Navigating AI Governance in Aerospace Industry
Navigating AI Governance in Aerospace Industry
Navigating AI Governance in Aerospace Industry
Akshat Gupta
Apr 3, 2024




As we usher into a new era of regulations, i.e., regulations on Artificial Intelligence, it becomes imperative to understand the governance landscape in each industry meticulously. AI is not a new technology and there are a lot of industries which have been using AI/ML, in some form, for more than a decade. Aerospace industry is one of them. Moreover, due to a very low risk-appetite, Aerospace technology has witnessed and compiled with strict regulatory measures drawn over time, as the situation demanded. This article aims at understanding the regulatory landscape in the Aerospace industry, in terms of various governing bodies and acts, requirements , and solutions to those requirements. We aim to talk more about AI Compliance with regulations in this article, which caters to overall AI governance by itself.
What has been done so far ?
When it comes to understanding the regulations drafted or implemented so far, EASA ( European Union Aviation Safety Agency ) and FAA ( Federal Aviation Administration ) are the major governing bodies in the EU and the USA respectively.
While the FAA is still in process to publish any concrete guidelines or restrictions on AI, EASA is way ahead with an extensive AI/ML regulation framework in place.
EASA, under its AI Roadmap, released version 2 of a concept paper establishing the guiding principles for Level 1 and 2 Machine Learning applications. This paper provides a first set of usable objectives in Aviation AI compliance and also serves as a base for formal regulatory developments to come into force. The paper aims to develop principles and guidance which can be integrated into rules and acceptable means of compliance (AMC) later on.
Let’s take a look at the framework.
The guidelines by EASA are so far the most extensive and well-defined set of steps published in any industry for ensuring a safe & ethical AI and regulatory compliance of that AI.

Source: EASA
The figure shows the building blocks for establishing trustworthy AI systems.
AI Trustworthiness Analysis
The AI trustworthiness analysis serves as a gateway to three other technical building blocks by aligning with EU Ethical Guidelines.
It involves characterising the AI application, conducting an ethics-based assessment, and performing safety and security assessments.
These assessments are crucial for the development and approval of AI/ML systems, following existing mandatory practices in industries like aviation.
While safety and security assessments maintain their principles developed originally for software, they require additional guidance to accommodate AI techniques.
AI Assurance
The AI assurance building block is intended to address the AI-specific guidance pertaining to the AIbased system. It encompasses three major topics -
Learning Assurance - It covers the paradigm shift from programming to learning, as the existing development assurance methods are not adapted to cover learning processes specific to AI/ML.

Source: EASA
W-Shaped process for Learning Assurance
Development & post-ops explainability - This deals with the capability to provide users with understandable, reliable and relevant information with the appropriate level of detail on how an AI/ML application produces its results.
Data recording capabilities - This addresses two specific operational and post operational purposes: on the one hand the continuous monitoring of the safety of the AI-based system and on the other hand the support to incident or accident investigation.
Human Factors for AI
This block introduces the necessary guidance to account for the specific human factors needs linked with the introduction of AI.
Among other aspects, AI operational explainability deals with the capability to provide the end users with understandable, reliable and relevant information with the appropriate level of detail and with appropriate timing on how an AI/ML application produces its results. This block also introduces the concept of human-AI teaming to ensure adequate cooperation or collaboration between end users and AI-based systems to achieve certain goals.
AI Safety and Risk Mitigation
This building block considers that we may not always be able to open the ‘AI
black box’ to satisfy the whole set of objectives defined for the AI assurance and the human factors for AI building blocks, and that the associated residual risk may need to be addressed to deal with the inherent uncertainty of AI.
How does RagaAI help ?
As we understand, under the purview of these guidelines, enterprises working on developing AI systems for applications in different aspects of aviation must be able to manage the associated risks well. While the enforcement and adoption of these obligations comes into full effect ( already started ), RagaAI offers an invaluable approach on helping expedite this endeavour. With its comprehensive solutions, RagaAI helps businesses identify, break-down and and comply with the obligations that the regulators propose. These solutions work across all modalities of data.
RagaAI provides comprehensive tests catering to the requirements of the act (laid out objectively), using cutting-edge methods, concrete frameworks and extensive visualisation techniques.
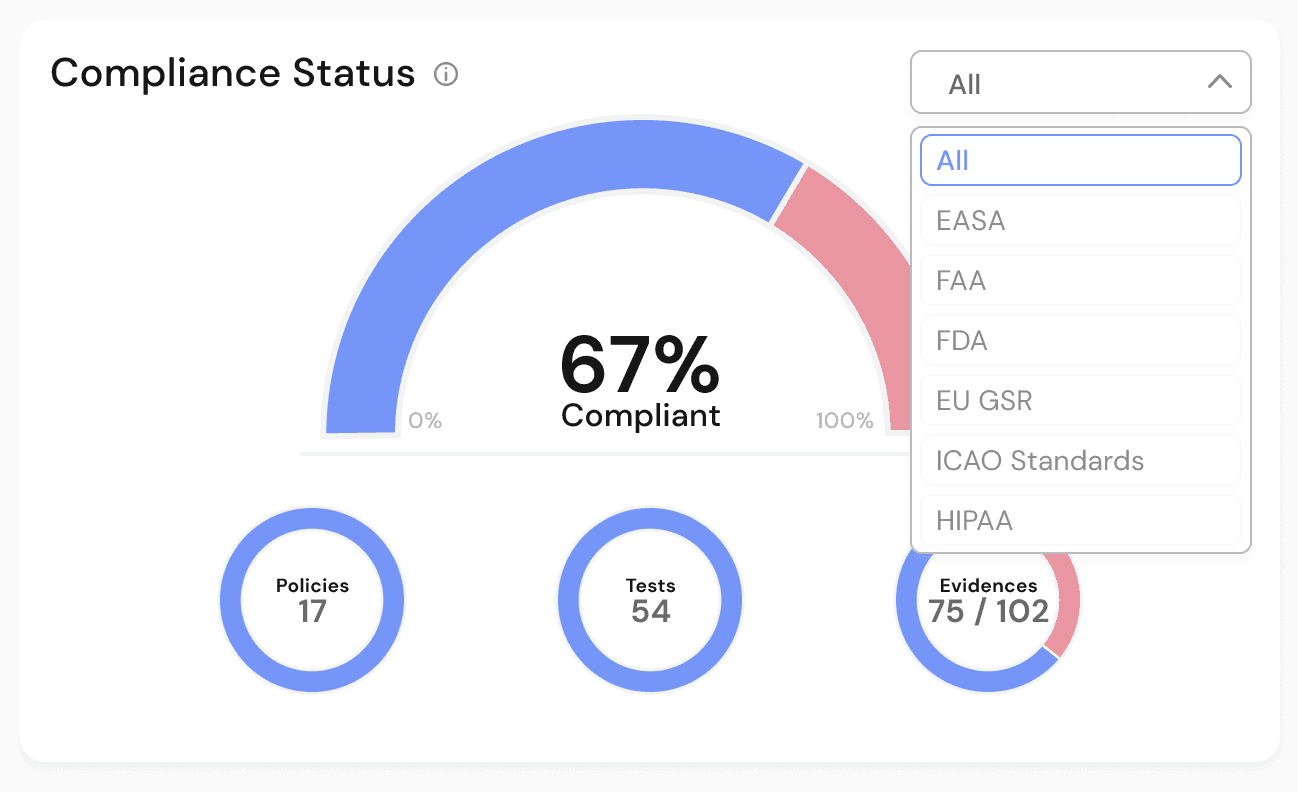
Source: RagaAI
Users can track overall compliance status with global standards put in place by various regulators and policies.

Source: RagaAI
The figure shows a sample of Raga AI framework for mapping various EASA objectives to RagaAI evaluation tests for achieving compliance to the objectives.
The website docs enlist and meticulously present the various tests which have been designed to comply with different aspects of AI regulatory regimes published by Aviation regulators.
Conclusion
This article only covers the AI Compliance landscape in aviation from the lens of EASA. Moreover, it does not get into details of HOW to ensure fulfilment of these vast sets of requirements and obligations. As the aerospace industry is a high-risk sector, the technology deployed at every step needs to be safe and repairable. EASA provides the best first step possible to fundamentally understand all the aspects of AI governance in action. Being highly comprehensive, it can also be extrapolated to other industries with ease.
We know there are tons of questions in your mind after reading this, and unfortunately we cannot cover all ground in this article. But we do give an open invitation to share our expertise with whoever wants to take this ride of AI governance in Aviation.
Get in touch with our Experts.
Want to know more ? Get in touch with our experts!
As we usher into a new era of regulations, i.e., regulations on Artificial Intelligence, it becomes imperative to understand the governance landscape in each industry meticulously. AI is not a new technology and there are a lot of industries which have been using AI/ML, in some form, for more than a decade. Aerospace industry is one of them. Moreover, due to a very low risk-appetite, Aerospace technology has witnessed and compiled with strict regulatory measures drawn over time, as the situation demanded. This article aims at understanding the regulatory landscape in the Aerospace industry, in terms of various governing bodies and acts, requirements , and solutions to those requirements. We aim to talk more about AI Compliance with regulations in this article, which caters to overall AI governance by itself.
What has been done so far ?
When it comes to understanding the regulations drafted or implemented so far, EASA ( European Union Aviation Safety Agency ) and FAA ( Federal Aviation Administration ) are the major governing bodies in the EU and the USA respectively.
While the FAA is still in process to publish any concrete guidelines or restrictions on AI, EASA is way ahead with an extensive AI/ML regulation framework in place.
EASA, under its AI Roadmap, released version 2 of a concept paper establishing the guiding principles for Level 1 and 2 Machine Learning applications. This paper provides a first set of usable objectives in Aviation AI compliance and also serves as a base for formal regulatory developments to come into force. The paper aims to develop principles and guidance which can be integrated into rules and acceptable means of compliance (AMC) later on.
Let’s take a look at the framework.
The guidelines by EASA are so far the most extensive and well-defined set of steps published in any industry for ensuring a safe & ethical AI and regulatory compliance of that AI.

Source: EASA
The figure shows the building blocks for establishing trustworthy AI systems.
AI Trustworthiness Analysis
The AI trustworthiness analysis serves as a gateway to three other technical building blocks by aligning with EU Ethical Guidelines.
It involves characterising the AI application, conducting an ethics-based assessment, and performing safety and security assessments.
These assessments are crucial for the development and approval of AI/ML systems, following existing mandatory practices in industries like aviation.
While safety and security assessments maintain their principles developed originally for software, they require additional guidance to accommodate AI techniques.
AI Assurance
The AI assurance building block is intended to address the AI-specific guidance pertaining to the AIbased system. It encompasses three major topics -
Learning Assurance - It covers the paradigm shift from programming to learning, as the existing development assurance methods are not adapted to cover learning processes specific to AI/ML.

Source: EASA
W-Shaped process for Learning Assurance
Development & post-ops explainability - This deals with the capability to provide users with understandable, reliable and relevant information with the appropriate level of detail on how an AI/ML application produces its results.
Data recording capabilities - This addresses two specific operational and post operational purposes: on the one hand the continuous monitoring of the safety of the AI-based system and on the other hand the support to incident or accident investigation.
Human Factors for AI
This block introduces the necessary guidance to account for the specific human factors needs linked with the introduction of AI.
Among other aspects, AI operational explainability deals with the capability to provide the end users with understandable, reliable and relevant information with the appropriate level of detail and with appropriate timing on how an AI/ML application produces its results. This block also introduces the concept of human-AI teaming to ensure adequate cooperation or collaboration between end users and AI-based systems to achieve certain goals.
AI Safety and Risk Mitigation
This building block considers that we may not always be able to open the ‘AI
black box’ to satisfy the whole set of objectives defined for the AI assurance and the human factors for AI building blocks, and that the associated residual risk may need to be addressed to deal with the inherent uncertainty of AI.
How does RagaAI help ?
As we understand, under the purview of these guidelines, enterprises working on developing AI systems for applications in different aspects of aviation must be able to manage the associated risks well. While the enforcement and adoption of these obligations comes into full effect ( already started ), RagaAI offers an invaluable approach on helping expedite this endeavour. With its comprehensive solutions, RagaAI helps businesses identify, break-down and and comply with the obligations that the regulators propose. These solutions work across all modalities of data.
RagaAI provides comprehensive tests catering to the requirements of the act (laid out objectively), using cutting-edge methods, concrete frameworks and extensive visualisation techniques.
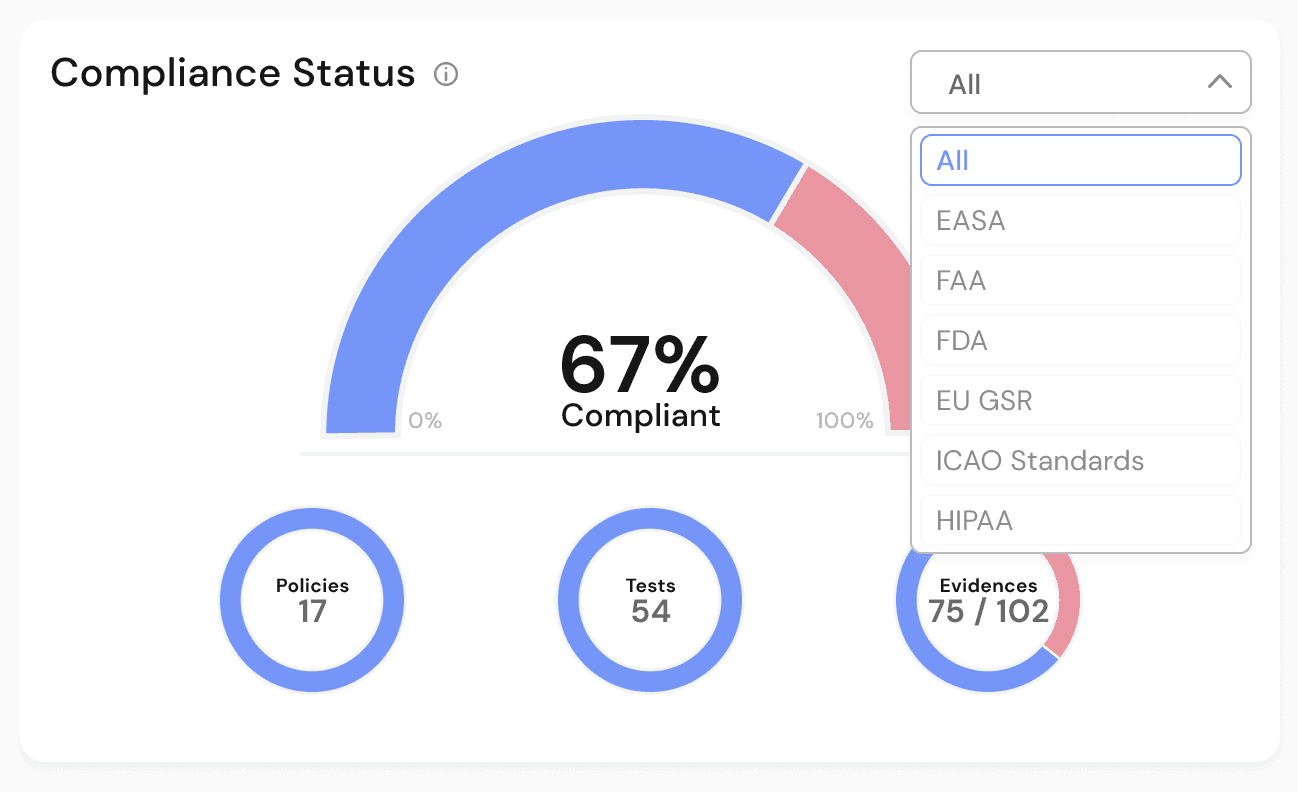
Source: RagaAI
Users can track overall compliance status with global standards put in place by various regulators and policies.

Source: RagaAI
The figure shows a sample of Raga AI framework for mapping various EASA objectives to RagaAI evaluation tests for achieving compliance to the objectives.
The website docs enlist and meticulously present the various tests which have been designed to comply with different aspects of AI regulatory regimes published by Aviation regulators.
Conclusion
This article only covers the AI Compliance landscape in aviation from the lens of EASA. Moreover, it does not get into details of HOW to ensure fulfilment of these vast sets of requirements and obligations. As the aerospace industry is a high-risk sector, the technology deployed at every step needs to be safe and repairable. EASA provides the best first step possible to fundamentally understand all the aspects of AI governance in action. Being highly comprehensive, it can also be extrapolated to other industries with ease.
We know there are tons of questions in your mind after reading this, and unfortunately we cannot cover all ground in this article. But we do give an open invitation to share our expertise with whoever wants to take this ride of AI governance in Aviation.
Get in touch with our Experts.
Want to know more ? Get in touch with our experts!
As we usher into a new era of regulations, i.e., regulations on Artificial Intelligence, it becomes imperative to understand the governance landscape in each industry meticulously. AI is not a new technology and there are a lot of industries which have been using AI/ML, in some form, for more than a decade. Aerospace industry is one of them. Moreover, due to a very low risk-appetite, Aerospace technology has witnessed and compiled with strict regulatory measures drawn over time, as the situation demanded. This article aims at understanding the regulatory landscape in the Aerospace industry, in terms of various governing bodies and acts, requirements , and solutions to those requirements. We aim to talk more about AI Compliance with regulations in this article, which caters to overall AI governance by itself.
What has been done so far ?
When it comes to understanding the regulations drafted or implemented so far, EASA ( European Union Aviation Safety Agency ) and FAA ( Federal Aviation Administration ) are the major governing bodies in the EU and the USA respectively.
While the FAA is still in process to publish any concrete guidelines or restrictions on AI, EASA is way ahead with an extensive AI/ML regulation framework in place.
EASA, under its AI Roadmap, released version 2 of a concept paper establishing the guiding principles for Level 1 and 2 Machine Learning applications. This paper provides a first set of usable objectives in Aviation AI compliance and also serves as a base for formal regulatory developments to come into force. The paper aims to develop principles and guidance which can be integrated into rules and acceptable means of compliance (AMC) later on.
Let’s take a look at the framework.
The guidelines by EASA are so far the most extensive and well-defined set of steps published in any industry for ensuring a safe & ethical AI and regulatory compliance of that AI.

Source: EASA
The figure shows the building blocks for establishing trustworthy AI systems.
AI Trustworthiness Analysis
The AI trustworthiness analysis serves as a gateway to three other technical building blocks by aligning with EU Ethical Guidelines.
It involves characterising the AI application, conducting an ethics-based assessment, and performing safety and security assessments.
These assessments are crucial for the development and approval of AI/ML systems, following existing mandatory practices in industries like aviation.
While safety and security assessments maintain their principles developed originally for software, they require additional guidance to accommodate AI techniques.
AI Assurance
The AI assurance building block is intended to address the AI-specific guidance pertaining to the AIbased system. It encompasses three major topics -
Learning Assurance - It covers the paradigm shift from programming to learning, as the existing development assurance methods are not adapted to cover learning processes specific to AI/ML.

Source: EASA
W-Shaped process for Learning Assurance
Development & post-ops explainability - This deals with the capability to provide users with understandable, reliable and relevant information with the appropriate level of detail on how an AI/ML application produces its results.
Data recording capabilities - This addresses two specific operational and post operational purposes: on the one hand the continuous monitoring of the safety of the AI-based system and on the other hand the support to incident or accident investigation.
Human Factors for AI
This block introduces the necessary guidance to account for the specific human factors needs linked with the introduction of AI.
Among other aspects, AI operational explainability deals with the capability to provide the end users with understandable, reliable and relevant information with the appropriate level of detail and with appropriate timing on how an AI/ML application produces its results. This block also introduces the concept of human-AI teaming to ensure adequate cooperation or collaboration between end users and AI-based systems to achieve certain goals.
AI Safety and Risk Mitigation
This building block considers that we may not always be able to open the ‘AI
black box’ to satisfy the whole set of objectives defined for the AI assurance and the human factors for AI building blocks, and that the associated residual risk may need to be addressed to deal with the inherent uncertainty of AI.
How does RagaAI help ?
As we understand, under the purview of these guidelines, enterprises working on developing AI systems for applications in different aspects of aviation must be able to manage the associated risks well. While the enforcement and adoption of these obligations comes into full effect ( already started ), RagaAI offers an invaluable approach on helping expedite this endeavour. With its comprehensive solutions, RagaAI helps businesses identify, break-down and and comply with the obligations that the regulators propose. These solutions work across all modalities of data.
RagaAI provides comprehensive tests catering to the requirements of the act (laid out objectively), using cutting-edge methods, concrete frameworks and extensive visualisation techniques.
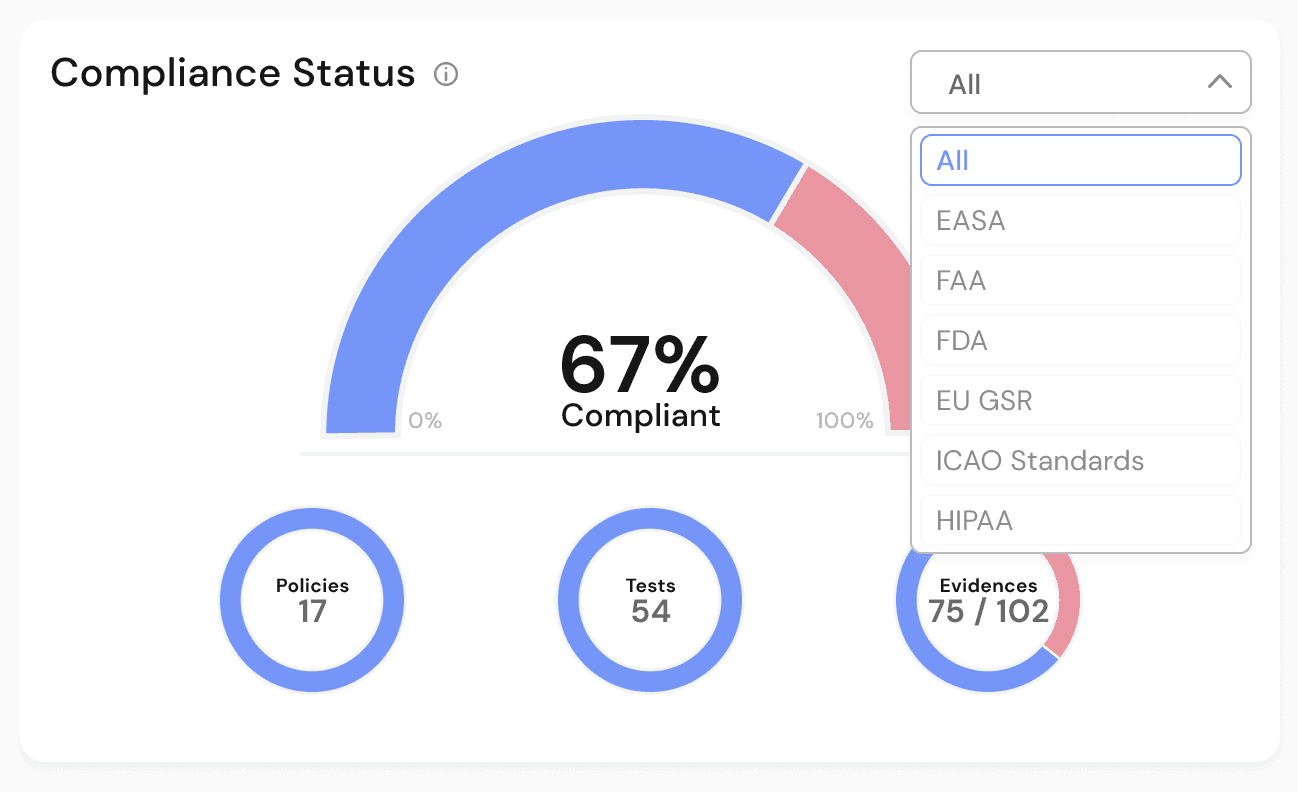
Source: RagaAI
Users can track overall compliance status with global standards put in place by various regulators and policies.

Source: RagaAI
The figure shows a sample of Raga AI framework for mapping various EASA objectives to RagaAI evaluation tests for achieving compliance to the objectives.
The website docs enlist and meticulously present the various tests which have been designed to comply with different aspects of AI regulatory regimes published by Aviation regulators.
Conclusion
This article only covers the AI Compliance landscape in aviation from the lens of EASA. Moreover, it does not get into details of HOW to ensure fulfilment of these vast sets of requirements and obligations. As the aerospace industry is a high-risk sector, the technology deployed at every step needs to be safe and repairable. EASA provides the best first step possible to fundamentally understand all the aspects of AI governance in action. Being highly comprehensive, it can also be extrapolated to other industries with ease.
We know there are tons of questions in your mind after reading this, and unfortunately we cannot cover all ground in this article. But we do give an open invitation to share our expertise with whoever wants to take this ride of AI governance in Aviation.
Get in touch with our Experts.
Want to know more ? Get in touch with our experts!
As we usher into a new era of regulations, i.e., regulations on Artificial Intelligence, it becomes imperative to understand the governance landscape in each industry meticulously. AI is not a new technology and there are a lot of industries which have been using AI/ML, in some form, for more than a decade. Aerospace industry is one of them. Moreover, due to a very low risk-appetite, Aerospace technology has witnessed and compiled with strict regulatory measures drawn over time, as the situation demanded. This article aims at understanding the regulatory landscape in the Aerospace industry, in terms of various governing bodies and acts, requirements , and solutions to those requirements. We aim to talk more about AI Compliance with regulations in this article, which caters to overall AI governance by itself.
What has been done so far ?
When it comes to understanding the regulations drafted or implemented so far, EASA ( European Union Aviation Safety Agency ) and FAA ( Federal Aviation Administration ) are the major governing bodies in the EU and the USA respectively.
While the FAA is still in process to publish any concrete guidelines or restrictions on AI, EASA is way ahead with an extensive AI/ML regulation framework in place.
EASA, under its AI Roadmap, released version 2 of a concept paper establishing the guiding principles for Level 1 and 2 Machine Learning applications. This paper provides a first set of usable objectives in Aviation AI compliance and also serves as a base for formal regulatory developments to come into force. The paper aims to develop principles and guidance which can be integrated into rules and acceptable means of compliance (AMC) later on.
Let’s take a look at the framework.
The guidelines by EASA are so far the most extensive and well-defined set of steps published in any industry for ensuring a safe & ethical AI and regulatory compliance of that AI.

Source: EASA
The figure shows the building blocks for establishing trustworthy AI systems.
AI Trustworthiness Analysis
The AI trustworthiness analysis serves as a gateway to three other technical building blocks by aligning with EU Ethical Guidelines.
It involves characterising the AI application, conducting an ethics-based assessment, and performing safety and security assessments.
These assessments are crucial for the development and approval of AI/ML systems, following existing mandatory practices in industries like aviation.
While safety and security assessments maintain their principles developed originally for software, they require additional guidance to accommodate AI techniques.
AI Assurance
The AI assurance building block is intended to address the AI-specific guidance pertaining to the AIbased system. It encompasses three major topics -
Learning Assurance - It covers the paradigm shift from programming to learning, as the existing development assurance methods are not adapted to cover learning processes specific to AI/ML.

Source: EASA
W-Shaped process for Learning Assurance
Development & post-ops explainability - This deals with the capability to provide users with understandable, reliable and relevant information with the appropriate level of detail on how an AI/ML application produces its results.
Data recording capabilities - This addresses two specific operational and post operational purposes: on the one hand the continuous monitoring of the safety of the AI-based system and on the other hand the support to incident or accident investigation.
Human Factors for AI
This block introduces the necessary guidance to account for the specific human factors needs linked with the introduction of AI.
Among other aspects, AI operational explainability deals with the capability to provide the end users with understandable, reliable and relevant information with the appropriate level of detail and with appropriate timing on how an AI/ML application produces its results. This block also introduces the concept of human-AI teaming to ensure adequate cooperation or collaboration between end users and AI-based systems to achieve certain goals.
AI Safety and Risk Mitigation
This building block considers that we may not always be able to open the ‘AI
black box’ to satisfy the whole set of objectives defined for the AI assurance and the human factors for AI building blocks, and that the associated residual risk may need to be addressed to deal with the inherent uncertainty of AI.
How does RagaAI help ?
As we understand, under the purview of these guidelines, enterprises working on developing AI systems for applications in different aspects of aviation must be able to manage the associated risks well. While the enforcement and adoption of these obligations comes into full effect ( already started ), RagaAI offers an invaluable approach on helping expedite this endeavour. With its comprehensive solutions, RagaAI helps businesses identify, break-down and and comply with the obligations that the regulators propose. These solutions work across all modalities of data.
RagaAI provides comprehensive tests catering to the requirements of the act (laid out objectively), using cutting-edge methods, concrete frameworks and extensive visualisation techniques.
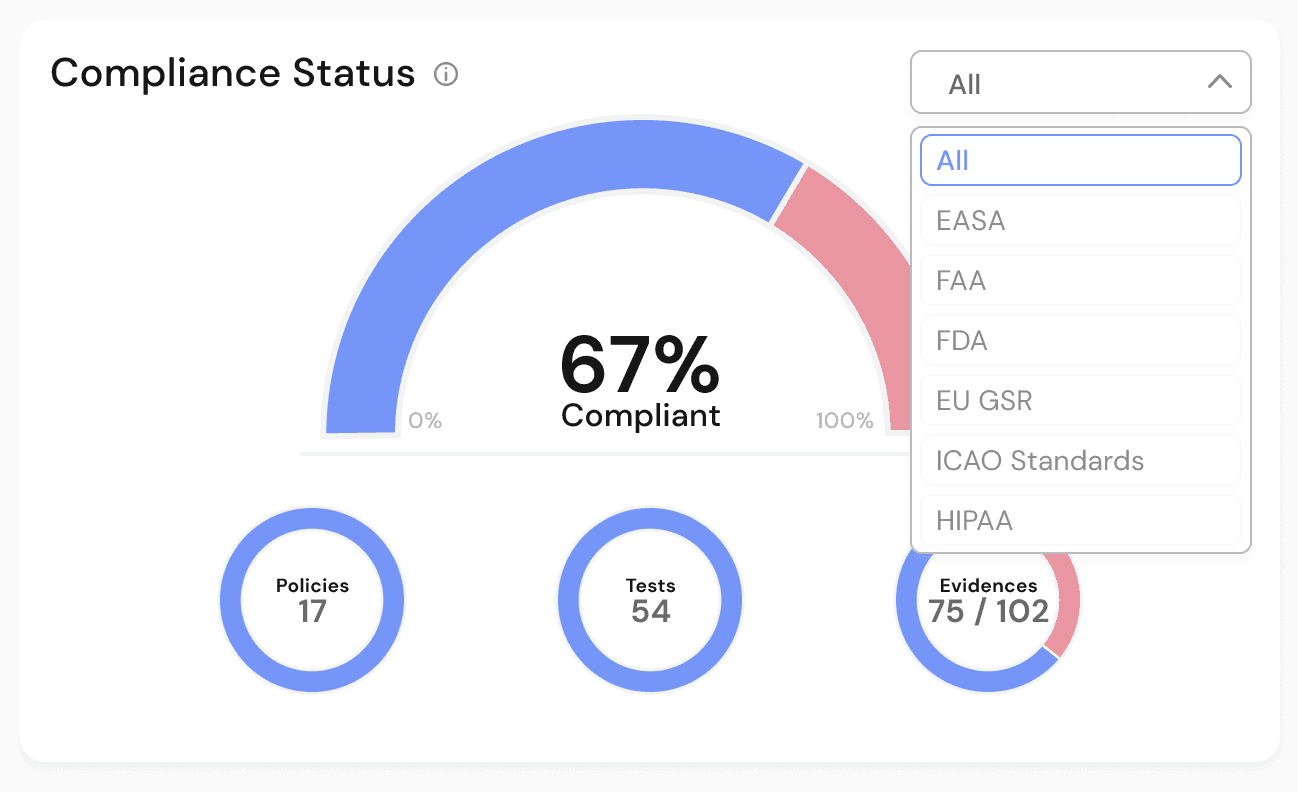
Source: RagaAI
Users can track overall compliance status with global standards put in place by various regulators and policies.

Source: RagaAI
The figure shows a sample of Raga AI framework for mapping various EASA objectives to RagaAI evaluation tests for achieving compliance to the objectives.
The website docs enlist and meticulously present the various tests which have been designed to comply with different aspects of AI regulatory regimes published by Aviation regulators.
Conclusion
This article only covers the AI Compliance landscape in aviation from the lens of EASA. Moreover, it does not get into details of HOW to ensure fulfilment of these vast sets of requirements and obligations. As the aerospace industry is a high-risk sector, the technology deployed at every step needs to be safe and repairable. EASA provides the best first step possible to fundamentally understand all the aspects of AI governance in action. Being highly comprehensive, it can also be extrapolated to other industries with ease.
We know there are tons of questions in your mind after reading this, and unfortunately we cannot cover all ground in this article. But we do give an open invitation to share our expertise with whoever wants to take this ride of AI governance in Aviation.
Get in touch with our Experts.
Want to know more ? Get in touch with our experts!
As we usher into a new era of regulations, i.e., regulations on Artificial Intelligence, it becomes imperative to understand the governance landscape in each industry meticulously. AI is not a new technology and there are a lot of industries which have been using AI/ML, in some form, for more than a decade. Aerospace industry is one of them. Moreover, due to a very low risk-appetite, Aerospace technology has witnessed and compiled with strict regulatory measures drawn over time, as the situation demanded. This article aims at understanding the regulatory landscape in the Aerospace industry, in terms of various governing bodies and acts, requirements , and solutions to those requirements. We aim to talk more about AI Compliance with regulations in this article, which caters to overall AI governance by itself.
What has been done so far ?
When it comes to understanding the regulations drafted or implemented so far, EASA ( European Union Aviation Safety Agency ) and FAA ( Federal Aviation Administration ) are the major governing bodies in the EU and the USA respectively.
While the FAA is still in process to publish any concrete guidelines or restrictions on AI, EASA is way ahead with an extensive AI/ML regulation framework in place.
EASA, under its AI Roadmap, released version 2 of a concept paper establishing the guiding principles for Level 1 and 2 Machine Learning applications. This paper provides a first set of usable objectives in Aviation AI compliance and also serves as a base for formal regulatory developments to come into force. The paper aims to develop principles and guidance which can be integrated into rules and acceptable means of compliance (AMC) later on.
Let’s take a look at the framework.
The guidelines by EASA are so far the most extensive and well-defined set of steps published in any industry for ensuring a safe & ethical AI and regulatory compliance of that AI.

Source: EASA
The figure shows the building blocks for establishing trustworthy AI systems.
AI Trustworthiness Analysis
The AI trustworthiness analysis serves as a gateway to three other technical building blocks by aligning with EU Ethical Guidelines.
It involves characterising the AI application, conducting an ethics-based assessment, and performing safety and security assessments.
These assessments are crucial for the development and approval of AI/ML systems, following existing mandatory practices in industries like aviation.
While safety and security assessments maintain their principles developed originally for software, they require additional guidance to accommodate AI techniques.
AI Assurance
The AI assurance building block is intended to address the AI-specific guidance pertaining to the AIbased system. It encompasses three major topics -
Learning Assurance - It covers the paradigm shift from programming to learning, as the existing development assurance methods are not adapted to cover learning processes specific to AI/ML.

Source: EASA
W-Shaped process for Learning Assurance
Development & post-ops explainability - This deals with the capability to provide users with understandable, reliable and relevant information with the appropriate level of detail on how an AI/ML application produces its results.
Data recording capabilities - This addresses two specific operational and post operational purposes: on the one hand the continuous monitoring of the safety of the AI-based system and on the other hand the support to incident or accident investigation.
Human Factors for AI
This block introduces the necessary guidance to account for the specific human factors needs linked with the introduction of AI.
Among other aspects, AI operational explainability deals with the capability to provide the end users with understandable, reliable and relevant information with the appropriate level of detail and with appropriate timing on how an AI/ML application produces its results. This block also introduces the concept of human-AI teaming to ensure adequate cooperation or collaboration between end users and AI-based systems to achieve certain goals.
AI Safety and Risk Mitigation
This building block considers that we may not always be able to open the ‘AI
black box’ to satisfy the whole set of objectives defined for the AI assurance and the human factors for AI building blocks, and that the associated residual risk may need to be addressed to deal with the inherent uncertainty of AI.
How does RagaAI help ?
As we understand, under the purview of these guidelines, enterprises working on developing AI systems for applications in different aspects of aviation must be able to manage the associated risks well. While the enforcement and adoption of these obligations comes into full effect ( already started ), RagaAI offers an invaluable approach on helping expedite this endeavour. With its comprehensive solutions, RagaAI helps businesses identify, break-down and and comply with the obligations that the regulators propose. These solutions work across all modalities of data.
RagaAI provides comprehensive tests catering to the requirements of the act (laid out objectively), using cutting-edge methods, concrete frameworks and extensive visualisation techniques.
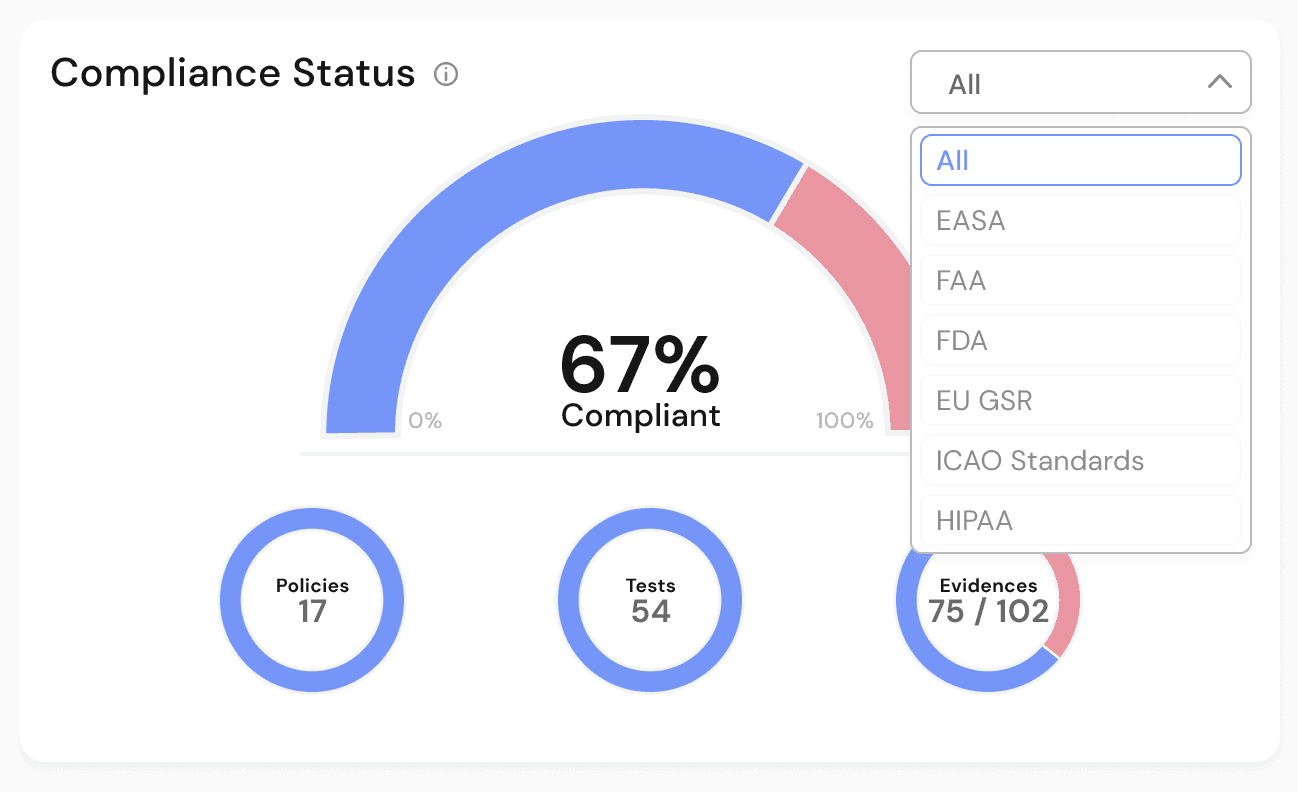
Source: RagaAI
Users can track overall compliance status with global standards put in place by various regulators and policies.

Source: RagaAI
The figure shows a sample of Raga AI framework for mapping various EASA objectives to RagaAI evaluation tests for achieving compliance to the objectives.
The website docs enlist and meticulously present the various tests which have been designed to comply with different aspects of AI regulatory regimes published by Aviation regulators.
Conclusion
This article only covers the AI Compliance landscape in aviation from the lens of EASA. Moreover, it does not get into details of HOW to ensure fulfilment of these vast sets of requirements and obligations. As the aerospace industry is a high-risk sector, the technology deployed at every step needs to be safe and repairable. EASA provides the best first step possible to fundamentally understand all the aspects of AI governance in action. Being highly comprehensive, it can also be extrapolated to other industries with ease.
We know there are tons of questions in your mind after reading this, and unfortunately we cannot cover all ground in this article. But we do give an open invitation to share our expertise with whoever wants to take this ride of AI governance in Aviation.
Get in touch with our Experts.
Want to know more ? Get in touch with our experts!