Understanding The Basics Of AI Governance
Akshat Gupta
Apr 15, 2024
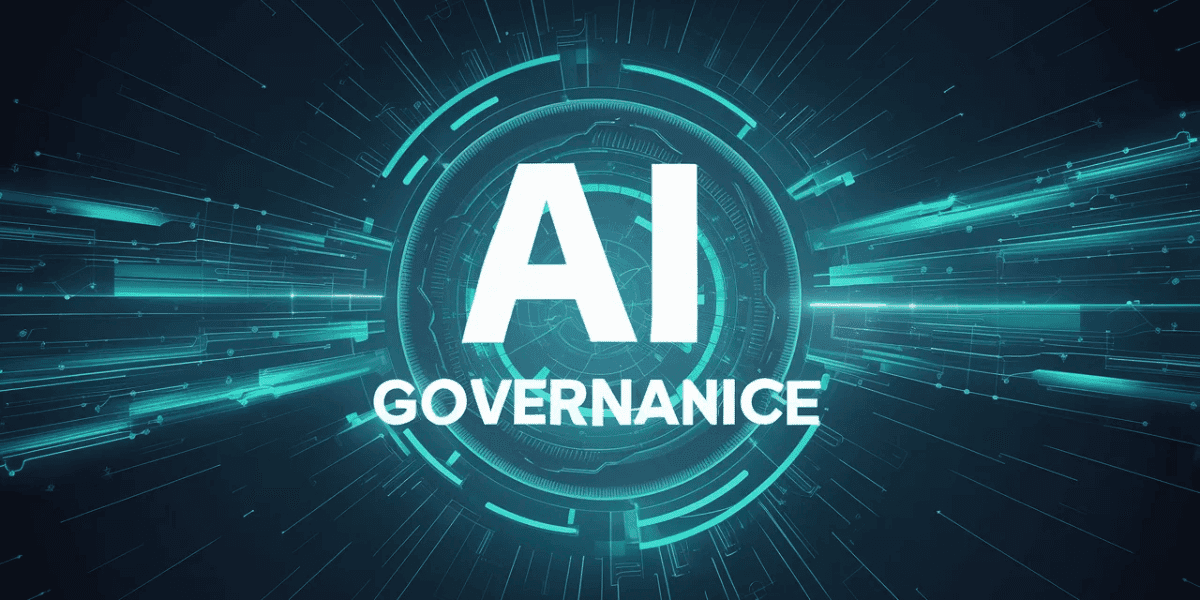
AI governance encompasses establishing frameworks, rules, and standards to ensure the ethical development and application of Artificial Intelligence technologies.
In today’s digital society, where AI systems play a crucial role in decision-making processes across various sectors, the significance of AI governance cannot be overstated.
It addresses critical oversight mechanisms for mitigating biases, ensuring Privacy, and preventing misuse while delineating the responsibilities of developers, policymakers, and ethicists in fostering responsible AI.
Technical Foundations of AI Governance
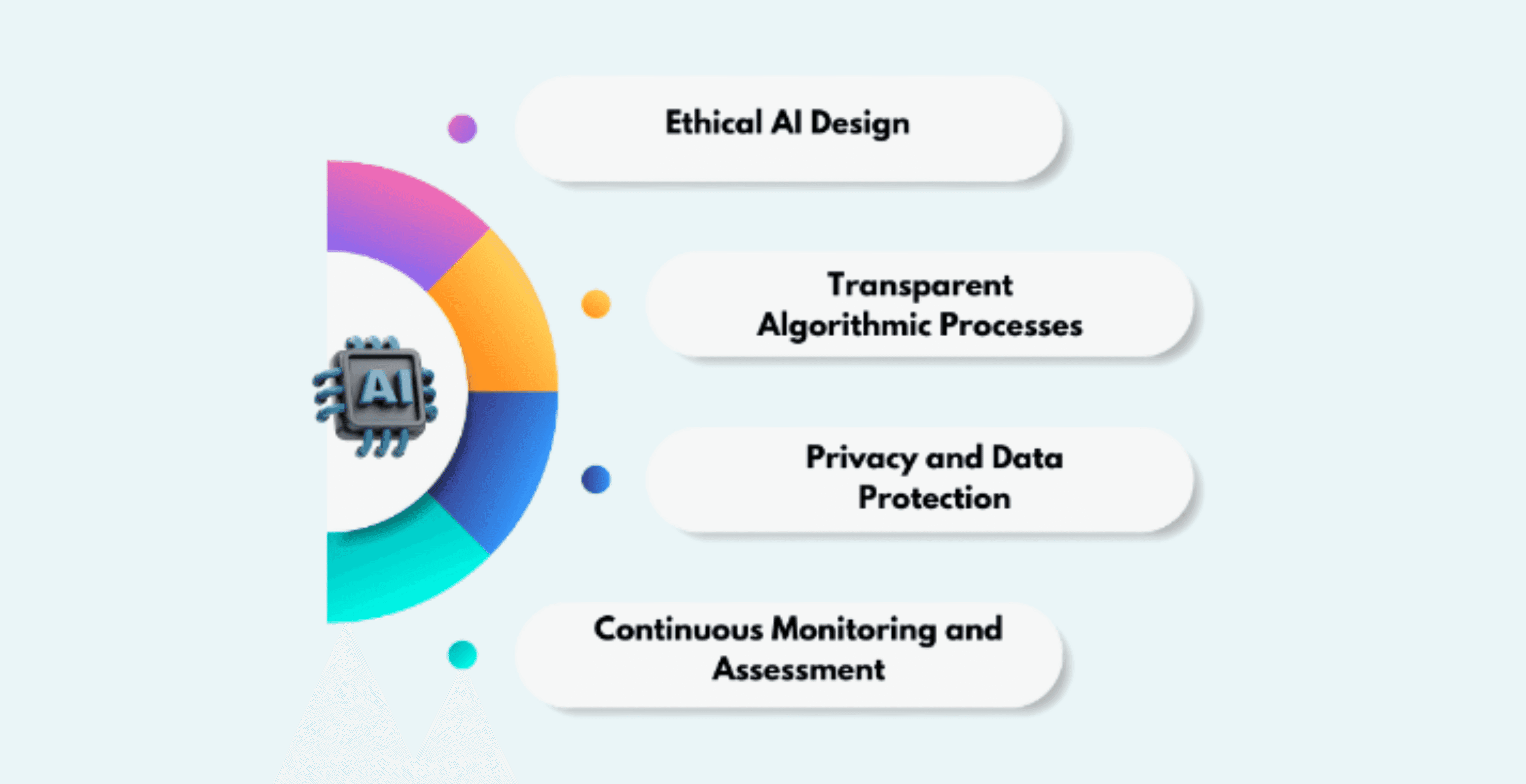
AI Governance is built upon several technical pillars that ensure AI systems are developed and operated under stringent ethical and operational guidelines:
Ethical AI Design: This involves incorporating ethical considerations into the AI design process, ensuring that AI systems are developed with safeguards against bias, discrimination, and other ethical pitfalls. It also involves integrating ethical decision-making algorithms and considering diverse stakeholder impacts during the design phase.
Transparent Algorithmic Processes: Advocates for developing AI systems with transparent, explainable algorithms. This transparency is crucial for auditing AI systems, understanding their decision-making processes, and ensuring accountability for the actions taken by AI systems. In addition, the algorithms need to be robust and reliable to ensure their performance.
Privacy and Data Protection: Enforces stringent data protection measures in AI systems, ensuring that personal data is processed securely, lawfully, and transparently. This includes adherence to global data protection regulations such as GDPR, implementing data anonymization techniques, and ensuring data is used solely for intended and consented purposes.
Continuous Monitoring and Assessment: Establishes ongoing monitoring mechanisms for AI systems, continuously assessing their performance, fairness, and impact on society. This includes using AI auditing tools, performance metrics, and feedback loops to identify and mitigate potential issues proactively.
Read more on A Guide to Evaluating LLM Applications and Enabling Guardrails Using Raga LLM Hub.
Societal Implications and Operational Frameworks
The societal implications of AI are profound, influencing everything from individual Privacy and employment to democracy and social justice. AI Governance seeks to navigate these implications through operational frameworks that balance innovation with societal welfare:
Regulatory Compliance: Aligns AI development and deployment with existing legal and regulatory frameworks. This involves compliance with data protection laws and emerging AI-specific regulations and standards that govern the use of AI in sensitive sectors.
Stakeholder Engagement: Emphasizes the importance of involving a broad spectrum of stakeholders in the AI Governance process. This includes engaging with policymakers, ethicists, civil society organizations, and the public to ensure diverse perspectives are considered in governance decisions.
Risk Management: Implements structured risk assessment and management processes for AI systems, identifying potential risks to individuals and society and establishing mitigation strategies. This encompasses identifying risks associated with bias, discrimination, Privacy breaches, and unintended consequences of AI applications.
Ethical AI Frameworks: Develop comprehensive ethical frameworks that guide AI development and use. These frameworks outline fairness, accountability, transparency, and privacy principles and are foundations for ethical AI practices.
Differentiating AI Governance from Data Governance
While AI and data governance intersect in several areas, they focus on distinct aspects of technology management. AI governance extends beyond data management to include considerations around the design, development, implementation, and operation of AI systems, emphasizing technical, legal, and ethical considerations.
In contrast, data governance primarily concerns data quality, management, and security, highlighting the broader scope of AI governance in addressing complex AI-specific challenges.
The following chart outlines key differences between AI Governance and Data Governance:
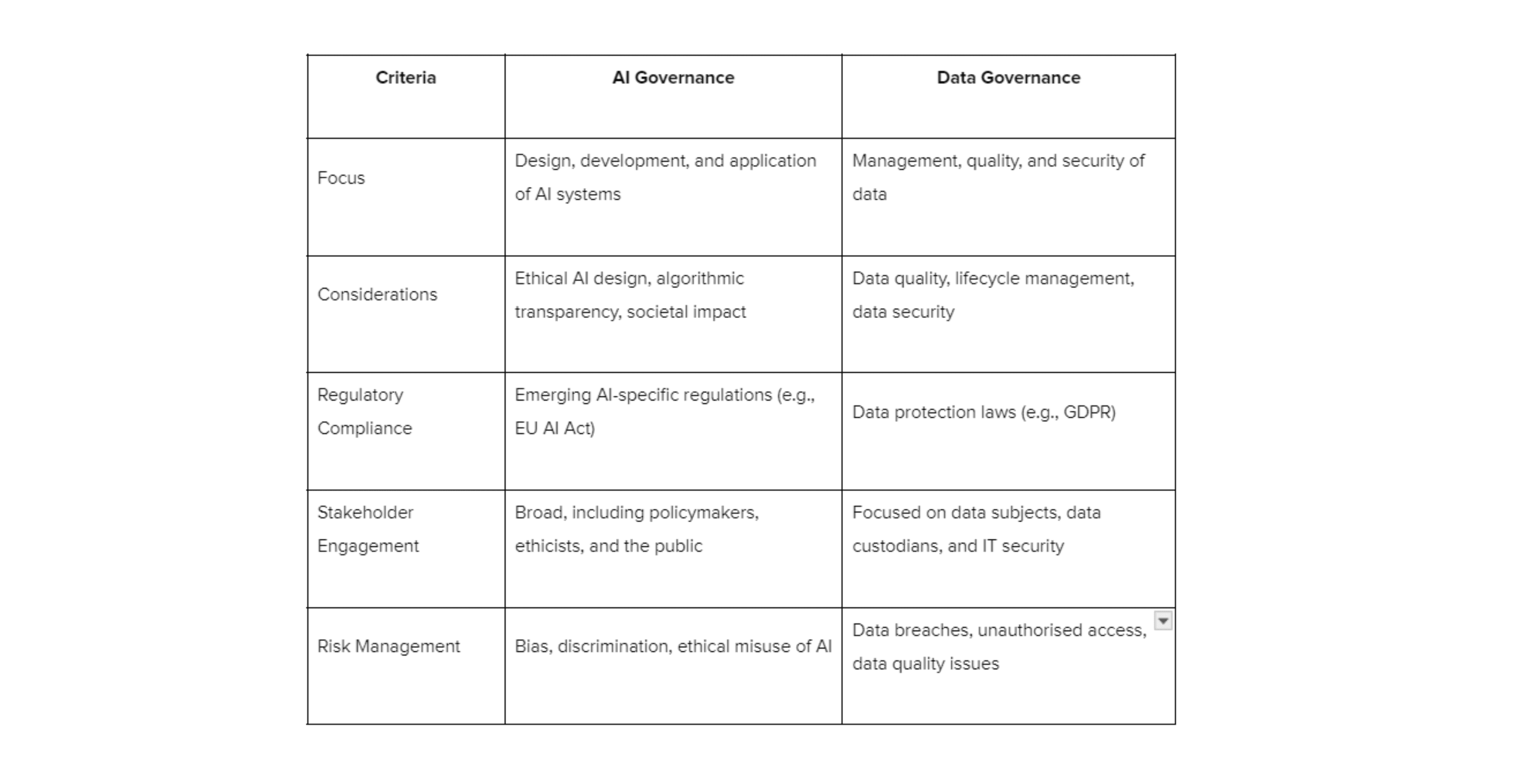
To get the essence of including ethical considerations from the start of AI projects, explore our insightful article on Advancing Geospatial Semantic Segmentation Through Automated Testing with RagaAI, which presents responsible AI development practices.
Components Of AI Governance
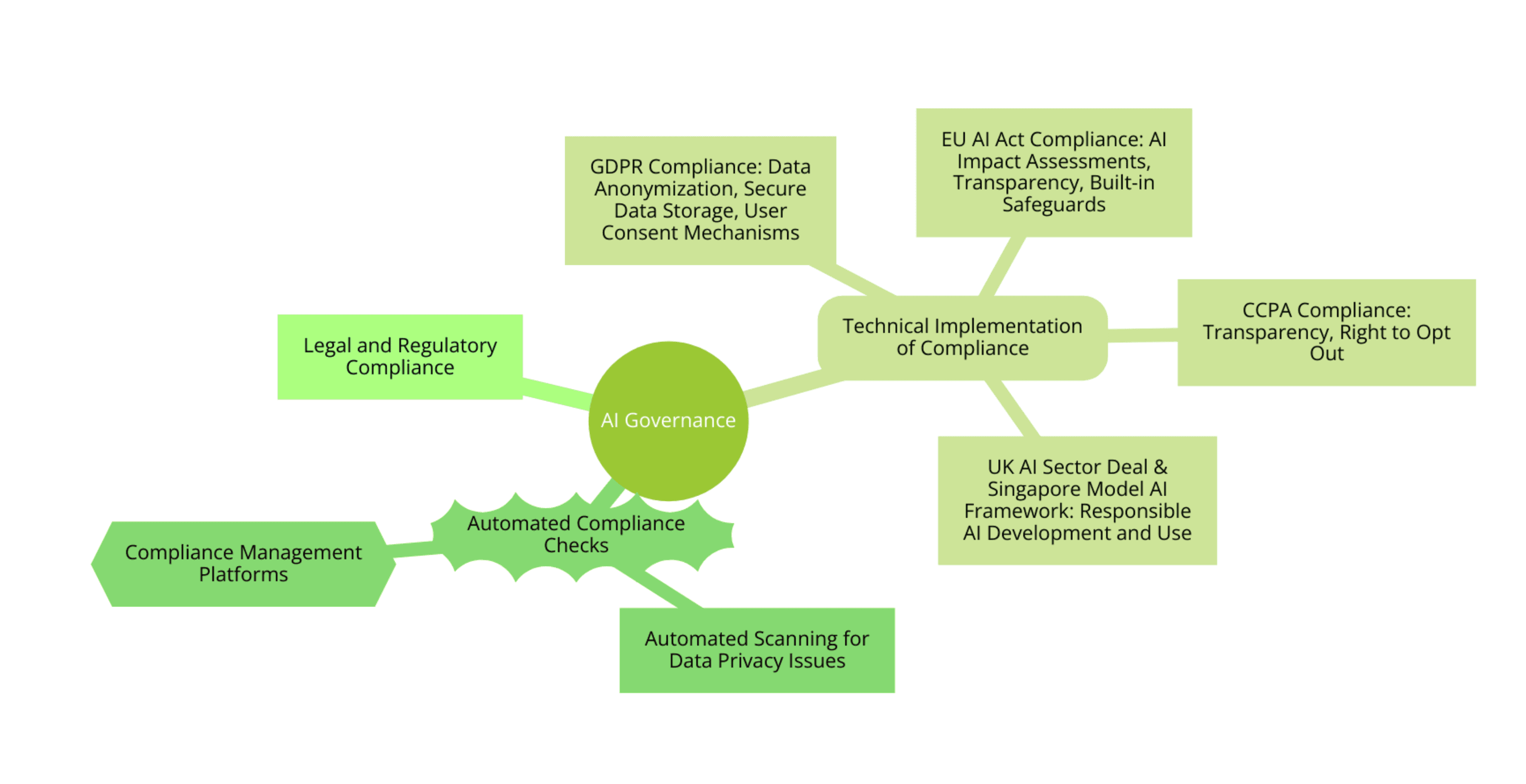
The backbone of effective AI governance lies in its components:
Legal and Regulatory Compliance
Technical Implementation of Compliance:
This involves integrating legal and regulatory requirements directly into the AI development lifecycle. For instance, ensuring GDPR compliance could involve technical measures like data anonymization, secure data storage, and processing protocols, alongside the implementation of user consent mechanisms for data collection and processing.
Compliance with the EU AI Act may require conducting AI impact assessments, adhering to transparency requirements, and ensuring AI systems are designed with built-in safeguards against potential misuse. California Consumer Privacy Act (CCPA) includes provisions related to businesses' collection, use, and sale of personal information, including transparency requirements and the right to opt out of the sale of personal information.
UK Government's AI Sector Deal aims to boost the UK's AI sector and ensure that AI is developed and used responsibly. Singapore's Model AI Governance Framework guides organizations in Singapore on how to develop and deploy AI systems responsibly and ethically
Automated Compliance Checks:
It utilizes automated tools to continuously monitor and verify the compliance of AI applications with relevant laws and regulations. This could include automated scanning of AI models to identify potential data Privacy issues or using compliance management platforms to track and manage regulatory obligations.
Ethical Considerations:
Ethical Frameworks and AI Ethics Committees:
Developing comprehensive ethical frameworks that outline fundamental principles and standards for AI development and use.
Establishing AI ethics committees within organizations can provide oversight, ensuring AI projects align with ethical guidelines. These committees can evaluate AI initiatives for potential ethical issues, provide guidance on moral dilemmas, and recommend corrective actions.
Impact Assessments:
They conduct ethical impact assessments for AI projects to identify and address potential societal impacts, biases, and moral risks. These assessments can inform the development process, guiding the design of AI systems that are fair, transparent, and aligned with societal values.
Read more on Navigating AI Governance: Building Trustworthy AI Amidst Evolving Regulations.
The Role of AI Governance in Risk Management
AI governance plays a pivotal role in risk management by structuring the monitoring of AI systems to mitigate human biases and errors. It ensures the responsible and ethical development and use of AI. It addresses risks related to discrimination, privacy violations, and potential harm, emphasizing the importance of structured oversight in safeguarding societal values.
Mitigating Human Biases and Errors
Bias Detection and Mitigation Tools: Implementing technical solutions to detect and mitigate biases in AI datasets and models. This can involve using bias detection algorithms that identify skewed data distributions or unfair model predictions, coupled with mitigation techniques like re-sampling, re-weighting, or algorithmic fairness interventions.
Diversity in AI Teams: Promoting diversity in AI development teams to bring a wide range of perspectives to designing and testing AI systems. Diverse teams are better equipped to identify potential biases and ethical issues, enhancing the fairness and inclusivity of AI applications.
Developing and Using AI Responsibly and Ethically
Responsible AI Development Practices: Adopt practices prioritizing ethical considerations, such as privacy-by-design, where Privacy safeguards are integrated at the design stage of AI projects. Implementing transparent AI development processes can also enhance accountability, allowing stakeholders to understand how AI systems make decisions.
Ethical AI Deployment: Ensuring the ethical deployment of AI involves continuous monitoring of AI applications in real-world settings to detect and address unintended consequences. Establishing feedback loops with users and affected communities can provide valuable insights into the impact of AI systems, informing ongoing improvements and ethical adjustments.
Addressing Risks such as Discrimination and Harm:
Risk Assessment and Mitigation Plans: Developing comprehensive risk assessment frameworks to systematically identify, evaluate, and mitigate risks associated with AI applications. This includes assessing risks of discrimination, Privacy breaches, and potential harm, with detailed mitigation plans outlining technical and procedural safeguards to protect against these risks.
Transparent Reporting and Accountability Mechanisms: Establishing mechanisms for transparent reporting on AI risks and the measures taken to address them. Creating precise lines of accountability within organizations ensures that decision-makers are responsible for managing AI risks, fostering a culture of responsibility and ethical AI use.
Understand safeguarding enterprise applications and enhancing reliability through our case study, Safeguarding Enterprise LLM Applications: Enhancing Reliability with RagaAI's Guardrails, to understand the significance of continuous AI system monitoring.
Regulations and Practices to Prevent AI Bias and Discrimination
Global Regulatory Frameworks
The U.S. SR-11-7 Guidance: This regulation offers a framework for risk management in financial institutions but is increasingly considered relevant for managing risks in AI deployments, emphasizing rigorous, data-driven risk assessment and mitigation strategies. Implementing SR-11-7 can involve conducting regular AI audits, stress testing AI models under various scenarios, and maintaining comprehensive documentation on decision-making processes to ensure transparency and accountability.
Europe’s AI Regulations: The European Union's approach to AI governance, including the proposed AI Act, strongly emphasizes high-risk applications of AI. It categorizes AI systems according to their risk level and imposes corresponding requirements, such as accuracy, transparency, and data governance standards. For organizations, this means implementing robust evaluation and testing protocols for AI models before deployment, especially in high-risk sectors like healthcare and criminal justice.
Technical Standards and Best Practices
Develop and adopt technical standards for AI models that prioritize fairness and non-discrimination. This includes using fairness metrics, such as equality of opportunity or demographic parity, to identify and mitigate bias during model training and evaluation.
Integrating ethical AI design principles from the outset of system development ensures that AI models are developed with an awareness of potential societal impacts, including the risk of perpetuating or amplifying biases.
Measuring AI Governance Effectiveness
Tailoring Governance to Organizational Needs:
We are conducting a comprehensive AI governance audit to identify specific focus areas, such as data quality, model transparency, or ethical AI use, depending on the organization's core activities and the AI applications.
Establish clear metrics and KPIs for AI governance, such as the frequency of bias audits, the number of identified and mitigated biases, or user satisfaction scores regarding AI interactions, to quantitatively assess governance effectiveness.
Adaptive and Continuous Improvement:
It is implementing an iterative governance process that allows for continuous feedback and improvement. This includes regular reviews of AI governance policies in light of new technological developments, regulatory changes, or shifts in societal expectations regarding AI.
Levels and Approaches in AI Governance
From Informal to Formal Governance:
Informal Governance: At the most basic level, informal governance may involve ad hoc committees or working groups addressing AI ethics and bias as part of an organization's broader technological or ethical discussions.
Ad Hoc Governance involves specific initiatives or projects aimed at addressing particular aspects of AI governance, such as bias mitigation in a new AI model or transparency in AI decision-making processes.
Formal Governance: Establishes dedicated structures and processes for AI governance, including permanent committees or departments, formalized policies and procedures for AI development and deployment, and regular reporting and accountability mechanisms.
Overseeing Automation and Societal Impact:
AI governance extends beyond the technical aspects of AI development to include the oversight of how AI systems are integrated into organizational processes and their broader societal impacts.
This includes evaluating the ethical implications of automated decision-making processes, assessing the potential for AI systems to impact human rights, and engaging with stakeholders, including affected communities and regulatory bodies, to ensure AI deployments align with societal values and expectations.
The development of comprehensive ethical frameworks is crucial for guiding AI practices. Learn more about RagaAI's approach in Introducing RagaAI DNA: The Multi-Modal Foundation Model for AI Testing, which underscores the importance of ethical considerations in AI development.
Conclusion
Effective AI governance requires a multifaceted approach that combines adherence to global regulatory frameworks, implementation of technical standards and best practices for bias mitigation, and development of tailored, adaptive governance structures.
By embracing a comprehensive and flexible approach to AI governance, organizations can ensure their AI systems are designed and deployed responsibly, contributing positively to societal progress and well-being.
Other articles

Agents Talk, Models Think: A2A + MCP for Enterprise Agentic AI

Riya Parikh, Nitai Agarwal
Apr 27, 2025
Read the article
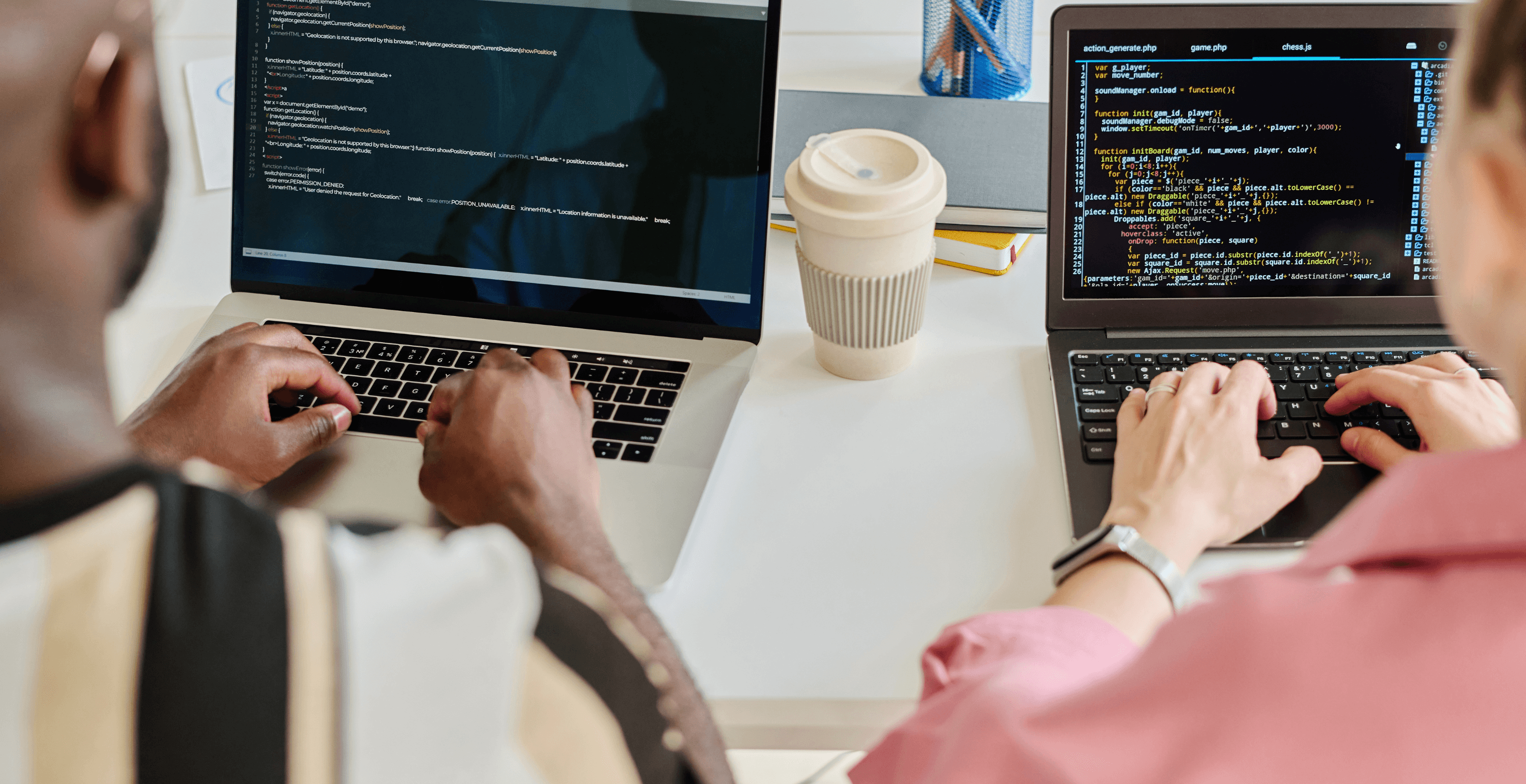
Understanding NeMo Guardrails: A Toolkit for LLM Security

Rehan Asif
Dec 24, 2024
Read the article

Understanding Differences in Large vs Small Language Models (LLM vs SLM)

Rehan Asif
Dec 21, 2024
Read the article