Understanding the Need and Possibilities of AI Guardrails Today
Understanding the Need and Possibilities of AI Guardrails Today
Understanding the Need and Possibilities of AI Guardrails Today
Jigar Gupta
Aug 19, 2024




In a globe where AI technologies are becoming an essential part of our lives, the significance of AI guardrails cannot be exaggerated. These systems ensure that users use AI ethically, securely, and fairly. Let’s learn the important role AI guardrails play in modern technology and explore the enormous potential they offer.
Ready to improve your AI safety and performance? Discover expert strategy in Building And Implementing Custom LLM Guardrails.
Introduction to AI Guardrails
Designers create AI Guardrails to observe, guide, and regulate AI systems, ensuring they work within ethical, legal, and safety boundaries. These guardrails are significant in modern technology as they help avert the unintended implications of AI deployment.
Developers and deployers ensure through these guardrails that AI technologies are used appropriately. They help sustain ethical standards, secure systems against risks, and nurture neutrality by alleviating partialities.
AI Guardrails predominantly cater to three purposes: detecting risks, quantifying potential impacts, and alleviating these risks. This procedure helps in creating powerful and dependable AI systems that can be trusted by users and investors alike.
Explore Real-World Success: Discover How AI Transformed Leading Businesses in Our In-Depth Case Studies.
The Risks Addressed by AI Guardrails
AI is transforming industries, but with great power comes great responsibility. Here’s how AI guardrails help you check the potential risks:
Protecting Against Unfair Bias
Ever stressed about AI systems being unfair or biased? Guardrails are your best buddies here. Guardrails ensure that AI systems treat everyone moderately by discovering and correcting biases. This means you can have faith in AI decisions to be unbiased, which is critical for sustaining equality and neutrality in your applications.
Securing AI from Threats
Security is a big deal, specifically with AI. Guardrails avert vicious manipulation and secure AI systems from potential risks. By enforcing powerful security measures, you safeguard your AI from hackers and ensure that your data stays safe and sound.
Prioritizing Privacy by Design
Guardrails help you address privacy concerns by incorporating Privacy by Design principles into your AI applications. This dynamic approach ensures that user information is safeguarded from the get-go, keeping privacy breaches at bay and building trust with your users.
Addressing Ethical Implications
Overpersonalization can be an ambiguous sword. While it enhances user experience, it can also interfere with privacy and autonomy. AI guardrails help strike a balance, ensuring that your AI respects user boundaries and maintains ethical standards.
Ensuring Accuracy and Trust
Eventually, you want your AI to be precise and trustworthy. Guardrails acknowledge the dependability of AI outputs, ensuring data incorporation and reducing the risk of errors. This means you can depend on your AI to offer congruous, high-quality outcomes that you and your users can trust.
Integrating AI guardrails is not just about compliance; it’s about building systems that are impartial, safe, private, ethical, and dependable. It’s about creating AI that you can proudly stand behind.
Ready to sleek your LLM evaluations and improve security? Discover the advantages of RagaAI-LLM Hub in this comprehensive guide on Evaluating LLM Applications and enabling Guardrails using Raga-LLM-Hub!
Types of AI Guardrails
AI technology provides vast advantages, but it also comes with risks. To ensure your AI works securely and ethically, enforcing AI guardrails is critical. Let’s learn the types of AI guardrails you should contemplate.
Input/Output Guards for Real-Time Risk Management
You need sturdy input/output guards to manage real-time risks efficiently. These guards observe data flowing into and out of your AI systems, ensuring that only apt and secure data is refined. This helps avert vicious inputs and ensures outputs are within adequate boundaries, sustaining the incorporation and dependability of your AI applications.
Privacy Safeguards Specific to AI Products
Privacy is paramount, specifically when dealing with AI products. You ensure that your AI respects user information and follows regulations such as GDPR and CCPA by integrating privacy safeguards. These safeguards indulge data anonymization, encryption, and strict access controls, ensuring that sensitive data is safeguarded and used responsibly.
Neutralizing Poisoned Content and Mitigating Multiturn Threats
Safeguard your AI from poisoned content and multiturn risks using advanced techniques. Poisoned content can degrade your AI models, leading to undependable outputs. Enforcing detection and neutralization strategies helps sustain the freshness of your data. In addition, multiturn threats, where vicious actors manipulate multi-step interactions, can be alleviated by observing and scrutinizing conversational flows, ensuring your AI remains secure and steadfast.
Implementing Policies and Classifiers for Responsible AI Use
To nurture liable AI use, you should pioneer clear policies and use classifiers. Policies guide the ethical use of AI, tracing acceptable practices and boundaries. Classifiers can involuntarily discover and flag content or behaviors that breach these policies, ensuring that your AI systems follow pioneered ethical standards and avert misuse.
Bias Mitigation and Fairness in AI Models
Bias in AI models can lead to unfair and discriminatory results. You can use numerous techniques to alleviate bias and improve fairness, like re-sampling data, adapting model parameters, and enforcing fairness restrictions during training. Frequent audits and updates to your models are also important to ensure they remain fair and unbiased over time.
By integrating these guardrails, you can improve the safety, privacy, and neutrality of your AI systems, unleashing their full potential while minimizing risks.
Implementing AI Guardrails
Implementing AI guardrails isn’t just a technical necessity; it’s the key to ensuring your AI systems work securely, ethically, and efficiently in a swiftly evolving digital scenario.
Technical Requirements: Integrating Guardrails into AI Systems
When you’re incorporating AI models into your existing systems, it’s critical to begin with a clear comprehension of your technical needs. First, evaluate your current AI infrastructure. Determine where guardrails are required most, whether it’s data input, refining, or output stages. Ensure conformity between the guardrails and your AI’s frameworks and libraries. You might need to update or adjust certain elements to smoothly integrate the guardrails. Concentrate on manageability and adaptability to allow for future adaptations and expansions as your AI system evolves.
Validator Assemblies and Guardrails Hub in Risk Management
Validator assemblies are your AI’s checkpoints, ensuring every decision made by the system is within set boundaries. The Guardrails Hub serves as the control centre, and it consolidates risk management and observance. By enforcing validator assemblies, you can methodically retrospect AI outputs, catching possible errors or biases before they cause issues. The Guardrails Hub expedites this process by providing a unified platform to sustain and adapt guardrail parameters, making risk management more effective and smooth.
Guardrail Implementations in Various AI Applications
To comprehend the practical application of AI guardrails, let’s look at a few instances. In healthcare, guardrails can avert diagnostic AI from suggesting treatments that are outside instructions. In finance, they ensure trading algorithms follow regulatory needs, averting risk investments. In autonomous driving, guardrails can stop vehicles from making unsafe maneuvers, prioritizing passenger security. These instances emphasize how guardrails can be customized to the precise requirements of numerous AI applications, improving dependability and trustworthiness.
By following these instructions, you can build and sustain AI guardrails that not only secure your functioning but also improve the overall performance and dependability of your AI systems.
Challenges and Solutions
Let’s learn the challenges and solutions surrounding AI guardrails today:
Balancing Innovation with Ethics and Safety
One of the difficult challenges is balancing the swift pace of AI innovations with the requirement of safety and ethics. You want AI to push boundaries and create new potentialities but without yielding to ethical standards. This solution lies in dynamic governance. By setting clear ethical instructions and safety protocols, you can nurture an environment where innovation flourishes responsibly. This means evolving AI that respects privacy, avoids bias, and ensures lucidity.
The Need for Ongoing Updates to Guardrail Mechanisms
AI is not immobile; it develops. Therefore, your guardrails must develop too. Constant updates and enhancements are critical to keep up with AI’s proactive nature. You can recognize potential risks and areas for improvement through frequent audits and reviews of AI systems. You can enforce flexible mechanisms that grasp new information and experiences, ensuring your AI remains safe and efficient over time.
Collaboration and Stakeholder Engagement for AI Safety Standards
AI safety isn’t a solo attempt. It needs collaboration and engagement from numerous investors, including developers, policymakers, and end-users. By nurturing open interaction and collaboration, you can evolve pragmatic safety standards that acknowledge disparate prospects and concerns. Engage with industry leaders, join ethics boards, and participate in public discussions to ensure your AI solutions are sturdy and well-rounded.
The requirement for AI guardrails is indisputable. By equating innovation with ethical contemplations, constantly updating mechanisms, nurturing collaboration, and grasping success stories, you can check the intricacies of AI evolution.
The Future of AI Guardrails
Imagine a globe where AI systems are not only savvy but also more secure, continually developing to safeguard against the latest threats.
Adaptive AI Guardrails for Evolving Security Threats
In the very-altering technology scenario, AI guardrails must constantly develop to keep up with occurring security risks. As cyber threats become more sophisticated, the guardrails designed to safeguard against them must also become more advanced. You must avoid these risks by enforcing flexible AI guardrails that can answer in real-time to new susceptibilities. This dynamic approach ensures that your system remains safe and adaptable in the face of continual change.
Cross-Industry Collaboration: Exchanging Best Practices
No single industry can tackle the challenges of AI security alone. By partnering across industries, you can share perceptions and best practices to nourish AI guardrails broadly. This cross-industry cooperation is critical for creating sturdy security frameworks that benefit everyone. By grasping each other’s experiences and innovations, you can build a more safe and trustworthy ecosystem.
Innovations in Guardrail Technology and Regulatory Impact
Technological advancements in AI guardrails constantly push the boundaries of what is possible. These innovations not only enhance safety but also accommodate developing regulatory frameworks. You must stay informed about these regulations to ensure your AI systems meet all necessary standards.
Conclusion
AI Guardrails are important for ensuring the ethical, secure, and fair use of AI technologies. They acknowledge risks such as prejudice, security risks, and seclusion concerns, maintaining trust in AI systems. Efficient enforcement requires comprehending technical requirements, using substantiator assemblies, and adhering to best practices.
Despite challenges, collaboration and congruous updates can improve AI safety standards. The future of AI guardrails involves adjusting to new threats, nurturing cross-industry collaboration, and encircling innovations. Lucidity and public faith are key to their success.
By using resources and community forums, you can stay informed and contribute to the liable expansion of Artificial Intelligence. Clasping AI guardrails ensures AI advantages society securely and efficiently.
Ready to transform your AI development process? RagaAI provides innovative test enforcement and guardrails to ensure your AI models perform immaculately and ethically. Sign Up today to use the power of our pragmatic AI governance hub and sleek your AI testing with certainty. Join the vanguard of AI innovation with RagaAI!
Begin your expedition now and experience the future of AI testing and governance.
In a globe where AI technologies are becoming an essential part of our lives, the significance of AI guardrails cannot be exaggerated. These systems ensure that users use AI ethically, securely, and fairly. Let’s learn the important role AI guardrails play in modern technology and explore the enormous potential they offer.
Ready to improve your AI safety and performance? Discover expert strategy in Building And Implementing Custom LLM Guardrails.
Introduction to AI Guardrails
Designers create AI Guardrails to observe, guide, and regulate AI systems, ensuring they work within ethical, legal, and safety boundaries. These guardrails are significant in modern technology as they help avert the unintended implications of AI deployment.
Developers and deployers ensure through these guardrails that AI technologies are used appropriately. They help sustain ethical standards, secure systems against risks, and nurture neutrality by alleviating partialities.
AI Guardrails predominantly cater to three purposes: detecting risks, quantifying potential impacts, and alleviating these risks. This procedure helps in creating powerful and dependable AI systems that can be trusted by users and investors alike.
Explore Real-World Success: Discover How AI Transformed Leading Businesses in Our In-Depth Case Studies.
The Risks Addressed by AI Guardrails
AI is transforming industries, but with great power comes great responsibility. Here’s how AI guardrails help you check the potential risks:
Protecting Against Unfair Bias
Ever stressed about AI systems being unfair or biased? Guardrails are your best buddies here. Guardrails ensure that AI systems treat everyone moderately by discovering and correcting biases. This means you can have faith in AI decisions to be unbiased, which is critical for sustaining equality and neutrality in your applications.
Securing AI from Threats
Security is a big deal, specifically with AI. Guardrails avert vicious manipulation and secure AI systems from potential risks. By enforcing powerful security measures, you safeguard your AI from hackers and ensure that your data stays safe and sound.
Prioritizing Privacy by Design
Guardrails help you address privacy concerns by incorporating Privacy by Design principles into your AI applications. This dynamic approach ensures that user information is safeguarded from the get-go, keeping privacy breaches at bay and building trust with your users.
Addressing Ethical Implications
Overpersonalization can be an ambiguous sword. While it enhances user experience, it can also interfere with privacy and autonomy. AI guardrails help strike a balance, ensuring that your AI respects user boundaries and maintains ethical standards.
Ensuring Accuracy and Trust
Eventually, you want your AI to be precise and trustworthy. Guardrails acknowledge the dependability of AI outputs, ensuring data incorporation and reducing the risk of errors. This means you can depend on your AI to offer congruous, high-quality outcomes that you and your users can trust.
Integrating AI guardrails is not just about compliance; it’s about building systems that are impartial, safe, private, ethical, and dependable. It’s about creating AI that you can proudly stand behind.
Ready to sleek your LLM evaluations and improve security? Discover the advantages of RagaAI-LLM Hub in this comprehensive guide on Evaluating LLM Applications and enabling Guardrails using Raga-LLM-Hub!
Types of AI Guardrails
AI technology provides vast advantages, but it also comes with risks. To ensure your AI works securely and ethically, enforcing AI guardrails is critical. Let’s learn the types of AI guardrails you should contemplate.
Input/Output Guards for Real-Time Risk Management
You need sturdy input/output guards to manage real-time risks efficiently. These guards observe data flowing into and out of your AI systems, ensuring that only apt and secure data is refined. This helps avert vicious inputs and ensures outputs are within adequate boundaries, sustaining the incorporation and dependability of your AI applications.
Privacy Safeguards Specific to AI Products
Privacy is paramount, specifically when dealing with AI products. You ensure that your AI respects user information and follows regulations such as GDPR and CCPA by integrating privacy safeguards. These safeguards indulge data anonymization, encryption, and strict access controls, ensuring that sensitive data is safeguarded and used responsibly.
Neutralizing Poisoned Content and Mitigating Multiturn Threats
Safeguard your AI from poisoned content and multiturn risks using advanced techniques. Poisoned content can degrade your AI models, leading to undependable outputs. Enforcing detection and neutralization strategies helps sustain the freshness of your data. In addition, multiturn threats, where vicious actors manipulate multi-step interactions, can be alleviated by observing and scrutinizing conversational flows, ensuring your AI remains secure and steadfast.
Implementing Policies and Classifiers for Responsible AI Use
To nurture liable AI use, you should pioneer clear policies and use classifiers. Policies guide the ethical use of AI, tracing acceptable practices and boundaries. Classifiers can involuntarily discover and flag content or behaviors that breach these policies, ensuring that your AI systems follow pioneered ethical standards and avert misuse.
Bias Mitigation and Fairness in AI Models
Bias in AI models can lead to unfair and discriminatory results. You can use numerous techniques to alleviate bias and improve fairness, like re-sampling data, adapting model parameters, and enforcing fairness restrictions during training. Frequent audits and updates to your models are also important to ensure they remain fair and unbiased over time.
By integrating these guardrails, you can improve the safety, privacy, and neutrality of your AI systems, unleashing their full potential while minimizing risks.
Implementing AI Guardrails
Implementing AI guardrails isn’t just a technical necessity; it’s the key to ensuring your AI systems work securely, ethically, and efficiently in a swiftly evolving digital scenario.
Technical Requirements: Integrating Guardrails into AI Systems
When you’re incorporating AI models into your existing systems, it’s critical to begin with a clear comprehension of your technical needs. First, evaluate your current AI infrastructure. Determine where guardrails are required most, whether it’s data input, refining, or output stages. Ensure conformity between the guardrails and your AI’s frameworks and libraries. You might need to update or adjust certain elements to smoothly integrate the guardrails. Concentrate on manageability and adaptability to allow for future adaptations and expansions as your AI system evolves.
Validator Assemblies and Guardrails Hub in Risk Management
Validator assemblies are your AI’s checkpoints, ensuring every decision made by the system is within set boundaries. The Guardrails Hub serves as the control centre, and it consolidates risk management and observance. By enforcing validator assemblies, you can methodically retrospect AI outputs, catching possible errors or biases before they cause issues. The Guardrails Hub expedites this process by providing a unified platform to sustain and adapt guardrail parameters, making risk management more effective and smooth.
Guardrail Implementations in Various AI Applications
To comprehend the practical application of AI guardrails, let’s look at a few instances. In healthcare, guardrails can avert diagnostic AI from suggesting treatments that are outside instructions. In finance, they ensure trading algorithms follow regulatory needs, averting risk investments. In autonomous driving, guardrails can stop vehicles from making unsafe maneuvers, prioritizing passenger security. These instances emphasize how guardrails can be customized to the precise requirements of numerous AI applications, improving dependability and trustworthiness.
By following these instructions, you can build and sustain AI guardrails that not only secure your functioning but also improve the overall performance and dependability of your AI systems.
Challenges and Solutions
Let’s learn the challenges and solutions surrounding AI guardrails today:
Balancing Innovation with Ethics and Safety
One of the difficult challenges is balancing the swift pace of AI innovations with the requirement of safety and ethics. You want AI to push boundaries and create new potentialities but without yielding to ethical standards. This solution lies in dynamic governance. By setting clear ethical instructions and safety protocols, you can nurture an environment where innovation flourishes responsibly. This means evolving AI that respects privacy, avoids bias, and ensures lucidity.
The Need for Ongoing Updates to Guardrail Mechanisms
AI is not immobile; it develops. Therefore, your guardrails must develop too. Constant updates and enhancements are critical to keep up with AI’s proactive nature. You can recognize potential risks and areas for improvement through frequent audits and reviews of AI systems. You can enforce flexible mechanisms that grasp new information and experiences, ensuring your AI remains safe and efficient over time.
Collaboration and Stakeholder Engagement for AI Safety Standards
AI safety isn’t a solo attempt. It needs collaboration and engagement from numerous investors, including developers, policymakers, and end-users. By nurturing open interaction and collaboration, you can evolve pragmatic safety standards that acknowledge disparate prospects and concerns. Engage with industry leaders, join ethics boards, and participate in public discussions to ensure your AI solutions are sturdy and well-rounded.
The requirement for AI guardrails is indisputable. By equating innovation with ethical contemplations, constantly updating mechanisms, nurturing collaboration, and grasping success stories, you can check the intricacies of AI evolution.
The Future of AI Guardrails
Imagine a globe where AI systems are not only savvy but also more secure, continually developing to safeguard against the latest threats.
Adaptive AI Guardrails for Evolving Security Threats
In the very-altering technology scenario, AI guardrails must constantly develop to keep up with occurring security risks. As cyber threats become more sophisticated, the guardrails designed to safeguard against them must also become more advanced. You must avoid these risks by enforcing flexible AI guardrails that can answer in real-time to new susceptibilities. This dynamic approach ensures that your system remains safe and adaptable in the face of continual change.
Cross-Industry Collaboration: Exchanging Best Practices
No single industry can tackle the challenges of AI security alone. By partnering across industries, you can share perceptions and best practices to nourish AI guardrails broadly. This cross-industry cooperation is critical for creating sturdy security frameworks that benefit everyone. By grasping each other’s experiences and innovations, you can build a more safe and trustworthy ecosystem.
Innovations in Guardrail Technology and Regulatory Impact
Technological advancements in AI guardrails constantly push the boundaries of what is possible. These innovations not only enhance safety but also accommodate developing regulatory frameworks. You must stay informed about these regulations to ensure your AI systems meet all necessary standards.
Conclusion
AI Guardrails are important for ensuring the ethical, secure, and fair use of AI technologies. They acknowledge risks such as prejudice, security risks, and seclusion concerns, maintaining trust in AI systems. Efficient enforcement requires comprehending technical requirements, using substantiator assemblies, and adhering to best practices.
Despite challenges, collaboration and congruous updates can improve AI safety standards. The future of AI guardrails involves adjusting to new threats, nurturing cross-industry collaboration, and encircling innovations. Lucidity and public faith are key to their success.
By using resources and community forums, you can stay informed and contribute to the liable expansion of Artificial Intelligence. Clasping AI guardrails ensures AI advantages society securely and efficiently.
Ready to transform your AI development process? RagaAI provides innovative test enforcement and guardrails to ensure your AI models perform immaculately and ethically. Sign Up today to use the power of our pragmatic AI governance hub and sleek your AI testing with certainty. Join the vanguard of AI innovation with RagaAI!
Begin your expedition now and experience the future of AI testing and governance.
In a globe where AI technologies are becoming an essential part of our lives, the significance of AI guardrails cannot be exaggerated. These systems ensure that users use AI ethically, securely, and fairly. Let’s learn the important role AI guardrails play in modern technology and explore the enormous potential they offer.
Ready to improve your AI safety and performance? Discover expert strategy in Building And Implementing Custom LLM Guardrails.
Introduction to AI Guardrails
Designers create AI Guardrails to observe, guide, and regulate AI systems, ensuring they work within ethical, legal, and safety boundaries. These guardrails are significant in modern technology as they help avert the unintended implications of AI deployment.
Developers and deployers ensure through these guardrails that AI technologies are used appropriately. They help sustain ethical standards, secure systems against risks, and nurture neutrality by alleviating partialities.
AI Guardrails predominantly cater to three purposes: detecting risks, quantifying potential impacts, and alleviating these risks. This procedure helps in creating powerful and dependable AI systems that can be trusted by users and investors alike.
Explore Real-World Success: Discover How AI Transformed Leading Businesses in Our In-Depth Case Studies.
The Risks Addressed by AI Guardrails
AI is transforming industries, but with great power comes great responsibility. Here’s how AI guardrails help you check the potential risks:
Protecting Against Unfair Bias
Ever stressed about AI systems being unfair or biased? Guardrails are your best buddies here. Guardrails ensure that AI systems treat everyone moderately by discovering and correcting biases. This means you can have faith in AI decisions to be unbiased, which is critical for sustaining equality and neutrality in your applications.
Securing AI from Threats
Security is a big deal, specifically with AI. Guardrails avert vicious manipulation and secure AI systems from potential risks. By enforcing powerful security measures, you safeguard your AI from hackers and ensure that your data stays safe and sound.
Prioritizing Privacy by Design
Guardrails help you address privacy concerns by incorporating Privacy by Design principles into your AI applications. This dynamic approach ensures that user information is safeguarded from the get-go, keeping privacy breaches at bay and building trust with your users.
Addressing Ethical Implications
Overpersonalization can be an ambiguous sword. While it enhances user experience, it can also interfere with privacy and autonomy. AI guardrails help strike a balance, ensuring that your AI respects user boundaries and maintains ethical standards.
Ensuring Accuracy and Trust
Eventually, you want your AI to be precise and trustworthy. Guardrails acknowledge the dependability of AI outputs, ensuring data incorporation and reducing the risk of errors. This means you can depend on your AI to offer congruous, high-quality outcomes that you and your users can trust.
Integrating AI guardrails is not just about compliance; it’s about building systems that are impartial, safe, private, ethical, and dependable. It’s about creating AI that you can proudly stand behind.
Ready to sleek your LLM evaluations and improve security? Discover the advantages of RagaAI-LLM Hub in this comprehensive guide on Evaluating LLM Applications and enabling Guardrails using Raga-LLM-Hub!
Types of AI Guardrails
AI technology provides vast advantages, but it also comes with risks. To ensure your AI works securely and ethically, enforcing AI guardrails is critical. Let’s learn the types of AI guardrails you should contemplate.
Input/Output Guards for Real-Time Risk Management
You need sturdy input/output guards to manage real-time risks efficiently. These guards observe data flowing into and out of your AI systems, ensuring that only apt and secure data is refined. This helps avert vicious inputs and ensures outputs are within adequate boundaries, sustaining the incorporation and dependability of your AI applications.
Privacy Safeguards Specific to AI Products
Privacy is paramount, specifically when dealing with AI products. You ensure that your AI respects user information and follows regulations such as GDPR and CCPA by integrating privacy safeguards. These safeguards indulge data anonymization, encryption, and strict access controls, ensuring that sensitive data is safeguarded and used responsibly.
Neutralizing Poisoned Content and Mitigating Multiturn Threats
Safeguard your AI from poisoned content and multiturn risks using advanced techniques. Poisoned content can degrade your AI models, leading to undependable outputs. Enforcing detection and neutralization strategies helps sustain the freshness of your data. In addition, multiturn threats, where vicious actors manipulate multi-step interactions, can be alleviated by observing and scrutinizing conversational flows, ensuring your AI remains secure and steadfast.
Implementing Policies and Classifiers for Responsible AI Use
To nurture liable AI use, you should pioneer clear policies and use classifiers. Policies guide the ethical use of AI, tracing acceptable practices and boundaries. Classifiers can involuntarily discover and flag content or behaviors that breach these policies, ensuring that your AI systems follow pioneered ethical standards and avert misuse.
Bias Mitigation and Fairness in AI Models
Bias in AI models can lead to unfair and discriminatory results. You can use numerous techniques to alleviate bias and improve fairness, like re-sampling data, adapting model parameters, and enforcing fairness restrictions during training. Frequent audits and updates to your models are also important to ensure they remain fair and unbiased over time.
By integrating these guardrails, you can improve the safety, privacy, and neutrality of your AI systems, unleashing their full potential while minimizing risks.
Implementing AI Guardrails
Implementing AI guardrails isn’t just a technical necessity; it’s the key to ensuring your AI systems work securely, ethically, and efficiently in a swiftly evolving digital scenario.
Technical Requirements: Integrating Guardrails into AI Systems
When you’re incorporating AI models into your existing systems, it’s critical to begin with a clear comprehension of your technical needs. First, evaluate your current AI infrastructure. Determine where guardrails are required most, whether it’s data input, refining, or output stages. Ensure conformity between the guardrails and your AI’s frameworks and libraries. You might need to update or adjust certain elements to smoothly integrate the guardrails. Concentrate on manageability and adaptability to allow for future adaptations and expansions as your AI system evolves.
Validator Assemblies and Guardrails Hub in Risk Management
Validator assemblies are your AI’s checkpoints, ensuring every decision made by the system is within set boundaries. The Guardrails Hub serves as the control centre, and it consolidates risk management and observance. By enforcing validator assemblies, you can methodically retrospect AI outputs, catching possible errors or biases before they cause issues. The Guardrails Hub expedites this process by providing a unified platform to sustain and adapt guardrail parameters, making risk management more effective and smooth.
Guardrail Implementations in Various AI Applications
To comprehend the practical application of AI guardrails, let’s look at a few instances. In healthcare, guardrails can avert diagnostic AI from suggesting treatments that are outside instructions. In finance, they ensure trading algorithms follow regulatory needs, averting risk investments. In autonomous driving, guardrails can stop vehicles from making unsafe maneuvers, prioritizing passenger security. These instances emphasize how guardrails can be customized to the precise requirements of numerous AI applications, improving dependability and trustworthiness.
By following these instructions, you can build and sustain AI guardrails that not only secure your functioning but also improve the overall performance and dependability of your AI systems.
Challenges and Solutions
Let’s learn the challenges and solutions surrounding AI guardrails today:
Balancing Innovation with Ethics and Safety
One of the difficult challenges is balancing the swift pace of AI innovations with the requirement of safety and ethics. You want AI to push boundaries and create new potentialities but without yielding to ethical standards. This solution lies in dynamic governance. By setting clear ethical instructions and safety protocols, you can nurture an environment where innovation flourishes responsibly. This means evolving AI that respects privacy, avoids bias, and ensures lucidity.
The Need for Ongoing Updates to Guardrail Mechanisms
AI is not immobile; it develops. Therefore, your guardrails must develop too. Constant updates and enhancements are critical to keep up with AI’s proactive nature. You can recognize potential risks and areas for improvement through frequent audits and reviews of AI systems. You can enforce flexible mechanisms that grasp new information and experiences, ensuring your AI remains safe and efficient over time.
Collaboration and Stakeholder Engagement for AI Safety Standards
AI safety isn’t a solo attempt. It needs collaboration and engagement from numerous investors, including developers, policymakers, and end-users. By nurturing open interaction and collaboration, you can evolve pragmatic safety standards that acknowledge disparate prospects and concerns. Engage with industry leaders, join ethics boards, and participate in public discussions to ensure your AI solutions are sturdy and well-rounded.
The requirement for AI guardrails is indisputable. By equating innovation with ethical contemplations, constantly updating mechanisms, nurturing collaboration, and grasping success stories, you can check the intricacies of AI evolution.
The Future of AI Guardrails
Imagine a globe where AI systems are not only savvy but also more secure, continually developing to safeguard against the latest threats.
Adaptive AI Guardrails for Evolving Security Threats
In the very-altering technology scenario, AI guardrails must constantly develop to keep up with occurring security risks. As cyber threats become more sophisticated, the guardrails designed to safeguard against them must also become more advanced. You must avoid these risks by enforcing flexible AI guardrails that can answer in real-time to new susceptibilities. This dynamic approach ensures that your system remains safe and adaptable in the face of continual change.
Cross-Industry Collaboration: Exchanging Best Practices
No single industry can tackle the challenges of AI security alone. By partnering across industries, you can share perceptions and best practices to nourish AI guardrails broadly. This cross-industry cooperation is critical for creating sturdy security frameworks that benefit everyone. By grasping each other’s experiences and innovations, you can build a more safe and trustworthy ecosystem.
Innovations in Guardrail Technology and Regulatory Impact
Technological advancements in AI guardrails constantly push the boundaries of what is possible. These innovations not only enhance safety but also accommodate developing regulatory frameworks. You must stay informed about these regulations to ensure your AI systems meet all necessary standards.
Conclusion
AI Guardrails are important for ensuring the ethical, secure, and fair use of AI technologies. They acknowledge risks such as prejudice, security risks, and seclusion concerns, maintaining trust in AI systems. Efficient enforcement requires comprehending technical requirements, using substantiator assemblies, and adhering to best practices.
Despite challenges, collaboration and congruous updates can improve AI safety standards. The future of AI guardrails involves adjusting to new threats, nurturing cross-industry collaboration, and encircling innovations. Lucidity and public faith are key to their success.
By using resources and community forums, you can stay informed and contribute to the liable expansion of Artificial Intelligence. Clasping AI guardrails ensures AI advantages society securely and efficiently.
Ready to transform your AI development process? RagaAI provides innovative test enforcement and guardrails to ensure your AI models perform immaculately and ethically. Sign Up today to use the power of our pragmatic AI governance hub and sleek your AI testing with certainty. Join the vanguard of AI innovation with RagaAI!
Begin your expedition now and experience the future of AI testing and governance.
In a globe where AI technologies are becoming an essential part of our lives, the significance of AI guardrails cannot be exaggerated. These systems ensure that users use AI ethically, securely, and fairly. Let’s learn the important role AI guardrails play in modern technology and explore the enormous potential they offer.
Ready to improve your AI safety and performance? Discover expert strategy in Building And Implementing Custom LLM Guardrails.
Introduction to AI Guardrails
Designers create AI Guardrails to observe, guide, and regulate AI systems, ensuring they work within ethical, legal, and safety boundaries. These guardrails are significant in modern technology as they help avert the unintended implications of AI deployment.
Developers and deployers ensure through these guardrails that AI technologies are used appropriately. They help sustain ethical standards, secure systems against risks, and nurture neutrality by alleviating partialities.
AI Guardrails predominantly cater to three purposes: detecting risks, quantifying potential impacts, and alleviating these risks. This procedure helps in creating powerful and dependable AI systems that can be trusted by users and investors alike.
Explore Real-World Success: Discover How AI Transformed Leading Businesses in Our In-Depth Case Studies.
The Risks Addressed by AI Guardrails
AI is transforming industries, but with great power comes great responsibility. Here’s how AI guardrails help you check the potential risks:
Protecting Against Unfair Bias
Ever stressed about AI systems being unfair or biased? Guardrails are your best buddies here. Guardrails ensure that AI systems treat everyone moderately by discovering and correcting biases. This means you can have faith in AI decisions to be unbiased, which is critical for sustaining equality and neutrality in your applications.
Securing AI from Threats
Security is a big deal, specifically with AI. Guardrails avert vicious manipulation and secure AI systems from potential risks. By enforcing powerful security measures, you safeguard your AI from hackers and ensure that your data stays safe and sound.
Prioritizing Privacy by Design
Guardrails help you address privacy concerns by incorporating Privacy by Design principles into your AI applications. This dynamic approach ensures that user information is safeguarded from the get-go, keeping privacy breaches at bay and building trust with your users.
Addressing Ethical Implications
Overpersonalization can be an ambiguous sword. While it enhances user experience, it can also interfere with privacy and autonomy. AI guardrails help strike a balance, ensuring that your AI respects user boundaries and maintains ethical standards.
Ensuring Accuracy and Trust
Eventually, you want your AI to be precise and trustworthy. Guardrails acknowledge the dependability of AI outputs, ensuring data incorporation and reducing the risk of errors. This means you can depend on your AI to offer congruous, high-quality outcomes that you and your users can trust.
Integrating AI guardrails is not just about compliance; it’s about building systems that are impartial, safe, private, ethical, and dependable. It’s about creating AI that you can proudly stand behind.
Ready to sleek your LLM evaluations and improve security? Discover the advantages of RagaAI-LLM Hub in this comprehensive guide on Evaluating LLM Applications and enabling Guardrails using Raga-LLM-Hub!
Types of AI Guardrails
AI technology provides vast advantages, but it also comes with risks. To ensure your AI works securely and ethically, enforcing AI guardrails is critical. Let’s learn the types of AI guardrails you should contemplate.
Input/Output Guards for Real-Time Risk Management
You need sturdy input/output guards to manage real-time risks efficiently. These guards observe data flowing into and out of your AI systems, ensuring that only apt and secure data is refined. This helps avert vicious inputs and ensures outputs are within adequate boundaries, sustaining the incorporation and dependability of your AI applications.
Privacy Safeguards Specific to AI Products
Privacy is paramount, specifically when dealing with AI products. You ensure that your AI respects user information and follows regulations such as GDPR and CCPA by integrating privacy safeguards. These safeguards indulge data anonymization, encryption, and strict access controls, ensuring that sensitive data is safeguarded and used responsibly.
Neutralizing Poisoned Content and Mitigating Multiturn Threats
Safeguard your AI from poisoned content and multiturn risks using advanced techniques. Poisoned content can degrade your AI models, leading to undependable outputs. Enforcing detection and neutralization strategies helps sustain the freshness of your data. In addition, multiturn threats, where vicious actors manipulate multi-step interactions, can be alleviated by observing and scrutinizing conversational flows, ensuring your AI remains secure and steadfast.
Implementing Policies and Classifiers for Responsible AI Use
To nurture liable AI use, you should pioneer clear policies and use classifiers. Policies guide the ethical use of AI, tracing acceptable practices and boundaries. Classifiers can involuntarily discover and flag content or behaviors that breach these policies, ensuring that your AI systems follow pioneered ethical standards and avert misuse.
Bias Mitigation and Fairness in AI Models
Bias in AI models can lead to unfair and discriminatory results. You can use numerous techniques to alleviate bias and improve fairness, like re-sampling data, adapting model parameters, and enforcing fairness restrictions during training. Frequent audits and updates to your models are also important to ensure they remain fair and unbiased over time.
By integrating these guardrails, you can improve the safety, privacy, and neutrality of your AI systems, unleashing their full potential while minimizing risks.
Implementing AI Guardrails
Implementing AI guardrails isn’t just a technical necessity; it’s the key to ensuring your AI systems work securely, ethically, and efficiently in a swiftly evolving digital scenario.
Technical Requirements: Integrating Guardrails into AI Systems
When you’re incorporating AI models into your existing systems, it’s critical to begin with a clear comprehension of your technical needs. First, evaluate your current AI infrastructure. Determine where guardrails are required most, whether it’s data input, refining, or output stages. Ensure conformity between the guardrails and your AI’s frameworks and libraries. You might need to update or adjust certain elements to smoothly integrate the guardrails. Concentrate on manageability and adaptability to allow for future adaptations and expansions as your AI system evolves.
Validator Assemblies and Guardrails Hub in Risk Management
Validator assemblies are your AI’s checkpoints, ensuring every decision made by the system is within set boundaries. The Guardrails Hub serves as the control centre, and it consolidates risk management and observance. By enforcing validator assemblies, you can methodically retrospect AI outputs, catching possible errors or biases before they cause issues. The Guardrails Hub expedites this process by providing a unified platform to sustain and adapt guardrail parameters, making risk management more effective and smooth.
Guardrail Implementations in Various AI Applications
To comprehend the practical application of AI guardrails, let’s look at a few instances. In healthcare, guardrails can avert diagnostic AI from suggesting treatments that are outside instructions. In finance, they ensure trading algorithms follow regulatory needs, averting risk investments. In autonomous driving, guardrails can stop vehicles from making unsafe maneuvers, prioritizing passenger security. These instances emphasize how guardrails can be customized to the precise requirements of numerous AI applications, improving dependability and trustworthiness.
By following these instructions, you can build and sustain AI guardrails that not only secure your functioning but also improve the overall performance and dependability of your AI systems.
Challenges and Solutions
Let’s learn the challenges and solutions surrounding AI guardrails today:
Balancing Innovation with Ethics and Safety
One of the difficult challenges is balancing the swift pace of AI innovations with the requirement of safety and ethics. You want AI to push boundaries and create new potentialities but without yielding to ethical standards. This solution lies in dynamic governance. By setting clear ethical instructions and safety protocols, you can nurture an environment where innovation flourishes responsibly. This means evolving AI that respects privacy, avoids bias, and ensures lucidity.
The Need for Ongoing Updates to Guardrail Mechanisms
AI is not immobile; it develops. Therefore, your guardrails must develop too. Constant updates and enhancements are critical to keep up with AI’s proactive nature. You can recognize potential risks and areas for improvement through frequent audits and reviews of AI systems. You can enforce flexible mechanisms that grasp new information and experiences, ensuring your AI remains safe and efficient over time.
Collaboration and Stakeholder Engagement for AI Safety Standards
AI safety isn’t a solo attempt. It needs collaboration and engagement from numerous investors, including developers, policymakers, and end-users. By nurturing open interaction and collaboration, you can evolve pragmatic safety standards that acknowledge disparate prospects and concerns. Engage with industry leaders, join ethics boards, and participate in public discussions to ensure your AI solutions are sturdy and well-rounded.
The requirement for AI guardrails is indisputable. By equating innovation with ethical contemplations, constantly updating mechanisms, nurturing collaboration, and grasping success stories, you can check the intricacies of AI evolution.
The Future of AI Guardrails
Imagine a globe where AI systems are not only savvy but also more secure, continually developing to safeguard against the latest threats.
Adaptive AI Guardrails for Evolving Security Threats
In the very-altering technology scenario, AI guardrails must constantly develop to keep up with occurring security risks. As cyber threats become more sophisticated, the guardrails designed to safeguard against them must also become more advanced. You must avoid these risks by enforcing flexible AI guardrails that can answer in real-time to new susceptibilities. This dynamic approach ensures that your system remains safe and adaptable in the face of continual change.
Cross-Industry Collaboration: Exchanging Best Practices
No single industry can tackle the challenges of AI security alone. By partnering across industries, you can share perceptions and best practices to nourish AI guardrails broadly. This cross-industry cooperation is critical for creating sturdy security frameworks that benefit everyone. By grasping each other’s experiences and innovations, you can build a more safe and trustworthy ecosystem.
Innovations in Guardrail Technology and Regulatory Impact
Technological advancements in AI guardrails constantly push the boundaries of what is possible. These innovations not only enhance safety but also accommodate developing regulatory frameworks. You must stay informed about these regulations to ensure your AI systems meet all necessary standards.
Conclusion
AI Guardrails are important for ensuring the ethical, secure, and fair use of AI technologies. They acknowledge risks such as prejudice, security risks, and seclusion concerns, maintaining trust in AI systems. Efficient enforcement requires comprehending technical requirements, using substantiator assemblies, and adhering to best practices.
Despite challenges, collaboration and congruous updates can improve AI safety standards. The future of AI guardrails involves adjusting to new threats, nurturing cross-industry collaboration, and encircling innovations. Lucidity and public faith are key to their success.
By using resources and community forums, you can stay informed and contribute to the liable expansion of Artificial Intelligence. Clasping AI guardrails ensures AI advantages society securely and efficiently.
Ready to transform your AI development process? RagaAI provides innovative test enforcement and guardrails to ensure your AI models perform immaculately and ethically. Sign Up today to use the power of our pragmatic AI governance hub and sleek your AI testing with certainty. Join the vanguard of AI innovation with RagaAI!
Begin your expedition now and experience the future of AI testing and governance.
In a globe where AI technologies are becoming an essential part of our lives, the significance of AI guardrails cannot be exaggerated. These systems ensure that users use AI ethically, securely, and fairly. Let’s learn the important role AI guardrails play in modern technology and explore the enormous potential they offer.
Ready to improve your AI safety and performance? Discover expert strategy in Building And Implementing Custom LLM Guardrails.
Introduction to AI Guardrails
Designers create AI Guardrails to observe, guide, and regulate AI systems, ensuring they work within ethical, legal, and safety boundaries. These guardrails are significant in modern technology as they help avert the unintended implications of AI deployment.
Developers and deployers ensure through these guardrails that AI technologies are used appropriately. They help sustain ethical standards, secure systems against risks, and nurture neutrality by alleviating partialities.
AI Guardrails predominantly cater to three purposes: detecting risks, quantifying potential impacts, and alleviating these risks. This procedure helps in creating powerful and dependable AI systems that can be trusted by users and investors alike.
Explore Real-World Success: Discover How AI Transformed Leading Businesses in Our In-Depth Case Studies.
The Risks Addressed by AI Guardrails
AI is transforming industries, but with great power comes great responsibility. Here’s how AI guardrails help you check the potential risks:
Protecting Against Unfair Bias
Ever stressed about AI systems being unfair or biased? Guardrails are your best buddies here. Guardrails ensure that AI systems treat everyone moderately by discovering and correcting biases. This means you can have faith in AI decisions to be unbiased, which is critical for sustaining equality and neutrality in your applications.
Securing AI from Threats
Security is a big deal, specifically with AI. Guardrails avert vicious manipulation and secure AI systems from potential risks. By enforcing powerful security measures, you safeguard your AI from hackers and ensure that your data stays safe and sound.
Prioritizing Privacy by Design
Guardrails help you address privacy concerns by incorporating Privacy by Design principles into your AI applications. This dynamic approach ensures that user information is safeguarded from the get-go, keeping privacy breaches at bay and building trust with your users.
Addressing Ethical Implications
Overpersonalization can be an ambiguous sword. While it enhances user experience, it can also interfere with privacy and autonomy. AI guardrails help strike a balance, ensuring that your AI respects user boundaries and maintains ethical standards.
Ensuring Accuracy and Trust
Eventually, you want your AI to be precise and trustworthy. Guardrails acknowledge the dependability of AI outputs, ensuring data incorporation and reducing the risk of errors. This means you can depend on your AI to offer congruous, high-quality outcomes that you and your users can trust.
Integrating AI guardrails is not just about compliance; it’s about building systems that are impartial, safe, private, ethical, and dependable. It’s about creating AI that you can proudly stand behind.
Ready to sleek your LLM evaluations and improve security? Discover the advantages of RagaAI-LLM Hub in this comprehensive guide on Evaluating LLM Applications and enabling Guardrails using Raga-LLM-Hub!
Types of AI Guardrails
AI technology provides vast advantages, but it also comes with risks. To ensure your AI works securely and ethically, enforcing AI guardrails is critical. Let’s learn the types of AI guardrails you should contemplate.
Input/Output Guards for Real-Time Risk Management
You need sturdy input/output guards to manage real-time risks efficiently. These guards observe data flowing into and out of your AI systems, ensuring that only apt and secure data is refined. This helps avert vicious inputs and ensures outputs are within adequate boundaries, sustaining the incorporation and dependability of your AI applications.
Privacy Safeguards Specific to AI Products
Privacy is paramount, specifically when dealing with AI products. You ensure that your AI respects user information and follows regulations such as GDPR and CCPA by integrating privacy safeguards. These safeguards indulge data anonymization, encryption, and strict access controls, ensuring that sensitive data is safeguarded and used responsibly.
Neutralizing Poisoned Content and Mitigating Multiturn Threats
Safeguard your AI from poisoned content and multiturn risks using advanced techniques. Poisoned content can degrade your AI models, leading to undependable outputs. Enforcing detection and neutralization strategies helps sustain the freshness of your data. In addition, multiturn threats, where vicious actors manipulate multi-step interactions, can be alleviated by observing and scrutinizing conversational flows, ensuring your AI remains secure and steadfast.
Implementing Policies and Classifiers for Responsible AI Use
To nurture liable AI use, you should pioneer clear policies and use classifiers. Policies guide the ethical use of AI, tracing acceptable practices and boundaries. Classifiers can involuntarily discover and flag content or behaviors that breach these policies, ensuring that your AI systems follow pioneered ethical standards and avert misuse.
Bias Mitigation and Fairness in AI Models
Bias in AI models can lead to unfair and discriminatory results. You can use numerous techniques to alleviate bias and improve fairness, like re-sampling data, adapting model parameters, and enforcing fairness restrictions during training. Frequent audits and updates to your models are also important to ensure they remain fair and unbiased over time.
By integrating these guardrails, you can improve the safety, privacy, and neutrality of your AI systems, unleashing their full potential while minimizing risks.
Implementing AI Guardrails
Implementing AI guardrails isn’t just a technical necessity; it’s the key to ensuring your AI systems work securely, ethically, and efficiently in a swiftly evolving digital scenario.
Technical Requirements: Integrating Guardrails into AI Systems
When you’re incorporating AI models into your existing systems, it’s critical to begin with a clear comprehension of your technical needs. First, evaluate your current AI infrastructure. Determine where guardrails are required most, whether it’s data input, refining, or output stages. Ensure conformity between the guardrails and your AI’s frameworks and libraries. You might need to update or adjust certain elements to smoothly integrate the guardrails. Concentrate on manageability and adaptability to allow for future adaptations and expansions as your AI system evolves.
Validator Assemblies and Guardrails Hub in Risk Management
Validator assemblies are your AI’s checkpoints, ensuring every decision made by the system is within set boundaries. The Guardrails Hub serves as the control centre, and it consolidates risk management and observance. By enforcing validator assemblies, you can methodically retrospect AI outputs, catching possible errors or biases before they cause issues. The Guardrails Hub expedites this process by providing a unified platform to sustain and adapt guardrail parameters, making risk management more effective and smooth.
Guardrail Implementations in Various AI Applications
To comprehend the practical application of AI guardrails, let’s look at a few instances. In healthcare, guardrails can avert diagnostic AI from suggesting treatments that are outside instructions. In finance, they ensure trading algorithms follow regulatory needs, averting risk investments. In autonomous driving, guardrails can stop vehicles from making unsafe maneuvers, prioritizing passenger security. These instances emphasize how guardrails can be customized to the precise requirements of numerous AI applications, improving dependability and trustworthiness.
By following these instructions, you can build and sustain AI guardrails that not only secure your functioning but also improve the overall performance and dependability of your AI systems.
Challenges and Solutions
Let’s learn the challenges and solutions surrounding AI guardrails today:
Balancing Innovation with Ethics and Safety
One of the difficult challenges is balancing the swift pace of AI innovations with the requirement of safety and ethics. You want AI to push boundaries and create new potentialities but without yielding to ethical standards. This solution lies in dynamic governance. By setting clear ethical instructions and safety protocols, you can nurture an environment where innovation flourishes responsibly. This means evolving AI that respects privacy, avoids bias, and ensures lucidity.
The Need for Ongoing Updates to Guardrail Mechanisms
AI is not immobile; it develops. Therefore, your guardrails must develop too. Constant updates and enhancements are critical to keep up with AI’s proactive nature. You can recognize potential risks and areas for improvement through frequent audits and reviews of AI systems. You can enforce flexible mechanisms that grasp new information and experiences, ensuring your AI remains safe and efficient over time.
Collaboration and Stakeholder Engagement for AI Safety Standards
AI safety isn’t a solo attempt. It needs collaboration and engagement from numerous investors, including developers, policymakers, and end-users. By nurturing open interaction and collaboration, you can evolve pragmatic safety standards that acknowledge disparate prospects and concerns. Engage with industry leaders, join ethics boards, and participate in public discussions to ensure your AI solutions are sturdy and well-rounded.
The requirement for AI guardrails is indisputable. By equating innovation with ethical contemplations, constantly updating mechanisms, nurturing collaboration, and grasping success stories, you can check the intricacies of AI evolution.
The Future of AI Guardrails
Imagine a globe where AI systems are not only savvy but also more secure, continually developing to safeguard against the latest threats.
Adaptive AI Guardrails for Evolving Security Threats
In the very-altering technology scenario, AI guardrails must constantly develop to keep up with occurring security risks. As cyber threats become more sophisticated, the guardrails designed to safeguard against them must also become more advanced. You must avoid these risks by enforcing flexible AI guardrails that can answer in real-time to new susceptibilities. This dynamic approach ensures that your system remains safe and adaptable in the face of continual change.
Cross-Industry Collaboration: Exchanging Best Practices
No single industry can tackle the challenges of AI security alone. By partnering across industries, you can share perceptions and best practices to nourish AI guardrails broadly. This cross-industry cooperation is critical for creating sturdy security frameworks that benefit everyone. By grasping each other’s experiences and innovations, you can build a more safe and trustworthy ecosystem.
Innovations in Guardrail Technology and Regulatory Impact
Technological advancements in AI guardrails constantly push the boundaries of what is possible. These innovations not only enhance safety but also accommodate developing regulatory frameworks. You must stay informed about these regulations to ensure your AI systems meet all necessary standards.
Conclusion
AI Guardrails are important for ensuring the ethical, secure, and fair use of AI technologies. They acknowledge risks such as prejudice, security risks, and seclusion concerns, maintaining trust in AI systems. Efficient enforcement requires comprehending technical requirements, using substantiator assemblies, and adhering to best practices.
Despite challenges, collaboration and congruous updates can improve AI safety standards. The future of AI guardrails involves adjusting to new threats, nurturing cross-industry collaboration, and encircling innovations. Lucidity and public faith are key to their success.
By using resources and community forums, you can stay informed and contribute to the liable expansion of Artificial Intelligence. Clasping AI guardrails ensures AI advantages society securely and efficiently.
Ready to transform your AI development process? RagaAI provides innovative test enforcement and guardrails to ensure your AI models perform immaculately and ethically. Sign Up today to use the power of our pragmatic AI governance hub and sleek your AI testing with certainty. Join the vanguard of AI innovation with RagaAI!
Begin your expedition now and experience the future of AI testing and governance.
Subscribe to our newsletter to never miss an update
Other articles


Agents Talk, Models Think: A2A + MCP for Enterprise Agentic AI

Riya Parikh, Nitai Agarwal
Apr 27, 2025
Read the article
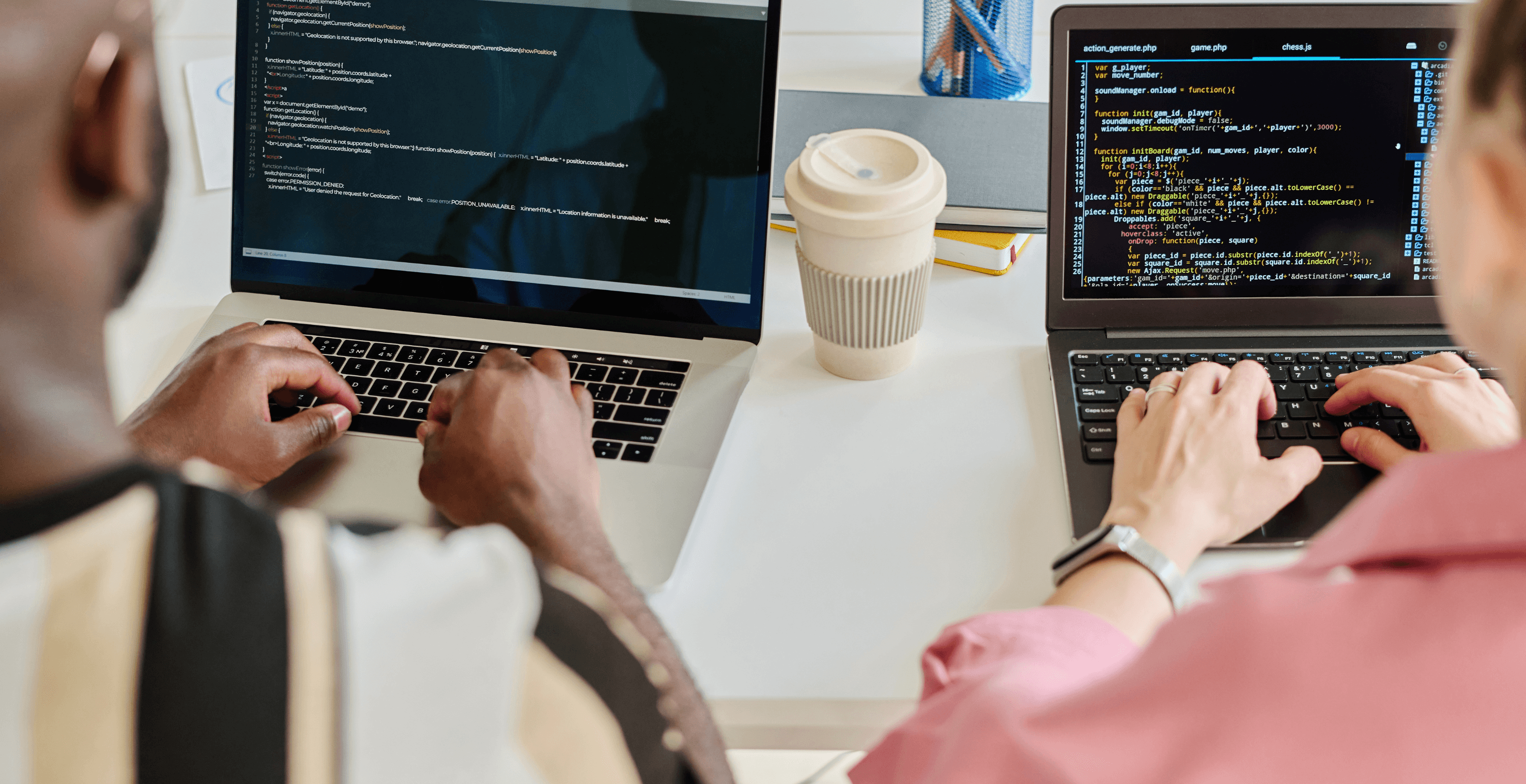
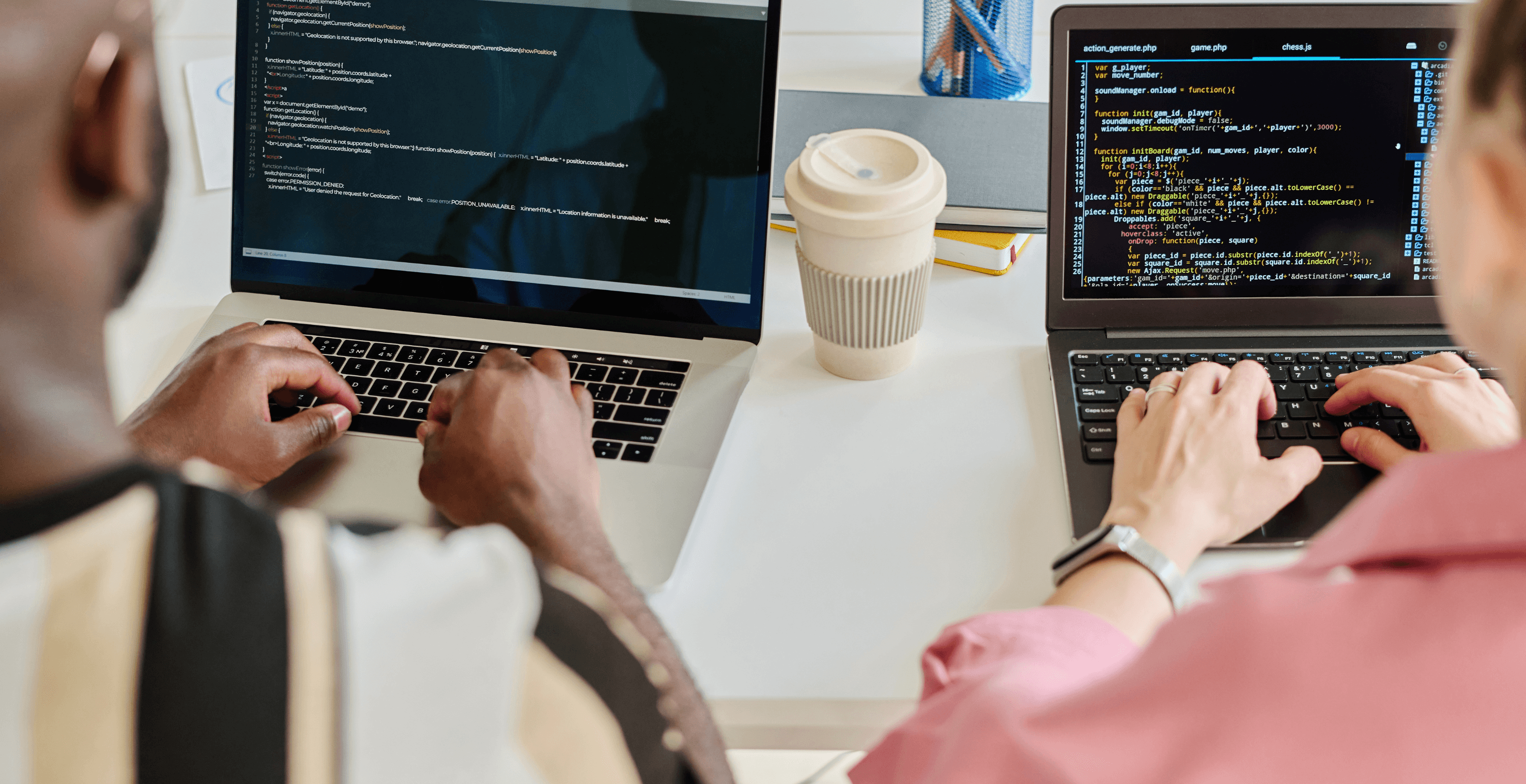
Understanding NeMo Guardrails: A Toolkit for LLM Security

Rehan Asif
Dec 24, 2024
Read the article


Understanding Differences in Large vs Small Language Models (LLM vs SLM)

Rehan Asif
Dec 21, 2024
Read the article