Understanding Large Action Models In AI
Understanding Large Action Models In AI
Understanding Large Action Models In AI
Rehan Asif
Jun 23, 2024




Are you among those who want to take their AI potential to the next level? Applying large action models (LAM) can help automate your decision-making. Understanding and applying LAMs can change the process of AI applications by providing more interactive, intelligent, and autonomous systems. This type of LAM enhances human capabilities and improves operational efficiency.
A large action model (LAM) is an advanced artificial intelligence (AI) foundation designed to execute tasks and make decisions autonomously. You can expect that the explanation of LAMs as a foundation model differs from large language models (LLMs). Generation and processing of language are the primary factors that make it different from others.
LAMs have performed a significant evolution in AI and can interact with the digital and physical world in ways that enhance human-computer interaction and streamline complex processes. LAMs are new-class AI models that work beyond the traditional Large Language Models or LLMS. You can expect that these systems can perform tasks by adding.
To better understand, you can book a demo for RagaAI LLM Development. It offers safe and secure deployment and carefully prioritizes decisions in your LLM Journey.
You can highlight "the capability of LAMs to perform tasks" autonomously and make decisions as active agents. It works as a passive interpreter of data without considering human participation and manages work decision-making.
The transition from LLMs to LAMs, highlighting their evolution and potential in AI, is a complex application. Still, Large Action models (LAMs) are more advanced than LLMs, and they can predict and understand human behavior. Now, let's have a look at what the basic mechanisms are working behind it,
The Mechanics Behind Large Action Models
LAM compositions are Structured and functionally active to mimic applications and human actions. They work precisely to understand a wide range of prompted tasks and are also structurally enabled. Large Action Models (LAMs) represent AI innovation and are designed to mimic human actions and applications with remarkable precision.
At their core, in evaluating "what a large action model" is, these models are structured to understand and execute a wide range of tasks. It can bridge the gap between human intentions and machine actions. It can enable a blend of neuro-symbolic programming to perform tasks. LAMs also harness the power of neural networks and symbolic reasoning to comprehend and execute complex actions effectively.
You can prompt an effective training process for assessing a large action model that adds 'imitation’ through demonstration. ' With these models, you can learn to execute practical tasks by observing human behavior, paving the way for seamless interaction between humans and machines.
Coming to the fundamental part of a large action model, we are supposed to know the difference between LLM and advanced LAM. In this approach, RagaAI DNA tests AI issues and fixes them.
Difference Between LAM and LLM
Let's discuss the visible difference between LAMs and their associated tools, like Large Language Models (LLMs). RagaAI considerably works in providing seamless AI testing services with RagaAI DNA.
You can be assured that in easy language, LLMs are all about generating text; in contrast, LAMs are more about acting. It's like talking about something and doing it practically. The primary difference behind this structural change is the shift in focus from performing actions to language generation. Raga AI is always working to prompt effective LLM services.
LAMs are designed to get things done for major interactive task completion. They mostly use a hybrid approach with different types of neural networks and symbolic reasoning to figure out the best way forward. When we compare the two, we can see where each shines brightest, showing us their unique strengths and how they can be used.
The basic comparative analysis of capabilities between LAMs and LLMs is significantly oriented in actions performed mostly in language generation and performance enhancement. RagaAI’s LLM is a crucial example of this action as it offers Customer Support, coding copilot, text summarization, and many more features. You can check global standards of AI regulations by RagaAI hereby for better understanding.
Understanding and Interaction Capabilities
One of the attractive and coolest things about LAMs is their capability to understand what we desire and aim to make it happen. It can realize human goals and translate them into actionable tasks like those friends who always know what to do in critical situations. Whether in the digital or physical world or out in the real one, LAMs make things easier for us.
They add more enhancements in terms of human-computer interaction and perform tasks to ease any difficulties. They are no less than the ultimate multitaskers, seamlessly connecting with other external systems and making real-life interaction a lot smoother.
Now, coming to the most important part of LAM, yes. It is its application. Though the application aspect is dynamic, here is a quick look.
Applications and Use Cases of LAMs
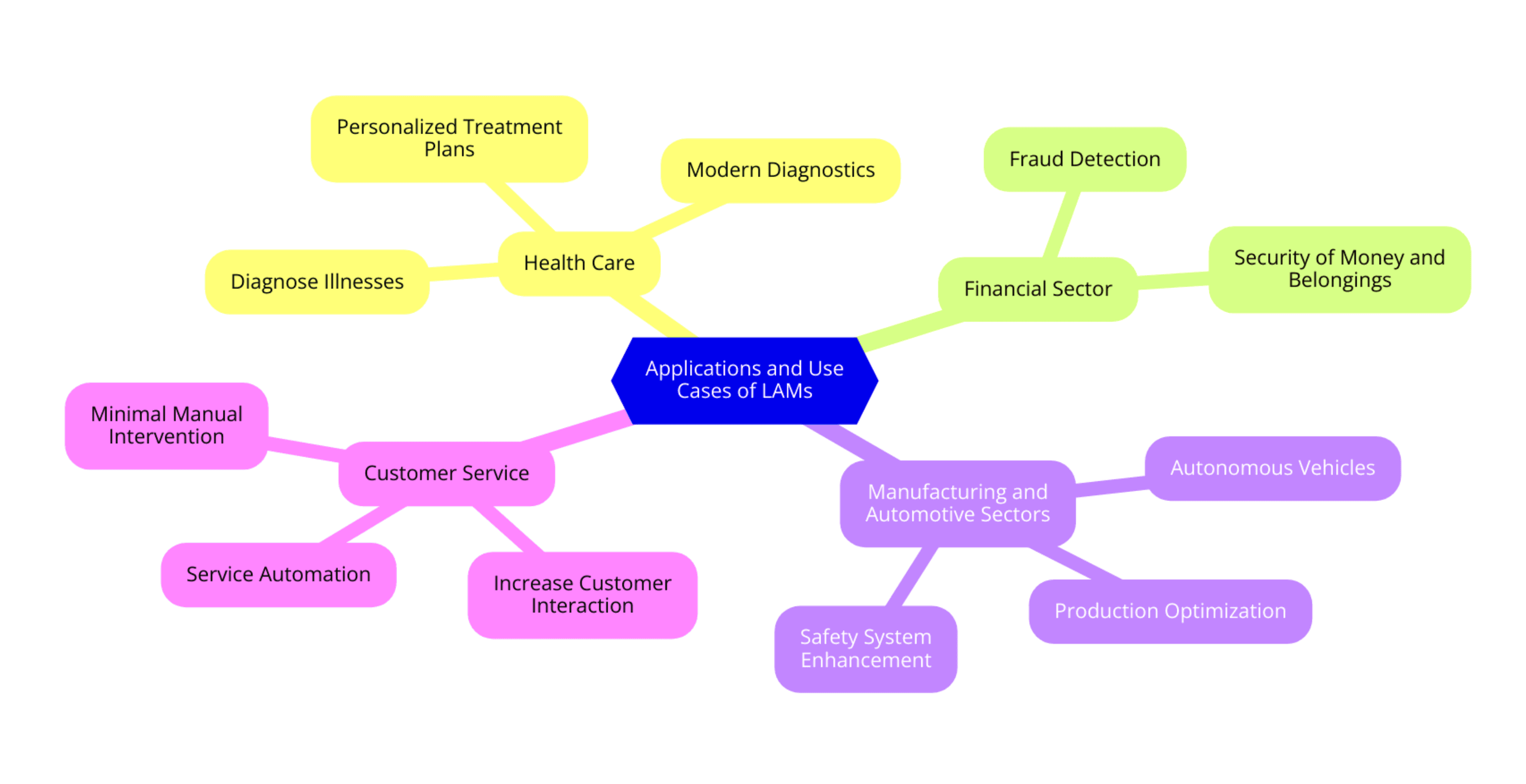
LAMs are not just about sitting around the corner—they can also work to make a difference in all sorts of domains.
Health care: You can take healthcare, for example. LAMs help doctors diagnose illnesses and develop personalized treatment plans using tailored treatment strategies and modern diagnostics.
Financial sector: They are like the key players in finance. They work to find fraud and keep our money and belongings safe. Moreover, they are lending a hand in the manufacturing and automotive sectors, making things run smoother and safer than ever.
Manufacturing and Automotive Sectors: it takes the considerations of the autonomous vehicles and optimizes production with safety system enhancement
Customer Service: And don't even get me started on customer service – LAMs are working on such things what used to be a chronic headache, now, has turned into a breeze. LAMs increase customer interaction, and service automation helps to apply minimal manual intervention.
Practical Implementation Examples
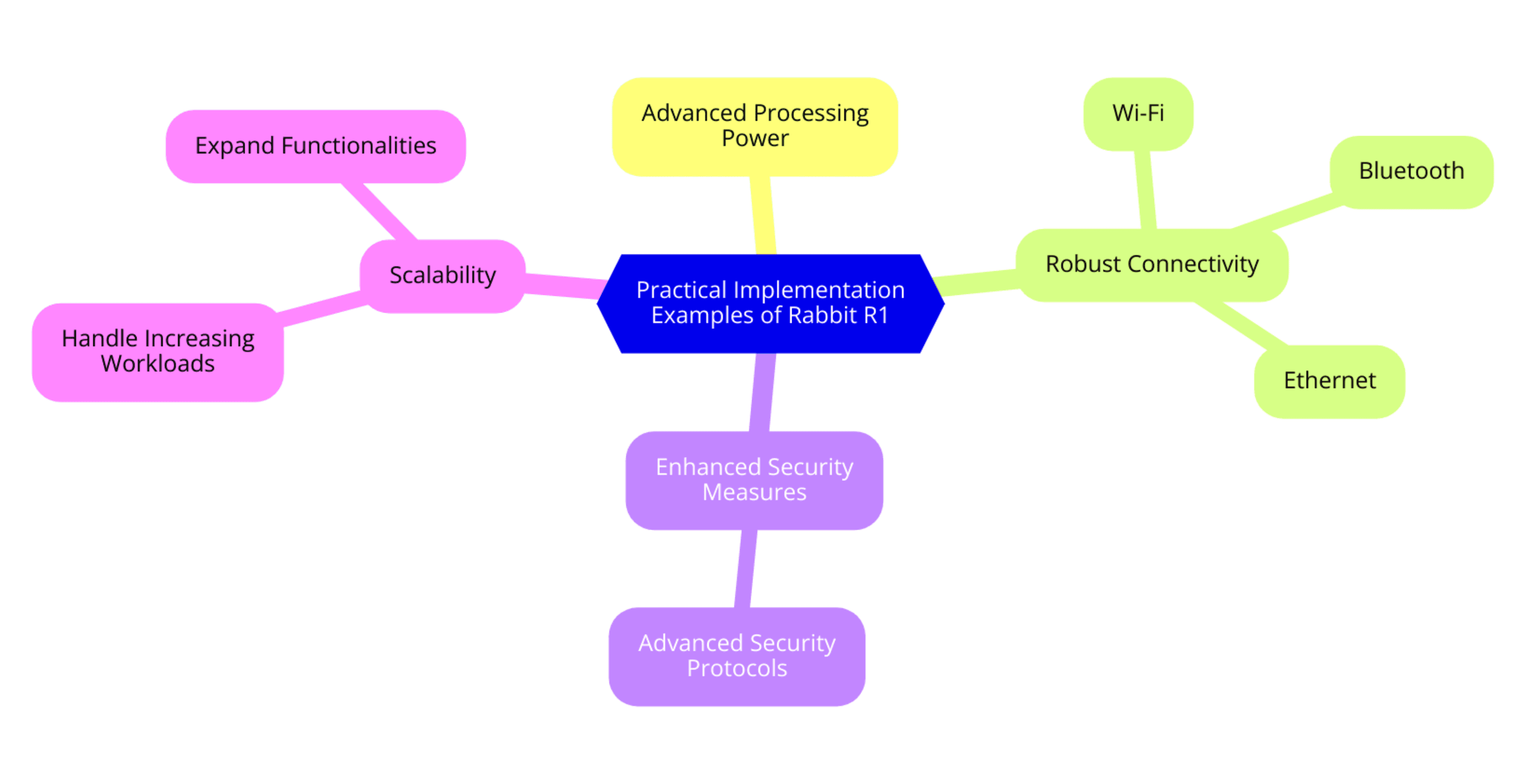
If you want to see what all the buzz is about a large action model (LAM), you just have to check out devices like Rabbit r1. It is like having your assistant but in a smarter way. Regarding virtual assistants, LAMs are the real MVPs, making our lives easier, one task at a time.
The Rabbit R1 device is a considerable development in Artificial Intelligence. It is formatted with state-of-the-art technology and features immense operational capabilities and AI applications.
Features:
Advanced Processing Power: Rabbit R1 has an extraordinary ability with the power of advanced processing
Robust Connectivity: It has different sets of connectivity options, such as Wi-Fi, Bluetooth, and Ethernet to perform seamless operations
Enhanced Security Measures: The Rabbit R1 has advanced security protocols to protect sensitive data
Scalability: Rabbit R1 can handle increasing workloads and expand its detailed functionalities.
Rabbit R1 device operates with advanced hardware and software capabilities to perform different AI tasks. It mostly uses machine learning algorithms to analyze data and recognize patterns before making decisions. Rabbit R1 can increase the performance and reliability of AI-driven systems.
It also has different features to perform operations. These qualities work for it and make it crucial in delivering intelligent solutions for various industries.
This statement means that AI and virtual assistants are integral parts of modern technology for Seamless Human-Computer Interaction. The Rabbit R1 device plays a noteworthy role in enhancing these interactions with its exclusive AI capabilities.
Ability to perform Natural Language Processing: Rabbit R1 can process and understand natural language with high accuracy with proper commands
Real-Time Data Processing: The Rabbit R1 is a powerful tool to process real-time data with AI-driven systems
Improved Personalization: Rabbit R1 holds systems with a highly accurate personalized approach that effectively follows commands as per the user prompts.
Enhanced Security and Privacy: it protects sensitive data and maintains all user security aspects.
Scalability for Diverse Applications: The Rabbit R1 device's scalability ensures that AI assistants can be deployed in different platforms and industries, such as healthcare and customer service.
Thus, the Rabbit R1 device has advancements in enabling AI technology. Its features and operational capabilities make it a critical asset in enhancing the functionality and reliability of complex AI systems.
Challenges and Future Considerations
This system is not free from specific challenges. Large Action Models (LAMs) in AI work to bring ethical, safety, and transparency challenges. Maintaining ethical guidelines is crucial to preventing misuse, as misusing is a significant challenge for this system.
You can expect this system to keep all safety protocols to ensure reliability in high-stakes environments, but a violation can happen in alternative situations. Transparency is a crucial concern in this scenario, and applying robust policies can ensure it.
Human involvement in LAM design and control increases trust in the system. However, from diverse perspectives, AI developers and users can foster human-in-the-loop systems to maintain consistent collaboration.
Explainable AI also works in favor of and helps users understand and verify LAM decisions. RagaAI is working simultaneously on this with extensive services.
LAMs might expand their capabilities to serve larger groups without considerable individual-centric applications. Developing more dynamics and sophisticated algorithms for real-time learning and adaptation and learning to improve performance continuously are also considerable future trends for LAMs.
Conclusion: The Impact and Future of LAMs in AI
Thus, to assess “what a large action model” is, large action models (LAMs) are mostly revolutionizing AI by working on the boundaries of being more autonomous and intelligent. These powerful models enable AI systems to perform complex tasks without massive human participation effectively.
LAMs are creating a transformative shift in how tasks are executed and interacted with. Imagine a world where repetitive and time-consuming processes are automated, freeing you to focus on a more strategic and creative journey. This new world offers unimagined productivity and opens innovative possibilities.
The future is bright, with the promise of enhanced human-machine collaboration. LAMs will work alongside you and can augment all your capabilities to perform day-to-day tasks with greater productivity and innovation.
Lastly, LAMs are advancing AI and reshaping how we live and work. We will have a future where LAMs help you unlock new levels of efficiency. Creativity, along with the dynamic scope of progress and innovation, is also a part of it. You can tryRagaAI today to enhance your LLM skills effectively.
Are you among those who want to take their AI potential to the next level? Applying large action models (LAM) can help automate your decision-making. Understanding and applying LAMs can change the process of AI applications by providing more interactive, intelligent, and autonomous systems. This type of LAM enhances human capabilities and improves operational efficiency.
A large action model (LAM) is an advanced artificial intelligence (AI) foundation designed to execute tasks and make decisions autonomously. You can expect that the explanation of LAMs as a foundation model differs from large language models (LLMs). Generation and processing of language are the primary factors that make it different from others.
LAMs have performed a significant evolution in AI and can interact with the digital and physical world in ways that enhance human-computer interaction and streamline complex processes. LAMs are new-class AI models that work beyond the traditional Large Language Models or LLMS. You can expect that these systems can perform tasks by adding.
To better understand, you can book a demo for RagaAI LLM Development. It offers safe and secure deployment and carefully prioritizes decisions in your LLM Journey.
You can highlight "the capability of LAMs to perform tasks" autonomously and make decisions as active agents. It works as a passive interpreter of data without considering human participation and manages work decision-making.
The transition from LLMs to LAMs, highlighting their evolution and potential in AI, is a complex application. Still, Large Action models (LAMs) are more advanced than LLMs, and they can predict and understand human behavior. Now, let's have a look at what the basic mechanisms are working behind it,
The Mechanics Behind Large Action Models
LAM compositions are Structured and functionally active to mimic applications and human actions. They work precisely to understand a wide range of prompted tasks and are also structurally enabled. Large Action Models (LAMs) represent AI innovation and are designed to mimic human actions and applications with remarkable precision.
At their core, in evaluating "what a large action model" is, these models are structured to understand and execute a wide range of tasks. It can bridge the gap between human intentions and machine actions. It can enable a blend of neuro-symbolic programming to perform tasks. LAMs also harness the power of neural networks and symbolic reasoning to comprehend and execute complex actions effectively.
You can prompt an effective training process for assessing a large action model that adds 'imitation’ through demonstration. ' With these models, you can learn to execute practical tasks by observing human behavior, paving the way for seamless interaction between humans and machines.
Coming to the fundamental part of a large action model, we are supposed to know the difference between LLM and advanced LAM. In this approach, RagaAI DNA tests AI issues and fixes them.
Difference Between LAM and LLM
Let's discuss the visible difference between LAMs and their associated tools, like Large Language Models (LLMs). RagaAI considerably works in providing seamless AI testing services with RagaAI DNA.
You can be assured that in easy language, LLMs are all about generating text; in contrast, LAMs are more about acting. It's like talking about something and doing it practically. The primary difference behind this structural change is the shift in focus from performing actions to language generation. Raga AI is always working to prompt effective LLM services.
LAMs are designed to get things done for major interactive task completion. They mostly use a hybrid approach with different types of neural networks and symbolic reasoning to figure out the best way forward. When we compare the two, we can see where each shines brightest, showing us their unique strengths and how they can be used.
The basic comparative analysis of capabilities between LAMs and LLMs is significantly oriented in actions performed mostly in language generation and performance enhancement. RagaAI’s LLM is a crucial example of this action as it offers Customer Support, coding copilot, text summarization, and many more features. You can check global standards of AI regulations by RagaAI hereby for better understanding.
Understanding and Interaction Capabilities
One of the attractive and coolest things about LAMs is their capability to understand what we desire and aim to make it happen. It can realize human goals and translate them into actionable tasks like those friends who always know what to do in critical situations. Whether in the digital or physical world or out in the real one, LAMs make things easier for us.
They add more enhancements in terms of human-computer interaction and perform tasks to ease any difficulties. They are no less than the ultimate multitaskers, seamlessly connecting with other external systems and making real-life interaction a lot smoother.
Now, coming to the most important part of LAM, yes. It is its application. Though the application aspect is dynamic, here is a quick look.
Applications and Use Cases of LAMs
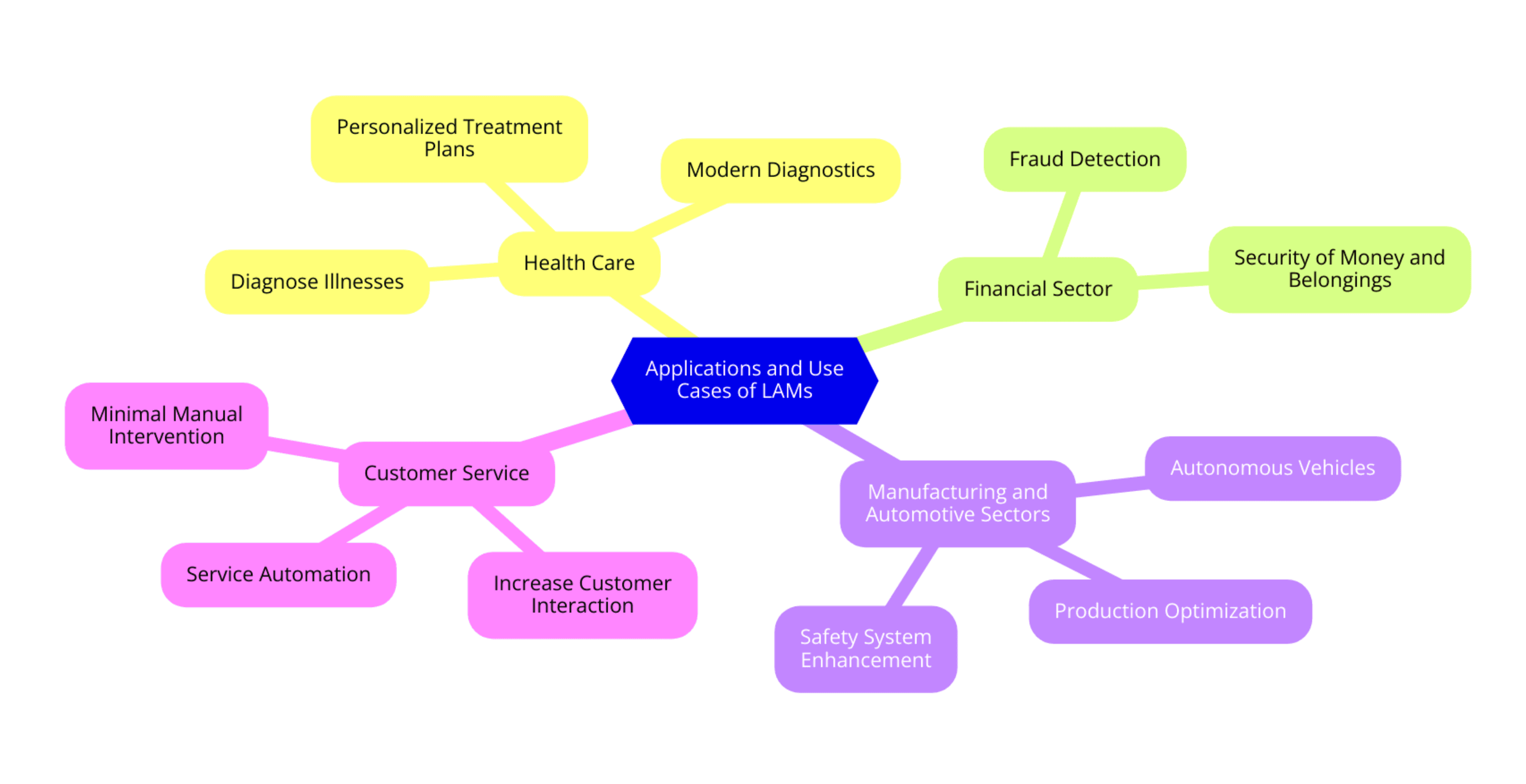
LAMs are not just about sitting around the corner—they can also work to make a difference in all sorts of domains.
Health care: You can take healthcare, for example. LAMs help doctors diagnose illnesses and develop personalized treatment plans using tailored treatment strategies and modern diagnostics.
Financial sector: They are like the key players in finance. They work to find fraud and keep our money and belongings safe. Moreover, they are lending a hand in the manufacturing and automotive sectors, making things run smoother and safer than ever.
Manufacturing and Automotive Sectors: it takes the considerations of the autonomous vehicles and optimizes production with safety system enhancement
Customer Service: And don't even get me started on customer service – LAMs are working on such things what used to be a chronic headache, now, has turned into a breeze. LAMs increase customer interaction, and service automation helps to apply minimal manual intervention.
Practical Implementation Examples
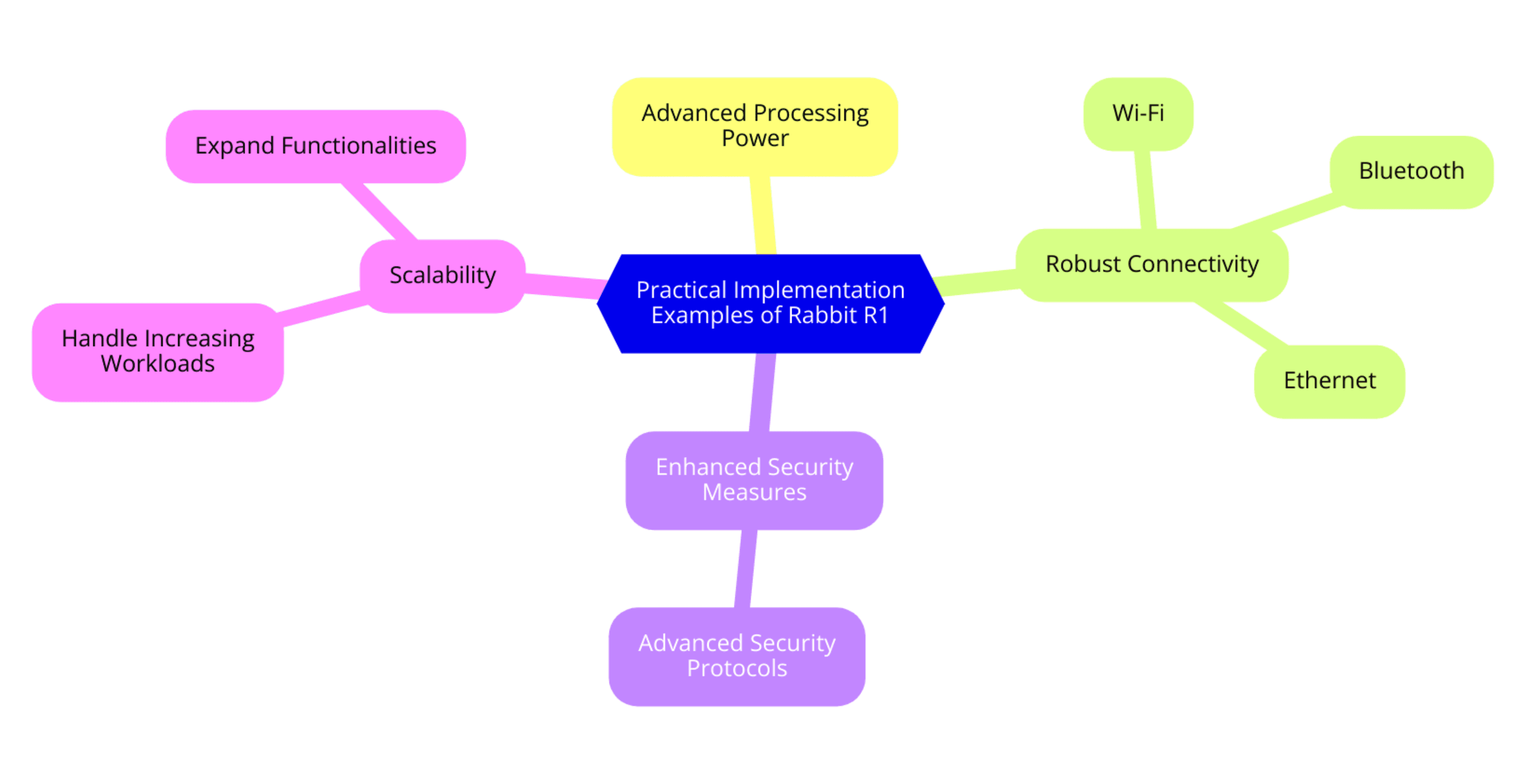
If you want to see what all the buzz is about a large action model (LAM), you just have to check out devices like Rabbit r1. It is like having your assistant but in a smarter way. Regarding virtual assistants, LAMs are the real MVPs, making our lives easier, one task at a time.
The Rabbit R1 device is a considerable development in Artificial Intelligence. It is formatted with state-of-the-art technology and features immense operational capabilities and AI applications.
Features:
Advanced Processing Power: Rabbit R1 has an extraordinary ability with the power of advanced processing
Robust Connectivity: It has different sets of connectivity options, such as Wi-Fi, Bluetooth, and Ethernet to perform seamless operations
Enhanced Security Measures: The Rabbit R1 has advanced security protocols to protect sensitive data
Scalability: Rabbit R1 can handle increasing workloads and expand its detailed functionalities.
Rabbit R1 device operates with advanced hardware and software capabilities to perform different AI tasks. It mostly uses machine learning algorithms to analyze data and recognize patterns before making decisions. Rabbit R1 can increase the performance and reliability of AI-driven systems.
It also has different features to perform operations. These qualities work for it and make it crucial in delivering intelligent solutions for various industries.
This statement means that AI and virtual assistants are integral parts of modern technology for Seamless Human-Computer Interaction. The Rabbit R1 device plays a noteworthy role in enhancing these interactions with its exclusive AI capabilities.
Ability to perform Natural Language Processing: Rabbit R1 can process and understand natural language with high accuracy with proper commands
Real-Time Data Processing: The Rabbit R1 is a powerful tool to process real-time data with AI-driven systems
Improved Personalization: Rabbit R1 holds systems with a highly accurate personalized approach that effectively follows commands as per the user prompts.
Enhanced Security and Privacy: it protects sensitive data and maintains all user security aspects.
Scalability for Diverse Applications: The Rabbit R1 device's scalability ensures that AI assistants can be deployed in different platforms and industries, such as healthcare and customer service.
Thus, the Rabbit R1 device has advancements in enabling AI technology. Its features and operational capabilities make it a critical asset in enhancing the functionality and reliability of complex AI systems.
Challenges and Future Considerations
This system is not free from specific challenges. Large Action Models (LAMs) in AI work to bring ethical, safety, and transparency challenges. Maintaining ethical guidelines is crucial to preventing misuse, as misusing is a significant challenge for this system.
You can expect this system to keep all safety protocols to ensure reliability in high-stakes environments, but a violation can happen in alternative situations. Transparency is a crucial concern in this scenario, and applying robust policies can ensure it.
Human involvement in LAM design and control increases trust in the system. However, from diverse perspectives, AI developers and users can foster human-in-the-loop systems to maintain consistent collaboration.
Explainable AI also works in favor of and helps users understand and verify LAM decisions. RagaAI is working simultaneously on this with extensive services.
LAMs might expand their capabilities to serve larger groups without considerable individual-centric applications. Developing more dynamics and sophisticated algorithms for real-time learning and adaptation and learning to improve performance continuously are also considerable future trends for LAMs.
Conclusion: The Impact and Future of LAMs in AI
Thus, to assess “what a large action model” is, large action models (LAMs) are mostly revolutionizing AI by working on the boundaries of being more autonomous and intelligent. These powerful models enable AI systems to perform complex tasks without massive human participation effectively.
LAMs are creating a transformative shift in how tasks are executed and interacted with. Imagine a world where repetitive and time-consuming processes are automated, freeing you to focus on a more strategic and creative journey. This new world offers unimagined productivity and opens innovative possibilities.
The future is bright, with the promise of enhanced human-machine collaboration. LAMs will work alongside you and can augment all your capabilities to perform day-to-day tasks with greater productivity and innovation.
Lastly, LAMs are advancing AI and reshaping how we live and work. We will have a future where LAMs help you unlock new levels of efficiency. Creativity, along with the dynamic scope of progress and innovation, is also a part of it. You can tryRagaAI today to enhance your LLM skills effectively.
Are you among those who want to take their AI potential to the next level? Applying large action models (LAM) can help automate your decision-making. Understanding and applying LAMs can change the process of AI applications by providing more interactive, intelligent, and autonomous systems. This type of LAM enhances human capabilities and improves operational efficiency.
A large action model (LAM) is an advanced artificial intelligence (AI) foundation designed to execute tasks and make decisions autonomously. You can expect that the explanation of LAMs as a foundation model differs from large language models (LLMs). Generation and processing of language are the primary factors that make it different from others.
LAMs have performed a significant evolution in AI and can interact with the digital and physical world in ways that enhance human-computer interaction and streamline complex processes. LAMs are new-class AI models that work beyond the traditional Large Language Models or LLMS. You can expect that these systems can perform tasks by adding.
To better understand, you can book a demo for RagaAI LLM Development. It offers safe and secure deployment and carefully prioritizes decisions in your LLM Journey.
You can highlight "the capability of LAMs to perform tasks" autonomously and make decisions as active agents. It works as a passive interpreter of data without considering human participation and manages work decision-making.
The transition from LLMs to LAMs, highlighting their evolution and potential in AI, is a complex application. Still, Large Action models (LAMs) are more advanced than LLMs, and they can predict and understand human behavior. Now, let's have a look at what the basic mechanisms are working behind it,
The Mechanics Behind Large Action Models
LAM compositions are Structured and functionally active to mimic applications and human actions. They work precisely to understand a wide range of prompted tasks and are also structurally enabled. Large Action Models (LAMs) represent AI innovation and are designed to mimic human actions and applications with remarkable precision.
At their core, in evaluating "what a large action model" is, these models are structured to understand and execute a wide range of tasks. It can bridge the gap between human intentions and machine actions. It can enable a blend of neuro-symbolic programming to perform tasks. LAMs also harness the power of neural networks and symbolic reasoning to comprehend and execute complex actions effectively.
You can prompt an effective training process for assessing a large action model that adds 'imitation’ through demonstration. ' With these models, you can learn to execute practical tasks by observing human behavior, paving the way for seamless interaction between humans and machines.
Coming to the fundamental part of a large action model, we are supposed to know the difference between LLM and advanced LAM. In this approach, RagaAI DNA tests AI issues and fixes them.
Difference Between LAM and LLM
Let's discuss the visible difference between LAMs and their associated tools, like Large Language Models (LLMs). RagaAI considerably works in providing seamless AI testing services with RagaAI DNA.
You can be assured that in easy language, LLMs are all about generating text; in contrast, LAMs are more about acting. It's like talking about something and doing it practically. The primary difference behind this structural change is the shift in focus from performing actions to language generation. Raga AI is always working to prompt effective LLM services.
LAMs are designed to get things done for major interactive task completion. They mostly use a hybrid approach with different types of neural networks and symbolic reasoning to figure out the best way forward. When we compare the two, we can see where each shines brightest, showing us their unique strengths and how they can be used.
The basic comparative analysis of capabilities between LAMs and LLMs is significantly oriented in actions performed mostly in language generation and performance enhancement. RagaAI’s LLM is a crucial example of this action as it offers Customer Support, coding copilot, text summarization, and many more features. You can check global standards of AI regulations by RagaAI hereby for better understanding.
Understanding and Interaction Capabilities
One of the attractive and coolest things about LAMs is their capability to understand what we desire and aim to make it happen. It can realize human goals and translate them into actionable tasks like those friends who always know what to do in critical situations. Whether in the digital or physical world or out in the real one, LAMs make things easier for us.
They add more enhancements in terms of human-computer interaction and perform tasks to ease any difficulties. They are no less than the ultimate multitaskers, seamlessly connecting with other external systems and making real-life interaction a lot smoother.
Now, coming to the most important part of LAM, yes. It is its application. Though the application aspect is dynamic, here is a quick look.
Applications and Use Cases of LAMs
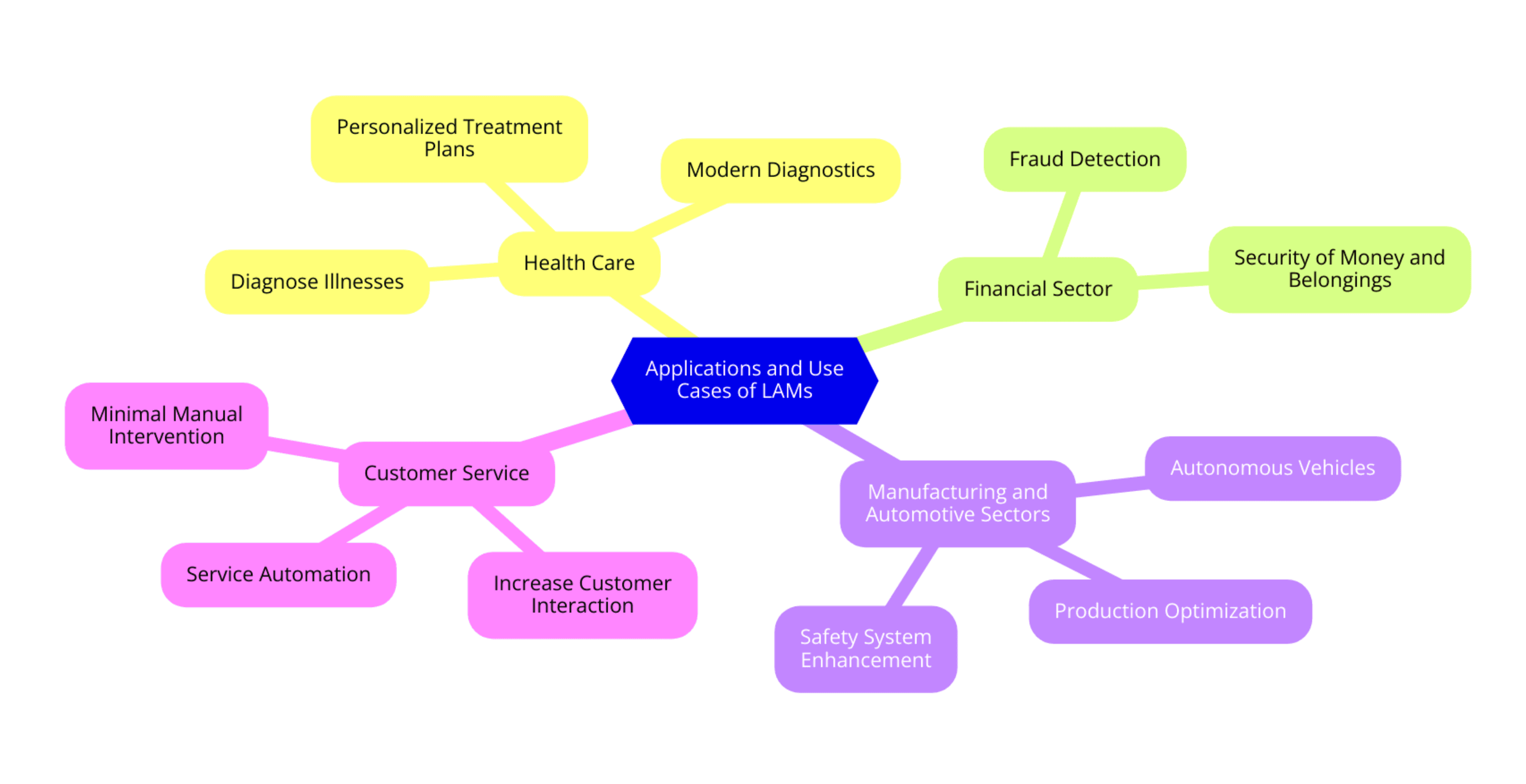
LAMs are not just about sitting around the corner—they can also work to make a difference in all sorts of domains.
Health care: You can take healthcare, for example. LAMs help doctors diagnose illnesses and develop personalized treatment plans using tailored treatment strategies and modern diagnostics.
Financial sector: They are like the key players in finance. They work to find fraud and keep our money and belongings safe. Moreover, they are lending a hand in the manufacturing and automotive sectors, making things run smoother and safer than ever.
Manufacturing and Automotive Sectors: it takes the considerations of the autonomous vehicles and optimizes production with safety system enhancement
Customer Service: And don't even get me started on customer service – LAMs are working on such things what used to be a chronic headache, now, has turned into a breeze. LAMs increase customer interaction, and service automation helps to apply minimal manual intervention.
Practical Implementation Examples
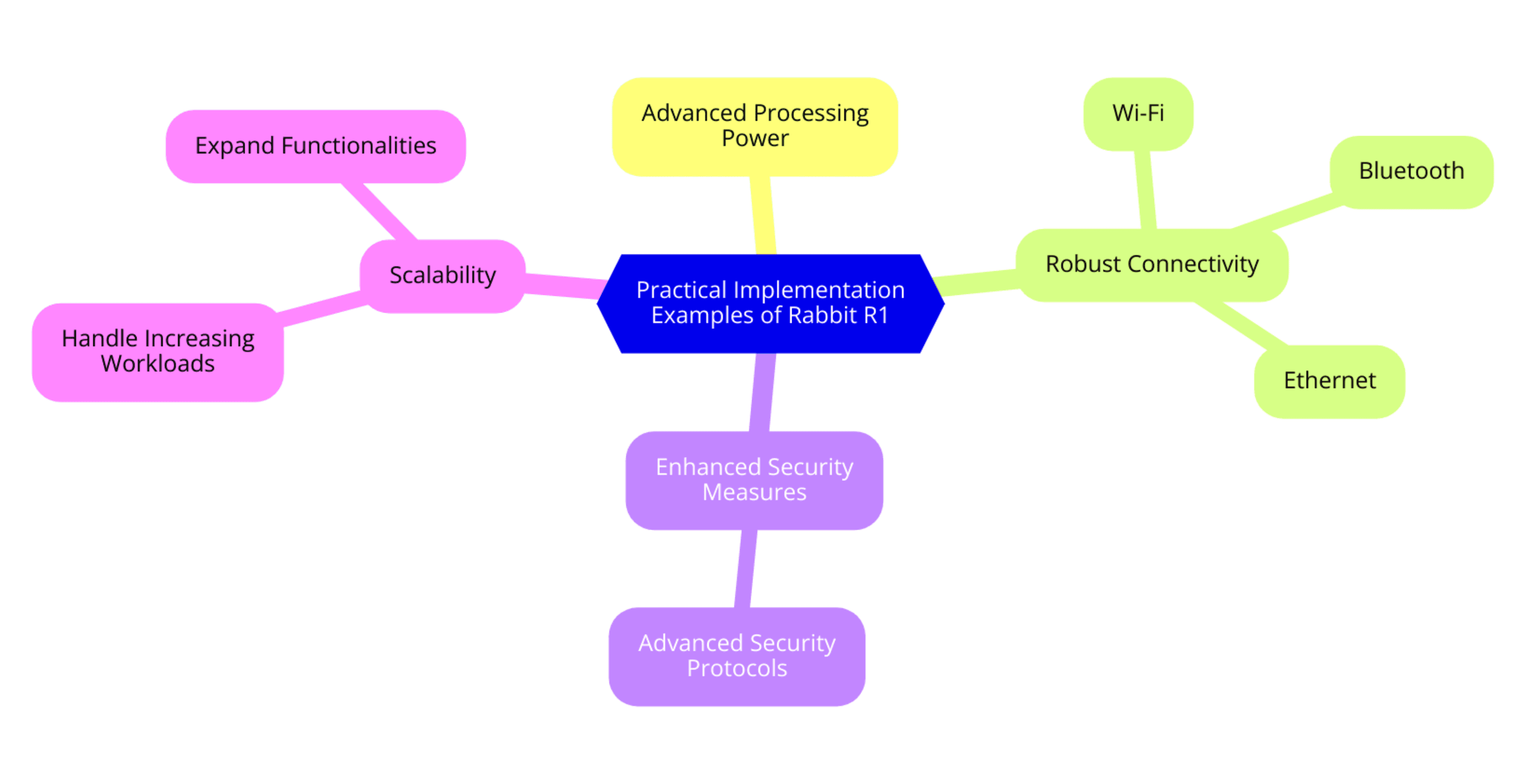
If you want to see what all the buzz is about a large action model (LAM), you just have to check out devices like Rabbit r1. It is like having your assistant but in a smarter way. Regarding virtual assistants, LAMs are the real MVPs, making our lives easier, one task at a time.
The Rabbit R1 device is a considerable development in Artificial Intelligence. It is formatted with state-of-the-art technology and features immense operational capabilities and AI applications.
Features:
Advanced Processing Power: Rabbit R1 has an extraordinary ability with the power of advanced processing
Robust Connectivity: It has different sets of connectivity options, such as Wi-Fi, Bluetooth, and Ethernet to perform seamless operations
Enhanced Security Measures: The Rabbit R1 has advanced security protocols to protect sensitive data
Scalability: Rabbit R1 can handle increasing workloads and expand its detailed functionalities.
Rabbit R1 device operates with advanced hardware and software capabilities to perform different AI tasks. It mostly uses machine learning algorithms to analyze data and recognize patterns before making decisions. Rabbit R1 can increase the performance and reliability of AI-driven systems.
It also has different features to perform operations. These qualities work for it and make it crucial in delivering intelligent solutions for various industries.
This statement means that AI and virtual assistants are integral parts of modern technology for Seamless Human-Computer Interaction. The Rabbit R1 device plays a noteworthy role in enhancing these interactions with its exclusive AI capabilities.
Ability to perform Natural Language Processing: Rabbit R1 can process and understand natural language with high accuracy with proper commands
Real-Time Data Processing: The Rabbit R1 is a powerful tool to process real-time data with AI-driven systems
Improved Personalization: Rabbit R1 holds systems with a highly accurate personalized approach that effectively follows commands as per the user prompts.
Enhanced Security and Privacy: it protects sensitive data and maintains all user security aspects.
Scalability for Diverse Applications: The Rabbit R1 device's scalability ensures that AI assistants can be deployed in different platforms and industries, such as healthcare and customer service.
Thus, the Rabbit R1 device has advancements in enabling AI technology. Its features and operational capabilities make it a critical asset in enhancing the functionality and reliability of complex AI systems.
Challenges and Future Considerations
This system is not free from specific challenges. Large Action Models (LAMs) in AI work to bring ethical, safety, and transparency challenges. Maintaining ethical guidelines is crucial to preventing misuse, as misusing is a significant challenge for this system.
You can expect this system to keep all safety protocols to ensure reliability in high-stakes environments, but a violation can happen in alternative situations. Transparency is a crucial concern in this scenario, and applying robust policies can ensure it.
Human involvement in LAM design and control increases trust in the system. However, from diverse perspectives, AI developers and users can foster human-in-the-loop systems to maintain consistent collaboration.
Explainable AI also works in favor of and helps users understand and verify LAM decisions. RagaAI is working simultaneously on this with extensive services.
LAMs might expand their capabilities to serve larger groups without considerable individual-centric applications. Developing more dynamics and sophisticated algorithms for real-time learning and adaptation and learning to improve performance continuously are also considerable future trends for LAMs.
Conclusion: The Impact and Future of LAMs in AI
Thus, to assess “what a large action model” is, large action models (LAMs) are mostly revolutionizing AI by working on the boundaries of being more autonomous and intelligent. These powerful models enable AI systems to perform complex tasks without massive human participation effectively.
LAMs are creating a transformative shift in how tasks are executed and interacted with. Imagine a world where repetitive and time-consuming processes are automated, freeing you to focus on a more strategic and creative journey. This new world offers unimagined productivity and opens innovative possibilities.
The future is bright, with the promise of enhanced human-machine collaboration. LAMs will work alongside you and can augment all your capabilities to perform day-to-day tasks with greater productivity and innovation.
Lastly, LAMs are advancing AI and reshaping how we live and work. We will have a future where LAMs help you unlock new levels of efficiency. Creativity, along with the dynamic scope of progress and innovation, is also a part of it. You can tryRagaAI today to enhance your LLM skills effectively.
Are you among those who want to take their AI potential to the next level? Applying large action models (LAM) can help automate your decision-making. Understanding and applying LAMs can change the process of AI applications by providing more interactive, intelligent, and autonomous systems. This type of LAM enhances human capabilities and improves operational efficiency.
A large action model (LAM) is an advanced artificial intelligence (AI) foundation designed to execute tasks and make decisions autonomously. You can expect that the explanation of LAMs as a foundation model differs from large language models (LLMs). Generation and processing of language are the primary factors that make it different from others.
LAMs have performed a significant evolution in AI and can interact with the digital and physical world in ways that enhance human-computer interaction and streamline complex processes. LAMs are new-class AI models that work beyond the traditional Large Language Models or LLMS. You can expect that these systems can perform tasks by adding.
To better understand, you can book a demo for RagaAI LLM Development. It offers safe and secure deployment and carefully prioritizes decisions in your LLM Journey.
You can highlight "the capability of LAMs to perform tasks" autonomously and make decisions as active agents. It works as a passive interpreter of data without considering human participation and manages work decision-making.
The transition from LLMs to LAMs, highlighting their evolution and potential in AI, is a complex application. Still, Large Action models (LAMs) are more advanced than LLMs, and they can predict and understand human behavior. Now, let's have a look at what the basic mechanisms are working behind it,
The Mechanics Behind Large Action Models
LAM compositions are Structured and functionally active to mimic applications and human actions. They work precisely to understand a wide range of prompted tasks and are also structurally enabled. Large Action Models (LAMs) represent AI innovation and are designed to mimic human actions and applications with remarkable precision.
At their core, in evaluating "what a large action model" is, these models are structured to understand and execute a wide range of tasks. It can bridge the gap between human intentions and machine actions. It can enable a blend of neuro-symbolic programming to perform tasks. LAMs also harness the power of neural networks and symbolic reasoning to comprehend and execute complex actions effectively.
You can prompt an effective training process for assessing a large action model that adds 'imitation’ through demonstration. ' With these models, you can learn to execute practical tasks by observing human behavior, paving the way for seamless interaction between humans and machines.
Coming to the fundamental part of a large action model, we are supposed to know the difference between LLM and advanced LAM. In this approach, RagaAI DNA tests AI issues and fixes them.
Difference Between LAM and LLM
Let's discuss the visible difference between LAMs and their associated tools, like Large Language Models (LLMs). RagaAI considerably works in providing seamless AI testing services with RagaAI DNA.
You can be assured that in easy language, LLMs are all about generating text; in contrast, LAMs are more about acting. It's like talking about something and doing it practically. The primary difference behind this structural change is the shift in focus from performing actions to language generation. Raga AI is always working to prompt effective LLM services.
LAMs are designed to get things done for major interactive task completion. They mostly use a hybrid approach with different types of neural networks and symbolic reasoning to figure out the best way forward. When we compare the two, we can see where each shines brightest, showing us their unique strengths and how they can be used.
The basic comparative analysis of capabilities between LAMs and LLMs is significantly oriented in actions performed mostly in language generation and performance enhancement. RagaAI’s LLM is a crucial example of this action as it offers Customer Support, coding copilot, text summarization, and many more features. You can check global standards of AI regulations by RagaAI hereby for better understanding.
Understanding and Interaction Capabilities
One of the attractive and coolest things about LAMs is their capability to understand what we desire and aim to make it happen. It can realize human goals and translate them into actionable tasks like those friends who always know what to do in critical situations. Whether in the digital or physical world or out in the real one, LAMs make things easier for us.
They add more enhancements in terms of human-computer interaction and perform tasks to ease any difficulties. They are no less than the ultimate multitaskers, seamlessly connecting with other external systems and making real-life interaction a lot smoother.
Now, coming to the most important part of LAM, yes. It is its application. Though the application aspect is dynamic, here is a quick look.
Applications and Use Cases of LAMs
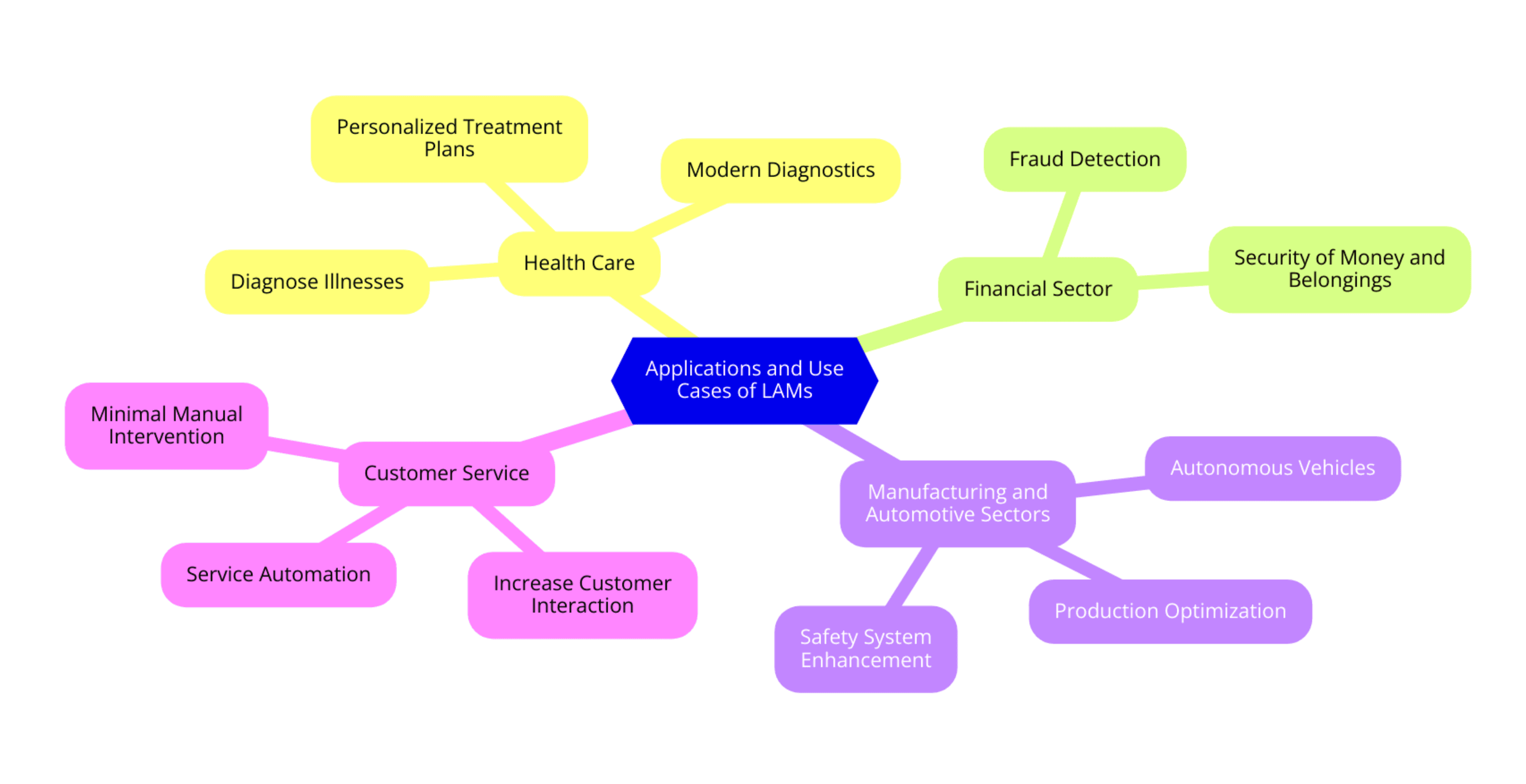
LAMs are not just about sitting around the corner—they can also work to make a difference in all sorts of domains.
Health care: You can take healthcare, for example. LAMs help doctors diagnose illnesses and develop personalized treatment plans using tailored treatment strategies and modern diagnostics.
Financial sector: They are like the key players in finance. They work to find fraud and keep our money and belongings safe. Moreover, they are lending a hand in the manufacturing and automotive sectors, making things run smoother and safer than ever.
Manufacturing and Automotive Sectors: it takes the considerations of the autonomous vehicles and optimizes production with safety system enhancement
Customer Service: And don't even get me started on customer service – LAMs are working on such things what used to be a chronic headache, now, has turned into a breeze. LAMs increase customer interaction, and service automation helps to apply minimal manual intervention.
Practical Implementation Examples
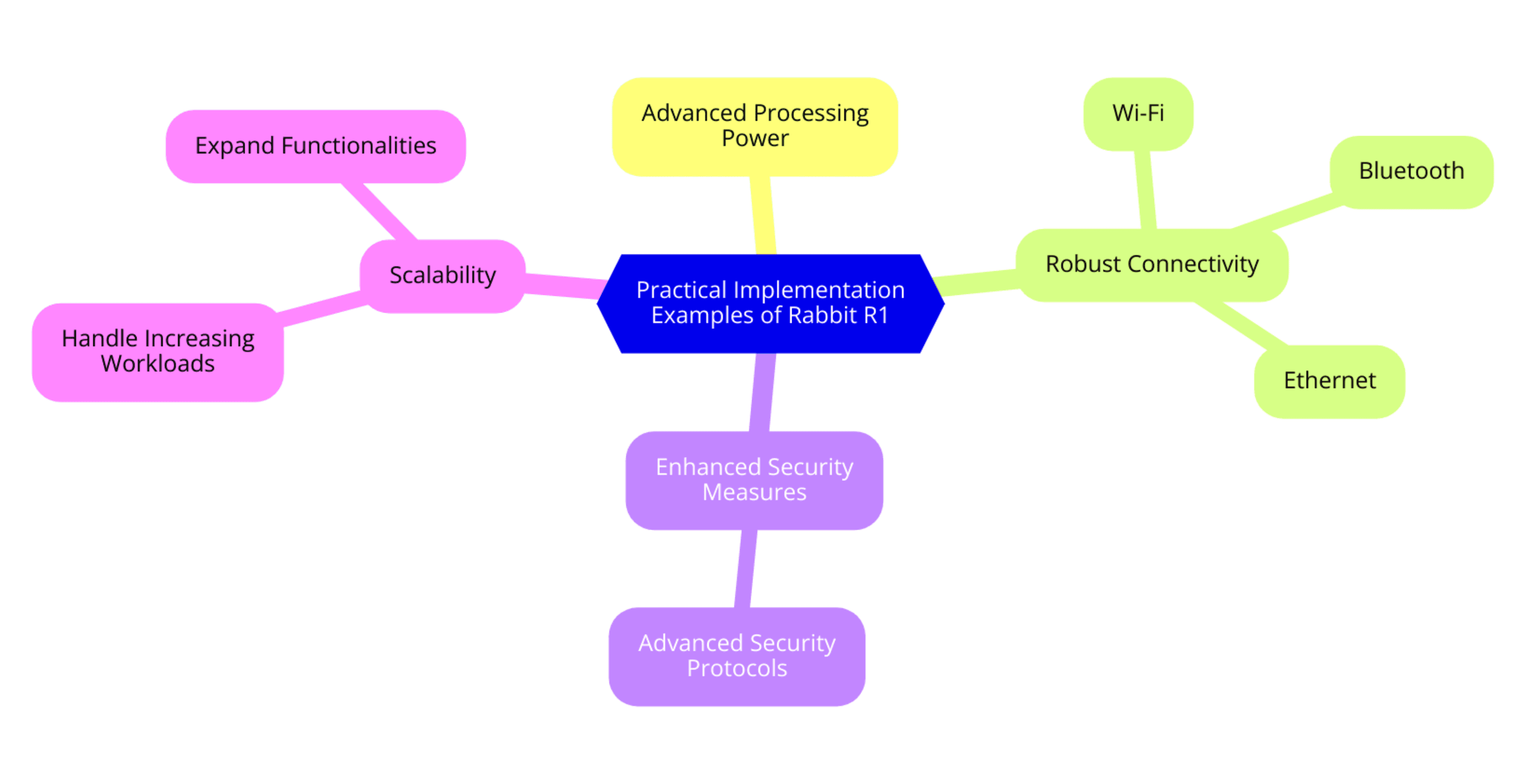
If you want to see what all the buzz is about a large action model (LAM), you just have to check out devices like Rabbit r1. It is like having your assistant but in a smarter way. Regarding virtual assistants, LAMs are the real MVPs, making our lives easier, one task at a time.
The Rabbit R1 device is a considerable development in Artificial Intelligence. It is formatted with state-of-the-art technology and features immense operational capabilities and AI applications.
Features:
Advanced Processing Power: Rabbit R1 has an extraordinary ability with the power of advanced processing
Robust Connectivity: It has different sets of connectivity options, such as Wi-Fi, Bluetooth, and Ethernet to perform seamless operations
Enhanced Security Measures: The Rabbit R1 has advanced security protocols to protect sensitive data
Scalability: Rabbit R1 can handle increasing workloads and expand its detailed functionalities.
Rabbit R1 device operates with advanced hardware and software capabilities to perform different AI tasks. It mostly uses machine learning algorithms to analyze data and recognize patterns before making decisions. Rabbit R1 can increase the performance and reliability of AI-driven systems.
It also has different features to perform operations. These qualities work for it and make it crucial in delivering intelligent solutions for various industries.
This statement means that AI and virtual assistants are integral parts of modern technology for Seamless Human-Computer Interaction. The Rabbit R1 device plays a noteworthy role in enhancing these interactions with its exclusive AI capabilities.
Ability to perform Natural Language Processing: Rabbit R1 can process and understand natural language with high accuracy with proper commands
Real-Time Data Processing: The Rabbit R1 is a powerful tool to process real-time data with AI-driven systems
Improved Personalization: Rabbit R1 holds systems with a highly accurate personalized approach that effectively follows commands as per the user prompts.
Enhanced Security and Privacy: it protects sensitive data and maintains all user security aspects.
Scalability for Diverse Applications: The Rabbit R1 device's scalability ensures that AI assistants can be deployed in different platforms and industries, such as healthcare and customer service.
Thus, the Rabbit R1 device has advancements in enabling AI technology. Its features and operational capabilities make it a critical asset in enhancing the functionality and reliability of complex AI systems.
Challenges and Future Considerations
This system is not free from specific challenges. Large Action Models (LAMs) in AI work to bring ethical, safety, and transparency challenges. Maintaining ethical guidelines is crucial to preventing misuse, as misusing is a significant challenge for this system.
You can expect this system to keep all safety protocols to ensure reliability in high-stakes environments, but a violation can happen in alternative situations. Transparency is a crucial concern in this scenario, and applying robust policies can ensure it.
Human involvement in LAM design and control increases trust in the system. However, from diverse perspectives, AI developers and users can foster human-in-the-loop systems to maintain consistent collaboration.
Explainable AI also works in favor of and helps users understand and verify LAM decisions. RagaAI is working simultaneously on this with extensive services.
LAMs might expand their capabilities to serve larger groups without considerable individual-centric applications. Developing more dynamics and sophisticated algorithms for real-time learning and adaptation and learning to improve performance continuously are also considerable future trends for LAMs.
Conclusion: The Impact and Future of LAMs in AI
Thus, to assess “what a large action model” is, large action models (LAMs) are mostly revolutionizing AI by working on the boundaries of being more autonomous and intelligent. These powerful models enable AI systems to perform complex tasks without massive human participation effectively.
LAMs are creating a transformative shift in how tasks are executed and interacted with. Imagine a world where repetitive and time-consuming processes are automated, freeing you to focus on a more strategic and creative journey. This new world offers unimagined productivity and opens innovative possibilities.
The future is bright, with the promise of enhanced human-machine collaboration. LAMs will work alongside you and can augment all your capabilities to perform day-to-day tasks with greater productivity and innovation.
Lastly, LAMs are advancing AI and reshaping how we live and work. We will have a future where LAMs help you unlock new levels of efficiency. Creativity, along with the dynamic scope of progress and innovation, is also a part of it. You can tryRagaAI today to enhance your LLM skills effectively.
Are you among those who want to take their AI potential to the next level? Applying large action models (LAM) can help automate your decision-making. Understanding and applying LAMs can change the process of AI applications by providing more interactive, intelligent, and autonomous systems. This type of LAM enhances human capabilities and improves operational efficiency.
A large action model (LAM) is an advanced artificial intelligence (AI) foundation designed to execute tasks and make decisions autonomously. You can expect that the explanation of LAMs as a foundation model differs from large language models (LLMs). Generation and processing of language are the primary factors that make it different from others.
LAMs have performed a significant evolution in AI and can interact with the digital and physical world in ways that enhance human-computer interaction and streamline complex processes. LAMs are new-class AI models that work beyond the traditional Large Language Models or LLMS. You can expect that these systems can perform tasks by adding.
To better understand, you can book a demo for RagaAI LLM Development. It offers safe and secure deployment and carefully prioritizes decisions in your LLM Journey.
You can highlight "the capability of LAMs to perform tasks" autonomously and make decisions as active agents. It works as a passive interpreter of data without considering human participation and manages work decision-making.
The transition from LLMs to LAMs, highlighting their evolution and potential in AI, is a complex application. Still, Large Action models (LAMs) are more advanced than LLMs, and they can predict and understand human behavior. Now, let's have a look at what the basic mechanisms are working behind it,
The Mechanics Behind Large Action Models
LAM compositions are Structured and functionally active to mimic applications and human actions. They work precisely to understand a wide range of prompted tasks and are also structurally enabled. Large Action Models (LAMs) represent AI innovation and are designed to mimic human actions and applications with remarkable precision.
At their core, in evaluating "what a large action model" is, these models are structured to understand and execute a wide range of tasks. It can bridge the gap between human intentions and machine actions. It can enable a blend of neuro-symbolic programming to perform tasks. LAMs also harness the power of neural networks and symbolic reasoning to comprehend and execute complex actions effectively.
You can prompt an effective training process for assessing a large action model that adds 'imitation’ through demonstration. ' With these models, you can learn to execute practical tasks by observing human behavior, paving the way for seamless interaction between humans and machines.
Coming to the fundamental part of a large action model, we are supposed to know the difference between LLM and advanced LAM. In this approach, RagaAI DNA tests AI issues and fixes them.
Difference Between LAM and LLM
Let's discuss the visible difference between LAMs and their associated tools, like Large Language Models (LLMs). RagaAI considerably works in providing seamless AI testing services with RagaAI DNA.
You can be assured that in easy language, LLMs are all about generating text; in contrast, LAMs are more about acting. It's like talking about something and doing it practically. The primary difference behind this structural change is the shift in focus from performing actions to language generation. Raga AI is always working to prompt effective LLM services.
LAMs are designed to get things done for major interactive task completion. They mostly use a hybrid approach with different types of neural networks and symbolic reasoning to figure out the best way forward. When we compare the two, we can see where each shines brightest, showing us their unique strengths and how they can be used.
The basic comparative analysis of capabilities between LAMs and LLMs is significantly oriented in actions performed mostly in language generation and performance enhancement. RagaAI’s LLM is a crucial example of this action as it offers Customer Support, coding copilot, text summarization, and many more features. You can check global standards of AI regulations by RagaAI hereby for better understanding.
Understanding and Interaction Capabilities
One of the attractive and coolest things about LAMs is their capability to understand what we desire and aim to make it happen. It can realize human goals and translate them into actionable tasks like those friends who always know what to do in critical situations. Whether in the digital or physical world or out in the real one, LAMs make things easier for us.
They add more enhancements in terms of human-computer interaction and perform tasks to ease any difficulties. They are no less than the ultimate multitaskers, seamlessly connecting with other external systems and making real-life interaction a lot smoother.
Now, coming to the most important part of LAM, yes. It is its application. Though the application aspect is dynamic, here is a quick look.
Applications and Use Cases of LAMs
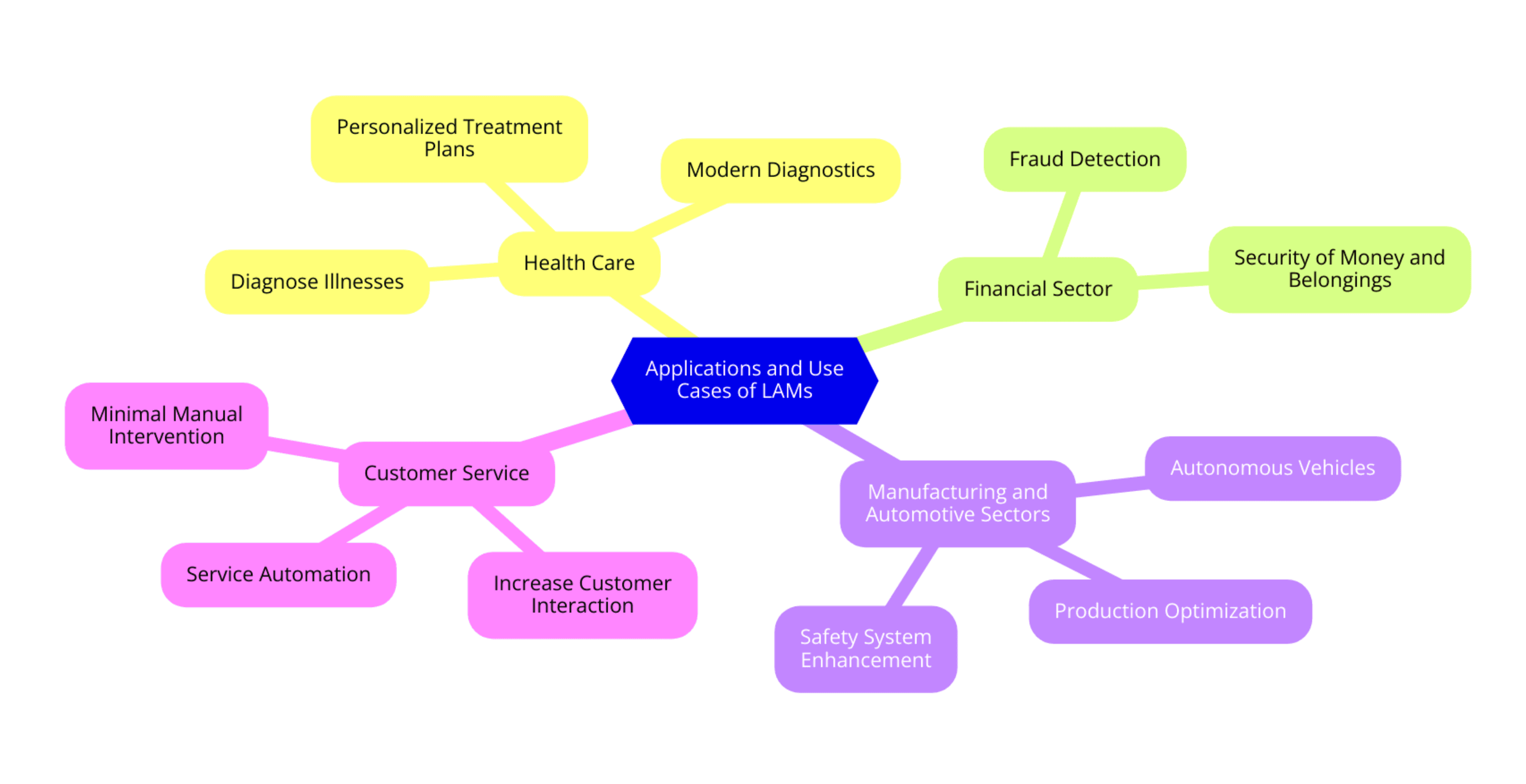
LAMs are not just about sitting around the corner—they can also work to make a difference in all sorts of domains.
Health care: You can take healthcare, for example. LAMs help doctors diagnose illnesses and develop personalized treatment plans using tailored treatment strategies and modern diagnostics.
Financial sector: They are like the key players in finance. They work to find fraud and keep our money and belongings safe. Moreover, they are lending a hand in the manufacturing and automotive sectors, making things run smoother and safer than ever.
Manufacturing and Automotive Sectors: it takes the considerations of the autonomous vehicles and optimizes production with safety system enhancement
Customer Service: And don't even get me started on customer service – LAMs are working on such things what used to be a chronic headache, now, has turned into a breeze. LAMs increase customer interaction, and service automation helps to apply minimal manual intervention.
Practical Implementation Examples
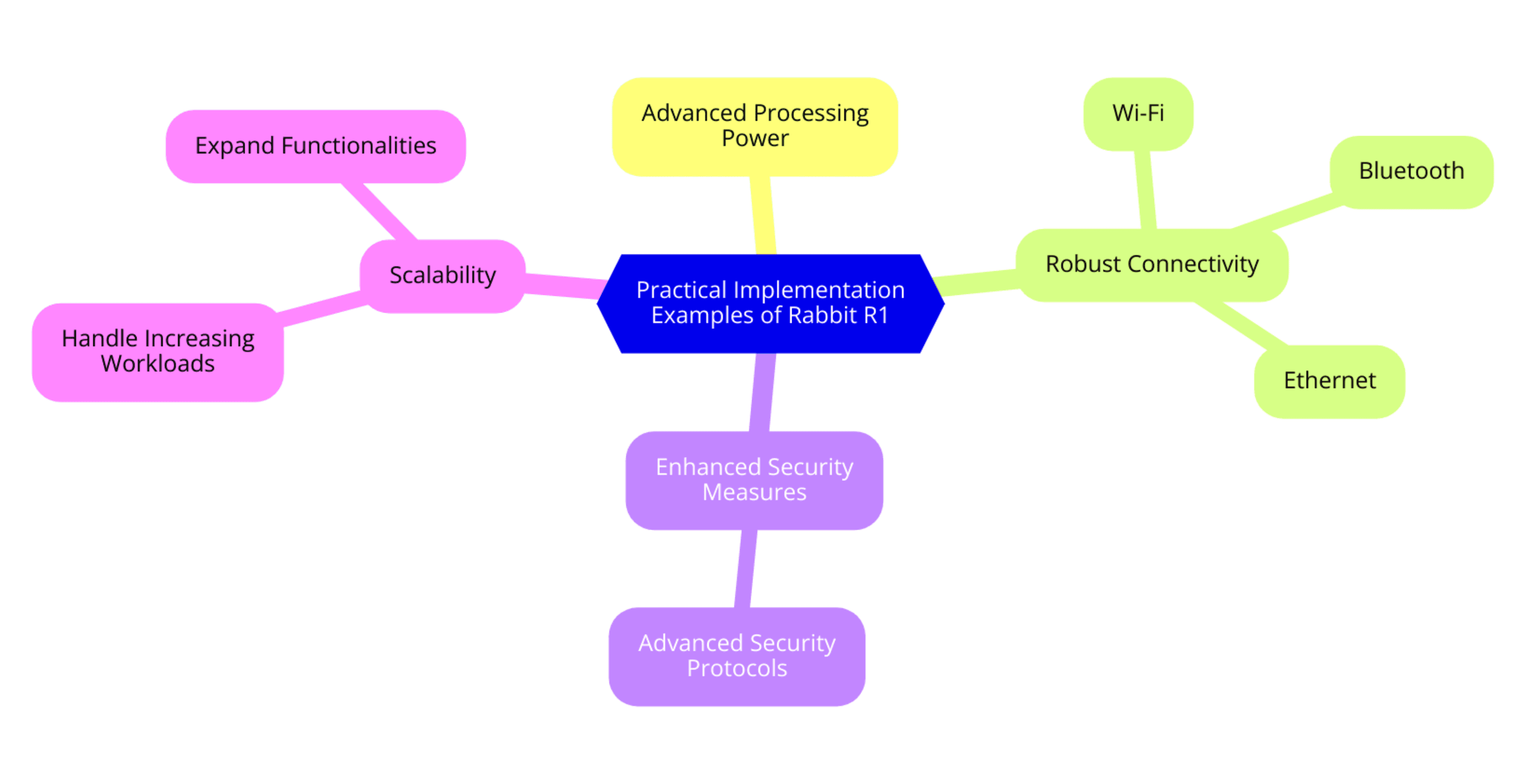
If you want to see what all the buzz is about a large action model (LAM), you just have to check out devices like Rabbit r1. It is like having your assistant but in a smarter way. Regarding virtual assistants, LAMs are the real MVPs, making our lives easier, one task at a time.
The Rabbit R1 device is a considerable development in Artificial Intelligence. It is formatted with state-of-the-art technology and features immense operational capabilities and AI applications.
Features:
Advanced Processing Power: Rabbit R1 has an extraordinary ability with the power of advanced processing
Robust Connectivity: It has different sets of connectivity options, such as Wi-Fi, Bluetooth, and Ethernet to perform seamless operations
Enhanced Security Measures: The Rabbit R1 has advanced security protocols to protect sensitive data
Scalability: Rabbit R1 can handle increasing workloads and expand its detailed functionalities.
Rabbit R1 device operates with advanced hardware and software capabilities to perform different AI tasks. It mostly uses machine learning algorithms to analyze data and recognize patterns before making decisions. Rabbit R1 can increase the performance and reliability of AI-driven systems.
It also has different features to perform operations. These qualities work for it and make it crucial in delivering intelligent solutions for various industries.
This statement means that AI and virtual assistants are integral parts of modern technology for Seamless Human-Computer Interaction. The Rabbit R1 device plays a noteworthy role in enhancing these interactions with its exclusive AI capabilities.
Ability to perform Natural Language Processing: Rabbit R1 can process and understand natural language with high accuracy with proper commands
Real-Time Data Processing: The Rabbit R1 is a powerful tool to process real-time data with AI-driven systems
Improved Personalization: Rabbit R1 holds systems with a highly accurate personalized approach that effectively follows commands as per the user prompts.
Enhanced Security and Privacy: it protects sensitive data and maintains all user security aspects.
Scalability for Diverse Applications: The Rabbit R1 device's scalability ensures that AI assistants can be deployed in different platforms and industries, such as healthcare and customer service.
Thus, the Rabbit R1 device has advancements in enabling AI technology. Its features and operational capabilities make it a critical asset in enhancing the functionality and reliability of complex AI systems.
Challenges and Future Considerations
This system is not free from specific challenges. Large Action Models (LAMs) in AI work to bring ethical, safety, and transparency challenges. Maintaining ethical guidelines is crucial to preventing misuse, as misusing is a significant challenge for this system.
You can expect this system to keep all safety protocols to ensure reliability in high-stakes environments, but a violation can happen in alternative situations. Transparency is a crucial concern in this scenario, and applying robust policies can ensure it.
Human involvement in LAM design and control increases trust in the system. However, from diverse perspectives, AI developers and users can foster human-in-the-loop systems to maintain consistent collaboration.
Explainable AI also works in favor of and helps users understand and verify LAM decisions. RagaAI is working simultaneously on this with extensive services.
LAMs might expand their capabilities to serve larger groups without considerable individual-centric applications. Developing more dynamics and sophisticated algorithms for real-time learning and adaptation and learning to improve performance continuously are also considerable future trends for LAMs.
Conclusion: The Impact and Future of LAMs in AI
Thus, to assess “what a large action model” is, large action models (LAMs) are mostly revolutionizing AI by working on the boundaries of being more autonomous and intelligent. These powerful models enable AI systems to perform complex tasks without massive human participation effectively.
LAMs are creating a transformative shift in how tasks are executed and interacted with. Imagine a world where repetitive and time-consuming processes are automated, freeing you to focus on a more strategic and creative journey. This new world offers unimagined productivity and opens innovative possibilities.
The future is bright, with the promise of enhanced human-machine collaboration. LAMs will work alongside you and can augment all your capabilities to perform day-to-day tasks with greater productivity and innovation.
Lastly, LAMs are advancing AI and reshaping how we live and work. We will have a future where LAMs help you unlock new levels of efficiency. Creativity, along with the dynamic scope of progress and innovation, is also a part of it. You can tryRagaAI today to enhance your LLM skills effectively.