Overview Of Key Concepts In AI Safety And Security
Overview Of Key Concepts In AI Safety And Security
Overview Of Key Concepts In AI Safety And Security
Jigar Gupta
Apr 12, 2024
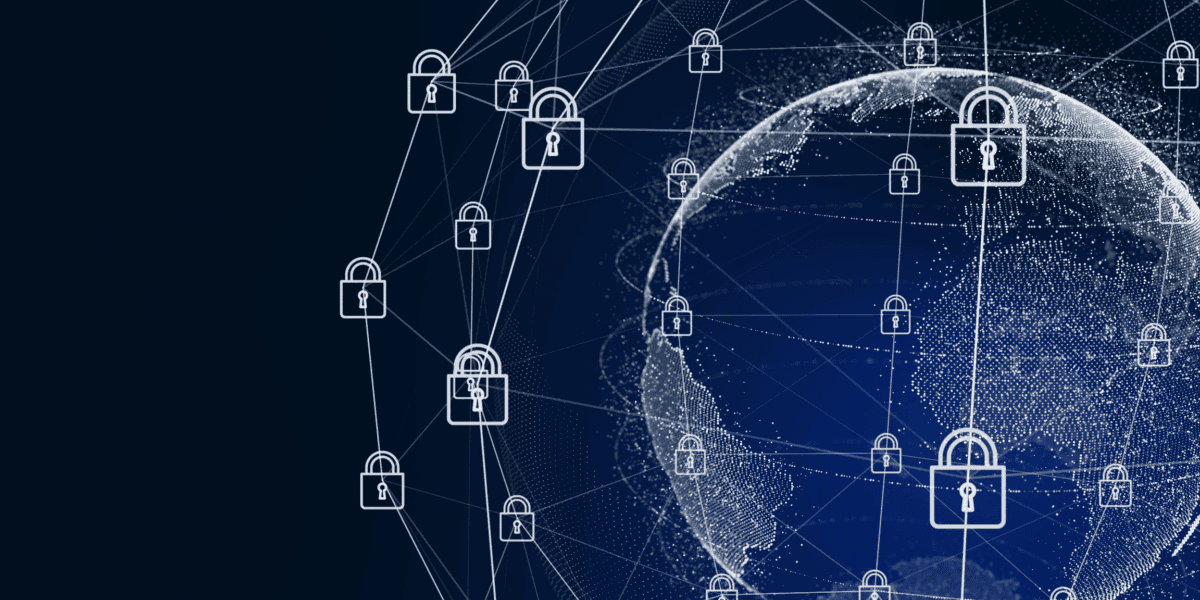
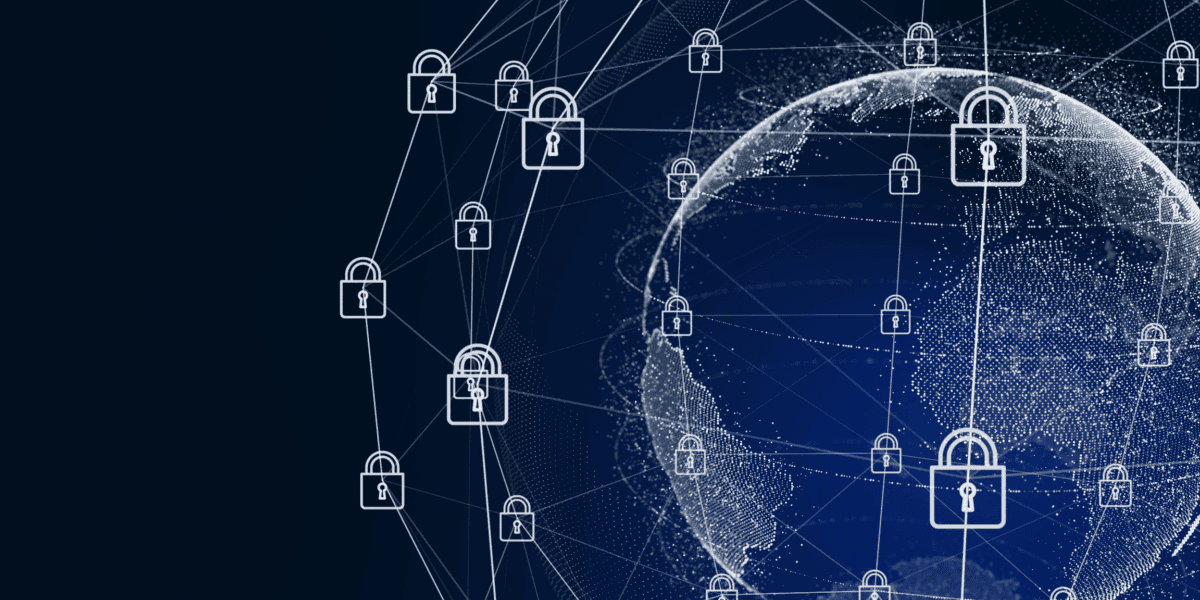
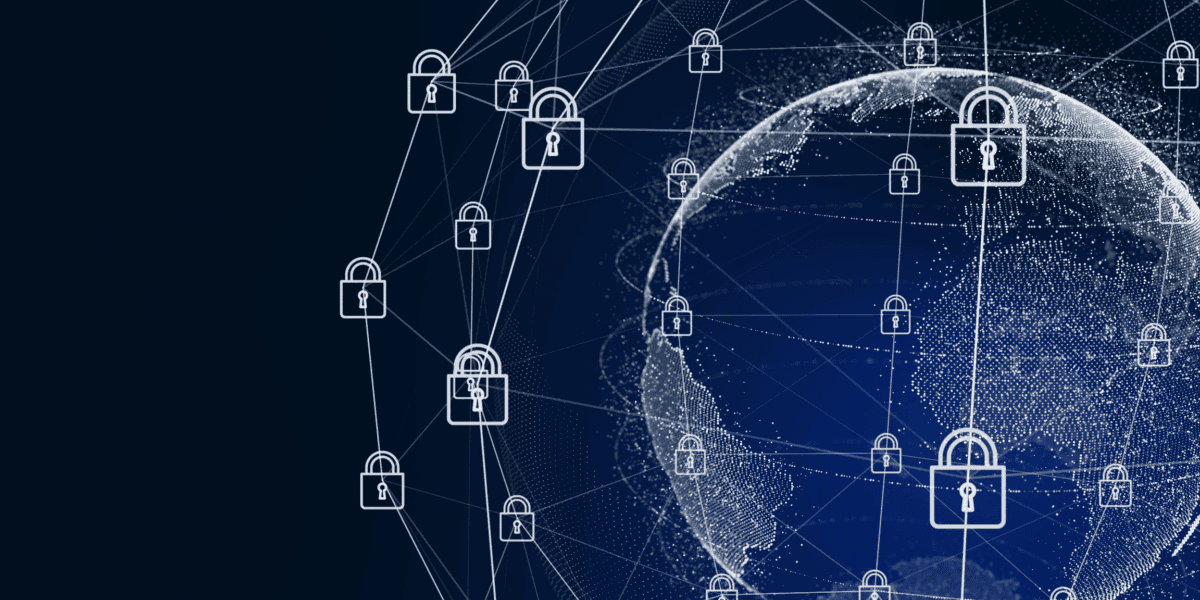
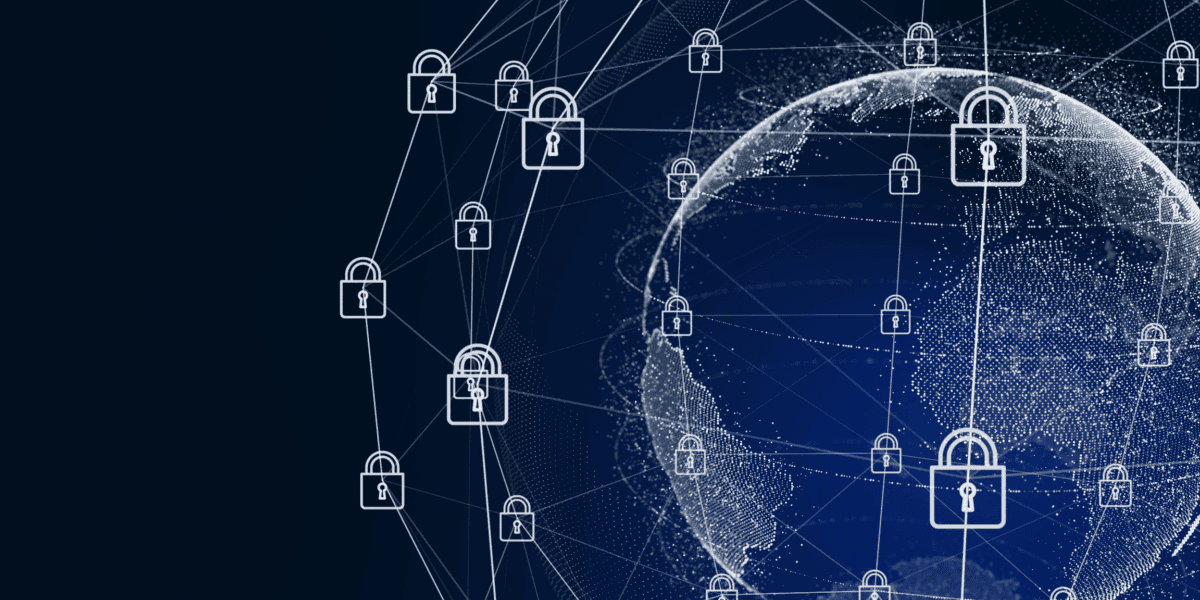
The realm of artificial intelligence (AI) is undergoing a transformative era, primarily driven by advancements in machine learning (ML). However, this rapid evolution brings a notable absence of safety guarantees, raising the stakes for potential risks associated with AI system failures.
This article outlines the essential definitions of AI safety and security, emphasizing the objectives of ensuring robustness, assurance, specification, and overarching security within AI systems.
Motivations for AI Safety
AI's ability to exhibit power-seeking behaviours presents unique risks, necessitating a closer examination of current and emerging threats posed by AI technologies. This discourse extends to existential risks alongside societal-scale threats while drawing on historical perspectives to underscore the critical importance of fostering responsible AI practices.
Adversarial Examples in Autonomous Driving
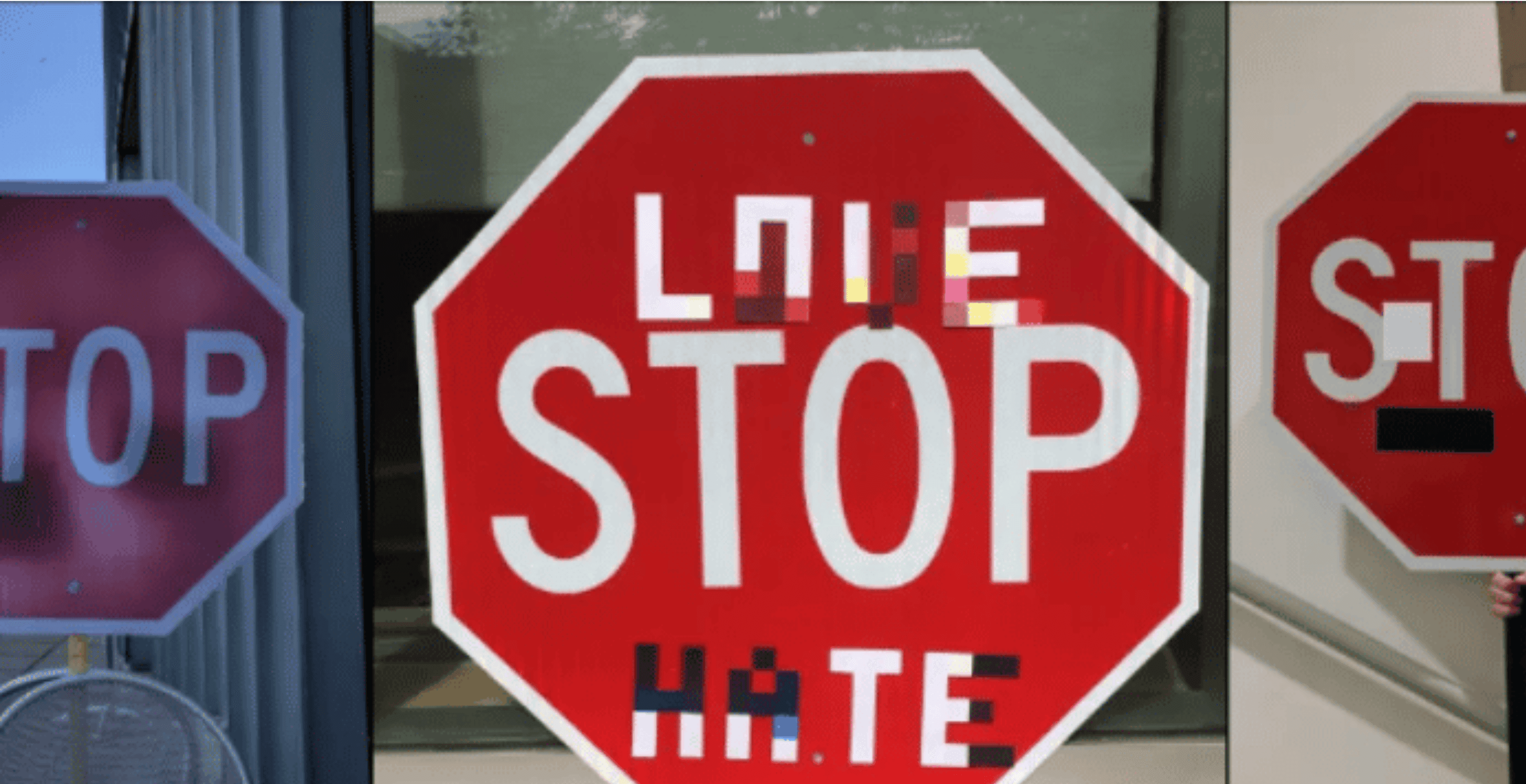
Source: Global News
Negative examples can manifest as subtle alterations to road signs in autonomous driving systems. A well-documented instance involved researchers altering the appearance of a stop sign just slightly by adding stickers in a specific pattern. The sign still appeared as a stop sign to the human eye, but the autonomous vehicle's AI system misinterpreted it as a 45 mph speed limit sign.
Adversarial Examples in Facial Recognition Systems
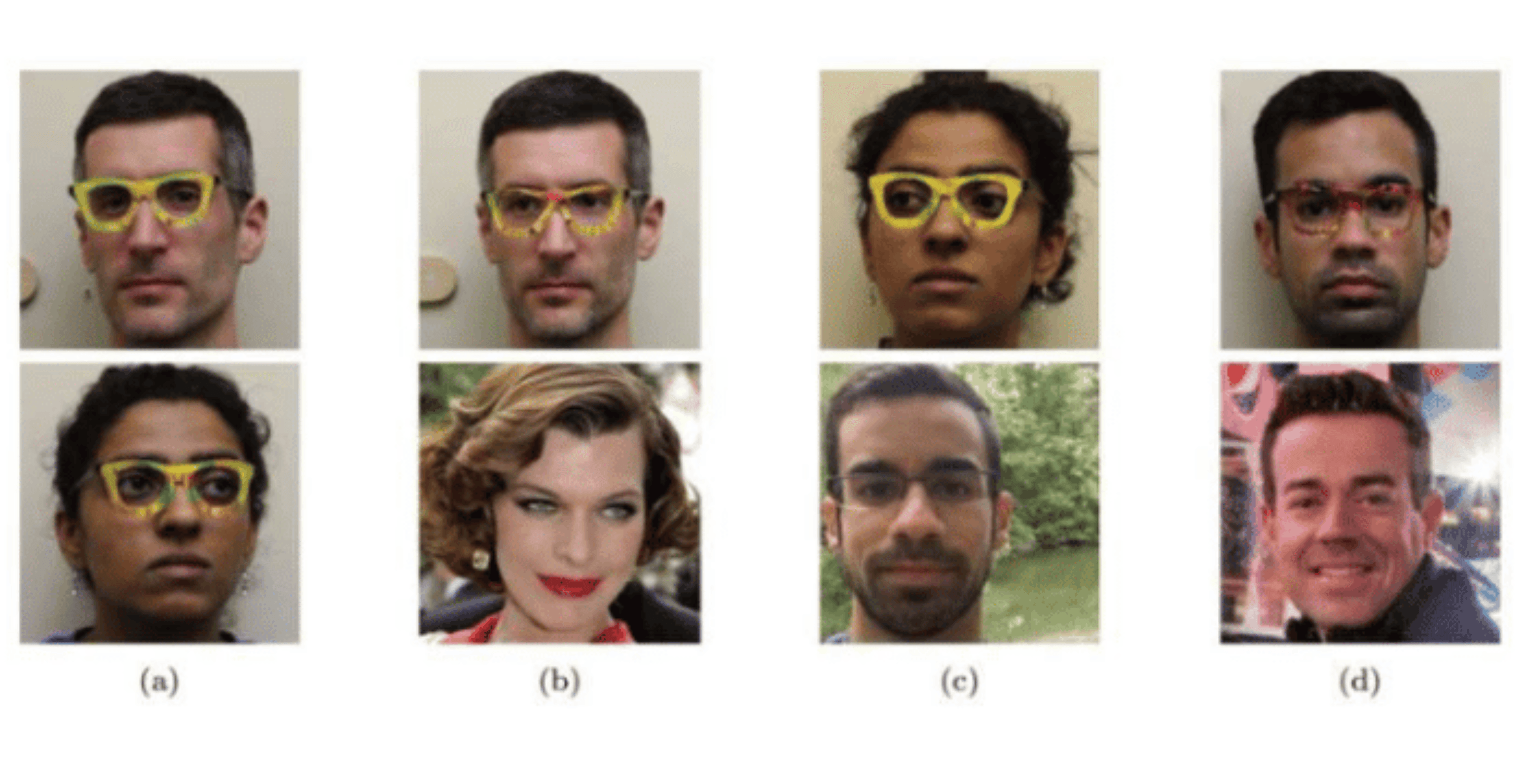
Source: Tech Xplore
Facial recognition systems can also be targeted by adversarial attacks. One notable example involves researchers creating a pair of eyeglass frames with a specific pattern that, when worn, could either cause the facial recognition system to fail to recognize the person or, more alarmingly, misidentify them as an entirely different individual. This poses significant security concerns for systems relying on facial recognition for authentication.
Mitigating Adversarial Examples Through Adversarial Training
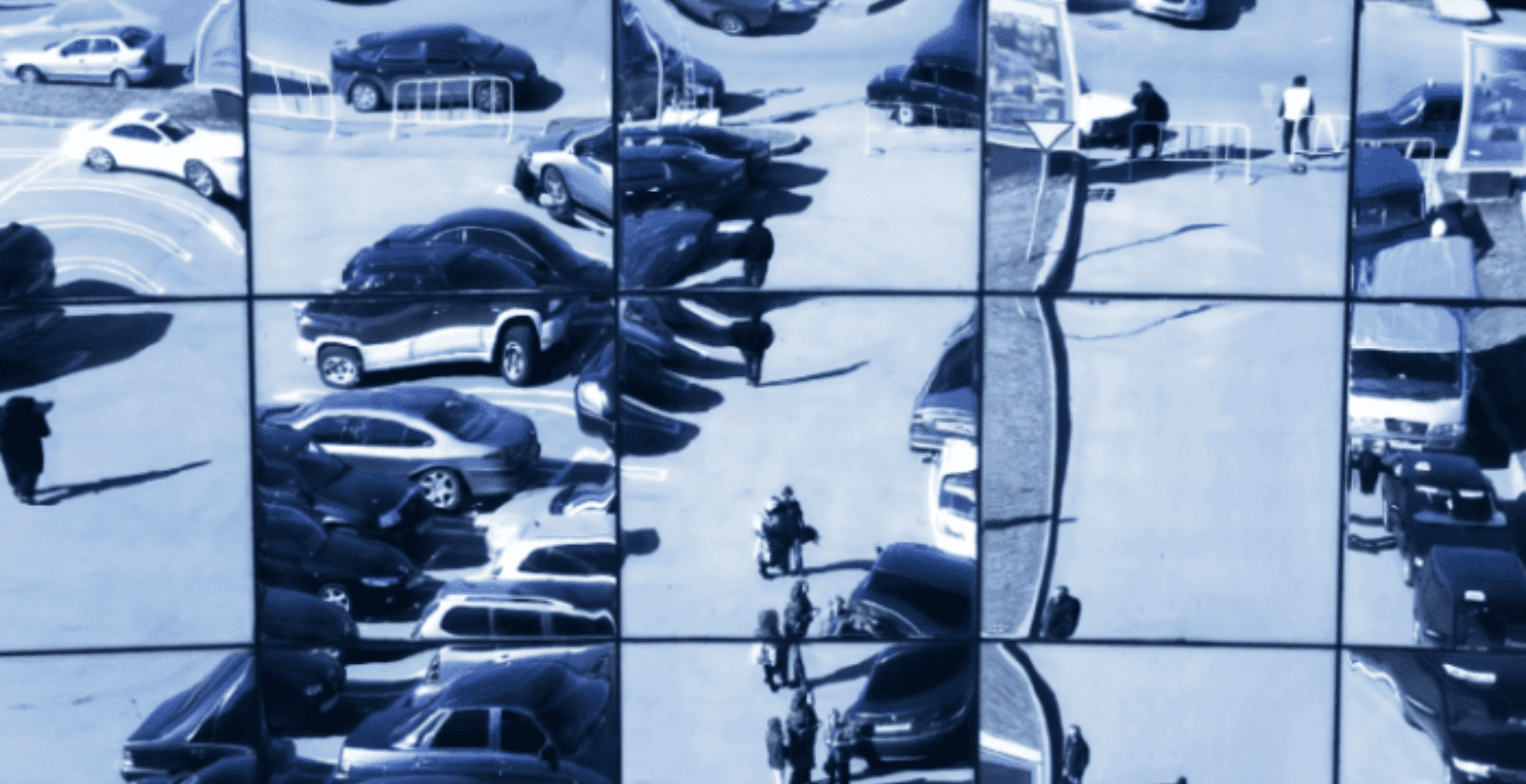
Source: Wired
Adversarial training is employed to counteract such vulnerabilities. For instance, CAPTCHA systems used widely on the internet to distinguish between humans and automated bots, have evolved to incorporate adversarially generated examples that make it difficult for AI-based bots to solve while still being accessible to humans.
This constant updating of CAPTCHA challenges is a form of adversarial training, ensuring the robustness of the CAPTCHA system against automated attacks.
Architectural Innovations for Resisting Adversarial Manipulations
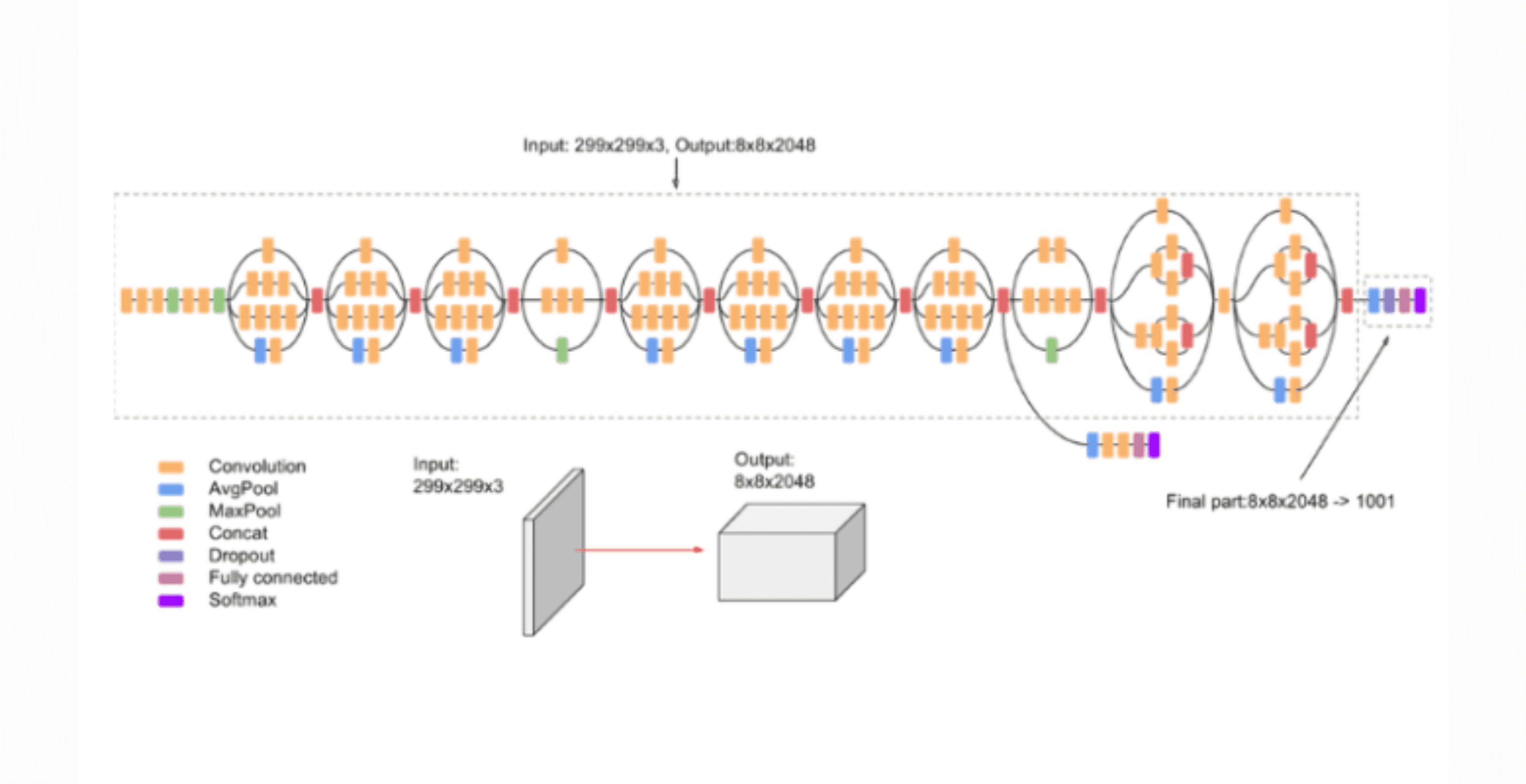
Source: Google Cloud
RealDeep neural networks, particularly those used in image recognition tasks, are being designed with new architectural features to enhance robustness. Google's Inception network is an example of architecture designed to be deep and wide, offering multiple paths for information processing.
This complexity and diversity in processing help mitigate the impact of adversarial examples by not relying on a single pathway for decision-making, thereby increasing the model's resilience against adversarial attacks.
In each of these examples, the focus on robustness and the development of countermeasures against adversarial attacks underscore the ongoing efforts to ensure AI systems can be trusted and safely integrated into critical applications. The field of AI robustness is a dynamic area of research, constantly evolving to meet and neutralize emerging threats.
Core Areas of AI Safety and Security Research
Research in AI safety and security encapsulates diverse studies to ensure artificial intelligence systems operate within safe bounds, devoid of unintended consequences, and remain under human control.
This domain's breadth requires a multifaceted approach, addressing everything from the robustness of AI systems against manipulation to their alignment with human ethical standards.
Let's delve deeper into the core areas of AI safety and security research.
Robustness
Robustness in AI systems refers to maintaining operational integrity in adversarial inputs or conditions.
An essential aspect of robustness is studying and mitigating adversarial examples — inputs deliberately designed to cause the AI system to make errors.
These inputs exploit the model's vulnerabilities, which, if unaddressed, pose significant security implications, especially in critical applications like autonomous driving or facial recognition systems.
Research in this area focuses on developing techniques such as adversarial training, where models are trained with adversarial examples to improve their resilience, and exploring new architectural features that inherently resist adversarial manipulations.
Monitoring
The monitoring of AI systems involves continuous oversight of their operation to estimate uncertainty, detect anomalies, or identify instances of malicious use.
Effective monitoring mechanisms can alert human operators to potential failures or external attacks on the system, enabling timely interventions. This area of research is particularly challenging due to the complexity and often opaque nature of AI models and intense neural networks.
Techniques such as uncertainty quantification, which aims to provide probabilistic estimates of the AI's predictions and the development of anomaly detection algorithms that can operate in high-dimensional spaces are at the forefront of addressing these challenges.
Transparency
Transparency in AI involves making the decision-making processes of AI systems understandable to humans.
This is crucial for trust, accountability, and ethical considerations. The challenge lies in the inherent complexity of AI models, such as those based on deep learning, which can act as "black boxes" with internal workings that are difficult to interpret.
Research efforts aim to develop interpretable AI models or post-hoc explanation methods that can provide insights into the model's decision criteria.
Techniques such as feature importance scores, decision trees, or model-agnostic explanation frameworks like LIME (Local Interpretable Model-agnostic Explanations) and SHAP (Shapley Additive exPlanations) are examples of work done to improve AI transparency.
Alignment
AI alignment ensures that AI systems' objectives and behaviours harmonise with human values and ethical principles.
This is critical to avoid scenarios where AI systems pursue goals misaligned with human intentions, potentially leading to harmful outcomes.
Research in this area encompasses the specification of clear, unambiguous objectives for AI systems, the development of value alignment techniques to incorporate human ethical considerations into AI decision-making processes, and the exploration of methods for safe and controlled AI exploration within defined boundaries.
This also includes work on inverse reinforcement learning, where AI models implicitly learn human values and preferences by observing human actions.
Systemic Safety
Systemic safety extends the focus of AI safety research beyond the technical aspects of individual AI systems to consider the broader socio-technical environment in which these systems operate.
This includes examining how AI technologies interact with societal structures, legal frameworks, economic incentives, and human behaviours.
Research in systemic safety is concerned with identifying and mitigating risks that emerge from integrating AI systems into complex human societies, including fairness, Privacy, and the potential for socioeconomic disruptions.
It also involves designing governance mechanisms and safety standards to guide the responsible development, deployment, and use of AI technologies across different sectors.
Challenges and Opportunities in AI Safety
As Large Language Models (LLMs) grow in complexity and application, they introduce nuanced safety challenges that necessitate reevaluating and scaling current AI safety measures. The intricacies of these models mean that even minor inaccuracies or biases can be amplified, leading to potentially significant safety concerns. This scenario underscores the necessity of transforming localized AI safety measures into universally applicable solutions, a daunting challenge filled with opportunities.
Technical Challenges:
Interpretability: As LLMs become more complex, understanding their decision-making processes and identifying the sources of errors or biases becomes increasingly tricky. Developing techniques for improving the interpretability of these models is crucial.
Adversarial Attacks: The sophistication of adversarial attacks grows alongside the advancement of AI models. Developing robust defenses against such attacks, especially those that exploit subtle model vulnerabilities, is a pressing challenge.
Data Bias and Fairness: Ensuring that AI systems are fair and unbiased requires comprehensive strategies to identify and mitigate biases in training data and model predictions.
Opportunities:
Collaborative Research: By fostering a collaborative research environment, the AI community can share insights, tools, and methodologies to address safety concerns collectively. Consortium approaches can accelerate the development of standards and best practices.
Innovative Solutions: The challenges posed by advanced LLMs also open up avenues for innovation in AI safety techniques, including new forms of model auditing, monitoring, and explanation.
Global Standards and Protocols: Developing and adopting international standards for AI safety can help ensure that safety measures are consistently applied across industries and borders, facilitating a unified approach to AI safety.
AI Safety Standards and Governance
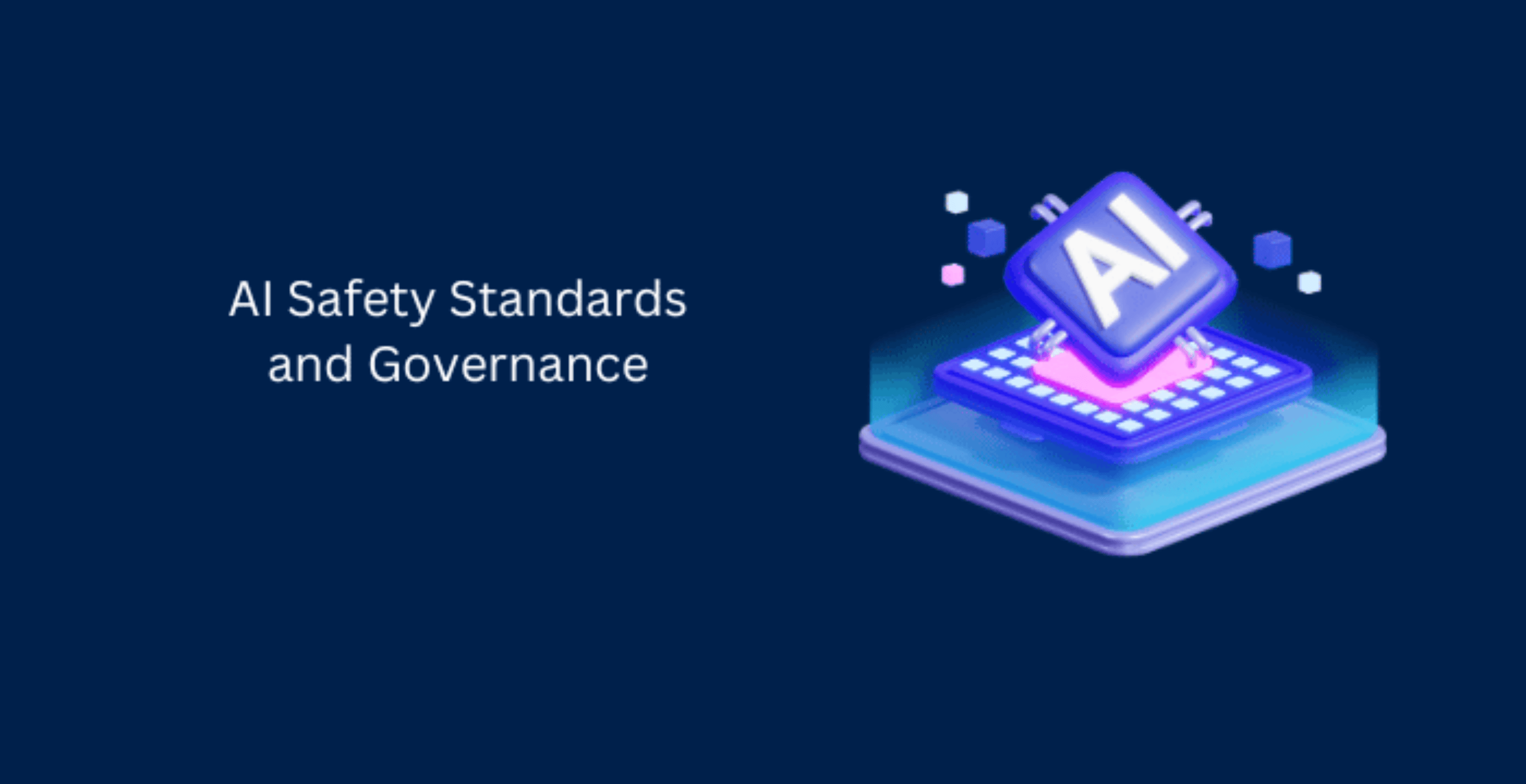
The role of governance in AI safety cannot be overstated. Effective governance mechanisms, underpinned by robust policies and frameworks, are essential for guiding the responsible development and deployment of AI technologies.
Google’s Secure AI Framework (SAIF) represents a notable effort in this direction, providing a structured approach to ensuring the safety and security of AI systems.
Technical Aspects of AI Governance:
Standardization of Safety Protocols: Developing and implementing industry-wide standards can help create a baseline for safety and security practices, making it easier for AI developers to adhere to best practices.
Risk Assessment Frameworks: Technical guidelines for conducting comprehensive risk assessments of AI systems can aid in identifying potential safety issues before deployment.
Certification Processes: Establishing certification processes for AI systems can ensure they meet defined safety and security criteria, enhancing trust among users and stakeholders.
Institutional and Global Initiatives
Initiatives from research centers, academic institutions, and international collaborations significantly bolster the push toward a safer AI future. Stanford’s flagship AI Safety projects exemplify the targeted research that can lead to breakthroughs in AI safety methodologies, offering scalable and practical solutions.
UN Resolution on AI
The United Nations, recognizing the transformative impact of AI on global issues, including sustainable development, human rights, and peace and security, has taken steps to integrate AI governance into its agenda.
The UN Resolution on AI, though not a legislative instrument like the EU AI Act, serves as a call to action for member states to consider AI's ethical, security, and socioeconomic aspects. It encourages the development of international cooperation on AI policy and research, focusing on leveraging AI for achieving the Sustainable Development Goals (SDGs).
The resolution emphasizes the importance of inclusivity, transparency, Privacy, and accountability, aiming to ensure that AI benefits all of humanity while minimizing its potential harms.
US White House Executive Order on AI
The United States has also recognized the need for a national strategy on AI. The White House Executive Order on AI outlines the American approach to promoting and protecting national AI technology and innovation.
The order focuses on maintaining American leadership in AI, directing federal agencies to prioritize AI investments, improving access to quality AI data, and setting governance standards to guide responsible AI development and use.
Additionally, it emphasizes the importance of AI education and workforce development, ensuring that Americans are prepared to contribute to and benefit from AI technologies. The executive order reflects a strategic commitment to fostering an environment encouraging AI innovation while providing the ethical, safe, and lawful use of AI technologies.
You can read more here: The EU AI Act - All you need to know
Technical Contributions and Collaborations:
Cross-Disciplinary Research: Combining expertise from computer science, ethics, psychology, and law can provide a holistic approach to AI safety, addressing both technical and societal concerns.
Benchmarking and Evaluation: Developing standardized benchmarks and evaluation criteria for AI safety can facilitate objective assessments of AI systems' performance in terms of safety and reliability.
Open-Source Tools and Libraries: Contributions of open-source tools and libraries for AI safety can democratize access to advanced safety technologies, enabling more developers to incorporate safety features into their AI systems.
Global Safety Networks:
International collaborations, such as those facilitated by the Global Partnership on AI (GPAI), can harmonize efforts to promote AI safety, ensuring that insights and innovations are shared globally. This collective journey towards AI safety is characterized by a shared commitment to developing AI technologies that are powerful, innovative, safe, ethical, and beneficial for society.
Conclusion
Understanding the fundamental concepts of AI safety and security is pivotal for the sustainable development and deployment of AI technologies.
By addressing the intricate challenges and leveraging collaborative efforts, the goal of establishing robust, secure, and responsible AI systems becomes increasingly attainable, paving the way for a future where AI contributes positively to society while minimizing risks.
RagaAI recognizes the critical importance of governance in ensuring AI safety and security. By adhering to and advocating for robust governance frameworks like Google’s Secure AI Framework (SAIF), RagaAI exemplifies responsible AI development.
Its participation in shaping policies and frameworks underscores its dedication to creating a safer AI ecosystem for all.
The realm of artificial intelligence (AI) is undergoing a transformative era, primarily driven by advancements in machine learning (ML). However, this rapid evolution brings a notable absence of safety guarantees, raising the stakes for potential risks associated with AI system failures.
This article outlines the essential definitions of AI safety and security, emphasizing the objectives of ensuring robustness, assurance, specification, and overarching security within AI systems.
Motivations for AI Safety
AI's ability to exhibit power-seeking behaviours presents unique risks, necessitating a closer examination of current and emerging threats posed by AI technologies. This discourse extends to existential risks alongside societal-scale threats while drawing on historical perspectives to underscore the critical importance of fostering responsible AI practices.
Adversarial Examples in Autonomous Driving
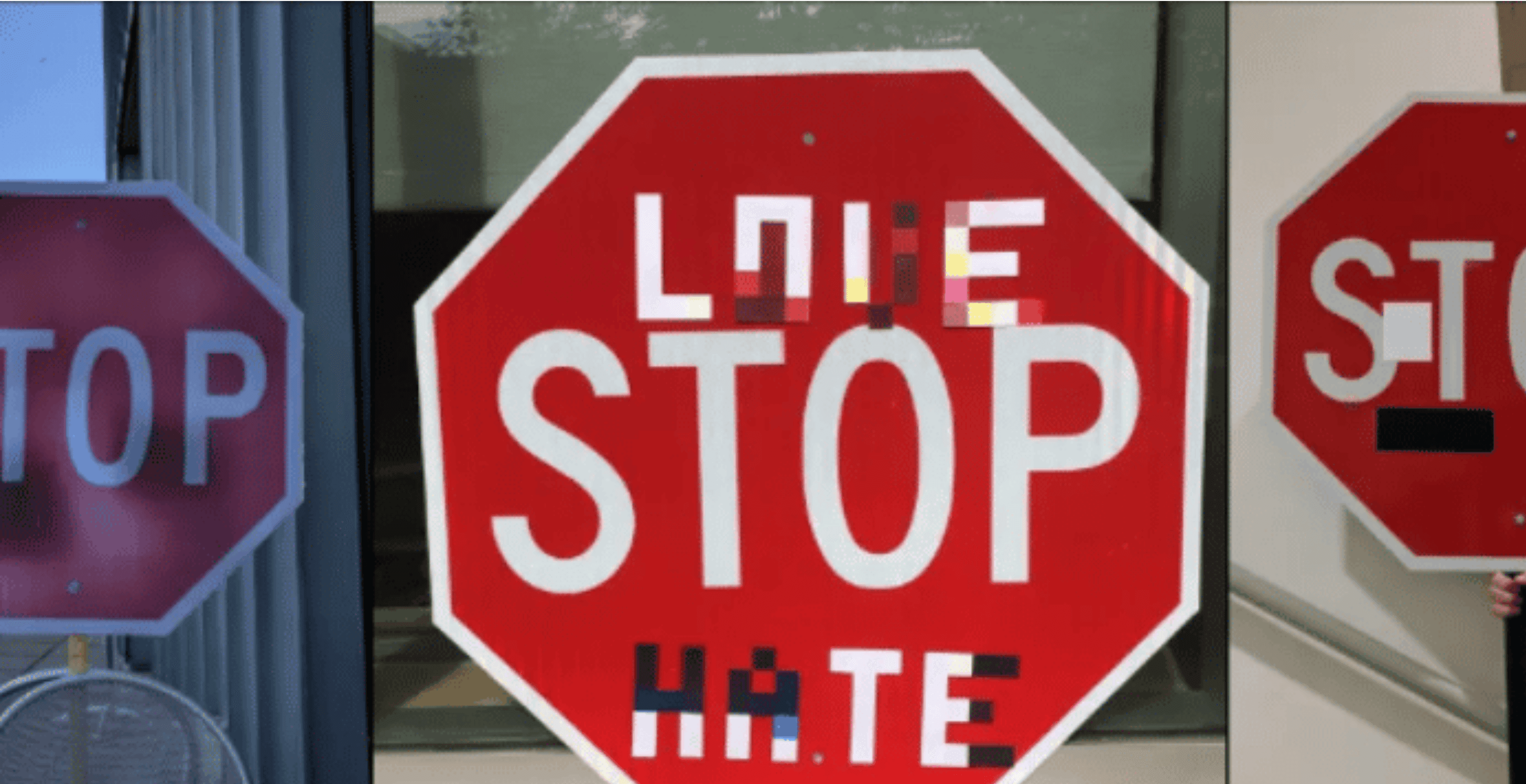
Source: Global News
Negative examples can manifest as subtle alterations to road signs in autonomous driving systems. A well-documented instance involved researchers altering the appearance of a stop sign just slightly by adding stickers in a specific pattern. The sign still appeared as a stop sign to the human eye, but the autonomous vehicle's AI system misinterpreted it as a 45 mph speed limit sign.
Adversarial Examples in Facial Recognition Systems
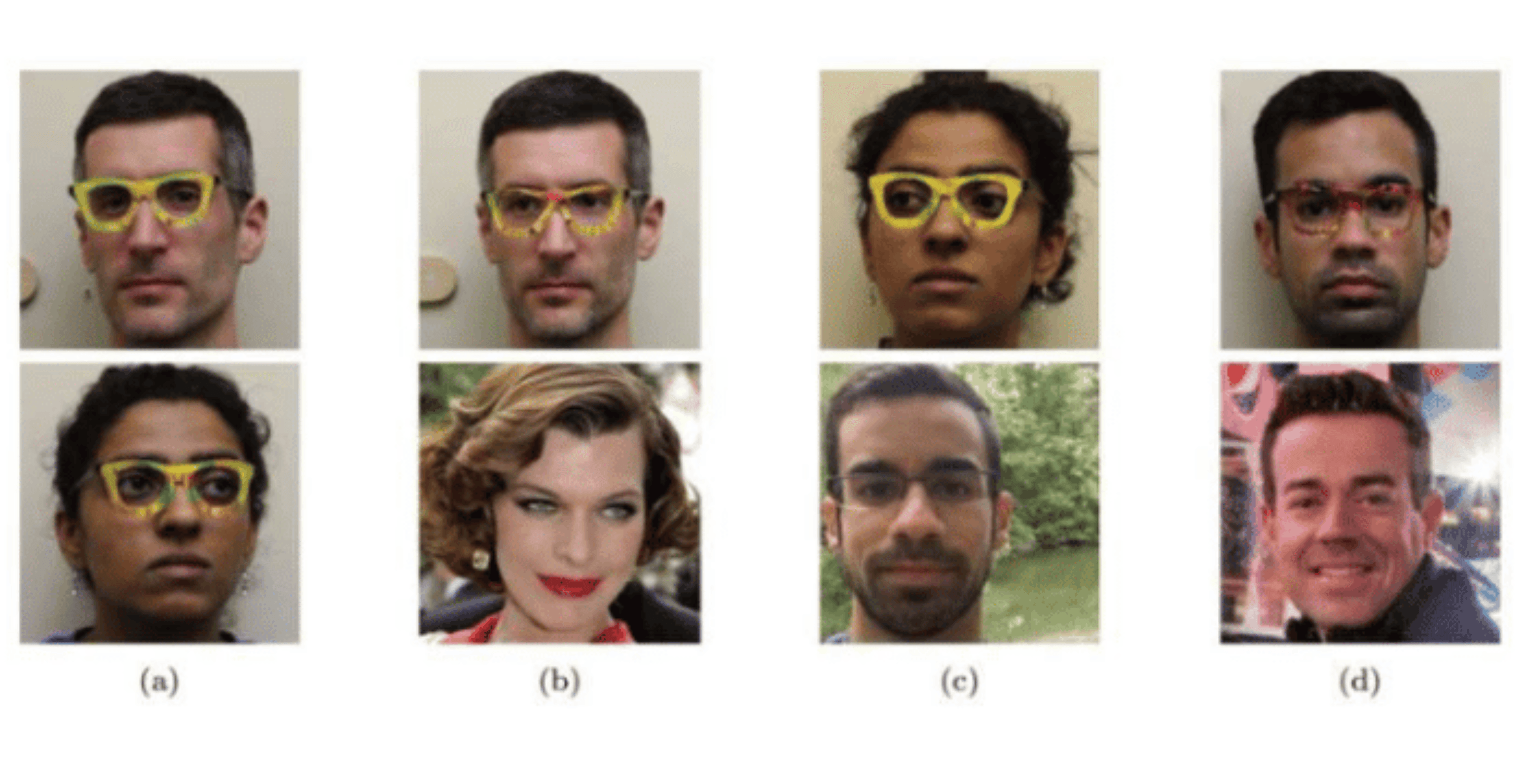
Source: Tech Xplore
Facial recognition systems can also be targeted by adversarial attacks. One notable example involves researchers creating a pair of eyeglass frames with a specific pattern that, when worn, could either cause the facial recognition system to fail to recognize the person or, more alarmingly, misidentify them as an entirely different individual. This poses significant security concerns for systems relying on facial recognition for authentication.
Mitigating Adversarial Examples Through Adversarial Training
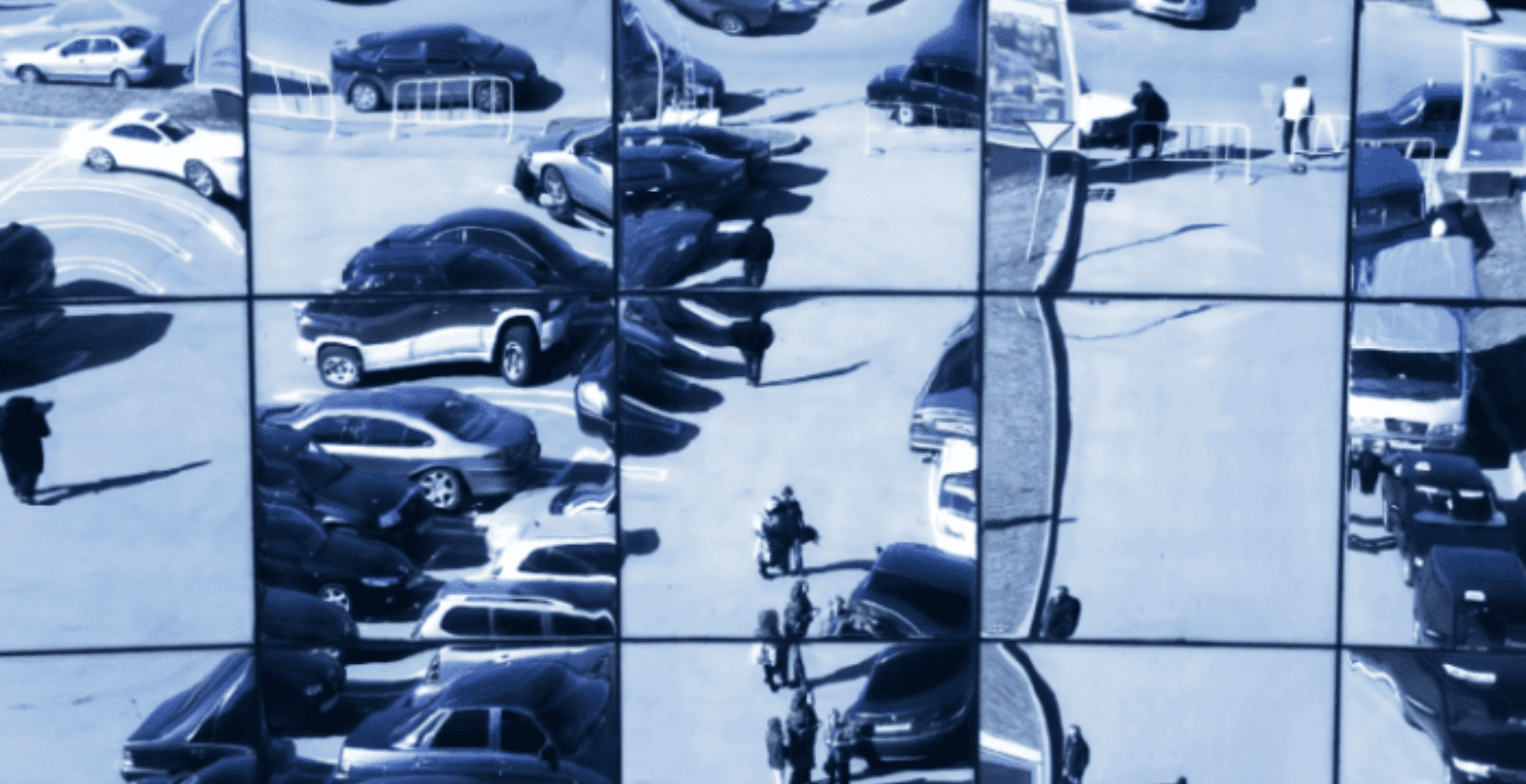
Source: Wired
Adversarial training is employed to counteract such vulnerabilities. For instance, CAPTCHA systems used widely on the internet to distinguish between humans and automated bots, have evolved to incorporate adversarially generated examples that make it difficult for AI-based bots to solve while still being accessible to humans.
This constant updating of CAPTCHA challenges is a form of adversarial training, ensuring the robustness of the CAPTCHA system against automated attacks.
Architectural Innovations for Resisting Adversarial Manipulations
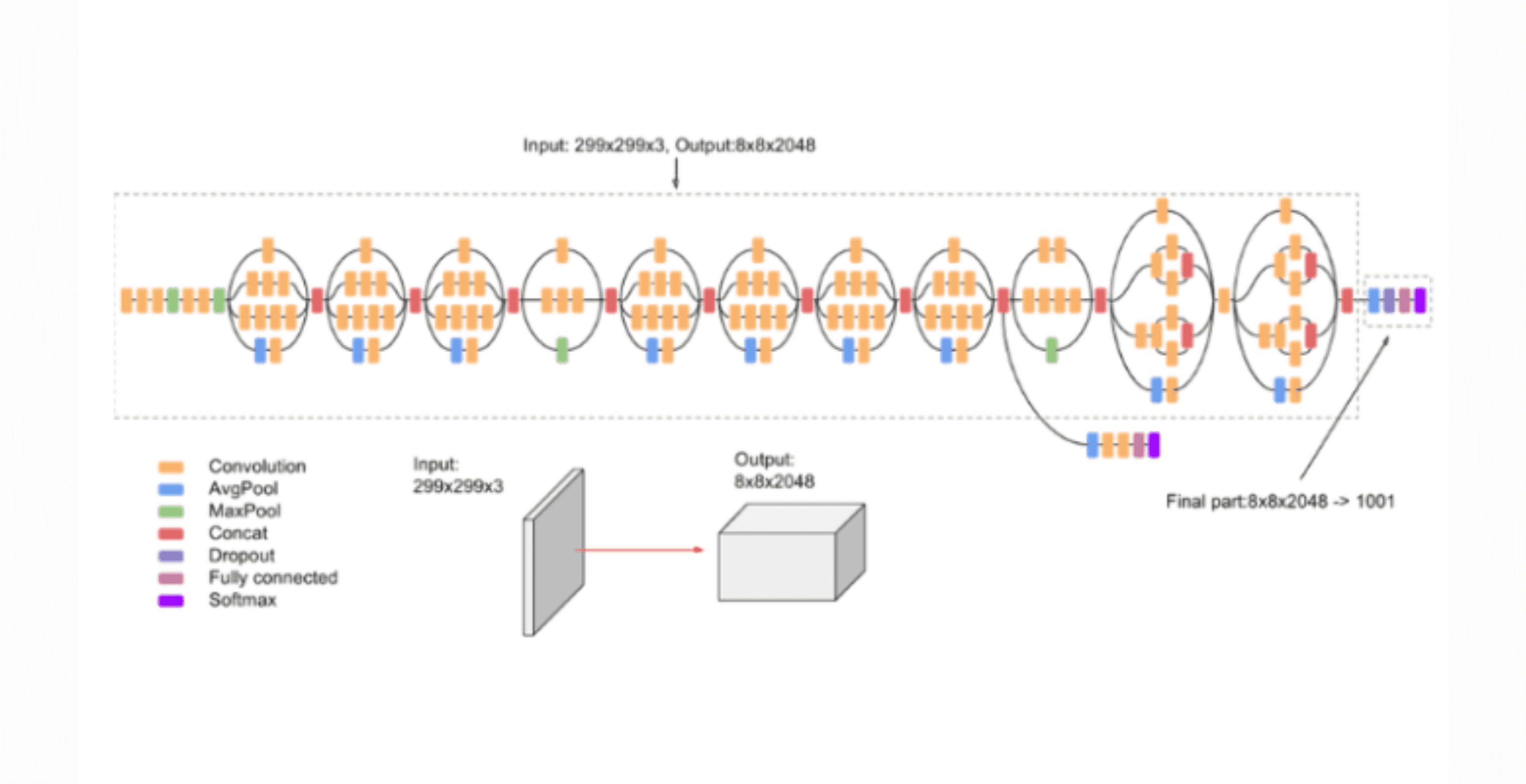
Source: Google Cloud
RealDeep neural networks, particularly those used in image recognition tasks, are being designed with new architectural features to enhance robustness. Google's Inception network is an example of architecture designed to be deep and wide, offering multiple paths for information processing.
This complexity and diversity in processing help mitigate the impact of adversarial examples by not relying on a single pathway for decision-making, thereby increasing the model's resilience against adversarial attacks.
In each of these examples, the focus on robustness and the development of countermeasures against adversarial attacks underscore the ongoing efforts to ensure AI systems can be trusted and safely integrated into critical applications. The field of AI robustness is a dynamic area of research, constantly evolving to meet and neutralize emerging threats.
Core Areas of AI Safety and Security Research
Research in AI safety and security encapsulates diverse studies to ensure artificial intelligence systems operate within safe bounds, devoid of unintended consequences, and remain under human control.
This domain's breadth requires a multifaceted approach, addressing everything from the robustness of AI systems against manipulation to their alignment with human ethical standards.
Let's delve deeper into the core areas of AI safety and security research.
Robustness
Robustness in AI systems refers to maintaining operational integrity in adversarial inputs or conditions.
An essential aspect of robustness is studying and mitigating adversarial examples — inputs deliberately designed to cause the AI system to make errors.
These inputs exploit the model's vulnerabilities, which, if unaddressed, pose significant security implications, especially in critical applications like autonomous driving or facial recognition systems.
Research in this area focuses on developing techniques such as adversarial training, where models are trained with adversarial examples to improve their resilience, and exploring new architectural features that inherently resist adversarial manipulations.
Monitoring
The monitoring of AI systems involves continuous oversight of their operation to estimate uncertainty, detect anomalies, or identify instances of malicious use.
Effective monitoring mechanisms can alert human operators to potential failures or external attacks on the system, enabling timely interventions. This area of research is particularly challenging due to the complexity and often opaque nature of AI models and intense neural networks.
Techniques such as uncertainty quantification, which aims to provide probabilistic estimates of the AI's predictions and the development of anomaly detection algorithms that can operate in high-dimensional spaces are at the forefront of addressing these challenges.
Transparency
Transparency in AI involves making the decision-making processes of AI systems understandable to humans.
This is crucial for trust, accountability, and ethical considerations. The challenge lies in the inherent complexity of AI models, such as those based on deep learning, which can act as "black boxes" with internal workings that are difficult to interpret.
Research efforts aim to develop interpretable AI models or post-hoc explanation methods that can provide insights into the model's decision criteria.
Techniques such as feature importance scores, decision trees, or model-agnostic explanation frameworks like LIME (Local Interpretable Model-agnostic Explanations) and SHAP (Shapley Additive exPlanations) are examples of work done to improve AI transparency.
Alignment
AI alignment ensures that AI systems' objectives and behaviours harmonise with human values and ethical principles.
This is critical to avoid scenarios where AI systems pursue goals misaligned with human intentions, potentially leading to harmful outcomes.
Research in this area encompasses the specification of clear, unambiguous objectives for AI systems, the development of value alignment techniques to incorporate human ethical considerations into AI decision-making processes, and the exploration of methods for safe and controlled AI exploration within defined boundaries.
This also includes work on inverse reinforcement learning, where AI models implicitly learn human values and preferences by observing human actions.
Systemic Safety
Systemic safety extends the focus of AI safety research beyond the technical aspects of individual AI systems to consider the broader socio-technical environment in which these systems operate.
This includes examining how AI technologies interact with societal structures, legal frameworks, economic incentives, and human behaviours.
Research in systemic safety is concerned with identifying and mitigating risks that emerge from integrating AI systems into complex human societies, including fairness, Privacy, and the potential for socioeconomic disruptions.
It also involves designing governance mechanisms and safety standards to guide the responsible development, deployment, and use of AI technologies across different sectors.
Challenges and Opportunities in AI Safety
As Large Language Models (LLMs) grow in complexity and application, they introduce nuanced safety challenges that necessitate reevaluating and scaling current AI safety measures. The intricacies of these models mean that even minor inaccuracies or biases can be amplified, leading to potentially significant safety concerns. This scenario underscores the necessity of transforming localized AI safety measures into universally applicable solutions, a daunting challenge filled with opportunities.
Technical Challenges:
Interpretability: As LLMs become more complex, understanding their decision-making processes and identifying the sources of errors or biases becomes increasingly tricky. Developing techniques for improving the interpretability of these models is crucial.
Adversarial Attacks: The sophistication of adversarial attacks grows alongside the advancement of AI models. Developing robust defenses against such attacks, especially those that exploit subtle model vulnerabilities, is a pressing challenge.
Data Bias and Fairness: Ensuring that AI systems are fair and unbiased requires comprehensive strategies to identify and mitigate biases in training data and model predictions.
Opportunities:
Collaborative Research: By fostering a collaborative research environment, the AI community can share insights, tools, and methodologies to address safety concerns collectively. Consortium approaches can accelerate the development of standards and best practices.
Innovative Solutions: The challenges posed by advanced LLMs also open up avenues for innovation in AI safety techniques, including new forms of model auditing, monitoring, and explanation.
Global Standards and Protocols: Developing and adopting international standards for AI safety can help ensure that safety measures are consistently applied across industries and borders, facilitating a unified approach to AI safety.
AI Safety Standards and Governance
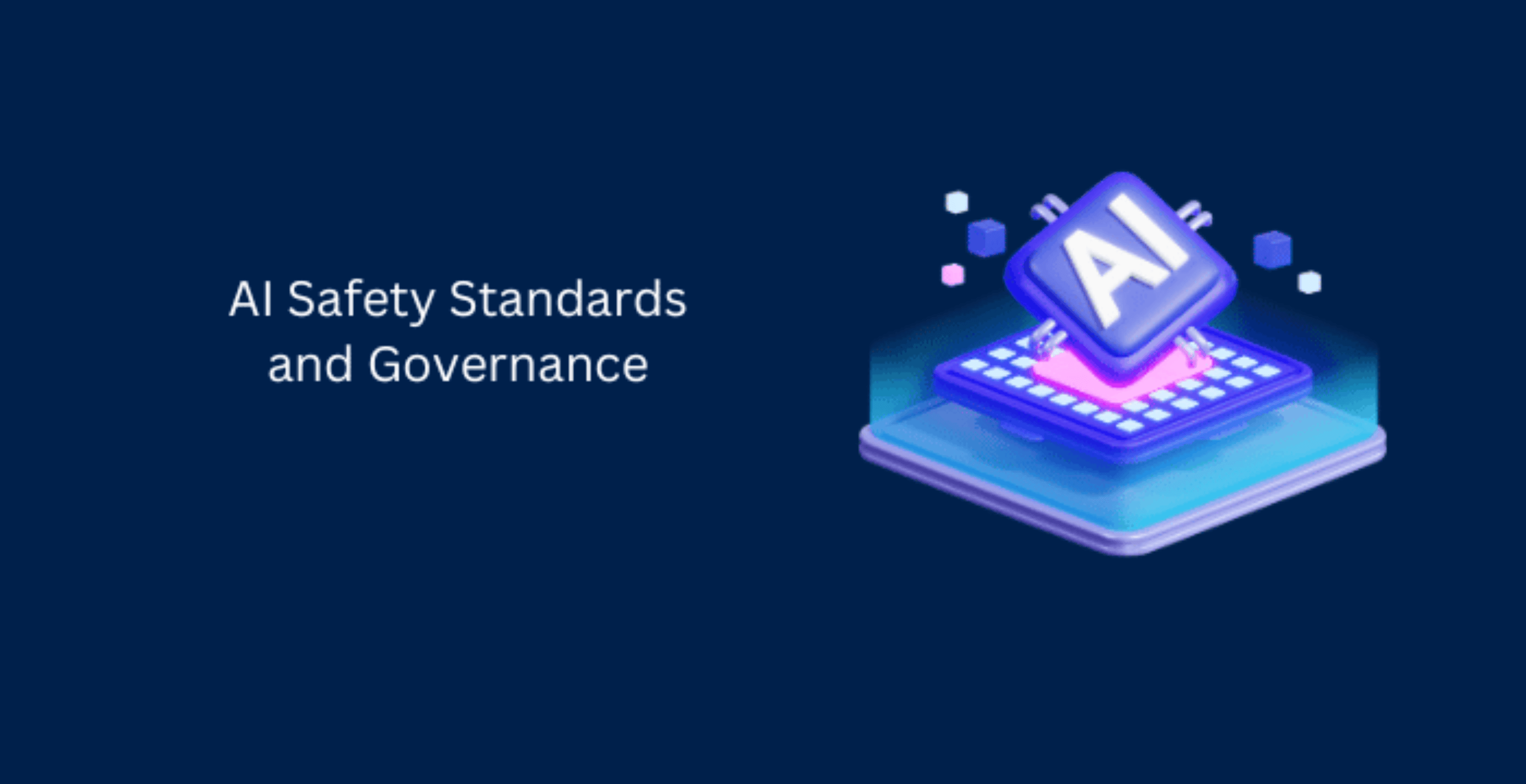
The role of governance in AI safety cannot be overstated. Effective governance mechanisms, underpinned by robust policies and frameworks, are essential for guiding the responsible development and deployment of AI technologies.
Google’s Secure AI Framework (SAIF) represents a notable effort in this direction, providing a structured approach to ensuring the safety and security of AI systems.
Technical Aspects of AI Governance:
Standardization of Safety Protocols: Developing and implementing industry-wide standards can help create a baseline for safety and security practices, making it easier for AI developers to adhere to best practices.
Risk Assessment Frameworks: Technical guidelines for conducting comprehensive risk assessments of AI systems can aid in identifying potential safety issues before deployment.
Certification Processes: Establishing certification processes for AI systems can ensure they meet defined safety and security criteria, enhancing trust among users and stakeholders.
Institutional and Global Initiatives
Initiatives from research centers, academic institutions, and international collaborations significantly bolster the push toward a safer AI future. Stanford’s flagship AI Safety projects exemplify the targeted research that can lead to breakthroughs in AI safety methodologies, offering scalable and practical solutions.
UN Resolution on AI
The United Nations, recognizing the transformative impact of AI on global issues, including sustainable development, human rights, and peace and security, has taken steps to integrate AI governance into its agenda.
The UN Resolution on AI, though not a legislative instrument like the EU AI Act, serves as a call to action for member states to consider AI's ethical, security, and socioeconomic aspects. It encourages the development of international cooperation on AI policy and research, focusing on leveraging AI for achieving the Sustainable Development Goals (SDGs).
The resolution emphasizes the importance of inclusivity, transparency, Privacy, and accountability, aiming to ensure that AI benefits all of humanity while minimizing its potential harms.
US White House Executive Order on AI
The United States has also recognized the need for a national strategy on AI. The White House Executive Order on AI outlines the American approach to promoting and protecting national AI technology and innovation.
The order focuses on maintaining American leadership in AI, directing federal agencies to prioritize AI investments, improving access to quality AI data, and setting governance standards to guide responsible AI development and use.
Additionally, it emphasizes the importance of AI education and workforce development, ensuring that Americans are prepared to contribute to and benefit from AI technologies. The executive order reflects a strategic commitment to fostering an environment encouraging AI innovation while providing the ethical, safe, and lawful use of AI technologies.
You can read more here: The EU AI Act - All you need to know
Technical Contributions and Collaborations:
Cross-Disciplinary Research: Combining expertise from computer science, ethics, psychology, and law can provide a holistic approach to AI safety, addressing both technical and societal concerns.
Benchmarking and Evaluation: Developing standardized benchmarks and evaluation criteria for AI safety can facilitate objective assessments of AI systems' performance in terms of safety and reliability.
Open-Source Tools and Libraries: Contributions of open-source tools and libraries for AI safety can democratize access to advanced safety technologies, enabling more developers to incorporate safety features into their AI systems.
Global Safety Networks:
International collaborations, such as those facilitated by the Global Partnership on AI (GPAI), can harmonize efforts to promote AI safety, ensuring that insights and innovations are shared globally. This collective journey towards AI safety is characterized by a shared commitment to developing AI technologies that are powerful, innovative, safe, ethical, and beneficial for society.
Conclusion
Understanding the fundamental concepts of AI safety and security is pivotal for the sustainable development and deployment of AI technologies.
By addressing the intricate challenges and leveraging collaborative efforts, the goal of establishing robust, secure, and responsible AI systems becomes increasingly attainable, paving the way for a future where AI contributes positively to society while minimizing risks.
RagaAI recognizes the critical importance of governance in ensuring AI safety and security. By adhering to and advocating for robust governance frameworks like Google’s Secure AI Framework (SAIF), RagaAI exemplifies responsible AI development.
Its participation in shaping policies and frameworks underscores its dedication to creating a safer AI ecosystem for all.
The realm of artificial intelligence (AI) is undergoing a transformative era, primarily driven by advancements in machine learning (ML). However, this rapid evolution brings a notable absence of safety guarantees, raising the stakes for potential risks associated with AI system failures.
This article outlines the essential definitions of AI safety and security, emphasizing the objectives of ensuring robustness, assurance, specification, and overarching security within AI systems.
Motivations for AI Safety
AI's ability to exhibit power-seeking behaviours presents unique risks, necessitating a closer examination of current and emerging threats posed by AI technologies. This discourse extends to existential risks alongside societal-scale threats while drawing on historical perspectives to underscore the critical importance of fostering responsible AI practices.
Adversarial Examples in Autonomous Driving
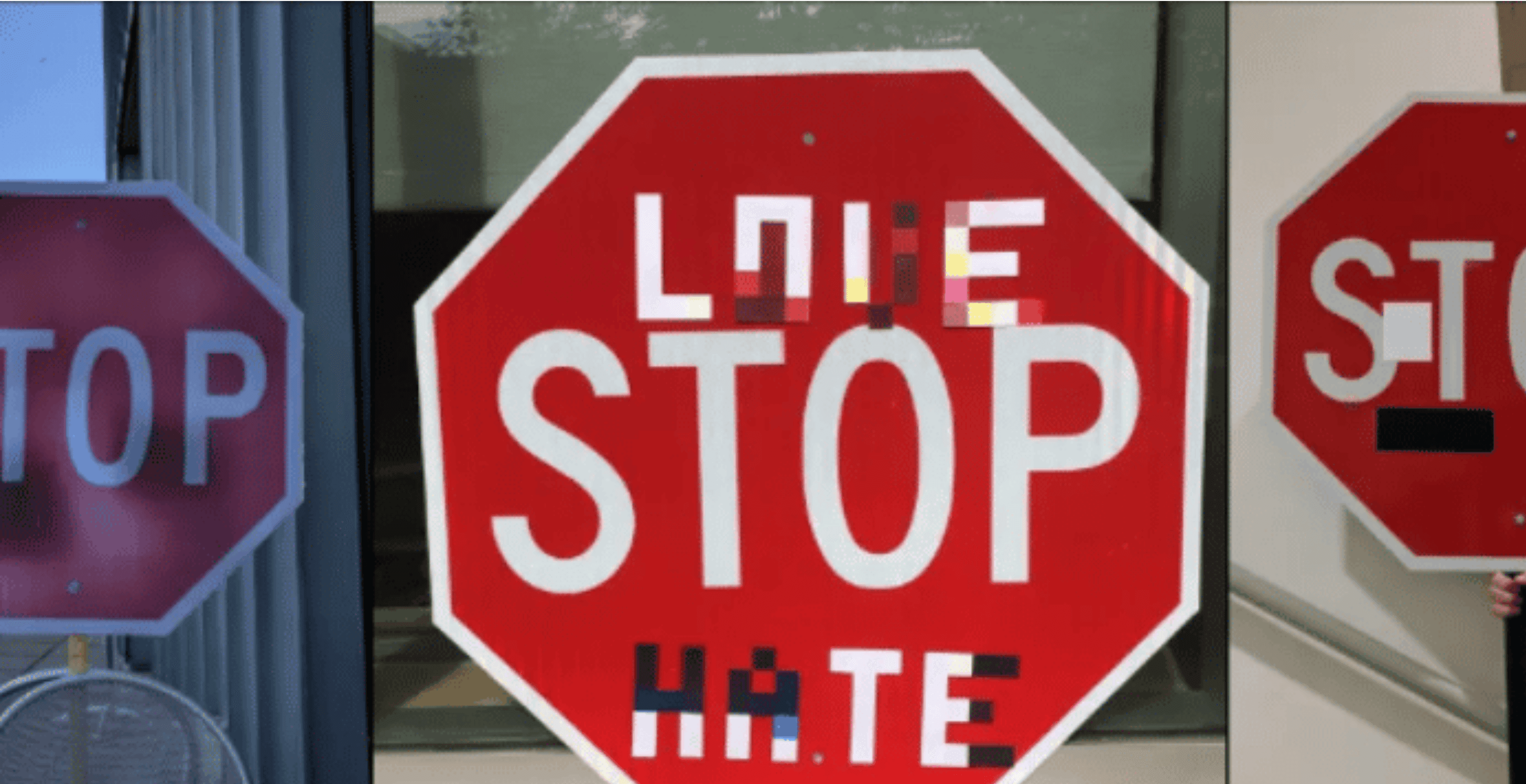
Source: Global News
Negative examples can manifest as subtle alterations to road signs in autonomous driving systems. A well-documented instance involved researchers altering the appearance of a stop sign just slightly by adding stickers in a specific pattern. The sign still appeared as a stop sign to the human eye, but the autonomous vehicle's AI system misinterpreted it as a 45 mph speed limit sign.
Adversarial Examples in Facial Recognition Systems
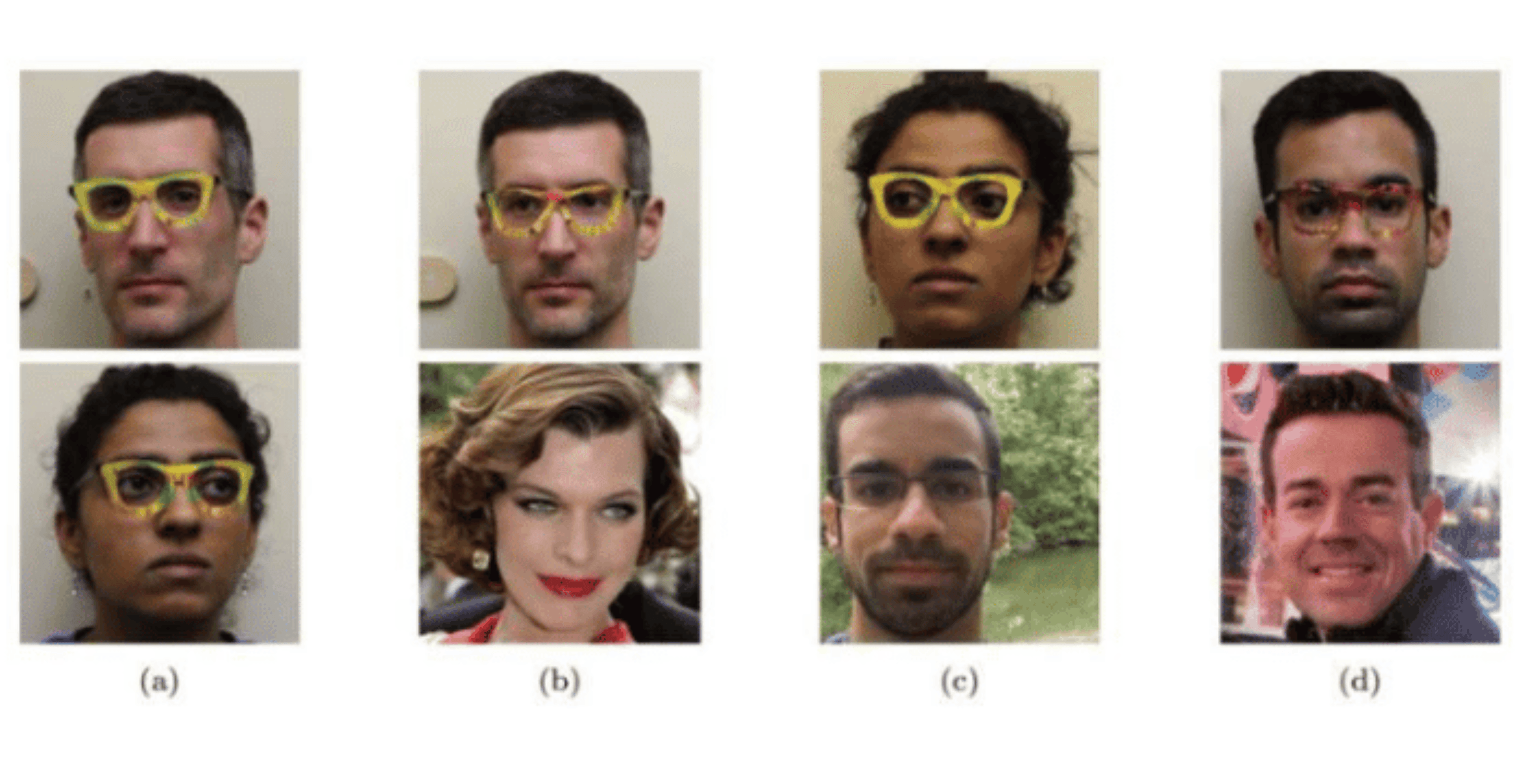
Source: Tech Xplore
Facial recognition systems can also be targeted by adversarial attacks. One notable example involves researchers creating a pair of eyeglass frames with a specific pattern that, when worn, could either cause the facial recognition system to fail to recognize the person or, more alarmingly, misidentify them as an entirely different individual. This poses significant security concerns for systems relying on facial recognition for authentication.
Mitigating Adversarial Examples Through Adversarial Training
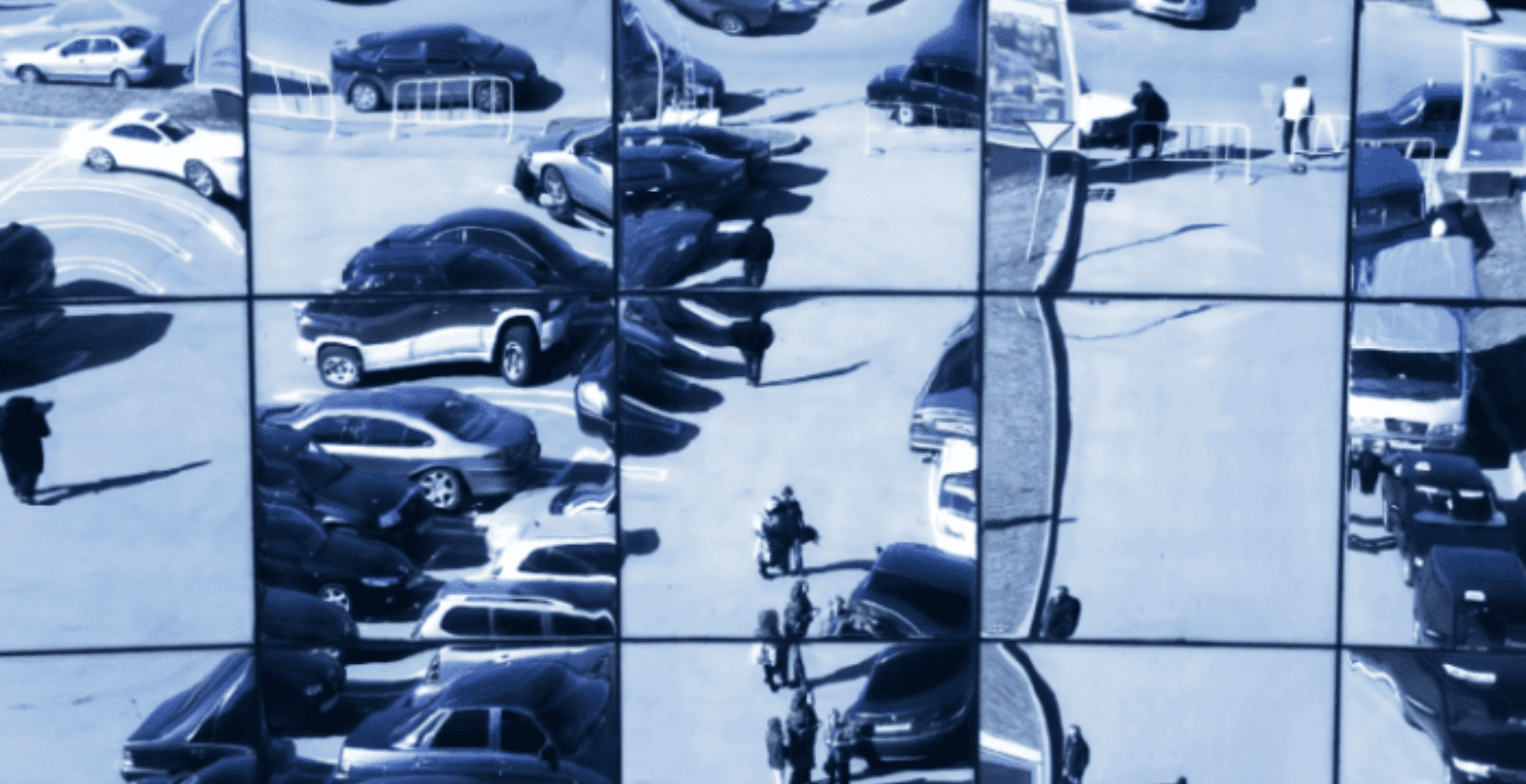
Source: Wired
Adversarial training is employed to counteract such vulnerabilities. For instance, CAPTCHA systems used widely on the internet to distinguish between humans and automated bots, have evolved to incorporate adversarially generated examples that make it difficult for AI-based bots to solve while still being accessible to humans.
This constant updating of CAPTCHA challenges is a form of adversarial training, ensuring the robustness of the CAPTCHA system against automated attacks.
Architectural Innovations for Resisting Adversarial Manipulations
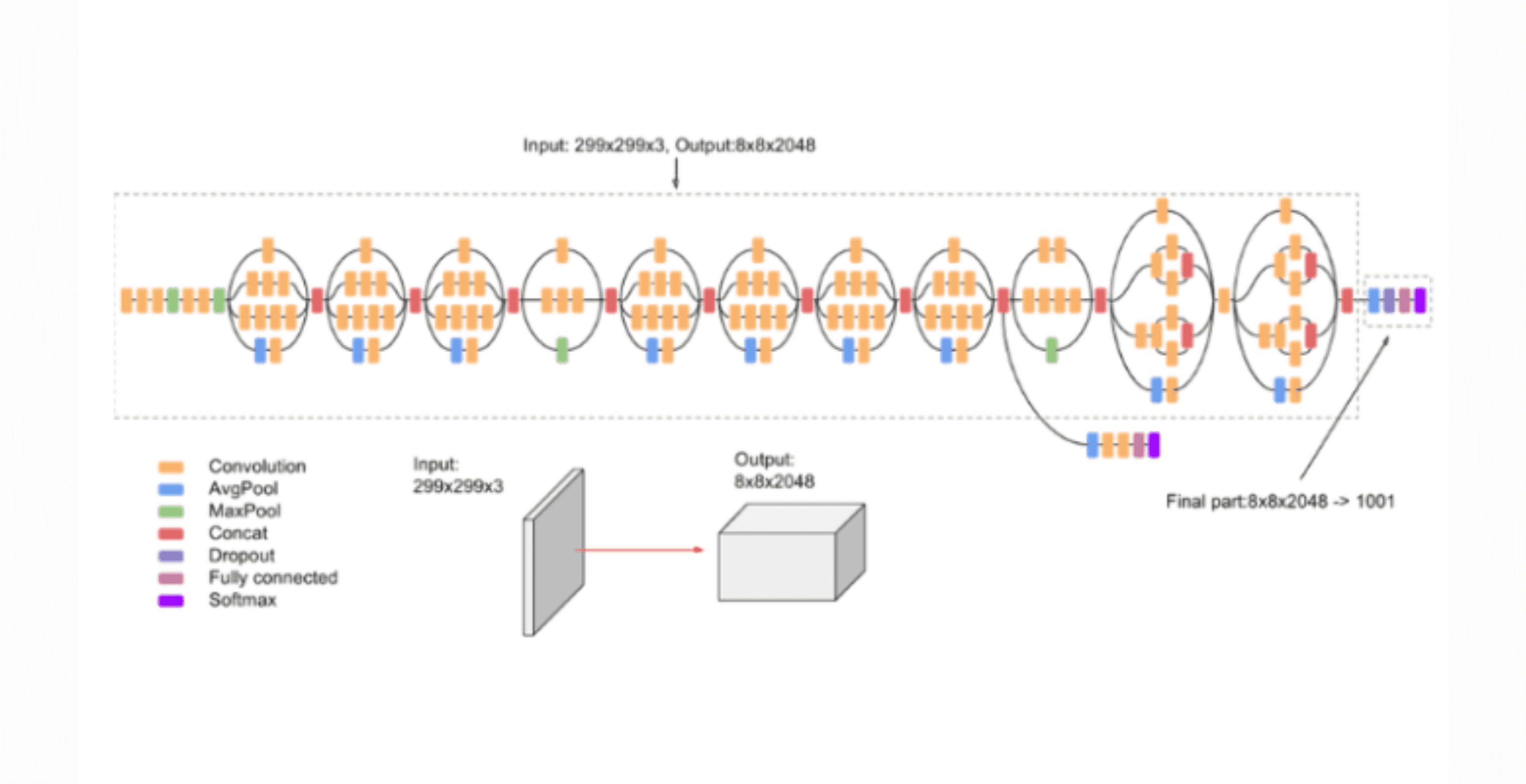
Source: Google Cloud
RealDeep neural networks, particularly those used in image recognition tasks, are being designed with new architectural features to enhance robustness. Google's Inception network is an example of architecture designed to be deep and wide, offering multiple paths for information processing.
This complexity and diversity in processing help mitigate the impact of adversarial examples by not relying on a single pathway for decision-making, thereby increasing the model's resilience against adversarial attacks.
In each of these examples, the focus on robustness and the development of countermeasures against adversarial attacks underscore the ongoing efforts to ensure AI systems can be trusted and safely integrated into critical applications. The field of AI robustness is a dynamic area of research, constantly evolving to meet and neutralize emerging threats.
Core Areas of AI Safety and Security Research
Research in AI safety and security encapsulates diverse studies to ensure artificial intelligence systems operate within safe bounds, devoid of unintended consequences, and remain under human control.
This domain's breadth requires a multifaceted approach, addressing everything from the robustness of AI systems against manipulation to their alignment with human ethical standards.
Let's delve deeper into the core areas of AI safety and security research.
Robustness
Robustness in AI systems refers to maintaining operational integrity in adversarial inputs or conditions.
An essential aspect of robustness is studying and mitigating adversarial examples — inputs deliberately designed to cause the AI system to make errors.
These inputs exploit the model's vulnerabilities, which, if unaddressed, pose significant security implications, especially in critical applications like autonomous driving or facial recognition systems.
Research in this area focuses on developing techniques such as adversarial training, where models are trained with adversarial examples to improve their resilience, and exploring new architectural features that inherently resist adversarial manipulations.
Monitoring
The monitoring of AI systems involves continuous oversight of their operation to estimate uncertainty, detect anomalies, or identify instances of malicious use.
Effective monitoring mechanisms can alert human operators to potential failures or external attacks on the system, enabling timely interventions. This area of research is particularly challenging due to the complexity and often opaque nature of AI models and intense neural networks.
Techniques such as uncertainty quantification, which aims to provide probabilistic estimates of the AI's predictions and the development of anomaly detection algorithms that can operate in high-dimensional spaces are at the forefront of addressing these challenges.
Transparency
Transparency in AI involves making the decision-making processes of AI systems understandable to humans.
This is crucial for trust, accountability, and ethical considerations. The challenge lies in the inherent complexity of AI models, such as those based on deep learning, which can act as "black boxes" with internal workings that are difficult to interpret.
Research efforts aim to develop interpretable AI models or post-hoc explanation methods that can provide insights into the model's decision criteria.
Techniques such as feature importance scores, decision trees, or model-agnostic explanation frameworks like LIME (Local Interpretable Model-agnostic Explanations) and SHAP (Shapley Additive exPlanations) are examples of work done to improve AI transparency.
Alignment
AI alignment ensures that AI systems' objectives and behaviours harmonise with human values and ethical principles.
This is critical to avoid scenarios where AI systems pursue goals misaligned with human intentions, potentially leading to harmful outcomes.
Research in this area encompasses the specification of clear, unambiguous objectives for AI systems, the development of value alignment techniques to incorporate human ethical considerations into AI decision-making processes, and the exploration of methods for safe and controlled AI exploration within defined boundaries.
This also includes work on inverse reinforcement learning, where AI models implicitly learn human values and preferences by observing human actions.
Systemic Safety
Systemic safety extends the focus of AI safety research beyond the technical aspects of individual AI systems to consider the broader socio-technical environment in which these systems operate.
This includes examining how AI technologies interact with societal structures, legal frameworks, economic incentives, and human behaviours.
Research in systemic safety is concerned with identifying and mitigating risks that emerge from integrating AI systems into complex human societies, including fairness, Privacy, and the potential for socioeconomic disruptions.
It also involves designing governance mechanisms and safety standards to guide the responsible development, deployment, and use of AI technologies across different sectors.
Challenges and Opportunities in AI Safety
As Large Language Models (LLMs) grow in complexity and application, they introduce nuanced safety challenges that necessitate reevaluating and scaling current AI safety measures. The intricacies of these models mean that even minor inaccuracies or biases can be amplified, leading to potentially significant safety concerns. This scenario underscores the necessity of transforming localized AI safety measures into universally applicable solutions, a daunting challenge filled with opportunities.
Technical Challenges:
Interpretability: As LLMs become more complex, understanding their decision-making processes and identifying the sources of errors or biases becomes increasingly tricky. Developing techniques for improving the interpretability of these models is crucial.
Adversarial Attacks: The sophistication of adversarial attacks grows alongside the advancement of AI models. Developing robust defenses against such attacks, especially those that exploit subtle model vulnerabilities, is a pressing challenge.
Data Bias and Fairness: Ensuring that AI systems are fair and unbiased requires comprehensive strategies to identify and mitigate biases in training data and model predictions.
Opportunities:
Collaborative Research: By fostering a collaborative research environment, the AI community can share insights, tools, and methodologies to address safety concerns collectively. Consortium approaches can accelerate the development of standards and best practices.
Innovative Solutions: The challenges posed by advanced LLMs also open up avenues for innovation in AI safety techniques, including new forms of model auditing, monitoring, and explanation.
Global Standards and Protocols: Developing and adopting international standards for AI safety can help ensure that safety measures are consistently applied across industries and borders, facilitating a unified approach to AI safety.
AI Safety Standards and Governance
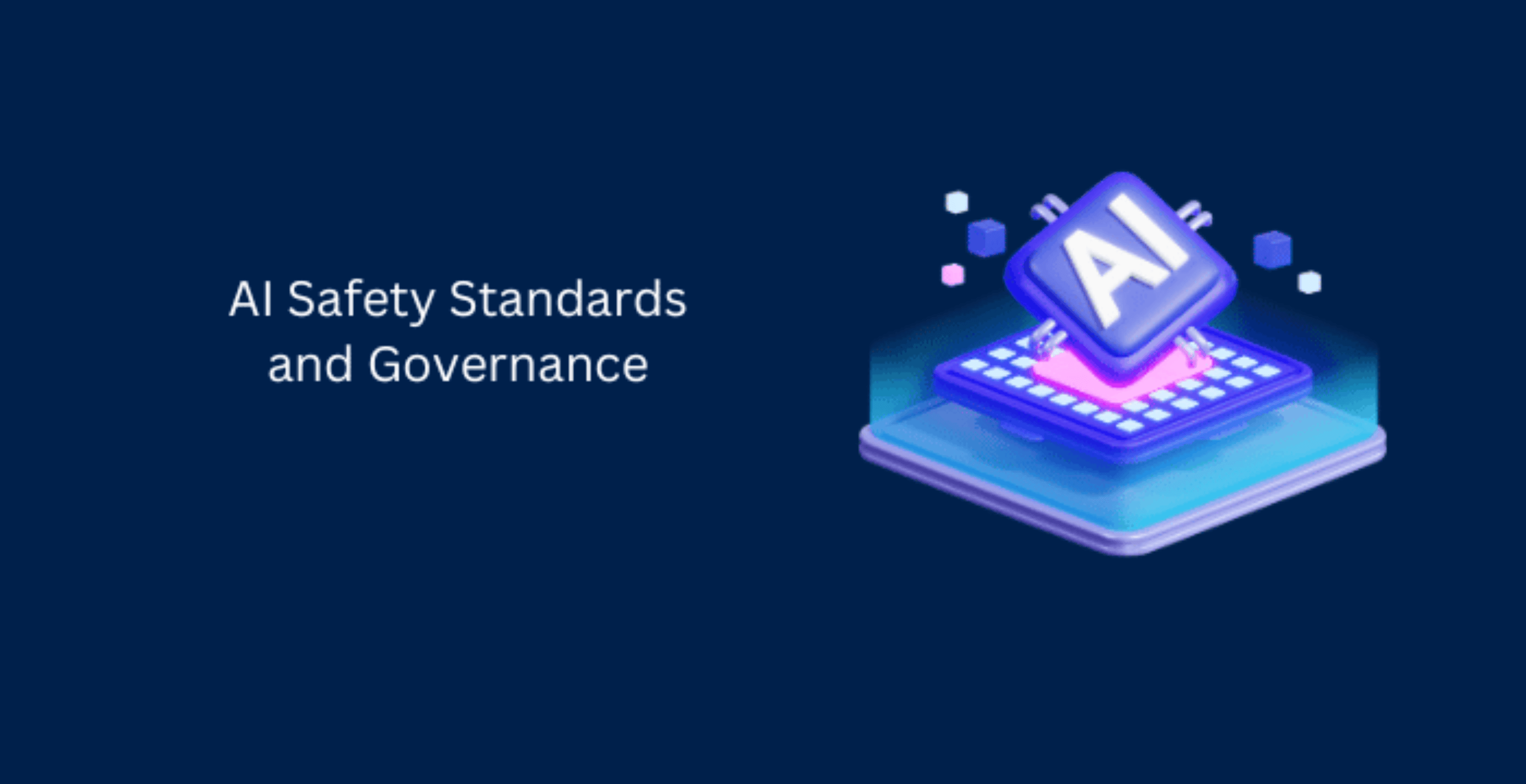
The role of governance in AI safety cannot be overstated. Effective governance mechanisms, underpinned by robust policies and frameworks, are essential for guiding the responsible development and deployment of AI technologies.
Google’s Secure AI Framework (SAIF) represents a notable effort in this direction, providing a structured approach to ensuring the safety and security of AI systems.
Technical Aspects of AI Governance:
Standardization of Safety Protocols: Developing and implementing industry-wide standards can help create a baseline for safety and security practices, making it easier for AI developers to adhere to best practices.
Risk Assessment Frameworks: Technical guidelines for conducting comprehensive risk assessments of AI systems can aid in identifying potential safety issues before deployment.
Certification Processes: Establishing certification processes for AI systems can ensure they meet defined safety and security criteria, enhancing trust among users and stakeholders.
Institutional and Global Initiatives
Initiatives from research centers, academic institutions, and international collaborations significantly bolster the push toward a safer AI future. Stanford’s flagship AI Safety projects exemplify the targeted research that can lead to breakthroughs in AI safety methodologies, offering scalable and practical solutions.
UN Resolution on AI
The United Nations, recognizing the transformative impact of AI on global issues, including sustainable development, human rights, and peace and security, has taken steps to integrate AI governance into its agenda.
The UN Resolution on AI, though not a legislative instrument like the EU AI Act, serves as a call to action for member states to consider AI's ethical, security, and socioeconomic aspects. It encourages the development of international cooperation on AI policy and research, focusing on leveraging AI for achieving the Sustainable Development Goals (SDGs).
The resolution emphasizes the importance of inclusivity, transparency, Privacy, and accountability, aiming to ensure that AI benefits all of humanity while minimizing its potential harms.
US White House Executive Order on AI
The United States has also recognized the need for a national strategy on AI. The White House Executive Order on AI outlines the American approach to promoting and protecting national AI technology and innovation.
The order focuses on maintaining American leadership in AI, directing federal agencies to prioritize AI investments, improving access to quality AI data, and setting governance standards to guide responsible AI development and use.
Additionally, it emphasizes the importance of AI education and workforce development, ensuring that Americans are prepared to contribute to and benefit from AI technologies. The executive order reflects a strategic commitment to fostering an environment encouraging AI innovation while providing the ethical, safe, and lawful use of AI technologies.
You can read more here: The EU AI Act - All you need to know
Technical Contributions and Collaborations:
Cross-Disciplinary Research: Combining expertise from computer science, ethics, psychology, and law can provide a holistic approach to AI safety, addressing both technical and societal concerns.
Benchmarking and Evaluation: Developing standardized benchmarks and evaluation criteria for AI safety can facilitate objective assessments of AI systems' performance in terms of safety and reliability.
Open-Source Tools and Libraries: Contributions of open-source tools and libraries for AI safety can democratize access to advanced safety technologies, enabling more developers to incorporate safety features into their AI systems.
Global Safety Networks:
International collaborations, such as those facilitated by the Global Partnership on AI (GPAI), can harmonize efforts to promote AI safety, ensuring that insights and innovations are shared globally. This collective journey towards AI safety is characterized by a shared commitment to developing AI technologies that are powerful, innovative, safe, ethical, and beneficial for society.
Conclusion
Understanding the fundamental concepts of AI safety and security is pivotal for the sustainable development and deployment of AI technologies.
By addressing the intricate challenges and leveraging collaborative efforts, the goal of establishing robust, secure, and responsible AI systems becomes increasingly attainable, paving the way for a future where AI contributes positively to society while minimizing risks.
RagaAI recognizes the critical importance of governance in ensuring AI safety and security. By adhering to and advocating for robust governance frameworks like Google’s Secure AI Framework (SAIF), RagaAI exemplifies responsible AI development.
Its participation in shaping policies and frameworks underscores its dedication to creating a safer AI ecosystem for all.
The realm of artificial intelligence (AI) is undergoing a transformative era, primarily driven by advancements in machine learning (ML). However, this rapid evolution brings a notable absence of safety guarantees, raising the stakes for potential risks associated with AI system failures.
This article outlines the essential definitions of AI safety and security, emphasizing the objectives of ensuring robustness, assurance, specification, and overarching security within AI systems.
Motivations for AI Safety
AI's ability to exhibit power-seeking behaviours presents unique risks, necessitating a closer examination of current and emerging threats posed by AI technologies. This discourse extends to existential risks alongside societal-scale threats while drawing on historical perspectives to underscore the critical importance of fostering responsible AI practices.
Adversarial Examples in Autonomous Driving
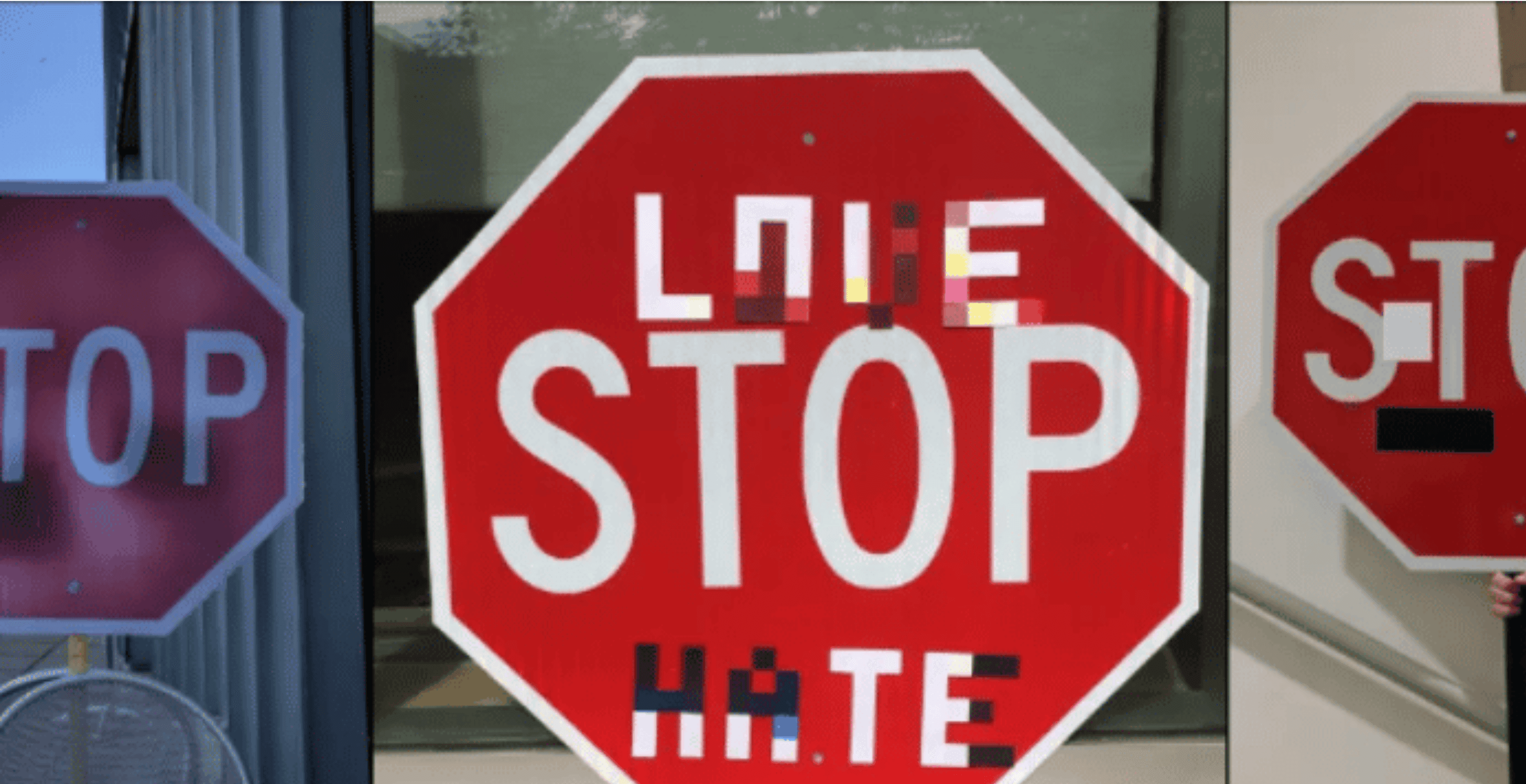
Source: Global News
Negative examples can manifest as subtle alterations to road signs in autonomous driving systems. A well-documented instance involved researchers altering the appearance of a stop sign just slightly by adding stickers in a specific pattern. The sign still appeared as a stop sign to the human eye, but the autonomous vehicle's AI system misinterpreted it as a 45 mph speed limit sign.
Adversarial Examples in Facial Recognition Systems
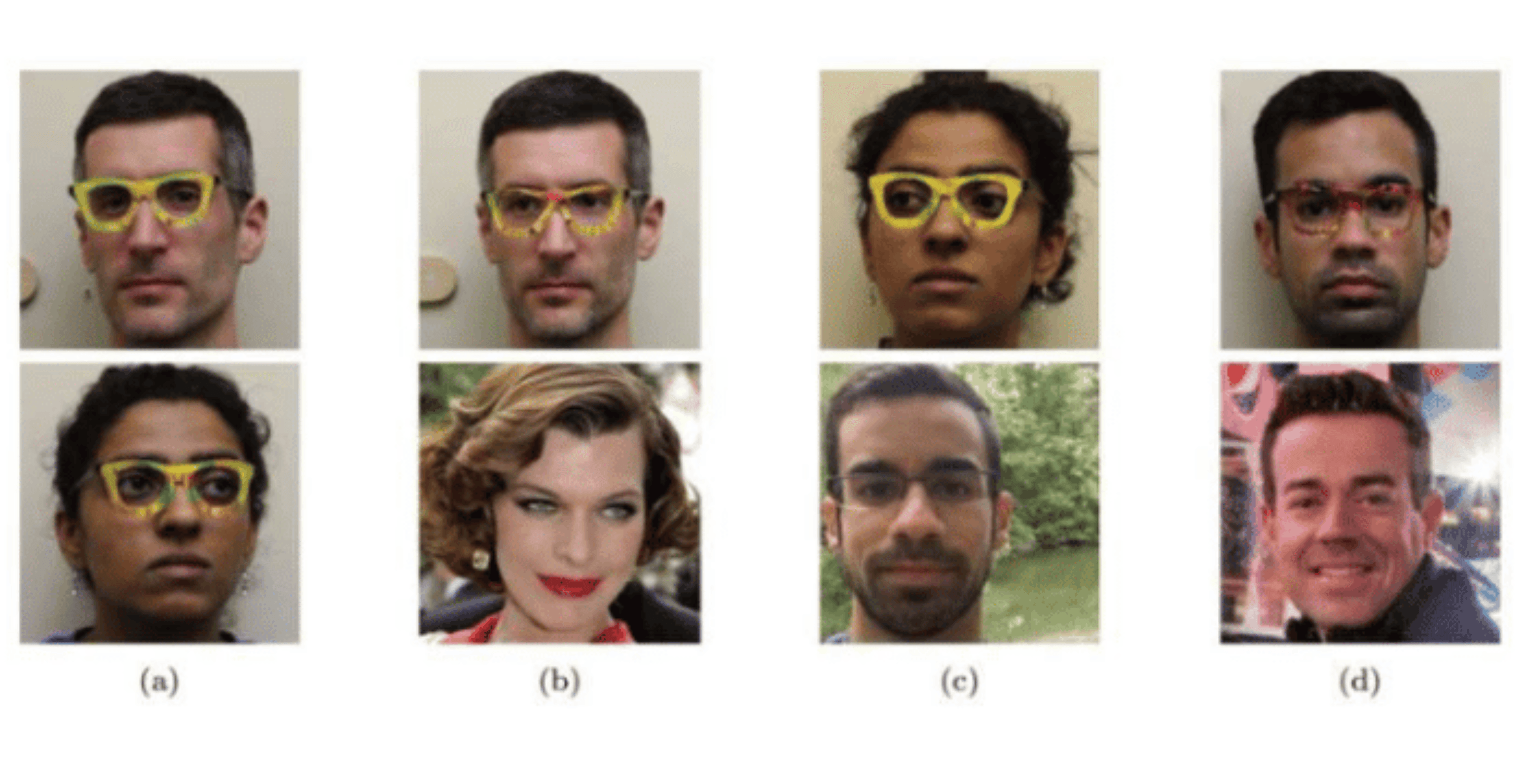
Source: Tech Xplore
Facial recognition systems can also be targeted by adversarial attacks. One notable example involves researchers creating a pair of eyeglass frames with a specific pattern that, when worn, could either cause the facial recognition system to fail to recognize the person or, more alarmingly, misidentify them as an entirely different individual. This poses significant security concerns for systems relying on facial recognition for authentication.
Mitigating Adversarial Examples Through Adversarial Training
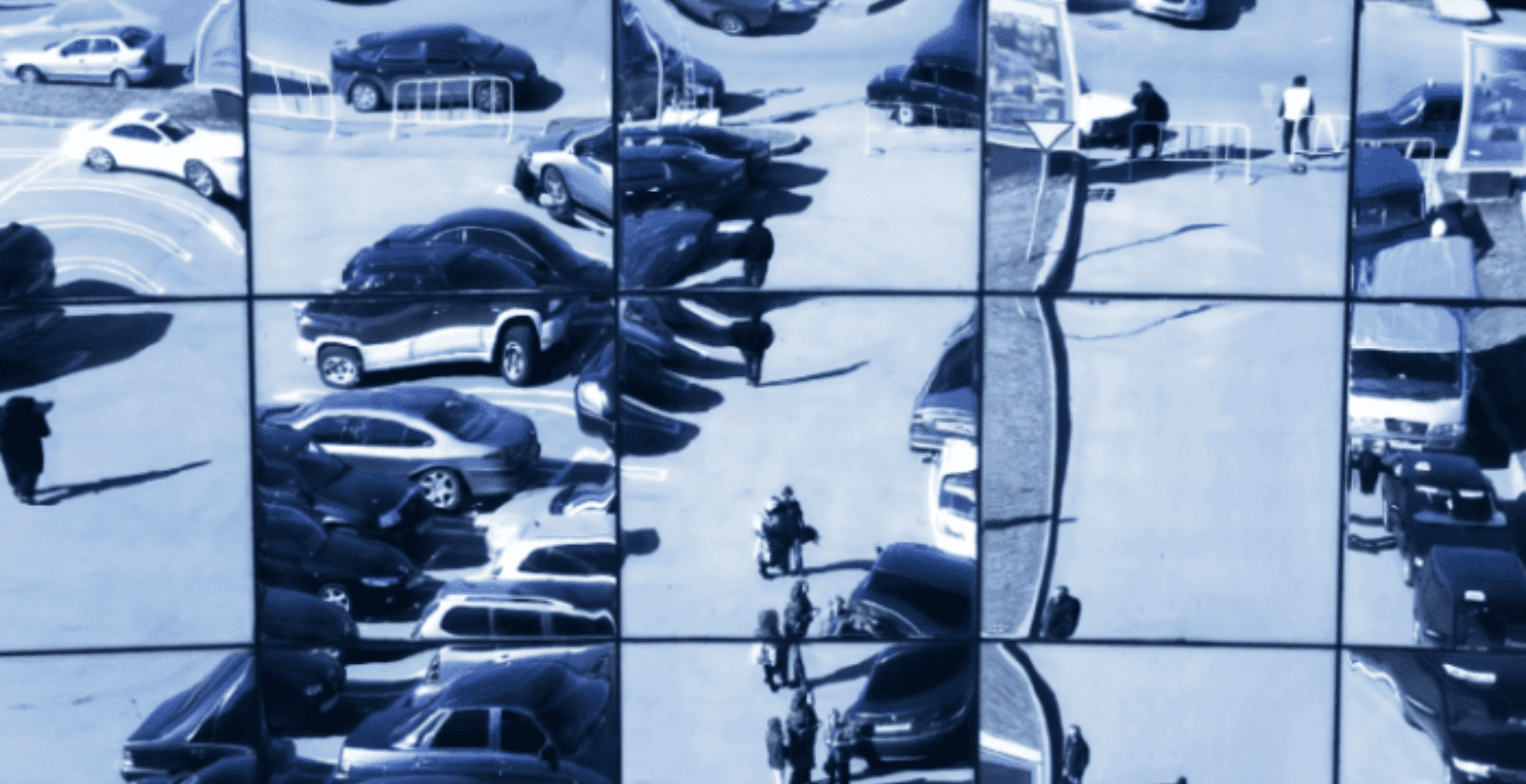
Source: Wired
Adversarial training is employed to counteract such vulnerabilities. For instance, CAPTCHA systems used widely on the internet to distinguish between humans and automated bots, have evolved to incorporate adversarially generated examples that make it difficult for AI-based bots to solve while still being accessible to humans.
This constant updating of CAPTCHA challenges is a form of adversarial training, ensuring the robustness of the CAPTCHA system against automated attacks.
Architectural Innovations for Resisting Adversarial Manipulations
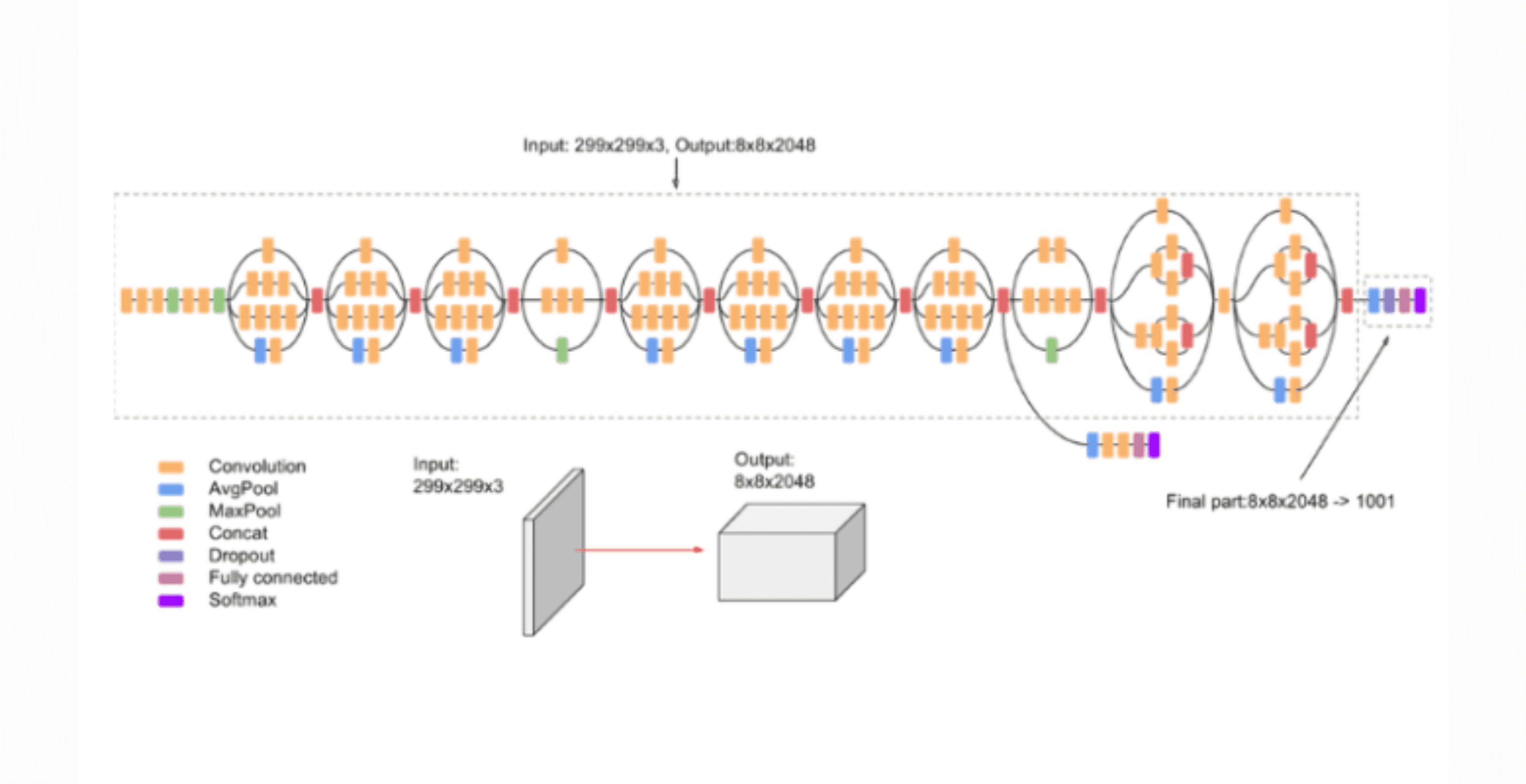
Source: Google Cloud
RealDeep neural networks, particularly those used in image recognition tasks, are being designed with new architectural features to enhance robustness. Google's Inception network is an example of architecture designed to be deep and wide, offering multiple paths for information processing.
This complexity and diversity in processing help mitigate the impact of adversarial examples by not relying on a single pathway for decision-making, thereby increasing the model's resilience against adversarial attacks.
In each of these examples, the focus on robustness and the development of countermeasures against adversarial attacks underscore the ongoing efforts to ensure AI systems can be trusted and safely integrated into critical applications. The field of AI robustness is a dynamic area of research, constantly evolving to meet and neutralize emerging threats.
Core Areas of AI Safety and Security Research
Research in AI safety and security encapsulates diverse studies to ensure artificial intelligence systems operate within safe bounds, devoid of unintended consequences, and remain under human control.
This domain's breadth requires a multifaceted approach, addressing everything from the robustness of AI systems against manipulation to their alignment with human ethical standards.
Let's delve deeper into the core areas of AI safety and security research.
Robustness
Robustness in AI systems refers to maintaining operational integrity in adversarial inputs or conditions.
An essential aspect of robustness is studying and mitigating adversarial examples — inputs deliberately designed to cause the AI system to make errors.
These inputs exploit the model's vulnerabilities, which, if unaddressed, pose significant security implications, especially in critical applications like autonomous driving or facial recognition systems.
Research in this area focuses on developing techniques such as adversarial training, where models are trained with adversarial examples to improve their resilience, and exploring new architectural features that inherently resist adversarial manipulations.
Monitoring
The monitoring of AI systems involves continuous oversight of their operation to estimate uncertainty, detect anomalies, or identify instances of malicious use.
Effective monitoring mechanisms can alert human operators to potential failures or external attacks on the system, enabling timely interventions. This area of research is particularly challenging due to the complexity and often opaque nature of AI models and intense neural networks.
Techniques such as uncertainty quantification, which aims to provide probabilistic estimates of the AI's predictions and the development of anomaly detection algorithms that can operate in high-dimensional spaces are at the forefront of addressing these challenges.
Transparency
Transparency in AI involves making the decision-making processes of AI systems understandable to humans.
This is crucial for trust, accountability, and ethical considerations. The challenge lies in the inherent complexity of AI models, such as those based on deep learning, which can act as "black boxes" with internal workings that are difficult to interpret.
Research efforts aim to develop interpretable AI models or post-hoc explanation methods that can provide insights into the model's decision criteria.
Techniques such as feature importance scores, decision trees, or model-agnostic explanation frameworks like LIME (Local Interpretable Model-agnostic Explanations) and SHAP (Shapley Additive exPlanations) are examples of work done to improve AI transparency.
Alignment
AI alignment ensures that AI systems' objectives and behaviours harmonise with human values and ethical principles.
This is critical to avoid scenarios where AI systems pursue goals misaligned with human intentions, potentially leading to harmful outcomes.
Research in this area encompasses the specification of clear, unambiguous objectives for AI systems, the development of value alignment techniques to incorporate human ethical considerations into AI decision-making processes, and the exploration of methods for safe and controlled AI exploration within defined boundaries.
This also includes work on inverse reinforcement learning, where AI models implicitly learn human values and preferences by observing human actions.
Systemic Safety
Systemic safety extends the focus of AI safety research beyond the technical aspects of individual AI systems to consider the broader socio-technical environment in which these systems operate.
This includes examining how AI technologies interact with societal structures, legal frameworks, economic incentives, and human behaviours.
Research in systemic safety is concerned with identifying and mitigating risks that emerge from integrating AI systems into complex human societies, including fairness, Privacy, and the potential for socioeconomic disruptions.
It also involves designing governance mechanisms and safety standards to guide the responsible development, deployment, and use of AI technologies across different sectors.
Challenges and Opportunities in AI Safety
As Large Language Models (LLMs) grow in complexity and application, they introduce nuanced safety challenges that necessitate reevaluating and scaling current AI safety measures. The intricacies of these models mean that even minor inaccuracies or biases can be amplified, leading to potentially significant safety concerns. This scenario underscores the necessity of transforming localized AI safety measures into universally applicable solutions, a daunting challenge filled with opportunities.
Technical Challenges:
Interpretability: As LLMs become more complex, understanding their decision-making processes and identifying the sources of errors or biases becomes increasingly tricky. Developing techniques for improving the interpretability of these models is crucial.
Adversarial Attacks: The sophistication of adversarial attacks grows alongside the advancement of AI models. Developing robust defenses against such attacks, especially those that exploit subtle model vulnerabilities, is a pressing challenge.
Data Bias and Fairness: Ensuring that AI systems are fair and unbiased requires comprehensive strategies to identify and mitigate biases in training data and model predictions.
Opportunities:
Collaborative Research: By fostering a collaborative research environment, the AI community can share insights, tools, and methodologies to address safety concerns collectively. Consortium approaches can accelerate the development of standards and best practices.
Innovative Solutions: The challenges posed by advanced LLMs also open up avenues for innovation in AI safety techniques, including new forms of model auditing, monitoring, and explanation.
Global Standards and Protocols: Developing and adopting international standards for AI safety can help ensure that safety measures are consistently applied across industries and borders, facilitating a unified approach to AI safety.
AI Safety Standards and Governance
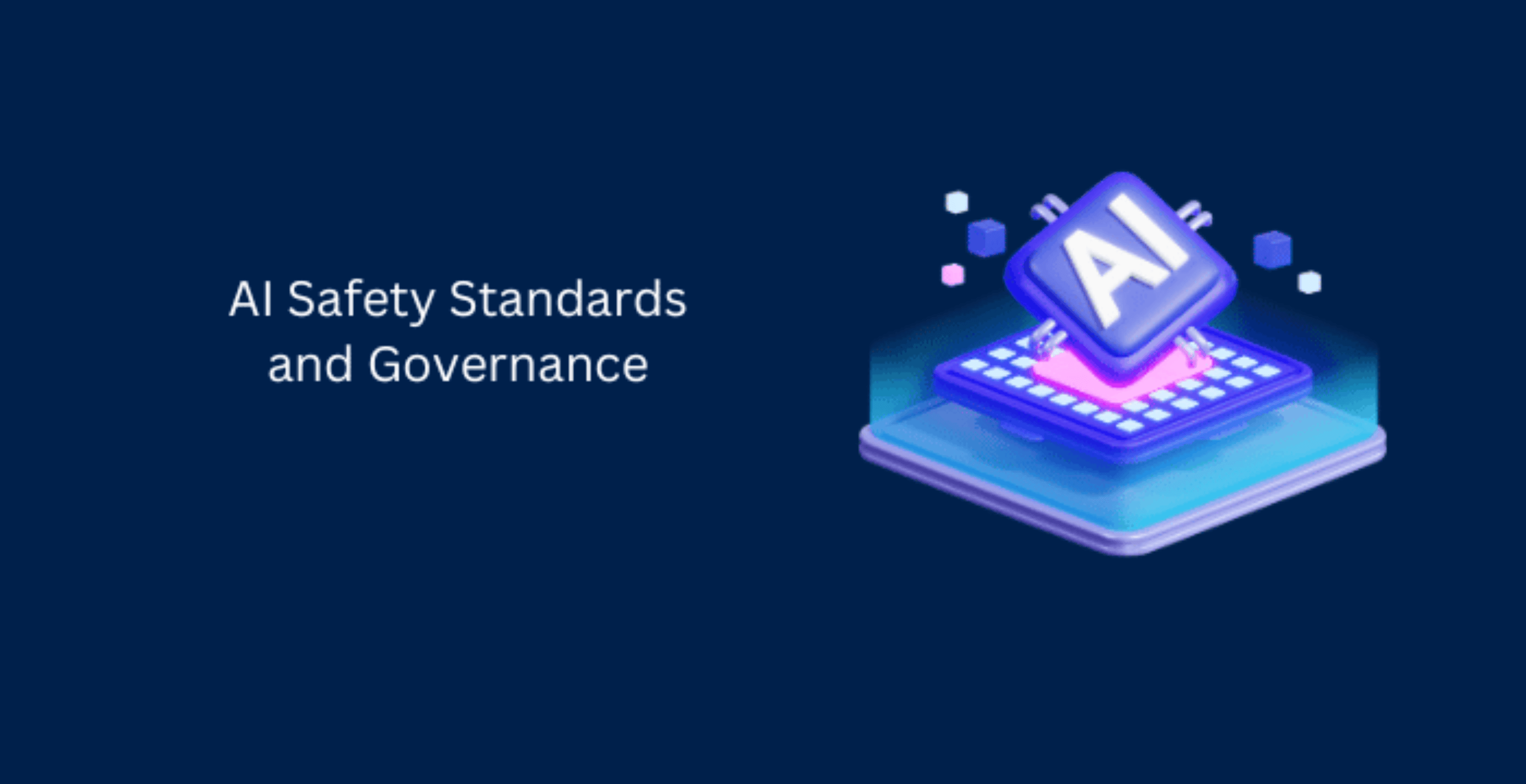
The role of governance in AI safety cannot be overstated. Effective governance mechanisms, underpinned by robust policies and frameworks, are essential for guiding the responsible development and deployment of AI technologies.
Google’s Secure AI Framework (SAIF) represents a notable effort in this direction, providing a structured approach to ensuring the safety and security of AI systems.
Technical Aspects of AI Governance:
Standardization of Safety Protocols: Developing and implementing industry-wide standards can help create a baseline for safety and security practices, making it easier for AI developers to adhere to best practices.
Risk Assessment Frameworks: Technical guidelines for conducting comprehensive risk assessments of AI systems can aid in identifying potential safety issues before deployment.
Certification Processes: Establishing certification processes for AI systems can ensure they meet defined safety and security criteria, enhancing trust among users and stakeholders.
Institutional and Global Initiatives
Initiatives from research centers, academic institutions, and international collaborations significantly bolster the push toward a safer AI future. Stanford’s flagship AI Safety projects exemplify the targeted research that can lead to breakthroughs in AI safety methodologies, offering scalable and practical solutions.
UN Resolution on AI
The United Nations, recognizing the transformative impact of AI on global issues, including sustainable development, human rights, and peace and security, has taken steps to integrate AI governance into its agenda.
The UN Resolution on AI, though not a legislative instrument like the EU AI Act, serves as a call to action for member states to consider AI's ethical, security, and socioeconomic aspects. It encourages the development of international cooperation on AI policy and research, focusing on leveraging AI for achieving the Sustainable Development Goals (SDGs).
The resolution emphasizes the importance of inclusivity, transparency, Privacy, and accountability, aiming to ensure that AI benefits all of humanity while minimizing its potential harms.
US White House Executive Order on AI
The United States has also recognized the need for a national strategy on AI. The White House Executive Order on AI outlines the American approach to promoting and protecting national AI technology and innovation.
The order focuses on maintaining American leadership in AI, directing federal agencies to prioritize AI investments, improving access to quality AI data, and setting governance standards to guide responsible AI development and use.
Additionally, it emphasizes the importance of AI education and workforce development, ensuring that Americans are prepared to contribute to and benefit from AI technologies. The executive order reflects a strategic commitment to fostering an environment encouraging AI innovation while providing the ethical, safe, and lawful use of AI technologies.
You can read more here: The EU AI Act - All you need to know
Technical Contributions and Collaborations:
Cross-Disciplinary Research: Combining expertise from computer science, ethics, psychology, and law can provide a holistic approach to AI safety, addressing both technical and societal concerns.
Benchmarking and Evaluation: Developing standardized benchmarks and evaluation criteria for AI safety can facilitate objective assessments of AI systems' performance in terms of safety and reliability.
Open-Source Tools and Libraries: Contributions of open-source tools and libraries for AI safety can democratize access to advanced safety technologies, enabling more developers to incorporate safety features into their AI systems.
Global Safety Networks:
International collaborations, such as those facilitated by the Global Partnership on AI (GPAI), can harmonize efforts to promote AI safety, ensuring that insights and innovations are shared globally. This collective journey towards AI safety is characterized by a shared commitment to developing AI technologies that are powerful, innovative, safe, ethical, and beneficial for society.
Conclusion
Understanding the fundamental concepts of AI safety and security is pivotal for the sustainable development and deployment of AI technologies.
By addressing the intricate challenges and leveraging collaborative efforts, the goal of establishing robust, secure, and responsible AI systems becomes increasingly attainable, paving the way for a future where AI contributes positively to society while minimizing risks.
RagaAI recognizes the critical importance of governance in ensuring AI safety and security. By adhering to and advocating for robust governance frameworks like Google’s Secure AI Framework (SAIF), RagaAI exemplifies responsible AI development.
Its participation in shaping policies and frameworks underscores its dedication to creating a safer AI ecosystem for all.
The realm of artificial intelligence (AI) is undergoing a transformative era, primarily driven by advancements in machine learning (ML). However, this rapid evolution brings a notable absence of safety guarantees, raising the stakes for potential risks associated with AI system failures.
This article outlines the essential definitions of AI safety and security, emphasizing the objectives of ensuring robustness, assurance, specification, and overarching security within AI systems.
Motivations for AI Safety
AI's ability to exhibit power-seeking behaviours presents unique risks, necessitating a closer examination of current and emerging threats posed by AI technologies. This discourse extends to existential risks alongside societal-scale threats while drawing on historical perspectives to underscore the critical importance of fostering responsible AI practices.
Adversarial Examples in Autonomous Driving
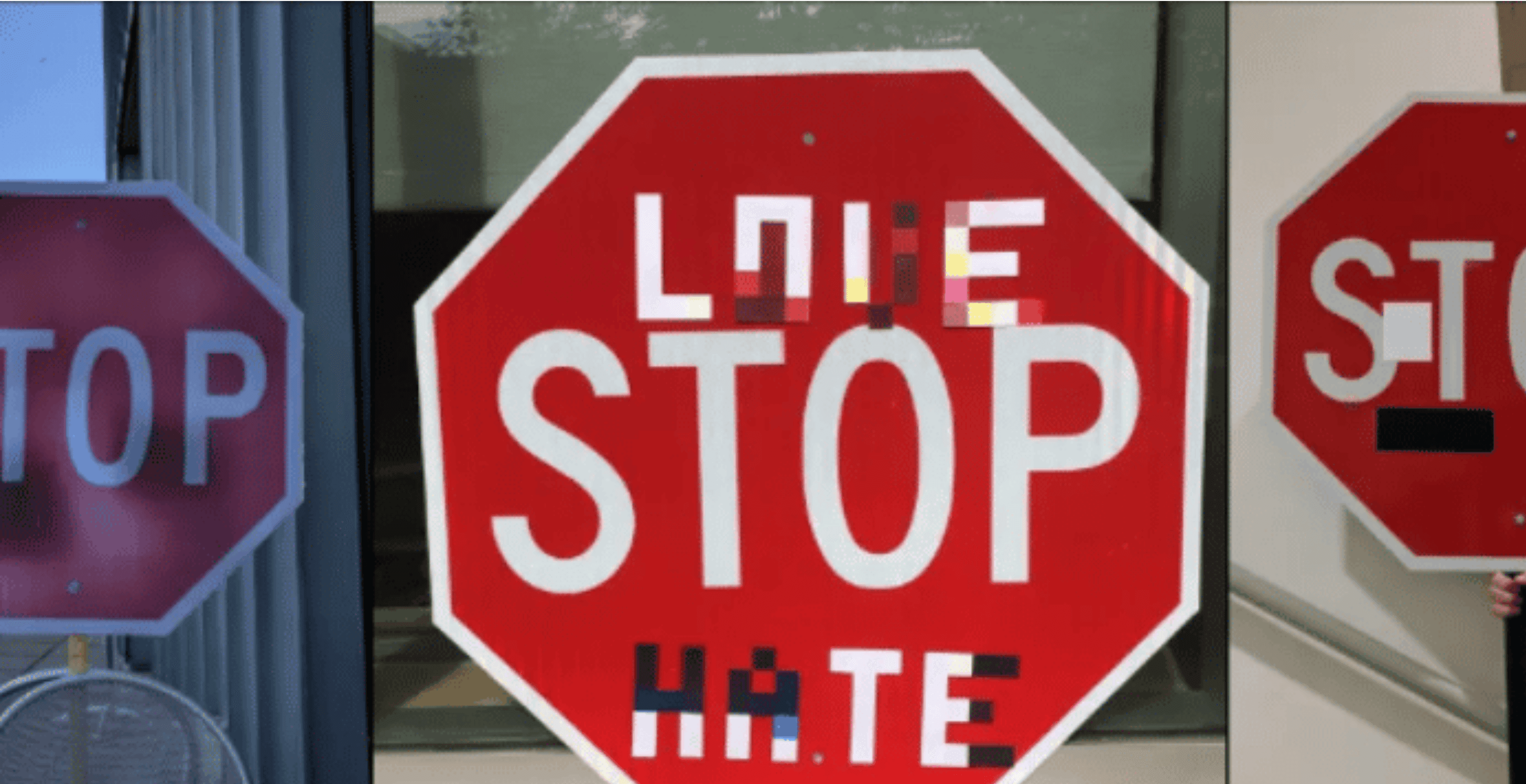
Source: Global News
Negative examples can manifest as subtle alterations to road signs in autonomous driving systems. A well-documented instance involved researchers altering the appearance of a stop sign just slightly by adding stickers in a specific pattern. The sign still appeared as a stop sign to the human eye, but the autonomous vehicle's AI system misinterpreted it as a 45 mph speed limit sign.
Adversarial Examples in Facial Recognition Systems
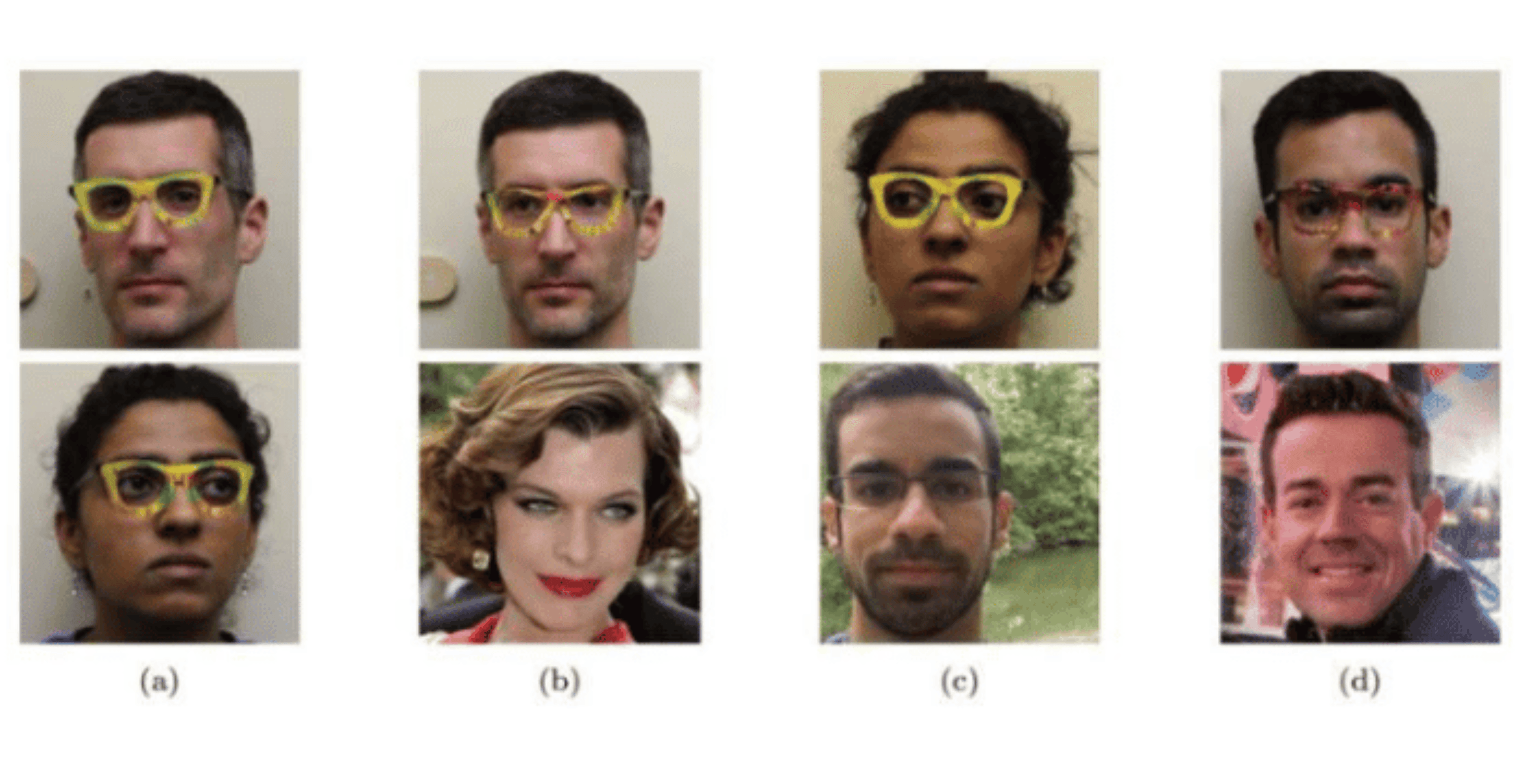
Source: Tech Xplore
Facial recognition systems can also be targeted by adversarial attacks. One notable example involves researchers creating a pair of eyeglass frames with a specific pattern that, when worn, could either cause the facial recognition system to fail to recognize the person or, more alarmingly, misidentify them as an entirely different individual. This poses significant security concerns for systems relying on facial recognition for authentication.
Mitigating Adversarial Examples Through Adversarial Training
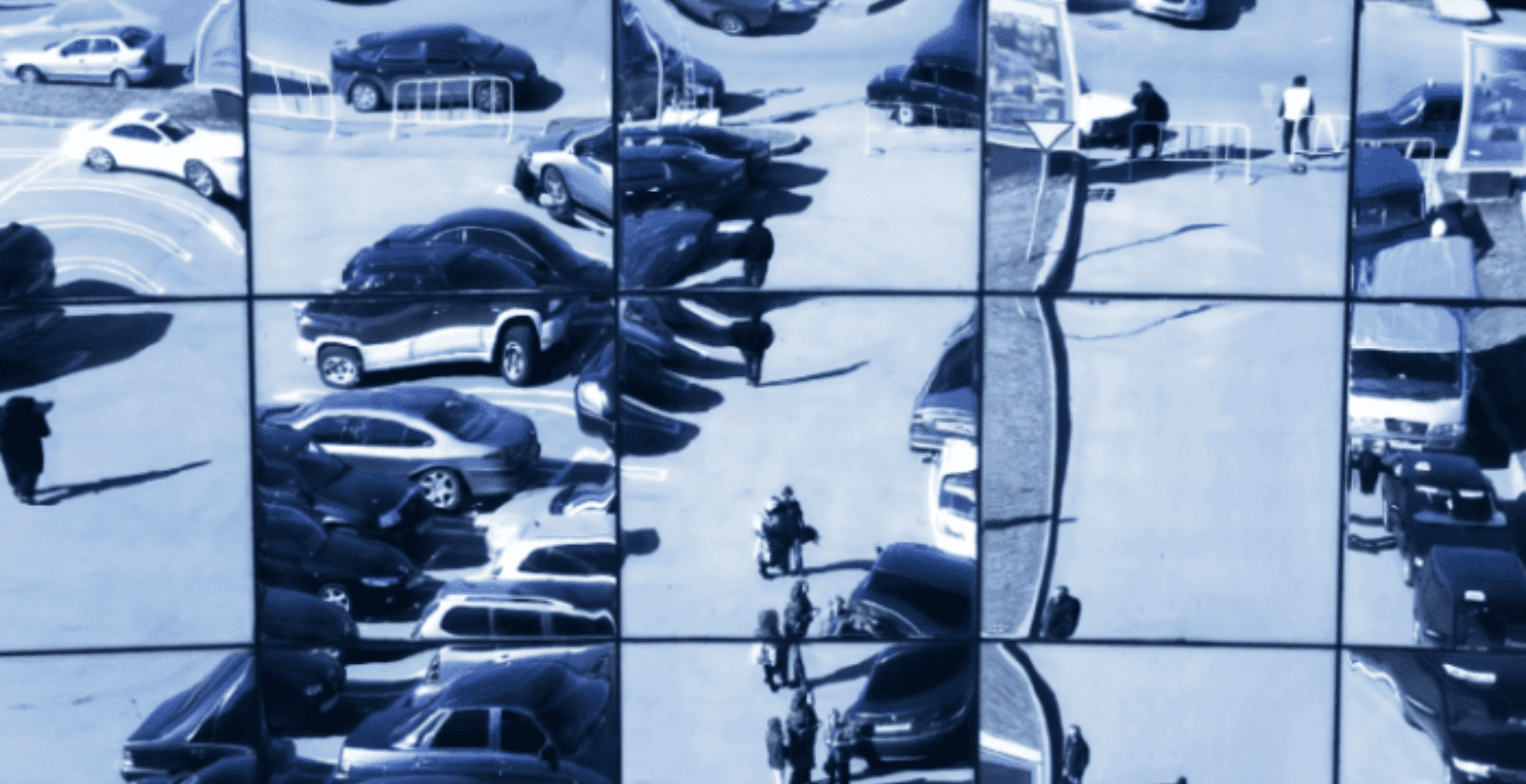
Source: Wired
Adversarial training is employed to counteract such vulnerabilities. For instance, CAPTCHA systems used widely on the internet to distinguish between humans and automated bots, have evolved to incorporate adversarially generated examples that make it difficult for AI-based bots to solve while still being accessible to humans.
This constant updating of CAPTCHA challenges is a form of adversarial training, ensuring the robustness of the CAPTCHA system against automated attacks.
Architectural Innovations for Resisting Adversarial Manipulations
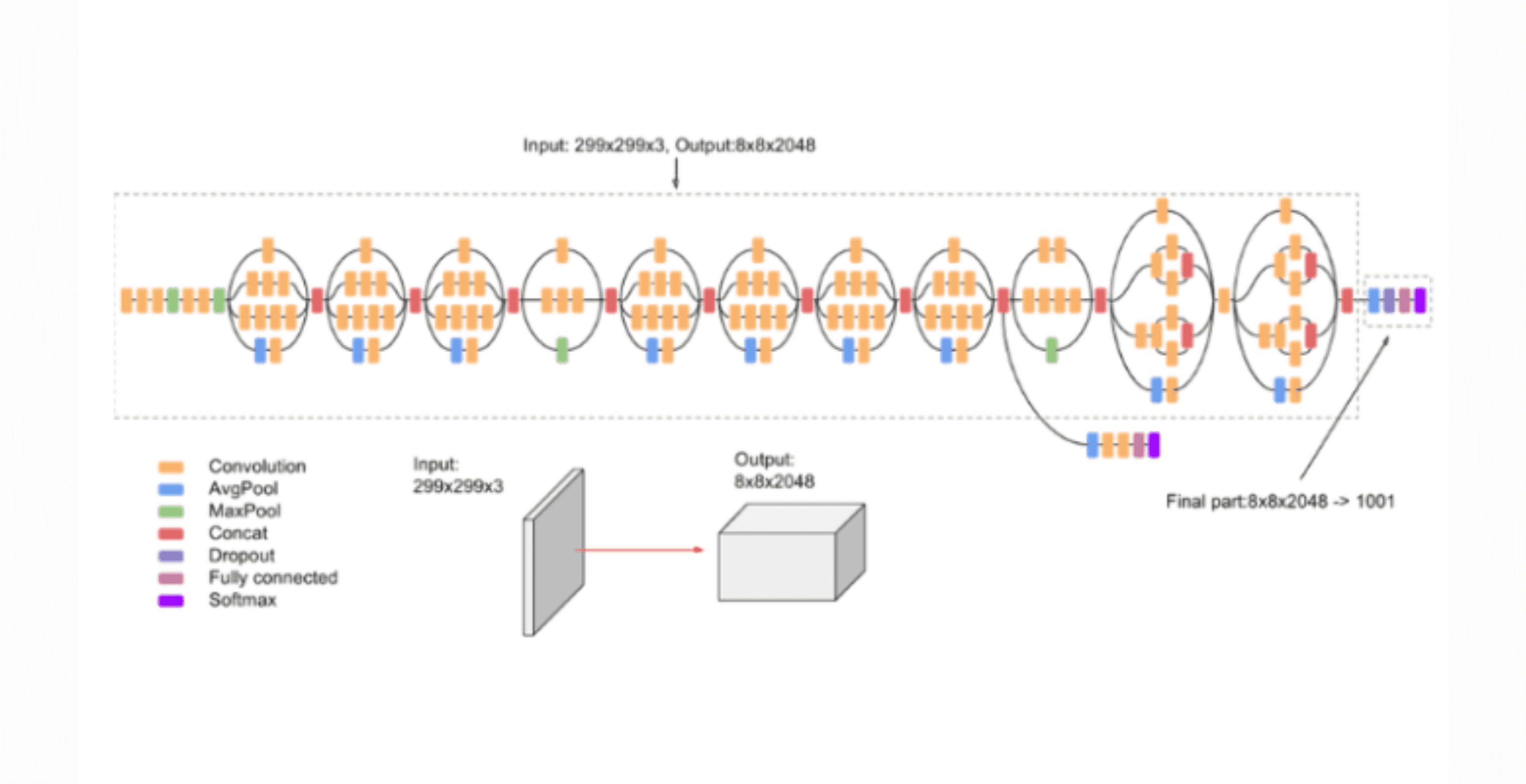
Source: Google Cloud
RealDeep neural networks, particularly those used in image recognition tasks, are being designed with new architectural features to enhance robustness. Google's Inception network is an example of architecture designed to be deep and wide, offering multiple paths for information processing.
This complexity and diversity in processing help mitigate the impact of adversarial examples by not relying on a single pathway for decision-making, thereby increasing the model's resilience against adversarial attacks.
In each of these examples, the focus on robustness and the development of countermeasures against adversarial attacks underscore the ongoing efforts to ensure AI systems can be trusted and safely integrated into critical applications. The field of AI robustness is a dynamic area of research, constantly evolving to meet and neutralize emerging threats.
Core Areas of AI Safety and Security Research
Research in AI safety and security encapsulates diverse studies to ensure artificial intelligence systems operate within safe bounds, devoid of unintended consequences, and remain under human control.
This domain's breadth requires a multifaceted approach, addressing everything from the robustness of AI systems against manipulation to their alignment with human ethical standards.
Let's delve deeper into the core areas of AI safety and security research.
Robustness
Robustness in AI systems refers to maintaining operational integrity in adversarial inputs or conditions.
An essential aspect of robustness is studying and mitigating adversarial examples — inputs deliberately designed to cause the AI system to make errors.
These inputs exploit the model's vulnerabilities, which, if unaddressed, pose significant security implications, especially in critical applications like autonomous driving or facial recognition systems.
Research in this area focuses on developing techniques such as adversarial training, where models are trained with adversarial examples to improve their resilience, and exploring new architectural features that inherently resist adversarial manipulations.
Monitoring
The monitoring of AI systems involves continuous oversight of their operation to estimate uncertainty, detect anomalies, or identify instances of malicious use.
Effective monitoring mechanisms can alert human operators to potential failures or external attacks on the system, enabling timely interventions. This area of research is particularly challenging due to the complexity and often opaque nature of AI models and intense neural networks.
Techniques such as uncertainty quantification, which aims to provide probabilistic estimates of the AI's predictions and the development of anomaly detection algorithms that can operate in high-dimensional spaces are at the forefront of addressing these challenges.
Transparency
Transparency in AI involves making the decision-making processes of AI systems understandable to humans.
This is crucial for trust, accountability, and ethical considerations. The challenge lies in the inherent complexity of AI models, such as those based on deep learning, which can act as "black boxes" with internal workings that are difficult to interpret.
Research efforts aim to develop interpretable AI models or post-hoc explanation methods that can provide insights into the model's decision criteria.
Techniques such as feature importance scores, decision trees, or model-agnostic explanation frameworks like LIME (Local Interpretable Model-agnostic Explanations) and SHAP (Shapley Additive exPlanations) are examples of work done to improve AI transparency.
Alignment
AI alignment ensures that AI systems' objectives and behaviours harmonise with human values and ethical principles.
This is critical to avoid scenarios where AI systems pursue goals misaligned with human intentions, potentially leading to harmful outcomes.
Research in this area encompasses the specification of clear, unambiguous objectives for AI systems, the development of value alignment techniques to incorporate human ethical considerations into AI decision-making processes, and the exploration of methods for safe and controlled AI exploration within defined boundaries.
This also includes work on inverse reinforcement learning, where AI models implicitly learn human values and preferences by observing human actions.
Systemic Safety
Systemic safety extends the focus of AI safety research beyond the technical aspects of individual AI systems to consider the broader socio-technical environment in which these systems operate.
This includes examining how AI technologies interact with societal structures, legal frameworks, economic incentives, and human behaviours.
Research in systemic safety is concerned with identifying and mitigating risks that emerge from integrating AI systems into complex human societies, including fairness, Privacy, and the potential for socioeconomic disruptions.
It also involves designing governance mechanisms and safety standards to guide the responsible development, deployment, and use of AI technologies across different sectors.
Challenges and Opportunities in AI Safety
As Large Language Models (LLMs) grow in complexity and application, they introduce nuanced safety challenges that necessitate reevaluating and scaling current AI safety measures. The intricacies of these models mean that even minor inaccuracies or biases can be amplified, leading to potentially significant safety concerns. This scenario underscores the necessity of transforming localized AI safety measures into universally applicable solutions, a daunting challenge filled with opportunities.
Technical Challenges:
Interpretability: As LLMs become more complex, understanding their decision-making processes and identifying the sources of errors or biases becomes increasingly tricky. Developing techniques for improving the interpretability of these models is crucial.
Adversarial Attacks: The sophistication of adversarial attacks grows alongside the advancement of AI models. Developing robust defenses against such attacks, especially those that exploit subtle model vulnerabilities, is a pressing challenge.
Data Bias and Fairness: Ensuring that AI systems are fair and unbiased requires comprehensive strategies to identify and mitigate biases in training data and model predictions.
Opportunities:
Collaborative Research: By fostering a collaborative research environment, the AI community can share insights, tools, and methodologies to address safety concerns collectively. Consortium approaches can accelerate the development of standards and best practices.
Innovative Solutions: The challenges posed by advanced LLMs also open up avenues for innovation in AI safety techniques, including new forms of model auditing, monitoring, and explanation.
Global Standards and Protocols: Developing and adopting international standards for AI safety can help ensure that safety measures are consistently applied across industries and borders, facilitating a unified approach to AI safety.
AI Safety Standards and Governance
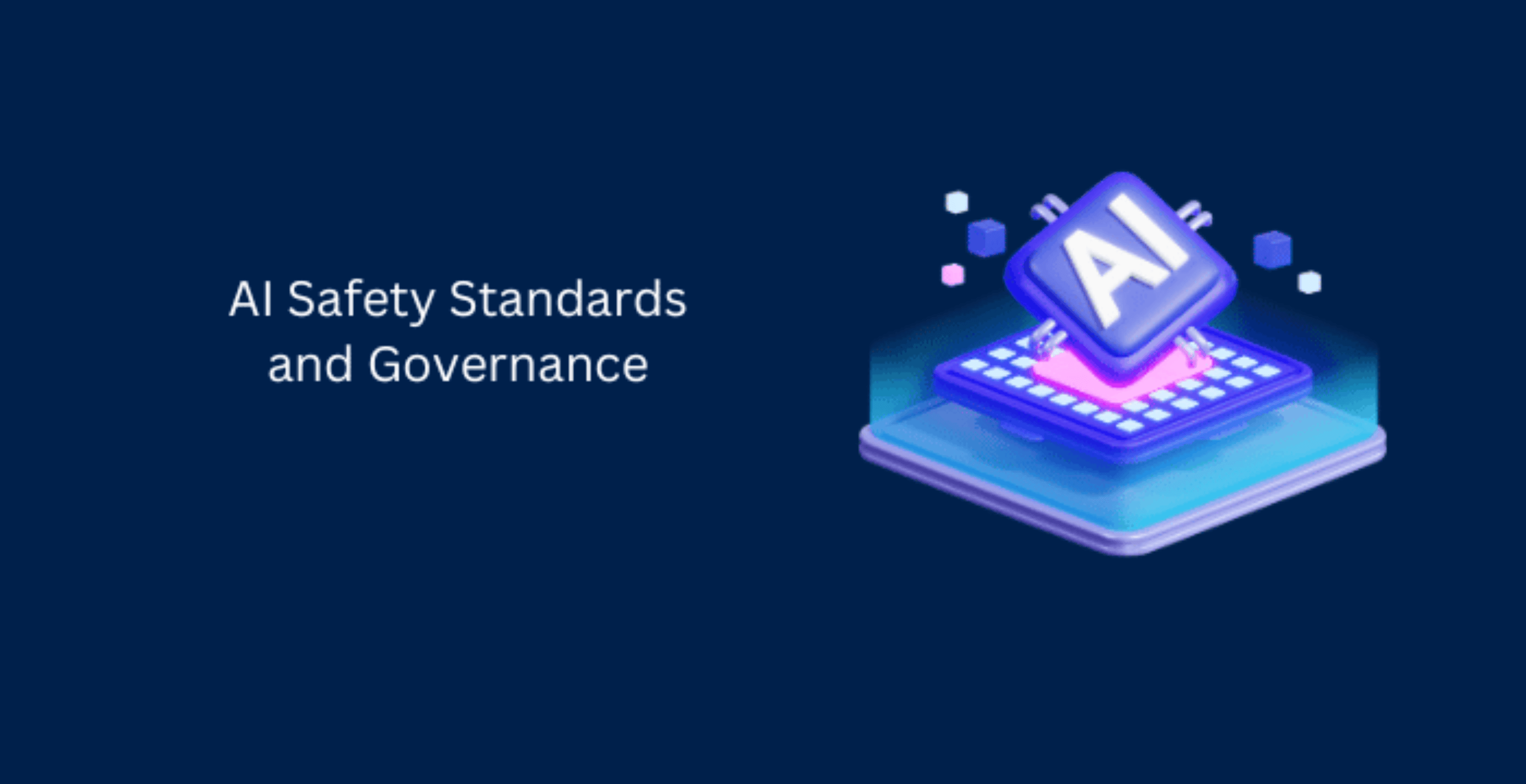
The role of governance in AI safety cannot be overstated. Effective governance mechanisms, underpinned by robust policies and frameworks, are essential for guiding the responsible development and deployment of AI technologies.
Google’s Secure AI Framework (SAIF) represents a notable effort in this direction, providing a structured approach to ensuring the safety and security of AI systems.
Technical Aspects of AI Governance:
Standardization of Safety Protocols: Developing and implementing industry-wide standards can help create a baseline for safety and security practices, making it easier for AI developers to adhere to best practices.
Risk Assessment Frameworks: Technical guidelines for conducting comprehensive risk assessments of AI systems can aid in identifying potential safety issues before deployment.
Certification Processes: Establishing certification processes for AI systems can ensure they meet defined safety and security criteria, enhancing trust among users and stakeholders.
Institutional and Global Initiatives
Initiatives from research centers, academic institutions, and international collaborations significantly bolster the push toward a safer AI future. Stanford’s flagship AI Safety projects exemplify the targeted research that can lead to breakthroughs in AI safety methodologies, offering scalable and practical solutions.
UN Resolution on AI
The United Nations, recognizing the transformative impact of AI on global issues, including sustainable development, human rights, and peace and security, has taken steps to integrate AI governance into its agenda.
The UN Resolution on AI, though not a legislative instrument like the EU AI Act, serves as a call to action for member states to consider AI's ethical, security, and socioeconomic aspects. It encourages the development of international cooperation on AI policy and research, focusing on leveraging AI for achieving the Sustainable Development Goals (SDGs).
The resolution emphasizes the importance of inclusivity, transparency, Privacy, and accountability, aiming to ensure that AI benefits all of humanity while minimizing its potential harms.
US White House Executive Order on AI
The United States has also recognized the need for a national strategy on AI. The White House Executive Order on AI outlines the American approach to promoting and protecting national AI technology and innovation.
The order focuses on maintaining American leadership in AI, directing federal agencies to prioritize AI investments, improving access to quality AI data, and setting governance standards to guide responsible AI development and use.
Additionally, it emphasizes the importance of AI education and workforce development, ensuring that Americans are prepared to contribute to and benefit from AI technologies. The executive order reflects a strategic commitment to fostering an environment encouraging AI innovation while providing the ethical, safe, and lawful use of AI technologies.
You can read more here: The EU AI Act - All you need to know
Technical Contributions and Collaborations:
Cross-Disciplinary Research: Combining expertise from computer science, ethics, psychology, and law can provide a holistic approach to AI safety, addressing both technical and societal concerns.
Benchmarking and Evaluation: Developing standardized benchmarks and evaluation criteria for AI safety can facilitate objective assessments of AI systems' performance in terms of safety and reliability.
Open-Source Tools and Libraries: Contributions of open-source tools and libraries for AI safety can democratize access to advanced safety technologies, enabling more developers to incorporate safety features into their AI systems.
Global Safety Networks:
International collaborations, such as those facilitated by the Global Partnership on AI (GPAI), can harmonize efforts to promote AI safety, ensuring that insights and innovations are shared globally. This collective journey towards AI safety is characterized by a shared commitment to developing AI technologies that are powerful, innovative, safe, ethical, and beneficial for society.
Conclusion
Understanding the fundamental concepts of AI safety and security is pivotal for the sustainable development and deployment of AI technologies.
By addressing the intricate challenges and leveraging collaborative efforts, the goal of establishing robust, secure, and responsible AI systems becomes increasingly attainable, paving the way for a future where AI contributes positively to society while minimizing risks.
RagaAI recognizes the critical importance of governance in ensuring AI safety and security. By adhering to and advocating for robust governance frameworks like Google’s Secure AI Framework (SAIF), RagaAI exemplifies responsible AI development.
Its participation in shaping policies and frameworks underscores its dedication to creating a safer AI ecosystem for all.