential of AI in optimizing industrial processes, reducing costs, and enhancing overall operational efficiency in the manufacturing sector.
Marketing and Advertising
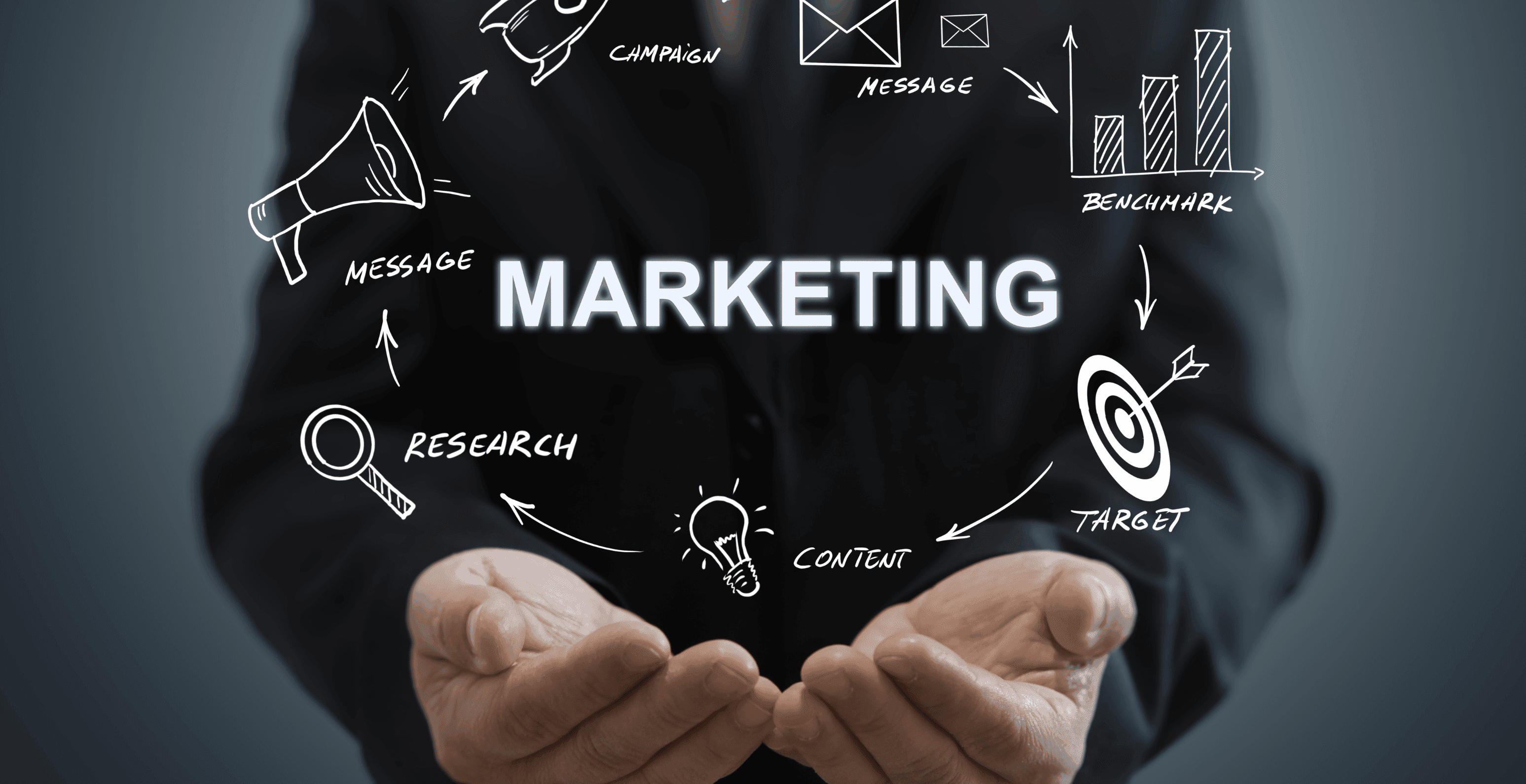
AI for Targeted Advertising and Campaign Optimization
Vanguard, one of the world's largest investment firms, utilized AI for targeted advertising and campaign optimization by partnering with the AI language platform Persado to conduct highly personalized advertising on LinkedIn, the only platform where they could run ads due to regulations.
Leveraging AI technology, Vanguard hyper-personalized its ads and tested them at scale to determine the most effective approaches that resonated with consumers. This strategic use of AI resulted in a significant 15% increase in conversion rates for Vanguard's advertising campaigns4.
Vanguard's Approach to AI-Powered Advertising:
Hyper-Personalization: Vanguard employed AI to create highly personalized ads tailored to individual consumers based on their preferences and behaviors.
Scale Testing: AI allowed Vanguard to test multiple ad variations at scale to identify the most effective messaging and creative elements.
Regulatory Compliance: Due to regulatory restrictions, Vanguard focused its AI-powered advertising efforts on LinkedIn, optimizing its campaigns for this specific platform.
Conversion Rate Optimization: By leveraging AI insights, Vanguard was able to optimize its advertising campaigns to drive a 15% increase in conversion rates.
Impact and Benefits:
Increased Conversions: The use of AI for targeted advertising led to a substantial 15% increase in conversion rates for Vanguard's campaigns.
Personalized Engagement: AI-driven hyper-personalization enhanced customer engagement by delivering tailored messages that resonated with individual preferences.
Efficiency and Effectiveness: AI technology enabled Vanguard to optimize its advertising efforts, ensuring that resources were allocated to the most impactful campaigns.
Regulatory Compliance: By focusing on LinkedIn for AI-powered advertising, Vanguard navigated regulatory constraints while still achieving significant performance improvements.
Future Applications:
Further Personalization: Vanguard can continue to refine its AI-powered advertising strategies to deliver even more personalized and targeted campaigns.
Multi-Platform Optimization: Expanding AI-driven advertising efforts to other platforms while maintaining regulatory compliance to reach a broader audience.
Continuous Testing and Optimization: Vanguard can leverage AI for ongoing testing and optimization of ad campaigns to adapt to changing consumer behaviors and preferences.
Vanguard's success in leveraging AI for targeted advertising and campaign optimization exemplifies the transformative impact of AI in enhancing marketing strategies, driving conversions, and delivering personalized experiences to consumers.
Education and Learning
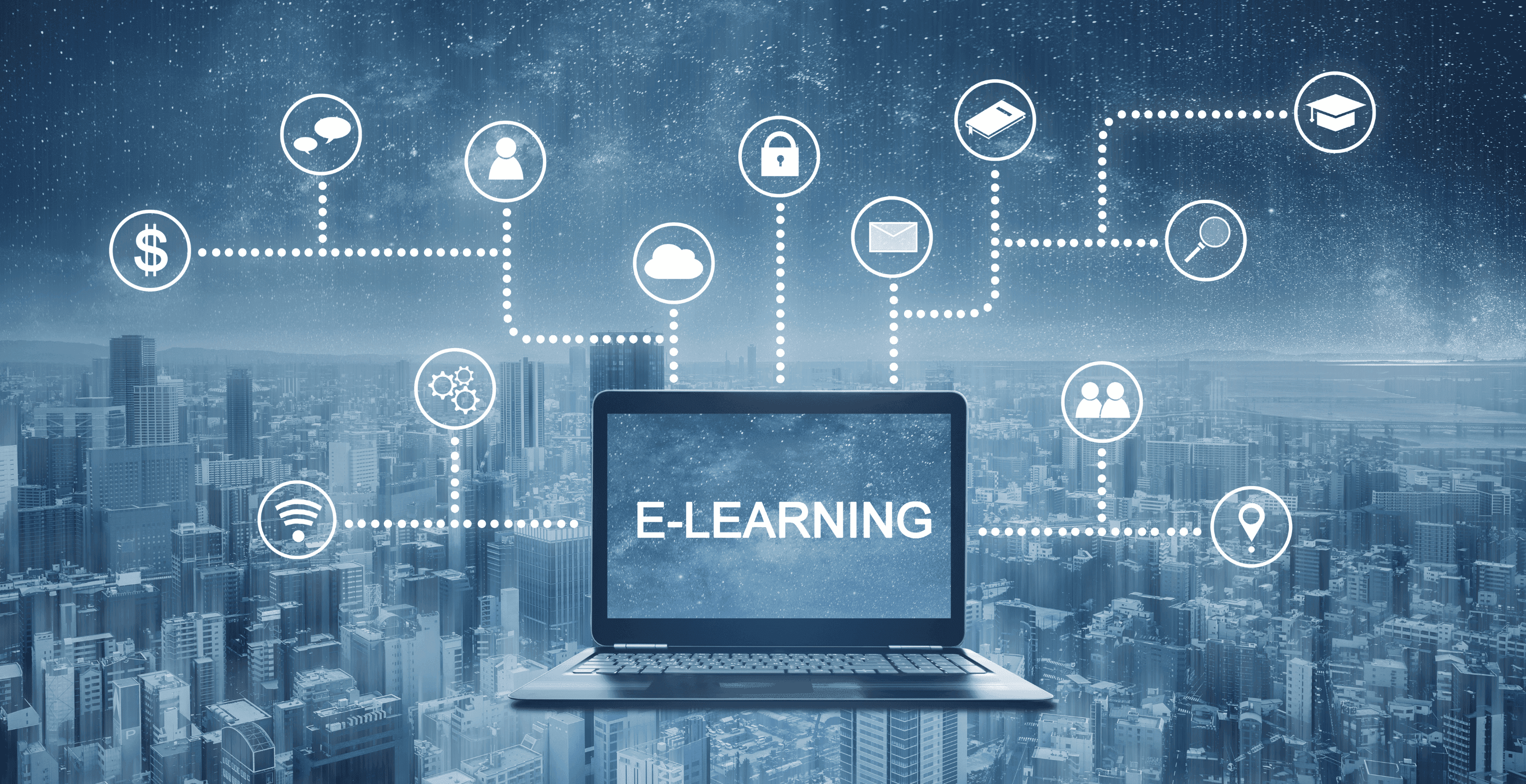
AI-Powered Virtual Tutors and Teaching Assistants
A notable example of AI-powered virtual tutors and teaching assistants is the work of Riiid Labs. This subsidiary of Riiid develops AI-based learning and assessment technologies that can be integrated into existing applications or used as standalone platforms13.
By leveraging machine learning capabilities, Riiid Labs' technology assists teachers in tracking student performance, automating assignment scoring, and personalizing curriculum needs for each student.
Riiid Labs' AI-Powered Virtual Tutoring System
Riiid Labs' AI-powered virtual tutoring system utilizes advanced machine learning algorithms to provide personalized learning experiences for students. The system works by:
Collecting and analyzing student data: The platform gathers information on each student's performance, learning patterns, and progress to create a personalized profile.
Adapting learning content: Based on the student's profile, the AI system selects and presents the most appropriate learning materials, exercises, and assessments tailored to their individual needs and learning style.
Providing real-time feedback: The virtual tutor offers immediate feedback and guidance to students, helping them identify areas of strength and weakness and providing targeted support.
Automating grading and scoring: The AI system can automatically grade assignments and assessments, freeing up teachers' time and providing consistent and timely feedback to students.
Predicting future performance: By analyzing student data over time, the AI can predict each student's future academic performance and identify potential areas of concern, allowing teachers to intervene early and provide additional support.
Impact and Benefits
The implementation of Riiid Labs' AI-powered virtual tutoring system has resulted in several key benefits for both students and teachers:
Personalized learning experiences: Students receive tailored instruction and support based on their individual needs, leading to improved engagement and learning outcomes.
Increased efficiency: Teachers can focus on providing high-quality instruction and mentorship while the AI system handles routine tasks such as grading and performance tracking.
Early intervention: By predicting potential academic challenges, teachers can intervene early and provide targeted support to students, preventing them from falling behind.
Scalability: The AI-powered system can provide personalized learning experiences to a large number of students simultaneously, making it a scalable solution for educational institutions.
Continuous improvement: The AI system learns and adapts over time, continuously improving its ability to provide personalized learning experiences and predict student performance.
Riiid Labs' success in developing AI-powered virtual tutoring systems demonstrates the transformative potential of AI in enhancing teaching and learning experiences, leading to improved student outcomes and increased efficiency for educators.
Conclusion
We've journeyed across a landscape where AI's influence is profound and pervasive. From diagnosing diseases with uncanny accuracy to managing global supply chains, and from personalizing learning experiences to revolutionizing customer interactions, AI’s capabilities are vast and varied.
These success stories and case studies not only illuminate AI's potential to enhance business outcomes but also highlight its role in driving competitive advantages in a rapidly evolving world.
As businesses and sectors continue to harness AI's power, the pathway to innovation seems increasingly intertwined with intelligent technology.
The future, rich with advancements in machine learning, natural language processing, and more, promises even more exciting prospects. For any industry looking to remain competitive and innovative, embracing AI is not just an option but a necessity.
Further Reading and Resources
For those intrigued by AI’s expansive role across various sectors and looking to dive deeper into its capabilities and real-world applications, numerous resources are available.
Books like "AI Superpowers" by Kai-Fu Lee offer insights into how AI is shaping global industries. Journals like "Artificial Intelligence" and online platforms such as Google Scholar provide access to the latest research and case studies.
Microsoft AI Customer Stories:
Microsoft AI showcases various customer stories where AI technologies are being applied across different sectors.
Examples include Zammo's conversational AI platform, CarMax's customer review summaries, Broward College's student support strategies, and IPLAND's retail display optimization using image recognition technology.
Amazon AWS Case Studies:
Amazon AWS presents customer success stories, including BMW Group's utilization of AWS technology to power over 1,000 microservices processing billions of requests daily with high reliability.
The platform offers a range of case studies demonstrating how organizations accelerate innovation, increase agility, and lower costs through AWS cloud services.
Whatfix Case Studies:
Whatfix showcases case studies on how organizations drive technology adoption for customers and employees through contextual in-app guidance and self-help support
These case studies cover a variety of industries and applications, providing insights into how AI adoption is enhancing user experiences and driving technology utilization.
By exploring these sources, you can gain a comprehensive understanding of how AI adoption is shaping industries and driving innovation across various sectors.
AI, a combination of machine learning, data analytics, and automation, is not just a futuristic concept but a present-day powerhouse driving innovation across various sectors.
From healthcare to finance, retail to manufacturing, and beyond, AI is at the forefront of revolutionizing how businesses operate, enhancing efficiency, and crafting personalized user experiences
The importance of AI adoption cannot be overstated. As we delve into this topic, we aim to shed light on the myriad ways AI technologies are being integrated into different industries, not just to streamline operations but to solve complex problems and create opportunities that were unimaginable just a few decades ago.
Our journey through this landscape will highlight significant success stories and insightful case studies that demonstrate the profound impact of AI.
Healthcare Industry
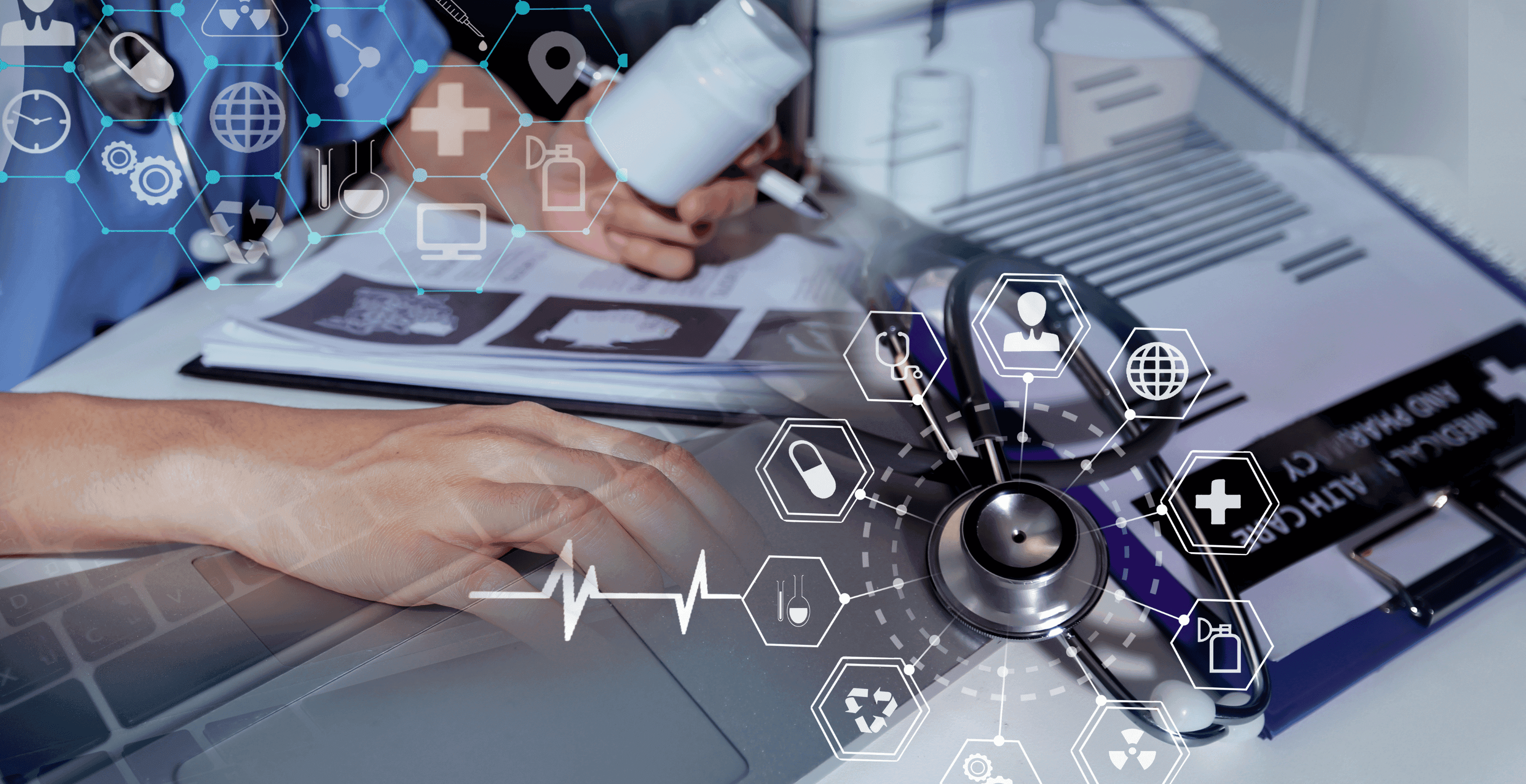
AI-Assisted Drug Discovery and Development
One notable example of AI's impact on drug discovery is the work of Baidu Research, which developed deep learning algorithms that outperform humans in identifying breast cancer metastasis.
These algorithms demonstrate AI's ability to analyze complex datasets, including genomic information, patient records, and drug compound libraries, to uncover insights that may not be immediately apparent to human researchers.
AI has significantly improved the efficiency of drug discovery at companies like Baidu Research in several key ways:
Accelerating lead compound discovery: AI-powered virtual screening tools can rapidly evaluate millions of chemical compounds to identify those with the highest potential for therapeutic activity, speeding up the lead compound discovery process.
Optimizing mRNA vaccine design: Baidu Research developed an AI algorithm called LinearDesign that can rapidly design highly stable COVID-19 mRNA vaccine sequences, achieving a 128-fold increase in antibody response compared to previous methods. This breakthrough can be applied to a wider range of therapeutic proteins.
Predicting drug properties: Baidu Research has developed novel machine learning-based computational biology algorithms for predicting drug properties, binding affinity, and designing new drug candidates, aiming to provide an AI-enhanced tool for facilitating drug discovery.
Leveraging knowledge graphs: Baidu's AI technologies, such as knowledge graphs, are being used to improve drug target discovery and validation, as well as small-molecule design. Knowledge graphs help connect disparate data sources to uncover new insights.
Optimizing workflows: By integrating AI into various stages of the drug discovery pipeline, including target identification, lead optimization, and preclinical testing, Baidu Research is able to streamline workflows and make the overall process more efficient.
These AI-driven advancements have the potential to significantly reduce the time and cost associated with drug development, which is typically a lengthy and expensive process. Baidu Research's work demonstrates the transformative impact AI can have on accelerating drug discovery and development.
Despite the promising results, it's important to note that AI is not a panacea for drug discovery challenges. Careful validation and integration with human expertise are crucial to ensure the reliability and safety of AI-generated insights.
Ongoing research and collaboration between AI experts and pharmaceutical researchers will be key to fully realizing the potential of AI in accelerating drug discovery and development.
AI for Fraud Detection and Risk Management
One notable success story in AI for fraud detection and risk management is the case of JPMorgan Chase. The bank implemented AI algorithms to enhance fraud detection capabilities, resulting in a significant reduction in false positives and improved detection accuracy.
By leveraging machine learning models, JPMorgan Chase was able to analyze vast amounts of transaction data in real-time, identifying suspicious patterns and anomalies that could indicate fraudulent activities.
JPMorgan Chase’s Use of AI:
Data Analysis: JPMorgan Chase's AI system processes large volumes of transaction data, looking for patterns indicative of fraudulent behavior.
Machine Learning Models: The bank uses sophisticated machine learning algorithms to detect anomalies and predict potential fraud.
Real-time Monitoring: The AI system operates in real-time, allowing for immediate detection and response to suspicious activities.
Continuous Learning: The system continuously learns from new data and feedback, improving its accuracy and staying ahead of evolving fraud tactics.
Reduced False Positives: By leveraging AI, JPMorgan Chase has significantly reduced false positives, minimizing disruptions to legitimate transactions.
Enhanced Detection Accuracy: The AI algorithms have improved the bank's ability to detect fraudulent activities early, preventing financial losses and protecting customers.
Impact:
Improved Fraud Prevention: JPMorgan Chase's AI implementation has strengthened its fraud prevention capabilities, safeguarding customer assets and financial transactions.
Cost Savings: By reducing false positives and improving detection accuracy, the bank has saved resources previously spent on investigating false alarms.
Enhanced Customer Trust: The proactive fraud detection enabled by AI has enhanced customer trust and confidence in the bank's security measures.
Industry Leadership: JPMorgan Chase's success in AI-driven fraud detection has positioned it as a leader in leveraging technology for risk management in the banking sector.
The success of JPMorgan Chase's AI implementation showcases the power of AI in combating financial fraud, enhancing risk management practices, and protecting both the institution and its customers from fraudulent activities.
Retail and E-commerce
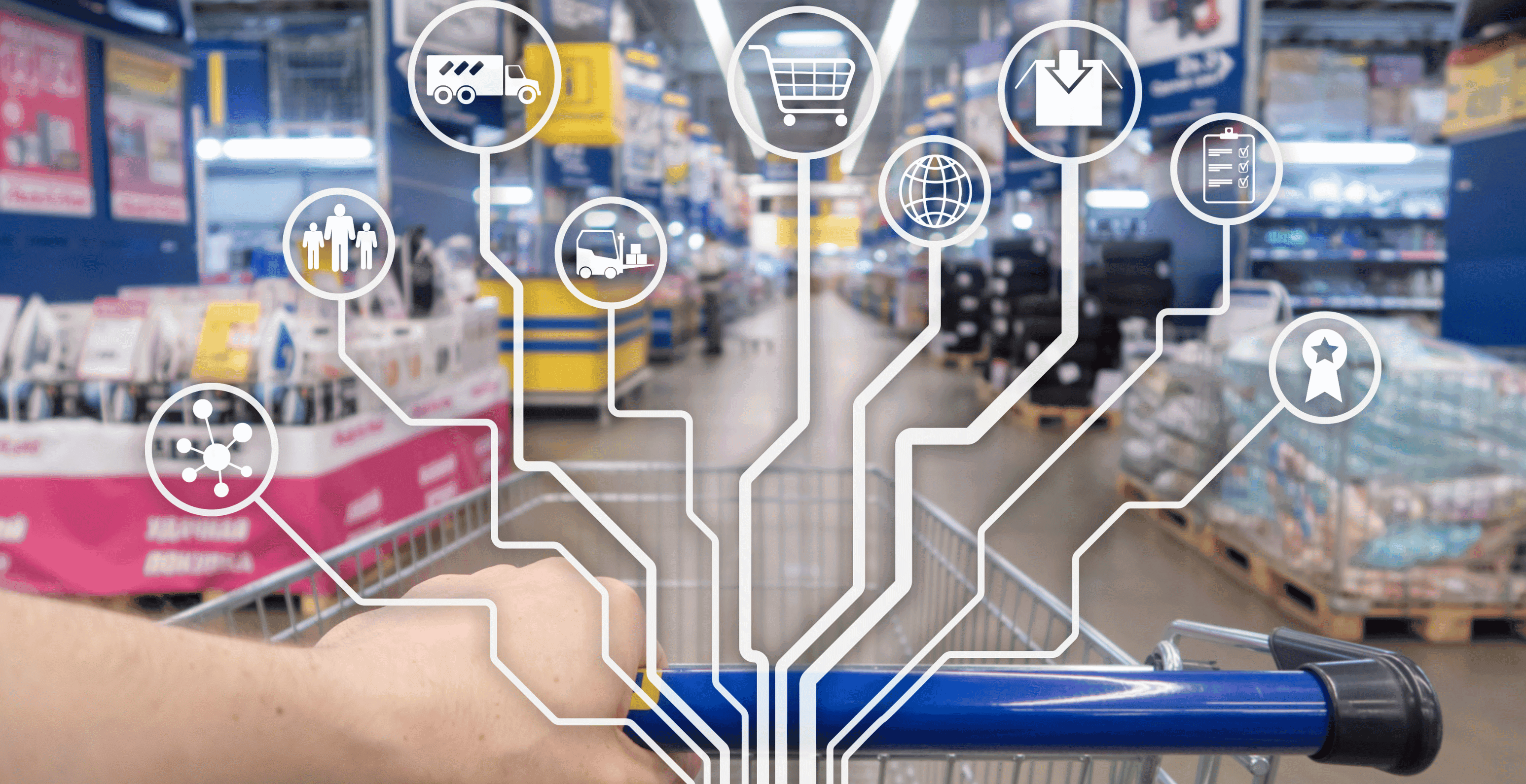
AI for Product Recommendation and Personalization
Amazon, the e-commerce giant, has been at the forefront of leveraging AI for product recommendation and personalization.
By analyzing customer browsing history, purchase patterns, and preferences, Amazon's AI algorithms generate highly accurate and personalized product recommendations123. This has significantly boosted customer engagement, increased sales, and improved the overall shopping experience.
How Amazon's AI-Powered Recommendations Work?
Amazon's recommendation system collects and analyzes vast amounts of customer data, including:
Browsing history: The products a customer has viewed or searched for
Purchase history: The products a customer has bought in the past
Ratings and reviews: The customer's ratings and reviews of products
Demographic data: The customer's age, gender, location, and other relevant information
Using this data, Amazon's AI algorithms identify patterns and correlations to predict what a customer might be interested in.
The system also takes into account the behavior of similar customers to provide even more personalized recommendations1.
Benefits of AI-Powered Recommendations
By implementing AI-driven product recommendations, Amazon has achieved several key benefits:
Increased sales: Personalized recommendations have been shown to drive a significant increase in sales, as customers are more likely to purchase products that are tailored to their preferences2.
Enhanced customer engagement: Relevant and engaging product recommendations keep customers on the site for longer, leading to increased browsing and exploration of Amazon's vast product catalog1.
Improved customer satisfaction: Customers appreciate the convenience and relevance of personalized recommendations, leading to higher satisfaction levels and increased loyalty to the Amazon brand3.
Competitive advantage: Amazon's advanced AI capabilities in product recommendation and personalization have given it a significant edge over competitors in the e-commerce space2.
Future Developments
As AI technology continues to advance, Amazon is likely to further refine and enhance its product recommendation system. Potential future developments may include:
Incorporating real-time data: Using AI to analyze customer behavior and preferences in real-time, allowing for even more dynamic and responsive recommendations4.
Expanding to new channels: Applying AI-powered recommendations to other channels, such as mobile apps and voice assistants, to provide a seamless and consistent experience across all touchpoints5.
Leveraging new data sources: Integrating additional data sources, such as social media activity and external market trends, to further improve the accuracy and relevance of recommendations3.
By continuously investing in and improving its AI capabilities, Amazon is well-positioned to maintain its leadership in the e-commerce industry and deliver exceptional customer experiences through personalized product recommendations.
Manufacturing and Industrial Automation
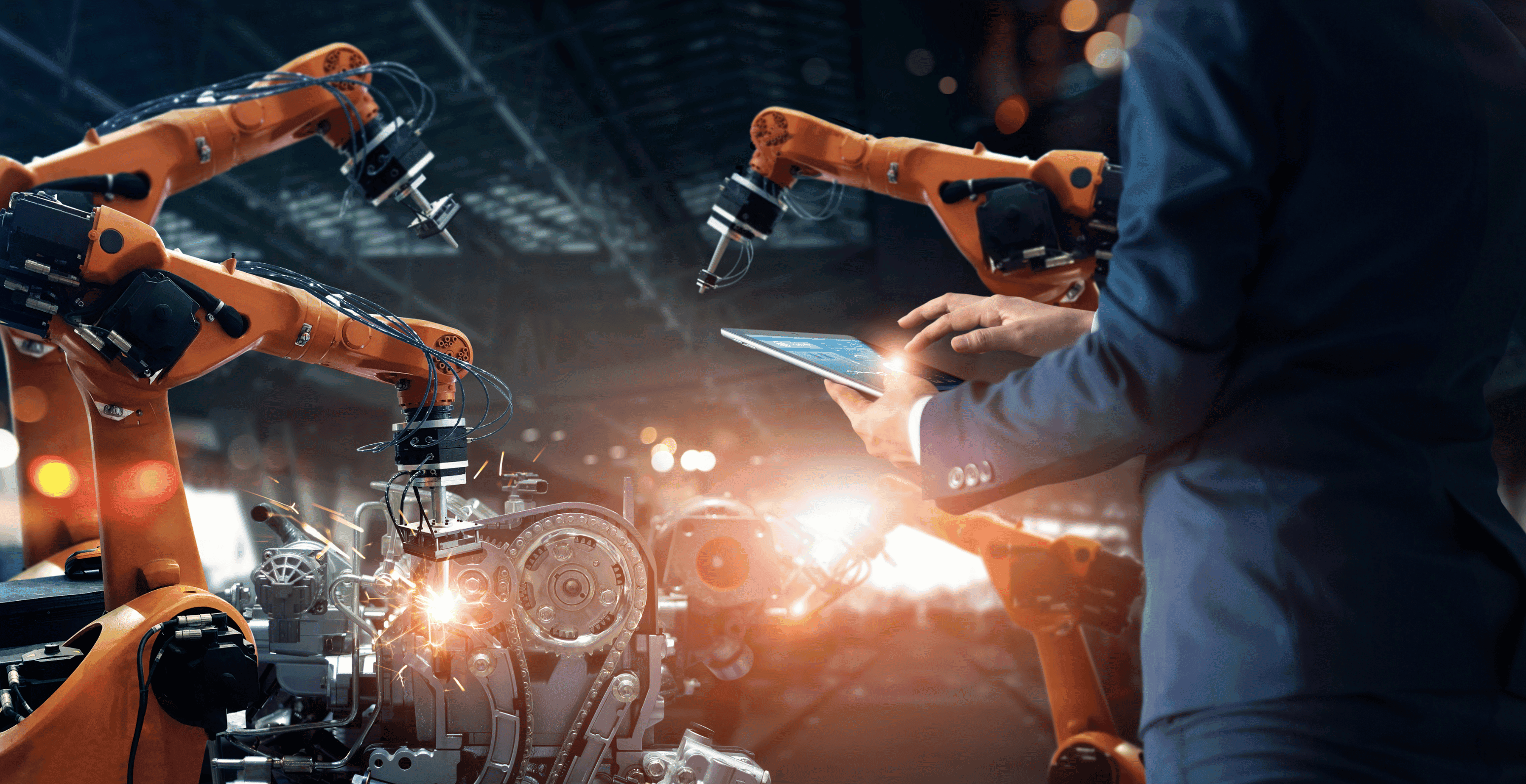
AI for Predictive Maintenance and Quality Control
A notable example of AI for predictive maintenance and quality control is the implementation by Siemens. The company leverages AI and machine learning to maximize productivity in the industrial and manufacturing sectors.
By exploring the capabilities of AI and machine learning, Siemens has developed technologies that reduce machinery costs, improve consistency with automation, and deliver instant data for quickly adjusting workflows 2.
Siemens' Approach to AI in Predictive Maintenance and Quality Control
Siemens utilizes AI and machine learning in the following ways to enhance predictive maintenance and quality control:
Predictive Maintenance: By analyzing real-time data from sensors embedded in machinery, Siemens' AI algorithms can predict potential equipment failures before they occur. This proactive approach allows for timely maintenance, reducing downtime and minimizing repair costs.
Quality Control: AI-driven quality control systems analyze production data to predict potential quality issues before they occur. By identifying anomalies and deviations in the manufacturing process, Siemens can take corrective actions to maintain product quality standards.
Automation and Consistency: AI technologies enable automation of manual processes, ensuring consistency in manufacturing operations. By leveraging machine learning algorithms, Siemens can optimize workflows, reduce errors, and improve operational efficiency.
Data-Driven Decision-Making: Siemens' AI systems provide instant data insights, allowing for quick adjustments in production processes. Real-time analytics enable agile decision-making, enhancing overall productivity and responsiveness to changing demands.
Impact and Benefits
The implementation of AI for predictive maintenance and quality control at Siemens has resulted in several key benefits:
Maximized Productivity: By leveraging AI technologies, Siemens has optimized machinery performance, reduced downtime, and maximized productivity in manufacturing operations.
Cost Reduction: Predictive maintenance has helped Siemens reduce machinery costs by proactively addressing maintenance needs and avoiding costly breakdowns.
Improved Quality: AI-driven quality control systems have enhanced product quality by identifying and addressing potential issues early in the manufacturing process.
Enhanced Automation: Automation through AI has improved consistency, reduced errors, and streamlined workflows, leading to increased operational efficiency.
Agile Decision-Making: Real-time data insights provided by AI systems enable Siemens to make quick and informed decisions, ensuring responsiveness to market demands and operational changes.
Siemens' successful integration of AI for predictive maintenance and quality control showcases the transformative potential of AI in optimizing industrial processes, reducing costs, and enhancing overall operational efficiency in the manufacturing sector.
Marketing and Advertising
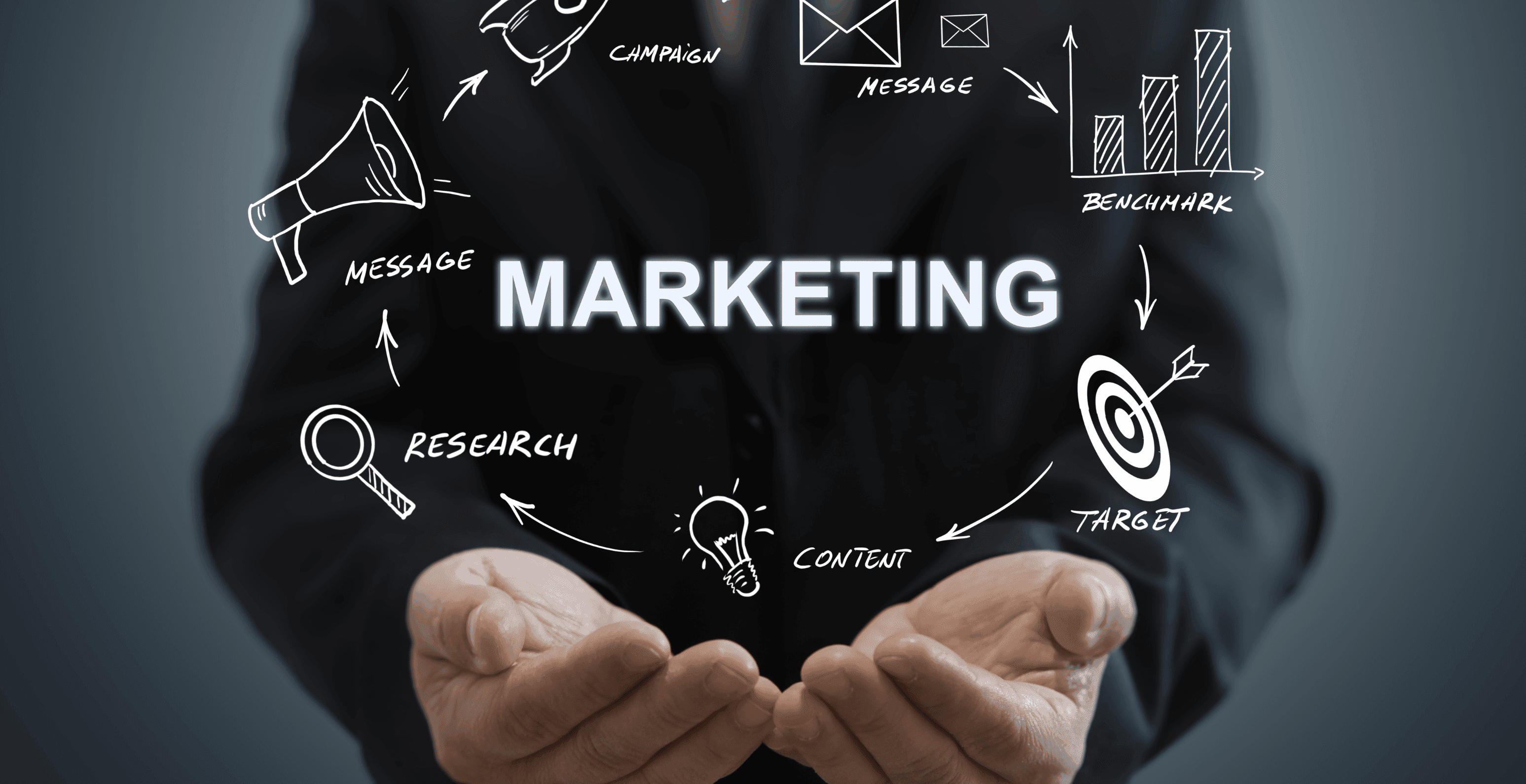
AI for Targeted Advertising and Campaign Optimization
Vanguard, one of the world's largest investment firms, utilized AI for targeted advertising and campaign optimization by partnering with the AI language platform Persado to conduct highly personalized advertising on LinkedIn, the only platform where they could run ads due to regulations.
Leveraging AI technology, Vanguard hyper-personalized its ads and tested them at scale to determine the most effective approaches that resonated with consumers. This strategic use of AI resulted in a significant 15% increase in conversion rates for Vanguard's advertising campaigns4.
Vanguard's Approach to AI-Powered Advertising:
Hyper-Personalization: Vanguard employed AI to create highly personalized ads tailored to individual consumers based on their preferences and behaviors.
Scale Testing: AI allowed Vanguard to test multiple ad variations at scale to identify the most effective messaging and creative elements.
Regulatory Compliance: Due to regulatory restrictions, Vanguard focused its AI-powered advertising efforts on LinkedIn, optimizing its campaigns for this specific platform.
Conversion Rate Optimization: By leveraging AI insights, Vanguard was able to optimize its advertising campaigns to drive a 15% increase in conversion rates.
Impact and Benefits:
Increased Conversions: The use of AI for targeted advertising led to a substantial 15% increase in conversion rates for Vanguard's campaigns.
Personalized Engagement: AI-driven hyper-personalization enhanced customer engagement by delivering tailored messages that resonated with individual preferences.
Efficiency and Effectiveness: AI technology enabled Vanguard to optimize its advertising efforts, ensuring that resources were allocated to the most impactful campaigns.
Regulatory Compliance: By focusing on LinkedIn for AI-powered advertising, Vanguard navigated regulatory constraints while still achieving significant performance improvements.
Future Applications:
Further Personalization: Vanguard can continue to refine its AI-powered advertising strategies to deliver even more personalized and targeted campaigns.
Multi-Platform Optimization: Expanding AI-driven advertising efforts to other platforms while maintaining regulatory compliance to reach a broader audience.
Continuous Testing and Optimization: Vanguard can leverage AI for ongoing testing and optimization of ad campaigns to adapt to changing consumer behaviors and preferences.
Vanguard's success in leveraging AI for targeted advertising and campaign optimization exemplifies the transformative impact of AI in enhancing marketing strategies, driving conversions, and delivering personalized experiences to consumers.
Education and Learning
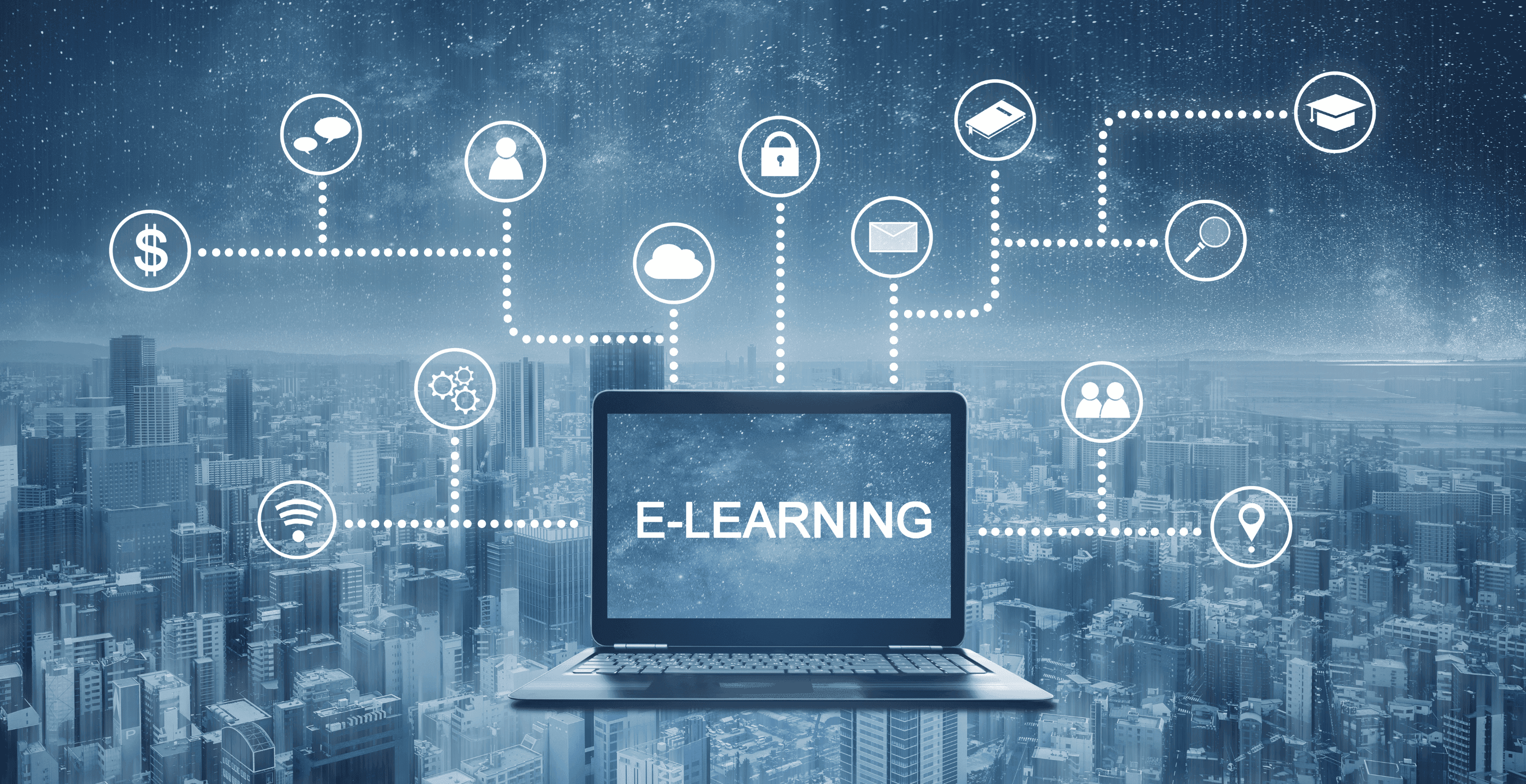
AI-Powered Virtual Tutors and Teaching Assistants
A notable example of AI-powered virtual tutors and teaching assistants is the work of Riiid Labs. This subsidiary of Riiid develops AI-based learning and assessment technologies that can be integrated into existing applications or used as standalone platforms13.
By leveraging machine learning capabilities, Riiid Labs' technology assists teachers in tracking student performance, automating assignment scoring, and personalizing curriculum needs for each student.
Riiid Labs' AI-Powered Virtual Tutoring System
Riiid Labs' AI-powered virtual tutoring system utilizes advanced machine learning algorithms to provide personalized learning experiences for students. The system works by:
Collecting and analyzing student data: The platform gathers information on each student's performance, learning patterns, and progress to create a personalized profile.
Adapting learning content: Based on the student's profile, the AI system selects and presents the most appropriate learning materials, exercises, and assessments tailored to their individual needs and learning style.
Providing real-time feedback: The virtual tutor offers immediate feedback and guidance to students, helping them identify areas of strength and weakness and providing targeted support.
Automating grading and scoring: The AI system can automatically grade assignments and assessments, freeing up teachers' time and providing consistent and timely feedback to students.
Predicting future performance: By analyzing student data over time, the AI can predict each student's future academic performance and identify potential areas of concern, allowing teachers to intervene early and provide additional support.
Impact and Benefits
The implementation of Riiid Labs' AI-powered virtual tutoring system has resulted in several key benefits for both students and teachers:
Personalized learning experiences: Students receive tailored instruction and support based on their individual needs, leading to improved engagement and learning outcomes.
Increased efficiency: Teachers can focus on providing high-quality instruction and mentorship while the AI system handles routine tasks such as grading and performance tracking.
Early intervention: By predicting potential academic challenges, teachers can intervene early and provide targeted support to students, preventing them from falling behind.
Scalability: The AI-powered system can provide personalized learning experiences to a large number of students simultaneously, making it a scalable solution for educational institutions.
Continuous improvement: The AI system learns and adapts over time, continuously improving its ability to provide personalized learning experiences and predict student performance.
Riiid Labs' success in developing AI-powered virtual tutoring systems demonstrates the transformative potential of AI in enhancing teaching and learning experiences, leading to improved student outcomes and increased efficiency for educators.
Conclusion
We've journeyed across a landscape where AI's influence is profound and pervasive. From diagnosing diseases with uncanny accuracy to managing global supply chains, and from personalizing learning experiences to revolutionizing customer interactions, AI’s capabilities are vast and varied.
These success stories and case studies not only illuminate AI's potential to enhance business outcomes but also highlight its role in driving competitive advantages in a rapidly evolving world.
As businesses and sectors continue to harness AI's power, the pathway to innovation seems increasingly intertwined with intelligent technology.
The future, rich with advancements in machine learning, natural language processing, and more, promises even more exciting prospects. For any industry looking to remain competitive and innovative, embracing AI is not just an option but a necessity.
Further Reading and Resources
For those intrigued by AI’s expansive role across various sectors and looking to dive deeper into its capabilities and real-world applications, numerous resources are available.
Books like "AI Superpowers" by Kai-Fu Lee offer insights into how AI is shaping global industries. Journals like "Artificial Intelligence" and online platforms such as Google Scholar provide access to the latest research and case studies.
Microsoft AI Customer Stories:
Microsoft AI showcases various customer stories where AI technologies are being applied across different sectors.
Examples include Zammo's conversational AI platform, CarMax's customer review summaries, Broward College's student support strategies, and IPLAND's retail display optimization using image recognition technology.
Amazon AWS Case Studies:
Amazon AWS presents customer success stories, including BMW Group's utilization of AWS technology to power over 1,000 microservices processing billions of requests daily with high reliability.
The platform offers a range of case studies demonstrating how organizations accelerate innovation, increase agility, and lower costs through AWS cloud services.
Whatfix Case Studies:
Whatfix showcases case studies on how organizations drive technology adoption for customers and employees through contextual in-app guidance and self-help support
These case studies cover a variety of industries and applications, providing insights into how AI adoption is enhancing user experiences and driving technology utilization.
By exploring these sources, you can gain a comprehensive understanding of how AI adoption is shaping industries and driving innovation across various sectors.
AI, a combination of machine learning, data analytics, and automation, is not just a futuristic concept but a present-day powerhouse driving innovation across various sectors.
From healthcare to finance, retail to manufacturing, and beyond, AI is at the forefront of revolutionizing how businesses operate, enhancing efficiency, and crafting personalized user experiences
The importance of AI adoption cannot be overstated. As we delve into this topic, we aim to shed light on the myriad ways AI technologies are being integrated into different industries, not just to streamline operations but to solve complex problems and create opportunities that were unimaginable just a few decades ago.
Our journey through this landscape will highlight significant success stories and insightful case studies that demonstrate the profound impact of AI.
Healthcare Industry
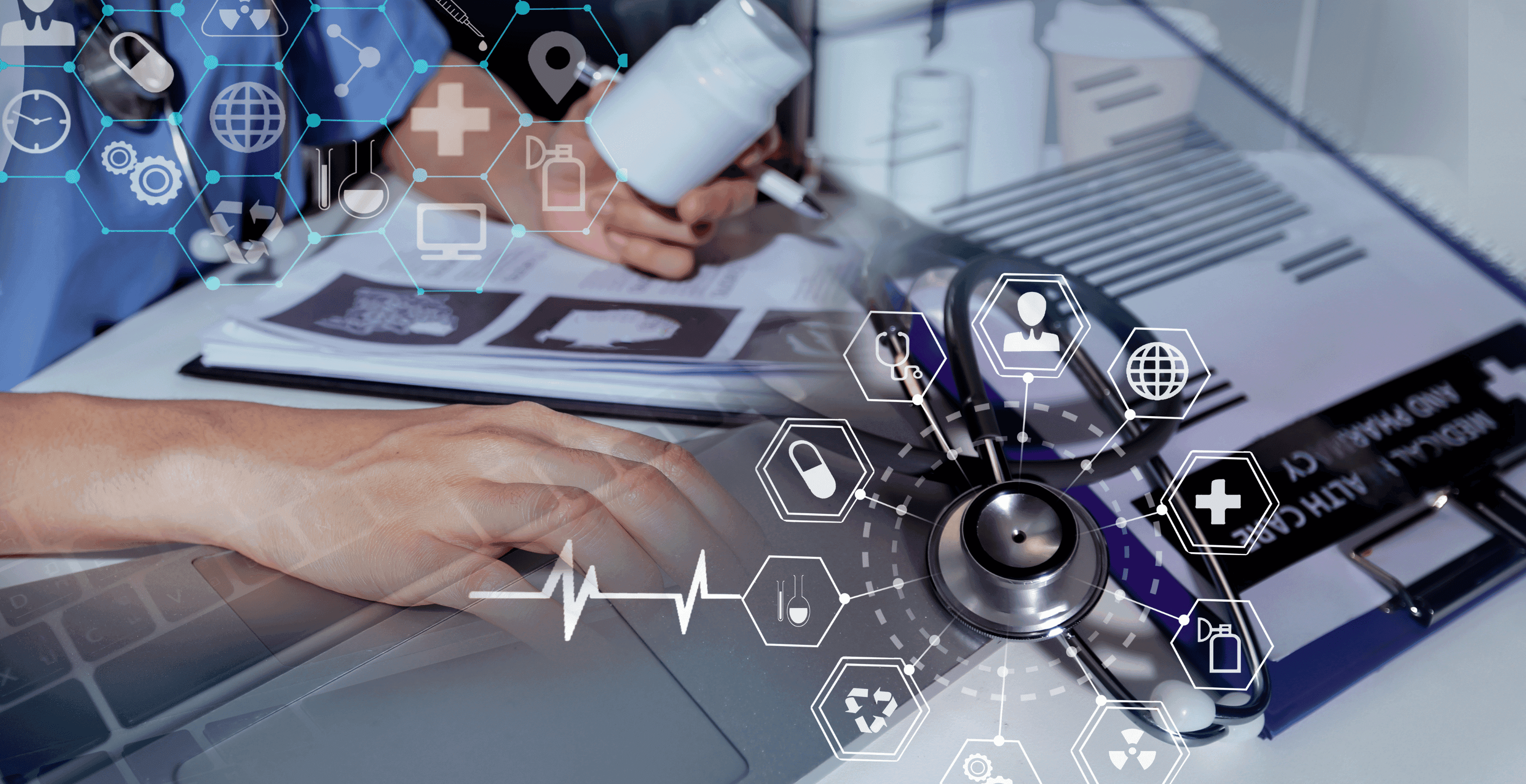
AI-Assisted Drug Discovery and Development
One notable example of AI's impact on drug discovery is the work of Baidu Research, which developed deep learning algorithms that outperform humans in identifying breast cancer metastasis.
These algorithms demonstrate AI's ability to analyze complex datasets, including genomic information, patient records, and drug compound libraries, to uncover insights that may not be immediately apparent to human researchers.
AI has significantly improved the efficiency of drug discovery at companies like Baidu Research in several key ways:
Accelerating lead compound discovery: AI-powered virtual screening tools can rapidly evaluate millions of chemical compounds to identify those with the highest potential for therapeutic activity, speeding up the lead compound discovery process.
Optimizing mRNA vaccine design: Baidu Research developed an AI algorithm called LinearDesign that can rapidly design highly stable COVID-19 mRNA vaccine sequences, achieving a 128-fold increase in antibody response compared to previous methods. This breakthrough can be applied to a wider range of therapeutic proteins.
Predicting drug properties: Baidu Research has developed novel machine learning-based computational biology algorithms for predicting drug properties, binding affinity, and designing new drug candidates, aiming to provide an AI-enhanced tool for facilitating drug discovery.
Leveraging knowledge graphs: Baidu's AI technologies, such as knowledge graphs, are being used to improve drug target discovery and validation, as well as small-molecule design. Knowledge graphs help connect disparate data sources to uncover new insights.
Optimizing workflows: By integrating AI into various stages of the drug discovery pipeline, including target identification, lead optimization, and preclinical testing, Baidu Research is able to streamline workflows and make the overall process more efficient.
These AI-driven advancements have the potential to significantly reduce the time and cost associated with drug development, which is typically a lengthy and expensive process. Baidu Research's work demonstrates the transformative impact AI can have on accelerating drug discovery and development.
Despite the promising results, it's important to note that AI is not a panacea for drug discovery challenges. Careful validation and integration with human expertise are crucial to ensure the reliability and safety of AI-generated insights.
Ongoing research and collaboration between AI experts and pharmaceutical researchers will be key to fully realizing the potential of AI in accelerating drug discovery and development.
AI for Fraud Detection and Risk Management
One notable success story in AI for fraud detection and risk management is the case of JPMorgan Chase. The bank implemented AI algorithms to enhance fraud detection capabilities, resulting in a significant reduction in false positives and improved detection accuracy.
By leveraging machine learning models, JPMorgan Chase was able to analyze vast amounts of transaction data in real-time, identifying suspicious patterns and anomalies that could indicate fraudulent activities.
JPMorgan Chase’s Use of AI:
Data Analysis: JPMorgan Chase's AI system processes large volumes of transaction data, looking for patterns indicative of fraudulent behavior.
Machine Learning Models: The bank uses sophisticated machine learning algorithms to detect anomalies and predict potential fraud.
Real-time Monitoring: The AI system operates in real-time, allowing for immediate detection and response to suspicious activities.
Continuous Learning: The system continuously learns from new data and feedback, improving its accuracy and staying ahead of evolving fraud tactics.
Reduced False Positives: By leveraging AI, JPMorgan Chase has significantly reduced false positives, minimizing disruptions to legitimate transactions.
Enhanced Detection Accuracy: The AI algorithms have improved the bank's ability to detect fraudulent activities early, preventing financial losses and protecting customers.
Impact:
Improved Fraud Prevention: JPMorgan Chase's AI implementation has strengthened its fraud prevention capabilities, safeguarding customer assets and financial transactions.
Cost Savings: By reducing false positives and improving detection accuracy, the bank has saved resources previously spent on investigating false alarms.
Enhanced Customer Trust: The proactive fraud detection enabled by AI has enhanced customer trust and confidence in the bank's security measures.
Industry Leadership: JPMorgan Chase's success in AI-driven fraud detection has positioned it as a leader in leveraging technology for risk management in the banking sector.
The success of JPMorgan Chase's AI implementation showcases the power of AI in combating financial fraud, enhancing risk management practices, and protecting both the institution and its customers from fraudulent activities.
Retail and E-commerce
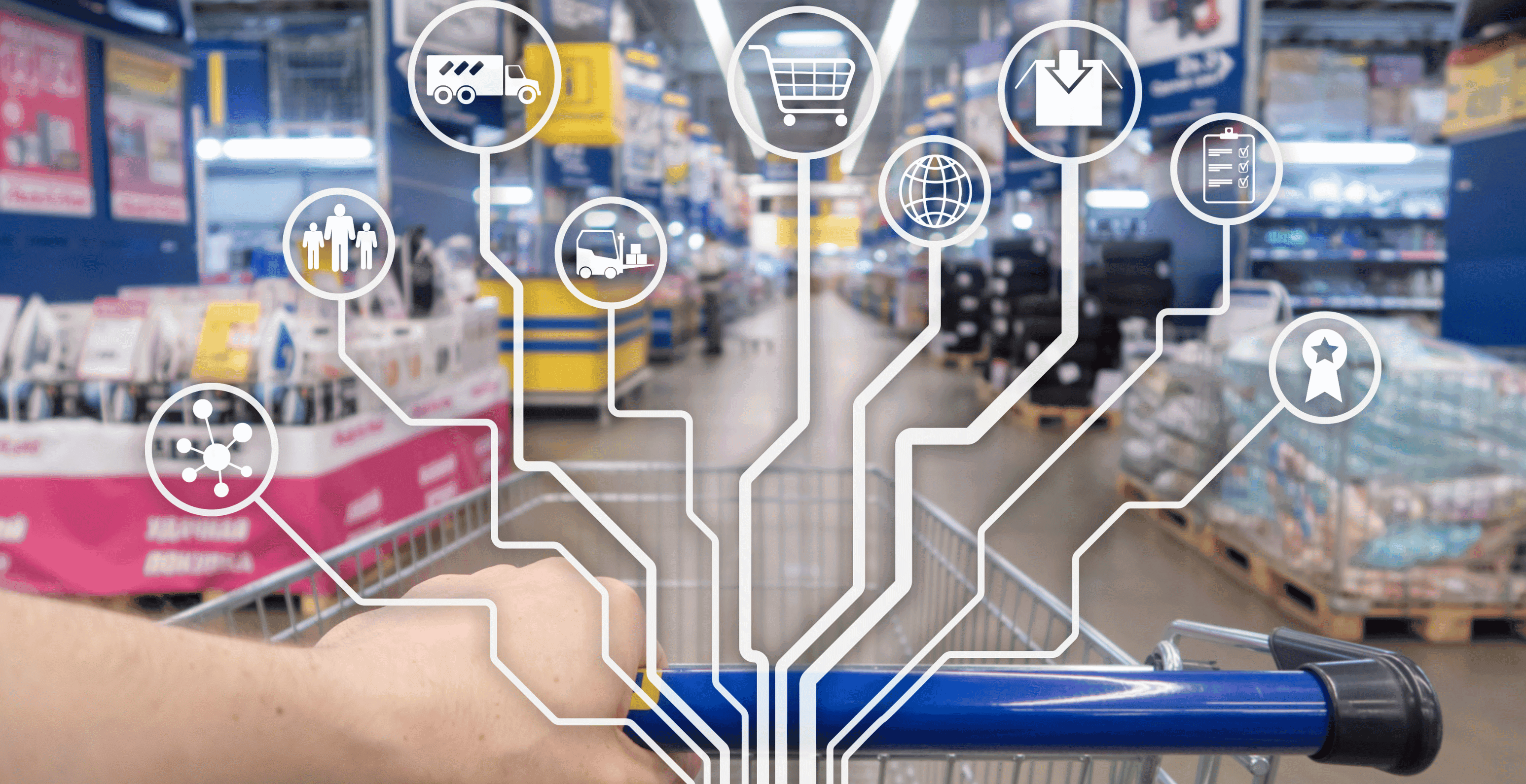
AI for Product Recommendation and Personalization
Amazon, the e-commerce giant, has been at the forefront of leveraging AI for product recommendation and personalization.
By analyzing customer browsing history, purchase patterns, and preferences, Amazon's AI algorithms generate highly accurate and personalized product recommendations123. This has significantly boosted customer engagement, increased sales, and improved the overall shopping experience.
How Amazon's AI-Powered Recommendations Work?
Amazon's recommendation system collects and analyzes vast amounts of customer data, including:
Browsing history: The products a customer has viewed or searched for
Purchase history: The products a customer has bought in the past
Ratings and reviews: The customer's ratings and reviews of products
Demographic data: The customer's age, gender, location, and other relevant information
Using this data, Amazon's AI algorithms identify patterns and correlations to predict what a customer might be interested in.
The system also takes into account the behavior of similar customers to provide even more personalized recommendations1.
Benefits of AI-Powered Recommendations
By implementing AI-driven product recommendations, Amazon has achieved several key benefits:
Increased sales: Personalized recommendations have been shown to drive a significant increase in sales, as customers are more likely to purchase products that are tailored to their preferences2.
Enhanced customer engagement: Relevant and engaging product recommendations keep customers on the site for longer, leading to increased browsing and exploration of Amazon's vast product catalog1.
Improved customer satisfaction: Customers appreciate the convenience and relevance of personalized recommendations, leading to higher satisfaction levels and increased loyalty to the Amazon brand3.
Competitive advantage: Amazon's advanced AI capabilities in product recommendation and personalization have given it a significant edge over competitors in the e-commerce space2.
Future Developments
As AI technology continues to advance, Amazon is likely to further refine and enhance its product recommendation system. Potential future developments may include:
Incorporating real-time data: Using AI to analyze customer behavior and preferences in real-time, allowing for even more dynamic and responsive recommendations4.
Expanding to new channels: Applying AI-powered recommendations to other channels, such as mobile apps and voice assistants, to provide a seamless and consistent experience across all touchpoints5.
Leveraging new data sources: Integrating additional data sources, such as social media activity and external market trends, to further improve the accuracy and relevance of recommendations3.
By continuously investing in and improving its AI capabilities, Amazon is well-positioned to maintain its leadership in the e-commerce industry and deliver exceptional customer experiences through personalized product recommendations.
Manufacturing and Industrial Automation
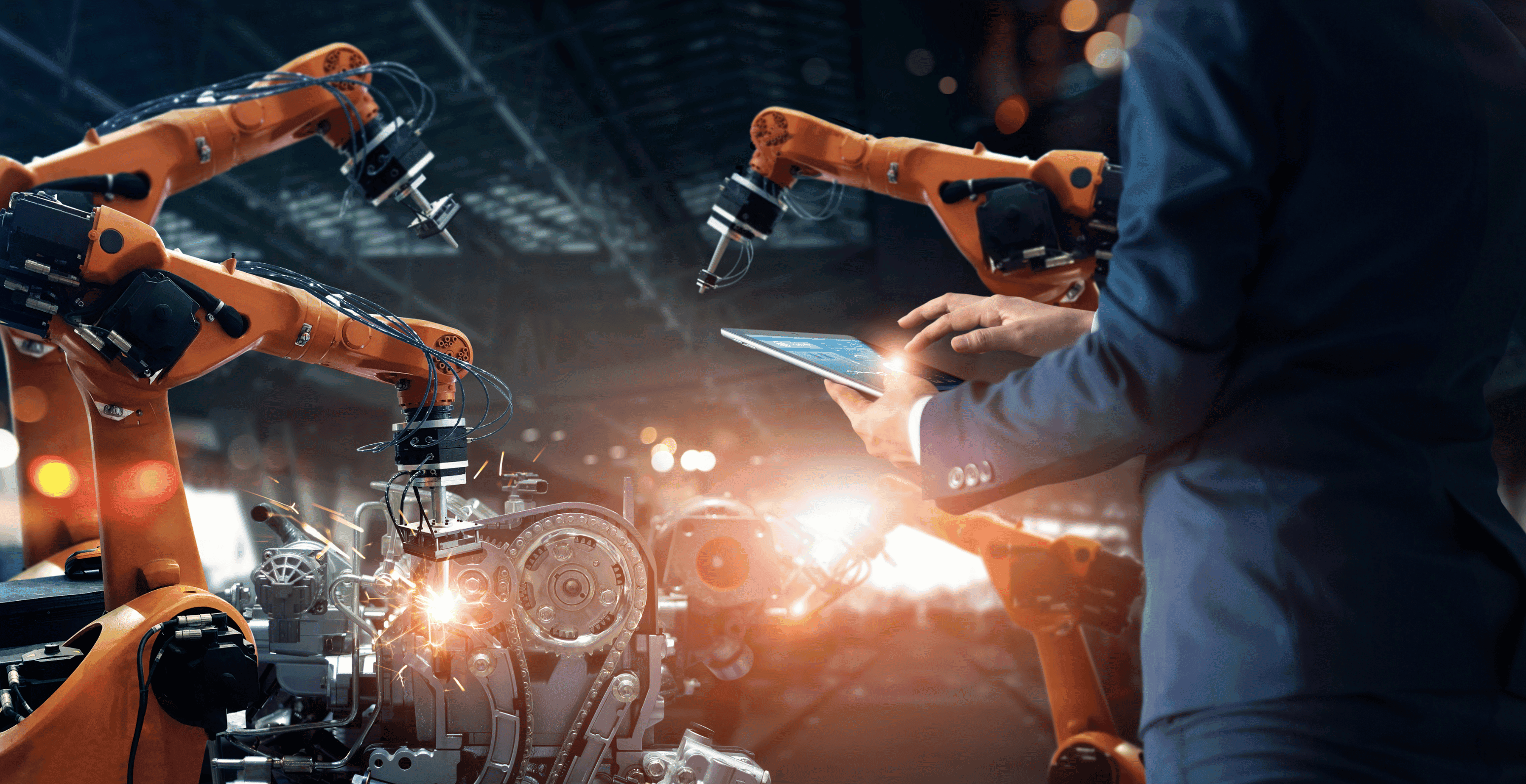
AI for Predictive Maintenance and Quality Control
A notable example of AI for predictive maintenance and quality control is the implementation by Siemens. The company leverages AI and machine learning to maximize productivity in the industrial and manufacturing sectors.
By exploring the capabilities of AI and machine learning, Siemens has developed technologies that reduce machinery costs, improve consistency with automation, and deliver instant data for quickly adjusting workflows 2.
Siemens' Approach to AI in Predictive Maintenance and Quality Control
Siemens utilizes AI and machine learning in the following ways to enhance predictive maintenance and quality control:
Predictive Maintenance: By analyzing real-time data from sensors embedded in machinery, Siemens' AI algorithms can predict potential equipment failures before they occur. This proactive approach allows for timely maintenance, reducing downtime and minimizing repair costs.
Quality Control: AI-driven quality control systems analyze production data to predict potential quality issues before they occur. By identifying anomalies and deviations in the manufacturing process, Siemens can take corrective actions to maintain product quality standards.
Automation and Consistency: AI technologies enable automation of manual processes, ensuring consistency in manufacturing operations. By leveraging machine learning algorithms, Siemens can optimize workflows, reduce errors, and improve operational efficiency.
Data-Driven Decision-Making: Siemens' AI systems provide instant data insights, allowing for quick adjustments in production processes. Real-time analytics enable agile decision-making, enhancing overall productivity and responsiveness to changing demands.
Impact and Benefits
The implementation of AI for predictive maintenance and quality control at Siemens has resulted in several key benefits:
Maximized Productivity: By leveraging AI technologies, Siemens has optimized machinery performance, reduced downtime, and maximized productivity in manufacturing operations.
Cost Reduction: Predictive maintenance has helped Siemens reduce machinery costs by proactively addressing maintenance needs and avoiding costly breakdowns.
Improved Quality: AI-driven quality control systems have enhanced product quality by identifying and addressing potential issues early in the manufacturing process.
Enhanced Automation: Automation through AI has improved consistency, reduced errors, and streamlined workflows, leading to increased operational efficiency.
Agile Decision-Making: Real-time data insights provided by AI systems enable Siemens to make quick and informed decisions, ensuring responsiveness to market demands and operational changes.
Siemens' successful integration of AI for predictive maintenance and quality control showcases the transformative potential of AI in optimizing industrial processes, reducing costs, and enhancing overall operational efficiency in the manufacturing sector.
Marketing and Advertising
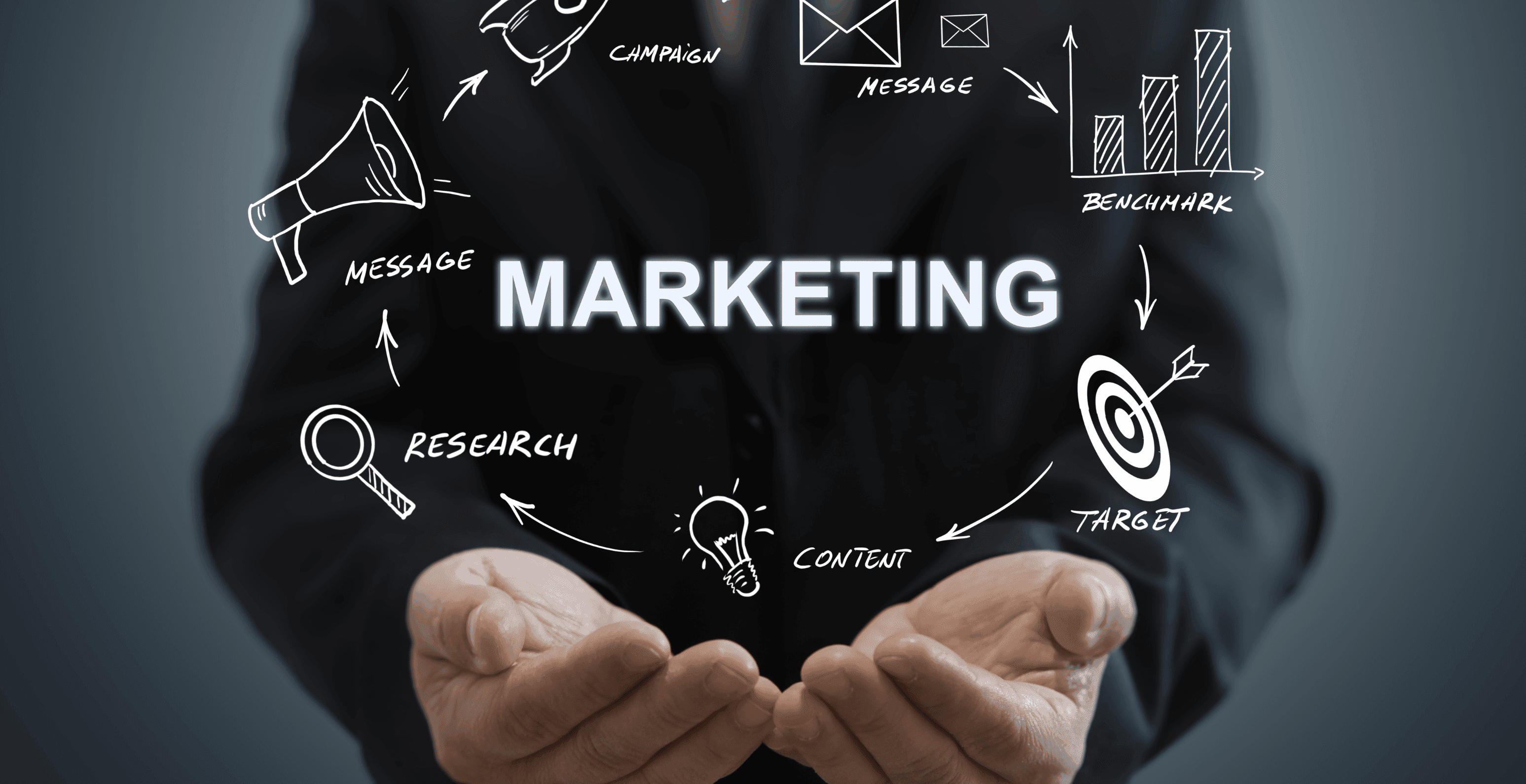
AI for Targeted Advertising and Campaign Optimization
Vanguard, one of the world's largest investment firms, utilized AI for targeted advertising and campaign optimization by partnering with the AI language platform Persado to conduct highly personalized advertising on LinkedIn, the only platform where they could run ads due to regulations.
Leveraging AI technology, Vanguard hyper-personalized its ads and tested them at scale to determine the most effective approaches that resonated with consumers. This strategic use of AI resulted in a significant 15% increase in conversion rates for Vanguard's advertising campaigns4.
Vanguard's Approach to AI-Powered Advertising:
Hyper-Personalization: Vanguard employed AI to create highly personalized ads tailored to individual consumers based on their preferences and behaviors.
Scale Testing: AI allowed Vanguard to test multiple ad variations at scale to identify the most effective messaging and creative elements.
Regulatory Compliance: Due to regulatory restrictions, Vanguard focused its AI-powered advertising efforts on LinkedIn, optimizing its campaigns for this specific platform.
Conversion Rate Optimization: By leveraging AI insights, Vanguard was able to optimize its advertising campaigns to drive a 15% increase in conversion rates.
Impact and Benefits:
Increased Conversions: The use of AI for targeted advertising led to a substantial 15% increase in conversion rates for Vanguard's campaigns.
Personalized Engagement: AI-driven hyper-personalization enhanced customer engagement by delivering tailored messages that resonated with individual preferences.
Efficiency and Effectiveness: AI technology enabled Vanguard to optimize its advertising efforts, ensuring that resources were allocated to the most impactful campaigns.
Regulatory Compliance: By focusing on LinkedIn for AI-powered advertising, Vanguard navigated regulatory constraints while still achieving significant performance improvements.
Future Applications:
Further Personalization: Vanguard can continue to refine its AI-powered advertising strategies to deliver even more personalized and targeted campaigns.
Multi-Platform Optimization: Expanding AI-driven advertising efforts to other platforms while maintaining regulatory compliance to reach a broader audience.
Continuous Testing and Optimization: Vanguard can leverage AI for ongoing testing and optimization of ad campaigns to adapt to changing consumer behaviors and preferences.
Vanguard's success in leveraging AI for targeted advertising and campaign optimization exemplifies the transformative impact of AI in enhancing marketing strategies, driving conversions, and delivering personalized experiences to consumers.
Education and Learning
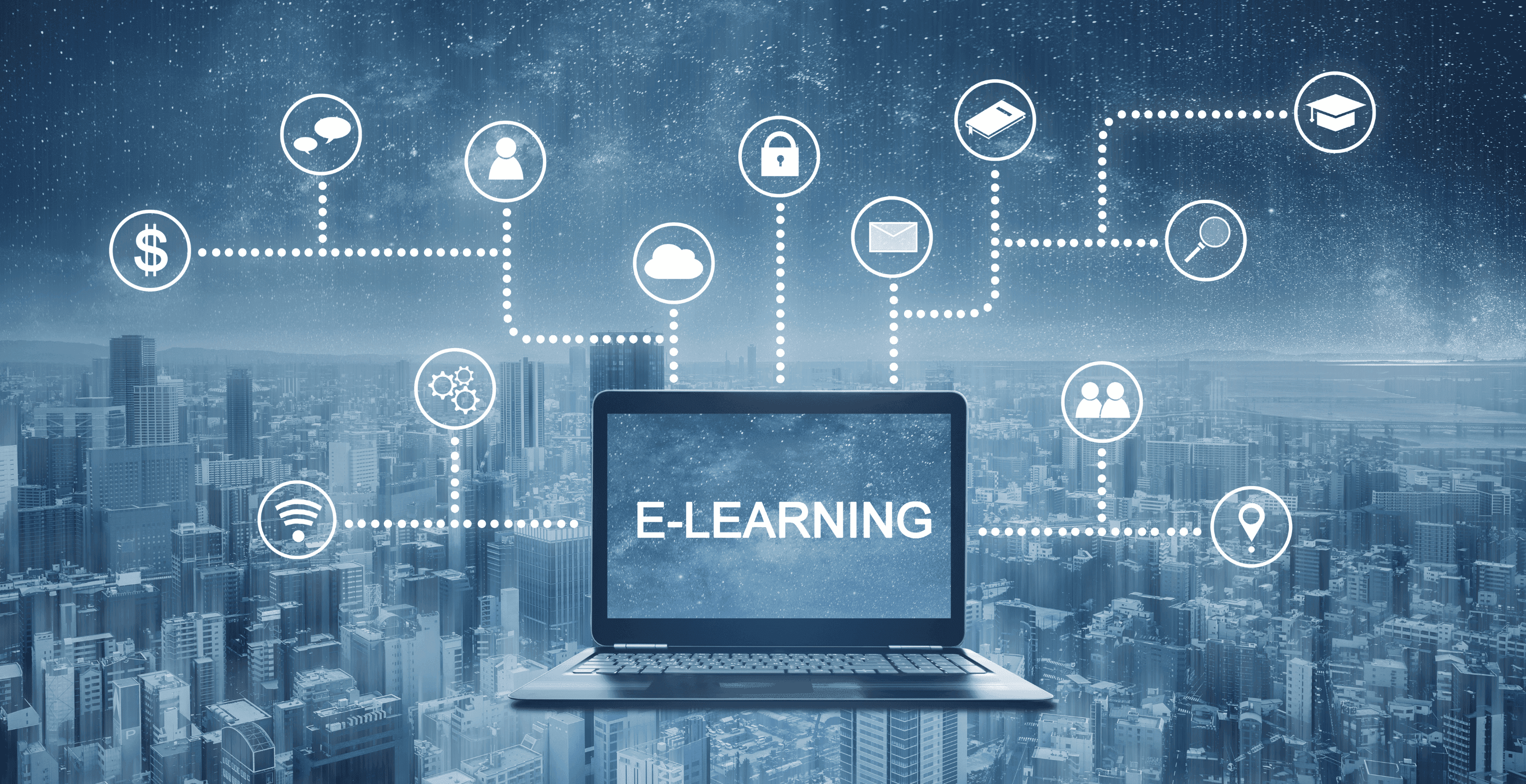
AI-Powered Virtual Tutors and Teaching Assistants
A notable example of AI-powered virtual tutors and teaching assistants is the work of Riiid Labs. This subsidiary of Riiid develops AI-based learning and assessment technologies that can be integrated into existing applications or used as standalone platforms13.
By leveraging machine learning capabilities, Riiid Labs' technology assists teachers in tracking student performance, automating assignment scoring, and personalizing curriculum needs for each student.
Riiid Labs' AI-Powered Virtual Tutoring System
Riiid Labs' AI-powered virtual tutoring system utilizes advanced machine learning algorithms to provide personalized learning experiences for students. The system works by:
Collecting and analyzing student data: The platform gathers information on each student's performance, learning patterns, and progress to create a personalized profile.
Adapting learning content: Based on the student's profile, the AI system selects and presents the most appropriate learning materials, exercises, and assessments tailored to their individual needs and learning style.
Providing real-time feedback: The virtual tutor offers immediate feedback and guidance to students, helping them identify areas of strength and weakness and providing targeted support.
Automating grading and scoring: The AI system can automatically grade assignments and assessments, freeing up teachers' time and providing consistent and timely feedback to students.
Predicting future performance: By analyzing student data over time, the AI can predict each student's future academic performance and identify potential areas of concern, allowing teachers to intervene early and provide additional support.
Impact and Benefits
The implementation of Riiid Labs' AI-powered virtual tutoring system has resulted in several key benefits for both students and teachers:
Personalized learning experiences: Students receive tailored instruction and support based on their individual needs, leading to improved engagement and learning outcomes.
Increased efficiency: Teachers can focus on providing high-quality instruction and mentorship while the AI system handles routine tasks such as grading and performance tracking.
Early intervention: By predicting potential academic challenges, teachers can intervene early and provide targeted support to students, preventing them from falling behind.
Scalability: The AI-powered system can provide personalized learning experiences to a large number of students simultaneously, making it a scalable solution for educational institutions.
Continuous improvement: The AI system learns and adapts over time, continuously improving its ability to provide personalized learning experiences and predict student performance.
Riiid Labs' success in developing AI-powered virtual tutoring systems demonstrates the transformative potential of AI in enhancing teaching and learning experiences, leading to improved student outcomes and increased efficiency for educators.
Conclusion
We've journeyed across a landscape where AI's influence is profound and pervasive. From diagnosing diseases with uncanny accuracy to managing global supply chains, and from personalizing learning experiences to revolutionizing customer interactions, AI’s capabilities are vast and varied.
These success stories and case studies not only illuminate AI's potential to enhance business outcomes but also highlight its role in driving competitive advantages in a rapidly evolving world.
As businesses and sectors continue to harness AI's power, the pathway to innovation seems increasingly intertwined with intelligent technology.
The future, rich with advancements in machine learning, natural language processing, and more, promises even more exciting prospects. For any industry looking to remain competitive and innovative, embracing AI is not just an option but a necessity.
Further Reading and Resources
For those intrigued by AI’s expansive role across various sectors and looking to dive deeper into its capabilities and real-world applications, numerous resources are available.
Books like "AI Superpowers" by Kai-Fu Lee offer insights into how AI is shaping global industries. Journals like "Artificial Intelligence" and online platforms such as Google Scholar provide access to the latest research and case studies.
Microsoft AI Customer Stories:
Microsoft AI showcases various customer stories where AI technologies are being applied across different sectors.
Examples include Zammo's conversational AI platform, CarMax's customer review summaries, Broward College's student support strategies, and IPLAND's retail display optimization using image recognition technology.
Amazon AWS Case Studies:
Amazon AWS presents customer success stories, including BMW Group's utilization of AWS technology to power over 1,000 microservices processing billions of requests daily with high reliability.
The platform offers a range of case studies demonstrating how organizations accelerate innovation, increase agility, and lower costs through AWS cloud services.
Whatfix Case Studies:
Whatfix showcases case studies on how organizations drive technology adoption for customers and employees through contextual in-app guidance and self-help support
These case studies cover a variety of industries and applications, providing insights into how AI adoption is enhancing user experiences and driving technology utilization.
By exploring these sources, you can gain a comprehensive understanding of how AI adoption is shaping industries and driving innovation across various sectors.
AI, a combination of machine learning, data analytics, and automation, is not just a futuristic concept but a present-day powerhouse driving innovation across various sectors.
From healthcare to finance, retail to manufacturing, and beyond, AI is at the forefront of revolutionizing how businesses operate, enhancing efficiency, and crafting personalized user experiences
The importance of AI adoption cannot be overstated. As we delve into this topic, we aim to shed light on the myriad ways AI technologies are being integrated into different industries, not just to streamline operations but to solve complex problems and create opportunities that were unimaginable just a few decades ago.
Our journey through this landscape will highlight significant success stories and insightful case studies that demonstrate the profound impact of AI.
Healthcare Industry
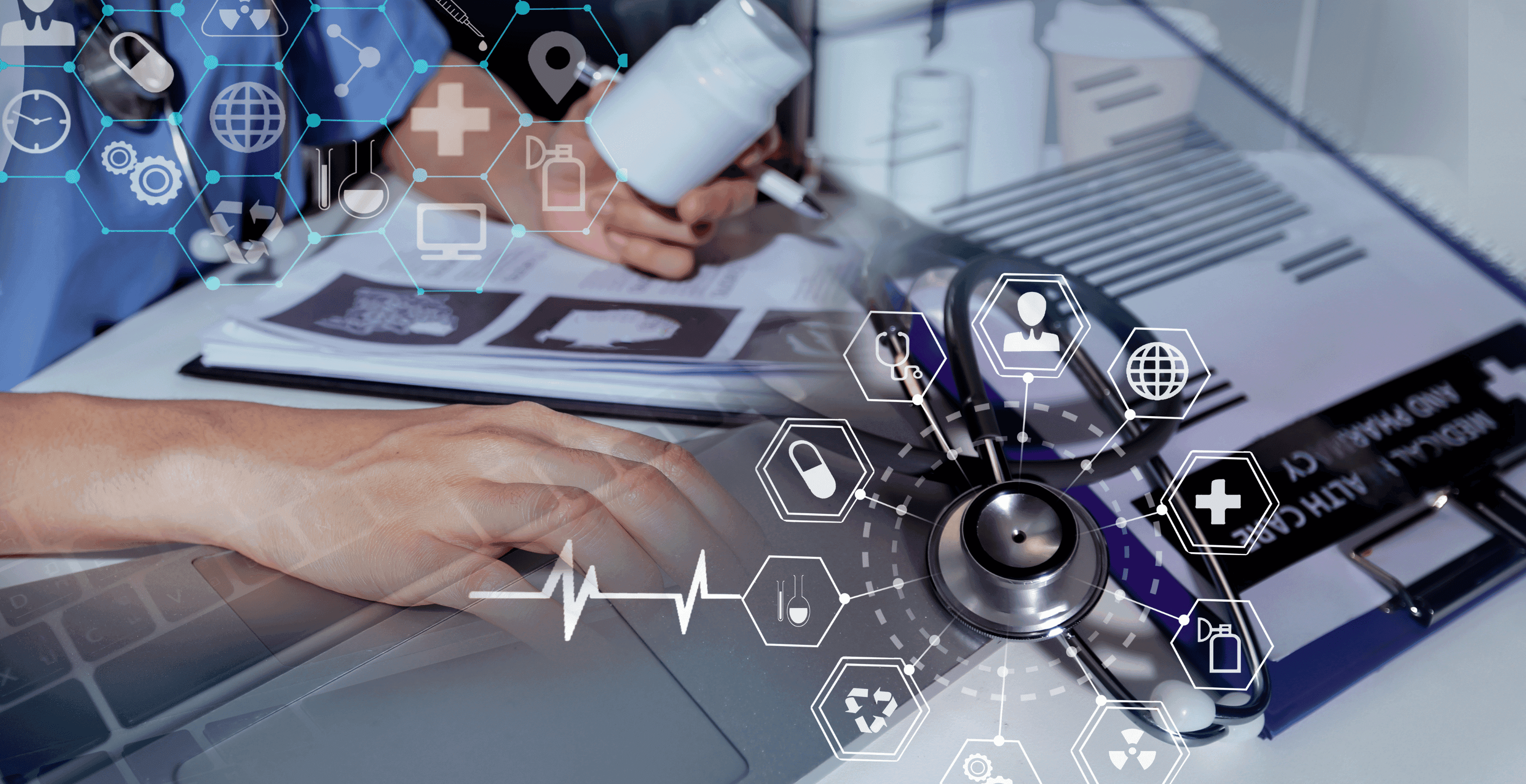
AI-Assisted Drug Discovery and Development
One notable example of AI's impact on drug discovery is the work of Baidu Research, which developed deep learning algorithms that outperform humans in identifying breast cancer metastasis.
These algorithms demonstrate AI's ability to analyze complex datasets, including genomic information, patient records, and drug compound libraries, to uncover insights that may not be immediately apparent to human researchers.
AI has significantly improved the efficiency of drug discovery at companies like Baidu Research in several key ways:
Accelerating lead compound discovery: AI-powered virtual screening tools can rapidly evaluate millions of chemical compounds to identify those with the highest potential for therapeutic activity, speeding up the lead compound discovery process.
Optimizing mRNA vaccine design: Baidu Research developed an AI algorithm called LinearDesign that can rapidly design highly stable COVID-19 mRNA vaccine sequences, achieving a 128-fold increase in antibody response compared to previous methods. This breakthrough can be applied to a wider range of therapeutic proteins.
Predicting drug properties: Baidu Research has developed novel machine learning-based computational biology algorithms for predicting drug properties, binding affinity, and designing new drug candidates, aiming to provide an AI-enhanced tool for facilitating drug discovery.
Leveraging knowledge graphs: Baidu's AI technologies, such as knowledge graphs, are being used to improve drug target discovery and validation, as well as small-molecule design. Knowledge graphs help connect disparate data sources to uncover new insights.
Optimizing workflows: By integrating AI into various stages of the drug discovery pipeline, including target identification, lead optimization, and preclinical testing, Baidu Research is able to streamline workflows and make the overall process more efficient.
These AI-driven advancements have the potential to significantly reduce the time and cost associated with drug development, which is typically a lengthy and expensive process. Baidu Research's work demonstrates the transformative impact AI can have on accelerating drug discovery and development.
Despite the promising results, it's important to note that AI is not a panacea for drug discovery challenges. Careful validation and integration with human expertise are crucial to ensure the reliability and safety of AI-generated insights.
Ongoing research and collaboration between AI experts and pharmaceutical researchers will be key to fully realizing the potential of AI in accelerating drug discovery and development.
AI for Fraud Detection and Risk Management
One notable success story in AI for fraud detection and risk management is the case of JPMorgan Chase. The bank implemented AI algorithms to enhance fraud detection capabilities, resulting in a significant reduction in false positives and improved detection accuracy.
By leveraging machine learning models, JPMorgan Chase was able to analyze vast amounts of transaction data in real-time, identifying suspicious patterns and anomalies that could indicate fraudulent activities.
JPMorgan Chase’s Use of AI:
Data Analysis: JPMorgan Chase's AI system processes large volumes of transaction data, looking for patterns indicative of fraudulent behavior.
Machine Learning Models: The bank uses sophisticated machine learning algorithms to detect anomalies and predict potential fraud.
Real-time Monitoring: The AI system operates in real-time, allowing for immediate detection and response to suspicious activities.
Continuous Learning: The system continuously learns from new data and feedback, improving its accuracy and staying ahead of evolving fraud tactics.
Reduced False Positives: By leveraging AI, JPMorgan Chase has significantly reduced false positives, minimizing disruptions to legitimate transactions.
Enhanced Detection Accuracy: The AI algorithms have improved the bank's ability to detect fraudulent activities early, preventing financial losses and protecting customers.
Impact:
Improved Fraud Prevention: JPMorgan Chase's AI implementation has strengthened its fraud prevention capabilities, safeguarding customer assets and financial transactions.
Cost Savings: By reducing false positives and improving detection accuracy, the bank has saved resources previously spent on investigating false alarms.
Enhanced Customer Trust: The proactive fraud detection enabled by AI has enhanced customer trust and confidence in the bank's security measures.
Industry Leadership: JPMorgan Chase's success in AI-driven fraud detection has positioned it as a leader in leveraging technology for risk management in the banking sector.
The success of JPMorgan Chase's AI implementation showcases the power of AI in combating financial fraud, enhancing risk management practices, and protecting both the institution and its customers from fraudulent activities.
Retail and E-commerce
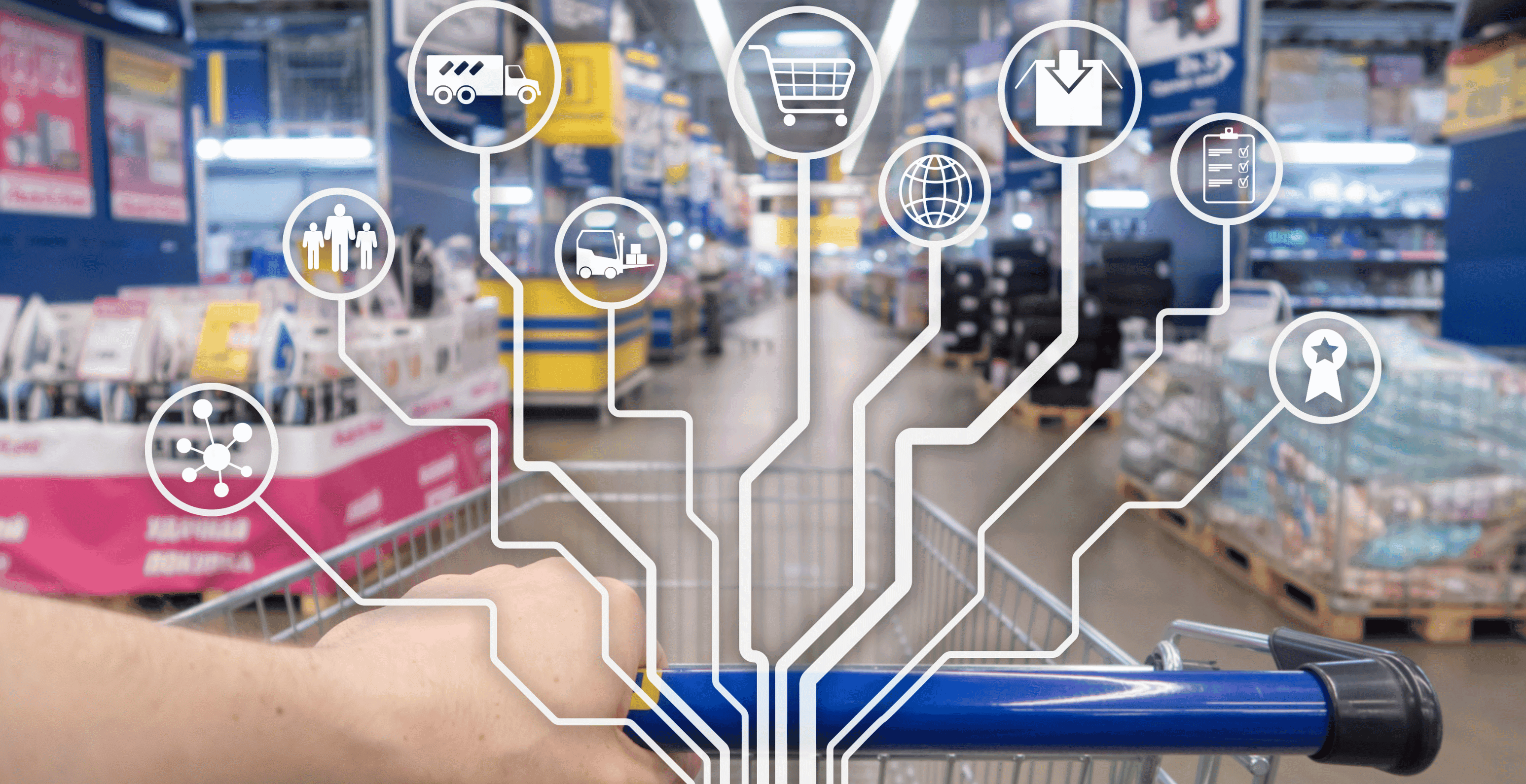
AI for Product Recommendation and Personalization
Amazon, the e-commerce giant, has been at the forefront of leveraging AI for product recommendation and personalization.
By analyzing customer browsing history, purchase patterns, and preferences, Amazon's AI algorithms generate highly accurate and personalized product recommendations123. This has significantly boosted customer engagement, increased sales, and improved the overall shopping experience.
How Amazon's AI-Powered Recommendations Work?
Amazon's recommendation system collects and analyzes vast amounts of customer data, including:
Browsing history: The products a customer has viewed or searched for
Purchase history: The products a customer has bought in the past
Ratings and reviews: The customer's ratings and reviews of products
Demographic data: The customer's age, gender, location, and other relevant information
Using this data, Amazon's AI algorithms identify patterns and correlations to predict what a customer might be interested in.
The system also takes into account the behavior of similar customers to provide even more personalized recommendations1.
Benefits of AI-Powered Recommendations
By implementing AI-driven product recommendations, Amazon has achieved several key benefits:
Increased sales: Personalized recommendations have been shown to drive a significant increase in sales, as customers are more likely to purchase products that are tailored to their preferences2.
Enhanced customer engagement: Relevant and engaging product recommendations keep customers on the site for longer, leading to increased browsing and exploration of Amazon's vast product catalog1.
Improved customer satisfaction: Customers appreciate the convenience and relevance of personalized recommendations, leading to higher satisfaction levels and increased loyalty to the Amazon brand3.
Competitive advantage: Amazon's advanced AI capabilities in product recommendation and personalization have given it a significant edge over competitors in the e-commerce space2.
Future Developments
As AI technology continues to advance, Amazon is likely to further refine and enhance its product recommendation system. Potential future developments may include:
Incorporating real-time data: Using AI to analyze customer behavior and preferences in real-time, allowing for even more dynamic and responsive recommendations4.
Expanding to new channels: Applying AI-powered recommendations to other channels, such as mobile apps and voice assistants, to provide a seamless and consistent experience across all touchpoints5.
Leveraging new data sources: Integrating additional data sources, such as social media activity and external market trends, to further improve the accuracy and relevance of recommendations3.
By continuously investing in and improving its AI capabilities, Amazon is well-positioned to maintain its leadership in the e-commerce industry and deliver exceptional customer experiences through personalized product recommendations.
Manufacturing and Industrial Automation
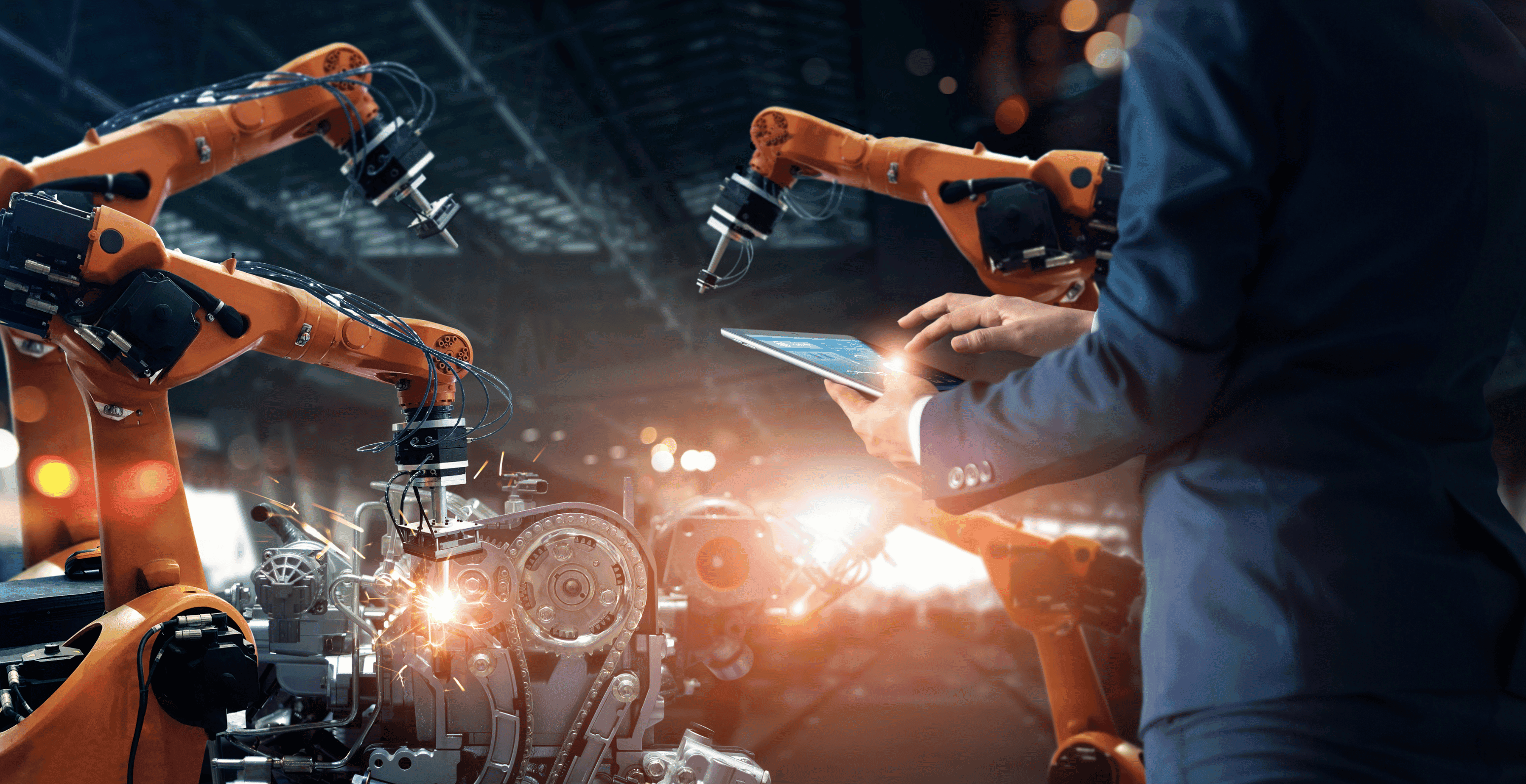
AI for Predictive Maintenance and Quality Control
A notable example of AI for predictive maintenance and quality control is the implementation by Siemens. The company leverages AI and machine learning to maximize productivity in the industrial and manufacturing sectors.
By exploring the capabilities of AI and machine learning, Siemens has developed technologies that reduce machinery costs, improve consistency with automation, and deliver instant data for quickly adjusting workflows 2.
Siemens' Approach to AI in Predictive Maintenance and Quality Control
Siemens utilizes AI and machine learning in the following ways to enhance predictive maintenance and quality control:
Predictive Maintenance: By analyzing real-time data from sensors embedded in machinery, Siemens' AI algorithms can predict potential equipment failures before they occur. This proactive approach allows for timely maintenance, reducing downtime and minimizing repair costs.
Quality Control: AI-driven quality control systems analyze production data to predict potential quality issues before they occur. By identifying anomalies and deviations in the manufacturing process, Siemens can take corrective actions to maintain product quality standards.
Automation and Consistency: AI technologies enable automation of manual processes, ensuring consistency in manufacturing operations. By leveraging machine learning algorithms, Siemens can optimize workflows, reduce errors, and improve operational efficiency.
Data-Driven Decision-Making: Siemens' AI systems provide instant data insights, allowing for quick adjustments in production processes. Real-time analytics enable agile decision-making, enhancing overall productivity and responsiveness to changing demands.
Impact and Benefits
The implementation of AI for predictive maintenance and quality control at Siemens has resulted in several key benefits:
Maximized Productivity: By leveraging AI technologies, Siemens has optimized machinery performance, reduced downtime, and maximized productivity in manufacturing operations.
Cost Reduction: Predictive maintenance has helped Siemens reduce machinery costs by proactively addressing maintenance needs and avoiding costly breakdowns.
Improved Quality: AI-driven quality control systems have enhanced product quality by identifying and addressing potential issues early in the manufacturing process.
Enhanced Automation: Automation through AI has improved consistency, reduced errors, and streamlined workflows, leading to increased operational efficiency.
Agile Decision-Making: Real-time data insights provided by AI systems enable Siemens to make quick and informed decisions, ensuring responsiveness to market demands and operational changes.
Siemens' successful integration of AI for predictive maintenance and quality control showcases the transformative potential of AI in optimizing industrial processes, reducing costs, and enhancing overall operational efficiency in the manufacturing sector.
Marketing and Advertising
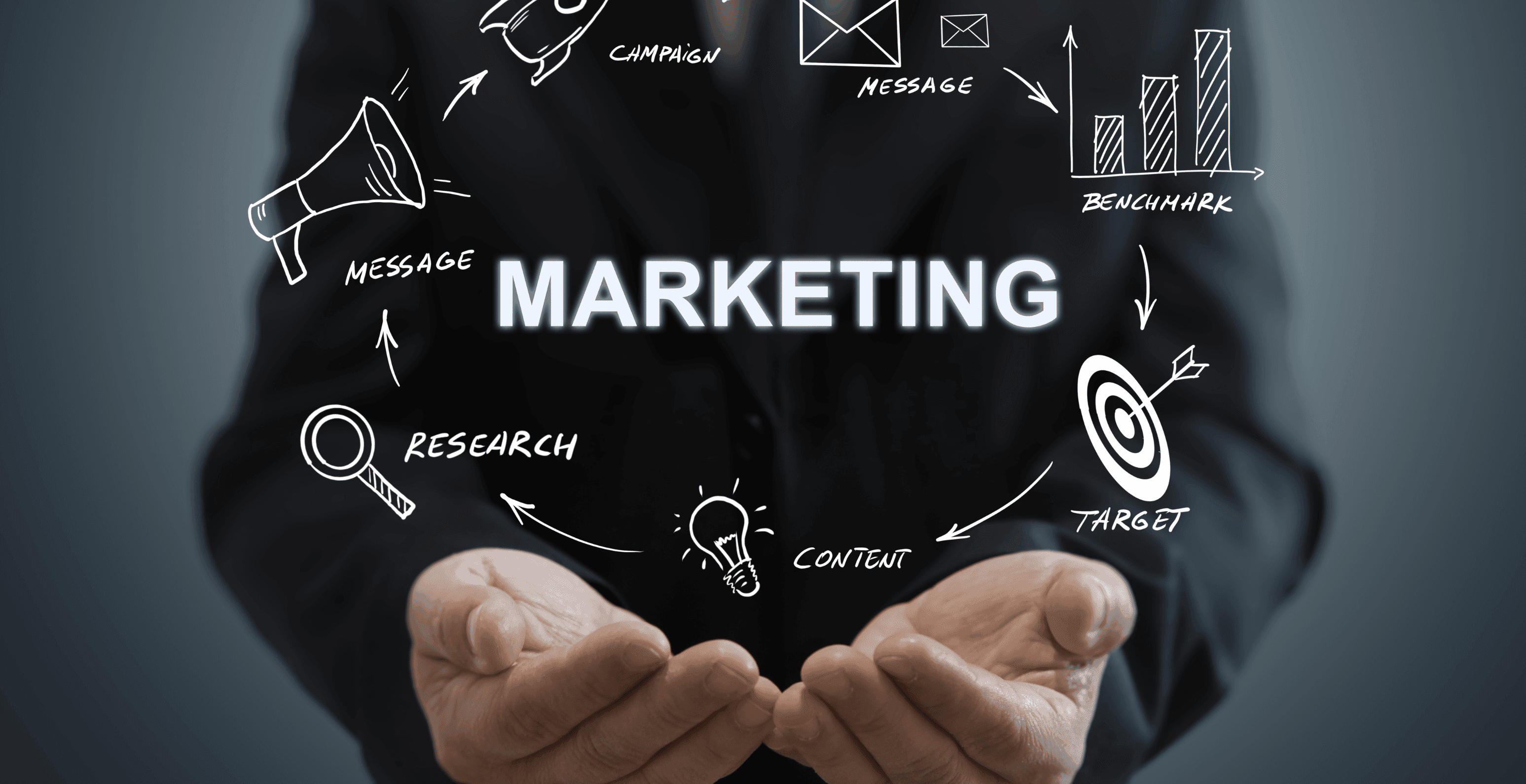
AI for Targeted Advertising and Campaign Optimization
Vanguard, one of the world's largest investment firms, utilized AI for targeted advertising and campaign optimization by partnering with the AI language platform Persado to conduct highly personalized advertising on LinkedIn, the only platform where they could run ads due to regulations.
Leveraging AI technology, Vanguard hyper-personalized its ads and tested them at scale to determine the most effective approaches that resonated with consumers. This strategic use of AI resulted in a significant 15% increase in conversion rates for Vanguard's advertising campaigns4.
Vanguard's Approach to AI-Powered Advertising:
Hyper-Personalization: Vanguard employed AI to create highly personalized ads tailored to individual consumers based on their preferences and behaviors.
Scale Testing: AI allowed Vanguard to test multiple ad variations at scale to identify the most effective messaging and creative elements.
Regulatory Compliance: Due to regulatory restrictions, Vanguard focused its AI-powered advertising efforts on LinkedIn, optimizing its campaigns for this specific platform.
Conversion Rate Optimization: By leveraging AI insights, Vanguard was able to optimize its advertising campaigns to drive a 15% increase in conversion rates.
Impact and Benefits:
Increased Conversions: The use of AI for targeted advertising led to a substantial 15% increase in conversion rates for Vanguard's campaigns.
Personalized Engagement: AI-driven hyper-personalization enhanced customer engagement by delivering tailored messages that resonated with individual preferences.
Efficiency and Effectiveness: AI technology enabled Vanguard to optimize its advertising efforts, ensuring that resources were allocated to the most impactful campaigns.
Regulatory Compliance: By focusing on LinkedIn for AI-powered advertising, Vanguard navigated regulatory constraints while still achieving significant performance improvements.
Future Applications:
Further Personalization: Vanguard can continue to refine its AI-powered advertising strategies to deliver even more personalized and targeted campaigns.
Multi-Platform Optimization: Expanding AI-driven advertising efforts to other platforms while maintaining regulatory compliance to reach a broader audience.
Continuous Testing and Optimization: Vanguard can leverage AI for ongoing testing and optimization of ad campaigns to adapt to changing consumer behaviors and preferences.
Vanguard's success in leveraging AI for targeted advertising and campaign optimization exemplifies the transformative impact of AI in enhancing marketing strategies, driving conversions, and delivering personalized experiences to consumers.
Education and Learning
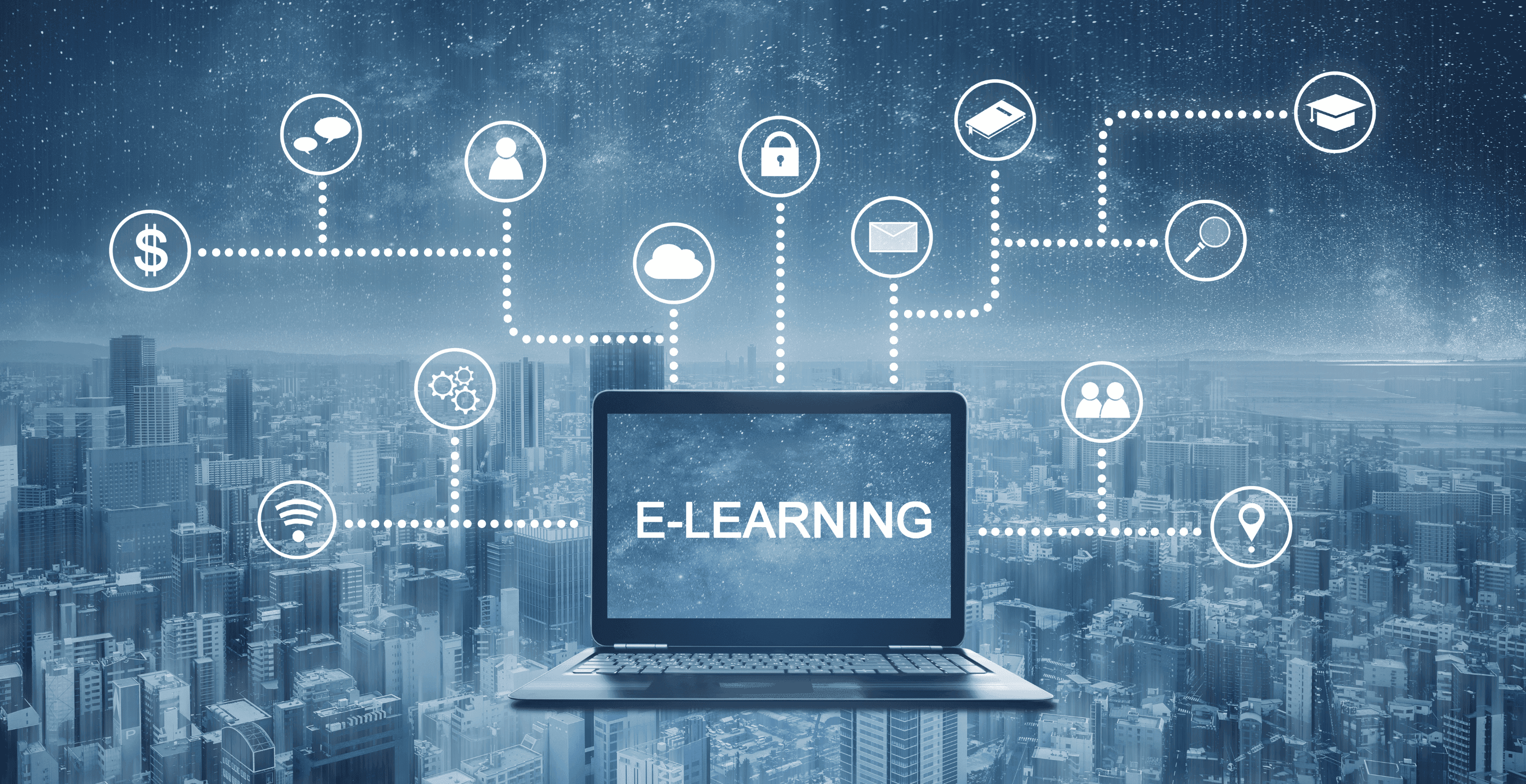
AI-Powered Virtual Tutors and Teaching Assistants
A notable example of AI-powered virtual tutors and teaching assistants is the work of Riiid Labs. This subsidiary of Riiid develops AI-based learning and assessment technologies that can be integrated into existing applications or used as standalone platforms13.
By leveraging machine learning capabilities, Riiid Labs' technology assists teachers in tracking student performance, automating assignment scoring, and personalizing curriculum needs for each student.
Riiid Labs' AI-Powered Virtual Tutoring System
Riiid Labs' AI-powered virtual tutoring system utilizes advanced machine learning algorithms to provide personalized learning experiences for students. The system works by:
Collecting and analyzing student data: The platform gathers information on each student's performance, learning patterns, and progress to create a personalized profile.
Adapting learning content: Based on the student's profile, the AI system selects and presents the most appropriate learning materials, exercises, and assessments tailored to their individual needs and learning style.
Providing real-time feedback: The virtual tutor offers immediate feedback and guidance to students, helping them identify areas of strength and weakness and providing targeted support.
Automating grading and scoring: The AI system can automatically grade assignments and assessments, freeing up teachers' time and providing consistent and timely feedback to students.
Predicting future performance: By analyzing student data over time, the AI can predict each student's future academic performance and identify potential areas of concern, allowing teachers to intervene early and provide additional support.
Impact and Benefits
The implementation of Riiid Labs' AI-powered virtual tutoring system has resulted in several key benefits for both students and teachers:
Personalized learning experiences: Students receive tailored instruction and support based on their individual needs, leading to improved engagement and learning outcomes.
Increased efficiency: Teachers can focus on providing high-quality instruction and mentorship while the AI system handles routine tasks such as grading and performance tracking.
Early intervention: By predicting potential academic challenges, teachers can intervene early and provide targeted support to students, preventing them from falling behind.
Scalability: The AI-powered system can provide personalized learning experiences to a large number of students simultaneously, making it a scalable solution for educational institutions.
Continuous improvement: The AI system learns and adapts over time, continuously improving its ability to provide personalized learning experiences and predict student performance.
Riiid Labs' success in developing AI-powered virtual tutoring systems demonstrates the transformative potential of AI in enhancing teaching and learning experiences, leading to improved student outcomes and increased efficiency for educators.
Conclusion
We've journeyed across a landscape where AI's influence is profound and pervasive. From diagnosing diseases with uncanny accuracy to managing global supply chains, and from personalizing learning experiences to revolutionizing customer interactions, AI’s capabilities are vast and varied.
These success stories and case studies not only illuminate AI's potential to enhance business outcomes but also highlight its role in driving competitive advantages in a rapidly evolving world.
As businesses and sectors continue to harness AI's power, the pathway to innovation seems increasingly intertwined with intelligent technology.
The future, rich with advancements in machine learning, natural language processing, and more, promises even more exciting prospects. For any industry looking to remain competitive and innovative, embracing AI is not just an option but a necessity.
Further Reading and Resources
For those intrigued by AI’s expansive role across various sectors and looking to dive deeper into its capabilities and real-world applications, numerous resources are available.
Books like "AI Superpowers" by Kai-Fu Lee offer insights into how AI is shaping global industries. Journals like "Artificial Intelligence" and online platforms such as Google Scholar provide access to the latest research and case studies.
Microsoft AI Customer Stories:
Microsoft AI showcases various customer stories where AI technologies are being applied across different sectors.
Examples include Zammo's conversational AI platform, CarMax's customer review summaries, Broward College's student support strategies, and IPLAND's retail display optimization using image recognition technology.
Amazon AWS Case Studies:
Amazon AWS presents customer success stories, including BMW Group's utilization of AWS technology to power over 1,000 microservices processing billions of requests daily with high reliability.
The platform offers a range of case studies demonstrating how organizations accelerate innovation, increase agility, and lower costs through AWS cloud services.
Whatfix Case Studies:
Whatfix showcases case studies on how organizations drive technology adoption for customers and employees through contextual in-app guidance and self-help support
These case studies cover a variety of industries and applications, providing insights into how AI adoption is enhancing user experiences and driving technology utilization.
By exploring these sources, you can gain a comprehensive understanding of how AI adoption is shaping industries and driving innovation across various sectors.
AI, a combination of machine learning, data analytics, and automation, is not just a futuristic concept but a present-day powerhouse driving innovation across various sectors.
From healthcare to finance, retail to manufacturing, and beyond, AI is at the forefront of revolutionizing how businesses operate, enhancing efficiency, and crafting personalized user experiences
The importance of AI adoption cannot be overstated. As we delve into this topic, we aim to shed light on the myriad ways AI technologies are being integrated into different industries, not just to streamline operations but to solve complex problems and create opportunities that were unimaginable just a few decades ago.
Our journey through this landscape will highlight significant success stories and insightful case studies that demonstrate the profound impact of AI.
Healthcare Industry
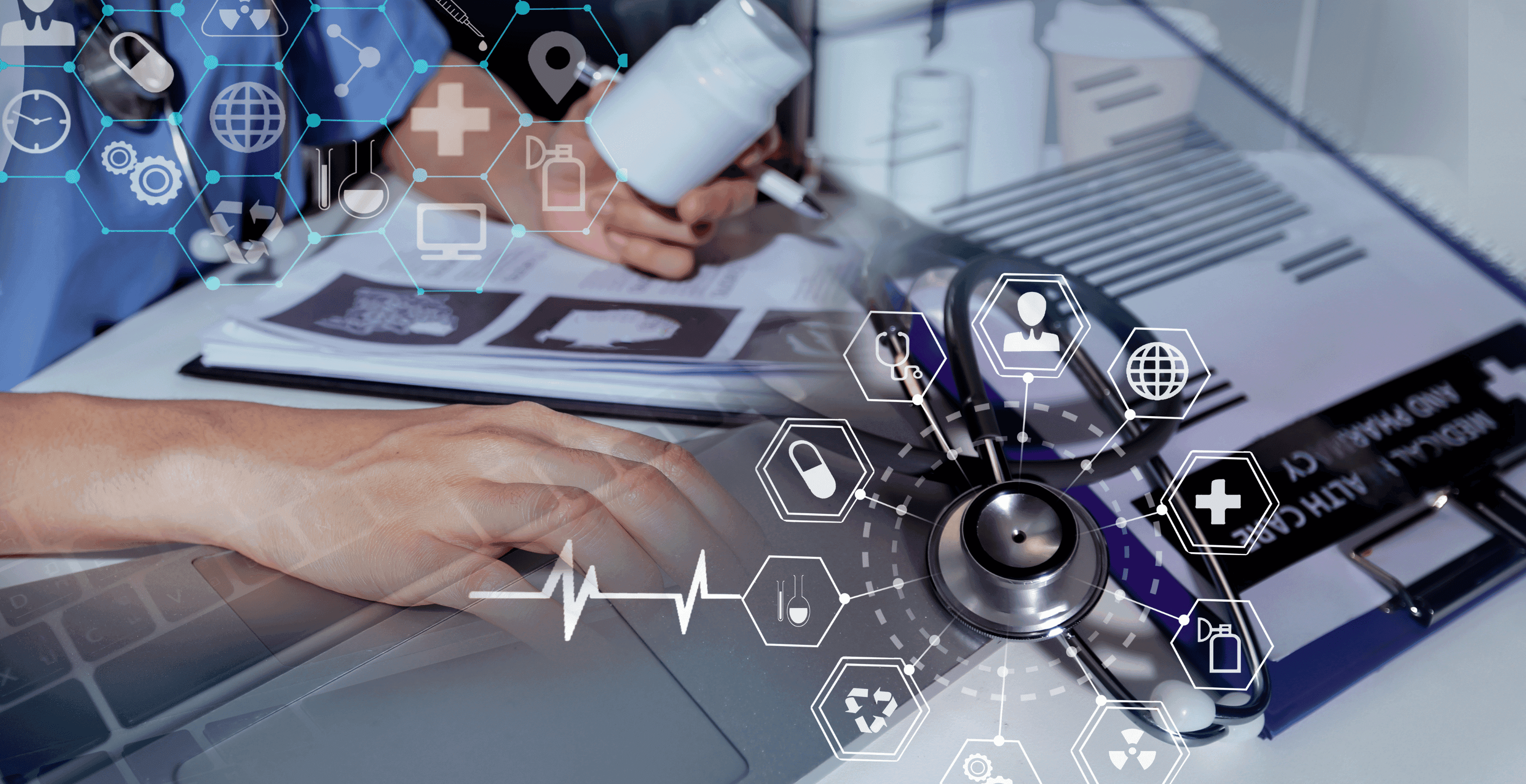
AI-Assisted Drug Discovery and Development
One notable example of AI's impact on drug discovery is the work of Baidu Research, which developed deep learning algorithms that outperform humans in identifying breast cancer metastasis.
These algorithms demonstrate AI's ability to analyze complex datasets, including genomic information, patient records, and drug compound libraries, to uncover insights that may not be immediately apparent to human researchers.
AI has significantly improved the efficiency of drug discovery at companies like Baidu Research in several key ways:
Accelerating lead compound discovery: AI-powered virtual screening tools can rapidly evaluate millions of chemical compounds to identify those with the highest potential for therapeutic activity, speeding up the lead compound discovery process.
Optimizing mRNA vaccine design: Baidu Research developed an AI algorithm called LinearDesign that can rapidly design highly stable COVID-19 mRNA vaccine sequences, achieving a 128-fold increase in antibody response compared to previous methods. This breakthrough can be applied to a wider range of therapeutic proteins.
Predicting drug properties: Baidu Research has developed novel machine learning-based computational biology algorithms for predicting drug properties, binding affinity, and designing new drug candidates, aiming to provide an AI-enhanced tool for facilitating drug discovery.
Leveraging knowledge graphs: Baidu's AI technologies, such as knowledge graphs, are being used to improve drug target discovery and validation, as well as small-molecule design. Knowledge graphs help connect disparate data sources to uncover new insights.
Optimizing workflows: By integrating AI into various stages of the drug discovery pipeline, including target identification, lead optimization, and preclinical testing, Baidu Research is able to streamline workflows and make the overall process more efficient.
These AI-driven advancements have the potential to significantly reduce the time and cost associated with drug development, which is typically a lengthy and expensive process. Baidu Research's work demonstrates the transformative impact AI can have on accelerating drug discovery and development.
Despite the promising results, it's important to note that AI is not a panacea for drug discovery challenges. Careful validation and integration with human expertise are crucial to ensure the reliability and safety of AI-generated insights.
Ongoing research and collaboration between AI experts and pharmaceutical researchers will be key to fully realizing the potential of AI in accelerating drug discovery and development.
AI for Fraud Detection and Risk Management
One notable success story in AI for fraud detection and risk management is the case of JPMorgan Chase. The bank implemented AI algorithms to enhance fraud detection capabilities, resulting in a significant reduction in false positives and improved detection accuracy.
By leveraging machine learning models, JPMorgan Chase was able to analyze vast amounts of transaction data in real-time, identifying suspicious patterns and anomalies that could indicate fraudulent activities.
JPMorgan Chase’s Use of AI:
Data Analysis: JPMorgan Chase's AI system processes large volumes of transaction data, looking for patterns indicative of fraudulent behavior.
Machine Learning Models: The bank uses sophisticated machine learning algorithms to detect anomalies and predict potential fraud.
Real-time Monitoring: The AI system operates in real-time, allowing for immediate detection and response to suspicious activities.
Continuous Learning: The system continuously learns from new data and feedback, improving its accuracy and staying ahead of evolving fraud tactics.
Reduced False Positives: By leveraging AI, JPMorgan Chase has significantly reduced false positives, minimizing disruptions to legitimate transactions.
Enhanced Detection Accuracy: The AI algorithms have improved the bank's ability to detect fraudulent activities early, preventing financial losses and protecting customers.
Impact:
Improved Fraud Prevention: JPMorgan Chase's AI implementation has strengthened its fraud prevention capabilities, safeguarding customer assets and financial transactions.
Cost Savings: By reducing false positives and improving detection accuracy, the bank has saved resources previously spent on investigating false alarms.
Enhanced Customer Trust: The proactive fraud detection enabled by AI has enhanced customer trust and confidence in the bank's security measures.
Industry Leadership: JPMorgan Chase's success in AI-driven fraud detection has positioned it as a leader in leveraging technology for risk management in the banking sector.
The success of JPMorgan Chase's AI implementation showcases the power of AI in combating financial fraud, enhancing risk management practices, and protecting both the institution and its customers from fraudulent activities.
Retail and E-commerce
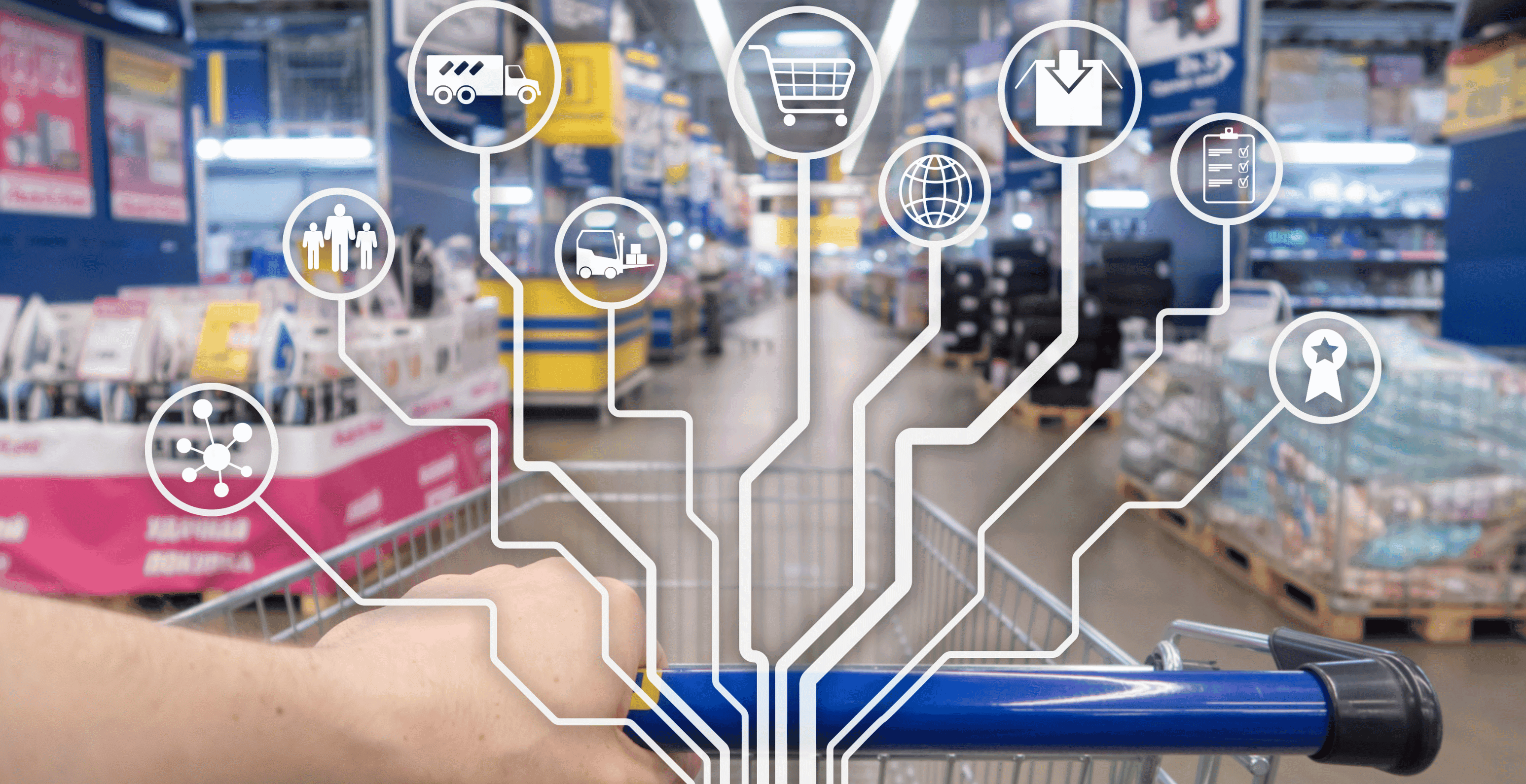
AI for Product Recommendation and Personalization
Amazon, the e-commerce giant, has been at the forefront of leveraging AI for product recommendation and personalization.
By analyzing customer browsing history, purchase patterns, and preferences, Amazon's AI algorithms generate highly accurate and personalized product recommendations123. This has significantly boosted customer engagement, increased sales, and improved the overall shopping experience.
How Amazon's AI-Powered Recommendations Work?
Amazon's recommendation system collects and analyzes vast amounts of customer data, including:
Browsing history: The products a customer has viewed or searched for
Purchase history: The products a customer has bought in the past
Ratings and reviews: The customer's ratings and reviews of products
Demographic data: The customer's age, gender, location, and other relevant information
Using this data, Amazon's AI algorithms identify patterns and correlations to predict what a customer might be interested in.
The system also takes into account the behavior of similar customers to provide even more personalized recommendations1.
Benefits of AI-Powered Recommendations
By implementing AI-driven product recommendations, Amazon has achieved several key benefits:
Increased sales: Personalized recommendations have been shown to drive a significant increase in sales, as customers are more likely to purchase products that are tailored to their preferences2.
Enhanced customer engagement: Relevant and engaging product recommendations keep customers on the site for longer, leading to increased browsing and exploration of Amazon's vast product catalog1.
Improved customer satisfaction: Customers appreciate the convenience and relevance of personalized recommendations, leading to higher satisfaction levels and increased loyalty to the Amazon brand3.
Competitive advantage: Amazon's advanced AI capabilities in product recommendation and personalization have given it a significant edge over competitors in the e-commerce space2.
Future Developments
As AI technology continues to advance, Amazon is likely to further refine and enhance its product recommendation system. Potential future developments may include:
Incorporating real-time data: Using AI to analyze customer behavior and preferences in real-time, allowing for even more dynamic and responsive recommendations4.
Expanding to new channels: Applying AI-powered recommendations to other channels, such as mobile apps and voice assistants, to provide a seamless and consistent experience across all touchpoints5.
Leveraging new data sources: Integrating additional data sources, such as social media activity and external market trends, to further improve the accuracy and relevance of recommendations3.
By continuously investing in and improving its AI capabilities, Amazon is well-positioned to maintain its leadership in the e-commerce industry and deliver exceptional customer experiences through personalized product recommendations.
Manufacturing and Industrial Automation
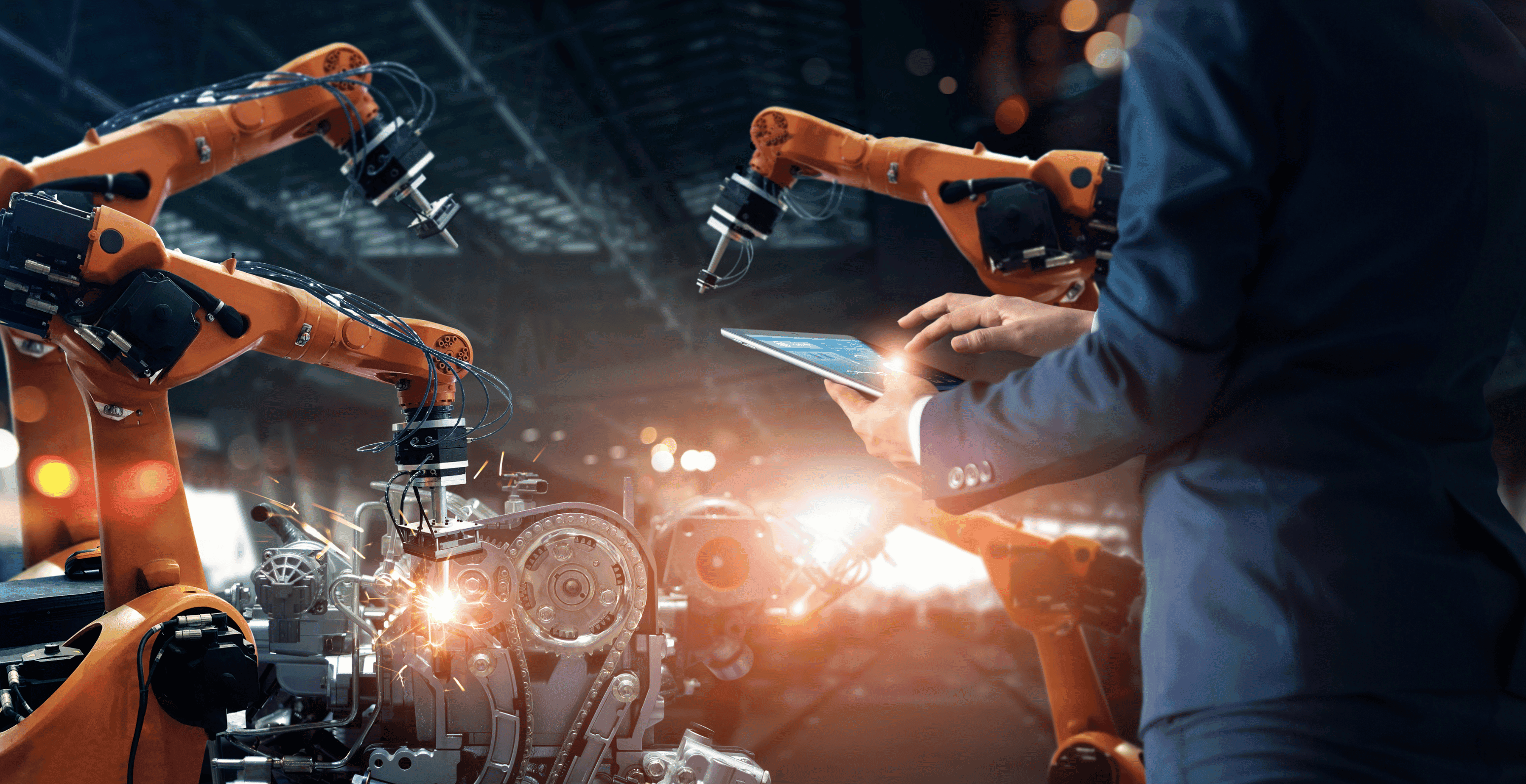
AI for Predictive Maintenance and Quality Control
A notable example of AI for predictive maintenance and quality control is the implementation by Siemens. The company leverages AI and machine learning to maximize productivity in the industrial and manufacturing sectors.
By exploring the capabilities of AI and machine learning, Siemens has developed technologies that reduce machinery costs, improve consistency with automation, and deliver instant data for quickly adjusting workflows 2.
Siemens' Approach to AI in Predictive Maintenance and Quality Control
Siemens utilizes AI and machine learning in the following ways to enhance predictive maintenance and quality control:
Predictive Maintenance: By analyzing real-time data from sensors embedded in machinery, Siemens' AI algorithms can predict potential equipment failures before they occur. This proactive approach allows for timely maintenance, reducing downtime and minimizing repair costs.
Quality Control: AI-driven quality control systems analyze production data to predict potential quality issues before they occur. By identifying anomalies and deviations in the manufacturing process, Siemens can take corrective actions to maintain product quality standards.
Automation and Consistency: AI technologies enable automation of manual processes, ensuring consistency in manufacturing operations. By leveraging machine learning algorithms, Siemens can optimize workflows, reduce errors, and improve operational efficiency.
Data-Driven Decision-Making: Siemens' AI systems provide instant data insights, allowing for quick adjustments in production processes. Real-time analytics enable agile decision-making, enhancing overall productivity and responsiveness to changing demands.
Impact and Benefits
The implementation of AI for predictive maintenance and quality control at Siemens has resulted in several key benefits:
Maximized Productivity: By leveraging AI technologies, Siemens has optimized machinery performance, reduced downtime, and maximized productivity in manufacturing operations.
Cost Reduction: Predictive maintenance has helped Siemens reduce machinery costs by proactively addressing maintenance needs and avoiding costly breakdowns.
Improved Quality: AI-driven quality control systems have enhanced product quality by identifying and addressing potential issues early in the manufacturing process.
Enhanced Automation: Automation through AI has improved consistency, reduced errors, and streamlined workflows, leading to increased operational efficiency.
Agile Decision-Making: Real-time data insights provided by AI systems enable Siemens to make quick and informed decisions, ensuring responsiveness to market demands and operational changes.
Siemens' successful integration of AI for predictive maintenance and quality control showcases the transformative potential of AI in optimizing industrial processes, reducing costs, and enhancing overall operational efficiency in the manufacturing sector.
Marketing and Advertising
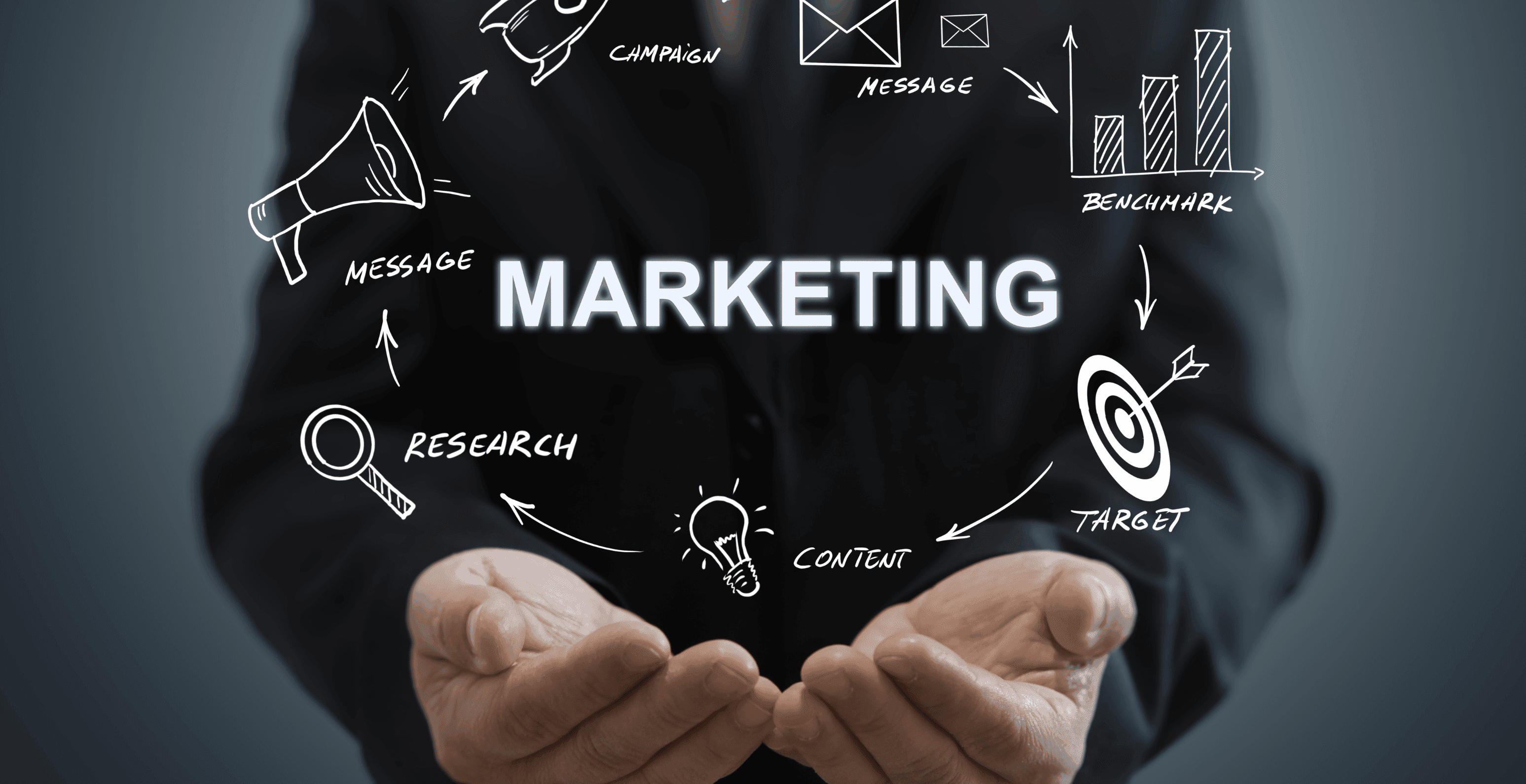
AI for Targeted Advertising and Campaign Optimization
Vanguard, one of the world's largest investment firms, utilized AI for targeted advertising and campaign optimization by partnering with the AI language platform Persado to conduct highly personalized advertising on LinkedIn, the only platform where they could run ads due to regulations.
Leveraging AI technology, Vanguard hyper-personalized its ads and tested them at scale to determine the most effective approaches that resonated with consumers. This strategic use of AI resulted in a significant 15% increase in conversion rates for Vanguard's advertising campaigns4.
Vanguard's Approach to AI-Powered Advertising:
Hyper-Personalization: Vanguard employed AI to create highly personalized ads tailored to individual consumers based on their preferences and behaviors.
Scale Testing: AI allowed Vanguard to test multiple ad variations at scale to identify the most effective messaging and creative elements.
Regulatory Compliance: Due to regulatory restrictions, Vanguard focused its AI-powered advertising efforts on LinkedIn, optimizing its campaigns for this specific platform.
Conversion Rate Optimization: By leveraging AI insights, Vanguard was able to optimize its advertising campaigns to drive a 15% increase in conversion rates.
Impact and Benefits:
Increased Conversions: The use of AI for targeted advertising led to a substantial 15% increase in conversion rates for Vanguard's campaigns.
Personalized Engagement: AI-driven hyper-personalization enhanced customer engagement by delivering tailored messages that resonated with individual preferences.
Efficiency and Effectiveness: AI technology enabled Vanguard to optimize its advertising efforts, ensuring that resources were allocated to the most impactful campaigns.
Regulatory Compliance: By focusing on LinkedIn for AI-powered advertising, Vanguard navigated regulatory constraints while still achieving significant performance improvements.
Future Applications:
Further Personalization: Vanguard can continue to refine its AI-powered advertising strategies to deliver even more personalized and targeted campaigns.
Multi-Platform Optimization: Expanding AI-driven advertising efforts to other platforms while maintaining regulatory compliance to reach a broader audience.
Continuous Testing and Optimization: Vanguard can leverage AI for ongoing testing and optimization of ad campaigns to adapt to changing consumer behaviors and preferences.
Vanguard's success in leveraging AI for targeted advertising and campaign optimization exemplifies the transformative impact of AI in enhancing marketing strategies, driving conversions, and delivering personalized experiences to consumers.
Education and Learning
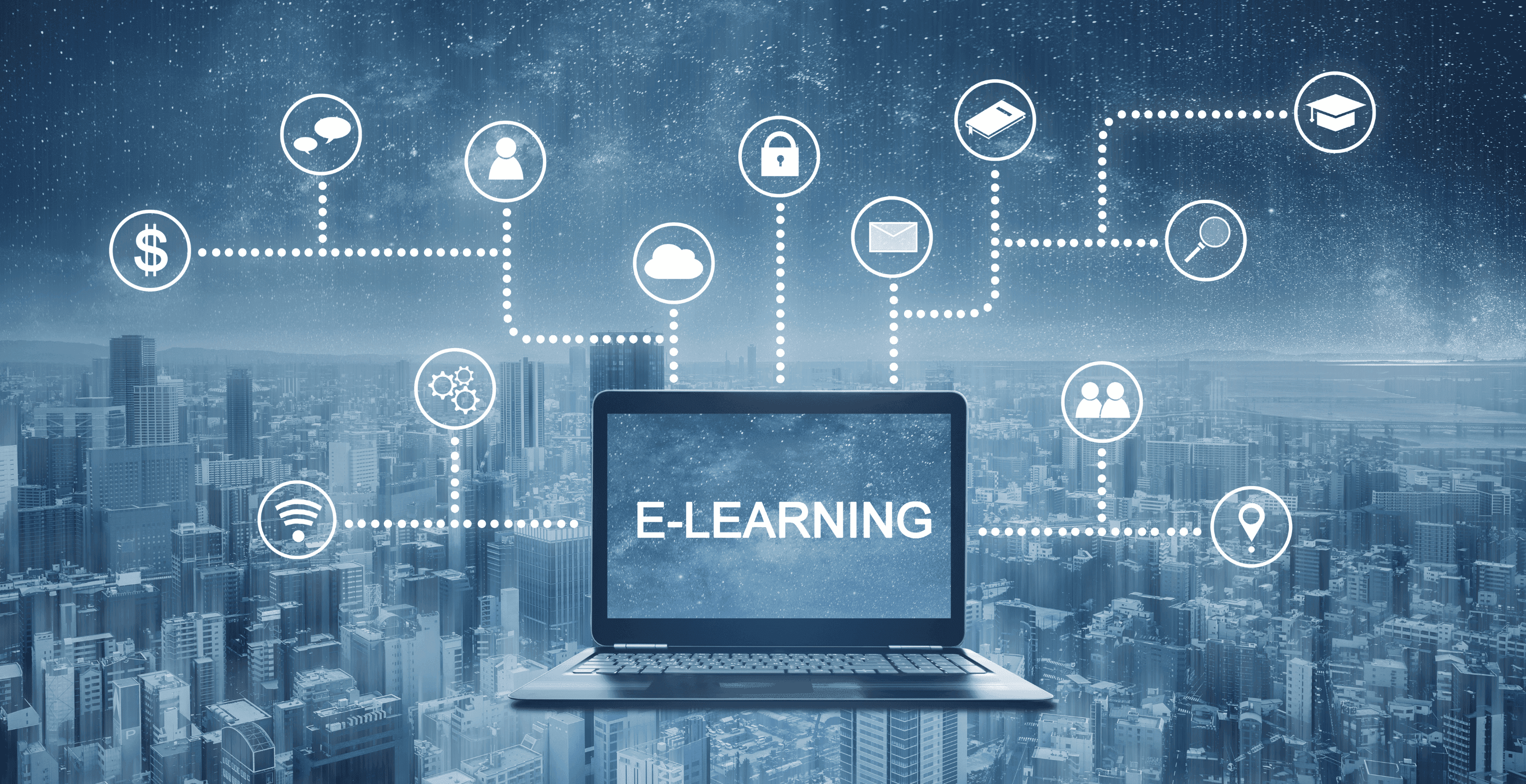
AI-Powered Virtual Tutors and Teaching Assistants
A notable example of AI-powered virtual tutors and teaching assistants is the work of Riiid Labs. This subsidiary of Riiid develops AI-based learning and assessment technologies that can be integrated into existing applications or used as standalone platforms13.
By leveraging machine learning capabilities, Riiid Labs' technology assists teachers in tracking student performance, automating assignment scoring, and personalizing curriculum needs for each student.
Riiid Labs' AI-Powered Virtual Tutoring System
Riiid Labs' AI-powered virtual tutoring system utilizes advanced machine learning algorithms to provide personalized learning experiences for students. The system works by:
Collecting and analyzing student data: The platform gathers information on each student's performance, learning patterns, and progress to create a personalized profile.
Adapting learning content: Based on the student's profile, the AI system selects and presents the most appropriate learning materials, exercises, and assessments tailored to their individual needs and learning style.
Providing real-time feedback: The virtual tutor offers immediate feedback and guidance to students, helping them identify areas of strength and weakness and providing targeted support.
Automating grading and scoring: The AI system can automatically grade assignments and assessments, freeing up teachers' time and providing consistent and timely feedback to students.
Predicting future performance: By analyzing student data over time, the AI can predict each student's future academic performance and identify potential areas of concern, allowing teachers to intervene early and provide additional support.
Impact and Benefits
The implementation of Riiid Labs' AI-powered virtual tutoring system has resulted in several key benefits for both students and teachers:
Personalized learning experiences: Students receive tailored instruction and support based on their individual needs, leading to improved engagement and learning outcomes.
Increased efficiency: Teachers can focus on providing high-quality instruction and mentorship while the AI system handles routine tasks such as grading and performance tracking.
Early intervention: By predicting potential academic challenges, teachers can intervene early and provide targeted support to students, preventing them from falling behind.
Scalability: The AI-powered system can provide personalized learning experiences to a large number of students simultaneously, making it a scalable solution for educational institutions.
Continuous improvement: The AI system learns and adapts over time, continuously improving its ability to provide personalized learning experiences and predict student performance.
Riiid Labs' success in developing AI-powered virtual tutoring systems demonstrates the transformative potential of AI in enhancing teaching and learning experiences, leading to improved student outcomes and increased efficiency for educators.
Conclusion
We've journeyed across a landscape where AI's influence is profound and pervasive. From diagnosing diseases with uncanny accuracy to managing global supply chains, and from personalizing learning experiences to revolutionizing customer interactions, AI’s capabilities are vast and varied.
These success stories and case studies not only illuminate AI's potential to enhance business outcomes but also highlight its role in driving competitive advantages in a rapidly evolving world.
As businesses and sectors continue to harness AI's power, the pathway to innovation seems increasingly intertwined with intelligent technology.
The future, rich with advancements in machine learning, natural language processing, and more, promises even more exciting prospects. For any industry looking to remain competitive and innovative, embracing AI is not just an option but a necessity.
Further Reading and Resources
For those intrigued by AI’s expansive role across various sectors and looking to dive deeper into its capabilities and real-world applications, numerous resources are available.
Books like "AI Superpowers" by Kai-Fu Lee offer insights into how AI is shaping global industries. Journals like "Artificial Intelligence" and online platforms such as Google Scholar provide access to the latest research and case studies.
Microsoft AI Customer Stories:
Microsoft AI showcases various customer stories where AI technologies are being applied across different sectors.
Examples include Zammo's conversational AI platform, CarMax's customer review summaries, Broward College's student support strategies, and IPLAND's retail display optimization using image recognition technology.
Amazon AWS Case Studies:
Amazon AWS presents customer success stories, including BMW Group's utilization of AWS technology to power over 1,000 microservices processing billions of requests daily with high reliability.
The platform offers a range of case studies demonstrating how organizations accelerate innovation, increase agility, and lower costs through AWS cloud services.
Whatfix Case Studies:
Whatfix showcases case studies on how organizations drive technology adoption for customers and employees through contextual in-app guidance and self-help support
These case studies cover a variety of industries and applications, providing insights into how AI adoption is enhancing user experiences and driving technology utilization.
By exploring these sources, you can gain a comprehensive understanding of how AI adoption is shaping industries and driving innovation across various sectors.