Understanding the Use of Guardrail Metrics in Ensuring LLM Safety
Understanding the Use of Guardrail Metrics in Ensuring LLM Safety
Understanding the Use of Guardrail Metrics in Ensuring LLM Safety
Rehan Asif
Jul 13, 2024
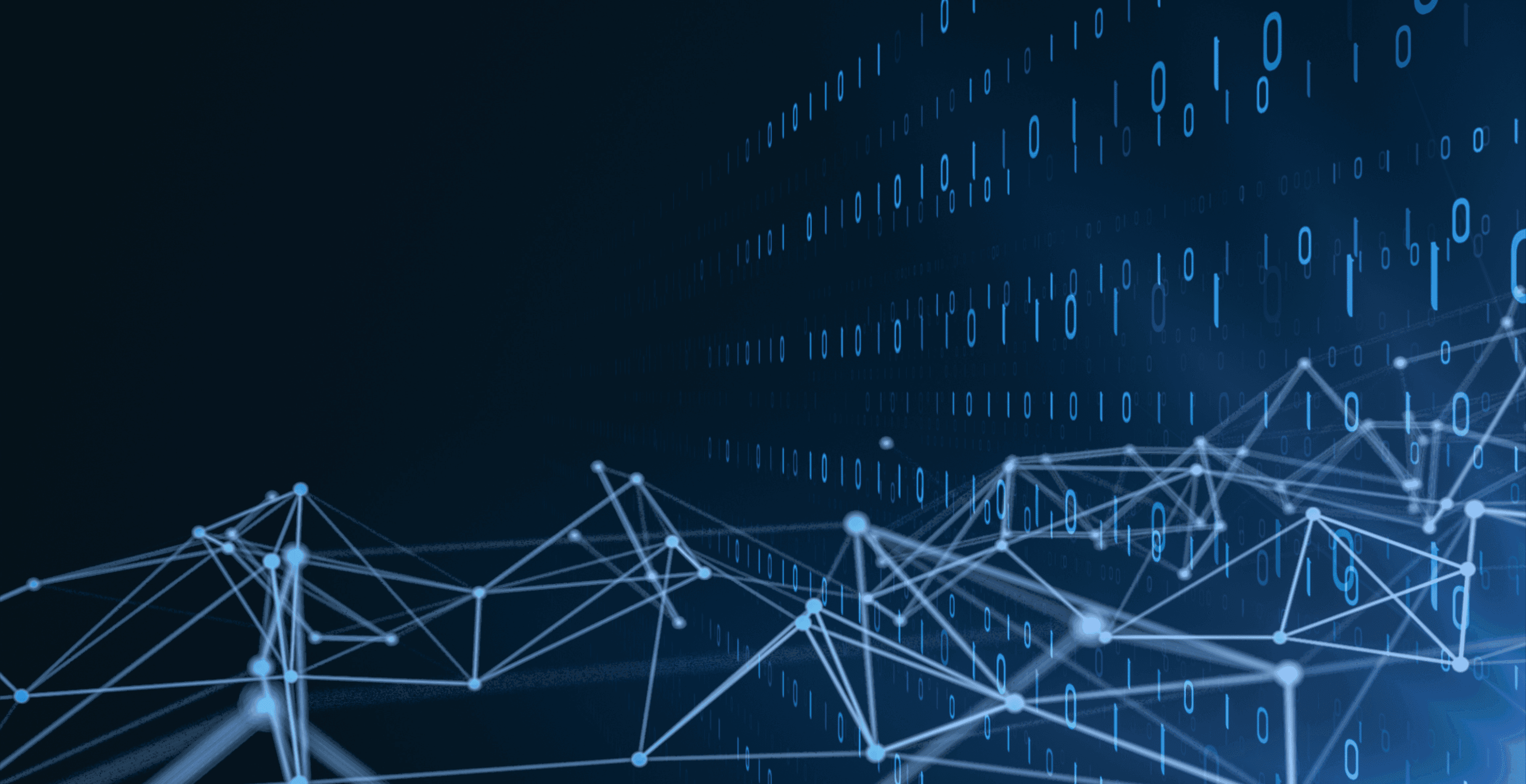
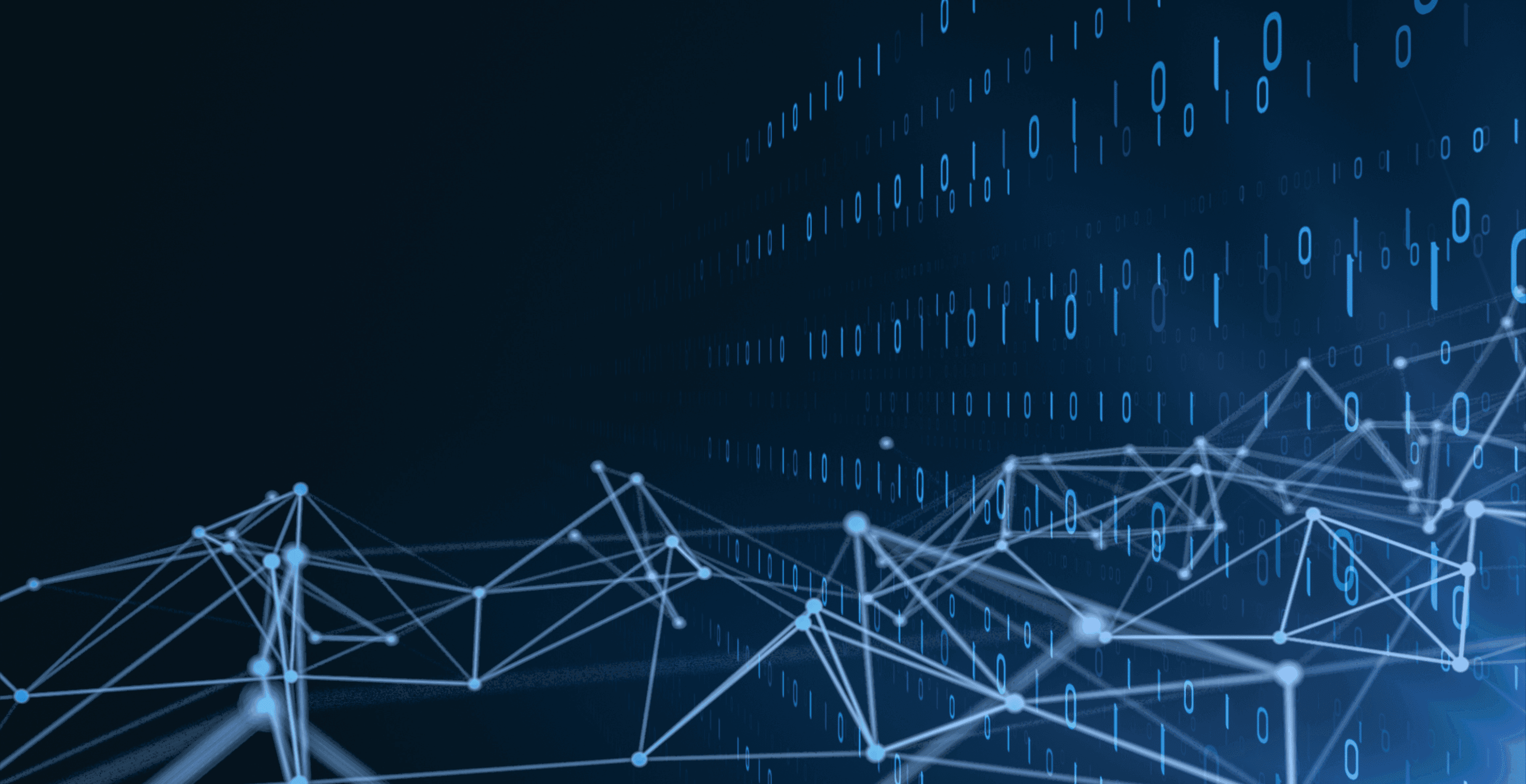
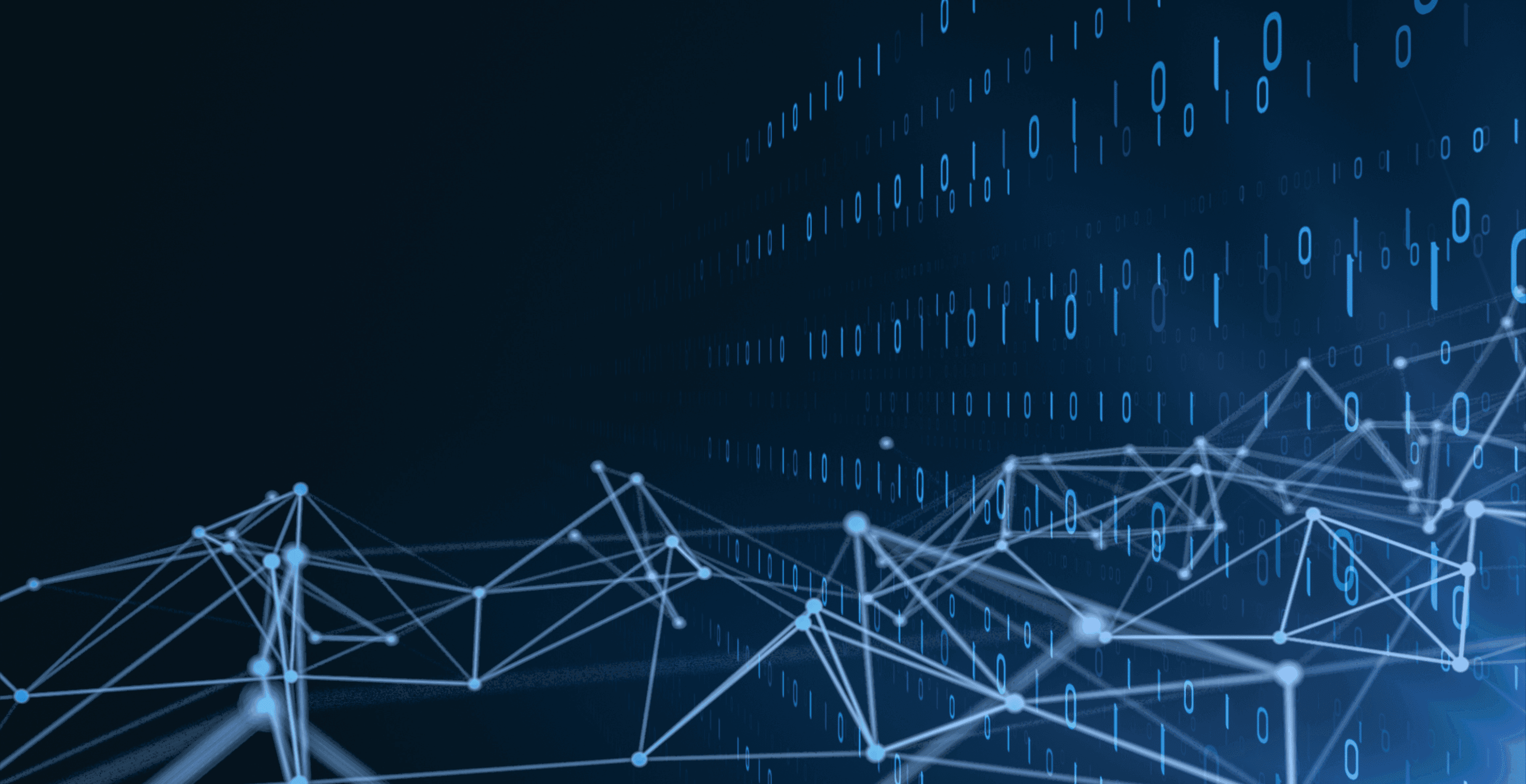
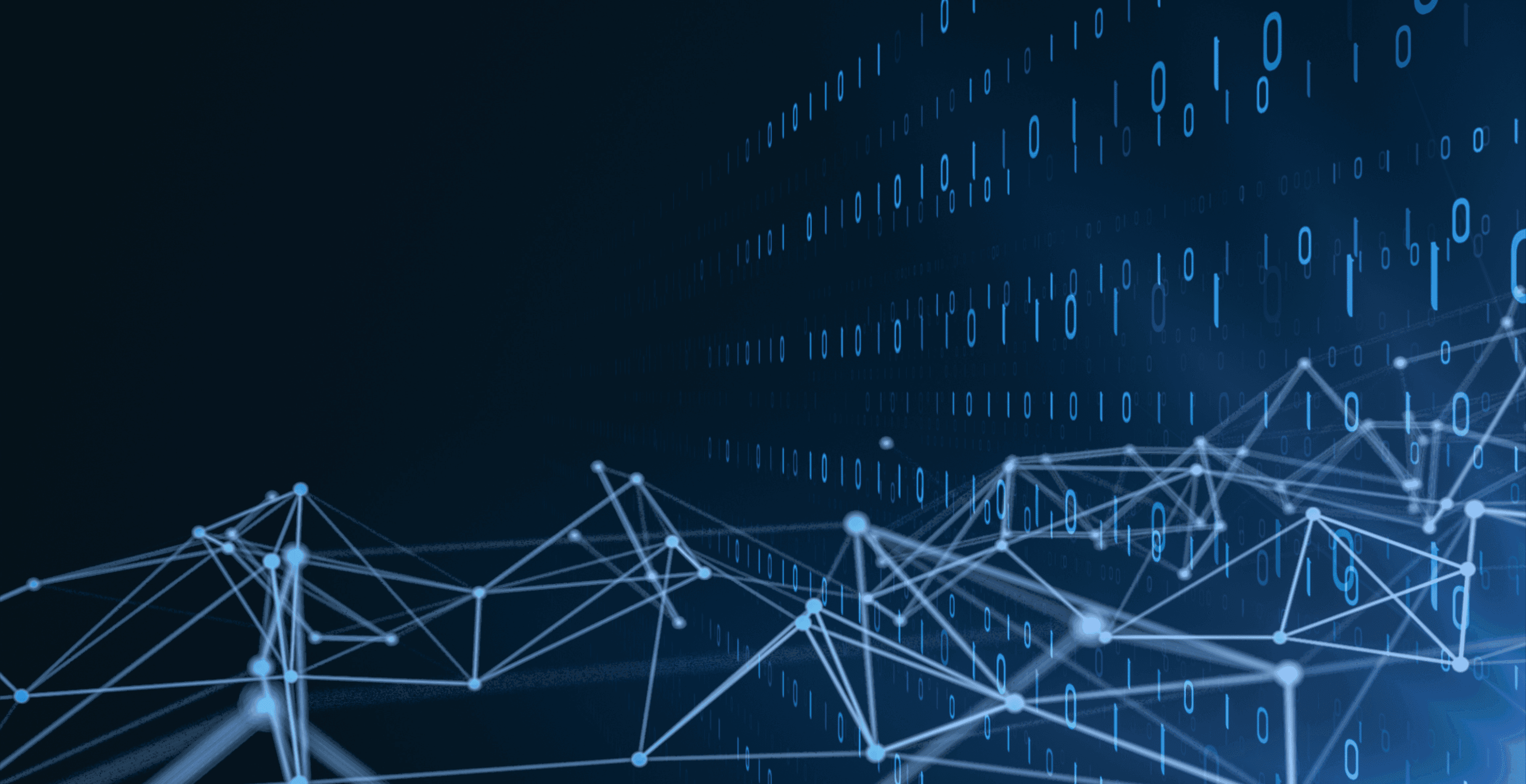
In the swiftly developing field of Artificial Intelligence, ensuring the safety and quality of large language models (LLMs) is paramount. Guardrail metrics, especially customized to generative AI applications, play a critical role in this attempt. You designed these metrics to avert negative alterations and probable harms, not just to serve as another set of information points.
Unlike traditional metrics used in venture or product development, which concentrate on performance and magnification, guardrail metrics for LLMs are about security and quality. They help us ensure that LLMs produce precise and apt yields, minimizing the threat of unintentional outcomes.
Explore our comprehensive article on Building and Implementing Custom LLM Guardrails to better understand the practical aspects of securing AI models.
The Role of Guardrail Metrics in LLM Safety
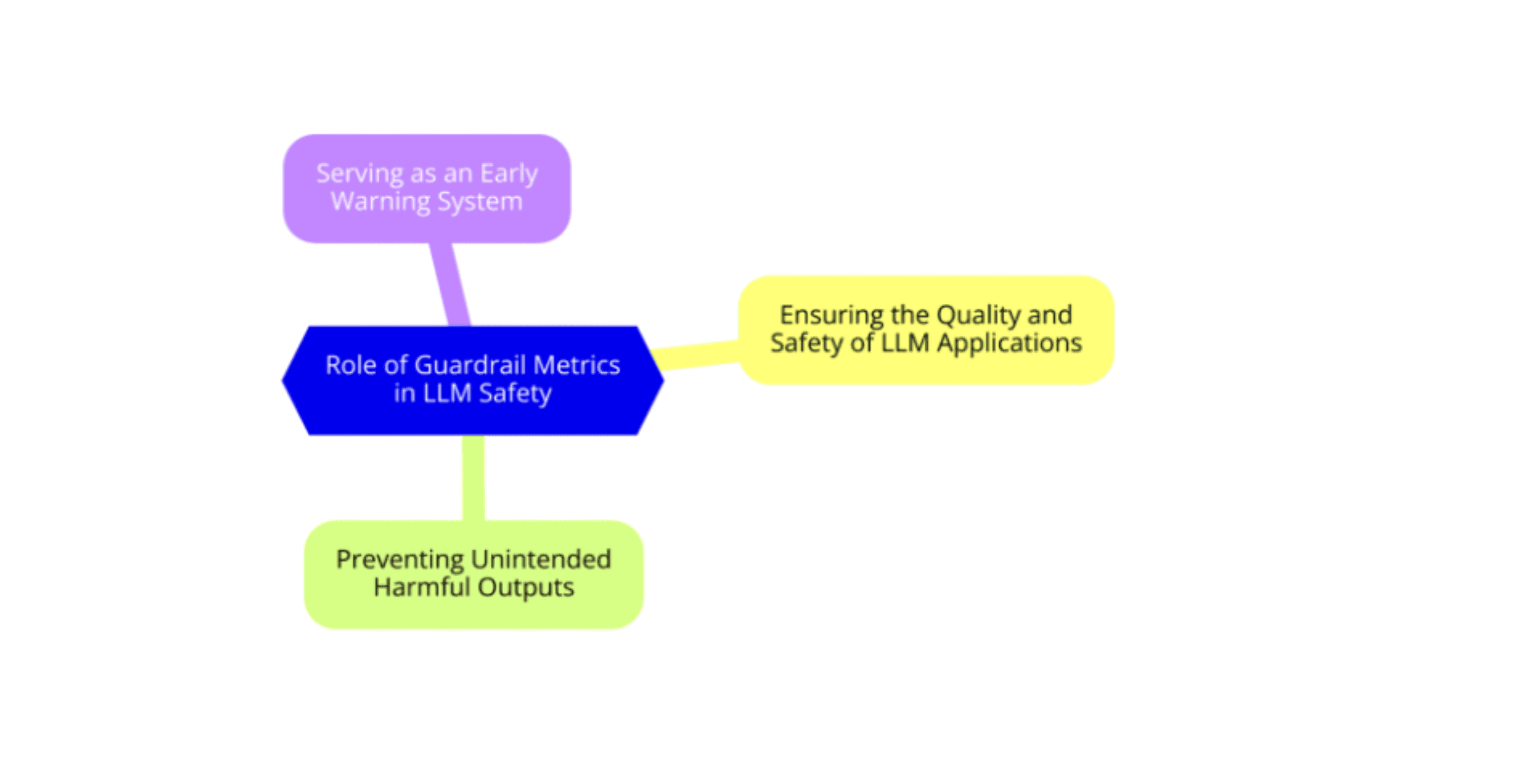
Guardrail metrics are critical in ensuring the safety and dependability of large language models (LLMs). They act as essential checkpoints that help sustain and observe the performance of these sophisticated systems, ensuring they operate as planned.
Ensuring the Quality and Safety of LLM Applications
You want your LLM applications to deliver high-quality and secure communications, right? Guardrail metrics are your ideal tools for this. By constantly monitoring and assessing the yields of your LLM, these metrics ensure that your model follows the set quality standards, giving precise and safe answers. They help you catch any peculiarity or divergence from anticipated behavior, keeping your application dependable and trustworthy.
Preventing Unintended Harmful Outputs
Envision an LLM producing improper and detrimental content during user interactions. That’s a torment synopsis you want to avoid. Guardrail metrics come to the redemption by closely monitoring precise aspects of the LLMs interactions. They help you determine and avert unintended dangerous yields, ensuring that your LLM remains a helpful and safe tool for users.
Serving as an Early Warning System
None like surprises, specifically when it comes to the performance of intricate models. Guardrail metrics serve as an early cautioning system, notifying you of probable problems before they become significant problems. By discerning peculiarities and irregularities, you can acknowledge and resolve them rapidly, sustaining the overall health and functionality of your LLM.
So, now that we understand the critical nature of guardrail metrics let's dive into the various types available for LLMs.
For more comprehensive insights into assessing LLM applications and enforcing guardrails using Raga-LLM-Hub, check out our guide: A Guide to Evaluating LLM Applications and Enabling Guardrails Using Raga-LLM-Hub.
Types of Guardrail Metrics for LLMs
In the Large Language Models (LLMs) scenario, guardrail metrics are your essential tools for sustaining model incorporation and safety. These metrics include sustaining yield quality, securing input safety, and supporting context adherence. Output quality metrics such as BLEU and ROUGE ensure your model’s answers are precise and pertinent. RAG quality metrics concentrate on sustaining the context, providing the model’s yields are coherent and harmonious with the given input. In addition, input quality metrics help you refine detrimental and inappropriate content, securing the communication environment. These guardrail metrics form a sturdy structure to ensure your LLMs deliver safe, dependable, and high-quality performance.
Introduction to Guardrail Metrics for LLM Safety
Ensuring the safety of Large Language Models (LLMs) is critical. Guardrail metrics are your security, ensuring these models perform dependably and ethically. These metrics come in numerous forms, including quality and behavior metrics, customized to keep LLMs in check. Let’s explore these guardrail metrics in more detail.
Output Quality Metrics: BLEU and ROUGE
Regarding output quality, you depend on metrics such as BLEU (Bilingual Evaluation Understudy) and ROUGE (Recall-Oriented Understudy for Gisting Evaluation). BLEU assesses how closely your model’s yield matches a set of reference translations, ensuring content precision. Conversely, ROUGE gauges the overlap of n-grams, word series, and word pairs between the procured and reference texts, concentrating on pertinence. These metrics help you measure whether the content produced by your LLM is precise and pertinent, giving transparent suspicions of the model’s performance.
RAG Quality Metrics
RAG (Retrieval-Augmented Generation) quality metrics are essential for sustaining context adherence. These metrics ensure that your model recovers pertinent data and produces answers that are accurate to the given context. By observing how well your LLM follows the input context, RAG metrics help you avert off-topic or absurd answers, thereby improving the model’s dependability.
Input Quality Metrics: Safety Measures
Ensuring the quality of inputs is just as significant as assessing yields. Input quality metrics concentrate on discerning and alleviating hazardous or inappropriate inputs. You can enforce safety assessments like content filters and pathogenic detectors to screen inputs before they reach the LLM. These metrics help you sustain a safe and regardful interaction environment, protecting users from detrimental content and ensuring the ethical use of LLMs.
Protecting Personal Identifiable Information (PII)
When dealing with LLMs, ensuring the ammunition of Personal Identifiable Information (PII) is paramount. Suppose your AI accidentally exposing someone’s social security number or address. Not cool, right?
To avoid this, you can enforce PII Detection Metrics. These metrics scan outputs for any sensitive data and ensure it’s censored or not produced in the first place. It’s like having a vigilant security guard that questions anything suspicious.
Tackling Bias in AI
Bias in AI can be an enormous problem. Think about it: if your LLM constantly generates biased outcomes, it could commemorate stereotypes or unfair treatment. This is where bias detection metrics comes into play.
These metrics assess the outputs for any unfair bias based on race, gender, age, or other attributes. By flagging and ackowedging biased answers, you ensure your AI remains impartial and balanced. It’s like giving your AI a moral compass to check tricky legitimate ethical terrains.
Reducing Toxicity
No one likes communicating with a toxic AI. Whether it’s spitting out detrimental language or nurturing negative behavior, toxicity in LLMs can lead to serious reputational detriment. Toxicity detection metrics help you observe and minimize detrimental language.
These metrics can determine the language produced and flag anything that’s insulting, hazardous, or otherwise inappropriate. Think of it as having a filter that cleanses your AI’s language before it reaches the user.
By comprehending and enforcing these guardrail metrics, you can ensure that your LLMs function safely and efficiently, providing precise and contextually pertinent yields while securing against detrimental inputs.
Are you curious about how to implement guardrail metrics in your generative AI applications? Let's walk through the necessary steps next.
Check out our guide on Evaluating Large Language Models: Methods And Metrics to investigate ways we gauge AI.
Implementing Guardrail Metrics in Generative AI
Implementing guardrail metrics in generative AI is critical for ensuring security and quality in your Large Language Models (LLMs). Creating and tracing these metrics can avert probable problems and sustain control over your AI applications. Here’s how you can get started.
Creating and Tracking Guardrail Metrics Specific to LLM Use Cases
First, determine the precise use cases for your LLMs. Each application might need to observe distinct guardrail metrics. For example, if your LLM is used in a customer service chatbot, you can trace metrics such as response precision, user contentment, and conversation culmination rates. Customizing these metrics to your use cases ensures that the guardrails are pertinent and efficient.
Next, pioneer an inception for these metrics. This indulges gathering initial information to comprehend the current performance of your LLMs. With this inception, you can set sensible targets and verges that depict adequate performance levels. Frequently trace these metrics to identify trends and anomalies, which can specify probable problems before they expand.
Managing and Monitoring Metrics with Automation
Use automated systems and tools to manage and observe your guardrail metrics effectively. Automated systems not only save time but also accelerate observation precision. Tools such as AI dashboards, metric tracking software, and anomaly detection systems can offer real-time perceptions of your LLMs' performance.
For instance, an automated dashboard can exhibit your key metrics at a glimpse, permitting you to gauge the health of your LLM rapidly. In addition, these tools can send warnings when metrics diverge from the pioneered verges, enabling you to take expeditious action to acknowledge any problems. Transforming to automated systems helps sleek the observation process and ensures you stay on top of your LLM performance.
Incorporating Industry Metrics with In-House ML Research
Incorporating industry-standard metrics is another crucial step in enforcing guardrails. These metrics are extensively determined and provide a standard for assessing your LLMs' performance. Instances include precision, recall, F1 score, and user engagement rates. Using these structured metrics, you can contrast your LLMs' performance against industry norms and ensure you meet pioneered standards.
Alongside these industry-standard metrics, integrate discoveries from your in-house machine learning research. Your team’s unique insights can result in the expansion of auxiliary metrics customized to your precise needs. Merging industry standards and in-house investigation ensures a thorough approach to observing your LLMs.
Now that we've covered how to implement guardrail metrics let's address the value of custom guardrail metrics tailored specifically to your needs.
Custom Guardrail Metrics for LLMs
While standard metrics are essential, adaptability in adding tailored metrics can substantially improve your LLMs' safety and quality control. These custom metrics can acknowledge distinct challenges and must be precise to your applications.
Adding Custom Metrics for Specific LLM Application Needs
Custom guardrail metrics provide the adaptability to acknowledge the precise needs of your LLM applications. For instance, if your LLM produces content for a sensitive domain such as healthcare, you might create metrics to trace medical precision and docility with regulations. Custom metrics permit you to customize the observation process to fit the nuances of your LLM use cases, ensuring more tormented and efficient guardrails.
Examples of Custom Metrics for Enhanced Safety and Quality Control
To improve safety and quality control, contemplate the following examples of custom metrics:
Contextual Accuracy: Assesses how precisely the LLM comprehends and responds within the context of a conversation or document.
Bias Detection: Observe the LLM for any signs of bias in its yields, ensuring neutrality and objectivity.
User Feedback and Integration: The LLM tracks user feedback and integrates it into metric evaluations to continuously enhance its performance.
Ethical Compliance: Ensures the LLMs yield with ethical guidelines and industry standards.
By enforcing these custom metrics, you create a sturdy structure that acknowledges the precise needs of your LLM applications. Transforming from standard to custom metrics permits more accurate and pertinent observation, improving your generative AI systems' overall safety and quality.
With all the information in hand, let's wrap up with a few final thoughts on the importance and future of guardrail metrics.
For comprehensive steps for setting up and managing Large Language Models in real-globe synopsis, check out our Practical Guide For Deploying LLMs In Production.
Conclusion
To conclude the article, guardrail metrics play a critical role in maintaining the security and standard of LLM applications. They act as an attentive observing system, catching probable problems before they expand into substantial issues. Integrating a balanced and pragmatic approach to these metrics ensures that your LLM functions securely and efficiently, giving dependable and valuable interactions.
Guardrail metrics are not just averting harm; they are about building certainty and dependability in the Artificial Intelligence systems we rely on. As we persist in pioneering and developing more sophisticated LLMs, these metrics will remain a backbone of AI security, accompanying us towards safer and more reliable AI applications.
Explore the future of AI-driven perceptions and automation. Join RagaAI today to unleash unparalleled effectiveness and innovation in your functioning. Don’t miss out–register now and lead your industry with cutting-edge technology.
In the swiftly developing field of Artificial Intelligence, ensuring the safety and quality of large language models (LLMs) is paramount. Guardrail metrics, especially customized to generative AI applications, play a critical role in this attempt. You designed these metrics to avert negative alterations and probable harms, not just to serve as another set of information points.
Unlike traditional metrics used in venture or product development, which concentrate on performance and magnification, guardrail metrics for LLMs are about security and quality. They help us ensure that LLMs produce precise and apt yields, minimizing the threat of unintentional outcomes.
Explore our comprehensive article on Building and Implementing Custom LLM Guardrails to better understand the practical aspects of securing AI models.
The Role of Guardrail Metrics in LLM Safety
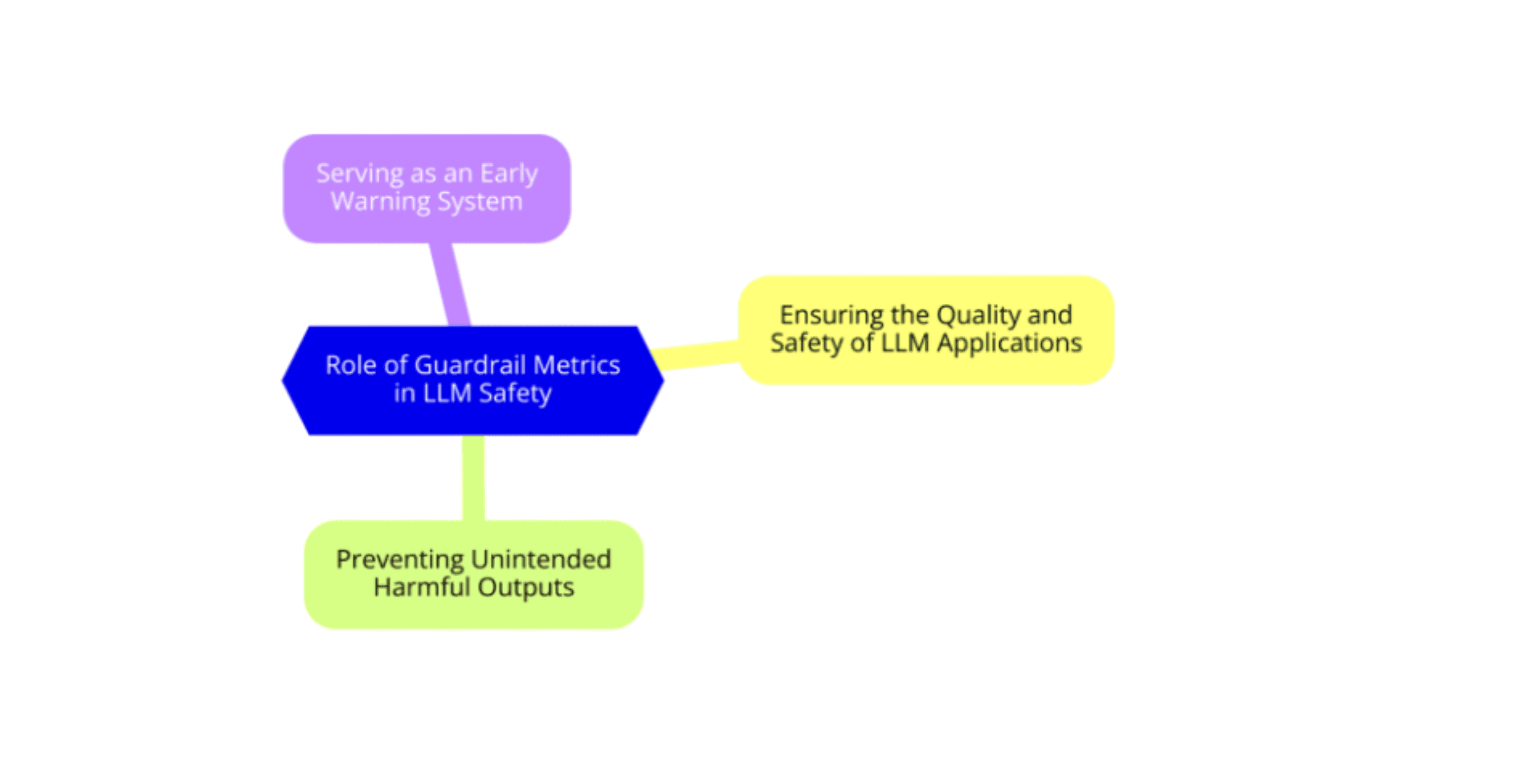
Guardrail metrics are critical in ensuring the safety and dependability of large language models (LLMs). They act as essential checkpoints that help sustain and observe the performance of these sophisticated systems, ensuring they operate as planned.
Ensuring the Quality and Safety of LLM Applications
You want your LLM applications to deliver high-quality and secure communications, right? Guardrail metrics are your ideal tools for this. By constantly monitoring and assessing the yields of your LLM, these metrics ensure that your model follows the set quality standards, giving precise and safe answers. They help you catch any peculiarity or divergence from anticipated behavior, keeping your application dependable and trustworthy.
Preventing Unintended Harmful Outputs
Envision an LLM producing improper and detrimental content during user interactions. That’s a torment synopsis you want to avoid. Guardrail metrics come to the redemption by closely monitoring precise aspects of the LLMs interactions. They help you determine and avert unintended dangerous yields, ensuring that your LLM remains a helpful and safe tool for users.
Serving as an Early Warning System
None like surprises, specifically when it comes to the performance of intricate models. Guardrail metrics serve as an early cautioning system, notifying you of probable problems before they become significant problems. By discerning peculiarities and irregularities, you can acknowledge and resolve them rapidly, sustaining the overall health and functionality of your LLM.
So, now that we understand the critical nature of guardrail metrics let's dive into the various types available for LLMs.
For more comprehensive insights into assessing LLM applications and enforcing guardrails using Raga-LLM-Hub, check out our guide: A Guide to Evaluating LLM Applications and Enabling Guardrails Using Raga-LLM-Hub.
Types of Guardrail Metrics for LLMs
In the Large Language Models (LLMs) scenario, guardrail metrics are your essential tools for sustaining model incorporation and safety. These metrics include sustaining yield quality, securing input safety, and supporting context adherence. Output quality metrics such as BLEU and ROUGE ensure your model’s answers are precise and pertinent. RAG quality metrics concentrate on sustaining the context, providing the model’s yields are coherent and harmonious with the given input. In addition, input quality metrics help you refine detrimental and inappropriate content, securing the communication environment. These guardrail metrics form a sturdy structure to ensure your LLMs deliver safe, dependable, and high-quality performance.
Introduction to Guardrail Metrics for LLM Safety
Ensuring the safety of Large Language Models (LLMs) is critical. Guardrail metrics are your security, ensuring these models perform dependably and ethically. These metrics come in numerous forms, including quality and behavior metrics, customized to keep LLMs in check. Let’s explore these guardrail metrics in more detail.
Output Quality Metrics: BLEU and ROUGE
Regarding output quality, you depend on metrics such as BLEU (Bilingual Evaluation Understudy) and ROUGE (Recall-Oriented Understudy for Gisting Evaluation). BLEU assesses how closely your model’s yield matches a set of reference translations, ensuring content precision. Conversely, ROUGE gauges the overlap of n-grams, word series, and word pairs between the procured and reference texts, concentrating on pertinence. These metrics help you measure whether the content produced by your LLM is precise and pertinent, giving transparent suspicions of the model’s performance.
RAG Quality Metrics
RAG (Retrieval-Augmented Generation) quality metrics are essential for sustaining context adherence. These metrics ensure that your model recovers pertinent data and produces answers that are accurate to the given context. By observing how well your LLM follows the input context, RAG metrics help you avert off-topic or absurd answers, thereby improving the model’s dependability.
Input Quality Metrics: Safety Measures
Ensuring the quality of inputs is just as significant as assessing yields. Input quality metrics concentrate on discerning and alleviating hazardous or inappropriate inputs. You can enforce safety assessments like content filters and pathogenic detectors to screen inputs before they reach the LLM. These metrics help you sustain a safe and regardful interaction environment, protecting users from detrimental content and ensuring the ethical use of LLMs.
Protecting Personal Identifiable Information (PII)
When dealing with LLMs, ensuring the ammunition of Personal Identifiable Information (PII) is paramount. Suppose your AI accidentally exposing someone’s social security number or address. Not cool, right?
To avoid this, you can enforce PII Detection Metrics. These metrics scan outputs for any sensitive data and ensure it’s censored or not produced in the first place. It’s like having a vigilant security guard that questions anything suspicious.
Tackling Bias in AI
Bias in AI can be an enormous problem. Think about it: if your LLM constantly generates biased outcomes, it could commemorate stereotypes or unfair treatment. This is where bias detection metrics comes into play.
These metrics assess the outputs for any unfair bias based on race, gender, age, or other attributes. By flagging and ackowedging biased answers, you ensure your AI remains impartial and balanced. It’s like giving your AI a moral compass to check tricky legitimate ethical terrains.
Reducing Toxicity
No one likes communicating with a toxic AI. Whether it’s spitting out detrimental language or nurturing negative behavior, toxicity in LLMs can lead to serious reputational detriment. Toxicity detection metrics help you observe and minimize detrimental language.
These metrics can determine the language produced and flag anything that’s insulting, hazardous, or otherwise inappropriate. Think of it as having a filter that cleanses your AI’s language before it reaches the user.
By comprehending and enforcing these guardrail metrics, you can ensure that your LLMs function safely and efficiently, providing precise and contextually pertinent yields while securing against detrimental inputs.
Are you curious about how to implement guardrail metrics in your generative AI applications? Let's walk through the necessary steps next.
Check out our guide on Evaluating Large Language Models: Methods And Metrics to investigate ways we gauge AI.
Implementing Guardrail Metrics in Generative AI
Implementing guardrail metrics in generative AI is critical for ensuring security and quality in your Large Language Models (LLMs). Creating and tracing these metrics can avert probable problems and sustain control over your AI applications. Here’s how you can get started.
Creating and Tracking Guardrail Metrics Specific to LLM Use Cases
First, determine the precise use cases for your LLMs. Each application might need to observe distinct guardrail metrics. For example, if your LLM is used in a customer service chatbot, you can trace metrics such as response precision, user contentment, and conversation culmination rates. Customizing these metrics to your use cases ensures that the guardrails are pertinent and efficient.
Next, pioneer an inception for these metrics. This indulges gathering initial information to comprehend the current performance of your LLMs. With this inception, you can set sensible targets and verges that depict adequate performance levels. Frequently trace these metrics to identify trends and anomalies, which can specify probable problems before they expand.
Managing and Monitoring Metrics with Automation
Use automated systems and tools to manage and observe your guardrail metrics effectively. Automated systems not only save time but also accelerate observation precision. Tools such as AI dashboards, metric tracking software, and anomaly detection systems can offer real-time perceptions of your LLMs' performance.
For instance, an automated dashboard can exhibit your key metrics at a glimpse, permitting you to gauge the health of your LLM rapidly. In addition, these tools can send warnings when metrics diverge from the pioneered verges, enabling you to take expeditious action to acknowledge any problems. Transforming to automated systems helps sleek the observation process and ensures you stay on top of your LLM performance.
Incorporating Industry Metrics with In-House ML Research
Incorporating industry-standard metrics is another crucial step in enforcing guardrails. These metrics are extensively determined and provide a standard for assessing your LLMs' performance. Instances include precision, recall, F1 score, and user engagement rates. Using these structured metrics, you can contrast your LLMs' performance against industry norms and ensure you meet pioneered standards.
Alongside these industry-standard metrics, integrate discoveries from your in-house machine learning research. Your team’s unique insights can result in the expansion of auxiliary metrics customized to your precise needs. Merging industry standards and in-house investigation ensures a thorough approach to observing your LLMs.
Now that we've covered how to implement guardrail metrics let's address the value of custom guardrail metrics tailored specifically to your needs.
Custom Guardrail Metrics for LLMs
While standard metrics are essential, adaptability in adding tailored metrics can substantially improve your LLMs' safety and quality control. These custom metrics can acknowledge distinct challenges and must be precise to your applications.
Adding Custom Metrics for Specific LLM Application Needs
Custom guardrail metrics provide the adaptability to acknowledge the precise needs of your LLM applications. For instance, if your LLM produces content for a sensitive domain such as healthcare, you might create metrics to trace medical precision and docility with regulations. Custom metrics permit you to customize the observation process to fit the nuances of your LLM use cases, ensuring more tormented and efficient guardrails.
Examples of Custom Metrics for Enhanced Safety and Quality Control
To improve safety and quality control, contemplate the following examples of custom metrics:
Contextual Accuracy: Assesses how precisely the LLM comprehends and responds within the context of a conversation or document.
Bias Detection: Observe the LLM for any signs of bias in its yields, ensuring neutrality and objectivity.
User Feedback and Integration: The LLM tracks user feedback and integrates it into metric evaluations to continuously enhance its performance.
Ethical Compliance: Ensures the LLMs yield with ethical guidelines and industry standards.
By enforcing these custom metrics, you create a sturdy structure that acknowledges the precise needs of your LLM applications. Transforming from standard to custom metrics permits more accurate and pertinent observation, improving your generative AI systems' overall safety and quality.
With all the information in hand, let's wrap up with a few final thoughts on the importance and future of guardrail metrics.
For comprehensive steps for setting up and managing Large Language Models in real-globe synopsis, check out our Practical Guide For Deploying LLMs In Production.
Conclusion
To conclude the article, guardrail metrics play a critical role in maintaining the security and standard of LLM applications. They act as an attentive observing system, catching probable problems before they expand into substantial issues. Integrating a balanced and pragmatic approach to these metrics ensures that your LLM functions securely and efficiently, giving dependable and valuable interactions.
Guardrail metrics are not just averting harm; they are about building certainty and dependability in the Artificial Intelligence systems we rely on. As we persist in pioneering and developing more sophisticated LLMs, these metrics will remain a backbone of AI security, accompanying us towards safer and more reliable AI applications.
Explore the future of AI-driven perceptions and automation. Join RagaAI today to unleash unparalleled effectiveness and innovation in your functioning. Don’t miss out–register now and lead your industry with cutting-edge technology.
In the swiftly developing field of Artificial Intelligence, ensuring the safety and quality of large language models (LLMs) is paramount. Guardrail metrics, especially customized to generative AI applications, play a critical role in this attempt. You designed these metrics to avert negative alterations and probable harms, not just to serve as another set of information points.
Unlike traditional metrics used in venture or product development, which concentrate on performance and magnification, guardrail metrics for LLMs are about security and quality. They help us ensure that LLMs produce precise and apt yields, minimizing the threat of unintentional outcomes.
Explore our comprehensive article on Building and Implementing Custom LLM Guardrails to better understand the practical aspects of securing AI models.
The Role of Guardrail Metrics in LLM Safety
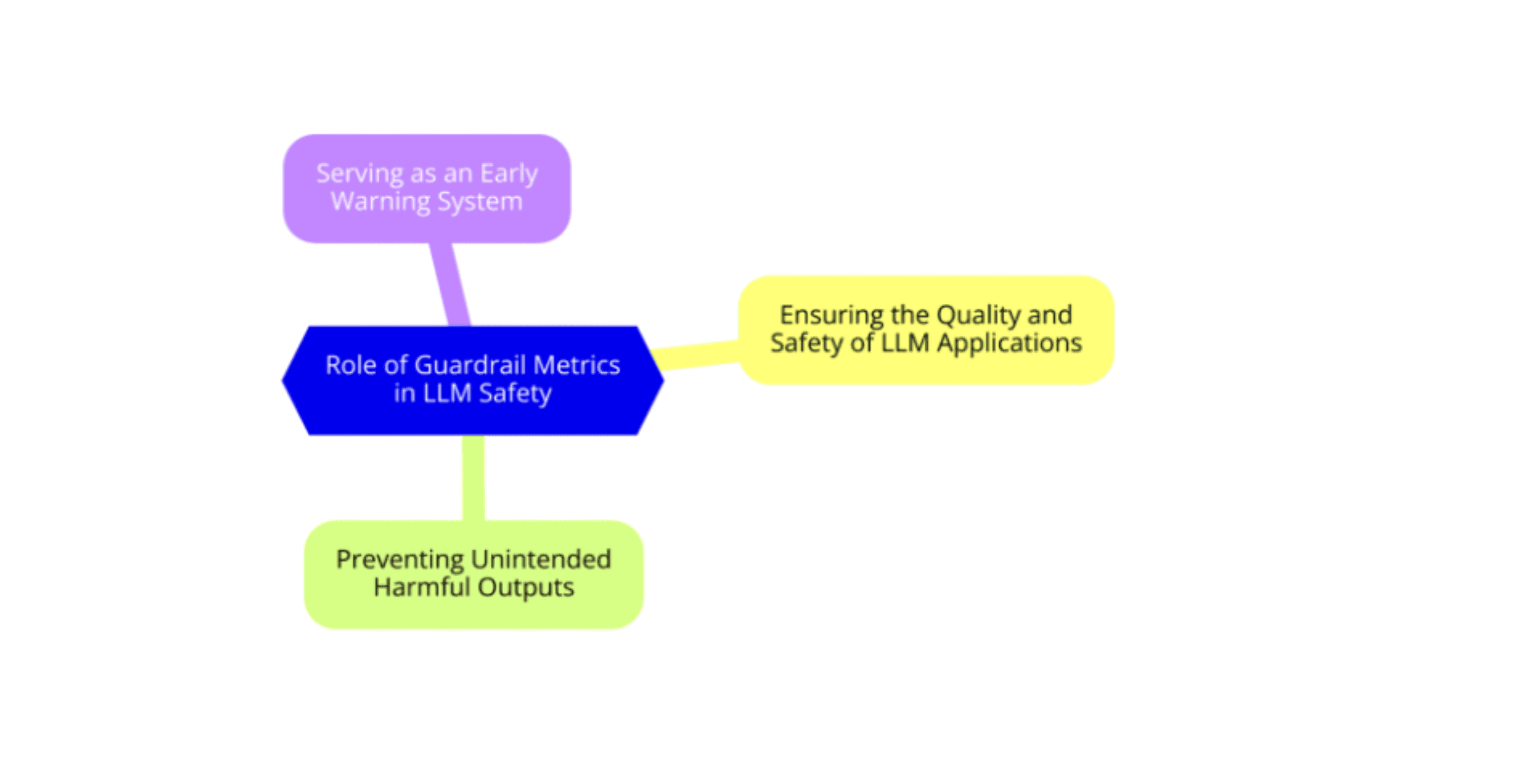
Guardrail metrics are critical in ensuring the safety and dependability of large language models (LLMs). They act as essential checkpoints that help sustain and observe the performance of these sophisticated systems, ensuring they operate as planned.
Ensuring the Quality and Safety of LLM Applications
You want your LLM applications to deliver high-quality and secure communications, right? Guardrail metrics are your ideal tools for this. By constantly monitoring and assessing the yields of your LLM, these metrics ensure that your model follows the set quality standards, giving precise and safe answers. They help you catch any peculiarity or divergence from anticipated behavior, keeping your application dependable and trustworthy.
Preventing Unintended Harmful Outputs
Envision an LLM producing improper and detrimental content during user interactions. That’s a torment synopsis you want to avoid. Guardrail metrics come to the redemption by closely monitoring precise aspects of the LLMs interactions. They help you determine and avert unintended dangerous yields, ensuring that your LLM remains a helpful and safe tool for users.
Serving as an Early Warning System
None like surprises, specifically when it comes to the performance of intricate models. Guardrail metrics serve as an early cautioning system, notifying you of probable problems before they become significant problems. By discerning peculiarities and irregularities, you can acknowledge and resolve them rapidly, sustaining the overall health and functionality of your LLM.
So, now that we understand the critical nature of guardrail metrics let's dive into the various types available for LLMs.
For more comprehensive insights into assessing LLM applications and enforcing guardrails using Raga-LLM-Hub, check out our guide: A Guide to Evaluating LLM Applications and Enabling Guardrails Using Raga-LLM-Hub.
Types of Guardrail Metrics for LLMs
In the Large Language Models (LLMs) scenario, guardrail metrics are your essential tools for sustaining model incorporation and safety. These metrics include sustaining yield quality, securing input safety, and supporting context adherence. Output quality metrics such as BLEU and ROUGE ensure your model’s answers are precise and pertinent. RAG quality metrics concentrate on sustaining the context, providing the model’s yields are coherent and harmonious with the given input. In addition, input quality metrics help you refine detrimental and inappropriate content, securing the communication environment. These guardrail metrics form a sturdy structure to ensure your LLMs deliver safe, dependable, and high-quality performance.
Introduction to Guardrail Metrics for LLM Safety
Ensuring the safety of Large Language Models (LLMs) is critical. Guardrail metrics are your security, ensuring these models perform dependably and ethically. These metrics come in numerous forms, including quality and behavior metrics, customized to keep LLMs in check. Let’s explore these guardrail metrics in more detail.
Output Quality Metrics: BLEU and ROUGE
Regarding output quality, you depend on metrics such as BLEU (Bilingual Evaluation Understudy) and ROUGE (Recall-Oriented Understudy for Gisting Evaluation). BLEU assesses how closely your model’s yield matches a set of reference translations, ensuring content precision. Conversely, ROUGE gauges the overlap of n-grams, word series, and word pairs between the procured and reference texts, concentrating on pertinence. These metrics help you measure whether the content produced by your LLM is precise and pertinent, giving transparent suspicions of the model’s performance.
RAG Quality Metrics
RAG (Retrieval-Augmented Generation) quality metrics are essential for sustaining context adherence. These metrics ensure that your model recovers pertinent data and produces answers that are accurate to the given context. By observing how well your LLM follows the input context, RAG metrics help you avert off-topic or absurd answers, thereby improving the model’s dependability.
Input Quality Metrics: Safety Measures
Ensuring the quality of inputs is just as significant as assessing yields. Input quality metrics concentrate on discerning and alleviating hazardous or inappropriate inputs. You can enforce safety assessments like content filters and pathogenic detectors to screen inputs before they reach the LLM. These metrics help you sustain a safe and regardful interaction environment, protecting users from detrimental content and ensuring the ethical use of LLMs.
Protecting Personal Identifiable Information (PII)
When dealing with LLMs, ensuring the ammunition of Personal Identifiable Information (PII) is paramount. Suppose your AI accidentally exposing someone’s social security number or address. Not cool, right?
To avoid this, you can enforce PII Detection Metrics. These metrics scan outputs for any sensitive data and ensure it’s censored or not produced in the first place. It’s like having a vigilant security guard that questions anything suspicious.
Tackling Bias in AI
Bias in AI can be an enormous problem. Think about it: if your LLM constantly generates biased outcomes, it could commemorate stereotypes or unfair treatment. This is where bias detection metrics comes into play.
These metrics assess the outputs for any unfair bias based on race, gender, age, or other attributes. By flagging and ackowedging biased answers, you ensure your AI remains impartial and balanced. It’s like giving your AI a moral compass to check tricky legitimate ethical terrains.
Reducing Toxicity
No one likes communicating with a toxic AI. Whether it’s spitting out detrimental language or nurturing negative behavior, toxicity in LLMs can lead to serious reputational detriment. Toxicity detection metrics help you observe and minimize detrimental language.
These metrics can determine the language produced and flag anything that’s insulting, hazardous, or otherwise inappropriate. Think of it as having a filter that cleanses your AI’s language before it reaches the user.
By comprehending and enforcing these guardrail metrics, you can ensure that your LLMs function safely and efficiently, providing precise and contextually pertinent yields while securing against detrimental inputs.
Are you curious about how to implement guardrail metrics in your generative AI applications? Let's walk through the necessary steps next.
Check out our guide on Evaluating Large Language Models: Methods And Metrics to investigate ways we gauge AI.
Implementing Guardrail Metrics in Generative AI
Implementing guardrail metrics in generative AI is critical for ensuring security and quality in your Large Language Models (LLMs). Creating and tracing these metrics can avert probable problems and sustain control over your AI applications. Here’s how you can get started.
Creating and Tracking Guardrail Metrics Specific to LLM Use Cases
First, determine the precise use cases for your LLMs. Each application might need to observe distinct guardrail metrics. For example, if your LLM is used in a customer service chatbot, you can trace metrics such as response precision, user contentment, and conversation culmination rates. Customizing these metrics to your use cases ensures that the guardrails are pertinent and efficient.
Next, pioneer an inception for these metrics. This indulges gathering initial information to comprehend the current performance of your LLMs. With this inception, you can set sensible targets and verges that depict adequate performance levels. Frequently trace these metrics to identify trends and anomalies, which can specify probable problems before they expand.
Managing and Monitoring Metrics with Automation
Use automated systems and tools to manage and observe your guardrail metrics effectively. Automated systems not only save time but also accelerate observation precision. Tools such as AI dashboards, metric tracking software, and anomaly detection systems can offer real-time perceptions of your LLMs' performance.
For instance, an automated dashboard can exhibit your key metrics at a glimpse, permitting you to gauge the health of your LLM rapidly. In addition, these tools can send warnings when metrics diverge from the pioneered verges, enabling you to take expeditious action to acknowledge any problems. Transforming to automated systems helps sleek the observation process and ensures you stay on top of your LLM performance.
Incorporating Industry Metrics with In-House ML Research
Incorporating industry-standard metrics is another crucial step in enforcing guardrails. These metrics are extensively determined and provide a standard for assessing your LLMs' performance. Instances include precision, recall, F1 score, and user engagement rates. Using these structured metrics, you can contrast your LLMs' performance against industry norms and ensure you meet pioneered standards.
Alongside these industry-standard metrics, integrate discoveries from your in-house machine learning research. Your team’s unique insights can result in the expansion of auxiliary metrics customized to your precise needs. Merging industry standards and in-house investigation ensures a thorough approach to observing your LLMs.
Now that we've covered how to implement guardrail metrics let's address the value of custom guardrail metrics tailored specifically to your needs.
Custom Guardrail Metrics for LLMs
While standard metrics are essential, adaptability in adding tailored metrics can substantially improve your LLMs' safety and quality control. These custom metrics can acknowledge distinct challenges and must be precise to your applications.
Adding Custom Metrics for Specific LLM Application Needs
Custom guardrail metrics provide the adaptability to acknowledge the precise needs of your LLM applications. For instance, if your LLM produces content for a sensitive domain such as healthcare, you might create metrics to trace medical precision and docility with regulations. Custom metrics permit you to customize the observation process to fit the nuances of your LLM use cases, ensuring more tormented and efficient guardrails.
Examples of Custom Metrics for Enhanced Safety and Quality Control
To improve safety and quality control, contemplate the following examples of custom metrics:
Contextual Accuracy: Assesses how precisely the LLM comprehends and responds within the context of a conversation or document.
Bias Detection: Observe the LLM for any signs of bias in its yields, ensuring neutrality and objectivity.
User Feedback and Integration: The LLM tracks user feedback and integrates it into metric evaluations to continuously enhance its performance.
Ethical Compliance: Ensures the LLMs yield with ethical guidelines and industry standards.
By enforcing these custom metrics, you create a sturdy structure that acknowledges the precise needs of your LLM applications. Transforming from standard to custom metrics permits more accurate and pertinent observation, improving your generative AI systems' overall safety and quality.
With all the information in hand, let's wrap up with a few final thoughts on the importance and future of guardrail metrics.
For comprehensive steps for setting up and managing Large Language Models in real-globe synopsis, check out our Practical Guide For Deploying LLMs In Production.
Conclusion
To conclude the article, guardrail metrics play a critical role in maintaining the security and standard of LLM applications. They act as an attentive observing system, catching probable problems before they expand into substantial issues. Integrating a balanced and pragmatic approach to these metrics ensures that your LLM functions securely and efficiently, giving dependable and valuable interactions.
Guardrail metrics are not just averting harm; they are about building certainty and dependability in the Artificial Intelligence systems we rely on. As we persist in pioneering and developing more sophisticated LLMs, these metrics will remain a backbone of AI security, accompanying us towards safer and more reliable AI applications.
Explore the future of AI-driven perceptions and automation. Join RagaAI today to unleash unparalleled effectiveness and innovation in your functioning. Don’t miss out–register now and lead your industry with cutting-edge technology.
In the swiftly developing field of Artificial Intelligence, ensuring the safety and quality of large language models (LLMs) is paramount. Guardrail metrics, especially customized to generative AI applications, play a critical role in this attempt. You designed these metrics to avert negative alterations and probable harms, not just to serve as another set of information points.
Unlike traditional metrics used in venture or product development, which concentrate on performance and magnification, guardrail metrics for LLMs are about security and quality. They help us ensure that LLMs produce precise and apt yields, minimizing the threat of unintentional outcomes.
Explore our comprehensive article on Building and Implementing Custom LLM Guardrails to better understand the practical aspects of securing AI models.
The Role of Guardrail Metrics in LLM Safety
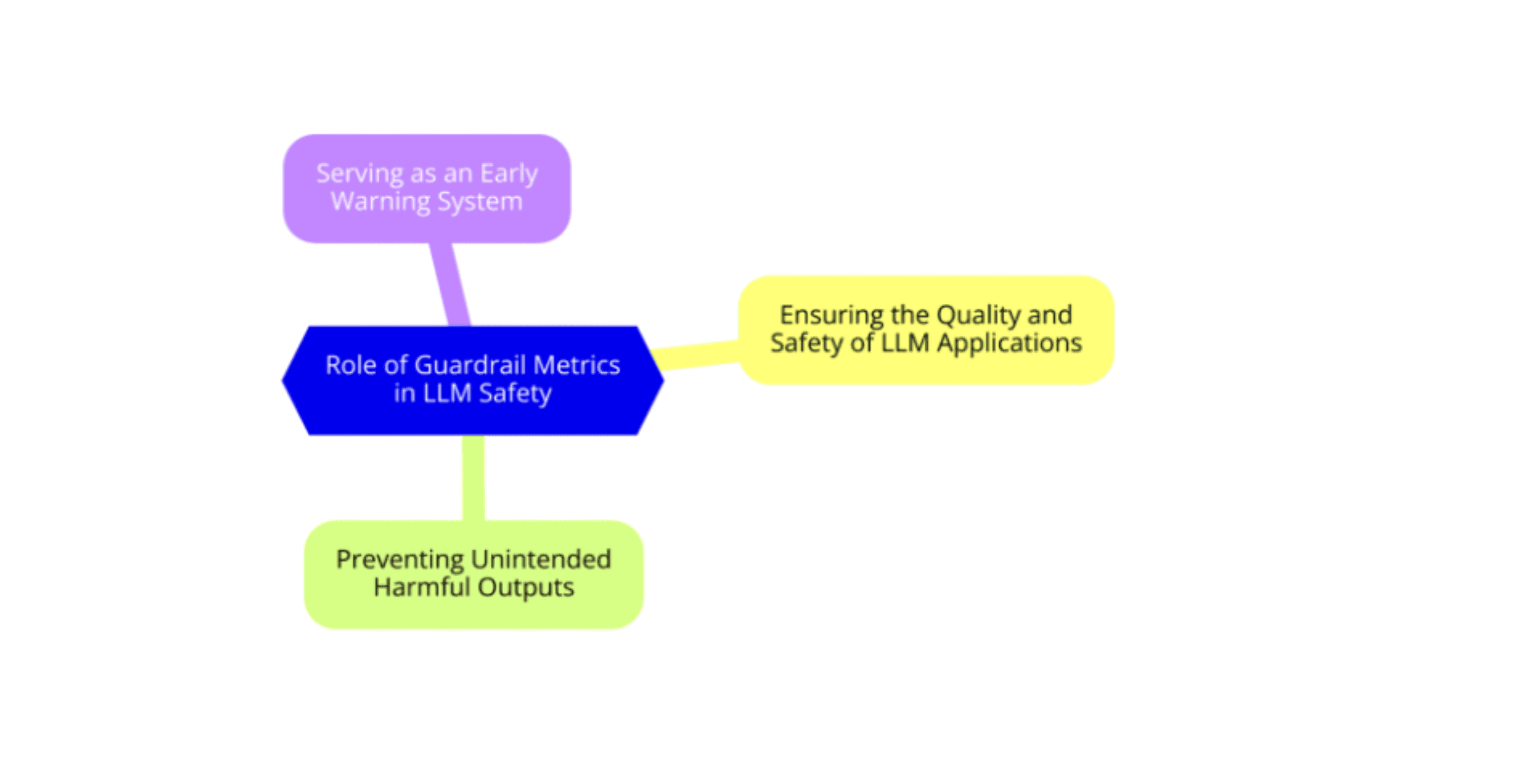
Guardrail metrics are critical in ensuring the safety and dependability of large language models (LLMs). They act as essential checkpoints that help sustain and observe the performance of these sophisticated systems, ensuring they operate as planned.
Ensuring the Quality and Safety of LLM Applications
You want your LLM applications to deliver high-quality and secure communications, right? Guardrail metrics are your ideal tools for this. By constantly monitoring and assessing the yields of your LLM, these metrics ensure that your model follows the set quality standards, giving precise and safe answers. They help you catch any peculiarity or divergence from anticipated behavior, keeping your application dependable and trustworthy.
Preventing Unintended Harmful Outputs
Envision an LLM producing improper and detrimental content during user interactions. That’s a torment synopsis you want to avoid. Guardrail metrics come to the redemption by closely monitoring precise aspects of the LLMs interactions. They help you determine and avert unintended dangerous yields, ensuring that your LLM remains a helpful and safe tool for users.
Serving as an Early Warning System
None like surprises, specifically when it comes to the performance of intricate models. Guardrail metrics serve as an early cautioning system, notifying you of probable problems before they become significant problems. By discerning peculiarities and irregularities, you can acknowledge and resolve them rapidly, sustaining the overall health and functionality of your LLM.
So, now that we understand the critical nature of guardrail metrics let's dive into the various types available for LLMs.
For more comprehensive insights into assessing LLM applications and enforcing guardrails using Raga-LLM-Hub, check out our guide: A Guide to Evaluating LLM Applications and Enabling Guardrails Using Raga-LLM-Hub.
Types of Guardrail Metrics for LLMs
In the Large Language Models (LLMs) scenario, guardrail metrics are your essential tools for sustaining model incorporation and safety. These metrics include sustaining yield quality, securing input safety, and supporting context adherence. Output quality metrics such as BLEU and ROUGE ensure your model’s answers are precise and pertinent. RAG quality metrics concentrate on sustaining the context, providing the model’s yields are coherent and harmonious with the given input. In addition, input quality metrics help you refine detrimental and inappropriate content, securing the communication environment. These guardrail metrics form a sturdy structure to ensure your LLMs deliver safe, dependable, and high-quality performance.
Introduction to Guardrail Metrics for LLM Safety
Ensuring the safety of Large Language Models (LLMs) is critical. Guardrail metrics are your security, ensuring these models perform dependably and ethically. These metrics come in numerous forms, including quality and behavior metrics, customized to keep LLMs in check. Let’s explore these guardrail metrics in more detail.
Output Quality Metrics: BLEU and ROUGE
Regarding output quality, you depend on metrics such as BLEU (Bilingual Evaluation Understudy) and ROUGE (Recall-Oriented Understudy for Gisting Evaluation). BLEU assesses how closely your model’s yield matches a set of reference translations, ensuring content precision. Conversely, ROUGE gauges the overlap of n-grams, word series, and word pairs between the procured and reference texts, concentrating on pertinence. These metrics help you measure whether the content produced by your LLM is precise and pertinent, giving transparent suspicions of the model’s performance.
RAG Quality Metrics
RAG (Retrieval-Augmented Generation) quality metrics are essential for sustaining context adherence. These metrics ensure that your model recovers pertinent data and produces answers that are accurate to the given context. By observing how well your LLM follows the input context, RAG metrics help you avert off-topic or absurd answers, thereby improving the model’s dependability.
Input Quality Metrics: Safety Measures
Ensuring the quality of inputs is just as significant as assessing yields. Input quality metrics concentrate on discerning and alleviating hazardous or inappropriate inputs. You can enforce safety assessments like content filters and pathogenic detectors to screen inputs before they reach the LLM. These metrics help you sustain a safe and regardful interaction environment, protecting users from detrimental content and ensuring the ethical use of LLMs.
Protecting Personal Identifiable Information (PII)
When dealing with LLMs, ensuring the ammunition of Personal Identifiable Information (PII) is paramount. Suppose your AI accidentally exposing someone’s social security number or address. Not cool, right?
To avoid this, you can enforce PII Detection Metrics. These metrics scan outputs for any sensitive data and ensure it’s censored or not produced in the first place. It’s like having a vigilant security guard that questions anything suspicious.
Tackling Bias in AI
Bias in AI can be an enormous problem. Think about it: if your LLM constantly generates biased outcomes, it could commemorate stereotypes or unfair treatment. This is where bias detection metrics comes into play.
These metrics assess the outputs for any unfair bias based on race, gender, age, or other attributes. By flagging and ackowedging biased answers, you ensure your AI remains impartial and balanced. It’s like giving your AI a moral compass to check tricky legitimate ethical terrains.
Reducing Toxicity
No one likes communicating with a toxic AI. Whether it’s spitting out detrimental language or nurturing negative behavior, toxicity in LLMs can lead to serious reputational detriment. Toxicity detection metrics help you observe and minimize detrimental language.
These metrics can determine the language produced and flag anything that’s insulting, hazardous, or otherwise inappropriate. Think of it as having a filter that cleanses your AI’s language before it reaches the user.
By comprehending and enforcing these guardrail metrics, you can ensure that your LLMs function safely and efficiently, providing precise and contextually pertinent yields while securing against detrimental inputs.
Are you curious about how to implement guardrail metrics in your generative AI applications? Let's walk through the necessary steps next.
Check out our guide on Evaluating Large Language Models: Methods And Metrics to investigate ways we gauge AI.
Implementing Guardrail Metrics in Generative AI
Implementing guardrail metrics in generative AI is critical for ensuring security and quality in your Large Language Models (LLMs). Creating and tracing these metrics can avert probable problems and sustain control over your AI applications. Here’s how you can get started.
Creating and Tracking Guardrail Metrics Specific to LLM Use Cases
First, determine the precise use cases for your LLMs. Each application might need to observe distinct guardrail metrics. For example, if your LLM is used in a customer service chatbot, you can trace metrics such as response precision, user contentment, and conversation culmination rates. Customizing these metrics to your use cases ensures that the guardrails are pertinent and efficient.
Next, pioneer an inception for these metrics. This indulges gathering initial information to comprehend the current performance of your LLMs. With this inception, you can set sensible targets and verges that depict adequate performance levels. Frequently trace these metrics to identify trends and anomalies, which can specify probable problems before they expand.
Managing and Monitoring Metrics with Automation
Use automated systems and tools to manage and observe your guardrail metrics effectively. Automated systems not only save time but also accelerate observation precision. Tools such as AI dashboards, metric tracking software, and anomaly detection systems can offer real-time perceptions of your LLMs' performance.
For instance, an automated dashboard can exhibit your key metrics at a glimpse, permitting you to gauge the health of your LLM rapidly. In addition, these tools can send warnings when metrics diverge from the pioneered verges, enabling you to take expeditious action to acknowledge any problems. Transforming to automated systems helps sleek the observation process and ensures you stay on top of your LLM performance.
Incorporating Industry Metrics with In-House ML Research
Incorporating industry-standard metrics is another crucial step in enforcing guardrails. These metrics are extensively determined and provide a standard for assessing your LLMs' performance. Instances include precision, recall, F1 score, and user engagement rates. Using these structured metrics, you can contrast your LLMs' performance against industry norms and ensure you meet pioneered standards.
Alongside these industry-standard metrics, integrate discoveries from your in-house machine learning research. Your team’s unique insights can result in the expansion of auxiliary metrics customized to your precise needs. Merging industry standards and in-house investigation ensures a thorough approach to observing your LLMs.
Now that we've covered how to implement guardrail metrics let's address the value of custom guardrail metrics tailored specifically to your needs.
Custom Guardrail Metrics for LLMs
While standard metrics are essential, adaptability in adding tailored metrics can substantially improve your LLMs' safety and quality control. These custom metrics can acknowledge distinct challenges and must be precise to your applications.
Adding Custom Metrics for Specific LLM Application Needs
Custom guardrail metrics provide the adaptability to acknowledge the precise needs of your LLM applications. For instance, if your LLM produces content for a sensitive domain such as healthcare, you might create metrics to trace medical precision and docility with regulations. Custom metrics permit you to customize the observation process to fit the nuances of your LLM use cases, ensuring more tormented and efficient guardrails.
Examples of Custom Metrics for Enhanced Safety and Quality Control
To improve safety and quality control, contemplate the following examples of custom metrics:
Contextual Accuracy: Assesses how precisely the LLM comprehends and responds within the context of a conversation or document.
Bias Detection: Observe the LLM for any signs of bias in its yields, ensuring neutrality and objectivity.
User Feedback and Integration: The LLM tracks user feedback and integrates it into metric evaluations to continuously enhance its performance.
Ethical Compliance: Ensures the LLMs yield with ethical guidelines and industry standards.
By enforcing these custom metrics, you create a sturdy structure that acknowledges the precise needs of your LLM applications. Transforming from standard to custom metrics permits more accurate and pertinent observation, improving your generative AI systems' overall safety and quality.
With all the information in hand, let's wrap up with a few final thoughts on the importance and future of guardrail metrics.
For comprehensive steps for setting up and managing Large Language Models in real-globe synopsis, check out our Practical Guide For Deploying LLMs In Production.
Conclusion
To conclude the article, guardrail metrics play a critical role in maintaining the security and standard of LLM applications. They act as an attentive observing system, catching probable problems before they expand into substantial issues. Integrating a balanced and pragmatic approach to these metrics ensures that your LLM functions securely and efficiently, giving dependable and valuable interactions.
Guardrail metrics are not just averting harm; they are about building certainty and dependability in the Artificial Intelligence systems we rely on. As we persist in pioneering and developing more sophisticated LLMs, these metrics will remain a backbone of AI security, accompanying us towards safer and more reliable AI applications.
Explore the future of AI-driven perceptions and automation. Join RagaAI today to unleash unparalleled effectiveness and innovation in your functioning. Don’t miss out–register now and lead your industry with cutting-edge technology.
In the swiftly developing field of Artificial Intelligence, ensuring the safety and quality of large language models (LLMs) is paramount. Guardrail metrics, especially customized to generative AI applications, play a critical role in this attempt. You designed these metrics to avert negative alterations and probable harms, not just to serve as another set of information points.
Unlike traditional metrics used in venture or product development, which concentrate on performance and magnification, guardrail metrics for LLMs are about security and quality. They help us ensure that LLMs produce precise and apt yields, minimizing the threat of unintentional outcomes.
Explore our comprehensive article on Building and Implementing Custom LLM Guardrails to better understand the practical aspects of securing AI models.
The Role of Guardrail Metrics in LLM Safety
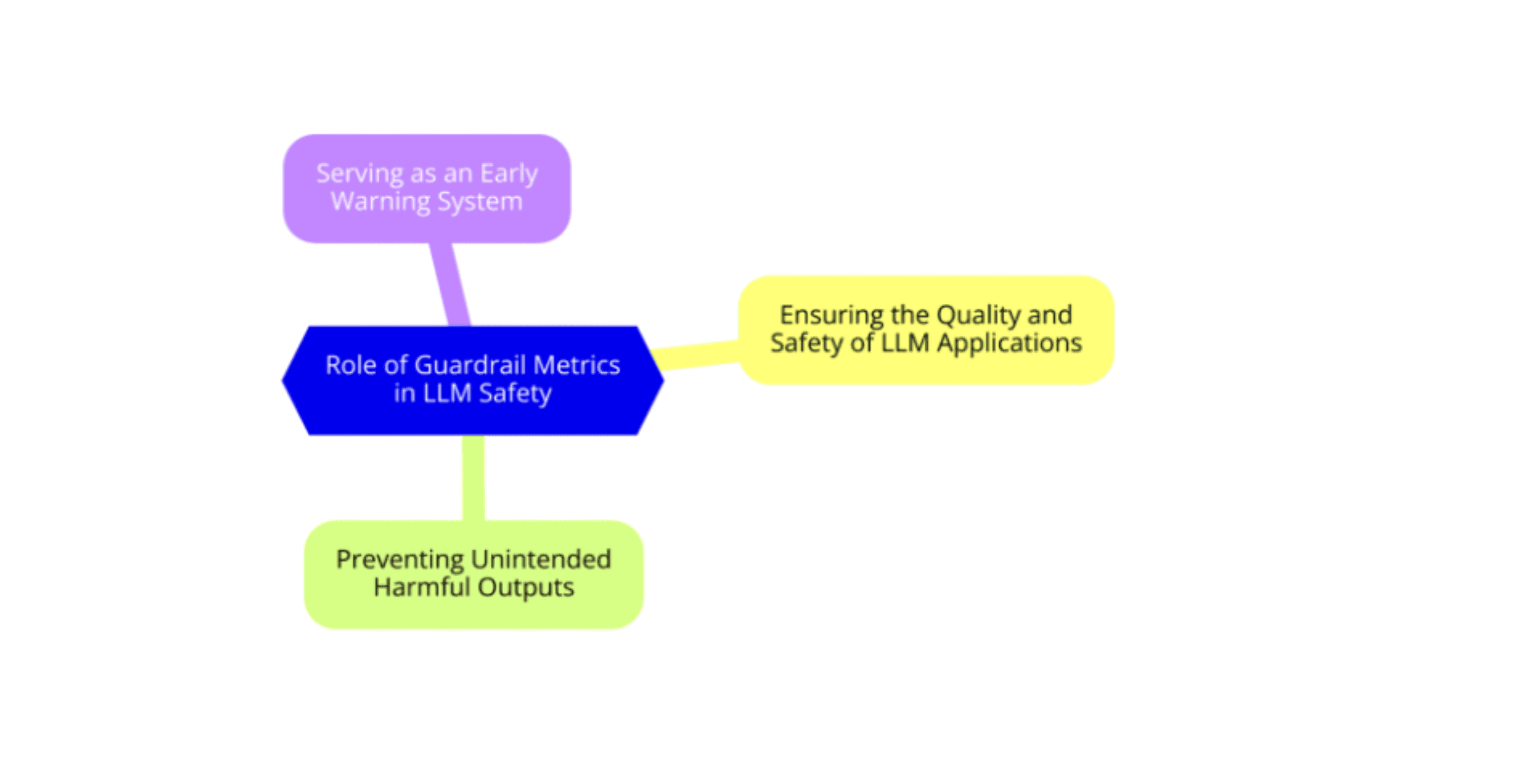
Guardrail metrics are critical in ensuring the safety and dependability of large language models (LLMs). They act as essential checkpoints that help sustain and observe the performance of these sophisticated systems, ensuring they operate as planned.
Ensuring the Quality and Safety of LLM Applications
You want your LLM applications to deliver high-quality and secure communications, right? Guardrail metrics are your ideal tools for this. By constantly monitoring and assessing the yields of your LLM, these metrics ensure that your model follows the set quality standards, giving precise and safe answers. They help you catch any peculiarity or divergence from anticipated behavior, keeping your application dependable and trustworthy.
Preventing Unintended Harmful Outputs
Envision an LLM producing improper and detrimental content during user interactions. That’s a torment synopsis you want to avoid. Guardrail metrics come to the redemption by closely monitoring precise aspects of the LLMs interactions. They help you determine and avert unintended dangerous yields, ensuring that your LLM remains a helpful and safe tool for users.
Serving as an Early Warning System
None like surprises, specifically when it comes to the performance of intricate models. Guardrail metrics serve as an early cautioning system, notifying you of probable problems before they become significant problems. By discerning peculiarities and irregularities, you can acknowledge and resolve them rapidly, sustaining the overall health and functionality of your LLM.
So, now that we understand the critical nature of guardrail metrics let's dive into the various types available for LLMs.
For more comprehensive insights into assessing LLM applications and enforcing guardrails using Raga-LLM-Hub, check out our guide: A Guide to Evaluating LLM Applications and Enabling Guardrails Using Raga-LLM-Hub.
Types of Guardrail Metrics for LLMs
In the Large Language Models (LLMs) scenario, guardrail metrics are your essential tools for sustaining model incorporation and safety. These metrics include sustaining yield quality, securing input safety, and supporting context adherence. Output quality metrics such as BLEU and ROUGE ensure your model’s answers are precise and pertinent. RAG quality metrics concentrate on sustaining the context, providing the model’s yields are coherent and harmonious with the given input. In addition, input quality metrics help you refine detrimental and inappropriate content, securing the communication environment. These guardrail metrics form a sturdy structure to ensure your LLMs deliver safe, dependable, and high-quality performance.
Introduction to Guardrail Metrics for LLM Safety
Ensuring the safety of Large Language Models (LLMs) is critical. Guardrail metrics are your security, ensuring these models perform dependably and ethically. These metrics come in numerous forms, including quality and behavior metrics, customized to keep LLMs in check. Let’s explore these guardrail metrics in more detail.
Output Quality Metrics: BLEU and ROUGE
Regarding output quality, you depend on metrics such as BLEU (Bilingual Evaluation Understudy) and ROUGE (Recall-Oriented Understudy for Gisting Evaluation). BLEU assesses how closely your model’s yield matches a set of reference translations, ensuring content precision. Conversely, ROUGE gauges the overlap of n-grams, word series, and word pairs between the procured and reference texts, concentrating on pertinence. These metrics help you measure whether the content produced by your LLM is precise and pertinent, giving transparent suspicions of the model’s performance.
RAG Quality Metrics
RAG (Retrieval-Augmented Generation) quality metrics are essential for sustaining context adherence. These metrics ensure that your model recovers pertinent data and produces answers that are accurate to the given context. By observing how well your LLM follows the input context, RAG metrics help you avert off-topic or absurd answers, thereby improving the model’s dependability.
Input Quality Metrics: Safety Measures
Ensuring the quality of inputs is just as significant as assessing yields. Input quality metrics concentrate on discerning and alleviating hazardous or inappropriate inputs. You can enforce safety assessments like content filters and pathogenic detectors to screen inputs before they reach the LLM. These metrics help you sustain a safe and regardful interaction environment, protecting users from detrimental content and ensuring the ethical use of LLMs.
Protecting Personal Identifiable Information (PII)
When dealing with LLMs, ensuring the ammunition of Personal Identifiable Information (PII) is paramount. Suppose your AI accidentally exposing someone’s social security number or address. Not cool, right?
To avoid this, you can enforce PII Detection Metrics. These metrics scan outputs for any sensitive data and ensure it’s censored or not produced in the first place. It’s like having a vigilant security guard that questions anything suspicious.
Tackling Bias in AI
Bias in AI can be an enormous problem. Think about it: if your LLM constantly generates biased outcomes, it could commemorate stereotypes or unfair treatment. This is where bias detection metrics comes into play.
These metrics assess the outputs for any unfair bias based on race, gender, age, or other attributes. By flagging and ackowedging biased answers, you ensure your AI remains impartial and balanced. It’s like giving your AI a moral compass to check tricky legitimate ethical terrains.
Reducing Toxicity
No one likes communicating with a toxic AI. Whether it’s spitting out detrimental language or nurturing negative behavior, toxicity in LLMs can lead to serious reputational detriment. Toxicity detection metrics help you observe and minimize detrimental language.
These metrics can determine the language produced and flag anything that’s insulting, hazardous, or otherwise inappropriate. Think of it as having a filter that cleanses your AI’s language before it reaches the user.
By comprehending and enforcing these guardrail metrics, you can ensure that your LLMs function safely and efficiently, providing precise and contextually pertinent yields while securing against detrimental inputs.
Are you curious about how to implement guardrail metrics in your generative AI applications? Let's walk through the necessary steps next.
Check out our guide on Evaluating Large Language Models: Methods And Metrics to investigate ways we gauge AI.
Implementing Guardrail Metrics in Generative AI
Implementing guardrail metrics in generative AI is critical for ensuring security and quality in your Large Language Models (LLMs). Creating and tracing these metrics can avert probable problems and sustain control over your AI applications. Here’s how you can get started.
Creating and Tracking Guardrail Metrics Specific to LLM Use Cases
First, determine the precise use cases for your LLMs. Each application might need to observe distinct guardrail metrics. For example, if your LLM is used in a customer service chatbot, you can trace metrics such as response precision, user contentment, and conversation culmination rates. Customizing these metrics to your use cases ensures that the guardrails are pertinent and efficient.
Next, pioneer an inception for these metrics. This indulges gathering initial information to comprehend the current performance of your LLMs. With this inception, you can set sensible targets and verges that depict adequate performance levels. Frequently trace these metrics to identify trends and anomalies, which can specify probable problems before they expand.
Managing and Monitoring Metrics with Automation
Use automated systems and tools to manage and observe your guardrail metrics effectively. Automated systems not only save time but also accelerate observation precision. Tools such as AI dashboards, metric tracking software, and anomaly detection systems can offer real-time perceptions of your LLMs' performance.
For instance, an automated dashboard can exhibit your key metrics at a glimpse, permitting you to gauge the health of your LLM rapidly. In addition, these tools can send warnings when metrics diverge from the pioneered verges, enabling you to take expeditious action to acknowledge any problems. Transforming to automated systems helps sleek the observation process and ensures you stay on top of your LLM performance.
Incorporating Industry Metrics with In-House ML Research
Incorporating industry-standard metrics is another crucial step in enforcing guardrails. These metrics are extensively determined and provide a standard for assessing your LLMs' performance. Instances include precision, recall, F1 score, and user engagement rates. Using these structured metrics, you can contrast your LLMs' performance against industry norms and ensure you meet pioneered standards.
Alongside these industry-standard metrics, integrate discoveries from your in-house machine learning research. Your team’s unique insights can result in the expansion of auxiliary metrics customized to your precise needs. Merging industry standards and in-house investigation ensures a thorough approach to observing your LLMs.
Now that we've covered how to implement guardrail metrics let's address the value of custom guardrail metrics tailored specifically to your needs.
Custom Guardrail Metrics for LLMs
While standard metrics are essential, adaptability in adding tailored metrics can substantially improve your LLMs' safety and quality control. These custom metrics can acknowledge distinct challenges and must be precise to your applications.
Adding Custom Metrics for Specific LLM Application Needs
Custom guardrail metrics provide the adaptability to acknowledge the precise needs of your LLM applications. For instance, if your LLM produces content for a sensitive domain such as healthcare, you might create metrics to trace medical precision and docility with regulations. Custom metrics permit you to customize the observation process to fit the nuances of your LLM use cases, ensuring more tormented and efficient guardrails.
Examples of Custom Metrics for Enhanced Safety and Quality Control
To improve safety and quality control, contemplate the following examples of custom metrics:
Contextual Accuracy: Assesses how precisely the LLM comprehends and responds within the context of a conversation or document.
Bias Detection: Observe the LLM for any signs of bias in its yields, ensuring neutrality and objectivity.
User Feedback and Integration: The LLM tracks user feedback and integrates it into metric evaluations to continuously enhance its performance.
Ethical Compliance: Ensures the LLMs yield with ethical guidelines and industry standards.
By enforcing these custom metrics, you create a sturdy structure that acknowledges the precise needs of your LLM applications. Transforming from standard to custom metrics permits more accurate and pertinent observation, improving your generative AI systems' overall safety and quality.
With all the information in hand, let's wrap up with a few final thoughts on the importance and future of guardrail metrics.
For comprehensive steps for setting up and managing Large Language Models in real-globe synopsis, check out our Practical Guide For Deploying LLMs In Production.
Conclusion
To conclude the article, guardrail metrics play a critical role in maintaining the security and standard of LLM applications. They act as an attentive observing system, catching probable problems before they expand into substantial issues. Integrating a balanced and pragmatic approach to these metrics ensures that your LLM functions securely and efficiently, giving dependable and valuable interactions.
Guardrail metrics are not just averting harm; they are about building certainty and dependability in the Artificial Intelligence systems we rely on. As we persist in pioneering and developing more sophisticated LLMs, these metrics will remain a backbone of AI security, accompanying us towards safer and more reliable AI applications.
Explore the future of AI-driven perceptions and automation. Join RagaAI today to unleash unparalleled effectiveness and innovation in your functioning. Don’t miss out–register now and lead your industry with cutting-edge technology.
Subscribe to our newsletter to never miss an update
Other articles


Agents Talk, Models Think: A2A + MCP for Enterprise Agentic AI

Riya Parikh, Nitai Agarwal
Apr 27, 2025
Read the article
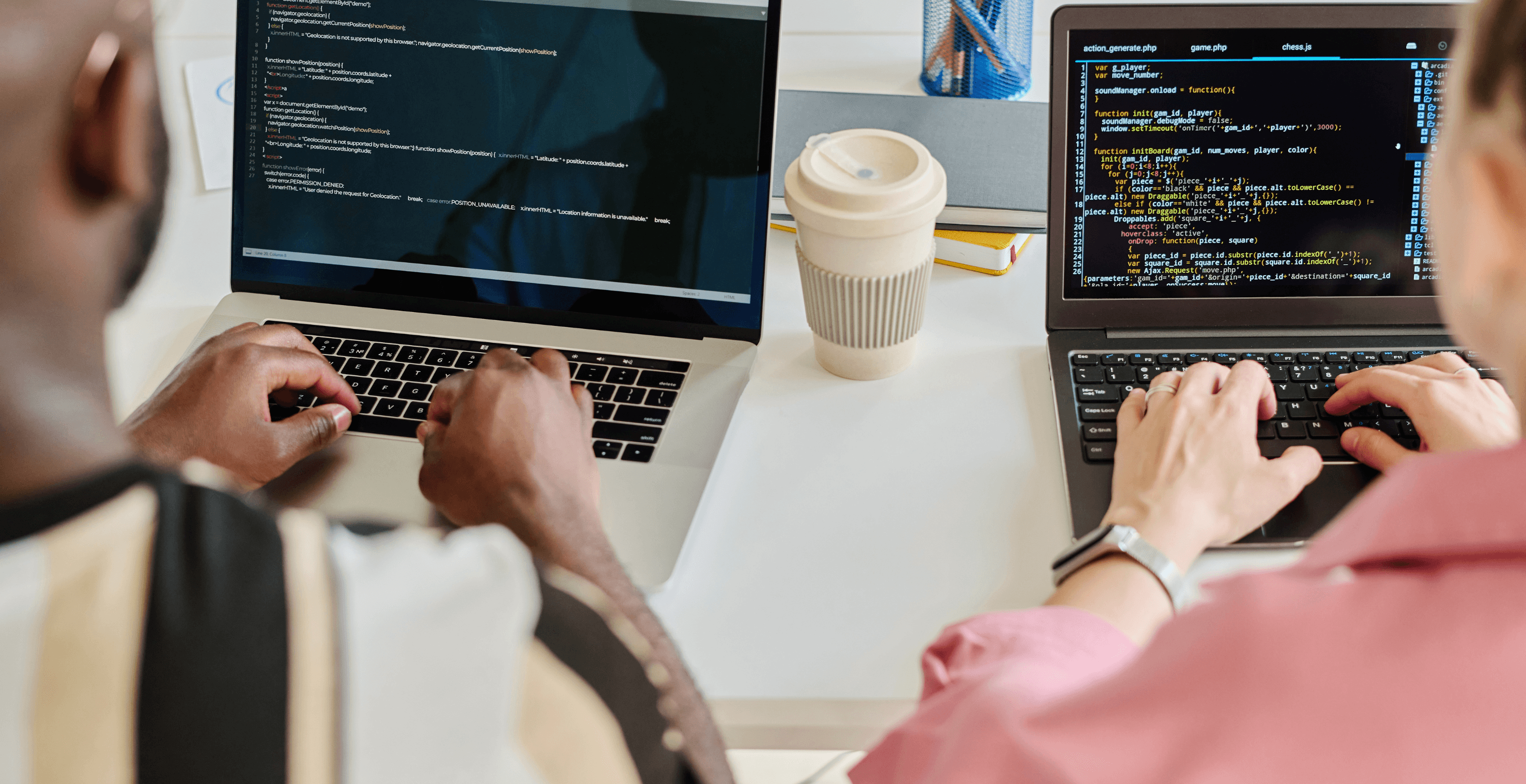
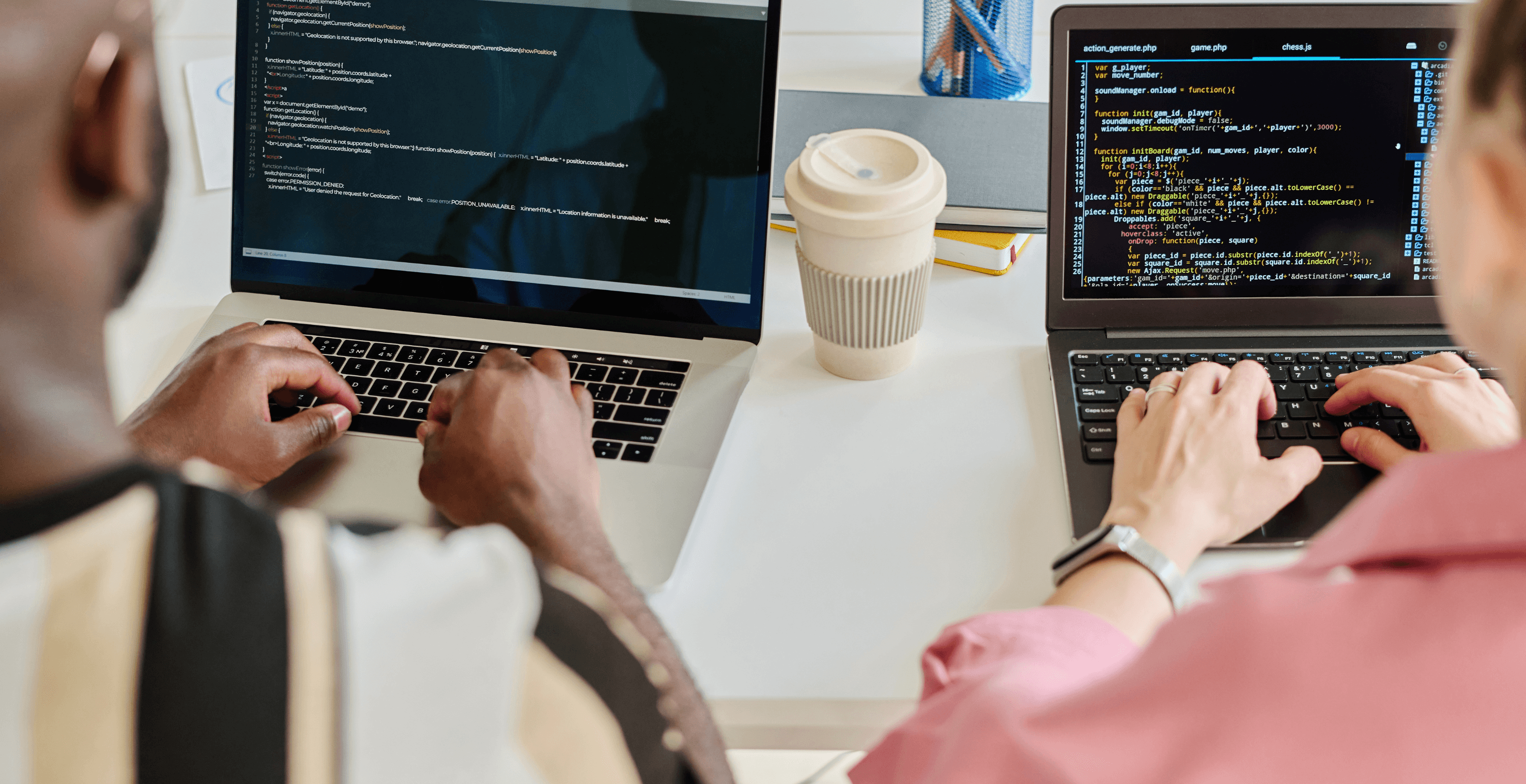
Understanding NeMo Guardrails: A Toolkit for LLM Security

Rehan Asif
Dec 24, 2024
Read the article


Understanding Differences in Large vs Small Language Models (LLM vs SLM)

Rehan Asif
Dec 21, 2024
Read the article