Importance of Accuracy and Reliability in Tabular Data Models
Importance of Accuracy and Reliability in Tabular Data Models
Importance of Accuracy and Reliability in Tabular Data Models
Jigar Gupta
Jul 1, 2024
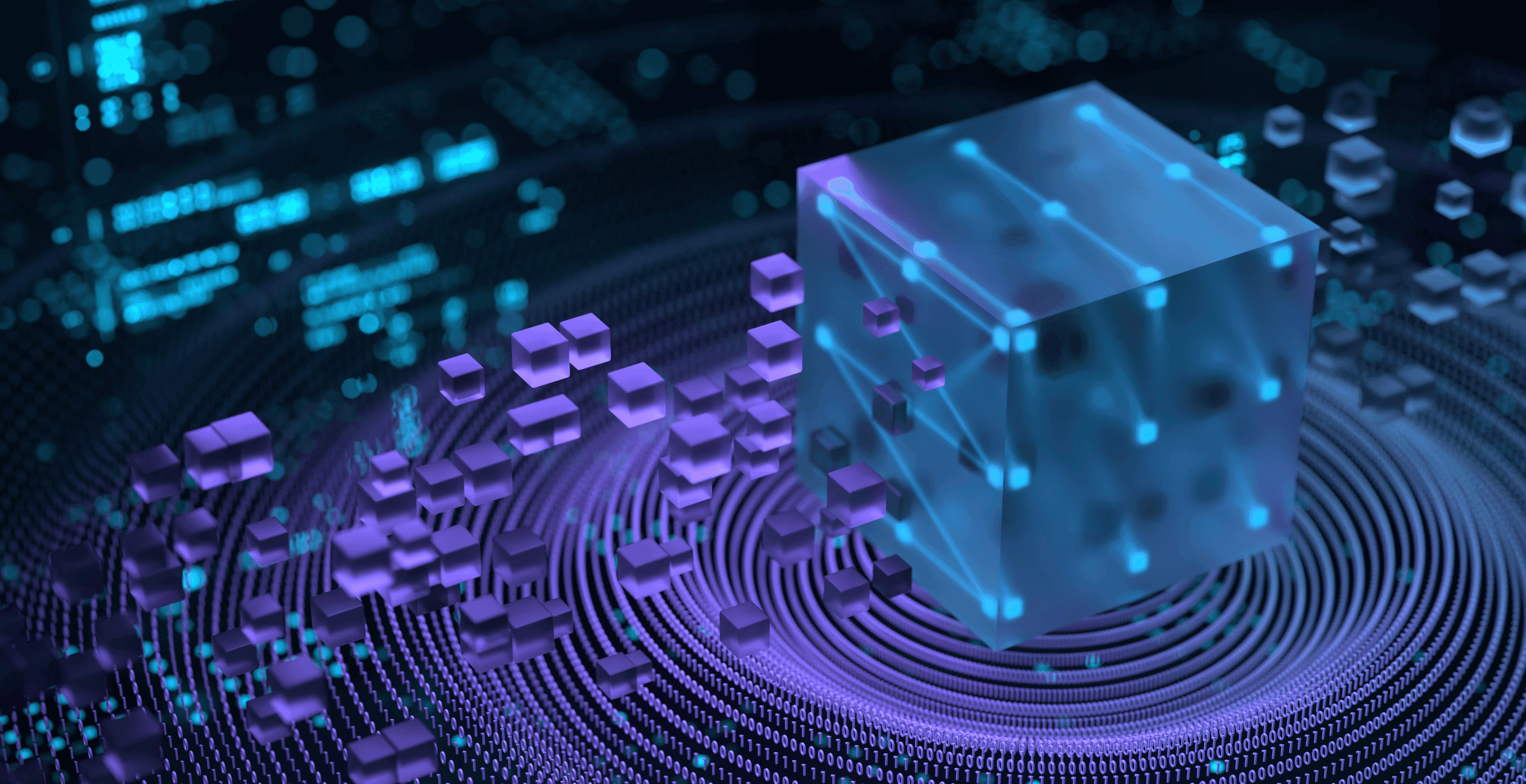
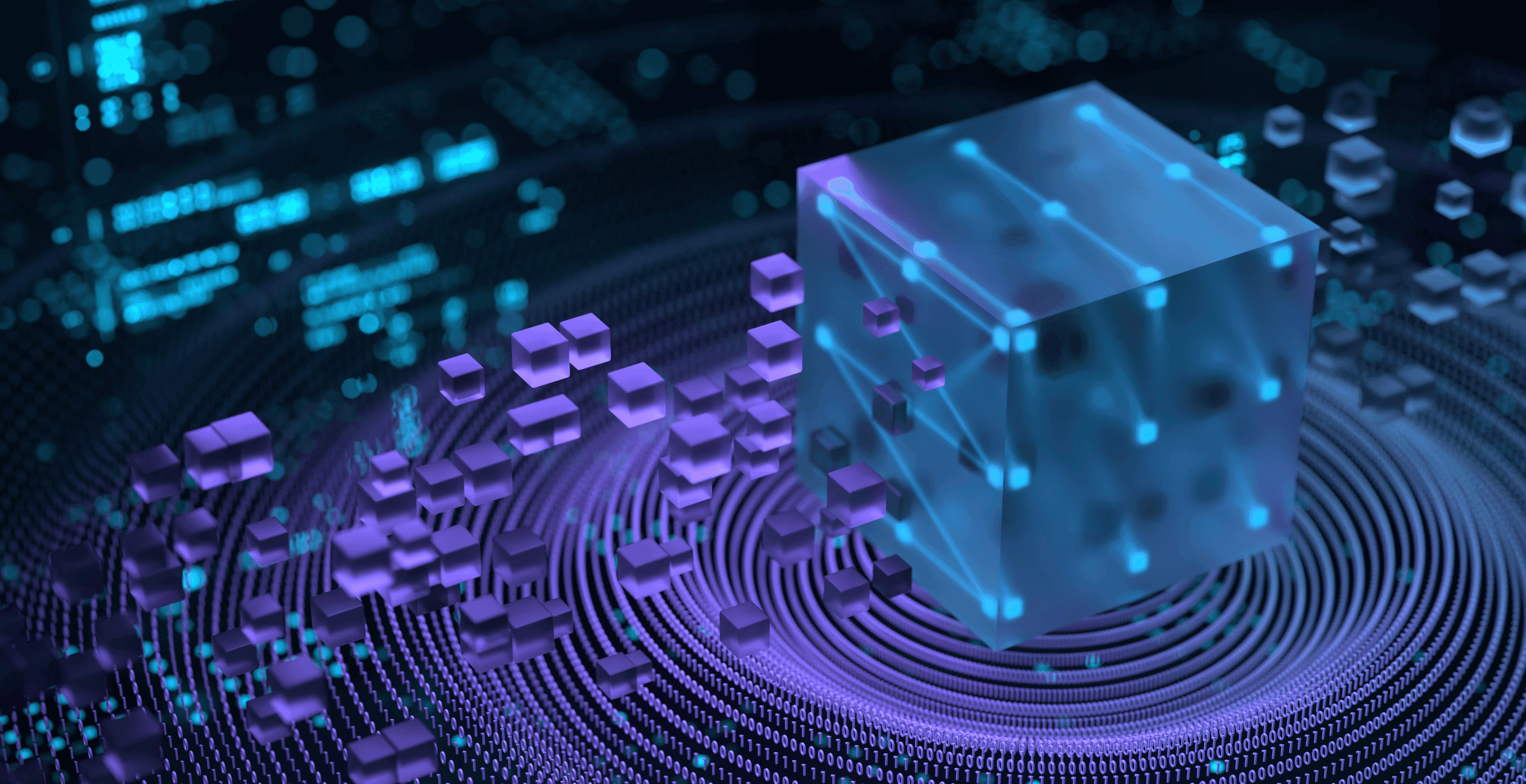
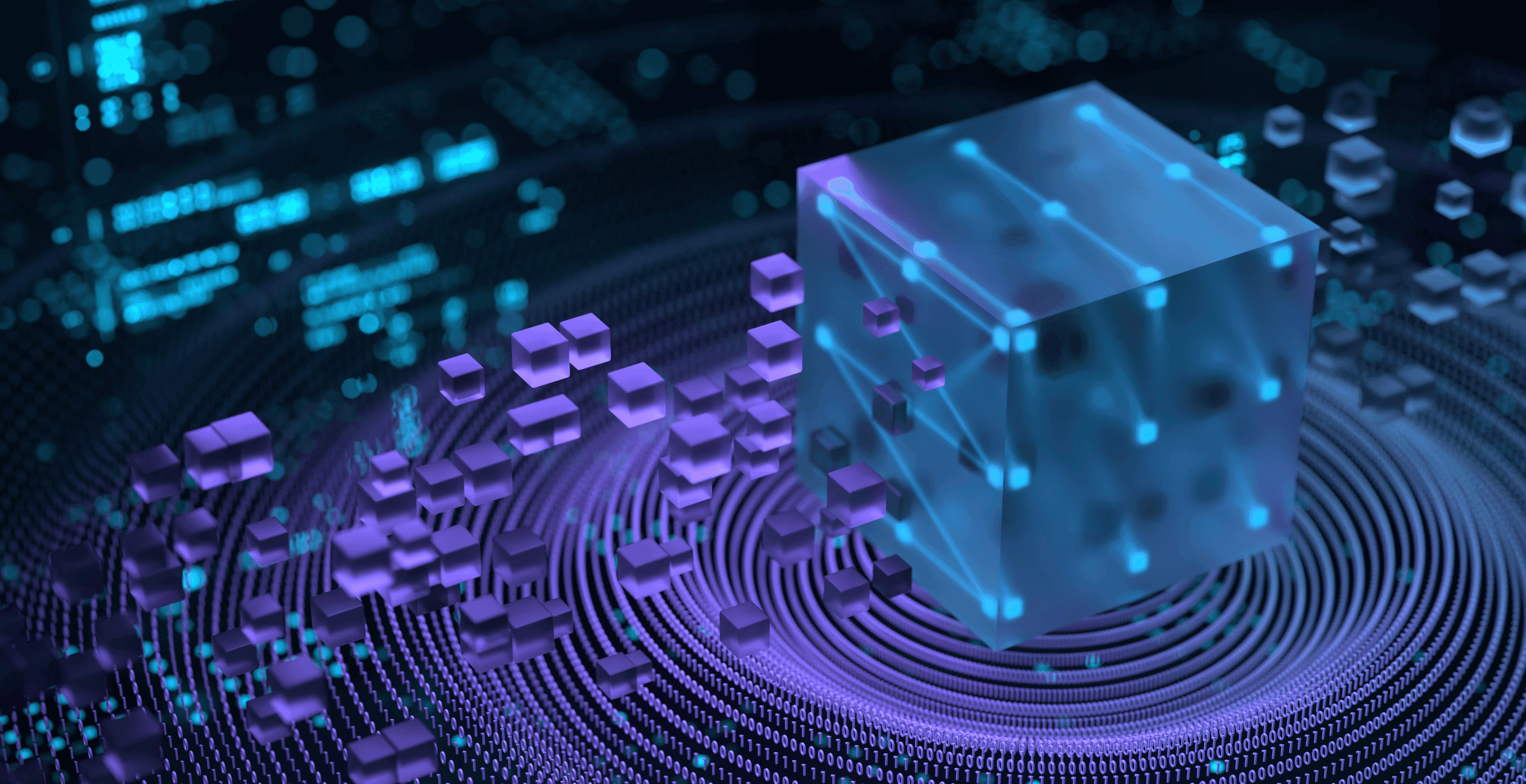
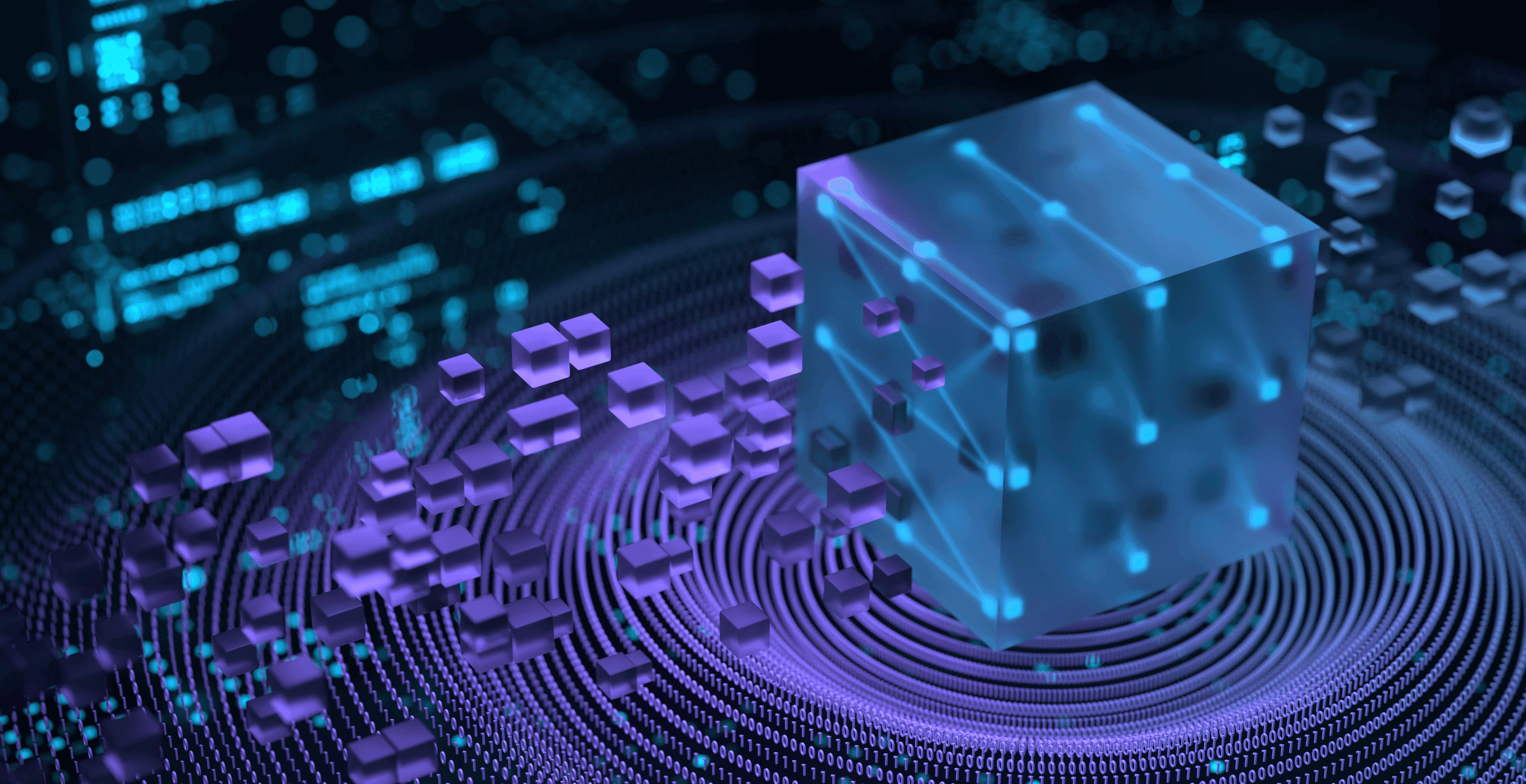
When you are operating with tabular data models, the data quality is not just significant- it’s integral. Poor quality of data can substantially affect the accuracy and reliability in tabular data models. This leads to mislead in outcomes and ineffective decisions. So, how can you assure that your data is up to par? By acknowledging errors of data directly, you can substantially improve your tabular data model’s performance.
Alright, ready to dive a bit deeper? Let's peel back the layers on tabular data and figure out what actually makes it tick.
Understanding Tabular Data and Its Challenges
Before you learn about the importance of Accuracy and Reliability in Tabular data models, let’s understand tabular data and its challenges first:-
Definition and Characteristics of Tabular Data within the Context of Machine Learning
Tabular data means a standardized layout of data that is organized into rows and columns, looking like a table in a spreadsheet. Each row in the table depicts an individual record or data point, and each column depicts an exact characteristic or attribute of the data. In the machine learning context, tabular data is a familiar data layout utilized to train models, giving a clear and organized way to input characteristics into algorithms.
Let’s now take a look at the characteristics of tabular data:-
Structured Format: Data is elegantly structured, which often refines the handling of data and pre-processing.
Heterogeneity: Columns can contain various types of data such as numeric, categorical, or datetime, each requiring different preprocessing methods.
Dimensionality: The number of characteristics in the data can differ widely, influencing the intricacy of the model and the arithmetical resources required.
Label Association: In controlled learning, each row often indulges a label or target value, which the model remembers to foretell.
Common Challenges in Tabular Data
Operating with tabular data in machine learning gives numerous challenges that can impact the performance and precision of models:
Noise: Data can include mistakes and irrelevant details, which can deceive the process of training and deteriorate the performance of a model. Noise may derive from measurement mistakes, data gathering problems, or during processing of data. For example, if you’re operating with sensor data from a manufacturing process, occasional faulty sensor readings can acquire noise, resulting in inaccurate forecasts about machine maintenance requirements.
Mislabeling: Precise labels are important for model training in supervised learning. Mislabelled data can lead to inaccurate learning and prophecies, substantially affecting the efficacy of the model. This can happen due to human mistakes in labeling of data or mistakes in automated labeling systems. For instance, if you’re instructing a model to determine types of fruits from images and some apples are erroneously labeled as oranges, the model will struggle to grasp the correct differences.
Missing Values: It’s familiar for datasets to have missing entries for some characteristics. Handling missing data needs careful approach because inappropriate handling can lead to partial or inappropriate models. Methods to handle missing data include attribution, where missing values are filled based on other data, or utilizing models that can manage missing values fundamentally. For example, if you’re inspecting patient records and some entries lack age data, you might use the median age of the dataset to fill in the gaps.
Imbalanced Data: Often, the distribution of target labels or classes in a dataset is imbalanced, which can lead to models that are biased towards the majority class. For instance, if you’re developing a fraud detection system and only 1% of your transactions are deceptive, your model might gradually become overly partial towards forecasts of non-deceptive transactions, missing many actual deceptive cases.
Correlation and Collinearity: High correlation or collinearity between features can cause issues for some models, particularly linear models, leading to inadequate performance or high variability in their predictions. It is important to discover and handle correlated characteristics for structuring healthy models. For instance, if you have two attributes in your dataset that both replicates a person’s name but in distinct units (e.g., monthly salary and annual salary), their high resemblance could veer the model’s outcomes.
Comprehending and acknowledging these challenges is crucial for efficient machine learning with tabular data, compelling extensive data preprocessing, experimental data analysis, and planned model choice.
Now that you've got the lay of the land with tabular data, let's see how it impacts data quality on model performance.
Impact on Data Quality on Model Performance
Now that you have understood Tabular data and its challenges in detail, let’s now take a look at the impact on data quality and model performance:-
The Relationship Between Data Cleanliness and the Accuracy of Models
Understanding Data Cleanliness
Data cleanliness refers to the preciseness, entirety, and correspondence of data. Clean data is mandatory for any machine learning model because the output of the model is directly influenced by its input quality. The old saying “garbage in, garbage out” completely captures this reliability, highlighting that no method, no matter how progressed, can conquer the basic flaws in the data it processes.
Impact on Model Accuracy
Clean data ensures that the model studies the right pattern and not for the noise. For instance, in a conjecturing maintenance model for machinery, precise and compatible data about machine operation times and maintenance schedules is important. False data can lead to incorrect foretelling of setbacks, causing irrelevant checks, or missed maintenance, eventually consuming time and resources.
Examples Demonstrating How Mislabeled or Noisy Data can Compromise Model Reliability
Case Study: Healthcare Diagnostics
Contemplate a model trained to identify diseases from lab results. If the training data includes conflated samples, the model might catch the inappropriate patterns, leading to wrong positives and negatives. This not only impacts the model’s dependency but also has serious indications for patient care.
Impact of Noisy Data in Financial Forecasting
In a financial situation, noisy data could indulge mistakes in transaction history or mis-classified financial data. A predictive model utilized for forecasting the trend in the market or analyzing risk of credit might then make choices based on defective theories. This could lead to poor investment approaches and inaccurate credit estimates, possibly leading to crucial financial losses.
Addressing Data Quality Issues
Rigorous data cleaning procedures are important to alleviate these problems. Techniques might include:-
Data Validation: Enforcing checks during data entry to avert mistakes and ensure coherence.
Anomaly Detection: Utilizing analytical tools or machine learning to determine exceptions that may specify data quality issues.
Regular Audits: Frequently reviewing the data and the processes that generate it to assure sustained data credibility.
Examples of Data Quality Issues
Duplicate Records: Duplicate entries can veer inspection, resulting in misleading inferences. For example, if customer information is duplicated, it may appear that there are more unique customers than there literally are, impacting marketing plans and inventory management.
Missing Values: Missing information can lead to incomplete analysis. For instance, if significant fields such as "date of purchase" or "customer age” are missing, it becomes complex to segment information and dissect trends precisely.
Inconsistent Data: Inconsistencies in data formats or entries can cause perplexity and mistakes in inspection. For example, if dates are transcribed in distinct formats (MM/DD/YYYY vs. DD/MM/YYYY), it can result in incorrect analysis of timelines or trends.
Feeling geared up? Time to roll up our sleeves and tackle how we can boost that data reliability to the next level.
Strategies for Enhancing Data Reliability
Assuring accuracy and reliability in tabular data models is important for efficient decision-making and reliable forecasting dissections. Below you will learn strategies to acknowledge these challenges, concentrating on determining and correcting misclassified data and executing no-code solutions for cleaning of data and model re-training.
Overview of Techniques for Identifying and Correcting Mislabeled Data
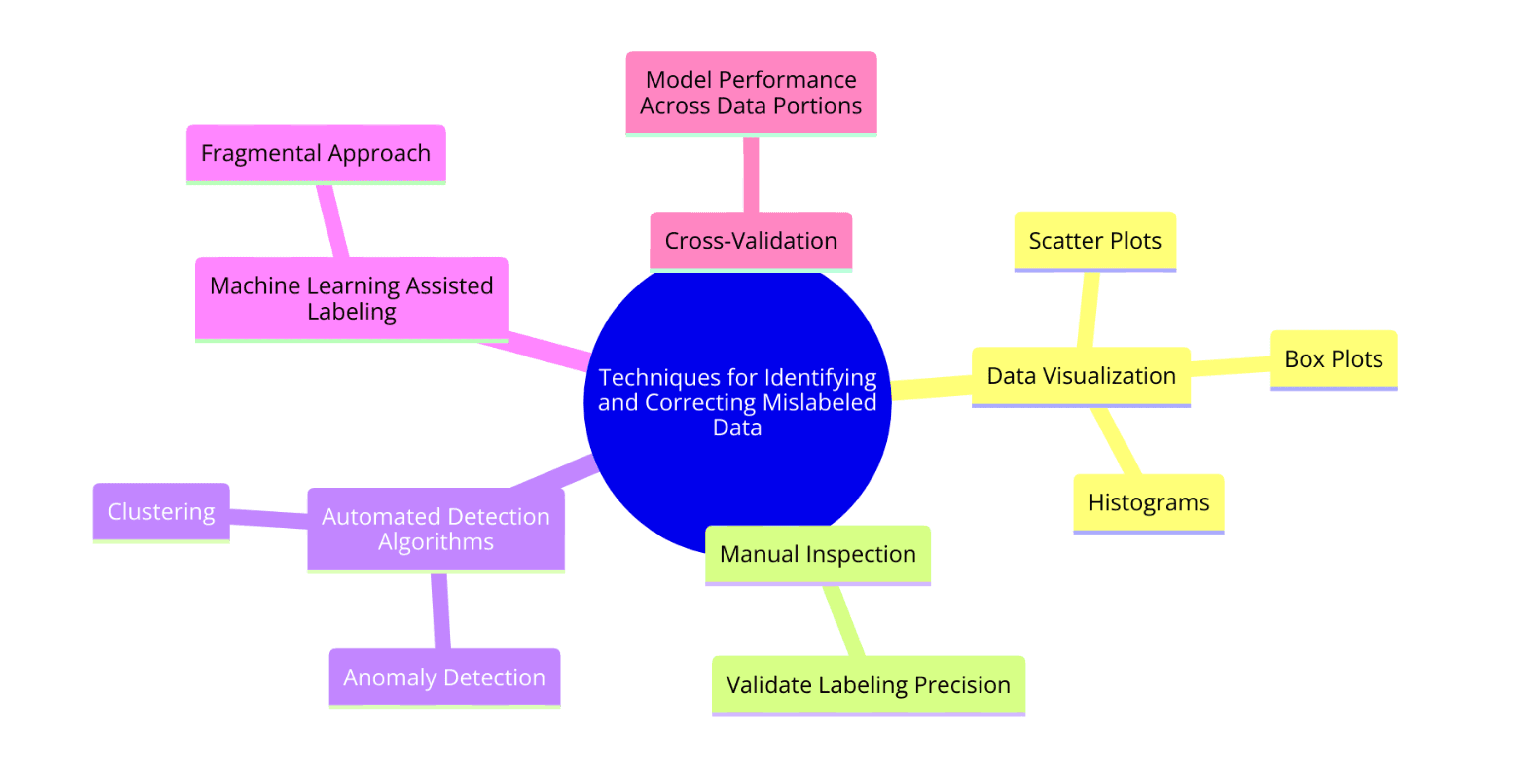
Conflating data can substantially deteriorate the performance of machine learning models. Below given are some techniques to identify and correct such data:
Data Visualization:- Utilize visualization tools to identify exceptions and anomalies that may specify mislabeling. Histograms, scatter plots and box plots can be efficient in identifying inconsistencies.
Manual Inspection: Although time-taking, manually inspecting a random sample of data can help validate labeling precision, especially in crucial areas where errors are expensive.
Automated Detection Algorithms: Execute algorithms like anomaly detection or gathering to locate data points that diverge from the norm, which might be misclassified.
Machine Learning Assisted Labeling: Use a fragmental approach where a little amount of correctly classified data helps to label a wide database.
Cross- Validation: Use a cross-validation approach to ensure that the model's performance is good across distinct portions of data, which can help identify labeling mistakes indirectly by pointing data subsets where the model performs inadequately.
Implementing No-Code Solutions and Tools for Data Cleaning and Retraining Models
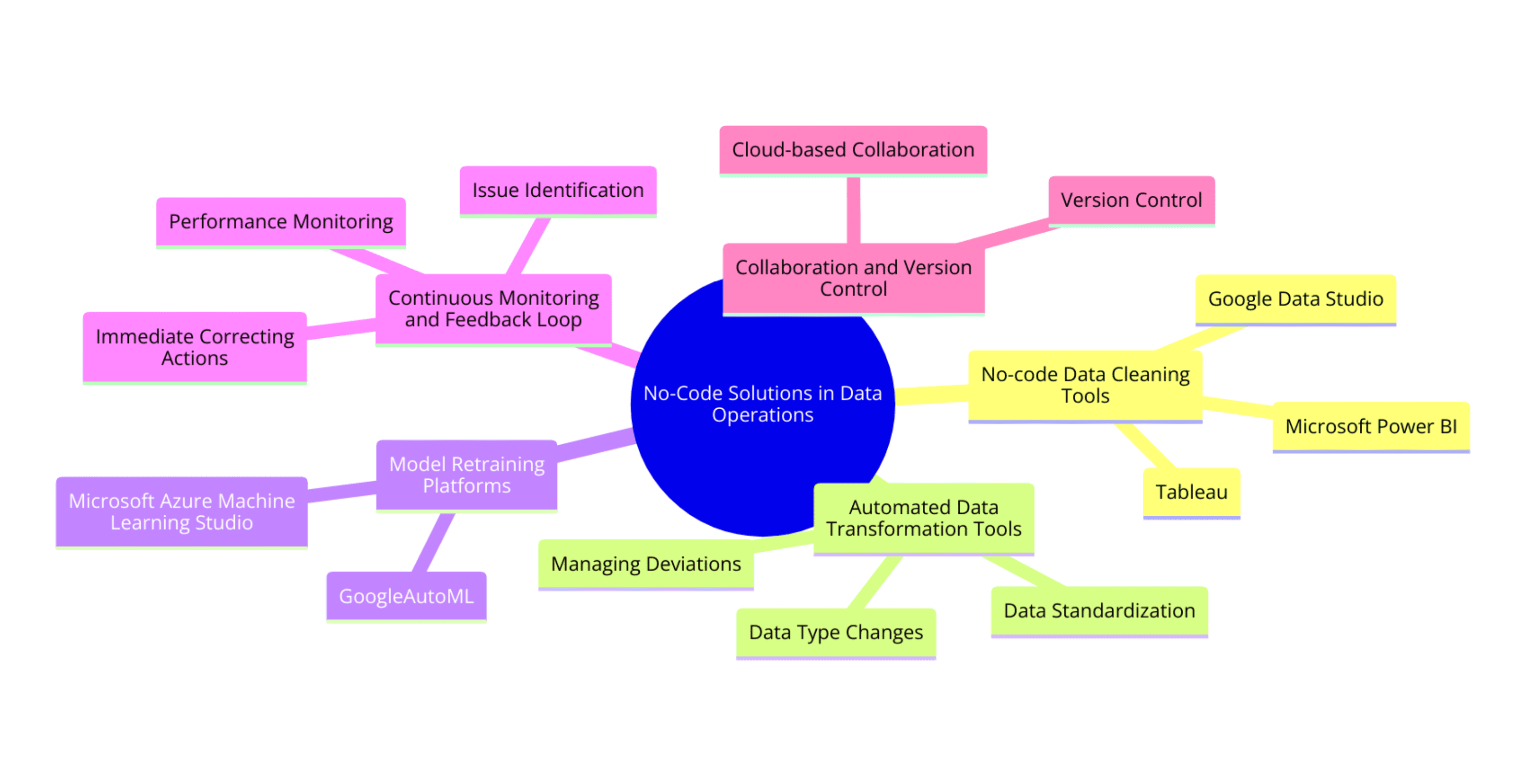
No-code tools have normalized access to intricate data operations, permitting non-specialists to perform the cleaning of data and model training. Below given are some strategies involving no-code solutions:-
No-code Data Cleaning Tools: There are many platforms which help in cleaning data like Microsoft Power BI, Google Data Studio and Tableau permits to clean data without programming. They give instinctive interfaces for abolishing copies, managing missing values, and applying restricted configuration.
Automated Data Transformation Tools: Tools like Trifacta and Alteryx instinctively detect and suggest transformations for data standardization, changing of data types, and managing deviations.
Model Retraining Platforms: No-code platforms such as GoogleAutoML and Microsoft Azure’s Machine Learning Studio offers user-friendly interfaces for training, assessing and retraining models. They often indulge characteristics for automatic data tearing, feature gauging and algorithm selection.
Continuous Monitoring and Feedback Loop: Implement systems that continuously monitor the performance of deployed models and identify potential issues with data quality and model accuracy. No-code platforms like DataRobot and H2O.ai progressively provide these characteristics, permitting immediate correcting actions.
Collaboration and Version Control: Use cloud based no-code platforms such as Airtable and Notion that accelerates partnership among team members and maintain version control of set of information and models, ensuring compatibility and detectability of modifications.
By incorporating these strategies, associations can improve the dependency of their data and the precision of their tabular data models, resulting in better venture insights and decisions.
Got everything sorted so far? Cool, let's pivot to figuring out how noise affects our data and what we can do about it.
Evaluating Model Accuracy in the Presence of Noisy Data
Now that you have know what strategies to implement to enhance data the reliability of data, let’s now move on to learning evaluating model accuracy in the presence of noisy data:
Methods for Training Models on Noisy Labeled Data and Assessing Impact on Performance
Robust Loss Functions: Loss functions that are least unresponsive to deviation and misclassified data can be helpful. Instances include the Huber loss or log-cosh loss, which unified the best properties of MSE and MAE but are less impacted by anomalies in data.
Data Cleaning: Determining and correcting mis-classifications can enhance the model accuracy before training. Approaches like manual examining, utilizing unsupervised learning to find deviation, or engaging anomaly detection, algorithms are familiar.
Regularization Techniques: Techniques such as dropout, L1/L2 regularization or early ceasing can avert the model from overfitting to the noise in the data.
Noise Robust Methods: Bootstrapping and ensemble techniques can help reduce variance and improve a model's resilience against noise.
Noise-Adaptation Layers: Integrating layers or methods that adjust to the noise level in the training data can help by vigorously adapting the training process based on the discovered noise level.
Case Studies on the Importance of Using Out-of-Sample Predictions for Finding Label Issues
Out-of-Sample Testing: Utilizing a split verification set not seen during training helps in comprehending how the model performs on new, hidden data. This can emphasize potent problems with overfitting on noisy training data.
Cross Validation: Using cross-validation approaches can give a more sturdy evaluation of performance of the model and help discover inconsistencies in the labeling of data.
Real-World Examples: Case studies often involve outlines where inaugural models trained on noisy data performed poorly when positioned in real-world environments. Re-evaluating the model with out-of-sample forecasting often leads to finding significant labeling errors.
Continuous Monitoring: Continuous monitoring model predictions against new incoming data can also help in discovering label imprecision. If forecasting constantly deviates from awaiting outcomes, it may specify problems with the training labels.
Feedback Loops: Demonstrating a feedback mechanism where forecasts are constantly retrospected and verified can help enhance the quality of labels over time. This is specifically common in fields like medical imaging or speech identification.
Integrating these techniques can substantially improve the strength and precision of models trained on noisy data. Each technique helps alleviate distinct aspects of the noise challenge and can be customized based on the particular features of the dataset and the noise present.
Feeling like a data detective yet? There's always more to discover. Here's where you can dig even deeper.
Further Research and Tools on Data Quality Improvement
List of Resources for Deepening Knowledge on Data Quality Improvement
Books: Acknowledge titles like “The Data Warehouse Toolkit” by Ralph Kimball or "Data Quality: The Accuracy Dimension" by Jack E. Olson.
Online Courses: There are many online platforms providing knowledge on Data Quality Improvement through their courses such as Coursera, Udemy and Linkedin. These platforms offer courses on data management, data quality and data governance.
Research Papers and Journals: Discover publications in journals such as the Journal of Data and Information Quality, which prospects into the latest inspection on data quality methods.
Encouragement to Participate in the Development and Use of Data Cleaning Tool
Get Involved: Immerse with open source projects on platforms such as GitHub to contribute to the growth of data cleaning tools.
Utilize Tools: Acquaint yourself with the data cleaning tools such as OpenRefine, Talend, and Data Ladder to comprehend their characteristics and applications in real-world scenarios.
Community Participation: Join pertinent online forums and discussion groups, like those on Stack Overflow or special data administration forums, to exchange thoughts and get support from the community.
Phew, that was a lot, wasn't it? Before we wrap up, let's recap why all this fuss about data quality is worth it.
Conclusion
To conclude the article, the significance of accuracy and reliability in tabular data models cannot be exaggerated. These models serve as a substratum for crucial decision-making procedures in several industries, prompting everything from planned venture decisions and to scientific research outcomes.
Ensuring that these models are both precise and reliable assures that the perception originated is responsible, which in turn improves the efficacy of decisions made based on this data. This dedication to accuracy not only secures against costly mistakes but also accelerates the confidence in data-driven planning, eventually contributing to enhanced results and inventiveness.
As data constantly evolves to grow in volume and intricacy, the concentration on maintaining the incorporation of tabular data models becomes even more critical, emphasizing the need for robust techniques and tools that affirms these necessary standards.
Ready to boost your AI models? Sign Up at RagaAI now to explore the globe’s first automated platform designed to perfect your AI applications.
When you are operating with tabular data models, the data quality is not just significant- it’s integral. Poor quality of data can substantially affect the accuracy and reliability in tabular data models. This leads to mislead in outcomes and ineffective decisions. So, how can you assure that your data is up to par? By acknowledging errors of data directly, you can substantially improve your tabular data model’s performance.
Alright, ready to dive a bit deeper? Let's peel back the layers on tabular data and figure out what actually makes it tick.
Understanding Tabular Data and Its Challenges
Before you learn about the importance of Accuracy and Reliability in Tabular data models, let’s understand tabular data and its challenges first:-
Definition and Characteristics of Tabular Data within the Context of Machine Learning
Tabular data means a standardized layout of data that is organized into rows and columns, looking like a table in a spreadsheet. Each row in the table depicts an individual record or data point, and each column depicts an exact characteristic or attribute of the data. In the machine learning context, tabular data is a familiar data layout utilized to train models, giving a clear and organized way to input characteristics into algorithms.
Let’s now take a look at the characteristics of tabular data:-
Structured Format: Data is elegantly structured, which often refines the handling of data and pre-processing.
Heterogeneity: Columns can contain various types of data such as numeric, categorical, or datetime, each requiring different preprocessing methods.
Dimensionality: The number of characteristics in the data can differ widely, influencing the intricacy of the model and the arithmetical resources required.
Label Association: In controlled learning, each row often indulges a label or target value, which the model remembers to foretell.
Common Challenges in Tabular Data
Operating with tabular data in machine learning gives numerous challenges that can impact the performance and precision of models:
Noise: Data can include mistakes and irrelevant details, which can deceive the process of training and deteriorate the performance of a model. Noise may derive from measurement mistakes, data gathering problems, or during processing of data. For example, if you’re operating with sensor data from a manufacturing process, occasional faulty sensor readings can acquire noise, resulting in inaccurate forecasts about machine maintenance requirements.
Mislabeling: Precise labels are important for model training in supervised learning. Mislabelled data can lead to inaccurate learning and prophecies, substantially affecting the efficacy of the model. This can happen due to human mistakes in labeling of data or mistakes in automated labeling systems. For instance, if you’re instructing a model to determine types of fruits from images and some apples are erroneously labeled as oranges, the model will struggle to grasp the correct differences.
Missing Values: It’s familiar for datasets to have missing entries for some characteristics. Handling missing data needs careful approach because inappropriate handling can lead to partial or inappropriate models. Methods to handle missing data include attribution, where missing values are filled based on other data, or utilizing models that can manage missing values fundamentally. For example, if you’re inspecting patient records and some entries lack age data, you might use the median age of the dataset to fill in the gaps.
Imbalanced Data: Often, the distribution of target labels or classes in a dataset is imbalanced, which can lead to models that are biased towards the majority class. For instance, if you’re developing a fraud detection system and only 1% of your transactions are deceptive, your model might gradually become overly partial towards forecasts of non-deceptive transactions, missing many actual deceptive cases.
Correlation and Collinearity: High correlation or collinearity between features can cause issues for some models, particularly linear models, leading to inadequate performance or high variability in their predictions. It is important to discover and handle correlated characteristics for structuring healthy models. For instance, if you have two attributes in your dataset that both replicates a person’s name but in distinct units (e.g., monthly salary and annual salary), their high resemblance could veer the model’s outcomes.
Comprehending and acknowledging these challenges is crucial for efficient machine learning with tabular data, compelling extensive data preprocessing, experimental data analysis, and planned model choice.
Now that you've got the lay of the land with tabular data, let's see how it impacts data quality on model performance.
Impact on Data Quality on Model Performance
Now that you have understood Tabular data and its challenges in detail, let’s now take a look at the impact on data quality and model performance:-
The Relationship Between Data Cleanliness and the Accuracy of Models
Understanding Data Cleanliness
Data cleanliness refers to the preciseness, entirety, and correspondence of data. Clean data is mandatory for any machine learning model because the output of the model is directly influenced by its input quality. The old saying “garbage in, garbage out” completely captures this reliability, highlighting that no method, no matter how progressed, can conquer the basic flaws in the data it processes.
Impact on Model Accuracy
Clean data ensures that the model studies the right pattern and not for the noise. For instance, in a conjecturing maintenance model for machinery, precise and compatible data about machine operation times and maintenance schedules is important. False data can lead to incorrect foretelling of setbacks, causing irrelevant checks, or missed maintenance, eventually consuming time and resources.
Examples Demonstrating How Mislabeled or Noisy Data can Compromise Model Reliability
Case Study: Healthcare Diagnostics
Contemplate a model trained to identify diseases from lab results. If the training data includes conflated samples, the model might catch the inappropriate patterns, leading to wrong positives and negatives. This not only impacts the model’s dependency but also has serious indications for patient care.
Impact of Noisy Data in Financial Forecasting
In a financial situation, noisy data could indulge mistakes in transaction history or mis-classified financial data. A predictive model utilized for forecasting the trend in the market or analyzing risk of credit might then make choices based on defective theories. This could lead to poor investment approaches and inaccurate credit estimates, possibly leading to crucial financial losses.
Addressing Data Quality Issues
Rigorous data cleaning procedures are important to alleviate these problems. Techniques might include:-
Data Validation: Enforcing checks during data entry to avert mistakes and ensure coherence.
Anomaly Detection: Utilizing analytical tools or machine learning to determine exceptions that may specify data quality issues.
Regular Audits: Frequently reviewing the data and the processes that generate it to assure sustained data credibility.
Examples of Data Quality Issues
Duplicate Records: Duplicate entries can veer inspection, resulting in misleading inferences. For example, if customer information is duplicated, it may appear that there are more unique customers than there literally are, impacting marketing plans and inventory management.
Missing Values: Missing information can lead to incomplete analysis. For instance, if significant fields such as "date of purchase" or "customer age” are missing, it becomes complex to segment information and dissect trends precisely.
Inconsistent Data: Inconsistencies in data formats or entries can cause perplexity and mistakes in inspection. For example, if dates are transcribed in distinct formats (MM/DD/YYYY vs. DD/MM/YYYY), it can result in incorrect analysis of timelines or trends.
Feeling geared up? Time to roll up our sleeves and tackle how we can boost that data reliability to the next level.
Strategies for Enhancing Data Reliability
Assuring accuracy and reliability in tabular data models is important for efficient decision-making and reliable forecasting dissections. Below you will learn strategies to acknowledge these challenges, concentrating on determining and correcting misclassified data and executing no-code solutions for cleaning of data and model re-training.
Overview of Techniques for Identifying and Correcting Mislabeled Data
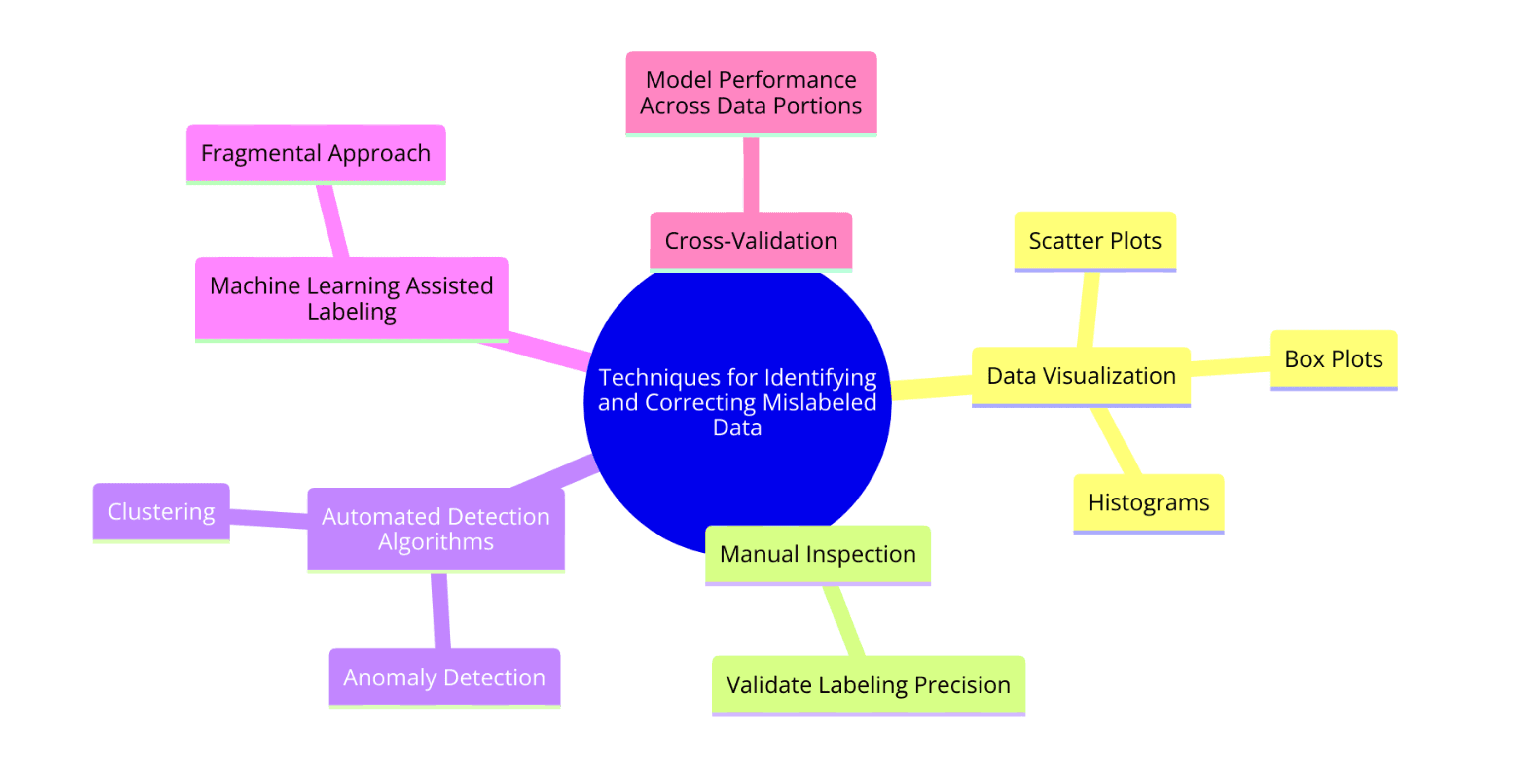
Conflating data can substantially deteriorate the performance of machine learning models. Below given are some techniques to identify and correct such data:
Data Visualization:- Utilize visualization tools to identify exceptions and anomalies that may specify mislabeling. Histograms, scatter plots and box plots can be efficient in identifying inconsistencies.
Manual Inspection: Although time-taking, manually inspecting a random sample of data can help validate labeling precision, especially in crucial areas where errors are expensive.
Automated Detection Algorithms: Execute algorithms like anomaly detection or gathering to locate data points that diverge from the norm, which might be misclassified.
Machine Learning Assisted Labeling: Use a fragmental approach where a little amount of correctly classified data helps to label a wide database.
Cross- Validation: Use a cross-validation approach to ensure that the model's performance is good across distinct portions of data, which can help identify labeling mistakes indirectly by pointing data subsets where the model performs inadequately.
Implementing No-Code Solutions and Tools for Data Cleaning and Retraining Models
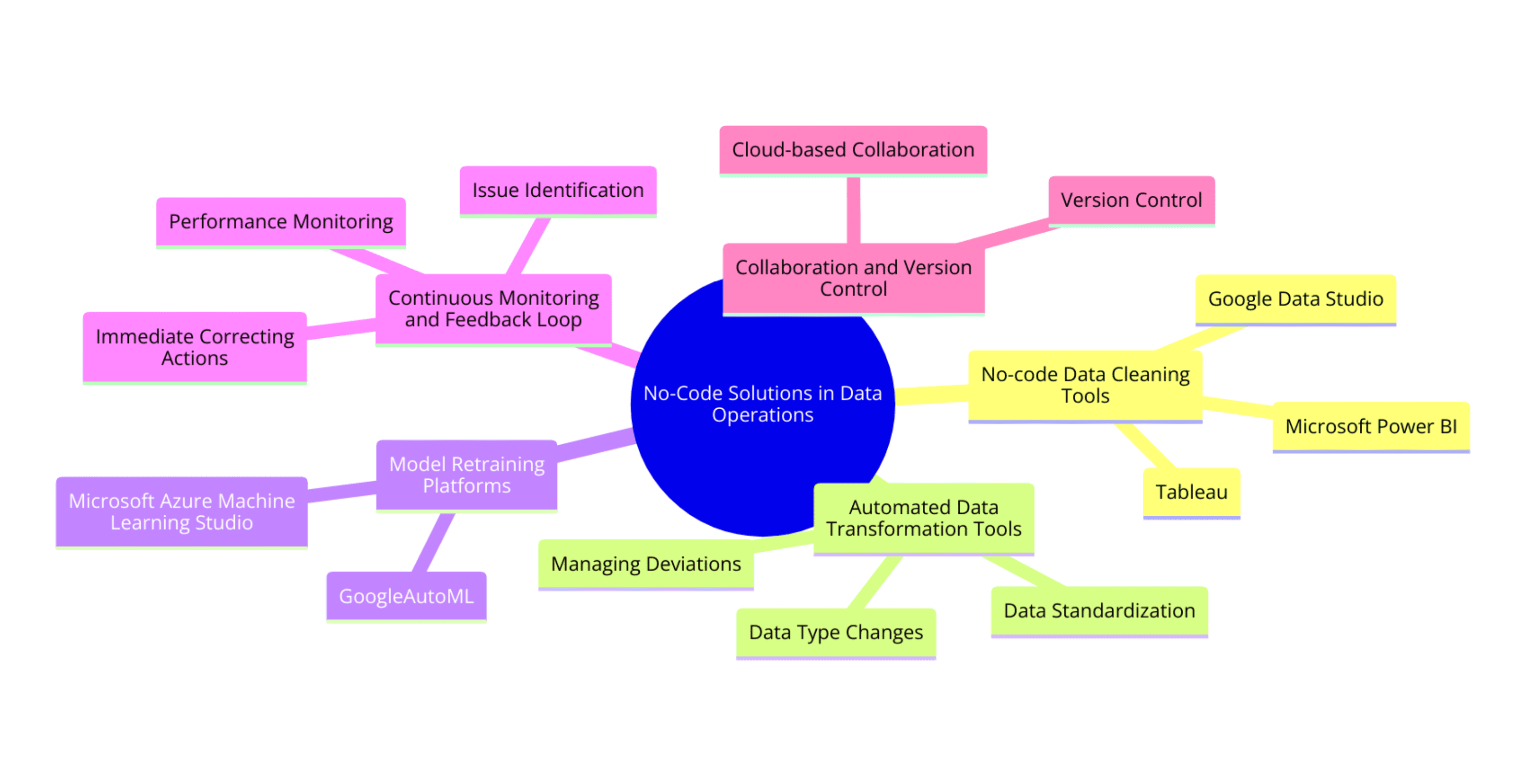
No-code tools have normalized access to intricate data operations, permitting non-specialists to perform the cleaning of data and model training. Below given are some strategies involving no-code solutions:-
No-code Data Cleaning Tools: There are many platforms which help in cleaning data like Microsoft Power BI, Google Data Studio and Tableau permits to clean data without programming. They give instinctive interfaces for abolishing copies, managing missing values, and applying restricted configuration.
Automated Data Transformation Tools: Tools like Trifacta and Alteryx instinctively detect and suggest transformations for data standardization, changing of data types, and managing deviations.
Model Retraining Platforms: No-code platforms such as GoogleAutoML and Microsoft Azure’s Machine Learning Studio offers user-friendly interfaces for training, assessing and retraining models. They often indulge characteristics for automatic data tearing, feature gauging and algorithm selection.
Continuous Monitoring and Feedback Loop: Implement systems that continuously monitor the performance of deployed models and identify potential issues with data quality and model accuracy. No-code platforms like DataRobot and H2O.ai progressively provide these characteristics, permitting immediate correcting actions.
Collaboration and Version Control: Use cloud based no-code platforms such as Airtable and Notion that accelerates partnership among team members and maintain version control of set of information and models, ensuring compatibility and detectability of modifications.
By incorporating these strategies, associations can improve the dependency of their data and the precision of their tabular data models, resulting in better venture insights and decisions.
Got everything sorted so far? Cool, let's pivot to figuring out how noise affects our data and what we can do about it.
Evaluating Model Accuracy in the Presence of Noisy Data
Now that you have know what strategies to implement to enhance data the reliability of data, let’s now move on to learning evaluating model accuracy in the presence of noisy data:
Methods for Training Models on Noisy Labeled Data and Assessing Impact on Performance
Robust Loss Functions: Loss functions that are least unresponsive to deviation and misclassified data can be helpful. Instances include the Huber loss or log-cosh loss, which unified the best properties of MSE and MAE but are less impacted by anomalies in data.
Data Cleaning: Determining and correcting mis-classifications can enhance the model accuracy before training. Approaches like manual examining, utilizing unsupervised learning to find deviation, or engaging anomaly detection, algorithms are familiar.
Regularization Techniques: Techniques such as dropout, L1/L2 regularization or early ceasing can avert the model from overfitting to the noise in the data.
Noise Robust Methods: Bootstrapping and ensemble techniques can help reduce variance and improve a model's resilience against noise.
Noise-Adaptation Layers: Integrating layers or methods that adjust to the noise level in the training data can help by vigorously adapting the training process based on the discovered noise level.
Case Studies on the Importance of Using Out-of-Sample Predictions for Finding Label Issues
Out-of-Sample Testing: Utilizing a split verification set not seen during training helps in comprehending how the model performs on new, hidden data. This can emphasize potent problems with overfitting on noisy training data.
Cross Validation: Using cross-validation approaches can give a more sturdy evaluation of performance of the model and help discover inconsistencies in the labeling of data.
Real-World Examples: Case studies often involve outlines where inaugural models trained on noisy data performed poorly when positioned in real-world environments. Re-evaluating the model with out-of-sample forecasting often leads to finding significant labeling errors.
Continuous Monitoring: Continuous monitoring model predictions against new incoming data can also help in discovering label imprecision. If forecasting constantly deviates from awaiting outcomes, it may specify problems with the training labels.
Feedback Loops: Demonstrating a feedback mechanism where forecasts are constantly retrospected and verified can help enhance the quality of labels over time. This is specifically common in fields like medical imaging or speech identification.
Integrating these techniques can substantially improve the strength and precision of models trained on noisy data. Each technique helps alleviate distinct aspects of the noise challenge and can be customized based on the particular features of the dataset and the noise present.
Feeling like a data detective yet? There's always more to discover. Here's where you can dig even deeper.
Further Research and Tools on Data Quality Improvement
List of Resources for Deepening Knowledge on Data Quality Improvement
Books: Acknowledge titles like “The Data Warehouse Toolkit” by Ralph Kimball or "Data Quality: The Accuracy Dimension" by Jack E. Olson.
Online Courses: There are many online platforms providing knowledge on Data Quality Improvement through their courses such as Coursera, Udemy and Linkedin. These platforms offer courses on data management, data quality and data governance.
Research Papers and Journals: Discover publications in journals such as the Journal of Data and Information Quality, which prospects into the latest inspection on data quality methods.
Encouragement to Participate in the Development and Use of Data Cleaning Tool
Get Involved: Immerse with open source projects on platforms such as GitHub to contribute to the growth of data cleaning tools.
Utilize Tools: Acquaint yourself with the data cleaning tools such as OpenRefine, Talend, and Data Ladder to comprehend their characteristics and applications in real-world scenarios.
Community Participation: Join pertinent online forums and discussion groups, like those on Stack Overflow or special data administration forums, to exchange thoughts and get support from the community.
Phew, that was a lot, wasn't it? Before we wrap up, let's recap why all this fuss about data quality is worth it.
Conclusion
To conclude the article, the significance of accuracy and reliability in tabular data models cannot be exaggerated. These models serve as a substratum for crucial decision-making procedures in several industries, prompting everything from planned venture decisions and to scientific research outcomes.
Ensuring that these models are both precise and reliable assures that the perception originated is responsible, which in turn improves the efficacy of decisions made based on this data. This dedication to accuracy not only secures against costly mistakes but also accelerates the confidence in data-driven planning, eventually contributing to enhanced results and inventiveness.
As data constantly evolves to grow in volume and intricacy, the concentration on maintaining the incorporation of tabular data models becomes even more critical, emphasizing the need for robust techniques and tools that affirms these necessary standards.
Ready to boost your AI models? Sign Up at RagaAI now to explore the globe’s first automated platform designed to perfect your AI applications.
When you are operating with tabular data models, the data quality is not just significant- it’s integral. Poor quality of data can substantially affect the accuracy and reliability in tabular data models. This leads to mislead in outcomes and ineffective decisions. So, how can you assure that your data is up to par? By acknowledging errors of data directly, you can substantially improve your tabular data model’s performance.
Alright, ready to dive a bit deeper? Let's peel back the layers on tabular data and figure out what actually makes it tick.
Understanding Tabular Data and Its Challenges
Before you learn about the importance of Accuracy and Reliability in Tabular data models, let’s understand tabular data and its challenges first:-
Definition and Characteristics of Tabular Data within the Context of Machine Learning
Tabular data means a standardized layout of data that is organized into rows and columns, looking like a table in a spreadsheet. Each row in the table depicts an individual record or data point, and each column depicts an exact characteristic or attribute of the data. In the machine learning context, tabular data is a familiar data layout utilized to train models, giving a clear and organized way to input characteristics into algorithms.
Let’s now take a look at the characteristics of tabular data:-
Structured Format: Data is elegantly structured, which often refines the handling of data and pre-processing.
Heterogeneity: Columns can contain various types of data such as numeric, categorical, or datetime, each requiring different preprocessing methods.
Dimensionality: The number of characteristics in the data can differ widely, influencing the intricacy of the model and the arithmetical resources required.
Label Association: In controlled learning, each row often indulges a label or target value, which the model remembers to foretell.
Common Challenges in Tabular Data
Operating with tabular data in machine learning gives numerous challenges that can impact the performance and precision of models:
Noise: Data can include mistakes and irrelevant details, which can deceive the process of training and deteriorate the performance of a model. Noise may derive from measurement mistakes, data gathering problems, or during processing of data. For example, if you’re operating with sensor data from a manufacturing process, occasional faulty sensor readings can acquire noise, resulting in inaccurate forecasts about machine maintenance requirements.
Mislabeling: Precise labels are important for model training in supervised learning. Mislabelled data can lead to inaccurate learning and prophecies, substantially affecting the efficacy of the model. This can happen due to human mistakes in labeling of data or mistakes in automated labeling systems. For instance, if you’re instructing a model to determine types of fruits from images and some apples are erroneously labeled as oranges, the model will struggle to grasp the correct differences.
Missing Values: It’s familiar for datasets to have missing entries for some characteristics. Handling missing data needs careful approach because inappropriate handling can lead to partial or inappropriate models. Methods to handle missing data include attribution, where missing values are filled based on other data, or utilizing models that can manage missing values fundamentally. For example, if you’re inspecting patient records and some entries lack age data, you might use the median age of the dataset to fill in the gaps.
Imbalanced Data: Often, the distribution of target labels or classes in a dataset is imbalanced, which can lead to models that are biased towards the majority class. For instance, if you’re developing a fraud detection system and only 1% of your transactions are deceptive, your model might gradually become overly partial towards forecasts of non-deceptive transactions, missing many actual deceptive cases.
Correlation and Collinearity: High correlation or collinearity between features can cause issues for some models, particularly linear models, leading to inadequate performance or high variability in their predictions. It is important to discover and handle correlated characteristics for structuring healthy models. For instance, if you have two attributes in your dataset that both replicates a person’s name but in distinct units (e.g., monthly salary and annual salary), their high resemblance could veer the model’s outcomes.
Comprehending and acknowledging these challenges is crucial for efficient machine learning with tabular data, compelling extensive data preprocessing, experimental data analysis, and planned model choice.
Now that you've got the lay of the land with tabular data, let's see how it impacts data quality on model performance.
Impact on Data Quality on Model Performance
Now that you have understood Tabular data and its challenges in detail, let’s now take a look at the impact on data quality and model performance:-
The Relationship Between Data Cleanliness and the Accuracy of Models
Understanding Data Cleanliness
Data cleanliness refers to the preciseness, entirety, and correspondence of data. Clean data is mandatory for any machine learning model because the output of the model is directly influenced by its input quality. The old saying “garbage in, garbage out” completely captures this reliability, highlighting that no method, no matter how progressed, can conquer the basic flaws in the data it processes.
Impact on Model Accuracy
Clean data ensures that the model studies the right pattern and not for the noise. For instance, in a conjecturing maintenance model for machinery, precise and compatible data about machine operation times and maintenance schedules is important. False data can lead to incorrect foretelling of setbacks, causing irrelevant checks, or missed maintenance, eventually consuming time and resources.
Examples Demonstrating How Mislabeled or Noisy Data can Compromise Model Reliability
Case Study: Healthcare Diagnostics
Contemplate a model trained to identify diseases from lab results. If the training data includes conflated samples, the model might catch the inappropriate patterns, leading to wrong positives and negatives. This not only impacts the model’s dependency but also has serious indications for patient care.
Impact of Noisy Data in Financial Forecasting
In a financial situation, noisy data could indulge mistakes in transaction history or mis-classified financial data. A predictive model utilized for forecasting the trend in the market or analyzing risk of credit might then make choices based on defective theories. This could lead to poor investment approaches and inaccurate credit estimates, possibly leading to crucial financial losses.
Addressing Data Quality Issues
Rigorous data cleaning procedures are important to alleviate these problems. Techniques might include:-
Data Validation: Enforcing checks during data entry to avert mistakes and ensure coherence.
Anomaly Detection: Utilizing analytical tools or machine learning to determine exceptions that may specify data quality issues.
Regular Audits: Frequently reviewing the data and the processes that generate it to assure sustained data credibility.
Examples of Data Quality Issues
Duplicate Records: Duplicate entries can veer inspection, resulting in misleading inferences. For example, if customer information is duplicated, it may appear that there are more unique customers than there literally are, impacting marketing plans and inventory management.
Missing Values: Missing information can lead to incomplete analysis. For instance, if significant fields such as "date of purchase" or "customer age” are missing, it becomes complex to segment information and dissect trends precisely.
Inconsistent Data: Inconsistencies in data formats or entries can cause perplexity and mistakes in inspection. For example, if dates are transcribed in distinct formats (MM/DD/YYYY vs. DD/MM/YYYY), it can result in incorrect analysis of timelines or trends.
Feeling geared up? Time to roll up our sleeves and tackle how we can boost that data reliability to the next level.
Strategies for Enhancing Data Reliability
Assuring accuracy and reliability in tabular data models is important for efficient decision-making and reliable forecasting dissections. Below you will learn strategies to acknowledge these challenges, concentrating on determining and correcting misclassified data and executing no-code solutions for cleaning of data and model re-training.
Overview of Techniques for Identifying and Correcting Mislabeled Data
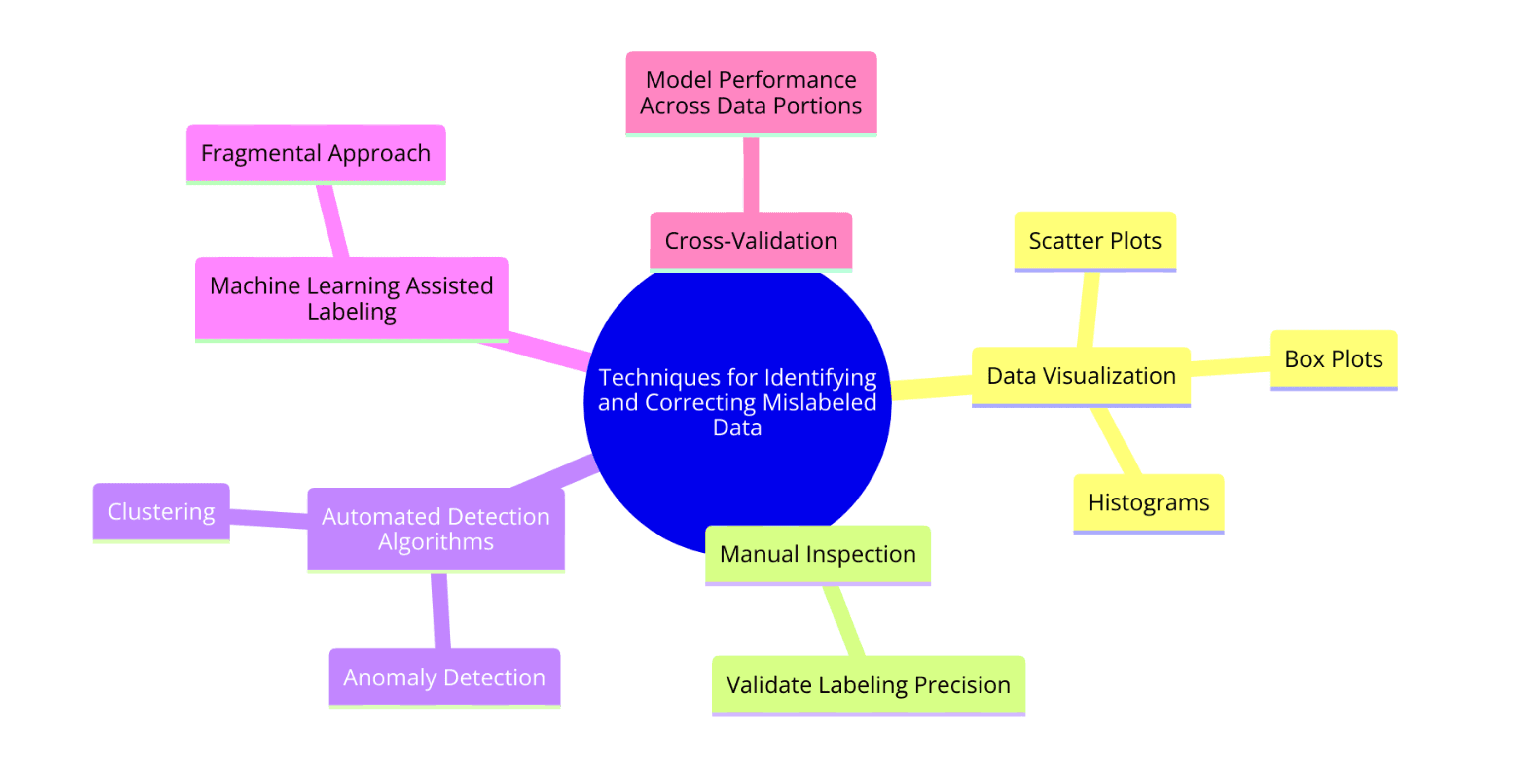
Conflating data can substantially deteriorate the performance of machine learning models. Below given are some techniques to identify and correct such data:
Data Visualization:- Utilize visualization tools to identify exceptions and anomalies that may specify mislabeling. Histograms, scatter plots and box plots can be efficient in identifying inconsistencies.
Manual Inspection: Although time-taking, manually inspecting a random sample of data can help validate labeling precision, especially in crucial areas where errors are expensive.
Automated Detection Algorithms: Execute algorithms like anomaly detection or gathering to locate data points that diverge from the norm, which might be misclassified.
Machine Learning Assisted Labeling: Use a fragmental approach where a little amount of correctly classified data helps to label a wide database.
Cross- Validation: Use a cross-validation approach to ensure that the model's performance is good across distinct portions of data, which can help identify labeling mistakes indirectly by pointing data subsets where the model performs inadequately.
Implementing No-Code Solutions and Tools for Data Cleaning and Retraining Models
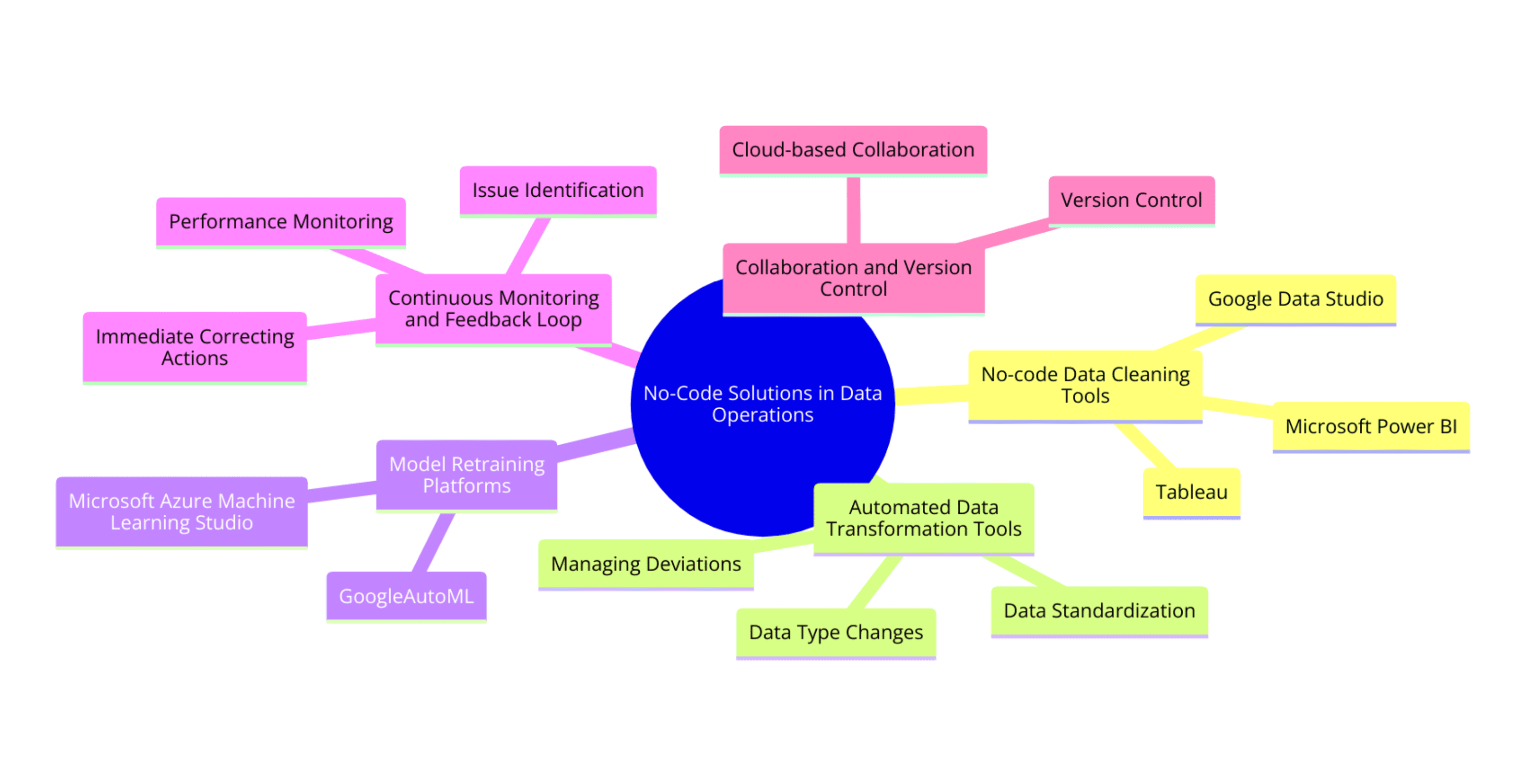
No-code tools have normalized access to intricate data operations, permitting non-specialists to perform the cleaning of data and model training. Below given are some strategies involving no-code solutions:-
No-code Data Cleaning Tools: There are many platforms which help in cleaning data like Microsoft Power BI, Google Data Studio and Tableau permits to clean data without programming. They give instinctive interfaces for abolishing copies, managing missing values, and applying restricted configuration.
Automated Data Transformation Tools: Tools like Trifacta and Alteryx instinctively detect and suggest transformations for data standardization, changing of data types, and managing deviations.
Model Retraining Platforms: No-code platforms such as GoogleAutoML and Microsoft Azure’s Machine Learning Studio offers user-friendly interfaces for training, assessing and retraining models. They often indulge characteristics for automatic data tearing, feature gauging and algorithm selection.
Continuous Monitoring and Feedback Loop: Implement systems that continuously monitor the performance of deployed models and identify potential issues with data quality and model accuracy. No-code platforms like DataRobot and H2O.ai progressively provide these characteristics, permitting immediate correcting actions.
Collaboration and Version Control: Use cloud based no-code platforms such as Airtable and Notion that accelerates partnership among team members and maintain version control of set of information and models, ensuring compatibility and detectability of modifications.
By incorporating these strategies, associations can improve the dependency of their data and the precision of their tabular data models, resulting in better venture insights and decisions.
Got everything sorted so far? Cool, let's pivot to figuring out how noise affects our data and what we can do about it.
Evaluating Model Accuracy in the Presence of Noisy Data
Now that you have know what strategies to implement to enhance data the reliability of data, let’s now move on to learning evaluating model accuracy in the presence of noisy data:
Methods for Training Models on Noisy Labeled Data and Assessing Impact on Performance
Robust Loss Functions: Loss functions that are least unresponsive to deviation and misclassified data can be helpful. Instances include the Huber loss or log-cosh loss, which unified the best properties of MSE and MAE but are less impacted by anomalies in data.
Data Cleaning: Determining and correcting mis-classifications can enhance the model accuracy before training. Approaches like manual examining, utilizing unsupervised learning to find deviation, or engaging anomaly detection, algorithms are familiar.
Regularization Techniques: Techniques such as dropout, L1/L2 regularization or early ceasing can avert the model from overfitting to the noise in the data.
Noise Robust Methods: Bootstrapping and ensemble techniques can help reduce variance and improve a model's resilience against noise.
Noise-Adaptation Layers: Integrating layers or methods that adjust to the noise level in the training data can help by vigorously adapting the training process based on the discovered noise level.
Case Studies on the Importance of Using Out-of-Sample Predictions for Finding Label Issues
Out-of-Sample Testing: Utilizing a split verification set not seen during training helps in comprehending how the model performs on new, hidden data. This can emphasize potent problems with overfitting on noisy training data.
Cross Validation: Using cross-validation approaches can give a more sturdy evaluation of performance of the model and help discover inconsistencies in the labeling of data.
Real-World Examples: Case studies often involve outlines where inaugural models trained on noisy data performed poorly when positioned in real-world environments. Re-evaluating the model with out-of-sample forecasting often leads to finding significant labeling errors.
Continuous Monitoring: Continuous monitoring model predictions against new incoming data can also help in discovering label imprecision. If forecasting constantly deviates from awaiting outcomes, it may specify problems with the training labels.
Feedback Loops: Demonstrating a feedback mechanism where forecasts are constantly retrospected and verified can help enhance the quality of labels over time. This is specifically common in fields like medical imaging or speech identification.
Integrating these techniques can substantially improve the strength and precision of models trained on noisy data. Each technique helps alleviate distinct aspects of the noise challenge and can be customized based on the particular features of the dataset and the noise present.
Feeling like a data detective yet? There's always more to discover. Here's where you can dig even deeper.
Further Research and Tools on Data Quality Improvement
List of Resources for Deepening Knowledge on Data Quality Improvement
Books: Acknowledge titles like “The Data Warehouse Toolkit” by Ralph Kimball or "Data Quality: The Accuracy Dimension" by Jack E. Olson.
Online Courses: There are many online platforms providing knowledge on Data Quality Improvement through their courses such as Coursera, Udemy and Linkedin. These platforms offer courses on data management, data quality and data governance.
Research Papers and Journals: Discover publications in journals such as the Journal of Data and Information Quality, which prospects into the latest inspection on data quality methods.
Encouragement to Participate in the Development and Use of Data Cleaning Tool
Get Involved: Immerse with open source projects on platforms such as GitHub to contribute to the growth of data cleaning tools.
Utilize Tools: Acquaint yourself with the data cleaning tools such as OpenRefine, Talend, and Data Ladder to comprehend their characteristics and applications in real-world scenarios.
Community Participation: Join pertinent online forums and discussion groups, like those on Stack Overflow or special data administration forums, to exchange thoughts and get support from the community.
Phew, that was a lot, wasn't it? Before we wrap up, let's recap why all this fuss about data quality is worth it.
Conclusion
To conclude the article, the significance of accuracy and reliability in tabular data models cannot be exaggerated. These models serve as a substratum for crucial decision-making procedures in several industries, prompting everything from planned venture decisions and to scientific research outcomes.
Ensuring that these models are both precise and reliable assures that the perception originated is responsible, which in turn improves the efficacy of decisions made based on this data. This dedication to accuracy not only secures against costly mistakes but also accelerates the confidence in data-driven planning, eventually contributing to enhanced results and inventiveness.
As data constantly evolves to grow in volume and intricacy, the concentration on maintaining the incorporation of tabular data models becomes even more critical, emphasizing the need for robust techniques and tools that affirms these necessary standards.
Ready to boost your AI models? Sign Up at RagaAI now to explore the globe’s first automated platform designed to perfect your AI applications.
When you are operating with tabular data models, the data quality is not just significant- it’s integral. Poor quality of data can substantially affect the accuracy and reliability in tabular data models. This leads to mislead in outcomes and ineffective decisions. So, how can you assure that your data is up to par? By acknowledging errors of data directly, you can substantially improve your tabular data model’s performance.
Alright, ready to dive a bit deeper? Let's peel back the layers on tabular data and figure out what actually makes it tick.
Understanding Tabular Data and Its Challenges
Before you learn about the importance of Accuracy and Reliability in Tabular data models, let’s understand tabular data and its challenges first:-
Definition and Characteristics of Tabular Data within the Context of Machine Learning
Tabular data means a standardized layout of data that is organized into rows and columns, looking like a table in a spreadsheet. Each row in the table depicts an individual record or data point, and each column depicts an exact characteristic or attribute of the data. In the machine learning context, tabular data is a familiar data layout utilized to train models, giving a clear and organized way to input characteristics into algorithms.
Let’s now take a look at the characteristics of tabular data:-
Structured Format: Data is elegantly structured, which often refines the handling of data and pre-processing.
Heterogeneity: Columns can contain various types of data such as numeric, categorical, or datetime, each requiring different preprocessing methods.
Dimensionality: The number of characteristics in the data can differ widely, influencing the intricacy of the model and the arithmetical resources required.
Label Association: In controlled learning, each row often indulges a label or target value, which the model remembers to foretell.
Common Challenges in Tabular Data
Operating with tabular data in machine learning gives numerous challenges that can impact the performance and precision of models:
Noise: Data can include mistakes and irrelevant details, which can deceive the process of training and deteriorate the performance of a model. Noise may derive from measurement mistakes, data gathering problems, or during processing of data. For example, if you’re operating with sensor data from a manufacturing process, occasional faulty sensor readings can acquire noise, resulting in inaccurate forecasts about machine maintenance requirements.
Mislabeling: Precise labels are important for model training in supervised learning. Mislabelled data can lead to inaccurate learning and prophecies, substantially affecting the efficacy of the model. This can happen due to human mistakes in labeling of data or mistakes in automated labeling systems. For instance, if you’re instructing a model to determine types of fruits from images and some apples are erroneously labeled as oranges, the model will struggle to grasp the correct differences.
Missing Values: It’s familiar for datasets to have missing entries for some characteristics. Handling missing data needs careful approach because inappropriate handling can lead to partial or inappropriate models. Methods to handle missing data include attribution, where missing values are filled based on other data, or utilizing models that can manage missing values fundamentally. For example, if you’re inspecting patient records and some entries lack age data, you might use the median age of the dataset to fill in the gaps.
Imbalanced Data: Often, the distribution of target labels or classes in a dataset is imbalanced, which can lead to models that are biased towards the majority class. For instance, if you’re developing a fraud detection system and only 1% of your transactions are deceptive, your model might gradually become overly partial towards forecasts of non-deceptive transactions, missing many actual deceptive cases.
Correlation and Collinearity: High correlation or collinearity between features can cause issues for some models, particularly linear models, leading to inadequate performance or high variability in their predictions. It is important to discover and handle correlated characteristics for structuring healthy models. For instance, if you have two attributes in your dataset that both replicates a person’s name but in distinct units (e.g., monthly salary and annual salary), their high resemblance could veer the model’s outcomes.
Comprehending and acknowledging these challenges is crucial for efficient machine learning with tabular data, compelling extensive data preprocessing, experimental data analysis, and planned model choice.
Now that you've got the lay of the land with tabular data, let's see how it impacts data quality on model performance.
Impact on Data Quality on Model Performance
Now that you have understood Tabular data and its challenges in detail, let’s now take a look at the impact on data quality and model performance:-
The Relationship Between Data Cleanliness and the Accuracy of Models
Understanding Data Cleanliness
Data cleanliness refers to the preciseness, entirety, and correspondence of data. Clean data is mandatory for any machine learning model because the output of the model is directly influenced by its input quality. The old saying “garbage in, garbage out” completely captures this reliability, highlighting that no method, no matter how progressed, can conquer the basic flaws in the data it processes.
Impact on Model Accuracy
Clean data ensures that the model studies the right pattern and not for the noise. For instance, in a conjecturing maintenance model for machinery, precise and compatible data about machine operation times and maintenance schedules is important. False data can lead to incorrect foretelling of setbacks, causing irrelevant checks, or missed maintenance, eventually consuming time and resources.
Examples Demonstrating How Mislabeled or Noisy Data can Compromise Model Reliability
Case Study: Healthcare Diagnostics
Contemplate a model trained to identify diseases from lab results. If the training data includes conflated samples, the model might catch the inappropriate patterns, leading to wrong positives and negatives. This not only impacts the model’s dependency but also has serious indications for patient care.
Impact of Noisy Data in Financial Forecasting
In a financial situation, noisy data could indulge mistakes in transaction history or mis-classified financial data. A predictive model utilized for forecasting the trend in the market or analyzing risk of credit might then make choices based on defective theories. This could lead to poor investment approaches and inaccurate credit estimates, possibly leading to crucial financial losses.
Addressing Data Quality Issues
Rigorous data cleaning procedures are important to alleviate these problems. Techniques might include:-
Data Validation: Enforcing checks during data entry to avert mistakes and ensure coherence.
Anomaly Detection: Utilizing analytical tools or machine learning to determine exceptions that may specify data quality issues.
Regular Audits: Frequently reviewing the data and the processes that generate it to assure sustained data credibility.
Examples of Data Quality Issues
Duplicate Records: Duplicate entries can veer inspection, resulting in misleading inferences. For example, if customer information is duplicated, it may appear that there are more unique customers than there literally are, impacting marketing plans and inventory management.
Missing Values: Missing information can lead to incomplete analysis. For instance, if significant fields such as "date of purchase" or "customer age” are missing, it becomes complex to segment information and dissect trends precisely.
Inconsistent Data: Inconsistencies in data formats or entries can cause perplexity and mistakes in inspection. For example, if dates are transcribed in distinct formats (MM/DD/YYYY vs. DD/MM/YYYY), it can result in incorrect analysis of timelines or trends.
Feeling geared up? Time to roll up our sleeves and tackle how we can boost that data reliability to the next level.
Strategies for Enhancing Data Reliability
Assuring accuracy and reliability in tabular data models is important for efficient decision-making and reliable forecasting dissections. Below you will learn strategies to acknowledge these challenges, concentrating on determining and correcting misclassified data and executing no-code solutions for cleaning of data and model re-training.
Overview of Techniques for Identifying and Correcting Mislabeled Data
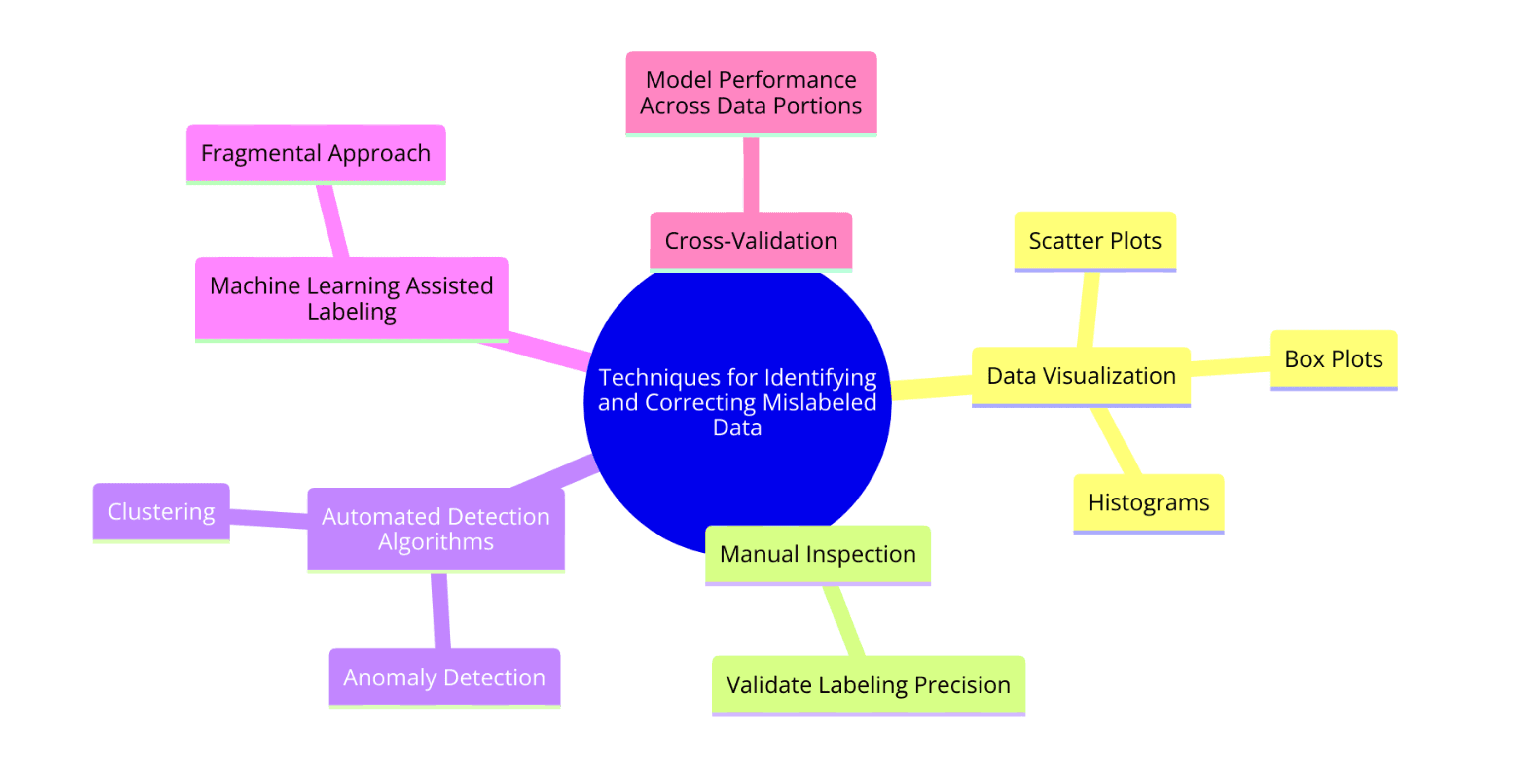
Conflating data can substantially deteriorate the performance of machine learning models. Below given are some techniques to identify and correct such data:
Data Visualization:- Utilize visualization tools to identify exceptions and anomalies that may specify mislabeling. Histograms, scatter plots and box plots can be efficient in identifying inconsistencies.
Manual Inspection: Although time-taking, manually inspecting a random sample of data can help validate labeling precision, especially in crucial areas where errors are expensive.
Automated Detection Algorithms: Execute algorithms like anomaly detection or gathering to locate data points that diverge from the norm, which might be misclassified.
Machine Learning Assisted Labeling: Use a fragmental approach where a little amount of correctly classified data helps to label a wide database.
Cross- Validation: Use a cross-validation approach to ensure that the model's performance is good across distinct portions of data, which can help identify labeling mistakes indirectly by pointing data subsets where the model performs inadequately.
Implementing No-Code Solutions and Tools for Data Cleaning and Retraining Models
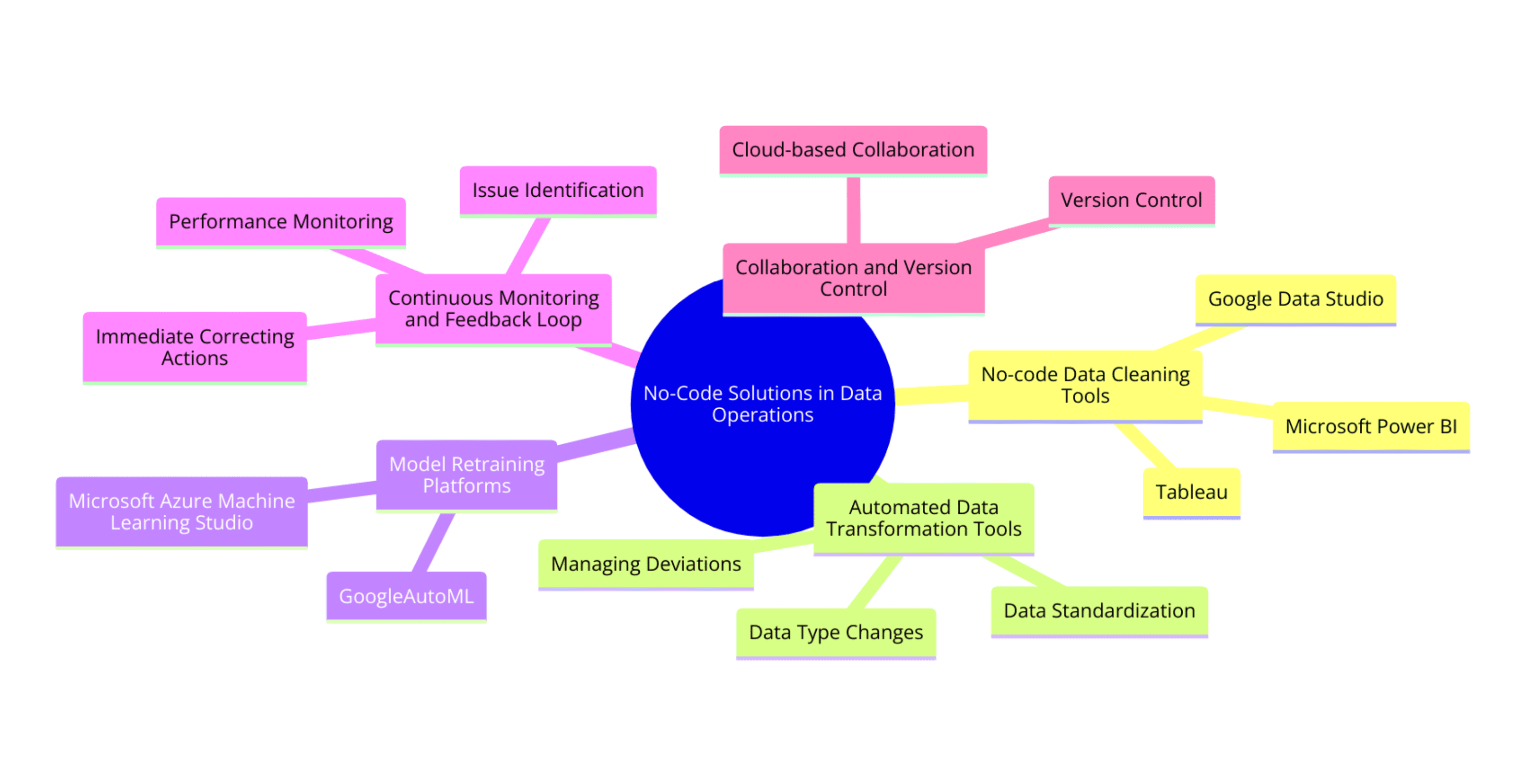
No-code tools have normalized access to intricate data operations, permitting non-specialists to perform the cleaning of data and model training. Below given are some strategies involving no-code solutions:-
No-code Data Cleaning Tools: There are many platforms which help in cleaning data like Microsoft Power BI, Google Data Studio and Tableau permits to clean data without programming. They give instinctive interfaces for abolishing copies, managing missing values, and applying restricted configuration.
Automated Data Transformation Tools: Tools like Trifacta and Alteryx instinctively detect and suggest transformations for data standardization, changing of data types, and managing deviations.
Model Retraining Platforms: No-code platforms such as GoogleAutoML and Microsoft Azure’s Machine Learning Studio offers user-friendly interfaces for training, assessing and retraining models. They often indulge characteristics for automatic data tearing, feature gauging and algorithm selection.
Continuous Monitoring and Feedback Loop: Implement systems that continuously monitor the performance of deployed models and identify potential issues with data quality and model accuracy. No-code platforms like DataRobot and H2O.ai progressively provide these characteristics, permitting immediate correcting actions.
Collaboration and Version Control: Use cloud based no-code platforms such as Airtable and Notion that accelerates partnership among team members and maintain version control of set of information and models, ensuring compatibility and detectability of modifications.
By incorporating these strategies, associations can improve the dependency of their data and the precision of their tabular data models, resulting in better venture insights and decisions.
Got everything sorted so far? Cool, let's pivot to figuring out how noise affects our data and what we can do about it.
Evaluating Model Accuracy in the Presence of Noisy Data
Now that you have know what strategies to implement to enhance data the reliability of data, let’s now move on to learning evaluating model accuracy in the presence of noisy data:
Methods for Training Models on Noisy Labeled Data and Assessing Impact on Performance
Robust Loss Functions: Loss functions that are least unresponsive to deviation and misclassified data can be helpful. Instances include the Huber loss or log-cosh loss, which unified the best properties of MSE and MAE but are less impacted by anomalies in data.
Data Cleaning: Determining and correcting mis-classifications can enhance the model accuracy before training. Approaches like manual examining, utilizing unsupervised learning to find deviation, or engaging anomaly detection, algorithms are familiar.
Regularization Techniques: Techniques such as dropout, L1/L2 regularization or early ceasing can avert the model from overfitting to the noise in the data.
Noise Robust Methods: Bootstrapping and ensemble techniques can help reduce variance and improve a model's resilience against noise.
Noise-Adaptation Layers: Integrating layers or methods that adjust to the noise level in the training data can help by vigorously adapting the training process based on the discovered noise level.
Case Studies on the Importance of Using Out-of-Sample Predictions for Finding Label Issues
Out-of-Sample Testing: Utilizing a split verification set not seen during training helps in comprehending how the model performs on new, hidden data. This can emphasize potent problems with overfitting on noisy training data.
Cross Validation: Using cross-validation approaches can give a more sturdy evaluation of performance of the model and help discover inconsistencies in the labeling of data.
Real-World Examples: Case studies often involve outlines where inaugural models trained on noisy data performed poorly when positioned in real-world environments. Re-evaluating the model with out-of-sample forecasting often leads to finding significant labeling errors.
Continuous Monitoring: Continuous monitoring model predictions against new incoming data can also help in discovering label imprecision. If forecasting constantly deviates from awaiting outcomes, it may specify problems with the training labels.
Feedback Loops: Demonstrating a feedback mechanism where forecasts are constantly retrospected and verified can help enhance the quality of labels over time. This is specifically common in fields like medical imaging or speech identification.
Integrating these techniques can substantially improve the strength and precision of models trained on noisy data. Each technique helps alleviate distinct aspects of the noise challenge and can be customized based on the particular features of the dataset and the noise present.
Feeling like a data detective yet? There's always more to discover. Here's where you can dig even deeper.
Further Research and Tools on Data Quality Improvement
List of Resources for Deepening Knowledge on Data Quality Improvement
Books: Acknowledge titles like “The Data Warehouse Toolkit” by Ralph Kimball or "Data Quality: The Accuracy Dimension" by Jack E. Olson.
Online Courses: There are many online platforms providing knowledge on Data Quality Improvement through their courses such as Coursera, Udemy and Linkedin. These platforms offer courses on data management, data quality and data governance.
Research Papers and Journals: Discover publications in journals such as the Journal of Data and Information Quality, which prospects into the latest inspection on data quality methods.
Encouragement to Participate in the Development and Use of Data Cleaning Tool
Get Involved: Immerse with open source projects on platforms such as GitHub to contribute to the growth of data cleaning tools.
Utilize Tools: Acquaint yourself with the data cleaning tools such as OpenRefine, Talend, and Data Ladder to comprehend their characteristics and applications in real-world scenarios.
Community Participation: Join pertinent online forums and discussion groups, like those on Stack Overflow or special data administration forums, to exchange thoughts and get support from the community.
Phew, that was a lot, wasn't it? Before we wrap up, let's recap why all this fuss about data quality is worth it.
Conclusion
To conclude the article, the significance of accuracy and reliability in tabular data models cannot be exaggerated. These models serve as a substratum for crucial decision-making procedures in several industries, prompting everything from planned venture decisions and to scientific research outcomes.
Ensuring that these models are both precise and reliable assures that the perception originated is responsible, which in turn improves the efficacy of decisions made based on this data. This dedication to accuracy not only secures against costly mistakes but also accelerates the confidence in data-driven planning, eventually contributing to enhanced results and inventiveness.
As data constantly evolves to grow in volume and intricacy, the concentration on maintaining the incorporation of tabular data models becomes even more critical, emphasizing the need for robust techniques and tools that affirms these necessary standards.
Ready to boost your AI models? Sign Up at RagaAI now to explore the globe’s first automated platform designed to perfect your AI applications.
When you are operating with tabular data models, the data quality is not just significant- it’s integral. Poor quality of data can substantially affect the accuracy and reliability in tabular data models. This leads to mislead in outcomes and ineffective decisions. So, how can you assure that your data is up to par? By acknowledging errors of data directly, you can substantially improve your tabular data model’s performance.
Alright, ready to dive a bit deeper? Let's peel back the layers on tabular data and figure out what actually makes it tick.
Understanding Tabular Data and Its Challenges
Before you learn about the importance of Accuracy and Reliability in Tabular data models, let’s understand tabular data and its challenges first:-
Definition and Characteristics of Tabular Data within the Context of Machine Learning
Tabular data means a standardized layout of data that is organized into rows and columns, looking like a table in a spreadsheet. Each row in the table depicts an individual record or data point, and each column depicts an exact characteristic or attribute of the data. In the machine learning context, tabular data is a familiar data layout utilized to train models, giving a clear and organized way to input characteristics into algorithms.
Let’s now take a look at the characteristics of tabular data:-
Structured Format: Data is elegantly structured, which often refines the handling of data and pre-processing.
Heterogeneity: Columns can contain various types of data such as numeric, categorical, or datetime, each requiring different preprocessing methods.
Dimensionality: The number of characteristics in the data can differ widely, influencing the intricacy of the model and the arithmetical resources required.
Label Association: In controlled learning, each row often indulges a label or target value, which the model remembers to foretell.
Common Challenges in Tabular Data
Operating with tabular data in machine learning gives numerous challenges that can impact the performance and precision of models:
Noise: Data can include mistakes and irrelevant details, which can deceive the process of training and deteriorate the performance of a model. Noise may derive from measurement mistakes, data gathering problems, or during processing of data. For example, if you’re operating with sensor data from a manufacturing process, occasional faulty sensor readings can acquire noise, resulting in inaccurate forecasts about machine maintenance requirements.
Mislabeling: Precise labels are important for model training in supervised learning. Mislabelled data can lead to inaccurate learning and prophecies, substantially affecting the efficacy of the model. This can happen due to human mistakes in labeling of data or mistakes in automated labeling systems. For instance, if you’re instructing a model to determine types of fruits from images and some apples are erroneously labeled as oranges, the model will struggle to grasp the correct differences.
Missing Values: It’s familiar for datasets to have missing entries for some characteristics. Handling missing data needs careful approach because inappropriate handling can lead to partial or inappropriate models. Methods to handle missing data include attribution, where missing values are filled based on other data, or utilizing models that can manage missing values fundamentally. For example, if you’re inspecting patient records and some entries lack age data, you might use the median age of the dataset to fill in the gaps.
Imbalanced Data: Often, the distribution of target labels or classes in a dataset is imbalanced, which can lead to models that are biased towards the majority class. For instance, if you’re developing a fraud detection system and only 1% of your transactions are deceptive, your model might gradually become overly partial towards forecasts of non-deceptive transactions, missing many actual deceptive cases.
Correlation and Collinearity: High correlation or collinearity between features can cause issues for some models, particularly linear models, leading to inadequate performance or high variability in their predictions. It is important to discover and handle correlated characteristics for structuring healthy models. For instance, if you have two attributes in your dataset that both replicates a person’s name but in distinct units (e.g., monthly salary and annual salary), their high resemblance could veer the model’s outcomes.
Comprehending and acknowledging these challenges is crucial for efficient machine learning with tabular data, compelling extensive data preprocessing, experimental data analysis, and planned model choice.
Now that you've got the lay of the land with tabular data, let's see how it impacts data quality on model performance.
Impact on Data Quality on Model Performance
Now that you have understood Tabular data and its challenges in detail, let’s now take a look at the impact on data quality and model performance:-
The Relationship Between Data Cleanliness and the Accuracy of Models
Understanding Data Cleanliness
Data cleanliness refers to the preciseness, entirety, and correspondence of data. Clean data is mandatory for any machine learning model because the output of the model is directly influenced by its input quality. The old saying “garbage in, garbage out” completely captures this reliability, highlighting that no method, no matter how progressed, can conquer the basic flaws in the data it processes.
Impact on Model Accuracy
Clean data ensures that the model studies the right pattern and not for the noise. For instance, in a conjecturing maintenance model for machinery, precise and compatible data about machine operation times and maintenance schedules is important. False data can lead to incorrect foretelling of setbacks, causing irrelevant checks, or missed maintenance, eventually consuming time and resources.
Examples Demonstrating How Mislabeled or Noisy Data can Compromise Model Reliability
Case Study: Healthcare Diagnostics
Contemplate a model trained to identify diseases from lab results. If the training data includes conflated samples, the model might catch the inappropriate patterns, leading to wrong positives and negatives. This not only impacts the model’s dependency but also has serious indications for patient care.
Impact of Noisy Data in Financial Forecasting
In a financial situation, noisy data could indulge mistakes in transaction history or mis-classified financial data. A predictive model utilized for forecasting the trend in the market or analyzing risk of credit might then make choices based on defective theories. This could lead to poor investment approaches and inaccurate credit estimates, possibly leading to crucial financial losses.
Addressing Data Quality Issues
Rigorous data cleaning procedures are important to alleviate these problems. Techniques might include:-
Data Validation: Enforcing checks during data entry to avert mistakes and ensure coherence.
Anomaly Detection: Utilizing analytical tools or machine learning to determine exceptions that may specify data quality issues.
Regular Audits: Frequently reviewing the data and the processes that generate it to assure sustained data credibility.
Examples of Data Quality Issues
Duplicate Records: Duplicate entries can veer inspection, resulting in misleading inferences. For example, if customer information is duplicated, it may appear that there are more unique customers than there literally are, impacting marketing plans and inventory management.
Missing Values: Missing information can lead to incomplete analysis. For instance, if significant fields such as "date of purchase" or "customer age” are missing, it becomes complex to segment information and dissect trends precisely.
Inconsistent Data: Inconsistencies in data formats or entries can cause perplexity and mistakes in inspection. For example, if dates are transcribed in distinct formats (MM/DD/YYYY vs. DD/MM/YYYY), it can result in incorrect analysis of timelines or trends.
Feeling geared up? Time to roll up our sleeves and tackle how we can boost that data reliability to the next level.
Strategies for Enhancing Data Reliability
Assuring accuracy and reliability in tabular data models is important for efficient decision-making and reliable forecasting dissections. Below you will learn strategies to acknowledge these challenges, concentrating on determining and correcting misclassified data and executing no-code solutions for cleaning of data and model re-training.
Overview of Techniques for Identifying and Correcting Mislabeled Data
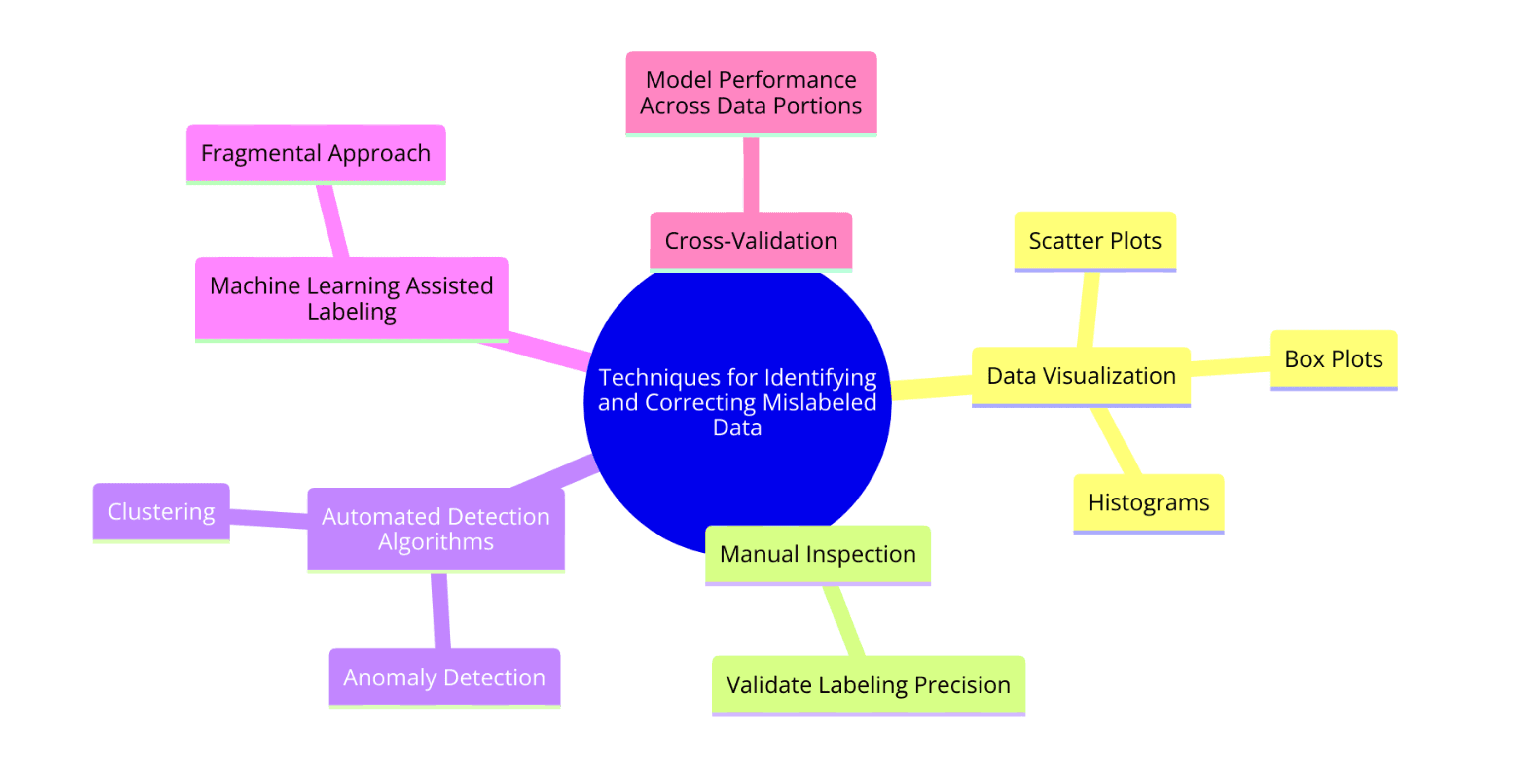
Conflating data can substantially deteriorate the performance of machine learning models. Below given are some techniques to identify and correct such data:
Data Visualization:- Utilize visualization tools to identify exceptions and anomalies that may specify mislabeling. Histograms, scatter plots and box plots can be efficient in identifying inconsistencies.
Manual Inspection: Although time-taking, manually inspecting a random sample of data can help validate labeling precision, especially in crucial areas where errors are expensive.
Automated Detection Algorithms: Execute algorithms like anomaly detection or gathering to locate data points that diverge from the norm, which might be misclassified.
Machine Learning Assisted Labeling: Use a fragmental approach where a little amount of correctly classified data helps to label a wide database.
Cross- Validation: Use a cross-validation approach to ensure that the model's performance is good across distinct portions of data, which can help identify labeling mistakes indirectly by pointing data subsets where the model performs inadequately.
Implementing No-Code Solutions and Tools for Data Cleaning and Retraining Models
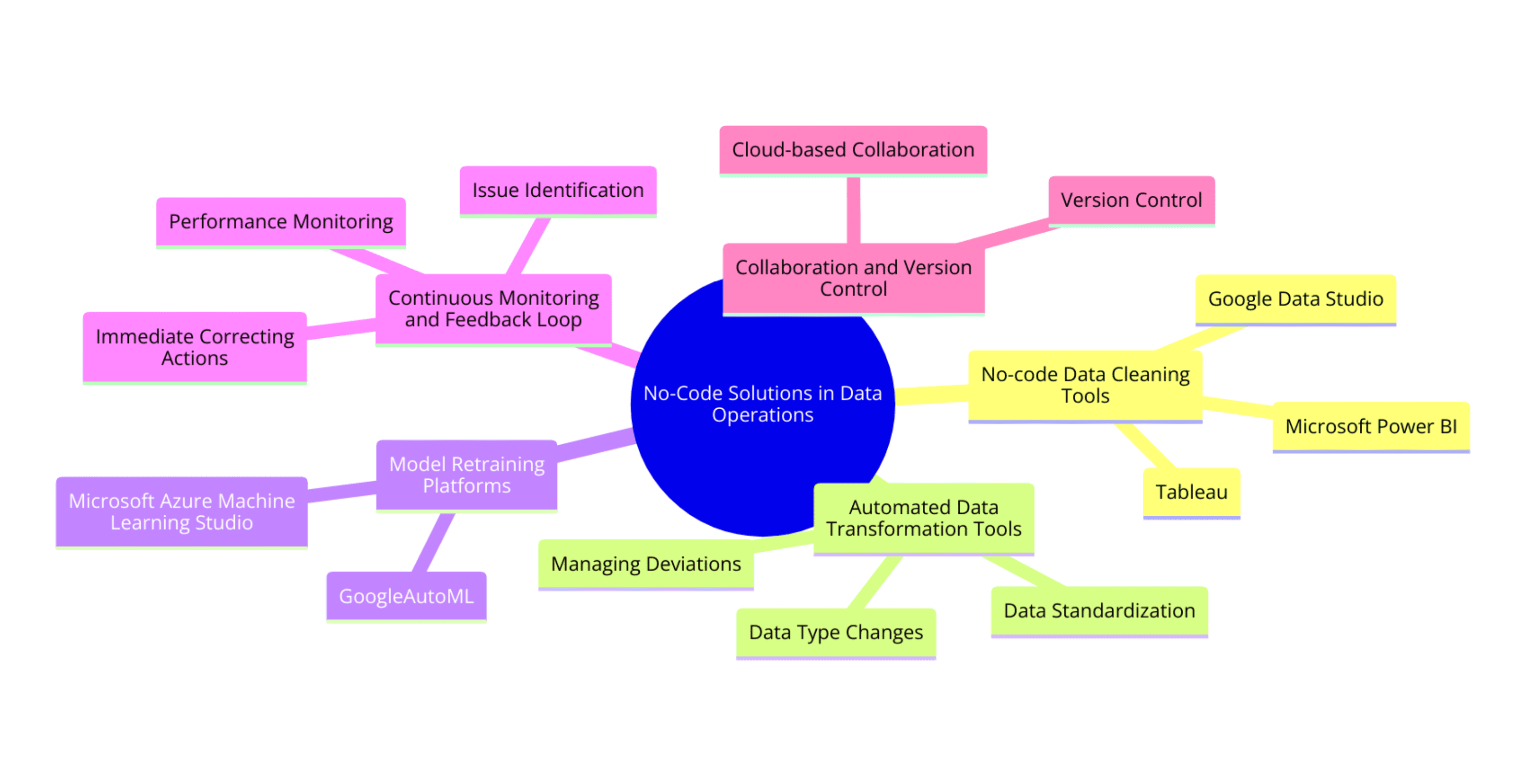
No-code tools have normalized access to intricate data operations, permitting non-specialists to perform the cleaning of data and model training. Below given are some strategies involving no-code solutions:-
No-code Data Cleaning Tools: There are many platforms which help in cleaning data like Microsoft Power BI, Google Data Studio and Tableau permits to clean data without programming. They give instinctive interfaces for abolishing copies, managing missing values, and applying restricted configuration.
Automated Data Transformation Tools: Tools like Trifacta and Alteryx instinctively detect and suggest transformations for data standardization, changing of data types, and managing deviations.
Model Retraining Platforms: No-code platforms such as GoogleAutoML and Microsoft Azure’s Machine Learning Studio offers user-friendly interfaces for training, assessing and retraining models. They often indulge characteristics for automatic data tearing, feature gauging and algorithm selection.
Continuous Monitoring and Feedback Loop: Implement systems that continuously monitor the performance of deployed models and identify potential issues with data quality and model accuracy. No-code platforms like DataRobot and H2O.ai progressively provide these characteristics, permitting immediate correcting actions.
Collaboration and Version Control: Use cloud based no-code platforms such as Airtable and Notion that accelerates partnership among team members and maintain version control of set of information and models, ensuring compatibility and detectability of modifications.
By incorporating these strategies, associations can improve the dependency of their data and the precision of their tabular data models, resulting in better venture insights and decisions.
Got everything sorted so far? Cool, let's pivot to figuring out how noise affects our data and what we can do about it.
Evaluating Model Accuracy in the Presence of Noisy Data
Now that you have know what strategies to implement to enhance data the reliability of data, let’s now move on to learning evaluating model accuracy in the presence of noisy data:
Methods for Training Models on Noisy Labeled Data and Assessing Impact on Performance
Robust Loss Functions: Loss functions that are least unresponsive to deviation and misclassified data can be helpful. Instances include the Huber loss or log-cosh loss, which unified the best properties of MSE and MAE but are less impacted by anomalies in data.
Data Cleaning: Determining and correcting mis-classifications can enhance the model accuracy before training. Approaches like manual examining, utilizing unsupervised learning to find deviation, or engaging anomaly detection, algorithms are familiar.
Regularization Techniques: Techniques such as dropout, L1/L2 regularization or early ceasing can avert the model from overfitting to the noise in the data.
Noise Robust Methods: Bootstrapping and ensemble techniques can help reduce variance and improve a model's resilience against noise.
Noise-Adaptation Layers: Integrating layers or methods that adjust to the noise level in the training data can help by vigorously adapting the training process based on the discovered noise level.
Case Studies on the Importance of Using Out-of-Sample Predictions for Finding Label Issues
Out-of-Sample Testing: Utilizing a split verification set not seen during training helps in comprehending how the model performs on new, hidden data. This can emphasize potent problems with overfitting on noisy training data.
Cross Validation: Using cross-validation approaches can give a more sturdy evaluation of performance of the model and help discover inconsistencies in the labeling of data.
Real-World Examples: Case studies often involve outlines where inaugural models trained on noisy data performed poorly when positioned in real-world environments. Re-evaluating the model with out-of-sample forecasting often leads to finding significant labeling errors.
Continuous Monitoring: Continuous monitoring model predictions against new incoming data can also help in discovering label imprecision. If forecasting constantly deviates from awaiting outcomes, it may specify problems with the training labels.
Feedback Loops: Demonstrating a feedback mechanism where forecasts are constantly retrospected and verified can help enhance the quality of labels over time. This is specifically common in fields like medical imaging or speech identification.
Integrating these techniques can substantially improve the strength and precision of models trained on noisy data. Each technique helps alleviate distinct aspects of the noise challenge and can be customized based on the particular features of the dataset and the noise present.
Feeling like a data detective yet? There's always more to discover. Here's where you can dig even deeper.
Further Research and Tools on Data Quality Improvement
List of Resources for Deepening Knowledge on Data Quality Improvement
Books: Acknowledge titles like “The Data Warehouse Toolkit” by Ralph Kimball or "Data Quality: The Accuracy Dimension" by Jack E. Olson.
Online Courses: There are many online platforms providing knowledge on Data Quality Improvement through their courses such as Coursera, Udemy and Linkedin. These platforms offer courses on data management, data quality and data governance.
Research Papers and Journals: Discover publications in journals such as the Journal of Data and Information Quality, which prospects into the latest inspection on data quality methods.
Encouragement to Participate in the Development and Use of Data Cleaning Tool
Get Involved: Immerse with open source projects on platforms such as GitHub to contribute to the growth of data cleaning tools.
Utilize Tools: Acquaint yourself with the data cleaning tools such as OpenRefine, Talend, and Data Ladder to comprehend their characteristics and applications in real-world scenarios.
Community Participation: Join pertinent online forums and discussion groups, like those on Stack Overflow or special data administration forums, to exchange thoughts and get support from the community.
Phew, that was a lot, wasn't it? Before we wrap up, let's recap why all this fuss about data quality is worth it.
Conclusion
To conclude the article, the significance of accuracy and reliability in tabular data models cannot be exaggerated. These models serve as a substratum for crucial decision-making procedures in several industries, prompting everything from planned venture decisions and to scientific research outcomes.
Ensuring that these models are both precise and reliable assures that the perception originated is responsible, which in turn improves the efficacy of decisions made based on this data. This dedication to accuracy not only secures against costly mistakes but also accelerates the confidence in data-driven planning, eventually contributing to enhanced results and inventiveness.
As data constantly evolves to grow in volume and intricacy, the concentration on maintaining the incorporation of tabular data models becomes even more critical, emphasizing the need for robust techniques and tools that affirms these necessary standards.
Ready to boost your AI models? Sign Up at RagaAI now to explore the globe’s first automated platform designed to perfect your AI applications.
Subscribe to our newsletter to never miss an update
Other articles


Agents Talk, Models Think: A2A + MCP for Enterprise Agentic AI

Riya Parikh, Nitai Agarwal
Apr 27, 2025
Read the article
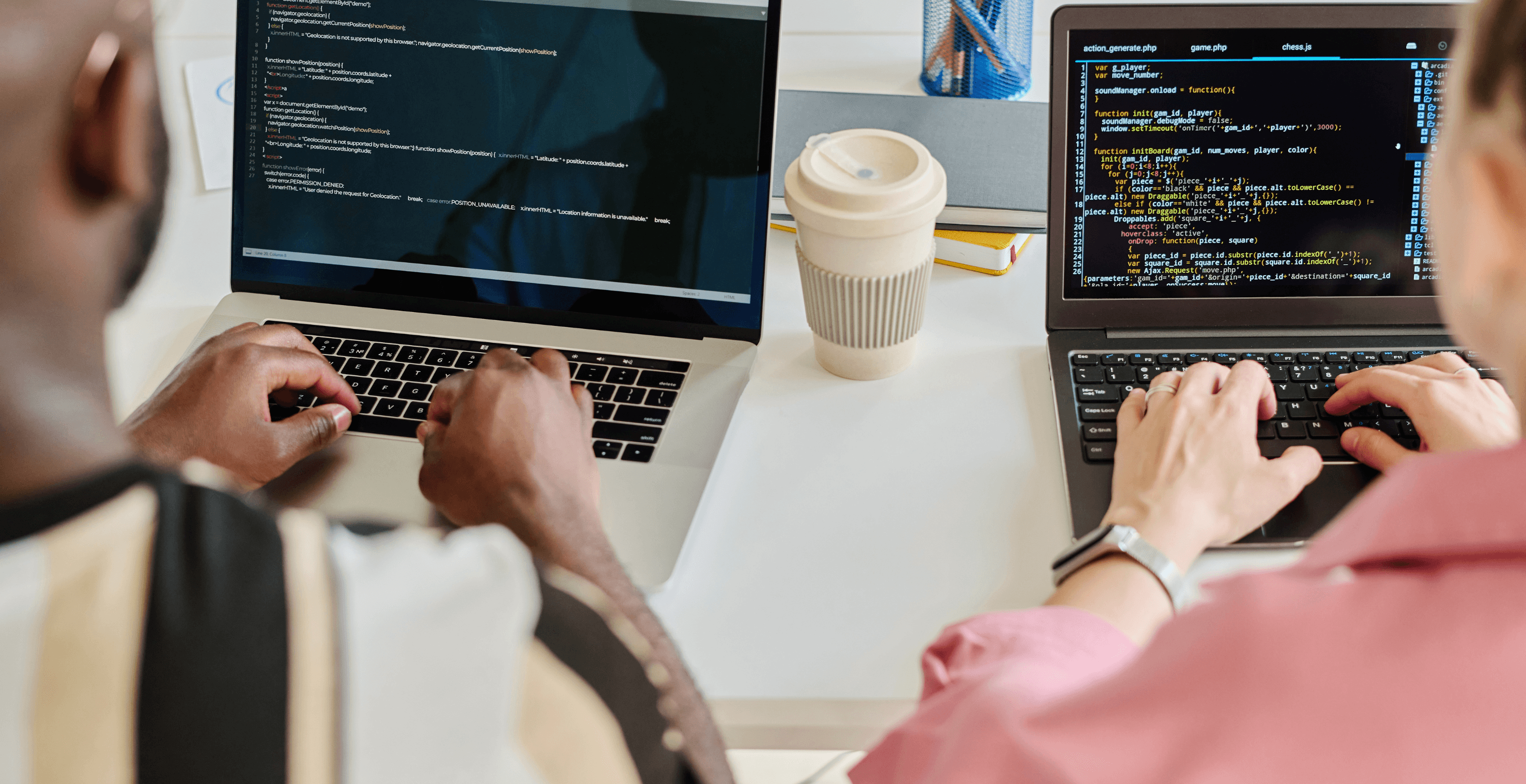
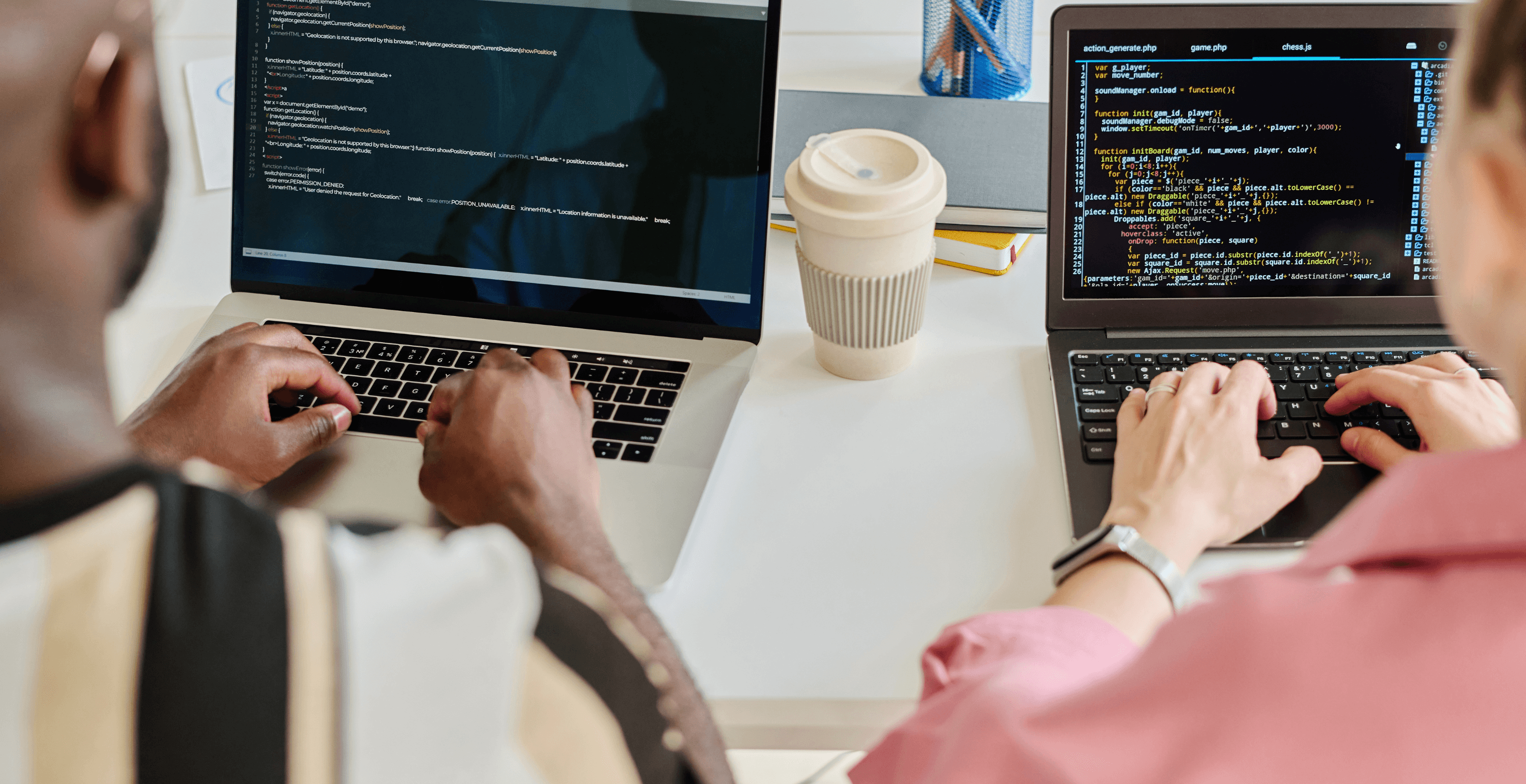
Understanding NeMo Guardrails: A Toolkit for LLM Security

Rehan Asif
Dec 24, 2024
Read the article


Understanding Differences in Large vs Small Language Models (LLM vs SLM)

Rehan Asif
Dec 21, 2024
Read the article