Best Practices In Data Governance For AI
Best Practices In Data Governance For AI
Best Practices In Data Governance For AI
Rehan Asif
Jun 10, 2024
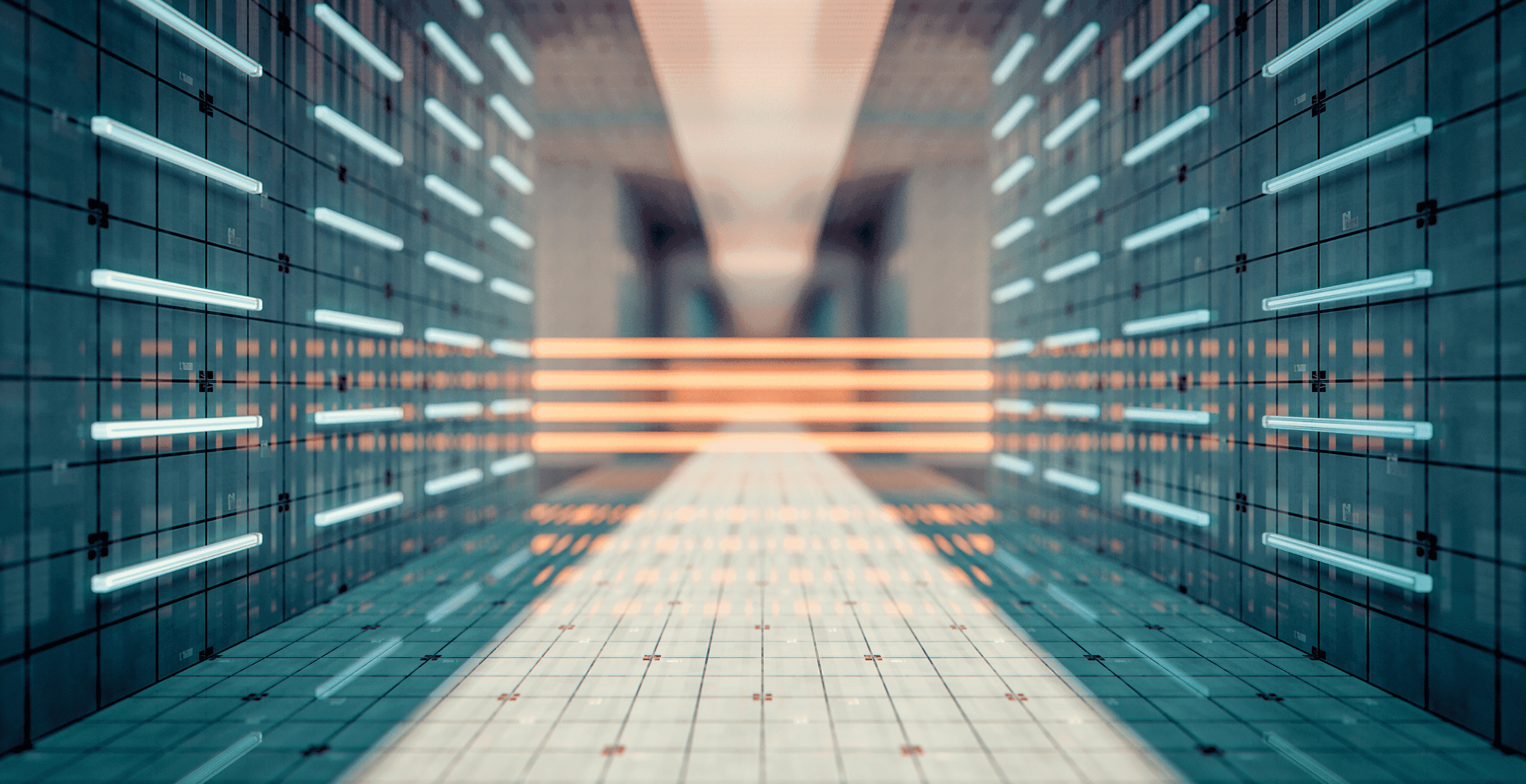
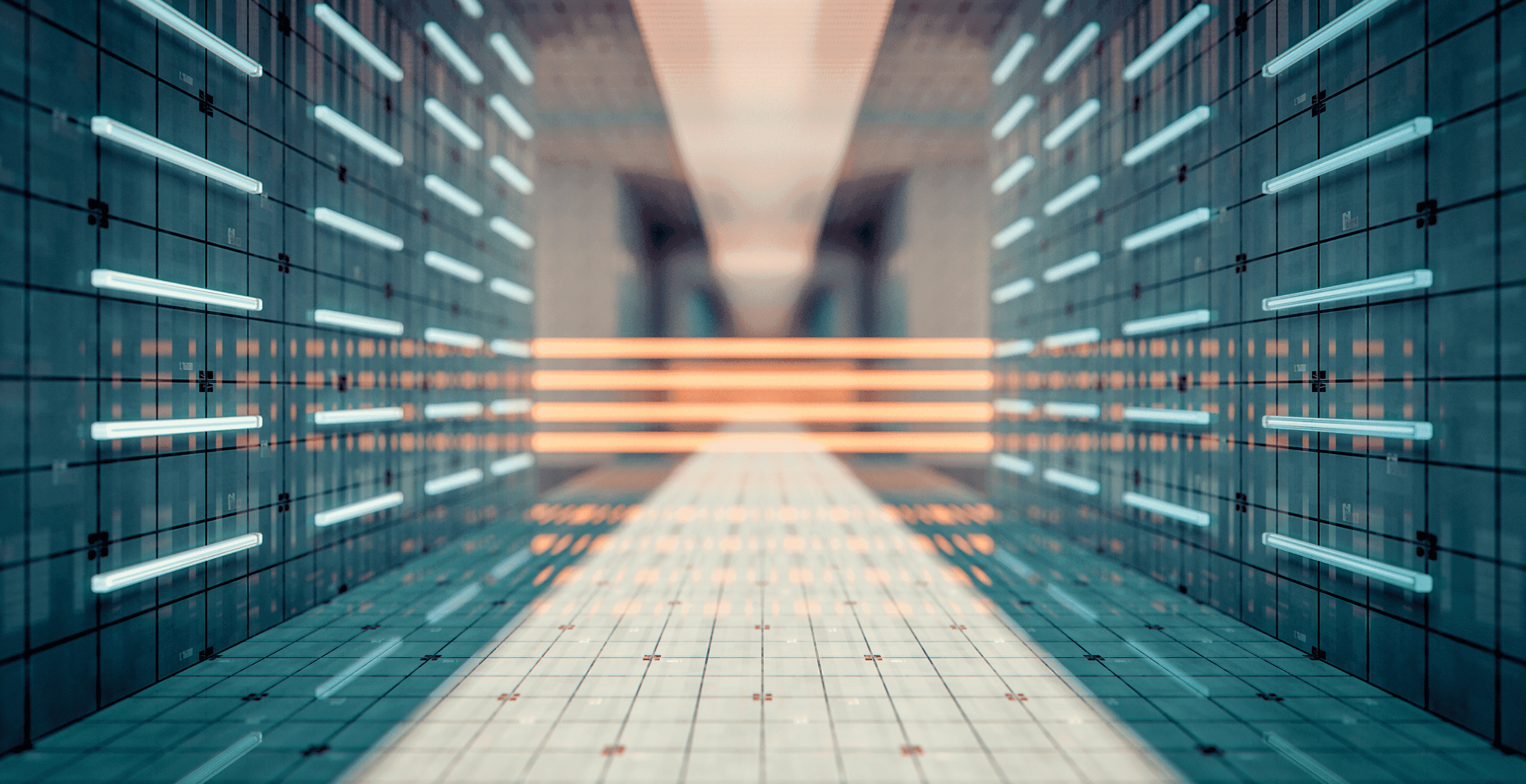
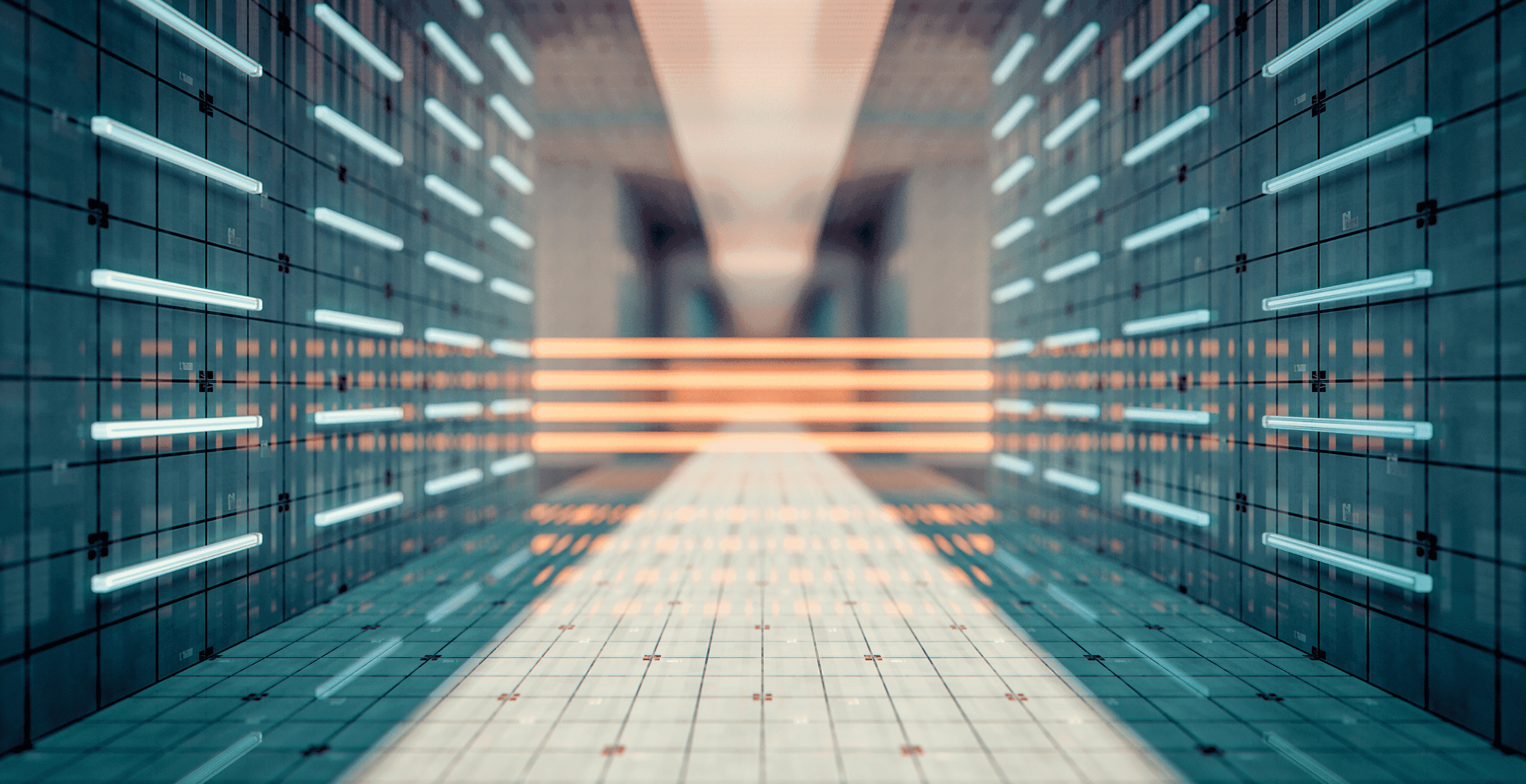
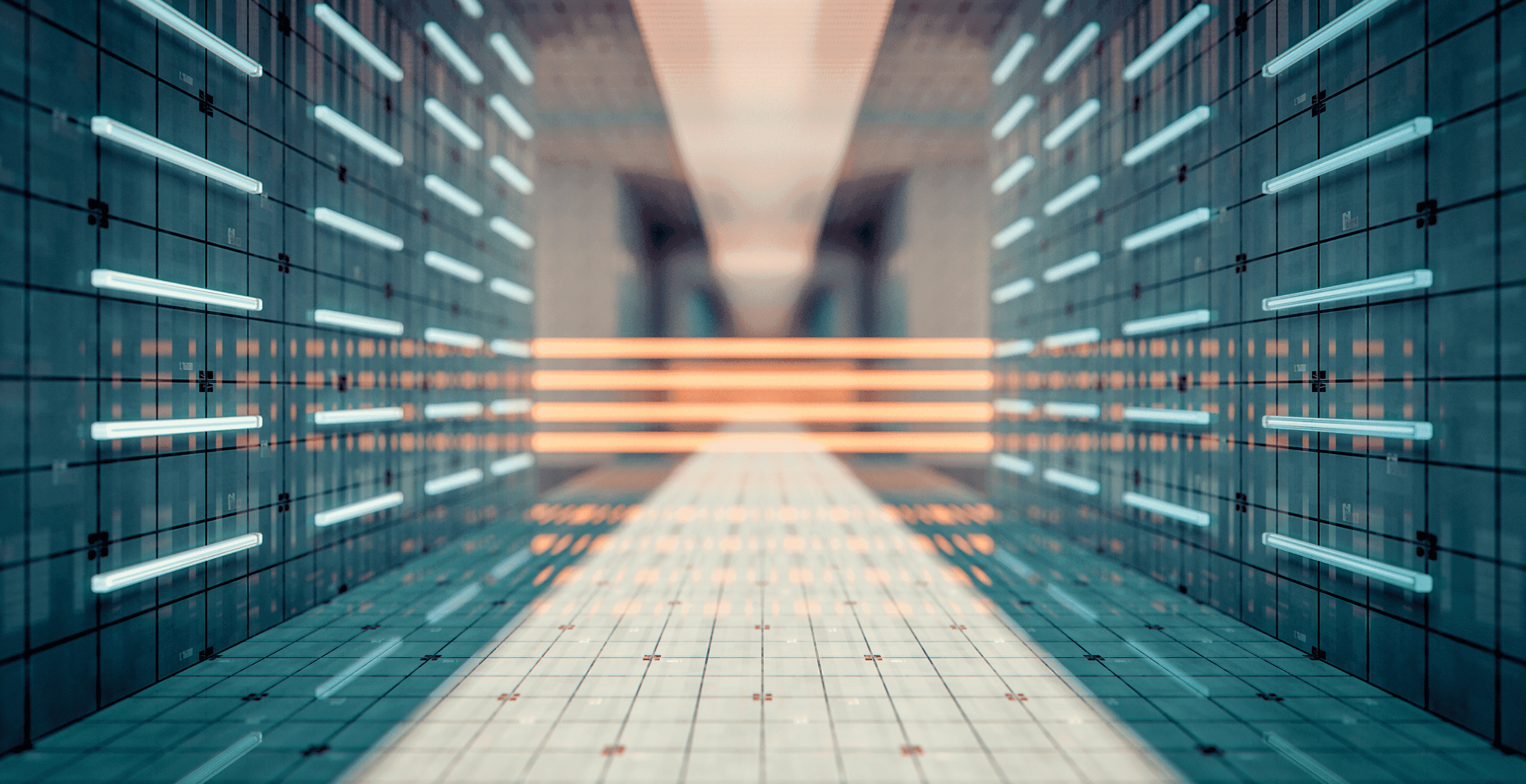
Entering the age of AI without a solid data governance plan is like skydiving without a parachute – thrilling but perilous.
In the swiftly progressing field of Artificial Intelligence (AI), Governance and data handling play an important role. Efficient data governance ensures not only the precision and security of the data utilized for Artificial Intelligence but also its ethical sourcing and compliance with appropriate laws.
This guide explores the best practices in data governance for AI, providing a strategy for associations looking to utilize the power of AI responsibly and efficiently.
Introduction
Importance of Data Governance in AI
Data Governance is critical in AI because it ensures the incorporation, quality, and security of data in Artificial Intelligence systems. Efficient data governance assists in maintaining the precision and reliability of Artificial Intelligence outputs, significant for making informed choices and achieving desired results.
With the expanding dependency on Artificial Intelligence, Robust data governance structures protect against data misuse and ensure compliance with executive standards.
Challenges Of Data Management For AI Systems
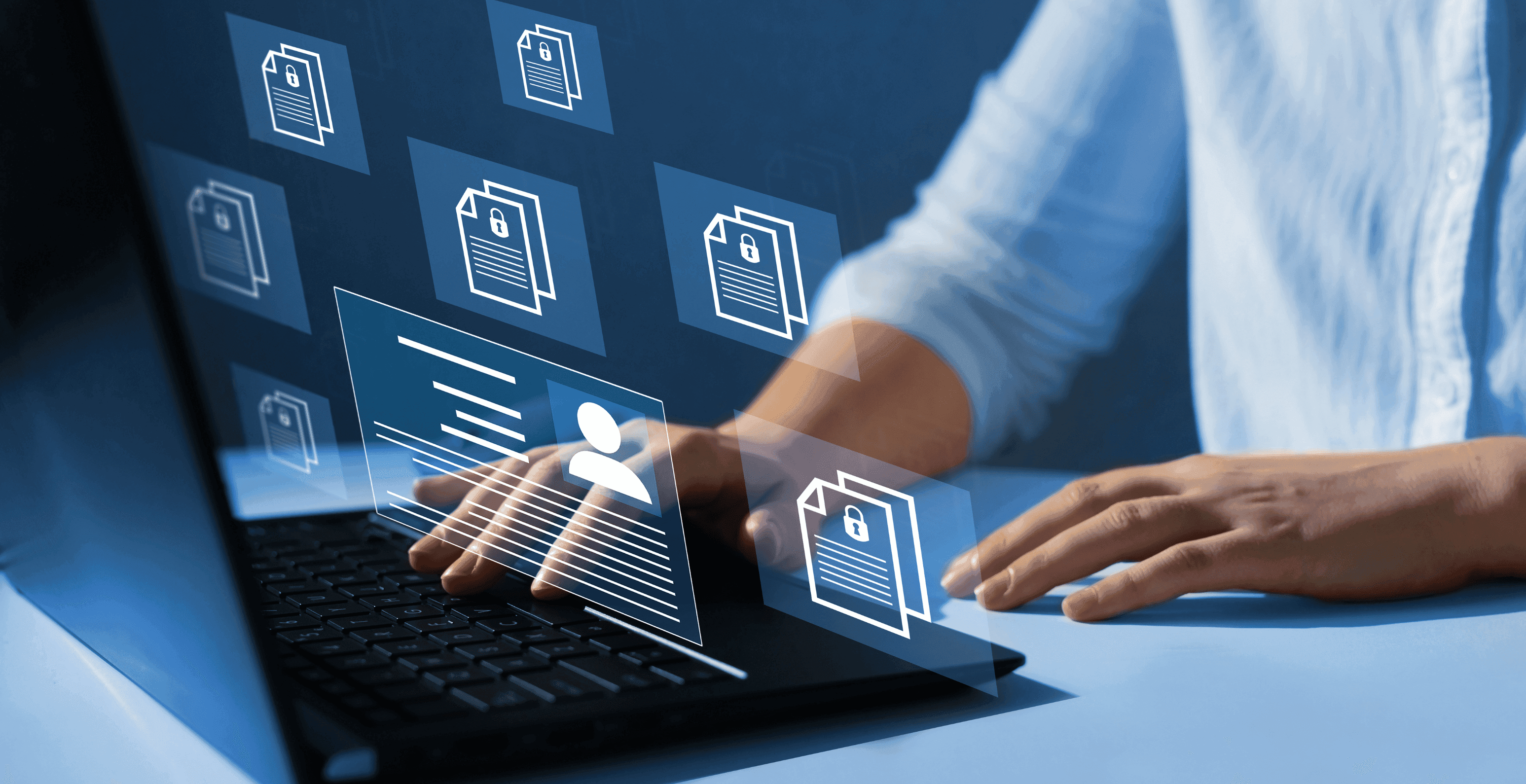
Data Management for Artificial Intelligence systems pose numerous challenges such as:
Data Quality and Consistency: Ensuring that data is precise, complete, and compatible is significant for training dependable Artificial Intelligence models.
Data Privacy and Security: Safeguarding sensitive data and maintaining assent with privacy laws such as GDPR and CCPA is crucial.
Data Integration and Silos: Incorporating data from numerous sources and breaking down data silos can be complicated but important for thorough analysis.
Ethical Considerations: Acknowledging tendencies in data and ensuring ethical utilization of Artificial Intelligence is crucial to avoid bigotry and unfair practices.
Scalability: Handling large dataset effectively and scaling systems to encompass evolving data requirements is significant for nourished AI performance.
Overview Of Data Governance Frameworks
Data governance structures offer structures approaches for handling data efficiently. These structures usually indulge:
Policies and Standards: Endowing recommendations for usage of data, security and privacy.
Roles and Responsibilities: Defining roles such as data housekeepers, data proprietors, and data caretakers to ensure liability.
Data Quality Management: Enforcing processes to monitor and enhance the quality of data.
Compliance and Audit: Ensuring compliance to legal and regulatory needs and imparting regular audits.
Data Lifecycle Management: Handling data from its formation to its removal, ensuring appropriate management at each stage.
Technology and Tools:- Using advanced technologies and tools for data handling, incorporation and dissecting.
Establishing A Data Governance Framework
Defining Organizational Data Strategy And Objectives
To begin, it’s important to clearly define your administrative data plans and purposes. This involves understanding the specific goals your institution aims to achieve through data governance.
Whether it's enhancing the quality of data, ensuring assent with regulations, or using data for strategic decision-making, having an explicit plan to provide a plan for all data-related activities.
Set Clear Goals: Decide what you want to accomplish with your data governance efforts. This could range from enhancing data precision to improving data security or upgrading data utilization for enhanced venture insights.
Align with Business Objectives: Ensure that your data plans correspond with your overall venture aims. This layout helps in giving priority to data capabilities that offer most value to an organization.
Develop a Data Management Plan: Summarize the processes and practices for handling data throughout its lifetime. This indulges data gathering, storage, usage and positioning.
Identifying Stakeholders and Roles
Successful data governance requires participation from various stakeholders across the association. Determining these stakeholders and clearly defining their roles is critical for the structure’s efficiency.
Identify Key Stakeholders: These usually include managers, data owners, data stewards, Information Technology staff and end-users. Each group has a suited interest in how data is handled and used.
Define Roles and Responsibilities: Evidently summarize the roles and responsibilities for each investor group. For instance, data stewards might be liable for handling data quality, while IT staff may manage data security.
Establish A Data Governance Council: Shape a governance council or committee that indulges deputies from key stakeholders groups. This council supervises the execution and administration of data governance policies.
Creating Data Governance Policies and Standards
Creating rigorous data governance policies and standards ensures that data is consistently and efficiently supervised across the association.
Develop Thorough Policies: These should cover numerous phases of data management, indulging the quality of data, data security, data seclusion and data lifespan handling. Policies should be transparent, applicable and executable.
Set Data Standards: Pioneer standards for data formats, naming customs, metadata handling, and data incorporation. Compatible standards help in maintaining the quality of data and conformity.
Implement Data Compliance Measures: Ensure that your strategies adhere with appropriate laws and standards, like GDPR or CCPA. Frequently check and update these strategies to stay compliant.
By adhering to these steps, your firm can pioneer a solid data governance structure that not only improves data management practices but also supports accomplishing strategic venture goals.
Data Quality Management
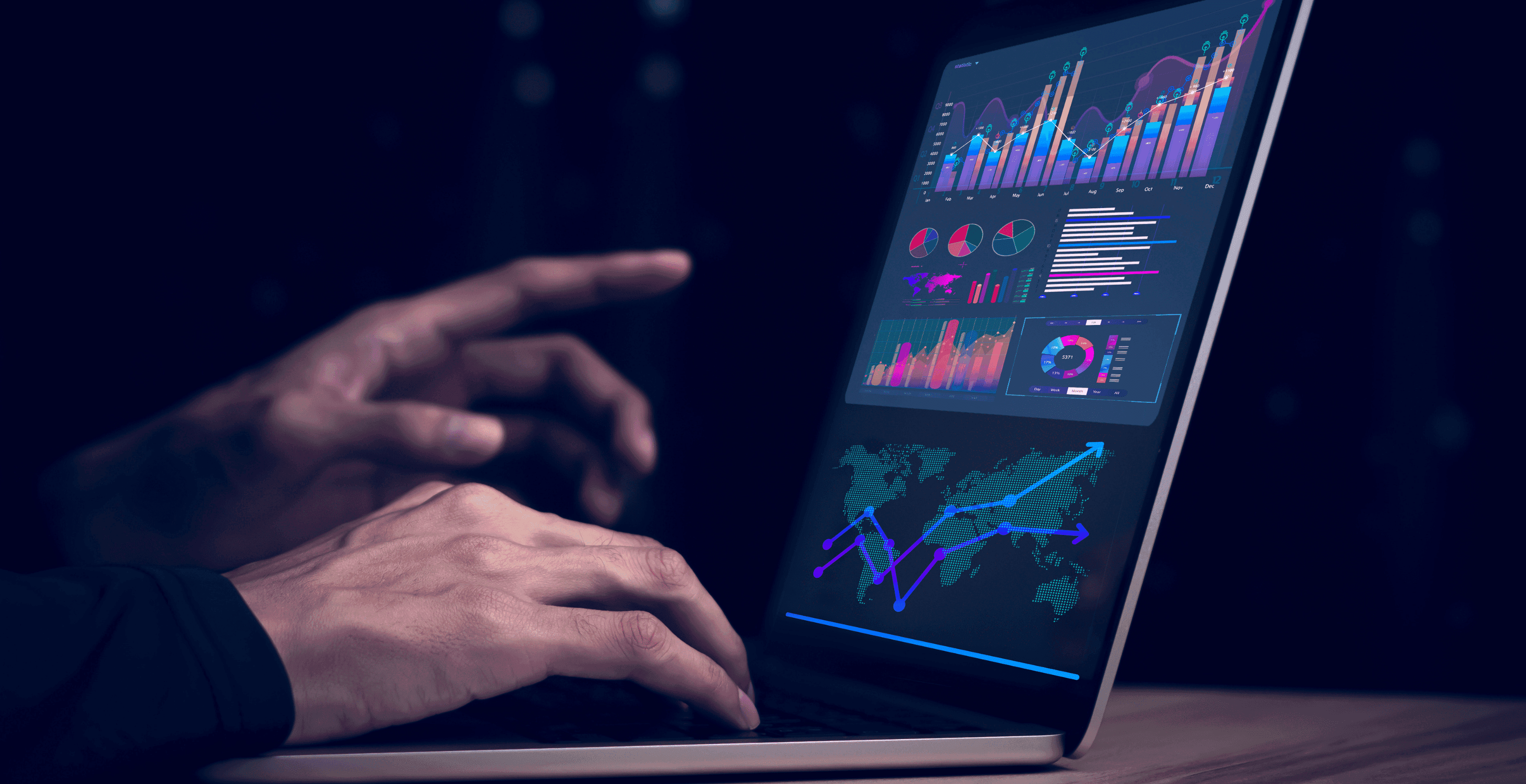
When managing data quality, organizations aim to ensure that their data is accurate, reliable, and useful for decision-making. Here’s an outline of chief aspects indulged in data-quality management:
Data Quality Dimensions
Accuracy: Refers to the accuracy of data after comparison with responsible sources or references. Accurate data appropriately imitates the real-world values it is aiming to represent.
Completeness: Covers to the limit to which all needed data is attainable. Incomplete data can result in examination that is possibly partial or misleading.
Consistency: Ensures that the information across varied datasets or information are compatible without any strife or modifications. Consistency is important for data to be dependable and trustworthy.
Data Profiling and Quality Assessment
Data Profiling: This is the initial step in comprehending the existing form of data. It indulges in investigating the data attainable and gathering statistics and information about that data. The process usually identifies problems with preciseness, distinctiveness, correspondence and entirety.
Quality Assessment: Continues from profiling, indulging more profound inspection to comprehend the root causes of data problems and to identify their effects on appropriate ventures processes. This indulges in setting standards for quality quality, estimating data against these criterias, and reporting the standing of data quality.
Data Cleansing And Transformation Processes
Data Cleansing:
Error Correction: Fixing mistakes determined in the data, like typos, spelling errors, or incorrect data entries.
Normalization: Normalizing the data structures, labels and data types to ensure correspondence across the dataset.
Missing Data Handling: Filling missing values through methods such as attribution or deciding to ignore or drop them based on the impact evaluation.
Data Transformation:
Data Integration: Merging data from various sources to give a unified view. This often indulges resolving incompatibilities and supporting the entirety of data.
Data Conversion: Transforming data from one format to another, usually to incorporate with other systems or to correspond with the needs of a new system.
Data Enrichment: Accelerating existing data with data from added sources to improve the data’s information or entirety.
Efficient data quality management ensures that data is a precious asset for decision-making, docility, and working procedures.
It needs constant efforts and incorporation into the daily data management processes to handle the quality of data throughout its lifespan.
Data Lineage and Traceability
Tracking Data Sources And Transformations
Understanding where data comes from and how it is transformed is crucial for maintaining the integrity of data throughout its lifespan.
Maintaining Metadata and Catalogs
Maintaining comprehensive metadata and data catalog is important for ensuring that data is easily attainable and understood by all investors.
Ensuring Data Provenance And Auditability
Data Provenance and ensuring auditability are important to follow with laws and maintain trust in Artificial Intelligence systems.
Data Security And Privacy
When discussing data safety and privacy, it’s crucial to contemplate numerous key aspects to ensure thorough security and assent. Below is an overview of the chief elements:
Data Protection And Access Control
Encryption: Encrypting data both at rest and in transit to safeguard it from improper access. This indulges using secure conventions such as HTTPS, SSL/TLS, and end-to-end encoding for contact.
Access Controls: Enforcing vigorous access control mechanisms like intricate validation (MFA), multifactor access control, and minimal advantage access strategies to ensure that only authorized individuals can ingress sensitive details.
Audit Trails: Handling comprehensive logs of data ingress and alterations to detect and retrospect how data is maintained and by whom. This helps in determining and replying to potent security infringements more efficiently.
Compliance With Regulations
Regulatory Understanding: Understanding the precise needs of regulations like the General Data Protection Regulation (GDPR) for European Union citizens, the Health Insurance Portability and Accountability Act (HIPAA), for health details in the United States, and other data safeguarding regulations.
Data Protection Officer (DPO): Designating a DPO to supervise data safeguarding plans, acquiescence with data safeguarding laws, and act as a point of connection for data subjects and regulatory authorities.
Data Subject Rights: Enforcing processes to acknowledge the data subject rights like the right to access, right to correction, right to erasure, and right to data flexibility.
Secure Data Handling And Storage Practices
Data Minimization: Gathering only the data that is important for the precise aim and reserving it only as long as it is required to serve those intentions.
Secure Data Storage Solutions: Using secure information and storage solutions that provides built-in security attributes such as automated backups, data repetition and safeguard against the loss of information.
Regular Audits and Penetration Testing: Managing regular security audits and penetration tests to determine and correct security susceptibilities. This also indulges upgrading and fixing software and systems to safeguard against known risks.
By following these practices, organizations can substantially improve their data security and privacy measures, ensuring that they not only safeguard sensitive details but also follow applicable laws, thereby handling trust and integrity in the digital ecosystem.
Data Ethics and Bias Mitigation
Ethical Principles For Data Collection And Use
Data gathering and utilization must follow ethical principles like neutrality, clarity, and respect for privacy. Associations should ensure that the data gathered is pertinent and significant for the contemplated purposes and gathered with specific permission from individuals.
Additionally, it’s important to inform participants about how their data will be utilized, ensuring their rights are safeguarded throughout the data lifespan.
Identifying And Mitigating Bias In Data
Partiality in data can result in uneven Artificial Intelligence results, affecting neutrality and effectiveness. To lessen this, it’s important to:
Determining potential sources of partiality across distinct phases of data gathering, refining and utilization.
Assort data sources to conceal a wide range of enumerations and synopsis.
Enforce bias detection methods like statistical examining and machine learning algorithms, to inspect and rectify partiality in data sets.
Fostering Transparency and Accountability
Transparency in Artificial Intelligence indulges in clear contact about how Artificial Intelligence systems operate, and the decision-making processes they adhere to. This can be accomplished by:
Establishing all data utilization and model decisions in a thorough manner.
Enforcing audit trails that trace data and selections for retrospection .
Engaging external audits to estimate AI practices and strategies.
Data Governance For AI Lifecycle
Data Governance in Model Development
Efficient data governance during model development ensures proper data use and that models are developed under appropriate guidelines. This indulges:
Endowing data quality standards to ensure data incorporation.
Regulating data access within the association to avert improper use.
Defining clear roles and authorities for team members indulged in data management and model development.
Data Governance in Model Deployment and Monitoring
Once models are deployed, constant monitoring is crucial to ensure they perform as intended and adhere to ethical standards. Data governance in this stage indulges:
Observing the performance of the model for preciseness and neutrality in real-time.
Modernizing data-management etiquettes as new data is gathered and as external circumstances change.
Ensuring model security against external risks and internal embezzle.
Continuous Improvement And Feedback Loops
The lifespan of Artificial Intelligence systems indulge apparatus for constant improvement:-
Feedback Loops that permits users to report problems or suggest enhancements.
Frequent updates to models based on new data, commuting circumstances and feedback.
Ongoing training for Artificial Intelligence teams on the newest technologies, ethical instructions, and governance etiquettes.
These details ensure that Artificial Intelligence systems are established and worked in a manner that is ethical, clear, accountable, and constantly enhancing, thus nurturing trust and dependability in AI applications.
Organizational Readiness and Change Management
Establishing Data Governance Roles And Responsibilities
To manage data effectively as a strategic asset, clear roles and responsibilities must be defined within the organization. This indulges setting up a data governance structure that indulges roles like Data Proprietors, Data Stewards, and Data keepers. Each role should have transparently defined duties:-
Data Proprietors are usually senior managers who are responsible for data quality and security in their respective areas.
Data Stewards are liable for the handling and fitness of data components, both content and metadata.
Data Keepers manage the technical aspect and directory handling, ensuring data is attainable yet secure.
Training and Awareness Programs
Training and Awareness programs are crucial for equipping team members with the necessary skills and knowledge to manage data responsibly.
These programs should cover topics like data privacy, data security strategies, and the significance of the data quality, and the organization’s precise data governance practices.
Frequent training classes ensure that all workers comprehend their roles in data governance and are up-to-date with the newest data handling procedures.
Fostering A Data-Driven Culture
Creating a data-driven culture requires more than just tools and policies; it requires a shift in attitude at every level of the organization.
Fostering open conversation about information, praising data-driven successes, and integrating data-based decision-making in daily working are all efficient strategies. Leaders must also master data governance by setting instances and constantly executing the value of data-driven insights.
Tools and Technologies for Data Governance
Data Catalogs and Metadata Management
Data Catalogs play a critical role in providing a single source of reference for organizational data assets, helping users find the data they need for their requirements.
These tools often trait metadata handling abilities that permits for analysis, classification, and concealment of data, making data more comprehensible and functional.
Metadata Management is crucial for comprehending data within its conditions, indulging its source, frameworks, reliabilities, or utilization policies.
Data Quality and Lineage Tools
Maintaining high data quality is essential for effective data governance. Tools for data quality ensures that data is precise, transparent and dependable. In addition, data lineage tools offers a transparent view of the data journey through the association, helping to trace the origin, movement or features and quality of data:
Data Lineage Tools help associations design the data lifespan, inspecting how data transforms, and derives from source to end of the line, which is critical for resolving and docility.
Data Governance Platforms and Solutions
Comprehensive data governance platforms include various functionalities to support data quality, compliance, policy management, and more. These platforms often indulge:
Automated Governance Tools that help in executing data governance strategies spontaneously across numerous data stores.
Integrated Solutions that gives an amalgamate of data cataloging, quality control, and lineage tracking, giving a comprehensive approach to data governance.
Enforcing these tools and technologies, besides a vigorous governing receptivity and change management plans, lays a powerful foundation for efficient data governance.
This planned approach ensures that data is handled as a precious asset, leading to enhanced working effectiveness, compliance and choice making abilities.
Now, let's dive into the world of case studies, where we'll see these best practices spring to life and truly make an impact.
Best Practices and Case Studies
Successful Data Governance Implementations
In successful data governance implementations, comprehensive data management procedures ensure that data remains accurate, accessible, and secure. For example, a reputable financial institution enforced a consolidated data governance structure that indulged organized data access rules and pioneering clear data possession.
They used metadata management tools to maintain a constant data dictionary, which substantially decreased the repetition of data and enhanced data quality across departments. This institution also engaged data lineage tools to track data from its source through its lifespan, improving clarity and responsibility.
Lessons Learned and Pitfalls to Avoid
Prevalent pitfalls in data governance indulges in minimizing the significance of organizational lifestyle and investor engagement. A case in point indulges a MNC that encountered resistance when enforcing a new governance program because it didn’t properly indulge predominant investors in the strategy stage.
This mistake led to a lack of continuity between ventures' aims and data practices, leading to poor adoption rates. Lessons learned features the significance of appealing to all investors early in the procedures, ensuring governance strategies align with actual assignment, and constantly teaching employees about the advantages of proper data handling.
Future Trends and Challenges
Emerging Data Governance Challenges
As technology progresses, data governance faces new difficulties like handling artificial data and co-operated learning environments. Artificial Data, propounds unique difficulties in ensuring the data imitates precise and impartial details.
Meanwhile, federated learning, a machine learning technique that trains computations across disparate devices or servers without swapping the data itself, presents intricacies in handling data privacy and security.
Governance plans must develop to acknowledge these problems, ensuring vigorous protocols for data verification and model clarity are in place.
Incorporating AI and Machine Learning in Data Governance
The incorporation of Artificial Intelligence and machine learning in data governance is becoming progressively predominant. AI can mechanize intricate data management tasks like data classification, anomaly detection, and predictive maintenance.
For example, AI driven tools can strongly adapt access controls and data quality regulations based on ongoing data utilization patterns and compliance needs. Hence, integrating these technologies requires robust structures to handle the AI lifespan, indulging model evolution, training, positioning, and monitoring.
Ensuring Artificial Intelligence systems administer data ethically and clearly remains an important challenge, requiring steady alertness and adjustment of governance structures to keep pace with technological progression.
Conclusion
To conclude the article, we have examined the critical role that data governance plays in utilizing the power of AI efficiently and legally. We explored pioneering a robust data governance structure, the complexities of handling data quality, and the significance of ensuring data lineage and traceability.
Furthermore, we accentuated the critical significance of maintaining the security and privacy of data, acknowledged the challenges of data ethics and bias mitigation, and discussed the thorough approach required for data governance across the AI lifespan.
The best practices and case studies give applicable insights and learnings from successful execution, emphasizing the practical phases of data governance in real-world scenarios.
Looking ahead, the arising challenges and future trends underline the robust nature of data governance, specifically in an era gradually conquered by AI inventiveness like synthetic data or federated learning.
For organizations intending to succeed in this AI-driven environment, the enforcement of sturdy data isn’t just advantageous; it is a requirement for imperishable success. Efficient data governance ensures that data stays safe, compliant and operable, which in turn, energizes AI systems that are strong, ethical and liable.
By following the practices, defined in this article, organizations can expect better decision-making abilities, improved working effectiveness, and an important fierce edge in the virtual age. Grasp these principles, and you will be fine on your way to unleashing the full potential of your data and AI investments.
Entering the age of AI without a solid data governance plan is like skydiving without a parachute – thrilling but perilous.
In the swiftly progressing field of Artificial Intelligence (AI), Governance and data handling play an important role. Efficient data governance ensures not only the precision and security of the data utilized for Artificial Intelligence but also its ethical sourcing and compliance with appropriate laws.
This guide explores the best practices in data governance for AI, providing a strategy for associations looking to utilize the power of AI responsibly and efficiently.
Introduction
Importance of Data Governance in AI
Data Governance is critical in AI because it ensures the incorporation, quality, and security of data in Artificial Intelligence systems. Efficient data governance assists in maintaining the precision and reliability of Artificial Intelligence outputs, significant for making informed choices and achieving desired results.
With the expanding dependency on Artificial Intelligence, Robust data governance structures protect against data misuse and ensure compliance with executive standards.
Challenges Of Data Management For AI Systems
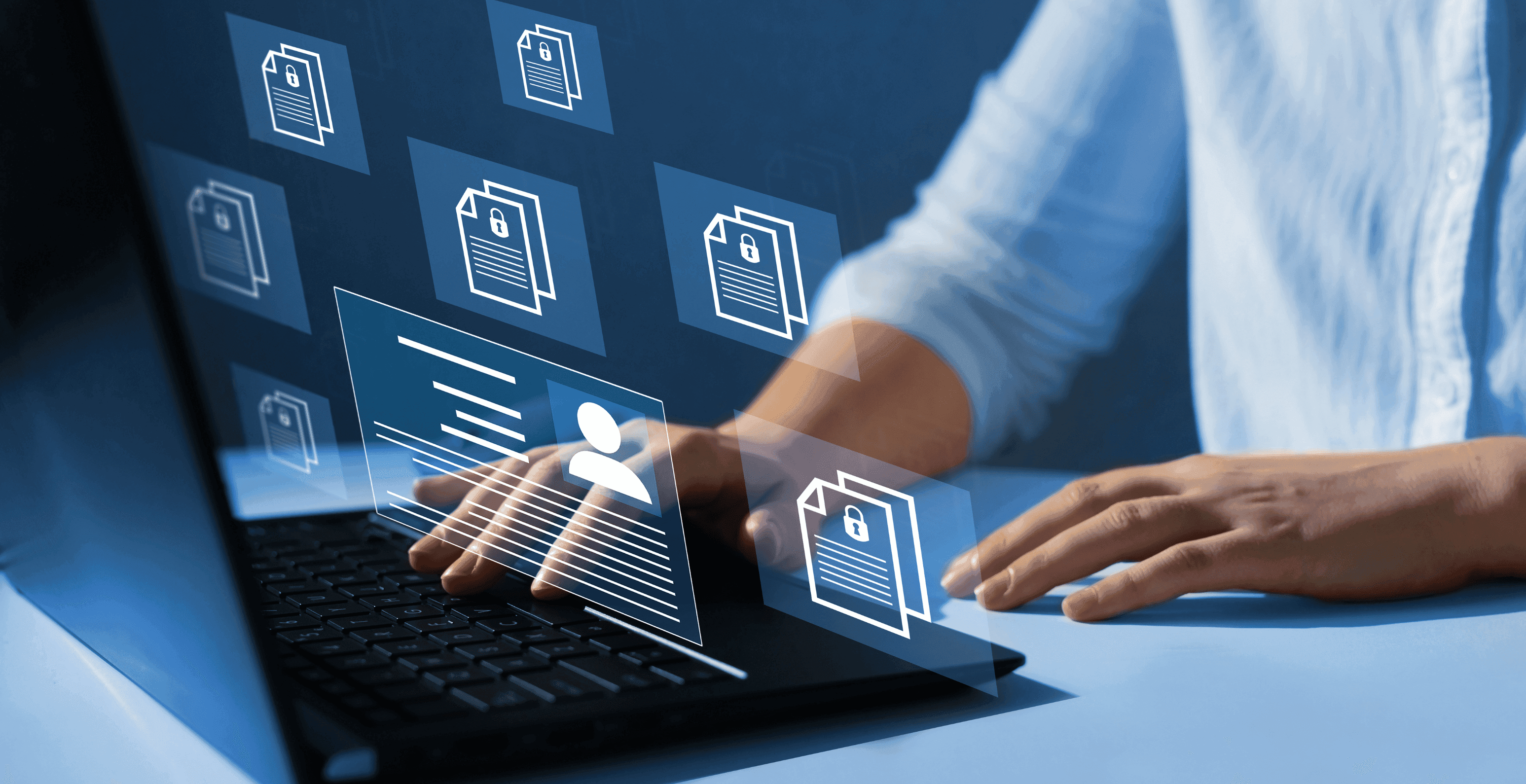
Data Management for Artificial Intelligence systems pose numerous challenges such as:
Data Quality and Consistency: Ensuring that data is precise, complete, and compatible is significant for training dependable Artificial Intelligence models.
Data Privacy and Security: Safeguarding sensitive data and maintaining assent with privacy laws such as GDPR and CCPA is crucial.
Data Integration and Silos: Incorporating data from numerous sources and breaking down data silos can be complicated but important for thorough analysis.
Ethical Considerations: Acknowledging tendencies in data and ensuring ethical utilization of Artificial Intelligence is crucial to avoid bigotry and unfair practices.
Scalability: Handling large dataset effectively and scaling systems to encompass evolving data requirements is significant for nourished AI performance.
Overview Of Data Governance Frameworks
Data governance structures offer structures approaches for handling data efficiently. These structures usually indulge:
Policies and Standards: Endowing recommendations for usage of data, security and privacy.
Roles and Responsibilities: Defining roles such as data housekeepers, data proprietors, and data caretakers to ensure liability.
Data Quality Management: Enforcing processes to monitor and enhance the quality of data.
Compliance and Audit: Ensuring compliance to legal and regulatory needs and imparting regular audits.
Data Lifecycle Management: Handling data from its formation to its removal, ensuring appropriate management at each stage.
Technology and Tools:- Using advanced technologies and tools for data handling, incorporation and dissecting.
Establishing A Data Governance Framework
Defining Organizational Data Strategy And Objectives
To begin, it’s important to clearly define your administrative data plans and purposes. This involves understanding the specific goals your institution aims to achieve through data governance.
Whether it's enhancing the quality of data, ensuring assent with regulations, or using data for strategic decision-making, having an explicit plan to provide a plan for all data-related activities.
Set Clear Goals: Decide what you want to accomplish with your data governance efforts. This could range from enhancing data precision to improving data security or upgrading data utilization for enhanced venture insights.
Align with Business Objectives: Ensure that your data plans correspond with your overall venture aims. This layout helps in giving priority to data capabilities that offer most value to an organization.
Develop a Data Management Plan: Summarize the processes and practices for handling data throughout its lifetime. This indulges data gathering, storage, usage and positioning.
Identifying Stakeholders and Roles
Successful data governance requires participation from various stakeholders across the association. Determining these stakeholders and clearly defining their roles is critical for the structure’s efficiency.
Identify Key Stakeholders: These usually include managers, data owners, data stewards, Information Technology staff and end-users. Each group has a suited interest in how data is handled and used.
Define Roles and Responsibilities: Evidently summarize the roles and responsibilities for each investor group. For instance, data stewards might be liable for handling data quality, while IT staff may manage data security.
Establish A Data Governance Council: Shape a governance council or committee that indulges deputies from key stakeholders groups. This council supervises the execution and administration of data governance policies.
Creating Data Governance Policies and Standards
Creating rigorous data governance policies and standards ensures that data is consistently and efficiently supervised across the association.
Develop Thorough Policies: These should cover numerous phases of data management, indulging the quality of data, data security, data seclusion and data lifespan handling. Policies should be transparent, applicable and executable.
Set Data Standards: Pioneer standards for data formats, naming customs, metadata handling, and data incorporation. Compatible standards help in maintaining the quality of data and conformity.
Implement Data Compliance Measures: Ensure that your strategies adhere with appropriate laws and standards, like GDPR or CCPA. Frequently check and update these strategies to stay compliant.
By adhering to these steps, your firm can pioneer a solid data governance structure that not only improves data management practices but also supports accomplishing strategic venture goals.
Data Quality Management
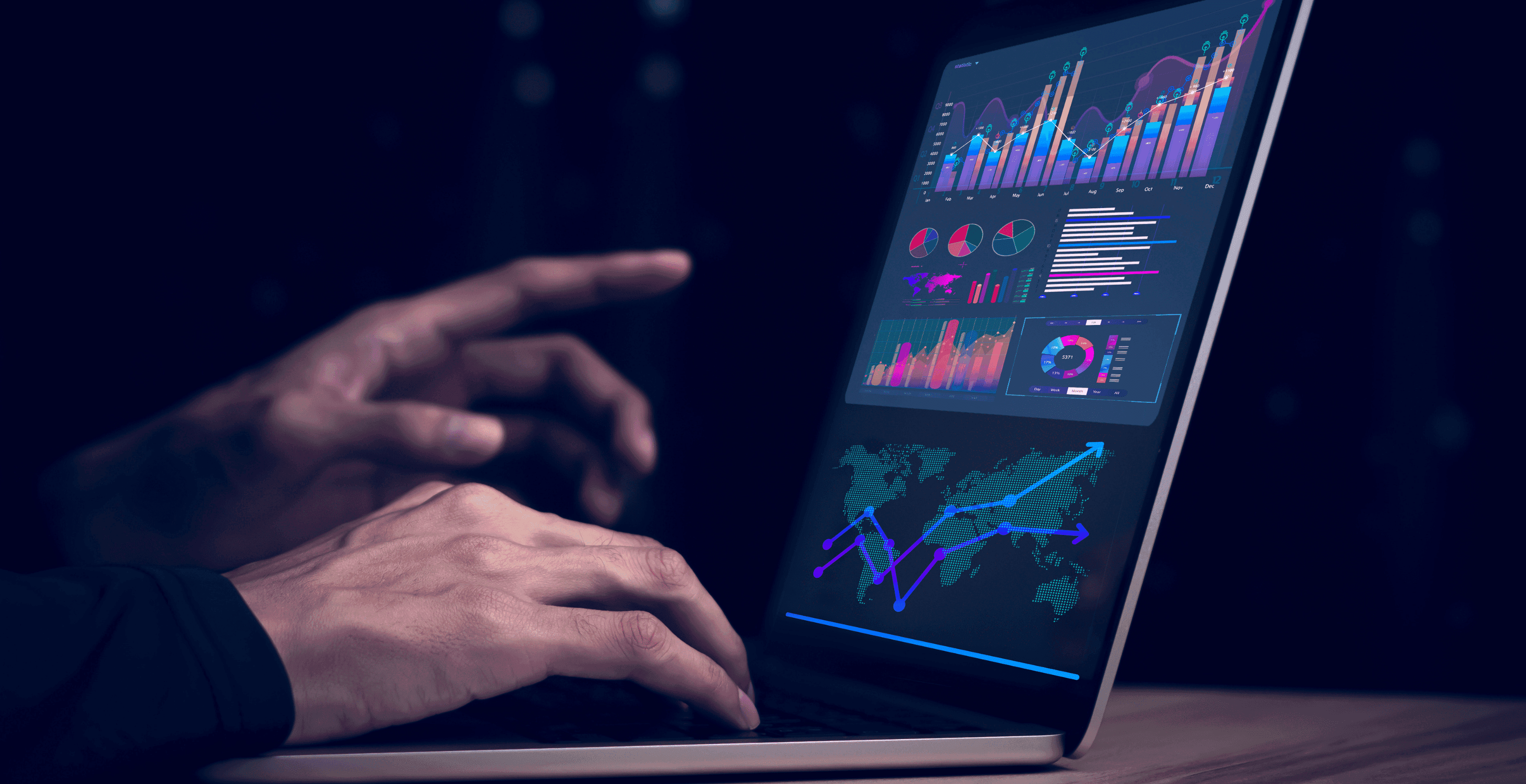
When managing data quality, organizations aim to ensure that their data is accurate, reliable, and useful for decision-making. Here’s an outline of chief aspects indulged in data-quality management:
Data Quality Dimensions
Accuracy: Refers to the accuracy of data after comparison with responsible sources or references. Accurate data appropriately imitates the real-world values it is aiming to represent.
Completeness: Covers to the limit to which all needed data is attainable. Incomplete data can result in examination that is possibly partial or misleading.
Consistency: Ensures that the information across varied datasets or information are compatible without any strife or modifications. Consistency is important for data to be dependable and trustworthy.
Data Profiling and Quality Assessment
Data Profiling: This is the initial step in comprehending the existing form of data. It indulges in investigating the data attainable and gathering statistics and information about that data. The process usually identifies problems with preciseness, distinctiveness, correspondence and entirety.
Quality Assessment: Continues from profiling, indulging more profound inspection to comprehend the root causes of data problems and to identify their effects on appropriate ventures processes. This indulges in setting standards for quality quality, estimating data against these criterias, and reporting the standing of data quality.
Data Cleansing And Transformation Processes
Data Cleansing:
Error Correction: Fixing mistakes determined in the data, like typos, spelling errors, or incorrect data entries.
Normalization: Normalizing the data structures, labels and data types to ensure correspondence across the dataset.
Missing Data Handling: Filling missing values through methods such as attribution or deciding to ignore or drop them based on the impact evaluation.
Data Transformation:
Data Integration: Merging data from various sources to give a unified view. This often indulges resolving incompatibilities and supporting the entirety of data.
Data Conversion: Transforming data from one format to another, usually to incorporate with other systems or to correspond with the needs of a new system.
Data Enrichment: Accelerating existing data with data from added sources to improve the data’s information or entirety.
Efficient data quality management ensures that data is a precious asset for decision-making, docility, and working procedures.
It needs constant efforts and incorporation into the daily data management processes to handle the quality of data throughout its lifespan.
Data Lineage and Traceability
Tracking Data Sources And Transformations
Understanding where data comes from and how it is transformed is crucial for maintaining the integrity of data throughout its lifespan.
Maintaining Metadata and Catalogs
Maintaining comprehensive metadata and data catalog is important for ensuring that data is easily attainable and understood by all investors.
Ensuring Data Provenance And Auditability
Data Provenance and ensuring auditability are important to follow with laws and maintain trust in Artificial Intelligence systems.
Data Security And Privacy
When discussing data safety and privacy, it’s crucial to contemplate numerous key aspects to ensure thorough security and assent. Below is an overview of the chief elements:
Data Protection And Access Control
Encryption: Encrypting data both at rest and in transit to safeguard it from improper access. This indulges using secure conventions such as HTTPS, SSL/TLS, and end-to-end encoding for contact.
Access Controls: Enforcing vigorous access control mechanisms like intricate validation (MFA), multifactor access control, and minimal advantage access strategies to ensure that only authorized individuals can ingress sensitive details.
Audit Trails: Handling comprehensive logs of data ingress and alterations to detect and retrospect how data is maintained and by whom. This helps in determining and replying to potent security infringements more efficiently.
Compliance With Regulations
Regulatory Understanding: Understanding the precise needs of regulations like the General Data Protection Regulation (GDPR) for European Union citizens, the Health Insurance Portability and Accountability Act (HIPAA), for health details in the United States, and other data safeguarding regulations.
Data Protection Officer (DPO): Designating a DPO to supervise data safeguarding plans, acquiescence with data safeguarding laws, and act as a point of connection for data subjects and regulatory authorities.
Data Subject Rights: Enforcing processes to acknowledge the data subject rights like the right to access, right to correction, right to erasure, and right to data flexibility.
Secure Data Handling And Storage Practices
Data Minimization: Gathering only the data that is important for the precise aim and reserving it only as long as it is required to serve those intentions.
Secure Data Storage Solutions: Using secure information and storage solutions that provides built-in security attributes such as automated backups, data repetition and safeguard against the loss of information.
Regular Audits and Penetration Testing: Managing regular security audits and penetration tests to determine and correct security susceptibilities. This also indulges upgrading and fixing software and systems to safeguard against known risks.
By following these practices, organizations can substantially improve their data security and privacy measures, ensuring that they not only safeguard sensitive details but also follow applicable laws, thereby handling trust and integrity in the digital ecosystem.
Data Ethics and Bias Mitigation
Ethical Principles For Data Collection And Use
Data gathering and utilization must follow ethical principles like neutrality, clarity, and respect for privacy. Associations should ensure that the data gathered is pertinent and significant for the contemplated purposes and gathered with specific permission from individuals.
Additionally, it’s important to inform participants about how their data will be utilized, ensuring their rights are safeguarded throughout the data lifespan.
Identifying And Mitigating Bias In Data
Partiality in data can result in uneven Artificial Intelligence results, affecting neutrality and effectiveness. To lessen this, it’s important to:
Determining potential sources of partiality across distinct phases of data gathering, refining and utilization.
Assort data sources to conceal a wide range of enumerations and synopsis.
Enforce bias detection methods like statistical examining and machine learning algorithms, to inspect and rectify partiality in data sets.
Fostering Transparency and Accountability
Transparency in Artificial Intelligence indulges in clear contact about how Artificial Intelligence systems operate, and the decision-making processes they adhere to. This can be accomplished by:
Establishing all data utilization and model decisions in a thorough manner.
Enforcing audit trails that trace data and selections for retrospection .
Engaging external audits to estimate AI practices and strategies.
Data Governance For AI Lifecycle
Data Governance in Model Development
Efficient data governance during model development ensures proper data use and that models are developed under appropriate guidelines. This indulges:
Endowing data quality standards to ensure data incorporation.
Regulating data access within the association to avert improper use.
Defining clear roles and authorities for team members indulged in data management and model development.
Data Governance in Model Deployment and Monitoring
Once models are deployed, constant monitoring is crucial to ensure they perform as intended and adhere to ethical standards. Data governance in this stage indulges:
Observing the performance of the model for preciseness and neutrality in real-time.
Modernizing data-management etiquettes as new data is gathered and as external circumstances change.
Ensuring model security against external risks and internal embezzle.
Continuous Improvement And Feedback Loops
The lifespan of Artificial Intelligence systems indulge apparatus for constant improvement:-
Feedback Loops that permits users to report problems or suggest enhancements.
Frequent updates to models based on new data, commuting circumstances and feedback.
Ongoing training for Artificial Intelligence teams on the newest technologies, ethical instructions, and governance etiquettes.
These details ensure that Artificial Intelligence systems are established and worked in a manner that is ethical, clear, accountable, and constantly enhancing, thus nurturing trust and dependability in AI applications.
Organizational Readiness and Change Management
Establishing Data Governance Roles And Responsibilities
To manage data effectively as a strategic asset, clear roles and responsibilities must be defined within the organization. This indulges setting up a data governance structure that indulges roles like Data Proprietors, Data Stewards, and Data keepers. Each role should have transparently defined duties:-
Data Proprietors are usually senior managers who are responsible for data quality and security in their respective areas.
Data Stewards are liable for the handling and fitness of data components, both content and metadata.
Data Keepers manage the technical aspect and directory handling, ensuring data is attainable yet secure.
Training and Awareness Programs
Training and Awareness programs are crucial for equipping team members with the necessary skills and knowledge to manage data responsibly.
These programs should cover topics like data privacy, data security strategies, and the significance of the data quality, and the organization’s precise data governance practices.
Frequent training classes ensure that all workers comprehend their roles in data governance and are up-to-date with the newest data handling procedures.
Fostering A Data-Driven Culture
Creating a data-driven culture requires more than just tools and policies; it requires a shift in attitude at every level of the organization.
Fostering open conversation about information, praising data-driven successes, and integrating data-based decision-making in daily working are all efficient strategies. Leaders must also master data governance by setting instances and constantly executing the value of data-driven insights.
Tools and Technologies for Data Governance
Data Catalogs and Metadata Management
Data Catalogs play a critical role in providing a single source of reference for organizational data assets, helping users find the data they need for their requirements.
These tools often trait metadata handling abilities that permits for analysis, classification, and concealment of data, making data more comprehensible and functional.
Metadata Management is crucial for comprehending data within its conditions, indulging its source, frameworks, reliabilities, or utilization policies.
Data Quality and Lineage Tools
Maintaining high data quality is essential for effective data governance. Tools for data quality ensures that data is precise, transparent and dependable. In addition, data lineage tools offers a transparent view of the data journey through the association, helping to trace the origin, movement or features and quality of data:
Data Lineage Tools help associations design the data lifespan, inspecting how data transforms, and derives from source to end of the line, which is critical for resolving and docility.
Data Governance Platforms and Solutions
Comprehensive data governance platforms include various functionalities to support data quality, compliance, policy management, and more. These platforms often indulge:
Automated Governance Tools that help in executing data governance strategies spontaneously across numerous data stores.
Integrated Solutions that gives an amalgamate of data cataloging, quality control, and lineage tracking, giving a comprehensive approach to data governance.
Enforcing these tools and technologies, besides a vigorous governing receptivity and change management plans, lays a powerful foundation for efficient data governance.
This planned approach ensures that data is handled as a precious asset, leading to enhanced working effectiveness, compliance and choice making abilities.
Now, let's dive into the world of case studies, where we'll see these best practices spring to life and truly make an impact.
Best Practices and Case Studies
Successful Data Governance Implementations
In successful data governance implementations, comprehensive data management procedures ensure that data remains accurate, accessible, and secure. For example, a reputable financial institution enforced a consolidated data governance structure that indulged organized data access rules and pioneering clear data possession.
They used metadata management tools to maintain a constant data dictionary, which substantially decreased the repetition of data and enhanced data quality across departments. This institution also engaged data lineage tools to track data from its source through its lifespan, improving clarity and responsibility.
Lessons Learned and Pitfalls to Avoid
Prevalent pitfalls in data governance indulges in minimizing the significance of organizational lifestyle and investor engagement. A case in point indulges a MNC that encountered resistance when enforcing a new governance program because it didn’t properly indulge predominant investors in the strategy stage.
This mistake led to a lack of continuity between ventures' aims and data practices, leading to poor adoption rates. Lessons learned features the significance of appealing to all investors early in the procedures, ensuring governance strategies align with actual assignment, and constantly teaching employees about the advantages of proper data handling.
Future Trends and Challenges
Emerging Data Governance Challenges
As technology progresses, data governance faces new difficulties like handling artificial data and co-operated learning environments. Artificial Data, propounds unique difficulties in ensuring the data imitates precise and impartial details.
Meanwhile, federated learning, a machine learning technique that trains computations across disparate devices or servers without swapping the data itself, presents intricacies in handling data privacy and security.
Governance plans must develop to acknowledge these problems, ensuring vigorous protocols for data verification and model clarity are in place.
Incorporating AI and Machine Learning in Data Governance
The incorporation of Artificial Intelligence and machine learning in data governance is becoming progressively predominant. AI can mechanize intricate data management tasks like data classification, anomaly detection, and predictive maintenance.
For example, AI driven tools can strongly adapt access controls and data quality regulations based on ongoing data utilization patterns and compliance needs. Hence, integrating these technologies requires robust structures to handle the AI lifespan, indulging model evolution, training, positioning, and monitoring.
Ensuring Artificial Intelligence systems administer data ethically and clearly remains an important challenge, requiring steady alertness and adjustment of governance structures to keep pace with technological progression.
Conclusion
To conclude the article, we have examined the critical role that data governance plays in utilizing the power of AI efficiently and legally. We explored pioneering a robust data governance structure, the complexities of handling data quality, and the significance of ensuring data lineage and traceability.
Furthermore, we accentuated the critical significance of maintaining the security and privacy of data, acknowledged the challenges of data ethics and bias mitigation, and discussed the thorough approach required for data governance across the AI lifespan.
The best practices and case studies give applicable insights and learnings from successful execution, emphasizing the practical phases of data governance in real-world scenarios.
Looking ahead, the arising challenges and future trends underline the robust nature of data governance, specifically in an era gradually conquered by AI inventiveness like synthetic data or federated learning.
For organizations intending to succeed in this AI-driven environment, the enforcement of sturdy data isn’t just advantageous; it is a requirement for imperishable success. Efficient data governance ensures that data stays safe, compliant and operable, which in turn, energizes AI systems that are strong, ethical and liable.
By following the practices, defined in this article, organizations can expect better decision-making abilities, improved working effectiveness, and an important fierce edge in the virtual age. Grasp these principles, and you will be fine on your way to unleashing the full potential of your data and AI investments.
Entering the age of AI without a solid data governance plan is like skydiving without a parachute – thrilling but perilous.
In the swiftly progressing field of Artificial Intelligence (AI), Governance and data handling play an important role. Efficient data governance ensures not only the precision and security of the data utilized for Artificial Intelligence but also its ethical sourcing and compliance with appropriate laws.
This guide explores the best practices in data governance for AI, providing a strategy for associations looking to utilize the power of AI responsibly and efficiently.
Introduction
Importance of Data Governance in AI
Data Governance is critical in AI because it ensures the incorporation, quality, and security of data in Artificial Intelligence systems. Efficient data governance assists in maintaining the precision and reliability of Artificial Intelligence outputs, significant for making informed choices and achieving desired results.
With the expanding dependency on Artificial Intelligence, Robust data governance structures protect against data misuse and ensure compliance with executive standards.
Challenges Of Data Management For AI Systems
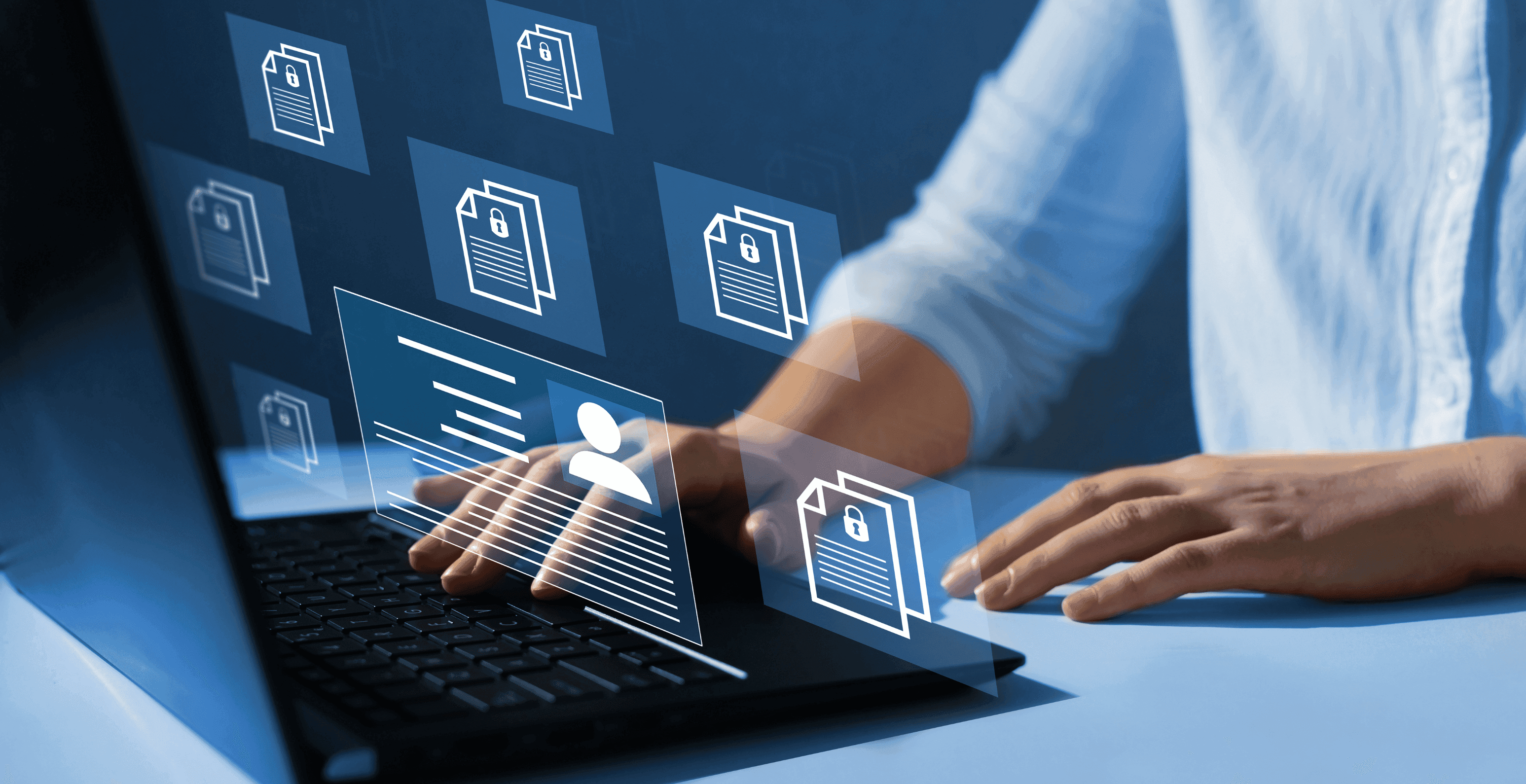
Data Management for Artificial Intelligence systems pose numerous challenges such as:
Data Quality and Consistency: Ensuring that data is precise, complete, and compatible is significant for training dependable Artificial Intelligence models.
Data Privacy and Security: Safeguarding sensitive data and maintaining assent with privacy laws such as GDPR and CCPA is crucial.
Data Integration and Silos: Incorporating data from numerous sources and breaking down data silos can be complicated but important for thorough analysis.
Ethical Considerations: Acknowledging tendencies in data and ensuring ethical utilization of Artificial Intelligence is crucial to avoid bigotry and unfair practices.
Scalability: Handling large dataset effectively and scaling systems to encompass evolving data requirements is significant for nourished AI performance.
Overview Of Data Governance Frameworks
Data governance structures offer structures approaches for handling data efficiently. These structures usually indulge:
Policies and Standards: Endowing recommendations for usage of data, security and privacy.
Roles and Responsibilities: Defining roles such as data housekeepers, data proprietors, and data caretakers to ensure liability.
Data Quality Management: Enforcing processes to monitor and enhance the quality of data.
Compliance and Audit: Ensuring compliance to legal and regulatory needs and imparting regular audits.
Data Lifecycle Management: Handling data from its formation to its removal, ensuring appropriate management at each stage.
Technology and Tools:- Using advanced technologies and tools for data handling, incorporation and dissecting.
Establishing A Data Governance Framework
Defining Organizational Data Strategy And Objectives
To begin, it’s important to clearly define your administrative data plans and purposes. This involves understanding the specific goals your institution aims to achieve through data governance.
Whether it's enhancing the quality of data, ensuring assent with regulations, or using data for strategic decision-making, having an explicit plan to provide a plan for all data-related activities.
Set Clear Goals: Decide what you want to accomplish with your data governance efforts. This could range from enhancing data precision to improving data security or upgrading data utilization for enhanced venture insights.
Align with Business Objectives: Ensure that your data plans correspond with your overall venture aims. This layout helps in giving priority to data capabilities that offer most value to an organization.
Develop a Data Management Plan: Summarize the processes and practices for handling data throughout its lifetime. This indulges data gathering, storage, usage and positioning.
Identifying Stakeholders and Roles
Successful data governance requires participation from various stakeholders across the association. Determining these stakeholders and clearly defining their roles is critical for the structure’s efficiency.
Identify Key Stakeholders: These usually include managers, data owners, data stewards, Information Technology staff and end-users. Each group has a suited interest in how data is handled and used.
Define Roles and Responsibilities: Evidently summarize the roles and responsibilities for each investor group. For instance, data stewards might be liable for handling data quality, while IT staff may manage data security.
Establish A Data Governance Council: Shape a governance council or committee that indulges deputies from key stakeholders groups. This council supervises the execution and administration of data governance policies.
Creating Data Governance Policies and Standards
Creating rigorous data governance policies and standards ensures that data is consistently and efficiently supervised across the association.
Develop Thorough Policies: These should cover numerous phases of data management, indulging the quality of data, data security, data seclusion and data lifespan handling. Policies should be transparent, applicable and executable.
Set Data Standards: Pioneer standards for data formats, naming customs, metadata handling, and data incorporation. Compatible standards help in maintaining the quality of data and conformity.
Implement Data Compliance Measures: Ensure that your strategies adhere with appropriate laws and standards, like GDPR or CCPA. Frequently check and update these strategies to stay compliant.
By adhering to these steps, your firm can pioneer a solid data governance structure that not only improves data management practices but also supports accomplishing strategic venture goals.
Data Quality Management
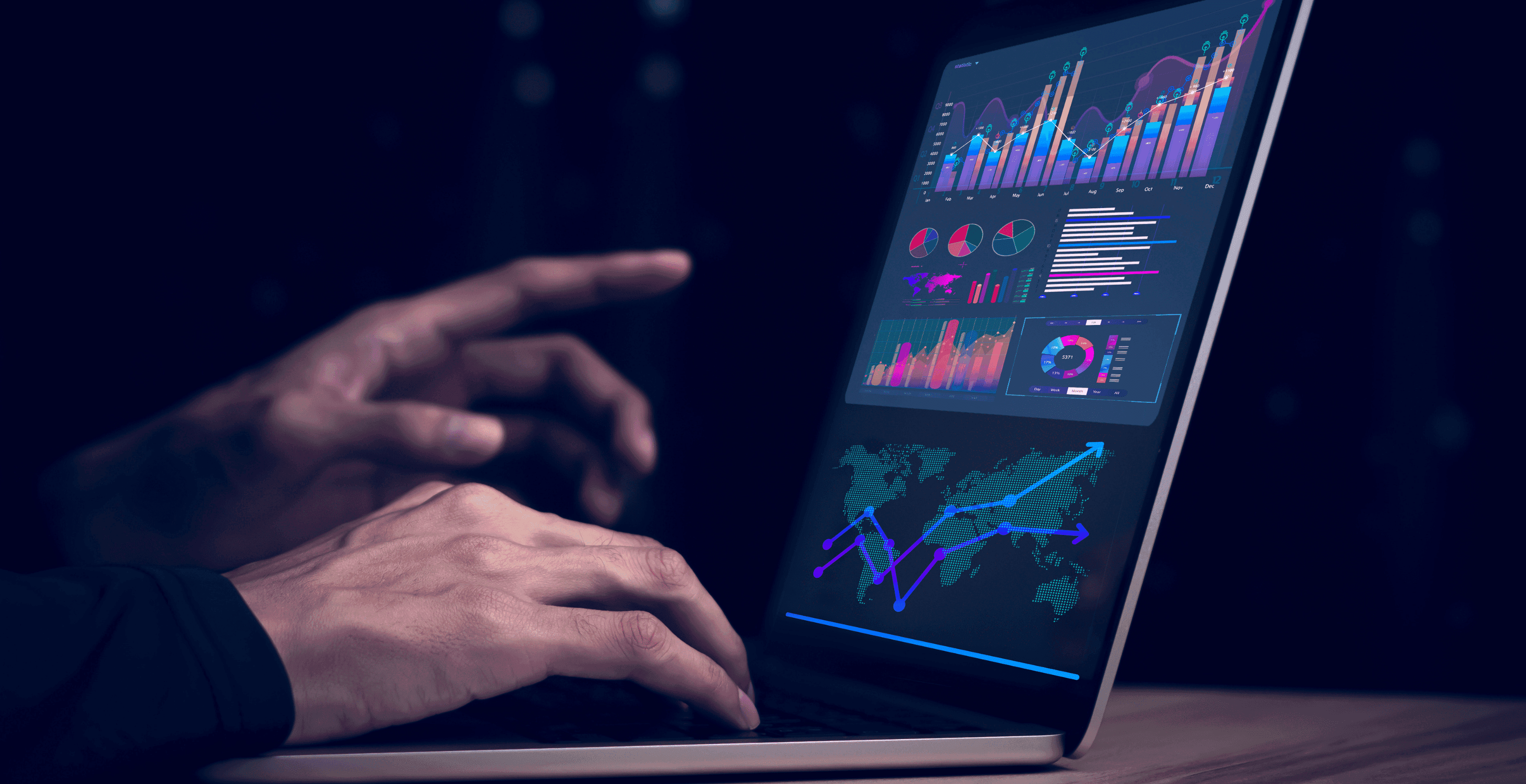
When managing data quality, organizations aim to ensure that their data is accurate, reliable, and useful for decision-making. Here’s an outline of chief aspects indulged in data-quality management:
Data Quality Dimensions
Accuracy: Refers to the accuracy of data after comparison with responsible sources or references. Accurate data appropriately imitates the real-world values it is aiming to represent.
Completeness: Covers to the limit to which all needed data is attainable. Incomplete data can result in examination that is possibly partial or misleading.
Consistency: Ensures that the information across varied datasets or information are compatible without any strife or modifications. Consistency is important for data to be dependable and trustworthy.
Data Profiling and Quality Assessment
Data Profiling: This is the initial step in comprehending the existing form of data. It indulges in investigating the data attainable and gathering statistics and information about that data. The process usually identifies problems with preciseness, distinctiveness, correspondence and entirety.
Quality Assessment: Continues from profiling, indulging more profound inspection to comprehend the root causes of data problems and to identify their effects on appropriate ventures processes. This indulges in setting standards for quality quality, estimating data against these criterias, and reporting the standing of data quality.
Data Cleansing And Transformation Processes
Data Cleansing:
Error Correction: Fixing mistakes determined in the data, like typos, spelling errors, or incorrect data entries.
Normalization: Normalizing the data structures, labels and data types to ensure correspondence across the dataset.
Missing Data Handling: Filling missing values through methods such as attribution or deciding to ignore or drop them based on the impact evaluation.
Data Transformation:
Data Integration: Merging data from various sources to give a unified view. This often indulges resolving incompatibilities and supporting the entirety of data.
Data Conversion: Transforming data from one format to another, usually to incorporate with other systems or to correspond with the needs of a new system.
Data Enrichment: Accelerating existing data with data from added sources to improve the data’s information or entirety.
Efficient data quality management ensures that data is a precious asset for decision-making, docility, and working procedures.
It needs constant efforts and incorporation into the daily data management processes to handle the quality of data throughout its lifespan.
Data Lineage and Traceability
Tracking Data Sources And Transformations
Understanding where data comes from and how it is transformed is crucial for maintaining the integrity of data throughout its lifespan.
Maintaining Metadata and Catalogs
Maintaining comprehensive metadata and data catalog is important for ensuring that data is easily attainable and understood by all investors.
Ensuring Data Provenance And Auditability
Data Provenance and ensuring auditability are important to follow with laws and maintain trust in Artificial Intelligence systems.
Data Security And Privacy
When discussing data safety and privacy, it’s crucial to contemplate numerous key aspects to ensure thorough security and assent. Below is an overview of the chief elements:
Data Protection And Access Control
Encryption: Encrypting data both at rest and in transit to safeguard it from improper access. This indulges using secure conventions such as HTTPS, SSL/TLS, and end-to-end encoding for contact.
Access Controls: Enforcing vigorous access control mechanisms like intricate validation (MFA), multifactor access control, and minimal advantage access strategies to ensure that only authorized individuals can ingress sensitive details.
Audit Trails: Handling comprehensive logs of data ingress and alterations to detect and retrospect how data is maintained and by whom. This helps in determining and replying to potent security infringements more efficiently.
Compliance With Regulations
Regulatory Understanding: Understanding the precise needs of regulations like the General Data Protection Regulation (GDPR) for European Union citizens, the Health Insurance Portability and Accountability Act (HIPAA), for health details in the United States, and other data safeguarding regulations.
Data Protection Officer (DPO): Designating a DPO to supervise data safeguarding plans, acquiescence with data safeguarding laws, and act as a point of connection for data subjects and regulatory authorities.
Data Subject Rights: Enforcing processes to acknowledge the data subject rights like the right to access, right to correction, right to erasure, and right to data flexibility.
Secure Data Handling And Storage Practices
Data Minimization: Gathering only the data that is important for the precise aim and reserving it only as long as it is required to serve those intentions.
Secure Data Storage Solutions: Using secure information and storage solutions that provides built-in security attributes such as automated backups, data repetition and safeguard against the loss of information.
Regular Audits and Penetration Testing: Managing regular security audits and penetration tests to determine and correct security susceptibilities. This also indulges upgrading and fixing software and systems to safeguard against known risks.
By following these practices, organizations can substantially improve their data security and privacy measures, ensuring that they not only safeguard sensitive details but also follow applicable laws, thereby handling trust and integrity in the digital ecosystem.
Data Ethics and Bias Mitigation
Ethical Principles For Data Collection And Use
Data gathering and utilization must follow ethical principles like neutrality, clarity, and respect for privacy. Associations should ensure that the data gathered is pertinent and significant for the contemplated purposes and gathered with specific permission from individuals.
Additionally, it’s important to inform participants about how their data will be utilized, ensuring their rights are safeguarded throughout the data lifespan.
Identifying And Mitigating Bias In Data
Partiality in data can result in uneven Artificial Intelligence results, affecting neutrality and effectiveness. To lessen this, it’s important to:
Determining potential sources of partiality across distinct phases of data gathering, refining and utilization.
Assort data sources to conceal a wide range of enumerations and synopsis.
Enforce bias detection methods like statistical examining and machine learning algorithms, to inspect and rectify partiality in data sets.
Fostering Transparency and Accountability
Transparency in Artificial Intelligence indulges in clear contact about how Artificial Intelligence systems operate, and the decision-making processes they adhere to. This can be accomplished by:
Establishing all data utilization and model decisions in a thorough manner.
Enforcing audit trails that trace data and selections for retrospection .
Engaging external audits to estimate AI practices and strategies.
Data Governance For AI Lifecycle
Data Governance in Model Development
Efficient data governance during model development ensures proper data use and that models are developed under appropriate guidelines. This indulges:
Endowing data quality standards to ensure data incorporation.
Regulating data access within the association to avert improper use.
Defining clear roles and authorities for team members indulged in data management and model development.
Data Governance in Model Deployment and Monitoring
Once models are deployed, constant monitoring is crucial to ensure they perform as intended and adhere to ethical standards. Data governance in this stage indulges:
Observing the performance of the model for preciseness and neutrality in real-time.
Modernizing data-management etiquettes as new data is gathered and as external circumstances change.
Ensuring model security against external risks and internal embezzle.
Continuous Improvement And Feedback Loops
The lifespan of Artificial Intelligence systems indulge apparatus for constant improvement:-
Feedback Loops that permits users to report problems or suggest enhancements.
Frequent updates to models based on new data, commuting circumstances and feedback.
Ongoing training for Artificial Intelligence teams on the newest technologies, ethical instructions, and governance etiquettes.
These details ensure that Artificial Intelligence systems are established and worked in a manner that is ethical, clear, accountable, and constantly enhancing, thus nurturing trust and dependability in AI applications.
Organizational Readiness and Change Management
Establishing Data Governance Roles And Responsibilities
To manage data effectively as a strategic asset, clear roles and responsibilities must be defined within the organization. This indulges setting up a data governance structure that indulges roles like Data Proprietors, Data Stewards, and Data keepers. Each role should have transparently defined duties:-
Data Proprietors are usually senior managers who are responsible for data quality and security in their respective areas.
Data Stewards are liable for the handling and fitness of data components, both content and metadata.
Data Keepers manage the technical aspect and directory handling, ensuring data is attainable yet secure.
Training and Awareness Programs
Training and Awareness programs are crucial for equipping team members with the necessary skills and knowledge to manage data responsibly.
These programs should cover topics like data privacy, data security strategies, and the significance of the data quality, and the organization’s precise data governance practices.
Frequent training classes ensure that all workers comprehend their roles in data governance and are up-to-date with the newest data handling procedures.
Fostering A Data-Driven Culture
Creating a data-driven culture requires more than just tools and policies; it requires a shift in attitude at every level of the organization.
Fostering open conversation about information, praising data-driven successes, and integrating data-based decision-making in daily working are all efficient strategies. Leaders must also master data governance by setting instances and constantly executing the value of data-driven insights.
Tools and Technologies for Data Governance
Data Catalogs and Metadata Management
Data Catalogs play a critical role in providing a single source of reference for organizational data assets, helping users find the data they need for their requirements.
These tools often trait metadata handling abilities that permits for analysis, classification, and concealment of data, making data more comprehensible and functional.
Metadata Management is crucial for comprehending data within its conditions, indulging its source, frameworks, reliabilities, or utilization policies.
Data Quality and Lineage Tools
Maintaining high data quality is essential for effective data governance. Tools for data quality ensures that data is precise, transparent and dependable. In addition, data lineage tools offers a transparent view of the data journey through the association, helping to trace the origin, movement or features and quality of data:
Data Lineage Tools help associations design the data lifespan, inspecting how data transforms, and derives from source to end of the line, which is critical for resolving and docility.
Data Governance Platforms and Solutions
Comprehensive data governance platforms include various functionalities to support data quality, compliance, policy management, and more. These platforms often indulge:
Automated Governance Tools that help in executing data governance strategies spontaneously across numerous data stores.
Integrated Solutions that gives an amalgamate of data cataloging, quality control, and lineage tracking, giving a comprehensive approach to data governance.
Enforcing these tools and technologies, besides a vigorous governing receptivity and change management plans, lays a powerful foundation for efficient data governance.
This planned approach ensures that data is handled as a precious asset, leading to enhanced working effectiveness, compliance and choice making abilities.
Now, let's dive into the world of case studies, where we'll see these best practices spring to life and truly make an impact.
Best Practices and Case Studies
Successful Data Governance Implementations
In successful data governance implementations, comprehensive data management procedures ensure that data remains accurate, accessible, and secure. For example, a reputable financial institution enforced a consolidated data governance structure that indulged organized data access rules and pioneering clear data possession.
They used metadata management tools to maintain a constant data dictionary, which substantially decreased the repetition of data and enhanced data quality across departments. This institution also engaged data lineage tools to track data from its source through its lifespan, improving clarity and responsibility.
Lessons Learned and Pitfalls to Avoid
Prevalent pitfalls in data governance indulges in minimizing the significance of organizational lifestyle and investor engagement. A case in point indulges a MNC that encountered resistance when enforcing a new governance program because it didn’t properly indulge predominant investors in the strategy stage.
This mistake led to a lack of continuity between ventures' aims and data practices, leading to poor adoption rates. Lessons learned features the significance of appealing to all investors early in the procedures, ensuring governance strategies align with actual assignment, and constantly teaching employees about the advantages of proper data handling.
Future Trends and Challenges
Emerging Data Governance Challenges
As technology progresses, data governance faces new difficulties like handling artificial data and co-operated learning environments. Artificial Data, propounds unique difficulties in ensuring the data imitates precise and impartial details.
Meanwhile, federated learning, a machine learning technique that trains computations across disparate devices or servers without swapping the data itself, presents intricacies in handling data privacy and security.
Governance plans must develop to acknowledge these problems, ensuring vigorous protocols for data verification and model clarity are in place.
Incorporating AI and Machine Learning in Data Governance
The incorporation of Artificial Intelligence and machine learning in data governance is becoming progressively predominant. AI can mechanize intricate data management tasks like data classification, anomaly detection, and predictive maintenance.
For example, AI driven tools can strongly adapt access controls and data quality regulations based on ongoing data utilization patterns and compliance needs. Hence, integrating these technologies requires robust structures to handle the AI lifespan, indulging model evolution, training, positioning, and monitoring.
Ensuring Artificial Intelligence systems administer data ethically and clearly remains an important challenge, requiring steady alertness and adjustment of governance structures to keep pace with technological progression.
Conclusion
To conclude the article, we have examined the critical role that data governance plays in utilizing the power of AI efficiently and legally. We explored pioneering a robust data governance structure, the complexities of handling data quality, and the significance of ensuring data lineage and traceability.
Furthermore, we accentuated the critical significance of maintaining the security and privacy of data, acknowledged the challenges of data ethics and bias mitigation, and discussed the thorough approach required for data governance across the AI lifespan.
The best practices and case studies give applicable insights and learnings from successful execution, emphasizing the practical phases of data governance in real-world scenarios.
Looking ahead, the arising challenges and future trends underline the robust nature of data governance, specifically in an era gradually conquered by AI inventiveness like synthetic data or federated learning.
For organizations intending to succeed in this AI-driven environment, the enforcement of sturdy data isn’t just advantageous; it is a requirement for imperishable success. Efficient data governance ensures that data stays safe, compliant and operable, which in turn, energizes AI systems that are strong, ethical and liable.
By following the practices, defined in this article, organizations can expect better decision-making abilities, improved working effectiveness, and an important fierce edge in the virtual age. Grasp these principles, and you will be fine on your way to unleashing the full potential of your data and AI investments.
Entering the age of AI without a solid data governance plan is like skydiving without a parachute – thrilling but perilous.
In the swiftly progressing field of Artificial Intelligence (AI), Governance and data handling play an important role. Efficient data governance ensures not only the precision and security of the data utilized for Artificial Intelligence but also its ethical sourcing and compliance with appropriate laws.
This guide explores the best practices in data governance for AI, providing a strategy for associations looking to utilize the power of AI responsibly and efficiently.
Introduction
Importance of Data Governance in AI
Data Governance is critical in AI because it ensures the incorporation, quality, and security of data in Artificial Intelligence systems. Efficient data governance assists in maintaining the precision and reliability of Artificial Intelligence outputs, significant for making informed choices and achieving desired results.
With the expanding dependency on Artificial Intelligence, Robust data governance structures protect against data misuse and ensure compliance with executive standards.
Challenges Of Data Management For AI Systems
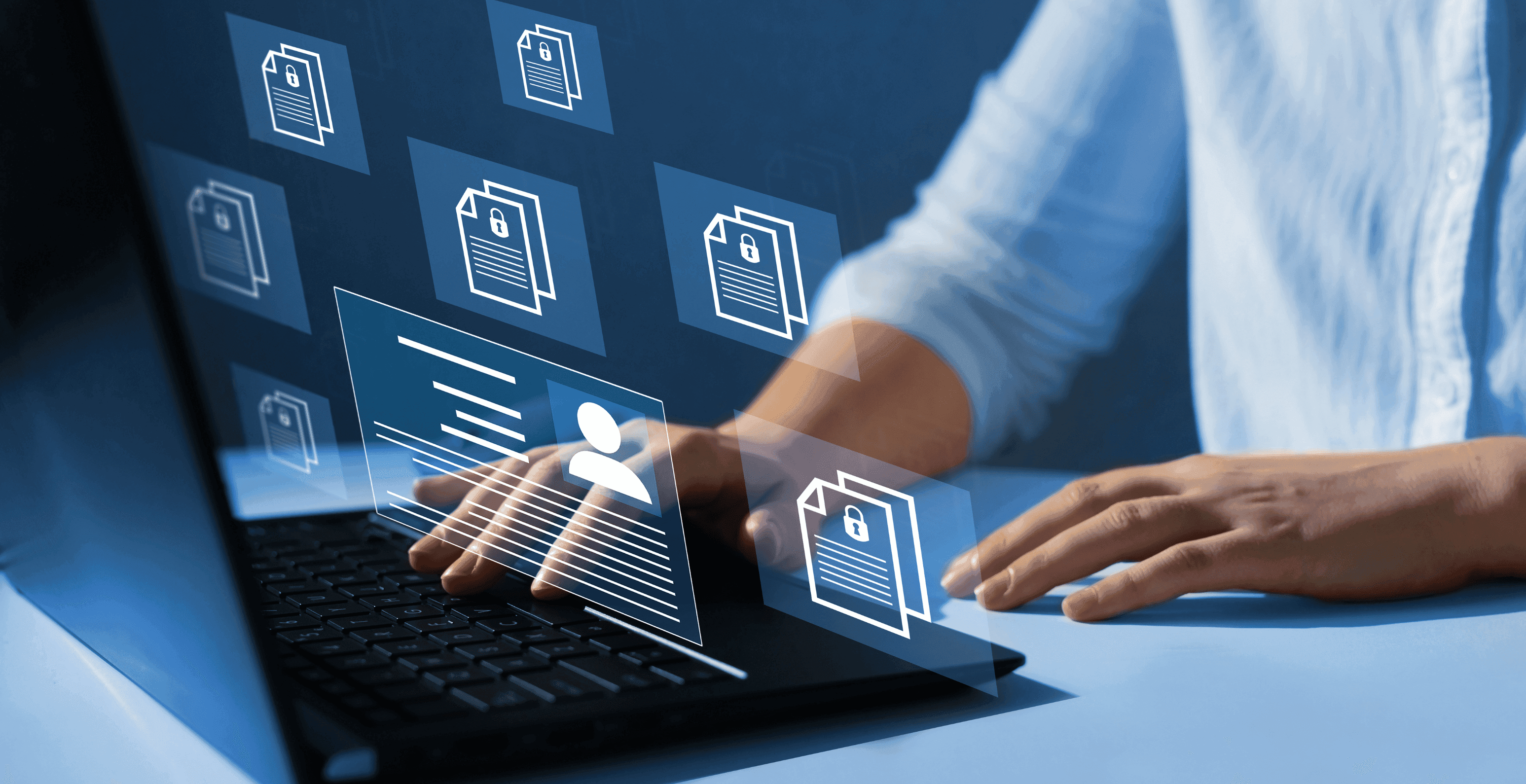
Data Management for Artificial Intelligence systems pose numerous challenges such as:
Data Quality and Consistency: Ensuring that data is precise, complete, and compatible is significant for training dependable Artificial Intelligence models.
Data Privacy and Security: Safeguarding sensitive data and maintaining assent with privacy laws such as GDPR and CCPA is crucial.
Data Integration and Silos: Incorporating data from numerous sources and breaking down data silos can be complicated but important for thorough analysis.
Ethical Considerations: Acknowledging tendencies in data and ensuring ethical utilization of Artificial Intelligence is crucial to avoid bigotry and unfair practices.
Scalability: Handling large dataset effectively and scaling systems to encompass evolving data requirements is significant for nourished AI performance.
Overview Of Data Governance Frameworks
Data governance structures offer structures approaches for handling data efficiently. These structures usually indulge:
Policies and Standards: Endowing recommendations for usage of data, security and privacy.
Roles and Responsibilities: Defining roles such as data housekeepers, data proprietors, and data caretakers to ensure liability.
Data Quality Management: Enforcing processes to monitor and enhance the quality of data.
Compliance and Audit: Ensuring compliance to legal and regulatory needs and imparting regular audits.
Data Lifecycle Management: Handling data from its formation to its removal, ensuring appropriate management at each stage.
Technology and Tools:- Using advanced technologies and tools for data handling, incorporation and dissecting.
Establishing A Data Governance Framework
Defining Organizational Data Strategy And Objectives
To begin, it’s important to clearly define your administrative data plans and purposes. This involves understanding the specific goals your institution aims to achieve through data governance.
Whether it's enhancing the quality of data, ensuring assent with regulations, or using data for strategic decision-making, having an explicit plan to provide a plan for all data-related activities.
Set Clear Goals: Decide what you want to accomplish with your data governance efforts. This could range from enhancing data precision to improving data security or upgrading data utilization for enhanced venture insights.
Align with Business Objectives: Ensure that your data plans correspond with your overall venture aims. This layout helps in giving priority to data capabilities that offer most value to an organization.
Develop a Data Management Plan: Summarize the processes and practices for handling data throughout its lifetime. This indulges data gathering, storage, usage and positioning.
Identifying Stakeholders and Roles
Successful data governance requires participation from various stakeholders across the association. Determining these stakeholders and clearly defining their roles is critical for the structure’s efficiency.
Identify Key Stakeholders: These usually include managers, data owners, data stewards, Information Technology staff and end-users. Each group has a suited interest in how data is handled and used.
Define Roles and Responsibilities: Evidently summarize the roles and responsibilities for each investor group. For instance, data stewards might be liable for handling data quality, while IT staff may manage data security.
Establish A Data Governance Council: Shape a governance council or committee that indulges deputies from key stakeholders groups. This council supervises the execution and administration of data governance policies.
Creating Data Governance Policies and Standards
Creating rigorous data governance policies and standards ensures that data is consistently and efficiently supervised across the association.
Develop Thorough Policies: These should cover numerous phases of data management, indulging the quality of data, data security, data seclusion and data lifespan handling. Policies should be transparent, applicable and executable.
Set Data Standards: Pioneer standards for data formats, naming customs, metadata handling, and data incorporation. Compatible standards help in maintaining the quality of data and conformity.
Implement Data Compliance Measures: Ensure that your strategies adhere with appropriate laws and standards, like GDPR or CCPA. Frequently check and update these strategies to stay compliant.
By adhering to these steps, your firm can pioneer a solid data governance structure that not only improves data management practices but also supports accomplishing strategic venture goals.
Data Quality Management
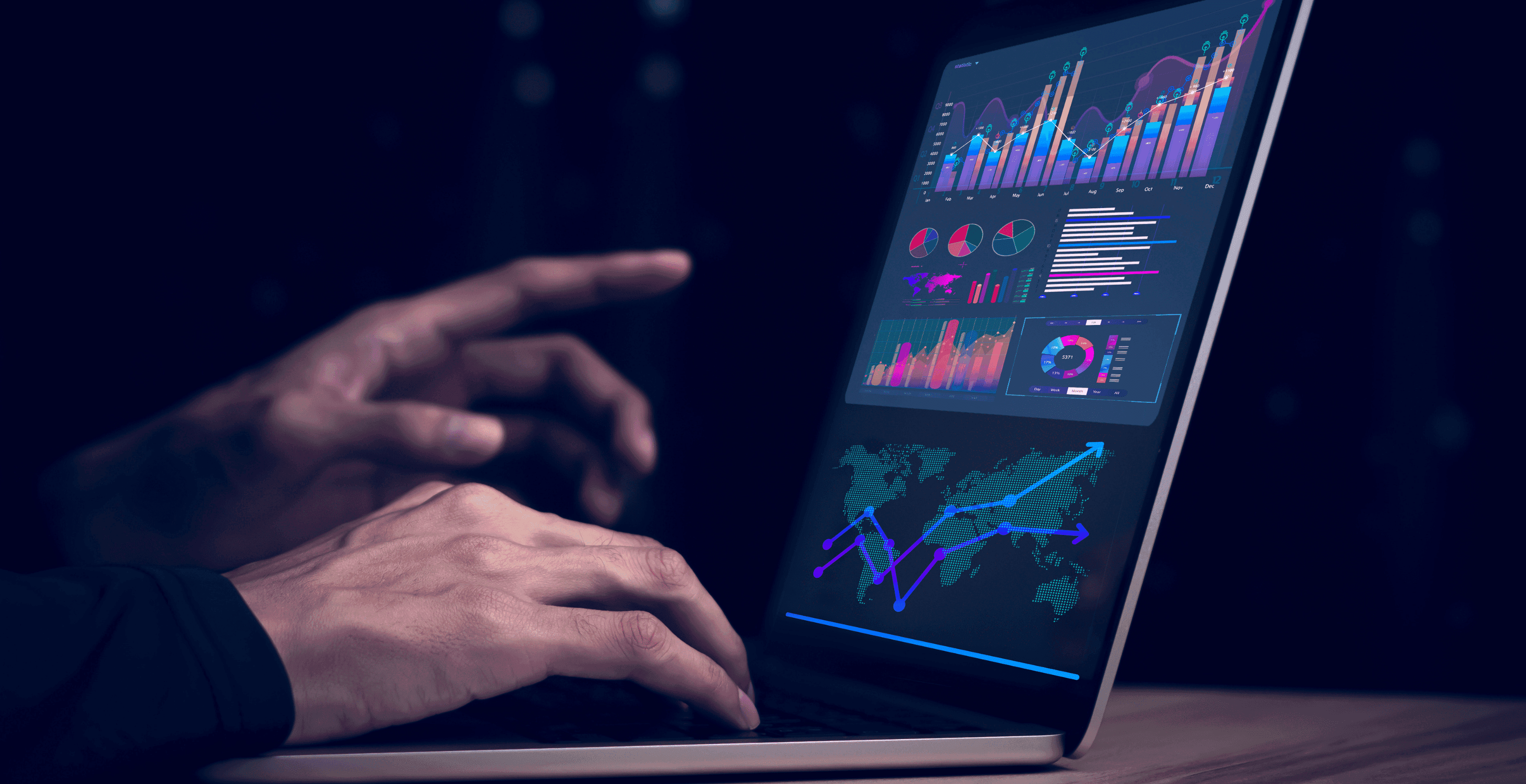
When managing data quality, organizations aim to ensure that their data is accurate, reliable, and useful for decision-making. Here’s an outline of chief aspects indulged in data-quality management:
Data Quality Dimensions
Accuracy: Refers to the accuracy of data after comparison with responsible sources or references. Accurate data appropriately imitates the real-world values it is aiming to represent.
Completeness: Covers to the limit to which all needed data is attainable. Incomplete data can result in examination that is possibly partial or misleading.
Consistency: Ensures that the information across varied datasets or information are compatible without any strife or modifications. Consistency is important for data to be dependable and trustworthy.
Data Profiling and Quality Assessment
Data Profiling: This is the initial step in comprehending the existing form of data. It indulges in investigating the data attainable and gathering statistics and information about that data. The process usually identifies problems with preciseness, distinctiveness, correspondence and entirety.
Quality Assessment: Continues from profiling, indulging more profound inspection to comprehend the root causes of data problems and to identify their effects on appropriate ventures processes. This indulges in setting standards for quality quality, estimating data against these criterias, and reporting the standing of data quality.
Data Cleansing And Transformation Processes
Data Cleansing:
Error Correction: Fixing mistakes determined in the data, like typos, spelling errors, or incorrect data entries.
Normalization: Normalizing the data structures, labels and data types to ensure correspondence across the dataset.
Missing Data Handling: Filling missing values through methods such as attribution or deciding to ignore or drop them based on the impact evaluation.
Data Transformation:
Data Integration: Merging data from various sources to give a unified view. This often indulges resolving incompatibilities and supporting the entirety of data.
Data Conversion: Transforming data from one format to another, usually to incorporate with other systems or to correspond with the needs of a new system.
Data Enrichment: Accelerating existing data with data from added sources to improve the data’s information or entirety.
Efficient data quality management ensures that data is a precious asset for decision-making, docility, and working procedures.
It needs constant efforts and incorporation into the daily data management processes to handle the quality of data throughout its lifespan.
Data Lineage and Traceability
Tracking Data Sources And Transformations
Understanding where data comes from and how it is transformed is crucial for maintaining the integrity of data throughout its lifespan.
Maintaining Metadata and Catalogs
Maintaining comprehensive metadata and data catalog is important for ensuring that data is easily attainable and understood by all investors.
Ensuring Data Provenance And Auditability
Data Provenance and ensuring auditability are important to follow with laws and maintain trust in Artificial Intelligence systems.
Data Security And Privacy
When discussing data safety and privacy, it’s crucial to contemplate numerous key aspects to ensure thorough security and assent. Below is an overview of the chief elements:
Data Protection And Access Control
Encryption: Encrypting data both at rest and in transit to safeguard it from improper access. This indulges using secure conventions such as HTTPS, SSL/TLS, and end-to-end encoding for contact.
Access Controls: Enforcing vigorous access control mechanisms like intricate validation (MFA), multifactor access control, and minimal advantage access strategies to ensure that only authorized individuals can ingress sensitive details.
Audit Trails: Handling comprehensive logs of data ingress and alterations to detect and retrospect how data is maintained and by whom. This helps in determining and replying to potent security infringements more efficiently.
Compliance With Regulations
Regulatory Understanding: Understanding the precise needs of regulations like the General Data Protection Regulation (GDPR) for European Union citizens, the Health Insurance Portability and Accountability Act (HIPAA), for health details in the United States, and other data safeguarding regulations.
Data Protection Officer (DPO): Designating a DPO to supervise data safeguarding plans, acquiescence with data safeguarding laws, and act as a point of connection for data subjects and regulatory authorities.
Data Subject Rights: Enforcing processes to acknowledge the data subject rights like the right to access, right to correction, right to erasure, and right to data flexibility.
Secure Data Handling And Storage Practices
Data Minimization: Gathering only the data that is important for the precise aim and reserving it only as long as it is required to serve those intentions.
Secure Data Storage Solutions: Using secure information and storage solutions that provides built-in security attributes such as automated backups, data repetition and safeguard against the loss of information.
Regular Audits and Penetration Testing: Managing regular security audits and penetration tests to determine and correct security susceptibilities. This also indulges upgrading and fixing software and systems to safeguard against known risks.
By following these practices, organizations can substantially improve their data security and privacy measures, ensuring that they not only safeguard sensitive details but also follow applicable laws, thereby handling trust and integrity in the digital ecosystem.
Data Ethics and Bias Mitigation
Ethical Principles For Data Collection And Use
Data gathering and utilization must follow ethical principles like neutrality, clarity, and respect for privacy. Associations should ensure that the data gathered is pertinent and significant for the contemplated purposes and gathered with specific permission from individuals.
Additionally, it’s important to inform participants about how their data will be utilized, ensuring their rights are safeguarded throughout the data lifespan.
Identifying And Mitigating Bias In Data
Partiality in data can result in uneven Artificial Intelligence results, affecting neutrality and effectiveness. To lessen this, it’s important to:
Determining potential sources of partiality across distinct phases of data gathering, refining and utilization.
Assort data sources to conceal a wide range of enumerations and synopsis.
Enforce bias detection methods like statistical examining and machine learning algorithms, to inspect and rectify partiality in data sets.
Fostering Transparency and Accountability
Transparency in Artificial Intelligence indulges in clear contact about how Artificial Intelligence systems operate, and the decision-making processes they adhere to. This can be accomplished by:
Establishing all data utilization and model decisions in a thorough manner.
Enforcing audit trails that trace data and selections for retrospection .
Engaging external audits to estimate AI practices and strategies.
Data Governance For AI Lifecycle
Data Governance in Model Development
Efficient data governance during model development ensures proper data use and that models are developed under appropriate guidelines. This indulges:
Endowing data quality standards to ensure data incorporation.
Regulating data access within the association to avert improper use.
Defining clear roles and authorities for team members indulged in data management and model development.
Data Governance in Model Deployment and Monitoring
Once models are deployed, constant monitoring is crucial to ensure they perform as intended and adhere to ethical standards. Data governance in this stage indulges:
Observing the performance of the model for preciseness and neutrality in real-time.
Modernizing data-management etiquettes as new data is gathered and as external circumstances change.
Ensuring model security against external risks and internal embezzle.
Continuous Improvement And Feedback Loops
The lifespan of Artificial Intelligence systems indulge apparatus for constant improvement:-
Feedback Loops that permits users to report problems or suggest enhancements.
Frequent updates to models based on new data, commuting circumstances and feedback.
Ongoing training for Artificial Intelligence teams on the newest technologies, ethical instructions, and governance etiquettes.
These details ensure that Artificial Intelligence systems are established and worked in a manner that is ethical, clear, accountable, and constantly enhancing, thus nurturing trust and dependability in AI applications.
Organizational Readiness and Change Management
Establishing Data Governance Roles And Responsibilities
To manage data effectively as a strategic asset, clear roles and responsibilities must be defined within the organization. This indulges setting up a data governance structure that indulges roles like Data Proprietors, Data Stewards, and Data keepers. Each role should have transparently defined duties:-
Data Proprietors are usually senior managers who are responsible for data quality and security in their respective areas.
Data Stewards are liable for the handling and fitness of data components, both content and metadata.
Data Keepers manage the technical aspect and directory handling, ensuring data is attainable yet secure.
Training and Awareness Programs
Training and Awareness programs are crucial for equipping team members with the necessary skills and knowledge to manage data responsibly.
These programs should cover topics like data privacy, data security strategies, and the significance of the data quality, and the organization’s precise data governance practices.
Frequent training classes ensure that all workers comprehend their roles in data governance and are up-to-date with the newest data handling procedures.
Fostering A Data-Driven Culture
Creating a data-driven culture requires more than just tools and policies; it requires a shift in attitude at every level of the organization.
Fostering open conversation about information, praising data-driven successes, and integrating data-based decision-making in daily working are all efficient strategies. Leaders must also master data governance by setting instances and constantly executing the value of data-driven insights.
Tools and Technologies for Data Governance
Data Catalogs and Metadata Management
Data Catalogs play a critical role in providing a single source of reference for organizational data assets, helping users find the data they need for their requirements.
These tools often trait metadata handling abilities that permits for analysis, classification, and concealment of data, making data more comprehensible and functional.
Metadata Management is crucial for comprehending data within its conditions, indulging its source, frameworks, reliabilities, or utilization policies.
Data Quality and Lineage Tools
Maintaining high data quality is essential for effective data governance. Tools for data quality ensures that data is precise, transparent and dependable. In addition, data lineage tools offers a transparent view of the data journey through the association, helping to trace the origin, movement or features and quality of data:
Data Lineage Tools help associations design the data lifespan, inspecting how data transforms, and derives from source to end of the line, which is critical for resolving and docility.
Data Governance Platforms and Solutions
Comprehensive data governance platforms include various functionalities to support data quality, compliance, policy management, and more. These platforms often indulge:
Automated Governance Tools that help in executing data governance strategies spontaneously across numerous data stores.
Integrated Solutions that gives an amalgamate of data cataloging, quality control, and lineage tracking, giving a comprehensive approach to data governance.
Enforcing these tools and technologies, besides a vigorous governing receptivity and change management plans, lays a powerful foundation for efficient data governance.
This planned approach ensures that data is handled as a precious asset, leading to enhanced working effectiveness, compliance and choice making abilities.
Now, let's dive into the world of case studies, where we'll see these best practices spring to life and truly make an impact.
Best Practices and Case Studies
Successful Data Governance Implementations
In successful data governance implementations, comprehensive data management procedures ensure that data remains accurate, accessible, and secure. For example, a reputable financial institution enforced a consolidated data governance structure that indulged organized data access rules and pioneering clear data possession.
They used metadata management tools to maintain a constant data dictionary, which substantially decreased the repetition of data and enhanced data quality across departments. This institution also engaged data lineage tools to track data from its source through its lifespan, improving clarity and responsibility.
Lessons Learned and Pitfalls to Avoid
Prevalent pitfalls in data governance indulges in minimizing the significance of organizational lifestyle and investor engagement. A case in point indulges a MNC that encountered resistance when enforcing a new governance program because it didn’t properly indulge predominant investors in the strategy stage.
This mistake led to a lack of continuity between ventures' aims and data practices, leading to poor adoption rates. Lessons learned features the significance of appealing to all investors early in the procedures, ensuring governance strategies align with actual assignment, and constantly teaching employees about the advantages of proper data handling.
Future Trends and Challenges
Emerging Data Governance Challenges
As technology progresses, data governance faces new difficulties like handling artificial data and co-operated learning environments. Artificial Data, propounds unique difficulties in ensuring the data imitates precise and impartial details.
Meanwhile, federated learning, a machine learning technique that trains computations across disparate devices or servers without swapping the data itself, presents intricacies in handling data privacy and security.
Governance plans must develop to acknowledge these problems, ensuring vigorous protocols for data verification and model clarity are in place.
Incorporating AI and Machine Learning in Data Governance
The incorporation of Artificial Intelligence and machine learning in data governance is becoming progressively predominant. AI can mechanize intricate data management tasks like data classification, anomaly detection, and predictive maintenance.
For example, AI driven tools can strongly adapt access controls and data quality regulations based on ongoing data utilization patterns and compliance needs. Hence, integrating these technologies requires robust structures to handle the AI lifespan, indulging model evolution, training, positioning, and monitoring.
Ensuring Artificial Intelligence systems administer data ethically and clearly remains an important challenge, requiring steady alertness and adjustment of governance structures to keep pace with technological progression.
Conclusion
To conclude the article, we have examined the critical role that data governance plays in utilizing the power of AI efficiently and legally. We explored pioneering a robust data governance structure, the complexities of handling data quality, and the significance of ensuring data lineage and traceability.
Furthermore, we accentuated the critical significance of maintaining the security and privacy of data, acknowledged the challenges of data ethics and bias mitigation, and discussed the thorough approach required for data governance across the AI lifespan.
The best practices and case studies give applicable insights and learnings from successful execution, emphasizing the practical phases of data governance in real-world scenarios.
Looking ahead, the arising challenges and future trends underline the robust nature of data governance, specifically in an era gradually conquered by AI inventiveness like synthetic data or federated learning.
For organizations intending to succeed in this AI-driven environment, the enforcement of sturdy data isn’t just advantageous; it is a requirement for imperishable success. Efficient data governance ensures that data stays safe, compliant and operable, which in turn, energizes AI systems that are strong, ethical and liable.
By following the practices, defined in this article, organizations can expect better decision-making abilities, improved working effectiveness, and an important fierce edge in the virtual age. Grasp these principles, and you will be fine on your way to unleashing the full potential of your data and AI investments.
Entering the age of AI without a solid data governance plan is like skydiving without a parachute – thrilling but perilous.
In the swiftly progressing field of Artificial Intelligence (AI), Governance and data handling play an important role. Efficient data governance ensures not only the precision and security of the data utilized for Artificial Intelligence but also its ethical sourcing and compliance with appropriate laws.
This guide explores the best practices in data governance for AI, providing a strategy for associations looking to utilize the power of AI responsibly and efficiently.
Introduction
Importance of Data Governance in AI
Data Governance is critical in AI because it ensures the incorporation, quality, and security of data in Artificial Intelligence systems. Efficient data governance assists in maintaining the precision and reliability of Artificial Intelligence outputs, significant for making informed choices and achieving desired results.
With the expanding dependency on Artificial Intelligence, Robust data governance structures protect against data misuse and ensure compliance with executive standards.
Challenges Of Data Management For AI Systems
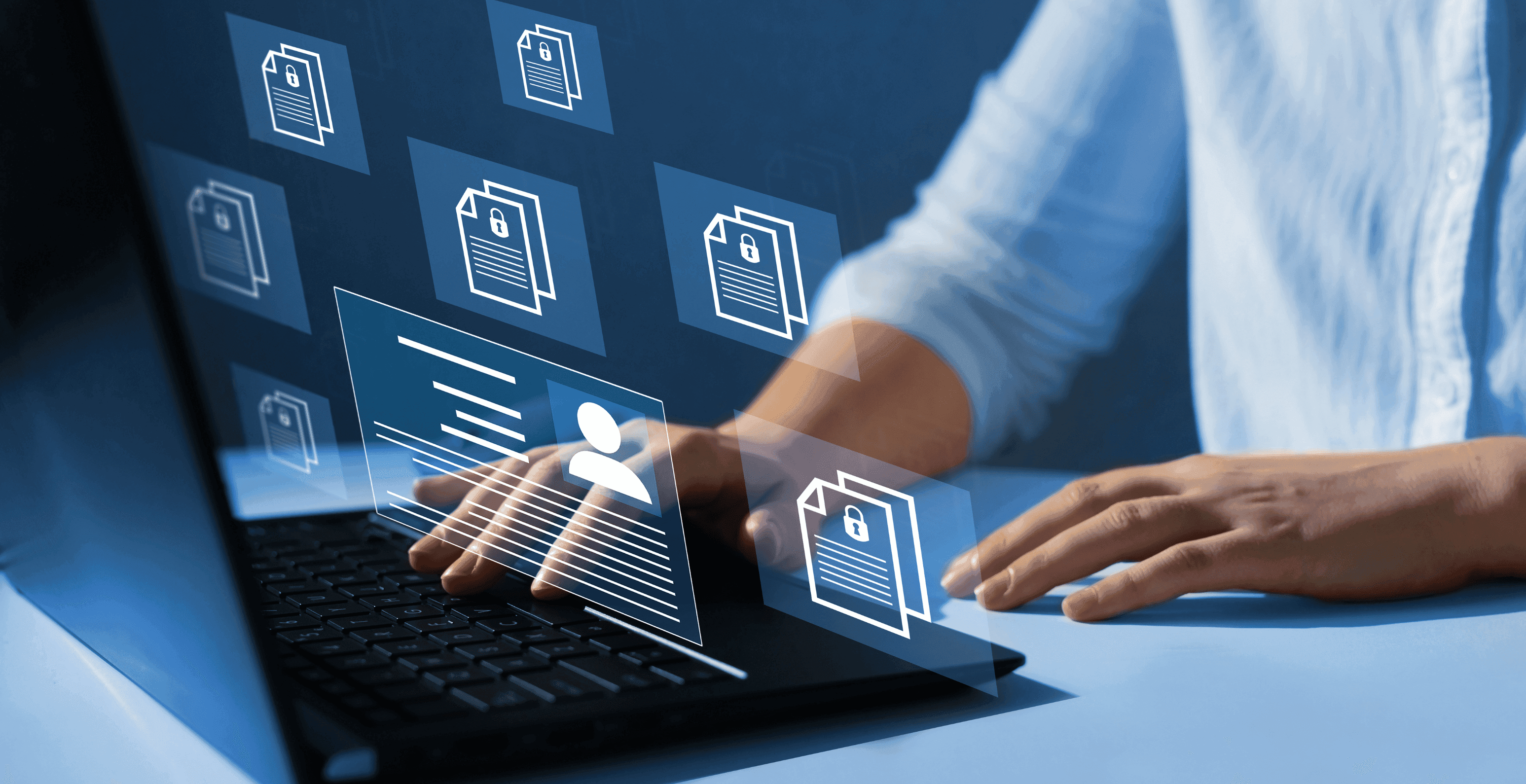
Data Management for Artificial Intelligence systems pose numerous challenges such as:
Data Quality and Consistency: Ensuring that data is precise, complete, and compatible is significant for training dependable Artificial Intelligence models.
Data Privacy and Security: Safeguarding sensitive data and maintaining assent with privacy laws such as GDPR and CCPA is crucial.
Data Integration and Silos: Incorporating data from numerous sources and breaking down data silos can be complicated but important for thorough analysis.
Ethical Considerations: Acknowledging tendencies in data and ensuring ethical utilization of Artificial Intelligence is crucial to avoid bigotry and unfair practices.
Scalability: Handling large dataset effectively and scaling systems to encompass evolving data requirements is significant for nourished AI performance.
Overview Of Data Governance Frameworks
Data governance structures offer structures approaches for handling data efficiently. These structures usually indulge:
Policies and Standards: Endowing recommendations for usage of data, security and privacy.
Roles and Responsibilities: Defining roles such as data housekeepers, data proprietors, and data caretakers to ensure liability.
Data Quality Management: Enforcing processes to monitor and enhance the quality of data.
Compliance and Audit: Ensuring compliance to legal and regulatory needs and imparting regular audits.
Data Lifecycle Management: Handling data from its formation to its removal, ensuring appropriate management at each stage.
Technology and Tools:- Using advanced technologies and tools for data handling, incorporation and dissecting.
Establishing A Data Governance Framework
Defining Organizational Data Strategy And Objectives
To begin, it’s important to clearly define your administrative data plans and purposes. This involves understanding the specific goals your institution aims to achieve through data governance.
Whether it's enhancing the quality of data, ensuring assent with regulations, or using data for strategic decision-making, having an explicit plan to provide a plan for all data-related activities.
Set Clear Goals: Decide what you want to accomplish with your data governance efforts. This could range from enhancing data precision to improving data security or upgrading data utilization for enhanced venture insights.
Align with Business Objectives: Ensure that your data plans correspond with your overall venture aims. This layout helps in giving priority to data capabilities that offer most value to an organization.
Develop a Data Management Plan: Summarize the processes and practices for handling data throughout its lifetime. This indulges data gathering, storage, usage and positioning.
Identifying Stakeholders and Roles
Successful data governance requires participation from various stakeholders across the association. Determining these stakeholders and clearly defining their roles is critical for the structure’s efficiency.
Identify Key Stakeholders: These usually include managers, data owners, data stewards, Information Technology staff and end-users. Each group has a suited interest in how data is handled and used.
Define Roles and Responsibilities: Evidently summarize the roles and responsibilities for each investor group. For instance, data stewards might be liable for handling data quality, while IT staff may manage data security.
Establish A Data Governance Council: Shape a governance council or committee that indulges deputies from key stakeholders groups. This council supervises the execution and administration of data governance policies.
Creating Data Governance Policies and Standards
Creating rigorous data governance policies and standards ensures that data is consistently and efficiently supervised across the association.
Develop Thorough Policies: These should cover numerous phases of data management, indulging the quality of data, data security, data seclusion and data lifespan handling. Policies should be transparent, applicable and executable.
Set Data Standards: Pioneer standards for data formats, naming customs, metadata handling, and data incorporation. Compatible standards help in maintaining the quality of data and conformity.
Implement Data Compliance Measures: Ensure that your strategies adhere with appropriate laws and standards, like GDPR or CCPA. Frequently check and update these strategies to stay compliant.
By adhering to these steps, your firm can pioneer a solid data governance structure that not only improves data management practices but also supports accomplishing strategic venture goals.
Data Quality Management
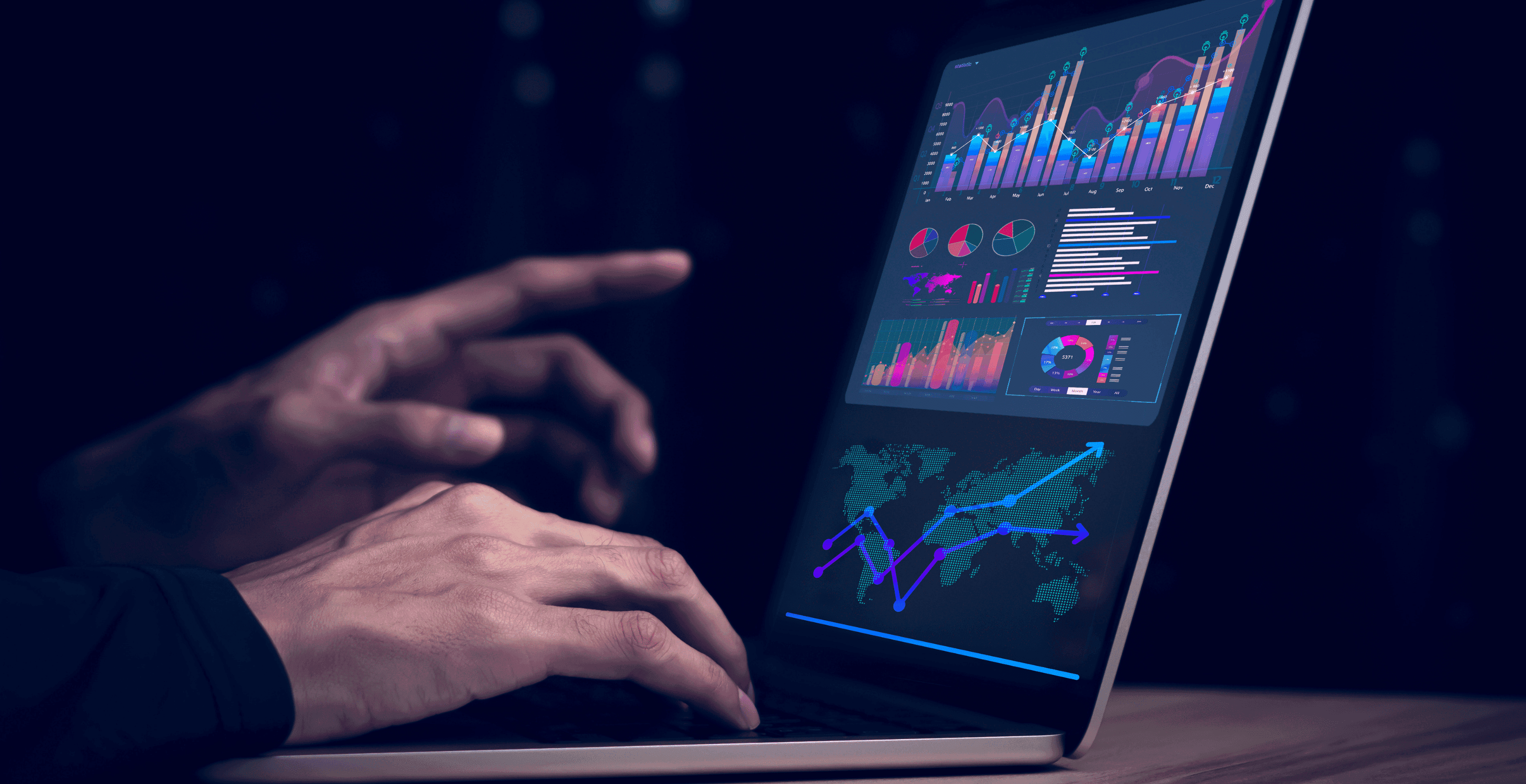
When managing data quality, organizations aim to ensure that their data is accurate, reliable, and useful for decision-making. Here’s an outline of chief aspects indulged in data-quality management:
Data Quality Dimensions
Accuracy: Refers to the accuracy of data after comparison with responsible sources or references. Accurate data appropriately imitates the real-world values it is aiming to represent.
Completeness: Covers to the limit to which all needed data is attainable. Incomplete data can result in examination that is possibly partial or misleading.
Consistency: Ensures that the information across varied datasets or information are compatible without any strife or modifications. Consistency is important for data to be dependable and trustworthy.
Data Profiling and Quality Assessment
Data Profiling: This is the initial step in comprehending the existing form of data. It indulges in investigating the data attainable and gathering statistics and information about that data. The process usually identifies problems with preciseness, distinctiveness, correspondence and entirety.
Quality Assessment: Continues from profiling, indulging more profound inspection to comprehend the root causes of data problems and to identify their effects on appropriate ventures processes. This indulges in setting standards for quality quality, estimating data against these criterias, and reporting the standing of data quality.
Data Cleansing And Transformation Processes
Data Cleansing:
Error Correction: Fixing mistakes determined in the data, like typos, spelling errors, or incorrect data entries.
Normalization: Normalizing the data structures, labels and data types to ensure correspondence across the dataset.
Missing Data Handling: Filling missing values through methods such as attribution or deciding to ignore or drop them based on the impact evaluation.
Data Transformation:
Data Integration: Merging data from various sources to give a unified view. This often indulges resolving incompatibilities and supporting the entirety of data.
Data Conversion: Transforming data from one format to another, usually to incorporate with other systems or to correspond with the needs of a new system.
Data Enrichment: Accelerating existing data with data from added sources to improve the data’s information or entirety.
Efficient data quality management ensures that data is a precious asset for decision-making, docility, and working procedures.
It needs constant efforts and incorporation into the daily data management processes to handle the quality of data throughout its lifespan.
Data Lineage and Traceability
Tracking Data Sources And Transformations
Understanding where data comes from and how it is transformed is crucial for maintaining the integrity of data throughout its lifespan.
Maintaining Metadata and Catalogs
Maintaining comprehensive metadata and data catalog is important for ensuring that data is easily attainable and understood by all investors.
Ensuring Data Provenance And Auditability
Data Provenance and ensuring auditability are important to follow with laws and maintain trust in Artificial Intelligence systems.
Data Security And Privacy
When discussing data safety and privacy, it’s crucial to contemplate numerous key aspects to ensure thorough security and assent. Below is an overview of the chief elements:
Data Protection And Access Control
Encryption: Encrypting data both at rest and in transit to safeguard it from improper access. This indulges using secure conventions such as HTTPS, SSL/TLS, and end-to-end encoding for contact.
Access Controls: Enforcing vigorous access control mechanisms like intricate validation (MFA), multifactor access control, and minimal advantage access strategies to ensure that only authorized individuals can ingress sensitive details.
Audit Trails: Handling comprehensive logs of data ingress and alterations to detect and retrospect how data is maintained and by whom. This helps in determining and replying to potent security infringements more efficiently.
Compliance With Regulations
Regulatory Understanding: Understanding the precise needs of regulations like the General Data Protection Regulation (GDPR) for European Union citizens, the Health Insurance Portability and Accountability Act (HIPAA), for health details in the United States, and other data safeguarding regulations.
Data Protection Officer (DPO): Designating a DPO to supervise data safeguarding plans, acquiescence with data safeguarding laws, and act as a point of connection for data subjects and regulatory authorities.
Data Subject Rights: Enforcing processes to acknowledge the data subject rights like the right to access, right to correction, right to erasure, and right to data flexibility.
Secure Data Handling And Storage Practices
Data Minimization: Gathering only the data that is important for the precise aim and reserving it only as long as it is required to serve those intentions.
Secure Data Storage Solutions: Using secure information and storage solutions that provides built-in security attributes such as automated backups, data repetition and safeguard against the loss of information.
Regular Audits and Penetration Testing: Managing regular security audits and penetration tests to determine and correct security susceptibilities. This also indulges upgrading and fixing software and systems to safeguard against known risks.
By following these practices, organizations can substantially improve their data security and privacy measures, ensuring that they not only safeguard sensitive details but also follow applicable laws, thereby handling trust and integrity in the digital ecosystem.
Data Ethics and Bias Mitigation
Ethical Principles For Data Collection And Use
Data gathering and utilization must follow ethical principles like neutrality, clarity, and respect for privacy. Associations should ensure that the data gathered is pertinent and significant for the contemplated purposes and gathered with specific permission from individuals.
Additionally, it’s important to inform participants about how their data will be utilized, ensuring their rights are safeguarded throughout the data lifespan.
Identifying And Mitigating Bias In Data
Partiality in data can result in uneven Artificial Intelligence results, affecting neutrality and effectiveness. To lessen this, it’s important to:
Determining potential sources of partiality across distinct phases of data gathering, refining and utilization.
Assort data sources to conceal a wide range of enumerations and synopsis.
Enforce bias detection methods like statistical examining and machine learning algorithms, to inspect and rectify partiality in data sets.
Fostering Transparency and Accountability
Transparency in Artificial Intelligence indulges in clear contact about how Artificial Intelligence systems operate, and the decision-making processes they adhere to. This can be accomplished by:
Establishing all data utilization and model decisions in a thorough manner.
Enforcing audit trails that trace data and selections for retrospection .
Engaging external audits to estimate AI practices and strategies.
Data Governance For AI Lifecycle
Data Governance in Model Development
Efficient data governance during model development ensures proper data use and that models are developed under appropriate guidelines. This indulges:
Endowing data quality standards to ensure data incorporation.
Regulating data access within the association to avert improper use.
Defining clear roles and authorities for team members indulged in data management and model development.
Data Governance in Model Deployment and Monitoring
Once models are deployed, constant monitoring is crucial to ensure they perform as intended and adhere to ethical standards. Data governance in this stage indulges:
Observing the performance of the model for preciseness and neutrality in real-time.
Modernizing data-management etiquettes as new data is gathered and as external circumstances change.
Ensuring model security against external risks and internal embezzle.
Continuous Improvement And Feedback Loops
The lifespan of Artificial Intelligence systems indulge apparatus for constant improvement:-
Feedback Loops that permits users to report problems or suggest enhancements.
Frequent updates to models based on new data, commuting circumstances and feedback.
Ongoing training for Artificial Intelligence teams on the newest technologies, ethical instructions, and governance etiquettes.
These details ensure that Artificial Intelligence systems are established and worked in a manner that is ethical, clear, accountable, and constantly enhancing, thus nurturing trust and dependability in AI applications.
Organizational Readiness and Change Management
Establishing Data Governance Roles And Responsibilities
To manage data effectively as a strategic asset, clear roles and responsibilities must be defined within the organization. This indulges setting up a data governance structure that indulges roles like Data Proprietors, Data Stewards, and Data keepers. Each role should have transparently defined duties:-
Data Proprietors are usually senior managers who are responsible for data quality and security in their respective areas.
Data Stewards are liable for the handling and fitness of data components, both content and metadata.
Data Keepers manage the technical aspect and directory handling, ensuring data is attainable yet secure.
Training and Awareness Programs
Training and Awareness programs are crucial for equipping team members with the necessary skills and knowledge to manage data responsibly.
These programs should cover topics like data privacy, data security strategies, and the significance of the data quality, and the organization’s precise data governance practices.
Frequent training classes ensure that all workers comprehend their roles in data governance and are up-to-date with the newest data handling procedures.
Fostering A Data-Driven Culture
Creating a data-driven culture requires more than just tools and policies; it requires a shift in attitude at every level of the organization.
Fostering open conversation about information, praising data-driven successes, and integrating data-based decision-making in daily working are all efficient strategies. Leaders must also master data governance by setting instances and constantly executing the value of data-driven insights.
Tools and Technologies for Data Governance
Data Catalogs and Metadata Management
Data Catalogs play a critical role in providing a single source of reference for organizational data assets, helping users find the data they need for their requirements.
These tools often trait metadata handling abilities that permits for analysis, classification, and concealment of data, making data more comprehensible and functional.
Metadata Management is crucial for comprehending data within its conditions, indulging its source, frameworks, reliabilities, or utilization policies.
Data Quality and Lineage Tools
Maintaining high data quality is essential for effective data governance. Tools for data quality ensures that data is precise, transparent and dependable. In addition, data lineage tools offers a transparent view of the data journey through the association, helping to trace the origin, movement or features and quality of data:
Data Lineage Tools help associations design the data lifespan, inspecting how data transforms, and derives from source to end of the line, which is critical for resolving and docility.
Data Governance Platforms and Solutions
Comprehensive data governance platforms include various functionalities to support data quality, compliance, policy management, and more. These platforms often indulge:
Automated Governance Tools that help in executing data governance strategies spontaneously across numerous data stores.
Integrated Solutions that gives an amalgamate of data cataloging, quality control, and lineage tracking, giving a comprehensive approach to data governance.
Enforcing these tools and technologies, besides a vigorous governing receptivity and change management plans, lays a powerful foundation for efficient data governance.
This planned approach ensures that data is handled as a precious asset, leading to enhanced working effectiveness, compliance and choice making abilities.
Now, let's dive into the world of case studies, where we'll see these best practices spring to life and truly make an impact.
Best Practices and Case Studies
Successful Data Governance Implementations
In successful data governance implementations, comprehensive data management procedures ensure that data remains accurate, accessible, and secure. For example, a reputable financial institution enforced a consolidated data governance structure that indulged organized data access rules and pioneering clear data possession.
They used metadata management tools to maintain a constant data dictionary, which substantially decreased the repetition of data and enhanced data quality across departments. This institution also engaged data lineage tools to track data from its source through its lifespan, improving clarity and responsibility.
Lessons Learned and Pitfalls to Avoid
Prevalent pitfalls in data governance indulges in minimizing the significance of organizational lifestyle and investor engagement. A case in point indulges a MNC that encountered resistance when enforcing a new governance program because it didn’t properly indulge predominant investors in the strategy stage.
This mistake led to a lack of continuity between ventures' aims and data practices, leading to poor adoption rates. Lessons learned features the significance of appealing to all investors early in the procedures, ensuring governance strategies align with actual assignment, and constantly teaching employees about the advantages of proper data handling.
Future Trends and Challenges
Emerging Data Governance Challenges
As technology progresses, data governance faces new difficulties like handling artificial data and co-operated learning environments. Artificial Data, propounds unique difficulties in ensuring the data imitates precise and impartial details.
Meanwhile, federated learning, a machine learning technique that trains computations across disparate devices or servers without swapping the data itself, presents intricacies in handling data privacy and security.
Governance plans must develop to acknowledge these problems, ensuring vigorous protocols for data verification and model clarity are in place.
Incorporating AI and Machine Learning in Data Governance
The incorporation of Artificial Intelligence and machine learning in data governance is becoming progressively predominant. AI can mechanize intricate data management tasks like data classification, anomaly detection, and predictive maintenance.
For example, AI driven tools can strongly adapt access controls and data quality regulations based on ongoing data utilization patterns and compliance needs. Hence, integrating these technologies requires robust structures to handle the AI lifespan, indulging model evolution, training, positioning, and monitoring.
Ensuring Artificial Intelligence systems administer data ethically and clearly remains an important challenge, requiring steady alertness and adjustment of governance structures to keep pace with technological progression.
Conclusion
To conclude the article, we have examined the critical role that data governance plays in utilizing the power of AI efficiently and legally. We explored pioneering a robust data governance structure, the complexities of handling data quality, and the significance of ensuring data lineage and traceability.
Furthermore, we accentuated the critical significance of maintaining the security and privacy of data, acknowledged the challenges of data ethics and bias mitigation, and discussed the thorough approach required for data governance across the AI lifespan.
The best practices and case studies give applicable insights and learnings from successful execution, emphasizing the practical phases of data governance in real-world scenarios.
Looking ahead, the arising challenges and future trends underline the robust nature of data governance, specifically in an era gradually conquered by AI inventiveness like synthetic data or federated learning.
For organizations intending to succeed in this AI-driven environment, the enforcement of sturdy data isn’t just advantageous; it is a requirement for imperishable success. Efficient data governance ensures that data stays safe, compliant and operable, which in turn, energizes AI systems that are strong, ethical and liable.
By following the practices, defined in this article, organizations can expect better decision-making abilities, improved working effectiveness, and an important fierce edge in the virtual age. Grasp these principles, and you will be fine on your way to unleashing the full potential of your data and AI investments.
Subscribe to our newsletter to never miss an update
Other articles


Agents Talk, Models Think: A2A + MCP for Enterprise Agentic AI

Riya Parikh, Nitai Agarwal
Apr 27, 2025
Read the article
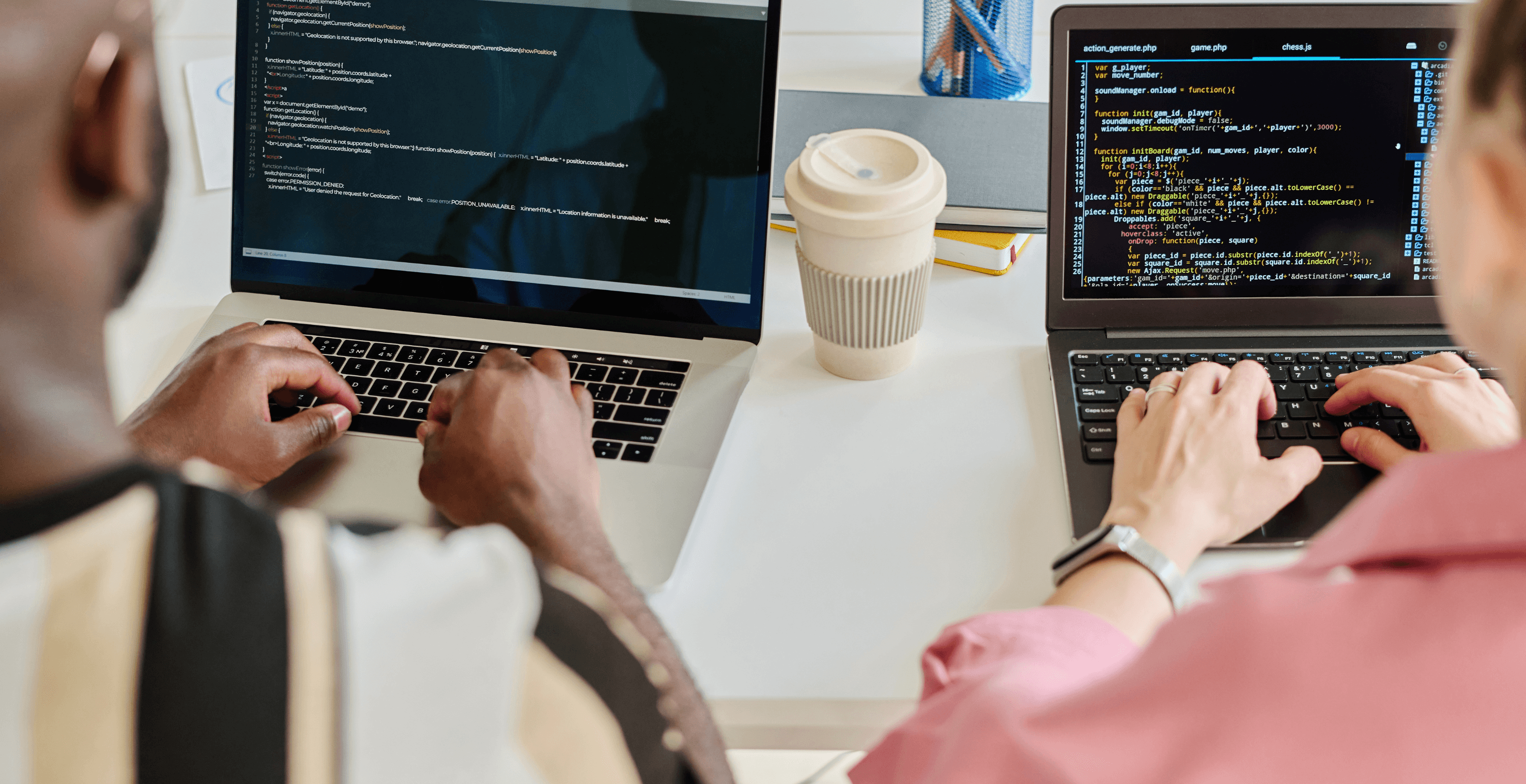
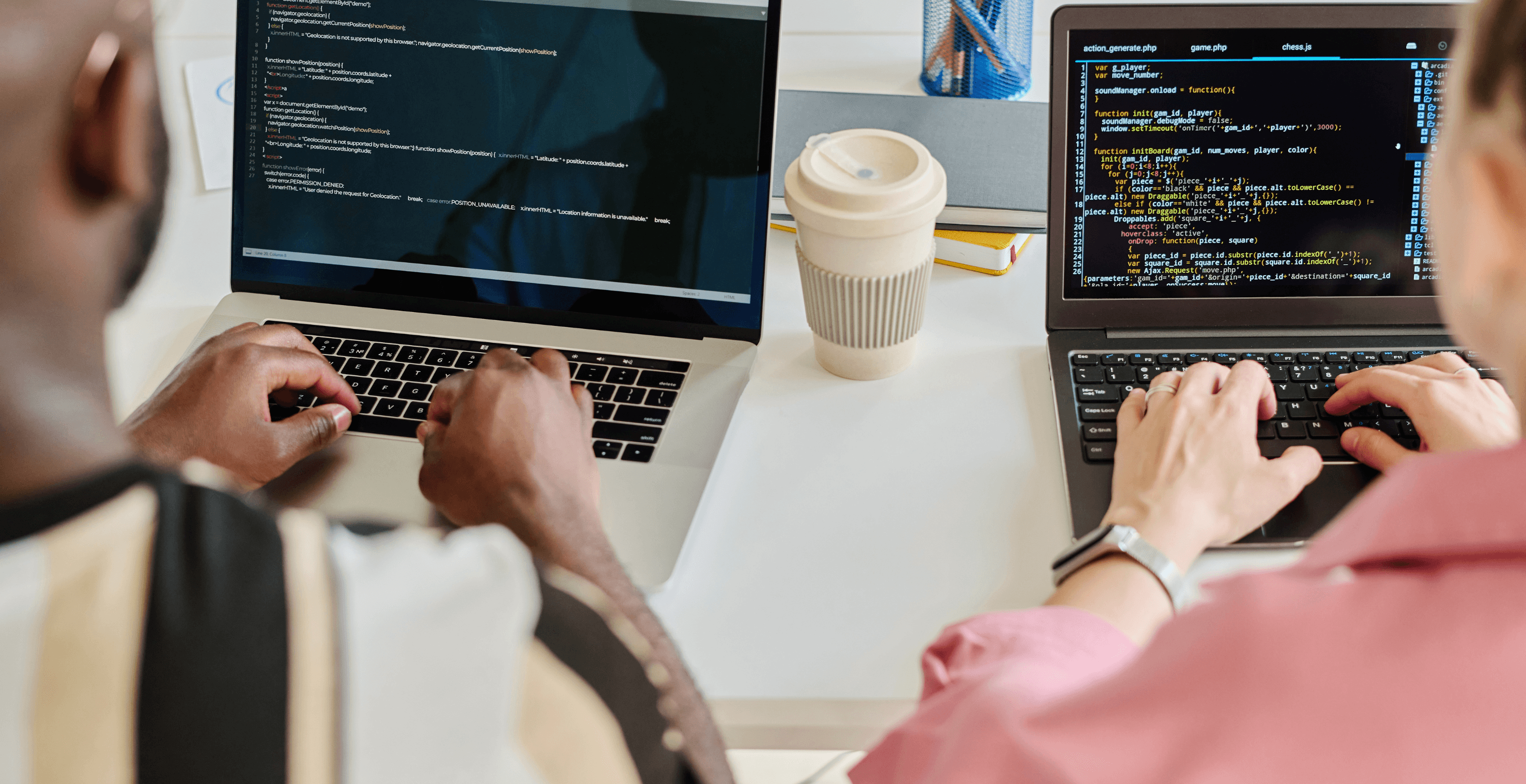
Understanding NeMo Guardrails: A Toolkit for LLM Security

Rehan Asif
Dec 24, 2024
Read the article


Understanding Differences in Large vs Small Language Models (LLM vs SLM)

Rehan Asif
Dec 21, 2024
Read the article