Introduction to LLM Powered Autonomous Agents
Introduction to LLM Powered Autonomous Agents
Introduction to LLM Powered Autonomous Agents
Rehan Asif
Jul 1, 2024




Imagine having an AI that doesn't just talk but takes action, solves problems, and improves on its own. When you dive into the world of artificial intelligence, you'll quickly encounter one of the most groundbreaking advancements: LLM-powered autonomous agents.
These agents are more than just sophisticated chatbots; they are action-takers, problem-solvers, and efficiency enhancers. By blending the language prowess of Large Language Models (LLMs) with the capability to detect, diagnose, and fix issues, these agents transform how we interact with AI.
Gone are the days of static LLMs that only generate text. Now, we have dynamic agents that actively improve their environments, setting the stage for smarter, faster, and more reliable AI systems. Let's understand the concept of LLM powered autonomous Agents.
LLM Powered Autonomous Agents: Overview
LLM-Powered Autonomous Agents are advanced AI systems that combine the language capabilities of Large Language Models (LLMs) with functionalities like reasoning, planning, tool use, and memory. When you hear about LLM-powered autonomous agents, think of them as supercharged versions of the AI models you already know.
These agents do more than understand and generate text. By integrating capabilities such as reasoning, specific knowledge, planning, tool use, and memory, they move from passive responders to active participants in various processes. To maintain their effectiveness, it's essential to understand how to detect and fix AI issues.
They take meaningful actions based on their understanding, allowing them to solve complex problems, streamline tasks, and improve outcomes.
Transition from LLMs to Autonomous Agents
The shift from standalone Large Language Models (LLMs) to autonomous agents marks a game-changing moment in AI development. Initially, LLMs like OpenAI’s GPT-4 or Google’s Gemini were impressive for their ability to generate human-like text.
These models could provide insightful responses and assist with a variety of language tasks.
However, their capabilities were limited to text generation, and they lacked the ability to take action or make decisions based on their outputs. So, a more dynamic approach was needed, leading to the development of LLM-powered autonomous agents.
These agents not only generate text but can also perform other tasks.
Because of this, autonomous agents are becoming invaluable in various fields, from customer service and marketing to scientific research and beyond. They significantly increase productivity and reduce the need for human intervention. A comprehensive LLM evaluation framework can help measure and enhance the performance of these autonomous agents.
Traditional Large Language Models (LLMs) vs Autonomous Agents
Traditional LLMs are sophisticated text generators. They excel at understanding and producing language but fall short when it comes to taking action or making decisions. In contrast, LLM-powered autonomous agents integrate several advanced functionalities:
Reasoning: They can think through problems and come up with solutions.
Specific Knowledge: They utilize domain-specific information to enhance their responses.
Planning: They break down tasks into manageable steps and follow through.
Tool Use: They can access and use external tools and databases via APIs.
Memory: They have both short-term and long-term memory, allowing them to learn from past interactions and store information for future use.
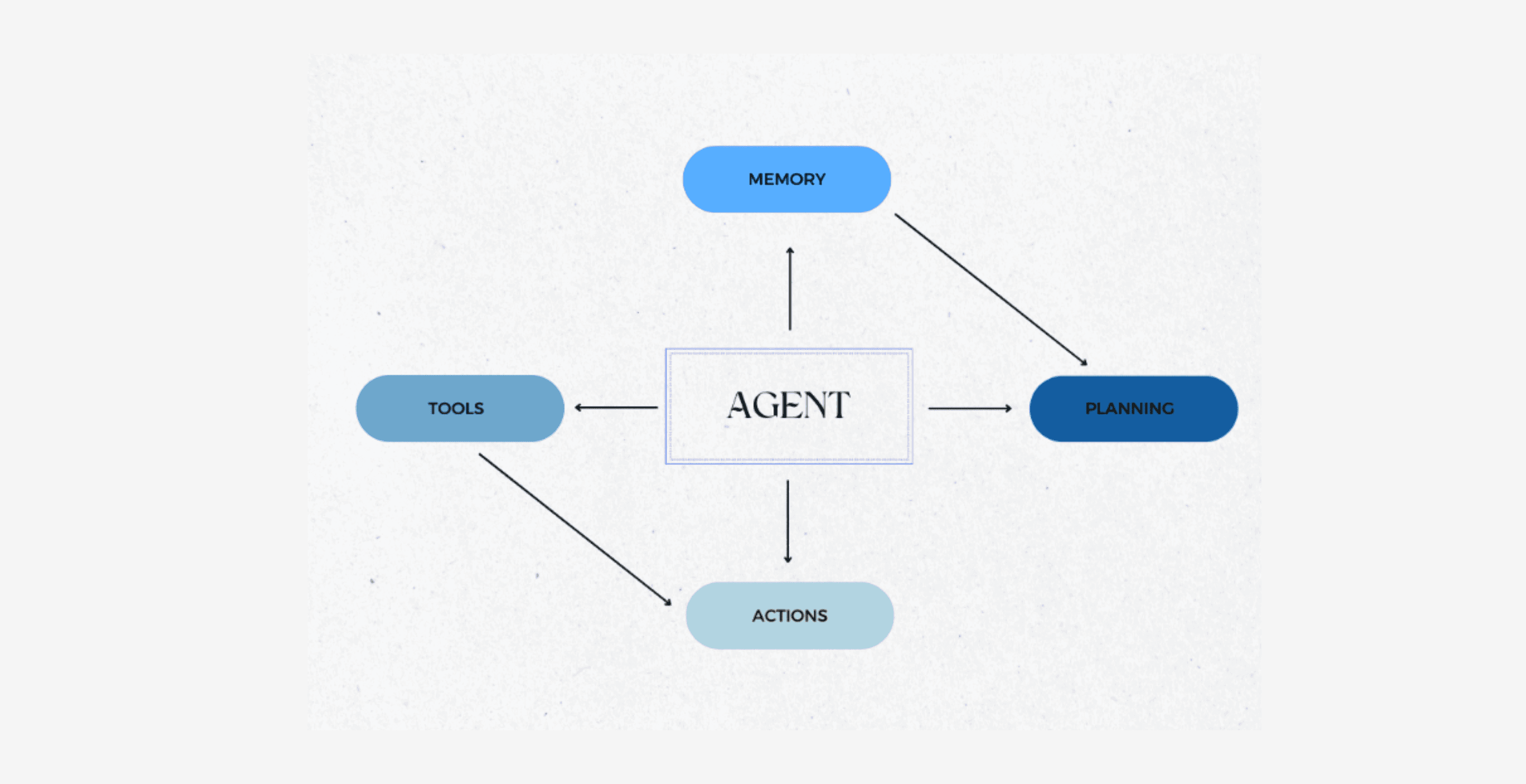
These enhancements allow agents to perform complex tasks that traditional LLMs cannot handle.
LLMs Beyond Simple Command-Response Mechanisms
The true power of LLM-powered autonomous agents lies in their expanded capabilities. Traditional LLMs operate on a simple command-response basis—you ask a question, and they provide an answer.
As previously explained, autonomous agents go far beyond this. They can interpret your instructions, create a plan of action, and execute that plan, all while using their built-in reasoning and memory capabilities to adapt and improve.
This means you can rely on them to handle more intricate tasks, such as managing workflows, optimizing processes, and even making data-driven decisions. For further insights on maintaining ethical standards, it is essential to understand the details of how correct AI governance is performed.
LLM Powered Autonomous Agents: Core Components
As explained above, LLM-powered autonomous agents are sophisticated advancements in artificial intelligence that combine the capabilities of large language models (LLMs) with additional functionalities like reasoning, planning, memory, and tool use.
They can not only generate and understand text but also execute tasks, make decisions, and interact dynamically with their environments. Evaluating and monitoring the performance of these agents is crucial for ensuring reliability, as outlined in the guide to evaluating LLM applications and enabling guardrails.
Let's understand the core components of the LLM-powered autonomous agents. They are as follows:
Planning
Planning is a fundamental component of LLM-powered autonomous agents. You can understand the overview of the Planning component from the bullet points provided below:
Task Decomposition: Agents break down complex tasks into smaller, manageable subgoals. This step-by-step approach simplifies the execution of intricate projects. It ensures that each segment of the task is handled effectively.
Self-Reflection: Advanced agents analyze their past actions. They learn from mistakes to refine their strategies. This continuous improvement mechanism enhances the quality of outcomes, increasing their ability to tackle increasingly complex tasks.
Memory
Memory is crucial for the effective functioning of LLM-powered autonomous agents. It is typically divided into short-term and long-term memory:
Short-Term Memory: This type of memory allows agents to retain and manipulate information relevant to the immediate task at hand, similar to how humans use short-term memory to recall recent events or information.
Long-Term Memory: This enables agents to store vast amounts of information over extended periods, often through external storage systems. It acts like a library of knowledge that the agent can access when necessary, supporting more informed decision-making and task execution.
The combination of these memory types allows agents to adapt to new information, learn from past experiences, and maintain a robust knowledge base.
Tools Usage:
Tool use is the added capability embedded in the LLM-powered autonomous agents that gives them the ability to augment capabilities. Tools usage can be summarized into two bullet points:
Extended Functionality: LLM-powered autonomous agents interact with external tools via APIs, accessing real-time data, executing code, and utilizing specialized databases.
Versatility: This ability to use tools transforms LLM-powered autonomous agents from mere language processors into versatile problem solvers, adapting to various scenarios and requirements.
Action
The decision-making process in LLM-powered autonomous agents is pivotal to their operation. When an agent decides to act, it can be either internal or external:
Internal Actions: These are confined to the agent's thought processes, such as generating and refining responses.
External Actions: These involve tangible outputs, like sending emails, managing data, or interacting with other systems.
The ability to choose and execute the appropriate type of action based on the context and objectives is a key feature that sets autonomous agents apart from traditional LLMs. This dual nature of actions allows them to be effective in a wide array of applications, from customer service to complex data analysis.
The overview of the components of an LLM-powered autonomous agent is shown below for your reference.

Pic: Overview of an LLM-powered autonomous agent with its system (Image source: Weng, Lilian. 2023)
Agent Component One: Planning
Planning plays a pivotal role in the operation of an LLM-powered autonomous agent. It's not just about understanding the steps involved in a complex task but also about strategizing for efficient execution. This is crucial for the development of effective strategies in LLM-powered autonomous agents.
By breaking down complex tasks into manageable steps, agents can approach intricate projects systematically. Let's understand the techniques involved in the "task decomposition" part of the Planning component used by LLM-powered autonomous agents.
Task Decomposition
Chain of thought (CoT; Wei et al., 2022) involves guiding the agent to think step-by-step, breaking down a task into a sequence of smaller, logical steps. It enhances the agent's performance by making its thought process transparent and methodical.
This technique has become a standard prompting technique for enhancing model performance on complex tasks. CoT transforms big tasks into multiple manageable tasks and sheds light on an interpretation of the model's thinking process.
Tree of Thoughts (Yao et al., 2023) is a further refinement of CoT and extends it by exploring multiple reasoning paths at each step, creating a tree structure. This technique explores multiple reasoning possibilities at each step. Under this, the task/problem is first decomposed into multiple thought steps, out of which multiple thoughts per step are generated, which creates a tree structure.
Each branch under the Tree of Thoughts represents a different potential solution, allowing the agent to evaluate various approaches and choose the most effective one. The search procedure offers both breadth-first search (BFS) and depth-first search (DFS) options. A classifier (via a prompt) or majority vote can assess each state.
Task decomposition under the Planning component can happen through the following methods:
(1) by LLM using straightforward questions such as "What are the subgoals for achieving XYZ?" and "Steps for XYZ. 1."
Using task-specific instructions, e.g. "Write a blog outline." for writing a specific topic
Using human inputs
Continuous Improvement Through Self-Reflection
LLM-powered autonomous agents continuously improve through the process of self-reflection, which plays a crucial role. Two key frameworks that facilitate self-reflection are ReAct and Reflexion:
ReAct Framework
Reflexion Framework
Let us understand both of these frameworks in detail.
ReAct Framework
ReAct Framework (Yao et al. 2023) Integrates reasoning and action, enabling agents to generate reasoning traces and perform task-specific actions. Reasoning enables the agent to interact with the environment (e.g., use Wikipedia search API). Acting prompts the powered agent to generate reasoning traces in natural language. This combination helps refine the agents' responses and actions based on real-time feedback.
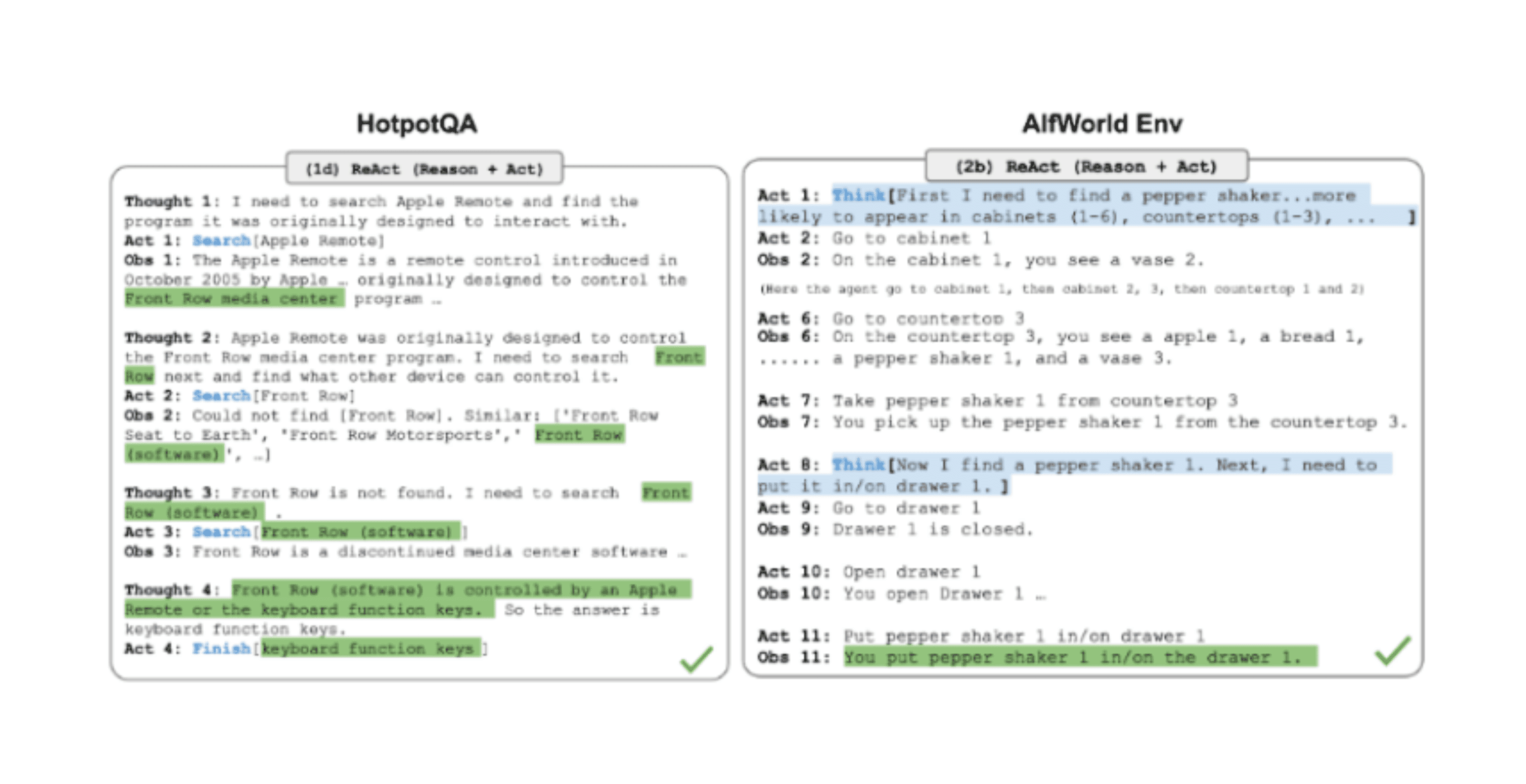
Pic: Examples of reasoning trajectories for knowledge-intensive tasks (e.g. HotpotQA, FEVER) and decision-making tasks (e.g. AlfWorld Env, WebShop). (Image source: Yao et al. 2023).
Reflexion Framework
Reflexion (Shinn & Labash, 2023) equips agents with dynamic memory and self-reflection capabilities, which improve their reasoning skills. This framework equips agents with dynamic memory and self-reflection capabilities. By analyzing past actions and outcomes, agents can identify errors and inefficiencies, allowing them to adjust their strategies and improve future performance.
Reflexion uses a basic reinforcement learning architecture, where the task-specific action space is enhanced with language to facilitate sophisticated reasoning stages. At the same time, the reward model offers a straightforward binary payout. Following every action, the agent calculates a heuristic and may choose to reset the environment to begin a new trial based on the outcomes of its self-reflection.
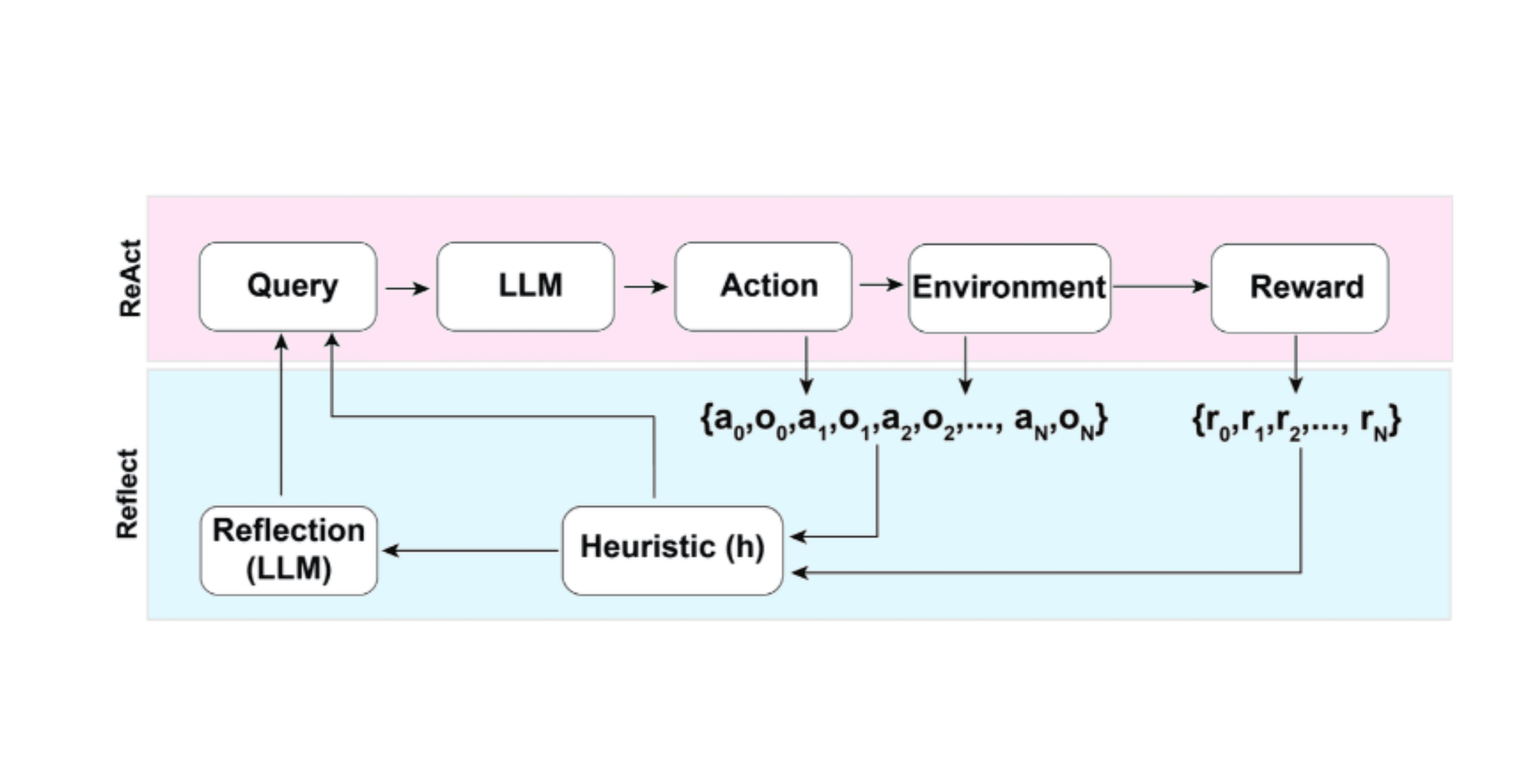
Pic: Illustration of the Reflexion framework. (Image source: Shinn & Labash, 2023)
Enhanced Long-Horizon Planning: Integrating Classical Planners with LLMs
For more complex, long-horizon tasks, integrating classical planners with LLMs can enhance planning capabilities for complex, long-horizon tasks. This approach involves translating the problem into PDDL, using an external classical planner to generate a detailed plan, and then translating the plan back into natural language for the agent to execute.
Translation to PDDL: The LLM translates a given problem into a PDDL format, defining the planning problem in a structured way.
Classical Planning: An external classical planner generates a detailed plan based on the PDDL description, utilizing domain-specific knowledge and algorithms.
Translation Back to Natural Language: The LLM translates the generated plan back into natural language, making it actionable for the agent.
Agent Component Two: Memory
Memory management is a cornerstone of LLM-powered autonomous agents, enabling them to store and recall information crucial for their operations. Memory in LLM-powered autonomous agents serves the same purpose as it does in a human brain.
Let's understand the types of memory in both the context of Human Memory and LLM-powered autonomous agents.
Classification of Memory: Human Brain
The procedures required to gather, store, hold onto, and then retrieve information are collectively referred to as memory. Human brains have multiple types of memory.
Human memory is broadly understood under the classification of:
Sensory Memory: The ability to hold onto impressions of sensory information (visual, aural, etc.) after the initial stimulation has finished is known as sensory memory, which is the first stage of memory. Usually, sensory memory is limited to a few seconds. There are three subcategories: haptic memory (touch), auditory echoic memory, and iconic memory (visual).
Short-Term Memory (STM) or Working Memory: It retains knowledge that we now possess and is required to perform sophisticated mental operations like learning and reasoning. According to Miller (1956), short-term memory may hold seven items and last 20 to 30 seconds.
Long-Term Memory (LTM): Information can be stored in long-term memory for a very long period—from a few days to decades—and it has practically infinite storage space. LTM comes in two subtypes:
Explicit/declarative memory: This memory refers to the consciously recalled recollections of facts and events, such as episodic memory (experiences and events) and semantic memory (concepts and facts).
Implicit/procedural memory: This kind of memory is unconscious and pertains to automatic skills and routines, such as riding a bike or using a keyboard.
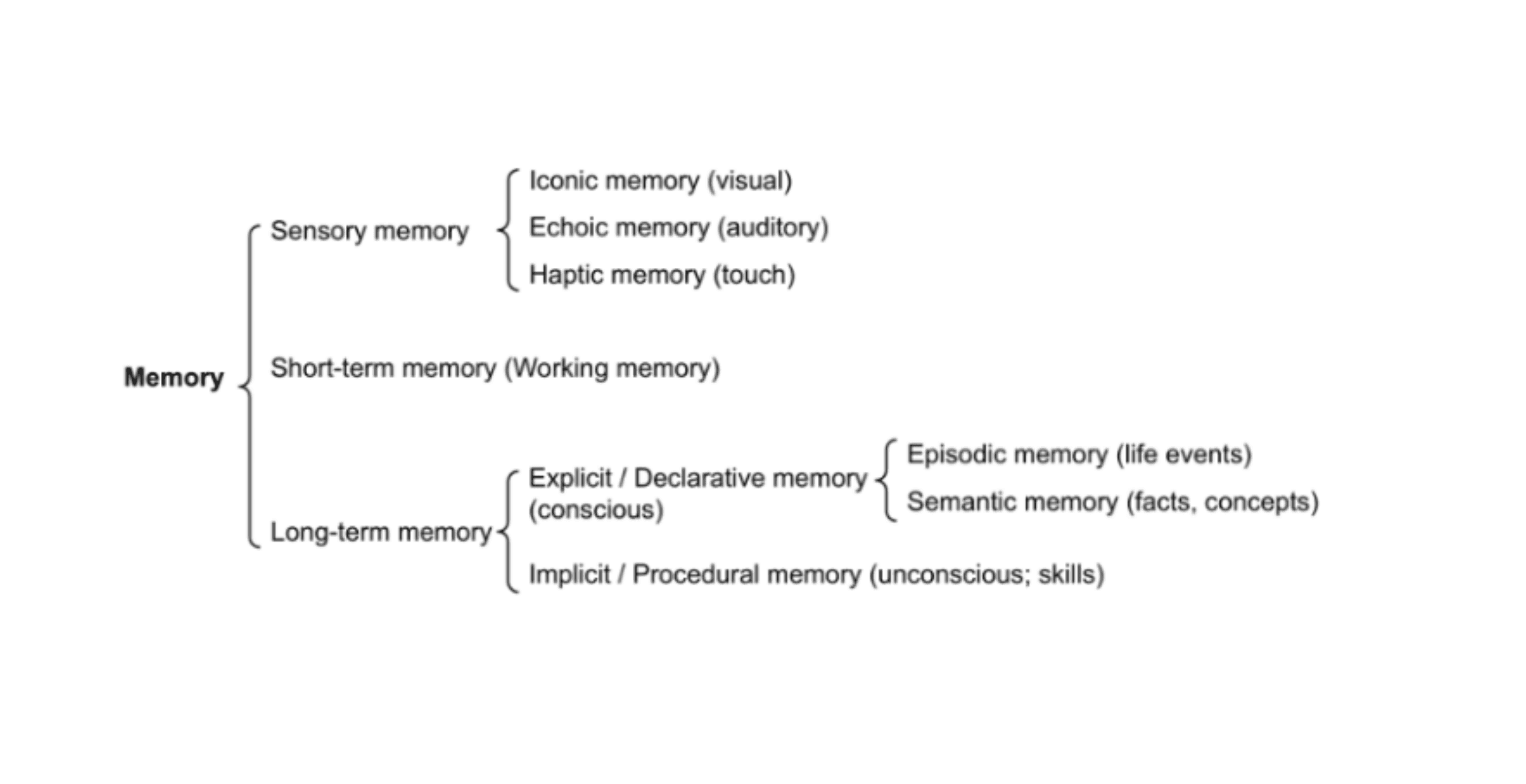
Pic: Classification and Categorization of Human Memory
Classification of Memory: LLM-Powered Autonomous Agents
Now that you have understood the classification of memory as per the human brain, it becomes easier to understand how memory is classified in the context of LLM-Powered Autonomous Agents.
The classifications are as follows:
Short-Term Memory (STM): This type of memory allows agents to hold and manipulate information relevant to the immediate task at hand. It is similar to how humans remember recent events or data points for short periods. STM is crucial for in-context learning, where the agent uses recent interactions to inform its current actions and responses
Long-term Memory (LTM): LTM enables agents to store vast amounts of information over extended periods. External storage systems often facilitate this memory, acting as repositories of knowledge that the agent can access as needed. LTM is essential for retaining information that might be required for future tasks or for drawing upon past experiences to improve performance.
Practical Implementation of Memory For Agents
In practical applications, implementing memory in LLM-powered autonomous agents often involves the use of external vector stores.
These stores are specialized databases that efficiently manage and retrieve large volumes of data. Here are a few examples:
Customer Support Systems: Agents can use short-term memory to handle ongoing customer interactions effectively, while long-term memory stores customer history, preferences, and past interactions, allowing the agent to provide personalized support.
Research and Development: In scientific research, agents can store and recall extensive datasets, research papers, and experimental results. Short-term memory helps in handling current research tasks, while long-term memory retains valuable historical data for future reference.
Educational Tools: Educational agents can use short-term memory to adapt to a student's immediate learning needs and questions, while long-term memory stores the student's progress, strengths, and weaknesses over time, enabling tailored educational experiences.
Importance of Memory in LLM-Powered Autonomous Agents
Memory plays a pivotal role in the effectiveness and adaptability of LLM-powered autonomous agents. Here's why it is crucial:
In-Context Learning: Short-term memory enables agents to utilize recent information to make informed decisions and generate accurate responses. This capability is vital for maintaining continuity and relevance in interactions, especially in dynamic and multi-step tasks.
Information Retention: Long-term memory allows agents to store knowledge and experiences over extended periods. This retention is essential for building a comprehensive understanding of various domains, improving problem-solving skills, and enhancing overall performance. By retaining information, agents can draw upon past experiences to navigate new challenges more effectively.
Component Three: Tool Utilization
LLM-powered autonomous agents extend their capabilities by interacting with external APIs, which allows them to access real-time data, execute code, and retrieve information from specialized databases.
This interaction significantly enhances their functionality beyond their pre-trained knowledge. By leveraging APIs, these agents can:
Access up-to-date information that is not part of their initial training data.
Perform specific tasks that require external computational resources or data.
Integrate specialized functions and tools, thereby increasing their versatility and applicability in various domains.
Examples of MRKL Architecture and Specific Projects like TAL and Toolformer
To better understand how LLM agents utilize external tools, let's look at some specific architectures and projects:
MRKL Architecture (Karpas et al. 2022): MRKL stands for Modular Reasoning, Knowledge, and Language. It is a neuro-symbolic architecture designed to enhance the reasoning capabilities of LLMs by integrating various expert modules. In MRKL systems, the LLM acts as a router, directing inquiries to the appropriate expert module, whether it be a neural network, symbolic system, or other specialized tools. This modular approach allows the agent to perform complex reasoning tasks more effectively.
TAL (Tool Augmented Language Models) (Parisi et al., 2022): TAL involves fine-tuning LLMs to learn how to call external APIs. The model is trained to recognize when and how to use these APIs to improve the quality of its outputs. By incorporating API calls into its responses, TAL can provide more accurate and contextually relevant information.
Toolformer (Schick et al., 2023): Similar to TAL, Toolformer is a project where LLMs are fine-tuned to use external tools automatically. The training process includes adding API call annotations to the dataset, allowing the model to learn when and how to make these calls to enhance its performance. Toolformer showcases the practical implementation of tool use in LLM agents, demonstrating significant improvements in task execution.
Significance of External Tools in Enhancing Problem-Solving Capabilities
The use of external tools is crucial in elevating the problem-solving abilities of LLM-powered autonomous agents. Here is why:
Enhanced Data Access: External tools allow agents to access a broader range of data sources, including real-time information and proprietary databases, which are essential for making informed decisions.
Specialized Functionality: By integrating with tools designed for specific tasks, such as mathematical computation, data analysis, or language translation, agents can perform these tasks more accurately and efficiently.
Adaptability: The ability to use external tools makes agents more adaptable to different scenarios and requirements. They can switch between various tools depending on the task at hand, providing a more versatile and robust solution.
Incorporating external tools transforms LLM agents from mere language processors into comprehensive problem solvers. This capability enables them to tackle a wide array of tasks, from simple information retrieval to complex data analysis, making them invaluable assets in both professional and personal applications.
LLM Powered Autonomous Agents: Capabilities and Applications
The difference between LLM-powered autonomous agents and traditional large language models (LLMs) lies in the range and complexity of tasks they can perform autonomously.
Traditional LLMs are primarily designed to generate text and respond to queries based on pre-trained data. They excel at understanding language and producing coherent responses but lack the ability to take meaningful actions or make decisions beyond text generation.
In contrast, LLM-powered autonomous agents integrate advanced functionalities such as reasoning, planning, memory, and tool use, enabling them to execute tasks independently. They can:
Plan and execute multi-step tasks: Decompose complex projects into manageable subgoals and follow through with action plans.
Interact with external systems: Use APIs to access real-time data, execute commands, and integrate specialized tools.
Learn and adapt: Utilize short-term and long-term memory to retain information and improve performance over time.
Make informed decisions: Apply reasoning capabilities to choose the best course of action based on context and available data.
This expanded spectrum of tasks makes autonomous agents far more versatile and capable than traditional LLMs.
Practical Applications of Agents
LLM-powered autonomous agents are revolutionizing various industries by enhancing efficiency and effectiveness in numerous applications:
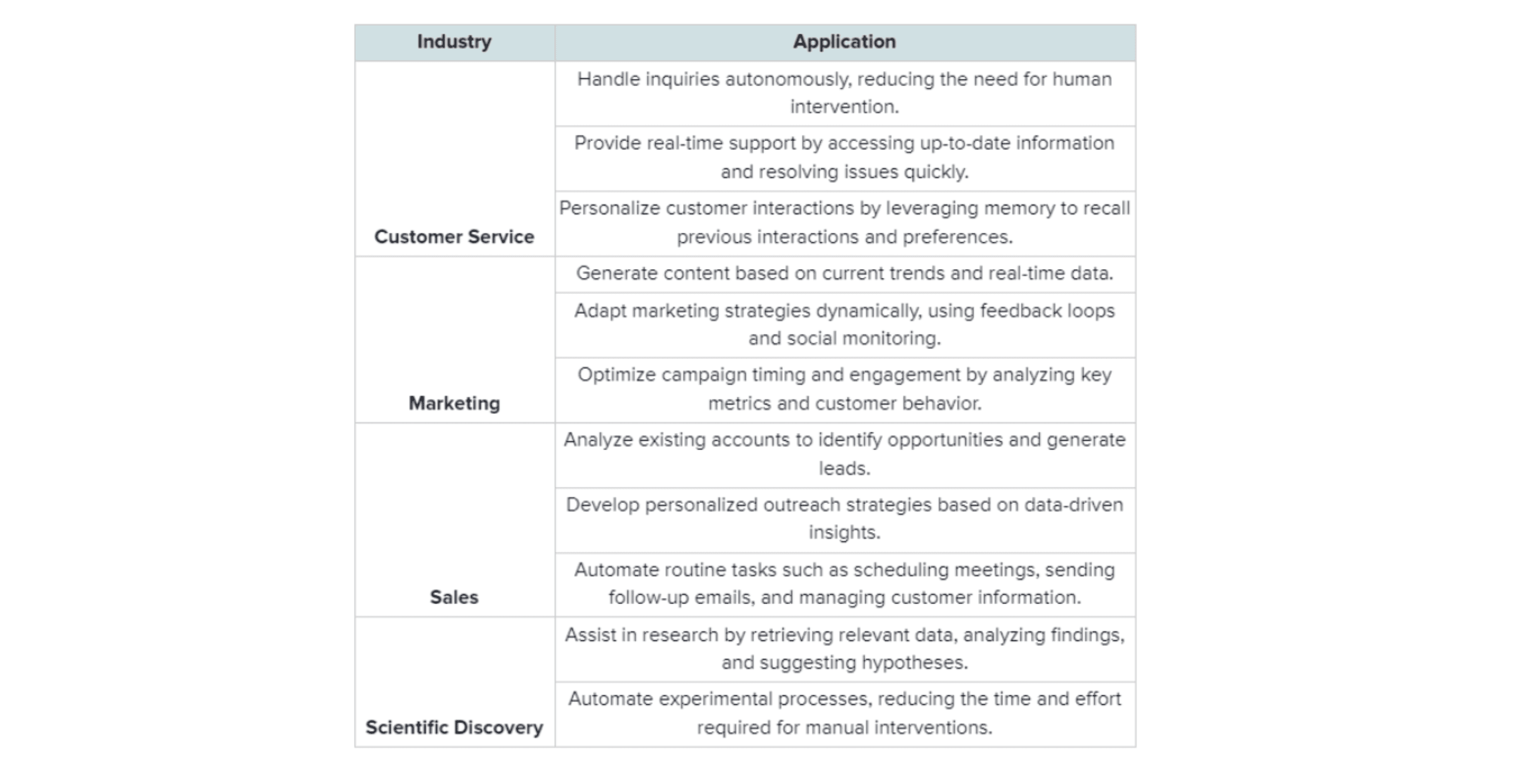
Pic: Industry Specific Applications for LLM Powered Autonoumous Agents
Autonomy in Task Execution Leading from Agents
The autonomy of LLM-powered agents in task execution is driving significant advancements in productivity and automation. By independently handling complex and routine tasks, these agents free up human resources to focus on higher-level strategic activities. Key benefits include:
Increased Efficiency: Autonomous agents can operate continuously without fatigue, ensuring tasks are completed faster and more accurately.
Reduced Costs: Automating routine and repetitive tasks reduces the need for extensive human labor, leading to cost savings.
Scalability: Agents can handle large volumes of tasks simultaneously, making it easier to scale operations without proportional increases in human resources.
Innovation: With routine tasks automated, humans can focus on creative and innovative aspects of their work, driving further advancements and improvements.
In summary, the capabilities and applications of LLM-powered autonomous agents are transforming industries by enhancing efficiency, reducing costs, and enabling more innovative and productive work environments.
LLM-Powered Autonomous Agents: Challenges and Future Directions
While LLM-powered autonomous agents represent a significant advancement in AI, they come with their own set of challenges:
Finite Context Length: One of the primary limitations is the finite context length of LLMs. This restricts the amount of historical information and detailed instructions that the agent can process at any given time, which can impact its ability to handle long and complex tasks effectively.
Long-Term Planning Difficulties: Despite improvements, long-term planning remains a challenge. Agents can struggle to maintain coherence and effectiveness over extended tasks, particularly those requiring intricate, multi-step processes.
Reliability of Natural Language Interfaces: The natural language interface, while powerful, is not infallible. Agents may occasionally produce incorrect or ambiguous outputs, make formatting errors, or misinterpret complex instructions. This can lead to issues in task execution and reduce the reliability of the agents.
LLM Powered Autonomous Agents: Future
The future development of LLM-powered autonomous agents is likely to focus on enhancing their autonomy and expanding their capabilities:
Extended Context Lengths: Improvements in AI architecture could allow agents to process more information, enhancing their ability to handle long-term tasks.
Improved Long-Term Planning: Advanced planning algorithms and learning techniques will help agents manage complex tasks more effectively.
Enhanced Natural Language Understanding: Better natural language processing will make agents more reliable in interpreting and executing complex instructions.
Advanced Tool Integration: Agents will become better at utilizing a wider range of external tools, increasing their versatility.
Evolving Landscape of AI and Potential Future Impact
The landscape of AI applications and impacts will continue to grow:
Broader Adoption: LLM-powered agents will be adopted across more industries, automating and enhancing complex tasks.
Human-AI Collaboration: Agents will take on more routine and complex tasks, allowing humans to focus on strategic and creative endeavors.
Ethical and Regulatory Considerations: There will be a greater emphasis on ensuring transparency, fairness, and compliance with global standards.
Innovation and Advancements: Ongoing AI research will lead to the development of more sophisticated agents, improving cognitive functions and decision-making.
Conclusion
In conclusion, LLM-powered autonomous agents represent a transformative leap in AI technology. They combine language processing with advanced capabilities like planning, memory, and tool use. These agents can handle complex tasks, make informed decisions, and interact dynamically with their environments, offering a versatile solution for various industries.
RAGA AI leverages these advancements to ensure the quality and consistency of AI applications through its comprehensive testing platform.
By detecting, diagnosing, and fixing AI issues autonomously, RAGA AI accelerates the development lifecycle and reduces risk. Sign up for our comprehensive RagaAI Testing Platform today.
Imagine having an AI that doesn't just talk but takes action, solves problems, and improves on its own. When you dive into the world of artificial intelligence, you'll quickly encounter one of the most groundbreaking advancements: LLM-powered autonomous agents.
These agents are more than just sophisticated chatbots; they are action-takers, problem-solvers, and efficiency enhancers. By blending the language prowess of Large Language Models (LLMs) with the capability to detect, diagnose, and fix issues, these agents transform how we interact with AI.
Gone are the days of static LLMs that only generate text. Now, we have dynamic agents that actively improve their environments, setting the stage for smarter, faster, and more reliable AI systems. Let's understand the concept of LLM powered autonomous Agents.
LLM Powered Autonomous Agents: Overview
LLM-Powered Autonomous Agents are advanced AI systems that combine the language capabilities of Large Language Models (LLMs) with functionalities like reasoning, planning, tool use, and memory. When you hear about LLM-powered autonomous agents, think of them as supercharged versions of the AI models you already know.
These agents do more than understand and generate text. By integrating capabilities such as reasoning, specific knowledge, planning, tool use, and memory, they move from passive responders to active participants in various processes. To maintain their effectiveness, it's essential to understand how to detect and fix AI issues.
They take meaningful actions based on their understanding, allowing them to solve complex problems, streamline tasks, and improve outcomes.
Transition from LLMs to Autonomous Agents
The shift from standalone Large Language Models (LLMs) to autonomous agents marks a game-changing moment in AI development. Initially, LLMs like OpenAI’s GPT-4 or Google’s Gemini were impressive for their ability to generate human-like text.
These models could provide insightful responses and assist with a variety of language tasks.
However, their capabilities were limited to text generation, and they lacked the ability to take action or make decisions based on their outputs. So, a more dynamic approach was needed, leading to the development of LLM-powered autonomous agents.
These agents not only generate text but can also perform other tasks.
Because of this, autonomous agents are becoming invaluable in various fields, from customer service and marketing to scientific research and beyond. They significantly increase productivity and reduce the need for human intervention. A comprehensive LLM evaluation framework can help measure and enhance the performance of these autonomous agents.
Traditional Large Language Models (LLMs) vs Autonomous Agents
Traditional LLMs are sophisticated text generators. They excel at understanding and producing language but fall short when it comes to taking action or making decisions. In contrast, LLM-powered autonomous agents integrate several advanced functionalities:
Reasoning: They can think through problems and come up with solutions.
Specific Knowledge: They utilize domain-specific information to enhance their responses.
Planning: They break down tasks into manageable steps and follow through.
Tool Use: They can access and use external tools and databases via APIs.
Memory: They have both short-term and long-term memory, allowing them to learn from past interactions and store information for future use.
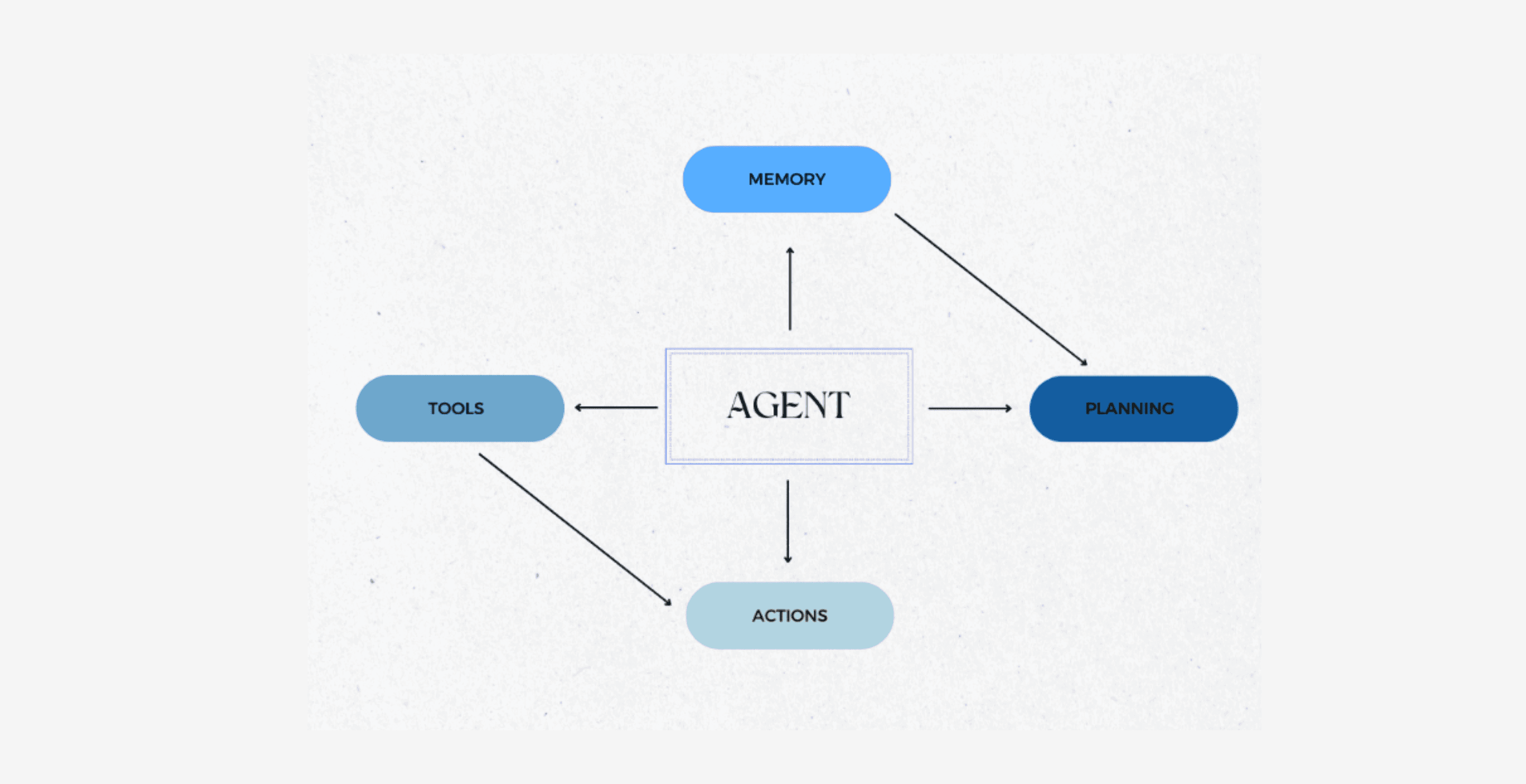
These enhancements allow agents to perform complex tasks that traditional LLMs cannot handle.
LLMs Beyond Simple Command-Response Mechanisms
The true power of LLM-powered autonomous agents lies in their expanded capabilities. Traditional LLMs operate on a simple command-response basis—you ask a question, and they provide an answer.
As previously explained, autonomous agents go far beyond this. They can interpret your instructions, create a plan of action, and execute that plan, all while using their built-in reasoning and memory capabilities to adapt and improve.
This means you can rely on them to handle more intricate tasks, such as managing workflows, optimizing processes, and even making data-driven decisions. For further insights on maintaining ethical standards, it is essential to understand the details of how correct AI governance is performed.
LLM Powered Autonomous Agents: Core Components
As explained above, LLM-powered autonomous agents are sophisticated advancements in artificial intelligence that combine the capabilities of large language models (LLMs) with additional functionalities like reasoning, planning, memory, and tool use.
They can not only generate and understand text but also execute tasks, make decisions, and interact dynamically with their environments. Evaluating and monitoring the performance of these agents is crucial for ensuring reliability, as outlined in the guide to evaluating LLM applications and enabling guardrails.
Let's understand the core components of the LLM-powered autonomous agents. They are as follows:
Planning
Planning is a fundamental component of LLM-powered autonomous agents. You can understand the overview of the Planning component from the bullet points provided below:
Task Decomposition: Agents break down complex tasks into smaller, manageable subgoals. This step-by-step approach simplifies the execution of intricate projects. It ensures that each segment of the task is handled effectively.
Self-Reflection: Advanced agents analyze their past actions. They learn from mistakes to refine their strategies. This continuous improvement mechanism enhances the quality of outcomes, increasing their ability to tackle increasingly complex tasks.
Memory
Memory is crucial for the effective functioning of LLM-powered autonomous agents. It is typically divided into short-term and long-term memory:
Short-Term Memory: This type of memory allows agents to retain and manipulate information relevant to the immediate task at hand, similar to how humans use short-term memory to recall recent events or information.
Long-Term Memory: This enables agents to store vast amounts of information over extended periods, often through external storage systems. It acts like a library of knowledge that the agent can access when necessary, supporting more informed decision-making and task execution.
The combination of these memory types allows agents to adapt to new information, learn from past experiences, and maintain a robust knowledge base.
Tools Usage:
Tool use is the added capability embedded in the LLM-powered autonomous agents that gives them the ability to augment capabilities. Tools usage can be summarized into two bullet points:
Extended Functionality: LLM-powered autonomous agents interact with external tools via APIs, accessing real-time data, executing code, and utilizing specialized databases.
Versatility: This ability to use tools transforms LLM-powered autonomous agents from mere language processors into versatile problem solvers, adapting to various scenarios and requirements.
Action
The decision-making process in LLM-powered autonomous agents is pivotal to their operation. When an agent decides to act, it can be either internal or external:
Internal Actions: These are confined to the agent's thought processes, such as generating and refining responses.
External Actions: These involve tangible outputs, like sending emails, managing data, or interacting with other systems.
The ability to choose and execute the appropriate type of action based on the context and objectives is a key feature that sets autonomous agents apart from traditional LLMs. This dual nature of actions allows them to be effective in a wide array of applications, from customer service to complex data analysis.
The overview of the components of an LLM-powered autonomous agent is shown below for your reference.

Pic: Overview of an LLM-powered autonomous agent with its system (Image source: Weng, Lilian. 2023)
Agent Component One: Planning
Planning plays a pivotal role in the operation of an LLM-powered autonomous agent. It's not just about understanding the steps involved in a complex task but also about strategizing for efficient execution. This is crucial for the development of effective strategies in LLM-powered autonomous agents.
By breaking down complex tasks into manageable steps, agents can approach intricate projects systematically. Let's understand the techniques involved in the "task decomposition" part of the Planning component used by LLM-powered autonomous agents.
Task Decomposition
Chain of thought (CoT; Wei et al., 2022) involves guiding the agent to think step-by-step, breaking down a task into a sequence of smaller, logical steps. It enhances the agent's performance by making its thought process transparent and methodical.
This technique has become a standard prompting technique for enhancing model performance on complex tasks. CoT transforms big tasks into multiple manageable tasks and sheds light on an interpretation of the model's thinking process.
Tree of Thoughts (Yao et al., 2023) is a further refinement of CoT and extends it by exploring multiple reasoning paths at each step, creating a tree structure. This technique explores multiple reasoning possibilities at each step. Under this, the task/problem is first decomposed into multiple thought steps, out of which multiple thoughts per step are generated, which creates a tree structure.
Each branch under the Tree of Thoughts represents a different potential solution, allowing the agent to evaluate various approaches and choose the most effective one. The search procedure offers both breadth-first search (BFS) and depth-first search (DFS) options. A classifier (via a prompt) or majority vote can assess each state.
Task decomposition under the Planning component can happen through the following methods:
(1) by LLM using straightforward questions such as "What are the subgoals for achieving XYZ?" and "Steps for XYZ. 1."
Using task-specific instructions, e.g. "Write a blog outline." for writing a specific topic
Using human inputs
Continuous Improvement Through Self-Reflection
LLM-powered autonomous agents continuously improve through the process of self-reflection, which plays a crucial role. Two key frameworks that facilitate self-reflection are ReAct and Reflexion:
ReAct Framework
Reflexion Framework
Let us understand both of these frameworks in detail.
ReAct Framework
ReAct Framework (Yao et al. 2023) Integrates reasoning and action, enabling agents to generate reasoning traces and perform task-specific actions. Reasoning enables the agent to interact with the environment (e.g., use Wikipedia search API). Acting prompts the powered agent to generate reasoning traces in natural language. This combination helps refine the agents' responses and actions based on real-time feedback.
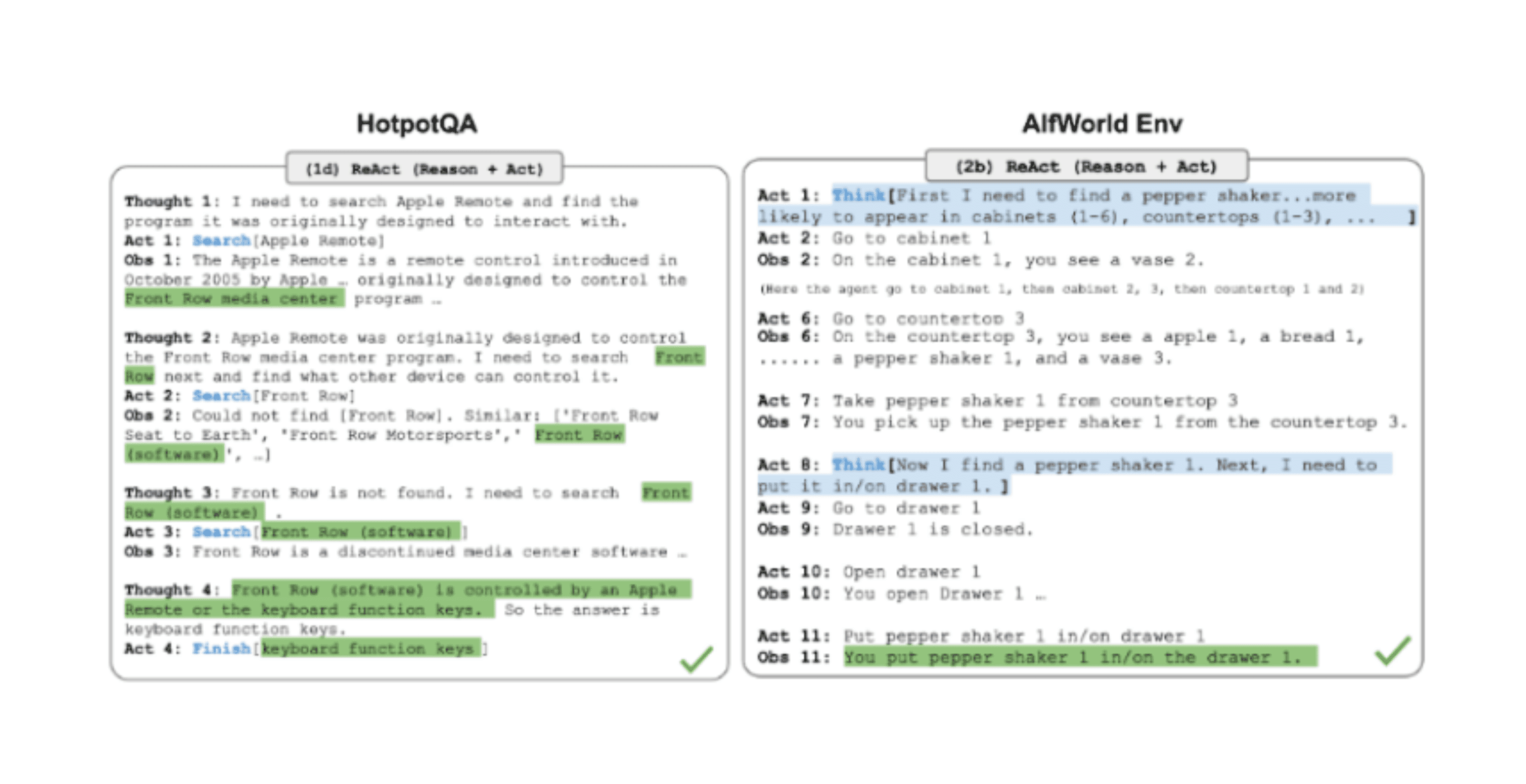
Pic: Examples of reasoning trajectories for knowledge-intensive tasks (e.g. HotpotQA, FEVER) and decision-making tasks (e.g. AlfWorld Env, WebShop). (Image source: Yao et al. 2023).
Reflexion Framework
Reflexion (Shinn & Labash, 2023) equips agents with dynamic memory and self-reflection capabilities, which improve their reasoning skills. This framework equips agents with dynamic memory and self-reflection capabilities. By analyzing past actions and outcomes, agents can identify errors and inefficiencies, allowing them to adjust their strategies and improve future performance.
Reflexion uses a basic reinforcement learning architecture, where the task-specific action space is enhanced with language to facilitate sophisticated reasoning stages. At the same time, the reward model offers a straightforward binary payout. Following every action, the agent calculates a heuristic and may choose to reset the environment to begin a new trial based on the outcomes of its self-reflection.
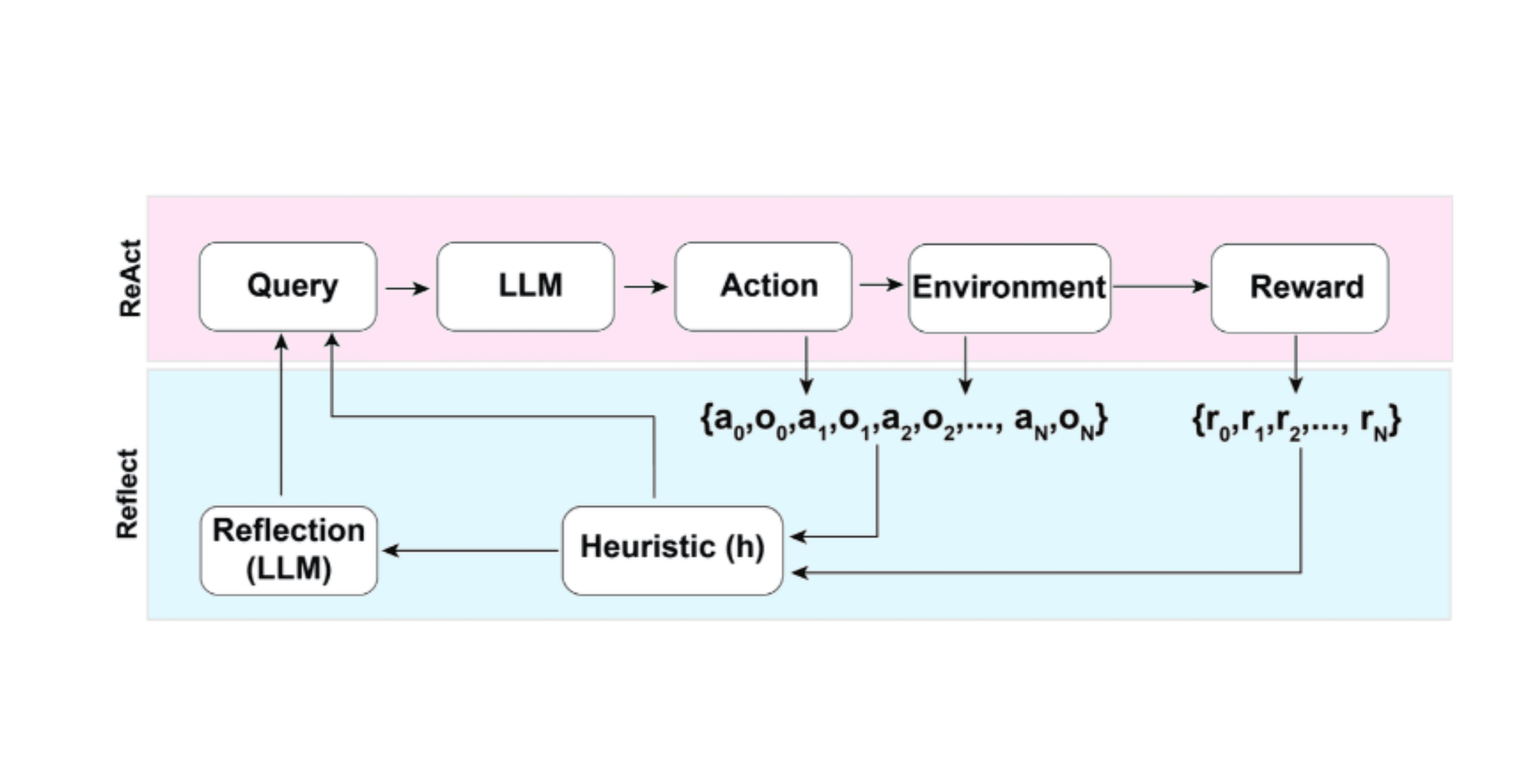
Pic: Illustration of the Reflexion framework. (Image source: Shinn & Labash, 2023)
Enhanced Long-Horizon Planning: Integrating Classical Planners with LLMs
For more complex, long-horizon tasks, integrating classical planners with LLMs can enhance planning capabilities for complex, long-horizon tasks. This approach involves translating the problem into PDDL, using an external classical planner to generate a detailed plan, and then translating the plan back into natural language for the agent to execute.
Translation to PDDL: The LLM translates a given problem into a PDDL format, defining the planning problem in a structured way.
Classical Planning: An external classical planner generates a detailed plan based on the PDDL description, utilizing domain-specific knowledge and algorithms.
Translation Back to Natural Language: The LLM translates the generated plan back into natural language, making it actionable for the agent.
Agent Component Two: Memory
Memory management is a cornerstone of LLM-powered autonomous agents, enabling them to store and recall information crucial for their operations. Memory in LLM-powered autonomous agents serves the same purpose as it does in a human brain.
Let's understand the types of memory in both the context of Human Memory and LLM-powered autonomous agents.
Classification of Memory: Human Brain
The procedures required to gather, store, hold onto, and then retrieve information are collectively referred to as memory. Human brains have multiple types of memory.
Human memory is broadly understood under the classification of:
Sensory Memory: The ability to hold onto impressions of sensory information (visual, aural, etc.) after the initial stimulation has finished is known as sensory memory, which is the first stage of memory. Usually, sensory memory is limited to a few seconds. There are three subcategories: haptic memory (touch), auditory echoic memory, and iconic memory (visual).
Short-Term Memory (STM) or Working Memory: It retains knowledge that we now possess and is required to perform sophisticated mental operations like learning and reasoning. According to Miller (1956), short-term memory may hold seven items and last 20 to 30 seconds.
Long-Term Memory (LTM): Information can be stored in long-term memory for a very long period—from a few days to decades—and it has practically infinite storage space. LTM comes in two subtypes:
Explicit/declarative memory: This memory refers to the consciously recalled recollections of facts and events, such as episodic memory (experiences and events) and semantic memory (concepts and facts).
Implicit/procedural memory: This kind of memory is unconscious and pertains to automatic skills and routines, such as riding a bike or using a keyboard.
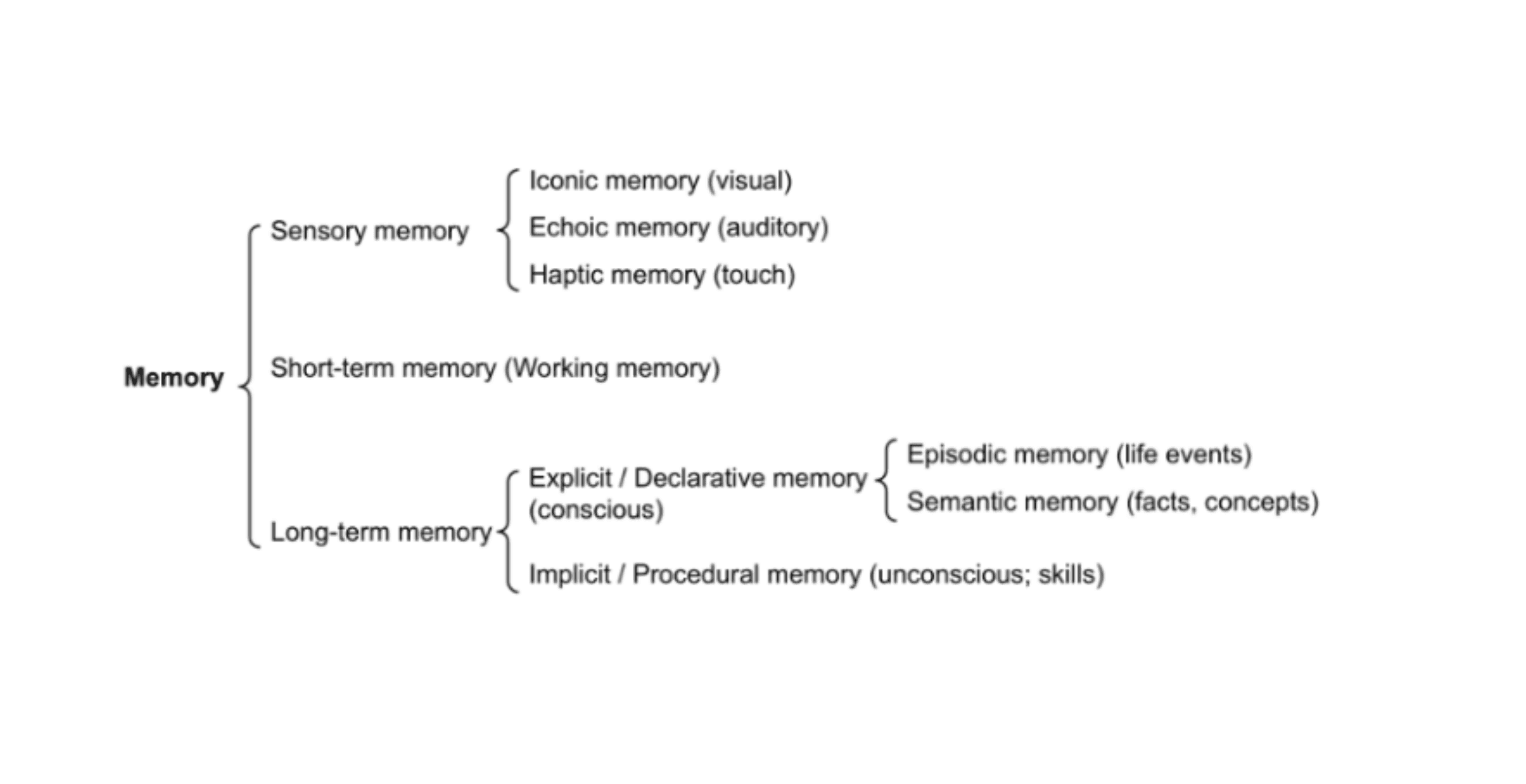
Pic: Classification and Categorization of Human Memory
Classification of Memory: LLM-Powered Autonomous Agents
Now that you have understood the classification of memory as per the human brain, it becomes easier to understand how memory is classified in the context of LLM-Powered Autonomous Agents.
The classifications are as follows:
Short-Term Memory (STM): This type of memory allows agents to hold and manipulate information relevant to the immediate task at hand. It is similar to how humans remember recent events or data points for short periods. STM is crucial for in-context learning, where the agent uses recent interactions to inform its current actions and responses
Long-term Memory (LTM): LTM enables agents to store vast amounts of information over extended periods. External storage systems often facilitate this memory, acting as repositories of knowledge that the agent can access as needed. LTM is essential for retaining information that might be required for future tasks or for drawing upon past experiences to improve performance.
Practical Implementation of Memory For Agents
In practical applications, implementing memory in LLM-powered autonomous agents often involves the use of external vector stores.
These stores are specialized databases that efficiently manage and retrieve large volumes of data. Here are a few examples:
Customer Support Systems: Agents can use short-term memory to handle ongoing customer interactions effectively, while long-term memory stores customer history, preferences, and past interactions, allowing the agent to provide personalized support.
Research and Development: In scientific research, agents can store and recall extensive datasets, research papers, and experimental results. Short-term memory helps in handling current research tasks, while long-term memory retains valuable historical data for future reference.
Educational Tools: Educational agents can use short-term memory to adapt to a student's immediate learning needs and questions, while long-term memory stores the student's progress, strengths, and weaknesses over time, enabling tailored educational experiences.
Importance of Memory in LLM-Powered Autonomous Agents
Memory plays a pivotal role in the effectiveness and adaptability of LLM-powered autonomous agents. Here's why it is crucial:
In-Context Learning: Short-term memory enables agents to utilize recent information to make informed decisions and generate accurate responses. This capability is vital for maintaining continuity and relevance in interactions, especially in dynamic and multi-step tasks.
Information Retention: Long-term memory allows agents to store knowledge and experiences over extended periods. This retention is essential for building a comprehensive understanding of various domains, improving problem-solving skills, and enhancing overall performance. By retaining information, agents can draw upon past experiences to navigate new challenges more effectively.
Component Three: Tool Utilization
LLM-powered autonomous agents extend their capabilities by interacting with external APIs, which allows them to access real-time data, execute code, and retrieve information from specialized databases.
This interaction significantly enhances their functionality beyond their pre-trained knowledge. By leveraging APIs, these agents can:
Access up-to-date information that is not part of their initial training data.
Perform specific tasks that require external computational resources or data.
Integrate specialized functions and tools, thereby increasing their versatility and applicability in various domains.
Examples of MRKL Architecture and Specific Projects like TAL and Toolformer
To better understand how LLM agents utilize external tools, let's look at some specific architectures and projects:
MRKL Architecture (Karpas et al. 2022): MRKL stands for Modular Reasoning, Knowledge, and Language. It is a neuro-symbolic architecture designed to enhance the reasoning capabilities of LLMs by integrating various expert modules. In MRKL systems, the LLM acts as a router, directing inquiries to the appropriate expert module, whether it be a neural network, symbolic system, or other specialized tools. This modular approach allows the agent to perform complex reasoning tasks more effectively.
TAL (Tool Augmented Language Models) (Parisi et al., 2022): TAL involves fine-tuning LLMs to learn how to call external APIs. The model is trained to recognize when and how to use these APIs to improve the quality of its outputs. By incorporating API calls into its responses, TAL can provide more accurate and contextually relevant information.
Toolformer (Schick et al., 2023): Similar to TAL, Toolformer is a project where LLMs are fine-tuned to use external tools automatically. The training process includes adding API call annotations to the dataset, allowing the model to learn when and how to make these calls to enhance its performance. Toolformer showcases the practical implementation of tool use in LLM agents, demonstrating significant improvements in task execution.
Significance of External Tools in Enhancing Problem-Solving Capabilities
The use of external tools is crucial in elevating the problem-solving abilities of LLM-powered autonomous agents. Here is why:
Enhanced Data Access: External tools allow agents to access a broader range of data sources, including real-time information and proprietary databases, which are essential for making informed decisions.
Specialized Functionality: By integrating with tools designed for specific tasks, such as mathematical computation, data analysis, or language translation, agents can perform these tasks more accurately and efficiently.
Adaptability: The ability to use external tools makes agents more adaptable to different scenarios and requirements. They can switch between various tools depending on the task at hand, providing a more versatile and robust solution.
Incorporating external tools transforms LLM agents from mere language processors into comprehensive problem solvers. This capability enables them to tackle a wide array of tasks, from simple information retrieval to complex data analysis, making them invaluable assets in both professional and personal applications.
LLM Powered Autonomous Agents: Capabilities and Applications
The difference between LLM-powered autonomous agents and traditional large language models (LLMs) lies in the range and complexity of tasks they can perform autonomously.
Traditional LLMs are primarily designed to generate text and respond to queries based on pre-trained data. They excel at understanding language and producing coherent responses but lack the ability to take meaningful actions or make decisions beyond text generation.
In contrast, LLM-powered autonomous agents integrate advanced functionalities such as reasoning, planning, memory, and tool use, enabling them to execute tasks independently. They can:
Plan and execute multi-step tasks: Decompose complex projects into manageable subgoals and follow through with action plans.
Interact with external systems: Use APIs to access real-time data, execute commands, and integrate specialized tools.
Learn and adapt: Utilize short-term and long-term memory to retain information and improve performance over time.
Make informed decisions: Apply reasoning capabilities to choose the best course of action based on context and available data.
This expanded spectrum of tasks makes autonomous agents far more versatile and capable than traditional LLMs.
Practical Applications of Agents
LLM-powered autonomous agents are revolutionizing various industries by enhancing efficiency and effectiveness in numerous applications:
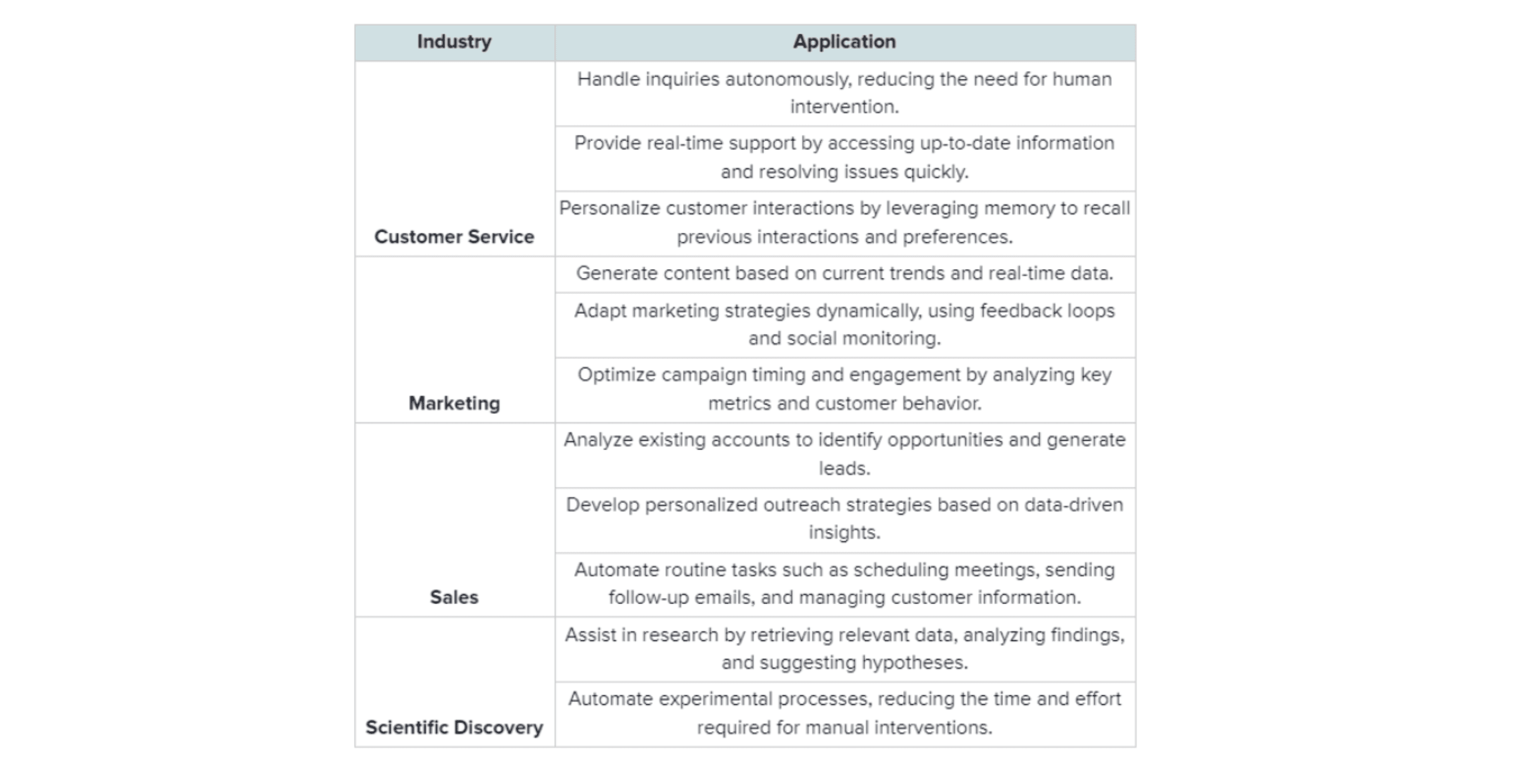
Pic: Industry Specific Applications for LLM Powered Autonoumous Agents
Autonomy in Task Execution Leading from Agents
The autonomy of LLM-powered agents in task execution is driving significant advancements in productivity and automation. By independently handling complex and routine tasks, these agents free up human resources to focus on higher-level strategic activities. Key benefits include:
Increased Efficiency: Autonomous agents can operate continuously without fatigue, ensuring tasks are completed faster and more accurately.
Reduced Costs: Automating routine and repetitive tasks reduces the need for extensive human labor, leading to cost savings.
Scalability: Agents can handle large volumes of tasks simultaneously, making it easier to scale operations without proportional increases in human resources.
Innovation: With routine tasks automated, humans can focus on creative and innovative aspects of their work, driving further advancements and improvements.
In summary, the capabilities and applications of LLM-powered autonomous agents are transforming industries by enhancing efficiency, reducing costs, and enabling more innovative and productive work environments.
LLM-Powered Autonomous Agents: Challenges and Future Directions
While LLM-powered autonomous agents represent a significant advancement in AI, they come with their own set of challenges:
Finite Context Length: One of the primary limitations is the finite context length of LLMs. This restricts the amount of historical information and detailed instructions that the agent can process at any given time, which can impact its ability to handle long and complex tasks effectively.
Long-Term Planning Difficulties: Despite improvements, long-term planning remains a challenge. Agents can struggle to maintain coherence and effectiveness over extended tasks, particularly those requiring intricate, multi-step processes.
Reliability of Natural Language Interfaces: The natural language interface, while powerful, is not infallible. Agents may occasionally produce incorrect or ambiguous outputs, make formatting errors, or misinterpret complex instructions. This can lead to issues in task execution and reduce the reliability of the agents.
LLM Powered Autonomous Agents: Future
The future development of LLM-powered autonomous agents is likely to focus on enhancing their autonomy and expanding their capabilities:
Extended Context Lengths: Improvements in AI architecture could allow agents to process more information, enhancing their ability to handle long-term tasks.
Improved Long-Term Planning: Advanced planning algorithms and learning techniques will help agents manage complex tasks more effectively.
Enhanced Natural Language Understanding: Better natural language processing will make agents more reliable in interpreting and executing complex instructions.
Advanced Tool Integration: Agents will become better at utilizing a wider range of external tools, increasing their versatility.
Evolving Landscape of AI and Potential Future Impact
The landscape of AI applications and impacts will continue to grow:
Broader Adoption: LLM-powered agents will be adopted across more industries, automating and enhancing complex tasks.
Human-AI Collaboration: Agents will take on more routine and complex tasks, allowing humans to focus on strategic and creative endeavors.
Ethical and Regulatory Considerations: There will be a greater emphasis on ensuring transparency, fairness, and compliance with global standards.
Innovation and Advancements: Ongoing AI research will lead to the development of more sophisticated agents, improving cognitive functions and decision-making.
Conclusion
In conclusion, LLM-powered autonomous agents represent a transformative leap in AI technology. They combine language processing with advanced capabilities like planning, memory, and tool use. These agents can handle complex tasks, make informed decisions, and interact dynamically with their environments, offering a versatile solution for various industries.
RAGA AI leverages these advancements to ensure the quality and consistency of AI applications through its comprehensive testing platform.
By detecting, diagnosing, and fixing AI issues autonomously, RAGA AI accelerates the development lifecycle and reduces risk. Sign up for our comprehensive RagaAI Testing Platform today.
Imagine having an AI that doesn't just talk but takes action, solves problems, and improves on its own. When you dive into the world of artificial intelligence, you'll quickly encounter one of the most groundbreaking advancements: LLM-powered autonomous agents.
These agents are more than just sophisticated chatbots; they are action-takers, problem-solvers, and efficiency enhancers. By blending the language prowess of Large Language Models (LLMs) with the capability to detect, diagnose, and fix issues, these agents transform how we interact with AI.
Gone are the days of static LLMs that only generate text. Now, we have dynamic agents that actively improve their environments, setting the stage for smarter, faster, and more reliable AI systems. Let's understand the concept of LLM powered autonomous Agents.
LLM Powered Autonomous Agents: Overview
LLM-Powered Autonomous Agents are advanced AI systems that combine the language capabilities of Large Language Models (LLMs) with functionalities like reasoning, planning, tool use, and memory. When you hear about LLM-powered autonomous agents, think of them as supercharged versions of the AI models you already know.
These agents do more than understand and generate text. By integrating capabilities such as reasoning, specific knowledge, planning, tool use, and memory, they move from passive responders to active participants in various processes. To maintain their effectiveness, it's essential to understand how to detect and fix AI issues.
They take meaningful actions based on their understanding, allowing them to solve complex problems, streamline tasks, and improve outcomes.
Transition from LLMs to Autonomous Agents
The shift from standalone Large Language Models (LLMs) to autonomous agents marks a game-changing moment in AI development. Initially, LLMs like OpenAI’s GPT-4 or Google’s Gemini were impressive for their ability to generate human-like text.
These models could provide insightful responses and assist with a variety of language tasks.
However, their capabilities were limited to text generation, and they lacked the ability to take action or make decisions based on their outputs. So, a more dynamic approach was needed, leading to the development of LLM-powered autonomous agents.
These agents not only generate text but can also perform other tasks.
Because of this, autonomous agents are becoming invaluable in various fields, from customer service and marketing to scientific research and beyond. They significantly increase productivity and reduce the need for human intervention. A comprehensive LLM evaluation framework can help measure and enhance the performance of these autonomous agents.
Traditional Large Language Models (LLMs) vs Autonomous Agents
Traditional LLMs are sophisticated text generators. They excel at understanding and producing language but fall short when it comes to taking action or making decisions. In contrast, LLM-powered autonomous agents integrate several advanced functionalities:
Reasoning: They can think through problems and come up with solutions.
Specific Knowledge: They utilize domain-specific information to enhance their responses.
Planning: They break down tasks into manageable steps and follow through.
Tool Use: They can access and use external tools and databases via APIs.
Memory: They have both short-term and long-term memory, allowing them to learn from past interactions and store information for future use.
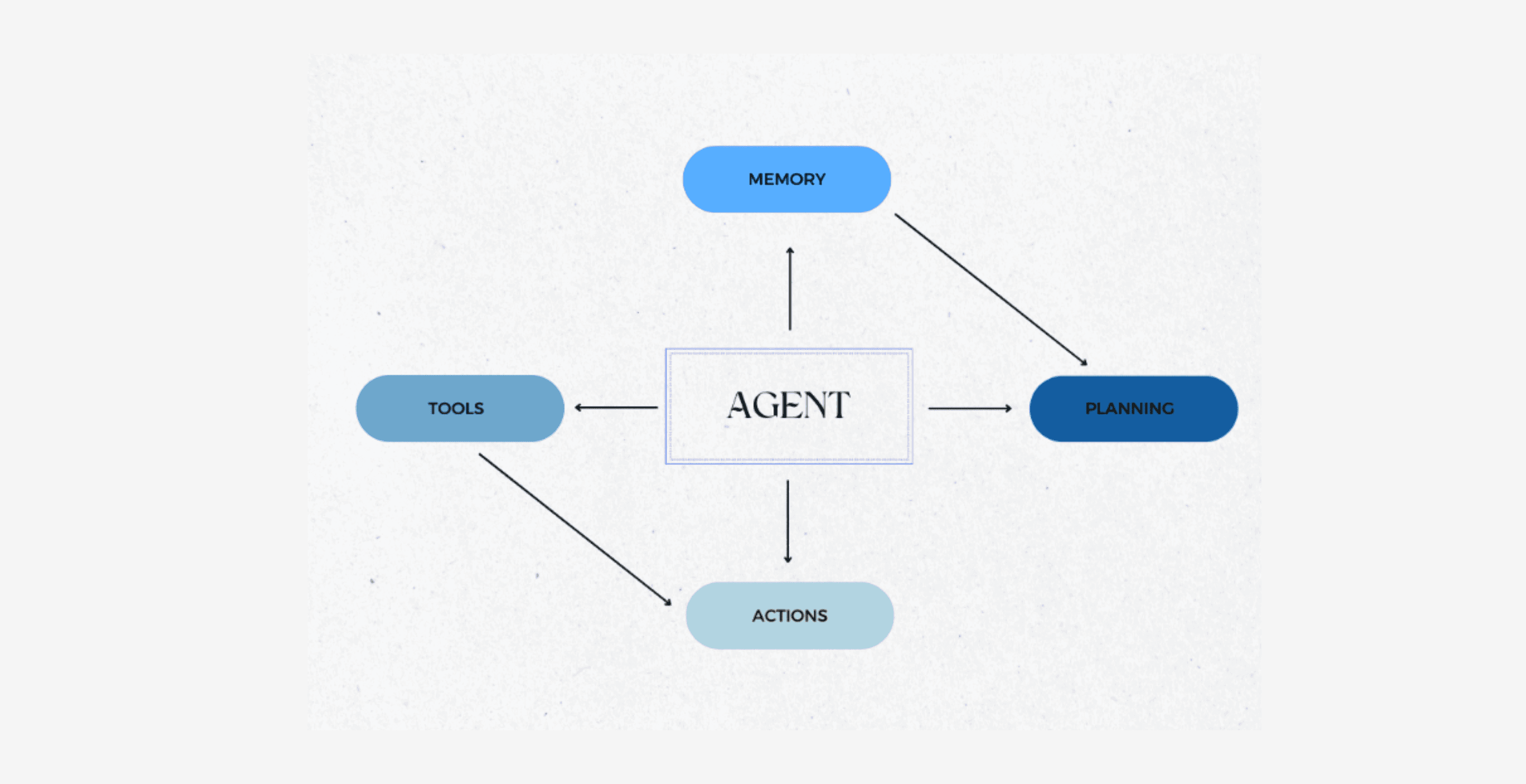
These enhancements allow agents to perform complex tasks that traditional LLMs cannot handle.
LLMs Beyond Simple Command-Response Mechanisms
The true power of LLM-powered autonomous agents lies in their expanded capabilities. Traditional LLMs operate on a simple command-response basis—you ask a question, and they provide an answer.
As previously explained, autonomous agents go far beyond this. They can interpret your instructions, create a plan of action, and execute that plan, all while using their built-in reasoning and memory capabilities to adapt and improve.
This means you can rely on them to handle more intricate tasks, such as managing workflows, optimizing processes, and even making data-driven decisions. For further insights on maintaining ethical standards, it is essential to understand the details of how correct AI governance is performed.
LLM Powered Autonomous Agents: Core Components
As explained above, LLM-powered autonomous agents are sophisticated advancements in artificial intelligence that combine the capabilities of large language models (LLMs) with additional functionalities like reasoning, planning, memory, and tool use.
They can not only generate and understand text but also execute tasks, make decisions, and interact dynamically with their environments. Evaluating and monitoring the performance of these agents is crucial for ensuring reliability, as outlined in the guide to evaluating LLM applications and enabling guardrails.
Let's understand the core components of the LLM-powered autonomous agents. They are as follows:
Planning
Planning is a fundamental component of LLM-powered autonomous agents. You can understand the overview of the Planning component from the bullet points provided below:
Task Decomposition: Agents break down complex tasks into smaller, manageable subgoals. This step-by-step approach simplifies the execution of intricate projects. It ensures that each segment of the task is handled effectively.
Self-Reflection: Advanced agents analyze their past actions. They learn from mistakes to refine their strategies. This continuous improvement mechanism enhances the quality of outcomes, increasing their ability to tackle increasingly complex tasks.
Memory
Memory is crucial for the effective functioning of LLM-powered autonomous agents. It is typically divided into short-term and long-term memory:
Short-Term Memory: This type of memory allows agents to retain and manipulate information relevant to the immediate task at hand, similar to how humans use short-term memory to recall recent events or information.
Long-Term Memory: This enables agents to store vast amounts of information over extended periods, often through external storage systems. It acts like a library of knowledge that the agent can access when necessary, supporting more informed decision-making and task execution.
The combination of these memory types allows agents to adapt to new information, learn from past experiences, and maintain a robust knowledge base.
Tools Usage:
Tool use is the added capability embedded in the LLM-powered autonomous agents that gives them the ability to augment capabilities. Tools usage can be summarized into two bullet points:
Extended Functionality: LLM-powered autonomous agents interact with external tools via APIs, accessing real-time data, executing code, and utilizing specialized databases.
Versatility: This ability to use tools transforms LLM-powered autonomous agents from mere language processors into versatile problem solvers, adapting to various scenarios and requirements.
Action
The decision-making process in LLM-powered autonomous agents is pivotal to their operation. When an agent decides to act, it can be either internal or external:
Internal Actions: These are confined to the agent's thought processes, such as generating and refining responses.
External Actions: These involve tangible outputs, like sending emails, managing data, or interacting with other systems.
The ability to choose and execute the appropriate type of action based on the context and objectives is a key feature that sets autonomous agents apart from traditional LLMs. This dual nature of actions allows them to be effective in a wide array of applications, from customer service to complex data analysis.
The overview of the components of an LLM-powered autonomous agent is shown below for your reference.

Pic: Overview of an LLM-powered autonomous agent with its system (Image source: Weng, Lilian. 2023)
Agent Component One: Planning
Planning plays a pivotal role in the operation of an LLM-powered autonomous agent. It's not just about understanding the steps involved in a complex task but also about strategizing for efficient execution. This is crucial for the development of effective strategies in LLM-powered autonomous agents.
By breaking down complex tasks into manageable steps, agents can approach intricate projects systematically. Let's understand the techniques involved in the "task decomposition" part of the Planning component used by LLM-powered autonomous agents.
Task Decomposition
Chain of thought (CoT; Wei et al., 2022) involves guiding the agent to think step-by-step, breaking down a task into a sequence of smaller, logical steps. It enhances the agent's performance by making its thought process transparent and methodical.
This technique has become a standard prompting technique for enhancing model performance on complex tasks. CoT transforms big tasks into multiple manageable tasks and sheds light on an interpretation of the model's thinking process.
Tree of Thoughts (Yao et al., 2023) is a further refinement of CoT and extends it by exploring multiple reasoning paths at each step, creating a tree structure. This technique explores multiple reasoning possibilities at each step. Under this, the task/problem is first decomposed into multiple thought steps, out of which multiple thoughts per step are generated, which creates a tree structure.
Each branch under the Tree of Thoughts represents a different potential solution, allowing the agent to evaluate various approaches and choose the most effective one. The search procedure offers both breadth-first search (BFS) and depth-first search (DFS) options. A classifier (via a prompt) or majority vote can assess each state.
Task decomposition under the Planning component can happen through the following methods:
(1) by LLM using straightforward questions such as "What are the subgoals for achieving XYZ?" and "Steps for XYZ. 1."
Using task-specific instructions, e.g. "Write a blog outline." for writing a specific topic
Using human inputs
Continuous Improvement Through Self-Reflection
LLM-powered autonomous agents continuously improve through the process of self-reflection, which plays a crucial role. Two key frameworks that facilitate self-reflection are ReAct and Reflexion:
ReAct Framework
Reflexion Framework
Let us understand both of these frameworks in detail.
ReAct Framework
ReAct Framework (Yao et al. 2023) Integrates reasoning and action, enabling agents to generate reasoning traces and perform task-specific actions. Reasoning enables the agent to interact with the environment (e.g., use Wikipedia search API). Acting prompts the powered agent to generate reasoning traces in natural language. This combination helps refine the agents' responses and actions based on real-time feedback.
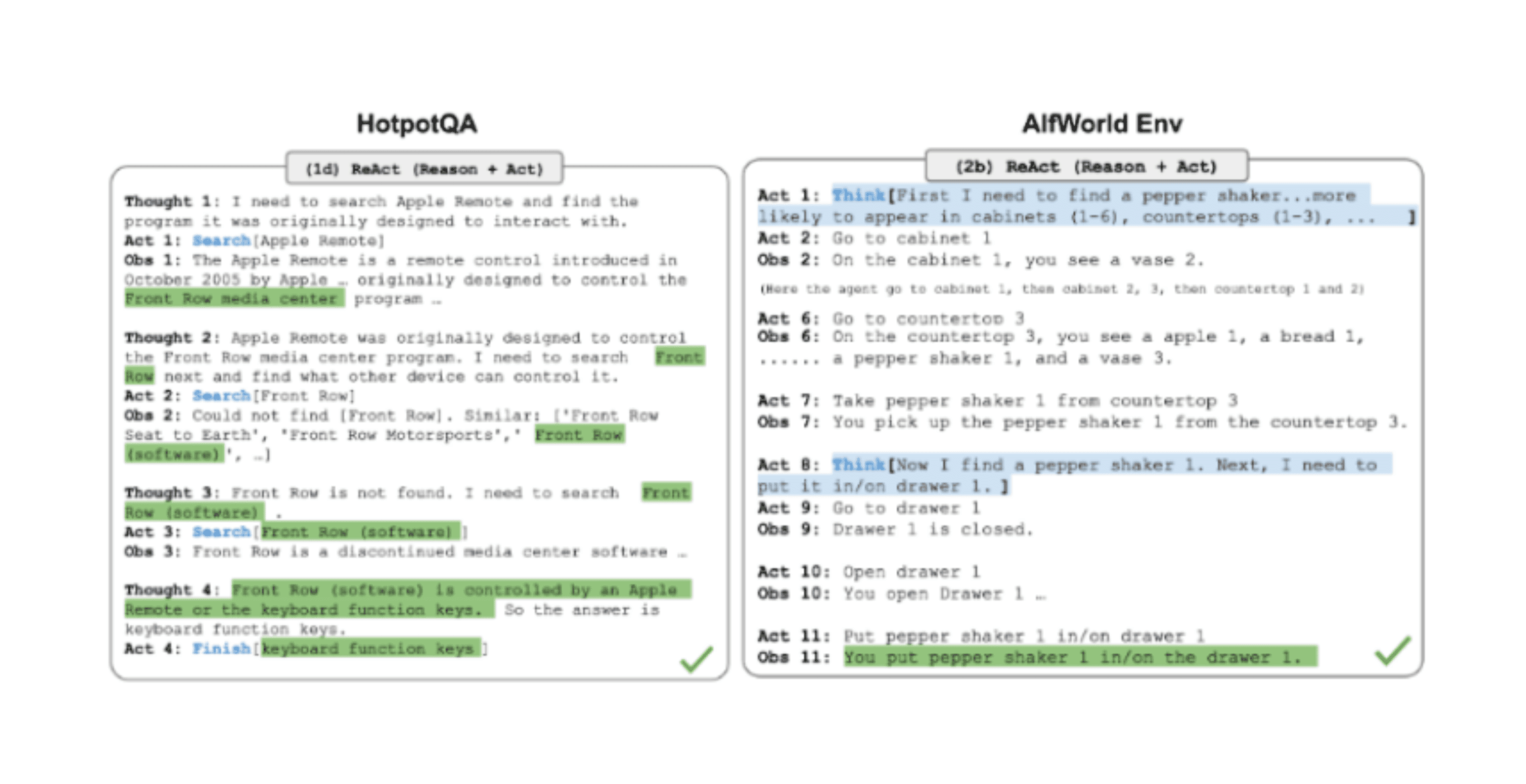
Pic: Examples of reasoning trajectories for knowledge-intensive tasks (e.g. HotpotQA, FEVER) and decision-making tasks (e.g. AlfWorld Env, WebShop). (Image source: Yao et al. 2023).
Reflexion Framework
Reflexion (Shinn & Labash, 2023) equips agents with dynamic memory and self-reflection capabilities, which improve their reasoning skills. This framework equips agents with dynamic memory and self-reflection capabilities. By analyzing past actions and outcomes, agents can identify errors and inefficiencies, allowing them to adjust their strategies and improve future performance.
Reflexion uses a basic reinforcement learning architecture, where the task-specific action space is enhanced with language to facilitate sophisticated reasoning stages. At the same time, the reward model offers a straightforward binary payout. Following every action, the agent calculates a heuristic and may choose to reset the environment to begin a new trial based on the outcomes of its self-reflection.
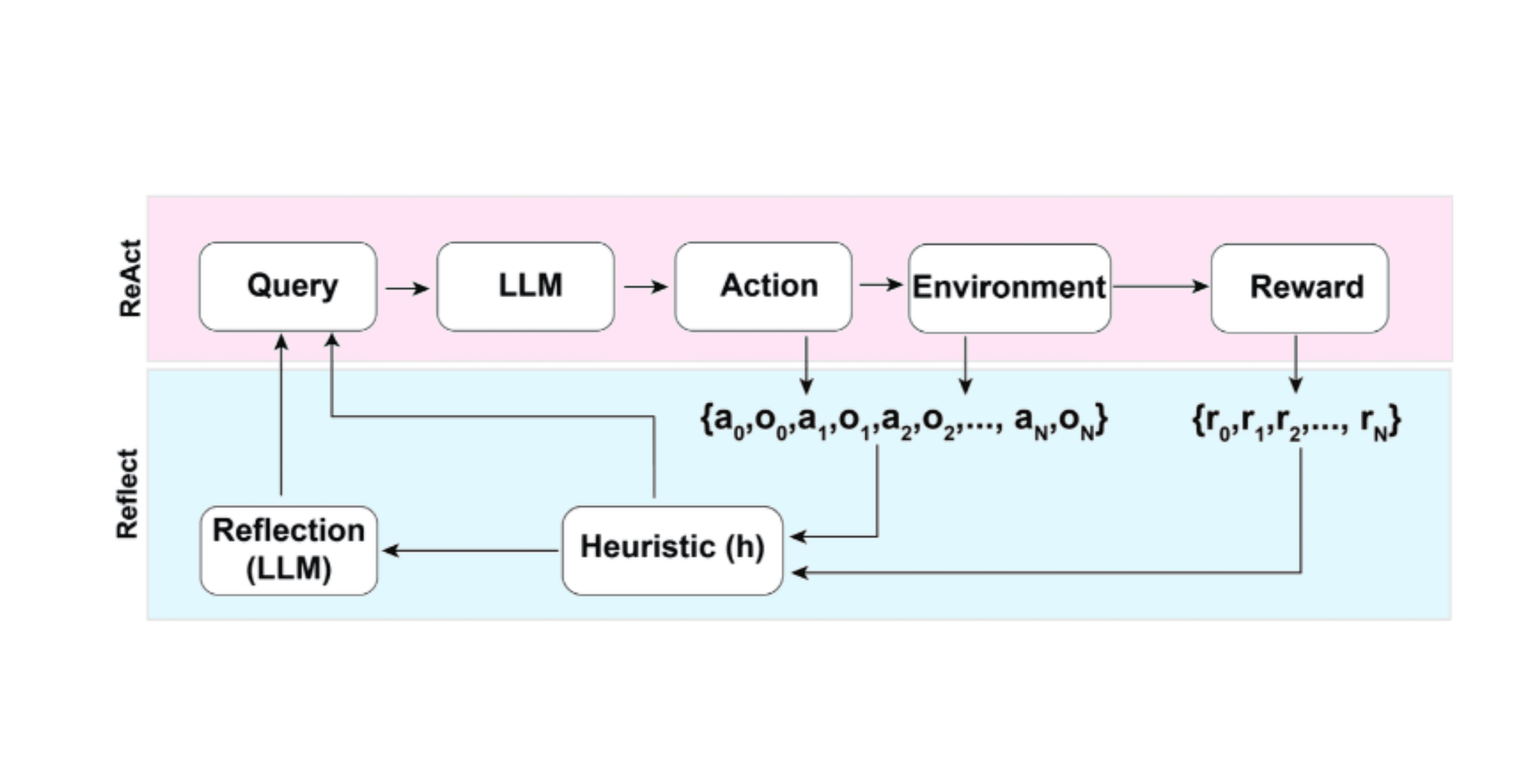
Pic: Illustration of the Reflexion framework. (Image source: Shinn & Labash, 2023)
Enhanced Long-Horizon Planning: Integrating Classical Planners with LLMs
For more complex, long-horizon tasks, integrating classical planners with LLMs can enhance planning capabilities for complex, long-horizon tasks. This approach involves translating the problem into PDDL, using an external classical planner to generate a detailed plan, and then translating the plan back into natural language for the agent to execute.
Translation to PDDL: The LLM translates a given problem into a PDDL format, defining the planning problem in a structured way.
Classical Planning: An external classical planner generates a detailed plan based on the PDDL description, utilizing domain-specific knowledge and algorithms.
Translation Back to Natural Language: The LLM translates the generated plan back into natural language, making it actionable for the agent.
Agent Component Two: Memory
Memory management is a cornerstone of LLM-powered autonomous agents, enabling them to store and recall information crucial for their operations. Memory in LLM-powered autonomous agents serves the same purpose as it does in a human brain.
Let's understand the types of memory in both the context of Human Memory and LLM-powered autonomous agents.
Classification of Memory: Human Brain
The procedures required to gather, store, hold onto, and then retrieve information are collectively referred to as memory. Human brains have multiple types of memory.
Human memory is broadly understood under the classification of:
Sensory Memory: The ability to hold onto impressions of sensory information (visual, aural, etc.) after the initial stimulation has finished is known as sensory memory, which is the first stage of memory. Usually, sensory memory is limited to a few seconds. There are three subcategories: haptic memory (touch), auditory echoic memory, and iconic memory (visual).
Short-Term Memory (STM) or Working Memory: It retains knowledge that we now possess and is required to perform sophisticated mental operations like learning and reasoning. According to Miller (1956), short-term memory may hold seven items and last 20 to 30 seconds.
Long-Term Memory (LTM): Information can be stored in long-term memory for a very long period—from a few days to decades—and it has practically infinite storage space. LTM comes in two subtypes:
Explicit/declarative memory: This memory refers to the consciously recalled recollections of facts and events, such as episodic memory (experiences and events) and semantic memory (concepts and facts).
Implicit/procedural memory: This kind of memory is unconscious and pertains to automatic skills and routines, such as riding a bike or using a keyboard.
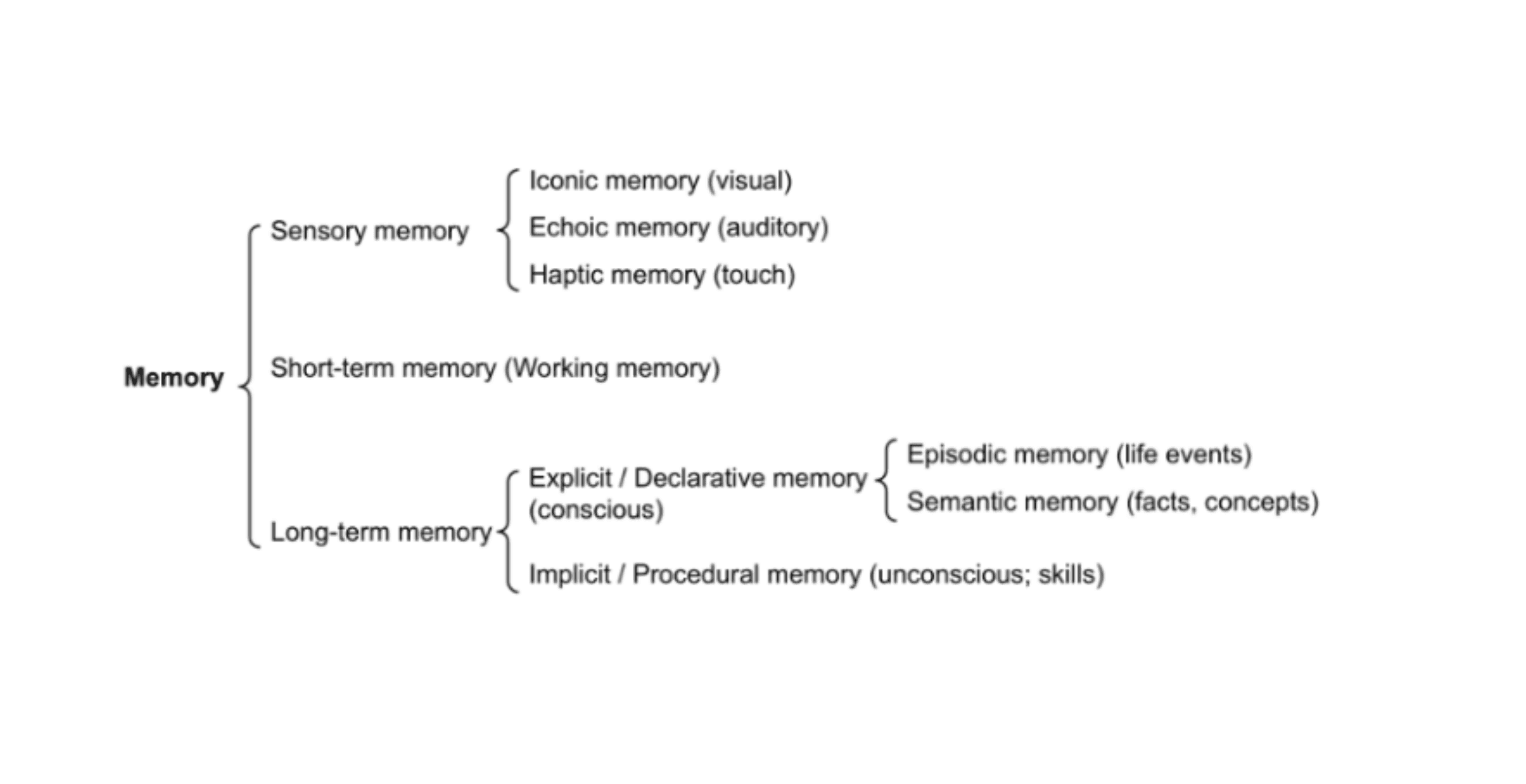
Pic: Classification and Categorization of Human Memory
Classification of Memory: LLM-Powered Autonomous Agents
Now that you have understood the classification of memory as per the human brain, it becomes easier to understand how memory is classified in the context of LLM-Powered Autonomous Agents.
The classifications are as follows:
Short-Term Memory (STM): This type of memory allows agents to hold and manipulate information relevant to the immediate task at hand. It is similar to how humans remember recent events or data points for short periods. STM is crucial for in-context learning, where the agent uses recent interactions to inform its current actions and responses
Long-term Memory (LTM): LTM enables agents to store vast amounts of information over extended periods. External storage systems often facilitate this memory, acting as repositories of knowledge that the agent can access as needed. LTM is essential for retaining information that might be required for future tasks or for drawing upon past experiences to improve performance.
Practical Implementation of Memory For Agents
In practical applications, implementing memory in LLM-powered autonomous agents often involves the use of external vector stores.
These stores are specialized databases that efficiently manage and retrieve large volumes of data. Here are a few examples:
Customer Support Systems: Agents can use short-term memory to handle ongoing customer interactions effectively, while long-term memory stores customer history, preferences, and past interactions, allowing the agent to provide personalized support.
Research and Development: In scientific research, agents can store and recall extensive datasets, research papers, and experimental results. Short-term memory helps in handling current research tasks, while long-term memory retains valuable historical data for future reference.
Educational Tools: Educational agents can use short-term memory to adapt to a student's immediate learning needs and questions, while long-term memory stores the student's progress, strengths, and weaknesses over time, enabling tailored educational experiences.
Importance of Memory in LLM-Powered Autonomous Agents
Memory plays a pivotal role in the effectiveness and adaptability of LLM-powered autonomous agents. Here's why it is crucial:
In-Context Learning: Short-term memory enables agents to utilize recent information to make informed decisions and generate accurate responses. This capability is vital for maintaining continuity and relevance in interactions, especially in dynamic and multi-step tasks.
Information Retention: Long-term memory allows agents to store knowledge and experiences over extended periods. This retention is essential for building a comprehensive understanding of various domains, improving problem-solving skills, and enhancing overall performance. By retaining information, agents can draw upon past experiences to navigate new challenges more effectively.
Component Three: Tool Utilization
LLM-powered autonomous agents extend their capabilities by interacting with external APIs, which allows them to access real-time data, execute code, and retrieve information from specialized databases.
This interaction significantly enhances their functionality beyond their pre-trained knowledge. By leveraging APIs, these agents can:
Access up-to-date information that is not part of their initial training data.
Perform specific tasks that require external computational resources or data.
Integrate specialized functions and tools, thereby increasing their versatility and applicability in various domains.
Examples of MRKL Architecture and Specific Projects like TAL and Toolformer
To better understand how LLM agents utilize external tools, let's look at some specific architectures and projects:
MRKL Architecture (Karpas et al. 2022): MRKL stands for Modular Reasoning, Knowledge, and Language. It is a neuro-symbolic architecture designed to enhance the reasoning capabilities of LLMs by integrating various expert modules. In MRKL systems, the LLM acts as a router, directing inquiries to the appropriate expert module, whether it be a neural network, symbolic system, or other specialized tools. This modular approach allows the agent to perform complex reasoning tasks more effectively.
TAL (Tool Augmented Language Models) (Parisi et al., 2022): TAL involves fine-tuning LLMs to learn how to call external APIs. The model is trained to recognize when and how to use these APIs to improve the quality of its outputs. By incorporating API calls into its responses, TAL can provide more accurate and contextually relevant information.
Toolformer (Schick et al., 2023): Similar to TAL, Toolformer is a project where LLMs are fine-tuned to use external tools automatically. The training process includes adding API call annotations to the dataset, allowing the model to learn when and how to make these calls to enhance its performance. Toolformer showcases the practical implementation of tool use in LLM agents, demonstrating significant improvements in task execution.
Significance of External Tools in Enhancing Problem-Solving Capabilities
The use of external tools is crucial in elevating the problem-solving abilities of LLM-powered autonomous agents. Here is why:
Enhanced Data Access: External tools allow agents to access a broader range of data sources, including real-time information and proprietary databases, which are essential for making informed decisions.
Specialized Functionality: By integrating with tools designed for specific tasks, such as mathematical computation, data analysis, or language translation, agents can perform these tasks more accurately and efficiently.
Adaptability: The ability to use external tools makes agents more adaptable to different scenarios and requirements. They can switch between various tools depending on the task at hand, providing a more versatile and robust solution.
Incorporating external tools transforms LLM agents from mere language processors into comprehensive problem solvers. This capability enables them to tackle a wide array of tasks, from simple information retrieval to complex data analysis, making them invaluable assets in both professional and personal applications.
LLM Powered Autonomous Agents: Capabilities and Applications
The difference between LLM-powered autonomous agents and traditional large language models (LLMs) lies in the range and complexity of tasks they can perform autonomously.
Traditional LLMs are primarily designed to generate text and respond to queries based on pre-trained data. They excel at understanding language and producing coherent responses but lack the ability to take meaningful actions or make decisions beyond text generation.
In contrast, LLM-powered autonomous agents integrate advanced functionalities such as reasoning, planning, memory, and tool use, enabling them to execute tasks independently. They can:
Plan and execute multi-step tasks: Decompose complex projects into manageable subgoals and follow through with action plans.
Interact with external systems: Use APIs to access real-time data, execute commands, and integrate specialized tools.
Learn and adapt: Utilize short-term and long-term memory to retain information and improve performance over time.
Make informed decisions: Apply reasoning capabilities to choose the best course of action based on context and available data.
This expanded spectrum of tasks makes autonomous agents far more versatile and capable than traditional LLMs.
Practical Applications of Agents
LLM-powered autonomous agents are revolutionizing various industries by enhancing efficiency and effectiveness in numerous applications:
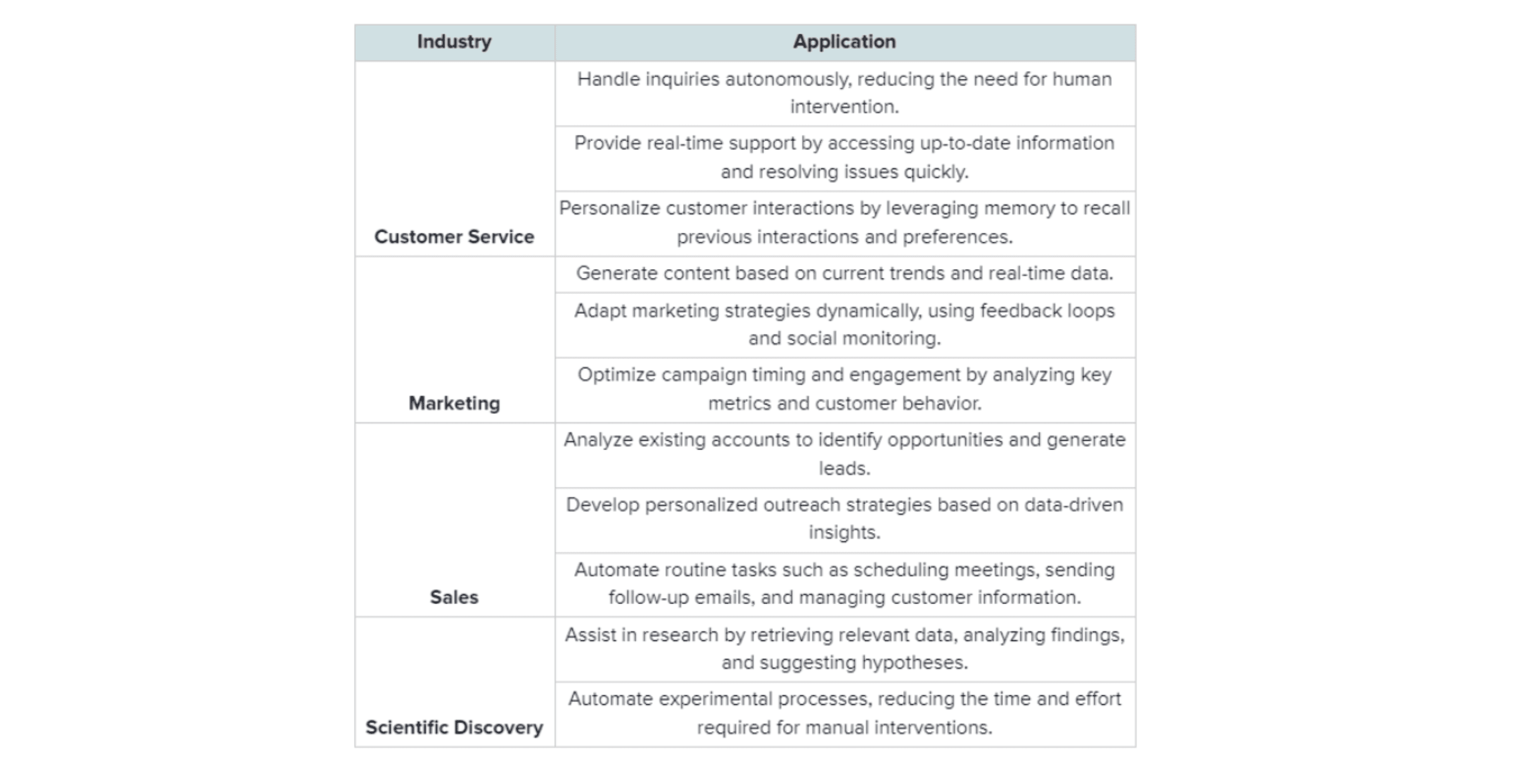
Pic: Industry Specific Applications for LLM Powered Autonoumous Agents
Autonomy in Task Execution Leading from Agents
The autonomy of LLM-powered agents in task execution is driving significant advancements in productivity and automation. By independently handling complex and routine tasks, these agents free up human resources to focus on higher-level strategic activities. Key benefits include:
Increased Efficiency: Autonomous agents can operate continuously without fatigue, ensuring tasks are completed faster and more accurately.
Reduced Costs: Automating routine and repetitive tasks reduces the need for extensive human labor, leading to cost savings.
Scalability: Agents can handle large volumes of tasks simultaneously, making it easier to scale operations without proportional increases in human resources.
Innovation: With routine tasks automated, humans can focus on creative and innovative aspects of their work, driving further advancements and improvements.
In summary, the capabilities and applications of LLM-powered autonomous agents are transforming industries by enhancing efficiency, reducing costs, and enabling more innovative and productive work environments.
LLM-Powered Autonomous Agents: Challenges and Future Directions
While LLM-powered autonomous agents represent a significant advancement in AI, they come with their own set of challenges:
Finite Context Length: One of the primary limitations is the finite context length of LLMs. This restricts the amount of historical information and detailed instructions that the agent can process at any given time, which can impact its ability to handle long and complex tasks effectively.
Long-Term Planning Difficulties: Despite improvements, long-term planning remains a challenge. Agents can struggle to maintain coherence and effectiveness over extended tasks, particularly those requiring intricate, multi-step processes.
Reliability of Natural Language Interfaces: The natural language interface, while powerful, is not infallible. Agents may occasionally produce incorrect or ambiguous outputs, make formatting errors, or misinterpret complex instructions. This can lead to issues in task execution and reduce the reliability of the agents.
LLM Powered Autonomous Agents: Future
The future development of LLM-powered autonomous agents is likely to focus on enhancing their autonomy and expanding their capabilities:
Extended Context Lengths: Improvements in AI architecture could allow agents to process more information, enhancing their ability to handle long-term tasks.
Improved Long-Term Planning: Advanced planning algorithms and learning techniques will help agents manage complex tasks more effectively.
Enhanced Natural Language Understanding: Better natural language processing will make agents more reliable in interpreting and executing complex instructions.
Advanced Tool Integration: Agents will become better at utilizing a wider range of external tools, increasing their versatility.
Evolving Landscape of AI and Potential Future Impact
The landscape of AI applications and impacts will continue to grow:
Broader Adoption: LLM-powered agents will be adopted across more industries, automating and enhancing complex tasks.
Human-AI Collaboration: Agents will take on more routine and complex tasks, allowing humans to focus on strategic and creative endeavors.
Ethical and Regulatory Considerations: There will be a greater emphasis on ensuring transparency, fairness, and compliance with global standards.
Innovation and Advancements: Ongoing AI research will lead to the development of more sophisticated agents, improving cognitive functions and decision-making.
Conclusion
In conclusion, LLM-powered autonomous agents represent a transformative leap in AI technology. They combine language processing with advanced capabilities like planning, memory, and tool use. These agents can handle complex tasks, make informed decisions, and interact dynamically with their environments, offering a versatile solution for various industries.
RAGA AI leverages these advancements to ensure the quality and consistency of AI applications through its comprehensive testing platform.
By detecting, diagnosing, and fixing AI issues autonomously, RAGA AI accelerates the development lifecycle and reduces risk. Sign up for our comprehensive RagaAI Testing Platform today.
Imagine having an AI that doesn't just talk but takes action, solves problems, and improves on its own. When you dive into the world of artificial intelligence, you'll quickly encounter one of the most groundbreaking advancements: LLM-powered autonomous agents.
These agents are more than just sophisticated chatbots; they are action-takers, problem-solvers, and efficiency enhancers. By blending the language prowess of Large Language Models (LLMs) with the capability to detect, diagnose, and fix issues, these agents transform how we interact with AI.
Gone are the days of static LLMs that only generate text. Now, we have dynamic agents that actively improve their environments, setting the stage for smarter, faster, and more reliable AI systems. Let's understand the concept of LLM powered autonomous Agents.
LLM Powered Autonomous Agents: Overview
LLM-Powered Autonomous Agents are advanced AI systems that combine the language capabilities of Large Language Models (LLMs) with functionalities like reasoning, planning, tool use, and memory. When you hear about LLM-powered autonomous agents, think of them as supercharged versions of the AI models you already know.
These agents do more than understand and generate text. By integrating capabilities such as reasoning, specific knowledge, planning, tool use, and memory, they move from passive responders to active participants in various processes. To maintain their effectiveness, it's essential to understand how to detect and fix AI issues.
They take meaningful actions based on their understanding, allowing them to solve complex problems, streamline tasks, and improve outcomes.
Transition from LLMs to Autonomous Agents
The shift from standalone Large Language Models (LLMs) to autonomous agents marks a game-changing moment in AI development. Initially, LLMs like OpenAI’s GPT-4 or Google’s Gemini were impressive for their ability to generate human-like text.
These models could provide insightful responses and assist with a variety of language tasks.
However, their capabilities were limited to text generation, and they lacked the ability to take action or make decisions based on their outputs. So, a more dynamic approach was needed, leading to the development of LLM-powered autonomous agents.
These agents not only generate text but can also perform other tasks.
Because of this, autonomous agents are becoming invaluable in various fields, from customer service and marketing to scientific research and beyond. They significantly increase productivity and reduce the need for human intervention. A comprehensive LLM evaluation framework can help measure and enhance the performance of these autonomous agents.
Traditional Large Language Models (LLMs) vs Autonomous Agents
Traditional LLMs are sophisticated text generators. They excel at understanding and producing language but fall short when it comes to taking action or making decisions. In contrast, LLM-powered autonomous agents integrate several advanced functionalities:
Reasoning: They can think through problems and come up with solutions.
Specific Knowledge: They utilize domain-specific information to enhance their responses.
Planning: They break down tasks into manageable steps and follow through.
Tool Use: They can access and use external tools and databases via APIs.
Memory: They have both short-term and long-term memory, allowing them to learn from past interactions and store information for future use.
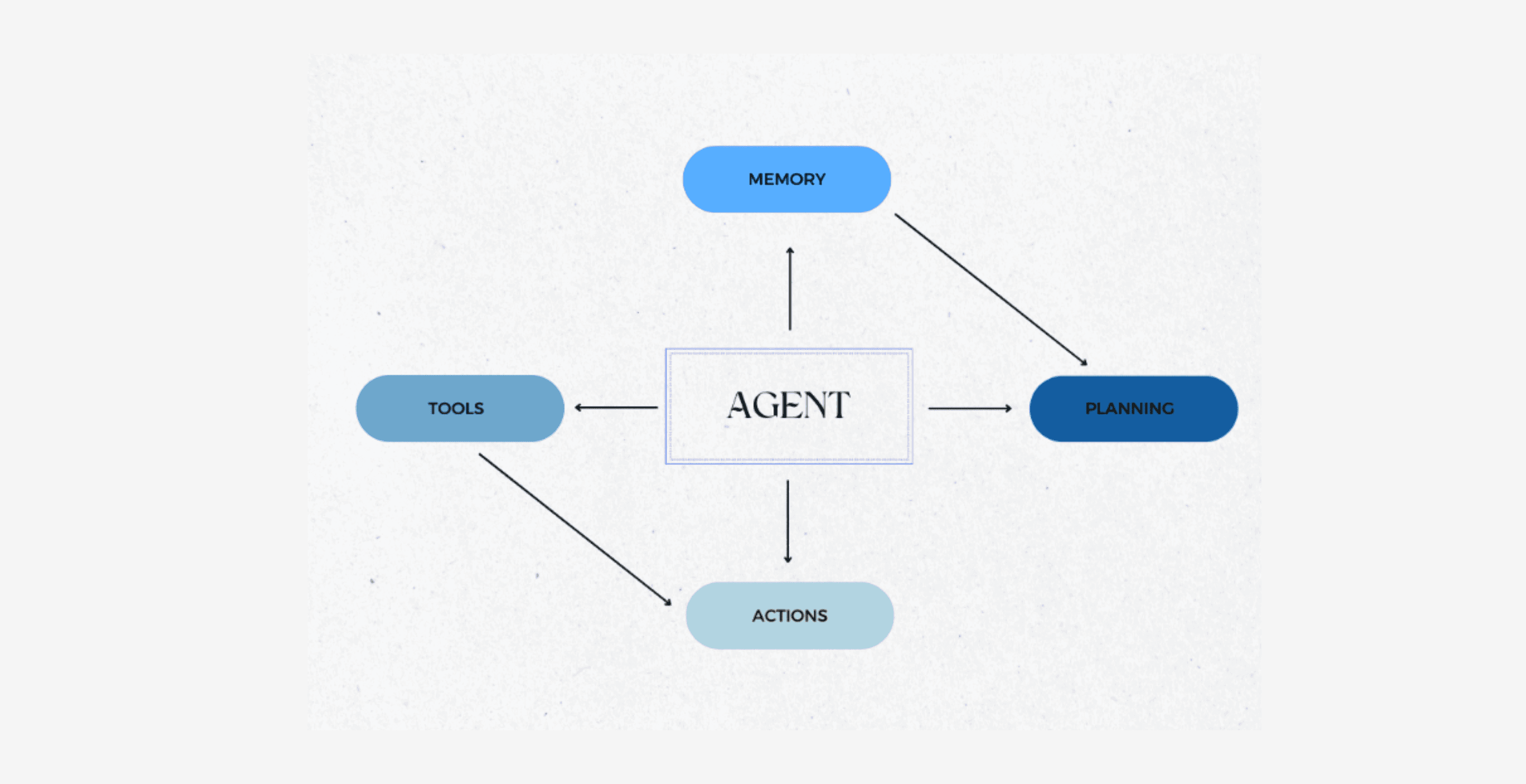
These enhancements allow agents to perform complex tasks that traditional LLMs cannot handle.
LLMs Beyond Simple Command-Response Mechanisms
The true power of LLM-powered autonomous agents lies in their expanded capabilities. Traditional LLMs operate on a simple command-response basis—you ask a question, and they provide an answer.
As previously explained, autonomous agents go far beyond this. They can interpret your instructions, create a plan of action, and execute that plan, all while using their built-in reasoning and memory capabilities to adapt and improve.
This means you can rely on them to handle more intricate tasks, such as managing workflows, optimizing processes, and even making data-driven decisions. For further insights on maintaining ethical standards, it is essential to understand the details of how correct AI governance is performed.
LLM Powered Autonomous Agents: Core Components
As explained above, LLM-powered autonomous agents are sophisticated advancements in artificial intelligence that combine the capabilities of large language models (LLMs) with additional functionalities like reasoning, planning, memory, and tool use.
They can not only generate and understand text but also execute tasks, make decisions, and interact dynamically with their environments. Evaluating and monitoring the performance of these agents is crucial for ensuring reliability, as outlined in the guide to evaluating LLM applications and enabling guardrails.
Let's understand the core components of the LLM-powered autonomous agents. They are as follows:
Planning
Planning is a fundamental component of LLM-powered autonomous agents. You can understand the overview of the Planning component from the bullet points provided below:
Task Decomposition: Agents break down complex tasks into smaller, manageable subgoals. This step-by-step approach simplifies the execution of intricate projects. It ensures that each segment of the task is handled effectively.
Self-Reflection: Advanced agents analyze their past actions. They learn from mistakes to refine their strategies. This continuous improvement mechanism enhances the quality of outcomes, increasing their ability to tackle increasingly complex tasks.
Memory
Memory is crucial for the effective functioning of LLM-powered autonomous agents. It is typically divided into short-term and long-term memory:
Short-Term Memory: This type of memory allows agents to retain and manipulate information relevant to the immediate task at hand, similar to how humans use short-term memory to recall recent events or information.
Long-Term Memory: This enables agents to store vast amounts of information over extended periods, often through external storage systems. It acts like a library of knowledge that the agent can access when necessary, supporting more informed decision-making and task execution.
The combination of these memory types allows agents to adapt to new information, learn from past experiences, and maintain a robust knowledge base.
Tools Usage:
Tool use is the added capability embedded in the LLM-powered autonomous agents that gives them the ability to augment capabilities. Tools usage can be summarized into two bullet points:
Extended Functionality: LLM-powered autonomous agents interact with external tools via APIs, accessing real-time data, executing code, and utilizing specialized databases.
Versatility: This ability to use tools transforms LLM-powered autonomous agents from mere language processors into versatile problem solvers, adapting to various scenarios and requirements.
Action
The decision-making process in LLM-powered autonomous agents is pivotal to their operation. When an agent decides to act, it can be either internal or external:
Internal Actions: These are confined to the agent's thought processes, such as generating and refining responses.
External Actions: These involve tangible outputs, like sending emails, managing data, or interacting with other systems.
The ability to choose and execute the appropriate type of action based on the context and objectives is a key feature that sets autonomous agents apart from traditional LLMs. This dual nature of actions allows them to be effective in a wide array of applications, from customer service to complex data analysis.
The overview of the components of an LLM-powered autonomous agent is shown below for your reference.

Pic: Overview of an LLM-powered autonomous agent with its system (Image source: Weng, Lilian. 2023)
Agent Component One: Planning
Planning plays a pivotal role in the operation of an LLM-powered autonomous agent. It's not just about understanding the steps involved in a complex task but also about strategizing for efficient execution. This is crucial for the development of effective strategies in LLM-powered autonomous agents.
By breaking down complex tasks into manageable steps, agents can approach intricate projects systematically. Let's understand the techniques involved in the "task decomposition" part of the Planning component used by LLM-powered autonomous agents.
Task Decomposition
Chain of thought (CoT; Wei et al., 2022) involves guiding the agent to think step-by-step, breaking down a task into a sequence of smaller, logical steps. It enhances the agent's performance by making its thought process transparent and methodical.
This technique has become a standard prompting technique for enhancing model performance on complex tasks. CoT transforms big tasks into multiple manageable tasks and sheds light on an interpretation of the model's thinking process.
Tree of Thoughts (Yao et al., 2023) is a further refinement of CoT and extends it by exploring multiple reasoning paths at each step, creating a tree structure. This technique explores multiple reasoning possibilities at each step. Under this, the task/problem is first decomposed into multiple thought steps, out of which multiple thoughts per step are generated, which creates a tree structure.
Each branch under the Tree of Thoughts represents a different potential solution, allowing the agent to evaluate various approaches and choose the most effective one. The search procedure offers both breadth-first search (BFS) and depth-first search (DFS) options. A classifier (via a prompt) or majority vote can assess each state.
Task decomposition under the Planning component can happen through the following methods:
(1) by LLM using straightforward questions such as "What are the subgoals for achieving XYZ?" and "Steps for XYZ. 1."
Using task-specific instructions, e.g. "Write a blog outline." for writing a specific topic
Using human inputs
Continuous Improvement Through Self-Reflection
LLM-powered autonomous agents continuously improve through the process of self-reflection, which plays a crucial role. Two key frameworks that facilitate self-reflection are ReAct and Reflexion:
ReAct Framework
Reflexion Framework
Let us understand both of these frameworks in detail.
ReAct Framework
ReAct Framework (Yao et al. 2023) Integrates reasoning and action, enabling agents to generate reasoning traces and perform task-specific actions. Reasoning enables the agent to interact with the environment (e.g., use Wikipedia search API). Acting prompts the powered agent to generate reasoning traces in natural language. This combination helps refine the agents' responses and actions based on real-time feedback.
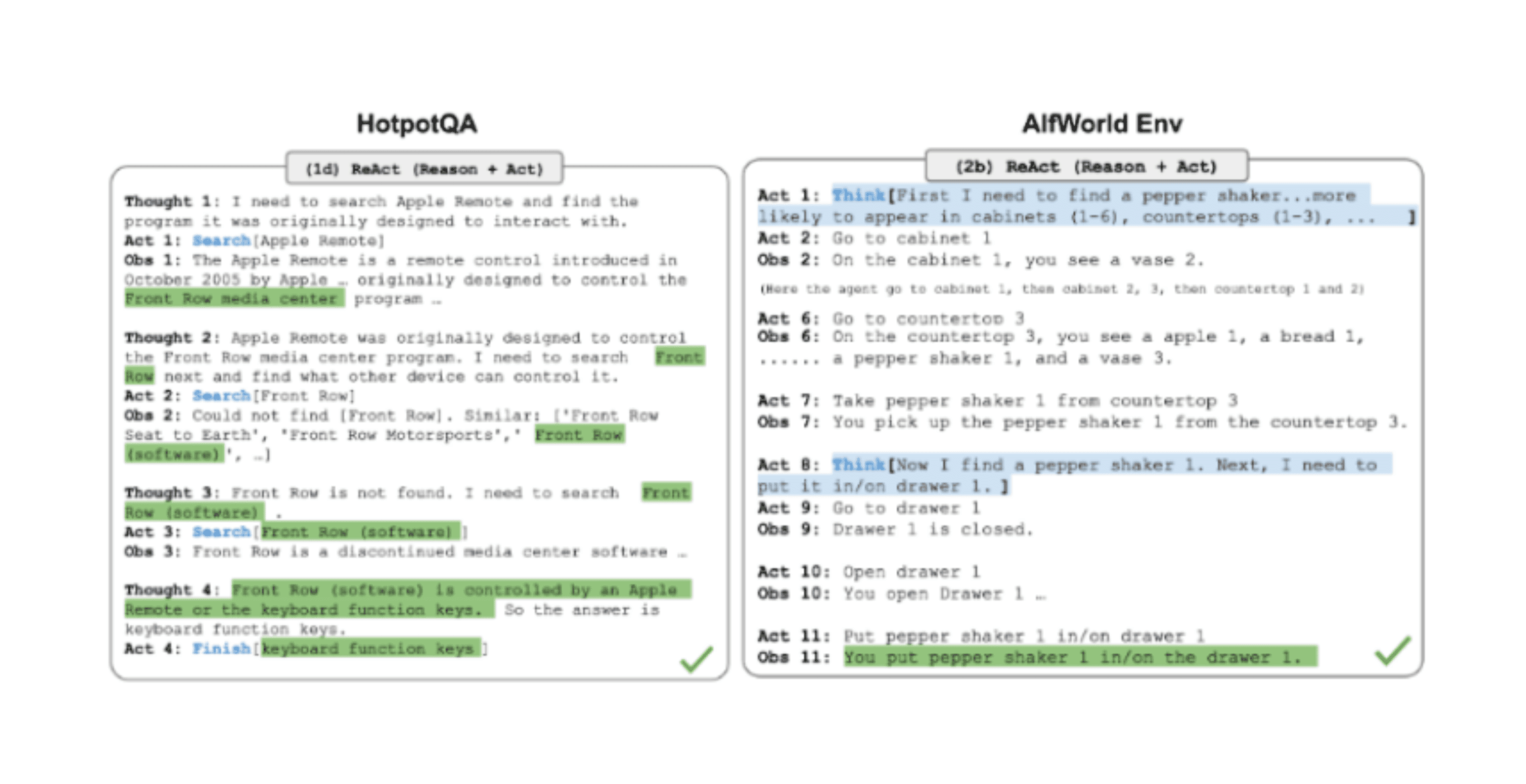
Pic: Examples of reasoning trajectories for knowledge-intensive tasks (e.g. HotpotQA, FEVER) and decision-making tasks (e.g. AlfWorld Env, WebShop). (Image source: Yao et al. 2023).
Reflexion Framework
Reflexion (Shinn & Labash, 2023) equips agents with dynamic memory and self-reflection capabilities, which improve their reasoning skills. This framework equips agents with dynamic memory and self-reflection capabilities. By analyzing past actions and outcomes, agents can identify errors and inefficiencies, allowing them to adjust their strategies and improve future performance.
Reflexion uses a basic reinforcement learning architecture, where the task-specific action space is enhanced with language to facilitate sophisticated reasoning stages. At the same time, the reward model offers a straightforward binary payout. Following every action, the agent calculates a heuristic and may choose to reset the environment to begin a new trial based on the outcomes of its self-reflection.
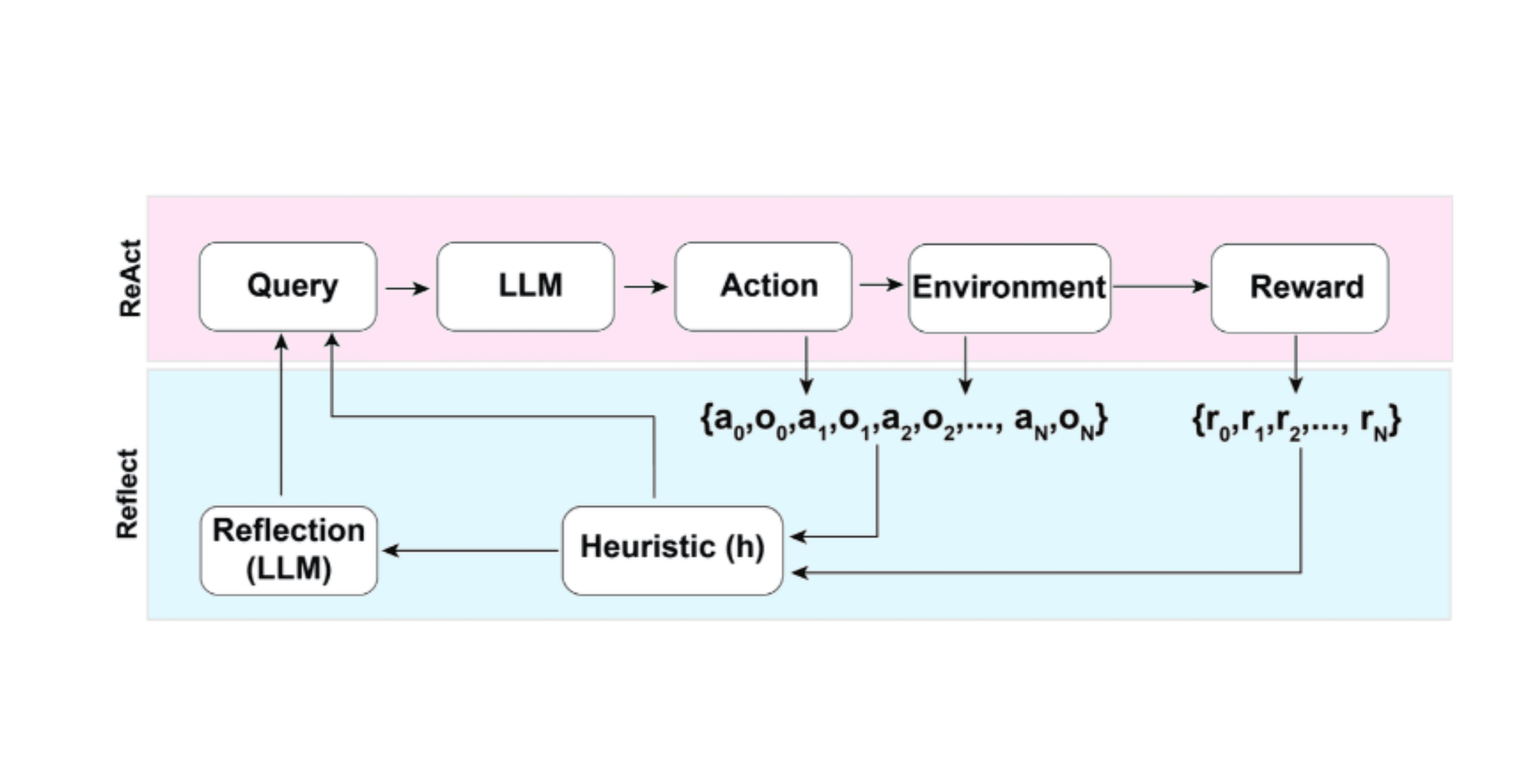
Pic: Illustration of the Reflexion framework. (Image source: Shinn & Labash, 2023)
Enhanced Long-Horizon Planning: Integrating Classical Planners with LLMs
For more complex, long-horizon tasks, integrating classical planners with LLMs can enhance planning capabilities for complex, long-horizon tasks. This approach involves translating the problem into PDDL, using an external classical planner to generate a detailed plan, and then translating the plan back into natural language for the agent to execute.
Translation to PDDL: The LLM translates a given problem into a PDDL format, defining the planning problem in a structured way.
Classical Planning: An external classical planner generates a detailed plan based on the PDDL description, utilizing domain-specific knowledge and algorithms.
Translation Back to Natural Language: The LLM translates the generated plan back into natural language, making it actionable for the agent.
Agent Component Two: Memory
Memory management is a cornerstone of LLM-powered autonomous agents, enabling them to store and recall information crucial for their operations. Memory in LLM-powered autonomous agents serves the same purpose as it does in a human brain.
Let's understand the types of memory in both the context of Human Memory and LLM-powered autonomous agents.
Classification of Memory: Human Brain
The procedures required to gather, store, hold onto, and then retrieve information are collectively referred to as memory. Human brains have multiple types of memory.
Human memory is broadly understood under the classification of:
Sensory Memory: The ability to hold onto impressions of sensory information (visual, aural, etc.) after the initial stimulation has finished is known as sensory memory, which is the first stage of memory. Usually, sensory memory is limited to a few seconds. There are three subcategories: haptic memory (touch), auditory echoic memory, and iconic memory (visual).
Short-Term Memory (STM) or Working Memory: It retains knowledge that we now possess and is required to perform sophisticated mental operations like learning and reasoning. According to Miller (1956), short-term memory may hold seven items and last 20 to 30 seconds.
Long-Term Memory (LTM): Information can be stored in long-term memory for a very long period—from a few days to decades—and it has practically infinite storage space. LTM comes in two subtypes:
Explicit/declarative memory: This memory refers to the consciously recalled recollections of facts and events, such as episodic memory (experiences and events) and semantic memory (concepts and facts).
Implicit/procedural memory: This kind of memory is unconscious and pertains to automatic skills and routines, such as riding a bike or using a keyboard.
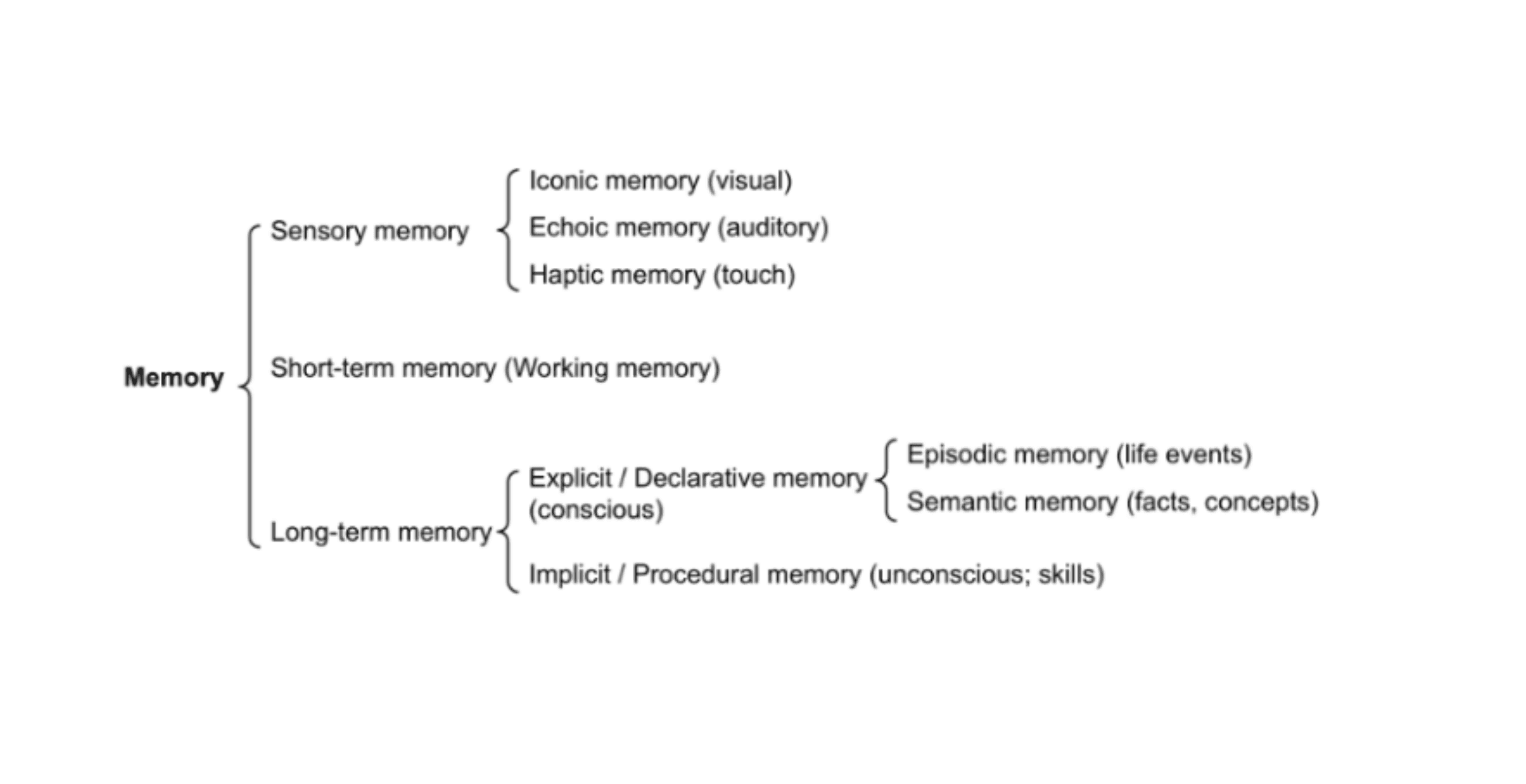
Pic: Classification and Categorization of Human Memory
Classification of Memory: LLM-Powered Autonomous Agents
Now that you have understood the classification of memory as per the human brain, it becomes easier to understand how memory is classified in the context of LLM-Powered Autonomous Agents.
The classifications are as follows:
Short-Term Memory (STM): This type of memory allows agents to hold and manipulate information relevant to the immediate task at hand. It is similar to how humans remember recent events or data points for short periods. STM is crucial for in-context learning, where the agent uses recent interactions to inform its current actions and responses
Long-term Memory (LTM): LTM enables agents to store vast amounts of information over extended periods. External storage systems often facilitate this memory, acting as repositories of knowledge that the agent can access as needed. LTM is essential for retaining information that might be required for future tasks or for drawing upon past experiences to improve performance.
Practical Implementation of Memory For Agents
In practical applications, implementing memory in LLM-powered autonomous agents often involves the use of external vector stores.
These stores are specialized databases that efficiently manage and retrieve large volumes of data. Here are a few examples:
Customer Support Systems: Agents can use short-term memory to handle ongoing customer interactions effectively, while long-term memory stores customer history, preferences, and past interactions, allowing the agent to provide personalized support.
Research and Development: In scientific research, agents can store and recall extensive datasets, research papers, and experimental results. Short-term memory helps in handling current research tasks, while long-term memory retains valuable historical data for future reference.
Educational Tools: Educational agents can use short-term memory to adapt to a student's immediate learning needs and questions, while long-term memory stores the student's progress, strengths, and weaknesses over time, enabling tailored educational experiences.
Importance of Memory in LLM-Powered Autonomous Agents
Memory plays a pivotal role in the effectiveness and adaptability of LLM-powered autonomous agents. Here's why it is crucial:
In-Context Learning: Short-term memory enables agents to utilize recent information to make informed decisions and generate accurate responses. This capability is vital for maintaining continuity and relevance in interactions, especially in dynamic and multi-step tasks.
Information Retention: Long-term memory allows agents to store knowledge and experiences over extended periods. This retention is essential for building a comprehensive understanding of various domains, improving problem-solving skills, and enhancing overall performance. By retaining information, agents can draw upon past experiences to navigate new challenges more effectively.
Component Three: Tool Utilization
LLM-powered autonomous agents extend their capabilities by interacting with external APIs, which allows them to access real-time data, execute code, and retrieve information from specialized databases.
This interaction significantly enhances their functionality beyond their pre-trained knowledge. By leveraging APIs, these agents can:
Access up-to-date information that is not part of their initial training data.
Perform specific tasks that require external computational resources or data.
Integrate specialized functions and tools, thereby increasing their versatility and applicability in various domains.
Examples of MRKL Architecture and Specific Projects like TAL and Toolformer
To better understand how LLM agents utilize external tools, let's look at some specific architectures and projects:
MRKL Architecture (Karpas et al. 2022): MRKL stands for Modular Reasoning, Knowledge, and Language. It is a neuro-symbolic architecture designed to enhance the reasoning capabilities of LLMs by integrating various expert modules. In MRKL systems, the LLM acts as a router, directing inquiries to the appropriate expert module, whether it be a neural network, symbolic system, or other specialized tools. This modular approach allows the agent to perform complex reasoning tasks more effectively.
TAL (Tool Augmented Language Models) (Parisi et al., 2022): TAL involves fine-tuning LLMs to learn how to call external APIs. The model is trained to recognize when and how to use these APIs to improve the quality of its outputs. By incorporating API calls into its responses, TAL can provide more accurate and contextually relevant information.
Toolformer (Schick et al., 2023): Similar to TAL, Toolformer is a project where LLMs are fine-tuned to use external tools automatically. The training process includes adding API call annotations to the dataset, allowing the model to learn when and how to make these calls to enhance its performance. Toolformer showcases the practical implementation of tool use in LLM agents, demonstrating significant improvements in task execution.
Significance of External Tools in Enhancing Problem-Solving Capabilities
The use of external tools is crucial in elevating the problem-solving abilities of LLM-powered autonomous agents. Here is why:
Enhanced Data Access: External tools allow agents to access a broader range of data sources, including real-time information and proprietary databases, which are essential for making informed decisions.
Specialized Functionality: By integrating with tools designed for specific tasks, such as mathematical computation, data analysis, or language translation, agents can perform these tasks more accurately and efficiently.
Adaptability: The ability to use external tools makes agents more adaptable to different scenarios and requirements. They can switch between various tools depending on the task at hand, providing a more versatile and robust solution.
Incorporating external tools transforms LLM agents from mere language processors into comprehensive problem solvers. This capability enables them to tackle a wide array of tasks, from simple information retrieval to complex data analysis, making them invaluable assets in both professional and personal applications.
LLM Powered Autonomous Agents: Capabilities and Applications
The difference between LLM-powered autonomous agents and traditional large language models (LLMs) lies in the range and complexity of tasks they can perform autonomously.
Traditional LLMs are primarily designed to generate text and respond to queries based on pre-trained data. They excel at understanding language and producing coherent responses but lack the ability to take meaningful actions or make decisions beyond text generation.
In contrast, LLM-powered autonomous agents integrate advanced functionalities such as reasoning, planning, memory, and tool use, enabling them to execute tasks independently. They can:
Plan and execute multi-step tasks: Decompose complex projects into manageable subgoals and follow through with action plans.
Interact with external systems: Use APIs to access real-time data, execute commands, and integrate specialized tools.
Learn and adapt: Utilize short-term and long-term memory to retain information and improve performance over time.
Make informed decisions: Apply reasoning capabilities to choose the best course of action based on context and available data.
This expanded spectrum of tasks makes autonomous agents far more versatile and capable than traditional LLMs.
Practical Applications of Agents
LLM-powered autonomous agents are revolutionizing various industries by enhancing efficiency and effectiveness in numerous applications:
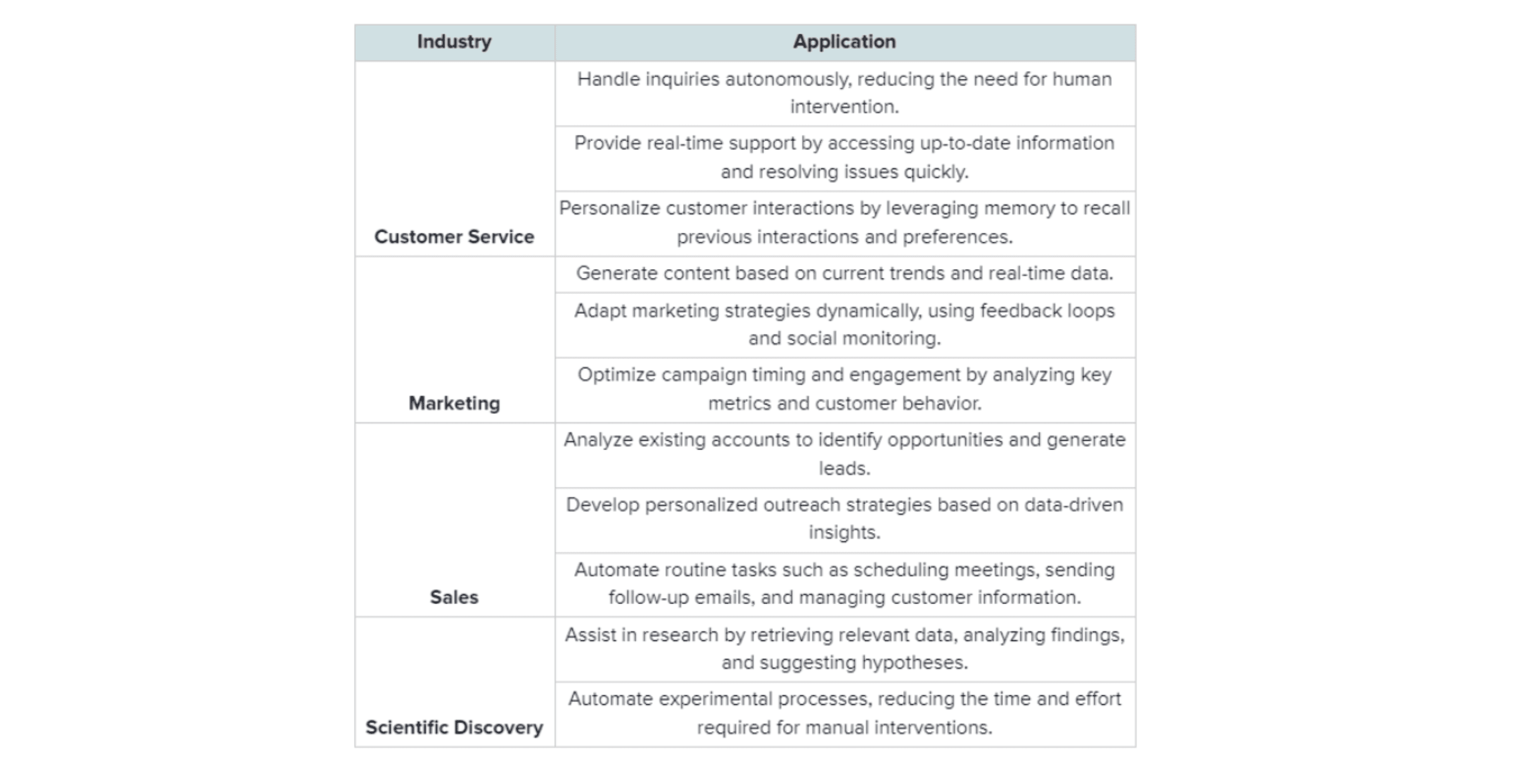
Pic: Industry Specific Applications for LLM Powered Autonoumous Agents
Autonomy in Task Execution Leading from Agents
The autonomy of LLM-powered agents in task execution is driving significant advancements in productivity and automation. By independently handling complex and routine tasks, these agents free up human resources to focus on higher-level strategic activities. Key benefits include:
Increased Efficiency: Autonomous agents can operate continuously without fatigue, ensuring tasks are completed faster and more accurately.
Reduced Costs: Automating routine and repetitive tasks reduces the need for extensive human labor, leading to cost savings.
Scalability: Agents can handle large volumes of tasks simultaneously, making it easier to scale operations without proportional increases in human resources.
Innovation: With routine tasks automated, humans can focus on creative and innovative aspects of their work, driving further advancements and improvements.
In summary, the capabilities and applications of LLM-powered autonomous agents are transforming industries by enhancing efficiency, reducing costs, and enabling more innovative and productive work environments.
LLM-Powered Autonomous Agents: Challenges and Future Directions
While LLM-powered autonomous agents represent a significant advancement in AI, they come with their own set of challenges:
Finite Context Length: One of the primary limitations is the finite context length of LLMs. This restricts the amount of historical information and detailed instructions that the agent can process at any given time, which can impact its ability to handle long and complex tasks effectively.
Long-Term Planning Difficulties: Despite improvements, long-term planning remains a challenge. Agents can struggle to maintain coherence and effectiveness over extended tasks, particularly those requiring intricate, multi-step processes.
Reliability of Natural Language Interfaces: The natural language interface, while powerful, is not infallible. Agents may occasionally produce incorrect or ambiguous outputs, make formatting errors, or misinterpret complex instructions. This can lead to issues in task execution and reduce the reliability of the agents.
LLM Powered Autonomous Agents: Future
The future development of LLM-powered autonomous agents is likely to focus on enhancing their autonomy and expanding their capabilities:
Extended Context Lengths: Improvements in AI architecture could allow agents to process more information, enhancing their ability to handle long-term tasks.
Improved Long-Term Planning: Advanced planning algorithms and learning techniques will help agents manage complex tasks more effectively.
Enhanced Natural Language Understanding: Better natural language processing will make agents more reliable in interpreting and executing complex instructions.
Advanced Tool Integration: Agents will become better at utilizing a wider range of external tools, increasing their versatility.
Evolving Landscape of AI and Potential Future Impact
The landscape of AI applications and impacts will continue to grow:
Broader Adoption: LLM-powered agents will be adopted across more industries, automating and enhancing complex tasks.
Human-AI Collaboration: Agents will take on more routine and complex tasks, allowing humans to focus on strategic and creative endeavors.
Ethical and Regulatory Considerations: There will be a greater emphasis on ensuring transparency, fairness, and compliance with global standards.
Innovation and Advancements: Ongoing AI research will lead to the development of more sophisticated agents, improving cognitive functions and decision-making.
Conclusion
In conclusion, LLM-powered autonomous agents represent a transformative leap in AI technology. They combine language processing with advanced capabilities like planning, memory, and tool use. These agents can handle complex tasks, make informed decisions, and interact dynamically with their environments, offering a versatile solution for various industries.
RAGA AI leverages these advancements to ensure the quality and consistency of AI applications through its comprehensive testing platform.
By detecting, diagnosing, and fixing AI issues autonomously, RAGA AI accelerates the development lifecycle and reduces risk. Sign up for our comprehensive RagaAI Testing Platform today.
Imagine having an AI that doesn't just talk but takes action, solves problems, and improves on its own. When you dive into the world of artificial intelligence, you'll quickly encounter one of the most groundbreaking advancements: LLM-powered autonomous agents.
These agents are more than just sophisticated chatbots; they are action-takers, problem-solvers, and efficiency enhancers. By blending the language prowess of Large Language Models (LLMs) with the capability to detect, diagnose, and fix issues, these agents transform how we interact with AI.
Gone are the days of static LLMs that only generate text. Now, we have dynamic agents that actively improve their environments, setting the stage for smarter, faster, and more reliable AI systems. Let's understand the concept of LLM powered autonomous Agents.
LLM Powered Autonomous Agents: Overview
LLM-Powered Autonomous Agents are advanced AI systems that combine the language capabilities of Large Language Models (LLMs) with functionalities like reasoning, planning, tool use, and memory. When you hear about LLM-powered autonomous agents, think of them as supercharged versions of the AI models you already know.
These agents do more than understand and generate text. By integrating capabilities such as reasoning, specific knowledge, planning, tool use, and memory, they move from passive responders to active participants in various processes. To maintain their effectiveness, it's essential to understand how to detect and fix AI issues.
They take meaningful actions based on their understanding, allowing them to solve complex problems, streamline tasks, and improve outcomes.
Transition from LLMs to Autonomous Agents
The shift from standalone Large Language Models (LLMs) to autonomous agents marks a game-changing moment in AI development. Initially, LLMs like OpenAI’s GPT-4 or Google’s Gemini were impressive for their ability to generate human-like text.
These models could provide insightful responses and assist with a variety of language tasks.
However, their capabilities were limited to text generation, and they lacked the ability to take action or make decisions based on their outputs. So, a more dynamic approach was needed, leading to the development of LLM-powered autonomous agents.
These agents not only generate text but can also perform other tasks.
Because of this, autonomous agents are becoming invaluable in various fields, from customer service and marketing to scientific research and beyond. They significantly increase productivity and reduce the need for human intervention. A comprehensive LLM evaluation framework can help measure and enhance the performance of these autonomous agents.
Traditional Large Language Models (LLMs) vs Autonomous Agents
Traditional LLMs are sophisticated text generators. They excel at understanding and producing language but fall short when it comes to taking action or making decisions. In contrast, LLM-powered autonomous agents integrate several advanced functionalities:
Reasoning: They can think through problems and come up with solutions.
Specific Knowledge: They utilize domain-specific information to enhance their responses.
Planning: They break down tasks into manageable steps and follow through.
Tool Use: They can access and use external tools and databases via APIs.
Memory: They have both short-term and long-term memory, allowing them to learn from past interactions and store information for future use.
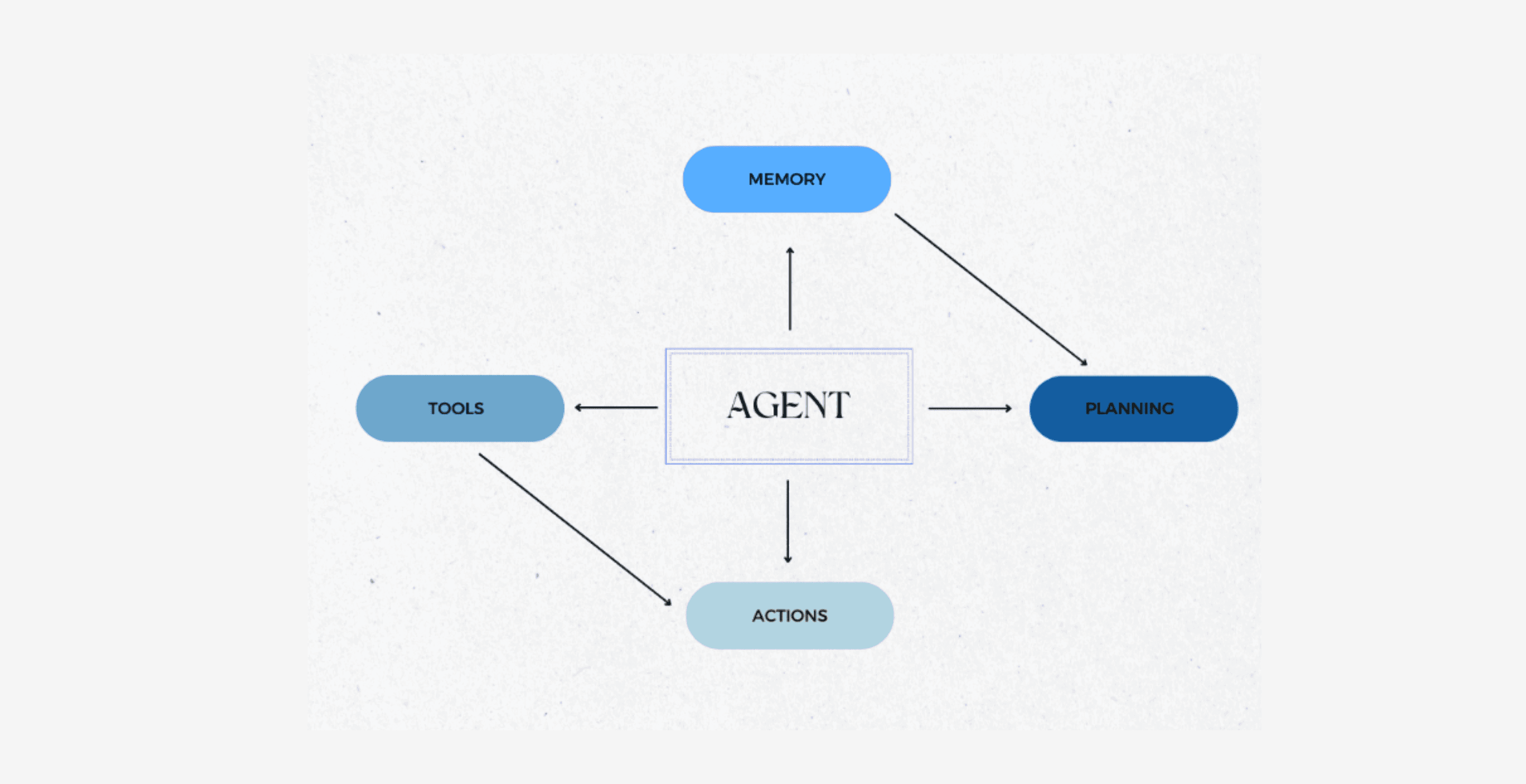
These enhancements allow agents to perform complex tasks that traditional LLMs cannot handle.
LLMs Beyond Simple Command-Response Mechanisms
The true power of LLM-powered autonomous agents lies in their expanded capabilities. Traditional LLMs operate on a simple command-response basis—you ask a question, and they provide an answer.
As previously explained, autonomous agents go far beyond this. They can interpret your instructions, create a plan of action, and execute that plan, all while using their built-in reasoning and memory capabilities to adapt and improve.
This means you can rely on them to handle more intricate tasks, such as managing workflows, optimizing processes, and even making data-driven decisions. For further insights on maintaining ethical standards, it is essential to understand the details of how correct AI governance is performed.
LLM Powered Autonomous Agents: Core Components
As explained above, LLM-powered autonomous agents are sophisticated advancements in artificial intelligence that combine the capabilities of large language models (LLMs) with additional functionalities like reasoning, planning, memory, and tool use.
They can not only generate and understand text but also execute tasks, make decisions, and interact dynamically with their environments. Evaluating and monitoring the performance of these agents is crucial for ensuring reliability, as outlined in the guide to evaluating LLM applications and enabling guardrails.
Let's understand the core components of the LLM-powered autonomous agents. They are as follows:
Planning
Planning is a fundamental component of LLM-powered autonomous agents. You can understand the overview of the Planning component from the bullet points provided below:
Task Decomposition: Agents break down complex tasks into smaller, manageable subgoals. This step-by-step approach simplifies the execution of intricate projects. It ensures that each segment of the task is handled effectively.
Self-Reflection: Advanced agents analyze their past actions. They learn from mistakes to refine their strategies. This continuous improvement mechanism enhances the quality of outcomes, increasing their ability to tackle increasingly complex tasks.
Memory
Memory is crucial for the effective functioning of LLM-powered autonomous agents. It is typically divided into short-term and long-term memory:
Short-Term Memory: This type of memory allows agents to retain and manipulate information relevant to the immediate task at hand, similar to how humans use short-term memory to recall recent events or information.
Long-Term Memory: This enables agents to store vast amounts of information over extended periods, often through external storage systems. It acts like a library of knowledge that the agent can access when necessary, supporting more informed decision-making and task execution.
The combination of these memory types allows agents to adapt to new information, learn from past experiences, and maintain a robust knowledge base.
Tools Usage:
Tool use is the added capability embedded in the LLM-powered autonomous agents that gives them the ability to augment capabilities. Tools usage can be summarized into two bullet points:
Extended Functionality: LLM-powered autonomous agents interact with external tools via APIs, accessing real-time data, executing code, and utilizing specialized databases.
Versatility: This ability to use tools transforms LLM-powered autonomous agents from mere language processors into versatile problem solvers, adapting to various scenarios and requirements.
Action
The decision-making process in LLM-powered autonomous agents is pivotal to their operation. When an agent decides to act, it can be either internal or external:
Internal Actions: These are confined to the agent's thought processes, such as generating and refining responses.
External Actions: These involve tangible outputs, like sending emails, managing data, or interacting with other systems.
The ability to choose and execute the appropriate type of action based on the context and objectives is a key feature that sets autonomous agents apart from traditional LLMs. This dual nature of actions allows them to be effective in a wide array of applications, from customer service to complex data analysis.
The overview of the components of an LLM-powered autonomous agent is shown below for your reference.

Pic: Overview of an LLM-powered autonomous agent with its system (Image source: Weng, Lilian. 2023)
Agent Component One: Planning
Planning plays a pivotal role in the operation of an LLM-powered autonomous agent. It's not just about understanding the steps involved in a complex task but also about strategizing for efficient execution. This is crucial for the development of effective strategies in LLM-powered autonomous agents.
By breaking down complex tasks into manageable steps, agents can approach intricate projects systematically. Let's understand the techniques involved in the "task decomposition" part of the Planning component used by LLM-powered autonomous agents.
Task Decomposition
Chain of thought (CoT; Wei et al., 2022) involves guiding the agent to think step-by-step, breaking down a task into a sequence of smaller, logical steps. It enhances the agent's performance by making its thought process transparent and methodical.
This technique has become a standard prompting technique for enhancing model performance on complex tasks. CoT transforms big tasks into multiple manageable tasks and sheds light on an interpretation of the model's thinking process.
Tree of Thoughts (Yao et al., 2023) is a further refinement of CoT and extends it by exploring multiple reasoning paths at each step, creating a tree structure. This technique explores multiple reasoning possibilities at each step. Under this, the task/problem is first decomposed into multiple thought steps, out of which multiple thoughts per step are generated, which creates a tree structure.
Each branch under the Tree of Thoughts represents a different potential solution, allowing the agent to evaluate various approaches and choose the most effective one. The search procedure offers both breadth-first search (BFS) and depth-first search (DFS) options. A classifier (via a prompt) or majority vote can assess each state.
Task decomposition under the Planning component can happen through the following methods:
(1) by LLM using straightforward questions such as "What are the subgoals for achieving XYZ?" and "Steps for XYZ. 1."
Using task-specific instructions, e.g. "Write a blog outline." for writing a specific topic
Using human inputs
Continuous Improvement Through Self-Reflection
LLM-powered autonomous agents continuously improve through the process of self-reflection, which plays a crucial role. Two key frameworks that facilitate self-reflection are ReAct and Reflexion:
ReAct Framework
Reflexion Framework
Let us understand both of these frameworks in detail.
ReAct Framework
ReAct Framework (Yao et al. 2023) Integrates reasoning and action, enabling agents to generate reasoning traces and perform task-specific actions. Reasoning enables the agent to interact with the environment (e.g., use Wikipedia search API). Acting prompts the powered agent to generate reasoning traces in natural language. This combination helps refine the agents' responses and actions based on real-time feedback.
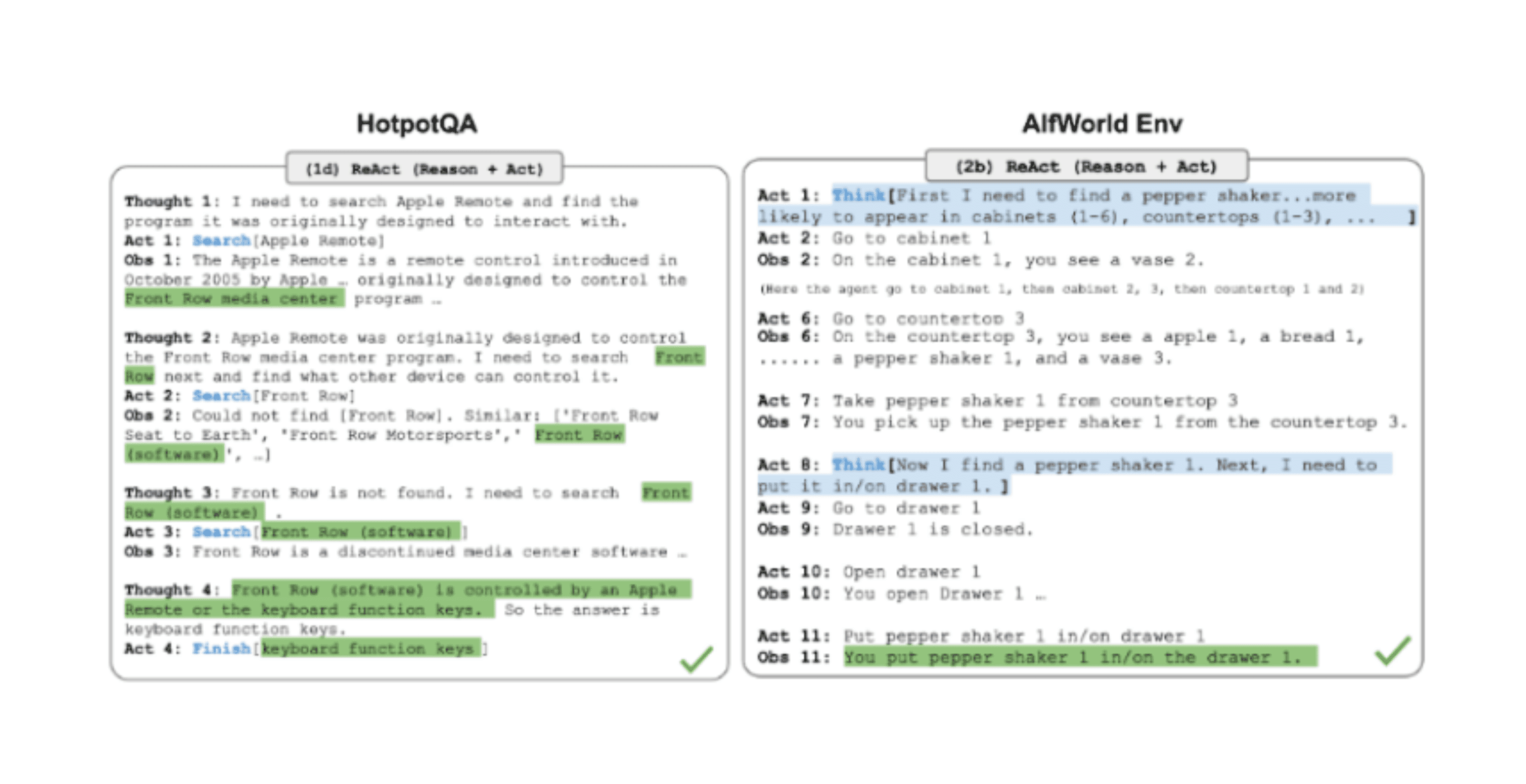
Pic: Examples of reasoning trajectories for knowledge-intensive tasks (e.g. HotpotQA, FEVER) and decision-making tasks (e.g. AlfWorld Env, WebShop). (Image source: Yao et al. 2023).
Reflexion Framework
Reflexion (Shinn & Labash, 2023) equips agents with dynamic memory and self-reflection capabilities, which improve their reasoning skills. This framework equips agents with dynamic memory and self-reflection capabilities. By analyzing past actions and outcomes, agents can identify errors and inefficiencies, allowing them to adjust their strategies and improve future performance.
Reflexion uses a basic reinforcement learning architecture, where the task-specific action space is enhanced with language to facilitate sophisticated reasoning stages. At the same time, the reward model offers a straightforward binary payout. Following every action, the agent calculates a heuristic and may choose to reset the environment to begin a new trial based on the outcomes of its self-reflection.
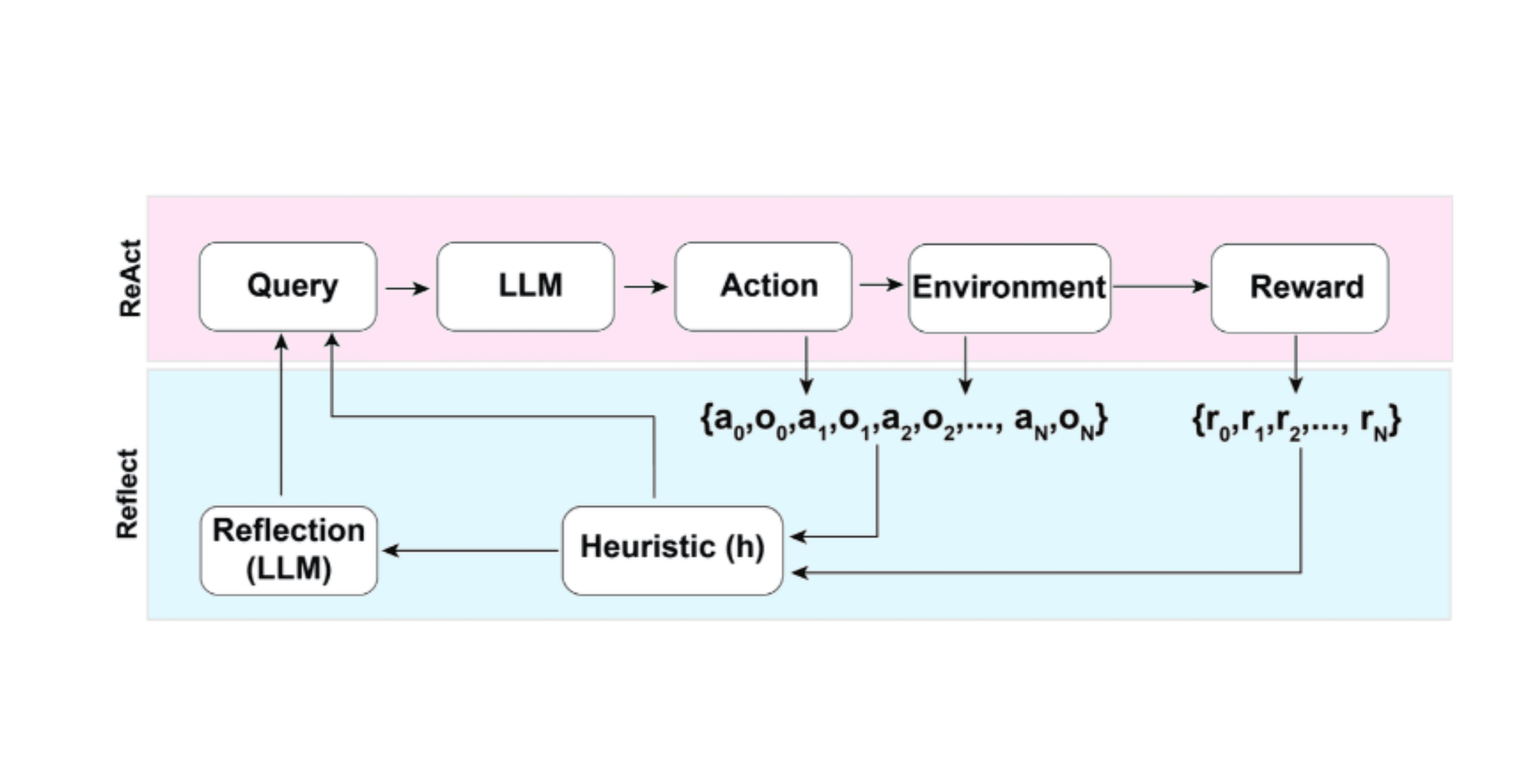
Pic: Illustration of the Reflexion framework. (Image source: Shinn & Labash, 2023)
Enhanced Long-Horizon Planning: Integrating Classical Planners with LLMs
For more complex, long-horizon tasks, integrating classical planners with LLMs can enhance planning capabilities for complex, long-horizon tasks. This approach involves translating the problem into PDDL, using an external classical planner to generate a detailed plan, and then translating the plan back into natural language for the agent to execute.
Translation to PDDL: The LLM translates a given problem into a PDDL format, defining the planning problem in a structured way.
Classical Planning: An external classical planner generates a detailed plan based on the PDDL description, utilizing domain-specific knowledge and algorithms.
Translation Back to Natural Language: The LLM translates the generated plan back into natural language, making it actionable for the agent.
Agent Component Two: Memory
Memory management is a cornerstone of LLM-powered autonomous agents, enabling them to store and recall information crucial for their operations. Memory in LLM-powered autonomous agents serves the same purpose as it does in a human brain.
Let's understand the types of memory in both the context of Human Memory and LLM-powered autonomous agents.
Classification of Memory: Human Brain
The procedures required to gather, store, hold onto, and then retrieve information are collectively referred to as memory. Human brains have multiple types of memory.
Human memory is broadly understood under the classification of:
Sensory Memory: The ability to hold onto impressions of sensory information (visual, aural, etc.) after the initial stimulation has finished is known as sensory memory, which is the first stage of memory. Usually, sensory memory is limited to a few seconds. There are three subcategories: haptic memory (touch), auditory echoic memory, and iconic memory (visual).
Short-Term Memory (STM) or Working Memory: It retains knowledge that we now possess and is required to perform sophisticated mental operations like learning and reasoning. According to Miller (1956), short-term memory may hold seven items and last 20 to 30 seconds.
Long-Term Memory (LTM): Information can be stored in long-term memory for a very long period—from a few days to decades—and it has practically infinite storage space. LTM comes in two subtypes:
Explicit/declarative memory: This memory refers to the consciously recalled recollections of facts and events, such as episodic memory (experiences and events) and semantic memory (concepts and facts).
Implicit/procedural memory: This kind of memory is unconscious and pertains to automatic skills and routines, such as riding a bike or using a keyboard.
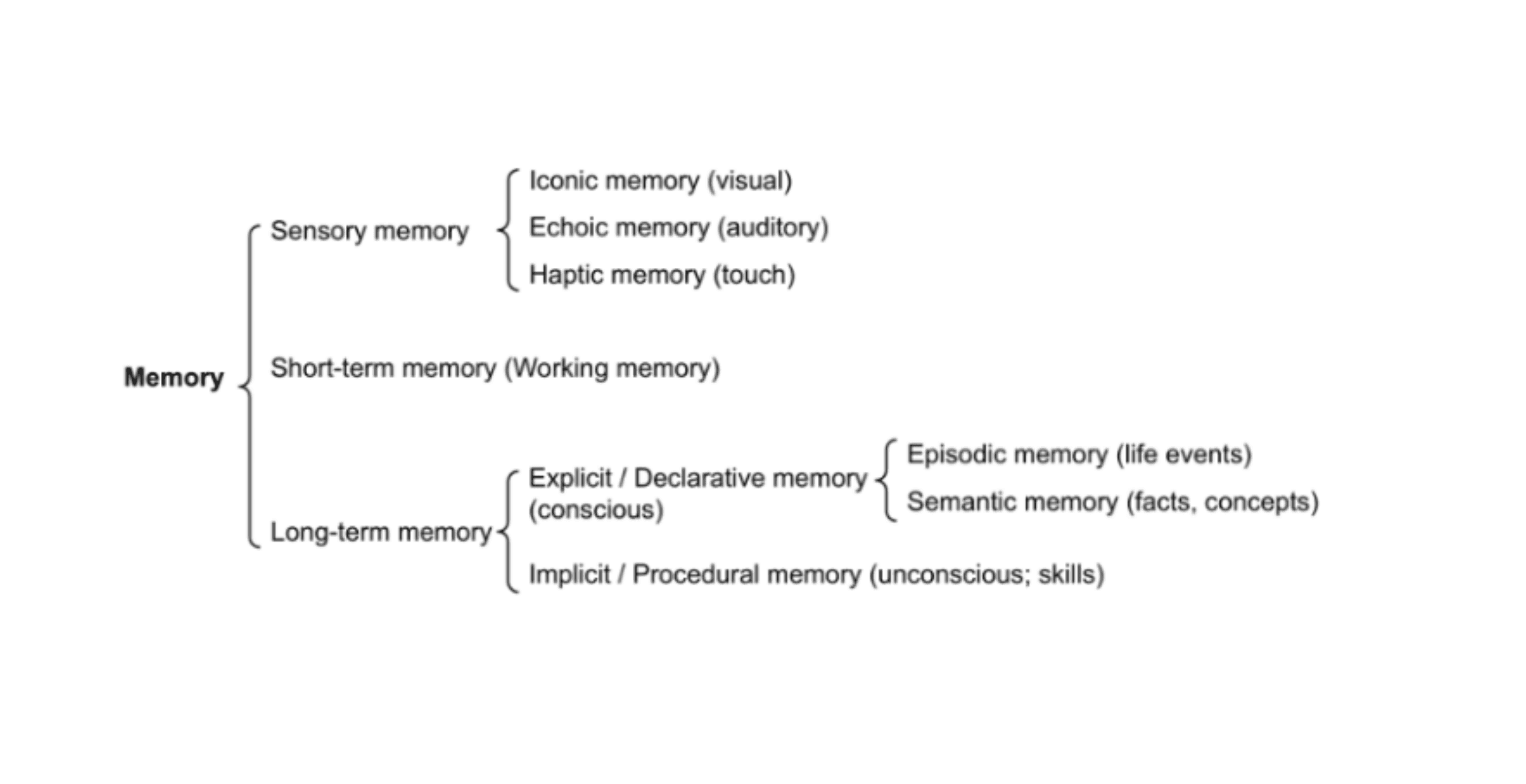
Pic: Classification and Categorization of Human Memory
Classification of Memory: LLM-Powered Autonomous Agents
Now that you have understood the classification of memory as per the human brain, it becomes easier to understand how memory is classified in the context of LLM-Powered Autonomous Agents.
The classifications are as follows:
Short-Term Memory (STM): This type of memory allows agents to hold and manipulate information relevant to the immediate task at hand. It is similar to how humans remember recent events or data points for short periods. STM is crucial for in-context learning, where the agent uses recent interactions to inform its current actions and responses
Long-term Memory (LTM): LTM enables agents to store vast amounts of information over extended periods. External storage systems often facilitate this memory, acting as repositories of knowledge that the agent can access as needed. LTM is essential for retaining information that might be required for future tasks or for drawing upon past experiences to improve performance.
Practical Implementation of Memory For Agents
In practical applications, implementing memory in LLM-powered autonomous agents often involves the use of external vector stores.
These stores are specialized databases that efficiently manage and retrieve large volumes of data. Here are a few examples:
Customer Support Systems: Agents can use short-term memory to handle ongoing customer interactions effectively, while long-term memory stores customer history, preferences, and past interactions, allowing the agent to provide personalized support.
Research and Development: In scientific research, agents can store and recall extensive datasets, research papers, and experimental results. Short-term memory helps in handling current research tasks, while long-term memory retains valuable historical data for future reference.
Educational Tools: Educational agents can use short-term memory to adapt to a student's immediate learning needs and questions, while long-term memory stores the student's progress, strengths, and weaknesses over time, enabling tailored educational experiences.
Importance of Memory in LLM-Powered Autonomous Agents
Memory plays a pivotal role in the effectiveness and adaptability of LLM-powered autonomous agents. Here's why it is crucial:
In-Context Learning: Short-term memory enables agents to utilize recent information to make informed decisions and generate accurate responses. This capability is vital for maintaining continuity and relevance in interactions, especially in dynamic and multi-step tasks.
Information Retention: Long-term memory allows agents to store knowledge and experiences over extended periods. This retention is essential for building a comprehensive understanding of various domains, improving problem-solving skills, and enhancing overall performance. By retaining information, agents can draw upon past experiences to navigate new challenges more effectively.
Component Three: Tool Utilization
LLM-powered autonomous agents extend their capabilities by interacting with external APIs, which allows them to access real-time data, execute code, and retrieve information from specialized databases.
This interaction significantly enhances their functionality beyond their pre-trained knowledge. By leveraging APIs, these agents can:
Access up-to-date information that is not part of their initial training data.
Perform specific tasks that require external computational resources or data.
Integrate specialized functions and tools, thereby increasing their versatility and applicability in various domains.
Examples of MRKL Architecture and Specific Projects like TAL and Toolformer
To better understand how LLM agents utilize external tools, let's look at some specific architectures and projects:
MRKL Architecture (Karpas et al. 2022): MRKL stands for Modular Reasoning, Knowledge, and Language. It is a neuro-symbolic architecture designed to enhance the reasoning capabilities of LLMs by integrating various expert modules. In MRKL systems, the LLM acts as a router, directing inquiries to the appropriate expert module, whether it be a neural network, symbolic system, or other specialized tools. This modular approach allows the agent to perform complex reasoning tasks more effectively.
TAL (Tool Augmented Language Models) (Parisi et al., 2022): TAL involves fine-tuning LLMs to learn how to call external APIs. The model is trained to recognize when and how to use these APIs to improve the quality of its outputs. By incorporating API calls into its responses, TAL can provide more accurate and contextually relevant information.
Toolformer (Schick et al., 2023): Similar to TAL, Toolformer is a project where LLMs are fine-tuned to use external tools automatically. The training process includes adding API call annotations to the dataset, allowing the model to learn when and how to make these calls to enhance its performance. Toolformer showcases the practical implementation of tool use in LLM agents, demonstrating significant improvements in task execution.
Significance of External Tools in Enhancing Problem-Solving Capabilities
The use of external tools is crucial in elevating the problem-solving abilities of LLM-powered autonomous agents. Here is why:
Enhanced Data Access: External tools allow agents to access a broader range of data sources, including real-time information and proprietary databases, which are essential for making informed decisions.
Specialized Functionality: By integrating with tools designed for specific tasks, such as mathematical computation, data analysis, or language translation, agents can perform these tasks more accurately and efficiently.
Adaptability: The ability to use external tools makes agents more adaptable to different scenarios and requirements. They can switch between various tools depending on the task at hand, providing a more versatile and robust solution.
Incorporating external tools transforms LLM agents from mere language processors into comprehensive problem solvers. This capability enables them to tackle a wide array of tasks, from simple information retrieval to complex data analysis, making them invaluable assets in both professional and personal applications.
LLM Powered Autonomous Agents: Capabilities and Applications
The difference between LLM-powered autonomous agents and traditional large language models (LLMs) lies in the range and complexity of tasks they can perform autonomously.
Traditional LLMs are primarily designed to generate text and respond to queries based on pre-trained data. They excel at understanding language and producing coherent responses but lack the ability to take meaningful actions or make decisions beyond text generation.
In contrast, LLM-powered autonomous agents integrate advanced functionalities such as reasoning, planning, memory, and tool use, enabling them to execute tasks independently. They can:
Plan and execute multi-step tasks: Decompose complex projects into manageable subgoals and follow through with action plans.
Interact with external systems: Use APIs to access real-time data, execute commands, and integrate specialized tools.
Learn and adapt: Utilize short-term and long-term memory to retain information and improve performance over time.
Make informed decisions: Apply reasoning capabilities to choose the best course of action based on context and available data.
This expanded spectrum of tasks makes autonomous agents far more versatile and capable than traditional LLMs.
Practical Applications of Agents
LLM-powered autonomous agents are revolutionizing various industries by enhancing efficiency and effectiveness in numerous applications:
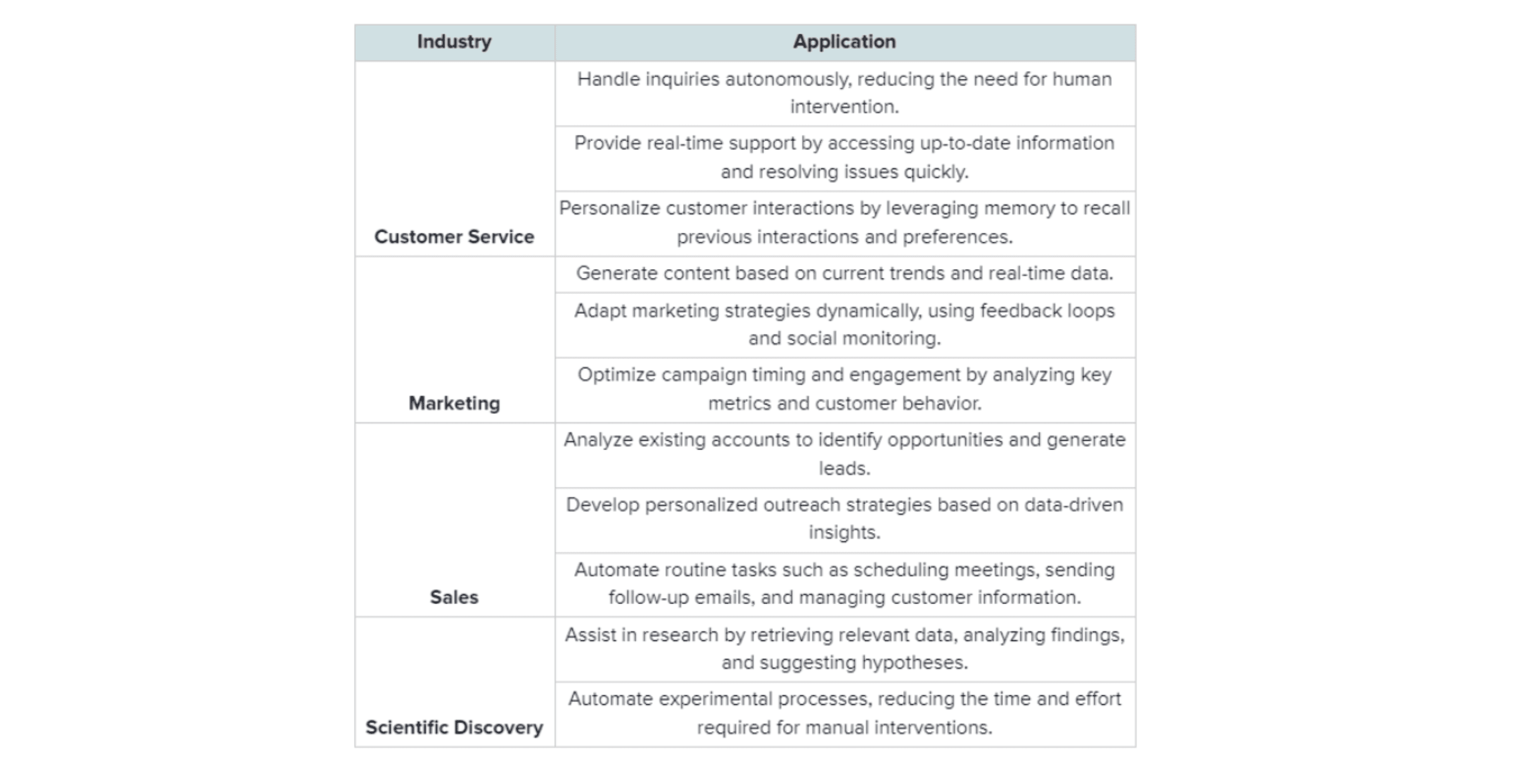
Pic: Industry Specific Applications for LLM Powered Autonoumous Agents
Autonomy in Task Execution Leading from Agents
The autonomy of LLM-powered agents in task execution is driving significant advancements in productivity and automation. By independently handling complex and routine tasks, these agents free up human resources to focus on higher-level strategic activities. Key benefits include:
Increased Efficiency: Autonomous agents can operate continuously without fatigue, ensuring tasks are completed faster and more accurately.
Reduced Costs: Automating routine and repetitive tasks reduces the need for extensive human labor, leading to cost savings.
Scalability: Agents can handle large volumes of tasks simultaneously, making it easier to scale operations without proportional increases in human resources.
Innovation: With routine tasks automated, humans can focus on creative and innovative aspects of their work, driving further advancements and improvements.
In summary, the capabilities and applications of LLM-powered autonomous agents are transforming industries by enhancing efficiency, reducing costs, and enabling more innovative and productive work environments.
LLM-Powered Autonomous Agents: Challenges and Future Directions
While LLM-powered autonomous agents represent a significant advancement in AI, they come with their own set of challenges:
Finite Context Length: One of the primary limitations is the finite context length of LLMs. This restricts the amount of historical information and detailed instructions that the agent can process at any given time, which can impact its ability to handle long and complex tasks effectively.
Long-Term Planning Difficulties: Despite improvements, long-term planning remains a challenge. Agents can struggle to maintain coherence and effectiveness over extended tasks, particularly those requiring intricate, multi-step processes.
Reliability of Natural Language Interfaces: The natural language interface, while powerful, is not infallible. Agents may occasionally produce incorrect or ambiguous outputs, make formatting errors, or misinterpret complex instructions. This can lead to issues in task execution and reduce the reliability of the agents.
LLM Powered Autonomous Agents: Future
The future development of LLM-powered autonomous agents is likely to focus on enhancing their autonomy and expanding their capabilities:
Extended Context Lengths: Improvements in AI architecture could allow agents to process more information, enhancing their ability to handle long-term tasks.
Improved Long-Term Planning: Advanced planning algorithms and learning techniques will help agents manage complex tasks more effectively.
Enhanced Natural Language Understanding: Better natural language processing will make agents more reliable in interpreting and executing complex instructions.
Advanced Tool Integration: Agents will become better at utilizing a wider range of external tools, increasing their versatility.
Evolving Landscape of AI and Potential Future Impact
The landscape of AI applications and impacts will continue to grow:
Broader Adoption: LLM-powered agents will be adopted across more industries, automating and enhancing complex tasks.
Human-AI Collaboration: Agents will take on more routine and complex tasks, allowing humans to focus on strategic and creative endeavors.
Ethical and Regulatory Considerations: There will be a greater emphasis on ensuring transparency, fairness, and compliance with global standards.
Innovation and Advancements: Ongoing AI research will lead to the development of more sophisticated agents, improving cognitive functions and decision-making.
Conclusion
In conclusion, LLM-powered autonomous agents represent a transformative leap in AI technology. They combine language processing with advanced capabilities like planning, memory, and tool use. These agents can handle complex tasks, make informed decisions, and interact dynamically with their environments, offering a versatile solution for various industries.
RAGA AI leverages these advancements to ensure the quality and consistency of AI applications through its comprehensive testing platform.
By detecting, diagnosing, and fixing AI issues autonomously, RAGA AI accelerates the development lifecycle and reduces risk. Sign up for our comprehensive RagaAI Testing Platform today.