Building And Implementing Custom LLM Guardrails
Building And Implementing Custom LLM Guardrails
Building And Implementing Custom LLM Guardrails
Rehan Asif
Jun 12, 2024




Ever tried herding cats? Now imagine doing that with AI – that's where LLM guardrails come into play!
Custom guardrails in Large Language Models (LLMs) are precise rules and structures that control the yields these advanced AI systems generate. These guardrails ensure that the retaliations and actions of an LLM correspond with desired moral, legitimate and contextual standards.
The predominant purpose of custom guardrails is to avert harmful or unsuitable yields, thus protecting users and investors from possible threats associated with AI-produced content.
So, how do we even begin to set up these guardrails in LLMs? Let’s know through this guide!
Types of Custom Guardrails for LLMs
Compliance Guardrails: Aligning Outputs with Legal Standards
Compliance guardrails ensure that the yields of an LLM follow pertinent laws and regulations. For instance, in the financial industry, you can design guardrails to avert the LLM from making unauthorized investment suggestions or revealing sensitive financial information. These guardrails help safeguard your organization from legitimate consequences and maintain compliance with industry norms.
Ethical considerations are next on the agenda, but trust me, it's more intriguing than it sounds!
Ethical Guardrails: Ensuring Outputs Meet Ethical Guidelines
Ethical guardrails are designed to align LLM yields with pioneered ethical principles. This includes averting biased, unfair or abusive language. For example, an LLM in employment should be shaped to avoid gender-biased language when assessing candidates. By enforcing ethical guardrails, you can promote a more comprehensive and respectful AI environment.
Now, let’s dive into the quirks and nuances of context-specific guardrails. Hang tight; it’s going to be insightful!
Contextual Guardrails: Tailoring Responses to Specific Contexts or Domains
Contextual guardrails customize the LLMs responses to fit precise contexts or domains. For instance, an LLM used in customer service for a technology firm should give precise and pertinent technical support information, rather than general knowledge or dissimilar guidance. These guardrails ensure that the AI’s yields are pertinent and beneficial to the deliberated audience.
Next up, let’s talk about security—because nobody wants their AI to turn into a cyber nincompoop.
Security Guardrails: Safeguarding Against Vulnerabilities and Threats
Security Guardrails safeguard against possible vulnerabilities and risks, like data infringement or unauthorized access. For example, in an LLM used for commercial interactions, guardrails can be set to avert the revelation of sensitive information or to filter out counterfeit efforts. These security measures are important for safeguarding both the AI systems and its users from cyber attacks.
Did someone say adaptability? Let’s get into how these guardrails can evolve and keep your AI sharp.
Adaptive Guardrails: Evolving with Changing Standards and Regulations
Adaptive guardrails are designed to develop and update in feedback to changing norms and regulations. This is specifically significant in industries with swiftly altering compliance needs. For instance, in the healthcare sector, adaptive guardrails can be updated to reflect new patient seclusion laws and medical guidelines. By enforcing adaptive guardrails, you ensure that your AI system stays compliant and efficient over time.
Ready to locate the best guardrails for your requirements? Delve into our thorough comparison of the leading large language models (LLMs). Comprehend their capabilities, powers and inventive attributes to make an informed choice.
Alright, now that we have discussed the various types of guardrails, let’s switch gears and get into the actual design process.
Designing Custom Guardrails
Analyzing Requirements: Identifying Specific Needs and Risks
Dissect the precise requirements and threats associated with your application as the initial step in designing custom guardrails.
This indulges in determining possible areas where the LLMs yields could be detrimental and non-compliant. For instance, if you are developing an LLM for informative purposes, you need to ensure it offers precise and age-accurate content. Conducting a pragmatic risk assessment helps you distinguish the crucial areas where guardrails are required.
Construction Methodologies: From Policy Enforcement to Continuous Adaptability
Once you have determined the needs, you can start creating the guardrails. This involves creating strategies and rules that the LLM must adhere to. For example, you might execute policies that limit the utilization of assured language and need fact-checking before data is given. Also, design guardrails that can adjust over time, allowing constant enhancement and updating as new threats and standards arise.
Technical Considerations: Balancing Accuracy, Latency, and System Integrations
When designing guardrails, it's important to contemplate the technical elements, like precision, suspension and system incorporations.
Ensure precision so that the guardrails efficiently filter unsuitable content without hampering the LLM’s performance. Latency involves minimizing detentions caused by guardrail checks, ensuring a sleek user experience. In addition, incorporating guardrails with existing systems and productivity is critical for streamlined functioning.
Examples of Custom Guardrail Designs for Diverse Industry Needs
When it comes to ensuring security in numerous industries, having the right guardrails in place is important. Customize custom guardrails to precise requirements to improve security and offer a sleek fit for a distinct environment. Let’s discover some instances of custom guardrail design that serve disparate industry needs, concentrating on how LLM guardrails can make a substantial impact.
Warehouse and Industry Guardrails
In occupied warehouses and industry settings, disaster can happen in the blink of an eye. Custom guardrails here are created to resist heavy impacts from freight elevators and other machinery. They can be customized to fit around machinery, safeguard climbers, walkways and separate work areas. Envision installing LLM guardrails in your warehouse; they not only provide sturdy security but also ensure that outline is effective and secure for all workers.
Parking Lot Guardrails
Parking lots often see a lot of automotive traffic, and guardrails play a pivotal role in averting accidents. Custom guardrails for parking lots are created to guide the flow of traffic, safeguard climbers, and avert cars from collisions into structures. By using LLM guardrails, you can create a secure and arranged parking area that curtails the risk of accidents and improves the overall user experience.
Public Park and Recreational Area Guardrails
Public parks and recreational areas need guardrails that amalgamate with the environment while ensuring security. Custom guardrails in these settings are often designed with elegance in mind, utilizing materials and colors that adjunct with natural surroundings. With LLM guardrails, you can accomplish a perfect balance of security and beauty, making the park a delightful place for everyone to relish.
Hospital and Healthcare Facility Guardrails
In hospitals and healthcare facilities, safety is the highest priority. Custom guardrails here are designed to safeguard patients, staff and guests from possible dangers. Often install them in corridors, around medical apparatus, and in parking areas. Use LLM guardrails to offer significant safety while making them easy to clean and maintain, which is significant in a healthcare environment.
Commercial Building Guardrails
Commercial buildings, indulging offices and shopping malls, benefit from custom guardrails that improve security without yielding on aesthetics. These guardrails are designed to amalgamate flawlessly with the building’s architecture while offering sturdy protection. Choose LLM guardrails to ensure that your commercial space is both secure and tangibly fascinating, thereby improving the overall experience for employees and guests.
Construction Site Guardrails
Construction sites are fundamentally risky, and having the right guardrails is significant to safeguard workers and visitors. Design custom guardrails for construction sites to be delicate and adjustable to altering site conditions. LLM guardrails can be personalized to meet precise safety norms and regulations, ensuring that your site stays compliant and secure.
Select custom LLM guardrails for your precise industry requirements to ensure maximum security and effectiveness. These instances embellish how customized fixes can make a substantial difference in numerous environments, giving peace of mind and protection where it’s required the most.
The release of RagaAI’s thorough assessment and guardrail platform marks a substantial progression in the field of large language models (LLMs). By acknowledging the crucial requirement for averting catastrophic setbacks, this platform sets a new norm for security and dependability in Artificial Intelligence applications. As the AI prospect persists to develop, tools such as RagaAI’s platform will be important in ensuring that these influential technologies can be deployed responsibly and efficiently.
Find how RagaAI’s platform can protect your AI enforcements. Read more here.
Implementing Custom Guardrails in LLM Systems
Preprocessing Inputs: Techniques for Input Validation and Sanitization
Before your LLM refines any input, it’s critical to verify and sanitize the data to avert vicious or detrimental content. Enforce input verification by inspecting for predefined formats, adequate characters, and coherent consistency. For instance, if your LLM is designed to manage email queries, ensure that the input adheres to email syntax and circumvents possibly dangerous scripts.
Sanitize by cleaning the input to extract and compensate for possibly detrimental components. Utilize libraries such as OWASP for Python or DOMPurify for Javascript to clear out vicious code from user inputs. This step safeguards your system and improves the quality of the output by ensuring the LLM receives clean and pertinent data.
Real-time Output Filtering: Strategies for Moderating and Fact-checking LLM Outputs
Real-time output filtering is significant for handling the incorporation and safety of LLM yields. Enforce moderation tools that dissect the LLMs responses for harmful, partial, or unsuitable content. Methods like keyword extracting, opinion analysis, and machine learning codification can help discover and alleviate troublesome outputs.
Rectification is another crucial aspect. Enforce real-time fact-checking APIs, like those Factmata and Full Fact offer, to validate the data the LLM generates. This ensures that the responses are not only secure but also precise and steadfast.
User Engagement and Feedback Mechanisms: Enabling Continuous Improvement
Engage users and collect responses for constant enhancement of your LLM system. Enforce feedback mechanisms that permit users to appraise feedback, flag problems and write comments. Tools such as user surveys, in-app feedback forms, and communicative prompts can facilitate this procedure.
Inspecting user responses helps determine common problems and areas for improvement. Use this information to fine-tune your LLM, update training datasets, and adapt algorithms. Constant learning from user interactions ensures that your LLM evolves to meet user requirements more efficiently.
Integration with LLM APIs and Platforms: Practical Implementation Steps
Incorporate system guardrails with LLM APIs and the platform using a standard approach. Start by choosing a suitable LLM platform, like OpenAI or HuggingFace, that aids the incorporation of custom guardrails. Acquaint yourself with the platform’s API documentation and capabilities.
Develop middleware that connects the user inputs and the guardrail mechanisms. This middleware should manage input predigestion, real-time output refining, and response gathering smoothly. For instance, use Flask or Express.js to make an API layer that handles these processes.
In the end, examine your incorporated system considerably to ensure the guardrails function as aimed without shuffling the user experience. Conduct both computerized and manual testing to cover a wide range of synopsis and edge cases.
By executing these strategies, you can create a sturdy LLM system that delivers dependable, precise and secure outputs, improving user trust and experience.
Monitoring and Evaluating Guardrail Effectiveness
Setting Up Metrics and KPIs for Guardrail Performance
When you set up metrics and Key Performance Indicators (KPIs) for your guardrails, you’re pioneering a clear structure to gauge their success. Begin by determining the predominant intentions of your guardrails. Are they designed to improve security, enhance functional effectiveness, or ensure docility with regulations.
Contemplate using metrics like the number of safety incidents averted, the regularity of guardrail activations, and the abatement in functional disruptions. KPIs might indulge the probability curtailed in disasters, the response duration to incidents, and user docility rates. For instance, if your guardrails are in place to curtail data infringement, a substantial KPI would be the abatement in unauthorized access efforts.
Tools and Techniques for Monitoring Guardrail Effectiveness
Observing the efficiency of your guardrails needs the right tools and methods. Enforce real-time monitoring systems that can trace guardrail initiations and provide instantaneous vigilantism. Tools such as Splunk or ELK stack can collect and dissect log data, providing perceptions into guardrail performance.
Utilize machine learning models to forecast possible failures or infringements and use dashboards to visualize performance metrics. Use these tools to comprehend trends and irregularities, thereby permitting energetic adaptations. In addition, frequent audits and docility checks ensure that guardrails stay efficient over time.
Adjusting Guardrails Based on Feedback and Performance Data
Adapt guardrails based on response and performance data to maintain their efficiency. Gather data from several sources, indulging system logs, user response and accident reports. Dissect this data to discover motifs and areas to enhance.
For example, if you observe a high prevalence of erroneous positives, fine-tune the perceptivity of your guardrails. Engage with investors to collect instinctive responses and ensure the adaptations align with their requirements. Frequently overhaul your guardrails to adjust to new risks and functional alterations to ensure they stay pertinent and efficient.
Case Studies on the Impact of Custom Guardrails in Real-World Applications
Let’s now discover some real-world instances to comprehend the impact of custom guardrails. In the financial sector, a chief bank enforced custom guardrails to avert deceptive transactions. By setting up precise KPIs and utilizing advanced observing tools, they curtail deceit attempts by 30% within the first 6 months.
Another instance is a healthcare supplier that used custom guardrails to safeguard the patient records. They observed data access motifs and adapted their guardrails based on user behavior This approach leads to 40% decrease in data infringement and enhanced patient trust.
In both cases, the amalgamation of well-defined metrics, efficient monitoring tools, and versatile adaptations based on performance data substantially improved the efficiency of the guardrails.
Ready to boost the security and dependability of your enterprise LLM applications? Explore how RagaAI’s advanced guardrails can protect your data and upgrade performance. Contact us today to learn more about enforcing sturdy solutions to your venture requirements. Secure your future with RagaAI!
Best Practices and Challenges Of Guardrails
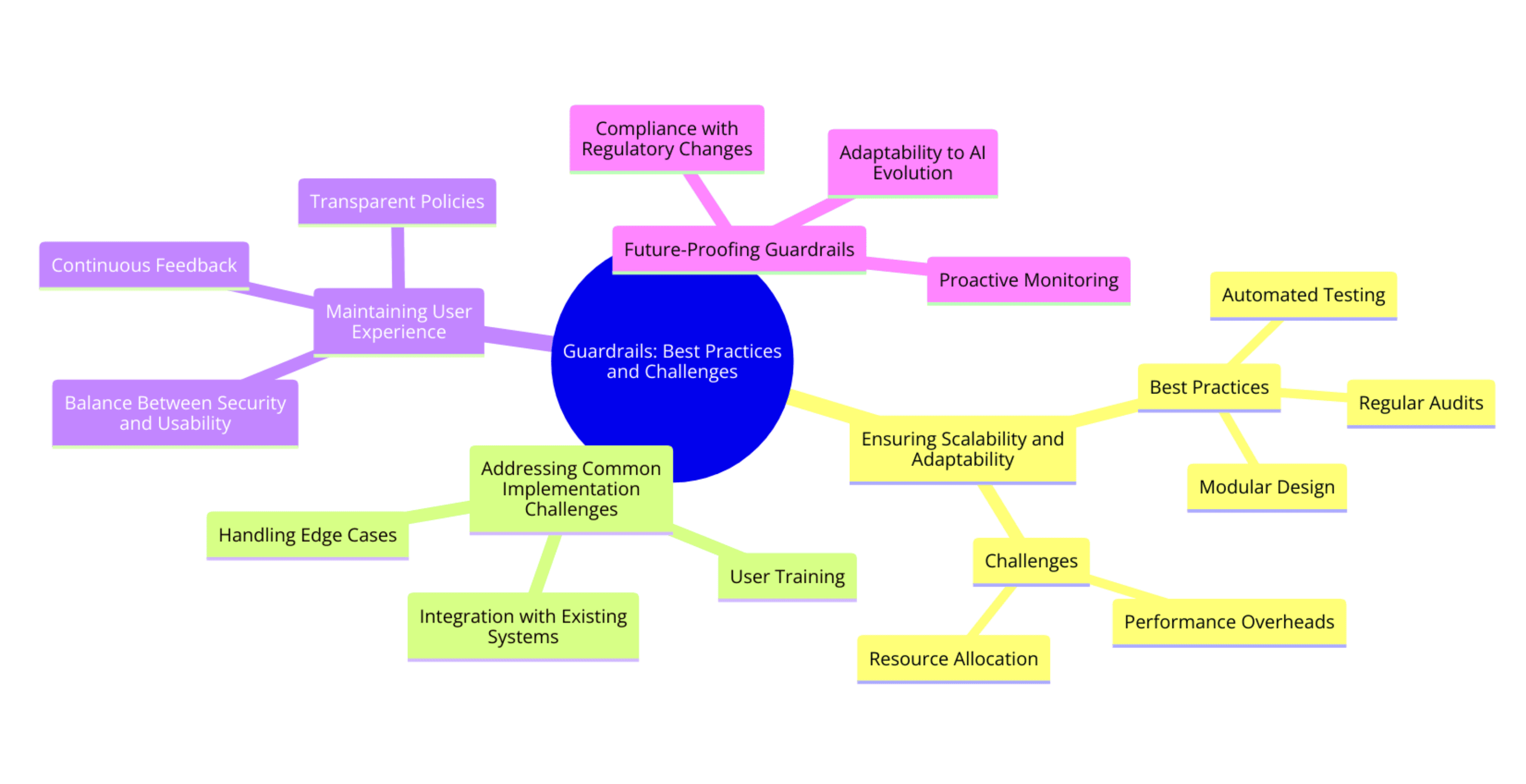
Ensuring Scalability and Adaptability of Guardrails
When enforcing LLM guardrails, ensuring manageability and flexibility is critical. You need to design guardrails that can manage the gradual loads of data and communications without yielding performance. This involves using adjustable and adaptable architecture.
By engaging microservices, you can easily scale distinct elements solely. In addition, contemplate using machine learning methods to constantly update and process guardrails based on new data. For instance, if you are operating with a consumer service chatbot, scalable guardrails ensure that as the number of users evolves, the system persists to execute rules efficiently without slowing down.
Addressing Common Implementation Challenges
Enforcing LLM guardrails comes with numerous challenges. One common problem is compensating the rigidity of the guardrails with the instability of natural language.
Overly strict guardrails can choke the AIs capability to produce natural, engaging responses. To acknowledge this, use a hybrid approach that merges rule-based systems with machine learning models.
For example, while rigid rules might be significant to avoid precise detrimental behaviors, machine learning models can offer more subtle moderation. Another challenge is the incorporation of these guardrails into existing systems.
Here, pragmatic testing and repetitive evolution are key. Begin with a small pilot, collect responses, and process the guardrails before full-scale disperse.
Maintaining User Experience While Enforcing Strict Guardrails
One of the peskiest parts of enforcing LLM guardrails is handling a positive user experience. Users anticipate fluid, natural communications, which can be disorganized by overly limited guardrails. To smack a balance, enforce flexible guardrails that can adapt based on context.
For example, a communicative agent might need to be more compassionate in a casual conversation but scrupulous in a professional setting. In addition, provide users with clarity. If a guardrail blocks an action, elucidate why in a friendly manner and recommend alternatives. This not only maintains trust but also improves user satisfaction.
Future-Proofing Guardrails Amidst Evolving AI Capabilities and Regulatory Landscapes
The AI prospect is swiftly progressing, and so are regulations ruling its utilization. To future-proof your guardrails, you need to assimilate a proactive approach. Stay informed about the newest evolvements in AI technology and executive alterations.
Design your guardrails to be easily upgradeable, permitting you to integrate new rules and standards as they appear. In addition, contemplate the possibility for new kinds of interactions and data.
For instance, as multimodal AI systems become more common, your guardrails should be able to manage texts, images and even video content. Frequently retrospect and upgrade your guardrails to ensure they remain efficient and compliant with the newest instructions.
Also Read:- Multimodal LLMS Using Image And Text
Conclusion
Building and enforcing custom LLM guardrails is significant for handling the incorporation and safety of AI communications. These guardrails not only avert detrimental or unsuitable behavior but also ensure that the AI controls within the wanted boundaries, offering users with a compatible and trustworthy experience.
Custom guardrails play a crucial role in shaping the future of ethical and liable AI. AI executing transparent instructions and standards, they help alleviate threats associated with AI utilization, like bias, false details and privacy concerns. This nurtures a secure and more dependable AI ecosystem, aiding both users and developers.
Delve into the future of AI-powered communication. Locate how our advanced language models can revolutionize your venture, smooth workflow, and improve customer experience.
Get Started Today!
Discover RagaAI LLM Hub and see the distinctiveness innovative AI can make. Don’t miss out on the transformation.
Join now and Boost your AI capabilities!
Ever tried herding cats? Now imagine doing that with AI – that's where LLM guardrails come into play!
Custom guardrails in Large Language Models (LLMs) are precise rules and structures that control the yields these advanced AI systems generate. These guardrails ensure that the retaliations and actions of an LLM correspond with desired moral, legitimate and contextual standards.
The predominant purpose of custom guardrails is to avert harmful or unsuitable yields, thus protecting users and investors from possible threats associated with AI-produced content.
So, how do we even begin to set up these guardrails in LLMs? Let’s know through this guide!
Types of Custom Guardrails for LLMs
Compliance Guardrails: Aligning Outputs with Legal Standards
Compliance guardrails ensure that the yields of an LLM follow pertinent laws and regulations. For instance, in the financial industry, you can design guardrails to avert the LLM from making unauthorized investment suggestions or revealing sensitive financial information. These guardrails help safeguard your organization from legitimate consequences and maintain compliance with industry norms.
Ethical considerations are next on the agenda, but trust me, it's more intriguing than it sounds!
Ethical Guardrails: Ensuring Outputs Meet Ethical Guidelines
Ethical guardrails are designed to align LLM yields with pioneered ethical principles. This includes averting biased, unfair or abusive language. For example, an LLM in employment should be shaped to avoid gender-biased language when assessing candidates. By enforcing ethical guardrails, you can promote a more comprehensive and respectful AI environment.
Now, let’s dive into the quirks and nuances of context-specific guardrails. Hang tight; it’s going to be insightful!
Contextual Guardrails: Tailoring Responses to Specific Contexts or Domains
Contextual guardrails customize the LLMs responses to fit precise contexts or domains. For instance, an LLM used in customer service for a technology firm should give precise and pertinent technical support information, rather than general knowledge or dissimilar guidance. These guardrails ensure that the AI’s yields are pertinent and beneficial to the deliberated audience.
Next up, let’s talk about security—because nobody wants their AI to turn into a cyber nincompoop.
Security Guardrails: Safeguarding Against Vulnerabilities and Threats
Security Guardrails safeguard against possible vulnerabilities and risks, like data infringement or unauthorized access. For example, in an LLM used for commercial interactions, guardrails can be set to avert the revelation of sensitive information or to filter out counterfeit efforts. These security measures are important for safeguarding both the AI systems and its users from cyber attacks.
Did someone say adaptability? Let’s get into how these guardrails can evolve and keep your AI sharp.
Adaptive Guardrails: Evolving with Changing Standards and Regulations
Adaptive guardrails are designed to develop and update in feedback to changing norms and regulations. This is specifically significant in industries with swiftly altering compliance needs. For instance, in the healthcare sector, adaptive guardrails can be updated to reflect new patient seclusion laws and medical guidelines. By enforcing adaptive guardrails, you ensure that your AI system stays compliant and efficient over time.
Ready to locate the best guardrails for your requirements? Delve into our thorough comparison of the leading large language models (LLMs). Comprehend their capabilities, powers and inventive attributes to make an informed choice.
Alright, now that we have discussed the various types of guardrails, let’s switch gears and get into the actual design process.
Designing Custom Guardrails
Analyzing Requirements: Identifying Specific Needs and Risks
Dissect the precise requirements and threats associated with your application as the initial step in designing custom guardrails.
This indulges in determining possible areas where the LLMs yields could be detrimental and non-compliant. For instance, if you are developing an LLM for informative purposes, you need to ensure it offers precise and age-accurate content. Conducting a pragmatic risk assessment helps you distinguish the crucial areas where guardrails are required.
Construction Methodologies: From Policy Enforcement to Continuous Adaptability
Once you have determined the needs, you can start creating the guardrails. This involves creating strategies and rules that the LLM must adhere to. For example, you might execute policies that limit the utilization of assured language and need fact-checking before data is given. Also, design guardrails that can adjust over time, allowing constant enhancement and updating as new threats and standards arise.
Technical Considerations: Balancing Accuracy, Latency, and System Integrations
When designing guardrails, it's important to contemplate the technical elements, like precision, suspension and system incorporations.
Ensure precision so that the guardrails efficiently filter unsuitable content without hampering the LLM’s performance. Latency involves minimizing detentions caused by guardrail checks, ensuring a sleek user experience. In addition, incorporating guardrails with existing systems and productivity is critical for streamlined functioning.
Examples of Custom Guardrail Designs for Diverse Industry Needs
When it comes to ensuring security in numerous industries, having the right guardrails in place is important. Customize custom guardrails to precise requirements to improve security and offer a sleek fit for a distinct environment. Let’s discover some instances of custom guardrail design that serve disparate industry needs, concentrating on how LLM guardrails can make a substantial impact.
Warehouse and Industry Guardrails
In occupied warehouses and industry settings, disaster can happen in the blink of an eye. Custom guardrails here are created to resist heavy impacts from freight elevators and other machinery. They can be customized to fit around machinery, safeguard climbers, walkways and separate work areas. Envision installing LLM guardrails in your warehouse; they not only provide sturdy security but also ensure that outline is effective and secure for all workers.
Parking Lot Guardrails
Parking lots often see a lot of automotive traffic, and guardrails play a pivotal role in averting accidents. Custom guardrails for parking lots are created to guide the flow of traffic, safeguard climbers, and avert cars from collisions into structures. By using LLM guardrails, you can create a secure and arranged parking area that curtails the risk of accidents and improves the overall user experience.
Public Park and Recreational Area Guardrails
Public parks and recreational areas need guardrails that amalgamate with the environment while ensuring security. Custom guardrails in these settings are often designed with elegance in mind, utilizing materials and colors that adjunct with natural surroundings. With LLM guardrails, you can accomplish a perfect balance of security and beauty, making the park a delightful place for everyone to relish.
Hospital and Healthcare Facility Guardrails
In hospitals and healthcare facilities, safety is the highest priority. Custom guardrails here are designed to safeguard patients, staff and guests from possible dangers. Often install them in corridors, around medical apparatus, and in parking areas. Use LLM guardrails to offer significant safety while making them easy to clean and maintain, which is significant in a healthcare environment.
Commercial Building Guardrails
Commercial buildings, indulging offices and shopping malls, benefit from custom guardrails that improve security without yielding on aesthetics. These guardrails are designed to amalgamate flawlessly with the building’s architecture while offering sturdy protection. Choose LLM guardrails to ensure that your commercial space is both secure and tangibly fascinating, thereby improving the overall experience for employees and guests.
Construction Site Guardrails
Construction sites are fundamentally risky, and having the right guardrails is significant to safeguard workers and visitors. Design custom guardrails for construction sites to be delicate and adjustable to altering site conditions. LLM guardrails can be personalized to meet precise safety norms and regulations, ensuring that your site stays compliant and secure.
Select custom LLM guardrails for your precise industry requirements to ensure maximum security and effectiveness. These instances embellish how customized fixes can make a substantial difference in numerous environments, giving peace of mind and protection where it’s required the most.
The release of RagaAI’s thorough assessment and guardrail platform marks a substantial progression in the field of large language models (LLMs). By acknowledging the crucial requirement for averting catastrophic setbacks, this platform sets a new norm for security and dependability in Artificial Intelligence applications. As the AI prospect persists to develop, tools such as RagaAI’s platform will be important in ensuring that these influential technologies can be deployed responsibly and efficiently.
Find how RagaAI’s platform can protect your AI enforcements. Read more here.
Implementing Custom Guardrails in LLM Systems
Preprocessing Inputs: Techniques for Input Validation and Sanitization
Before your LLM refines any input, it’s critical to verify and sanitize the data to avert vicious or detrimental content. Enforce input verification by inspecting for predefined formats, adequate characters, and coherent consistency. For instance, if your LLM is designed to manage email queries, ensure that the input adheres to email syntax and circumvents possibly dangerous scripts.
Sanitize by cleaning the input to extract and compensate for possibly detrimental components. Utilize libraries such as OWASP for Python or DOMPurify for Javascript to clear out vicious code from user inputs. This step safeguards your system and improves the quality of the output by ensuring the LLM receives clean and pertinent data.
Real-time Output Filtering: Strategies for Moderating and Fact-checking LLM Outputs
Real-time output filtering is significant for handling the incorporation and safety of LLM yields. Enforce moderation tools that dissect the LLMs responses for harmful, partial, or unsuitable content. Methods like keyword extracting, opinion analysis, and machine learning codification can help discover and alleviate troublesome outputs.
Rectification is another crucial aspect. Enforce real-time fact-checking APIs, like those Factmata and Full Fact offer, to validate the data the LLM generates. This ensures that the responses are not only secure but also precise and steadfast.
User Engagement and Feedback Mechanisms: Enabling Continuous Improvement
Engage users and collect responses for constant enhancement of your LLM system. Enforce feedback mechanisms that permit users to appraise feedback, flag problems and write comments. Tools such as user surveys, in-app feedback forms, and communicative prompts can facilitate this procedure.
Inspecting user responses helps determine common problems and areas for improvement. Use this information to fine-tune your LLM, update training datasets, and adapt algorithms. Constant learning from user interactions ensures that your LLM evolves to meet user requirements more efficiently.
Integration with LLM APIs and Platforms: Practical Implementation Steps
Incorporate system guardrails with LLM APIs and the platform using a standard approach. Start by choosing a suitable LLM platform, like OpenAI or HuggingFace, that aids the incorporation of custom guardrails. Acquaint yourself with the platform’s API documentation and capabilities.
Develop middleware that connects the user inputs and the guardrail mechanisms. This middleware should manage input predigestion, real-time output refining, and response gathering smoothly. For instance, use Flask or Express.js to make an API layer that handles these processes.
In the end, examine your incorporated system considerably to ensure the guardrails function as aimed without shuffling the user experience. Conduct both computerized and manual testing to cover a wide range of synopsis and edge cases.
By executing these strategies, you can create a sturdy LLM system that delivers dependable, precise and secure outputs, improving user trust and experience.
Monitoring and Evaluating Guardrail Effectiveness
Setting Up Metrics and KPIs for Guardrail Performance
When you set up metrics and Key Performance Indicators (KPIs) for your guardrails, you’re pioneering a clear structure to gauge their success. Begin by determining the predominant intentions of your guardrails. Are they designed to improve security, enhance functional effectiveness, or ensure docility with regulations.
Contemplate using metrics like the number of safety incidents averted, the regularity of guardrail activations, and the abatement in functional disruptions. KPIs might indulge the probability curtailed in disasters, the response duration to incidents, and user docility rates. For instance, if your guardrails are in place to curtail data infringement, a substantial KPI would be the abatement in unauthorized access efforts.
Tools and Techniques for Monitoring Guardrail Effectiveness
Observing the efficiency of your guardrails needs the right tools and methods. Enforce real-time monitoring systems that can trace guardrail initiations and provide instantaneous vigilantism. Tools such as Splunk or ELK stack can collect and dissect log data, providing perceptions into guardrail performance.
Utilize machine learning models to forecast possible failures or infringements and use dashboards to visualize performance metrics. Use these tools to comprehend trends and irregularities, thereby permitting energetic adaptations. In addition, frequent audits and docility checks ensure that guardrails stay efficient over time.
Adjusting Guardrails Based on Feedback and Performance Data
Adapt guardrails based on response and performance data to maintain their efficiency. Gather data from several sources, indulging system logs, user response and accident reports. Dissect this data to discover motifs and areas to enhance.
For example, if you observe a high prevalence of erroneous positives, fine-tune the perceptivity of your guardrails. Engage with investors to collect instinctive responses and ensure the adaptations align with their requirements. Frequently overhaul your guardrails to adjust to new risks and functional alterations to ensure they stay pertinent and efficient.
Case Studies on the Impact of Custom Guardrails in Real-World Applications
Let’s now discover some real-world instances to comprehend the impact of custom guardrails. In the financial sector, a chief bank enforced custom guardrails to avert deceptive transactions. By setting up precise KPIs and utilizing advanced observing tools, they curtail deceit attempts by 30% within the first 6 months.
Another instance is a healthcare supplier that used custom guardrails to safeguard the patient records. They observed data access motifs and adapted their guardrails based on user behavior This approach leads to 40% decrease in data infringement and enhanced patient trust.
In both cases, the amalgamation of well-defined metrics, efficient monitoring tools, and versatile adaptations based on performance data substantially improved the efficiency of the guardrails.
Ready to boost the security and dependability of your enterprise LLM applications? Explore how RagaAI’s advanced guardrails can protect your data and upgrade performance. Contact us today to learn more about enforcing sturdy solutions to your venture requirements. Secure your future with RagaAI!
Best Practices and Challenges Of Guardrails
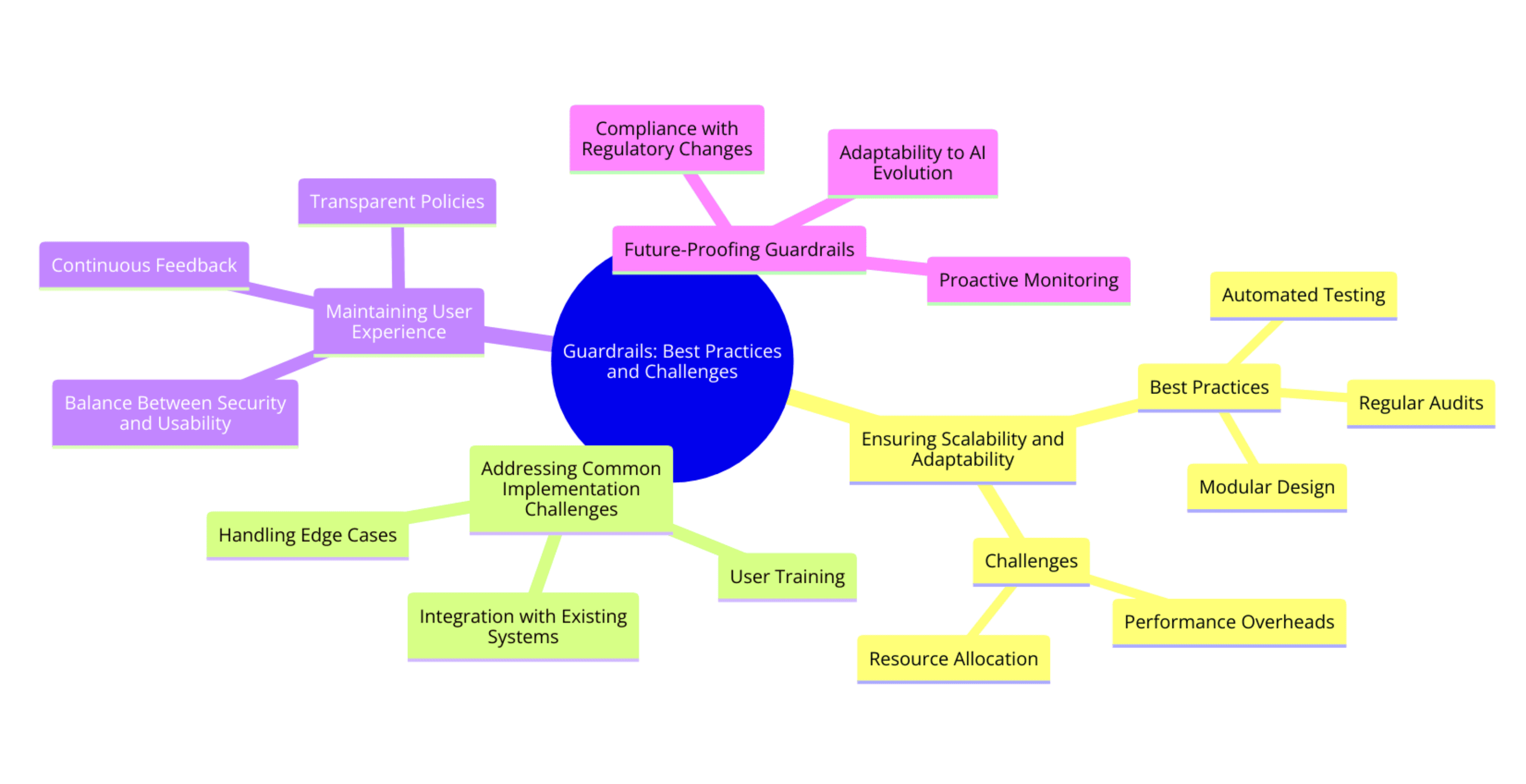
Ensuring Scalability and Adaptability of Guardrails
When enforcing LLM guardrails, ensuring manageability and flexibility is critical. You need to design guardrails that can manage the gradual loads of data and communications without yielding performance. This involves using adjustable and adaptable architecture.
By engaging microservices, you can easily scale distinct elements solely. In addition, contemplate using machine learning methods to constantly update and process guardrails based on new data. For instance, if you are operating with a consumer service chatbot, scalable guardrails ensure that as the number of users evolves, the system persists to execute rules efficiently without slowing down.
Addressing Common Implementation Challenges
Enforcing LLM guardrails comes with numerous challenges. One common problem is compensating the rigidity of the guardrails with the instability of natural language.
Overly strict guardrails can choke the AIs capability to produce natural, engaging responses. To acknowledge this, use a hybrid approach that merges rule-based systems with machine learning models.
For example, while rigid rules might be significant to avoid precise detrimental behaviors, machine learning models can offer more subtle moderation. Another challenge is the incorporation of these guardrails into existing systems.
Here, pragmatic testing and repetitive evolution are key. Begin with a small pilot, collect responses, and process the guardrails before full-scale disperse.
Maintaining User Experience While Enforcing Strict Guardrails
One of the peskiest parts of enforcing LLM guardrails is handling a positive user experience. Users anticipate fluid, natural communications, which can be disorganized by overly limited guardrails. To smack a balance, enforce flexible guardrails that can adapt based on context.
For example, a communicative agent might need to be more compassionate in a casual conversation but scrupulous in a professional setting. In addition, provide users with clarity. If a guardrail blocks an action, elucidate why in a friendly manner and recommend alternatives. This not only maintains trust but also improves user satisfaction.
Future-Proofing Guardrails Amidst Evolving AI Capabilities and Regulatory Landscapes
The AI prospect is swiftly progressing, and so are regulations ruling its utilization. To future-proof your guardrails, you need to assimilate a proactive approach. Stay informed about the newest evolvements in AI technology and executive alterations.
Design your guardrails to be easily upgradeable, permitting you to integrate new rules and standards as they appear. In addition, contemplate the possibility for new kinds of interactions and data.
For instance, as multimodal AI systems become more common, your guardrails should be able to manage texts, images and even video content. Frequently retrospect and upgrade your guardrails to ensure they remain efficient and compliant with the newest instructions.
Also Read:- Multimodal LLMS Using Image And Text
Conclusion
Building and enforcing custom LLM guardrails is significant for handling the incorporation and safety of AI communications. These guardrails not only avert detrimental or unsuitable behavior but also ensure that the AI controls within the wanted boundaries, offering users with a compatible and trustworthy experience.
Custom guardrails play a crucial role in shaping the future of ethical and liable AI. AI executing transparent instructions and standards, they help alleviate threats associated with AI utilization, like bias, false details and privacy concerns. This nurtures a secure and more dependable AI ecosystem, aiding both users and developers.
Delve into the future of AI-powered communication. Locate how our advanced language models can revolutionize your venture, smooth workflow, and improve customer experience.
Get Started Today!
Discover RagaAI LLM Hub and see the distinctiveness innovative AI can make. Don’t miss out on the transformation.
Join now and Boost your AI capabilities!
Ever tried herding cats? Now imagine doing that with AI – that's where LLM guardrails come into play!
Custom guardrails in Large Language Models (LLMs) are precise rules and structures that control the yields these advanced AI systems generate. These guardrails ensure that the retaliations and actions of an LLM correspond with desired moral, legitimate and contextual standards.
The predominant purpose of custom guardrails is to avert harmful or unsuitable yields, thus protecting users and investors from possible threats associated with AI-produced content.
So, how do we even begin to set up these guardrails in LLMs? Let’s know through this guide!
Types of Custom Guardrails for LLMs
Compliance Guardrails: Aligning Outputs with Legal Standards
Compliance guardrails ensure that the yields of an LLM follow pertinent laws and regulations. For instance, in the financial industry, you can design guardrails to avert the LLM from making unauthorized investment suggestions or revealing sensitive financial information. These guardrails help safeguard your organization from legitimate consequences and maintain compliance with industry norms.
Ethical considerations are next on the agenda, but trust me, it's more intriguing than it sounds!
Ethical Guardrails: Ensuring Outputs Meet Ethical Guidelines
Ethical guardrails are designed to align LLM yields with pioneered ethical principles. This includes averting biased, unfair or abusive language. For example, an LLM in employment should be shaped to avoid gender-biased language when assessing candidates. By enforcing ethical guardrails, you can promote a more comprehensive and respectful AI environment.
Now, let’s dive into the quirks and nuances of context-specific guardrails. Hang tight; it’s going to be insightful!
Contextual Guardrails: Tailoring Responses to Specific Contexts or Domains
Contextual guardrails customize the LLMs responses to fit precise contexts or domains. For instance, an LLM used in customer service for a technology firm should give precise and pertinent technical support information, rather than general knowledge or dissimilar guidance. These guardrails ensure that the AI’s yields are pertinent and beneficial to the deliberated audience.
Next up, let’s talk about security—because nobody wants their AI to turn into a cyber nincompoop.
Security Guardrails: Safeguarding Against Vulnerabilities and Threats
Security Guardrails safeguard against possible vulnerabilities and risks, like data infringement or unauthorized access. For example, in an LLM used for commercial interactions, guardrails can be set to avert the revelation of sensitive information or to filter out counterfeit efforts. These security measures are important for safeguarding both the AI systems and its users from cyber attacks.
Did someone say adaptability? Let’s get into how these guardrails can evolve and keep your AI sharp.
Adaptive Guardrails: Evolving with Changing Standards and Regulations
Adaptive guardrails are designed to develop and update in feedback to changing norms and regulations. This is specifically significant in industries with swiftly altering compliance needs. For instance, in the healthcare sector, adaptive guardrails can be updated to reflect new patient seclusion laws and medical guidelines. By enforcing adaptive guardrails, you ensure that your AI system stays compliant and efficient over time.
Ready to locate the best guardrails for your requirements? Delve into our thorough comparison of the leading large language models (LLMs). Comprehend their capabilities, powers and inventive attributes to make an informed choice.
Alright, now that we have discussed the various types of guardrails, let’s switch gears and get into the actual design process.
Designing Custom Guardrails
Analyzing Requirements: Identifying Specific Needs and Risks
Dissect the precise requirements and threats associated with your application as the initial step in designing custom guardrails.
This indulges in determining possible areas where the LLMs yields could be detrimental and non-compliant. For instance, if you are developing an LLM for informative purposes, you need to ensure it offers precise and age-accurate content. Conducting a pragmatic risk assessment helps you distinguish the crucial areas where guardrails are required.
Construction Methodologies: From Policy Enforcement to Continuous Adaptability
Once you have determined the needs, you can start creating the guardrails. This involves creating strategies and rules that the LLM must adhere to. For example, you might execute policies that limit the utilization of assured language and need fact-checking before data is given. Also, design guardrails that can adjust over time, allowing constant enhancement and updating as new threats and standards arise.
Technical Considerations: Balancing Accuracy, Latency, and System Integrations
When designing guardrails, it's important to contemplate the technical elements, like precision, suspension and system incorporations.
Ensure precision so that the guardrails efficiently filter unsuitable content without hampering the LLM’s performance. Latency involves minimizing detentions caused by guardrail checks, ensuring a sleek user experience. In addition, incorporating guardrails with existing systems and productivity is critical for streamlined functioning.
Examples of Custom Guardrail Designs for Diverse Industry Needs
When it comes to ensuring security in numerous industries, having the right guardrails in place is important. Customize custom guardrails to precise requirements to improve security and offer a sleek fit for a distinct environment. Let’s discover some instances of custom guardrail design that serve disparate industry needs, concentrating on how LLM guardrails can make a substantial impact.
Warehouse and Industry Guardrails
In occupied warehouses and industry settings, disaster can happen in the blink of an eye. Custom guardrails here are created to resist heavy impacts from freight elevators and other machinery. They can be customized to fit around machinery, safeguard climbers, walkways and separate work areas. Envision installing LLM guardrails in your warehouse; they not only provide sturdy security but also ensure that outline is effective and secure for all workers.
Parking Lot Guardrails
Parking lots often see a lot of automotive traffic, and guardrails play a pivotal role in averting accidents. Custom guardrails for parking lots are created to guide the flow of traffic, safeguard climbers, and avert cars from collisions into structures. By using LLM guardrails, you can create a secure and arranged parking area that curtails the risk of accidents and improves the overall user experience.
Public Park and Recreational Area Guardrails
Public parks and recreational areas need guardrails that amalgamate with the environment while ensuring security. Custom guardrails in these settings are often designed with elegance in mind, utilizing materials and colors that adjunct with natural surroundings. With LLM guardrails, you can accomplish a perfect balance of security and beauty, making the park a delightful place for everyone to relish.
Hospital and Healthcare Facility Guardrails
In hospitals and healthcare facilities, safety is the highest priority. Custom guardrails here are designed to safeguard patients, staff and guests from possible dangers. Often install them in corridors, around medical apparatus, and in parking areas. Use LLM guardrails to offer significant safety while making them easy to clean and maintain, which is significant in a healthcare environment.
Commercial Building Guardrails
Commercial buildings, indulging offices and shopping malls, benefit from custom guardrails that improve security without yielding on aesthetics. These guardrails are designed to amalgamate flawlessly with the building’s architecture while offering sturdy protection. Choose LLM guardrails to ensure that your commercial space is both secure and tangibly fascinating, thereby improving the overall experience for employees and guests.
Construction Site Guardrails
Construction sites are fundamentally risky, and having the right guardrails is significant to safeguard workers and visitors. Design custom guardrails for construction sites to be delicate and adjustable to altering site conditions. LLM guardrails can be personalized to meet precise safety norms and regulations, ensuring that your site stays compliant and secure.
Select custom LLM guardrails for your precise industry requirements to ensure maximum security and effectiveness. These instances embellish how customized fixes can make a substantial difference in numerous environments, giving peace of mind and protection where it’s required the most.
The release of RagaAI’s thorough assessment and guardrail platform marks a substantial progression in the field of large language models (LLMs). By acknowledging the crucial requirement for averting catastrophic setbacks, this platform sets a new norm for security and dependability in Artificial Intelligence applications. As the AI prospect persists to develop, tools such as RagaAI’s platform will be important in ensuring that these influential technologies can be deployed responsibly and efficiently.
Find how RagaAI’s platform can protect your AI enforcements. Read more here.
Implementing Custom Guardrails in LLM Systems
Preprocessing Inputs: Techniques for Input Validation and Sanitization
Before your LLM refines any input, it’s critical to verify and sanitize the data to avert vicious or detrimental content. Enforce input verification by inspecting for predefined formats, adequate characters, and coherent consistency. For instance, if your LLM is designed to manage email queries, ensure that the input adheres to email syntax and circumvents possibly dangerous scripts.
Sanitize by cleaning the input to extract and compensate for possibly detrimental components. Utilize libraries such as OWASP for Python or DOMPurify for Javascript to clear out vicious code from user inputs. This step safeguards your system and improves the quality of the output by ensuring the LLM receives clean and pertinent data.
Real-time Output Filtering: Strategies for Moderating and Fact-checking LLM Outputs
Real-time output filtering is significant for handling the incorporation and safety of LLM yields. Enforce moderation tools that dissect the LLMs responses for harmful, partial, or unsuitable content. Methods like keyword extracting, opinion analysis, and machine learning codification can help discover and alleviate troublesome outputs.
Rectification is another crucial aspect. Enforce real-time fact-checking APIs, like those Factmata and Full Fact offer, to validate the data the LLM generates. This ensures that the responses are not only secure but also precise and steadfast.
User Engagement and Feedback Mechanisms: Enabling Continuous Improvement
Engage users and collect responses for constant enhancement of your LLM system. Enforce feedback mechanisms that permit users to appraise feedback, flag problems and write comments. Tools such as user surveys, in-app feedback forms, and communicative prompts can facilitate this procedure.
Inspecting user responses helps determine common problems and areas for improvement. Use this information to fine-tune your LLM, update training datasets, and adapt algorithms. Constant learning from user interactions ensures that your LLM evolves to meet user requirements more efficiently.
Integration with LLM APIs and Platforms: Practical Implementation Steps
Incorporate system guardrails with LLM APIs and the platform using a standard approach. Start by choosing a suitable LLM platform, like OpenAI or HuggingFace, that aids the incorporation of custom guardrails. Acquaint yourself with the platform’s API documentation and capabilities.
Develop middleware that connects the user inputs and the guardrail mechanisms. This middleware should manage input predigestion, real-time output refining, and response gathering smoothly. For instance, use Flask or Express.js to make an API layer that handles these processes.
In the end, examine your incorporated system considerably to ensure the guardrails function as aimed without shuffling the user experience. Conduct both computerized and manual testing to cover a wide range of synopsis and edge cases.
By executing these strategies, you can create a sturdy LLM system that delivers dependable, precise and secure outputs, improving user trust and experience.
Monitoring and Evaluating Guardrail Effectiveness
Setting Up Metrics and KPIs for Guardrail Performance
When you set up metrics and Key Performance Indicators (KPIs) for your guardrails, you’re pioneering a clear structure to gauge their success. Begin by determining the predominant intentions of your guardrails. Are they designed to improve security, enhance functional effectiveness, or ensure docility with regulations.
Contemplate using metrics like the number of safety incidents averted, the regularity of guardrail activations, and the abatement in functional disruptions. KPIs might indulge the probability curtailed in disasters, the response duration to incidents, and user docility rates. For instance, if your guardrails are in place to curtail data infringement, a substantial KPI would be the abatement in unauthorized access efforts.
Tools and Techniques for Monitoring Guardrail Effectiveness
Observing the efficiency of your guardrails needs the right tools and methods. Enforce real-time monitoring systems that can trace guardrail initiations and provide instantaneous vigilantism. Tools such as Splunk or ELK stack can collect and dissect log data, providing perceptions into guardrail performance.
Utilize machine learning models to forecast possible failures or infringements and use dashboards to visualize performance metrics. Use these tools to comprehend trends and irregularities, thereby permitting energetic adaptations. In addition, frequent audits and docility checks ensure that guardrails stay efficient over time.
Adjusting Guardrails Based on Feedback and Performance Data
Adapt guardrails based on response and performance data to maintain their efficiency. Gather data from several sources, indulging system logs, user response and accident reports. Dissect this data to discover motifs and areas to enhance.
For example, if you observe a high prevalence of erroneous positives, fine-tune the perceptivity of your guardrails. Engage with investors to collect instinctive responses and ensure the adaptations align with their requirements. Frequently overhaul your guardrails to adjust to new risks and functional alterations to ensure they stay pertinent and efficient.
Case Studies on the Impact of Custom Guardrails in Real-World Applications
Let’s now discover some real-world instances to comprehend the impact of custom guardrails. In the financial sector, a chief bank enforced custom guardrails to avert deceptive transactions. By setting up precise KPIs and utilizing advanced observing tools, they curtail deceit attempts by 30% within the first 6 months.
Another instance is a healthcare supplier that used custom guardrails to safeguard the patient records. They observed data access motifs and adapted their guardrails based on user behavior This approach leads to 40% decrease in data infringement and enhanced patient trust.
In both cases, the amalgamation of well-defined metrics, efficient monitoring tools, and versatile adaptations based on performance data substantially improved the efficiency of the guardrails.
Ready to boost the security and dependability of your enterprise LLM applications? Explore how RagaAI’s advanced guardrails can protect your data and upgrade performance. Contact us today to learn more about enforcing sturdy solutions to your venture requirements. Secure your future with RagaAI!
Best Practices and Challenges Of Guardrails
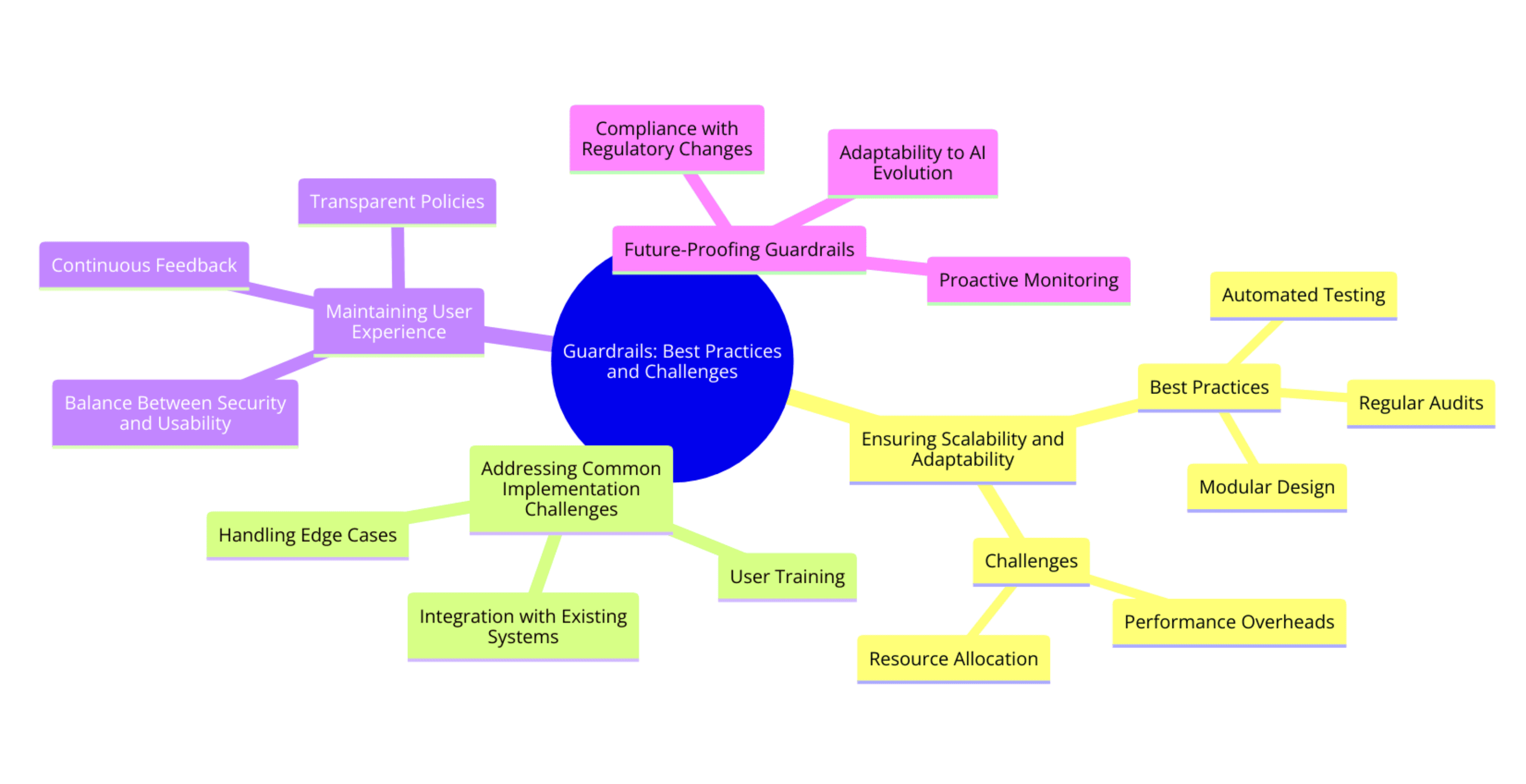
Ensuring Scalability and Adaptability of Guardrails
When enforcing LLM guardrails, ensuring manageability and flexibility is critical. You need to design guardrails that can manage the gradual loads of data and communications without yielding performance. This involves using adjustable and adaptable architecture.
By engaging microservices, you can easily scale distinct elements solely. In addition, contemplate using machine learning methods to constantly update and process guardrails based on new data. For instance, if you are operating with a consumer service chatbot, scalable guardrails ensure that as the number of users evolves, the system persists to execute rules efficiently without slowing down.
Addressing Common Implementation Challenges
Enforcing LLM guardrails comes with numerous challenges. One common problem is compensating the rigidity of the guardrails with the instability of natural language.
Overly strict guardrails can choke the AIs capability to produce natural, engaging responses. To acknowledge this, use a hybrid approach that merges rule-based systems with machine learning models.
For example, while rigid rules might be significant to avoid precise detrimental behaviors, machine learning models can offer more subtle moderation. Another challenge is the incorporation of these guardrails into existing systems.
Here, pragmatic testing and repetitive evolution are key. Begin with a small pilot, collect responses, and process the guardrails before full-scale disperse.
Maintaining User Experience While Enforcing Strict Guardrails
One of the peskiest parts of enforcing LLM guardrails is handling a positive user experience. Users anticipate fluid, natural communications, which can be disorganized by overly limited guardrails. To smack a balance, enforce flexible guardrails that can adapt based on context.
For example, a communicative agent might need to be more compassionate in a casual conversation but scrupulous in a professional setting. In addition, provide users with clarity. If a guardrail blocks an action, elucidate why in a friendly manner and recommend alternatives. This not only maintains trust but also improves user satisfaction.
Future-Proofing Guardrails Amidst Evolving AI Capabilities and Regulatory Landscapes
The AI prospect is swiftly progressing, and so are regulations ruling its utilization. To future-proof your guardrails, you need to assimilate a proactive approach. Stay informed about the newest evolvements in AI technology and executive alterations.
Design your guardrails to be easily upgradeable, permitting you to integrate new rules and standards as they appear. In addition, contemplate the possibility for new kinds of interactions and data.
For instance, as multimodal AI systems become more common, your guardrails should be able to manage texts, images and even video content. Frequently retrospect and upgrade your guardrails to ensure they remain efficient and compliant with the newest instructions.
Also Read:- Multimodal LLMS Using Image And Text
Conclusion
Building and enforcing custom LLM guardrails is significant for handling the incorporation and safety of AI communications. These guardrails not only avert detrimental or unsuitable behavior but also ensure that the AI controls within the wanted boundaries, offering users with a compatible and trustworthy experience.
Custom guardrails play a crucial role in shaping the future of ethical and liable AI. AI executing transparent instructions and standards, they help alleviate threats associated with AI utilization, like bias, false details and privacy concerns. This nurtures a secure and more dependable AI ecosystem, aiding both users and developers.
Delve into the future of AI-powered communication. Locate how our advanced language models can revolutionize your venture, smooth workflow, and improve customer experience.
Get Started Today!
Discover RagaAI LLM Hub and see the distinctiveness innovative AI can make. Don’t miss out on the transformation.
Join now and Boost your AI capabilities!
Ever tried herding cats? Now imagine doing that with AI – that's where LLM guardrails come into play!
Custom guardrails in Large Language Models (LLMs) are precise rules and structures that control the yields these advanced AI systems generate. These guardrails ensure that the retaliations and actions of an LLM correspond with desired moral, legitimate and contextual standards.
The predominant purpose of custom guardrails is to avert harmful or unsuitable yields, thus protecting users and investors from possible threats associated with AI-produced content.
So, how do we even begin to set up these guardrails in LLMs? Let’s know through this guide!
Types of Custom Guardrails for LLMs
Compliance Guardrails: Aligning Outputs with Legal Standards
Compliance guardrails ensure that the yields of an LLM follow pertinent laws and regulations. For instance, in the financial industry, you can design guardrails to avert the LLM from making unauthorized investment suggestions or revealing sensitive financial information. These guardrails help safeguard your organization from legitimate consequences and maintain compliance with industry norms.
Ethical considerations are next on the agenda, but trust me, it's more intriguing than it sounds!
Ethical Guardrails: Ensuring Outputs Meet Ethical Guidelines
Ethical guardrails are designed to align LLM yields with pioneered ethical principles. This includes averting biased, unfair or abusive language. For example, an LLM in employment should be shaped to avoid gender-biased language when assessing candidates. By enforcing ethical guardrails, you can promote a more comprehensive and respectful AI environment.
Now, let’s dive into the quirks and nuances of context-specific guardrails. Hang tight; it’s going to be insightful!
Contextual Guardrails: Tailoring Responses to Specific Contexts or Domains
Contextual guardrails customize the LLMs responses to fit precise contexts or domains. For instance, an LLM used in customer service for a technology firm should give precise and pertinent technical support information, rather than general knowledge or dissimilar guidance. These guardrails ensure that the AI’s yields are pertinent and beneficial to the deliberated audience.
Next up, let’s talk about security—because nobody wants their AI to turn into a cyber nincompoop.
Security Guardrails: Safeguarding Against Vulnerabilities and Threats
Security Guardrails safeguard against possible vulnerabilities and risks, like data infringement or unauthorized access. For example, in an LLM used for commercial interactions, guardrails can be set to avert the revelation of sensitive information or to filter out counterfeit efforts. These security measures are important for safeguarding both the AI systems and its users from cyber attacks.
Did someone say adaptability? Let’s get into how these guardrails can evolve and keep your AI sharp.
Adaptive Guardrails: Evolving with Changing Standards and Regulations
Adaptive guardrails are designed to develop and update in feedback to changing norms and regulations. This is specifically significant in industries with swiftly altering compliance needs. For instance, in the healthcare sector, adaptive guardrails can be updated to reflect new patient seclusion laws and medical guidelines. By enforcing adaptive guardrails, you ensure that your AI system stays compliant and efficient over time.
Ready to locate the best guardrails for your requirements? Delve into our thorough comparison of the leading large language models (LLMs). Comprehend their capabilities, powers and inventive attributes to make an informed choice.
Alright, now that we have discussed the various types of guardrails, let’s switch gears and get into the actual design process.
Designing Custom Guardrails
Analyzing Requirements: Identifying Specific Needs and Risks
Dissect the precise requirements and threats associated with your application as the initial step in designing custom guardrails.
This indulges in determining possible areas where the LLMs yields could be detrimental and non-compliant. For instance, if you are developing an LLM for informative purposes, you need to ensure it offers precise and age-accurate content. Conducting a pragmatic risk assessment helps you distinguish the crucial areas where guardrails are required.
Construction Methodologies: From Policy Enforcement to Continuous Adaptability
Once you have determined the needs, you can start creating the guardrails. This involves creating strategies and rules that the LLM must adhere to. For example, you might execute policies that limit the utilization of assured language and need fact-checking before data is given. Also, design guardrails that can adjust over time, allowing constant enhancement and updating as new threats and standards arise.
Technical Considerations: Balancing Accuracy, Latency, and System Integrations
When designing guardrails, it's important to contemplate the technical elements, like precision, suspension and system incorporations.
Ensure precision so that the guardrails efficiently filter unsuitable content without hampering the LLM’s performance. Latency involves minimizing detentions caused by guardrail checks, ensuring a sleek user experience. In addition, incorporating guardrails with existing systems and productivity is critical for streamlined functioning.
Examples of Custom Guardrail Designs for Diverse Industry Needs
When it comes to ensuring security in numerous industries, having the right guardrails in place is important. Customize custom guardrails to precise requirements to improve security and offer a sleek fit for a distinct environment. Let’s discover some instances of custom guardrail design that serve disparate industry needs, concentrating on how LLM guardrails can make a substantial impact.
Warehouse and Industry Guardrails
In occupied warehouses and industry settings, disaster can happen in the blink of an eye. Custom guardrails here are created to resist heavy impacts from freight elevators and other machinery. They can be customized to fit around machinery, safeguard climbers, walkways and separate work areas. Envision installing LLM guardrails in your warehouse; they not only provide sturdy security but also ensure that outline is effective and secure for all workers.
Parking Lot Guardrails
Parking lots often see a lot of automotive traffic, and guardrails play a pivotal role in averting accidents. Custom guardrails for parking lots are created to guide the flow of traffic, safeguard climbers, and avert cars from collisions into structures. By using LLM guardrails, you can create a secure and arranged parking area that curtails the risk of accidents and improves the overall user experience.
Public Park and Recreational Area Guardrails
Public parks and recreational areas need guardrails that amalgamate with the environment while ensuring security. Custom guardrails in these settings are often designed with elegance in mind, utilizing materials and colors that adjunct with natural surroundings. With LLM guardrails, you can accomplish a perfect balance of security and beauty, making the park a delightful place for everyone to relish.
Hospital and Healthcare Facility Guardrails
In hospitals and healthcare facilities, safety is the highest priority. Custom guardrails here are designed to safeguard patients, staff and guests from possible dangers. Often install them in corridors, around medical apparatus, and in parking areas. Use LLM guardrails to offer significant safety while making them easy to clean and maintain, which is significant in a healthcare environment.
Commercial Building Guardrails
Commercial buildings, indulging offices and shopping malls, benefit from custom guardrails that improve security without yielding on aesthetics. These guardrails are designed to amalgamate flawlessly with the building’s architecture while offering sturdy protection. Choose LLM guardrails to ensure that your commercial space is both secure and tangibly fascinating, thereby improving the overall experience for employees and guests.
Construction Site Guardrails
Construction sites are fundamentally risky, and having the right guardrails is significant to safeguard workers and visitors. Design custom guardrails for construction sites to be delicate and adjustable to altering site conditions. LLM guardrails can be personalized to meet precise safety norms and regulations, ensuring that your site stays compliant and secure.
Select custom LLM guardrails for your precise industry requirements to ensure maximum security and effectiveness. These instances embellish how customized fixes can make a substantial difference in numerous environments, giving peace of mind and protection where it’s required the most.
The release of RagaAI’s thorough assessment and guardrail platform marks a substantial progression in the field of large language models (LLMs). By acknowledging the crucial requirement for averting catastrophic setbacks, this platform sets a new norm for security and dependability in Artificial Intelligence applications. As the AI prospect persists to develop, tools such as RagaAI’s platform will be important in ensuring that these influential technologies can be deployed responsibly and efficiently.
Find how RagaAI’s platform can protect your AI enforcements. Read more here.
Implementing Custom Guardrails in LLM Systems
Preprocessing Inputs: Techniques for Input Validation and Sanitization
Before your LLM refines any input, it’s critical to verify and sanitize the data to avert vicious or detrimental content. Enforce input verification by inspecting for predefined formats, adequate characters, and coherent consistency. For instance, if your LLM is designed to manage email queries, ensure that the input adheres to email syntax and circumvents possibly dangerous scripts.
Sanitize by cleaning the input to extract and compensate for possibly detrimental components. Utilize libraries such as OWASP for Python or DOMPurify for Javascript to clear out vicious code from user inputs. This step safeguards your system and improves the quality of the output by ensuring the LLM receives clean and pertinent data.
Real-time Output Filtering: Strategies for Moderating and Fact-checking LLM Outputs
Real-time output filtering is significant for handling the incorporation and safety of LLM yields. Enforce moderation tools that dissect the LLMs responses for harmful, partial, or unsuitable content. Methods like keyword extracting, opinion analysis, and machine learning codification can help discover and alleviate troublesome outputs.
Rectification is another crucial aspect. Enforce real-time fact-checking APIs, like those Factmata and Full Fact offer, to validate the data the LLM generates. This ensures that the responses are not only secure but also precise and steadfast.
User Engagement and Feedback Mechanisms: Enabling Continuous Improvement
Engage users and collect responses for constant enhancement of your LLM system. Enforce feedback mechanisms that permit users to appraise feedback, flag problems and write comments. Tools such as user surveys, in-app feedback forms, and communicative prompts can facilitate this procedure.
Inspecting user responses helps determine common problems and areas for improvement. Use this information to fine-tune your LLM, update training datasets, and adapt algorithms. Constant learning from user interactions ensures that your LLM evolves to meet user requirements more efficiently.
Integration with LLM APIs and Platforms: Practical Implementation Steps
Incorporate system guardrails with LLM APIs and the platform using a standard approach. Start by choosing a suitable LLM platform, like OpenAI or HuggingFace, that aids the incorporation of custom guardrails. Acquaint yourself with the platform’s API documentation and capabilities.
Develop middleware that connects the user inputs and the guardrail mechanisms. This middleware should manage input predigestion, real-time output refining, and response gathering smoothly. For instance, use Flask or Express.js to make an API layer that handles these processes.
In the end, examine your incorporated system considerably to ensure the guardrails function as aimed without shuffling the user experience. Conduct both computerized and manual testing to cover a wide range of synopsis and edge cases.
By executing these strategies, you can create a sturdy LLM system that delivers dependable, precise and secure outputs, improving user trust and experience.
Monitoring and Evaluating Guardrail Effectiveness
Setting Up Metrics and KPIs for Guardrail Performance
When you set up metrics and Key Performance Indicators (KPIs) for your guardrails, you’re pioneering a clear structure to gauge their success. Begin by determining the predominant intentions of your guardrails. Are they designed to improve security, enhance functional effectiveness, or ensure docility with regulations.
Contemplate using metrics like the number of safety incidents averted, the regularity of guardrail activations, and the abatement in functional disruptions. KPIs might indulge the probability curtailed in disasters, the response duration to incidents, and user docility rates. For instance, if your guardrails are in place to curtail data infringement, a substantial KPI would be the abatement in unauthorized access efforts.
Tools and Techniques for Monitoring Guardrail Effectiveness
Observing the efficiency of your guardrails needs the right tools and methods. Enforce real-time monitoring systems that can trace guardrail initiations and provide instantaneous vigilantism. Tools such as Splunk or ELK stack can collect and dissect log data, providing perceptions into guardrail performance.
Utilize machine learning models to forecast possible failures or infringements and use dashboards to visualize performance metrics. Use these tools to comprehend trends and irregularities, thereby permitting energetic adaptations. In addition, frequent audits and docility checks ensure that guardrails stay efficient over time.
Adjusting Guardrails Based on Feedback and Performance Data
Adapt guardrails based on response and performance data to maintain their efficiency. Gather data from several sources, indulging system logs, user response and accident reports. Dissect this data to discover motifs and areas to enhance.
For example, if you observe a high prevalence of erroneous positives, fine-tune the perceptivity of your guardrails. Engage with investors to collect instinctive responses and ensure the adaptations align with their requirements. Frequently overhaul your guardrails to adjust to new risks and functional alterations to ensure they stay pertinent and efficient.
Case Studies on the Impact of Custom Guardrails in Real-World Applications
Let’s now discover some real-world instances to comprehend the impact of custom guardrails. In the financial sector, a chief bank enforced custom guardrails to avert deceptive transactions. By setting up precise KPIs and utilizing advanced observing tools, they curtail deceit attempts by 30% within the first 6 months.
Another instance is a healthcare supplier that used custom guardrails to safeguard the patient records. They observed data access motifs and adapted their guardrails based on user behavior This approach leads to 40% decrease in data infringement and enhanced patient trust.
In both cases, the amalgamation of well-defined metrics, efficient monitoring tools, and versatile adaptations based on performance data substantially improved the efficiency of the guardrails.
Ready to boost the security and dependability of your enterprise LLM applications? Explore how RagaAI’s advanced guardrails can protect your data and upgrade performance. Contact us today to learn more about enforcing sturdy solutions to your venture requirements. Secure your future with RagaAI!
Best Practices and Challenges Of Guardrails
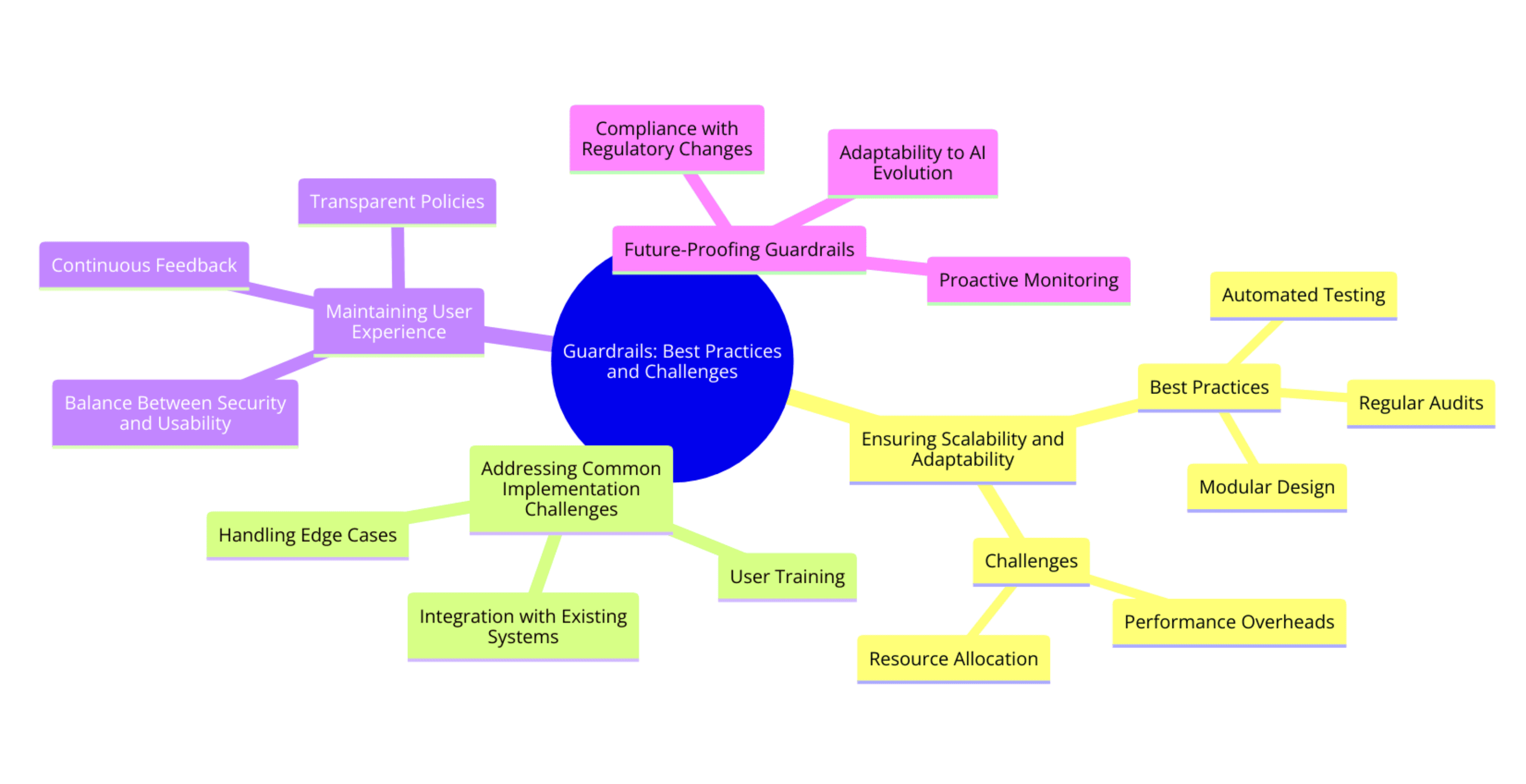
Ensuring Scalability and Adaptability of Guardrails
When enforcing LLM guardrails, ensuring manageability and flexibility is critical. You need to design guardrails that can manage the gradual loads of data and communications without yielding performance. This involves using adjustable and adaptable architecture.
By engaging microservices, you can easily scale distinct elements solely. In addition, contemplate using machine learning methods to constantly update and process guardrails based on new data. For instance, if you are operating with a consumer service chatbot, scalable guardrails ensure that as the number of users evolves, the system persists to execute rules efficiently without slowing down.
Addressing Common Implementation Challenges
Enforcing LLM guardrails comes with numerous challenges. One common problem is compensating the rigidity of the guardrails with the instability of natural language.
Overly strict guardrails can choke the AIs capability to produce natural, engaging responses. To acknowledge this, use a hybrid approach that merges rule-based systems with machine learning models.
For example, while rigid rules might be significant to avoid precise detrimental behaviors, machine learning models can offer more subtle moderation. Another challenge is the incorporation of these guardrails into existing systems.
Here, pragmatic testing and repetitive evolution are key. Begin with a small pilot, collect responses, and process the guardrails before full-scale disperse.
Maintaining User Experience While Enforcing Strict Guardrails
One of the peskiest parts of enforcing LLM guardrails is handling a positive user experience. Users anticipate fluid, natural communications, which can be disorganized by overly limited guardrails. To smack a balance, enforce flexible guardrails that can adapt based on context.
For example, a communicative agent might need to be more compassionate in a casual conversation but scrupulous in a professional setting. In addition, provide users with clarity. If a guardrail blocks an action, elucidate why in a friendly manner and recommend alternatives. This not only maintains trust but also improves user satisfaction.
Future-Proofing Guardrails Amidst Evolving AI Capabilities and Regulatory Landscapes
The AI prospect is swiftly progressing, and so are regulations ruling its utilization. To future-proof your guardrails, you need to assimilate a proactive approach. Stay informed about the newest evolvements in AI technology and executive alterations.
Design your guardrails to be easily upgradeable, permitting you to integrate new rules and standards as they appear. In addition, contemplate the possibility for new kinds of interactions and data.
For instance, as multimodal AI systems become more common, your guardrails should be able to manage texts, images and even video content. Frequently retrospect and upgrade your guardrails to ensure they remain efficient and compliant with the newest instructions.
Also Read:- Multimodal LLMS Using Image And Text
Conclusion
Building and enforcing custom LLM guardrails is significant for handling the incorporation and safety of AI communications. These guardrails not only avert detrimental or unsuitable behavior but also ensure that the AI controls within the wanted boundaries, offering users with a compatible and trustworthy experience.
Custom guardrails play a crucial role in shaping the future of ethical and liable AI. AI executing transparent instructions and standards, they help alleviate threats associated with AI utilization, like bias, false details and privacy concerns. This nurtures a secure and more dependable AI ecosystem, aiding both users and developers.
Delve into the future of AI-powered communication. Locate how our advanced language models can revolutionize your venture, smooth workflow, and improve customer experience.
Get Started Today!
Discover RagaAI LLM Hub and see the distinctiveness innovative AI can make. Don’t miss out on the transformation.
Join now and Boost your AI capabilities!
Ever tried herding cats? Now imagine doing that with AI – that's where LLM guardrails come into play!
Custom guardrails in Large Language Models (LLMs) are precise rules and structures that control the yields these advanced AI systems generate. These guardrails ensure that the retaliations and actions of an LLM correspond with desired moral, legitimate and contextual standards.
The predominant purpose of custom guardrails is to avert harmful or unsuitable yields, thus protecting users and investors from possible threats associated with AI-produced content.
So, how do we even begin to set up these guardrails in LLMs? Let’s know through this guide!
Types of Custom Guardrails for LLMs
Compliance Guardrails: Aligning Outputs with Legal Standards
Compliance guardrails ensure that the yields of an LLM follow pertinent laws and regulations. For instance, in the financial industry, you can design guardrails to avert the LLM from making unauthorized investment suggestions or revealing sensitive financial information. These guardrails help safeguard your organization from legitimate consequences and maintain compliance with industry norms.
Ethical considerations are next on the agenda, but trust me, it's more intriguing than it sounds!
Ethical Guardrails: Ensuring Outputs Meet Ethical Guidelines
Ethical guardrails are designed to align LLM yields with pioneered ethical principles. This includes averting biased, unfair or abusive language. For example, an LLM in employment should be shaped to avoid gender-biased language when assessing candidates. By enforcing ethical guardrails, you can promote a more comprehensive and respectful AI environment.
Now, let’s dive into the quirks and nuances of context-specific guardrails. Hang tight; it’s going to be insightful!
Contextual Guardrails: Tailoring Responses to Specific Contexts or Domains
Contextual guardrails customize the LLMs responses to fit precise contexts or domains. For instance, an LLM used in customer service for a technology firm should give precise and pertinent technical support information, rather than general knowledge or dissimilar guidance. These guardrails ensure that the AI’s yields are pertinent and beneficial to the deliberated audience.
Next up, let’s talk about security—because nobody wants their AI to turn into a cyber nincompoop.
Security Guardrails: Safeguarding Against Vulnerabilities and Threats
Security Guardrails safeguard against possible vulnerabilities and risks, like data infringement or unauthorized access. For example, in an LLM used for commercial interactions, guardrails can be set to avert the revelation of sensitive information or to filter out counterfeit efforts. These security measures are important for safeguarding both the AI systems and its users from cyber attacks.
Did someone say adaptability? Let’s get into how these guardrails can evolve and keep your AI sharp.
Adaptive Guardrails: Evolving with Changing Standards and Regulations
Adaptive guardrails are designed to develop and update in feedback to changing norms and regulations. This is specifically significant in industries with swiftly altering compliance needs. For instance, in the healthcare sector, adaptive guardrails can be updated to reflect new patient seclusion laws and medical guidelines. By enforcing adaptive guardrails, you ensure that your AI system stays compliant and efficient over time.
Ready to locate the best guardrails for your requirements? Delve into our thorough comparison of the leading large language models (LLMs). Comprehend their capabilities, powers and inventive attributes to make an informed choice.
Alright, now that we have discussed the various types of guardrails, let’s switch gears and get into the actual design process.
Designing Custom Guardrails
Analyzing Requirements: Identifying Specific Needs and Risks
Dissect the precise requirements and threats associated with your application as the initial step in designing custom guardrails.
This indulges in determining possible areas where the LLMs yields could be detrimental and non-compliant. For instance, if you are developing an LLM for informative purposes, you need to ensure it offers precise and age-accurate content. Conducting a pragmatic risk assessment helps you distinguish the crucial areas where guardrails are required.
Construction Methodologies: From Policy Enforcement to Continuous Adaptability
Once you have determined the needs, you can start creating the guardrails. This involves creating strategies and rules that the LLM must adhere to. For example, you might execute policies that limit the utilization of assured language and need fact-checking before data is given. Also, design guardrails that can adjust over time, allowing constant enhancement and updating as new threats and standards arise.
Technical Considerations: Balancing Accuracy, Latency, and System Integrations
When designing guardrails, it's important to contemplate the technical elements, like precision, suspension and system incorporations.
Ensure precision so that the guardrails efficiently filter unsuitable content without hampering the LLM’s performance. Latency involves minimizing detentions caused by guardrail checks, ensuring a sleek user experience. In addition, incorporating guardrails with existing systems and productivity is critical for streamlined functioning.
Examples of Custom Guardrail Designs for Diverse Industry Needs
When it comes to ensuring security in numerous industries, having the right guardrails in place is important. Customize custom guardrails to precise requirements to improve security and offer a sleek fit for a distinct environment. Let’s discover some instances of custom guardrail design that serve disparate industry needs, concentrating on how LLM guardrails can make a substantial impact.
Warehouse and Industry Guardrails
In occupied warehouses and industry settings, disaster can happen in the blink of an eye. Custom guardrails here are created to resist heavy impacts from freight elevators and other machinery. They can be customized to fit around machinery, safeguard climbers, walkways and separate work areas. Envision installing LLM guardrails in your warehouse; they not only provide sturdy security but also ensure that outline is effective and secure for all workers.
Parking Lot Guardrails
Parking lots often see a lot of automotive traffic, and guardrails play a pivotal role in averting accidents. Custom guardrails for parking lots are created to guide the flow of traffic, safeguard climbers, and avert cars from collisions into structures. By using LLM guardrails, you can create a secure and arranged parking area that curtails the risk of accidents and improves the overall user experience.
Public Park and Recreational Area Guardrails
Public parks and recreational areas need guardrails that amalgamate with the environment while ensuring security. Custom guardrails in these settings are often designed with elegance in mind, utilizing materials and colors that adjunct with natural surroundings. With LLM guardrails, you can accomplish a perfect balance of security and beauty, making the park a delightful place for everyone to relish.
Hospital and Healthcare Facility Guardrails
In hospitals and healthcare facilities, safety is the highest priority. Custom guardrails here are designed to safeguard patients, staff and guests from possible dangers. Often install them in corridors, around medical apparatus, and in parking areas. Use LLM guardrails to offer significant safety while making them easy to clean and maintain, which is significant in a healthcare environment.
Commercial Building Guardrails
Commercial buildings, indulging offices and shopping malls, benefit from custom guardrails that improve security without yielding on aesthetics. These guardrails are designed to amalgamate flawlessly with the building’s architecture while offering sturdy protection. Choose LLM guardrails to ensure that your commercial space is both secure and tangibly fascinating, thereby improving the overall experience for employees and guests.
Construction Site Guardrails
Construction sites are fundamentally risky, and having the right guardrails is significant to safeguard workers and visitors. Design custom guardrails for construction sites to be delicate and adjustable to altering site conditions. LLM guardrails can be personalized to meet precise safety norms and regulations, ensuring that your site stays compliant and secure.
Select custom LLM guardrails for your precise industry requirements to ensure maximum security and effectiveness. These instances embellish how customized fixes can make a substantial difference in numerous environments, giving peace of mind and protection where it’s required the most.
The release of RagaAI’s thorough assessment and guardrail platform marks a substantial progression in the field of large language models (LLMs). By acknowledging the crucial requirement for averting catastrophic setbacks, this platform sets a new norm for security and dependability in Artificial Intelligence applications. As the AI prospect persists to develop, tools such as RagaAI’s platform will be important in ensuring that these influential technologies can be deployed responsibly and efficiently.
Find how RagaAI’s platform can protect your AI enforcements. Read more here.
Implementing Custom Guardrails in LLM Systems
Preprocessing Inputs: Techniques for Input Validation and Sanitization
Before your LLM refines any input, it’s critical to verify and sanitize the data to avert vicious or detrimental content. Enforce input verification by inspecting for predefined formats, adequate characters, and coherent consistency. For instance, if your LLM is designed to manage email queries, ensure that the input adheres to email syntax and circumvents possibly dangerous scripts.
Sanitize by cleaning the input to extract and compensate for possibly detrimental components. Utilize libraries such as OWASP for Python or DOMPurify for Javascript to clear out vicious code from user inputs. This step safeguards your system and improves the quality of the output by ensuring the LLM receives clean and pertinent data.
Real-time Output Filtering: Strategies for Moderating and Fact-checking LLM Outputs
Real-time output filtering is significant for handling the incorporation and safety of LLM yields. Enforce moderation tools that dissect the LLMs responses for harmful, partial, or unsuitable content. Methods like keyword extracting, opinion analysis, and machine learning codification can help discover and alleviate troublesome outputs.
Rectification is another crucial aspect. Enforce real-time fact-checking APIs, like those Factmata and Full Fact offer, to validate the data the LLM generates. This ensures that the responses are not only secure but also precise and steadfast.
User Engagement and Feedback Mechanisms: Enabling Continuous Improvement
Engage users and collect responses for constant enhancement of your LLM system. Enforce feedback mechanisms that permit users to appraise feedback, flag problems and write comments. Tools such as user surveys, in-app feedback forms, and communicative prompts can facilitate this procedure.
Inspecting user responses helps determine common problems and areas for improvement. Use this information to fine-tune your LLM, update training datasets, and adapt algorithms. Constant learning from user interactions ensures that your LLM evolves to meet user requirements more efficiently.
Integration with LLM APIs and Platforms: Practical Implementation Steps
Incorporate system guardrails with LLM APIs and the platform using a standard approach. Start by choosing a suitable LLM platform, like OpenAI or HuggingFace, that aids the incorporation of custom guardrails. Acquaint yourself with the platform’s API documentation and capabilities.
Develop middleware that connects the user inputs and the guardrail mechanisms. This middleware should manage input predigestion, real-time output refining, and response gathering smoothly. For instance, use Flask or Express.js to make an API layer that handles these processes.
In the end, examine your incorporated system considerably to ensure the guardrails function as aimed without shuffling the user experience. Conduct both computerized and manual testing to cover a wide range of synopsis and edge cases.
By executing these strategies, you can create a sturdy LLM system that delivers dependable, precise and secure outputs, improving user trust and experience.
Monitoring and Evaluating Guardrail Effectiveness
Setting Up Metrics and KPIs for Guardrail Performance
When you set up metrics and Key Performance Indicators (KPIs) for your guardrails, you’re pioneering a clear structure to gauge their success. Begin by determining the predominant intentions of your guardrails. Are they designed to improve security, enhance functional effectiveness, or ensure docility with regulations.
Contemplate using metrics like the number of safety incidents averted, the regularity of guardrail activations, and the abatement in functional disruptions. KPIs might indulge the probability curtailed in disasters, the response duration to incidents, and user docility rates. For instance, if your guardrails are in place to curtail data infringement, a substantial KPI would be the abatement in unauthorized access efforts.
Tools and Techniques for Monitoring Guardrail Effectiveness
Observing the efficiency of your guardrails needs the right tools and methods. Enforce real-time monitoring systems that can trace guardrail initiations and provide instantaneous vigilantism. Tools such as Splunk or ELK stack can collect and dissect log data, providing perceptions into guardrail performance.
Utilize machine learning models to forecast possible failures or infringements and use dashboards to visualize performance metrics. Use these tools to comprehend trends and irregularities, thereby permitting energetic adaptations. In addition, frequent audits and docility checks ensure that guardrails stay efficient over time.
Adjusting Guardrails Based on Feedback and Performance Data
Adapt guardrails based on response and performance data to maintain their efficiency. Gather data from several sources, indulging system logs, user response and accident reports. Dissect this data to discover motifs and areas to enhance.
For example, if you observe a high prevalence of erroneous positives, fine-tune the perceptivity of your guardrails. Engage with investors to collect instinctive responses and ensure the adaptations align with their requirements. Frequently overhaul your guardrails to adjust to new risks and functional alterations to ensure they stay pertinent and efficient.
Case Studies on the Impact of Custom Guardrails in Real-World Applications
Let’s now discover some real-world instances to comprehend the impact of custom guardrails. In the financial sector, a chief bank enforced custom guardrails to avert deceptive transactions. By setting up precise KPIs and utilizing advanced observing tools, they curtail deceit attempts by 30% within the first 6 months.
Another instance is a healthcare supplier that used custom guardrails to safeguard the patient records. They observed data access motifs and adapted their guardrails based on user behavior This approach leads to 40% decrease in data infringement and enhanced patient trust.
In both cases, the amalgamation of well-defined metrics, efficient monitoring tools, and versatile adaptations based on performance data substantially improved the efficiency of the guardrails.
Ready to boost the security and dependability of your enterprise LLM applications? Explore how RagaAI’s advanced guardrails can protect your data and upgrade performance. Contact us today to learn more about enforcing sturdy solutions to your venture requirements. Secure your future with RagaAI!
Best Practices and Challenges Of Guardrails
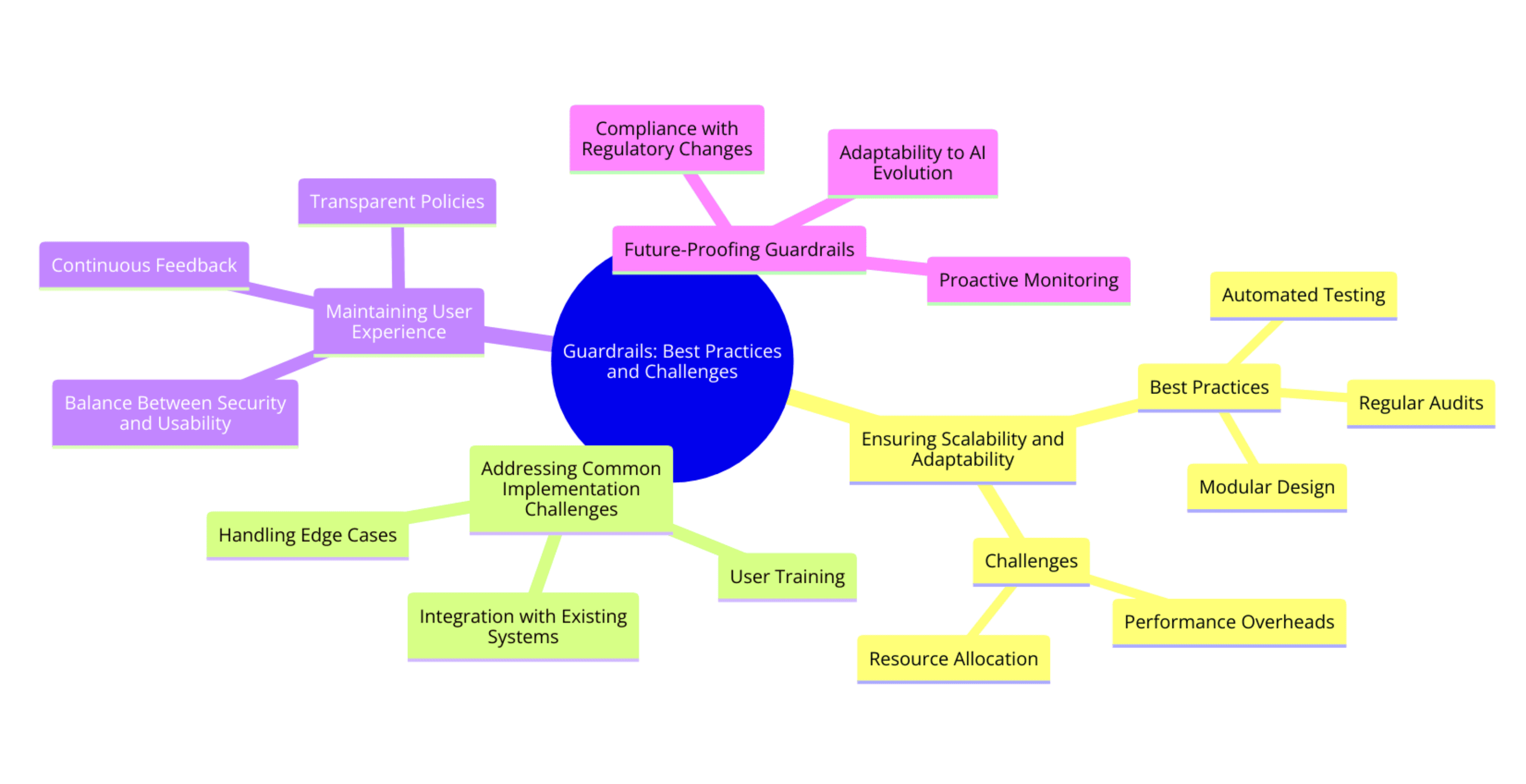
Ensuring Scalability and Adaptability of Guardrails
When enforcing LLM guardrails, ensuring manageability and flexibility is critical. You need to design guardrails that can manage the gradual loads of data and communications without yielding performance. This involves using adjustable and adaptable architecture.
By engaging microservices, you can easily scale distinct elements solely. In addition, contemplate using machine learning methods to constantly update and process guardrails based on new data. For instance, if you are operating with a consumer service chatbot, scalable guardrails ensure that as the number of users evolves, the system persists to execute rules efficiently without slowing down.
Addressing Common Implementation Challenges
Enforcing LLM guardrails comes with numerous challenges. One common problem is compensating the rigidity of the guardrails with the instability of natural language.
Overly strict guardrails can choke the AIs capability to produce natural, engaging responses. To acknowledge this, use a hybrid approach that merges rule-based systems with machine learning models.
For example, while rigid rules might be significant to avoid precise detrimental behaviors, machine learning models can offer more subtle moderation. Another challenge is the incorporation of these guardrails into existing systems.
Here, pragmatic testing and repetitive evolution are key. Begin with a small pilot, collect responses, and process the guardrails before full-scale disperse.
Maintaining User Experience While Enforcing Strict Guardrails
One of the peskiest parts of enforcing LLM guardrails is handling a positive user experience. Users anticipate fluid, natural communications, which can be disorganized by overly limited guardrails. To smack a balance, enforce flexible guardrails that can adapt based on context.
For example, a communicative agent might need to be more compassionate in a casual conversation but scrupulous in a professional setting. In addition, provide users with clarity. If a guardrail blocks an action, elucidate why in a friendly manner and recommend alternatives. This not only maintains trust but also improves user satisfaction.
Future-Proofing Guardrails Amidst Evolving AI Capabilities and Regulatory Landscapes
The AI prospect is swiftly progressing, and so are regulations ruling its utilization. To future-proof your guardrails, you need to assimilate a proactive approach. Stay informed about the newest evolvements in AI technology and executive alterations.
Design your guardrails to be easily upgradeable, permitting you to integrate new rules and standards as they appear. In addition, contemplate the possibility for new kinds of interactions and data.
For instance, as multimodal AI systems become more common, your guardrails should be able to manage texts, images and even video content. Frequently retrospect and upgrade your guardrails to ensure they remain efficient and compliant with the newest instructions.
Also Read:- Multimodal LLMS Using Image And Text
Conclusion
Building and enforcing custom LLM guardrails is significant for handling the incorporation and safety of AI communications. These guardrails not only avert detrimental or unsuitable behavior but also ensure that the AI controls within the wanted boundaries, offering users with a compatible and trustworthy experience.
Custom guardrails play a crucial role in shaping the future of ethical and liable AI. AI executing transparent instructions and standards, they help alleviate threats associated with AI utilization, like bias, false details and privacy concerns. This nurtures a secure and more dependable AI ecosystem, aiding both users and developers.
Delve into the future of AI-powered communication. Locate how our advanced language models can revolutionize your venture, smooth workflow, and improve customer experience.
Get Started Today!
Discover RagaAI LLM Hub and see the distinctiveness innovative AI can make. Don’t miss out on the transformation.
Join now and Boost your AI capabilities!