Agentic Design Patterns In LLM-Based Applications
Agentic Design Patterns In LLM-Based Applications
Agentic Design Patterns In LLM-Based Applications
Rehan Asif
Jul 16, 2024
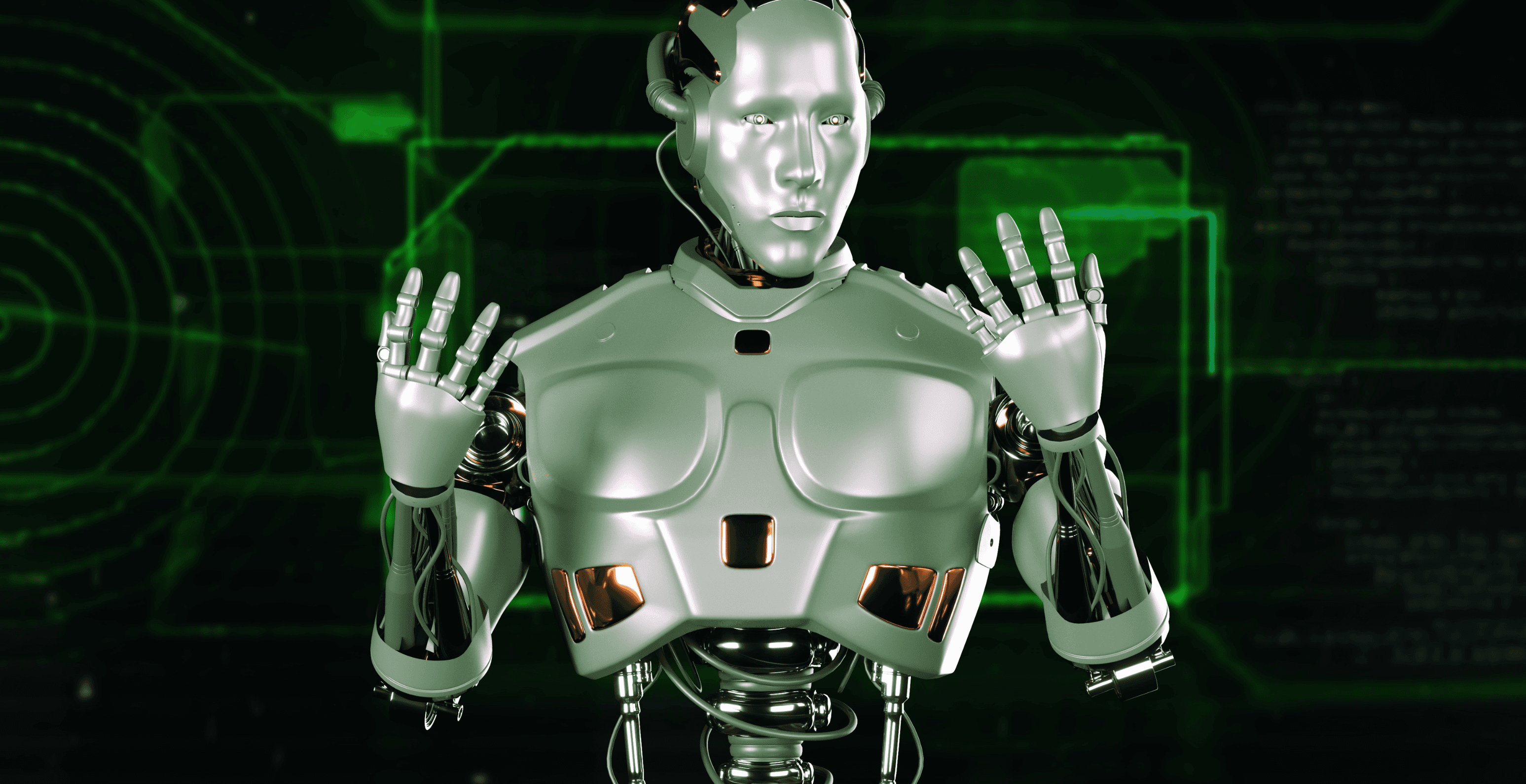
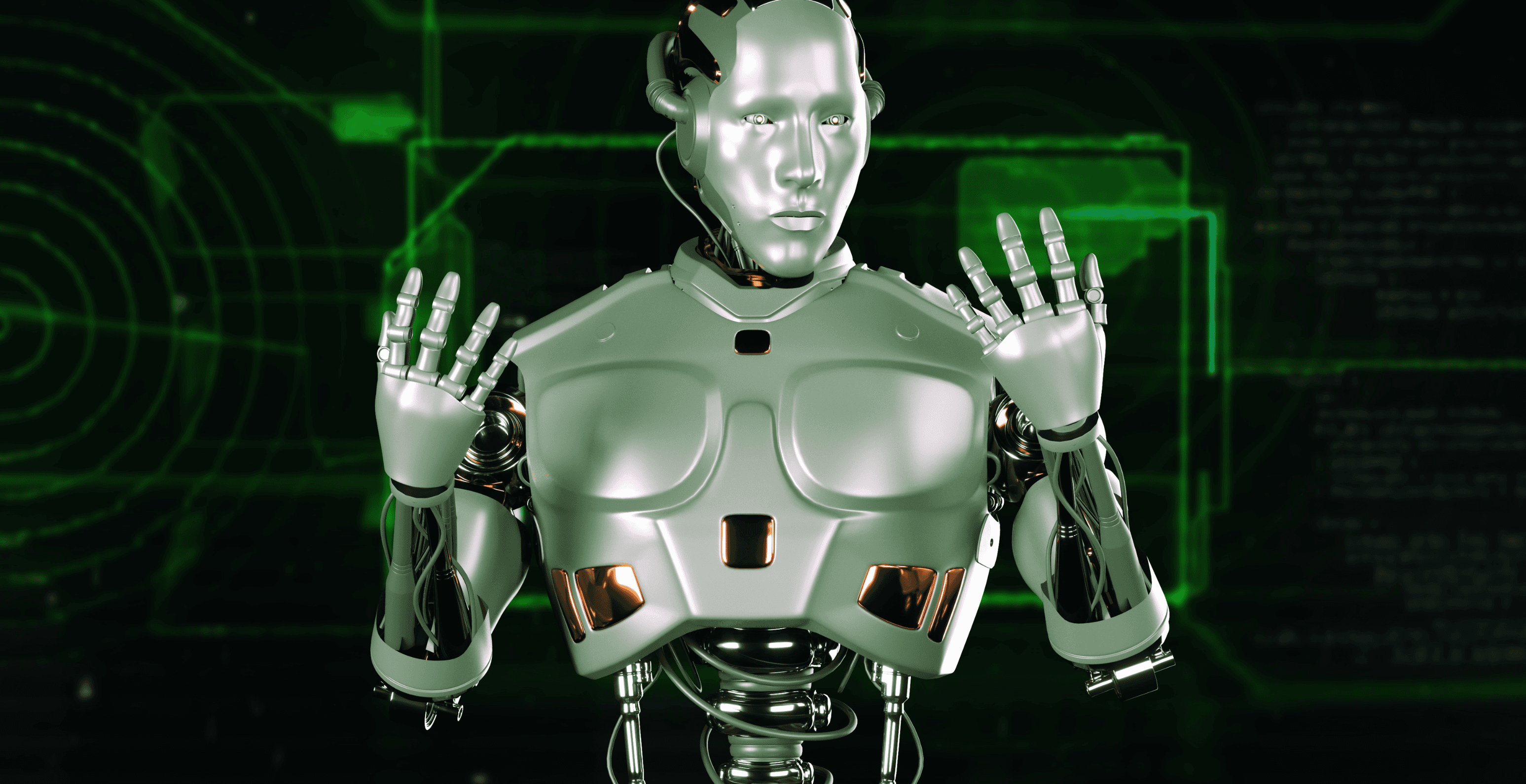
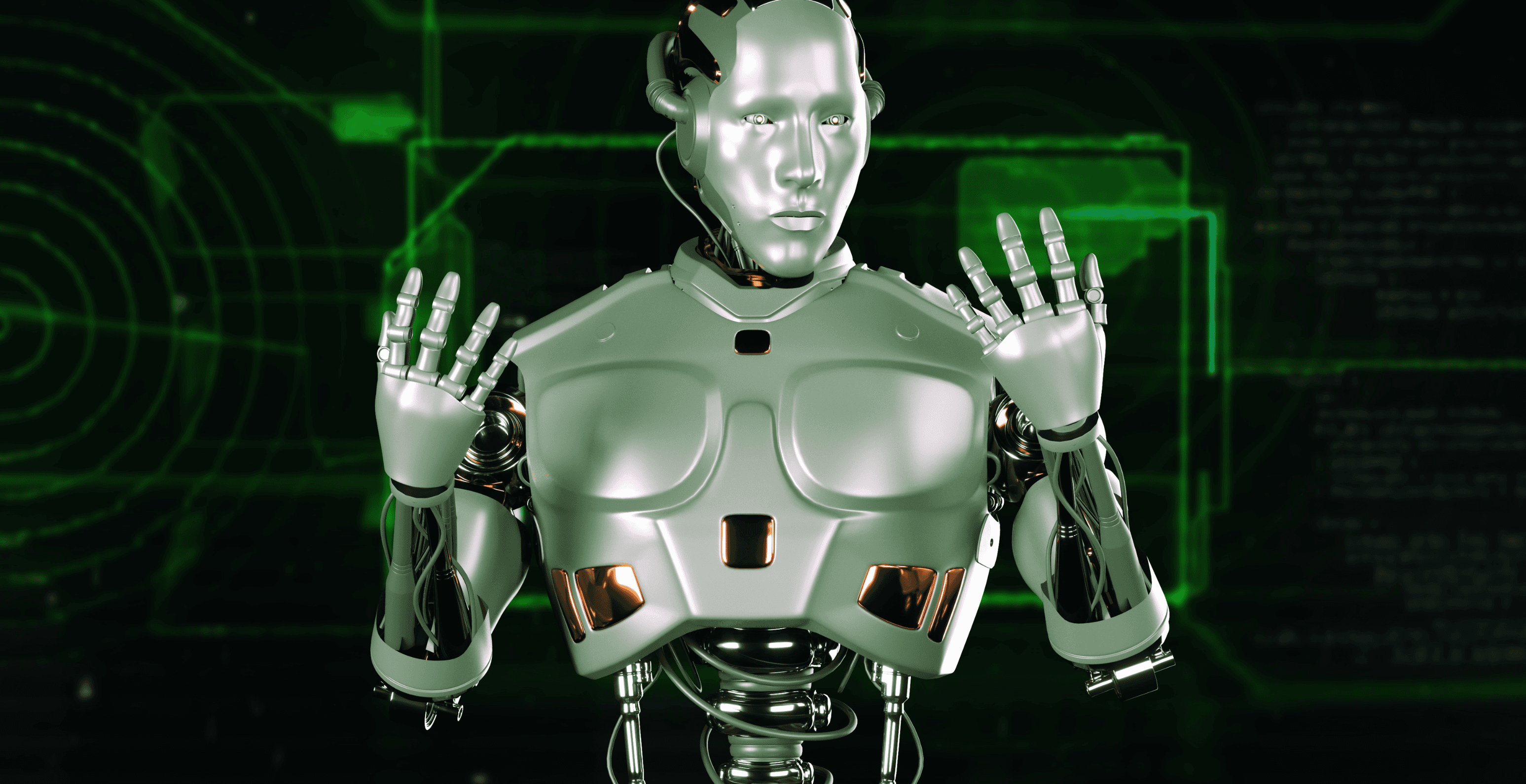
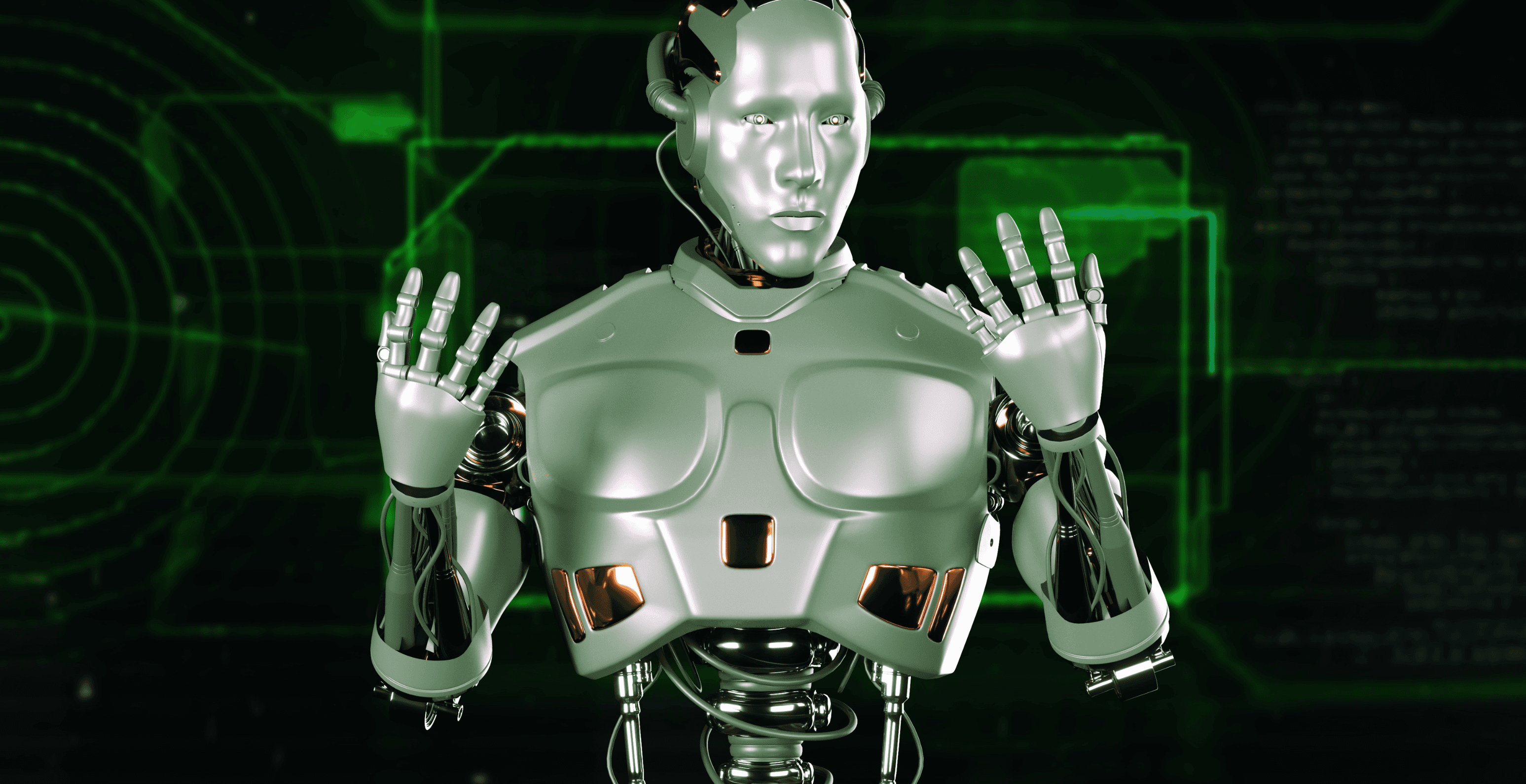
Hey there! Have you ever wondered how large language models (LLMs) such as GPT-4 manage to be so adaptable and effective? It’s all thanks to agentic design motifs. These patterns are transforming how we evolve and use applications with LLMs, making them more proficient and flexible. Let’s explore agentic design patterns further and see how they improve the abilities of LLMs in application development.
Don’t miss out on the chance to improve your skills and knowledge with these anticipating Large Language Models projects! Whether you’re a beginner or looking to amplify your skills, our well-chosen list of 20 LLM Project Ideas is designed to encourage and challenge you. Begin your expedition today and revolutionize your potential into reality.
Introduction to Agentic Design Patterns in LLM Applications
Agentic design patterns in LLM applications improve the abilities of language models by impacting resolute, aspirational behaviors. This introduction explores how these patterns can enhance user interactions, increase effectiveness, and nurture innovation in numerous industries. So, let’s know more in detail:
Explanation of Agentic Design Patterns
Agentic design patterns refer to a set of practices and structures that delegate AI systems to act more independently and effectively. These patterns structure AI agents to perform tasks, make decisions, and communicate with other systems in a more human-like and autonomous manner. By integrating these patterns, developers can create applications that are not only savvy but also more dependable and user-friendly.
Evolving Role of LLMs in App Development
Simple text generators were the starting point for LLMs, but they have come a long way since then. Today, numerous applications, from customer service bots to intricate data analysis tools, rely on them. Their capability to comprehend and generate human-like text has opened new horizons in application development, making tasks that once seemed futile now viable. Incorporating agentic design patterns into LLMs is the next step in this development, pushing the boundaries of what these models can accomplish.
Boosting LLM Capabilities with Agentic Workflows
Agentic workflows play a critical role in improving LLM capabilities. These workflows involve designing AI systems that can independently handle tasks, make informed choices, and grasp their experiences. By integrating agentic workflows, developers can ensure that LLMs are not only cognizant but also dynamic in their operations, leading to more effective applications.
The future of agentic workflows with LLMs is promising, with expected evolutions including more advanced reflection agents, enhanced multi-agent collaboration, and improved tool use. These expected advancements in agentic workflows will enable LLMs to strengthen their abilities, resulting in more effective applications.
But before we get too far into the future, let’s take a closer look at the tools that make these workflows possible right now.
Are you ready to use the full potential of Large Language Models (LLMs) for your venture? Understanding LLM Alignment is critical for creating AI that works smoothly and honorably. So, read now!
Tool Use in AI Agentic Workflows
The concept of “Agentic Design” in AI highlights creating systems that can work independently with a degree of initiative and objective. Tools and methods play a crucial role in developing such intelligent workflows, improving the overall capabilities and efficiency of AI agents. Here’s a detailed look at the key elements and contemplations in the tool used within AI agentic workflows:
Understanding Tool Use in Agentic Design
In the synopsis of AI agentic workflows, tool use stands as a critical radical design pattern. Suppose your AI is a sharp assistant, prepared to improve your workflow by smoothly incorporating numerous tools. This capability revolutionizes your AI from a simple communicative agent into a prominent, adaptable helper. By using various tools, your AI can execute intricate tasks, recover precise data, and sleek your productivity, making your communications more effective and impactful.
Examples of Tool Use: Web Searches, Databases, Productivity Tools
Let’s know some practical instances to embellish how tool use works. Picture yourself requiring the latest market trends; your AI conducts web searches to collect and condense this data rapidly. If you need precise customer data, it can access and query databases to retrieve the information you require. In addition, your AI can interface with workflow tools such as calendars, emails, and project management software, scheduling meetings, sending reminders, or updating task statuses. These instances emphasize how incorporating tool use can substantially boost the abilities of your AI, turning it into a versatile asset.
Prompting LLMs for Tool Selection
Prompting LLMs (Large Language Models) for involuntary tool selection is a developing art. You commence this process by giving your AI clear, brief instructions. For example, if you need a report on recent sales figures, you would prompt the AI with, "Create a report on sales figures for the last quarter." The AI then recognizes the most suitable tool–whether it’s a database query or a web search–to achieve this task. This involuntary selection process improves effectiveness, as the AI vigorously selects the best tools based on your requirements.
Evolution of Tool Functionality Beyond Basic Web Searches
AI tool use has developed far beyond mere web searches. At first, web search abilities permitted AIs to collect details from the Internet. However, modern AIs are now equipped with advanced tools that can execute specialized tasks. For instance, they can dissect datasets, generate visualizations, write code, and even counterfeit synopses based on the details they refine. This development has augmented the scope of what AI can accomplish, enabling it to aid more intricate and disparate productivity.
Managing Large-Scale Functions with Heuristics
When managing a comprehensive range of functions in large-scale AI enforcement, heuristics play a crucial role. Heuristics are inherently rule-of-thumb plans that can aid the AI in making decisions rapidly and effectively.
For example, if the AI regularly confronts similar types of tasks, heuristics enable it to determine patterns and apply the most efficient tool or technique involuntarily. This approach not only enhances the pace of task implementation but also ensures steadiness and precision in handling numerous functions. By using heuristics, you can manage the intricacies of large-scale AI operations, ensuring your AI remains a dependable and powerful tool in your productivity.
Now that we've covered the nuts and bolts of managing large-scale functions let's dive into the world of multi-agent collaboration and see how teamwork among AIs can elevate your projects.
Ready to boost your AI abilities? Find how custom guardrails can improve the performance, dependability, and security of your language models. Take the initial step towards smarter AI today. Read our article on Building And Implementing Custom LLM Guardrails and revolutionize your approach to LLMs!
Multi-agent Collaboration
In the swiftly developing field of AI, collaboration is not just a human attempt; it’s a key strategy for upgrading machine intelligence as well. Multi-agent collaboration in LLM-based applications depicts a cutting-edge approach where multiple language model agents operate together to solve intricate issues more effectively than ever before. Let’s take a look more in detail:
Introduction to Multi-agent Systems in LLM
Suppose a team of specialists operating together flawlessly to tackle an intricate issue. That’s what multi-agent systems in LLM applications bring to the table. Instead of depending on a single large language model to regulate everything, you envoy tasks to multiple specialized agents. These agents partner, each contributing their strengths, to offer a more effective solution. This collaboration approach not only improves performance but also opens up new potentiality for creativity in Artificial Intelligence.
Advantages of Delegating Complex Tasks to Multiple LLM Agents
By delegating intricate tasks to multiple LLM agents, you can use the power of expertise. Each agent can concentrate on a precise aspect of the issue, using its unique abilities to deliver exceptional outcomes. This division of labor reduces the cognitive load on individual agents, leading to rapid refining times and more precise results. In addition, this approach provides greater adaptability, as you can easily reconfigure the agents to acknowledge various challenges or incorporate new abilities as they arise.
Applications of Multi-Agent Systems in Software Development
In software development and problem-solving, multi-agent systems can transform the way you approach tasks. For example, in debugging, one agent could determine the bug, another could recommend potential solutions, and yet another could verify the solution. This collaborative effort boosts the evolution process and improves the quality of the final product. In problem-solving synopsis, multiple agents can operate together to explore various strategies, assess results, and assemble the most efficient solution. This approach is specifically valuable in intricate, flexible problems where a single agent might struggle to provide a thorough response.
Frameworks for Integrating Multiple Agents
To efficiently incorporate multiple agents, you need sturdy frameworks and mental models. One admired approach is the use of a central coordinator agent that manages the communication between skilled agents. This coordinator ensures that tasks are adequately delegated and that agents interact effectively. Another model involves decentralized coordination, where agents autonomously communicate based on predefined conventions. Both approaches have their advantages, and the choice relies on the precise needs of your application. Evolving a clear comprehension of these frameworks is critical for successful multi-agent incorporation.
From robust frameworks to practical examples, let's explore some emerging tools that are setting the standard for multi-agent systems.
Examples of Emerging Frameworks: AutoGen, Crew AI, LangGraph
Numerous emerging frameworks pave the way for more sophisticated multi-agent systems. AutoGen, for instance, automates the creation and management of agent workflows, making it easier to deploy and scale multi-agent systems. Crew AI concentrates on collaborative problem-solving, enabling agents to operate together in a highly coordinated manner. LangGraph, on the other hand, offers a graph-based approach to agent communication, permitting for more robust and adaptable coordination. These frameworks demonstrate the potential of multi-agent systems and provide applicable tools for enforcing them in real-globe applications.
Alright, let’s shift gears to another exciting aspect: reflection-based agents and how they can make your AI even smarter.
By comprehending and using these advanced concepts, you can unleash new levels of effectiveness and innovation in your Artificial Intelligence projects.
Unleash the full potential of your AI: Read our Practical Guide For Deploying LLMs In Production and revolutionize your venture with innovative technology. Get specialist perceptions, step-by-step guidelines, and demonstrated strategies to ensure a smooth and successful deployment. Begin transforming your operations today!
Reflection-based Agents
Let’s now know about Reflection-based agents in LLM-based applications:
Concept of Reflection in Improving AI Systems
Imagine if your Artificial Intelligence could think about its conceptions. That’s the core concept behind reflection in AI systems. By reflecting, an AI can assess its decisions, learn from its mistakes, and refine its procedures. This self-assessment makes the AI more flexible and smarter over time. Just like you might reflect on your day to enhance tomorrow, reflection aids AI systems in becoming more effective in handling intricate tasks.
System 1 and System 2 Architecture in LLMs
Think of your brain’s swift, instinctive answers as System 1 and your slower, more considered thinking as System 2. Large Language Models (LLMs) can be designed likewise. System 1 in LLMs handles rapid, involuntary answers, great for direct tasks. System 2 kicks in for more intricate problem-solving, needing deeper reasoning. This dual approach aids LLMs in equating pace and precision, ensuring they execute well under differing events.
Types of Reflection Agents: Basic, Reflexion, and LATS
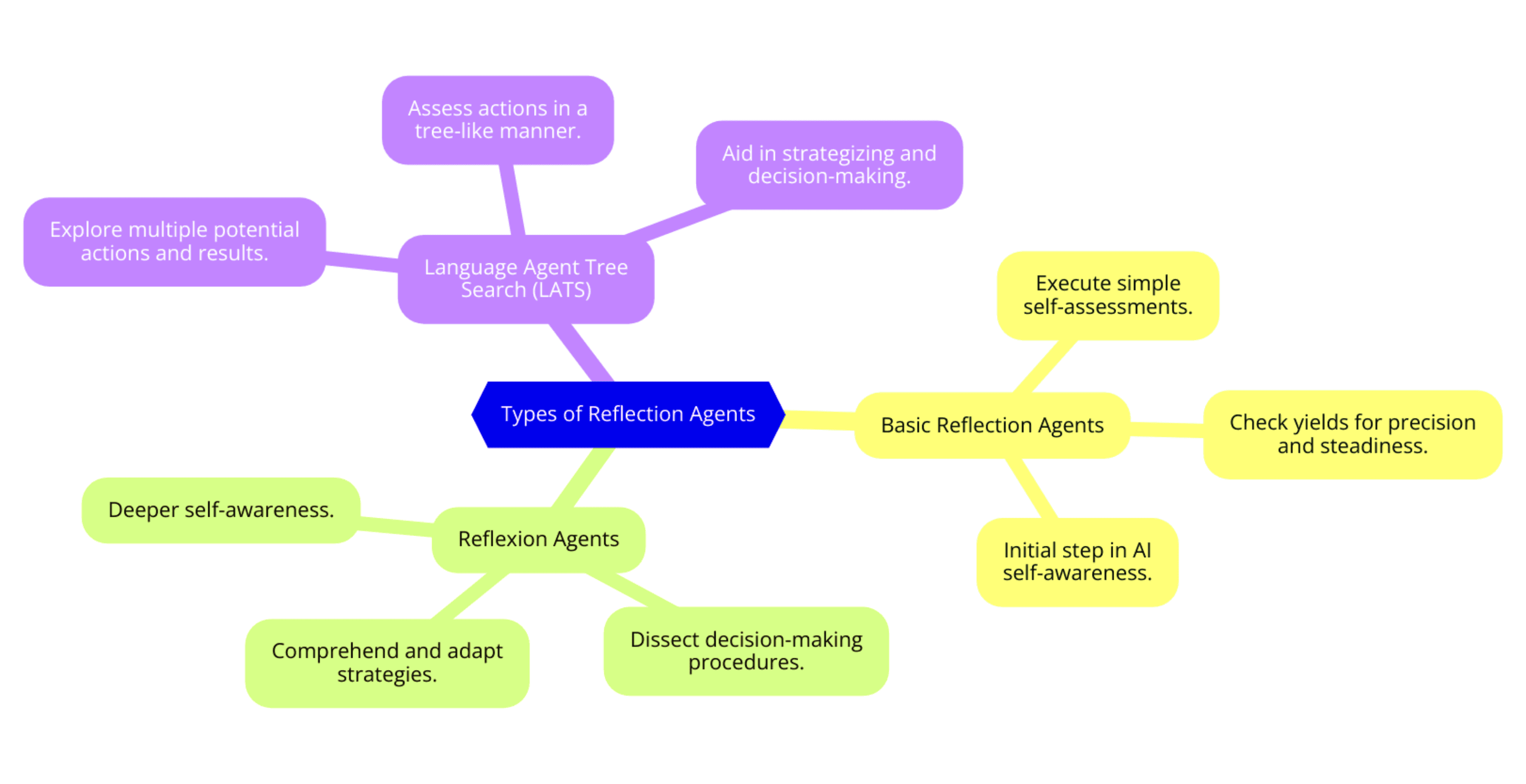
There are various types of reflection agents you can use to improve your AI:
Basic Reflection Agents: These agents execute simple self-assessments, checking their yields for precision and steadiness. They’re the initial step in making your AI more self-aware.
Reflexion Agents: These are more expanded and capable of deeper self-awareness. Reflexion agents can dissect their decision-making procedures, comprehend why certain decisions were made, and adapt their strategies appropriately.
Language Agent Tree Search (LATS): LATS agents take reflection to the next level. They can explore multiple potential actions and results, assessing them in a standardized, tree-like manner. This aids in strategizing and decision-making, specifically in intricate synopses where various paths are feasible.
Awesome, right? Now, let’s talk about how you can actually implement these reflection agents in your app development workflow.
Implementing Reflection Agents in App Development
Incorporating reflection agents into your applications can substantially elevate their performance. Begin by defining the scope of reflection for your AI. What decisions should it assess, and how often? Enforce basic reflection to observe and adapt yields. As your AI system matures, introduce Reflection agents to go deeper into its decision-making procedures. Eventually, use LATS for intricate problem-solving tasks, permitting your AI to contemplate different potentialities and results.
By integrating these reflection-based design patterns, you will create AI systems that not only perform tasks but also constantly enhance, becoming more effective and sharp with each communication. This dynamic flexibility makes reflection-based agents a groundbreaker in the world of AI development.
Ready to put this into practice? Let’s explore the role of an AI simulation assistant to see these concepts in action.
Unleash the full potential of your AI systems with innovative Large Action Models. Explore how they can revolutionize your venture today!
Implementing AI Simulation Assistant with Agentic Workflows
Have you ever considered having a sharp, intelligent assistant that can smoothly counterfeit intricate synopses and provide you with applicable perceptions, all powered by innovative AI and agentic workflows?
Overview of the Simulation Assistant Application
Ever wanted to have an AI simulation assistant at your fingertips that helps you model intricate synopses smoothly? This application uses advanced AI to provide pragmatic simulations for numerous requirements, from business predictions to educational tools. You ask, and the simulation assistant delivers, making intricate data understandable and applicable. The heart of this assistant is its capability to refine and simulate enormous amounts of information, giving you perceptions that are both deep and easy to comprehend.
Components and Roles of LLM-based Agents with Tools
The backbone of your AI simulation assistant comprises numerous key architectural elements, all operating in harmony. At the core, you have Large Language Model (LLM)- based agents, which are designed to comprehend and create human-like text. These agents don’t operate in solitude; specialized tools equip them to improve their abilities. Envision the LLM as the brain, capable of deep inspection and generation of intricate text, while the tools are like virtuoso advisors, offering accurate and domain-specific knowledge. Together, they form a sturdy system that can handle your most demanding simulation requests.
Life Cycle of an AI Assistant Simulation Request
When you capitulate a simulation request to your AI assistant, an appealing process starts. First, the request is analyzed and comprehended by the LLM-based agent. Then, it determines the significant tools and resources required to generate a precise simulation. This might involve accessing databases, running intricate algorithms, or even consulting other AI agents. Once the introductory information is collected, the simulation is created, with continuous processing and verification to ensure precision. Eventually, the outcomes are acquainted with you in a clear, easy-to-comprehend format, ready for your inspection and decision-making.
Simulation Workflow Integration Challenges and Solutions
Incorporating a simulation assistant into your existing productivity might seem challenging, but with the right approach, it’s quite tractable. One of the main challenges is ensuring smooth communication between the LLM-based agents and the tools they depend on. This requires sturdy APIs and well-defined protocols. In addition, maintaining data integrity and security is paramount, especially when handling sensitive data. To acknowledge these problems, you can enforce encryption and access controls, along with frequent inspections. Another obstacle is ensuring the AI’s yields are dependable and pertinent. This is tackled through constant training and updating of the LLMs and tools, ensuring they stay current with the latest information and techniques.
Future Directions for Simulation Assistant Development
Looking ahead, the future of AI simulation assistants is absolutely promising. You can anticipate these systems to become even more instinctive and receptive, able to handle more intricate and eclectic requests. Advances in machine learning and natural language processing will enable deeper comprehension and more precise simulations. In addition, the incorporation of real-time data sources will make simulations more robust and pertinent to your instant requirements. As these assistants develop, they will become invaluable tools in your decision-making arsenal, providing insights and foresight that are currently inconceivable. Enfold these progressions, and you will be at the vanguard of innovation, using AI to its fullest potential.
Before we wrap up, let's take a step back and look at what we've learned about agentic design patterns and their future outlook.
Conclusion and Future Outlook
Agentic design patterns hold enormous potential to improve the abilities of LLMs. They enable the evolution of smarter, more effective, and user-friendly applications that can handle intricate tasks autonomously.
Constant experimentation and evolution are critical in unleashing the full potential of LLM-based applications. By exploring new techniques and refining existing ones, developers can create more advanced and efficient AI systems.
The future of agentic workflows with LLMs is promising. We expect more advanced reflection agents, enhanced multi-agent collaboration, and improved tool use. These expansions will further improve LLMs' abilities, leading to more efficient applications.
But before you go, transform your testing workflow today with RagaAI’s intuitive platform. Sign up now for a free trial!
Hey there! Have you ever wondered how large language models (LLMs) such as GPT-4 manage to be so adaptable and effective? It’s all thanks to agentic design motifs. These patterns are transforming how we evolve and use applications with LLMs, making them more proficient and flexible. Let’s explore agentic design patterns further and see how they improve the abilities of LLMs in application development.
Don’t miss out on the chance to improve your skills and knowledge with these anticipating Large Language Models projects! Whether you’re a beginner or looking to amplify your skills, our well-chosen list of 20 LLM Project Ideas is designed to encourage and challenge you. Begin your expedition today and revolutionize your potential into reality.
Introduction to Agentic Design Patterns in LLM Applications
Agentic design patterns in LLM applications improve the abilities of language models by impacting resolute, aspirational behaviors. This introduction explores how these patterns can enhance user interactions, increase effectiveness, and nurture innovation in numerous industries. So, let’s know more in detail:
Explanation of Agentic Design Patterns
Agentic design patterns refer to a set of practices and structures that delegate AI systems to act more independently and effectively. These patterns structure AI agents to perform tasks, make decisions, and communicate with other systems in a more human-like and autonomous manner. By integrating these patterns, developers can create applications that are not only savvy but also more dependable and user-friendly.
Evolving Role of LLMs in App Development
Simple text generators were the starting point for LLMs, but they have come a long way since then. Today, numerous applications, from customer service bots to intricate data analysis tools, rely on them. Their capability to comprehend and generate human-like text has opened new horizons in application development, making tasks that once seemed futile now viable. Incorporating agentic design patterns into LLMs is the next step in this development, pushing the boundaries of what these models can accomplish.
Boosting LLM Capabilities with Agentic Workflows
Agentic workflows play a critical role in improving LLM capabilities. These workflows involve designing AI systems that can independently handle tasks, make informed choices, and grasp their experiences. By integrating agentic workflows, developers can ensure that LLMs are not only cognizant but also dynamic in their operations, leading to more effective applications.
The future of agentic workflows with LLMs is promising, with expected evolutions including more advanced reflection agents, enhanced multi-agent collaboration, and improved tool use. These expected advancements in agentic workflows will enable LLMs to strengthen their abilities, resulting in more effective applications.
But before we get too far into the future, let’s take a closer look at the tools that make these workflows possible right now.
Are you ready to use the full potential of Large Language Models (LLMs) for your venture? Understanding LLM Alignment is critical for creating AI that works smoothly and honorably. So, read now!
Tool Use in AI Agentic Workflows
The concept of “Agentic Design” in AI highlights creating systems that can work independently with a degree of initiative and objective. Tools and methods play a crucial role in developing such intelligent workflows, improving the overall capabilities and efficiency of AI agents. Here’s a detailed look at the key elements and contemplations in the tool used within AI agentic workflows:
Understanding Tool Use in Agentic Design
In the synopsis of AI agentic workflows, tool use stands as a critical radical design pattern. Suppose your AI is a sharp assistant, prepared to improve your workflow by smoothly incorporating numerous tools. This capability revolutionizes your AI from a simple communicative agent into a prominent, adaptable helper. By using various tools, your AI can execute intricate tasks, recover precise data, and sleek your productivity, making your communications more effective and impactful.
Examples of Tool Use: Web Searches, Databases, Productivity Tools
Let’s know some practical instances to embellish how tool use works. Picture yourself requiring the latest market trends; your AI conducts web searches to collect and condense this data rapidly. If you need precise customer data, it can access and query databases to retrieve the information you require. In addition, your AI can interface with workflow tools such as calendars, emails, and project management software, scheduling meetings, sending reminders, or updating task statuses. These instances emphasize how incorporating tool use can substantially boost the abilities of your AI, turning it into a versatile asset.
Prompting LLMs for Tool Selection
Prompting LLMs (Large Language Models) for involuntary tool selection is a developing art. You commence this process by giving your AI clear, brief instructions. For example, if you need a report on recent sales figures, you would prompt the AI with, "Create a report on sales figures for the last quarter." The AI then recognizes the most suitable tool–whether it’s a database query or a web search–to achieve this task. This involuntary selection process improves effectiveness, as the AI vigorously selects the best tools based on your requirements.
Evolution of Tool Functionality Beyond Basic Web Searches
AI tool use has developed far beyond mere web searches. At first, web search abilities permitted AIs to collect details from the Internet. However, modern AIs are now equipped with advanced tools that can execute specialized tasks. For instance, they can dissect datasets, generate visualizations, write code, and even counterfeit synopses based on the details they refine. This development has augmented the scope of what AI can accomplish, enabling it to aid more intricate and disparate productivity.
Managing Large-Scale Functions with Heuristics
When managing a comprehensive range of functions in large-scale AI enforcement, heuristics play a crucial role. Heuristics are inherently rule-of-thumb plans that can aid the AI in making decisions rapidly and effectively.
For example, if the AI regularly confronts similar types of tasks, heuristics enable it to determine patterns and apply the most efficient tool or technique involuntarily. This approach not only enhances the pace of task implementation but also ensures steadiness and precision in handling numerous functions. By using heuristics, you can manage the intricacies of large-scale AI operations, ensuring your AI remains a dependable and powerful tool in your productivity.
Now that we've covered the nuts and bolts of managing large-scale functions let's dive into the world of multi-agent collaboration and see how teamwork among AIs can elevate your projects.
Ready to boost your AI abilities? Find how custom guardrails can improve the performance, dependability, and security of your language models. Take the initial step towards smarter AI today. Read our article on Building And Implementing Custom LLM Guardrails and revolutionize your approach to LLMs!
Multi-agent Collaboration
In the swiftly developing field of AI, collaboration is not just a human attempt; it’s a key strategy for upgrading machine intelligence as well. Multi-agent collaboration in LLM-based applications depicts a cutting-edge approach where multiple language model agents operate together to solve intricate issues more effectively than ever before. Let’s take a look more in detail:
Introduction to Multi-agent Systems in LLM
Suppose a team of specialists operating together flawlessly to tackle an intricate issue. That’s what multi-agent systems in LLM applications bring to the table. Instead of depending on a single large language model to regulate everything, you envoy tasks to multiple specialized agents. These agents partner, each contributing their strengths, to offer a more effective solution. This collaboration approach not only improves performance but also opens up new potentiality for creativity in Artificial Intelligence.
Advantages of Delegating Complex Tasks to Multiple LLM Agents
By delegating intricate tasks to multiple LLM agents, you can use the power of expertise. Each agent can concentrate on a precise aspect of the issue, using its unique abilities to deliver exceptional outcomes. This division of labor reduces the cognitive load on individual agents, leading to rapid refining times and more precise results. In addition, this approach provides greater adaptability, as you can easily reconfigure the agents to acknowledge various challenges or incorporate new abilities as they arise.
Applications of Multi-Agent Systems in Software Development
In software development and problem-solving, multi-agent systems can transform the way you approach tasks. For example, in debugging, one agent could determine the bug, another could recommend potential solutions, and yet another could verify the solution. This collaborative effort boosts the evolution process and improves the quality of the final product. In problem-solving synopsis, multiple agents can operate together to explore various strategies, assess results, and assemble the most efficient solution. This approach is specifically valuable in intricate, flexible problems where a single agent might struggle to provide a thorough response.
Frameworks for Integrating Multiple Agents
To efficiently incorporate multiple agents, you need sturdy frameworks and mental models. One admired approach is the use of a central coordinator agent that manages the communication between skilled agents. This coordinator ensures that tasks are adequately delegated and that agents interact effectively. Another model involves decentralized coordination, where agents autonomously communicate based on predefined conventions. Both approaches have their advantages, and the choice relies on the precise needs of your application. Evolving a clear comprehension of these frameworks is critical for successful multi-agent incorporation.
From robust frameworks to practical examples, let's explore some emerging tools that are setting the standard for multi-agent systems.
Examples of Emerging Frameworks: AutoGen, Crew AI, LangGraph
Numerous emerging frameworks pave the way for more sophisticated multi-agent systems. AutoGen, for instance, automates the creation and management of agent workflows, making it easier to deploy and scale multi-agent systems. Crew AI concentrates on collaborative problem-solving, enabling agents to operate together in a highly coordinated manner. LangGraph, on the other hand, offers a graph-based approach to agent communication, permitting for more robust and adaptable coordination. These frameworks demonstrate the potential of multi-agent systems and provide applicable tools for enforcing them in real-globe applications.
Alright, let’s shift gears to another exciting aspect: reflection-based agents and how they can make your AI even smarter.
By comprehending and using these advanced concepts, you can unleash new levels of effectiveness and innovation in your Artificial Intelligence projects.
Unleash the full potential of your AI: Read our Practical Guide For Deploying LLMs In Production and revolutionize your venture with innovative technology. Get specialist perceptions, step-by-step guidelines, and demonstrated strategies to ensure a smooth and successful deployment. Begin transforming your operations today!
Reflection-based Agents
Let’s now know about Reflection-based agents in LLM-based applications:
Concept of Reflection in Improving AI Systems
Imagine if your Artificial Intelligence could think about its conceptions. That’s the core concept behind reflection in AI systems. By reflecting, an AI can assess its decisions, learn from its mistakes, and refine its procedures. This self-assessment makes the AI more flexible and smarter over time. Just like you might reflect on your day to enhance tomorrow, reflection aids AI systems in becoming more effective in handling intricate tasks.
System 1 and System 2 Architecture in LLMs
Think of your brain’s swift, instinctive answers as System 1 and your slower, more considered thinking as System 2. Large Language Models (LLMs) can be designed likewise. System 1 in LLMs handles rapid, involuntary answers, great for direct tasks. System 2 kicks in for more intricate problem-solving, needing deeper reasoning. This dual approach aids LLMs in equating pace and precision, ensuring they execute well under differing events.
Types of Reflection Agents: Basic, Reflexion, and LATS
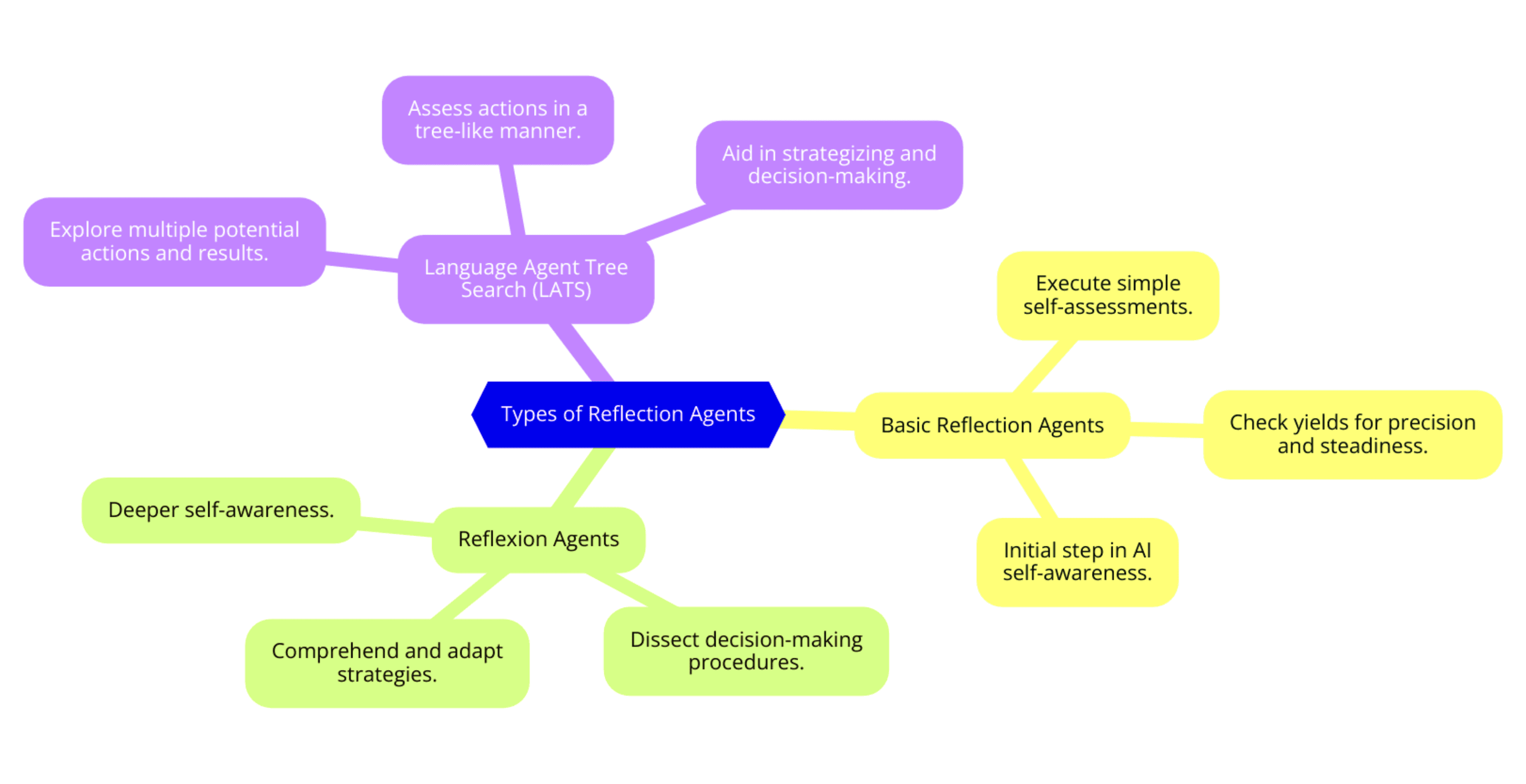
There are various types of reflection agents you can use to improve your AI:
Basic Reflection Agents: These agents execute simple self-assessments, checking their yields for precision and steadiness. They’re the initial step in making your AI more self-aware.
Reflexion Agents: These are more expanded and capable of deeper self-awareness. Reflexion agents can dissect their decision-making procedures, comprehend why certain decisions were made, and adapt their strategies appropriately.
Language Agent Tree Search (LATS): LATS agents take reflection to the next level. They can explore multiple potential actions and results, assessing them in a standardized, tree-like manner. This aids in strategizing and decision-making, specifically in intricate synopses where various paths are feasible.
Awesome, right? Now, let’s talk about how you can actually implement these reflection agents in your app development workflow.
Implementing Reflection Agents in App Development
Incorporating reflection agents into your applications can substantially elevate their performance. Begin by defining the scope of reflection for your AI. What decisions should it assess, and how often? Enforce basic reflection to observe and adapt yields. As your AI system matures, introduce Reflection agents to go deeper into its decision-making procedures. Eventually, use LATS for intricate problem-solving tasks, permitting your AI to contemplate different potentialities and results.
By integrating these reflection-based design patterns, you will create AI systems that not only perform tasks but also constantly enhance, becoming more effective and sharp with each communication. This dynamic flexibility makes reflection-based agents a groundbreaker in the world of AI development.
Ready to put this into practice? Let’s explore the role of an AI simulation assistant to see these concepts in action.
Unleash the full potential of your AI systems with innovative Large Action Models. Explore how they can revolutionize your venture today!
Implementing AI Simulation Assistant with Agentic Workflows
Have you ever considered having a sharp, intelligent assistant that can smoothly counterfeit intricate synopses and provide you with applicable perceptions, all powered by innovative AI and agentic workflows?
Overview of the Simulation Assistant Application
Ever wanted to have an AI simulation assistant at your fingertips that helps you model intricate synopses smoothly? This application uses advanced AI to provide pragmatic simulations for numerous requirements, from business predictions to educational tools. You ask, and the simulation assistant delivers, making intricate data understandable and applicable. The heart of this assistant is its capability to refine and simulate enormous amounts of information, giving you perceptions that are both deep and easy to comprehend.
Components and Roles of LLM-based Agents with Tools
The backbone of your AI simulation assistant comprises numerous key architectural elements, all operating in harmony. At the core, you have Large Language Model (LLM)- based agents, which are designed to comprehend and create human-like text. These agents don’t operate in solitude; specialized tools equip them to improve their abilities. Envision the LLM as the brain, capable of deep inspection and generation of intricate text, while the tools are like virtuoso advisors, offering accurate and domain-specific knowledge. Together, they form a sturdy system that can handle your most demanding simulation requests.
Life Cycle of an AI Assistant Simulation Request
When you capitulate a simulation request to your AI assistant, an appealing process starts. First, the request is analyzed and comprehended by the LLM-based agent. Then, it determines the significant tools and resources required to generate a precise simulation. This might involve accessing databases, running intricate algorithms, or even consulting other AI agents. Once the introductory information is collected, the simulation is created, with continuous processing and verification to ensure precision. Eventually, the outcomes are acquainted with you in a clear, easy-to-comprehend format, ready for your inspection and decision-making.
Simulation Workflow Integration Challenges and Solutions
Incorporating a simulation assistant into your existing productivity might seem challenging, but with the right approach, it’s quite tractable. One of the main challenges is ensuring smooth communication between the LLM-based agents and the tools they depend on. This requires sturdy APIs and well-defined protocols. In addition, maintaining data integrity and security is paramount, especially when handling sensitive data. To acknowledge these problems, you can enforce encryption and access controls, along with frequent inspections. Another obstacle is ensuring the AI’s yields are dependable and pertinent. This is tackled through constant training and updating of the LLMs and tools, ensuring they stay current with the latest information and techniques.
Future Directions for Simulation Assistant Development
Looking ahead, the future of AI simulation assistants is absolutely promising. You can anticipate these systems to become even more instinctive and receptive, able to handle more intricate and eclectic requests. Advances in machine learning and natural language processing will enable deeper comprehension and more precise simulations. In addition, the incorporation of real-time data sources will make simulations more robust and pertinent to your instant requirements. As these assistants develop, they will become invaluable tools in your decision-making arsenal, providing insights and foresight that are currently inconceivable. Enfold these progressions, and you will be at the vanguard of innovation, using AI to its fullest potential.
Before we wrap up, let's take a step back and look at what we've learned about agentic design patterns and their future outlook.
Conclusion and Future Outlook
Agentic design patterns hold enormous potential to improve the abilities of LLMs. They enable the evolution of smarter, more effective, and user-friendly applications that can handle intricate tasks autonomously.
Constant experimentation and evolution are critical in unleashing the full potential of LLM-based applications. By exploring new techniques and refining existing ones, developers can create more advanced and efficient AI systems.
The future of agentic workflows with LLMs is promising. We expect more advanced reflection agents, enhanced multi-agent collaboration, and improved tool use. These expansions will further improve LLMs' abilities, leading to more efficient applications.
But before you go, transform your testing workflow today with RagaAI’s intuitive platform. Sign up now for a free trial!
Hey there! Have you ever wondered how large language models (LLMs) such as GPT-4 manage to be so adaptable and effective? It’s all thanks to agentic design motifs. These patterns are transforming how we evolve and use applications with LLMs, making them more proficient and flexible. Let’s explore agentic design patterns further and see how they improve the abilities of LLMs in application development.
Don’t miss out on the chance to improve your skills and knowledge with these anticipating Large Language Models projects! Whether you’re a beginner or looking to amplify your skills, our well-chosen list of 20 LLM Project Ideas is designed to encourage and challenge you. Begin your expedition today and revolutionize your potential into reality.
Introduction to Agentic Design Patterns in LLM Applications
Agentic design patterns in LLM applications improve the abilities of language models by impacting resolute, aspirational behaviors. This introduction explores how these patterns can enhance user interactions, increase effectiveness, and nurture innovation in numerous industries. So, let’s know more in detail:
Explanation of Agentic Design Patterns
Agentic design patterns refer to a set of practices and structures that delegate AI systems to act more independently and effectively. These patterns structure AI agents to perform tasks, make decisions, and communicate with other systems in a more human-like and autonomous manner. By integrating these patterns, developers can create applications that are not only savvy but also more dependable and user-friendly.
Evolving Role of LLMs in App Development
Simple text generators were the starting point for LLMs, but they have come a long way since then. Today, numerous applications, from customer service bots to intricate data analysis tools, rely on them. Their capability to comprehend and generate human-like text has opened new horizons in application development, making tasks that once seemed futile now viable. Incorporating agentic design patterns into LLMs is the next step in this development, pushing the boundaries of what these models can accomplish.
Boosting LLM Capabilities with Agentic Workflows
Agentic workflows play a critical role in improving LLM capabilities. These workflows involve designing AI systems that can independently handle tasks, make informed choices, and grasp their experiences. By integrating agentic workflows, developers can ensure that LLMs are not only cognizant but also dynamic in their operations, leading to more effective applications.
The future of agentic workflows with LLMs is promising, with expected evolutions including more advanced reflection agents, enhanced multi-agent collaboration, and improved tool use. These expected advancements in agentic workflows will enable LLMs to strengthen their abilities, resulting in more effective applications.
But before we get too far into the future, let’s take a closer look at the tools that make these workflows possible right now.
Are you ready to use the full potential of Large Language Models (LLMs) for your venture? Understanding LLM Alignment is critical for creating AI that works smoothly and honorably. So, read now!
Tool Use in AI Agentic Workflows
The concept of “Agentic Design” in AI highlights creating systems that can work independently with a degree of initiative and objective. Tools and methods play a crucial role in developing such intelligent workflows, improving the overall capabilities and efficiency of AI agents. Here’s a detailed look at the key elements and contemplations in the tool used within AI agentic workflows:
Understanding Tool Use in Agentic Design
In the synopsis of AI agentic workflows, tool use stands as a critical radical design pattern. Suppose your AI is a sharp assistant, prepared to improve your workflow by smoothly incorporating numerous tools. This capability revolutionizes your AI from a simple communicative agent into a prominent, adaptable helper. By using various tools, your AI can execute intricate tasks, recover precise data, and sleek your productivity, making your communications more effective and impactful.
Examples of Tool Use: Web Searches, Databases, Productivity Tools
Let’s know some practical instances to embellish how tool use works. Picture yourself requiring the latest market trends; your AI conducts web searches to collect and condense this data rapidly. If you need precise customer data, it can access and query databases to retrieve the information you require. In addition, your AI can interface with workflow tools such as calendars, emails, and project management software, scheduling meetings, sending reminders, or updating task statuses. These instances emphasize how incorporating tool use can substantially boost the abilities of your AI, turning it into a versatile asset.
Prompting LLMs for Tool Selection
Prompting LLMs (Large Language Models) for involuntary tool selection is a developing art. You commence this process by giving your AI clear, brief instructions. For example, if you need a report on recent sales figures, you would prompt the AI with, "Create a report on sales figures for the last quarter." The AI then recognizes the most suitable tool–whether it’s a database query or a web search–to achieve this task. This involuntary selection process improves effectiveness, as the AI vigorously selects the best tools based on your requirements.
Evolution of Tool Functionality Beyond Basic Web Searches
AI tool use has developed far beyond mere web searches. At first, web search abilities permitted AIs to collect details from the Internet. However, modern AIs are now equipped with advanced tools that can execute specialized tasks. For instance, they can dissect datasets, generate visualizations, write code, and even counterfeit synopses based on the details they refine. This development has augmented the scope of what AI can accomplish, enabling it to aid more intricate and disparate productivity.
Managing Large-Scale Functions with Heuristics
When managing a comprehensive range of functions in large-scale AI enforcement, heuristics play a crucial role. Heuristics are inherently rule-of-thumb plans that can aid the AI in making decisions rapidly and effectively.
For example, if the AI regularly confronts similar types of tasks, heuristics enable it to determine patterns and apply the most efficient tool or technique involuntarily. This approach not only enhances the pace of task implementation but also ensures steadiness and precision in handling numerous functions. By using heuristics, you can manage the intricacies of large-scale AI operations, ensuring your AI remains a dependable and powerful tool in your productivity.
Now that we've covered the nuts and bolts of managing large-scale functions let's dive into the world of multi-agent collaboration and see how teamwork among AIs can elevate your projects.
Ready to boost your AI abilities? Find how custom guardrails can improve the performance, dependability, and security of your language models. Take the initial step towards smarter AI today. Read our article on Building And Implementing Custom LLM Guardrails and revolutionize your approach to LLMs!
Multi-agent Collaboration
In the swiftly developing field of AI, collaboration is not just a human attempt; it’s a key strategy for upgrading machine intelligence as well. Multi-agent collaboration in LLM-based applications depicts a cutting-edge approach where multiple language model agents operate together to solve intricate issues more effectively than ever before. Let’s take a look more in detail:
Introduction to Multi-agent Systems in LLM
Suppose a team of specialists operating together flawlessly to tackle an intricate issue. That’s what multi-agent systems in LLM applications bring to the table. Instead of depending on a single large language model to regulate everything, you envoy tasks to multiple specialized agents. These agents partner, each contributing their strengths, to offer a more effective solution. This collaboration approach not only improves performance but also opens up new potentiality for creativity in Artificial Intelligence.
Advantages of Delegating Complex Tasks to Multiple LLM Agents
By delegating intricate tasks to multiple LLM agents, you can use the power of expertise. Each agent can concentrate on a precise aspect of the issue, using its unique abilities to deliver exceptional outcomes. This division of labor reduces the cognitive load on individual agents, leading to rapid refining times and more precise results. In addition, this approach provides greater adaptability, as you can easily reconfigure the agents to acknowledge various challenges or incorporate new abilities as they arise.
Applications of Multi-Agent Systems in Software Development
In software development and problem-solving, multi-agent systems can transform the way you approach tasks. For example, in debugging, one agent could determine the bug, another could recommend potential solutions, and yet another could verify the solution. This collaborative effort boosts the evolution process and improves the quality of the final product. In problem-solving synopsis, multiple agents can operate together to explore various strategies, assess results, and assemble the most efficient solution. This approach is specifically valuable in intricate, flexible problems where a single agent might struggle to provide a thorough response.
Frameworks for Integrating Multiple Agents
To efficiently incorporate multiple agents, you need sturdy frameworks and mental models. One admired approach is the use of a central coordinator agent that manages the communication between skilled agents. This coordinator ensures that tasks are adequately delegated and that agents interact effectively. Another model involves decentralized coordination, where agents autonomously communicate based on predefined conventions. Both approaches have their advantages, and the choice relies on the precise needs of your application. Evolving a clear comprehension of these frameworks is critical for successful multi-agent incorporation.
From robust frameworks to practical examples, let's explore some emerging tools that are setting the standard for multi-agent systems.
Examples of Emerging Frameworks: AutoGen, Crew AI, LangGraph
Numerous emerging frameworks pave the way for more sophisticated multi-agent systems. AutoGen, for instance, automates the creation and management of agent workflows, making it easier to deploy and scale multi-agent systems. Crew AI concentrates on collaborative problem-solving, enabling agents to operate together in a highly coordinated manner. LangGraph, on the other hand, offers a graph-based approach to agent communication, permitting for more robust and adaptable coordination. These frameworks demonstrate the potential of multi-agent systems and provide applicable tools for enforcing them in real-globe applications.
Alright, let’s shift gears to another exciting aspect: reflection-based agents and how they can make your AI even smarter.
By comprehending and using these advanced concepts, you can unleash new levels of effectiveness and innovation in your Artificial Intelligence projects.
Unleash the full potential of your AI: Read our Practical Guide For Deploying LLMs In Production and revolutionize your venture with innovative technology. Get specialist perceptions, step-by-step guidelines, and demonstrated strategies to ensure a smooth and successful deployment. Begin transforming your operations today!
Reflection-based Agents
Let’s now know about Reflection-based agents in LLM-based applications:
Concept of Reflection in Improving AI Systems
Imagine if your Artificial Intelligence could think about its conceptions. That’s the core concept behind reflection in AI systems. By reflecting, an AI can assess its decisions, learn from its mistakes, and refine its procedures. This self-assessment makes the AI more flexible and smarter over time. Just like you might reflect on your day to enhance tomorrow, reflection aids AI systems in becoming more effective in handling intricate tasks.
System 1 and System 2 Architecture in LLMs
Think of your brain’s swift, instinctive answers as System 1 and your slower, more considered thinking as System 2. Large Language Models (LLMs) can be designed likewise. System 1 in LLMs handles rapid, involuntary answers, great for direct tasks. System 2 kicks in for more intricate problem-solving, needing deeper reasoning. This dual approach aids LLMs in equating pace and precision, ensuring they execute well under differing events.
Types of Reflection Agents: Basic, Reflexion, and LATS
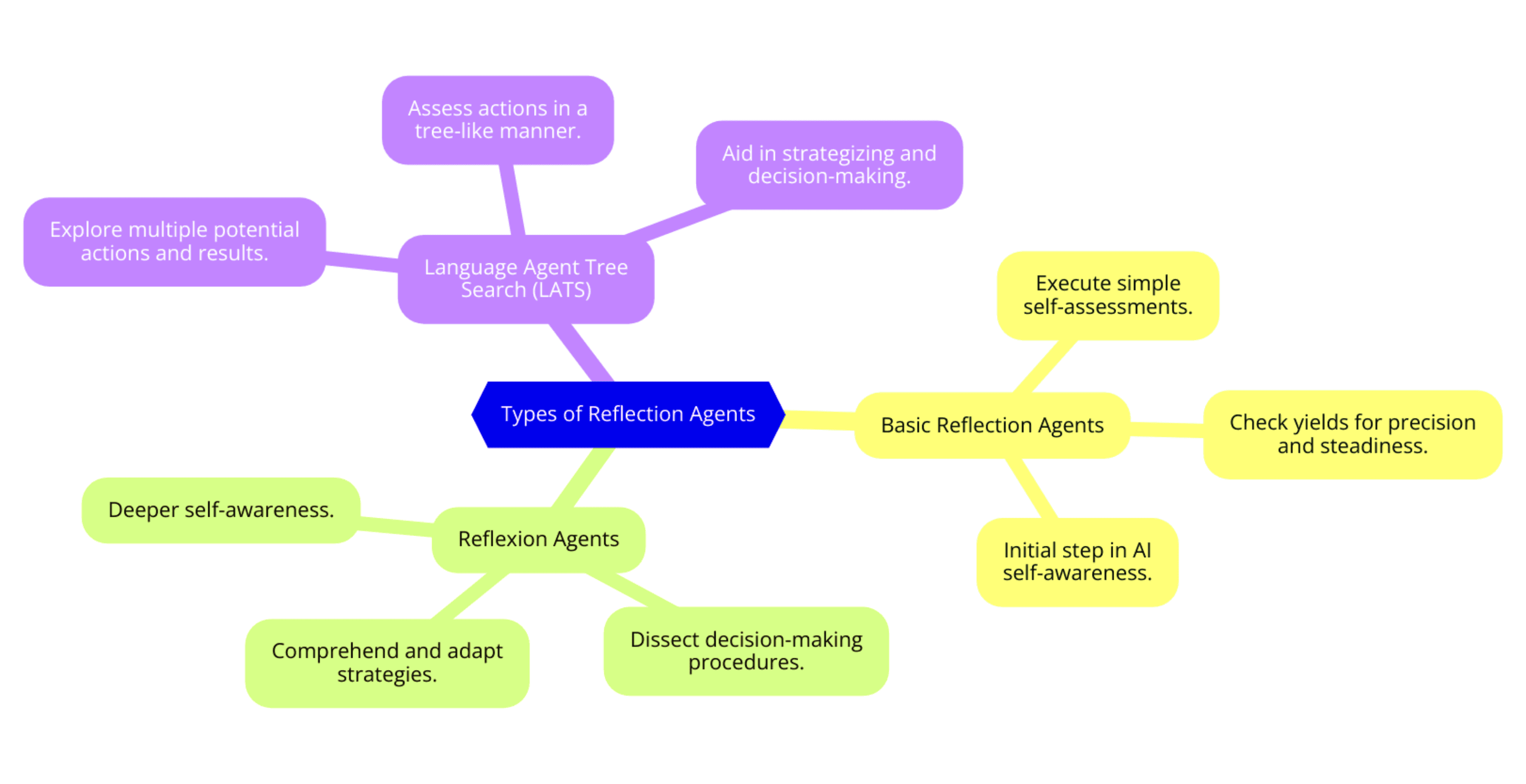
There are various types of reflection agents you can use to improve your AI:
Basic Reflection Agents: These agents execute simple self-assessments, checking their yields for precision and steadiness. They’re the initial step in making your AI more self-aware.
Reflexion Agents: These are more expanded and capable of deeper self-awareness. Reflexion agents can dissect their decision-making procedures, comprehend why certain decisions were made, and adapt their strategies appropriately.
Language Agent Tree Search (LATS): LATS agents take reflection to the next level. They can explore multiple potential actions and results, assessing them in a standardized, tree-like manner. This aids in strategizing and decision-making, specifically in intricate synopses where various paths are feasible.
Awesome, right? Now, let’s talk about how you can actually implement these reflection agents in your app development workflow.
Implementing Reflection Agents in App Development
Incorporating reflection agents into your applications can substantially elevate their performance. Begin by defining the scope of reflection for your AI. What decisions should it assess, and how often? Enforce basic reflection to observe and adapt yields. As your AI system matures, introduce Reflection agents to go deeper into its decision-making procedures. Eventually, use LATS for intricate problem-solving tasks, permitting your AI to contemplate different potentialities and results.
By integrating these reflection-based design patterns, you will create AI systems that not only perform tasks but also constantly enhance, becoming more effective and sharp with each communication. This dynamic flexibility makes reflection-based agents a groundbreaker in the world of AI development.
Ready to put this into practice? Let’s explore the role of an AI simulation assistant to see these concepts in action.
Unleash the full potential of your AI systems with innovative Large Action Models. Explore how they can revolutionize your venture today!
Implementing AI Simulation Assistant with Agentic Workflows
Have you ever considered having a sharp, intelligent assistant that can smoothly counterfeit intricate synopses and provide you with applicable perceptions, all powered by innovative AI and agentic workflows?
Overview of the Simulation Assistant Application
Ever wanted to have an AI simulation assistant at your fingertips that helps you model intricate synopses smoothly? This application uses advanced AI to provide pragmatic simulations for numerous requirements, from business predictions to educational tools. You ask, and the simulation assistant delivers, making intricate data understandable and applicable. The heart of this assistant is its capability to refine and simulate enormous amounts of information, giving you perceptions that are both deep and easy to comprehend.
Components and Roles of LLM-based Agents with Tools
The backbone of your AI simulation assistant comprises numerous key architectural elements, all operating in harmony. At the core, you have Large Language Model (LLM)- based agents, which are designed to comprehend and create human-like text. These agents don’t operate in solitude; specialized tools equip them to improve their abilities. Envision the LLM as the brain, capable of deep inspection and generation of intricate text, while the tools are like virtuoso advisors, offering accurate and domain-specific knowledge. Together, they form a sturdy system that can handle your most demanding simulation requests.
Life Cycle of an AI Assistant Simulation Request
When you capitulate a simulation request to your AI assistant, an appealing process starts. First, the request is analyzed and comprehended by the LLM-based agent. Then, it determines the significant tools and resources required to generate a precise simulation. This might involve accessing databases, running intricate algorithms, or even consulting other AI agents. Once the introductory information is collected, the simulation is created, with continuous processing and verification to ensure precision. Eventually, the outcomes are acquainted with you in a clear, easy-to-comprehend format, ready for your inspection and decision-making.
Simulation Workflow Integration Challenges and Solutions
Incorporating a simulation assistant into your existing productivity might seem challenging, but with the right approach, it’s quite tractable. One of the main challenges is ensuring smooth communication between the LLM-based agents and the tools they depend on. This requires sturdy APIs and well-defined protocols. In addition, maintaining data integrity and security is paramount, especially when handling sensitive data. To acknowledge these problems, you can enforce encryption and access controls, along with frequent inspections. Another obstacle is ensuring the AI’s yields are dependable and pertinent. This is tackled through constant training and updating of the LLMs and tools, ensuring they stay current with the latest information and techniques.
Future Directions for Simulation Assistant Development
Looking ahead, the future of AI simulation assistants is absolutely promising. You can anticipate these systems to become even more instinctive and receptive, able to handle more intricate and eclectic requests. Advances in machine learning and natural language processing will enable deeper comprehension and more precise simulations. In addition, the incorporation of real-time data sources will make simulations more robust and pertinent to your instant requirements. As these assistants develop, they will become invaluable tools in your decision-making arsenal, providing insights and foresight that are currently inconceivable. Enfold these progressions, and you will be at the vanguard of innovation, using AI to its fullest potential.
Before we wrap up, let's take a step back and look at what we've learned about agentic design patterns and their future outlook.
Conclusion and Future Outlook
Agentic design patterns hold enormous potential to improve the abilities of LLMs. They enable the evolution of smarter, more effective, and user-friendly applications that can handle intricate tasks autonomously.
Constant experimentation and evolution are critical in unleashing the full potential of LLM-based applications. By exploring new techniques and refining existing ones, developers can create more advanced and efficient AI systems.
The future of agentic workflows with LLMs is promising. We expect more advanced reflection agents, enhanced multi-agent collaboration, and improved tool use. These expansions will further improve LLMs' abilities, leading to more efficient applications.
But before you go, transform your testing workflow today with RagaAI’s intuitive platform. Sign up now for a free trial!
Hey there! Have you ever wondered how large language models (LLMs) such as GPT-4 manage to be so adaptable and effective? It’s all thanks to agentic design motifs. These patterns are transforming how we evolve and use applications with LLMs, making them more proficient and flexible. Let’s explore agentic design patterns further and see how they improve the abilities of LLMs in application development.
Don’t miss out on the chance to improve your skills and knowledge with these anticipating Large Language Models projects! Whether you’re a beginner or looking to amplify your skills, our well-chosen list of 20 LLM Project Ideas is designed to encourage and challenge you. Begin your expedition today and revolutionize your potential into reality.
Introduction to Agentic Design Patterns in LLM Applications
Agentic design patterns in LLM applications improve the abilities of language models by impacting resolute, aspirational behaviors. This introduction explores how these patterns can enhance user interactions, increase effectiveness, and nurture innovation in numerous industries. So, let’s know more in detail:
Explanation of Agentic Design Patterns
Agentic design patterns refer to a set of practices and structures that delegate AI systems to act more independently and effectively. These patterns structure AI agents to perform tasks, make decisions, and communicate with other systems in a more human-like and autonomous manner. By integrating these patterns, developers can create applications that are not only savvy but also more dependable and user-friendly.
Evolving Role of LLMs in App Development
Simple text generators were the starting point for LLMs, but they have come a long way since then. Today, numerous applications, from customer service bots to intricate data analysis tools, rely on them. Their capability to comprehend and generate human-like text has opened new horizons in application development, making tasks that once seemed futile now viable. Incorporating agentic design patterns into LLMs is the next step in this development, pushing the boundaries of what these models can accomplish.
Boosting LLM Capabilities with Agentic Workflows
Agentic workflows play a critical role in improving LLM capabilities. These workflows involve designing AI systems that can independently handle tasks, make informed choices, and grasp their experiences. By integrating agentic workflows, developers can ensure that LLMs are not only cognizant but also dynamic in their operations, leading to more effective applications.
The future of agentic workflows with LLMs is promising, with expected evolutions including more advanced reflection agents, enhanced multi-agent collaboration, and improved tool use. These expected advancements in agentic workflows will enable LLMs to strengthen their abilities, resulting in more effective applications.
But before we get too far into the future, let’s take a closer look at the tools that make these workflows possible right now.
Are you ready to use the full potential of Large Language Models (LLMs) for your venture? Understanding LLM Alignment is critical for creating AI that works smoothly and honorably. So, read now!
Tool Use in AI Agentic Workflows
The concept of “Agentic Design” in AI highlights creating systems that can work independently with a degree of initiative and objective. Tools and methods play a crucial role in developing such intelligent workflows, improving the overall capabilities and efficiency of AI agents. Here’s a detailed look at the key elements and contemplations in the tool used within AI agentic workflows:
Understanding Tool Use in Agentic Design
In the synopsis of AI agentic workflows, tool use stands as a critical radical design pattern. Suppose your AI is a sharp assistant, prepared to improve your workflow by smoothly incorporating numerous tools. This capability revolutionizes your AI from a simple communicative agent into a prominent, adaptable helper. By using various tools, your AI can execute intricate tasks, recover precise data, and sleek your productivity, making your communications more effective and impactful.
Examples of Tool Use: Web Searches, Databases, Productivity Tools
Let’s know some practical instances to embellish how tool use works. Picture yourself requiring the latest market trends; your AI conducts web searches to collect and condense this data rapidly. If you need precise customer data, it can access and query databases to retrieve the information you require. In addition, your AI can interface with workflow tools such as calendars, emails, and project management software, scheduling meetings, sending reminders, or updating task statuses. These instances emphasize how incorporating tool use can substantially boost the abilities of your AI, turning it into a versatile asset.
Prompting LLMs for Tool Selection
Prompting LLMs (Large Language Models) for involuntary tool selection is a developing art. You commence this process by giving your AI clear, brief instructions. For example, if you need a report on recent sales figures, you would prompt the AI with, "Create a report on sales figures for the last quarter." The AI then recognizes the most suitable tool–whether it’s a database query or a web search–to achieve this task. This involuntary selection process improves effectiveness, as the AI vigorously selects the best tools based on your requirements.
Evolution of Tool Functionality Beyond Basic Web Searches
AI tool use has developed far beyond mere web searches. At first, web search abilities permitted AIs to collect details from the Internet. However, modern AIs are now equipped with advanced tools that can execute specialized tasks. For instance, they can dissect datasets, generate visualizations, write code, and even counterfeit synopses based on the details they refine. This development has augmented the scope of what AI can accomplish, enabling it to aid more intricate and disparate productivity.
Managing Large-Scale Functions with Heuristics
When managing a comprehensive range of functions in large-scale AI enforcement, heuristics play a crucial role. Heuristics are inherently rule-of-thumb plans that can aid the AI in making decisions rapidly and effectively.
For example, if the AI regularly confronts similar types of tasks, heuristics enable it to determine patterns and apply the most efficient tool or technique involuntarily. This approach not only enhances the pace of task implementation but also ensures steadiness and precision in handling numerous functions. By using heuristics, you can manage the intricacies of large-scale AI operations, ensuring your AI remains a dependable and powerful tool in your productivity.
Now that we've covered the nuts and bolts of managing large-scale functions let's dive into the world of multi-agent collaboration and see how teamwork among AIs can elevate your projects.
Ready to boost your AI abilities? Find how custom guardrails can improve the performance, dependability, and security of your language models. Take the initial step towards smarter AI today. Read our article on Building And Implementing Custom LLM Guardrails and revolutionize your approach to LLMs!
Multi-agent Collaboration
In the swiftly developing field of AI, collaboration is not just a human attempt; it’s a key strategy for upgrading machine intelligence as well. Multi-agent collaboration in LLM-based applications depicts a cutting-edge approach where multiple language model agents operate together to solve intricate issues more effectively than ever before. Let’s take a look more in detail:
Introduction to Multi-agent Systems in LLM
Suppose a team of specialists operating together flawlessly to tackle an intricate issue. That’s what multi-agent systems in LLM applications bring to the table. Instead of depending on a single large language model to regulate everything, you envoy tasks to multiple specialized agents. These agents partner, each contributing their strengths, to offer a more effective solution. This collaboration approach not only improves performance but also opens up new potentiality for creativity in Artificial Intelligence.
Advantages of Delegating Complex Tasks to Multiple LLM Agents
By delegating intricate tasks to multiple LLM agents, you can use the power of expertise. Each agent can concentrate on a precise aspect of the issue, using its unique abilities to deliver exceptional outcomes. This division of labor reduces the cognitive load on individual agents, leading to rapid refining times and more precise results. In addition, this approach provides greater adaptability, as you can easily reconfigure the agents to acknowledge various challenges or incorporate new abilities as they arise.
Applications of Multi-Agent Systems in Software Development
In software development and problem-solving, multi-agent systems can transform the way you approach tasks. For example, in debugging, one agent could determine the bug, another could recommend potential solutions, and yet another could verify the solution. This collaborative effort boosts the evolution process and improves the quality of the final product. In problem-solving synopsis, multiple agents can operate together to explore various strategies, assess results, and assemble the most efficient solution. This approach is specifically valuable in intricate, flexible problems where a single agent might struggle to provide a thorough response.
Frameworks for Integrating Multiple Agents
To efficiently incorporate multiple agents, you need sturdy frameworks and mental models. One admired approach is the use of a central coordinator agent that manages the communication between skilled agents. This coordinator ensures that tasks are adequately delegated and that agents interact effectively. Another model involves decentralized coordination, where agents autonomously communicate based on predefined conventions. Both approaches have their advantages, and the choice relies on the precise needs of your application. Evolving a clear comprehension of these frameworks is critical for successful multi-agent incorporation.
From robust frameworks to practical examples, let's explore some emerging tools that are setting the standard for multi-agent systems.
Examples of Emerging Frameworks: AutoGen, Crew AI, LangGraph
Numerous emerging frameworks pave the way for more sophisticated multi-agent systems. AutoGen, for instance, automates the creation and management of agent workflows, making it easier to deploy and scale multi-agent systems. Crew AI concentrates on collaborative problem-solving, enabling agents to operate together in a highly coordinated manner. LangGraph, on the other hand, offers a graph-based approach to agent communication, permitting for more robust and adaptable coordination. These frameworks demonstrate the potential of multi-agent systems and provide applicable tools for enforcing them in real-globe applications.
Alright, let’s shift gears to another exciting aspect: reflection-based agents and how they can make your AI even smarter.
By comprehending and using these advanced concepts, you can unleash new levels of effectiveness and innovation in your Artificial Intelligence projects.
Unleash the full potential of your AI: Read our Practical Guide For Deploying LLMs In Production and revolutionize your venture with innovative technology. Get specialist perceptions, step-by-step guidelines, and demonstrated strategies to ensure a smooth and successful deployment. Begin transforming your operations today!
Reflection-based Agents
Let’s now know about Reflection-based agents in LLM-based applications:
Concept of Reflection in Improving AI Systems
Imagine if your Artificial Intelligence could think about its conceptions. That’s the core concept behind reflection in AI systems. By reflecting, an AI can assess its decisions, learn from its mistakes, and refine its procedures. This self-assessment makes the AI more flexible and smarter over time. Just like you might reflect on your day to enhance tomorrow, reflection aids AI systems in becoming more effective in handling intricate tasks.
System 1 and System 2 Architecture in LLMs
Think of your brain’s swift, instinctive answers as System 1 and your slower, more considered thinking as System 2. Large Language Models (LLMs) can be designed likewise. System 1 in LLMs handles rapid, involuntary answers, great for direct tasks. System 2 kicks in for more intricate problem-solving, needing deeper reasoning. This dual approach aids LLMs in equating pace and precision, ensuring they execute well under differing events.
Types of Reflection Agents: Basic, Reflexion, and LATS
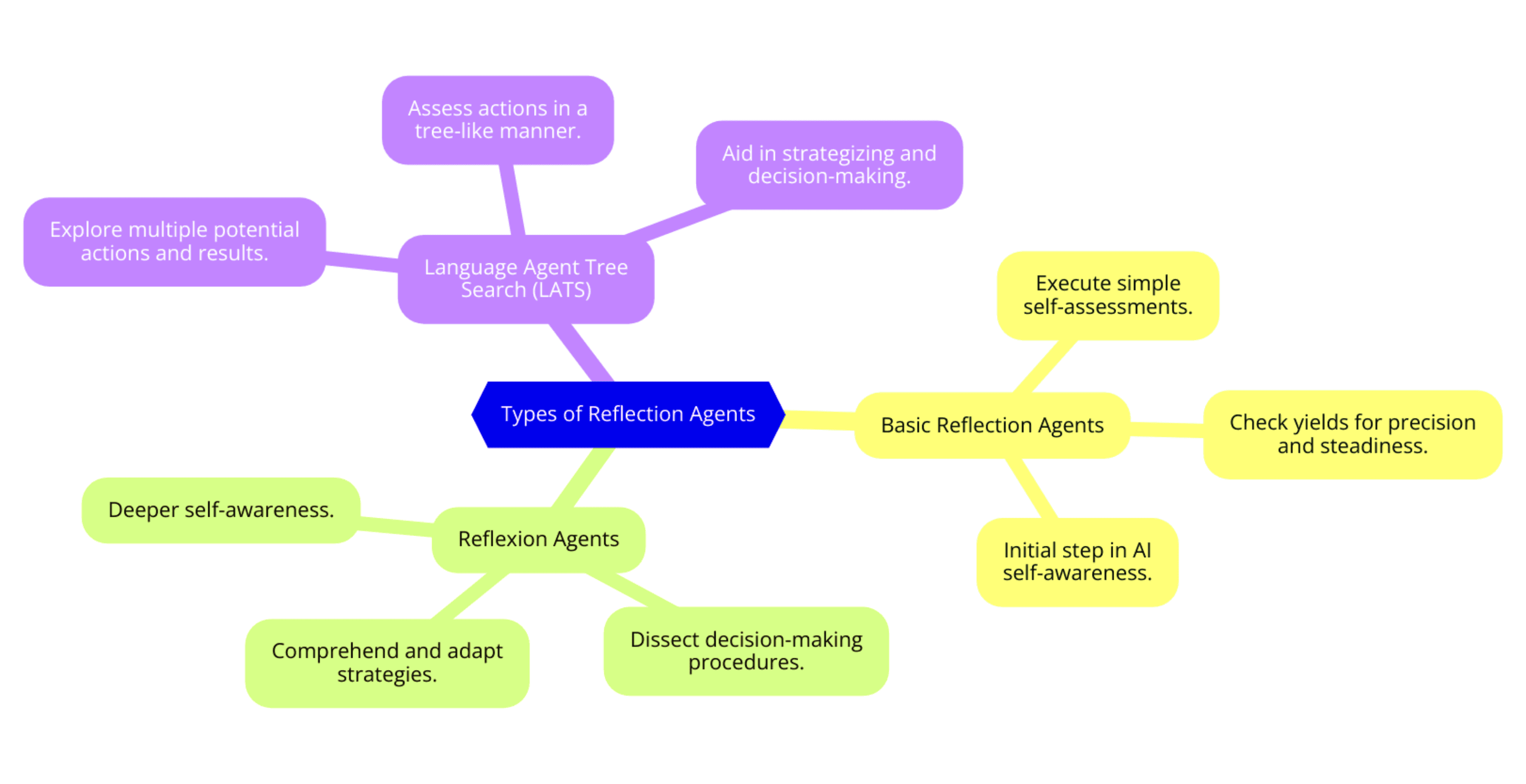
There are various types of reflection agents you can use to improve your AI:
Basic Reflection Agents: These agents execute simple self-assessments, checking their yields for precision and steadiness. They’re the initial step in making your AI more self-aware.
Reflexion Agents: These are more expanded and capable of deeper self-awareness. Reflexion agents can dissect their decision-making procedures, comprehend why certain decisions were made, and adapt their strategies appropriately.
Language Agent Tree Search (LATS): LATS agents take reflection to the next level. They can explore multiple potential actions and results, assessing them in a standardized, tree-like manner. This aids in strategizing and decision-making, specifically in intricate synopses where various paths are feasible.
Awesome, right? Now, let’s talk about how you can actually implement these reflection agents in your app development workflow.
Implementing Reflection Agents in App Development
Incorporating reflection agents into your applications can substantially elevate their performance. Begin by defining the scope of reflection for your AI. What decisions should it assess, and how often? Enforce basic reflection to observe and adapt yields. As your AI system matures, introduce Reflection agents to go deeper into its decision-making procedures. Eventually, use LATS for intricate problem-solving tasks, permitting your AI to contemplate different potentialities and results.
By integrating these reflection-based design patterns, you will create AI systems that not only perform tasks but also constantly enhance, becoming more effective and sharp with each communication. This dynamic flexibility makes reflection-based agents a groundbreaker in the world of AI development.
Ready to put this into practice? Let’s explore the role of an AI simulation assistant to see these concepts in action.
Unleash the full potential of your AI systems with innovative Large Action Models. Explore how they can revolutionize your venture today!
Implementing AI Simulation Assistant with Agentic Workflows
Have you ever considered having a sharp, intelligent assistant that can smoothly counterfeit intricate synopses and provide you with applicable perceptions, all powered by innovative AI and agentic workflows?
Overview of the Simulation Assistant Application
Ever wanted to have an AI simulation assistant at your fingertips that helps you model intricate synopses smoothly? This application uses advanced AI to provide pragmatic simulations for numerous requirements, from business predictions to educational tools. You ask, and the simulation assistant delivers, making intricate data understandable and applicable. The heart of this assistant is its capability to refine and simulate enormous amounts of information, giving you perceptions that are both deep and easy to comprehend.
Components and Roles of LLM-based Agents with Tools
The backbone of your AI simulation assistant comprises numerous key architectural elements, all operating in harmony. At the core, you have Large Language Model (LLM)- based agents, which are designed to comprehend and create human-like text. These agents don’t operate in solitude; specialized tools equip them to improve their abilities. Envision the LLM as the brain, capable of deep inspection and generation of intricate text, while the tools are like virtuoso advisors, offering accurate and domain-specific knowledge. Together, they form a sturdy system that can handle your most demanding simulation requests.
Life Cycle of an AI Assistant Simulation Request
When you capitulate a simulation request to your AI assistant, an appealing process starts. First, the request is analyzed and comprehended by the LLM-based agent. Then, it determines the significant tools and resources required to generate a precise simulation. This might involve accessing databases, running intricate algorithms, or even consulting other AI agents. Once the introductory information is collected, the simulation is created, with continuous processing and verification to ensure precision. Eventually, the outcomes are acquainted with you in a clear, easy-to-comprehend format, ready for your inspection and decision-making.
Simulation Workflow Integration Challenges and Solutions
Incorporating a simulation assistant into your existing productivity might seem challenging, but with the right approach, it’s quite tractable. One of the main challenges is ensuring smooth communication between the LLM-based agents and the tools they depend on. This requires sturdy APIs and well-defined protocols. In addition, maintaining data integrity and security is paramount, especially when handling sensitive data. To acknowledge these problems, you can enforce encryption and access controls, along with frequent inspections. Another obstacle is ensuring the AI’s yields are dependable and pertinent. This is tackled through constant training and updating of the LLMs and tools, ensuring they stay current with the latest information and techniques.
Future Directions for Simulation Assistant Development
Looking ahead, the future of AI simulation assistants is absolutely promising. You can anticipate these systems to become even more instinctive and receptive, able to handle more intricate and eclectic requests. Advances in machine learning and natural language processing will enable deeper comprehension and more precise simulations. In addition, the incorporation of real-time data sources will make simulations more robust and pertinent to your instant requirements. As these assistants develop, they will become invaluable tools in your decision-making arsenal, providing insights and foresight that are currently inconceivable. Enfold these progressions, and you will be at the vanguard of innovation, using AI to its fullest potential.
Before we wrap up, let's take a step back and look at what we've learned about agentic design patterns and their future outlook.
Conclusion and Future Outlook
Agentic design patterns hold enormous potential to improve the abilities of LLMs. They enable the evolution of smarter, more effective, and user-friendly applications that can handle intricate tasks autonomously.
Constant experimentation and evolution are critical in unleashing the full potential of LLM-based applications. By exploring new techniques and refining existing ones, developers can create more advanced and efficient AI systems.
The future of agentic workflows with LLMs is promising. We expect more advanced reflection agents, enhanced multi-agent collaboration, and improved tool use. These expansions will further improve LLMs' abilities, leading to more efficient applications.
But before you go, transform your testing workflow today with RagaAI’s intuitive platform. Sign up now for a free trial!
Hey there! Have you ever wondered how large language models (LLMs) such as GPT-4 manage to be so adaptable and effective? It’s all thanks to agentic design motifs. These patterns are transforming how we evolve and use applications with LLMs, making them more proficient and flexible. Let’s explore agentic design patterns further and see how they improve the abilities of LLMs in application development.
Don’t miss out on the chance to improve your skills and knowledge with these anticipating Large Language Models projects! Whether you’re a beginner or looking to amplify your skills, our well-chosen list of 20 LLM Project Ideas is designed to encourage and challenge you. Begin your expedition today and revolutionize your potential into reality.
Introduction to Agentic Design Patterns in LLM Applications
Agentic design patterns in LLM applications improve the abilities of language models by impacting resolute, aspirational behaviors. This introduction explores how these patterns can enhance user interactions, increase effectiveness, and nurture innovation in numerous industries. So, let’s know more in detail:
Explanation of Agentic Design Patterns
Agentic design patterns refer to a set of practices and structures that delegate AI systems to act more independently and effectively. These patterns structure AI agents to perform tasks, make decisions, and communicate with other systems in a more human-like and autonomous manner. By integrating these patterns, developers can create applications that are not only savvy but also more dependable and user-friendly.
Evolving Role of LLMs in App Development
Simple text generators were the starting point for LLMs, but they have come a long way since then. Today, numerous applications, from customer service bots to intricate data analysis tools, rely on them. Their capability to comprehend and generate human-like text has opened new horizons in application development, making tasks that once seemed futile now viable. Incorporating agentic design patterns into LLMs is the next step in this development, pushing the boundaries of what these models can accomplish.
Boosting LLM Capabilities with Agentic Workflows
Agentic workflows play a critical role in improving LLM capabilities. These workflows involve designing AI systems that can independently handle tasks, make informed choices, and grasp their experiences. By integrating agentic workflows, developers can ensure that LLMs are not only cognizant but also dynamic in their operations, leading to more effective applications.
The future of agentic workflows with LLMs is promising, with expected evolutions including more advanced reflection agents, enhanced multi-agent collaboration, and improved tool use. These expected advancements in agentic workflows will enable LLMs to strengthen their abilities, resulting in more effective applications.
But before we get too far into the future, let’s take a closer look at the tools that make these workflows possible right now.
Are you ready to use the full potential of Large Language Models (LLMs) for your venture? Understanding LLM Alignment is critical for creating AI that works smoothly and honorably. So, read now!
Tool Use in AI Agentic Workflows
The concept of “Agentic Design” in AI highlights creating systems that can work independently with a degree of initiative and objective. Tools and methods play a crucial role in developing such intelligent workflows, improving the overall capabilities and efficiency of AI agents. Here’s a detailed look at the key elements and contemplations in the tool used within AI agentic workflows:
Understanding Tool Use in Agentic Design
In the synopsis of AI agentic workflows, tool use stands as a critical radical design pattern. Suppose your AI is a sharp assistant, prepared to improve your workflow by smoothly incorporating numerous tools. This capability revolutionizes your AI from a simple communicative agent into a prominent, adaptable helper. By using various tools, your AI can execute intricate tasks, recover precise data, and sleek your productivity, making your communications more effective and impactful.
Examples of Tool Use: Web Searches, Databases, Productivity Tools
Let’s know some practical instances to embellish how tool use works. Picture yourself requiring the latest market trends; your AI conducts web searches to collect and condense this data rapidly. If you need precise customer data, it can access and query databases to retrieve the information you require. In addition, your AI can interface with workflow tools such as calendars, emails, and project management software, scheduling meetings, sending reminders, or updating task statuses. These instances emphasize how incorporating tool use can substantially boost the abilities of your AI, turning it into a versatile asset.
Prompting LLMs for Tool Selection
Prompting LLMs (Large Language Models) for involuntary tool selection is a developing art. You commence this process by giving your AI clear, brief instructions. For example, if you need a report on recent sales figures, you would prompt the AI with, "Create a report on sales figures for the last quarter." The AI then recognizes the most suitable tool–whether it’s a database query or a web search–to achieve this task. This involuntary selection process improves effectiveness, as the AI vigorously selects the best tools based on your requirements.
Evolution of Tool Functionality Beyond Basic Web Searches
AI tool use has developed far beyond mere web searches. At first, web search abilities permitted AIs to collect details from the Internet. However, modern AIs are now equipped with advanced tools that can execute specialized tasks. For instance, they can dissect datasets, generate visualizations, write code, and even counterfeit synopses based on the details they refine. This development has augmented the scope of what AI can accomplish, enabling it to aid more intricate and disparate productivity.
Managing Large-Scale Functions with Heuristics
When managing a comprehensive range of functions in large-scale AI enforcement, heuristics play a crucial role. Heuristics are inherently rule-of-thumb plans that can aid the AI in making decisions rapidly and effectively.
For example, if the AI regularly confronts similar types of tasks, heuristics enable it to determine patterns and apply the most efficient tool or technique involuntarily. This approach not only enhances the pace of task implementation but also ensures steadiness and precision in handling numerous functions. By using heuristics, you can manage the intricacies of large-scale AI operations, ensuring your AI remains a dependable and powerful tool in your productivity.
Now that we've covered the nuts and bolts of managing large-scale functions let's dive into the world of multi-agent collaboration and see how teamwork among AIs can elevate your projects.
Ready to boost your AI abilities? Find how custom guardrails can improve the performance, dependability, and security of your language models. Take the initial step towards smarter AI today. Read our article on Building And Implementing Custom LLM Guardrails and revolutionize your approach to LLMs!
Multi-agent Collaboration
In the swiftly developing field of AI, collaboration is not just a human attempt; it’s a key strategy for upgrading machine intelligence as well. Multi-agent collaboration in LLM-based applications depicts a cutting-edge approach where multiple language model agents operate together to solve intricate issues more effectively than ever before. Let’s take a look more in detail:
Introduction to Multi-agent Systems in LLM
Suppose a team of specialists operating together flawlessly to tackle an intricate issue. That’s what multi-agent systems in LLM applications bring to the table. Instead of depending on a single large language model to regulate everything, you envoy tasks to multiple specialized agents. These agents partner, each contributing their strengths, to offer a more effective solution. This collaboration approach not only improves performance but also opens up new potentiality for creativity in Artificial Intelligence.
Advantages of Delegating Complex Tasks to Multiple LLM Agents
By delegating intricate tasks to multiple LLM agents, you can use the power of expertise. Each agent can concentrate on a precise aspect of the issue, using its unique abilities to deliver exceptional outcomes. This division of labor reduces the cognitive load on individual agents, leading to rapid refining times and more precise results. In addition, this approach provides greater adaptability, as you can easily reconfigure the agents to acknowledge various challenges or incorporate new abilities as they arise.
Applications of Multi-Agent Systems in Software Development
In software development and problem-solving, multi-agent systems can transform the way you approach tasks. For example, in debugging, one agent could determine the bug, another could recommend potential solutions, and yet another could verify the solution. This collaborative effort boosts the evolution process and improves the quality of the final product. In problem-solving synopsis, multiple agents can operate together to explore various strategies, assess results, and assemble the most efficient solution. This approach is specifically valuable in intricate, flexible problems where a single agent might struggle to provide a thorough response.
Frameworks for Integrating Multiple Agents
To efficiently incorporate multiple agents, you need sturdy frameworks and mental models. One admired approach is the use of a central coordinator agent that manages the communication between skilled agents. This coordinator ensures that tasks are adequately delegated and that agents interact effectively. Another model involves decentralized coordination, where agents autonomously communicate based on predefined conventions. Both approaches have their advantages, and the choice relies on the precise needs of your application. Evolving a clear comprehension of these frameworks is critical for successful multi-agent incorporation.
From robust frameworks to practical examples, let's explore some emerging tools that are setting the standard for multi-agent systems.
Examples of Emerging Frameworks: AutoGen, Crew AI, LangGraph
Numerous emerging frameworks pave the way for more sophisticated multi-agent systems. AutoGen, for instance, automates the creation and management of agent workflows, making it easier to deploy and scale multi-agent systems. Crew AI concentrates on collaborative problem-solving, enabling agents to operate together in a highly coordinated manner. LangGraph, on the other hand, offers a graph-based approach to agent communication, permitting for more robust and adaptable coordination. These frameworks demonstrate the potential of multi-agent systems and provide applicable tools for enforcing them in real-globe applications.
Alright, let’s shift gears to another exciting aspect: reflection-based agents and how they can make your AI even smarter.
By comprehending and using these advanced concepts, you can unleash new levels of effectiveness and innovation in your Artificial Intelligence projects.
Unleash the full potential of your AI: Read our Practical Guide For Deploying LLMs In Production and revolutionize your venture with innovative technology. Get specialist perceptions, step-by-step guidelines, and demonstrated strategies to ensure a smooth and successful deployment. Begin transforming your operations today!
Reflection-based Agents
Let’s now know about Reflection-based agents in LLM-based applications:
Concept of Reflection in Improving AI Systems
Imagine if your Artificial Intelligence could think about its conceptions. That’s the core concept behind reflection in AI systems. By reflecting, an AI can assess its decisions, learn from its mistakes, and refine its procedures. This self-assessment makes the AI more flexible and smarter over time. Just like you might reflect on your day to enhance tomorrow, reflection aids AI systems in becoming more effective in handling intricate tasks.
System 1 and System 2 Architecture in LLMs
Think of your brain’s swift, instinctive answers as System 1 and your slower, more considered thinking as System 2. Large Language Models (LLMs) can be designed likewise. System 1 in LLMs handles rapid, involuntary answers, great for direct tasks. System 2 kicks in for more intricate problem-solving, needing deeper reasoning. This dual approach aids LLMs in equating pace and precision, ensuring they execute well under differing events.
Types of Reflection Agents: Basic, Reflexion, and LATS
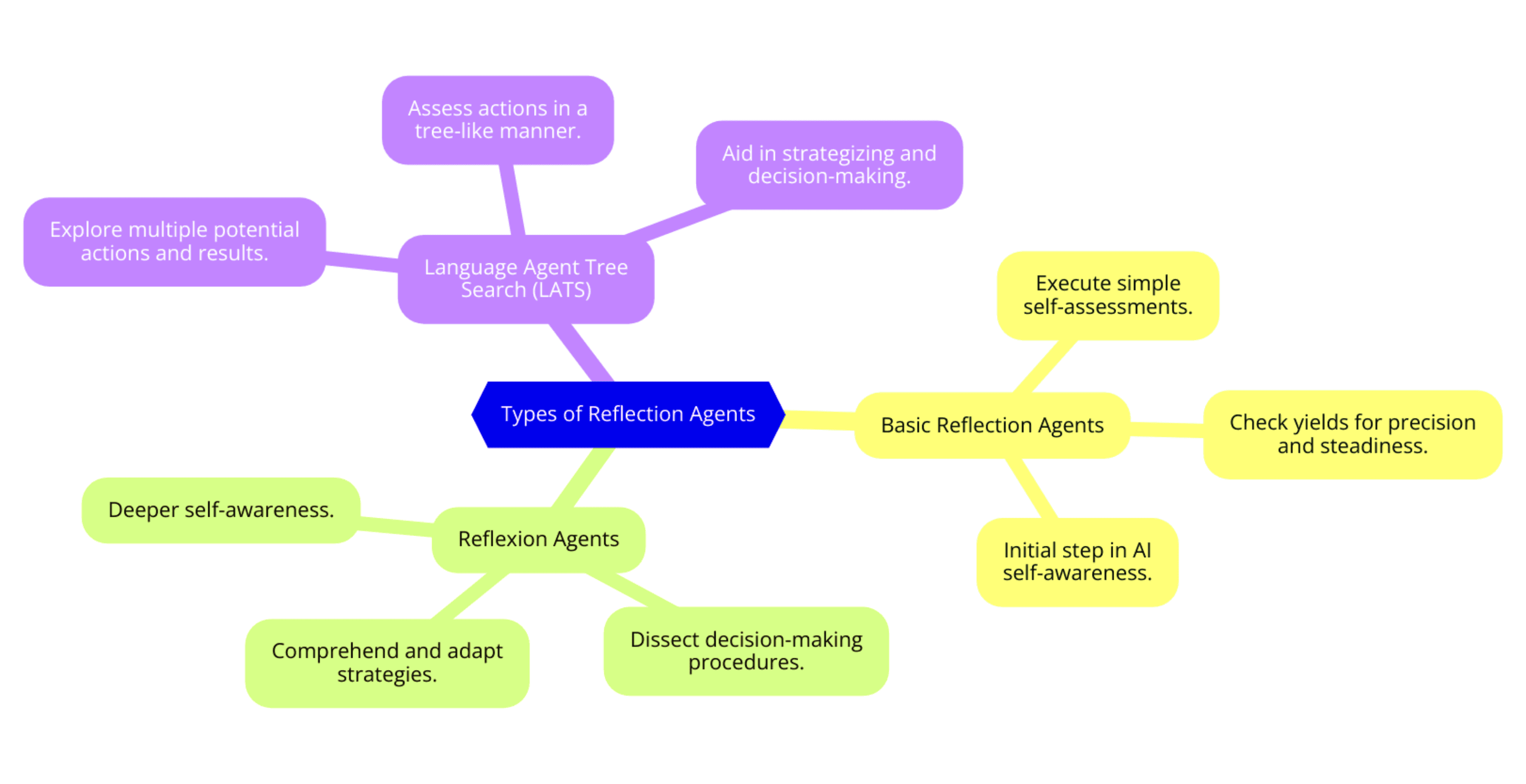
There are various types of reflection agents you can use to improve your AI:
Basic Reflection Agents: These agents execute simple self-assessments, checking their yields for precision and steadiness. They’re the initial step in making your AI more self-aware.
Reflexion Agents: These are more expanded and capable of deeper self-awareness. Reflexion agents can dissect their decision-making procedures, comprehend why certain decisions were made, and adapt their strategies appropriately.
Language Agent Tree Search (LATS): LATS agents take reflection to the next level. They can explore multiple potential actions and results, assessing them in a standardized, tree-like manner. This aids in strategizing and decision-making, specifically in intricate synopses where various paths are feasible.
Awesome, right? Now, let’s talk about how you can actually implement these reflection agents in your app development workflow.
Implementing Reflection Agents in App Development
Incorporating reflection agents into your applications can substantially elevate their performance. Begin by defining the scope of reflection for your AI. What decisions should it assess, and how often? Enforce basic reflection to observe and adapt yields. As your AI system matures, introduce Reflection agents to go deeper into its decision-making procedures. Eventually, use LATS for intricate problem-solving tasks, permitting your AI to contemplate different potentialities and results.
By integrating these reflection-based design patterns, you will create AI systems that not only perform tasks but also constantly enhance, becoming more effective and sharp with each communication. This dynamic flexibility makes reflection-based agents a groundbreaker in the world of AI development.
Ready to put this into practice? Let’s explore the role of an AI simulation assistant to see these concepts in action.
Unleash the full potential of your AI systems with innovative Large Action Models. Explore how they can revolutionize your venture today!
Implementing AI Simulation Assistant with Agentic Workflows
Have you ever considered having a sharp, intelligent assistant that can smoothly counterfeit intricate synopses and provide you with applicable perceptions, all powered by innovative AI and agentic workflows?
Overview of the Simulation Assistant Application
Ever wanted to have an AI simulation assistant at your fingertips that helps you model intricate synopses smoothly? This application uses advanced AI to provide pragmatic simulations for numerous requirements, from business predictions to educational tools. You ask, and the simulation assistant delivers, making intricate data understandable and applicable. The heart of this assistant is its capability to refine and simulate enormous amounts of information, giving you perceptions that are both deep and easy to comprehend.
Components and Roles of LLM-based Agents with Tools
The backbone of your AI simulation assistant comprises numerous key architectural elements, all operating in harmony. At the core, you have Large Language Model (LLM)- based agents, which are designed to comprehend and create human-like text. These agents don’t operate in solitude; specialized tools equip them to improve their abilities. Envision the LLM as the brain, capable of deep inspection and generation of intricate text, while the tools are like virtuoso advisors, offering accurate and domain-specific knowledge. Together, they form a sturdy system that can handle your most demanding simulation requests.
Life Cycle of an AI Assistant Simulation Request
When you capitulate a simulation request to your AI assistant, an appealing process starts. First, the request is analyzed and comprehended by the LLM-based agent. Then, it determines the significant tools and resources required to generate a precise simulation. This might involve accessing databases, running intricate algorithms, or even consulting other AI agents. Once the introductory information is collected, the simulation is created, with continuous processing and verification to ensure precision. Eventually, the outcomes are acquainted with you in a clear, easy-to-comprehend format, ready for your inspection and decision-making.
Simulation Workflow Integration Challenges and Solutions
Incorporating a simulation assistant into your existing productivity might seem challenging, but with the right approach, it’s quite tractable. One of the main challenges is ensuring smooth communication between the LLM-based agents and the tools they depend on. This requires sturdy APIs and well-defined protocols. In addition, maintaining data integrity and security is paramount, especially when handling sensitive data. To acknowledge these problems, you can enforce encryption and access controls, along with frequent inspections. Another obstacle is ensuring the AI’s yields are dependable and pertinent. This is tackled through constant training and updating of the LLMs and tools, ensuring they stay current with the latest information and techniques.
Future Directions for Simulation Assistant Development
Looking ahead, the future of AI simulation assistants is absolutely promising. You can anticipate these systems to become even more instinctive and receptive, able to handle more intricate and eclectic requests. Advances in machine learning and natural language processing will enable deeper comprehension and more precise simulations. In addition, the incorporation of real-time data sources will make simulations more robust and pertinent to your instant requirements. As these assistants develop, they will become invaluable tools in your decision-making arsenal, providing insights and foresight that are currently inconceivable. Enfold these progressions, and you will be at the vanguard of innovation, using AI to its fullest potential.
Before we wrap up, let's take a step back and look at what we've learned about agentic design patterns and their future outlook.
Conclusion and Future Outlook
Agentic design patterns hold enormous potential to improve the abilities of LLMs. They enable the evolution of smarter, more effective, and user-friendly applications that can handle intricate tasks autonomously.
Constant experimentation and evolution are critical in unleashing the full potential of LLM-based applications. By exploring new techniques and refining existing ones, developers can create more advanced and efficient AI systems.
The future of agentic workflows with LLMs is promising. We expect more advanced reflection agents, enhanced multi-agent collaboration, and improved tool use. These expansions will further improve LLMs' abilities, leading to more efficient applications.
But before you go, transform your testing workflow today with RagaAI’s intuitive platform. Sign up now for a free trial!