Understanding LLM Agent Architecture
Understanding LLM Agent Architecture
Understanding LLM Agent Architecture
Rehan Asif
Aug 19, 2024
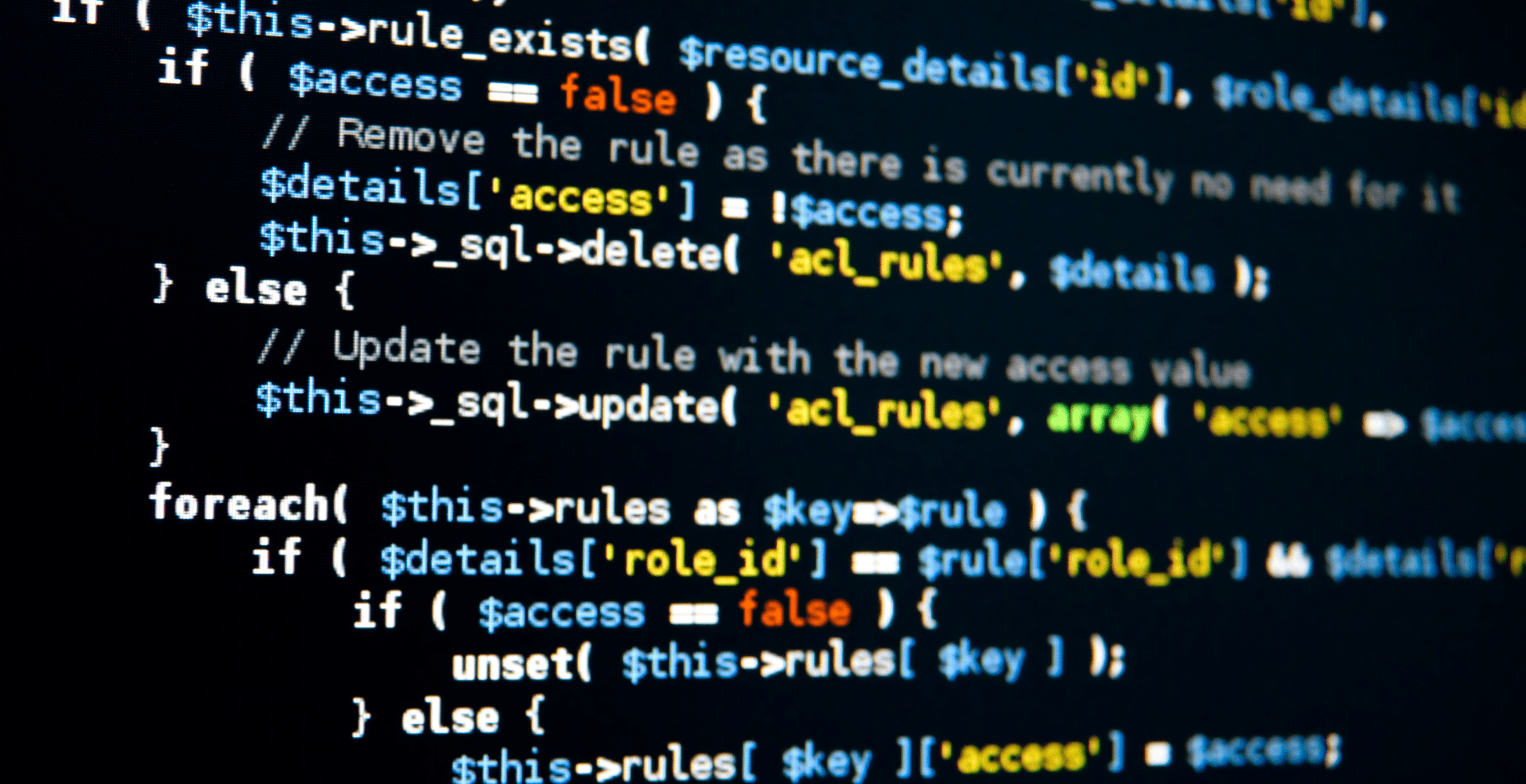
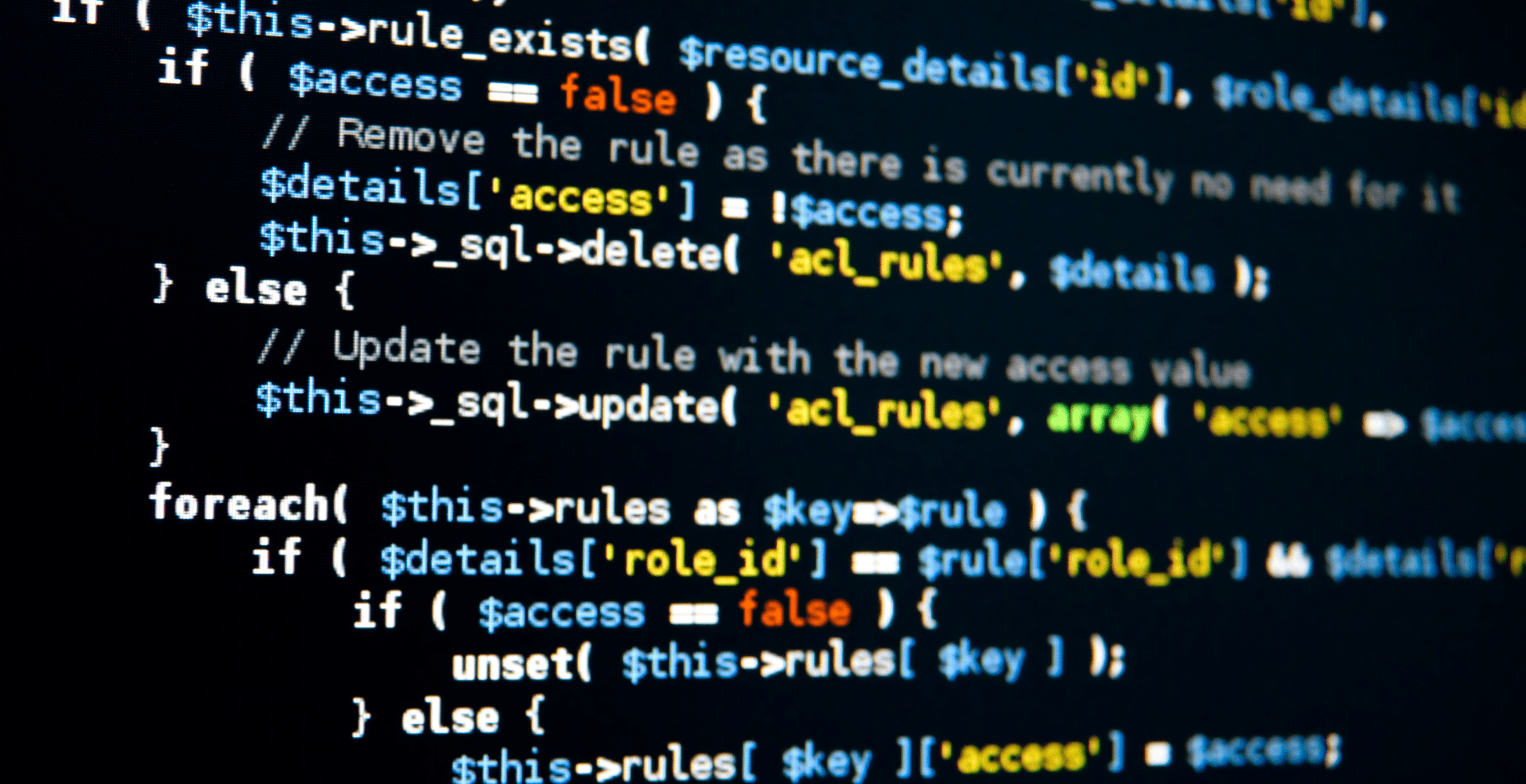
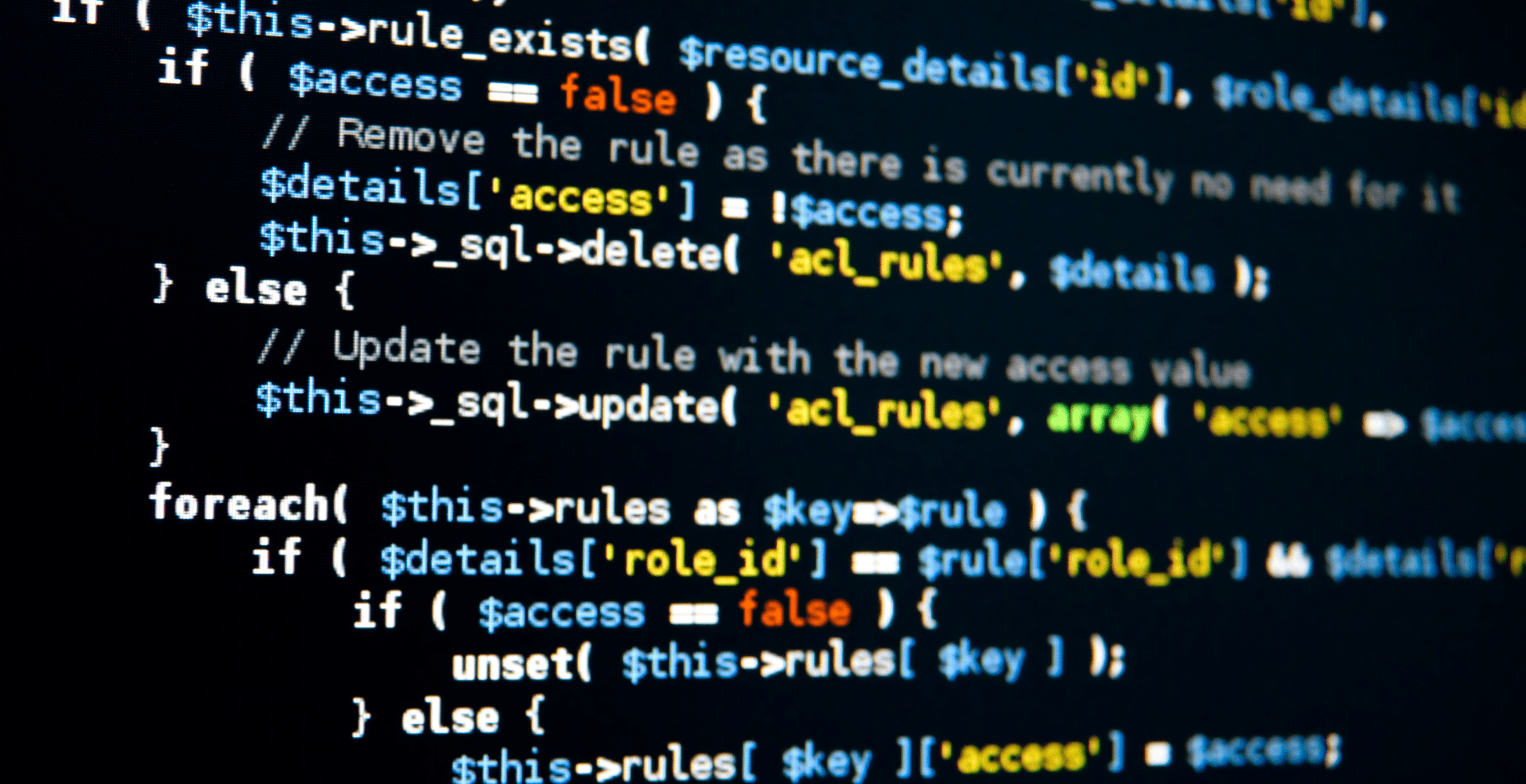
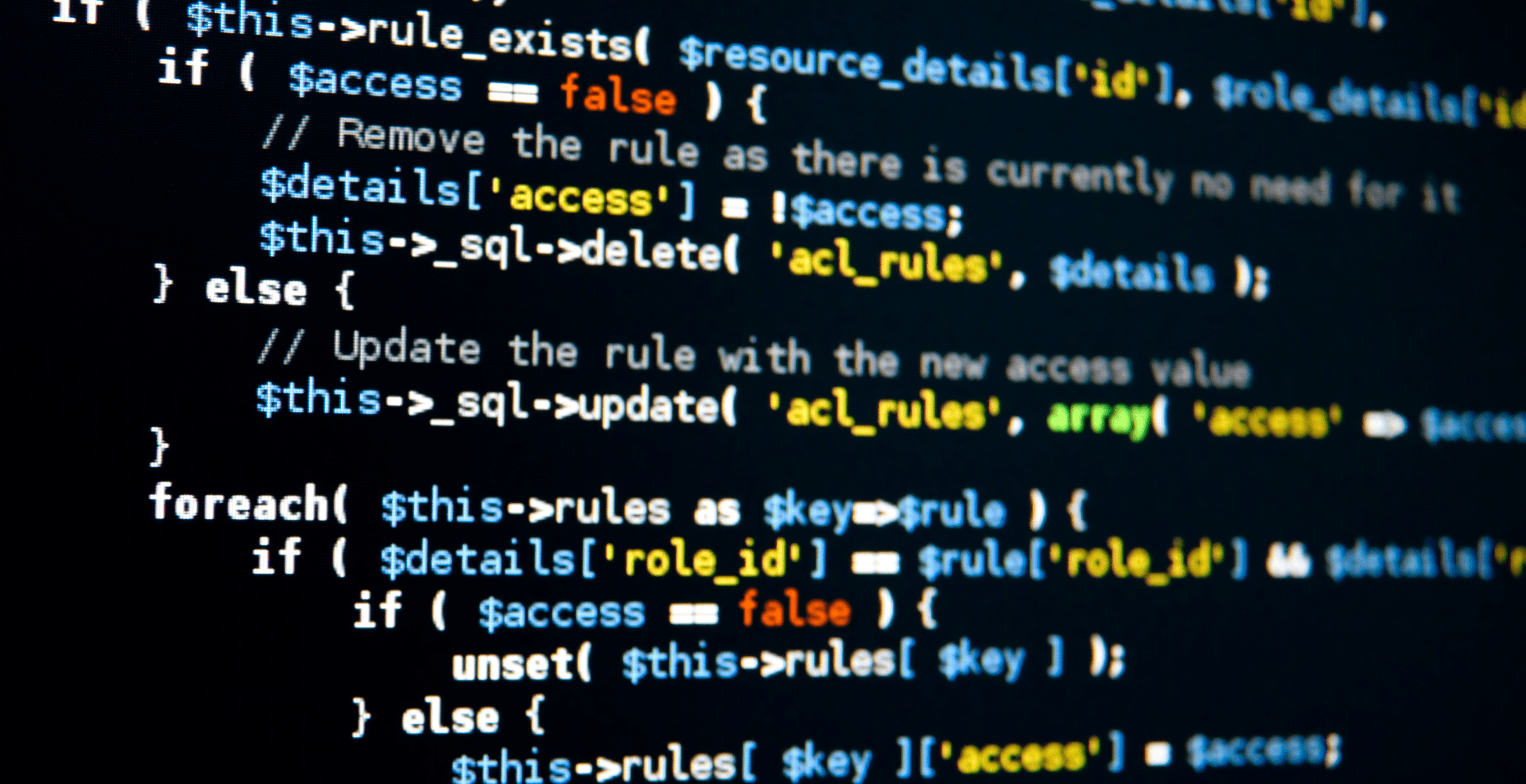
In the ever-evolving field of Artificial Intelligence, large language models (LLMs) have transformed the way we approach intricate tasks. You might wonder how these potent models can be structured into effective agents capable of performing complex functions. This article will walk you through the intriguing architecture of LLM-based agents, shedding light on their core elements and distinct capabilities.
Ready to take your AI abilities to the next level? Then, read our comprehensive guide on Understanding LLM Alignment: A Simple Guide.
Introduction
Explanation of LLM-Based Agents
Sophisticated AI systems use the comprehensive knowledge and language processing abilities of large language models to perform tasks. These agents can execute various functions, from responding to queries to generating creative content, making them flexible tools in multiple applications.
Defining LLM Agents and Their Unique Architecture
At their core, LLM agents combine the vast computational power of large language models (LLMs) with a specialized architecture that enables them to execute precise tasks. This architecture includes elements like memory modules, planning strategies, and tool incorporations, all designed to the agent’s effectiveness.
Building Agents with Large Language Models for Complex Tasks: Role and Intuition
Building agents with LLMs involves deeply comprehending how these models can elucidate and produce human-like text. This intuition behind this lies in using the model’s capability to refine natural language, enabling the agent to tackle intricate tasks by breaking them down into tractable parts and accurately performing them.
Want to know the distinctions between LLM Pre-Training and Fine-Tuning? Then, you can read our detailed guide on LLM Pre-Training and Fine-Tuning Differences.
Components of LLM Agents
Unleashing the secrets behind LLM agents, you’ll discover an intriguing blend of technology and brain power that powers some of the most advanced conversational systems now:
Key Components of LLM Agents

When knowing about Large Language Model (LLM) agents, you’ll locate numerous key components that tick these systems. These elements operate together to ensure the agents can comprehend, process, and respond to various tasks efficiently. Let’s break down these critical components to get a clear picture of what powers these expanded systems.
Training Data: The cornerstone of any LLM agent is its training data. You require enormous amounts of text data for the model to grasp language patterns, grammar, and context. The quality and assortment of this data substantially affect the agent’s performance. By feeding your model disparate and considerable data, you ensure it can handle various topics and variations in human language.
Architecture: An LLM agent's architecture is comparable to its skeleton. Models such as GPT-4 use a transformer architecture, which is prominent for its effectiveness in comprehending context and relationships in text. The design allows the agent to refine data in corresponding ways, making it faster and more robust. You benefit from this architecture as it enables the agent to produce coherent and contextually pertinent responses.
Tokenization: Tokenization breaks down words and sentences into tractable pieces called tokens. This step is critical because it alters words and sentences into a format the model can comprehend. By tokenizing text, you ensure that the agent can refine and dissect the information precisely, leading to better execution in producing responses.
Training Process: Training an LLM agent encompasses feeding it large datasets and fine-tuning it through numerous repetitions. This process helps the model grasp its errors and enhance its precision. You accomplish this by using techniques such as supervised learning, where the model is accompanied by correct instances, and reinforcement learning, where it is rewarded for producing the right yield.
Inference: Inference is when the trained model produces answers based on new input. When you input a query or a prompt, the model uses its grasped knowledge to give a response. This is where the wizardry happens, and you see the practical application of all training and fine-tuning.
General vs. Specific Prompts in Agent Functionality
Prompts are like the guidelines to give you to the LLM. They can be general or specific, and each type plays a pivotal role. General prompts are extensive and open-ended, permitting the LLM to produce more disparate and creative answers. In contrast, specific prompts give clear, narrow guidelines accompanying the LLM to deliver accurate and pertinent answers. Comprehending when to use each type can substantially improve the functionality and precision of the agent.
Dual Aspects of Memory in Agents: Working and Long-term
Memory is another crucial component. LLM agents use both short-term (working) memory and long-term memory. Short-term memory lets the agent keep track of the current conversation, sustaining context and coherence across interactions. Meanwhile, long-term memory enables the agent to remember past interactions, knowledge and facts, which is critical for offering congruous and informed responses over time.
The Importance of Knowledge in Solving Tasks
Knowledge is power, and for LLM agents, it’s important for comprehending and solving tasks. The more knowledge an agent has, the better it can understand intricate questions and provide precise responses. This knowledge base can indulge facts, concepts, processes, and perceptions, all of which contribute to the agent’s capability to perform a wide range of functions efficiently.
Planning: Essential for Task and Question Decomposition
Planning is a strategy behind the agent’s actions. It involves breaking down tasks and queries into tractable elements, permitting the agent to tackle them step by step. An efficient strategy ensures that the agent can handle intricate questions methodologically, enhancing both effectiveness and precision. By decomposing tasks, the agent can also better use its resources and abilities.
Discover the key to using advanced AI abilities. Grasp the best practices for Evaluating Large Language Models: Methods And Metrics to drive innovation and effectiveness in your projects. Begin your expedition today!
Agent Core
Ever thought about how Large Language Models work? Let’s learn about LLM architecture and discover how these agents set intentions, manage tasks, and interact with users like never before.
Insight into the Agent Core
The agent core lies at the heart of every language model. This core is responsible for a variety of functions that make the agent acutely adaptable and powerful.
Goal Setting: First and foremost, the agent core sets goals. These goals guide the agent’s actions and verdicts, ensuring it remains concentrated on delivering the best possible results for you. Whether you’re seeking responses to precise queries or need support with intricate tasks, the agent core keeps everything on track.
Execution Tools: Next up are the execution tools. Think of these as the agent’s toolbox, filled with numerous abilities that help it execute tasks effectively. From refining natural processing to generating responses, these tools operate together smoothly, providing you with precise and pertinent data.
Dynamic Memory Management: One of the most imposing aspects of the agent core is its dynamic memory management. The agent continually remembers past interactions and uses that data to enhance future answers. It’s like having personal support that gets wise and more helpful with each conversation.
Reasoning and Task Execution
An LLM agent isn’t just about ensuing orders. It can reason and perform tasks with notable precision. When you ask a query, the agent doesn’t simply recover or pre-programmed responses. Instead, it refines your question, comprehends the context, and composes customized responses to your requirements.
These reasoning abilities extend to intricate tasks as well. Whether you’re sustaining a project, systematizing data, or seeking advice, the agent can dissect the circumstances, contemplate numerous factors, and offer well-thought-out solutions.
Optional Persona Customization and Enhancing User-Agent Interaction
Optional Persona Customization
To make interactions even more appealing, you can tailor the agent’s persona. This optional attribute permits you to adjust the agent’s tone, style, and behaviour to suit your choices better. Suppose interact with an agent that not only comprehends your requirements but also matches your communication style finely.
Enhancing User-Agent Interaction
Tailoring improves the overall user-agent interaction, making it more peculiar and delightful. Whether you select a formal, professional tone or a friendly, casual vibe, persona customization ensures the agent feels more like a real conversation partner.
Unleash your potential with 20 LLM Project Ideas For Beginners Using Large Language Models! Let’s learn about Large Language Models (LLMs) and stimulate your expedition with these beginner-friendly projects. Begin exploring now and revolutionize your ideas into reality!
Memory Module
Every virtual support remembers your past order details or interactions, and that is why whenever you order something, you see your past orders and recommendations. This happens because of the memory module. Let’s know in detail:
Short-Term vs. Long-Term Memory in Agents
Imagine the human brain, how it remembers phone numbers for a few seconds but retains precious memories for years. Similarly, in LLM agent architecture, short-term memory handles instant, temporary information, think of it like a sticky note. It’s great for keeping track of your current chat or immediate tasks. On the other hand, long-term memory is the agent’s hard drive, storing data that it has grasped over time. This helps in sustaining context over prolonged interactions and enhancing answers based on past experiences. Knowing this distinction helps you comprehend how agents juggle instant tasks while building a knowledge base for the future.
Memory Techniques for Enhanced Agent Decision-Making
To make smart choices, LLM agents use numerous memory techniques. Recurrent Neural Networks (RNNs), for example, help in refining sequences of information by sustaining a form of short-term memory. Meanwhile, transformer models, which power most modern LLMs, use attention apparatus to concentrate on pertinent parts of the input data selectively. These techniques enable the agent to provide coherent, context-aware responses, making them important. You can think of this as the agent’s way of not just hearing but truly paying attention and comprehending the context of your queries.
Knowledge Integration and Management
An agent’s memory system is an intricate connection of integration and management. It’s like a librarian classifying and shelving books effectively. The agent continuously updates its knowledge base with new data while ensuring that the most pertinent information is easily attainable. Efficient knowledge integration means the agent smoothly integrates new facts into its existing structure, improving its decision-making abilities. Sustaining this memory system effectively is critical because it ensures the agent remains precise, pertinent and useful over time. So, when you interact with an LLM agent, you’re aiding from a well-organized, ever-growing knowledge storage.
Unleash the power of innovative AI technology with our thorough guide: Practical Strategies For Self-Hosting Large Language Models. Learn proven methods and best practices to smoothly incorporate advanced language models into your infrastructure!
Planning Module
Improve your LLM agents' capabilities with a thorough planning module designed to streamline task enforcement and elevate decision-making effectiveness. This crucial component ensures your AI works accurately and flexiblely, optimizing productivity and results.
Effective Strategies for Problem Solving
Efficient planning strategies are important to tackle complicated issues. One key strategy is task decomposition. By breaking down a large task into smaller, more tractable components, you can approach each part with a clear focus, making the whole process more effective. This method helps avert oppression and permits methodical progress.
Another critical strategy is self-reflection. Frequently assessing your approach and progress helps identify any flaws or ineffectiveness. By reflecting on what works and what doesn’t, you can adapt your techniques and constantly enhance your problem-solving skills.
Managing Complex Tasks with Subgoal Identification
Complex tasks can be challenging, but subgoal identification and decomposition make them more tractable. Begin by identifying major milestones you need to accomplish. These milestones become your subgoals. Then, break each subgoal into smaller, applicable steps.
For example, if you’re developing a new software attribute, your subgoals might include researching user requirements, designing the attribute, and testing it. Each of these subgoals can be further decomposed into precise tasks, like creating user surveys or writing code.
This approach not only makes the task less formidable but also gives a clear strategy to follow, ensuring that you stay on track and make congruous progress.
Using Reflection and Critique for Plan Refinement
Once you have a plan in place, it’s critical to assess and process it constantly. This is where reflection and critical techniques come into play. After enforcing a part of your plan, take the time to reflect on its efficiency. Ask yourself queries like: Did this approach work as anticipated? What hurdles did I encounter? How can I enhance this process?
In addition, adopt critic thinking. Be your own formidable critic by objectively analyzing your methods and results. Look for areas of enhancement and be open to making significant alterations.
By frequently reflecting and evaluating your strategies, you can fine-tune your strategy, leading to more effective problem-solving.
Want to know about Large Action Models? Read our pragmatic guide on Understanding Large Action Models In AI.
Tool Usage in LLM Agents
Comprehending the efficient use of tools in Large Language Models (LLMs) can be a groundbreaker. Let’s know in detail about the key tools usage ensuring your LLM agents work savvy, not arduous.
Enhancing Capabilities with Tool Augmentation
At their core, LLM agents are acutely skilled at refining and producing text. However, their real power emerges when you augment them with external tools. Think of tools as special skills or extensions that you could add to your agent, much like apps on your smartphone.
For example, you might incorporate an LLM agent with a data analysis tool. This allows the agent to comprehend your query about sales trends, dissect your sales data, and give applicable perceptions. Another instance is blending an LLM with a scheduling tool, enabling it to set up schedules and sustain your calendar effectively.
By combining natural language comprehension with precise functionalities, LLM agents can execute a broader range of tasks, making them indispensable in both personal and professional settings.
Executable Workflows: The Heart of Functionality
To truly use the potential of LLM agents, you must comprehend executable workflows. These workflows are significantly step-by-step procedures that the agent can follow to finish a task. Tools are essential to these workflows, providing significant functions to accomplish the desired results.
Contemplate a synopsis where you need to produce a financial report. An LLM agent equipped with data recovery, analysis, and report generation tools can automate this process. The productivity might involve retrieving data from numerous sources, dissecting it to identify key trends, and then composing the outcomes into a polished report. Each step in this productivity depends on various tools working smoothly together, orchestrated by the LLM agent’s language processing capabilities.
Beyond Training: Leveraging External Tools
One of the most intriguing aspects of LLM agents is their capability to use external tools for tasks beyond their inaugural training. While an LLM is trained on vast amounts of text, it doesn’t intrinsically know how to execute every possible task. That’s where external tools come into play.
For instance, an LLM agent might not have built-in knowledge about the latest stock market trends. However, by incorporating a real-time financial data API, the agent can attain up-to-date data and offer pertinent advice or perceptions. This ability to tap into external resources extends the agent’s usefulness far beyond its original training data.
Another conclusive use case in customer support is an LLM agent connected to a repository of common troubleshooting steps and solutions. When a customer presents an issue, the agent can quickly search the repository and provide the apt solution, substantially enhancing the effectiveness and precision of customer support.
Empower your AI with custom LLM guardrails by reading our detailed guide on Building And Implementing Custom LLM Guardrails.
Architectures and Types of LLM Agents
Using Large Language Models (LLMs) has become invaluable. But to truly use their potential, comprehending their architectures and types is critical.
Overview of LLM Agent Architectures and Applications
Large Language Models (LLMs) are revolutionizing how we communicate with technology. These models, built on neural network architectures, can refine and produce human-like text. Think of them as your digital assistants, capable of comprehending and answering your questions in a conversational manner.
Architecture of LLM agents differ, but the common thread is their capability to use vast amounts of data to execute tasks like text generation, translation and summarization. The most renowned architectures indulge in transformer models such as GPT (Generative Pre-trained Transformer), which is the backbone of many LLMs today. These models handle sequential data, making them ideal for comprehending context and producing coherent answers.
Applications of LLM agents are enormous. In customer service, they can handle queries and provide solutions effectively. In content creation, they aid in drafting articles, scripts and even poetry. For ventures, they dissect data, generate reports, and automate everyday tasks. Significantly, wherever there’s text, LLMs can step in and improve workflow.
Web Agents vs. Tool Agents: Key Differences and Problem-Solving Capabilities
Now, let's know about the types of LLM agents: Web Agents and Tool Agents.
Web Agents
Web Agents are designed to check the internet, pulling data from numerous sources to provide you with precise and thorough responses. Suppose you’re planning a trip and need information about flights, hotels and local attractions. A web agent can browse the website, collect the latest data, and present it to you systematically. Their ability to incorporate and demonstrate web data makes them indispensable for research, content aggregation, and real-time updates.
Problem-Solving Capabilities of Web Agents:
Data-Retrieval: Quickly collect data from multiple sources.
Research Assistance: Assemble and summarize research materials.
Real-Time Updates: Give the latest news, weather, and stock data.
Tool Agents
On the other hand, Tool Agents are designed to interact with precise software tools or systems to execute tasks. These agents are like your personal software experts. Need help with a spreadsheet or project management tool? A tool can step in, enforce commands, automate recurring tasks, and troubleshoot problems.
Problem-Solving Capabilities of Tool Agents:
Task Automation: Sleek recurring tasks within software tools.
Software Integration: Smoothly interact with numerous applications.
Efficiency Improvement: Optimize productivity and reduce manual effort.
Choosing the Right Agent
When selecting between web agents and tool agents, contemplate your requirements. If you need the latest data from the internet, a web agent is your go-to. If your tasks are more software-centric, a tool agent will be more advantageous. Both types of agents bring distinct strengths to the table, improving your workflow and problem-solving capabilities in various ways.
Comprehending these differences aids you in using LLM agents more efficiently, ensuring you choose the right tool for the job. Whether checking the website or optimizing software productivity, LLM agents are here to assist you every step of the way.
Discover the power of AI by checking our detailed guide on Comparing Different Large Language Models (LLM).
Conclusion
Comprehending the architecture and elements of LLM-based agents discloses the incorporated design and functionality that drive their splendid capabilities. These agents use the power of large language models, incorporating memory systems, planning modules, and external tools to execute intricate tasks effectively. From sustaining short-term and long-term memory to engaging advanced planning strategies and incorporating numerous tools, LLM agents depict a blend of sophisticated methods and intuitive designs. Whether you are a developer, researcher, or enthusiast, comprehending these principles equips you with the knowledge to use the full potential of LLM-based agents in your projects.
Boost your testing workflow with RagaAI’s cutting-edge platform. Relish smooth incorporation, advanced automation, and unmatched effectiveness that will transform your procedures. Don’t miss the chance to improve your productivity. Sign up for a free trial today and experience the distinction!
In the ever-evolving field of Artificial Intelligence, large language models (LLMs) have transformed the way we approach intricate tasks. You might wonder how these potent models can be structured into effective agents capable of performing complex functions. This article will walk you through the intriguing architecture of LLM-based agents, shedding light on their core elements and distinct capabilities.
Ready to take your AI abilities to the next level? Then, read our comprehensive guide on Understanding LLM Alignment: A Simple Guide.
Introduction
Explanation of LLM-Based Agents
Sophisticated AI systems use the comprehensive knowledge and language processing abilities of large language models to perform tasks. These agents can execute various functions, from responding to queries to generating creative content, making them flexible tools in multiple applications.
Defining LLM Agents and Their Unique Architecture
At their core, LLM agents combine the vast computational power of large language models (LLMs) with a specialized architecture that enables them to execute precise tasks. This architecture includes elements like memory modules, planning strategies, and tool incorporations, all designed to the agent’s effectiveness.
Building Agents with Large Language Models for Complex Tasks: Role and Intuition
Building agents with LLMs involves deeply comprehending how these models can elucidate and produce human-like text. This intuition behind this lies in using the model’s capability to refine natural language, enabling the agent to tackle intricate tasks by breaking them down into tractable parts and accurately performing them.
Want to know the distinctions between LLM Pre-Training and Fine-Tuning? Then, you can read our detailed guide on LLM Pre-Training and Fine-Tuning Differences.
Components of LLM Agents
Unleashing the secrets behind LLM agents, you’ll discover an intriguing blend of technology and brain power that powers some of the most advanced conversational systems now:
Key Components of LLM Agents

When knowing about Large Language Model (LLM) agents, you’ll locate numerous key components that tick these systems. These elements operate together to ensure the agents can comprehend, process, and respond to various tasks efficiently. Let’s break down these critical components to get a clear picture of what powers these expanded systems.
Training Data: The cornerstone of any LLM agent is its training data. You require enormous amounts of text data for the model to grasp language patterns, grammar, and context. The quality and assortment of this data substantially affect the agent’s performance. By feeding your model disparate and considerable data, you ensure it can handle various topics and variations in human language.
Architecture: An LLM agent's architecture is comparable to its skeleton. Models such as GPT-4 use a transformer architecture, which is prominent for its effectiveness in comprehending context and relationships in text. The design allows the agent to refine data in corresponding ways, making it faster and more robust. You benefit from this architecture as it enables the agent to produce coherent and contextually pertinent responses.
Tokenization: Tokenization breaks down words and sentences into tractable pieces called tokens. This step is critical because it alters words and sentences into a format the model can comprehend. By tokenizing text, you ensure that the agent can refine and dissect the information precisely, leading to better execution in producing responses.
Training Process: Training an LLM agent encompasses feeding it large datasets and fine-tuning it through numerous repetitions. This process helps the model grasp its errors and enhance its precision. You accomplish this by using techniques such as supervised learning, where the model is accompanied by correct instances, and reinforcement learning, where it is rewarded for producing the right yield.
Inference: Inference is when the trained model produces answers based on new input. When you input a query or a prompt, the model uses its grasped knowledge to give a response. This is where the wizardry happens, and you see the practical application of all training and fine-tuning.
General vs. Specific Prompts in Agent Functionality
Prompts are like the guidelines to give you to the LLM. They can be general or specific, and each type plays a pivotal role. General prompts are extensive and open-ended, permitting the LLM to produce more disparate and creative answers. In contrast, specific prompts give clear, narrow guidelines accompanying the LLM to deliver accurate and pertinent answers. Comprehending when to use each type can substantially improve the functionality and precision of the agent.
Dual Aspects of Memory in Agents: Working and Long-term
Memory is another crucial component. LLM agents use both short-term (working) memory and long-term memory. Short-term memory lets the agent keep track of the current conversation, sustaining context and coherence across interactions. Meanwhile, long-term memory enables the agent to remember past interactions, knowledge and facts, which is critical for offering congruous and informed responses over time.
The Importance of Knowledge in Solving Tasks
Knowledge is power, and for LLM agents, it’s important for comprehending and solving tasks. The more knowledge an agent has, the better it can understand intricate questions and provide precise responses. This knowledge base can indulge facts, concepts, processes, and perceptions, all of which contribute to the agent’s capability to perform a wide range of functions efficiently.
Planning: Essential for Task and Question Decomposition
Planning is a strategy behind the agent’s actions. It involves breaking down tasks and queries into tractable elements, permitting the agent to tackle them step by step. An efficient strategy ensures that the agent can handle intricate questions methodologically, enhancing both effectiveness and precision. By decomposing tasks, the agent can also better use its resources and abilities.
Discover the key to using advanced AI abilities. Grasp the best practices for Evaluating Large Language Models: Methods And Metrics to drive innovation and effectiveness in your projects. Begin your expedition today!
Agent Core
Ever thought about how Large Language Models work? Let’s learn about LLM architecture and discover how these agents set intentions, manage tasks, and interact with users like never before.
Insight into the Agent Core
The agent core lies at the heart of every language model. This core is responsible for a variety of functions that make the agent acutely adaptable and powerful.
Goal Setting: First and foremost, the agent core sets goals. These goals guide the agent’s actions and verdicts, ensuring it remains concentrated on delivering the best possible results for you. Whether you’re seeking responses to precise queries or need support with intricate tasks, the agent core keeps everything on track.
Execution Tools: Next up are the execution tools. Think of these as the agent’s toolbox, filled with numerous abilities that help it execute tasks effectively. From refining natural processing to generating responses, these tools operate together smoothly, providing you with precise and pertinent data.
Dynamic Memory Management: One of the most imposing aspects of the agent core is its dynamic memory management. The agent continually remembers past interactions and uses that data to enhance future answers. It’s like having personal support that gets wise and more helpful with each conversation.
Reasoning and Task Execution
An LLM agent isn’t just about ensuing orders. It can reason and perform tasks with notable precision. When you ask a query, the agent doesn’t simply recover or pre-programmed responses. Instead, it refines your question, comprehends the context, and composes customized responses to your requirements.
These reasoning abilities extend to intricate tasks as well. Whether you’re sustaining a project, systematizing data, or seeking advice, the agent can dissect the circumstances, contemplate numerous factors, and offer well-thought-out solutions.
Optional Persona Customization and Enhancing User-Agent Interaction
Optional Persona Customization
To make interactions even more appealing, you can tailor the agent’s persona. This optional attribute permits you to adjust the agent’s tone, style, and behaviour to suit your choices better. Suppose interact with an agent that not only comprehends your requirements but also matches your communication style finely.
Enhancing User-Agent Interaction
Tailoring improves the overall user-agent interaction, making it more peculiar and delightful. Whether you select a formal, professional tone or a friendly, casual vibe, persona customization ensures the agent feels more like a real conversation partner.
Unleash your potential with 20 LLM Project Ideas For Beginners Using Large Language Models! Let’s learn about Large Language Models (LLMs) and stimulate your expedition with these beginner-friendly projects. Begin exploring now and revolutionize your ideas into reality!
Memory Module
Every virtual support remembers your past order details or interactions, and that is why whenever you order something, you see your past orders and recommendations. This happens because of the memory module. Let’s know in detail:
Short-Term vs. Long-Term Memory in Agents
Imagine the human brain, how it remembers phone numbers for a few seconds but retains precious memories for years. Similarly, in LLM agent architecture, short-term memory handles instant, temporary information, think of it like a sticky note. It’s great for keeping track of your current chat or immediate tasks. On the other hand, long-term memory is the agent’s hard drive, storing data that it has grasped over time. This helps in sustaining context over prolonged interactions and enhancing answers based on past experiences. Knowing this distinction helps you comprehend how agents juggle instant tasks while building a knowledge base for the future.
Memory Techniques for Enhanced Agent Decision-Making
To make smart choices, LLM agents use numerous memory techniques. Recurrent Neural Networks (RNNs), for example, help in refining sequences of information by sustaining a form of short-term memory. Meanwhile, transformer models, which power most modern LLMs, use attention apparatus to concentrate on pertinent parts of the input data selectively. These techniques enable the agent to provide coherent, context-aware responses, making them important. You can think of this as the agent’s way of not just hearing but truly paying attention and comprehending the context of your queries.
Knowledge Integration and Management
An agent’s memory system is an intricate connection of integration and management. It’s like a librarian classifying and shelving books effectively. The agent continuously updates its knowledge base with new data while ensuring that the most pertinent information is easily attainable. Efficient knowledge integration means the agent smoothly integrates new facts into its existing structure, improving its decision-making abilities. Sustaining this memory system effectively is critical because it ensures the agent remains precise, pertinent and useful over time. So, when you interact with an LLM agent, you’re aiding from a well-organized, ever-growing knowledge storage.
Unleash the power of innovative AI technology with our thorough guide: Practical Strategies For Self-Hosting Large Language Models. Learn proven methods and best practices to smoothly incorporate advanced language models into your infrastructure!
Planning Module
Improve your LLM agents' capabilities with a thorough planning module designed to streamline task enforcement and elevate decision-making effectiveness. This crucial component ensures your AI works accurately and flexiblely, optimizing productivity and results.
Effective Strategies for Problem Solving
Efficient planning strategies are important to tackle complicated issues. One key strategy is task decomposition. By breaking down a large task into smaller, more tractable components, you can approach each part with a clear focus, making the whole process more effective. This method helps avert oppression and permits methodical progress.
Another critical strategy is self-reflection. Frequently assessing your approach and progress helps identify any flaws or ineffectiveness. By reflecting on what works and what doesn’t, you can adapt your techniques and constantly enhance your problem-solving skills.
Managing Complex Tasks with Subgoal Identification
Complex tasks can be challenging, but subgoal identification and decomposition make them more tractable. Begin by identifying major milestones you need to accomplish. These milestones become your subgoals. Then, break each subgoal into smaller, applicable steps.
For example, if you’re developing a new software attribute, your subgoals might include researching user requirements, designing the attribute, and testing it. Each of these subgoals can be further decomposed into precise tasks, like creating user surveys or writing code.
This approach not only makes the task less formidable but also gives a clear strategy to follow, ensuring that you stay on track and make congruous progress.
Using Reflection and Critique for Plan Refinement
Once you have a plan in place, it’s critical to assess and process it constantly. This is where reflection and critical techniques come into play. After enforcing a part of your plan, take the time to reflect on its efficiency. Ask yourself queries like: Did this approach work as anticipated? What hurdles did I encounter? How can I enhance this process?
In addition, adopt critic thinking. Be your own formidable critic by objectively analyzing your methods and results. Look for areas of enhancement and be open to making significant alterations.
By frequently reflecting and evaluating your strategies, you can fine-tune your strategy, leading to more effective problem-solving.
Want to know about Large Action Models? Read our pragmatic guide on Understanding Large Action Models In AI.
Tool Usage in LLM Agents
Comprehending the efficient use of tools in Large Language Models (LLMs) can be a groundbreaker. Let’s know in detail about the key tools usage ensuring your LLM agents work savvy, not arduous.
Enhancing Capabilities with Tool Augmentation
At their core, LLM agents are acutely skilled at refining and producing text. However, their real power emerges when you augment them with external tools. Think of tools as special skills or extensions that you could add to your agent, much like apps on your smartphone.
For example, you might incorporate an LLM agent with a data analysis tool. This allows the agent to comprehend your query about sales trends, dissect your sales data, and give applicable perceptions. Another instance is blending an LLM with a scheduling tool, enabling it to set up schedules and sustain your calendar effectively.
By combining natural language comprehension with precise functionalities, LLM agents can execute a broader range of tasks, making them indispensable in both personal and professional settings.
Executable Workflows: The Heart of Functionality
To truly use the potential of LLM agents, you must comprehend executable workflows. These workflows are significantly step-by-step procedures that the agent can follow to finish a task. Tools are essential to these workflows, providing significant functions to accomplish the desired results.
Contemplate a synopsis where you need to produce a financial report. An LLM agent equipped with data recovery, analysis, and report generation tools can automate this process. The productivity might involve retrieving data from numerous sources, dissecting it to identify key trends, and then composing the outcomes into a polished report. Each step in this productivity depends on various tools working smoothly together, orchestrated by the LLM agent’s language processing capabilities.
Beyond Training: Leveraging External Tools
One of the most intriguing aspects of LLM agents is their capability to use external tools for tasks beyond their inaugural training. While an LLM is trained on vast amounts of text, it doesn’t intrinsically know how to execute every possible task. That’s where external tools come into play.
For instance, an LLM agent might not have built-in knowledge about the latest stock market trends. However, by incorporating a real-time financial data API, the agent can attain up-to-date data and offer pertinent advice or perceptions. This ability to tap into external resources extends the agent’s usefulness far beyond its original training data.
Another conclusive use case in customer support is an LLM agent connected to a repository of common troubleshooting steps and solutions. When a customer presents an issue, the agent can quickly search the repository and provide the apt solution, substantially enhancing the effectiveness and precision of customer support.
Empower your AI with custom LLM guardrails by reading our detailed guide on Building And Implementing Custom LLM Guardrails.
Architectures and Types of LLM Agents
Using Large Language Models (LLMs) has become invaluable. But to truly use their potential, comprehending their architectures and types is critical.
Overview of LLM Agent Architectures and Applications
Large Language Models (LLMs) are revolutionizing how we communicate with technology. These models, built on neural network architectures, can refine and produce human-like text. Think of them as your digital assistants, capable of comprehending and answering your questions in a conversational manner.
Architecture of LLM agents differ, but the common thread is their capability to use vast amounts of data to execute tasks like text generation, translation and summarization. The most renowned architectures indulge in transformer models such as GPT (Generative Pre-trained Transformer), which is the backbone of many LLMs today. These models handle sequential data, making them ideal for comprehending context and producing coherent answers.
Applications of LLM agents are enormous. In customer service, they can handle queries and provide solutions effectively. In content creation, they aid in drafting articles, scripts and even poetry. For ventures, they dissect data, generate reports, and automate everyday tasks. Significantly, wherever there’s text, LLMs can step in and improve workflow.
Web Agents vs. Tool Agents: Key Differences and Problem-Solving Capabilities
Now, let's know about the types of LLM agents: Web Agents and Tool Agents.
Web Agents
Web Agents are designed to check the internet, pulling data from numerous sources to provide you with precise and thorough responses. Suppose you’re planning a trip and need information about flights, hotels and local attractions. A web agent can browse the website, collect the latest data, and present it to you systematically. Their ability to incorporate and demonstrate web data makes them indispensable for research, content aggregation, and real-time updates.
Problem-Solving Capabilities of Web Agents:
Data-Retrieval: Quickly collect data from multiple sources.
Research Assistance: Assemble and summarize research materials.
Real-Time Updates: Give the latest news, weather, and stock data.
Tool Agents
On the other hand, Tool Agents are designed to interact with precise software tools or systems to execute tasks. These agents are like your personal software experts. Need help with a spreadsheet or project management tool? A tool can step in, enforce commands, automate recurring tasks, and troubleshoot problems.
Problem-Solving Capabilities of Tool Agents:
Task Automation: Sleek recurring tasks within software tools.
Software Integration: Smoothly interact with numerous applications.
Efficiency Improvement: Optimize productivity and reduce manual effort.
Choosing the Right Agent
When selecting between web agents and tool agents, contemplate your requirements. If you need the latest data from the internet, a web agent is your go-to. If your tasks are more software-centric, a tool agent will be more advantageous. Both types of agents bring distinct strengths to the table, improving your workflow and problem-solving capabilities in various ways.
Comprehending these differences aids you in using LLM agents more efficiently, ensuring you choose the right tool for the job. Whether checking the website or optimizing software productivity, LLM agents are here to assist you every step of the way.
Discover the power of AI by checking our detailed guide on Comparing Different Large Language Models (LLM).
Conclusion
Comprehending the architecture and elements of LLM-based agents discloses the incorporated design and functionality that drive their splendid capabilities. These agents use the power of large language models, incorporating memory systems, planning modules, and external tools to execute intricate tasks effectively. From sustaining short-term and long-term memory to engaging advanced planning strategies and incorporating numerous tools, LLM agents depict a blend of sophisticated methods and intuitive designs. Whether you are a developer, researcher, or enthusiast, comprehending these principles equips you with the knowledge to use the full potential of LLM-based agents in your projects.
Boost your testing workflow with RagaAI’s cutting-edge platform. Relish smooth incorporation, advanced automation, and unmatched effectiveness that will transform your procedures. Don’t miss the chance to improve your productivity. Sign up for a free trial today and experience the distinction!
In the ever-evolving field of Artificial Intelligence, large language models (LLMs) have transformed the way we approach intricate tasks. You might wonder how these potent models can be structured into effective agents capable of performing complex functions. This article will walk you through the intriguing architecture of LLM-based agents, shedding light on their core elements and distinct capabilities.
Ready to take your AI abilities to the next level? Then, read our comprehensive guide on Understanding LLM Alignment: A Simple Guide.
Introduction
Explanation of LLM-Based Agents
Sophisticated AI systems use the comprehensive knowledge and language processing abilities of large language models to perform tasks. These agents can execute various functions, from responding to queries to generating creative content, making them flexible tools in multiple applications.
Defining LLM Agents and Their Unique Architecture
At their core, LLM agents combine the vast computational power of large language models (LLMs) with a specialized architecture that enables them to execute precise tasks. This architecture includes elements like memory modules, planning strategies, and tool incorporations, all designed to the agent’s effectiveness.
Building Agents with Large Language Models for Complex Tasks: Role and Intuition
Building agents with LLMs involves deeply comprehending how these models can elucidate and produce human-like text. This intuition behind this lies in using the model’s capability to refine natural language, enabling the agent to tackle intricate tasks by breaking them down into tractable parts and accurately performing them.
Want to know the distinctions between LLM Pre-Training and Fine-Tuning? Then, you can read our detailed guide on LLM Pre-Training and Fine-Tuning Differences.
Components of LLM Agents
Unleashing the secrets behind LLM agents, you’ll discover an intriguing blend of technology and brain power that powers some of the most advanced conversational systems now:
Key Components of LLM Agents

When knowing about Large Language Model (LLM) agents, you’ll locate numerous key components that tick these systems. These elements operate together to ensure the agents can comprehend, process, and respond to various tasks efficiently. Let’s break down these critical components to get a clear picture of what powers these expanded systems.
Training Data: The cornerstone of any LLM agent is its training data. You require enormous amounts of text data for the model to grasp language patterns, grammar, and context. The quality and assortment of this data substantially affect the agent’s performance. By feeding your model disparate and considerable data, you ensure it can handle various topics and variations in human language.
Architecture: An LLM agent's architecture is comparable to its skeleton. Models such as GPT-4 use a transformer architecture, which is prominent for its effectiveness in comprehending context and relationships in text. The design allows the agent to refine data in corresponding ways, making it faster and more robust. You benefit from this architecture as it enables the agent to produce coherent and contextually pertinent responses.
Tokenization: Tokenization breaks down words and sentences into tractable pieces called tokens. This step is critical because it alters words and sentences into a format the model can comprehend. By tokenizing text, you ensure that the agent can refine and dissect the information precisely, leading to better execution in producing responses.
Training Process: Training an LLM agent encompasses feeding it large datasets and fine-tuning it through numerous repetitions. This process helps the model grasp its errors and enhance its precision. You accomplish this by using techniques such as supervised learning, where the model is accompanied by correct instances, and reinforcement learning, where it is rewarded for producing the right yield.
Inference: Inference is when the trained model produces answers based on new input. When you input a query or a prompt, the model uses its grasped knowledge to give a response. This is where the wizardry happens, and you see the practical application of all training and fine-tuning.
General vs. Specific Prompts in Agent Functionality
Prompts are like the guidelines to give you to the LLM. They can be general or specific, and each type plays a pivotal role. General prompts are extensive and open-ended, permitting the LLM to produce more disparate and creative answers. In contrast, specific prompts give clear, narrow guidelines accompanying the LLM to deliver accurate and pertinent answers. Comprehending when to use each type can substantially improve the functionality and precision of the agent.
Dual Aspects of Memory in Agents: Working and Long-term
Memory is another crucial component. LLM agents use both short-term (working) memory and long-term memory. Short-term memory lets the agent keep track of the current conversation, sustaining context and coherence across interactions. Meanwhile, long-term memory enables the agent to remember past interactions, knowledge and facts, which is critical for offering congruous and informed responses over time.
The Importance of Knowledge in Solving Tasks
Knowledge is power, and for LLM agents, it’s important for comprehending and solving tasks. The more knowledge an agent has, the better it can understand intricate questions and provide precise responses. This knowledge base can indulge facts, concepts, processes, and perceptions, all of which contribute to the agent’s capability to perform a wide range of functions efficiently.
Planning: Essential for Task and Question Decomposition
Planning is a strategy behind the agent’s actions. It involves breaking down tasks and queries into tractable elements, permitting the agent to tackle them step by step. An efficient strategy ensures that the agent can handle intricate questions methodologically, enhancing both effectiveness and precision. By decomposing tasks, the agent can also better use its resources and abilities.
Discover the key to using advanced AI abilities. Grasp the best practices for Evaluating Large Language Models: Methods And Metrics to drive innovation and effectiveness in your projects. Begin your expedition today!
Agent Core
Ever thought about how Large Language Models work? Let’s learn about LLM architecture and discover how these agents set intentions, manage tasks, and interact with users like never before.
Insight into the Agent Core
The agent core lies at the heart of every language model. This core is responsible for a variety of functions that make the agent acutely adaptable and powerful.
Goal Setting: First and foremost, the agent core sets goals. These goals guide the agent’s actions and verdicts, ensuring it remains concentrated on delivering the best possible results for you. Whether you’re seeking responses to precise queries or need support with intricate tasks, the agent core keeps everything on track.
Execution Tools: Next up are the execution tools. Think of these as the agent’s toolbox, filled with numerous abilities that help it execute tasks effectively. From refining natural processing to generating responses, these tools operate together smoothly, providing you with precise and pertinent data.
Dynamic Memory Management: One of the most imposing aspects of the agent core is its dynamic memory management. The agent continually remembers past interactions and uses that data to enhance future answers. It’s like having personal support that gets wise and more helpful with each conversation.
Reasoning and Task Execution
An LLM agent isn’t just about ensuing orders. It can reason and perform tasks with notable precision. When you ask a query, the agent doesn’t simply recover or pre-programmed responses. Instead, it refines your question, comprehends the context, and composes customized responses to your requirements.
These reasoning abilities extend to intricate tasks as well. Whether you’re sustaining a project, systematizing data, or seeking advice, the agent can dissect the circumstances, contemplate numerous factors, and offer well-thought-out solutions.
Optional Persona Customization and Enhancing User-Agent Interaction
Optional Persona Customization
To make interactions even more appealing, you can tailor the agent’s persona. This optional attribute permits you to adjust the agent’s tone, style, and behaviour to suit your choices better. Suppose interact with an agent that not only comprehends your requirements but also matches your communication style finely.
Enhancing User-Agent Interaction
Tailoring improves the overall user-agent interaction, making it more peculiar and delightful. Whether you select a formal, professional tone or a friendly, casual vibe, persona customization ensures the agent feels more like a real conversation partner.
Unleash your potential with 20 LLM Project Ideas For Beginners Using Large Language Models! Let’s learn about Large Language Models (LLMs) and stimulate your expedition with these beginner-friendly projects. Begin exploring now and revolutionize your ideas into reality!
Memory Module
Every virtual support remembers your past order details or interactions, and that is why whenever you order something, you see your past orders and recommendations. This happens because of the memory module. Let’s know in detail:
Short-Term vs. Long-Term Memory in Agents
Imagine the human brain, how it remembers phone numbers for a few seconds but retains precious memories for years. Similarly, in LLM agent architecture, short-term memory handles instant, temporary information, think of it like a sticky note. It’s great for keeping track of your current chat or immediate tasks. On the other hand, long-term memory is the agent’s hard drive, storing data that it has grasped over time. This helps in sustaining context over prolonged interactions and enhancing answers based on past experiences. Knowing this distinction helps you comprehend how agents juggle instant tasks while building a knowledge base for the future.
Memory Techniques for Enhanced Agent Decision-Making
To make smart choices, LLM agents use numerous memory techniques. Recurrent Neural Networks (RNNs), for example, help in refining sequences of information by sustaining a form of short-term memory. Meanwhile, transformer models, which power most modern LLMs, use attention apparatus to concentrate on pertinent parts of the input data selectively. These techniques enable the agent to provide coherent, context-aware responses, making them important. You can think of this as the agent’s way of not just hearing but truly paying attention and comprehending the context of your queries.
Knowledge Integration and Management
An agent’s memory system is an intricate connection of integration and management. It’s like a librarian classifying and shelving books effectively. The agent continuously updates its knowledge base with new data while ensuring that the most pertinent information is easily attainable. Efficient knowledge integration means the agent smoothly integrates new facts into its existing structure, improving its decision-making abilities. Sustaining this memory system effectively is critical because it ensures the agent remains precise, pertinent and useful over time. So, when you interact with an LLM agent, you’re aiding from a well-organized, ever-growing knowledge storage.
Unleash the power of innovative AI technology with our thorough guide: Practical Strategies For Self-Hosting Large Language Models. Learn proven methods and best practices to smoothly incorporate advanced language models into your infrastructure!
Planning Module
Improve your LLM agents' capabilities with a thorough planning module designed to streamline task enforcement and elevate decision-making effectiveness. This crucial component ensures your AI works accurately and flexiblely, optimizing productivity and results.
Effective Strategies for Problem Solving
Efficient planning strategies are important to tackle complicated issues. One key strategy is task decomposition. By breaking down a large task into smaller, more tractable components, you can approach each part with a clear focus, making the whole process more effective. This method helps avert oppression and permits methodical progress.
Another critical strategy is self-reflection. Frequently assessing your approach and progress helps identify any flaws or ineffectiveness. By reflecting on what works and what doesn’t, you can adapt your techniques and constantly enhance your problem-solving skills.
Managing Complex Tasks with Subgoal Identification
Complex tasks can be challenging, but subgoal identification and decomposition make them more tractable. Begin by identifying major milestones you need to accomplish. These milestones become your subgoals. Then, break each subgoal into smaller, applicable steps.
For example, if you’re developing a new software attribute, your subgoals might include researching user requirements, designing the attribute, and testing it. Each of these subgoals can be further decomposed into precise tasks, like creating user surveys or writing code.
This approach not only makes the task less formidable but also gives a clear strategy to follow, ensuring that you stay on track and make congruous progress.
Using Reflection and Critique for Plan Refinement
Once you have a plan in place, it’s critical to assess and process it constantly. This is where reflection and critical techniques come into play. After enforcing a part of your plan, take the time to reflect on its efficiency. Ask yourself queries like: Did this approach work as anticipated? What hurdles did I encounter? How can I enhance this process?
In addition, adopt critic thinking. Be your own formidable critic by objectively analyzing your methods and results. Look for areas of enhancement and be open to making significant alterations.
By frequently reflecting and evaluating your strategies, you can fine-tune your strategy, leading to more effective problem-solving.
Want to know about Large Action Models? Read our pragmatic guide on Understanding Large Action Models In AI.
Tool Usage in LLM Agents
Comprehending the efficient use of tools in Large Language Models (LLMs) can be a groundbreaker. Let’s know in detail about the key tools usage ensuring your LLM agents work savvy, not arduous.
Enhancing Capabilities with Tool Augmentation
At their core, LLM agents are acutely skilled at refining and producing text. However, their real power emerges when you augment them with external tools. Think of tools as special skills or extensions that you could add to your agent, much like apps on your smartphone.
For example, you might incorporate an LLM agent with a data analysis tool. This allows the agent to comprehend your query about sales trends, dissect your sales data, and give applicable perceptions. Another instance is blending an LLM with a scheduling tool, enabling it to set up schedules and sustain your calendar effectively.
By combining natural language comprehension with precise functionalities, LLM agents can execute a broader range of tasks, making them indispensable in both personal and professional settings.
Executable Workflows: The Heart of Functionality
To truly use the potential of LLM agents, you must comprehend executable workflows. These workflows are significantly step-by-step procedures that the agent can follow to finish a task. Tools are essential to these workflows, providing significant functions to accomplish the desired results.
Contemplate a synopsis where you need to produce a financial report. An LLM agent equipped with data recovery, analysis, and report generation tools can automate this process. The productivity might involve retrieving data from numerous sources, dissecting it to identify key trends, and then composing the outcomes into a polished report. Each step in this productivity depends on various tools working smoothly together, orchestrated by the LLM agent’s language processing capabilities.
Beyond Training: Leveraging External Tools
One of the most intriguing aspects of LLM agents is their capability to use external tools for tasks beyond their inaugural training. While an LLM is trained on vast amounts of text, it doesn’t intrinsically know how to execute every possible task. That’s where external tools come into play.
For instance, an LLM agent might not have built-in knowledge about the latest stock market trends. However, by incorporating a real-time financial data API, the agent can attain up-to-date data and offer pertinent advice or perceptions. This ability to tap into external resources extends the agent’s usefulness far beyond its original training data.
Another conclusive use case in customer support is an LLM agent connected to a repository of common troubleshooting steps and solutions. When a customer presents an issue, the agent can quickly search the repository and provide the apt solution, substantially enhancing the effectiveness and precision of customer support.
Empower your AI with custom LLM guardrails by reading our detailed guide on Building And Implementing Custom LLM Guardrails.
Architectures and Types of LLM Agents
Using Large Language Models (LLMs) has become invaluable. But to truly use their potential, comprehending their architectures and types is critical.
Overview of LLM Agent Architectures and Applications
Large Language Models (LLMs) are revolutionizing how we communicate with technology. These models, built on neural network architectures, can refine and produce human-like text. Think of them as your digital assistants, capable of comprehending and answering your questions in a conversational manner.
Architecture of LLM agents differ, but the common thread is their capability to use vast amounts of data to execute tasks like text generation, translation and summarization. The most renowned architectures indulge in transformer models such as GPT (Generative Pre-trained Transformer), which is the backbone of many LLMs today. These models handle sequential data, making them ideal for comprehending context and producing coherent answers.
Applications of LLM agents are enormous. In customer service, they can handle queries and provide solutions effectively. In content creation, they aid in drafting articles, scripts and even poetry. For ventures, they dissect data, generate reports, and automate everyday tasks. Significantly, wherever there’s text, LLMs can step in and improve workflow.
Web Agents vs. Tool Agents: Key Differences and Problem-Solving Capabilities
Now, let's know about the types of LLM agents: Web Agents and Tool Agents.
Web Agents
Web Agents are designed to check the internet, pulling data from numerous sources to provide you with precise and thorough responses. Suppose you’re planning a trip and need information about flights, hotels and local attractions. A web agent can browse the website, collect the latest data, and present it to you systematically. Their ability to incorporate and demonstrate web data makes them indispensable for research, content aggregation, and real-time updates.
Problem-Solving Capabilities of Web Agents:
Data-Retrieval: Quickly collect data from multiple sources.
Research Assistance: Assemble and summarize research materials.
Real-Time Updates: Give the latest news, weather, and stock data.
Tool Agents
On the other hand, Tool Agents are designed to interact with precise software tools or systems to execute tasks. These agents are like your personal software experts. Need help with a spreadsheet or project management tool? A tool can step in, enforce commands, automate recurring tasks, and troubleshoot problems.
Problem-Solving Capabilities of Tool Agents:
Task Automation: Sleek recurring tasks within software tools.
Software Integration: Smoothly interact with numerous applications.
Efficiency Improvement: Optimize productivity and reduce manual effort.
Choosing the Right Agent
When selecting between web agents and tool agents, contemplate your requirements. If you need the latest data from the internet, a web agent is your go-to. If your tasks are more software-centric, a tool agent will be more advantageous. Both types of agents bring distinct strengths to the table, improving your workflow and problem-solving capabilities in various ways.
Comprehending these differences aids you in using LLM agents more efficiently, ensuring you choose the right tool for the job. Whether checking the website or optimizing software productivity, LLM agents are here to assist you every step of the way.
Discover the power of AI by checking our detailed guide on Comparing Different Large Language Models (LLM).
Conclusion
Comprehending the architecture and elements of LLM-based agents discloses the incorporated design and functionality that drive their splendid capabilities. These agents use the power of large language models, incorporating memory systems, planning modules, and external tools to execute intricate tasks effectively. From sustaining short-term and long-term memory to engaging advanced planning strategies and incorporating numerous tools, LLM agents depict a blend of sophisticated methods and intuitive designs. Whether you are a developer, researcher, or enthusiast, comprehending these principles equips you with the knowledge to use the full potential of LLM-based agents in your projects.
Boost your testing workflow with RagaAI’s cutting-edge platform. Relish smooth incorporation, advanced automation, and unmatched effectiveness that will transform your procedures. Don’t miss the chance to improve your productivity. Sign up for a free trial today and experience the distinction!
In the ever-evolving field of Artificial Intelligence, large language models (LLMs) have transformed the way we approach intricate tasks. You might wonder how these potent models can be structured into effective agents capable of performing complex functions. This article will walk you through the intriguing architecture of LLM-based agents, shedding light on their core elements and distinct capabilities.
Ready to take your AI abilities to the next level? Then, read our comprehensive guide on Understanding LLM Alignment: A Simple Guide.
Introduction
Explanation of LLM-Based Agents
Sophisticated AI systems use the comprehensive knowledge and language processing abilities of large language models to perform tasks. These agents can execute various functions, from responding to queries to generating creative content, making them flexible tools in multiple applications.
Defining LLM Agents and Their Unique Architecture
At their core, LLM agents combine the vast computational power of large language models (LLMs) with a specialized architecture that enables them to execute precise tasks. This architecture includes elements like memory modules, planning strategies, and tool incorporations, all designed to the agent’s effectiveness.
Building Agents with Large Language Models for Complex Tasks: Role and Intuition
Building agents with LLMs involves deeply comprehending how these models can elucidate and produce human-like text. This intuition behind this lies in using the model’s capability to refine natural language, enabling the agent to tackle intricate tasks by breaking them down into tractable parts and accurately performing them.
Want to know the distinctions between LLM Pre-Training and Fine-Tuning? Then, you can read our detailed guide on LLM Pre-Training and Fine-Tuning Differences.
Components of LLM Agents
Unleashing the secrets behind LLM agents, you’ll discover an intriguing blend of technology and brain power that powers some of the most advanced conversational systems now:
Key Components of LLM Agents

When knowing about Large Language Model (LLM) agents, you’ll locate numerous key components that tick these systems. These elements operate together to ensure the agents can comprehend, process, and respond to various tasks efficiently. Let’s break down these critical components to get a clear picture of what powers these expanded systems.
Training Data: The cornerstone of any LLM agent is its training data. You require enormous amounts of text data for the model to grasp language patterns, grammar, and context. The quality and assortment of this data substantially affect the agent’s performance. By feeding your model disparate and considerable data, you ensure it can handle various topics and variations in human language.
Architecture: An LLM agent's architecture is comparable to its skeleton. Models such as GPT-4 use a transformer architecture, which is prominent for its effectiveness in comprehending context and relationships in text. The design allows the agent to refine data in corresponding ways, making it faster and more robust. You benefit from this architecture as it enables the agent to produce coherent and contextually pertinent responses.
Tokenization: Tokenization breaks down words and sentences into tractable pieces called tokens. This step is critical because it alters words and sentences into a format the model can comprehend. By tokenizing text, you ensure that the agent can refine and dissect the information precisely, leading to better execution in producing responses.
Training Process: Training an LLM agent encompasses feeding it large datasets and fine-tuning it through numerous repetitions. This process helps the model grasp its errors and enhance its precision. You accomplish this by using techniques such as supervised learning, where the model is accompanied by correct instances, and reinforcement learning, where it is rewarded for producing the right yield.
Inference: Inference is when the trained model produces answers based on new input. When you input a query or a prompt, the model uses its grasped knowledge to give a response. This is where the wizardry happens, and you see the practical application of all training and fine-tuning.
General vs. Specific Prompts in Agent Functionality
Prompts are like the guidelines to give you to the LLM. They can be general or specific, and each type plays a pivotal role. General prompts are extensive and open-ended, permitting the LLM to produce more disparate and creative answers. In contrast, specific prompts give clear, narrow guidelines accompanying the LLM to deliver accurate and pertinent answers. Comprehending when to use each type can substantially improve the functionality and precision of the agent.
Dual Aspects of Memory in Agents: Working and Long-term
Memory is another crucial component. LLM agents use both short-term (working) memory and long-term memory. Short-term memory lets the agent keep track of the current conversation, sustaining context and coherence across interactions. Meanwhile, long-term memory enables the agent to remember past interactions, knowledge and facts, which is critical for offering congruous and informed responses over time.
The Importance of Knowledge in Solving Tasks
Knowledge is power, and for LLM agents, it’s important for comprehending and solving tasks. The more knowledge an agent has, the better it can understand intricate questions and provide precise responses. This knowledge base can indulge facts, concepts, processes, and perceptions, all of which contribute to the agent’s capability to perform a wide range of functions efficiently.
Planning: Essential for Task and Question Decomposition
Planning is a strategy behind the agent’s actions. It involves breaking down tasks and queries into tractable elements, permitting the agent to tackle them step by step. An efficient strategy ensures that the agent can handle intricate questions methodologically, enhancing both effectiveness and precision. By decomposing tasks, the agent can also better use its resources and abilities.
Discover the key to using advanced AI abilities. Grasp the best practices for Evaluating Large Language Models: Methods And Metrics to drive innovation and effectiveness in your projects. Begin your expedition today!
Agent Core
Ever thought about how Large Language Models work? Let’s learn about LLM architecture and discover how these agents set intentions, manage tasks, and interact with users like never before.
Insight into the Agent Core
The agent core lies at the heart of every language model. This core is responsible for a variety of functions that make the agent acutely adaptable and powerful.
Goal Setting: First and foremost, the agent core sets goals. These goals guide the agent’s actions and verdicts, ensuring it remains concentrated on delivering the best possible results for you. Whether you’re seeking responses to precise queries or need support with intricate tasks, the agent core keeps everything on track.
Execution Tools: Next up are the execution tools. Think of these as the agent’s toolbox, filled with numerous abilities that help it execute tasks effectively. From refining natural processing to generating responses, these tools operate together smoothly, providing you with precise and pertinent data.
Dynamic Memory Management: One of the most imposing aspects of the agent core is its dynamic memory management. The agent continually remembers past interactions and uses that data to enhance future answers. It’s like having personal support that gets wise and more helpful with each conversation.
Reasoning and Task Execution
An LLM agent isn’t just about ensuing orders. It can reason and perform tasks with notable precision. When you ask a query, the agent doesn’t simply recover or pre-programmed responses. Instead, it refines your question, comprehends the context, and composes customized responses to your requirements.
These reasoning abilities extend to intricate tasks as well. Whether you’re sustaining a project, systematizing data, or seeking advice, the agent can dissect the circumstances, contemplate numerous factors, and offer well-thought-out solutions.
Optional Persona Customization and Enhancing User-Agent Interaction
Optional Persona Customization
To make interactions even more appealing, you can tailor the agent’s persona. This optional attribute permits you to adjust the agent’s tone, style, and behaviour to suit your choices better. Suppose interact with an agent that not only comprehends your requirements but also matches your communication style finely.
Enhancing User-Agent Interaction
Tailoring improves the overall user-agent interaction, making it more peculiar and delightful. Whether you select a formal, professional tone or a friendly, casual vibe, persona customization ensures the agent feels more like a real conversation partner.
Unleash your potential with 20 LLM Project Ideas For Beginners Using Large Language Models! Let’s learn about Large Language Models (LLMs) and stimulate your expedition with these beginner-friendly projects. Begin exploring now and revolutionize your ideas into reality!
Memory Module
Every virtual support remembers your past order details or interactions, and that is why whenever you order something, you see your past orders and recommendations. This happens because of the memory module. Let’s know in detail:
Short-Term vs. Long-Term Memory in Agents
Imagine the human brain, how it remembers phone numbers for a few seconds but retains precious memories for years. Similarly, in LLM agent architecture, short-term memory handles instant, temporary information, think of it like a sticky note. It’s great for keeping track of your current chat or immediate tasks. On the other hand, long-term memory is the agent’s hard drive, storing data that it has grasped over time. This helps in sustaining context over prolonged interactions and enhancing answers based on past experiences. Knowing this distinction helps you comprehend how agents juggle instant tasks while building a knowledge base for the future.
Memory Techniques for Enhanced Agent Decision-Making
To make smart choices, LLM agents use numerous memory techniques. Recurrent Neural Networks (RNNs), for example, help in refining sequences of information by sustaining a form of short-term memory. Meanwhile, transformer models, which power most modern LLMs, use attention apparatus to concentrate on pertinent parts of the input data selectively. These techniques enable the agent to provide coherent, context-aware responses, making them important. You can think of this as the agent’s way of not just hearing but truly paying attention and comprehending the context of your queries.
Knowledge Integration and Management
An agent’s memory system is an intricate connection of integration and management. It’s like a librarian classifying and shelving books effectively. The agent continuously updates its knowledge base with new data while ensuring that the most pertinent information is easily attainable. Efficient knowledge integration means the agent smoothly integrates new facts into its existing structure, improving its decision-making abilities. Sustaining this memory system effectively is critical because it ensures the agent remains precise, pertinent and useful over time. So, when you interact with an LLM agent, you’re aiding from a well-organized, ever-growing knowledge storage.
Unleash the power of innovative AI technology with our thorough guide: Practical Strategies For Self-Hosting Large Language Models. Learn proven methods and best practices to smoothly incorporate advanced language models into your infrastructure!
Planning Module
Improve your LLM agents' capabilities with a thorough planning module designed to streamline task enforcement and elevate decision-making effectiveness. This crucial component ensures your AI works accurately and flexiblely, optimizing productivity and results.
Effective Strategies for Problem Solving
Efficient planning strategies are important to tackle complicated issues. One key strategy is task decomposition. By breaking down a large task into smaller, more tractable components, you can approach each part with a clear focus, making the whole process more effective. This method helps avert oppression and permits methodical progress.
Another critical strategy is self-reflection. Frequently assessing your approach and progress helps identify any flaws or ineffectiveness. By reflecting on what works and what doesn’t, you can adapt your techniques and constantly enhance your problem-solving skills.
Managing Complex Tasks with Subgoal Identification
Complex tasks can be challenging, but subgoal identification and decomposition make them more tractable. Begin by identifying major milestones you need to accomplish. These milestones become your subgoals. Then, break each subgoal into smaller, applicable steps.
For example, if you’re developing a new software attribute, your subgoals might include researching user requirements, designing the attribute, and testing it. Each of these subgoals can be further decomposed into precise tasks, like creating user surveys or writing code.
This approach not only makes the task less formidable but also gives a clear strategy to follow, ensuring that you stay on track and make congruous progress.
Using Reflection and Critique for Plan Refinement
Once you have a plan in place, it’s critical to assess and process it constantly. This is where reflection and critical techniques come into play. After enforcing a part of your plan, take the time to reflect on its efficiency. Ask yourself queries like: Did this approach work as anticipated? What hurdles did I encounter? How can I enhance this process?
In addition, adopt critic thinking. Be your own formidable critic by objectively analyzing your methods and results. Look for areas of enhancement and be open to making significant alterations.
By frequently reflecting and evaluating your strategies, you can fine-tune your strategy, leading to more effective problem-solving.
Want to know about Large Action Models? Read our pragmatic guide on Understanding Large Action Models In AI.
Tool Usage in LLM Agents
Comprehending the efficient use of tools in Large Language Models (LLMs) can be a groundbreaker. Let’s know in detail about the key tools usage ensuring your LLM agents work savvy, not arduous.
Enhancing Capabilities with Tool Augmentation
At their core, LLM agents are acutely skilled at refining and producing text. However, their real power emerges when you augment them with external tools. Think of tools as special skills or extensions that you could add to your agent, much like apps on your smartphone.
For example, you might incorporate an LLM agent with a data analysis tool. This allows the agent to comprehend your query about sales trends, dissect your sales data, and give applicable perceptions. Another instance is blending an LLM with a scheduling tool, enabling it to set up schedules and sustain your calendar effectively.
By combining natural language comprehension with precise functionalities, LLM agents can execute a broader range of tasks, making them indispensable in both personal and professional settings.
Executable Workflows: The Heart of Functionality
To truly use the potential of LLM agents, you must comprehend executable workflows. These workflows are significantly step-by-step procedures that the agent can follow to finish a task. Tools are essential to these workflows, providing significant functions to accomplish the desired results.
Contemplate a synopsis where you need to produce a financial report. An LLM agent equipped with data recovery, analysis, and report generation tools can automate this process. The productivity might involve retrieving data from numerous sources, dissecting it to identify key trends, and then composing the outcomes into a polished report. Each step in this productivity depends on various tools working smoothly together, orchestrated by the LLM agent’s language processing capabilities.
Beyond Training: Leveraging External Tools
One of the most intriguing aspects of LLM agents is their capability to use external tools for tasks beyond their inaugural training. While an LLM is trained on vast amounts of text, it doesn’t intrinsically know how to execute every possible task. That’s where external tools come into play.
For instance, an LLM agent might not have built-in knowledge about the latest stock market trends. However, by incorporating a real-time financial data API, the agent can attain up-to-date data and offer pertinent advice or perceptions. This ability to tap into external resources extends the agent’s usefulness far beyond its original training data.
Another conclusive use case in customer support is an LLM agent connected to a repository of common troubleshooting steps and solutions. When a customer presents an issue, the agent can quickly search the repository and provide the apt solution, substantially enhancing the effectiveness and precision of customer support.
Empower your AI with custom LLM guardrails by reading our detailed guide on Building And Implementing Custom LLM Guardrails.
Architectures and Types of LLM Agents
Using Large Language Models (LLMs) has become invaluable. But to truly use their potential, comprehending their architectures and types is critical.
Overview of LLM Agent Architectures and Applications
Large Language Models (LLMs) are revolutionizing how we communicate with technology. These models, built on neural network architectures, can refine and produce human-like text. Think of them as your digital assistants, capable of comprehending and answering your questions in a conversational manner.
Architecture of LLM agents differ, but the common thread is their capability to use vast amounts of data to execute tasks like text generation, translation and summarization. The most renowned architectures indulge in transformer models such as GPT (Generative Pre-trained Transformer), which is the backbone of many LLMs today. These models handle sequential data, making them ideal for comprehending context and producing coherent answers.
Applications of LLM agents are enormous. In customer service, they can handle queries and provide solutions effectively. In content creation, they aid in drafting articles, scripts and even poetry. For ventures, they dissect data, generate reports, and automate everyday tasks. Significantly, wherever there’s text, LLMs can step in and improve workflow.
Web Agents vs. Tool Agents: Key Differences and Problem-Solving Capabilities
Now, let's know about the types of LLM agents: Web Agents and Tool Agents.
Web Agents
Web Agents are designed to check the internet, pulling data from numerous sources to provide you with precise and thorough responses. Suppose you’re planning a trip and need information about flights, hotels and local attractions. A web agent can browse the website, collect the latest data, and present it to you systematically. Their ability to incorporate and demonstrate web data makes them indispensable for research, content aggregation, and real-time updates.
Problem-Solving Capabilities of Web Agents:
Data-Retrieval: Quickly collect data from multiple sources.
Research Assistance: Assemble and summarize research materials.
Real-Time Updates: Give the latest news, weather, and stock data.
Tool Agents
On the other hand, Tool Agents are designed to interact with precise software tools or systems to execute tasks. These agents are like your personal software experts. Need help with a spreadsheet or project management tool? A tool can step in, enforce commands, automate recurring tasks, and troubleshoot problems.
Problem-Solving Capabilities of Tool Agents:
Task Automation: Sleek recurring tasks within software tools.
Software Integration: Smoothly interact with numerous applications.
Efficiency Improvement: Optimize productivity and reduce manual effort.
Choosing the Right Agent
When selecting between web agents and tool agents, contemplate your requirements. If you need the latest data from the internet, a web agent is your go-to. If your tasks are more software-centric, a tool agent will be more advantageous. Both types of agents bring distinct strengths to the table, improving your workflow and problem-solving capabilities in various ways.
Comprehending these differences aids you in using LLM agents more efficiently, ensuring you choose the right tool for the job. Whether checking the website or optimizing software productivity, LLM agents are here to assist you every step of the way.
Discover the power of AI by checking our detailed guide on Comparing Different Large Language Models (LLM).
Conclusion
Comprehending the architecture and elements of LLM-based agents discloses the incorporated design and functionality that drive their splendid capabilities. These agents use the power of large language models, incorporating memory systems, planning modules, and external tools to execute intricate tasks effectively. From sustaining short-term and long-term memory to engaging advanced planning strategies and incorporating numerous tools, LLM agents depict a blend of sophisticated methods and intuitive designs. Whether you are a developer, researcher, or enthusiast, comprehending these principles equips you with the knowledge to use the full potential of LLM-based agents in your projects.
Boost your testing workflow with RagaAI’s cutting-edge platform. Relish smooth incorporation, advanced automation, and unmatched effectiveness that will transform your procedures. Don’t miss the chance to improve your productivity. Sign up for a free trial today and experience the distinction!
In the ever-evolving field of Artificial Intelligence, large language models (LLMs) have transformed the way we approach intricate tasks. You might wonder how these potent models can be structured into effective agents capable of performing complex functions. This article will walk you through the intriguing architecture of LLM-based agents, shedding light on their core elements and distinct capabilities.
Ready to take your AI abilities to the next level? Then, read our comprehensive guide on Understanding LLM Alignment: A Simple Guide.
Introduction
Explanation of LLM-Based Agents
Sophisticated AI systems use the comprehensive knowledge and language processing abilities of large language models to perform tasks. These agents can execute various functions, from responding to queries to generating creative content, making them flexible tools in multiple applications.
Defining LLM Agents and Their Unique Architecture
At their core, LLM agents combine the vast computational power of large language models (LLMs) with a specialized architecture that enables them to execute precise tasks. This architecture includes elements like memory modules, planning strategies, and tool incorporations, all designed to the agent’s effectiveness.
Building Agents with Large Language Models for Complex Tasks: Role and Intuition
Building agents with LLMs involves deeply comprehending how these models can elucidate and produce human-like text. This intuition behind this lies in using the model’s capability to refine natural language, enabling the agent to tackle intricate tasks by breaking them down into tractable parts and accurately performing them.
Want to know the distinctions between LLM Pre-Training and Fine-Tuning? Then, you can read our detailed guide on LLM Pre-Training and Fine-Tuning Differences.
Components of LLM Agents
Unleashing the secrets behind LLM agents, you’ll discover an intriguing blend of technology and brain power that powers some of the most advanced conversational systems now:
Key Components of LLM Agents

When knowing about Large Language Model (LLM) agents, you’ll locate numerous key components that tick these systems. These elements operate together to ensure the agents can comprehend, process, and respond to various tasks efficiently. Let’s break down these critical components to get a clear picture of what powers these expanded systems.
Training Data: The cornerstone of any LLM agent is its training data. You require enormous amounts of text data for the model to grasp language patterns, grammar, and context. The quality and assortment of this data substantially affect the agent’s performance. By feeding your model disparate and considerable data, you ensure it can handle various topics and variations in human language.
Architecture: An LLM agent's architecture is comparable to its skeleton. Models such as GPT-4 use a transformer architecture, which is prominent for its effectiveness in comprehending context and relationships in text. The design allows the agent to refine data in corresponding ways, making it faster and more robust. You benefit from this architecture as it enables the agent to produce coherent and contextually pertinent responses.
Tokenization: Tokenization breaks down words and sentences into tractable pieces called tokens. This step is critical because it alters words and sentences into a format the model can comprehend. By tokenizing text, you ensure that the agent can refine and dissect the information precisely, leading to better execution in producing responses.
Training Process: Training an LLM agent encompasses feeding it large datasets and fine-tuning it through numerous repetitions. This process helps the model grasp its errors and enhance its precision. You accomplish this by using techniques such as supervised learning, where the model is accompanied by correct instances, and reinforcement learning, where it is rewarded for producing the right yield.
Inference: Inference is when the trained model produces answers based on new input. When you input a query or a prompt, the model uses its grasped knowledge to give a response. This is where the wizardry happens, and you see the practical application of all training and fine-tuning.
General vs. Specific Prompts in Agent Functionality
Prompts are like the guidelines to give you to the LLM. They can be general or specific, and each type plays a pivotal role. General prompts are extensive and open-ended, permitting the LLM to produce more disparate and creative answers. In contrast, specific prompts give clear, narrow guidelines accompanying the LLM to deliver accurate and pertinent answers. Comprehending when to use each type can substantially improve the functionality and precision of the agent.
Dual Aspects of Memory in Agents: Working and Long-term
Memory is another crucial component. LLM agents use both short-term (working) memory and long-term memory. Short-term memory lets the agent keep track of the current conversation, sustaining context and coherence across interactions. Meanwhile, long-term memory enables the agent to remember past interactions, knowledge and facts, which is critical for offering congruous and informed responses over time.
The Importance of Knowledge in Solving Tasks
Knowledge is power, and for LLM agents, it’s important for comprehending and solving tasks. The more knowledge an agent has, the better it can understand intricate questions and provide precise responses. This knowledge base can indulge facts, concepts, processes, and perceptions, all of which contribute to the agent’s capability to perform a wide range of functions efficiently.
Planning: Essential for Task and Question Decomposition
Planning is a strategy behind the agent’s actions. It involves breaking down tasks and queries into tractable elements, permitting the agent to tackle them step by step. An efficient strategy ensures that the agent can handle intricate questions methodologically, enhancing both effectiveness and precision. By decomposing tasks, the agent can also better use its resources and abilities.
Discover the key to using advanced AI abilities. Grasp the best practices for Evaluating Large Language Models: Methods And Metrics to drive innovation and effectiveness in your projects. Begin your expedition today!
Agent Core
Ever thought about how Large Language Models work? Let’s learn about LLM architecture and discover how these agents set intentions, manage tasks, and interact with users like never before.
Insight into the Agent Core
The agent core lies at the heart of every language model. This core is responsible for a variety of functions that make the agent acutely adaptable and powerful.
Goal Setting: First and foremost, the agent core sets goals. These goals guide the agent’s actions and verdicts, ensuring it remains concentrated on delivering the best possible results for you. Whether you’re seeking responses to precise queries or need support with intricate tasks, the agent core keeps everything on track.
Execution Tools: Next up are the execution tools. Think of these as the agent’s toolbox, filled with numerous abilities that help it execute tasks effectively. From refining natural processing to generating responses, these tools operate together smoothly, providing you with precise and pertinent data.
Dynamic Memory Management: One of the most imposing aspects of the agent core is its dynamic memory management. The agent continually remembers past interactions and uses that data to enhance future answers. It’s like having personal support that gets wise and more helpful with each conversation.
Reasoning and Task Execution
An LLM agent isn’t just about ensuing orders. It can reason and perform tasks with notable precision. When you ask a query, the agent doesn’t simply recover or pre-programmed responses. Instead, it refines your question, comprehends the context, and composes customized responses to your requirements.
These reasoning abilities extend to intricate tasks as well. Whether you’re sustaining a project, systematizing data, or seeking advice, the agent can dissect the circumstances, contemplate numerous factors, and offer well-thought-out solutions.
Optional Persona Customization and Enhancing User-Agent Interaction
Optional Persona Customization
To make interactions even more appealing, you can tailor the agent’s persona. This optional attribute permits you to adjust the agent’s tone, style, and behaviour to suit your choices better. Suppose interact with an agent that not only comprehends your requirements but also matches your communication style finely.
Enhancing User-Agent Interaction
Tailoring improves the overall user-agent interaction, making it more peculiar and delightful. Whether you select a formal, professional tone or a friendly, casual vibe, persona customization ensures the agent feels more like a real conversation partner.
Unleash your potential with 20 LLM Project Ideas For Beginners Using Large Language Models! Let’s learn about Large Language Models (LLMs) and stimulate your expedition with these beginner-friendly projects. Begin exploring now and revolutionize your ideas into reality!
Memory Module
Every virtual support remembers your past order details or interactions, and that is why whenever you order something, you see your past orders and recommendations. This happens because of the memory module. Let’s know in detail:
Short-Term vs. Long-Term Memory in Agents
Imagine the human brain, how it remembers phone numbers for a few seconds but retains precious memories for years. Similarly, in LLM agent architecture, short-term memory handles instant, temporary information, think of it like a sticky note. It’s great for keeping track of your current chat or immediate tasks. On the other hand, long-term memory is the agent’s hard drive, storing data that it has grasped over time. This helps in sustaining context over prolonged interactions and enhancing answers based on past experiences. Knowing this distinction helps you comprehend how agents juggle instant tasks while building a knowledge base for the future.
Memory Techniques for Enhanced Agent Decision-Making
To make smart choices, LLM agents use numerous memory techniques. Recurrent Neural Networks (RNNs), for example, help in refining sequences of information by sustaining a form of short-term memory. Meanwhile, transformer models, which power most modern LLMs, use attention apparatus to concentrate on pertinent parts of the input data selectively. These techniques enable the agent to provide coherent, context-aware responses, making them important. You can think of this as the agent’s way of not just hearing but truly paying attention and comprehending the context of your queries.
Knowledge Integration and Management
An agent’s memory system is an intricate connection of integration and management. It’s like a librarian classifying and shelving books effectively. The agent continuously updates its knowledge base with new data while ensuring that the most pertinent information is easily attainable. Efficient knowledge integration means the agent smoothly integrates new facts into its existing structure, improving its decision-making abilities. Sustaining this memory system effectively is critical because it ensures the agent remains precise, pertinent and useful over time. So, when you interact with an LLM agent, you’re aiding from a well-organized, ever-growing knowledge storage.
Unleash the power of innovative AI technology with our thorough guide: Practical Strategies For Self-Hosting Large Language Models. Learn proven methods and best practices to smoothly incorporate advanced language models into your infrastructure!
Planning Module
Improve your LLM agents' capabilities with a thorough planning module designed to streamline task enforcement and elevate decision-making effectiveness. This crucial component ensures your AI works accurately and flexiblely, optimizing productivity and results.
Effective Strategies for Problem Solving
Efficient planning strategies are important to tackle complicated issues. One key strategy is task decomposition. By breaking down a large task into smaller, more tractable components, you can approach each part with a clear focus, making the whole process more effective. This method helps avert oppression and permits methodical progress.
Another critical strategy is self-reflection. Frequently assessing your approach and progress helps identify any flaws or ineffectiveness. By reflecting on what works and what doesn’t, you can adapt your techniques and constantly enhance your problem-solving skills.
Managing Complex Tasks with Subgoal Identification
Complex tasks can be challenging, but subgoal identification and decomposition make them more tractable. Begin by identifying major milestones you need to accomplish. These milestones become your subgoals. Then, break each subgoal into smaller, applicable steps.
For example, if you’re developing a new software attribute, your subgoals might include researching user requirements, designing the attribute, and testing it. Each of these subgoals can be further decomposed into precise tasks, like creating user surveys or writing code.
This approach not only makes the task less formidable but also gives a clear strategy to follow, ensuring that you stay on track and make congruous progress.
Using Reflection and Critique for Plan Refinement
Once you have a plan in place, it’s critical to assess and process it constantly. This is where reflection and critical techniques come into play. After enforcing a part of your plan, take the time to reflect on its efficiency. Ask yourself queries like: Did this approach work as anticipated? What hurdles did I encounter? How can I enhance this process?
In addition, adopt critic thinking. Be your own formidable critic by objectively analyzing your methods and results. Look for areas of enhancement and be open to making significant alterations.
By frequently reflecting and evaluating your strategies, you can fine-tune your strategy, leading to more effective problem-solving.
Want to know about Large Action Models? Read our pragmatic guide on Understanding Large Action Models In AI.
Tool Usage in LLM Agents
Comprehending the efficient use of tools in Large Language Models (LLMs) can be a groundbreaker. Let’s know in detail about the key tools usage ensuring your LLM agents work savvy, not arduous.
Enhancing Capabilities with Tool Augmentation
At their core, LLM agents are acutely skilled at refining and producing text. However, their real power emerges when you augment them with external tools. Think of tools as special skills or extensions that you could add to your agent, much like apps on your smartphone.
For example, you might incorporate an LLM agent with a data analysis tool. This allows the agent to comprehend your query about sales trends, dissect your sales data, and give applicable perceptions. Another instance is blending an LLM with a scheduling tool, enabling it to set up schedules and sustain your calendar effectively.
By combining natural language comprehension with precise functionalities, LLM agents can execute a broader range of tasks, making them indispensable in both personal and professional settings.
Executable Workflows: The Heart of Functionality
To truly use the potential of LLM agents, you must comprehend executable workflows. These workflows are significantly step-by-step procedures that the agent can follow to finish a task. Tools are essential to these workflows, providing significant functions to accomplish the desired results.
Contemplate a synopsis where you need to produce a financial report. An LLM agent equipped with data recovery, analysis, and report generation tools can automate this process. The productivity might involve retrieving data from numerous sources, dissecting it to identify key trends, and then composing the outcomes into a polished report. Each step in this productivity depends on various tools working smoothly together, orchestrated by the LLM agent’s language processing capabilities.
Beyond Training: Leveraging External Tools
One of the most intriguing aspects of LLM agents is their capability to use external tools for tasks beyond their inaugural training. While an LLM is trained on vast amounts of text, it doesn’t intrinsically know how to execute every possible task. That’s where external tools come into play.
For instance, an LLM agent might not have built-in knowledge about the latest stock market trends. However, by incorporating a real-time financial data API, the agent can attain up-to-date data and offer pertinent advice or perceptions. This ability to tap into external resources extends the agent’s usefulness far beyond its original training data.
Another conclusive use case in customer support is an LLM agent connected to a repository of common troubleshooting steps and solutions. When a customer presents an issue, the agent can quickly search the repository and provide the apt solution, substantially enhancing the effectiveness and precision of customer support.
Empower your AI with custom LLM guardrails by reading our detailed guide on Building And Implementing Custom LLM Guardrails.
Architectures and Types of LLM Agents
Using Large Language Models (LLMs) has become invaluable. But to truly use their potential, comprehending their architectures and types is critical.
Overview of LLM Agent Architectures and Applications
Large Language Models (LLMs) are revolutionizing how we communicate with technology. These models, built on neural network architectures, can refine and produce human-like text. Think of them as your digital assistants, capable of comprehending and answering your questions in a conversational manner.
Architecture of LLM agents differ, but the common thread is their capability to use vast amounts of data to execute tasks like text generation, translation and summarization. The most renowned architectures indulge in transformer models such as GPT (Generative Pre-trained Transformer), which is the backbone of many LLMs today. These models handle sequential data, making them ideal for comprehending context and producing coherent answers.
Applications of LLM agents are enormous. In customer service, they can handle queries and provide solutions effectively. In content creation, they aid in drafting articles, scripts and even poetry. For ventures, they dissect data, generate reports, and automate everyday tasks. Significantly, wherever there’s text, LLMs can step in and improve workflow.
Web Agents vs. Tool Agents: Key Differences and Problem-Solving Capabilities
Now, let's know about the types of LLM agents: Web Agents and Tool Agents.
Web Agents
Web Agents are designed to check the internet, pulling data from numerous sources to provide you with precise and thorough responses. Suppose you’re planning a trip and need information about flights, hotels and local attractions. A web agent can browse the website, collect the latest data, and present it to you systematically. Their ability to incorporate and demonstrate web data makes them indispensable for research, content aggregation, and real-time updates.
Problem-Solving Capabilities of Web Agents:
Data-Retrieval: Quickly collect data from multiple sources.
Research Assistance: Assemble and summarize research materials.
Real-Time Updates: Give the latest news, weather, and stock data.
Tool Agents
On the other hand, Tool Agents are designed to interact with precise software tools or systems to execute tasks. These agents are like your personal software experts. Need help with a spreadsheet or project management tool? A tool can step in, enforce commands, automate recurring tasks, and troubleshoot problems.
Problem-Solving Capabilities of Tool Agents:
Task Automation: Sleek recurring tasks within software tools.
Software Integration: Smoothly interact with numerous applications.
Efficiency Improvement: Optimize productivity and reduce manual effort.
Choosing the Right Agent
When selecting between web agents and tool agents, contemplate your requirements. If you need the latest data from the internet, a web agent is your go-to. If your tasks are more software-centric, a tool agent will be more advantageous. Both types of agents bring distinct strengths to the table, improving your workflow and problem-solving capabilities in various ways.
Comprehending these differences aids you in using LLM agents more efficiently, ensuring you choose the right tool for the job. Whether checking the website or optimizing software productivity, LLM agents are here to assist you every step of the way.
Discover the power of AI by checking our detailed guide on Comparing Different Large Language Models (LLM).
Conclusion
Comprehending the architecture and elements of LLM-based agents discloses the incorporated design and functionality that drive their splendid capabilities. These agents use the power of large language models, incorporating memory systems, planning modules, and external tools to execute intricate tasks effectively. From sustaining short-term and long-term memory to engaging advanced planning strategies and incorporating numerous tools, LLM agents depict a blend of sophisticated methods and intuitive designs. Whether you are a developer, researcher, or enthusiast, comprehending these principles equips you with the knowledge to use the full potential of LLM-based agents in your projects.
Boost your testing workflow with RagaAI’s cutting-edge platform. Relish smooth incorporation, advanced automation, and unmatched effectiveness that will transform your procedures. Don’t miss the chance to improve your productivity. Sign up for a free trial today and experience the distinction!
Subscribe to our newsletter to never miss an update
Other articles


Agents Talk, Models Think: A2A + MCP for Enterprise Agentic AI

Riya Parikh, Nitai Agarwal
Apr 27, 2025
Read the article
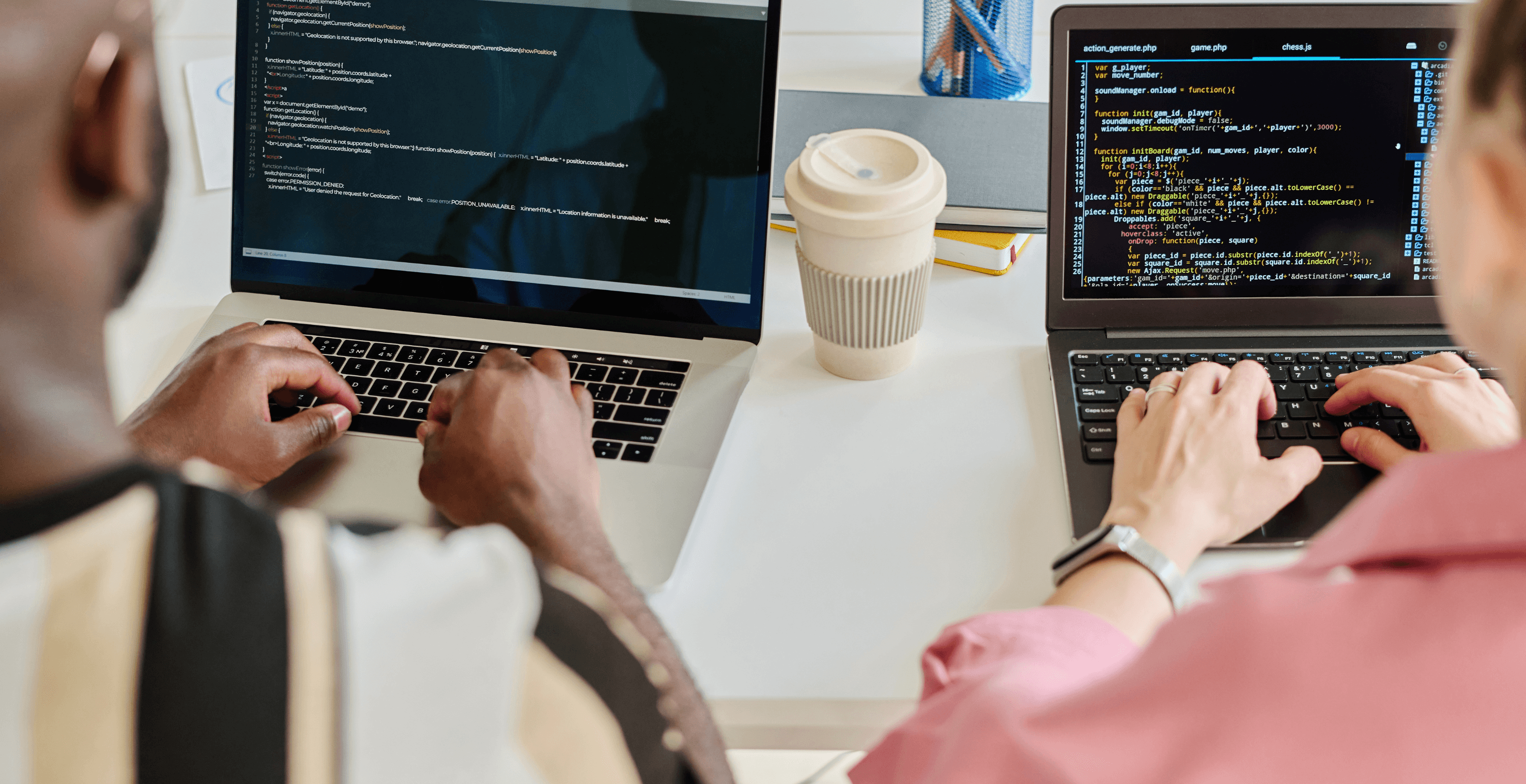
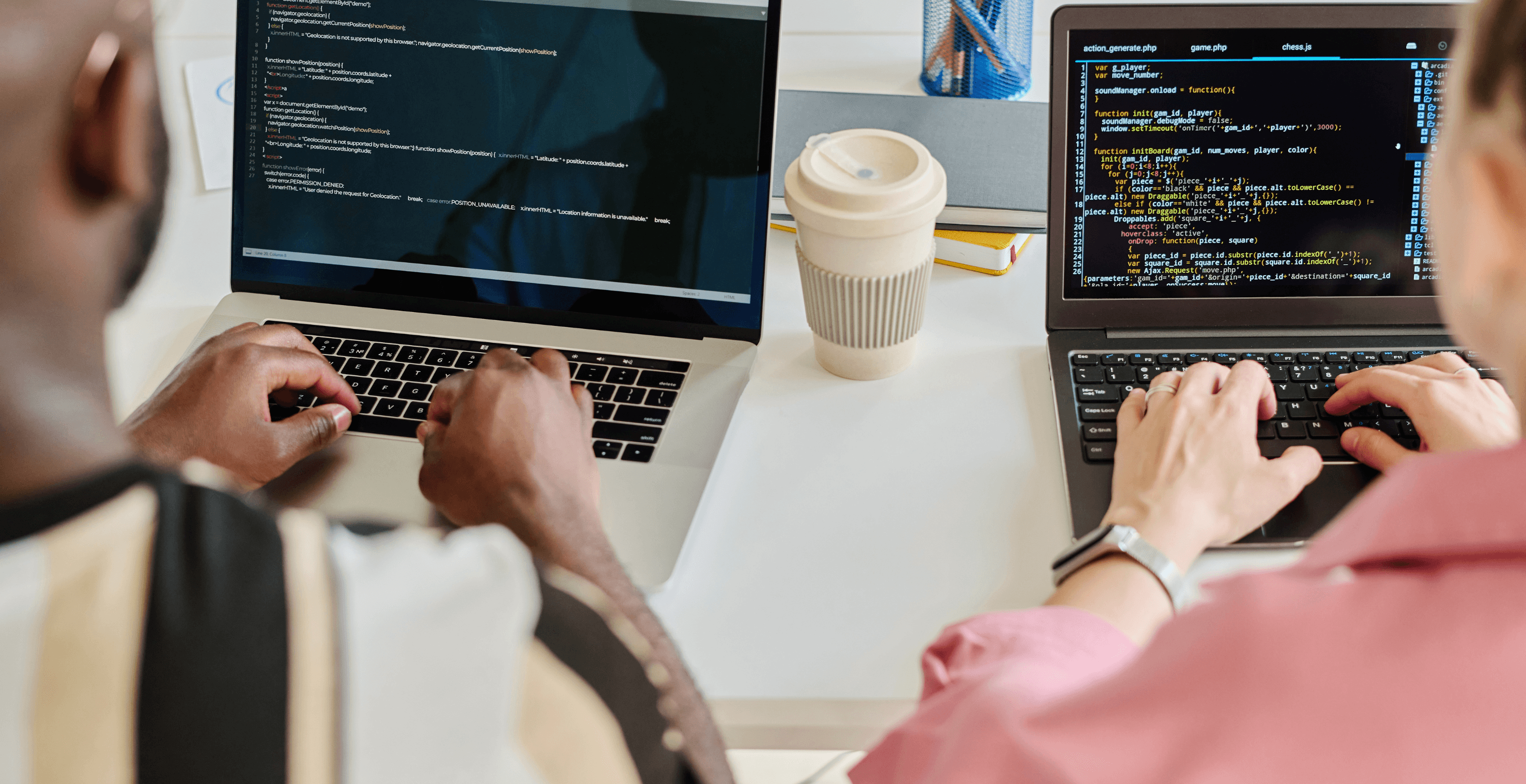
Understanding NeMo Guardrails: A Toolkit for LLM Security

Rehan Asif
Dec 24, 2024
Read the article


Understanding Differences in Large vs Small Language Models (LLM vs SLM)

Rehan Asif
Dec 21, 2024
Read the article