Understanding and Tackling Data Drift: Causes, Impact, and Automation Strategies
Understanding and Tackling Data Drift: Causes, Impact, and Automation Strategies
Understanding and Tackling Data Drift: Causes, Impact, and Automation Strategies
Jigar Gupta
Aug 14, 2024
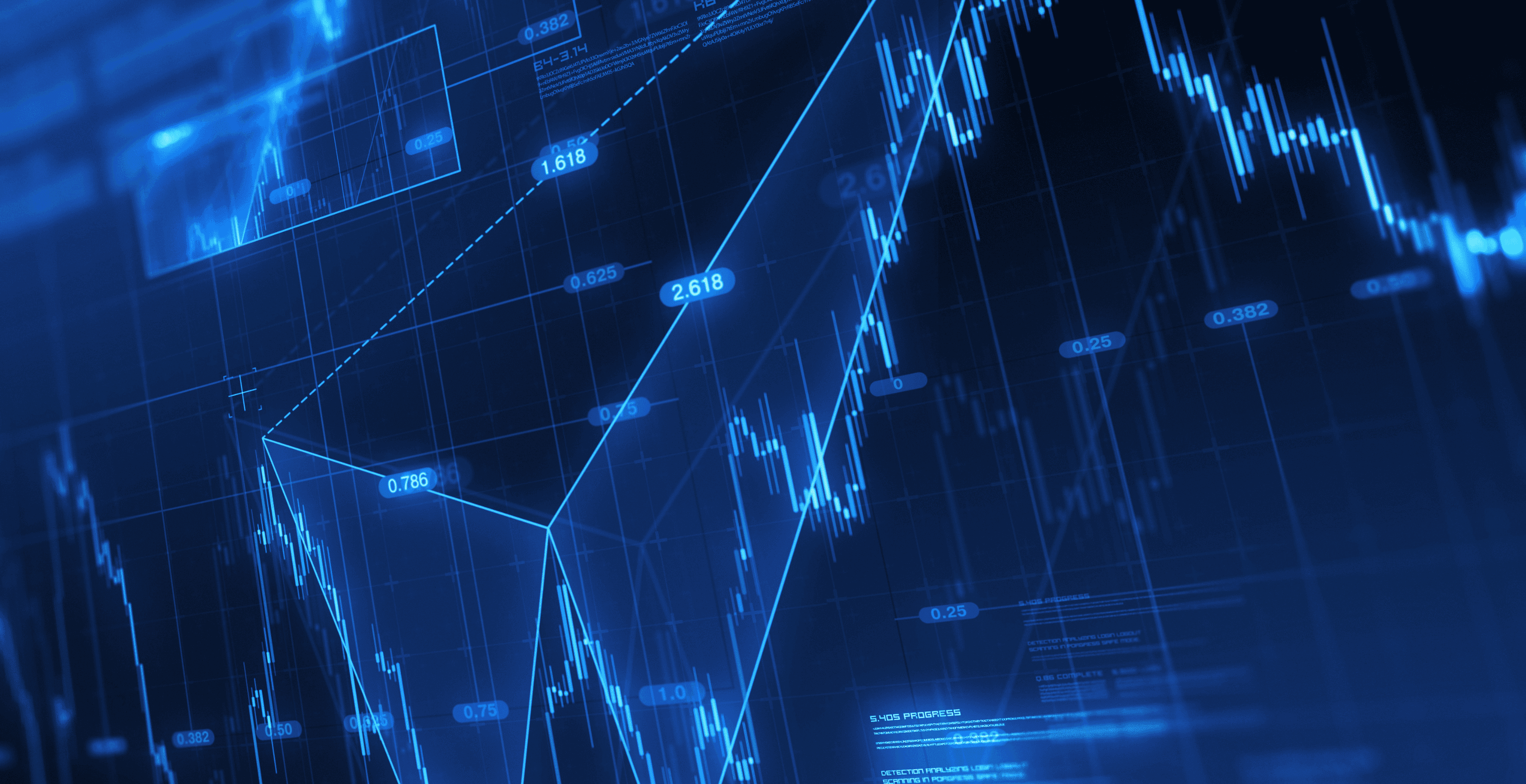
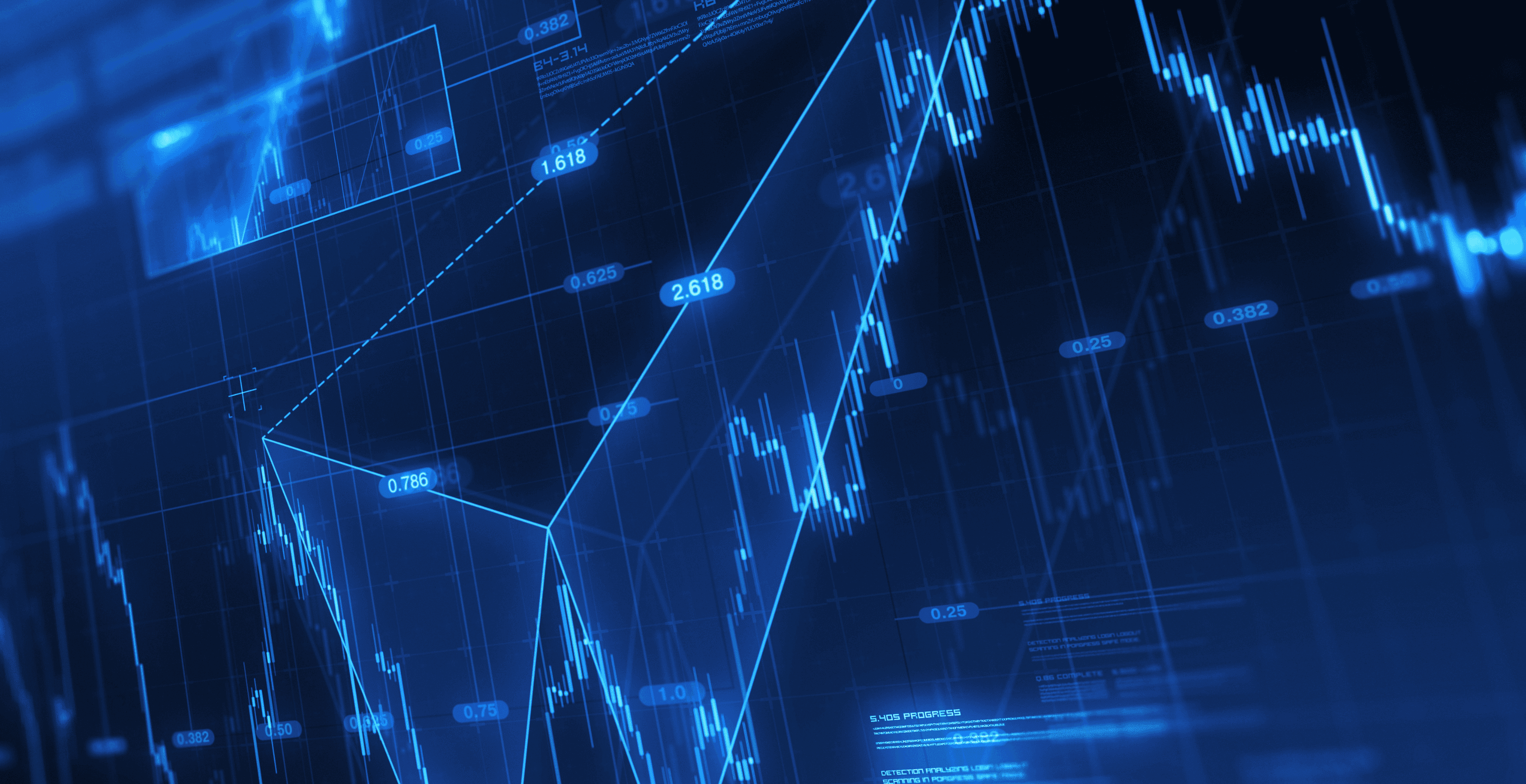
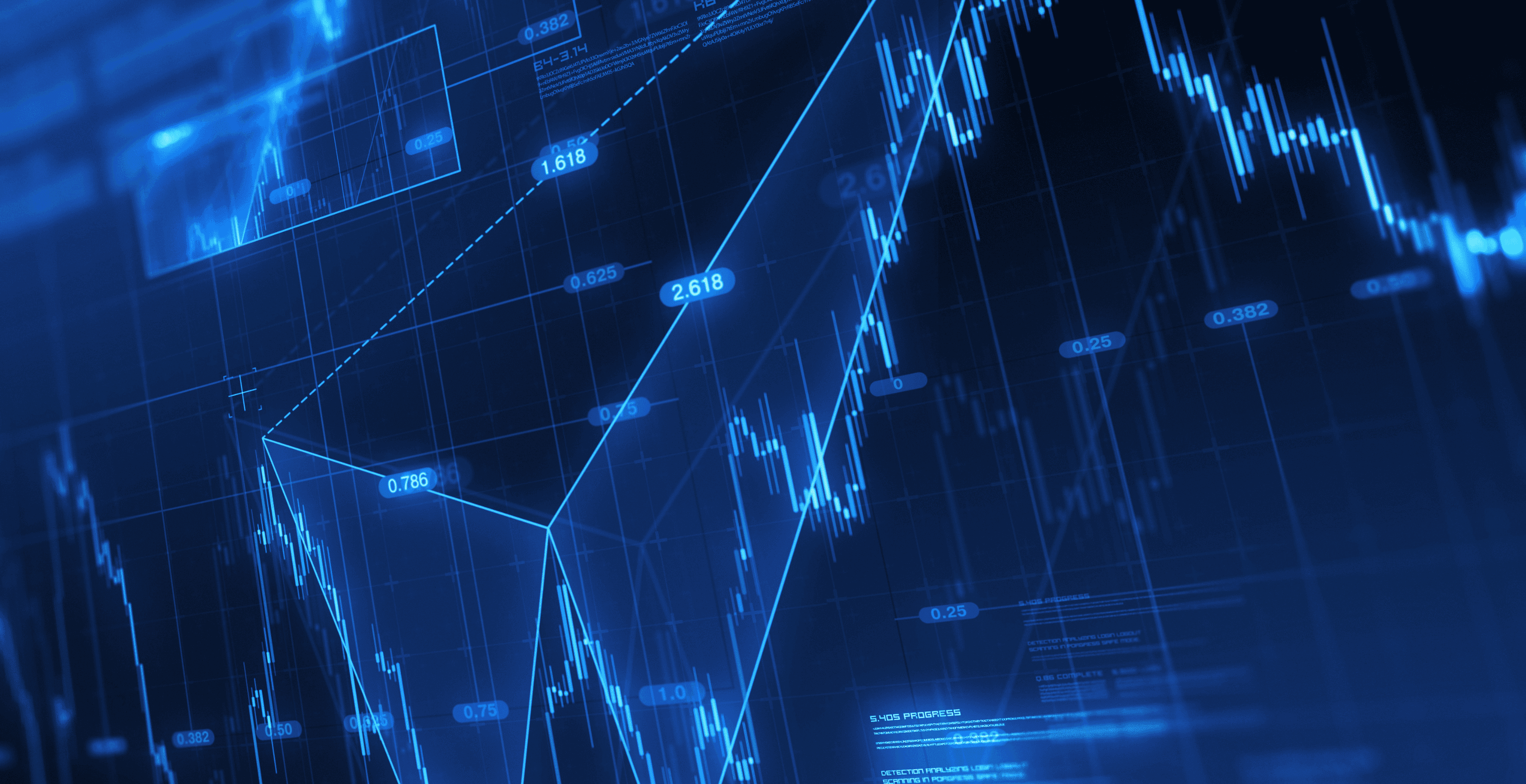
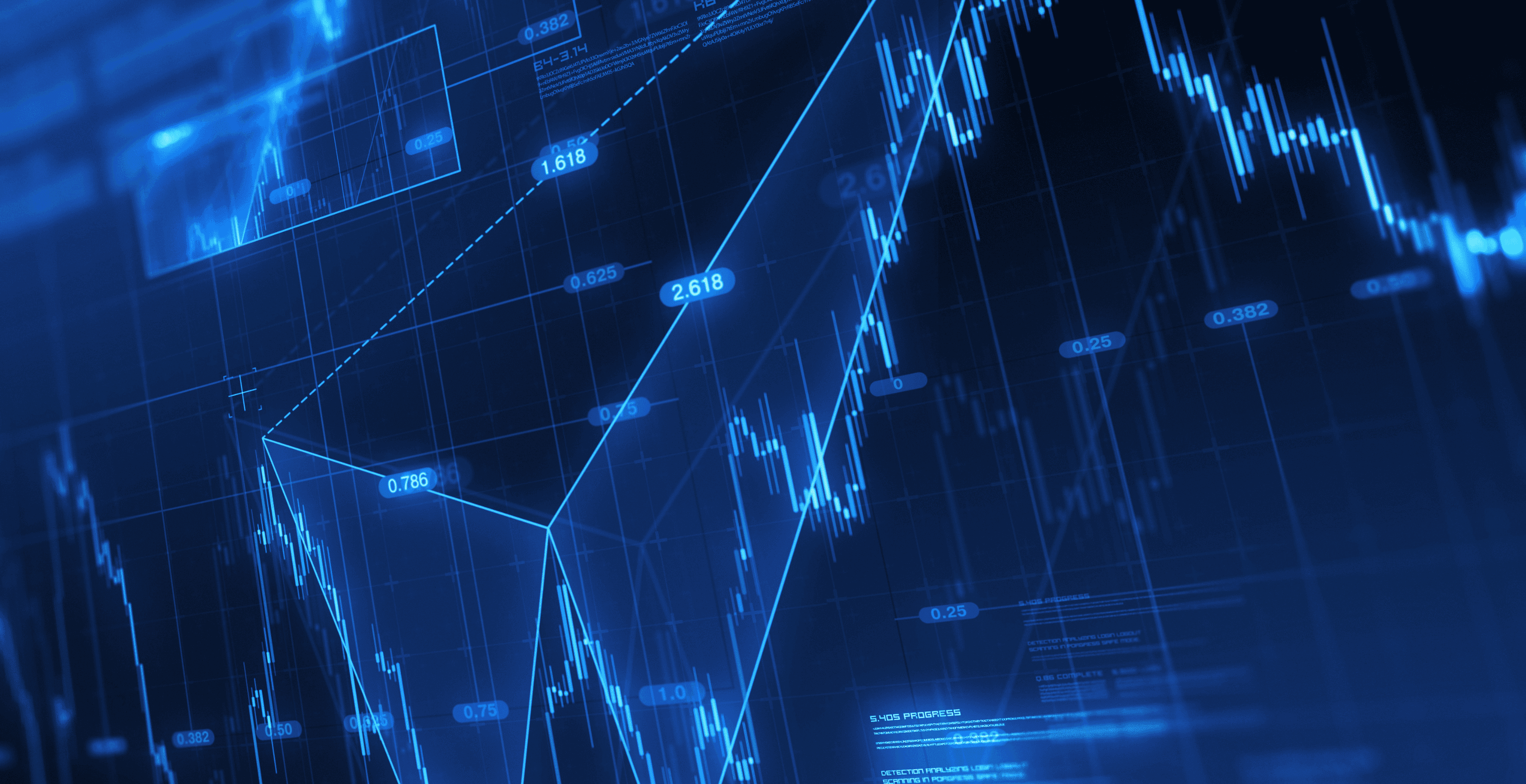
Ever wondered why your machine-learning models lose their efficacy over time? The culprit might be data drift. In the rapidly evolving scenario of machine learning, comprehending and acknowledging data drift is paramount to maintaining the precision and dependability of your models.
In the continuously progressing scenario of machine learning, one hidden opponent can inaudibly vandalize your model's performance: data drift.
Data Drift in the Context of Machine Learning
Data drift refers to the alterations in data dispensations over time, which can sternly affect the performance of machine learning models. As models are often trained on factual information, any shift in the fundamental data patterns—be it progressive or sudden—can lead to inaccuracies and reduced efficiency in auguring tasks. Comprehending and acknowledging data drift is vital for maintaining powerful and dependable ML systems.
ML Model Assumptions and Their Implications
When evolving ML models, numerous key theories are made about the information:
Stationarity: The statistical properties of the data remain perpetual over time.
IID (Independent and Identically Distributed): The training data is a delegate of the real-world data the model will confront.
Breaching these presumptions can lead to substantial performance deterioration. For instance, if the distribution of attributes in new data diverges from the training data, the model's forecasts may become less precise or even solely incorrect. This makes the monitoring and detection of data drift a crucial part of the ML lifecycle.
How Real-World Data Deviations Cause Data Drift
In the real world, data is sometimes stagnant. It unfolds due to numerous factors, such as alterations in user behavior, market trends, or external events. These deviations mean that the patterns the model learned during training no longer hold, leading to data drift. For example, a model forecasting customer behavior based on past buying data might become less precise if consumer choices shift due to a new trend. Consequently, constant monitoring and updating of models are necessary to adjust to these alterations and maintain performance.
Explore more insights on AI innovations in our guide to Enhancing Computer Vision with Synthetic Data: Advantages and Generation Techniques.
Understanding Data Drift
Ever felt like your data is betraying you? That's probably data drift at play. Let's dive into what it means and why it happens.
Definition and Types of Data Drift
Data drift is when the statistical properties of your data alter over time. This can mess up your machine-learning models, making them less precise and dependable. There are two main types of data drift you need to know about:
Concept Drift: This occurs when the relationship between input data and output changes. Imagine a model forecasting customer choices. Over time, as trends change, the choices shift, leading to concept drift.
Covariate Drift: This happens when the allocation of input data alters. For example, if you're dissecting website traffic and suddenly see a spike from a new region, that’s covariate drift.
Causes of Data Drift
Why does data drift happen? There are numerous reasons, but let’s concentrate on two big ones:
Time Dimension: Over time, everything advances. Customer choices, market trends, and even the data itself alter. This is a natural cause of data drift.
External Factors: Technological progressions and societal alterations can lead to data drift. For instance, a new social media platform might alter how people communicate online, or a universal event could shift buying behaviors.
Impact of Data Drift
Data drift can have a significant impact on your business decisions, model accuracy, and user behavior. Here’s how:
Business Decisions: Relying on outdated models can lead to poor business choices. If your model doesn’t account for current trends, you might miss out on opportunities or face unexpected challenges.
Model Accuracy: Data drift reduces the accuracy of your predictive models. An inaccurate model means poor predictions, leading to flawed strategies.
User Behavior: If your business depends on understanding user behavior, data drift can lead to misinterpretations. This affects everything from marketing campaigns to product recommendations.
Nuances Between Data Drift, Concept Drift, and Related Terms
Comprehending the nuances between these terms is critical. While data drift is an expansive concept, concept drift especially refers to alterations in the relationship between inputs and outputs. Meanwhile, covariate drift concentrates on alterations in the input data dispensation. Other related terms include:
Label Drift: When the allocation of the target variable alters over time.
Feature Drift: When individual attributes within the data alter independently of the others.
By determining and acknowledging data drift, you can maintain the precision and dependability of your models, ensuring better decision-making and user experiences. Stay alert and keep your data in check!
For an understanding of the Importance of Accuracy and Reliability in Tabular Data Models, check out our comprehensive guide.
The Importance of Data Drift Detection
Let’s now examine the significance of Data Drift detection:
Significance of Data Drift Monitoring
Do you know why your machine learning model's performance suddenly deteriorates? The perpetrator might be data drift. Discerning and monitoring data drift is vital to maintaining the quality of your model. When you observe data drift, you ensure your model stays precise and dependable over time.
Early Detection of Data Drift to Prevent Model Degradation
Data drift, the silent destroyer, can lead to substantial model deterioration. If you let it go rampant, it can render your model ineffectual, causing headaches and lost opportunities. That's why early detection is necessary. By detecting data drift early, you can take reformative actions before your model’s performance suffers.
Data Drift: Early Warnings and Model Debugging
Data drift detection isn't just about damage control; it plays a crucial role in model preservation. It acts as an early cautioning system, warning you of probable problems before they intensify. This dynamic approach helps in debugging models effectively, saving time and resources.
In short, monitoring data drift keeps your models sharp, helps you catch issues early, and aids sleek model maintenance.
Discover more insights on Understanding ML Model Monitoring in Production to ensure your models are performing at their best.
Methods and Tools for Data Drift Detection
Let's learn some efficient methods and tools for detecting data drift to ensure your models stay precise and dependable:
Overview of Data Drift Detection Methods
There are numerous ways to detect data drift, including statistical tests, distance metrics, and machine learning models. Each approach provides eccentric benefits:
Statistical tests contrast allocations to determine substantial alterations.
Distance metrics gauge the divergence between data dispensations.
Machine learning models forecast and flag possible drifts based on factual information.
Introduction to Specific Detection Methodologies
Let’s get more precise with some eminent methodologies:
Kolmogorov-Smirnov (K-S) Test: This non-parametric test contrasts the allocation of two datasets. It’s specifically advantageous for constant variables, emphasizing distinctions that could impact your model.
Population Stability Index (PSI): PSI gauges the firmness of unambiguous or numerical attributes over time. A high PSI indicates a substantial shift in data dispensation, waving potential drift.
Page-Hinkley Method: Designed for real-time monitoring, this method discovers sudden alterations in data streams. It’s gradually efficient for applications requiring prompt drift detection.
Automated Detection Tools
Why do it manually when automation can save you time and effort? Here are some automated tools you can use:
Evidently AI: This open-source library provides thorough reports on data drift, including statistical tests and visualizations.
Azure ML’s Data Drift Detection Features: Microsoft Azure’s machine learning platform provides built-in tools for monitoring and managing data drift, incorporating smoothly with your existing workflows.
Alternative Approaches: Other tools like DataRobot and Alteryx provide powerful drift detection capabilities, each with unique attributes customized to various requirements.
Implementing Data Drift Detection in Production
To efficiently scuffle data drift, follow these strategies:
Integrate Continuous Monitoring: Frequently monitor your data pipelines and model outputs. Automated warnings can inform you of potential drifts before they affect your model's performance.
Define Thresholds: Set clear thresholds for adequate data dispensation alterations. This will help you differentiate between minor changes and substantial drifts.
Leverage Version Control: Keep track of data and model versions to determine when and where drifts occur. This will provide faster diagnostics and refinement.
Regularly Retrain Models: Schedule frequent model retraining sessions based on updated data to ensure your models remain precise and efficient.
Detecting data drift is vital for maintaining the precision and dependability of your machine-learning models. By using statistical tests, distance metrics, machine learning models, and automated tools, you can stay ahead of data drift and ensure your models perform at their best.
For a dissection, check out our guide on Understanding the Basics of LLM Fine-Tuning with Custom Data.
Strategies for Identifying and Managing Data Drift
Let’s now know about strategic ways to stay ahead of the game. Here’s how you can tackle data drift like a pro:
Robust Continuous Monitoring
Can you drive your car without checking your mirrors? Observing your data constantly works the same way. You need to set up automated systems to trace alterations in your data's allocation over time. Use tools that give real-time insights into your data, helping you catch peculiarities before they turn into bigger problems. Think of it as a health inspection for your data, ensuring everything runs effortlessly and efficiently.
Master Data Drift: Retrain, Adjust, Learn
Once you detect data drift, it’s time to take action. Here are three robust approaches:
Retraining Models: Frequently retrain your models with the most recent data to keep them sharp and up-to-date. This helps maintain their auguring power and pertinence.
Adjusting Data Pipelines: Refine your data pipelines to adapt alterations in data patterns. This might mean tweaking preprocessing steps or distorting feature engineering methodologies.
Using Incremental Learning: Integrate gradual comprehension techniques that permit your models to adjust increasingly to new data without the requirement for complete retraining. This is specifically useful for large datasets where retraining can be resource-intensive.
Importance of Feature Selection in Drift Detection and Management
Not all attributes are created equal. Some can be more sensitive to alterations, leading to substantial drift. By precisely choosing and observing critical attributes, you can detect drift early and take corrective measures. Frequently retrospect your attribute set and exclude those that contribute little to the model’s performance or are prone to drift. This keeps your model lean, effective, and flexible against data drift.
Custom Alerts, Dataset Monitors, and Explainable AI
Stay ahead with personalized alerts and dataset monitors that notify you promptly when data drift occurs. Set thresholds and prompts accurate to your venture requirements, so you’re always in the loop. Moreover, using Explainable AI can provide insights into why your models are complying distinctly, providing a clearer picture of the underlying drift causes. This lucidity helps you make informed decisions and refine your strategies efficiently.
Ready to automate your data drift detection and save your valuable time? Let’s dive into why automation is a game changer.
Want to learn about Deep Learning Applications? Read our detailed article on Exploring The Frontiers Of Deep Learning Applications.
Automating Data Drift Detection
Are you languishing to keep your machine learning models precise and pertinent? Automating data drift detection could be your groundbreaker! Here's why you need it, how to set it up, and some alternatives if you prefer a more hands-on approach.
Benefits of Automating Data Drift Detection
Automated data drift detection provides effectiveness and readiness for supervising your machine learning models. You won't spend innumerable hours manually checking for inconsistencies or shifts in data dispensations. With automation, you can instantly identify any data alterations affecting your model's performance. This means you can swiftly adapt your models, maintaining their precision and dependability. In addition, automated systems manage large datasets and intricate data pipelines, making them indispensable for modern data-driven ventures.
Setting Up Automated Data Drift Detection in Azure ML
Setting up automated data drift detection in Azure ML is a direct process. Here’s a quick outline to get you started:
Create an Azure ML Workspace: Begin by setting up your workspace where all your experiments, models, and information will reside.
Ingest Your Data: Use Azure Data Factory or Azure Blob Storage to bring your data into the workspace.
Register Your Dataset: Register your threshold dataset (the original data) and the new incoming datasets.
Set Up a Data Drift Monitor: Azure ML provides a data drift detection attribute. Configure this by choosing the baseline dataset, the target dataset, and the attributes to observe.
Configure Alerts: Set up alerts to notify you when substantial data drift is discovered. You can choose email notifications or incorporate them with Azure DevOps for more sleek productivity.
Alternatives to Automated Tools
If you prefer more control over your data drift detection, you can opt for custom scripts or open-source libraries. Here are a few eminent options:
Custom Scripts: Writing your scripts in Python or R permits you to customize the drift detection procedure to your precise requirements. You can use libraries like Pandas, NumPy, and SciPy to create comprehensive drift reports.
Open-Source Libraries: Tools like Evidently and Scikit-Multiflow offer pre-built functions for data drift detection. These libraries offer adaptability and personalization, giving you the ability to correct the algorithms to suit your data and models better.
Incorporating Automation and Explainability
Incorporating both automation and explainability into your data drift management plan ensures that you not only detect drift quickly but also comprehend why it happens. Here’s how you can do it:
Automate Monitoring: Use automated tools to monitor your data for drift constantly. This reduces manual workload and ensures prompt discovery.
Explainable AI (XAI) Tools: Incorporate XAI tools like SHAP or LIME to elucidate the data drift. These tools help you comprehend which attributes are altering and how they affect your model's performance.
Documentation and Reporting: Keep comprehensive records of detected drifts and the steps taken to acknowledge them. This practice helps in tracking trends over time and enhances your overall data governance.
Discover the necessities of compliance automation in our guide on getting started with regulatory management.
How RagaAi Can Help in Tackling Data Drift
When it comes to tackling data drift, RagaAI provides a pragmatic solution designed to detect and address data anomalies effectively. Our platform provides powerful tools and methodologies to ensure your models remain precise and dependable over time. Here’s how RagaAI can assist:
Advanced Drift Detection: RagaAI’s Prism module includes an advanced drift detection system that recognizes shifts in data distribution. It observes input data and alerts you when substantial deviations occur, enabling instant corrective actions.
Real-time Monitoring: With RagaAI, you can set up real-time monitoring for your data streams. This ensures that any data drift is detected as soon as it happens, minimizing the impact on your models’ performance. The constant monitoring attribute helps in maintaining model precision and dependability.
Automated Response Mechanisms: RagaAI incorporates automated response mechanisms to manage data drift. Once drift is detected, predefined actions can be triggered automatically, such as retraining models or adapting data preprocessing steps. This automation reduces the time and effort needed to tackle drift manually.
Comprehensive Analytics: The platform provides comprehensive analytics and visualizations to comprehend the nature and cause of data drift. By using these insights, you can make informed decisions about how to acknowledge and alleviate drift in your models.
Easy Integration: RagaAi’s tools are designed for easy incorporation with your existing data pipelines. Whether you’re using cloud-based services or on-premise systems, RagaAI can smoothly incorporate constant drift monitoring and management.
Customizable Alerts: You can tailor alert thresholds and notifications according to your explicit requirements. This adaptability ensures that you are only informed of substantial drifts that could affect your models, reducing noise and permitting you to concentrate on integral issues.
By using RagaAI’s robust drift detection and management capabilities, ventures can ensure that their models remain powerful and precise, even as fundamental data alterations. This dynamic approach to managing data drift not only enhances model performance but also contributes to more dependable and trustworthy AI systems.
For more detailed insights on drift detection in object detection models, check out the Ragaai Prism documentation on drift detection.
Conclusion
Incorporating data drift management into the ML model lifecycle will improve model dependability and performance, driving better venture results and more precise forecasts.
By comprehending and acknowledging data drift, you can ensure your machine-learning models remain powerful, precise, and dependable. Stay alert, observe constantly, and clasp automation to tackle data drift efficiently.
Sign up now and revolutionize your AI quality management with Raga AI. Don't let data drift hold you back—join us and stay ahead of the curve!
Ever wondered why your machine-learning models lose their efficacy over time? The culprit might be data drift. In the rapidly evolving scenario of machine learning, comprehending and acknowledging data drift is paramount to maintaining the precision and dependability of your models.
In the continuously progressing scenario of machine learning, one hidden opponent can inaudibly vandalize your model's performance: data drift.
Data Drift in the Context of Machine Learning
Data drift refers to the alterations in data dispensations over time, which can sternly affect the performance of machine learning models. As models are often trained on factual information, any shift in the fundamental data patterns—be it progressive or sudden—can lead to inaccuracies and reduced efficiency in auguring tasks. Comprehending and acknowledging data drift is vital for maintaining powerful and dependable ML systems.
ML Model Assumptions and Their Implications
When evolving ML models, numerous key theories are made about the information:
Stationarity: The statistical properties of the data remain perpetual over time.
IID (Independent and Identically Distributed): The training data is a delegate of the real-world data the model will confront.
Breaching these presumptions can lead to substantial performance deterioration. For instance, if the distribution of attributes in new data diverges from the training data, the model's forecasts may become less precise or even solely incorrect. This makes the monitoring and detection of data drift a crucial part of the ML lifecycle.
How Real-World Data Deviations Cause Data Drift
In the real world, data is sometimes stagnant. It unfolds due to numerous factors, such as alterations in user behavior, market trends, or external events. These deviations mean that the patterns the model learned during training no longer hold, leading to data drift. For example, a model forecasting customer behavior based on past buying data might become less precise if consumer choices shift due to a new trend. Consequently, constant monitoring and updating of models are necessary to adjust to these alterations and maintain performance.
Explore more insights on AI innovations in our guide to Enhancing Computer Vision with Synthetic Data: Advantages and Generation Techniques.
Understanding Data Drift
Ever felt like your data is betraying you? That's probably data drift at play. Let's dive into what it means and why it happens.
Definition and Types of Data Drift
Data drift is when the statistical properties of your data alter over time. This can mess up your machine-learning models, making them less precise and dependable. There are two main types of data drift you need to know about:
Concept Drift: This occurs when the relationship between input data and output changes. Imagine a model forecasting customer choices. Over time, as trends change, the choices shift, leading to concept drift.
Covariate Drift: This happens when the allocation of input data alters. For example, if you're dissecting website traffic and suddenly see a spike from a new region, that’s covariate drift.
Causes of Data Drift
Why does data drift happen? There are numerous reasons, but let’s concentrate on two big ones:
Time Dimension: Over time, everything advances. Customer choices, market trends, and even the data itself alter. This is a natural cause of data drift.
External Factors: Technological progressions and societal alterations can lead to data drift. For instance, a new social media platform might alter how people communicate online, or a universal event could shift buying behaviors.
Impact of Data Drift
Data drift can have a significant impact on your business decisions, model accuracy, and user behavior. Here’s how:
Business Decisions: Relying on outdated models can lead to poor business choices. If your model doesn’t account for current trends, you might miss out on opportunities or face unexpected challenges.
Model Accuracy: Data drift reduces the accuracy of your predictive models. An inaccurate model means poor predictions, leading to flawed strategies.
User Behavior: If your business depends on understanding user behavior, data drift can lead to misinterpretations. This affects everything from marketing campaigns to product recommendations.
Nuances Between Data Drift, Concept Drift, and Related Terms
Comprehending the nuances between these terms is critical. While data drift is an expansive concept, concept drift especially refers to alterations in the relationship between inputs and outputs. Meanwhile, covariate drift concentrates on alterations in the input data dispensation. Other related terms include:
Label Drift: When the allocation of the target variable alters over time.
Feature Drift: When individual attributes within the data alter independently of the others.
By determining and acknowledging data drift, you can maintain the precision and dependability of your models, ensuring better decision-making and user experiences. Stay alert and keep your data in check!
For an understanding of the Importance of Accuracy and Reliability in Tabular Data Models, check out our comprehensive guide.
The Importance of Data Drift Detection
Let’s now examine the significance of Data Drift detection:
Significance of Data Drift Monitoring
Do you know why your machine learning model's performance suddenly deteriorates? The perpetrator might be data drift. Discerning and monitoring data drift is vital to maintaining the quality of your model. When you observe data drift, you ensure your model stays precise and dependable over time.
Early Detection of Data Drift to Prevent Model Degradation
Data drift, the silent destroyer, can lead to substantial model deterioration. If you let it go rampant, it can render your model ineffectual, causing headaches and lost opportunities. That's why early detection is necessary. By detecting data drift early, you can take reformative actions before your model’s performance suffers.
Data Drift: Early Warnings and Model Debugging
Data drift detection isn't just about damage control; it plays a crucial role in model preservation. It acts as an early cautioning system, warning you of probable problems before they intensify. This dynamic approach helps in debugging models effectively, saving time and resources.
In short, monitoring data drift keeps your models sharp, helps you catch issues early, and aids sleek model maintenance.
Discover more insights on Understanding ML Model Monitoring in Production to ensure your models are performing at their best.
Methods and Tools for Data Drift Detection
Let's learn some efficient methods and tools for detecting data drift to ensure your models stay precise and dependable:
Overview of Data Drift Detection Methods
There are numerous ways to detect data drift, including statistical tests, distance metrics, and machine learning models. Each approach provides eccentric benefits:
Statistical tests contrast allocations to determine substantial alterations.
Distance metrics gauge the divergence between data dispensations.
Machine learning models forecast and flag possible drifts based on factual information.
Introduction to Specific Detection Methodologies
Let’s get more precise with some eminent methodologies:
Kolmogorov-Smirnov (K-S) Test: This non-parametric test contrasts the allocation of two datasets. It’s specifically advantageous for constant variables, emphasizing distinctions that could impact your model.
Population Stability Index (PSI): PSI gauges the firmness of unambiguous or numerical attributes over time. A high PSI indicates a substantial shift in data dispensation, waving potential drift.
Page-Hinkley Method: Designed for real-time monitoring, this method discovers sudden alterations in data streams. It’s gradually efficient for applications requiring prompt drift detection.
Automated Detection Tools
Why do it manually when automation can save you time and effort? Here are some automated tools you can use:
Evidently AI: This open-source library provides thorough reports on data drift, including statistical tests and visualizations.
Azure ML’s Data Drift Detection Features: Microsoft Azure’s machine learning platform provides built-in tools for monitoring and managing data drift, incorporating smoothly with your existing workflows.
Alternative Approaches: Other tools like DataRobot and Alteryx provide powerful drift detection capabilities, each with unique attributes customized to various requirements.
Implementing Data Drift Detection in Production
To efficiently scuffle data drift, follow these strategies:
Integrate Continuous Monitoring: Frequently monitor your data pipelines and model outputs. Automated warnings can inform you of potential drifts before they affect your model's performance.
Define Thresholds: Set clear thresholds for adequate data dispensation alterations. This will help you differentiate between minor changes and substantial drifts.
Leverage Version Control: Keep track of data and model versions to determine when and where drifts occur. This will provide faster diagnostics and refinement.
Regularly Retrain Models: Schedule frequent model retraining sessions based on updated data to ensure your models remain precise and efficient.
Detecting data drift is vital for maintaining the precision and dependability of your machine-learning models. By using statistical tests, distance metrics, machine learning models, and automated tools, you can stay ahead of data drift and ensure your models perform at their best.
For a dissection, check out our guide on Understanding the Basics of LLM Fine-Tuning with Custom Data.
Strategies for Identifying and Managing Data Drift
Let’s now know about strategic ways to stay ahead of the game. Here’s how you can tackle data drift like a pro:
Robust Continuous Monitoring
Can you drive your car without checking your mirrors? Observing your data constantly works the same way. You need to set up automated systems to trace alterations in your data's allocation over time. Use tools that give real-time insights into your data, helping you catch peculiarities before they turn into bigger problems. Think of it as a health inspection for your data, ensuring everything runs effortlessly and efficiently.
Master Data Drift: Retrain, Adjust, Learn
Once you detect data drift, it’s time to take action. Here are three robust approaches:
Retraining Models: Frequently retrain your models with the most recent data to keep them sharp and up-to-date. This helps maintain their auguring power and pertinence.
Adjusting Data Pipelines: Refine your data pipelines to adapt alterations in data patterns. This might mean tweaking preprocessing steps or distorting feature engineering methodologies.
Using Incremental Learning: Integrate gradual comprehension techniques that permit your models to adjust increasingly to new data without the requirement for complete retraining. This is specifically useful for large datasets where retraining can be resource-intensive.
Importance of Feature Selection in Drift Detection and Management
Not all attributes are created equal. Some can be more sensitive to alterations, leading to substantial drift. By precisely choosing and observing critical attributes, you can detect drift early and take corrective measures. Frequently retrospect your attribute set and exclude those that contribute little to the model’s performance or are prone to drift. This keeps your model lean, effective, and flexible against data drift.
Custom Alerts, Dataset Monitors, and Explainable AI
Stay ahead with personalized alerts and dataset monitors that notify you promptly when data drift occurs. Set thresholds and prompts accurate to your venture requirements, so you’re always in the loop. Moreover, using Explainable AI can provide insights into why your models are complying distinctly, providing a clearer picture of the underlying drift causes. This lucidity helps you make informed decisions and refine your strategies efficiently.
Ready to automate your data drift detection and save your valuable time? Let’s dive into why automation is a game changer.
Want to learn about Deep Learning Applications? Read our detailed article on Exploring The Frontiers Of Deep Learning Applications.
Automating Data Drift Detection
Are you languishing to keep your machine learning models precise and pertinent? Automating data drift detection could be your groundbreaker! Here's why you need it, how to set it up, and some alternatives if you prefer a more hands-on approach.
Benefits of Automating Data Drift Detection
Automated data drift detection provides effectiveness and readiness for supervising your machine learning models. You won't spend innumerable hours manually checking for inconsistencies or shifts in data dispensations. With automation, you can instantly identify any data alterations affecting your model's performance. This means you can swiftly adapt your models, maintaining their precision and dependability. In addition, automated systems manage large datasets and intricate data pipelines, making them indispensable for modern data-driven ventures.
Setting Up Automated Data Drift Detection in Azure ML
Setting up automated data drift detection in Azure ML is a direct process. Here’s a quick outline to get you started:
Create an Azure ML Workspace: Begin by setting up your workspace where all your experiments, models, and information will reside.
Ingest Your Data: Use Azure Data Factory or Azure Blob Storage to bring your data into the workspace.
Register Your Dataset: Register your threshold dataset (the original data) and the new incoming datasets.
Set Up a Data Drift Monitor: Azure ML provides a data drift detection attribute. Configure this by choosing the baseline dataset, the target dataset, and the attributes to observe.
Configure Alerts: Set up alerts to notify you when substantial data drift is discovered. You can choose email notifications or incorporate them with Azure DevOps for more sleek productivity.
Alternatives to Automated Tools
If you prefer more control over your data drift detection, you can opt for custom scripts or open-source libraries. Here are a few eminent options:
Custom Scripts: Writing your scripts in Python or R permits you to customize the drift detection procedure to your precise requirements. You can use libraries like Pandas, NumPy, and SciPy to create comprehensive drift reports.
Open-Source Libraries: Tools like Evidently and Scikit-Multiflow offer pre-built functions for data drift detection. These libraries offer adaptability and personalization, giving you the ability to correct the algorithms to suit your data and models better.
Incorporating Automation and Explainability
Incorporating both automation and explainability into your data drift management plan ensures that you not only detect drift quickly but also comprehend why it happens. Here’s how you can do it:
Automate Monitoring: Use automated tools to monitor your data for drift constantly. This reduces manual workload and ensures prompt discovery.
Explainable AI (XAI) Tools: Incorporate XAI tools like SHAP or LIME to elucidate the data drift. These tools help you comprehend which attributes are altering and how they affect your model's performance.
Documentation and Reporting: Keep comprehensive records of detected drifts and the steps taken to acknowledge them. This practice helps in tracking trends over time and enhances your overall data governance.
Discover the necessities of compliance automation in our guide on getting started with regulatory management.
How RagaAi Can Help in Tackling Data Drift
When it comes to tackling data drift, RagaAI provides a pragmatic solution designed to detect and address data anomalies effectively. Our platform provides powerful tools and methodologies to ensure your models remain precise and dependable over time. Here’s how RagaAI can assist:
Advanced Drift Detection: RagaAI’s Prism module includes an advanced drift detection system that recognizes shifts in data distribution. It observes input data and alerts you when substantial deviations occur, enabling instant corrective actions.
Real-time Monitoring: With RagaAI, you can set up real-time monitoring for your data streams. This ensures that any data drift is detected as soon as it happens, minimizing the impact on your models’ performance. The constant monitoring attribute helps in maintaining model precision and dependability.
Automated Response Mechanisms: RagaAI incorporates automated response mechanisms to manage data drift. Once drift is detected, predefined actions can be triggered automatically, such as retraining models or adapting data preprocessing steps. This automation reduces the time and effort needed to tackle drift manually.
Comprehensive Analytics: The platform provides comprehensive analytics and visualizations to comprehend the nature and cause of data drift. By using these insights, you can make informed decisions about how to acknowledge and alleviate drift in your models.
Easy Integration: RagaAi’s tools are designed for easy incorporation with your existing data pipelines. Whether you’re using cloud-based services or on-premise systems, RagaAI can smoothly incorporate constant drift monitoring and management.
Customizable Alerts: You can tailor alert thresholds and notifications according to your explicit requirements. This adaptability ensures that you are only informed of substantial drifts that could affect your models, reducing noise and permitting you to concentrate on integral issues.
By using RagaAI’s robust drift detection and management capabilities, ventures can ensure that their models remain powerful and precise, even as fundamental data alterations. This dynamic approach to managing data drift not only enhances model performance but also contributes to more dependable and trustworthy AI systems.
For more detailed insights on drift detection in object detection models, check out the Ragaai Prism documentation on drift detection.
Conclusion
Incorporating data drift management into the ML model lifecycle will improve model dependability and performance, driving better venture results and more precise forecasts.
By comprehending and acknowledging data drift, you can ensure your machine-learning models remain powerful, precise, and dependable. Stay alert, observe constantly, and clasp automation to tackle data drift efficiently.
Sign up now and revolutionize your AI quality management with Raga AI. Don't let data drift hold you back—join us and stay ahead of the curve!
Ever wondered why your machine-learning models lose their efficacy over time? The culprit might be data drift. In the rapidly evolving scenario of machine learning, comprehending and acknowledging data drift is paramount to maintaining the precision and dependability of your models.
In the continuously progressing scenario of machine learning, one hidden opponent can inaudibly vandalize your model's performance: data drift.
Data Drift in the Context of Machine Learning
Data drift refers to the alterations in data dispensations over time, which can sternly affect the performance of machine learning models. As models are often trained on factual information, any shift in the fundamental data patterns—be it progressive or sudden—can lead to inaccuracies and reduced efficiency in auguring tasks. Comprehending and acknowledging data drift is vital for maintaining powerful and dependable ML systems.
ML Model Assumptions and Their Implications
When evolving ML models, numerous key theories are made about the information:
Stationarity: The statistical properties of the data remain perpetual over time.
IID (Independent and Identically Distributed): The training data is a delegate of the real-world data the model will confront.
Breaching these presumptions can lead to substantial performance deterioration. For instance, if the distribution of attributes in new data diverges from the training data, the model's forecasts may become less precise or even solely incorrect. This makes the monitoring and detection of data drift a crucial part of the ML lifecycle.
How Real-World Data Deviations Cause Data Drift
In the real world, data is sometimes stagnant. It unfolds due to numerous factors, such as alterations in user behavior, market trends, or external events. These deviations mean that the patterns the model learned during training no longer hold, leading to data drift. For example, a model forecasting customer behavior based on past buying data might become less precise if consumer choices shift due to a new trend. Consequently, constant monitoring and updating of models are necessary to adjust to these alterations and maintain performance.
Explore more insights on AI innovations in our guide to Enhancing Computer Vision with Synthetic Data: Advantages and Generation Techniques.
Understanding Data Drift
Ever felt like your data is betraying you? That's probably data drift at play. Let's dive into what it means and why it happens.
Definition and Types of Data Drift
Data drift is when the statistical properties of your data alter over time. This can mess up your machine-learning models, making them less precise and dependable. There are two main types of data drift you need to know about:
Concept Drift: This occurs when the relationship between input data and output changes. Imagine a model forecasting customer choices. Over time, as trends change, the choices shift, leading to concept drift.
Covariate Drift: This happens when the allocation of input data alters. For example, if you're dissecting website traffic and suddenly see a spike from a new region, that’s covariate drift.
Causes of Data Drift
Why does data drift happen? There are numerous reasons, but let’s concentrate on two big ones:
Time Dimension: Over time, everything advances. Customer choices, market trends, and even the data itself alter. This is a natural cause of data drift.
External Factors: Technological progressions and societal alterations can lead to data drift. For instance, a new social media platform might alter how people communicate online, or a universal event could shift buying behaviors.
Impact of Data Drift
Data drift can have a significant impact on your business decisions, model accuracy, and user behavior. Here’s how:
Business Decisions: Relying on outdated models can lead to poor business choices. If your model doesn’t account for current trends, you might miss out on opportunities or face unexpected challenges.
Model Accuracy: Data drift reduces the accuracy of your predictive models. An inaccurate model means poor predictions, leading to flawed strategies.
User Behavior: If your business depends on understanding user behavior, data drift can lead to misinterpretations. This affects everything from marketing campaigns to product recommendations.
Nuances Between Data Drift, Concept Drift, and Related Terms
Comprehending the nuances between these terms is critical. While data drift is an expansive concept, concept drift especially refers to alterations in the relationship between inputs and outputs. Meanwhile, covariate drift concentrates on alterations in the input data dispensation. Other related terms include:
Label Drift: When the allocation of the target variable alters over time.
Feature Drift: When individual attributes within the data alter independently of the others.
By determining and acknowledging data drift, you can maintain the precision and dependability of your models, ensuring better decision-making and user experiences. Stay alert and keep your data in check!
For an understanding of the Importance of Accuracy and Reliability in Tabular Data Models, check out our comprehensive guide.
The Importance of Data Drift Detection
Let’s now examine the significance of Data Drift detection:
Significance of Data Drift Monitoring
Do you know why your machine learning model's performance suddenly deteriorates? The perpetrator might be data drift. Discerning and monitoring data drift is vital to maintaining the quality of your model. When you observe data drift, you ensure your model stays precise and dependable over time.
Early Detection of Data Drift to Prevent Model Degradation
Data drift, the silent destroyer, can lead to substantial model deterioration. If you let it go rampant, it can render your model ineffectual, causing headaches and lost opportunities. That's why early detection is necessary. By detecting data drift early, you can take reformative actions before your model’s performance suffers.
Data Drift: Early Warnings and Model Debugging
Data drift detection isn't just about damage control; it plays a crucial role in model preservation. It acts as an early cautioning system, warning you of probable problems before they intensify. This dynamic approach helps in debugging models effectively, saving time and resources.
In short, monitoring data drift keeps your models sharp, helps you catch issues early, and aids sleek model maintenance.
Discover more insights on Understanding ML Model Monitoring in Production to ensure your models are performing at their best.
Methods and Tools for Data Drift Detection
Let's learn some efficient methods and tools for detecting data drift to ensure your models stay precise and dependable:
Overview of Data Drift Detection Methods
There are numerous ways to detect data drift, including statistical tests, distance metrics, and machine learning models. Each approach provides eccentric benefits:
Statistical tests contrast allocations to determine substantial alterations.
Distance metrics gauge the divergence between data dispensations.
Machine learning models forecast and flag possible drifts based on factual information.
Introduction to Specific Detection Methodologies
Let’s get more precise with some eminent methodologies:
Kolmogorov-Smirnov (K-S) Test: This non-parametric test contrasts the allocation of two datasets. It’s specifically advantageous for constant variables, emphasizing distinctions that could impact your model.
Population Stability Index (PSI): PSI gauges the firmness of unambiguous or numerical attributes over time. A high PSI indicates a substantial shift in data dispensation, waving potential drift.
Page-Hinkley Method: Designed for real-time monitoring, this method discovers sudden alterations in data streams. It’s gradually efficient for applications requiring prompt drift detection.
Automated Detection Tools
Why do it manually when automation can save you time and effort? Here are some automated tools you can use:
Evidently AI: This open-source library provides thorough reports on data drift, including statistical tests and visualizations.
Azure ML’s Data Drift Detection Features: Microsoft Azure’s machine learning platform provides built-in tools for monitoring and managing data drift, incorporating smoothly with your existing workflows.
Alternative Approaches: Other tools like DataRobot and Alteryx provide powerful drift detection capabilities, each with unique attributes customized to various requirements.
Implementing Data Drift Detection in Production
To efficiently scuffle data drift, follow these strategies:
Integrate Continuous Monitoring: Frequently monitor your data pipelines and model outputs. Automated warnings can inform you of potential drifts before they affect your model's performance.
Define Thresholds: Set clear thresholds for adequate data dispensation alterations. This will help you differentiate between minor changes and substantial drifts.
Leverage Version Control: Keep track of data and model versions to determine when and where drifts occur. This will provide faster diagnostics and refinement.
Regularly Retrain Models: Schedule frequent model retraining sessions based on updated data to ensure your models remain precise and efficient.
Detecting data drift is vital for maintaining the precision and dependability of your machine-learning models. By using statistical tests, distance metrics, machine learning models, and automated tools, you can stay ahead of data drift and ensure your models perform at their best.
For a dissection, check out our guide on Understanding the Basics of LLM Fine-Tuning with Custom Data.
Strategies for Identifying and Managing Data Drift
Let’s now know about strategic ways to stay ahead of the game. Here’s how you can tackle data drift like a pro:
Robust Continuous Monitoring
Can you drive your car without checking your mirrors? Observing your data constantly works the same way. You need to set up automated systems to trace alterations in your data's allocation over time. Use tools that give real-time insights into your data, helping you catch peculiarities before they turn into bigger problems. Think of it as a health inspection for your data, ensuring everything runs effortlessly and efficiently.
Master Data Drift: Retrain, Adjust, Learn
Once you detect data drift, it’s time to take action. Here are three robust approaches:
Retraining Models: Frequently retrain your models with the most recent data to keep them sharp and up-to-date. This helps maintain their auguring power and pertinence.
Adjusting Data Pipelines: Refine your data pipelines to adapt alterations in data patterns. This might mean tweaking preprocessing steps or distorting feature engineering methodologies.
Using Incremental Learning: Integrate gradual comprehension techniques that permit your models to adjust increasingly to new data without the requirement for complete retraining. This is specifically useful for large datasets where retraining can be resource-intensive.
Importance of Feature Selection in Drift Detection and Management
Not all attributes are created equal. Some can be more sensitive to alterations, leading to substantial drift. By precisely choosing and observing critical attributes, you can detect drift early and take corrective measures. Frequently retrospect your attribute set and exclude those that contribute little to the model’s performance or are prone to drift. This keeps your model lean, effective, and flexible against data drift.
Custom Alerts, Dataset Monitors, and Explainable AI
Stay ahead with personalized alerts and dataset monitors that notify you promptly when data drift occurs. Set thresholds and prompts accurate to your venture requirements, so you’re always in the loop. Moreover, using Explainable AI can provide insights into why your models are complying distinctly, providing a clearer picture of the underlying drift causes. This lucidity helps you make informed decisions and refine your strategies efficiently.
Ready to automate your data drift detection and save your valuable time? Let’s dive into why automation is a game changer.
Want to learn about Deep Learning Applications? Read our detailed article on Exploring The Frontiers Of Deep Learning Applications.
Automating Data Drift Detection
Are you languishing to keep your machine learning models precise and pertinent? Automating data drift detection could be your groundbreaker! Here's why you need it, how to set it up, and some alternatives if you prefer a more hands-on approach.
Benefits of Automating Data Drift Detection
Automated data drift detection provides effectiveness and readiness for supervising your machine learning models. You won't spend innumerable hours manually checking for inconsistencies or shifts in data dispensations. With automation, you can instantly identify any data alterations affecting your model's performance. This means you can swiftly adapt your models, maintaining their precision and dependability. In addition, automated systems manage large datasets and intricate data pipelines, making them indispensable for modern data-driven ventures.
Setting Up Automated Data Drift Detection in Azure ML
Setting up automated data drift detection in Azure ML is a direct process. Here’s a quick outline to get you started:
Create an Azure ML Workspace: Begin by setting up your workspace where all your experiments, models, and information will reside.
Ingest Your Data: Use Azure Data Factory or Azure Blob Storage to bring your data into the workspace.
Register Your Dataset: Register your threshold dataset (the original data) and the new incoming datasets.
Set Up a Data Drift Monitor: Azure ML provides a data drift detection attribute. Configure this by choosing the baseline dataset, the target dataset, and the attributes to observe.
Configure Alerts: Set up alerts to notify you when substantial data drift is discovered. You can choose email notifications or incorporate them with Azure DevOps for more sleek productivity.
Alternatives to Automated Tools
If you prefer more control over your data drift detection, you can opt for custom scripts or open-source libraries. Here are a few eminent options:
Custom Scripts: Writing your scripts in Python or R permits you to customize the drift detection procedure to your precise requirements. You can use libraries like Pandas, NumPy, and SciPy to create comprehensive drift reports.
Open-Source Libraries: Tools like Evidently and Scikit-Multiflow offer pre-built functions for data drift detection. These libraries offer adaptability and personalization, giving you the ability to correct the algorithms to suit your data and models better.
Incorporating Automation and Explainability
Incorporating both automation and explainability into your data drift management plan ensures that you not only detect drift quickly but also comprehend why it happens. Here’s how you can do it:
Automate Monitoring: Use automated tools to monitor your data for drift constantly. This reduces manual workload and ensures prompt discovery.
Explainable AI (XAI) Tools: Incorporate XAI tools like SHAP or LIME to elucidate the data drift. These tools help you comprehend which attributes are altering and how they affect your model's performance.
Documentation and Reporting: Keep comprehensive records of detected drifts and the steps taken to acknowledge them. This practice helps in tracking trends over time and enhances your overall data governance.
Discover the necessities of compliance automation in our guide on getting started with regulatory management.
How RagaAi Can Help in Tackling Data Drift
When it comes to tackling data drift, RagaAI provides a pragmatic solution designed to detect and address data anomalies effectively. Our platform provides powerful tools and methodologies to ensure your models remain precise and dependable over time. Here’s how RagaAI can assist:
Advanced Drift Detection: RagaAI’s Prism module includes an advanced drift detection system that recognizes shifts in data distribution. It observes input data and alerts you when substantial deviations occur, enabling instant corrective actions.
Real-time Monitoring: With RagaAI, you can set up real-time monitoring for your data streams. This ensures that any data drift is detected as soon as it happens, minimizing the impact on your models’ performance. The constant monitoring attribute helps in maintaining model precision and dependability.
Automated Response Mechanisms: RagaAI incorporates automated response mechanisms to manage data drift. Once drift is detected, predefined actions can be triggered automatically, such as retraining models or adapting data preprocessing steps. This automation reduces the time and effort needed to tackle drift manually.
Comprehensive Analytics: The platform provides comprehensive analytics and visualizations to comprehend the nature and cause of data drift. By using these insights, you can make informed decisions about how to acknowledge and alleviate drift in your models.
Easy Integration: RagaAi’s tools are designed for easy incorporation with your existing data pipelines. Whether you’re using cloud-based services or on-premise systems, RagaAI can smoothly incorporate constant drift monitoring and management.
Customizable Alerts: You can tailor alert thresholds and notifications according to your explicit requirements. This adaptability ensures that you are only informed of substantial drifts that could affect your models, reducing noise and permitting you to concentrate on integral issues.
By using RagaAI’s robust drift detection and management capabilities, ventures can ensure that their models remain powerful and precise, even as fundamental data alterations. This dynamic approach to managing data drift not only enhances model performance but also contributes to more dependable and trustworthy AI systems.
For more detailed insights on drift detection in object detection models, check out the Ragaai Prism documentation on drift detection.
Conclusion
Incorporating data drift management into the ML model lifecycle will improve model dependability and performance, driving better venture results and more precise forecasts.
By comprehending and acknowledging data drift, you can ensure your machine-learning models remain powerful, precise, and dependable. Stay alert, observe constantly, and clasp automation to tackle data drift efficiently.
Sign up now and revolutionize your AI quality management with Raga AI. Don't let data drift hold you back—join us and stay ahead of the curve!
Ever wondered why your machine-learning models lose their efficacy over time? The culprit might be data drift. In the rapidly evolving scenario of machine learning, comprehending and acknowledging data drift is paramount to maintaining the precision and dependability of your models.
In the continuously progressing scenario of machine learning, one hidden opponent can inaudibly vandalize your model's performance: data drift.
Data Drift in the Context of Machine Learning
Data drift refers to the alterations in data dispensations over time, which can sternly affect the performance of machine learning models. As models are often trained on factual information, any shift in the fundamental data patterns—be it progressive or sudden—can lead to inaccuracies and reduced efficiency in auguring tasks. Comprehending and acknowledging data drift is vital for maintaining powerful and dependable ML systems.
ML Model Assumptions and Their Implications
When evolving ML models, numerous key theories are made about the information:
Stationarity: The statistical properties of the data remain perpetual over time.
IID (Independent and Identically Distributed): The training data is a delegate of the real-world data the model will confront.
Breaching these presumptions can lead to substantial performance deterioration. For instance, if the distribution of attributes in new data diverges from the training data, the model's forecasts may become less precise or even solely incorrect. This makes the monitoring and detection of data drift a crucial part of the ML lifecycle.
How Real-World Data Deviations Cause Data Drift
In the real world, data is sometimes stagnant. It unfolds due to numerous factors, such as alterations in user behavior, market trends, or external events. These deviations mean that the patterns the model learned during training no longer hold, leading to data drift. For example, a model forecasting customer behavior based on past buying data might become less precise if consumer choices shift due to a new trend. Consequently, constant monitoring and updating of models are necessary to adjust to these alterations and maintain performance.
Explore more insights on AI innovations in our guide to Enhancing Computer Vision with Synthetic Data: Advantages and Generation Techniques.
Understanding Data Drift
Ever felt like your data is betraying you? That's probably data drift at play. Let's dive into what it means and why it happens.
Definition and Types of Data Drift
Data drift is when the statistical properties of your data alter over time. This can mess up your machine-learning models, making them less precise and dependable. There are two main types of data drift you need to know about:
Concept Drift: This occurs when the relationship between input data and output changes. Imagine a model forecasting customer choices. Over time, as trends change, the choices shift, leading to concept drift.
Covariate Drift: This happens when the allocation of input data alters. For example, if you're dissecting website traffic and suddenly see a spike from a new region, that’s covariate drift.
Causes of Data Drift
Why does data drift happen? There are numerous reasons, but let’s concentrate on two big ones:
Time Dimension: Over time, everything advances. Customer choices, market trends, and even the data itself alter. This is a natural cause of data drift.
External Factors: Technological progressions and societal alterations can lead to data drift. For instance, a new social media platform might alter how people communicate online, or a universal event could shift buying behaviors.
Impact of Data Drift
Data drift can have a significant impact on your business decisions, model accuracy, and user behavior. Here’s how:
Business Decisions: Relying on outdated models can lead to poor business choices. If your model doesn’t account for current trends, you might miss out on opportunities or face unexpected challenges.
Model Accuracy: Data drift reduces the accuracy of your predictive models. An inaccurate model means poor predictions, leading to flawed strategies.
User Behavior: If your business depends on understanding user behavior, data drift can lead to misinterpretations. This affects everything from marketing campaigns to product recommendations.
Nuances Between Data Drift, Concept Drift, and Related Terms
Comprehending the nuances between these terms is critical. While data drift is an expansive concept, concept drift especially refers to alterations in the relationship between inputs and outputs. Meanwhile, covariate drift concentrates on alterations in the input data dispensation. Other related terms include:
Label Drift: When the allocation of the target variable alters over time.
Feature Drift: When individual attributes within the data alter independently of the others.
By determining and acknowledging data drift, you can maintain the precision and dependability of your models, ensuring better decision-making and user experiences. Stay alert and keep your data in check!
For an understanding of the Importance of Accuracy and Reliability in Tabular Data Models, check out our comprehensive guide.
The Importance of Data Drift Detection
Let’s now examine the significance of Data Drift detection:
Significance of Data Drift Monitoring
Do you know why your machine learning model's performance suddenly deteriorates? The perpetrator might be data drift. Discerning and monitoring data drift is vital to maintaining the quality of your model. When you observe data drift, you ensure your model stays precise and dependable over time.
Early Detection of Data Drift to Prevent Model Degradation
Data drift, the silent destroyer, can lead to substantial model deterioration. If you let it go rampant, it can render your model ineffectual, causing headaches and lost opportunities. That's why early detection is necessary. By detecting data drift early, you can take reformative actions before your model’s performance suffers.
Data Drift: Early Warnings and Model Debugging
Data drift detection isn't just about damage control; it plays a crucial role in model preservation. It acts as an early cautioning system, warning you of probable problems before they intensify. This dynamic approach helps in debugging models effectively, saving time and resources.
In short, monitoring data drift keeps your models sharp, helps you catch issues early, and aids sleek model maintenance.
Discover more insights on Understanding ML Model Monitoring in Production to ensure your models are performing at their best.
Methods and Tools for Data Drift Detection
Let's learn some efficient methods and tools for detecting data drift to ensure your models stay precise and dependable:
Overview of Data Drift Detection Methods
There are numerous ways to detect data drift, including statistical tests, distance metrics, and machine learning models. Each approach provides eccentric benefits:
Statistical tests contrast allocations to determine substantial alterations.
Distance metrics gauge the divergence between data dispensations.
Machine learning models forecast and flag possible drifts based on factual information.
Introduction to Specific Detection Methodologies
Let’s get more precise with some eminent methodologies:
Kolmogorov-Smirnov (K-S) Test: This non-parametric test contrasts the allocation of two datasets. It’s specifically advantageous for constant variables, emphasizing distinctions that could impact your model.
Population Stability Index (PSI): PSI gauges the firmness of unambiguous or numerical attributes over time. A high PSI indicates a substantial shift in data dispensation, waving potential drift.
Page-Hinkley Method: Designed for real-time monitoring, this method discovers sudden alterations in data streams. It’s gradually efficient for applications requiring prompt drift detection.
Automated Detection Tools
Why do it manually when automation can save you time and effort? Here are some automated tools you can use:
Evidently AI: This open-source library provides thorough reports on data drift, including statistical tests and visualizations.
Azure ML’s Data Drift Detection Features: Microsoft Azure’s machine learning platform provides built-in tools for monitoring and managing data drift, incorporating smoothly with your existing workflows.
Alternative Approaches: Other tools like DataRobot and Alteryx provide powerful drift detection capabilities, each with unique attributes customized to various requirements.
Implementing Data Drift Detection in Production
To efficiently scuffle data drift, follow these strategies:
Integrate Continuous Monitoring: Frequently monitor your data pipelines and model outputs. Automated warnings can inform you of potential drifts before they affect your model's performance.
Define Thresholds: Set clear thresholds for adequate data dispensation alterations. This will help you differentiate between minor changes and substantial drifts.
Leverage Version Control: Keep track of data and model versions to determine when and where drifts occur. This will provide faster diagnostics and refinement.
Regularly Retrain Models: Schedule frequent model retraining sessions based on updated data to ensure your models remain precise and efficient.
Detecting data drift is vital for maintaining the precision and dependability of your machine-learning models. By using statistical tests, distance metrics, machine learning models, and automated tools, you can stay ahead of data drift and ensure your models perform at their best.
For a dissection, check out our guide on Understanding the Basics of LLM Fine-Tuning with Custom Data.
Strategies for Identifying and Managing Data Drift
Let’s now know about strategic ways to stay ahead of the game. Here’s how you can tackle data drift like a pro:
Robust Continuous Monitoring
Can you drive your car without checking your mirrors? Observing your data constantly works the same way. You need to set up automated systems to trace alterations in your data's allocation over time. Use tools that give real-time insights into your data, helping you catch peculiarities before they turn into bigger problems. Think of it as a health inspection for your data, ensuring everything runs effortlessly and efficiently.
Master Data Drift: Retrain, Adjust, Learn
Once you detect data drift, it’s time to take action. Here are three robust approaches:
Retraining Models: Frequently retrain your models with the most recent data to keep them sharp and up-to-date. This helps maintain their auguring power and pertinence.
Adjusting Data Pipelines: Refine your data pipelines to adapt alterations in data patterns. This might mean tweaking preprocessing steps or distorting feature engineering methodologies.
Using Incremental Learning: Integrate gradual comprehension techniques that permit your models to adjust increasingly to new data without the requirement for complete retraining. This is specifically useful for large datasets where retraining can be resource-intensive.
Importance of Feature Selection in Drift Detection and Management
Not all attributes are created equal. Some can be more sensitive to alterations, leading to substantial drift. By precisely choosing and observing critical attributes, you can detect drift early and take corrective measures. Frequently retrospect your attribute set and exclude those that contribute little to the model’s performance or are prone to drift. This keeps your model lean, effective, and flexible against data drift.
Custom Alerts, Dataset Monitors, and Explainable AI
Stay ahead with personalized alerts and dataset monitors that notify you promptly when data drift occurs. Set thresholds and prompts accurate to your venture requirements, so you’re always in the loop. Moreover, using Explainable AI can provide insights into why your models are complying distinctly, providing a clearer picture of the underlying drift causes. This lucidity helps you make informed decisions and refine your strategies efficiently.
Ready to automate your data drift detection and save your valuable time? Let’s dive into why automation is a game changer.
Want to learn about Deep Learning Applications? Read our detailed article on Exploring The Frontiers Of Deep Learning Applications.
Automating Data Drift Detection
Are you languishing to keep your machine learning models precise and pertinent? Automating data drift detection could be your groundbreaker! Here's why you need it, how to set it up, and some alternatives if you prefer a more hands-on approach.
Benefits of Automating Data Drift Detection
Automated data drift detection provides effectiveness and readiness for supervising your machine learning models. You won't spend innumerable hours manually checking for inconsistencies or shifts in data dispensations. With automation, you can instantly identify any data alterations affecting your model's performance. This means you can swiftly adapt your models, maintaining their precision and dependability. In addition, automated systems manage large datasets and intricate data pipelines, making them indispensable for modern data-driven ventures.
Setting Up Automated Data Drift Detection in Azure ML
Setting up automated data drift detection in Azure ML is a direct process. Here’s a quick outline to get you started:
Create an Azure ML Workspace: Begin by setting up your workspace where all your experiments, models, and information will reside.
Ingest Your Data: Use Azure Data Factory or Azure Blob Storage to bring your data into the workspace.
Register Your Dataset: Register your threshold dataset (the original data) and the new incoming datasets.
Set Up a Data Drift Monitor: Azure ML provides a data drift detection attribute. Configure this by choosing the baseline dataset, the target dataset, and the attributes to observe.
Configure Alerts: Set up alerts to notify you when substantial data drift is discovered. You can choose email notifications or incorporate them with Azure DevOps for more sleek productivity.
Alternatives to Automated Tools
If you prefer more control over your data drift detection, you can opt for custom scripts or open-source libraries. Here are a few eminent options:
Custom Scripts: Writing your scripts in Python or R permits you to customize the drift detection procedure to your precise requirements. You can use libraries like Pandas, NumPy, and SciPy to create comprehensive drift reports.
Open-Source Libraries: Tools like Evidently and Scikit-Multiflow offer pre-built functions for data drift detection. These libraries offer adaptability and personalization, giving you the ability to correct the algorithms to suit your data and models better.
Incorporating Automation and Explainability
Incorporating both automation and explainability into your data drift management plan ensures that you not only detect drift quickly but also comprehend why it happens. Here’s how you can do it:
Automate Monitoring: Use automated tools to monitor your data for drift constantly. This reduces manual workload and ensures prompt discovery.
Explainable AI (XAI) Tools: Incorporate XAI tools like SHAP or LIME to elucidate the data drift. These tools help you comprehend which attributes are altering and how they affect your model's performance.
Documentation and Reporting: Keep comprehensive records of detected drifts and the steps taken to acknowledge them. This practice helps in tracking trends over time and enhances your overall data governance.
Discover the necessities of compliance automation in our guide on getting started with regulatory management.
How RagaAi Can Help in Tackling Data Drift
When it comes to tackling data drift, RagaAI provides a pragmatic solution designed to detect and address data anomalies effectively. Our platform provides powerful tools and methodologies to ensure your models remain precise and dependable over time. Here’s how RagaAI can assist:
Advanced Drift Detection: RagaAI’s Prism module includes an advanced drift detection system that recognizes shifts in data distribution. It observes input data and alerts you when substantial deviations occur, enabling instant corrective actions.
Real-time Monitoring: With RagaAI, you can set up real-time monitoring for your data streams. This ensures that any data drift is detected as soon as it happens, minimizing the impact on your models’ performance. The constant monitoring attribute helps in maintaining model precision and dependability.
Automated Response Mechanisms: RagaAI incorporates automated response mechanisms to manage data drift. Once drift is detected, predefined actions can be triggered automatically, such as retraining models or adapting data preprocessing steps. This automation reduces the time and effort needed to tackle drift manually.
Comprehensive Analytics: The platform provides comprehensive analytics and visualizations to comprehend the nature and cause of data drift. By using these insights, you can make informed decisions about how to acknowledge and alleviate drift in your models.
Easy Integration: RagaAi’s tools are designed for easy incorporation with your existing data pipelines. Whether you’re using cloud-based services or on-premise systems, RagaAI can smoothly incorporate constant drift monitoring and management.
Customizable Alerts: You can tailor alert thresholds and notifications according to your explicit requirements. This adaptability ensures that you are only informed of substantial drifts that could affect your models, reducing noise and permitting you to concentrate on integral issues.
By using RagaAI’s robust drift detection and management capabilities, ventures can ensure that their models remain powerful and precise, even as fundamental data alterations. This dynamic approach to managing data drift not only enhances model performance but also contributes to more dependable and trustworthy AI systems.
For more detailed insights on drift detection in object detection models, check out the Ragaai Prism documentation on drift detection.
Conclusion
Incorporating data drift management into the ML model lifecycle will improve model dependability and performance, driving better venture results and more precise forecasts.
By comprehending and acknowledging data drift, you can ensure your machine-learning models remain powerful, precise, and dependable. Stay alert, observe constantly, and clasp automation to tackle data drift efficiently.
Sign up now and revolutionize your AI quality management with Raga AI. Don't let data drift hold you back—join us and stay ahead of the curve!
Ever wondered why your machine-learning models lose their efficacy over time? The culprit might be data drift. In the rapidly evolving scenario of machine learning, comprehending and acknowledging data drift is paramount to maintaining the precision and dependability of your models.
In the continuously progressing scenario of machine learning, one hidden opponent can inaudibly vandalize your model's performance: data drift.
Data Drift in the Context of Machine Learning
Data drift refers to the alterations in data dispensations over time, which can sternly affect the performance of machine learning models. As models are often trained on factual information, any shift in the fundamental data patterns—be it progressive or sudden—can lead to inaccuracies and reduced efficiency in auguring tasks. Comprehending and acknowledging data drift is vital for maintaining powerful and dependable ML systems.
ML Model Assumptions and Their Implications
When evolving ML models, numerous key theories are made about the information:
Stationarity: The statistical properties of the data remain perpetual over time.
IID (Independent and Identically Distributed): The training data is a delegate of the real-world data the model will confront.
Breaching these presumptions can lead to substantial performance deterioration. For instance, if the distribution of attributes in new data diverges from the training data, the model's forecasts may become less precise or even solely incorrect. This makes the monitoring and detection of data drift a crucial part of the ML lifecycle.
How Real-World Data Deviations Cause Data Drift
In the real world, data is sometimes stagnant. It unfolds due to numerous factors, such as alterations in user behavior, market trends, or external events. These deviations mean that the patterns the model learned during training no longer hold, leading to data drift. For example, a model forecasting customer behavior based on past buying data might become less precise if consumer choices shift due to a new trend. Consequently, constant monitoring and updating of models are necessary to adjust to these alterations and maintain performance.
Explore more insights on AI innovations in our guide to Enhancing Computer Vision with Synthetic Data: Advantages and Generation Techniques.
Understanding Data Drift
Ever felt like your data is betraying you? That's probably data drift at play. Let's dive into what it means and why it happens.
Definition and Types of Data Drift
Data drift is when the statistical properties of your data alter over time. This can mess up your machine-learning models, making them less precise and dependable. There are two main types of data drift you need to know about:
Concept Drift: This occurs when the relationship between input data and output changes. Imagine a model forecasting customer choices. Over time, as trends change, the choices shift, leading to concept drift.
Covariate Drift: This happens when the allocation of input data alters. For example, if you're dissecting website traffic and suddenly see a spike from a new region, that’s covariate drift.
Causes of Data Drift
Why does data drift happen? There are numerous reasons, but let’s concentrate on two big ones:
Time Dimension: Over time, everything advances. Customer choices, market trends, and even the data itself alter. This is a natural cause of data drift.
External Factors: Technological progressions and societal alterations can lead to data drift. For instance, a new social media platform might alter how people communicate online, or a universal event could shift buying behaviors.
Impact of Data Drift
Data drift can have a significant impact on your business decisions, model accuracy, and user behavior. Here’s how:
Business Decisions: Relying on outdated models can lead to poor business choices. If your model doesn’t account for current trends, you might miss out on opportunities or face unexpected challenges.
Model Accuracy: Data drift reduces the accuracy of your predictive models. An inaccurate model means poor predictions, leading to flawed strategies.
User Behavior: If your business depends on understanding user behavior, data drift can lead to misinterpretations. This affects everything from marketing campaigns to product recommendations.
Nuances Between Data Drift, Concept Drift, and Related Terms
Comprehending the nuances between these terms is critical. While data drift is an expansive concept, concept drift especially refers to alterations in the relationship between inputs and outputs. Meanwhile, covariate drift concentrates on alterations in the input data dispensation. Other related terms include:
Label Drift: When the allocation of the target variable alters over time.
Feature Drift: When individual attributes within the data alter independently of the others.
By determining and acknowledging data drift, you can maintain the precision and dependability of your models, ensuring better decision-making and user experiences. Stay alert and keep your data in check!
For an understanding of the Importance of Accuracy and Reliability in Tabular Data Models, check out our comprehensive guide.
The Importance of Data Drift Detection
Let’s now examine the significance of Data Drift detection:
Significance of Data Drift Monitoring
Do you know why your machine learning model's performance suddenly deteriorates? The perpetrator might be data drift. Discerning and monitoring data drift is vital to maintaining the quality of your model. When you observe data drift, you ensure your model stays precise and dependable over time.
Early Detection of Data Drift to Prevent Model Degradation
Data drift, the silent destroyer, can lead to substantial model deterioration. If you let it go rampant, it can render your model ineffectual, causing headaches and lost opportunities. That's why early detection is necessary. By detecting data drift early, you can take reformative actions before your model’s performance suffers.
Data Drift: Early Warnings and Model Debugging
Data drift detection isn't just about damage control; it plays a crucial role in model preservation. It acts as an early cautioning system, warning you of probable problems before they intensify. This dynamic approach helps in debugging models effectively, saving time and resources.
In short, monitoring data drift keeps your models sharp, helps you catch issues early, and aids sleek model maintenance.
Discover more insights on Understanding ML Model Monitoring in Production to ensure your models are performing at their best.
Methods and Tools for Data Drift Detection
Let's learn some efficient methods and tools for detecting data drift to ensure your models stay precise and dependable:
Overview of Data Drift Detection Methods
There are numerous ways to detect data drift, including statistical tests, distance metrics, and machine learning models. Each approach provides eccentric benefits:
Statistical tests contrast allocations to determine substantial alterations.
Distance metrics gauge the divergence between data dispensations.
Machine learning models forecast and flag possible drifts based on factual information.
Introduction to Specific Detection Methodologies
Let’s get more precise with some eminent methodologies:
Kolmogorov-Smirnov (K-S) Test: This non-parametric test contrasts the allocation of two datasets. It’s specifically advantageous for constant variables, emphasizing distinctions that could impact your model.
Population Stability Index (PSI): PSI gauges the firmness of unambiguous or numerical attributes over time. A high PSI indicates a substantial shift in data dispensation, waving potential drift.
Page-Hinkley Method: Designed for real-time monitoring, this method discovers sudden alterations in data streams. It’s gradually efficient for applications requiring prompt drift detection.
Automated Detection Tools
Why do it manually when automation can save you time and effort? Here are some automated tools you can use:
Evidently AI: This open-source library provides thorough reports on data drift, including statistical tests and visualizations.
Azure ML’s Data Drift Detection Features: Microsoft Azure’s machine learning platform provides built-in tools for monitoring and managing data drift, incorporating smoothly with your existing workflows.
Alternative Approaches: Other tools like DataRobot and Alteryx provide powerful drift detection capabilities, each with unique attributes customized to various requirements.
Implementing Data Drift Detection in Production
To efficiently scuffle data drift, follow these strategies:
Integrate Continuous Monitoring: Frequently monitor your data pipelines and model outputs. Automated warnings can inform you of potential drifts before they affect your model's performance.
Define Thresholds: Set clear thresholds for adequate data dispensation alterations. This will help you differentiate between minor changes and substantial drifts.
Leverage Version Control: Keep track of data and model versions to determine when and where drifts occur. This will provide faster diagnostics and refinement.
Regularly Retrain Models: Schedule frequent model retraining sessions based on updated data to ensure your models remain precise and efficient.
Detecting data drift is vital for maintaining the precision and dependability of your machine-learning models. By using statistical tests, distance metrics, machine learning models, and automated tools, you can stay ahead of data drift and ensure your models perform at their best.
For a dissection, check out our guide on Understanding the Basics of LLM Fine-Tuning with Custom Data.
Strategies for Identifying and Managing Data Drift
Let’s now know about strategic ways to stay ahead of the game. Here’s how you can tackle data drift like a pro:
Robust Continuous Monitoring
Can you drive your car without checking your mirrors? Observing your data constantly works the same way. You need to set up automated systems to trace alterations in your data's allocation over time. Use tools that give real-time insights into your data, helping you catch peculiarities before they turn into bigger problems. Think of it as a health inspection for your data, ensuring everything runs effortlessly and efficiently.
Master Data Drift: Retrain, Adjust, Learn
Once you detect data drift, it’s time to take action. Here are three robust approaches:
Retraining Models: Frequently retrain your models with the most recent data to keep them sharp and up-to-date. This helps maintain their auguring power and pertinence.
Adjusting Data Pipelines: Refine your data pipelines to adapt alterations in data patterns. This might mean tweaking preprocessing steps or distorting feature engineering methodologies.
Using Incremental Learning: Integrate gradual comprehension techniques that permit your models to adjust increasingly to new data without the requirement for complete retraining. This is specifically useful for large datasets where retraining can be resource-intensive.
Importance of Feature Selection in Drift Detection and Management
Not all attributes are created equal. Some can be more sensitive to alterations, leading to substantial drift. By precisely choosing and observing critical attributes, you can detect drift early and take corrective measures. Frequently retrospect your attribute set and exclude those that contribute little to the model’s performance or are prone to drift. This keeps your model lean, effective, and flexible against data drift.
Custom Alerts, Dataset Monitors, and Explainable AI
Stay ahead with personalized alerts and dataset monitors that notify you promptly when data drift occurs. Set thresholds and prompts accurate to your venture requirements, so you’re always in the loop. Moreover, using Explainable AI can provide insights into why your models are complying distinctly, providing a clearer picture of the underlying drift causes. This lucidity helps you make informed decisions and refine your strategies efficiently.
Ready to automate your data drift detection and save your valuable time? Let’s dive into why automation is a game changer.
Want to learn about Deep Learning Applications? Read our detailed article on Exploring The Frontiers Of Deep Learning Applications.
Automating Data Drift Detection
Are you languishing to keep your machine learning models precise and pertinent? Automating data drift detection could be your groundbreaker! Here's why you need it, how to set it up, and some alternatives if you prefer a more hands-on approach.
Benefits of Automating Data Drift Detection
Automated data drift detection provides effectiveness and readiness for supervising your machine learning models. You won't spend innumerable hours manually checking for inconsistencies or shifts in data dispensations. With automation, you can instantly identify any data alterations affecting your model's performance. This means you can swiftly adapt your models, maintaining their precision and dependability. In addition, automated systems manage large datasets and intricate data pipelines, making them indispensable for modern data-driven ventures.
Setting Up Automated Data Drift Detection in Azure ML
Setting up automated data drift detection in Azure ML is a direct process. Here’s a quick outline to get you started:
Create an Azure ML Workspace: Begin by setting up your workspace where all your experiments, models, and information will reside.
Ingest Your Data: Use Azure Data Factory or Azure Blob Storage to bring your data into the workspace.
Register Your Dataset: Register your threshold dataset (the original data) and the new incoming datasets.
Set Up a Data Drift Monitor: Azure ML provides a data drift detection attribute. Configure this by choosing the baseline dataset, the target dataset, and the attributes to observe.
Configure Alerts: Set up alerts to notify you when substantial data drift is discovered. You can choose email notifications or incorporate them with Azure DevOps for more sleek productivity.
Alternatives to Automated Tools
If you prefer more control over your data drift detection, you can opt for custom scripts or open-source libraries. Here are a few eminent options:
Custom Scripts: Writing your scripts in Python or R permits you to customize the drift detection procedure to your precise requirements. You can use libraries like Pandas, NumPy, and SciPy to create comprehensive drift reports.
Open-Source Libraries: Tools like Evidently and Scikit-Multiflow offer pre-built functions for data drift detection. These libraries offer adaptability and personalization, giving you the ability to correct the algorithms to suit your data and models better.
Incorporating Automation and Explainability
Incorporating both automation and explainability into your data drift management plan ensures that you not only detect drift quickly but also comprehend why it happens. Here’s how you can do it:
Automate Monitoring: Use automated tools to monitor your data for drift constantly. This reduces manual workload and ensures prompt discovery.
Explainable AI (XAI) Tools: Incorporate XAI tools like SHAP or LIME to elucidate the data drift. These tools help you comprehend which attributes are altering and how they affect your model's performance.
Documentation and Reporting: Keep comprehensive records of detected drifts and the steps taken to acknowledge them. This practice helps in tracking trends over time and enhances your overall data governance.
Discover the necessities of compliance automation in our guide on getting started with regulatory management.
How RagaAi Can Help in Tackling Data Drift
When it comes to tackling data drift, RagaAI provides a pragmatic solution designed to detect and address data anomalies effectively. Our platform provides powerful tools and methodologies to ensure your models remain precise and dependable over time. Here’s how RagaAI can assist:
Advanced Drift Detection: RagaAI’s Prism module includes an advanced drift detection system that recognizes shifts in data distribution. It observes input data and alerts you when substantial deviations occur, enabling instant corrective actions.
Real-time Monitoring: With RagaAI, you can set up real-time monitoring for your data streams. This ensures that any data drift is detected as soon as it happens, minimizing the impact on your models’ performance. The constant monitoring attribute helps in maintaining model precision and dependability.
Automated Response Mechanisms: RagaAI incorporates automated response mechanisms to manage data drift. Once drift is detected, predefined actions can be triggered automatically, such as retraining models or adapting data preprocessing steps. This automation reduces the time and effort needed to tackle drift manually.
Comprehensive Analytics: The platform provides comprehensive analytics and visualizations to comprehend the nature and cause of data drift. By using these insights, you can make informed decisions about how to acknowledge and alleviate drift in your models.
Easy Integration: RagaAi’s tools are designed for easy incorporation with your existing data pipelines. Whether you’re using cloud-based services or on-premise systems, RagaAI can smoothly incorporate constant drift monitoring and management.
Customizable Alerts: You can tailor alert thresholds and notifications according to your explicit requirements. This adaptability ensures that you are only informed of substantial drifts that could affect your models, reducing noise and permitting you to concentrate on integral issues.
By using RagaAI’s robust drift detection and management capabilities, ventures can ensure that their models remain powerful and precise, even as fundamental data alterations. This dynamic approach to managing data drift not only enhances model performance but also contributes to more dependable and trustworthy AI systems.
For more detailed insights on drift detection in object detection models, check out the Ragaai Prism documentation on drift detection.
Conclusion
Incorporating data drift management into the ML model lifecycle will improve model dependability and performance, driving better venture results and more precise forecasts.
By comprehending and acknowledging data drift, you can ensure your machine-learning models remain powerful, precise, and dependable. Stay alert, observe constantly, and clasp automation to tackle data drift efficiently.
Sign up now and revolutionize your AI quality management with Raga AI. Don't let data drift hold you back—join us and stay ahead of the curve!
Subscribe to our newsletter to never miss an update
Other articles


Agents Talk, Models Think: A2A + MCP for Enterprise Agentic AI

Riya Parikh, Nitai Agarwal
Apr 27, 2025
Read the article
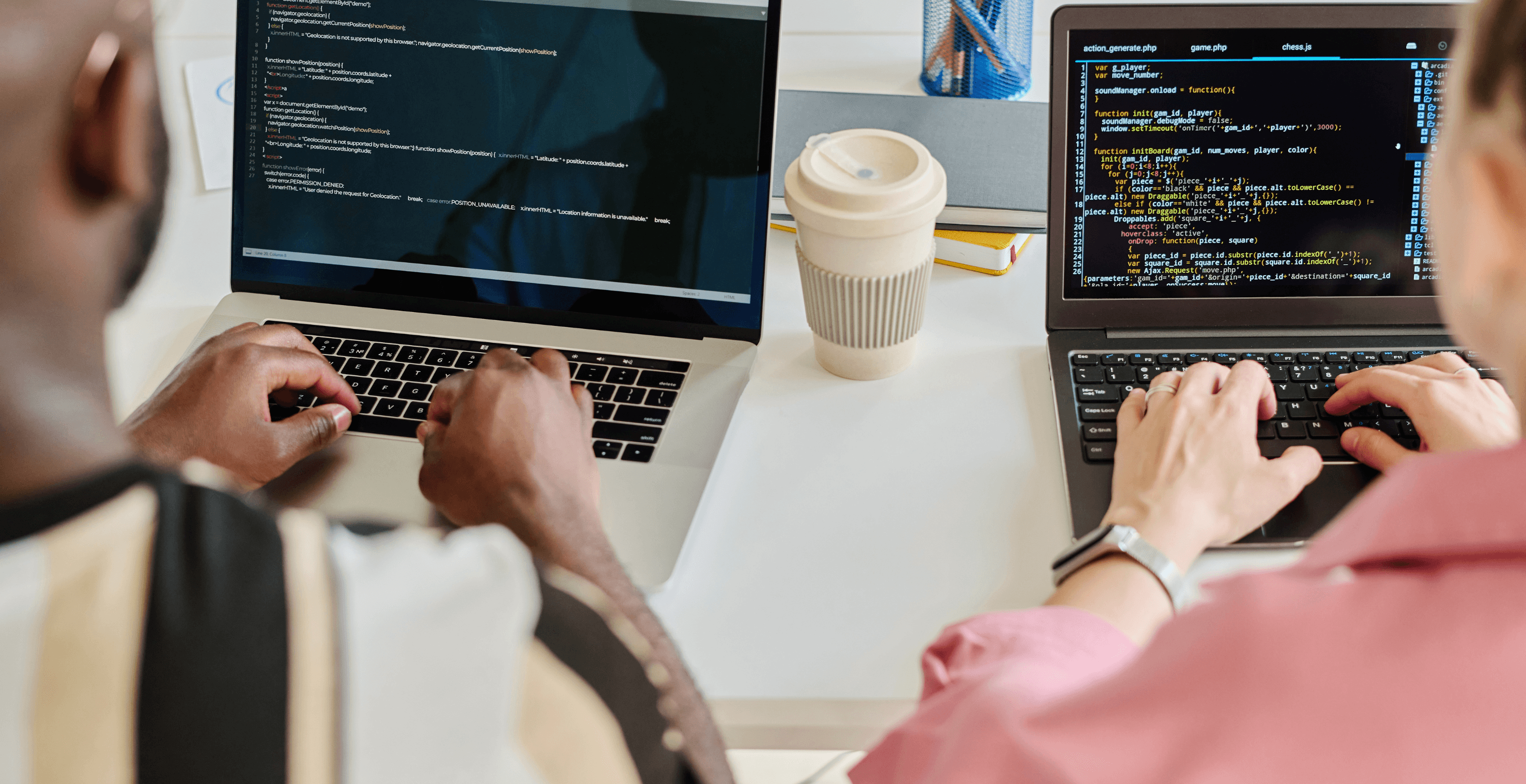
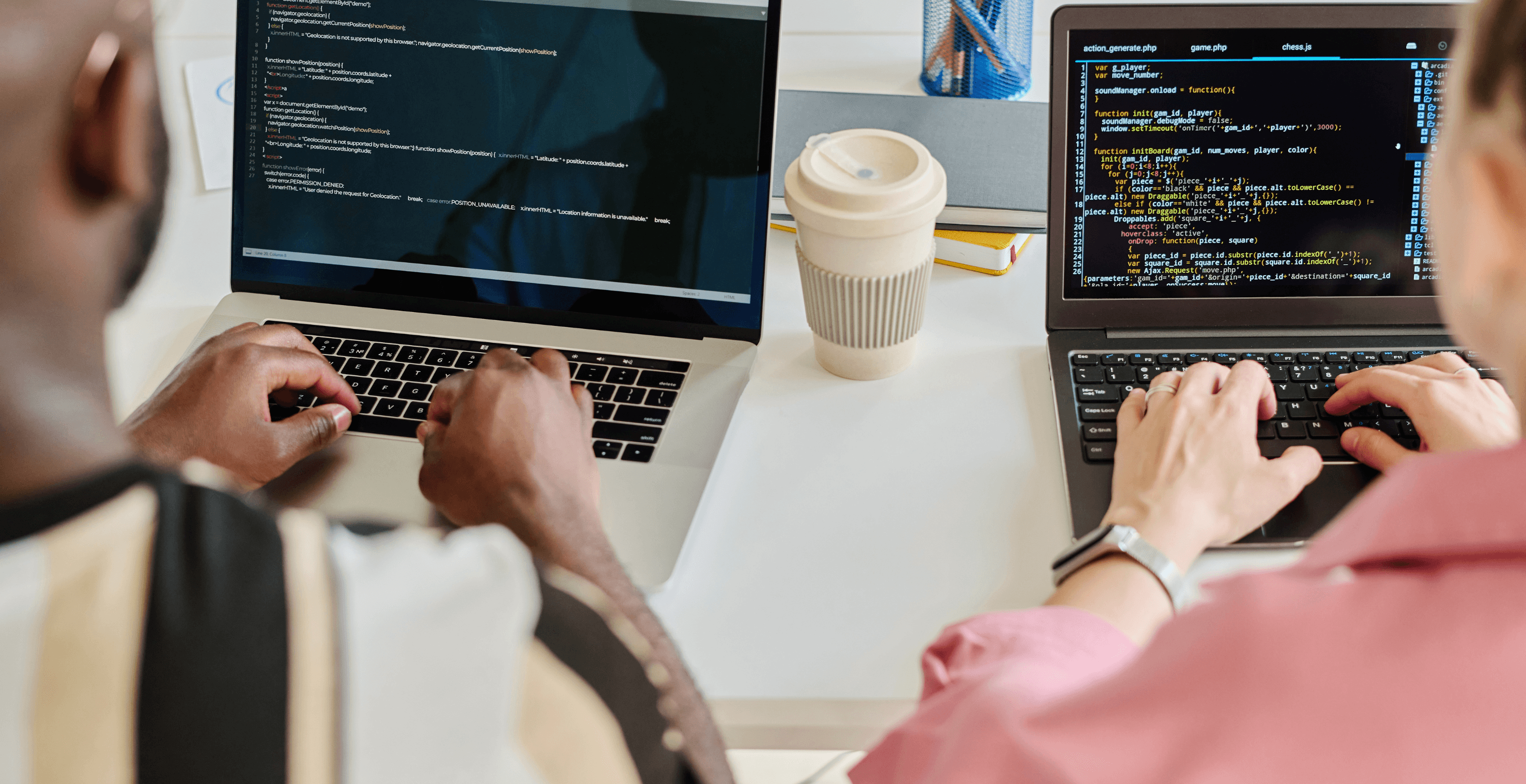
Understanding NeMo Guardrails: A Toolkit for LLM Security

Rehan Asif
Dec 24, 2024
Read the article


Understanding Differences in Large vs Small Language Models (LLM vs SLM)

Rehan Asif
Dec 21, 2024
Read the article