Evaluating and Enhancing LLM-as-a-Judge with Automated Tools
Evaluating and Enhancing LLM-as-a-Judge with Automated Tools
Evaluating and Enhancing LLM-as-a-Judge with Automated Tools
Rehan Asif
Dec 6, 2024
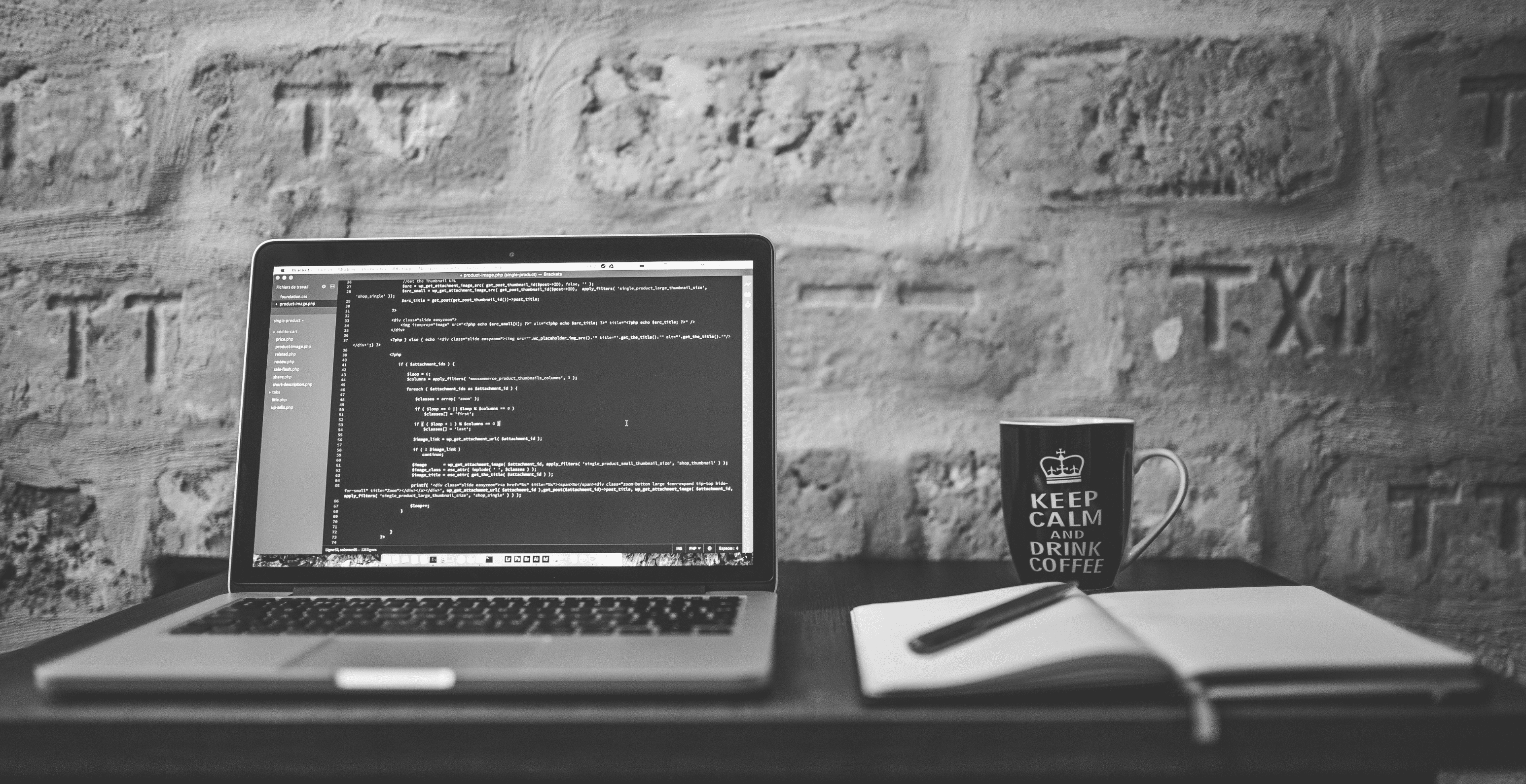
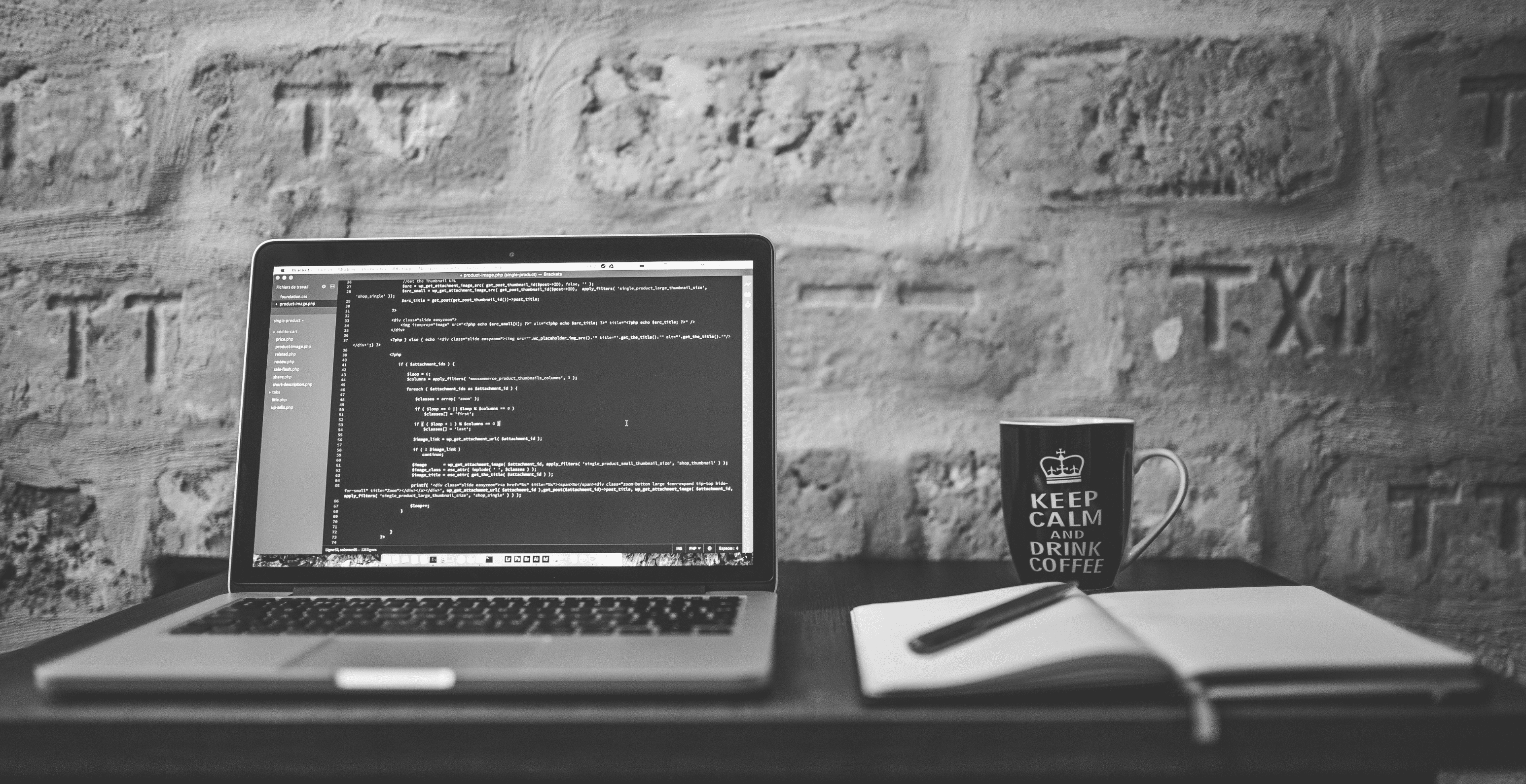
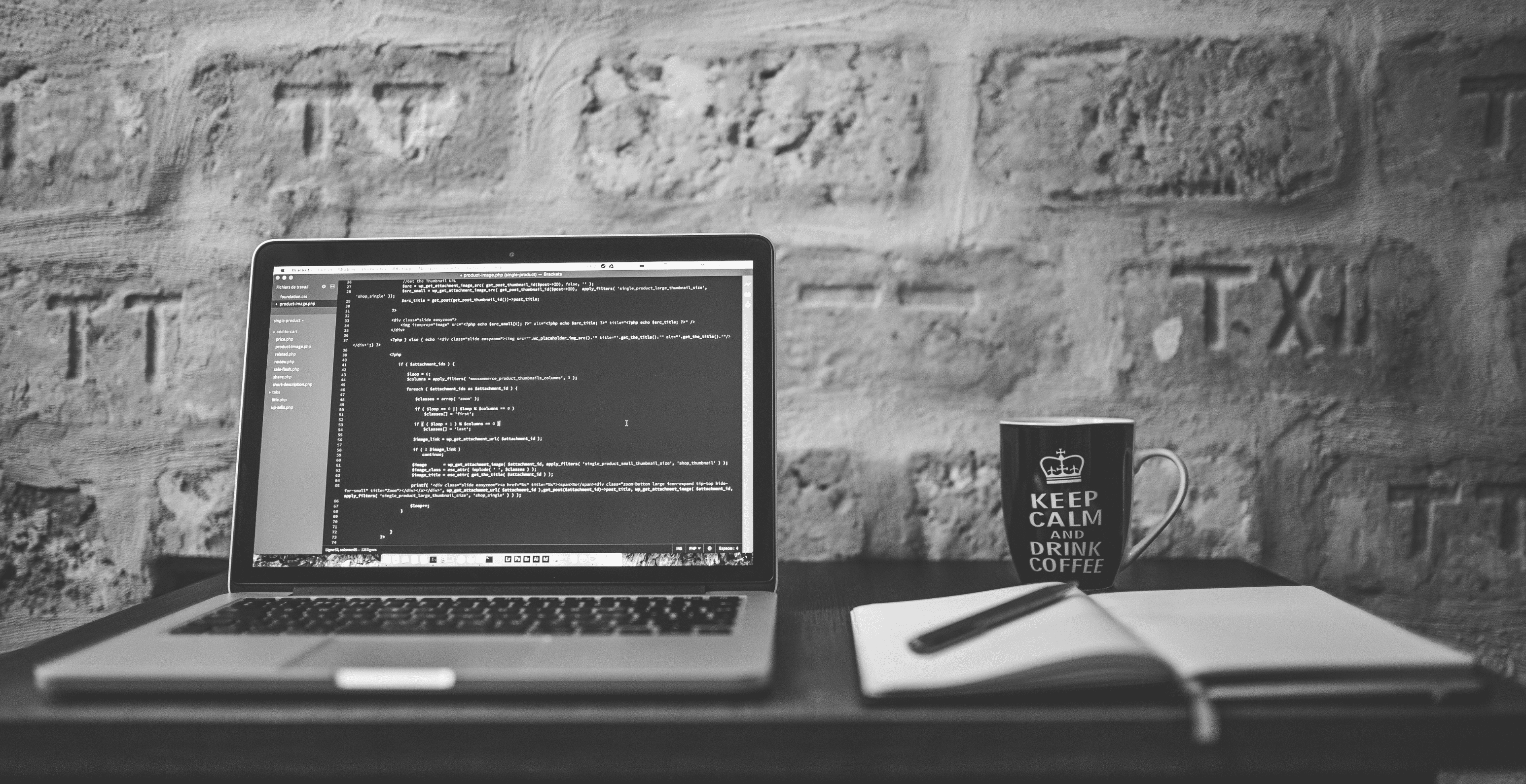
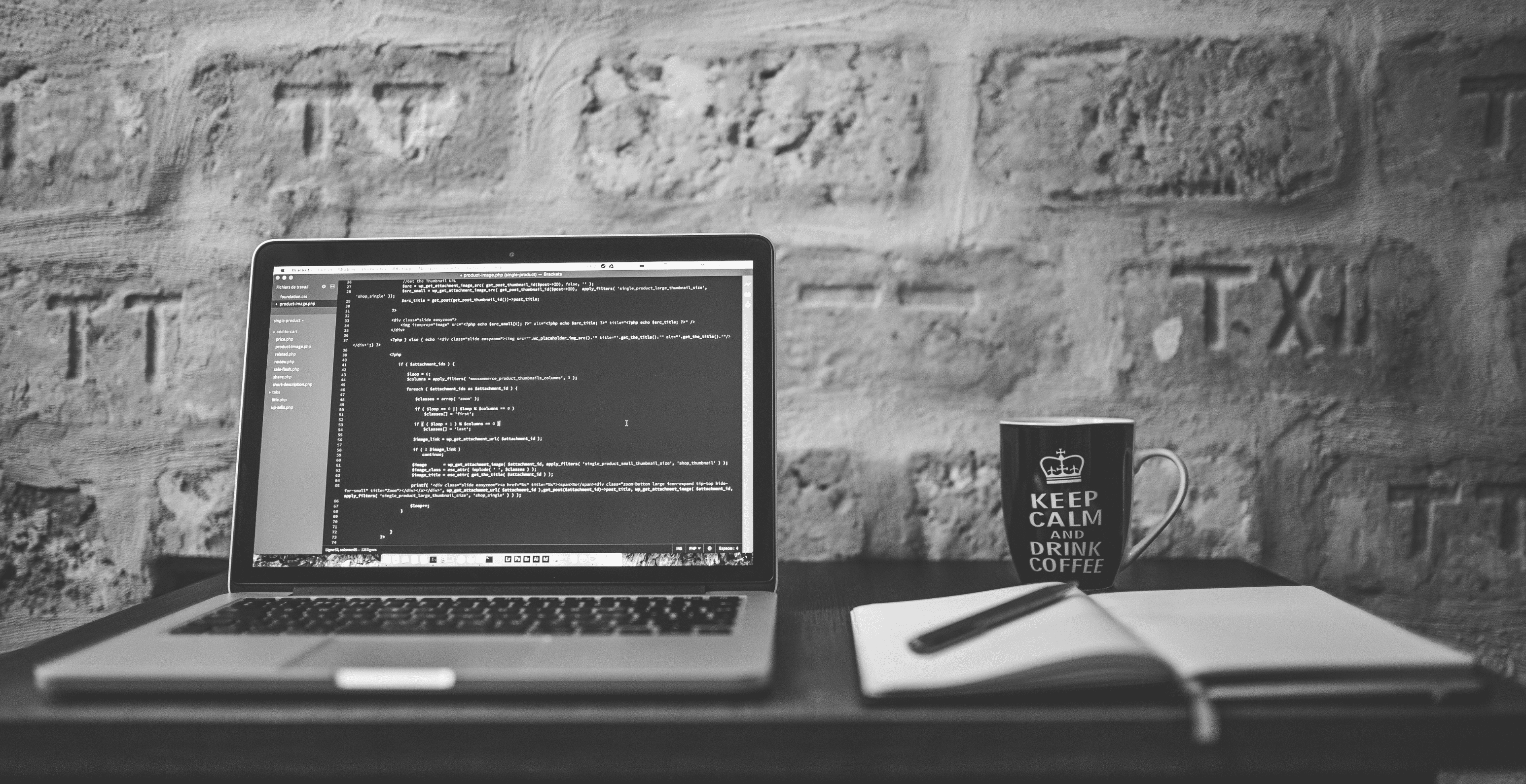
LLM as a judge is becoming an essential tool in assessing the outputs of large language models (LLMs). Traditional evaluation methods often fall short when dealing with the complexity and nuance of AI-generated content, making automated solutions not just beneficial but necessary.
You might find that using automated tools to evaluate LLMs greatly enhances accuracy and efficiency. These tools can identify, diagnose, and fix issues in real time, providing a more reliable and streamlined evaluation process. Understanding how to implement and benefit from these automated tools effectively is crucial for anyone looking to improve the performance of their AI applications.

Source: Using LLMs for Evaluation
Let's explore the challenges of traditional evaluation methods and how innovative automated tools are addressing these issues.
Introduction to Using LLM-as-a-Judge as a Solution
LLM-as-a-Judge offers a practical and efficient solution to the challenges of evaluating LLM outputs. This method involves using a large language model to assess the performance of another LLM.
By doing so, you can combine the nuanced understanding typically associated with human judgment and the speed and scalability of automated systems. This approach ensures that evaluations are consistent and thorough, reducing the time and effort required for manual assessments.
Key benefits of using LLM-as-a-Judge include:
Automated Evaluations: Automates the process of evaluating AI outputs, which significantly reduces the need for manual labor and speeds up the evaluation process.
Consistency: Ensures consistent application of evaluation criteria across all assessments, leading to more reliable and comparable results.
Additionally, LLM-as-a-Judge enhances the quality of evaluations by using the advanced understanding and contextual awareness inherent in LLMs. In the rapidly evolving field of large language models (LLMs), ensuring the safety, reliability, and quality of model outputs is paramount hence users must understand how to properly evaluate LLM.
This means you can quickly identify and diagnose issues in AI outputs, such as context adherence, coherence, and overall relevance, without the variability and bias that might come from human evaluators.

Source: LLM as a Judge
Importance of Automated Tools
Automated tools are vital in making the evaluation of LLMs more efficient and reliable. These tools can handle large volumes of data quickly, identifying patterns and providing insights that would take much longer to discover manually.
By using automated tools and LLM as a judge, you can streamline your evaluation processes, reduce human error, and ensure that your AI applications are performing optimally.
Key advantages of automated evaluation tools include:
Speed and Efficiency: Automated tools can process vast datasets much faster than manual methods, allowing you to keep up with the high volume of outputs generated by LLMs. This speed is crucial for timely evaluations and quick iterations.
Reduced Human Error: Human evaluators can make mistakes, especially when dealing with repetitive tasks or large amounts of data. Automated tools help minimize these errors, ensuring more accurate and consistent evaluations.
Continuous Monitoring: Automated tools can provide real-time feedback and continuous monitoring of your AI systems. This constant oversight helps in promptly identifying and addressing any issues, leading to more stable and reliable AI performance.
Comprehensive Analysis: These tools can perform detailed and comprehensive analyses of AI outputs, identifying subtle issues and patterns that might be missed in manual reviews. This thoroughness leads to deeper insights and better overall performance.
By integrating automated tools into your evaluation strategy, you can enhance the accuracy and reliability of your AI assessments. This integration leads to more efficient processes, better performance, and a higher quality of AI applications.
Next, let's delve into the specific challenges faced in traditional evaluation methods and how automated solutions are transforming this methodology.
Challenges in Traditional Evaluation Methods
Evaluating LLM outputs through traditional methods poses several challenges that can hinder the accuracy and reliability of assessments. The complexity and diversity of criteria involved in evaluating AI-generated content make it difficult to apply consistent and effective measures.
Additionally, traditional rule-based assessments and metrics often fall short of capturing the nuances of AI responses. Let's explore the challenges, complexity, and other aspects in detail below.
Complexity and Diversity in Traditional Evaluations
Evaluating the performance of LLMs involves considering a wide range of criteria, each with its own set of complexities. From context adherence to grammatical accuracy, the diversity of factors that need to be assessed can be overwhelming.
This complexity often leads to inconsistent evaluations, as human reviewers may struggle to apply the same standards across different assessments. Key complexities include:
Context Adherence: Ensuring the response is relevant to the given context.
Grammar and Syntax: Checking for grammatical correctness and proper sentence structure.
Coherence and Flow: Assessing the logical flow and coherence of the response.
Limitations of Rule-Based Assessments and Traditional Metrics
Rule-based assessments and traditional metrics such as ROUGE and BLEU are commonly used in evaluating LLM outputs, but they have significant limitations. These methods primarily focus on surface-level features and often miss deeper aspects of content quality.
For instance, they might accurately measure similarity to a reference text but fail to capture the semantic relevance or the overall coherence of the response. Limitations include:
Surface-Level Focus: Metrics like ROUGE and BLEU emphasize word overlap and syntactic similarity, neglecting deeper semantic meaning.
Lack of Nuance: These methods do not account for the nuances of context, tone, and intent in AI-generated responses.
Inflexibility: Rule-based assessments are rigid and cannot adapt to the diverse and evolving nature of AI outputs.
Examples of Common Issues in AI Assistant Responses
AI assistant responses often face several common issues that traditional evaluation methods struggle to address effectively. These issues can significantly impact the user experience and the perceived quality of the AI application.
Common issues include:
Context Misalignment: Responses that are not aligned with the given context or prompt.
Repetition: Unnecessary repetition of words or phrases, leading to redundancy.
Excessive Length: Responses that are overly long and verbose, lacking conciseness.
Lack of Coherence: Incoherent responses that do not logically flow from one point to another.
Addressing these issues requires more advanced evaluation methods that can capture the subtleties and nuances of AI-generated content.
Next, we'll explore the concept of automated tools for LLM-as-a-Judge and how it can provide a solution to these challenges.
Introduction to Automated Tools for LLM-as-a-Judge
Automated tools are essential for enhancing the capabilities of LLM-as-a-Judge. These tools can streamline the evaluation process, making it more efficient and comprehensive.
By integrating advanced metrics and evaluation frameworks, automated tools can provide deeper insights into the performance of LLMs, highlighting areas for improvement and ensuring that AI applications meet high standards of quality.
Benefits of using automated tools with LLM-as-a-Judge include:
Enhanced Precision: Automated tools can detect subtle issues and provide detailed feedback that might be missed in manual evaluations. This precise feedback helps in pinpointing specific areas that need improvement, ensuring that the LLM outputs are of the highest quality.
Scalability: These tools can handle large volumes of data, making it feasible to evaluate extensive datasets quickly. This scalability is crucial for businesses that need to process and evaluate large amounts of data without compromising on the quality of the assessments.
Continuous Improvement: Automated tools can continuously monitor and evaluate LLM outputs, providing real-time feedback that helps in iterative improvement. This ongoing evaluation process ensures that your AI systems are constantly being refined and improved, leading to better performance over time.
Next, we'll delve into the automated tools specifically designed to enhance the LLM-as-a-Judge process.
Automated Tools for Enhancing LLM-as-a-Judge
Grading Notes are a valuable tool for enhancing the LLM-as-a-Judge process. These notes provide detailed, structured feedback on AI-generated content, helping to identify specific strengths and weaknesses.
By using Grading Notes, you can ensure that your evaluations are comprehensive and focused on the most critical aspects of performance. Benefits of Grading Notes include:
Structured Feedback: Offers clear and organized feedback, making it easier to identify areas that need improvement.
Detailed Analysis: Provides in-depth insights into the performance of LLMs, helping to refine and improve AI models.
With Grading Notes, you can enhance the accuracy and reliability of your LLM evaluations, leading to better overall performance of your AI systems.
Introduction to Other Automated Tools
In addition to Grading Notes, other automated tools such as MT-Bench and Chatbot Arena play a crucial role in enhancing the capabilities of LLM-as-a-Judge. Each tool has unique features that contribute to a comprehensive evaluation framework for LLMs.

Pic: MT-Bench and Chabot Arena (Source: Link)
MT-Bench:
MT-Bench is specifically designed to benchmark the performance of LLMs across a variety of tasks, providing a detailed view of their strengths and weaknesses. This tool evaluates multiple dimensions of LLM performance, ensuring a thorough assessment that covers all critical aspects.
Pic: Average Win Rate of Six Models Under Different Judges in the MT-Bench

(Source: Link)
Comprehensive Benchmarking: MT-Bench covers a wide array of tasks, from simple question answering to complex problem-solving, giving you a full picture of the LLM's capabilities.
Task-Specific Insights: It breaks down performance by task, highlighting specific areas where the LLM excels or needs improvement. This granularity is essential for fine-tuning models to meet particular needs.
Performance Comparison: MT-Bench allows for direct comparisons between different LLMs, helping you choose the best model for your specific requirements. This comparison is based on clear, objective criteria, ensuring that your decision-making is well-informed.
Chatbot Arena:
Chatbot Arena focuses on evaluating conversational agents, ensuring they deliver high-quality, coherent, and contextually relevant interactions. This tool is essential for businesses that rely on chatbots to interact with customers, as it helps ensure that these interactions are effective and satisfactory.

Pic: A screenshot of the Chatbot Arena interface (Source: Link)
Specialized Evaluation: Chatbot Arena assesses the conversational abilities of chatbots, checking for coherence, relevance, and responsiveness. This specialized focus ensures that the chatbots perform well in real-world scenarios.
User Experience Focus: The tool evaluates interactions from the user's perspective, providing insights into how users perceive and interact with the chatbot. This feedback is crucial for improving user satisfaction and engagement.
Dialogue Coherence: Chatbot Arena ensures that the chatbot maintains context and logical flow throughout the conversation, reducing misunderstandings and enhancing the overall interaction quality. This coherence is key to creating a seamless and natural user experience.
Addressing Limitations and Biases Using Automated Tools
Automated tools are instrumental in addressing the limitations and biases inherent in traditional evaluation methods. By using these tools, you can ensure that your LLM evaluations are more objective, consistent, and comprehensive.
How automated tools address limitations and biases:
Objective Assessments: Automated tools provide unbiased evaluations based on predefined criteria, reducing the influence of subjective judgments.
Consistency: Ensures that the same evaluation standards are applied across all assessments, minimizing variability.
Comprehensive Analysis: Detects subtle issues and provides detailed feedback, ensuring that all aspects of LLM performance are thoroughly evaluated.
Improving Alignment Rates and Accuracy with Tools
Grading Notes and other automated tools significantly enhance the alignment rates and accuracy when evaluating long-form outputs from LLMs. These tools provide a structured framework for feedback, ensuring that evaluations are both detailed and actionable.
By aligning LLM outputs with predefined criteria, these tools ensure that the responses meet the required standards consistently. This structured approach helps identify specific areas for improvement, allowing for targeted enhancements in LLM performance.
Advantages of using Grading Notes and other automated tools include:
Improved Alignment: Automated tools help ensure that LLM outputs align with predefined criteria, maintaining a consistent quality across all evaluations. This alignment is crucial for meeting the standards expected in professional and practical applications.
Higher Accuracy: Tools like Grading Notes provide detailed feedback, highlighting subtle issues that might be overlooked in manual evaluations. This detailed analysis leads to more accurate assessments, improving the overall quality of LLM outputs.
Using Grading Notes and other automated tools enhances the overall quality of LLM evaluations, leading to more reliable and effective AI applications.
Next, we'll delve into how important human labeling is in improving consistency and alignment.
Improving Evaluation Consistency and Alignment
Human labeling is crucial for measuring the alignment rates of LLM judges. This process involves human evaluators reviewing AI-generated outputs and providing feedback on their accuracy and relevance. By comparing these human assessments with the evaluations made by LLMs, you can determine how well the AI aligns with human judgment.
This comparison helps in identifying any discrepancies and areas where the AI might need improvement. The human labeling process typically includes the following:
Selection of Evaluation Criteria: Defining the specific criteria that human evaluators will use to assess the AI outputs.
Training Human Evaluators: Ensuring that the evaluators understand the criteria and can apply them consistently.
Cross-Checking Results: Comparing the evaluations from multiple human reviewers to ensure consistency and accuracy.
Read our detailed article on the methods and metrics of evaluating Large language models for more information.
Agreement Rates Between LLM Judges and Human Judges
Understanding the agreement rates between LLM judges and human judges is critical for evaluating the reliability of AI assessments. By analyzing these rates, you can determine how closely LLM evaluations match those of human experts. High agreement rates indicate that the
LLM is effectively mimicking human judgment, while low rates suggest areas for improvement. This analysis involves:
Collecting Data: Gathering a substantial amount of evaluation data from both LLM judges and human judges.
Statistical Comparison: Using statistical methods to compare the evaluations and calculate agreement rates.
Identifying Discrepancies: Highlighting any significant differences in judgments and exploring the reasons behind them.
By regularly analyzing agreement rates, you can continuously refine the LLM evaluation process, ensuring that it remains aligned with human standards.
Next, we'll explore how to address biases and limitations involved in the evaluation process of LLM as a judge with automated tools involved.
Addressing Biases and Limitations
Biases in the evaluation process can significantly affect the reliability and fairness of LLM assessments. Human judges, despite their expertise, can introduce subjective biases based on their perspectives, experiences, and expectations. For example, a human judge might favor responses that align with their personal views or overlook errors that they are less sensitive to.
Similarly, Grading Notes, while structured and comprehensive, can also be influenced by the biases of the individuals who design and implement them. Common biases include:
Position Bias: This occurs when the order in which responses are presented affects their evaluation. Responses shown first or last might receive more favorable ratings simply due to their placement. This can skew the evaluation results and lead to inaccurate assessments.
Verbosity Bias: Longer responses might be perceived as more informative or accurate, even if they contain unnecessary information. This can result in evaluators overvaluing length over quality, leading to biased assessments that do not accurately reflect the response's true merit.
Self-Enhancement Bias: Evaluators might unconsciously rate outputs generated by systems they favor or are more familiar with more positively. This bias can affect the objectivity of the evaluations, as personal preferences or familiarity with certain systems can influence judgment.
Mitigation Strategies for Biases
To mitigate biases in the evaluation process, several strategies can be implemented. Addressing position bias involves randomizing the order of responses during evaluations. This ensures that no response consistently benefits from being positioned at the beginning or end of the list.
For verbosity bias, setting clear guidelines on the expected length and detail of responses can help evaluators focus on the quality rather than the quantity of information. Effective mitigation strategies include:
Randomization: By randomizing the order in which responses are evaluated, you can prevent position bias. This means that evaluators will assess each response based on its content rather than its placement, leading to more accurate and fair evaluations.
Clear Guidelines: Establishing clear guidelines for response length and detail helps evaluators concentrate on the substance of the content rather than its length. This reduces verbosity bias, ensuring that evaluations are based on the quality of information rather than the quantity.
Blind Evaluation: Conducting evaluations without revealing which system generated the response can help mitigate self-enhancement bias. When evaluators do not know the source of the responses, they are more likely to judge the content objectively, leading to fairer and more reliable assessments.
Role of Iterative Consensus-Building and Cross-Annotation
Iterative consensus-building and cross-annotation are crucial for enhancing the reliability of evaluations. Consensus-building involves multiple evaluators reviewing the same responses and discussing their assessments to reach a common agreement.
This process helps to standardize evaluations and reduce individual biases.
Cross-annotation, where different evaluators assess the same set of responses, provides a way to measure and improve consistency among judges. Benefits of these practices include:
Bias Reduction: Multiple perspectives help identify and mitigate individual biases, leading to a fairer evaluation process. By involving several evaluators, the influence of any single evaluator's bias is diminished, resulting in a more balanced assessment.
Quality Assurance: Ongoing collaboration among evaluators ensures that the evaluation criteria are applied uniformly, maintaining high standards of assessment. This collaborative approach helps to uphold the quality of evaluations and ensures that the criteria are consistently and accurately applied.
By integrating iterative consensus-building and cross-annotation into your evaluation strategy, you can significantly improve the accuracy and fairness of LLM assessments.
Next, we will discuss the future direction of the scenario of LLM as a judge, focusing on how the industry can go forth with the integration and implementation of the same.
Future Directions
Looking ahead, there are several exciting opportunities for enhancing LLM-as-a-Judge capabilities and setting new benchmarks. Future developments may include more sophisticated evaluation metrics that can better capture the nuances of AI-generated content. Additionally, integrating advanced machine learning techniques can help further reduce biases and improve the accuracy of evaluations.
Potential enhancements include:
Advanced Metrics: Developing new metrics that provide deeper insights into the quality and relevance of AI outputs.
Machine Learning Integration: Using advanced algorithms to refine evaluation processes and reduce biases.
Expanded Use Cases: Applying LLM-as-a-Judge to a broader range of applications, from content generation to customer support.
The current scenario of LLM as a judge and the generative AI infrastructure is bound to evolve rapidly in the future given the benefits and advantages of the same.
Encouragement for Industry Collaboration
The ongoing improvement of LLM evaluation processes benefits greatly from industry collaboration. By sharing insights, methodologies, and best practices, you can contribute to the collective advancement of AI technologies.
Encouraging open communication and collaboration across the industry fosters innovation and helps set higher standards for AI performance and evaluation. Benefits of industry collaboration include:
Knowledge Sharing: Pooling resources and expertise to develop better evaluation methods and tools.
Standardization: Establishing common benchmarks and standards for LLM evaluation, ensuring consistency and reliability across different systems.
Innovation: Driving innovation through collaborative efforts, leading to more advanced and effective AI solutions.
By promoting continuous improvement and industry collaboration, you can help create a more robust and dynamic AI aspect, ultimately leading to more reliable and effective AI applications.
Conclusion
Automated tools are essential for improving the evaluation and performance of LLMs, providing accuracy, efficiency, and continuous improvement. By adopting these tools and fostering industry collaboration, you can ensure your AI applications consistently deliver high-quality results. Embracing future advancements will further enhance LLM capabilities, driving innovation and reliability in AI technologies.
Raga AI offers advanced automated tools designed to enhance the evaluation and performance of LLMs, ensuring high accuracy and efficiency. By integrating Raga AI's solutions like RAGA AI catalyst and RAGA AI prism, you can achieve continuous improvement and reliable results in your AI applications.
Start your journey with Raga AI today and take your AI applications to the next level.
LLM as a judge is becoming an essential tool in assessing the outputs of large language models (LLMs). Traditional evaluation methods often fall short when dealing with the complexity and nuance of AI-generated content, making automated solutions not just beneficial but necessary.
You might find that using automated tools to evaluate LLMs greatly enhances accuracy and efficiency. These tools can identify, diagnose, and fix issues in real time, providing a more reliable and streamlined evaluation process. Understanding how to implement and benefit from these automated tools effectively is crucial for anyone looking to improve the performance of their AI applications.

Source: Using LLMs for Evaluation
Let's explore the challenges of traditional evaluation methods and how innovative automated tools are addressing these issues.
Introduction to Using LLM-as-a-Judge as a Solution
LLM-as-a-Judge offers a practical and efficient solution to the challenges of evaluating LLM outputs. This method involves using a large language model to assess the performance of another LLM.
By doing so, you can combine the nuanced understanding typically associated with human judgment and the speed and scalability of automated systems. This approach ensures that evaluations are consistent and thorough, reducing the time and effort required for manual assessments.
Key benefits of using LLM-as-a-Judge include:
Automated Evaluations: Automates the process of evaluating AI outputs, which significantly reduces the need for manual labor and speeds up the evaluation process.
Consistency: Ensures consistent application of evaluation criteria across all assessments, leading to more reliable and comparable results.
Additionally, LLM-as-a-Judge enhances the quality of evaluations by using the advanced understanding and contextual awareness inherent in LLMs. In the rapidly evolving field of large language models (LLMs), ensuring the safety, reliability, and quality of model outputs is paramount hence users must understand how to properly evaluate LLM.
This means you can quickly identify and diagnose issues in AI outputs, such as context adherence, coherence, and overall relevance, without the variability and bias that might come from human evaluators.

Source: LLM as a Judge
Importance of Automated Tools
Automated tools are vital in making the evaluation of LLMs more efficient and reliable. These tools can handle large volumes of data quickly, identifying patterns and providing insights that would take much longer to discover manually.
By using automated tools and LLM as a judge, you can streamline your evaluation processes, reduce human error, and ensure that your AI applications are performing optimally.
Key advantages of automated evaluation tools include:
Speed and Efficiency: Automated tools can process vast datasets much faster than manual methods, allowing you to keep up with the high volume of outputs generated by LLMs. This speed is crucial for timely evaluations and quick iterations.
Reduced Human Error: Human evaluators can make mistakes, especially when dealing with repetitive tasks or large amounts of data. Automated tools help minimize these errors, ensuring more accurate and consistent evaluations.
Continuous Monitoring: Automated tools can provide real-time feedback and continuous monitoring of your AI systems. This constant oversight helps in promptly identifying and addressing any issues, leading to more stable and reliable AI performance.
Comprehensive Analysis: These tools can perform detailed and comprehensive analyses of AI outputs, identifying subtle issues and patterns that might be missed in manual reviews. This thoroughness leads to deeper insights and better overall performance.
By integrating automated tools into your evaluation strategy, you can enhance the accuracy and reliability of your AI assessments. This integration leads to more efficient processes, better performance, and a higher quality of AI applications.
Next, let's delve into the specific challenges faced in traditional evaluation methods and how automated solutions are transforming this methodology.
Challenges in Traditional Evaluation Methods
Evaluating LLM outputs through traditional methods poses several challenges that can hinder the accuracy and reliability of assessments. The complexity and diversity of criteria involved in evaluating AI-generated content make it difficult to apply consistent and effective measures.
Additionally, traditional rule-based assessments and metrics often fall short of capturing the nuances of AI responses. Let's explore the challenges, complexity, and other aspects in detail below.
Complexity and Diversity in Traditional Evaluations
Evaluating the performance of LLMs involves considering a wide range of criteria, each with its own set of complexities. From context adherence to grammatical accuracy, the diversity of factors that need to be assessed can be overwhelming.
This complexity often leads to inconsistent evaluations, as human reviewers may struggle to apply the same standards across different assessments. Key complexities include:
Context Adherence: Ensuring the response is relevant to the given context.
Grammar and Syntax: Checking for grammatical correctness and proper sentence structure.
Coherence and Flow: Assessing the logical flow and coherence of the response.
Limitations of Rule-Based Assessments and Traditional Metrics
Rule-based assessments and traditional metrics such as ROUGE and BLEU are commonly used in evaluating LLM outputs, but they have significant limitations. These methods primarily focus on surface-level features and often miss deeper aspects of content quality.
For instance, they might accurately measure similarity to a reference text but fail to capture the semantic relevance or the overall coherence of the response. Limitations include:
Surface-Level Focus: Metrics like ROUGE and BLEU emphasize word overlap and syntactic similarity, neglecting deeper semantic meaning.
Lack of Nuance: These methods do not account for the nuances of context, tone, and intent in AI-generated responses.
Inflexibility: Rule-based assessments are rigid and cannot adapt to the diverse and evolving nature of AI outputs.
Examples of Common Issues in AI Assistant Responses
AI assistant responses often face several common issues that traditional evaluation methods struggle to address effectively. These issues can significantly impact the user experience and the perceived quality of the AI application.
Common issues include:
Context Misalignment: Responses that are not aligned with the given context or prompt.
Repetition: Unnecessary repetition of words or phrases, leading to redundancy.
Excessive Length: Responses that are overly long and verbose, lacking conciseness.
Lack of Coherence: Incoherent responses that do not logically flow from one point to another.
Addressing these issues requires more advanced evaluation methods that can capture the subtleties and nuances of AI-generated content.
Next, we'll explore the concept of automated tools for LLM-as-a-Judge and how it can provide a solution to these challenges.
Introduction to Automated Tools for LLM-as-a-Judge
Automated tools are essential for enhancing the capabilities of LLM-as-a-Judge. These tools can streamline the evaluation process, making it more efficient and comprehensive.
By integrating advanced metrics and evaluation frameworks, automated tools can provide deeper insights into the performance of LLMs, highlighting areas for improvement and ensuring that AI applications meet high standards of quality.
Benefits of using automated tools with LLM-as-a-Judge include:
Enhanced Precision: Automated tools can detect subtle issues and provide detailed feedback that might be missed in manual evaluations. This precise feedback helps in pinpointing specific areas that need improvement, ensuring that the LLM outputs are of the highest quality.
Scalability: These tools can handle large volumes of data, making it feasible to evaluate extensive datasets quickly. This scalability is crucial for businesses that need to process and evaluate large amounts of data without compromising on the quality of the assessments.
Continuous Improvement: Automated tools can continuously monitor and evaluate LLM outputs, providing real-time feedback that helps in iterative improvement. This ongoing evaluation process ensures that your AI systems are constantly being refined and improved, leading to better performance over time.
Next, we'll delve into the automated tools specifically designed to enhance the LLM-as-a-Judge process.
Automated Tools for Enhancing LLM-as-a-Judge
Grading Notes are a valuable tool for enhancing the LLM-as-a-Judge process. These notes provide detailed, structured feedback on AI-generated content, helping to identify specific strengths and weaknesses.
By using Grading Notes, you can ensure that your evaluations are comprehensive and focused on the most critical aspects of performance. Benefits of Grading Notes include:
Structured Feedback: Offers clear and organized feedback, making it easier to identify areas that need improvement.
Detailed Analysis: Provides in-depth insights into the performance of LLMs, helping to refine and improve AI models.
With Grading Notes, you can enhance the accuracy and reliability of your LLM evaluations, leading to better overall performance of your AI systems.
Introduction to Other Automated Tools
In addition to Grading Notes, other automated tools such as MT-Bench and Chatbot Arena play a crucial role in enhancing the capabilities of LLM-as-a-Judge. Each tool has unique features that contribute to a comprehensive evaluation framework for LLMs.

Pic: MT-Bench and Chabot Arena (Source: Link)
MT-Bench:
MT-Bench is specifically designed to benchmark the performance of LLMs across a variety of tasks, providing a detailed view of their strengths and weaknesses. This tool evaluates multiple dimensions of LLM performance, ensuring a thorough assessment that covers all critical aspects.
Pic: Average Win Rate of Six Models Under Different Judges in the MT-Bench

(Source: Link)
Comprehensive Benchmarking: MT-Bench covers a wide array of tasks, from simple question answering to complex problem-solving, giving you a full picture of the LLM's capabilities.
Task-Specific Insights: It breaks down performance by task, highlighting specific areas where the LLM excels or needs improvement. This granularity is essential for fine-tuning models to meet particular needs.
Performance Comparison: MT-Bench allows for direct comparisons between different LLMs, helping you choose the best model for your specific requirements. This comparison is based on clear, objective criteria, ensuring that your decision-making is well-informed.
Chatbot Arena:
Chatbot Arena focuses on evaluating conversational agents, ensuring they deliver high-quality, coherent, and contextually relevant interactions. This tool is essential for businesses that rely on chatbots to interact with customers, as it helps ensure that these interactions are effective and satisfactory.

Pic: A screenshot of the Chatbot Arena interface (Source: Link)
Specialized Evaluation: Chatbot Arena assesses the conversational abilities of chatbots, checking for coherence, relevance, and responsiveness. This specialized focus ensures that the chatbots perform well in real-world scenarios.
User Experience Focus: The tool evaluates interactions from the user's perspective, providing insights into how users perceive and interact with the chatbot. This feedback is crucial for improving user satisfaction and engagement.
Dialogue Coherence: Chatbot Arena ensures that the chatbot maintains context and logical flow throughout the conversation, reducing misunderstandings and enhancing the overall interaction quality. This coherence is key to creating a seamless and natural user experience.
Addressing Limitations and Biases Using Automated Tools
Automated tools are instrumental in addressing the limitations and biases inherent in traditional evaluation methods. By using these tools, you can ensure that your LLM evaluations are more objective, consistent, and comprehensive.
How automated tools address limitations and biases:
Objective Assessments: Automated tools provide unbiased evaluations based on predefined criteria, reducing the influence of subjective judgments.
Consistency: Ensures that the same evaluation standards are applied across all assessments, minimizing variability.
Comprehensive Analysis: Detects subtle issues and provides detailed feedback, ensuring that all aspects of LLM performance are thoroughly evaluated.
Improving Alignment Rates and Accuracy with Tools
Grading Notes and other automated tools significantly enhance the alignment rates and accuracy when evaluating long-form outputs from LLMs. These tools provide a structured framework for feedback, ensuring that evaluations are both detailed and actionable.
By aligning LLM outputs with predefined criteria, these tools ensure that the responses meet the required standards consistently. This structured approach helps identify specific areas for improvement, allowing for targeted enhancements in LLM performance.
Advantages of using Grading Notes and other automated tools include:
Improved Alignment: Automated tools help ensure that LLM outputs align with predefined criteria, maintaining a consistent quality across all evaluations. This alignment is crucial for meeting the standards expected in professional and practical applications.
Higher Accuracy: Tools like Grading Notes provide detailed feedback, highlighting subtle issues that might be overlooked in manual evaluations. This detailed analysis leads to more accurate assessments, improving the overall quality of LLM outputs.
Using Grading Notes and other automated tools enhances the overall quality of LLM evaluations, leading to more reliable and effective AI applications.
Next, we'll delve into how important human labeling is in improving consistency and alignment.
Improving Evaluation Consistency and Alignment
Human labeling is crucial for measuring the alignment rates of LLM judges. This process involves human evaluators reviewing AI-generated outputs and providing feedback on their accuracy and relevance. By comparing these human assessments with the evaluations made by LLMs, you can determine how well the AI aligns with human judgment.
This comparison helps in identifying any discrepancies and areas where the AI might need improvement. The human labeling process typically includes the following:
Selection of Evaluation Criteria: Defining the specific criteria that human evaluators will use to assess the AI outputs.
Training Human Evaluators: Ensuring that the evaluators understand the criteria and can apply them consistently.
Cross-Checking Results: Comparing the evaluations from multiple human reviewers to ensure consistency and accuracy.
Read our detailed article on the methods and metrics of evaluating Large language models for more information.
Agreement Rates Between LLM Judges and Human Judges
Understanding the agreement rates between LLM judges and human judges is critical for evaluating the reliability of AI assessments. By analyzing these rates, you can determine how closely LLM evaluations match those of human experts. High agreement rates indicate that the
LLM is effectively mimicking human judgment, while low rates suggest areas for improvement. This analysis involves:
Collecting Data: Gathering a substantial amount of evaluation data from both LLM judges and human judges.
Statistical Comparison: Using statistical methods to compare the evaluations and calculate agreement rates.
Identifying Discrepancies: Highlighting any significant differences in judgments and exploring the reasons behind them.
By regularly analyzing agreement rates, you can continuously refine the LLM evaluation process, ensuring that it remains aligned with human standards.
Next, we'll explore how to address biases and limitations involved in the evaluation process of LLM as a judge with automated tools involved.
Addressing Biases and Limitations
Biases in the evaluation process can significantly affect the reliability and fairness of LLM assessments. Human judges, despite their expertise, can introduce subjective biases based on their perspectives, experiences, and expectations. For example, a human judge might favor responses that align with their personal views or overlook errors that they are less sensitive to.
Similarly, Grading Notes, while structured and comprehensive, can also be influenced by the biases of the individuals who design and implement them. Common biases include:
Position Bias: This occurs when the order in which responses are presented affects their evaluation. Responses shown first or last might receive more favorable ratings simply due to their placement. This can skew the evaluation results and lead to inaccurate assessments.
Verbosity Bias: Longer responses might be perceived as more informative or accurate, even if they contain unnecessary information. This can result in evaluators overvaluing length over quality, leading to biased assessments that do not accurately reflect the response's true merit.
Self-Enhancement Bias: Evaluators might unconsciously rate outputs generated by systems they favor or are more familiar with more positively. This bias can affect the objectivity of the evaluations, as personal preferences or familiarity with certain systems can influence judgment.
Mitigation Strategies for Biases
To mitigate biases in the evaluation process, several strategies can be implemented. Addressing position bias involves randomizing the order of responses during evaluations. This ensures that no response consistently benefits from being positioned at the beginning or end of the list.
For verbosity bias, setting clear guidelines on the expected length and detail of responses can help evaluators focus on the quality rather than the quantity of information. Effective mitigation strategies include:
Randomization: By randomizing the order in which responses are evaluated, you can prevent position bias. This means that evaluators will assess each response based on its content rather than its placement, leading to more accurate and fair evaluations.
Clear Guidelines: Establishing clear guidelines for response length and detail helps evaluators concentrate on the substance of the content rather than its length. This reduces verbosity bias, ensuring that evaluations are based on the quality of information rather than the quantity.
Blind Evaluation: Conducting evaluations without revealing which system generated the response can help mitigate self-enhancement bias. When evaluators do not know the source of the responses, they are more likely to judge the content objectively, leading to fairer and more reliable assessments.
Role of Iterative Consensus-Building and Cross-Annotation
Iterative consensus-building and cross-annotation are crucial for enhancing the reliability of evaluations. Consensus-building involves multiple evaluators reviewing the same responses and discussing their assessments to reach a common agreement.
This process helps to standardize evaluations and reduce individual biases.
Cross-annotation, where different evaluators assess the same set of responses, provides a way to measure and improve consistency among judges. Benefits of these practices include:
Bias Reduction: Multiple perspectives help identify and mitigate individual biases, leading to a fairer evaluation process. By involving several evaluators, the influence of any single evaluator's bias is diminished, resulting in a more balanced assessment.
Quality Assurance: Ongoing collaboration among evaluators ensures that the evaluation criteria are applied uniformly, maintaining high standards of assessment. This collaborative approach helps to uphold the quality of evaluations and ensures that the criteria are consistently and accurately applied.
By integrating iterative consensus-building and cross-annotation into your evaluation strategy, you can significantly improve the accuracy and fairness of LLM assessments.
Next, we will discuss the future direction of the scenario of LLM as a judge, focusing on how the industry can go forth with the integration and implementation of the same.
Future Directions
Looking ahead, there are several exciting opportunities for enhancing LLM-as-a-Judge capabilities and setting new benchmarks. Future developments may include more sophisticated evaluation metrics that can better capture the nuances of AI-generated content. Additionally, integrating advanced machine learning techniques can help further reduce biases and improve the accuracy of evaluations.
Potential enhancements include:
Advanced Metrics: Developing new metrics that provide deeper insights into the quality and relevance of AI outputs.
Machine Learning Integration: Using advanced algorithms to refine evaluation processes and reduce biases.
Expanded Use Cases: Applying LLM-as-a-Judge to a broader range of applications, from content generation to customer support.
The current scenario of LLM as a judge and the generative AI infrastructure is bound to evolve rapidly in the future given the benefits and advantages of the same.
Encouragement for Industry Collaboration
The ongoing improvement of LLM evaluation processes benefits greatly from industry collaboration. By sharing insights, methodologies, and best practices, you can contribute to the collective advancement of AI technologies.
Encouraging open communication and collaboration across the industry fosters innovation and helps set higher standards for AI performance and evaluation. Benefits of industry collaboration include:
Knowledge Sharing: Pooling resources and expertise to develop better evaluation methods and tools.
Standardization: Establishing common benchmarks and standards for LLM evaluation, ensuring consistency and reliability across different systems.
Innovation: Driving innovation through collaborative efforts, leading to more advanced and effective AI solutions.
By promoting continuous improvement and industry collaboration, you can help create a more robust and dynamic AI aspect, ultimately leading to more reliable and effective AI applications.
Conclusion
Automated tools are essential for improving the evaluation and performance of LLMs, providing accuracy, efficiency, and continuous improvement. By adopting these tools and fostering industry collaboration, you can ensure your AI applications consistently deliver high-quality results. Embracing future advancements will further enhance LLM capabilities, driving innovation and reliability in AI technologies.
Raga AI offers advanced automated tools designed to enhance the evaluation and performance of LLMs, ensuring high accuracy and efficiency. By integrating Raga AI's solutions like RAGA AI catalyst and RAGA AI prism, you can achieve continuous improvement and reliable results in your AI applications.
Start your journey with Raga AI today and take your AI applications to the next level.
LLM as a judge is becoming an essential tool in assessing the outputs of large language models (LLMs). Traditional evaluation methods often fall short when dealing with the complexity and nuance of AI-generated content, making automated solutions not just beneficial but necessary.
You might find that using automated tools to evaluate LLMs greatly enhances accuracy and efficiency. These tools can identify, diagnose, and fix issues in real time, providing a more reliable and streamlined evaluation process. Understanding how to implement and benefit from these automated tools effectively is crucial for anyone looking to improve the performance of their AI applications.

Source: Using LLMs for Evaluation
Let's explore the challenges of traditional evaluation methods and how innovative automated tools are addressing these issues.
Introduction to Using LLM-as-a-Judge as a Solution
LLM-as-a-Judge offers a practical and efficient solution to the challenges of evaluating LLM outputs. This method involves using a large language model to assess the performance of another LLM.
By doing so, you can combine the nuanced understanding typically associated with human judgment and the speed and scalability of automated systems. This approach ensures that evaluations are consistent and thorough, reducing the time and effort required for manual assessments.
Key benefits of using LLM-as-a-Judge include:
Automated Evaluations: Automates the process of evaluating AI outputs, which significantly reduces the need for manual labor and speeds up the evaluation process.
Consistency: Ensures consistent application of evaluation criteria across all assessments, leading to more reliable and comparable results.
Additionally, LLM-as-a-Judge enhances the quality of evaluations by using the advanced understanding and contextual awareness inherent in LLMs. In the rapidly evolving field of large language models (LLMs), ensuring the safety, reliability, and quality of model outputs is paramount hence users must understand how to properly evaluate LLM.
This means you can quickly identify and diagnose issues in AI outputs, such as context adherence, coherence, and overall relevance, without the variability and bias that might come from human evaluators.

Source: LLM as a Judge
Importance of Automated Tools
Automated tools are vital in making the evaluation of LLMs more efficient and reliable. These tools can handle large volumes of data quickly, identifying patterns and providing insights that would take much longer to discover manually.
By using automated tools and LLM as a judge, you can streamline your evaluation processes, reduce human error, and ensure that your AI applications are performing optimally.
Key advantages of automated evaluation tools include:
Speed and Efficiency: Automated tools can process vast datasets much faster than manual methods, allowing you to keep up with the high volume of outputs generated by LLMs. This speed is crucial for timely evaluations and quick iterations.
Reduced Human Error: Human evaluators can make mistakes, especially when dealing with repetitive tasks or large amounts of data. Automated tools help minimize these errors, ensuring more accurate and consistent evaluations.
Continuous Monitoring: Automated tools can provide real-time feedback and continuous monitoring of your AI systems. This constant oversight helps in promptly identifying and addressing any issues, leading to more stable and reliable AI performance.
Comprehensive Analysis: These tools can perform detailed and comprehensive analyses of AI outputs, identifying subtle issues and patterns that might be missed in manual reviews. This thoroughness leads to deeper insights and better overall performance.
By integrating automated tools into your evaluation strategy, you can enhance the accuracy and reliability of your AI assessments. This integration leads to more efficient processes, better performance, and a higher quality of AI applications.
Next, let's delve into the specific challenges faced in traditional evaluation methods and how automated solutions are transforming this methodology.
Challenges in Traditional Evaluation Methods
Evaluating LLM outputs through traditional methods poses several challenges that can hinder the accuracy and reliability of assessments. The complexity and diversity of criteria involved in evaluating AI-generated content make it difficult to apply consistent and effective measures.
Additionally, traditional rule-based assessments and metrics often fall short of capturing the nuances of AI responses. Let's explore the challenges, complexity, and other aspects in detail below.
Complexity and Diversity in Traditional Evaluations
Evaluating the performance of LLMs involves considering a wide range of criteria, each with its own set of complexities. From context adherence to grammatical accuracy, the diversity of factors that need to be assessed can be overwhelming.
This complexity often leads to inconsistent evaluations, as human reviewers may struggle to apply the same standards across different assessments. Key complexities include:
Context Adherence: Ensuring the response is relevant to the given context.
Grammar and Syntax: Checking for grammatical correctness and proper sentence structure.
Coherence and Flow: Assessing the logical flow and coherence of the response.
Limitations of Rule-Based Assessments and Traditional Metrics
Rule-based assessments and traditional metrics such as ROUGE and BLEU are commonly used in evaluating LLM outputs, but they have significant limitations. These methods primarily focus on surface-level features and often miss deeper aspects of content quality.
For instance, they might accurately measure similarity to a reference text but fail to capture the semantic relevance or the overall coherence of the response. Limitations include:
Surface-Level Focus: Metrics like ROUGE and BLEU emphasize word overlap and syntactic similarity, neglecting deeper semantic meaning.
Lack of Nuance: These methods do not account for the nuances of context, tone, and intent in AI-generated responses.
Inflexibility: Rule-based assessments are rigid and cannot adapt to the diverse and evolving nature of AI outputs.
Examples of Common Issues in AI Assistant Responses
AI assistant responses often face several common issues that traditional evaluation methods struggle to address effectively. These issues can significantly impact the user experience and the perceived quality of the AI application.
Common issues include:
Context Misalignment: Responses that are not aligned with the given context or prompt.
Repetition: Unnecessary repetition of words or phrases, leading to redundancy.
Excessive Length: Responses that are overly long and verbose, lacking conciseness.
Lack of Coherence: Incoherent responses that do not logically flow from one point to another.
Addressing these issues requires more advanced evaluation methods that can capture the subtleties and nuances of AI-generated content.
Next, we'll explore the concept of automated tools for LLM-as-a-Judge and how it can provide a solution to these challenges.
Introduction to Automated Tools for LLM-as-a-Judge
Automated tools are essential for enhancing the capabilities of LLM-as-a-Judge. These tools can streamline the evaluation process, making it more efficient and comprehensive.
By integrating advanced metrics and evaluation frameworks, automated tools can provide deeper insights into the performance of LLMs, highlighting areas for improvement and ensuring that AI applications meet high standards of quality.
Benefits of using automated tools with LLM-as-a-Judge include:
Enhanced Precision: Automated tools can detect subtle issues and provide detailed feedback that might be missed in manual evaluations. This precise feedback helps in pinpointing specific areas that need improvement, ensuring that the LLM outputs are of the highest quality.
Scalability: These tools can handle large volumes of data, making it feasible to evaluate extensive datasets quickly. This scalability is crucial for businesses that need to process and evaluate large amounts of data without compromising on the quality of the assessments.
Continuous Improvement: Automated tools can continuously monitor and evaluate LLM outputs, providing real-time feedback that helps in iterative improvement. This ongoing evaluation process ensures that your AI systems are constantly being refined and improved, leading to better performance over time.
Next, we'll delve into the automated tools specifically designed to enhance the LLM-as-a-Judge process.
Automated Tools for Enhancing LLM-as-a-Judge
Grading Notes are a valuable tool for enhancing the LLM-as-a-Judge process. These notes provide detailed, structured feedback on AI-generated content, helping to identify specific strengths and weaknesses.
By using Grading Notes, you can ensure that your evaluations are comprehensive and focused on the most critical aspects of performance. Benefits of Grading Notes include:
Structured Feedback: Offers clear and organized feedback, making it easier to identify areas that need improvement.
Detailed Analysis: Provides in-depth insights into the performance of LLMs, helping to refine and improve AI models.
With Grading Notes, you can enhance the accuracy and reliability of your LLM evaluations, leading to better overall performance of your AI systems.
Introduction to Other Automated Tools
In addition to Grading Notes, other automated tools such as MT-Bench and Chatbot Arena play a crucial role in enhancing the capabilities of LLM-as-a-Judge. Each tool has unique features that contribute to a comprehensive evaluation framework for LLMs.

Pic: MT-Bench and Chabot Arena (Source: Link)
MT-Bench:
MT-Bench is specifically designed to benchmark the performance of LLMs across a variety of tasks, providing a detailed view of their strengths and weaknesses. This tool evaluates multiple dimensions of LLM performance, ensuring a thorough assessment that covers all critical aspects.
Pic: Average Win Rate of Six Models Under Different Judges in the MT-Bench

(Source: Link)
Comprehensive Benchmarking: MT-Bench covers a wide array of tasks, from simple question answering to complex problem-solving, giving you a full picture of the LLM's capabilities.
Task-Specific Insights: It breaks down performance by task, highlighting specific areas where the LLM excels or needs improvement. This granularity is essential for fine-tuning models to meet particular needs.
Performance Comparison: MT-Bench allows for direct comparisons between different LLMs, helping you choose the best model for your specific requirements. This comparison is based on clear, objective criteria, ensuring that your decision-making is well-informed.
Chatbot Arena:
Chatbot Arena focuses on evaluating conversational agents, ensuring they deliver high-quality, coherent, and contextually relevant interactions. This tool is essential for businesses that rely on chatbots to interact with customers, as it helps ensure that these interactions are effective and satisfactory.

Pic: A screenshot of the Chatbot Arena interface (Source: Link)
Specialized Evaluation: Chatbot Arena assesses the conversational abilities of chatbots, checking for coherence, relevance, and responsiveness. This specialized focus ensures that the chatbots perform well in real-world scenarios.
User Experience Focus: The tool evaluates interactions from the user's perspective, providing insights into how users perceive and interact with the chatbot. This feedback is crucial for improving user satisfaction and engagement.
Dialogue Coherence: Chatbot Arena ensures that the chatbot maintains context and logical flow throughout the conversation, reducing misunderstandings and enhancing the overall interaction quality. This coherence is key to creating a seamless and natural user experience.
Addressing Limitations and Biases Using Automated Tools
Automated tools are instrumental in addressing the limitations and biases inherent in traditional evaluation methods. By using these tools, you can ensure that your LLM evaluations are more objective, consistent, and comprehensive.
How automated tools address limitations and biases:
Objective Assessments: Automated tools provide unbiased evaluations based on predefined criteria, reducing the influence of subjective judgments.
Consistency: Ensures that the same evaluation standards are applied across all assessments, minimizing variability.
Comprehensive Analysis: Detects subtle issues and provides detailed feedback, ensuring that all aspects of LLM performance are thoroughly evaluated.
Improving Alignment Rates and Accuracy with Tools
Grading Notes and other automated tools significantly enhance the alignment rates and accuracy when evaluating long-form outputs from LLMs. These tools provide a structured framework for feedback, ensuring that evaluations are both detailed and actionable.
By aligning LLM outputs with predefined criteria, these tools ensure that the responses meet the required standards consistently. This structured approach helps identify specific areas for improvement, allowing for targeted enhancements in LLM performance.
Advantages of using Grading Notes and other automated tools include:
Improved Alignment: Automated tools help ensure that LLM outputs align with predefined criteria, maintaining a consistent quality across all evaluations. This alignment is crucial for meeting the standards expected in professional and practical applications.
Higher Accuracy: Tools like Grading Notes provide detailed feedback, highlighting subtle issues that might be overlooked in manual evaluations. This detailed analysis leads to more accurate assessments, improving the overall quality of LLM outputs.
Using Grading Notes and other automated tools enhances the overall quality of LLM evaluations, leading to more reliable and effective AI applications.
Next, we'll delve into how important human labeling is in improving consistency and alignment.
Improving Evaluation Consistency and Alignment
Human labeling is crucial for measuring the alignment rates of LLM judges. This process involves human evaluators reviewing AI-generated outputs and providing feedback on their accuracy and relevance. By comparing these human assessments with the evaluations made by LLMs, you can determine how well the AI aligns with human judgment.
This comparison helps in identifying any discrepancies and areas where the AI might need improvement. The human labeling process typically includes the following:
Selection of Evaluation Criteria: Defining the specific criteria that human evaluators will use to assess the AI outputs.
Training Human Evaluators: Ensuring that the evaluators understand the criteria and can apply them consistently.
Cross-Checking Results: Comparing the evaluations from multiple human reviewers to ensure consistency and accuracy.
Read our detailed article on the methods and metrics of evaluating Large language models for more information.
Agreement Rates Between LLM Judges and Human Judges
Understanding the agreement rates between LLM judges and human judges is critical for evaluating the reliability of AI assessments. By analyzing these rates, you can determine how closely LLM evaluations match those of human experts. High agreement rates indicate that the
LLM is effectively mimicking human judgment, while low rates suggest areas for improvement. This analysis involves:
Collecting Data: Gathering a substantial amount of evaluation data from both LLM judges and human judges.
Statistical Comparison: Using statistical methods to compare the evaluations and calculate agreement rates.
Identifying Discrepancies: Highlighting any significant differences in judgments and exploring the reasons behind them.
By regularly analyzing agreement rates, you can continuously refine the LLM evaluation process, ensuring that it remains aligned with human standards.
Next, we'll explore how to address biases and limitations involved in the evaluation process of LLM as a judge with automated tools involved.
Addressing Biases and Limitations
Biases in the evaluation process can significantly affect the reliability and fairness of LLM assessments. Human judges, despite their expertise, can introduce subjective biases based on their perspectives, experiences, and expectations. For example, a human judge might favor responses that align with their personal views or overlook errors that they are less sensitive to.
Similarly, Grading Notes, while structured and comprehensive, can also be influenced by the biases of the individuals who design and implement them. Common biases include:
Position Bias: This occurs when the order in which responses are presented affects their evaluation. Responses shown first or last might receive more favorable ratings simply due to their placement. This can skew the evaluation results and lead to inaccurate assessments.
Verbosity Bias: Longer responses might be perceived as more informative or accurate, even if they contain unnecessary information. This can result in evaluators overvaluing length over quality, leading to biased assessments that do not accurately reflect the response's true merit.
Self-Enhancement Bias: Evaluators might unconsciously rate outputs generated by systems they favor or are more familiar with more positively. This bias can affect the objectivity of the evaluations, as personal preferences or familiarity with certain systems can influence judgment.
Mitigation Strategies for Biases
To mitigate biases in the evaluation process, several strategies can be implemented. Addressing position bias involves randomizing the order of responses during evaluations. This ensures that no response consistently benefits from being positioned at the beginning or end of the list.
For verbosity bias, setting clear guidelines on the expected length and detail of responses can help evaluators focus on the quality rather than the quantity of information. Effective mitigation strategies include:
Randomization: By randomizing the order in which responses are evaluated, you can prevent position bias. This means that evaluators will assess each response based on its content rather than its placement, leading to more accurate and fair evaluations.
Clear Guidelines: Establishing clear guidelines for response length and detail helps evaluators concentrate on the substance of the content rather than its length. This reduces verbosity bias, ensuring that evaluations are based on the quality of information rather than the quantity.
Blind Evaluation: Conducting evaluations without revealing which system generated the response can help mitigate self-enhancement bias. When evaluators do not know the source of the responses, they are more likely to judge the content objectively, leading to fairer and more reliable assessments.
Role of Iterative Consensus-Building and Cross-Annotation
Iterative consensus-building and cross-annotation are crucial for enhancing the reliability of evaluations. Consensus-building involves multiple evaluators reviewing the same responses and discussing their assessments to reach a common agreement.
This process helps to standardize evaluations and reduce individual biases.
Cross-annotation, where different evaluators assess the same set of responses, provides a way to measure and improve consistency among judges. Benefits of these practices include:
Bias Reduction: Multiple perspectives help identify and mitigate individual biases, leading to a fairer evaluation process. By involving several evaluators, the influence of any single evaluator's bias is diminished, resulting in a more balanced assessment.
Quality Assurance: Ongoing collaboration among evaluators ensures that the evaluation criteria are applied uniformly, maintaining high standards of assessment. This collaborative approach helps to uphold the quality of evaluations and ensures that the criteria are consistently and accurately applied.
By integrating iterative consensus-building and cross-annotation into your evaluation strategy, you can significantly improve the accuracy and fairness of LLM assessments.
Next, we will discuss the future direction of the scenario of LLM as a judge, focusing on how the industry can go forth with the integration and implementation of the same.
Future Directions
Looking ahead, there are several exciting opportunities for enhancing LLM-as-a-Judge capabilities and setting new benchmarks. Future developments may include more sophisticated evaluation metrics that can better capture the nuances of AI-generated content. Additionally, integrating advanced machine learning techniques can help further reduce biases and improve the accuracy of evaluations.
Potential enhancements include:
Advanced Metrics: Developing new metrics that provide deeper insights into the quality and relevance of AI outputs.
Machine Learning Integration: Using advanced algorithms to refine evaluation processes and reduce biases.
Expanded Use Cases: Applying LLM-as-a-Judge to a broader range of applications, from content generation to customer support.
The current scenario of LLM as a judge and the generative AI infrastructure is bound to evolve rapidly in the future given the benefits and advantages of the same.
Encouragement for Industry Collaboration
The ongoing improvement of LLM evaluation processes benefits greatly from industry collaboration. By sharing insights, methodologies, and best practices, you can contribute to the collective advancement of AI technologies.
Encouraging open communication and collaboration across the industry fosters innovation and helps set higher standards for AI performance and evaluation. Benefits of industry collaboration include:
Knowledge Sharing: Pooling resources and expertise to develop better evaluation methods and tools.
Standardization: Establishing common benchmarks and standards for LLM evaluation, ensuring consistency and reliability across different systems.
Innovation: Driving innovation through collaborative efforts, leading to more advanced and effective AI solutions.
By promoting continuous improvement and industry collaboration, you can help create a more robust and dynamic AI aspect, ultimately leading to more reliable and effective AI applications.
Conclusion
Automated tools are essential for improving the evaluation and performance of LLMs, providing accuracy, efficiency, and continuous improvement. By adopting these tools and fostering industry collaboration, you can ensure your AI applications consistently deliver high-quality results. Embracing future advancements will further enhance LLM capabilities, driving innovation and reliability in AI technologies.
Raga AI offers advanced automated tools designed to enhance the evaluation and performance of LLMs, ensuring high accuracy and efficiency. By integrating Raga AI's solutions like RAGA AI catalyst and RAGA AI prism, you can achieve continuous improvement and reliable results in your AI applications.
Start your journey with Raga AI today and take your AI applications to the next level.
LLM as a judge is becoming an essential tool in assessing the outputs of large language models (LLMs). Traditional evaluation methods often fall short when dealing with the complexity and nuance of AI-generated content, making automated solutions not just beneficial but necessary.
You might find that using automated tools to evaluate LLMs greatly enhances accuracy and efficiency. These tools can identify, diagnose, and fix issues in real time, providing a more reliable and streamlined evaluation process. Understanding how to implement and benefit from these automated tools effectively is crucial for anyone looking to improve the performance of their AI applications.

Source: Using LLMs for Evaluation
Let's explore the challenges of traditional evaluation methods and how innovative automated tools are addressing these issues.
Introduction to Using LLM-as-a-Judge as a Solution
LLM-as-a-Judge offers a practical and efficient solution to the challenges of evaluating LLM outputs. This method involves using a large language model to assess the performance of another LLM.
By doing so, you can combine the nuanced understanding typically associated with human judgment and the speed and scalability of automated systems. This approach ensures that evaluations are consistent and thorough, reducing the time and effort required for manual assessments.
Key benefits of using LLM-as-a-Judge include:
Automated Evaluations: Automates the process of evaluating AI outputs, which significantly reduces the need for manual labor and speeds up the evaluation process.
Consistency: Ensures consistent application of evaluation criteria across all assessments, leading to more reliable and comparable results.
Additionally, LLM-as-a-Judge enhances the quality of evaluations by using the advanced understanding and contextual awareness inherent in LLMs. In the rapidly evolving field of large language models (LLMs), ensuring the safety, reliability, and quality of model outputs is paramount hence users must understand how to properly evaluate LLM.
This means you can quickly identify and diagnose issues in AI outputs, such as context adherence, coherence, and overall relevance, without the variability and bias that might come from human evaluators.

Source: LLM as a Judge
Importance of Automated Tools
Automated tools are vital in making the evaluation of LLMs more efficient and reliable. These tools can handle large volumes of data quickly, identifying patterns and providing insights that would take much longer to discover manually.
By using automated tools and LLM as a judge, you can streamline your evaluation processes, reduce human error, and ensure that your AI applications are performing optimally.
Key advantages of automated evaluation tools include:
Speed and Efficiency: Automated tools can process vast datasets much faster than manual methods, allowing you to keep up with the high volume of outputs generated by LLMs. This speed is crucial for timely evaluations and quick iterations.
Reduced Human Error: Human evaluators can make mistakes, especially when dealing with repetitive tasks or large amounts of data. Automated tools help minimize these errors, ensuring more accurate and consistent evaluations.
Continuous Monitoring: Automated tools can provide real-time feedback and continuous monitoring of your AI systems. This constant oversight helps in promptly identifying and addressing any issues, leading to more stable and reliable AI performance.
Comprehensive Analysis: These tools can perform detailed and comprehensive analyses of AI outputs, identifying subtle issues and patterns that might be missed in manual reviews. This thoroughness leads to deeper insights and better overall performance.
By integrating automated tools into your evaluation strategy, you can enhance the accuracy and reliability of your AI assessments. This integration leads to more efficient processes, better performance, and a higher quality of AI applications.
Next, let's delve into the specific challenges faced in traditional evaluation methods and how automated solutions are transforming this methodology.
Challenges in Traditional Evaluation Methods
Evaluating LLM outputs through traditional methods poses several challenges that can hinder the accuracy and reliability of assessments. The complexity and diversity of criteria involved in evaluating AI-generated content make it difficult to apply consistent and effective measures.
Additionally, traditional rule-based assessments and metrics often fall short of capturing the nuances of AI responses. Let's explore the challenges, complexity, and other aspects in detail below.
Complexity and Diversity in Traditional Evaluations
Evaluating the performance of LLMs involves considering a wide range of criteria, each with its own set of complexities. From context adherence to grammatical accuracy, the diversity of factors that need to be assessed can be overwhelming.
This complexity often leads to inconsistent evaluations, as human reviewers may struggle to apply the same standards across different assessments. Key complexities include:
Context Adherence: Ensuring the response is relevant to the given context.
Grammar and Syntax: Checking for grammatical correctness and proper sentence structure.
Coherence and Flow: Assessing the logical flow and coherence of the response.
Limitations of Rule-Based Assessments and Traditional Metrics
Rule-based assessments and traditional metrics such as ROUGE and BLEU are commonly used in evaluating LLM outputs, but they have significant limitations. These methods primarily focus on surface-level features and often miss deeper aspects of content quality.
For instance, they might accurately measure similarity to a reference text but fail to capture the semantic relevance or the overall coherence of the response. Limitations include:
Surface-Level Focus: Metrics like ROUGE and BLEU emphasize word overlap and syntactic similarity, neglecting deeper semantic meaning.
Lack of Nuance: These methods do not account for the nuances of context, tone, and intent in AI-generated responses.
Inflexibility: Rule-based assessments are rigid and cannot adapt to the diverse and evolving nature of AI outputs.
Examples of Common Issues in AI Assistant Responses
AI assistant responses often face several common issues that traditional evaluation methods struggle to address effectively. These issues can significantly impact the user experience and the perceived quality of the AI application.
Common issues include:
Context Misalignment: Responses that are not aligned with the given context or prompt.
Repetition: Unnecessary repetition of words or phrases, leading to redundancy.
Excessive Length: Responses that are overly long and verbose, lacking conciseness.
Lack of Coherence: Incoherent responses that do not logically flow from one point to another.
Addressing these issues requires more advanced evaluation methods that can capture the subtleties and nuances of AI-generated content.
Next, we'll explore the concept of automated tools for LLM-as-a-Judge and how it can provide a solution to these challenges.
Introduction to Automated Tools for LLM-as-a-Judge
Automated tools are essential for enhancing the capabilities of LLM-as-a-Judge. These tools can streamline the evaluation process, making it more efficient and comprehensive.
By integrating advanced metrics and evaluation frameworks, automated tools can provide deeper insights into the performance of LLMs, highlighting areas for improvement and ensuring that AI applications meet high standards of quality.
Benefits of using automated tools with LLM-as-a-Judge include:
Enhanced Precision: Automated tools can detect subtle issues and provide detailed feedback that might be missed in manual evaluations. This precise feedback helps in pinpointing specific areas that need improvement, ensuring that the LLM outputs are of the highest quality.
Scalability: These tools can handle large volumes of data, making it feasible to evaluate extensive datasets quickly. This scalability is crucial for businesses that need to process and evaluate large amounts of data without compromising on the quality of the assessments.
Continuous Improvement: Automated tools can continuously monitor and evaluate LLM outputs, providing real-time feedback that helps in iterative improvement. This ongoing evaluation process ensures that your AI systems are constantly being refined and improved, leading to better performance over time.
Next, we'll delve into the automated tools specifically designed to enhance the LLM-as-a-Judge process.
Automated Tools for Enhancing LLM-as-a-Judge
Grading Notes are a valuable tool for enhancing the LLM-as-a-Judge process. These notes provide detailed, structured feedback on AI-generated content, helping to identify specific strengths and weaknesses.
By using Grading Notes, you can ensure that your evaluations are comprehensive and focused on the most critical aspects of performance. Benefits of Grading Notes include:
Structured Feedback: Offers clear and organized feedback, making it easier to identify areas that need improvement.
Detailed Analysis: Provides in-depth insights into the performance of LLMs, helping to refine and improve AI models.
With Grading Notes, you can enhance the accuracy and reliability of your LLM evaluations, leading to better overall performance of your AI systems.
Introduction to Other Automated Tools
In addition to Grading Notes, other automated tools such as MT-Bench and Chatbot Arena play a crucial role in enhancing the capabilities of LLM-as-a-Judge. Each tool has unique features that contribute to a comprehensive evaluation framework for LLMs.

Pic: MT-Bench and Chabot Arena (Source: Link)
MT-Bench:
MT-Bench is specifically designed to benchmark the performance of LLMs across a variety of tasks, providing a detailed view of their strengths and weaknesses. This tool evaluates multiple dimensions of LLM performance, ensuring a thorough assessment that covers all critical aspects.
Pic: Average Win Rate of Six Models Under Different Judges in the MT-Bench

(Source: Link)
Comprehensive Benchmarking: MT-Bench covers a wide array of tasks, from simple question answering to complex problem-solving, giving you a full picture of the LLM's capabilities.
Task-Specific Insights: It breaks down performance by task, highlighting specific areas where the LLM excels or needs improvement. This granularity is essential for fine-tuning models to meet particular needs.
Performance Comparison: MT-Bench allows for direct comparisons between different LLMs, helping you choose the best model for your specific requirements. This comparison is based on clear, objective criteria, ensuring that your decision-making is well-informed.
Chatbot Arena:
Chatbot Arena focuses on evaluating conversational agents, ensuring they deliver high-quality, coherent, and contextually relevant interactions. This tool is essential for businesses that rely on chatbots to interact with customers, as it helps ensure that these interactions are effective and satisfactory.

Pic: A screenshot of the Chatbot Arena interface (Source: Link)
Specialized Evaluation: Chatbot Arena assesses the conversational abilities of chatbots, checking for coherence, relevance, and responsiveness. This specialized focus ensures that the chatbots perform well in real-world scenarios.
User Experience Focus: The tool evaluates interactions from the user's perspective, providing insights into how users perceive and interact with the chatbot. This feedback is crucial for improving user satisfaction and engagement.
Dialogue Coherence: Chatbot Arena ensures that the chatbot maintains context and logical flow throughout the conversation, reducing misunderstandings and enhancing the overall interaction quality. This coherence is key to creating a seamless and natural user experience.
Addressing Limitations and Biases Using Automated Tools
Automated tools are instrumental in addressing the limitations and biases inherent in traditional evaluation methods. By using these tools, you can ensure that your LLM evaluations are more objective, consistent, and comprehensive.
How automated tools address limitations and biases:
Objective Assessments: Automated tools provide unbiased evaluations based on predefined criteria, reducing the influence of subjective judgments.
Consistency: Ensures that the same evaluation standards are applied across all assessments, minimizing variability.
Comprehensive Analysis: Detects subtle issues and provides detailed feedback, ensuring that all aspects of LLM performance are thoroughly evaluated.
Improving Alignment Rates and Accuracy with Tools
Grading Notes and other automated tools significantly enhance the alignment rates and accuracy when evaluating long-form outputs from LLMs. These tools provide a structured framework for feedback, ensuring that evaluations are both detailed and actionable.
By aligning LLM outputs with predefined criteria, these tools ensure that the responses meet the required standards consistently. This structured approach helps identify specific areas for improvement, allowing for targeted enhancements in LLM performance.
Advantages of using Grading Notes and other automated tools include:
Improved Alignment: Automated tools help ensure that LLM outputs align with predefined criteria, maintaining a consistent quality across all evaluations. This alignment is crucial for meeting the standards expected in professional and practical applications.
Higher Accuracy: Tools like Grading Notes provide detailed feedback, highlighting subtle issues that might be overlooked in manual evaluations. This detailed analysis leads to more accurate assessments, improving the overall quality of LLM outputs.
Using Grading Notes and other automated tools enhances the overall quality of LLM evaluations, leading to more reliable and effective AI applications.
Next, we'll delve into how important human labeling is in improving consistency and alignment.
Improving Evaluation Consistency and Alignment
Human labeling is crucial for measuring the alignment rates of LLM judges. This process involves human evaluators reviewing AI-generated outputs and providing feedback on their accuracy and relevance. By comparing these human assessments with the evaluations made by LLMs, you can determine how well the AI aligns with human judgment.
This comparison helps in identifying any discrepancies and areas where the AI might need improvement. The human labeling process typically includes the following:
Selection of Evaluation Criteria: Defining the specific criteria that human evaluators will use to assess the AI outputs.
Training Human Evaluators: Ensuring that the evaluators understand the criteria and can apply them consistently.
Cross-Checking Results: Comparing the evaluations from multiple human reviewers to ensure consistency and accuracy.
Read our detailed article on the methods and metrics of evaluating Large language models for more information.
Agreement Rates Between LLM Judges and Human Judges
Understanding the agreement rates between LLM judges and human judges is critical for evaluating the reliability of AI assessments. By analyzing these rates, you can determine how closely LLM evaluations match those of human experts. High agreement rates indicate that the
LLM is effectively mimicking human judgment, while low rates suggest areas for improvement. This analysis involves:
Collecting Data: Gathering a substantial amount of evaluation data from both LLM judges and human judges.
Statistical Comparison: Using statistical methods to compare the evaluations and calculate agreement rates.
Identifying Discrepancies: Highlighting any significant differences in judgments and exploring the reasons behind them.
By regularly analyzing agreement rates, you can continuously refine the LLM evaluation process, ensuring that it remains aligned with human standards.
Next, we'll explore how to address biases and limitations involved in the evaluation process of LLM as a judge with automated tools involved.
Addressing Biases and Limitations
Biases in the evaluation process can significantly affect the reliability and fairness of LLM assessments. Human judges, despite their expertise, can introduce subjective biases based on their perspectives, experiences, and expectations. For example, a human judge might favor responses that align with their personal views or overlook errors that they are less sensitive to.
Similarly, Grading Notes, while structured and comprehensive, can also be influenced by the biases of the individuals who design and implement them. Common biases include:
Position Bias: This occurs when the order in which responses are presented affects their evaluation. Responses shown first or last might receive more favorable ratings simply due to their placement. This can skew the evaluation results and lead to inaccurate assessments.
Verbosity Bias: Longer responses might be perceived as more informative or accurate, even if they contain unnecessary information. This can result in evaluators overvaluing length over quality, leading to biased assessments that do not accurately reflect the response's true merit.
Self-Enhancement Bias: Evaluators might unconsciously rate outputs generated by systems they favor or are more familiar with more positively. This bias can affect the objectivity of the evaluations, as personal preferences or familiarity with certain systems can influence judgment.
Mitigation Strategies for Biases
To mitigate biases in the evaluation process, several strategies can be implemented. Addressing position bias involves randomizing the order of responses during evaluations. This ensures that no response consistently benefits from being positioned at the beginning or end of the list.
For verbosity bias, setting clear guidelines on the expected length and detail of responses can help evaluators focus on the quality rather than the quantity of information. Effective mitigation strategies include:
Randomization: By randomizing the order in which responses are evaluated, you can prevent position bias. This means that evaluators will assess each response based on its content rather than its placement, leading to more accurate and fair evaluations.
Clear Guidelines: Establishing clear guidelines for response length and detail helps evaluators concentrate on the substance of the content rather than its length. This reduces verbosity bias, ensuring that evaluations are based on the quality of information rather than the quantity.
Blind Evaluation: Conducting evaluations without revealing which system generated the response can help mitigate self-enhancement bias. When evaluators do not know the source of the responses, they are more likely to judge the content objectively, leading to fairer and more reliable assessments.
Role of Iterative Consensus-Building and Cross-Annotation
Iterative consensus-building and cross-annotation are crucial for enhancing the reliability of evaluations. Consensus-building involves multiple evaluators reviewing the same responses and discussing their assessments to reach a common agreement.
This process helps to standardize evaluations and reduce individual biases.
Cross-annotation, where different evaluators assess the same set of responses, provides a way to measure and improve consistency among judges. Benefits of these practices include:
Bias Reduction: Multiple perspectives help identify and mitigate individual biases, leading to a fairer evaluation process. By involving several evaluators, the influence of any single evaluator's bias is diminished, resulting in a more balanced assessment.
Quality Assurance: Ongoing collaboration among evaluators ensures that the evaluation criteria are applied uniformly, maintaining high standards of assessment. This collaborative approach helps to uphold the quality of evaluations and ensures that the criteria are consistently and accurately applied.
By integrating iterative consensus-building and cross-annotation into your evaluation strategy, you can significantly improve the accuracy and fairness of LLM assessments.
Next, we will discuss the future direction of the scenario of LLM as a judge, focusing on how the industry can go forth with the integration and implementation of the same.
Future Directions
Looking ahead, there are several exciting opportunities for enhancing LLM-as-a-Judge capabilities and setting new benchmarks. Future developments may include more sophisticated evaluation metrics that can better capture the nuances of AI-generated content. Additionally, integrating advanced machine learning techniques can help further reduce biases and improve the accuracy of evaluations.
Potential enhancements include:
Advanced Metrics: Developing new metrics that provide deeper insights into the quality and relevance of AI outputs.
Machine Learning Integration: Using advanced algorithms to refine evaluation processes and reduce biases.
Expanded Use Cases: Applying LLM-as-a-Judge to a broader range of applications, from content generation to customer support.
The current scenario of LLM as a judge and the generative AI infrastructure is bound to evolve rapidly in the future given the benefits and advantages of the same.
Encouragement for Industry Collaboration
The ongoing improvement of LLM evaluation processes benefits greatly from industry collaboration. By sharing insights, methodologies, and best practices, you can contribute to the collective advancement of AI technologies.
Encouraging open communication and collaboration across the industry fosters innovation and helps set higher standards for AI performance and evaluation. Benefits of industry collaboration include:
Knowledge Sharing: Pooling resources and expertise to develop better evaluation methods and tools.
Standardization: Establishing common benchmarks and standards for LLM evaluation, ensuring consistency and reliability across different systems.
Innovation: Driving innovation through collaborative efforts, leading to more advanced and effective AI solutions.
By promoting continuous improvement and industry collaboration, you can help create a more robust and dynamic AI aspect, ultimately leading to more reliable and effective AI applications.
Conclusion
Automated tools are essential for improving the evaluation and performance of LLMs, providing accuracy, efficiency, and continuous improvement. By adopting these tools and fostering industry collaboration, you can ensure your AI applications consistently deliver high-quality results. Embracing future advancements will further enhance LLM capabilities, driving innovation and reliability in AI technologies.
Raga AI offers advanced automated tools designed to enhance the evaluation and performance of LLMs, ensuring high accuracy and efficiency. By integrating Raga AI's solutions like RAGA AI catalyst and RAGA AI prism, you can achieve continuous improvement and reliable results in your AI applications.
Start your journey with Raga AI today and take your AI applications to the next level.
LLM as a judge is becoming an essential tool in assessing the outputs of large language models (LLMs). Traditional evaluation methods often fall short when dealing with the complexity and nuance of AI-generated content, making automated solutions not just beneficial but necessary.
You might find that using automated tools to evaluate LLMs greatly enhances accuracy and efficiency. These tools can identify, diagnose, and fix issues in real time, providing a more reliable and streamlined evaluation process. Understanding how to implement and benefit from these automated tools effectively is crucial for anyone looking to improve the performance of their AI applications.

Source: Using LLMs for Evaluation
Let's explore the challenges of traditional evaluation methods and how innovative automated tools are addressing these issues.
Introduction to Using LLM-as-a-Judge as a Solution
LLM-as-a-Judge offers a practical and efficient solution to the challenges of evaluating LLM outputs. This method involves using a large language model to assess the performance of another LLM.
By doing so, you can combine the nuanced understanding typically associated with human judgment and the speed and scalability of automated systems. This approach ensures that evaluations are consistent and thorough, reducing the time and effort required for manual assessments.
Key benefits of using LLM-as-a-Judge include:
Automated Evaluations: Automates the process of evaluating AI outputs, which significantly reduces the need for manual labor and speeds up the evaluation process.
Consistency: Ensures consistent application of evaluation criteria across all assessments, leading to more reliable and comparable results.
Additionally, LLM-as-a-Judge enhances the quality of evaluations by using the advanced understanding and contextual awareness inherent in LLMs. In the rapidly evolving field of large language models (LLMs), ensuring the safety, reliability, and quality of model outputs is paramount hence users must understand how to properly evaluate LLM.
This means you can quickly identify and diagnose issues in AI outputs, such as context adherence, coherence, and overall relevance, without the variability and bias that might come from human evaluators.

Source: LLM as a Judge
Importance of Automated Tools
Automated tools are vital in making the evaluation of LLMs more efficient and reliable. These tools can handle large volumes of data quickly, identifying patterns and providing insights that would take much longer to discover manually.
By using automated tools and LLM as a judge, you can streamline your evaluation processes, reduce human error, and ensure that your AI applications are performing optimally.
Key advantages of automated evaluation tools include:
Speed and Efficiency: Automated tools can process vast datasets much faster than manual methods, allowing you to keep up with the high volume of outputs generated by LLMs. This speed is crucial for timely evaluations and quick iterations.
Reduced Human Error: Human evaluators can make mistakes, especially when dealing with repetitive tasks or large amounts of data. Automated tools help minimize these errors, ensuring more accurate and consistent evaluations.
Continuous Monitoring: Automated tools can provide real-time feedback and continuous monitoring of your AI systems. This constant oversight helps in promptly identifying and addressing any issues, leading to more stable and reliable AI performance.
Comprehensive Analysis: These tools can perform detailed and comprehensive analyses of AI outputs, identifying subtle issues and patterns that might be missed in manual reviews. This thoroughness leads to deeper insights and better overall performance.
By integrating automated tools into your evaluation strategy, you can enhance the accuracy and reliability of your AI assessments. This integration leads to more efficient processes, better performance, and a higher quality of AI applications.
Next, let's delve into the specific challenges faced in traditional evaluation methods and how automated solutions are transforming this methodology.
Challenges in Traditional Evaluation Methods
Evaluating LLM outputs through traditional methods poses several challenges that can hinder the accuracy and reliability of assessments. The complexity and diversity of criteria involved in evaluating AI-generated content make it difficult to apply consistent and effective measures.
Additionally, traditional rule-based assessments and metrics often fall short of capturing the nuances of AI responses. Let's explore the challenges, complexity, and other aspects in detail below.
Complexity and Diversity in Traditional Evaluations
Evaluating the performance of LLMs involves considering a wide range of criteria, each with its own set of complexities. From context adherence to grammatical accuracy, the diversity of factors that need to be assessed can be overwhelming.
This complexity often leads to inconsistent evaluations, as human reviewers may struggle to apply the same standards across different assessments. Key complexities include:
Context Adherence: Ensuring the response is relevant to the given context.
Grammar and Syntax: Checking for grammatical correctness and proper sentence structure.
Coherence and Flow: Assessing the logical flow and coherence of the response.
Limitations of Rule-Based Assessments and Traditional Metrics
Rule-based assessments and traditional metrics such as ROUGE and BLEU are commonly used in evaluating LLM outputs, but they have significant limitations. These methods primarily focus on surface-level features and often miss deeper aspects of content quality.
For instance, they might accurately measure similarity to a reference text but fail to capture the semantic relevance or the overall coherence of the response. Limitations include:
Surface-Level Focus: Metrics like ROUGE and BLEU emphasize word overlap and syntactic similarity, neglecting deeper semantic meaning.
Lack of Nuance: These methods do not account for the nuances of context, tone, and intent in AI-generated responses.
Inflexibility: Rule-based assessments are rigid and cannot adapt to the diverse and evolving nature of AI outputs.
Examples of Common Issues in AI Assistant Responses
AI assistant responses often face several common issues that traditional evaluation methods struggle to address effectively. These issues can significantly impact the user experience and the perceived quality of the AI application.
Common issues include:
Context Misalignment: Responses that are not aligned with the given context or prompt.
Repetition: Unnecessary repetition of words or phrases, leading to redundancy.
Excessive Length: Responses that are overly long and verbose, lacking conciseness.
Lack of Coherence: Incoherent responses that do not logically flow from one point to another.
Addressing these issues requires more advanced evaluation methods that can capture the subtleties and nuances of AI-generated content.
Next, we'll explore the concept of automated tools for LLM-as-a-Judge and how it can provide a solution to these challenges.
Introduction to Automated Tools for LLM-as-a-Judge
Automated tools are essential for enhancing the capabilities of LLM-as-a-Judge. These tools can streamline the evaluation process, making it more efficient and comprehensive.
By integrating advanced metrics and evaluation frameworks, automated tools can provide deeper insights into the performance of LLMs, highlighting areas for improvement and ensuring that AI applications meet high standards of quality.
Benefits of using automated tools with LLM-as-a-Judge include:
Enhanced Precision: Automated tools can detect subtle issues and provide detailed feedback that might be missed in manual evaluations. This precise feedback helps in pinpointing specific areas that need improvement, ensuring that the LLM outputs are of the highest quality.
Scalability: These tools can handle large volumes of data, making it feasible to evaluate extensive datasets quickly. This scalability is crucial for businesses that need to process and evaluate large amounts of data without compromising on the quality of the assessments.
Continuous Improvement: Automated tools can continuously monitor and evaluate LLM outputs, providing real-time feedback that helps in iterative improvement. This ongoing evaluation process ensures that your AI systems are constantly being refined and improved, leading to better performance over time.
Next, we'll delve into the automated tools specifically designed to enhance the LLM-as-a-Judge process.
Automated Tools for Enhancing LLM-as-a-Judge
Grading Notes are a valuable tool for enhancing the LLM-as-a-Judge process. These notes provide detailed, structured feedback on AI-generated content, helping to identify specific strengths and weaknesses.
By using Grading Notes, you can ensure that your evaluations are comprehensive and focused on the most critical aspects of performance. Benefits of Grading Notes include:
Structured Feedback: Offers clear and organized feedback, making it easier to identify areas that need improvement.
Detailed Analysis: Provides in-depth insights into the performance of LLMs, helping to refine and improve AI models.
With Grading Notes, you can enhance the accuracy and reliability of your LLM evaluations, leading to better overall performance of your AI systems.
Introduction to Other Automated Tools
In addition to Grading Notes, other automated tools such as MT-Bench and Chatbot Arena play a crucial role in enhancing the capabilities of LLM-as-a-Judge. Each tool has unique features that contribute to a comprehensive evaluation framework for LLMs.

Pic: MT-Bench and Chabot Arena (Source: Link)
MT-Bench:
MT-Bench is specifically designed to benchmark the performance of LLMs across a variety of tasks, providing a detailed view of their strengths and weaknesses. This tool evaluates multiple dimensions of LLM performance, ensuring a thorough assessment that covers all critical aspects.
Pic: Average Win Rate of Six Models Under Different Judges in the MT-Bench

(Source: Link)
Comprehensive Benchmarking: MT-Bench covers a wide array of tasks, from simple question answering to complex problem-solving, giving you a full picture of the LLM's capabilities.
Task-Specific Insights: It breaks down performance by task, highlighting specific areas where the LLM excels or needs improvement. This granularity is essential for fine-tuning models to meet particular needs.
Performance Comparison: MT-Bench allows for direct comparisons between different LLMs, helping you choose the best model for your specific requirements. This comparison is based on clear, objective criteria, ensuring that your decision-making is well-informed.
Chatbot Arena:
Chatbot Arena focuses on evaluating conversational agents, ensuring they deliver high-quality, coherent, and contextually relevant interactions. This tool is essential for businesses that rely on chatbots to interact with customers, as it helps ensure that these interactions are effective and satisfactory.

Pic: A screenshot of the Chatbot Arena interface (Source: Link)
Specialized Evaluation: Chatbot Arena assesses the conversational abilities of chatbots, checking for coherence, relevance, and responsiveness. This specialized focus ensures that the chatbots perform well in real-world scenarios.
User Experience Focus: The tool evaluates interactions from the user's perspective, providing insights into how users perceive and interact with the chatbot. This feedback is crucial for improving user satisfaction and engagement.
Dialogue Coherence: Chatbot Arena ensures that the chatbot maintains context and logical flow throughout the conversation, reducing misunderstandings and enhancing the overall interaction quality. This coherence is key to creating a seamless and natural user experience.
Addressing Limitations and Biases Using Automated Tools
Automated tools are instrumental in addressing the limitations and biases inherent in traditional evaluation methods. By using these tools, you can ensure that your LLM evaluations are more objective, consistent, and comprehensive.
How automated tools address limitations and biases:
Objective Assessments: Automated tools provide unbiased evaluations based on predefined criteria, reducing the influence of subjective judgments.
Consistency: Ensures that the same evaluation standards are applied across all assessments, minimizing variability.
Comprehensive Analysis: Detects subtle issues and provides detailed feedback, ensuring that all aspects of LLM performance are thoroughly evaluated.
Improving Alignment Rates and Accuracy with Tools
Grading Notes and other automated tools significantly enhance the alignment rates and accuracy when evaluating long-form outputs from LLMs. These tools provide a structured framework for feedback, ensuring that evaluations are both detailed and actionable.
By aligning LLM outputs with predefined criteria, these tools ensure that the responses meet the required standards consistently. This structured approach helps identify specific areas for improvement, allowing for targeted enhancements in LLM performance.
Advantages of using Grading Notes and other automated tools include:
Improved Alignment: Automated tools help ensure that LLM outputs align with predefined criteria, maintaining a consistent quality across all evaluations. This alignment is crucial for meeting the standards expected in professional and practical applications.
Higher Accuracy: Tools like Grading Notes provide detailed feedback, highlighting subtle issues that might be overlooked in manual evaluations. This detailed analysis leads to more accurate assessments, improving the overall quality of LLM outputs.
Using Grading Notes and other automated tools enhances the overall quality of LLM evaluations, leading to more reliable and effective AI applications.
Next, we'll delve into how important human labeling is in improving consistency and alignment.
Improving Evaluation Consistency and Alignment
Human labeling is crucial for measuring the alignment rates of LLM judges. This process involves human evaluators reviewing AI-generated outputs and providing feedback on their accuracy and relevance. By comparing these human assessments with the evaluations made by LLMs, you can determine how well the AI aligns with human judgment.
This comparison helps in identifying any discrepancies and areas where the AI might need improvement. The human labeling process typically includes the following:
Selection of Evaluation Criteria: Defining the specific criteria that human evaluators will use to assess the AI outputs.
Training Human Evaluators: Ensuring that the evaluators understand the criteria and can apply them consistently.
Cross-Checking Results: Comparing the evaluations from multiple human reviewers to ensure consistency and accuracy.
Read our detailed article on the methods and metrics of evaluating Large language models for more information.
Agreement Rates Between LLM Judges and Human Judges
Understanding the agreement rates between LLM judges and human judges is critical for evaluating the reliability of AI assessments. By analyzing these rates, you can determine how closely LLM evaluations match those of human experts. High agreement rates indicate that the
LLM is effectively mimicking human judgment, while low rates suggest areas for improvement. This analysis involves:
Collecting Data: Gathering a substantial amount of evaluation data from both LLM judges and human judges.
Statistical Comparison: Using statistical methods to compare the evaluations and calculate agreement rates.
Identifying Discrepancies: Highlighting any significant differences in judgments and exploring the reasons behind them.
By regularly analyzing agreement rates, you can continuously refine the LLM evaluation process, ensuring that it remains aligned with human standards.
Next, we'll explore how to address biases and limitations involved in the evaluation process of LLM as a judge with automated tools involved.
Addressing Biases and Limitations
Biases in the evaluation process can significantly affect the reliability and fairness of LLM assessments. Human judges, despite their expertise, can introduce subjective biases based on their perspectives, experiences, and expectations. For example, a human judge might favor responses that align with their personal views or overlook errors that they are less sensitive to.
Similarly, Grading Notes, while structured and comprehensive, can also be influenced by the biases of the individuals who design and implement them. Common biases include:
Position Bias: This occurs when the order in which responses are presented affects their evaluation. Responses shown first or last might receive more favorable ratings simply due to their placement. This can skew the evaluation results and lead to inaccurate assessments.
Verbosity Bias: Longer responses might be perceived as more informative or accurate, even if they contain unnecessary information. This can result in evaluators overvaluing length over quality, leading to biased assessments that do not accurately reflect the response's true merit.
Self-Enhancement Bias: Evaluators might unconsciously rate outputs generated by systems they favor or are more familiar with more positively. This bias can affect the objectivity of the evaluations, as personal preferences or familiarity with certain systems can influence judgment.
Mitigation Strategies for Biases
To mitigate biases in the evaluation process, several strategies can be implemented. Addressing position bias involves randomizing the order of responses during evaluations. This ensures that no response consistently benefits from being positioned at the beginning or end of the list.
For verbosity bias, setting clear guidelines on the expected length and detail of responses can help evaluators focus on the quality rather than the quantity of information. Effective mitigation strategies include:
Randomization: By randomizing the order in which responses are evaluated, you can prevent position bias. This means that evaluators will assess each response based on its content rather than its placement, leading to more accurate and fair evaluations.
Clear Guidelines: Establishing clear guidelines for response length and detail helps evaluators concentrate on the substance of the content rather than its length. This reduces verbosity bias, ensuring that evaluations are based on the quality of information rather than the quantity.
Blind Evaluation: Conducting evaluations without revealing which system generated the response can help mitigate self-enhancement bias. When evaluators do not know the source of the responses, they are more likely to judge the content objectively, leading to fairer and more reliable assessments.
Role of Iterative Consensus-Building and Cross-Annotation
Iterative consensus-building and cross-annotation are crucial for enhancing the reliability of evaluations. Consensus-building involves multiple evaluators reviewing the same responses and discussing their assessments to reach a common agreement.
This process helps to standardize evaluations and reduce individual biases.
Cross-annotation, where different evaluators assess the same set of responses, provides a way to measure and improve consistency among judges. Benefits of these practices include:
Bias Reduction: Multiple perspectives help identify and mitigate individual biases, leading to a fairer evaluation process. By involving several evaluators, the influence of any single evaluator's bias is diminished, resulting in a more balanced assessment.
Quality Assurance: Ongoing collaboration among evaluators ensures that the evaluation criteria are applied uniformly, maintaining high standards of assessment. This collaborative approach helps to uphold the quality of evaluations and ensures that the criteria are consistently and accurately applied.
By integrating iterative consensus-building and cross-annotation into your evaluation strategy, you can significantly improve the accuracy and fairness of LLM assessments.
Next, we will discuss the future direction of the scenario of LLM as a judge, focusing on how the industry can go forth with the integration and implementation of the same.
Future Directions
Looking ahead, there are several exciting opportunities for enhancing LLM-as-a-Judge capabilities and setting new benchmarks. Future developments may include more sophisticated evaluation metrics that can better capture the nuances of AI-generated content. Additionally, integrating advanced machine learning techniques can help further reduce biases and improve the accuracy of evaluations.
Potential enhancements include:
Advanced Metrics: Developing new metrics that provide deeper insights into the quality and relevance of AI outputs.
Machine Learning Integration: Using advanced algorithms to refine evaluation processes and reduce biases.
Expanded Use Cases: Applying LLM-as-a-Judge to a broader range of applications, from content generation to customer support.
The current scenario of LLM as a judge and the generative AI infrastructure is bound to evolve rapidly in the future given the benefits and advantages of the same.
Encouragement for Industry Collaboration
The ongoing improvement of LLM evaluation processes benefits greatly from industry collaboration. By sharing insights, methodologies, and best practices, you can contribute to the collective advancement of AI technologies.
Encouraging open communication and collaboration across the industry fosters innovation and helps set higher standards for AI performance and evaluation. Benefits of industry collaboration include:
Knowledge Sharing: Pooling resources and expertise to develop better evaluation methods and tools.
Standardization: Establishing common benchmarks and standards for LLM evaluation, ensuring consistency and reliability across different systems.
Innovation: Driving innovation through collaborative efforts, leading to more advanced and effective AI solutions.
By promoting continuous improvement and industry collaboration, you can help create a more robust and dynamic AI aspect, ultimately leading to more reliable and effective AI applications.
Conclusion
Automated tools are essential for improving the evaluation and performance of LLMs, providing accuracy, efficiency, and continuous improvement. By adopting these tools and fostering industry collaboration, you can ensure your AI applications consistently deliver high-quality results. Embracing future advancements will further enhance LLM capabilities, driving innovation and reliability in AI technologies.
Raga AI offers advanced automated tools designed to enhance the evaluation and performance of LLMs, ensuring high accuracy and efficiency. By integrating Raga AI's solutions like RAGA AI catalyst and RAGA AI prism, you can achieve continuous improvement and reliable results in your AI applications.
Start your journey with Raga AI today and take your AI applications to the next level.
Subscribe to our newsletter to never miss an update
Other articles


Agents Talk, Models Think: A2A + MCP for Enterprise Agentic AI

Riya Parikh, Nitai Agarwal
Apr 27, 2025
Read the article
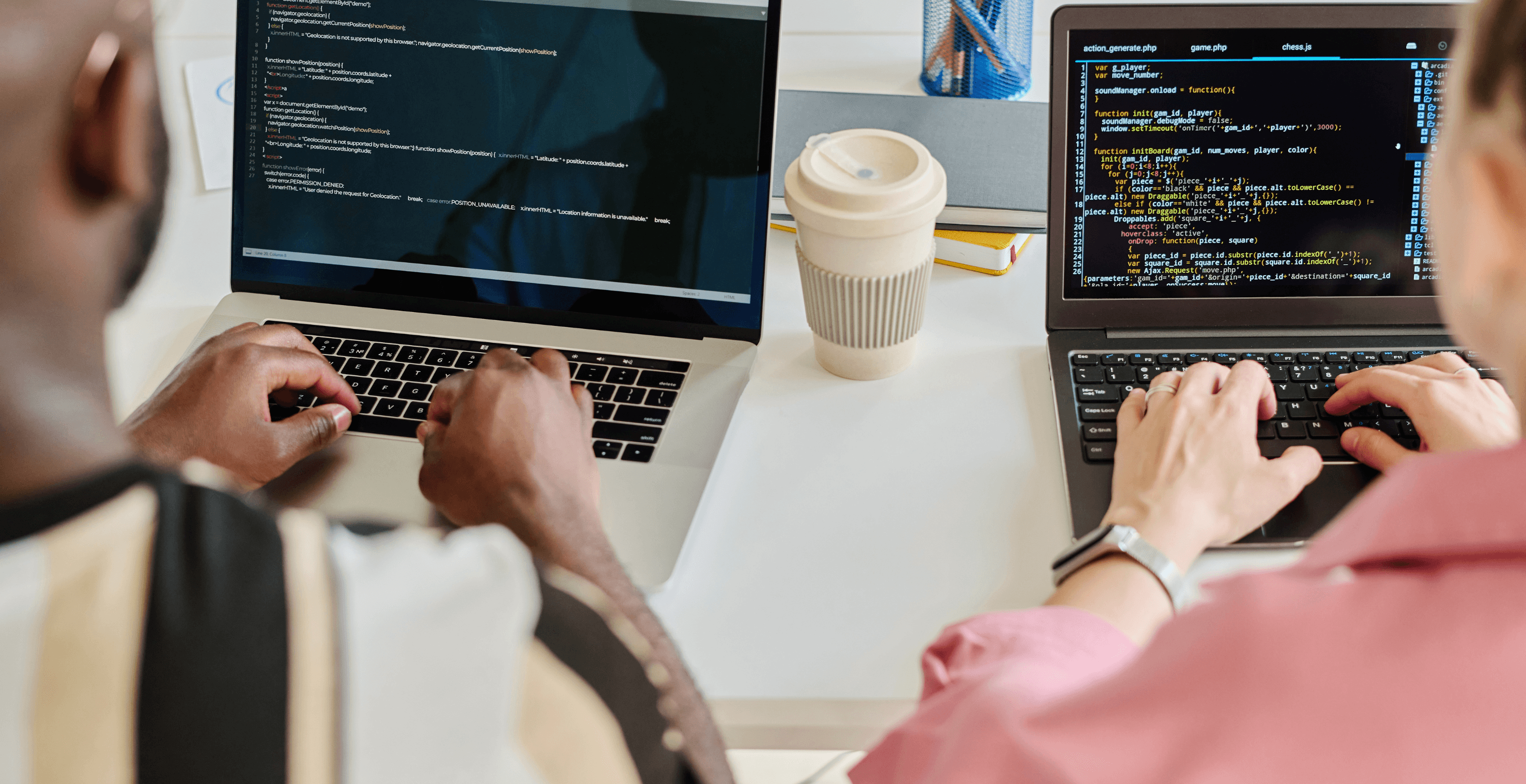
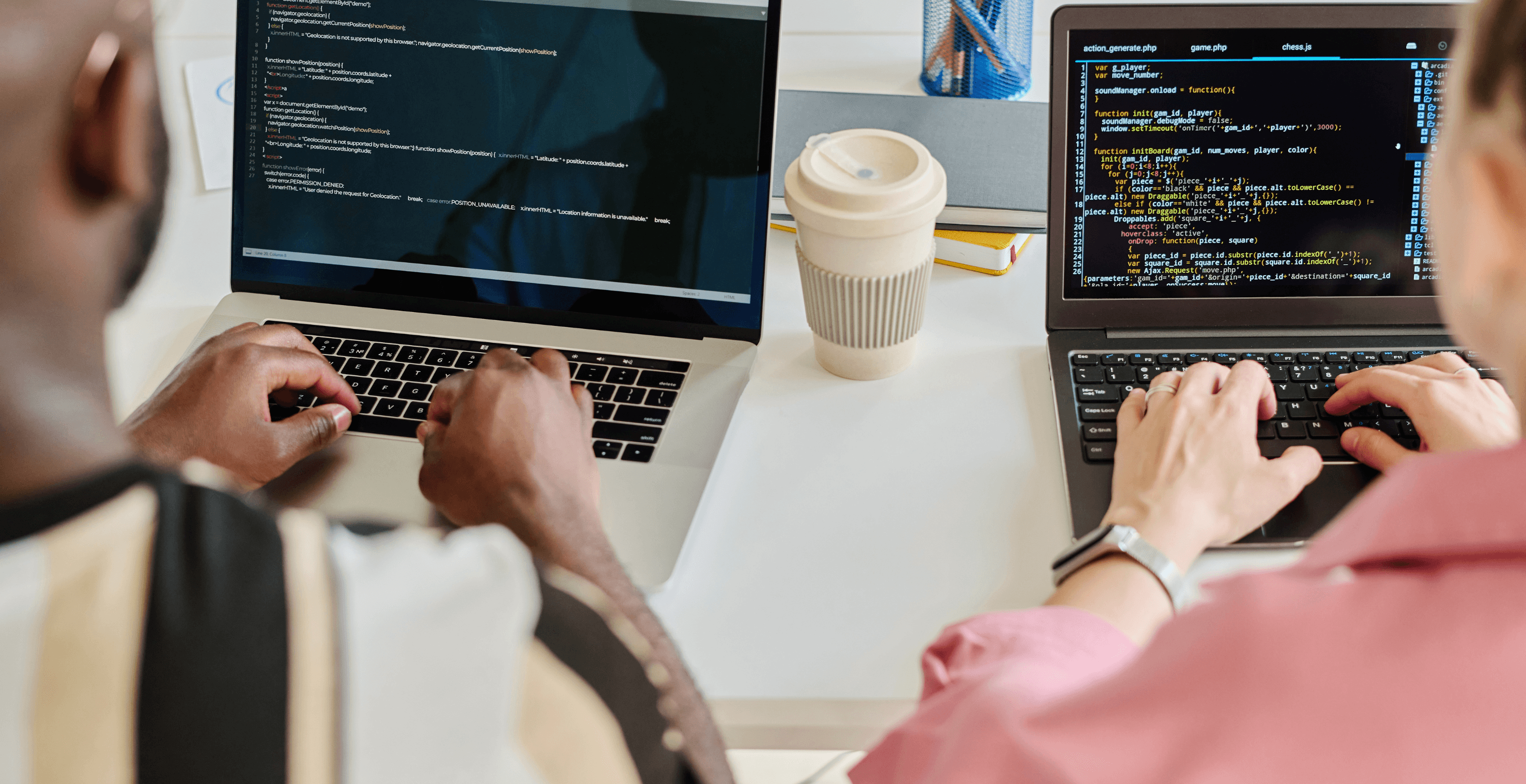
Understanding NeMo Guardrails: A Toolkit for LLM Security

Rehan Asif
Dec 24, 2024
Read the article


Understanding Differences in Large vs Small Language Models (LLM vs SLM)

Rehan Asif
Dec 21, 2024
Read the article