Enhancing Computer Vision with Synthetic Data: Advantages and Generation Techniques
Enhancing Computer Vision with Synthetic Data: Advantages and Generation Techniques
Enhancing Computer Vision with Synthetic Data: Advantages and Generation Techniques
Jigar Gupta
Apr 20, 2024




Synthetic data refers to any data that are artificially generated rather than obtained by direct measurement. In the realm of computer vision, synthetic data typically includes images or videos created digitally using various algorithms and simulation technologies.
This data serves as a stand-in for real-world data during the training phases of machine learning models, mainly when accurate data is scarce, expensive, or sensitive.
The demand for high-quality training data has skyrocketed as computer vision technologies evolve and find applications in diverse fields such as autonomous driving, medical imaging, and retail. Synthetic data has emerged as a crucial resource because it can be generated in large volumes and tailored to specific needs without the logistical and ethical complications of collecting real-world data.
Advantages of Synthetic Data
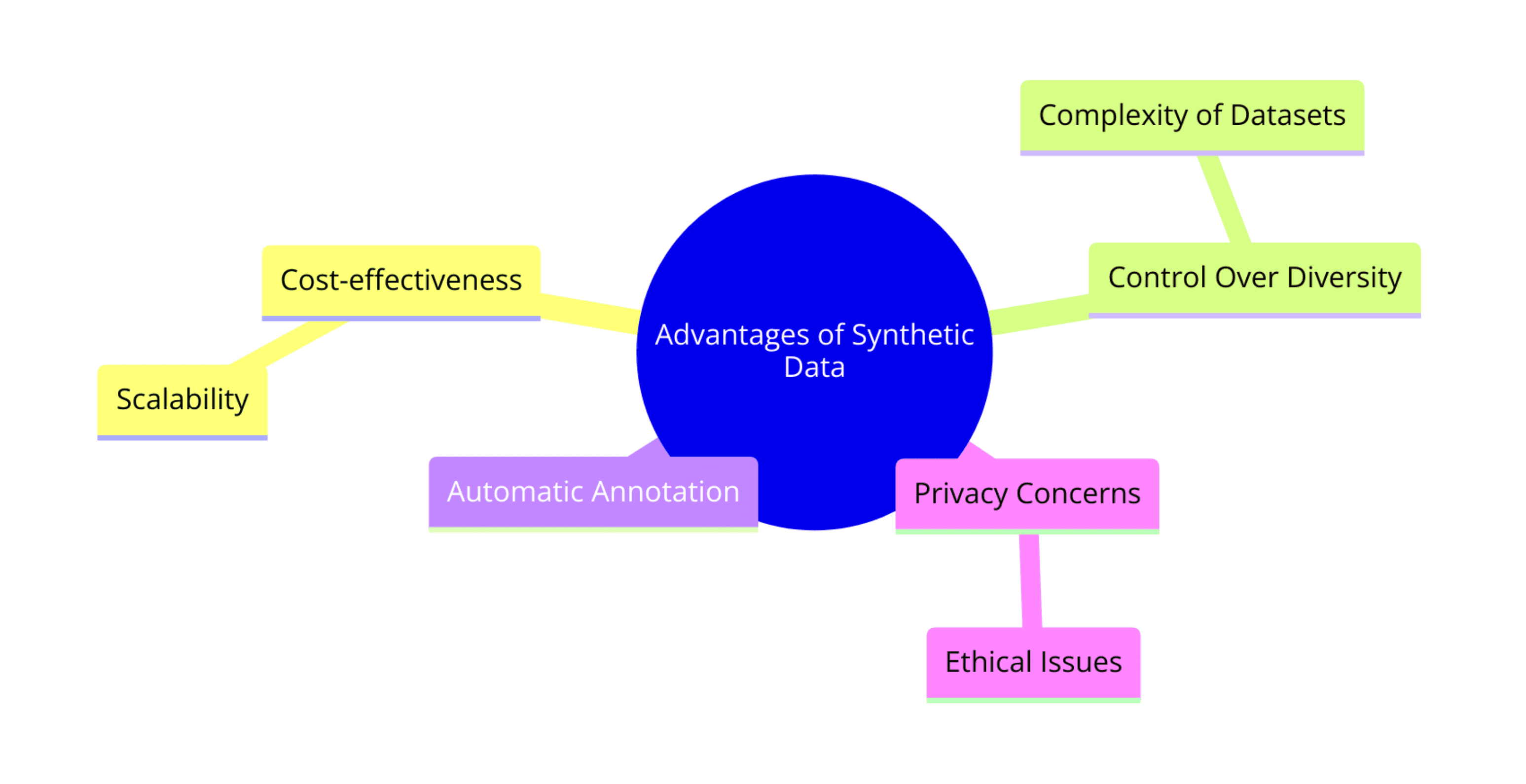
Let's explore synthetic data's significant advantages in computer vision, highlighting its cost-effectiveness, control over dataset properties, and other key benefits.
Cost-effectiveness and Scalability
One of the most compelling advantages of synthetic data is its cost-effectiveness. Generating synthetic images or videos typically requires only the initial investment in software or the development of digital environments.
Once set up, these systems can produce vast amounts of data at a fraction of the cost of traditional data-gathering methods. This scalability allows for the rapid expansion of datasets as needed without additional significant costs.
Discover how RagaAI Enhances Cost-effectiveness with RagaAI for synthetic data in computer vision.
Control Over Diversity and Complexity of Datasets
Synthetic data generation tools provide unprecedented control over the properties of the datasets. Developers can adjust and manipulate lighting, weather conditions, object configurations, and backgrounds. This ability is precious in fields like autonomous driving, where varied scenarios (like different weather and lighting conditions) are crucial for comprehensive systems training.
Ability to Generate Annotated Data Automatically
Another significant benefit of synthetic data is that it can be generated with automatic annotations. This is crucial for training machine learning models since labeled data (data tagged with information like object boundaries and classifications) is typically required.
Generating annotated data manually is often one of the most time-consuming and labor-intensive aspects of preparing training datasets. Synthetic data tools can automate this process, providing perfectly accurate labels and significantly reducing the time and labor involved.
Explore RagaAI's innovative approach to Controlling Dataset Diversity with RagaAI for synthetic data generation.
Reduction of Privacy Concerns and Ethical Issues
Using real-world data, especially in sensitive areas such as surveillance or personal devices, can raise significant privacy and ethical concerns. Synthetic data eliminates many of these issues as it does not involve real individuals or scenarios. This aspect not only simplifies compliance with data protection regulations but also opens up possibilities for research in areas where privacy concerns might restrict the use of accurate data.
These advantages make synthetic data a powerful tool in developing and training computer vision systems, offering challenging efficiencies and capabilities to achieve with real-world data alone.
Challenges in Using Synthetic Data
While synthetic data offers many advantages, it's essential to recognize and address its challenges to ensure effective outcomes in computer vision applications.
Maintaining Realistic Variations and Fidelity
One of the primary challenges with synthetic data is achieving a high level of realism and maintaining variations that accurately mimic the real world. Synthetic datasets must be designed to include realistic textures, lighting variations, and environmental dynamics. This requires sophisticated modeling techniques and a deep understanding of the physical properties being simulated, which can be complex and resource-intensive to develop.
Ensuring the Synthetic Data is Representative of Real-World Scenarios
Synthetic data must encompass various scenarios and conditions to effectively train robust computer vision models. Ensuring that these datasets represent real-world complexities involves significant domain expertise and continuous refinement of the simulation parameters.
There's a risk that models trained predominantly on synthetic data may perform poorly in real-life situations if the data lacks diversity or fails to cover rare but critical scenarios.
Bridging the Domain Gap Between Synthetic and Real Data
The domain gap — the difference in how models perform on synthetic versus accurate data — presents a significant challenge. Even high-quality synthetic data may not perfectly capture real-world data's subtleties and unpredictable nature, leading to models that are overfitted to the synthetic characteristics and underperform when faced with actual data.
This gap requires ongoing efforts in domain adaptation and continuous testing against real-world data sets to ensure that the models remain effective and reliable.
Synthetic Data Generation Techniques
Let's explore the various techniques employed to generate high-quality synthetic data, which are crucial in developing and training computer vision systems. These methods enhance realism and applicability, helping to overcome some of the challenges we've discussed.
Computer Graphics and 3D Modeling for Realistic Image and Video Generation
One of the primary methods for creating synthetic data is using advanced computer graphics and 3D modeling. These technologies allow for precise controlling of environmental variables, object properties, and scenarios. For instance, 3D modeling software can simulate complex urban environments for autonomous vehicle training or detailed anatomical models for medical imaging.
Use of Gaming Engines and Simulation Environments
Gaming engines such as Unreal Engine and Unity are increasingly used for synthetic data generation due to their sophisticated physics simulations and high-fidelity graphics.
These platforms can create dynamic, interactive worlds where developers can simulate a wide range of scenarios that are hard to capture in real life. For example, they can generate diverse driving conditions, including weather scenarios, traffic patterns, and pedestrian behaviors, essential for training autonomous driving systems.
Advancements in Generative Adversarial Networks (GANs) for Data Generation
Generative adversarial networks (GANs) are robust neural networks that generate synthetic data. GANs work by using two neural networks competing: one generates data (generator), while the other evaluates it (discriminator).
This setup helps produce highly realistic images and videos that can be difficult to distinguish from real data. GANs are useful for augmenting datasets with images that fill real-world data gaps, enhancing training datasets' diversity and quality.
Automated Annotation and Labeling Tools
Automating the annotation and labeling of synthetic data is crucial for scaling up the production and use of synthetic datasets. Tools automatically generating annotations help streamline training data preparation for machine learning models.
This automation saves significant time and resources and ensures high accuracy and consistency in the labels, which are critical for the performance of computer vision models.
These synthetic data generation techniques provide the tools to create diverse, realistic datasets that help train more accurate and robust computer vision models. By leveraging these technologies, developers can significantly enhance the effectiveness of their models and accelerate the development cycle.
Let's delve into how synthetic data is applied across various domains, highlighting specific use cases where it significantly enhances the performance and capabilities of computer vision models.
Applications and Use Cases
Synthetic data finds application in numerous fields, providing vital support in training and enhancing the accuracy of computer vision models. Its versatility allows for tailored scenario-specific data generation, crucial for addressing niche requirements.
Training and Enhancing the Accuracy of Computer Vision Models
One primary use of synthetic data is in the training and fine-tuning of computer vision models. Synthetic data provides diverse scenarios and images, helping these models learn to recognize patterns and objects more accurately.
For example, in autonomous vehicle development, synthetic data is used to simulate various driving conditions that help improve the vehicle's ability to recognize road signs, pedestrians, and other vehicles under different lighting and weather conditions.
Scenario-Specific Data Generation for Niche Applications
Synthetic data is precious in fields where real-world data is too complex or sensitive to collect. In medical imaging, for instance, synthetic data can generate detailed anatomical images for training diagnostic tools without extensive patient data, which can be hard to obtain due to privacy concerns.
Augmenting Real Datasets with Synthetic Data for Improved Model Robustness
Combining synthetic data with real datasets can significantly improve the robustness of machine learning models. This approach ensures that models are trained on a wide range of data and can generalize well across different environments.
For example, in facial recognition technology, synthetic data can provide a balanced set of facial images across various ethnicities, ages, and lighting conditions, which needs to be adequately represented in available real datasets.
These applications demonstrate the crucial role of synthetic data in pushing the boundaries of what computer vision technologies can achieve, making systems more reliable, inclusive, and capable of operating in diverse environments.
Next, we will discuss best practices for integrating synthetic data into machine learning workflows to maximize benefits and ensure successful outcomes.
Best Practices for Integrating Synthetic Data
Integrating synthetic data effectively into machine learning workflows is crucial for maximizing the potential benefits while minimizing the risks associated with data biases and domain gaps. Here are some best practices for using synthetic data in training computer vision models.
To ensure that synthetic data contributes positively to the development of robust and accurate computer vision models, it's essential to follow some established best practices:
Balancing Synthetic and Real Data in Training Datasets
While synthetic data can significantly enhance a dataset, it's crucial to balance it with real data to ensure that models remain grounded in real-world scenarios. This balance helps prevent models from learning unrealistic patterns that only appear in synthetic data.
Example: In autonomous driving systems, developers might use a mix of real-world driving footage and synthetic scenarios that include rare but possible driving conditions (like road debris or unexpected weather changes) to create a comprehensive training dataset.
Iteratively Testing and Validating Models with Both Data Types
Regularly testing and validating the performance of models using both synthetic and real data helps identify any discrepancies and adjust the training process accordingly. This iterative process ensures the model performs well in real-world conditions, not just theoretically modeled scenarios.
Example: A facial recognition system could be iteratively tested on synthetic faces and a diverse real-world dataset to ensure it accurately recognizes faces across different demographics and in various lighting conditions.
Continuous Evaluation of Model Performance in Real-World Tasks
It is essential to evaluate models' performance when deployed in real-world conditions continuously. This continuous monitoring allows developers to fine-tune models as new data becomes available or conditions change, ensuring they remain effective and reliable.
Example: Security systems that use computer vision to identify potential threats should continuously evaluate detection accuracy and update models with new synthetic data that simulate emerging security challenges.
By adhering to these best practices, developers can effectively leverage synthetic data to enhance the development of computer vision models, ensuring they are both powerful and practical for real-world applications. These strategies improve the accuracy and robustness of models and contribute to more ethical and equitable AI systems by addressing data diversity and representation issues.
Future Directions and Potential
Let's explore synthetic data's future directions and potential in computer vision. This perspective will help us understand the evolving trends and how they may shape the development and application of these technologies.
As technology advances, the potential and applications of synthetic data in computer vision are expanding. Here are some key trends and future directions in the evolution of synthetic data generation technologies.
Evolution of Synthetic Data Generation Technologies
Advancements in computer graphics, simulation technologies, and machine learning algorithms will continue to improve the realism and variety of synthetic data. This includes more sophisticated textural details, better simulation of physical properties, and enhanced behavioral modeling for dynamic scenarios.
Example: Future developments may allow for near-perfect simulations of human behavior in virtual environments, which could revolutionize how AI systems are trained in fields such as behavioral analysis and complex interaction systems.
Expanding the Scope of Computer Vision Applications Through Synthetic Data
As synthetic data becomes more realistic and easier to generate, its use in training computer vision models will expand into new areas. This includes fields that have been challenging due to data limitations, such as under-resourced medical research areas, advanced robotics, and highly personalized user interfaces.
Example: In robotics, synthetic data can be used to train robots on thousands of household tasks within virtual environments before interacting with the real world, greatly accelerating the learning process and reducing the risk of errors.
Potential Impact on Reducing Bias and Improving Fairness in AI Models
One of the most promising aspects of synthetic data is its potential to reduce biases in real-world datasets. By intentionally designing diverse and inclusive datasets, developers can help ensure that AI models perform fairly and accurately across different populations.
Example: Synthetic data can be used to balance datasets in facial recognition technology, ensuring that the models are equally accurate for all ethnicities and genders by generating a diverse range of faces that are underrepresented in available real datasets.
Conclusion
The future of synthetic data in computer vision looks promising. It has the potential to significantly enhance the accuracy, fairness, and applicability of AI models.
As we continue to innovate and push the boundaries of what synthetic data can achieve, it holds the promise of profoundly transforming the landscape of AI and technology.
Take the next step in your AI journey. Visit Raga AI's website today to learn more about how our synthetic data platforms can revolutionize your applications. Embrace the future of AI with Raga AI—where innovation meets integrity.
Synthetic data refers to any data that are artificially generated rather than obtained by direct measurement. In the realm of computer vision, synthetic data typically includes images or videos created digitally using various algorithms and simulation technologies.
This data serves as a stand-in for real-world data during the training phases of machine learning models, mainly when accurate data is scarce, expensive, or sensitive.
The demand for high-quality training data has skyrocketed as computer vision technologies evolve and find applications in diverse fields such as autonomous driving, medical imaging, and retail. Synthetic data has emerged as a crucial resource because it can be generated in large volumes and tailored to specific needs without the logistical and ethical complications of collecting real-world data.
Advantages of Synthetic Data
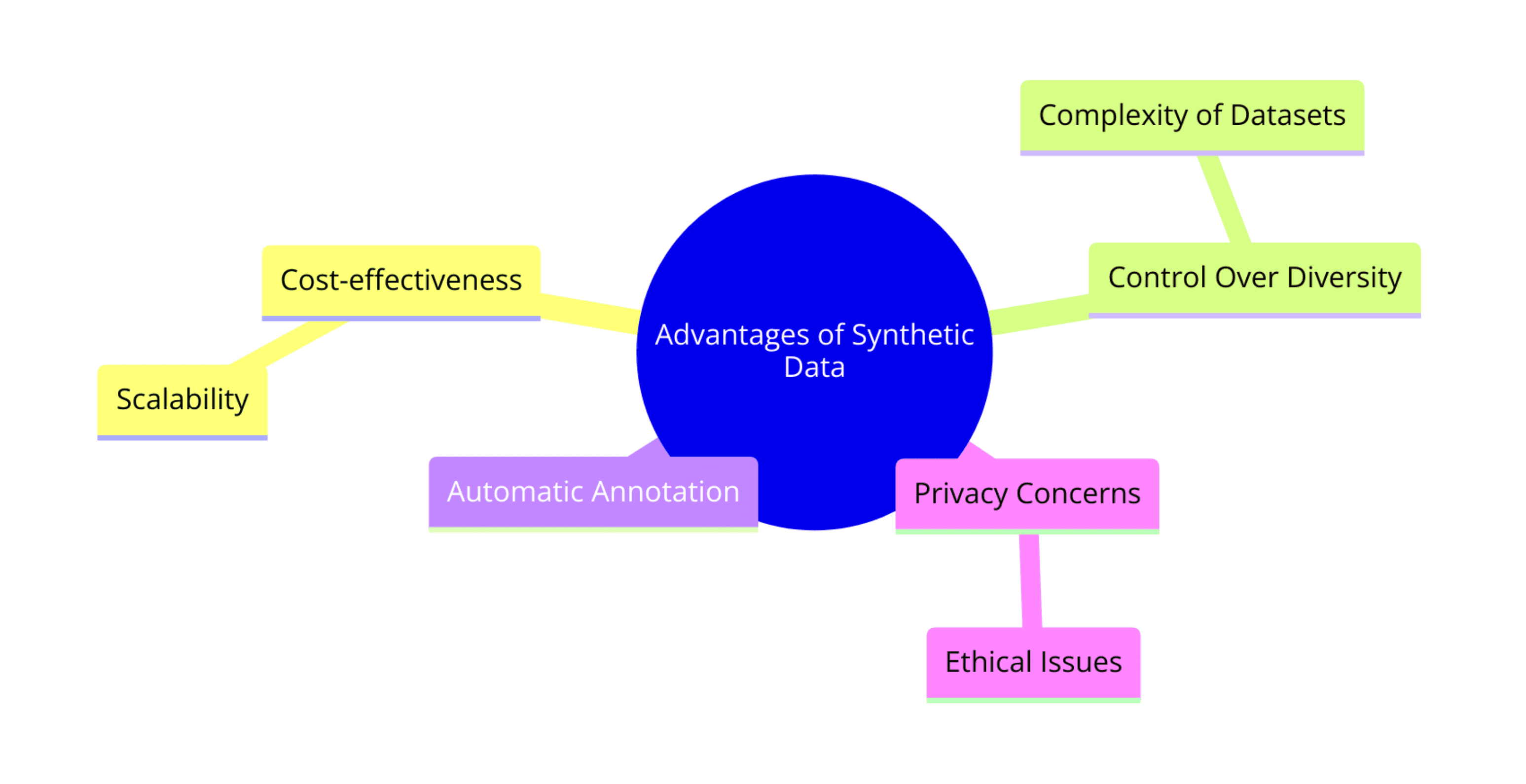
Let's explore synthetic data's significant advantages in computer vision, highlighting its cost-effectiveness, control over dataset properties, and other key benefits.
Cost-effectiveness and Scalability
One of the most compelling advantages of synthetic data is its cost-effectiveness. Generating synthetic images or videos typically requires only the initial investment in software or the development of digital environments.
Once set up, these systems can produce vast amounts of data at a fraction of the cost of traditional data-gathering methods. This scalability allows for the rapid expansion of datasets as needed without additional significant costs.
Discover how RagaAI Enhances Cost-effectiveness with RagaAI for synthetic data in computer vision.
Control Over Diversity and Complexity of Datasets
Synthetic data generation tools provide unprecedented control over the properties of the datasets. Developers can adjust and manipulate lighting, weather conditions, object configurations, and backgrounds. This ability is precious in fields like autonomous driving, where varied scenarios (like different weather and lighting conditions) are crucial for comprehensive systems training.
Ability to Generate Annotated Data Automatically
Another significant benefit of synthetic data is that it can be generated with automatic annotations. This is crucial for training machine learning models since labeled data (data tagged with information like object boundaries and classifications) is typically required.
Generating annotated data manually is often one of the most time-consuming and labor-intensive aspects of preparing training datasets. Synthetic data tools can automate this process, providing perfectly accurate labels and significantly reducing the time and labor involved.
Explore RagaAI's innovative approach to Controlling Dataset Diversity with RagaAI for synthetic data generation.
Reduction of Privacy Concerns and Ethical Issues
Using real-world data, especially in sensitive areas such as surveillance or personal devices, can raise significant privacy and ethical concerns. Synthetic data eliminates many of these issues as it does not involve real individuals or scenarios. This aspect not only simplifies compliance with data protection regulations but also opens up possibilities for research in areas where privacy concerns might restrict the use of accurate data.
These advantages make synthetic data a powerful tool in developing and training computer vision systems, offering challenging efficiencies and capabilities to achieve with real-world data alone.
Challenges in Using Synthetic Data
While synthetic data offers many advantages, it's essential to recognize and address its challenges to ensure effective outcomes in computer vision applications.
Maintaining Realistic Variations and Fidelity
One of the primary challenges with synthetic data is achieving a high level of realism and maintaining variations that accurately mimic the real world. Synthetic datasets must be designed to include realistic textures, lighting variations, and environmental dynamics. This requires sophisticated modeling techniques and a deep understanding of the physical properties being simulated, which can be complex and resource-intensive to develop.
Ensuring the Synthetic Data is Representative of Real-World Scenarios
Synthetic data must encompass various scenarios and conditions to effectively train robust computer vision models. Ensuring that these datasets represent real-world complexities involves significant domain expertise and continuous refinement of the simulation parameters.
There's a risk that models trained predominantly on synthetic data may perform poorly in real-life situations if the data lacks diversity or fails to cover rare but critical scenarios.
Bridging the Domain Gap Between Synthetic and Real Data
The domain gap — the difference in how models perform on synthetic versus accurate data — presents a significant challenge. Even high-quality synthetic data may not perfectly capture real-world data's subtleties and unpredictable nature, leading to models that are overfitted to the synthetic characteristics and underperform when faced with actual data.
This gap requires ongoing efforts in domain adaptation and continuous testing against real-world data sets to ensure that the models remain effective and reliable.
Synthetic Data Generation Techniques
Let's explore the various techniques employed to generate high-quality synthetic data, which are crucial in developing and training computer vision systems. These methods enhance realism and applicability, helping to overcome some of the challenges we've discussed.
Computer Graphics and 3D Modeling for Realistic Image and Video Generation
One of the primary methods for creating synthetic data is using advanced computer graphics and 3D modeling. These technologies allow for precise controlling of environmental variables, object properties, and scenarios. For instance, 3D modeling software can simulate complex urban environments for autonomous vehicle training or detailed anatomical models for medical imaging.
Use of Gaming Engines and Simulation Environments
Gaming engines such as Unreal Engine and Unity are increasingly used for synthetic data generation due to their sophisticated physics simulations and high-fidelity graphics.
These platforms can create dynamic, interactive worlds where developers can simulate a wide range of scenarios that are hard to capture in real life. For example, they can generate diverse driving conditions, including weather scenarios, traffic patterns, and pedestrian behaviors, essential for training autonomous driving systems.
Advancements in Generative Adversarial Networks (GANs) for Data Generation
Generative adversarial networks (GANs) are robust neural networks that generate synthetic data. GANs work by using two neural networks competing: one generates data (generator), while the other evaluates it (discriminator).
This setup helps produce highly realistic images and videos that can be difficult to distinguish from real data. GANs are useful for augmenting datasets with images that fill real-world data gaps, enhancing training datasets' diversity and quality.
Automated Annotation and Labeling Tools
Automating the annotation and labeling of synthetic data is crucial for scaling up the production and use of synthetic datasets. Tools automatically generating annotations help streamline training data preparation for machine learning models.
This automation saves significant time and resources and ensures high accuracy and consistency in the labels, which are critical for the performance of computer vision models.
These synthetic data generation techniques provide the tools to create diverse, realistic datasets that help train more accurate and robust computer vision models. By leveraging these technologies, developers can significantly enhance the effectiveness of their models and accelerate the development cycle.
Let's delve into how synthetic data is applied across various domains, highlighting specific use cases where it significantly enhances the performance and capabilities of computer vision models.
Applications and Use Cases
Synthetic data finds application in numerous fields, providing vital support in training and enhancing the accuracy of computer vision models. Its versatility allows for tailored scenario-specific data generation, crucial for addressing niche requirements.
Training and Enhancing the Accuracy of Computer Vision Models
One primary use of synthetic data is in the training and fine-tuning of computer vision models. Synthetic data provides diverse scenarios and images, helping these models learn to recognize patterns and objects more accurately.
For example, in autonomous vehicle development, synthetic data is used to simulate various driving conditions that help improve the vehicle's ability to recognize road signs, pedestrians, and other vehicles under different lighting and weather conditions.
Scenario-Specific Data Generation for Niche Applications
Synthetic data is precious in fields where real-world data is too complex or sensitive to collect. In medical imaging, for instance, synthetic data can generate detailed anatomical images for training diagnostic tools without extensive patient data, which can be hard to obtain due to privacy concerns.
Augmenting Real Datasets with Synthetic Data for Improved Model Robustness
Combining synthetic data with real datasets can significantly improve the robustness of machine learning models. This approach ensures that models are trained on a wide range of data and can generalize well across different environments.
For example, in facial recognition technology, synthetic data can provide a balanced set of facial images across various ethnicities, ages, and lighting conditions, which needs to be adequately represented in available real datasets.
These applications demonstrate the crucial role of synthetic data in pushing the boundaries of what computer vision technologies can achieve, making systems more reliable, inclusive, and capable of operating in diverse environments.
Next, we will discuss best practices for integrating synthetic data into machine learning workflows to maximize benefits and ensure successful outcomes.
Best Practices for Integrating Synthetic Data
Integrating synthetic data effectively into machine learning workflows is crucial for maximizing the potential benefits while minimizing the risks associated with data biases and domain gaps. Here are some best practices for using synthetic data in training computer vision models.
To ensure that synthetic data contributes positively to the development of robust and accurate computer vision models, it's essential to follow some established best practices:
Balancing Synthetic and Real Data in Training Datasets
While synthetic data can significantly enhance a dataset, it's crucial to balance it with real data to ensure that models remain grounded in real-world scenarios. This balance helps prevent models from learning unrealistic patterns that only appear in synthetic data.
Example: In autonomous driving systems, developers might use a mix of real-world driving footage and synthetic scenarios that include rare but possible driving conditions (like road debris or unexpected weather changes) to create a comprehensive training dataset.
Iteratively Testing and Validating Models with Both Data Types
Regularly testing and validating the performance of models using both synthetic and real data helps identify any discrepancies and adjust the training process accordingly. This iterative process ensures the model performs well in real-world conditions, not just theoretically modeled scenarios.
Example: A facial recognition system could be iteratively tested on synthetic faces and a diverse real-world dataset to ensure it accurately recognizes faces across different demographics and in various lighting conditions.
Continuous Evaluation of Model Performance in Real-World Tasks
It is essential to evaluate models' performance when deployed in real-world conditions continuously. This continuous monitoring allows developers to fine-tune models as new data becomes available or conditions change, ensuring they remain effective and reliable.
Example: Security systems that use computer vision to identify potential threats should continuously evaluate detection accuracy and update models with new synthetic data that simulate emerging security challenges.
By adhering to these best practices, developers can effectively leverage synthetic data to enhance the development of computer vision models, ensuring they are both powerful and practical for real-world applications. These strategies improve the accuracy and robustness of models and contribute to more ethical and equitable AI systems by addressing data diversity and representation issues.
Future Directions and Potential
Let's explore synthetic data's future directions and potential in computer vision. This perspective will help us understand the evolving trends and how they may shape the development and application of these technologies.
As technology advances, the potential and applications of synthetic data in computer vision are expanding. Here are some key trends and future directions in the evolution of synthetic data generation technologies.
Evolution of Synthetic Data Generation Technologies
Advancements in computer graphics, simulation technologies, and machine learning algorithms will continue to improve the realism and variety of synthetic data. This includes more sophisticated textural details, better simulation of physical properties, and enhanced behavioral modeling for dynamic scenarios.
Example: Future developments may allow for near-perfect simulations of human behavior in virtual environments, which could revolutionize how AI systems are trained in fields such as behavioral analysis and complex interaction systems.
Expanding the Scope of Computer Vision Applications Through Synthetic Data
As synthetic data becomes more realistic and easier to generate, its use in training computer vision models will expand into new areas. This includes fields that have been challenging due to data limitations, such as under-resourced medical research areas, advanced robotics, and highly personalized user interfaces.
Example: In robotics, synthetic data can be used to train robots on thousands of household tasks within virtual environments before interacting with the real world, greatly accelerating the learning process and reducing the risk of errors.
Potential Impact on Reducing Bias and Improving Fairness in AI Models
One of the most promising aspects of synthetic data is its potential to reduce biases in real-world datasets. By intentionally designing diverse and inclusive datasets, developers can help ensure that AI models perform fairly and accurately across different populations.
Example: Synthetic data can be used to balance datasets in facial recognition technology, ensuring that the models are equally accurate for all ethnicities and genders by generating a diverse range of faces that are underrepresented in available real datasets.
Conclusion
The future of synthetic data in computer vision looks promising. It has the potential to significantly enhance the accuracy, fairness, and applicability of AI models.
As we continue to innovate and push the boundaries of what synthetic data can achieve, it holds the promise of profoundly transforming the landscape of AI and technology.
Take the next step in your AI journey. Visit Raga AI's website today to learn more about how our synthetic data platforms can revolutionize your applications. Embrace the future of AI with Raga AI—where innovation meets integrity.
Synthetic data refers to any data that are artificially generated rather than obtained by direct measurement. In the realm of computer vision, synthetic data typically includes images or videos created digitally using various algorithms and simulation technologies.
This data serves as a stand-in for real-world data during the training phases of machine learning models, mainly when accurate data is scarce, expensive, or sensitive.
The demand for high-quality training data has skyrocketed as computer vision technologies evolve and find applications in diverse fields such as autonomous driving, medical imaging, and retail. Synthetic data has emerged as a crucial resource because it can be generated in large volumes and tailored to specific needs without the logistical and ethical complications of collecting real-world data.
Advantages of Synthetic Data
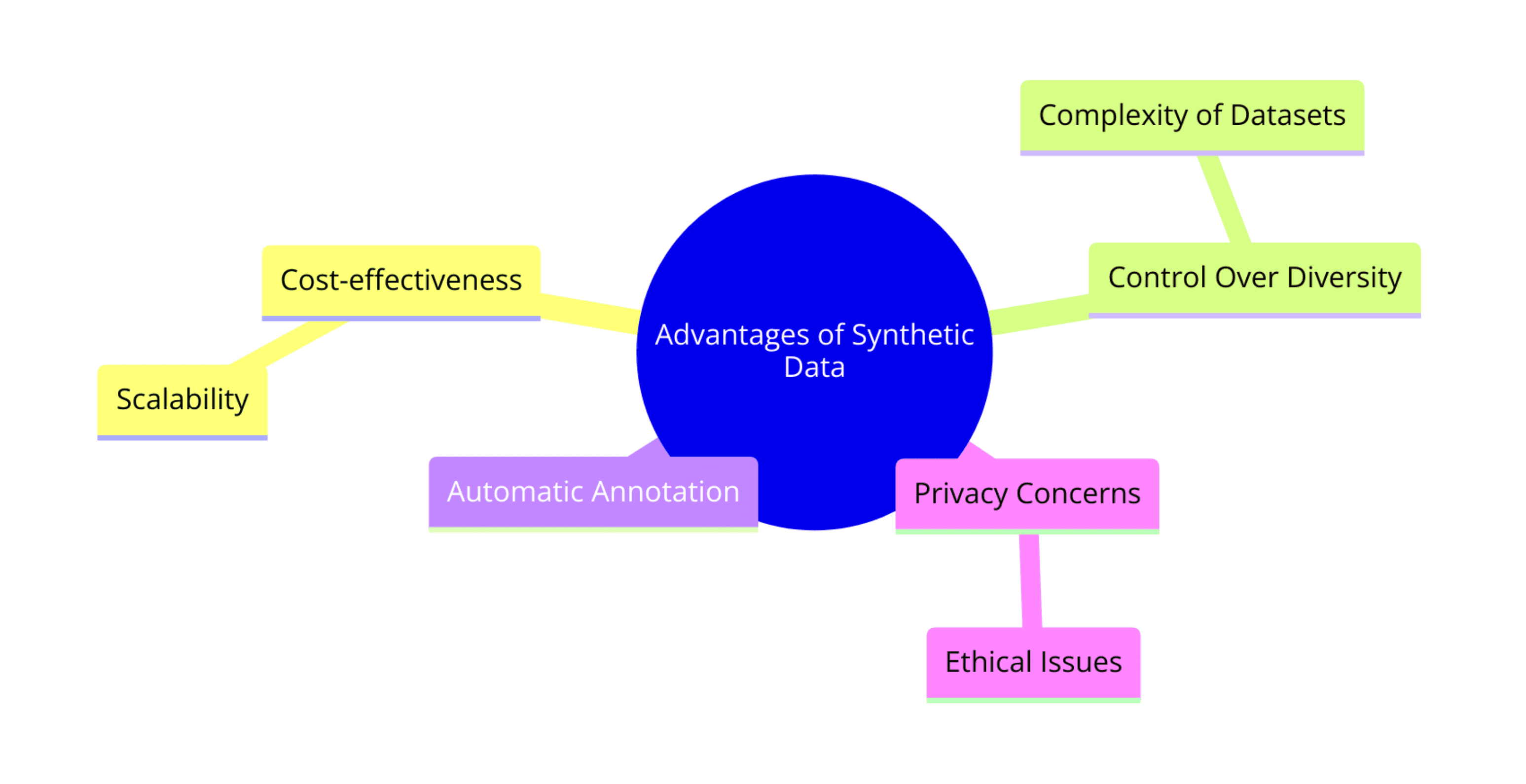
Let's explore synthetic data's significant advantages in computer vision, highlighting its cost-effectiveness, control over dataset properties, and other key benefits.
Cost-effectiveness and Scalability
One of the most compelling advantages of synthetic data is its cost-effectiveness. Generating synthetic images or videos typically requires only the initial investment in software or the development of digital environments.
Once set up, these systems can produce vast amounts of data at a fraction of the cost of traditional data-gathering methods. This scalability allows for the rapid expansion of datasets as needed without additional significant costs.
Discover how RagaAI Enhances Cost-effectiveness with RagaAI for synthetic data in computer vision.
Control Over Diversity and Complexity of Datasets
Synthetic data generation tools provide unprecedented control over the properties of the datasets. Developers can adjust and manipulate lighting, weather conditions, object configurations, and backgrounds. This ability is precious in fields like autonomous driving, where varied scenarios (like different weather and lighting conditions) are crucial for comprehensive systems training.
Ability to Generate Annotated Data Automatically
Another significant benefit of synthetic data is that it can be generated with automatic annotations. This is crucial for training machine learning models since labeled data (data tagged with information like object boundaries and classifications) is typically required.
Generating annotated data manually is often one of the most time-consuming and labor-intensive aspects of preparing training datasets. Synthetic data tools can automate this process, providing perfectly accurate labels and significantly reducing the time and labor involved.
Explore RagaAI's innovative approach to Controlling Dataset Diversity with RagaAI for synthetic data generation.
Reduction of Privacy Concerns and Ethical Issues
Using real-world data, especially in sensitive areas such as surveillance or personal devices, can raise significant privacy and ethical concerns. Synthetic data eliminates many of these issues as it does not involve real individuals or scenarios. This aspect not only simplifies compliance with data protection regulations but also opens up possibilities for research in areas where privacy concerns might restrict the use of accurate data.
These advantages make synthetic data a powerful tool in developing and training computer vision systems, offering challenging efficiencies and capabilities to achieve with real-world data alone.
Challenges in Using Synthetic Data
While synthetic data offers many advantages, it's essential to recognize and address its challenges to ensure effective outcomes in computer vision applications.
Maintaining Realistic Variations and Fidelity
One of the primary challenges with synthetic data is achieving a high level of realism and maintaining variations that accurately mimic the real world. Synthetic datasets must be designed to include realistic textures, lighting variations, and environmental dynamics. This requires sophisticated modeling techniques and a deep understanding of the physical properties being simulated, which can be complex and resource-intensive to develop.
Ensuring the Synthetic Data is Representative of Real-World Scenarios
Synthetic data must encompass various scenarios and conditions to effectively train robust computer vision models. Ensuring that these datasets represent real-world complexities involves significant domain expertise and continuous refinement of the simulation parameters.
There's a risk that models trained predominantly on synthetic data may perform poorly in real-life situations if the data lacks diversity or fails to cover rare but critical scenarios.
Bridging the Domain Gap Between Synthetic and Real Data
The domain gap — the difference in how models perform on synthetic versus accurate data — presents a significant challenge. Even high-quality synthetic data may not perfectly capture real-world data's subtleties and unpredictable nature, leading to models that are overfitted to the synthetic characteristics and underperform when faced with actual data.
This gap requires ongoing efforts in domain adaptation and continuous testing against real-world data sets to ensure that the models remain effective and reliable.
Synthetic Data Generation Techniques
Let's explore the various techniques employed to generate high-quality synthetic data, which are crucial in developing and training computer vision systems. These methods enhance realism and applicability, helping to overcome some of the challenges we've discussed.
Computer Graphics and 3D Modeling for Realistic Image and Video Generation
One of the primary methods for creating synthetic data is using advanced computer graphics and 3D modeling. These technologies allow for precise controlling of environmental variables, object properties, and scenarios. For instance, 3D modeling software can simulate complex urban environments for autonomous vehicle training or detailed anatomical models for medical imaging.
Use of Gaming Engines and Simulation Environments
Gaming engines such as Unreal Engine and Unity are increasingly used for synthetic data generation due to their sophisticated physics simulations and high-fidelity graphics.
These platforms can create dynamic, interactive worlds where developers can simulate a wide range of scenarios that are hard to capture in real life. For example, they can generate diverse driving conditions, including weather scenarios, traffic patterns, and pedestrian behaviors, essential for training autonomous driving systems.
Advancements in Generative Adversarial Networks (GANs) for Data Generation
Generative adversarial networks (GANs) are robust neural networks that generate synthetic data. GANs work by using two neural networks competing: one generates data (generator), while the other evaluates it (discriminator).
This setup helps produce highly realistic images and videos that can be difficult to distinguish from real data. GANs are useful for augmenting datasets with images that fill real-world data gaps, enhancing training datasets' diversity and quality.
Automated Annotation and Labeling Tools
Automating the annotation and labeling of synthetic data is crucial for scaling up the production and use of synthetic datasets. Tools automatically generating annotations help streamline training data preparation for machine learning models.
This automation saves significant time and resources and ensures high accuracy and consistency in the labels, which are critical for the performance of computer vision models.
These synthetic data generation techniques provide the tools to create diverse, realistic datasets that help train more accurate and robust computer vision models. By leveraging these technologies, developers can significantly enhance the effectiveness of their models and accelerate the development cycle.
Let's delve into how synthetic data is applied across various domains, highlighting specific use cases where it significantly enhances the performance and capabilities of computer vision models.
Applications and Use Cases
Synthetic data finds application in numerous fields, providing vital support in training and enhancing the accuracy of computer vision models. Its versatility allows for tailored scenario-specific data generation, crucial for addressing niche requirements.
Training and Enhancing the Accuracy of Computer Vision Models
One primary use of synthetic data is in the training and fine-tuning of computer vision models. Synthetic data provides diverse scenarios and images, helping these models learn to recognize patterns and objects more accurately.
For example, in autonomous vehicle development, synthetic data is used to simulate various driving conditions that help improve the vehicle's ability to recognize road signs, pedestrians, and other vehicles under different lighting and weather conditions.
Scenario-Specific Data Generation for Niche Applications
Synthetic data is precious in fields where real-world data is too complex or sensitive to collect. In medical imaging, for instance, synthetic data can generate detailed anatomical images for training diagnostic tools without extensive patient data, which can be hard to obtain due to privacy concerns.
Augmenting Real Datasets with Synthetic Data for Improved Model Robustness
Combining synthetic data with real datasets can significantly improve the robustness of machine learning models. This approach ensures that models are trained on a wide range of data and can generalize well across different environments.
For example, in facial recognition technology, synthetic data can provide a balanced set of facial images across various ethnicities, ages, and lighting conditions, which needs to be adequately represented in available real datasets.
These applications demonstrate the crucial role of synthetic data in pushing the boundaries of what computer vision technologies can achieve, making systems more reliable, inclusive, and capable of operating in diverse environments.
Next, we will discuss best practices for integrating synthetic data into machine learning workflows to maximize benefits and ensure successful outcomes.
Best Practices for Integrating Synthetic Data
Integrating synthetic data effectively into machine learning workflows is crucial for maximizing the potential benefits while minimizing the risks associated with data biases and domain gaps. Here are some best practices for using synthetic data in training computer vision models.
To ensure that synthetic data contributes positively to the development of robust and accurate computer vision models, it's essential to follow some established best practices:
Balancing Synthetic and Real Data in Training Datasets
While synthetic data can significantly enhance a dataset, it's crucial to balance it with real data to ensure that models remain grounded in real-world scenarios. This balance helps prevent models from learning unrealistic patterns that only appear in synthetic data.
Example: In autonomous driving systems, developers might use a mix of real-world driving footage and synthetic scenarios that include rare but possible driving conditions (like road debris or unexpected weather changes) to create a comprehensive training dataset.
Iteratively Testing and Validating Models with Both Data Types
Regularly testing and validating the performance of models using both synthetic and real data helps identify any discrepancies and adjust the training process accordingly. This iterative process ensures the model performs well in real-world conditions, not just theoretically modeled scenarios.
Example: A facial recognition system could be iteratively tested on synthetic faces and a diverse real-world dataset to ensure it accurately recognizes faces across different demographics and in various lighting conditions.
Continuous Evaluation of Model Performance in Real-World Tasks
It is essential to evaluate models' performance when deployed in real-world conditions continuously. This continuous monitoring allows developers to fine-tune models as new data becomes available or conditions change, ensuring they remain effective and reliable.
Example: Security systems that use computer vision to identify potential threats should continuously evaluate detection accuracy and update models with new synthetic data that simulate emerging security challenges.
By adhering to these best practices, developers can effectively leverage synthetic data to enhance the development of computer vision models, ensuring they are both powerful and practical for real-world applications. These strategies improve the accuracy and robustness of models and contribute to more ethical and equitable AI systems by addressing data diversity and representation issues.
Future Directions and Potential
Let's explore synthetic data's future directions and potential in computer vision. This perspective will help us understand the evolving trends and how they may shape the development and application of these technologies.
As technology advances, the potential and applications of synthetic data in computer vision are expanding. Here are some key trends and future directions in the evolution of synthetic data generation technologies.
Evolution of Synthetic Data Generation Technologies
Advancements in computer graphics, simulation technologies, and machine learning algorithms will continue to improve the realism and variety of synthetic data. This includes more sophisticated textural details, better simulation of physical properties, and enhanced behavioral modeling for dynamic scenarios.
Example: Future developments may allow for near-perfect simulations of human behavior in virtual environments, which could revolutionize how AI systems are trained in fields such as behavioral analysis and complex interaction systems.
Expanding the Scope of Computer Vision Applications Through Synthetic Data
As synthetic data becomes more realistic and easier to generate, its use in training computer vision models will expand into new areas. This includes fields that have been challenging due to data limitations, such as under-resourced medical research areas, advanced robotics, and highly personalized user interfaces.
Example: In robotics, synthetic data can be used to train robots on thousands of household tasks within virtual environments before interacting with the real world, greatly accelerating the learning process and reducing the risk of errors.
Potential Impact on Reducing Bias and Improving Fairness in AI Models
One of the most promising aspects of synthetic data is its potential to reduce biases in real-world datasets. By intentionally designing diverse and inclusive datasets, developers can help ensure that AI models perform fairly and accurately across different populations.
Example: Synthetic data can be used to balance datasets in facial recognition technology, ensuring that the models are equally accurate for all ethnicities and genders by generating a diverse range of faces that are underrepresented in available real datasets.
Conclusion
The future of synthetic data in computer vision looks promising. It has the potential to significantly enhance the accuracy, fairness, and applicability of AI models.
As we continue to innovate and push the boundaries of what synthetic data can achieve, it holds the promise of profoundly transforming the landscape of AI and technology.
Take the next step in your AI journey. Visit Raga AI's website today to learn more about how our synthetic data platforms can revolutionize your applications. Embrace the future of AI with Raga AI—where innovation meets integrity.
Synthetic data refers to any data that are artificially generated rather than obtained by direct measurement. In the realm of computer vision, synthetic data typically includes images or videos created digitally using various algorithms and simulation technologies.
This data serves as a stand-in for real-world data during the training phases of machine learning models, mainly when accurate data is scarce, expensive, or sensitive.
The demand for high-quality training data has skyrocketed as computer vision technologies evolve and find applications in diverse fields such as autonomous driving, medical imaging, and retail. Synthetic data has emerged as a crucial resource because it can be generated in large volumes and tailored to specific needs without the logistical and ethical complications of collecting real-world data.
Advantages of Synthetic Data
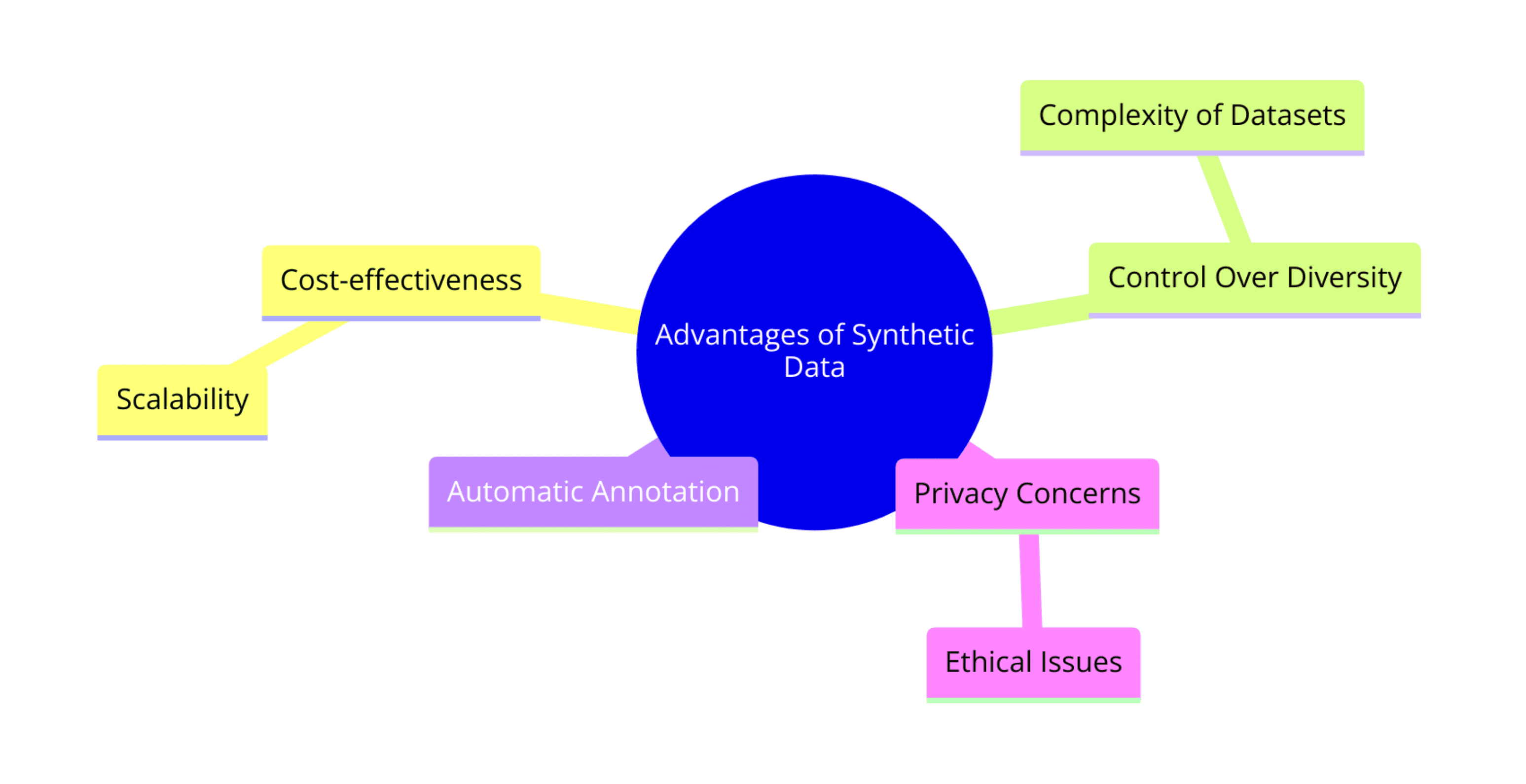
Let's explore synthetic data's significant advantages in computer vision, highlighting its cost-effectiveness, control over dataset properties, and other key benefits.
Cost-effectiveness and Scalability
One of the most compelling advantages of synthetic data is its cost-effectiveness. Generating synthetic images or videos typically requires only the initial investment in software or the development of digital environments.
Once set up, these systems can produce vast amounts of data at a fraction of the cost of traditional data-gathering methods. This scalability allows for the rapid expansion of datasets as needed without additional significant costs.
Discover how RagaAI Enhances Cost-effectiveness with RagaAI for synthetic data in computer vision.
Control Over Diversity and Complexity of Datasets
Synthetic data generation tools provide unprecedented control over the properties of the datasets. Developers can adjust and manipulate lighting, weather conditions, object configurations, and backgrounds. This ability is precious in fields like autonomous driving, where varied scenarios (like different weather and lighting conditions) are crucial for comprehensive systems training.
Ability to Generate Annotated Data Automatically
Another significant benefit of synthetic data is that it can be generated with automatic annotations. This is crucial for training machine learning models since labeled data (data tagged with information like object boundaries and classifications) is typically required.
Generating annotated data manually is often one of the most time-consuming and labor-intensive aspects of preparing training datasets. Synthetic data tools can automate this process, providing perfectly accurate labels and significantly reducing the time and labor involved.
Explore RagaAI's innovative approach to Controlling Dataset Diversity with RagaAI for synthetic data generation.
Reduction of Privacy Concerns and Ethical Issues
Using real-world data, especially in sensitive areas such as surveillance or personal devices, can raise significant privacy and ethical concerns. Synthetic data eliminates many of these issues as it does not involve real individuals or scenarios. This aspect not only simplifies compliance with data protection regulations but also opens up possibilities for research in areas where privacy concerns might restrict the use of accurate data.
These advantages make synthetic data a powerful tool in developing and training computer vision systems, offering challenging efficiencies and capabilities to achieve with real-world data alone.
Challenges in Using Synthetic Data
While synthetic data offers many advantages, it's essential to recognize and address its challenges to ensure effective outcomes in computer vision applications.
Maintaining Realistic Variations and Fidelity
One of the primary challenges with synthetic data is achieving a high level of realism and maintaining variations that accurately mimic the real world. Synthetic datasets must be designed to include realistic textures, lighting variations, and environmental dynamics. This requires sophisticated modeling techniques and a deep understanding of the physical properties being simulated, which can be complex and resource-intensive to develop.
Ensuring the Synthetic Data is Representative of Real-World Scenarios
Synthetic data must encompass various scenarios and conditions to effectively train robust computer vision models. Ensuring that these datasets represent real-world complexities involves significant domain expertise and continuous refinement of the simulation parameters.
There's a risk that models trained predominantly on synthetic data may perform poorly in real-life situations if the data lacks diversity or fails to cover rare but critical scenarios.
Bridging the Domain Gap Between Synthetic and Real Data
The domain gap — the difference in how models perform on synthetic versus accurate data — presents a significant challenge. Even high-quality synthetic data may not perfectly capture real-world data's subtleties and unpredictable nature, leading to models that are overfitted to the synthetic characteristics and underperform when faced with actual data.
This gap requires ongoing efforts in domain adaptation and continuous testing against real-world data sets to ensure that the models remain effective and reliable.
Synthetic Data Generation Techniques
Let's explore the various techniques employed to generate high-quality synthetic data, which are crucial in developing and training computer vision systems. These methods enhance realism and applicability, helping to overcome some of the challenges we've discussed.
Computer Graphics and 3D Modeling for Realistic Image and Video Generation
One of the primary methods for creating synthetic data is using advanced computer graphics and 3D modeling. These technologies allow for precise controlling of environmental variables, object properties, and scenarios. For instance, 3D modeling software can simulate complex urban environments for autonomous vehicle training or detailed anatomical models for medical imaging.
Use of Gaming Engines and Simulation Environments
Gaming engines such as Unreal Engine and Unity are increasingly used for synthetic data generation due to their sophisticated physics simulations and high-fidelity graphics.
These platforms can create dynamic, interactive worlds where developers can simulate a wide range of scenarios that are hard to capture in real life. For example, they can generate diverse driving conditions, including weather scenarios, traffic patterns, and pedestrian behaviors, essential for training autonomous driving systems.
Advancements in Generative Adversarial Networks (GANs) for Data Generation
Generative adversarial networks (GANs) are robust neural networks that generate synthetic data. GANs work by using two neural networks competing: one generates data (generator), while the other evaluates it (discriminator).
This setup helps produce highly realistic images and videos that can be difficult to distinguish from real data. GANs are useful for augmenting datasets with images that fill real-world data gaps, enhancing training datasets' diversity and quality.
Automated Annotation and Labeling Tools
Automating the annotation and labeling of synthetic data is crucial for scaling up the production and use of synthetic datasets. Tools automatically generating annotations help streamline training data preparation for machine learning models.
This automation saves significant time and resources and ensures high accuracy and consistency in the labels, which are critical for the performance of computer vision models.
These synthetic data generation techniques provide the tools to create diverse, realistic datasets that help train more accurate and robust computer vision models. By leveraging these technologies, developers can significantly enhance the effectiveness of their models and accelerate the development cycle.
Let's delve into how synthetic data is applied across various domains, highlighting specific use cases where it significantly enhances the performance and capabilities of computer vision models.
Applications and Use Cases
Synthetic data finds application in numerous fields, providing vital support in training and enhancing the accuracy of computer vision models. Its versatility allows for tailored scenario-specific data generation, crucial for addressing niche requirements.
Training and Enhancing the Accuracy of Computer Vision Models
One primary use of synthetic data is in the training and fine-tuning of computer vision models. Synthetic data provides diverse scenarios and images, helping these models learn to recognize patterns and objects more accurately.
For example, in autonomous vehicle development, synthetic data is used to simulate various driving conditions that help improve the vehicle's ability to recognize road signs, pedestrians, and other vehicles under different lighting and weather conditions.
Scenario-Specific Data Generation for Niche Applications
Synthetic data is precious in fields where real-world data is too complex or sensitive to collect. In medical imaging, for instance, synthetic data can generate detailed anatomical images for training diagnostic tools without extensive patient data, which can be hard to obtain due to privacy concerns.
Augmenting Real Datasets with Synthetic Data for Improved Model Robustness
Combining synthetic data with real datasets can significantly improve the robustness of machine learning models. This approach ensures that models are trained on a wide range of data and can generalize well across different environments.
For example, in facial recognition technology, synthetic data can provide a balanced set of facial images across various ethnicities, ages, and lighting conditions, which needs to be adequately represented in available real datasets.
These applications demonstrate the crucial role of synthetic data in pushing the boundaries of what computer vision technologies can achieve, making systems more reliable, inclusive, and capable of operating in diverse environments.
Next, we will discuss best practices for integrating synthetic data into machine learning workflows to maximize benefits and ensure successful outcomes.
Best Practices for Integrating Synthetic Data
Integrating synthetic data effectively into machine learning workflows is crucial for maximizing the potential benefits while minimizing the risks associated with data biases and domain gaps. Here are some best practices for using synthetic data in training computer vision models.
To ensure that synthetic data contributes positively to the development of robust and accurate computer vision models, it's essential to follow some established best practices:
Balancing Synthetic and Real Data in Training Datasets
While synthetic data can significantly enhance a dataset, it's crucial to balance it with real data to ensure that models remain grounded in real-world scenarios. This balance helps prevent models from learning unrealistic patterns that only appear in synthetic data.
Example: In autonomous driving systems, developers might use a mix of real-world driving footage and synthetic scenarios that include rare but possible driving conditions (like road debris or unexpected weather changes) to create a comprehensive training dataset.
Iteratively Testing and Validating Models with Both Data Types
Regularly testing and validating the performance of models using both synthetic and real data helps identify any discrepancies and adjust the training process accordingly. This iterative process ensures the model performs well in real-world conditions, not just theoretically modeled scenarios.
Example: A facial recognition system could be iteratively tested on synthetic faces and a diverse real-world dataset to ensure it accurately recognizes faces across different demographics and in various lighting conditions.
Continuous Evaluation of Model Performance in Real-World Tasks
It is essential to evaluate models' performance when deployed in real-world conditions continuously. This continuous monitoring allows developers to fine-tune models as new data becomes available or conditions change, ensuring they remain effective and reliable.
Example: Security systems that use computer vision to identify potential threats should continuously evaluate detection accuracy and update models with new synthetic data that simulate emerging security challenges.
By adhering to these best practices, developers can effectively leverage synthetic data to enhance the development of computer vision models, ensuring they are both powerful and practical for real-world applications. These strategies improve the accuracy and robustness of models and contribute to more ethical and equitable AI systems by addressing data diversity and representation issues.
Future Directions and Potential
Let's explore synthetic data's future directions and potential in computer vision. This perspective will help us understand the evolving trends and how they may shape the development and application of these technologies.
As technology advances, the potential and applications of synthetic data in computer vision are expanding. Here are some key trends and future directions in the evolution of synthetic data generation technologies.
Evolution of Synthetic Data Generation Technologies
Advancements in computer graphics, simulation technologies, and machine learning algorithms will continue to improve the realism and variety of synthetic data. This includes more sophisticated textural details, better simulation of physical properties, and enhanced behavioral modeling for dynamic scenarios.
Example: Future developments may allow for near-perfect simulations of human behavior in virtual environments, which could revolutionize how AI systems are trained in fields such as behavioral analysis and complex interaction systems.
Expanding the Scope of Computer Vision Applications Through Synthetic Data
As synthetic data becomes more realistic and easier to generate, its use in training computer vision models will expand into new areas. This includes fields that have been challenging due to data limitations, such as under-resourced medical research areas, advanced robotics, and highly personalized user interfaces.
Example: In robotics, synthetic data can be used to train robots on thousands of household tasks within virtual environments before interacting with the real world, greatly accelerating the learning process and reducing the risk of errors.
Potential Impact on Reducing Bias and Improving Fairness in AI Models
One of the most promising aspects of synthetic data is its potential to reduce biases in real-world datasets. By intentionally designing diverse and inclusive datasets, developers can help ensure that AI models perform fairly and accurately across different populations.
Example: Synthetic data can be used to balance datasets in facial recognition technology, ensuring that the models are equally accurate for all ethnicities and genders by generating a diverse range of faces that are underrepresented in available real datasets.
Conclusion
The future of synthetic data in computer vision looks promising. It has the potential to significantly enhance the accuracy, fairness, and applicability of AI models.
As we continue to innovate and push the boundaries of what synthetic data can achieve, it holds the promise of profoundly transforming the landscape of AI and technology.
Take the next step in your AI journey. Visit Raga AI's website today to learn more about how our synthetic data platforms can revolutionize your applications. Embrace the future of AI with Raga AI—where innovation meets integrity.
Synthetic data refers to any data that are artificially generated rather than obtained by direct measurement. In the realm of computer vision, synthetic data typically includes images or videos created digitally using various algorithms and simulation technologies.
This data serves as a stand-in for real-world data during the training phases of machine learning models, mainly when accurate data is scarce, expensive, or sensitive.
The demand for high-quality training data has skyrocketed as computer vision technologies evolve and find applications in diverse fields such as autonomous driving, medical imaging, and retail. Synthetic data has emerged as a crucial resource because it can be generated in large volumes and tailored to specific needs without the logistical and ethical complications of collecting real-world data.
Advantages of Synthetic Data
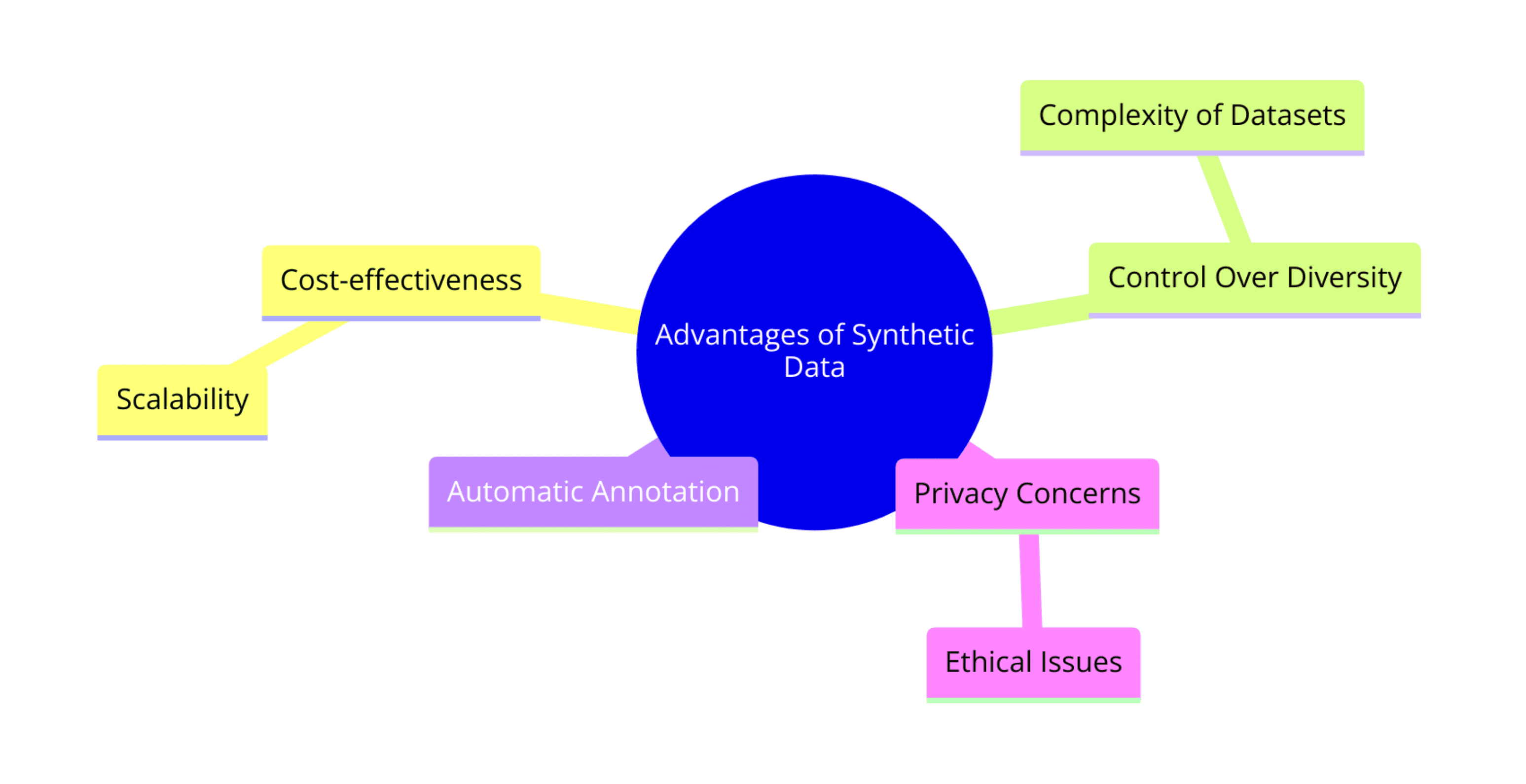
Let's explore synthetic data's significant advantages in computer vision, highlighting its cost-effectiveness, control over dataset properties, and other key benefits.
Cost-effectiveness and Scalability
One of the most compelling advantages of synthetic data is its cost-effectiveness. Generating synthetic images or videos typically requires only the initial investment in software or the development of digital environments.
Once set up, these systems can produce vast amounts of data at a fraction of the cost of traditional data-gathering methods. This scalability allows for the rapid expansion of datasets as needed without additional significant costs.
Discover how RagaAI Enhances Cost-effectiveness with RagaAI for synthetic data in computer vision.
Control Over Diversity and Complexity of Datasets
Synthetic data generation tools provide unprecedented control over the properties of the datasets. Developers can adjust and manipulate lighting, weather conditions, object configurations, and backgrounds. This ability is precious in fields like autonomous driving, where varied scenarios (like different weather and lighting conditions) are crucial for comprehensive systems training.
Ability to Generate Annotated Data Automatically
Another significant benefit of synthetic data is that it can be generated with automatic annotations. This is crucial for training machine learning models since labeled data (data tagged with information like object boundaries and classifications) is typically required.
Generating annotated data manually is often one of the most time-consuming and labor-intensive aspects of preparing training datasets. Synthetic data tools can automate this process, providing perfectly accurate labels and significantly reducing the time and labor involved.
Explore RagaAI's innovative approach to Controlling Dataset Diversity with RagaAI for synthetic data generation.
Reduction of Privacy Concerns and Ethical Issues
Using real-world data, especially in sensitive areas such as surveillance or personal devices, can raise significant privacy and ethical concerns. Synthetic data eliminates many of these issues as it does not involve real individuals or scenarios. This aspect not only simplifies compliance with data protection regulations but also opens up possibilities for research in areas where privacy concerns might restrict the use of accurate data.
These advantages make synthetic data a powerful tool in developing and training computer vision systems, offering challenging efficiencies and capabilities to achieve with real-world data alone.
Challenges in Using Synthetic Data
While synthetic data offers many advantages, it's essential to recognize and address its challenges to ensure effective outcomes in computer vision applications.
Maintaining Realistic Variations and Fidelity
One of the primary challenges with synthetic data is achieving a high level of realism and maintaining variations that accurately mimic the real world. Synthetic datasets must be designed to include realistic textures, lighting variations, and environmental dynamics. This requires sophisticated modeling techniques and a deep understanding of the physical properties being simulated, which can be complex and resource-intensive to develop.
Ensuring the Synthetic Data is Representative of Real-World Scenarios
Synthetic data must encompass various scenarios and conditions to effectively train robust computer vision models. Ensuring that these datasets represent real-world complexities involves significant domain expertise and continuous refinement of the simulation parameters.
There's a risk that models trained predominantly on synthetic data may perform poorly in real-life situations if the data lacks diversity or fails to cover rare but critical scenarios.
Bridging the Domain Gap Between Synthetic and Real Data
The domain gap — the difference in how models perform on synthetic versus accurate data — presents a significant challenge. Even high-quality synthetic data may not perfectly capture real-world data's subtleties and unpredictable nature, leading to models that are overfitted to the synthetic characteristics and underperform when faced with actual data.
This gap requires ongoing efforts in domain adaptation and continuous testing against real-world data sets to ensure that the models remain effective and reliable.
Synthetic Data Generation Techniques
Let's explore the various techniques employed to generate high-quality synthetic data, which are crucial in developing and training computer vision systems. These methods enhance realism and applicability, helping to overcome some of the challenges we've discussed.
Computer Graphics and 3D Modeling for Realistic Image and Video Generation
One of the primary methods for creating synthetic data is using advanced computer graphics and 3D modeling. These technologies allow for precise controlling of environmental variables, object properties, and scenarios. For instance, 3D modeling software can simulate complex urban environments for autonomous vehicle training or detailed anatomical models for medical imaging.
Use of Gaming Engines and Simulation Environments
Gaming engines such as Unreal Engine and Unity are increasingly used for synthetic data generation due to their sophisticated physics simulations and high-fidelity graphics.
These platforms can create dynamic, interactive worlds where developers can simulate a wide range of scenarios that are hard to capture in real life. For example, they can generate diverse driving conditions, including weather scenarios, traffic patterns, and pedestrian behaviors, essential for training autonomous driving systems.
Advancements in Generative Adversarial Networks (GANs) for Data Generation
Generative adversarial networks (GANs) are robust neural networks that generate synthetic data. GANs work by using two neural networks competing: one generates data (generator), while the other evaluates it (discriminator).
This setup helps produce highly realistic images and videos that can be difficult to distinguish from real data. GANs are useful for augmenting datasets with images that fill real-world data gaps, enhancing training datasets' diversity and quality.
Automated Annotation and Labeling Tools
Automating the annotation and labeling of synthetic data is crucial for scaling up the production and use of synthetic datasets. Tools automatically generating annotations help streamline training data preparation for machine learning models.
This automation saves significant time and resources and ensures high accuracy and consistency in the labels, which are critical for the performance of computer vision models.
These synthetic data generation techniques provide the tools to create diverse, realistic datasets that help train more accurate and robust computer vision models. By leveraging these technologies, developers can significantly enhance the effectiveness of their models and accelerate the development cycle.
Let's delve into how synthetic data is applied across various domains, highlighting specific use cases where it significantly enhances the performance and capabilities of computer vision models.
Applications and Use Cases
Synthetic data finds application in numerous fields, providing vital support in training and enhancing the accuracy of computer vision models. Its versatility allows for tailored scenario-specific data generation, crucial for addressing niche requirements.
Training and Enhancing the Accuracy of Computer Vision Models
One primary use of synthetic data is in the training and fine-tuning of computer vision models. Synthetic data provides diverse scenarios and images, helping these models learn to recognize patterns and objects more accurately.
For example, in autonomous vehicle development, synthetic data is used to simulate various driving conditions that help improve the vehicle's ability to recognize road signs, pedestrians, and other vehicles under different lighting and weather conditions.
Scenario-Specific Data Generation for Niche Applications
Synthetic data is precious in fields where real-world data is too complex or sensitive to collect. In medical imaging, for instance, synthetic data can generate detailed anatomical images for training diagnostic tools without extensive patient data, which can be hard to obtain due to privacy concerns.
Augmenting Real Datasets with Synthetic Data for Improved Model Robustness
Combining synthetic data with real datasets can significantly improve the robustness of machine learning models. This approach ensures that models are trained on a wide range of data and can generalize well across different environments.
For example, in facial recognition technology, synthetic data can provide a balanced set of facial images across various ethnicities, ages, and lighting conditions, which needs to be adequately represented in available real datasets.
These applications demonstrate the crucial role of synthetic data in pushing the boundaries of what computer vision technologies can achieve, making systems more reliable, inclusive, and capable of operating in diverse environments.
Next, we will discuss best practices for integrating synthetic data into machine learning workflows to maximize benefits and ensure successful outcomes.
Best Practices for Integrating Synthetic Data
Integrating synthetic data effectively into machine learning workflows is crucial for maximizing the potential benefits while minimizing the risks associated with data biases and domain gaps. Here are some best practices for using synthetic data in training computer vision models.
To ensure that synthetic data contributes positively to the development of robust and accurate computer vision models, it's essential to follow some established best practices:
Balancing Synthetic and Real Data in Training Datasets
While synthetic data can significantly enhance a dataset, it's crucial to balance it with real data to ensure that models remain grounded in real-world scenarios. This balance helps prevent models from learning unrealistic patterns that only appear in synthetic data.
Example: In autonomous driving systems, developers might use a mix of real-world driving footage and synthetic scenarios that include rare but possible driving conditions (like road debris or unexpected weather changes) to create a comprehensive training dataset.
Iteratively Testing and Validating Models with Both Data Types
Regularly testing and validating the performance of models using both synthetic and real data helps identify any discrepancies and adjust the training process accordingly. This iterative process ensures the model performs well in real-world conditions, not just theoretically modeled scenarios.
Example: A facial recognition system could be iteratively tested on synthetic faces and a diverse real-world dataset to ensure it accurately recognizes faces across different demographics and in various lighting conditions.
Continuous Evaluation of Model Performance in Real-World Tasks
It is essential to evaluate models' performance when deployed in real-world conditions continuously. This continuous monitoring allows developers to fine-tune models as new data becomes available or conditions change, ensuring they remain effective and reliable.
Example: Security systems that use computer vision to identify potential threats should continuously evaluate detection accuracy and update models with new synthetic data that simulate emerging security challenges.
By adhering to these best practices, developers can effectively leverage synthetic data to enhance the development of computer vision models, ensuring they are both powerful and practical for real-world applications. These strategies improve the accuracy and robustness of models and contribute to more ethical and equitable AI systems by addressing data diversity and representation issues.
Future Directions and Potential
Let's explore synthetic data's future directions and potential in computer vision. This perspective will help us understand the evolving trends and how they may shape the development and application of these technologies.
As technology advances, the potential and applications of synthetic data in computer vision are expanding. Here are some key trends and future directions in the evolution of synthetic data generation technologies.
Evolution of Synthetic Data Generation Technologies
Advancements in computer graphics, simulation technologies, and machine learning algorithms will continue to improve the realism and variety of synthetic data. This includes more sophisticated textural details, better simulation of physical properties, and enhanced behavioral modeling for dynamic scenarios.
Example: Future developments may allow for near-perfect simulations of human behavior in virtual environments, which could revolutionize how AI systems are trained in fields such as behavioral analysis and complex interaction systems.
Expanding the Scope of Computer Vision Applications Through Synthetic Data
As synthetic data becomes more realistic and easier to generate, its use in training computer vision models will expand into new areas. This includes fields that have been challenging due to data limitations, such as under-resourced medical research areas, advanced robotics, and highly personalized user interfaces.
Example: In robotics, synthetic data can be used to train robots on thousands of household tasks within virtual environments before interacting with the real world, greatly accelerating the learning process and reducing the risk of errors.
Potential Impact on Reducing Bias and Improving Fairness in AI Models
One of the most promising aspects of synthetic data is its potential to reduce biases in real-world datasets. By intentionally designing diverse and inclusive datasets, developers can help ensure that AI models perform fairly and accurately across different populations.
Example: Synthetic data can be used to balance datasets in facial recognition technology, ensuring that the models are equally accurate for all ethnicities and genders by generating a diverse range of faces that are underrepresented in available real datasets.
Conclusion
The future of synthetic data in computer vision looks promising. It has the potential to significantly enhance the accuracy, fairness, and applicability of AI models.
As we continue to innovate and push the boundaries of what synthetic data can achieve, it holds the promise of profoundly transforming the landscape of AI and technology.
Take the next step in your AI journey. Visit Raga AI's website today to learn more about how our synthetic data platforms can revolutionize your applications. Embrace the future of AI with Raga AI—where innovation meets integrity.