Practical Retrieval Augmented Generation: Use Cases And Impact
Jigar Gupta
Jun 24, 2024




Ever wondered if your AI assistant could fetch real-time data while still making clever quips? Welcome to the world of Retrieval-Augmented Generation (RAG) where your wish is its command!
Envision a world where AI systems can attain and incorporate real-time data from the web to offer you up-to-date, precise and contextually pertinent details. This is the power of Retrieval-Augmented Generation (RAG), an innovative approach transforming the abilities of language models.
By smoothly merging data retrieval with context-aware refining, RAG ensures that large language models (LLMs) are not just producing text from stagnant training data but are dynamically pulling in current, dependable facts from external databases.
This innovation clarifies data sources and alleviates concerns about bias in LLM yields, making it a groundbreaker in numerous fields. Let’s dive into what makes RAG so important and explore its disparate use cases.
Want to get a detailed analysis on distinct LLMs, check out our, “Comparing Different Large Language Models (LLMs)” article.
The Basics of Retrieval Augmented Generation
How RAG Works: Merging Dynamic Retrieval with Context-Aware Processing
Envisage a system that can retrieve the most pertinent data from a vast database and produce context-aware, coherent responses in real-time.
That’s Retrieval-Augmented Generation (RAG) in action. It merges 2 strong AI capabilities: dynamic information retrieval and context-aware processing. When you ask a question, RAG first retrieves relevant information from a knowledge base.
Then, it uses this data to produce a response that is both precise and contextually pertinent. This dual-step procedure ensures that the answers you get are not only pertinent but also pragmatic.
Distinction Between Two Types of RAG Models
There are two predominant RAG models: RAG-Token and RAG Sequence.
RAG-Token: This model recovers details at the token level. The model produces each word (token) of the response by actively pulling pertinent pieces of information. This approach permits highly correct and precise responses, as each token is informed by the latest information.
RAG-Sequence: Unlike RAG-Token, the RAG-Sequence model recovers information for the whole sequence of text before producing the responses. This technique ensures that the response is coherent and contextually congruous, as the entire sequence is based on a pragmatic comprehension of the recovered data.
Key Components of RAG
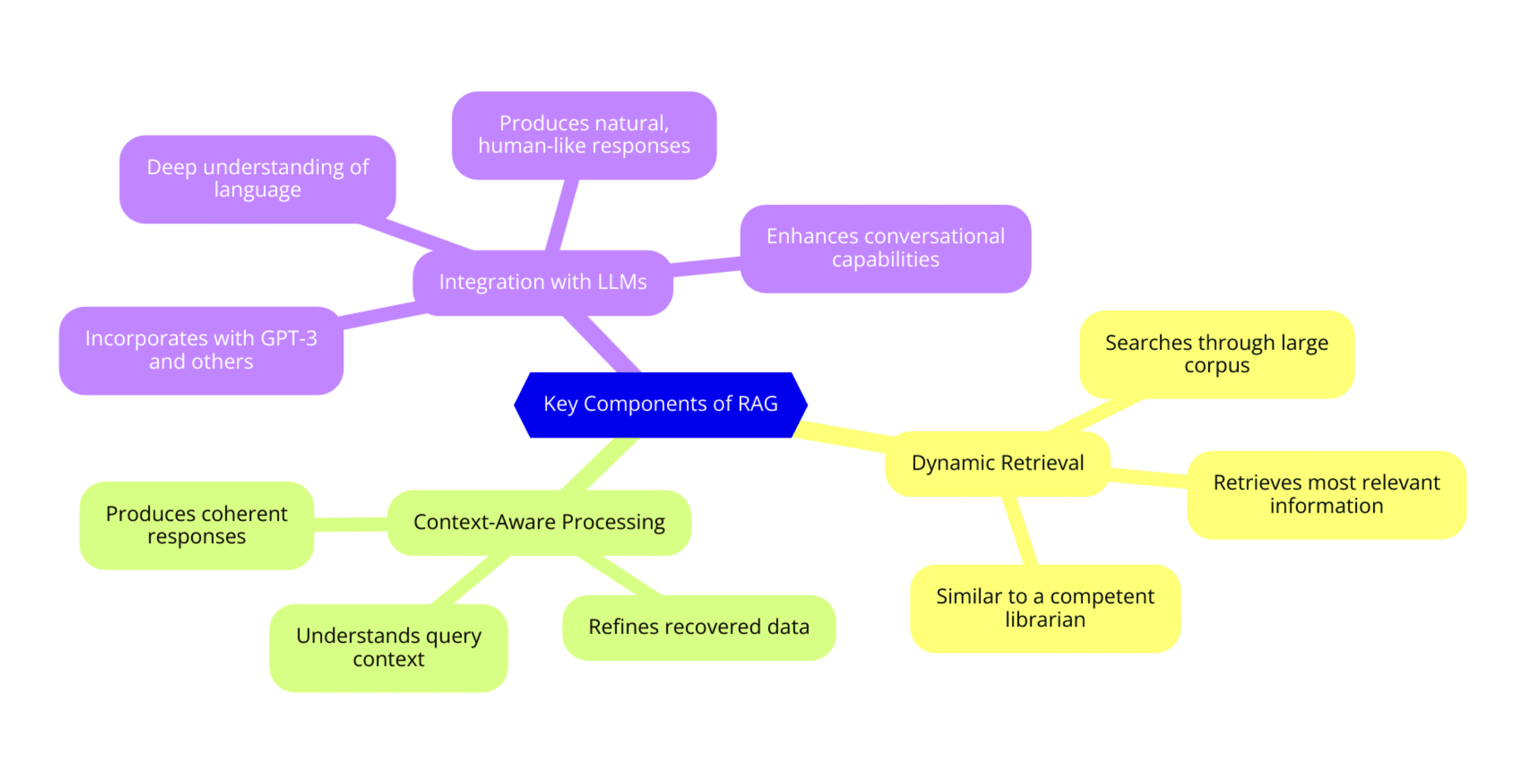
To entirely learn how RAG works, let’s break down its key components:
Dynamic Retrieval: This is the initial step, where the system searches through a huge corpus of information to retrieve the most relevant information. It resembles a competent librarian promptly locating the exact book or documents you need.
Context-Aware Processing: Once the pertinent data is recovered, the system refines it in a way that comprehends the context of your query. It ensures that the produced response is not just an arbitrarily collection of facts but a coherent and contextually apt response.
Integration with Large Language Models (LLMs): RAG incorporates smoothly with LLMs such as GPT-3. These models have a deep comprehension of language, enabling them to produce natural, human-like responses. The incorporation with LLMs permits RAG to use their conversational capabilities, improving the overall quality of the produced content.
Path of Development Since Its Creation by Meta Research in 2020
RAG’s expedition began in 2020, established by Meta Research (formerly Facebook AI). Since then, it has developed substantially. Initially, Meta Research designed RAG to improve the precision and pertinence of AI-produced responses, and it has since undergone numerous iterations to enhance its effectiveness and reliability.
Early versions concentrated on fine-tuning the dynamic recovery procedure, ensuring that the system could retrieve the most relevant information rapidly. Subsequent evolutions aimed to improve the context-aware refining abilities, making the responses more coherent and contextually felicitous.
Today, RAG stands as a sturdy structure that incorporates smoothly with LLMs, providing exceptional precision and pertinence in data retrieval and generation.
As you traverse the potential of RAG, you will find its applications in several fields, from customer assistance and education to investigation and content creation. Its capability to merge real-time data retrieval with advanced language generation makes it a groundbreaker in the AI world.
Now that we have a firm grasp on how RAG functions, let's dive into some real-world applications that showcase its true impact.
Practical Use Cases of RAG (Retrieval-Augmented Generation)
Document Question Answering Systems: Enhancing Access to Proprietary Documents
Envisage having an enormous base of proprietary documents and requiring precise details from them swiftly. Document Question Answering System, powered by RAG, can transform this process. By asking queries in natural language, you can promptly recover specific responses from your documents, saving time and enhancing effectiveness.
Conversational Agents: Customizing LLMs to Specific Guidelines or Manuals
Conversational agents can become even more efficient when customized to precise instructions or manuals. With RAG, you can tailor language models to adhere to concrete conventions and industry standards. This ensures that the AI interacts with precision while complying with specific needs.
Real-time Event Commentary with Live Data and LLMs
For live events, giving real-time commentary is critical. RAG can connect language models to live data feeds, permitting you to produce up-to-minute reports that improve the virtual experience. Whether it’s a sports game, a meeting, or a breaking news story, RAG keeps your audience engaged with the newest updates.
Content Generation: Personalizing Content and Ensuring Contextual Relevance
Generating customized content that reverberates with your audience can be challenging. RAG helps by using real-time data to create content that is not only pertinent but also gradually customized. This ensures that your readers find your content appealing and valuable, elevating your content’s efficiency.
Personalized Recommendation: Evolving Content Recommendations through LLMs
RAG can revolutionize how you provide customized suggestions. By incorporating retrieval mechanisms and language models, you can offer suggestions that develop based on user interactions and choices. This dynamic approach ensures that your suggestions remain pertinent and customized over time.
Virtual Assistants: Creating More Personalized User Experiences
Virtual Assistants equipped with RAG abilities can provide gradually customized user experiences. They can recover pertinent details and produce answers that serve specifically to the user’s requirement and context. This makes interactions more relevant and improves user contentment.
Customer Support Chatbots: Providing Up-to-date and Accurate Responses
Customer support chatbots need to deliver precise and prompt responses. With RAG, your chatbots can attain the most latest information, ensuring they give dependable and up-to-date details. This enhances customer service standards and decreases answering duration.
Business Intelligence and Analysis: Delivering Domain-specific, Relevant Insights
In the scenario of Business Intelligence, RAG can be a groundbreaker. By delivering domain-specific perceptions, RAG enables you to make informed decisions based on the newest and most pertinent information. This improves your inquisitive abilities and helps you stay ahead in your industry.
Healthcare Information Systems: Accessing Medical Research and Patient Data for Better Care
Healthcare professionals can take advantage of RAG by attaining medical investigation and patient records efficiently. RAG permits for swift recovery of relevant details, helping in better curing and treatment plans, eventually enhancing patient care.
Legal Research and Compliance: Assisting in the Analysis of Legal Documents and Regulatory Compliance
Legitimate professionals can use RAG to sleek the inspection of legitimate documents and ensure regulatory compliance. By recovering and producing pertinent legitimate data, RAG helps in comprehensive investigation and compliance checks, making legitimate processes more effective and precise.
And that's not all—RAG's utility is expanding into even more advanced, specialized areas. Check out some next-level use cases.
Advanced RAG Use Cases
Gaining Insights from Sales Rep Feedback
Imagine turning your sales representative’ remarks into gold mines of applicable insights. You can use Retrieval-Augmented Generation (RAG) to dissect sales feedback. By involuntarily classifying and amalgamating responses, you can pinpoint trends, common problems and opportunities.
This permits you to cautiously acknowledge concerns, customize your approach to customer requirements, and eventually drive better customer success results. It’s like having a 24/7 annotator that turns every piece of response into planned insights.
Medical Insights Miner: Enhancing Research with Real-Time PubMed Data
Stay ahead in medical investigation by pounding into real-time information from PubMed using RAG. This tool permits you to constantly observe and extract pertinent research discoveries, keeping you updated with the newest evolutions.
By incorporating these perceptions into your research process, you can improve the quality and promptness of your studies. This approach boosts discovery, helps in pinpointing emerging trends, and ensures that your work stays at the cutting edge of medical science.
L1/L2 Customer Support Assistant: Improving Customer Support Experiences
Elevate your customer support experience by using RAG to assist your L1 and L2 support teams. This tool can rapidly recover and present pertinent solutions from a wide knowledge base, ensuring that your support agents always have the correct data at their fingertips. By doing so, you can decrease response duration, increase solution rates, and improve overall customer contentment. It’s like giving your support team a significant support that never sleeps and always has the answers.
Compliance in Customer Contact Centers: Ensuring Behavior Analysis in Regulated Industries
Ensure your customer centers follow regulatory requirements using RAG. This tool can dissect interactions for compliance, discerning any divergence required conventions.
By giving real-time responses and recommendations, you can acknowledge problems instantly, ensuring that your functioning remains within the bounds of industry regulations. This proactive approach not only helps in sustaining compliance but also builds trust with your customers and investors.
Employee Knowledge Training Assessment: Enhancing Training Effectiveness Across Roles
Revolutionize your employee training programs with RAG. By inspecting training materials and employee responses, you can pinpoint gaps in knowledge and areas for enhancement.
This tool helps in customizing training sessions to acknowledge precise requirements, ensuring that employees across all roles receive the most efficient and pertinent training. By constantly evaluating and processing your training programs, you can elevate workflow, improve expertise, and ensure that your employees are always prepared to meet new challenges.
Global SOP Standardization: Analyzing and Improving Standard Operating Procedures
Sleek your worldwide operations by homogenizing your Standard Operating Procedures (SOPs) with RAG. This tool can dissect SOPs from distinct regions, dissect inconsistencies, and recommend enhancements.
By ensuring that all your SOPs are aligned and upgraded, you can improve functioning effectiveness, reduce errors, and ensure congruous quality across your organization. It’s like having a universal process examiner that ensures every process is up to par.
Operations Support Assistant in Manufacturing: Assisting Technical Productivity with Complex Machinery Maintenance
Improve your manufacturing operations with an RAG-powered support assistant. This tool can aid in sustaining intricate machinery by offering real-time troubleshooting and preserving data.
By rapidly recovering and presenting pertinent technical data, you can reduce interruption, enhance workflow, and lengthen the lifespan of your equipment. This approach ensures that your technical workforce always has the details they need to keep your operations running sleekly.
Of course, implementing RAG comes with its own set of best practices and considerations, and we'll explore those next.
Implementing RAG - Best Practices and Considerations
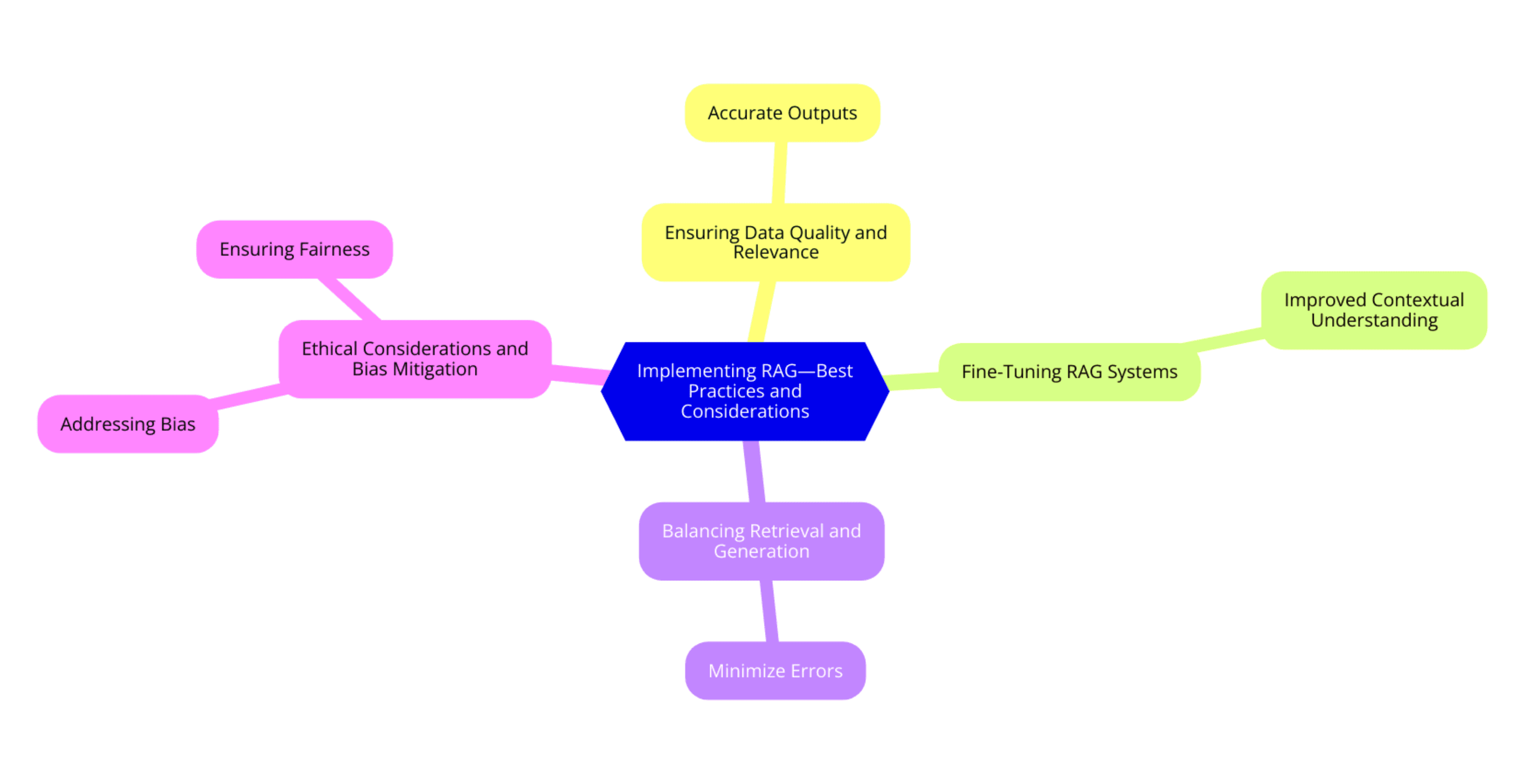
Ensuring Data Quality and Relevance for Accurate Outputs
When enforcing RAG (Retrieval-Augmented Generation), data quality and pertinence are your top priorities. You require to begin by consolidating a high-quality data set that is relevant to your domain.
This means using dependable sources, updating your data frequently, and removing any outdated or irrelevant data. High-quality information ensures that your RAG model recovers the most precise and helpful information, which results in more accurate and dependable yields.
Fine-Tuning RAG Systems for Improved Contextual Understanding
To get the most out of your RAG system, refining is important. This involves instructing your model on domain-specific data so it can comprehend the variations and context of your queries better. Use methods such as supervised refining with interpreted information to help your model grasp the correct answers.
This step is critical for improving the contextual comprehension of your system, making its yields more pertinent and precise.
Balancing Retrieval and Generation to Minimize Errors
Locating the right balance between retrieval and generation is key to minimizing mistakes and hallucinations. Too much dependency on generation can result in fabricated data, while over-dependence on recovery can restrict the creativity and profundity of the responses. You should adapt the emphasis of recovery and generation elements based on the nature of your application. Frequently assess the yields and modify the system to ensure it gives illuminating and dependable responses.
Ethical Considerations and Bias Mitigation in RAG Implementations
Ethics and bias mitigation should be essential to your RAG enforcement procedure. Begin by inspecting your dataset for biases and ensuring a disparate range of sources. Enforcing fairness-aware algorithms can help in lessening bias in the retrieval process. In addition, it’s important to sustain clarity with users about how your system operates and the sources it uses. By concentrating on ethical contemplations, you can build trust and ensure that your RAG system provides impartial and fair data.
So, what does the future hold for RAG? Let's explore the exciting advancements and potential impacts on various industries.
Future Directions and Impact of RAG
Exploration of Multimodal Capabilities and API Access
Envisage a globe where your applications can refine not just text, but pictures, audio and video smoothly. That’s where the probing of multimodal abilities in RAG (Retrieval-Augmented Generation) is heading. By incorporating numerous data types, you improve the opulence and context of data your application can handle.
Connected with API access, you can pull real-time information from disparate sources, making your solutions more robust and receptive. This means more reciprocal and captivating user experience, where your app can describe an image, condense a video, or even communicate through voice.
Broader Applications and Enhanced User Experiences
RAGs potential isn’t restricted to a single field. You can apply it across education, finance, healthcare and beyond. Picture an informational platform that not only answers student queries but also gives comprehensive elucidations, suggests further reading, and even interrogates students based on their learning growth. In healthcare, RAG can assist doctors by recovering the latest investigation and patient record, helping them make informed decisions.
The key is the enriched user experience, where communications feel more natural and private, making technology an inherent augmentation of human abilities.
Data Integration and Innovations in Multimodal Models
Incorporating distinct types of data such as text, images, and standardized data opens new frontiers in RAG applications. This incorporation permits for more sophisticated data refining and insights generation. Innovations in multimodal models mean you can evolve applications that comprehend and produce content across multiple formats.
For example, a customer service bot could dissect a customer’s voice tone and the content of their texts to offer more compassionate and precise answers. Such innovations drive forward a more associated and perceptive digital ecosystem.
LangChain and LLM RAG: Generative Search, Data Chat, and Next-Gen Customer Service
LanChain and large language model (LLM) RAG applications are revolutionizing how you communicate with data. With generative search, your doubts return not just pertinent documents, but integrated, brief responses drawn from numerous sources.
Chatting with data means engaging in a conversation with your datasets, asking intricate questions and getting comprehensible, applicable perceptions. In customer service, next-generation applications can handle more complex queries, giving prompt, precise support and freeing up human agents for more intricate tasks. This shift towards savvy, more receptive systems marks a substantial leap in how we use the power of AI to enhance daily communications and productivity.
By clasping these future guidance, you can use RAG to create more smart, receptive and flexible applications, pushing the boundaries of what’s possible in technology today.
To cap things off, let’s look at how you can seamlessly integrate these advanced RAG platforms into your existing systems to elevate productivity and effectiveness.
Conclusion
Retrieval-Augmented Generation is a revolutionary technology assured to transform numerous industries. By using real-time information and context-aware refining, RAG ensures that AI systems deliver the most precise and pertinent information, paving the way for innovative applications and improved user experiences.
Whether it’s healthcare, legitimate research, or customer support, RAG is set to make a substantial impact, driving effectiveness and precision across disparate domains.
Explore how you can smoothly integrate advanced RAG platforms into your current enterprise systems for improved effectiveness and productivity in our thorough guide on Integration Of RAG Platforms With Existing Enterprise Systems.
Ever wondered if your AI assistant could fetch real-time data while still making clever quips? Welcome to the world of Retrieval-Augmented Generation (RAG) where your wish is its command!
Envision a world where AI systems can attain and incorporate real-time data from the web to offer you up-to-date, precise and contextually pertinent details. This is the power of Retrieval-Augmented Generation (RAG), an innovative approach transforming the abilities of language models.
By smoothly merging data retrieval with context-aware refining, RAG ensures that large language models (LLMs) are not just producing text from stagnant training data but are dynamically pulling in current, dependable facts from external databases.
This innovation clarifies data sources and alleviates concerns about bias in LLM yields, making it a groundbreaker in numerous fields. Let’s dive into what makes RAG so important and explore its disparate use cases.
Want to get a detailed analysis on distinct LLMs, check out our, “Comparing Different Large Language Models (LLMs)” article.
The Basics of Retrieval Augmented Generation
How RAG Works: Merging Dynamic Retrieval with Context-Aware Processing
Envisage a system that can retrieve the most pertinent data from a vast database and produce context-aware, coherent responses in real-time.
That’s Retrieval-Augmented Generation (RAG) in action. It merges 2 strong AI capabilities: dynamic information retrieval and context-aware processing. When you ask a question, RAG first retrieves relevant information from a knowledge base.
Then, it uses this data to produce a response that is both precise and contextually pertinent. This dual-step procedure ensures that the answers you get are not only pertinent but also pragmatic.
Distinction Between Two Types of RAG Models
There are two predominant RAG models: RAG-Token and RAG Sequence.
RAG-Token: This model recovers details at the token level. The model produces each word (token) of the response by actively pulling pertinent pieces of information. This approach permits highly correct and precise responses, as each token is informed by the latest information.
RAG-Sequence: Unlike RAG-Token, the RAG-Sequence model recovers information for the whole sequence of text before producing the responses. This technique ensures that the response is coherent and contextually congruous, as the entire sequence is based on a pragmatic comprehension of the recovered data.
Key Components of RAG
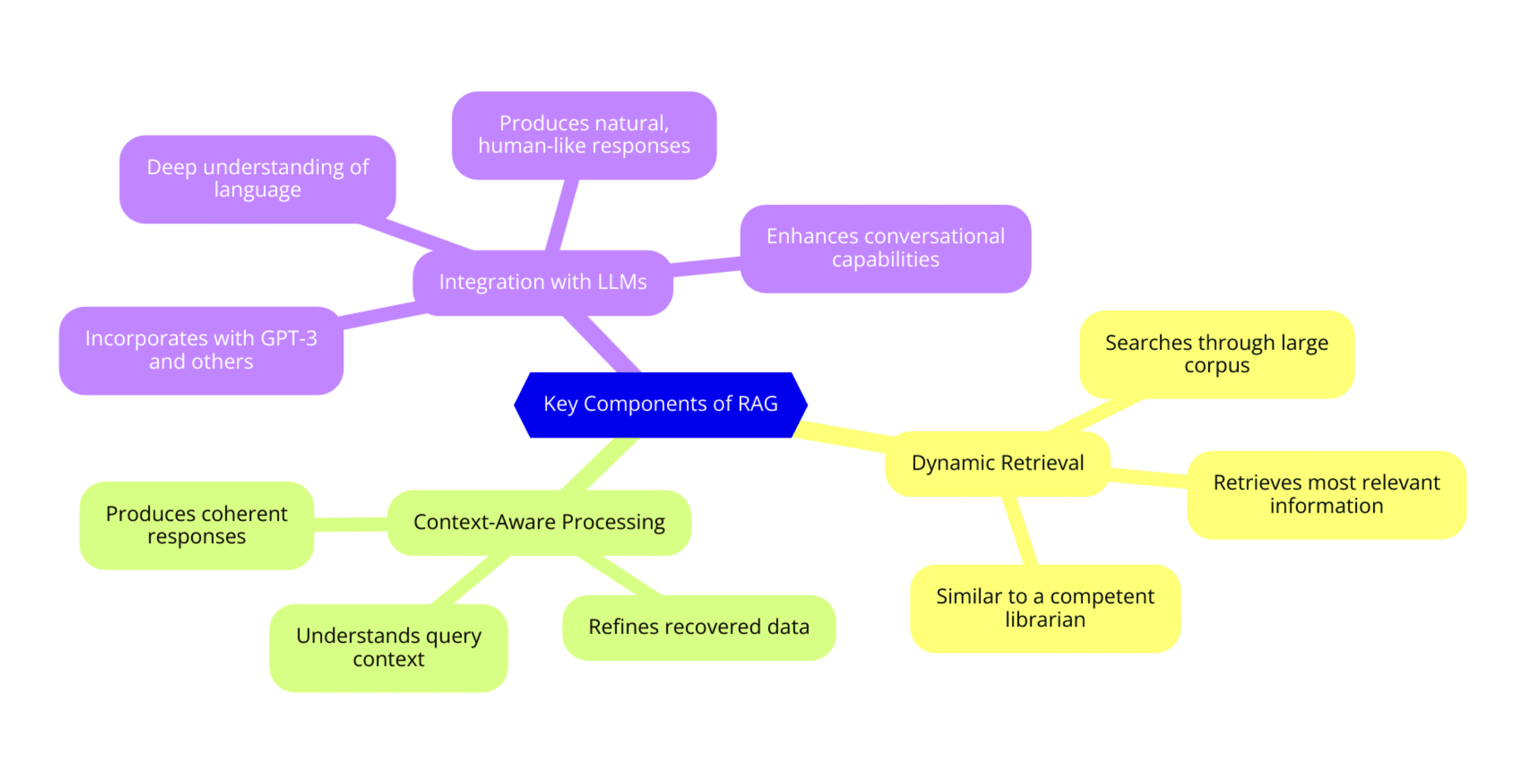
To entirely learn how RAG works, let’s break down its key components:
Dynamic Retrieval: This is the initial step, where the system searches through a huge corpus of information to retrieve the most relevant information. It resembles a competent librarian promptly locating the exact book or documents you need.
Context-Aware Processing: Once the pertinent data is recovered, the system refines it in a way that comprehends the context of your query. It ensures that the produced response is not just an arbitrarily collection of facts but a coherent and contextually apt response.
Integration with Large Language Models (LLMs): RAG incorporates smoothly with LLMs such as GPT-3. These models have a deep comprehension of language, enabling them to produce natural, human-like responses. The incorporation with LLMs permits RAG to use their conversational capabilities, improving the overall quality of the produced content.
Path of Development Since Its Creation by Meta Research in 2020
RAG’s expedition began in 2020, established by Meta Research (formerly Facebook AI). Since then, it has developed substantially. Initially, Meta Research designed RAG to improve the precision and pertinence of AI-produced responses, and it has since undergone numerous iterations to enhance its effectiveness and reliability.
Early versions concentrated on fine-tuning the dynamic recovery procedure, ensuring that the system could retrieve the most relevant information rapidly. Subsequent evolutions aimed to improve the context-aware refining abilities, making the responses more coherent and contextually felicitous.
Today, RAG stands as a sturdy structure that incorporates smoothly with LLMs, providing exceptional precision and pertinence in data retrieval and generation.
As you traverse the potential of RAG, you will find its applications in several fields, from customer assistance and education to investigation and content creation. Its capability to merge real-time data retrieval with advanced language generation makes it a groundbreaker in the AI world.
Now that we have a firm grasp on how RAG functions, let's dive into some real-world applications that showcase its true impact.
Practical Use Cases of RAG (Retrieval-Augmented Generation)
Document Question Answering Systems: Enhancing Access to Proprietary Documents
Envisage having an enormous base of proprietary documents and requiring precise details from them swiftly. Document Question Answering System, powered by RAG, can transform this process. By asking queries in natural language, you can promptly recover specific responses from your documents, saving time and enhancing effectiveness.
Conversational Agents: Customizing LLMs to Specific Guidelines or Manuals
Conversational agents can become even more efficient when customized to precise instructions or manuals. With RAG, you can tailor language models to adhere to concrete conventions and industry standards. This ensures that the AI interacts with precision while complying with specific needs.
Real-time Event Commentary with Live Data and LLMs
For live events, giving real-time commentary is critical. RAG can connect language models to live data feeds, permitting you to produce up-to-minute reports that improve the virtual experience. Whether it’s a sports game, a meeting, or a breaking news story, RAG keeps your audience engaged with the newest updates.
Content Generation: Personalizing Content and Ensuring Contextual Relevance
Generating customized content that reverberates with your audience can be challenging. RAG helps by using real-time data to create content that is not only pertinent but also gradually customized. This ensures that your readers find your content appealing and valuable, elevating your content’s efficiency.
Personalized Recommendation: Evolving Content Recommendations through LLMs
RAG can revolutionize how you provide customized suggestions. By incorporating retrieval mechanisms and language models, you can offer suggestions that develop based on user interactions and choices. This dynamic approach ensures that your suggestions remain pertinent and customized over time.
Virtual Assistants: Creating More Personalized User Experiences
Virtual Assistants equipped with RAG abilities can provide gradually customized user experiences. They can recover pertinent details and produce answers that serve specifically to the user’s requirement and context. This makes interactions more relevant and improves user contentment.
Customer Support Chatbots: Providing Up-to-date and Accurate Responses
Customer support chatbots need to deliver precise and prompt responses. With RAG, your chatbots can attain the most latest information, ensuring they give dependable and up-to-date details. This enhances customer service standards and decreases answering duration.
Business Intelligence and Analysis: Delivering Domain-specific, Relevant Insights
In the scenario of Business Intelligence, RAG can be a groundbreaker. By delivering domain-specific perceptions, RAG enables you to make informed decisions based on the newest and most pertinent information. This improves your inquisitive abilities and helps you stay ahead in your industry.
Healthcare Information Systems: Accessing Medical Research and Patient Data for Better Care
Healthcare professionals can take advantage of RAG by attaining medical investigation and patient records efficiently. RAG permits for swift recovery of relevant details, helping in better curing and treatment plans, eventually enhancing patient care.
Legal Research and Compliance: Assisting in the Analysis of Legal Documents and Regulatory Compliance
Legitimate professionals can use RAG to sleek the inspection of legitimate documents and ensure regulatory compliance. By recovering and producing pertinent legitimate data, RAG helps in comprehensive investigation and compliance checks, making legitimate processes more effective and precise.
And that's not all—RAG's utility is expanding into even more advanced, specialized areas. Check out some next-level use cases.
Advanced RAG Use Cases
Gaining Insights from Sales Rep Feedback
Imagine turning your sales representative’ remarks into gold mines of applicable insights. You can use Retrieval-Augmented Generation (RAG) to dissect sales feedback. By involuntarily classifying and amalgamating responses, you can pinpoint trends, common problems and opportunities.
This permits you to cautiously acknowledge concerns, customize your approach to customer requirements, and eventually drive better customer success results. It’s like having a 24/7 annotator that turns every piece of response into planned insights.
Medical Insights Miner: Enhancing Research with Real-Time PubMed Data
Stay ahead in medical investigation by pounding into real-time information from PubMed using RAG. This tool permits you to constantly observe and extract pertinent research discoveries, keeping you updated with the newest evolutions.
By incorporating these perceptions into your research process, you can improve the quality and promptness of your studies. This approach boosts discovery, helps in pinpointing emerging trends, and ensures that your work stays at the cutting edge of medical science.
L1/L2 Customer Support Assistant: Improving Customer Support Experiences
Elevate your customer support experience by using RAG to assist your L1 and L2 support teams. This tool can rapidly recover and present pertinent solutions from a wide knowledge base, ensuring that your support agents always have the correct data at their fingertips. By doing so, you can decrease response duration, increase solution rates, and improve overall customer contentment. It’s like giving your support team a significant support that never sleeps and always has the answers.
Compliance in Customer Contact Centers: Ensuring Behavior Analysis in Regulated Industries
Ensure your customer centers follow regulatory requirements using RAG. This tool can dissect interactions for compliance, discerning any divergence required conventions.
By giving real-time responses and recommendations, you can acknowledge problems instantly, ensuring that your functioning remains within the bounds of industry regulations. This proactive approach not only helps in sustaining compliance but also builds trust with your customers and investors.
Employee Knowledge Training Assessment: Enhancing Training Effectiveness Across Roles
Revolutionize your employee training programs with RAG. By inspecting training materials and employee responses, you can pinpoint gaps in knowledge and areas for enhancement.
This tool helps in customizing training sessions to acknowledge precise requirements, ensuring that employees across all roles receive the most efficient and pertinent training. By constantly evaluating and processing your training programs, you can elevate workflow, improve expertise, and ensure that your employees are always prepared to meet new challenges.
Global SOP Standardization: Analyzing and Improving Standard Operating Procedures
Sleek your worldwide operations by homogenizing your Standard Operating Procedures (SOPs) with RAG. This tool can dissect SOPs from distinct regions, dissect inconsistencies, and recommend enhancements.
By ensuring that all your SOPs are aligned and upgraded, you can improve functioning effectiveness, reduce errors, and ensure congruous quality across your organization. It’s like having a universal process examiner that ensures every process is up to par.
Operations Support Assistant in Manufacturing: Assisting Technical Productivity with Complex Machinery Maintenance
Improve your manufacturing operations with an RAG-powered support assistant. This tool can aid in sustaining intricate machinery by offering real-time troubleshooting and preserving data.
By rapidly recovering and presenting pertinent technical data, you can reduce interruption, enhance workflow, and lengthen the lifespan of your equipment. This approach ensures that your technical workforce always has the details they need to keep your operations running sleekly.
Of course, implementing RAG comes with its own set of best practices and considerations, and we'll explore those next.
Implementing RAG - Best Practices and Considerations
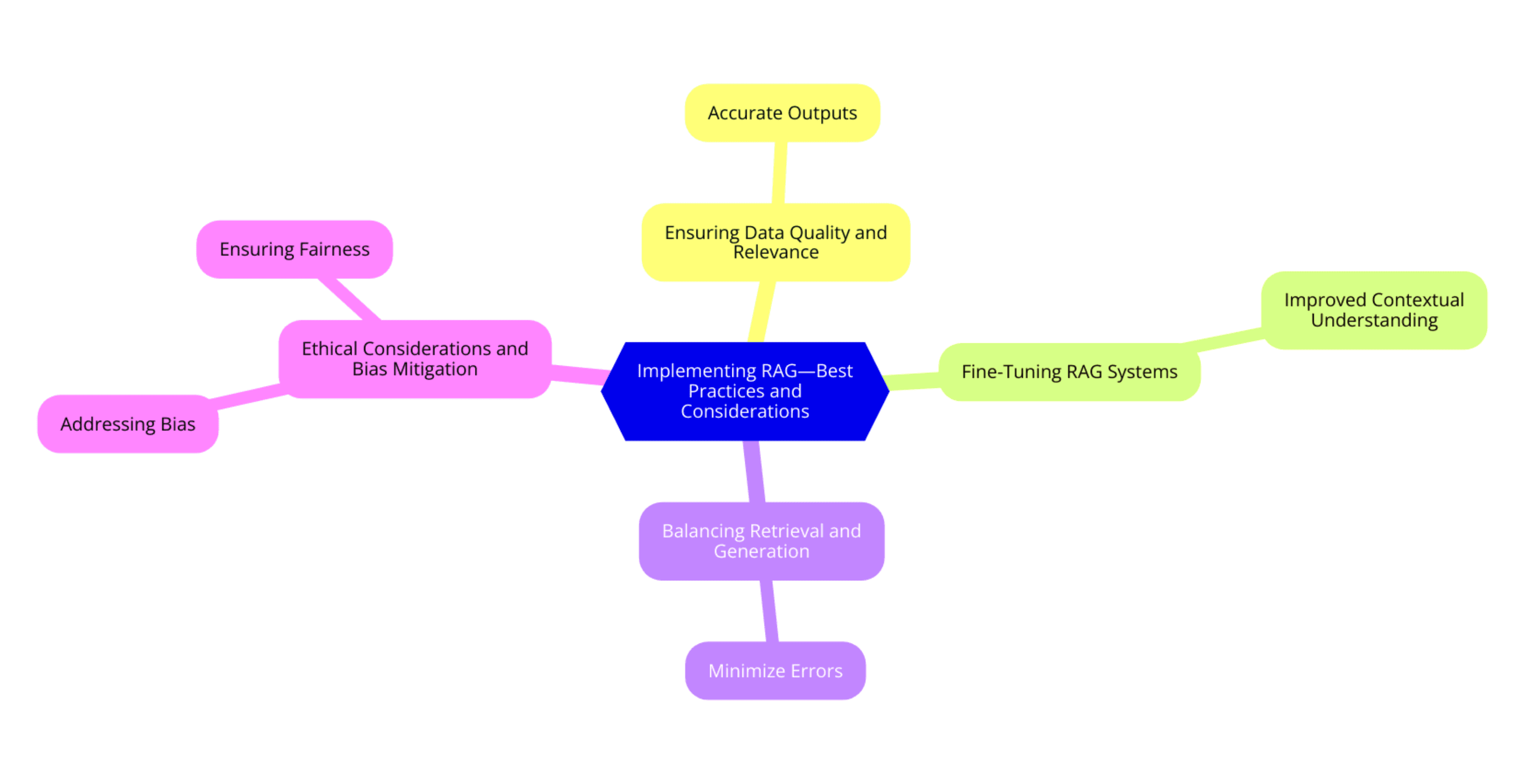
Ensuring Data Quality and Relevance for Accurate Outputs
When enforcing RAG (Retrieval-Augmented Generation), data quality and pertinence are your top priorities. You require to begin by consolidating a high-quality data set that is relevant to your domain.
This means using dependable sources, updating your data frequently, and removing any outdated or irrelevant data. High-quality information ensures that your RAG model recovers the most precise and helpful information, which results in more accurate and dependable yields.
Fine-Tuning RAG Systems for Improved Contextual Understanding
To get the most out of your RAG system, refining is important. This involves instructing your model on domain-specific data so it can comprehend the variations and context of your queries better. Use methods such as supervised refining with interpreted information to help your model grasp the correct answers.
This step is critical for improving the contextual comprehension of your system, making its yields more pertinent and precise.
Balancing Retrieval and Generation to Minimize Errors
Locating the right balance between retrieval and generation is key to minimizing mistakes and hallucinations. Too much dependency on generation can result in fabricated data, while over-dependence on recovery can restrict the creativity and profundity of the responses. You should adapt the emphasis of recovery and generation elements based on the nature of your application. Frequently assess the yields and modify the system to ensure it gives illuminating and dependable responses.
Ethical Considerations and Bias Mitigation in RAG Implementations
Ethics and bias mitigation should be essential to your RAG enforcement procedure. Begin by inspecting your dataset for biases and ensuring a disparate range of sources. Enforcing fairness-aware algorithms can help in lessening bias in the retrieval process. In addition, it’s important to sustain clarity with users about how your system operates and the sources it uses. By concentrating on ethical contemplations, you can build trust and ensure that your RAG system provides impartial and fair data.
So, what does the future hold for RAG? Let's explore the exciting advancements and potential impacts on various industries.
Future Directions and Impact of RAG
Exploration of Multimodal Capabilities and API Access
Envisage a globe where your applications can refine not just text, but pictures, audio and video smoothly. That’s where the probing of multimodal abilities in RAG (Retrieval-Augmented Generation) is heading. By incorporating numerous data types, you improve the opulence and context of data your application can handle.
Connected with API access, you can pull real-time information from disparate sources, making your solutions more robust and receptive. This means more reciprocal and captivating user experience, where your app can describe an image, condense a video, or even communicate through voice.
Broader Applications and Enhanced User Experiences
RAGs potential isn’t restricted to a single field. You can apply it across education, finance, healthcare and beyond. Picture an informational platform that not only answers student queries but also gives comprehensive elucidations, suggests further reading, and even interrogates students based on their learning growth. In healthcare, RAG can assist doctors by recovering the latest investigation and patient record, helping them make informed decisions.
The key is the enriched user experience, where communications feel more natural and private, making technology an inherent augmentation of human abilities.
Data Integration and Innovations in Multimodal Models
Incorporating distinct types of data such as text, images, and standardized data opens new frontiers in RAG applications. This incorporation permits for more sophisticated data refining and insights generation. Innovations in multimodal models mean you can evolve applications that comprehend and produce content across multiple formats.
For example, a customer service bot could dissect a customer’s voice tone and the content of their texts to offer more compassionate and precise answers. Such innovations drive forward a more associated and perceptive digital ecosystem.
LangChain and LLM RAG: Generative Search, Data Chat, and Next-Gen Customer Service
LanChain and large language model (LLM) RAG applications are revolutionizing how you communicate with data. With generative search, your doubts return not just pertinent documents, but integrated, brief responses drawn from numerous sources.
Chatting with data means engaging in a conversation with your datasets, asking intricate questions and getting comprehensible, applicable perceptions. In customer service, next-generation applications can handle more complex queries, giving prompt, precise support and freeing up human agents for more intricate tasks. This shift towards savvy, more receptive systems marks a substantial leap in how we use the power of AI to enhance daily communications and productivity.
By clasping these future guidance, you can use RAG to create more smart, receptive and flexible applications, pushing the boundaries of what’s possible in technology today.
To cap things off, let’s look at how you can seamlessly integrate these advanced RAG platforms into your existing systems to elevate productivity and effectiveness.
Conclusion
Retrieval-Augmented Generation is a revolutionary technology assured to transform numerous industries. By using real-time information and context-aware refining, RAG ensures that AI systems deliver the most precise and pertinent information, paving the way for innovative applications and improved user experiences.
Whether it’s healthcare, legitimate research, or customer support, RAG is set to make a substantial impact, driving effectiveness and precision across disparate domains.
Explore how you can smoothly integrate advanced RAG platforms into your current enterprise systems for improved effectiveness and productivity in our thorough guide on Integration Of RAG Platforms With Existing Enterprise Systems.
Ever wondered if your AI assistant could fetch real-time data while still making clever quips? Welcome to the world of Retrieval-Augmented Generation (RAG) where your wish is its command!
Envision a world where AI systems can attain and incorporate real-time data from the web to offer you up-to-date, precise and contextually pertinent details. This is the power of Retrieval-Augmented Generation (RAG), an innovative approach transforming the abilities of language models.
By smoothly merging data retrieval with context-aware refining, RAG ensures that large language models (LLMs) are not just producing text from stagnant training data but are dynamically pulling in current, dependable facts from external databases.
This innovation clarifies data sources and alleviates concerns about bias in LLM yields, making it a groundbreaker in numerous fields. Let’s dive into what makes RAG so important and explore its disparate use cases.
Want to get a detailed analysis on distinct LLMs, check out our, “Comparing Different Large Language Models (LLMs)” article.
The Basics of Retrieval Augmented Generation
How RAG Works: Merging Dynamic Retrieval with Context-Aware Processing
Envisage a system that can retrieve the most pertinent data from a vast database and produce context-aware, coherent responses in real-time.
That’s Retrieval-Augmented Generation (RAG) in action. It merges 2 strong AI capabilities: dynamic information retrieval and context-aware processing. When you ask a question, RAG first retrieves relevant information from a knowledge base.
Then, it uses this data to produce a response that is both precise and contextually pertinent. This dual-step procedure ensures that the answers you get are not only pertinent but also pragmatic.
Distinction Between Two Types of RAG Models
There are two predominant RAG models: RAG-Token and RAG Sequence.
RAG-Token: This model recovers details at the token level. The model produces each word (token) of the response by actively pulling pertinent pieces of information. This approach permits highly correct and precise responses, as each token is informed by the latest information.
RAG-Sequence: Unlike RAG-Token, the RAG-Sequence model recovers information for the whole sequence of text before producing the responses. This technique ensures that the response is coherent and contextually congruous, as the entire sequence is based on a pragmatic comprehension of the recovered data.
Key Components of RAG
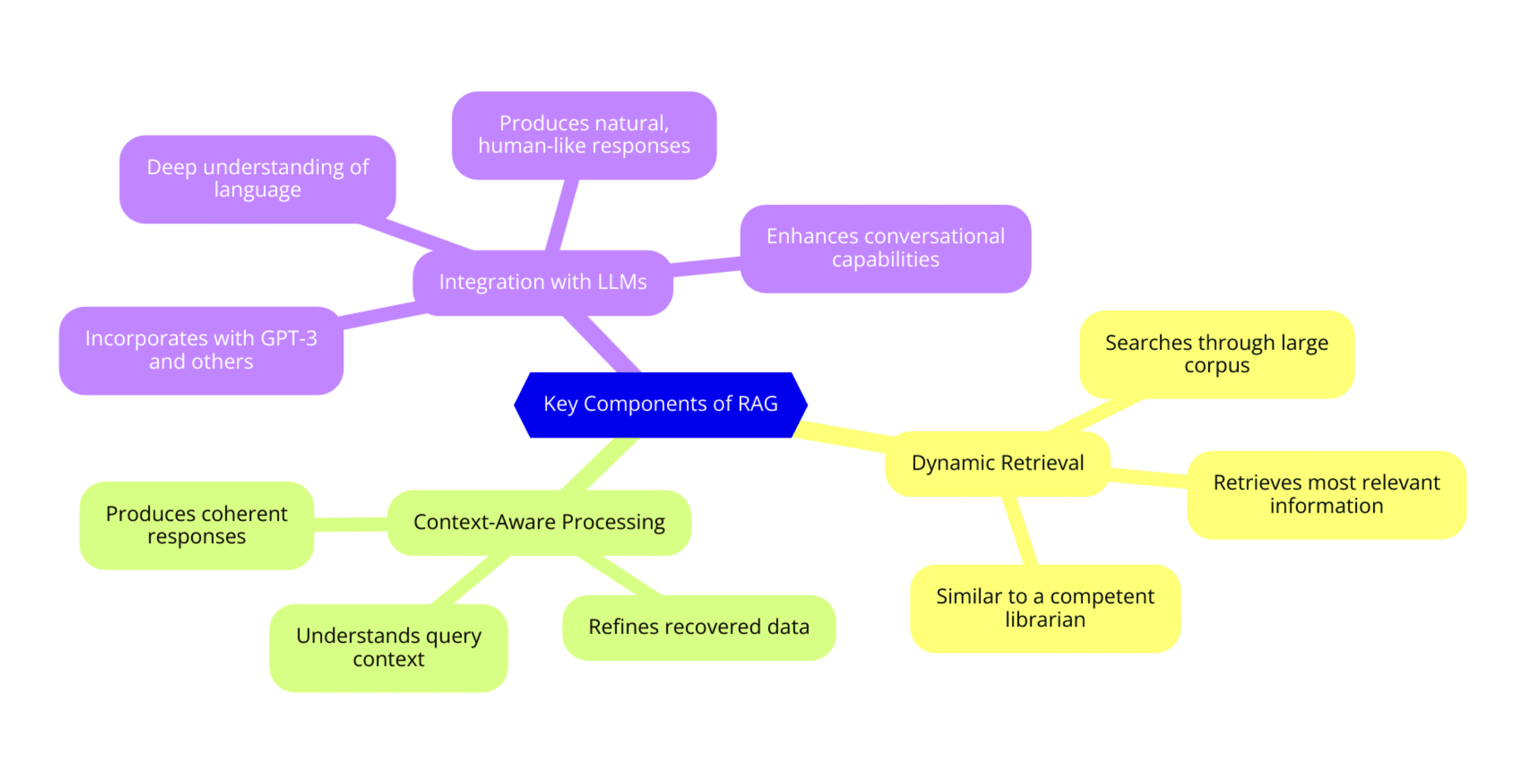
To entirely learn how RAG works, let’s break down its key components:
Dynamic Retrieval: This is the initial step, where the system searches through a huge corpus of information to retrieve the most relevant information. It resembles a competent librarian promptly locating the exact book or documents you need.
Context-Aware Processing: Once the pertinent data is recovered, the system refines it in a way that comprehends the context of your query. It ensures that the produced response is not just an arbitrarily collection of facts but a coherent and contextually apt response.
Integration with Large Language Models (LLMs): RAG incorporates smoothly with LLMs such as GPT-3. These models have a deep comprehension of language, enabling them to produce natural, human-like responses. The incorporation with LLMs permits RAG to use their conversational capabilities, improving the overall quality of the produced content.
Path of Development Since Its Creation by Meta Research in 2020
RAG’s expedition began in 2020, established by Meta Research (formerly Facebook AI). Since then, it has developed substantially. Initially, Meta Research designed RAG to improve the precision and pertinence of AI-produced responses, and it has since undergone numerous iterations to enhance its effectiveness and reliability.
Early versions concentrated on fine-tuning the dynamic recovery procedure, ensuring that the system could retrieve the most relevant information rapidly. Subsequent evolutions aimed to improve the context-aware refining abilities, making the responses more coherent and contextually felicitous.
Today, RAG stands as a sturdy structure that incorporates smoothly with LLMs, providing exceptional precision and pertinence in data retrieval and generation.
As you traverse the potential of RAG, you will find its applications in several fields, from customer assistance and education to investigation and content creation. Its capability to merge real-time data retrieval with advanced language generation makes it a groundbreaker in the AI world.
Now that we have a firm grasp on how RAG functions, let's dive into some real-world applications that showcase its true impact.
Practical Use Cases of RAG (Retrieval-Augmented Generation)
Document Question Answering Systems: Enhancing Access to Proprietary Documents
Envisage having an enormous base of proprietary documents and requiring precise details from them swiftly. Document Question Answering System, powered by RAG, can transform this process. By asking queries in natural language, you can promptly recover specific responses from your documents, saving time and enhancing effectiveness.
Conversational Agents: Customizing LLMs to Specific Guidelines or Manuals
Conversational agents can become even more efficient when customized to precise instructions or manuals. With RAG, you can tailor language models to adhere to concrete conventions and industry standards. This ensures that the AI interacts with precision while complying with specific needs.
Real-time Event Commentary with Live Data and LLMs
For live events, giving real-time commentary is critical. RAG can connect language models to live data feeds, permitting you to produce up-to-minute reports that improve the virtual experience. Whether it’s a sports game, a meeting, or a breaking news story, RAG keeps your audience engaged with the newest updates.
Content Generation: Personalizing Content and Ensuring Contextual Relevance
Generating customized content that reverberates with your audience can be challenging. RAG helps by using real-time data to create content that is not only pertinent but also gradually customized. This ensures that your readers find your content appealing and valuable, elevating your content’s efficiency.
Personalized Recommendation: Evolving Content Recommendations through LLMs
RAG can revolutionize how you provide customized suggestions. By incorporating retrieval mechanisms and language models, you can offer suggestions that develop based on user interactions and choices. This dynamic approach ensures that your suggestions remain pertinent and customized over time.
Virtual Assistants: Creating More Personalized User Experiences
Virtual Assistants equipped with RAG abilities can provide gradually customized user experiences. They can recover pertinent details and produce answers that serve specifically to the user’s requirement and context. This makes interactions more relevant and improves user contentment.
Customer Support Chatbots: Providing Up-to-date and Accurate Responses
Customer support chatbots need to deliver precise and prompt responses. With RAG, your chatbots can attain the most latest information, ensuring they give dependable and up-to-date details. This enhances customer service standards and decreases answering duration.
Business Intelligence and Analysis: Delivering Domain-specific, Relevant Insights
In the scenario of Business Intelligence, RAG can be a groundbreaker. By delivering domain-specific perceptions, RAG enables you to make informed decisions based on the newest and most pertinent information. This improves your inquisitive abilities and helps you stay ahead in your industry.
Healthcare Information Systems: Accessing Medical Research and Patient Data for Better Care
Healthcare professionals can take advantage of RAG by attaining medical investigation and patient records efficiently. RAG permits for swift recovery of relevant details, helping in better curing and treatment plans, eventually enhancing patient care.
Legal Research and Compliance: Assisting in the Analysis of Legal Documents and Regulatory Compliance
Legitimate professionals can use RAG to sleek the inspection of legitimate documents and ensure regulatory compliance. By recovering and producing pertinent legitimate data, RAG helps in comprehensive investigation and compliance checks, making legitimate processes more effective and precise.
And that's not all—RAG's utility is expanding into even more advanced, specialized areas. Check out some next-level use cases.
Advanced RAG Use Cases
Gaining Insights from Sales Rep Feedback
Imagine turning your sales representative’ remarks into gold mines of applicable insights. You can use Retrieval-Augmented Generation (RAG) to dissect sales feedback. By involuntarily classifying and amalgamating responses, you can pinpoint trends, common problems and opportunities.
This permits you to cautiously acknowledge concerns, customize your approach to customer requirements, and eventually drive better customer success results. It’s like having a 24/7 annotator that turns every piece of response into planned insights.
Medical Insights Miner: Enhancing Research with Real-Time PubMed Data
Stay ahead in medical investigation by pounding into real-time information from PubMed using RAG. This tool permits you to constantly observe and extract pertinent research discoveries, keeping you updated with the newest evolutions.
By incorporating these perceptions into your research process, you can improve the quality and promptness of your studies. This approach boosts discovery, helps in pinpointing emerging trends, and ensures that your work stays at the cutting edge of medical science.
L1/L2 Customer Support Assistant: Improving Customer Support Experiences
Elevate your customer support experience by using RAG to assist your L1 and L2 support teams. This tool can rapidly recover and present pertinent solutions from a wide knowledge base, ensuring that your support agents always have the correct data at their fingertips. By doing so, you can decrease response duration, increase solution rates, and improve overall customer contentment. It’s like giving your support team a significant support that never sleeps and always has the answers.
Compliance in Customer Contact Centers: Ensuring Behavior Analysis in Regulated Industries
Ensure your customer centers follow regulatory requirements using RAG. This tool can dissect interactions for compliance, discerning any divergence required conventions.
By giving real-time responses and recommendations, you can acknowledge problems instantly, ensuring that your functioning remains within the bounds of industry regulations. This proactive approach not only helps in sustaining compliance but also builds trust with your customers and investors.
Employee Knowledge Training Assessment: Enhancing Training Effectiveness Across Roles
Revolutionize your employee training programs with RAG. By inspecting training materials and employee responses, you can pinpoint gaps in knowledge and areas for enhancement.
This tool helps in customizing training sessions to acknowledge precise requirements, ensuring that employees across all roles receive the most efficient and pertinent training. By constantly evaluating and processing your training programs, you can elevate workflow, improve expertise, and ensure that your employees are always prepared to meet new challenges.
Global SOP Standardization: Analyzing and Improving Standard Operating Procedures
Sleek your worldwide operations by homogenizing your Standard Operating Procedures (SOPs) with RAG. This tool can dissect SOPs from distinct regions, dissect inconsistencies, and recommend enhancements.
By ensuring that all your SOPs are aligned and upgraded, you can improve functioning effectiveness, reduce errors, and ensure congruous quality across your organization. It’s like having a universal process examiner that ensures every process is up to par.
Operations Support Assistant in Manufacturing: Assisting Technical Productivity with Complex Machinery Maintenance
Improve your manufacturing operations with an RAG-powered support assistant. This tool can aid in sustaining intricate machinery by offering real-time troubleshooting and preserving data.
By rapidly recovering and presenting pertinent technical data, you can reduce interruption, enhance workflow, and lengthen the lifespan of your equipment. This approach ensures that your technical workforce always has the details they need to keep your operations running sleekly.
Of course, implementing RAG comes with its own set of best practices and considerations, and we'll explore those next.
Implementing RAG - Best Practices and Considerations
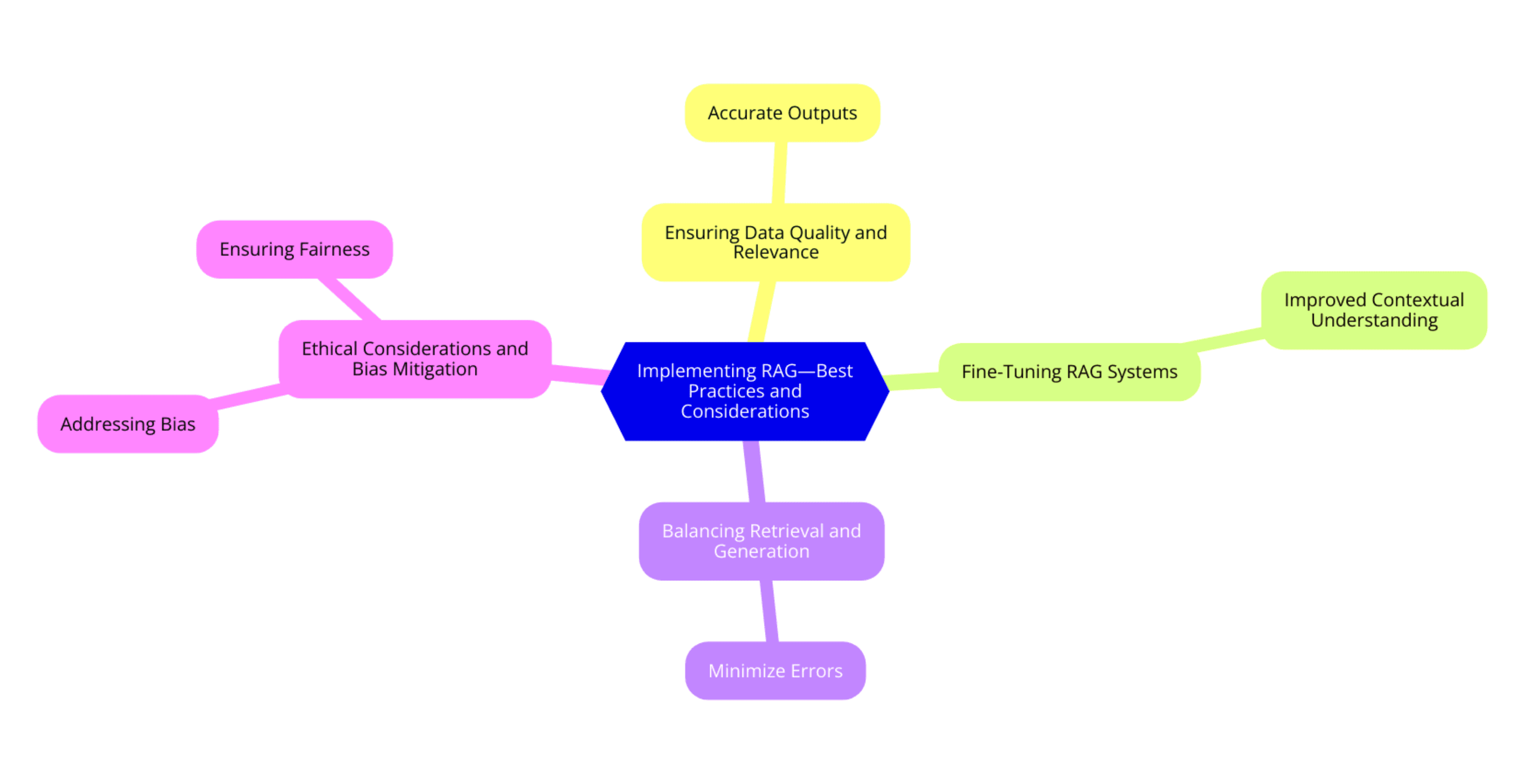
Ensuring Data Quality and Relevance for Accurate Outputs
When enforcing RAG (Retrieval-Augmented Generation), data quality and pertinence are your top priorities. You require to begin by consolidating a high-quality data set that is relevant to your domain.
This means using dependable sources, updating your data frequently, and removing any outdated or irrelevant data. High-quality information ensures that your RAG model recovers the most precise and helpful information, which results in more accurate and dependable yields.
Fine-Tuning RAG Systems for Improved Contextual Understanding
To get the most out of your RAG system, refining is important. This involves instructing your model on domain-specific data so it can comprehend the variations and context of your queries better. Use methods such as supervised refining with interpreted information to help your model grasp the correct answers.
This step is critical for improving the contextual comprehension of your system, making its yields more pertinent and precise.
Balancing Retrieval and Generation to Minimize Errors
Locating the right balance between retrieval and generation is key to minimizing mistakes and hallucinations. Too much dependency on generation can result in fabricated data, while over-dependence on recovery can restrict the creativity and profundity of the responses. You should adapt the emphasis of recovery and generation elements based on the nature of your application. Frequently assess the yields and modify the system to ensure it gives illuminating and dependable responses.
Ethical Considerations and Bias Mitigation in RAG Implementations
Ethics and bias mitigation should be essential to your RAG enforcement procedure. Begin by inspecting your dataset for biases and ensuring a disparate range of sources. Enforcing fairness-aware algorithms can help in lessening bias in the retrieval process. In addition, it’s important to sustain clarity with users about how your system operates and the sources it uses. By concentrating on ethical contemplations, you can build trust and ensure that your RAG system provides impartial and fair data.
So, what does the future hold for RAG? Let's explore the exciting advancements and potential impacts on various industries.
Future Directions and Impact of RAG
Exploration of Multimodal Capabilities and API Access
Envisage a globe where your applications can refine not just text, but pictures, audio and video smoothly. That’s where the probing of multimodal abilities in RAG (Retrieval-Augmented Generation) is heading. By incorporating numerous data types, you improve the opulence and context of data your application can handle.
Connected with API access, you can pull real-time information from disparate sources, making your solutions more robust and receptive. This means more reciprocal and captivating user experience, where your app can describe an image, condense a video, or even communicate through voice.
Broader Applications and Enhanced User Experiences
RAGs potential isn’t restricted to a single field. You can apply it across education, finance, healthcare and beyond. Picture an informational platform that not only answers student queries but also gives comprehensive elucidations, suggests further reading, and even interrogates students based on their learning growth. In healthcare, RAG can assist doctors by recovering the latest investigation and patient record, helping them make informed decisions.
The key is the enriched user experience, where communications feel more natural and private, making technology an inherent augmentation of human abilities.
Data Integration and Innovations in Multimodal Models
Incorporating distinct types of data such as text, images, and standardized data opens new frontiers in RAG applications. This incorporation permits for more sophisticated data refining and insights generation. Innovations in multimodal models mean you can evolve applications that comprehend and produce content across multiple formats.
For example, a customer service bot could dissect a customer’s voice tone and the content of their texts to offer more compassionate and precise answers. Such innovations drive forward a more associated and perceptive digital ecosystem.
LangChain and LLM RAG: Generative Search, Data Chat, and Next-Gen Customer Service
LanChain and large language model (LLM) RAG applications are revolutionizing how you communicate with data. With generative search, your doubts return not just pertinent documents, but integrated, brief responses drawn from numerous sources.
Chatting with data means engaging in a conversation with your datasets, asking intricate questions and getting comprehensible, applicable perceptions. In customer service, next-generation applications can handle more complex queries, giving prompt, precise support and freeing up human agents for more intricate tasks. This shift towards savvy, more receptive systems marks a substantial leap in how we use the power of AI to enhance daily communications and productivity.
By clasping these future guidance, you can use RAG to create more smart, receptive and flexible applications, pushing the boundaries of what’s possible in technology today.
To cap things off, let’s look at how you can seamlessly integrate these advanced RAG platforms into your existing systems to elevate productivity and effectiveness.
Conclusion
Retrieval-Augmented Generation is a revolutionary technology assured to transform numerous industries. By using real-time information and context-aware refining, RAG ensures that AI systems deliver the most precise and pertinent information, paving the way for innovative applications and improved user experiences.
Whether it’s healthcare, legitimate research, or customer support, RAG is set to make a substantial impact, driving effectiveness and precision across disparate domains.
Explore how you can smoothly integrate advanced RAG platforms into your current enterprise systems for improved effectiveness and productivity in our thorough guide on Integration Of RAG Platforms With Existing Enterprise Systems.
Ever wondered if your AI assistant could fetch real-time data while still making clever quips? Welcome to the world of Retrieval-Augmented Generation (RAG) where your wish is its command!
Envision a world where AI systems can attain and incorporate real-time data from the web to offer you up-to-date, precise and contextually pertinent details. This is the power of Retrieval-Augmented Generation (RAG), an innovative approach transforming the abilities of language models.
By smoothly merging data retrieval with context-aware refining, RAG ensures that large language models (LLMs) are not just producing text from stagnant training data but are dynamically pulling in current, dependable facts from external databases.
This innovation clarifies data sources and alleviates concerns about bias in LLM yields, making it a groundbreaker in numerous fields. Let’s dive into what makes RAG so important and explore its disparate use cases.
Want to get a detailed analysis on distinct LLMs, check out our, “Comparing Different Large Language Models (LLMs)” article.
The Basics of Retrieval Augmented Generation
How RAG Works: Merging Dynamic Retrieval with Context-Aware Processing
Envisage a system that can retrieve the most pertinent data from a vast database and produce context-aware, coherent responses in real-time.
That’s Retrieval-Augmented Generation (RAG) in action. It merges 2 strong AI capabilities: dynamic information retrieval and context-aware processing. When you ask a question, RAG first retrieves relevant information from a knowledge base.
Then, it uses this data to produce a response that is both precise and contextually pertinent. This dual-step procedure ensures that the answers you get are not only pertinent but also pragmatic.
Distinction Between Two Types of RAG Models
There are two predominant RAG models: RAG-Token and RAG Sequence.
RAG-Token: This model recovers details at the token level. The model produces each word (token) of the response by actively pulling pertinent pieces of information. This approach permits highly correct and precise responses, as each token is informed by the latest information.
RAG-Sequence: Unlike RAG-Token, the RAG-Sequence model recovers information for the whole sequence of text before producing the responses. This technique ensures that the response is coherent and contextually congruous, as the entire sequence is based on a pragmatic comprehension of the recovered data.
Key Components of RAG
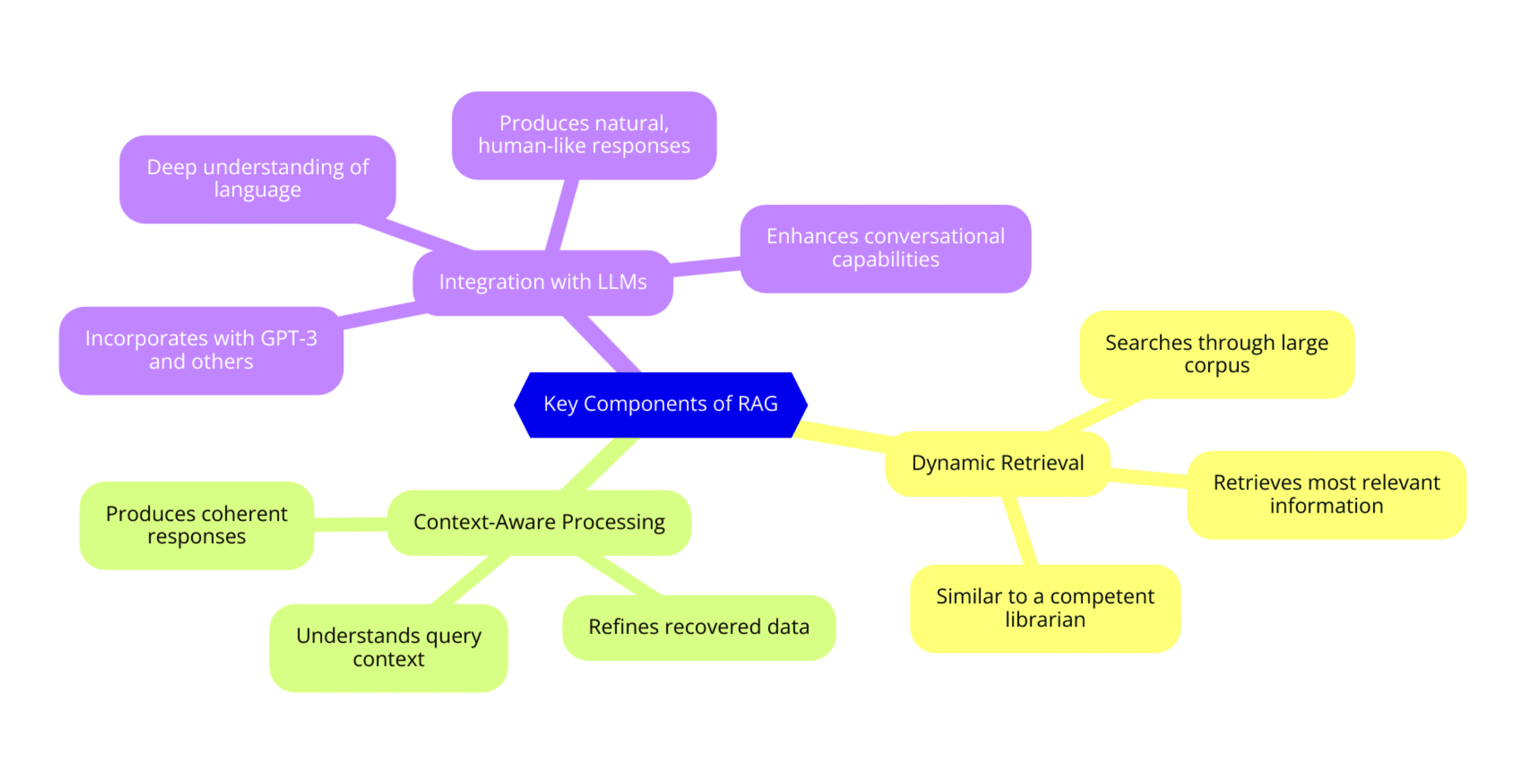
To entirely learn how RAG works, let’s break down its key components:
Dynamic Retrieval: This is the initial step, where the system searches through a huge corpus of information to retrieve the most relevant information. It resembles a competent librarian promptly locating the exact book or documents you need.
Context-Aware Processing: Once the pertinent data is recovered, the system refines it in a way that comprehends the context of your query. It ensures that the produced response is not just an arbitrarily collection of facts but a coherent and contextually apt response.
Integration with Large Language Models (LLMs): RAG incorporates smoothly with LLMs such as GPT-3. These models have a deep comprehension of language, enabling them to produce natural, human-like responses. The incorporation with LLMs permits RAG to use their conversational capabilities, improving the overall quality of the produced content.
Path of Development Since Its Creation by Meta Research in 2020
RAG’s expedition began in 2020, established by Meta Research (formerly Facebook AI). Since then, it has developed substantially. Initially, Meta Research designed RAG to improve the precision and pertinence of AI-produced responses, and it has since undergone numerous iterations to enhance its effectiveness and reliability.
Early versions concentrated on fine-tuning the dynamic recovery procedure, ensuring that the system could retrieve the most relevant information rapidly. Subsequent evolutions aimed to improve the context-aware refining abilities, making the responses more coherent and contextually felicitous.
Today, RAG stands as a sturdy structure that incorporates smoothly with LLMs, providing exceptional precision and pertinence in data retrieval and generation.
As you traverse the potential of RAG, you will find its applications in several fields, from customer assistance and education to investigation and content creation. Its capability to merge real-time data retrieval with advanced language generation makes it a groundbreaker in the AI world.
Now that we have a firm grasp on how RAG functions, let's dive into some real-world applications that showcase its true impact.
Practical Use Cases of RAG (Retrieval-Augmented Generation)
Document Question Answering Systems: Enhancing Access to Proprietary Documents
Envisage having an enormous base of proprietary documents and requiring precise details from them swiftly. Document Question Answering System, powered by RAG, can transform this process. By asking queries in natural language, you can promptly recover specific responses from your documents, saving time and enhancing effectiveness.
Conversational Agents: Customizing LLMs to Specific Guidelines or Manuals
Conversational agents can become even more efficient when customized to precise instructions or manuals. With RAG, you can tailor language models to adhere to concrete conventions and industry standards. This ensures that the AI interacts with precision while complying with specific needs.
Real-time Event Commentary with Live Data and LLMs
For live events, giving real-time commentary is critical. RAG can connect language models to live data feeds, permitting you to produce up-to-minute reports that improve the virtual experience. Whether it’s a sports game, a meeting, or a breaking news story, RAG keeps your audience engaged with the newest updates.
Content Generation: Personalizing Content and Ensuring Contextual Relevance
Generating customized content that reverberates with your audience can be challenging. RAG helps by using real-time data to create content that is not only pertinent but also gradually customized. This ensures that your readers find your content appealing and valuable, elevating your content’s efficiency.
Personalized Recommendation: Evolving Content Recommendations through LLMs
RAG can revolutionize how you provide customized suggestions. By incorporating retrieval mechanisms and language models, you can offer suggestions that develop based on user interactions and choices. This dynamic approach ensures that your suggestions remain pertinent and customized over time.
Virtual Assistants: Creating More Personalized User Experiences
Virtual Assistants equipped with RAG abilities can provide gradually customized user experiences. They can recover pertinent details and produce answers that serve specifically to the user’s requirement and context. This makes interactions more relevant and improves user contentment.
Customer Support Chatbots: Providing Up-to-date and Accurate Responses
Customer support chatbots need to deliver precise and prompt responses. With RAG, your chatbots can attain the most latest information, ensuring they give dependable and up-to-date details. This enhances customer service standards and decreases answering duration.
Business Intelligence and Analysis: Delivering Domain-specific, Relevant Insights
In the scenario of Business Intelligence, RAG can be a groundbreaker. By delivering domain-specific perceptions, RAG enables you to make informed decisions based on the newest and most pertinent information. This improves your inquisitive abilities and helps you stay ahead in your industry.
Healthcare Information Systems: Accessing Medical Research and Patient Data for Better Care
Healthcare professionals can take advantage of RAG by attaining medical investigation and patient records efficiently. RAG permits for swift recovery of relevant details, helping in better curing and treatment plans, eventually enhancing patient care.
Legal Research and Compliance: Assisting in the Analysis of Legal Documents and Regulatory Compliance
Legitimate professionals can use RAG to sleek the inspection of legitimate documents and ensure regulatory compliance. By recovering and producing pertinent legitimate data, RAG helps in comprehensive investigation and compliance checks, making legitimate processes more effective and precise.
And that's not all—RAG's utility is expanding into even more advanced, specialized areas. Check out some next-level use cases.
Advanced RAG Use Cases
Gaining Insights from Sales Rep Feedback
Imagine turning your sales representative’ remarks into gold mines of applicable insights. You can use Retrieval-Augmented Generation (RAG) to dissect sales feedback. By involuntarily classifying and amalgamating responses, you can pinpoint trends, common problems and opportunities.
This permits you to cautiously acknowledge concerns, customize your approach to customer requirements, and eventually drive better customer success results. It’s like having a 24/7 annotator that turns every piece of response into planned insights.
Medical Insights Miner: Enhancing Research with Real-Time PubMed Data
Stay ahead in medical investigation by pounding into real-time information from PubMed using RAG. This tool permits you to constantly observe and extract pertinent research discoveries, keeping you updated with the newest evolutions.
By incorporating these perceptions into your research process, you can improve the quality and promptness of your studies. This approach boosts discovery, helps in pinpointing emerging trends, and ensures that your work stays at the cutting edge of medical science.
L1/L2 Customer Support Assistant: Improving Customer Support Experiences
Elevate your customer support experience by using RAG to assist your L1 and L2 support teams. This tool can rapidly recover and present pertinent solutions from a wide knowledge base, ensuring that your support agents always have the correct data at their fingertips. By doing so, you can decrease response duration, increase solution rates, and improve overall customer contentment. It’s like giving your support team a significant support that never sleeps and always has the answers.
Compliance in Customer Contact Centers: Ensuring Behavior Analysis in Regulated Industries
Ensure your customer centers follow regulatory requirements using RAG. This tool can dissect interactions for compliance, discerning any divergence required conventions.
By giving real-time responses and recommendations, you can acknowledge problems instantly, ensuring that your functioning remains within the bounds of industry regulations. This proactive approach not only helps in sustaining compliance but also builds trust with your customers and investors.
Employee Knowledge Training Assessment: Enhancing Training Effectiveness Across Roles
Revolutionize your employee training programs with RAG. By inspecting training materials and employee responses, you can pinpoint gaps in knowledge and areas for enhancement.
This tool helps in customizing training sessions to acknowledge precise requirements, ensuring that employees across all roles receive the most efficient and pertinent training. By constantly evaluating and processing your training programs, you can elevate workflow, improve expertise, and ensure that your employees are always prepared to meet new challenges.
Global SOP Standardization: Analyzing and Improving Standard Operating Procedures
Sleek your worldwide operations by homogenizing your Standard Operating Procedures (SOPs) with RAG. This tool can dissect SOPs from distinct regions, dissect inconsistencies, and recommend enhancements.
By ensuring that all your SOPs are aligned and upgraded, you can improve functioning effectiveness, reduce errors, and ensure congruous quality across your organization. It’s like having a universal process examiner that ensures every process is up to par.
Operations Support Assistant in Manufacturing: Assisting Technical Productivity with Complex Machinery Maintenance
Improve your manufacturing operations with an RAG-powered support assistant. This tool can aid in sustaining intricate machinery by offering real-time troubleshooting and preserving data.
By rapidly recovering and presenting pertinent technical data, you can reduce interruption, enhance workflow, and lengthen the lifespan of your equipment. This approach ensures that your technical workforce always has the details they need to keep your operations running sleekly.
Of course, implementing RAG comes with its own set of best practices and considerations, and we'll explore those next.
Implementing RAG - Best Practices and Considerations
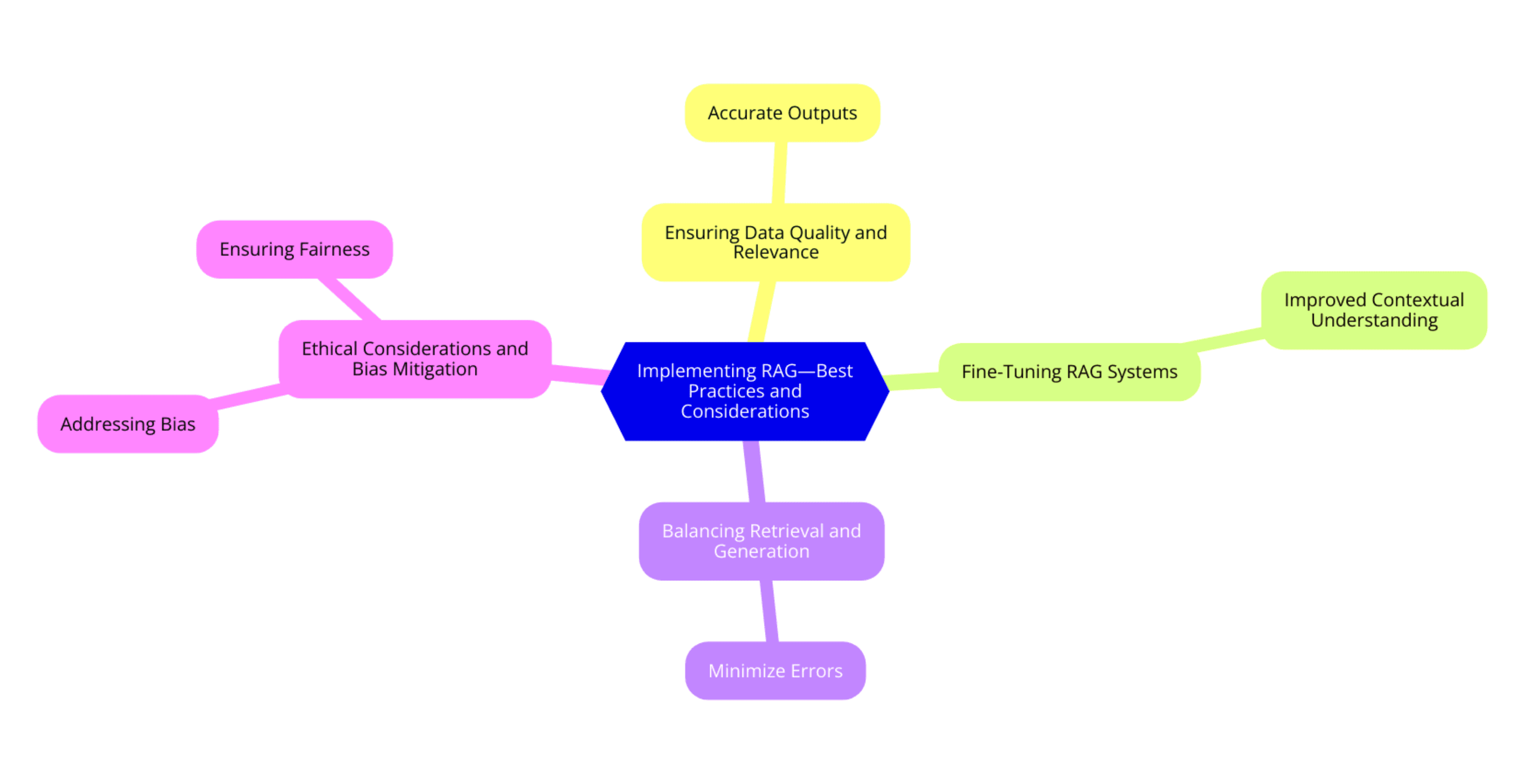
Ensuring Data Quality and Relevance for Accurate Outputs
When enforcing RAG (Retrieval-Augmented Generation), data quality and pertinence are your top priorities. You require to begin by consolidating a high-quality data set that is relevant to your domain.
This means using dependable sources, updating your data frequently, and removing any outdated or irrelevant data. High-quality information ensures that your RAG model recovers the most precise and helpful information, which results in more accurate and dependable yields.
Fine-Tuning RAG Systems for Improved Contextual Understanding
To get the most out of your RAG system, refining is important. This involves instructing your model on domain-specific data so it can comprehend the variations and context of your queries better. Use methods such as supervised refining with interpreted information to help your model grasp the correct answers.
This step is critical for improving the contextual comprehension of your system, making its yields more pertinent and precise.
Balancing Retrieval and Generation to Minimize Errors
Locating the right balance between retrieval and generation is key to minimizing mistakes and hallucinations. Too much dependency on generation can result in fabricated data, while over-dependence on recovery can restrict the creativity and profundity of the responses. You should adapt the emphasis of recovery and generation elements based on the nature of your application. Frequently assess the yields and modify the system to ensure it gives illuminating and dependable responses.
Ethical Considerations and Bias Mitigation in RAG Implementations
Ethics and bias mitigation should be essential to your RAG enforcement procedure. Begin by inspecting your dataset for biases and ensuring a disparate range of sources. Enforcing fairness-aware algorithms can help in lessening bias in the retrieval process. In addition, it’s important to sustain clarity with users about how your system operates and the sources it uses. By concentrating on ethical contemplations, you can build trust and ensure that your RAG system provides impartial and fair data.
So, what does the future hold for RAG? Let's explore the exciting advancements and potential impacts on various industries.
Future Directions and Impact of RAG
Exploration of Multimodal Capabilities and API Access
Envisage a globe where your applications can refine not just text, but pictures, audio and video smoothly. That’s where the probing of multimodal abilities in RAG (Retrieval-Augmented Generation) is heading. By incorporating numerous data types, you improve the opulence and context of data your application can handle.
Connected with API access, you can pull real-time information from disparate sources, making your solutions more robust and receptive. This means more reciprocal and captivating user experience, where your app can describe an image, condense a video, or even communicate through voice.
Broader Applications and Enhanced User Experiences
RAGs potential isn’t restricted to a single field. You can apply it across education, finance, healthcare and beyond. Picture an informational platform that not only answers student queries but also gives comprehensive elucidations, suggests further reading, and even interrogates students based on their learning growth. In healthcare, RAG can assist doctors by recovering the latest investigation and patient record, helping them make informed decisions.
The key is the enriched user experience, where communications feel more natural and private, making technology an inherent augmentation of human abilities.
Data Integration and Innovations in Multimodal Models
Incorporating distinct types of data such as text, images, and standardized data opens new frontiers in RAG applications. This incorporation permits for more sophisticated data refining and insights generation. Innovations in multimodal models mean you can evolve applications that comprehend and produce content across multiple formats.
For example, a customer service bot could dissect a customer’s voice tone and the content of their texts to offer more compassionate and precise answers. Such innovations drive forward a more associated and perceptive digital ecosystem.
LangChain and LLM RAG: Generative Search, Data Chat, and Next-Gen Customer Service
LanChain and large language model (LLM) RAG applications are revolutionizing how you communicate with data. With generative search, your doubts return not just pertinent documents, but integrated, brief responses drawn from numerous sources.
Chatting with data means engaging in a conversation with your datasets, asking intricate questions and getting comprehensible, applicable perceptions. In customer service, next-generation applications can handle more complex queries, giving prompt, precise support and freeing up human agents for more intricate tasks. This shift towards savvy, more receptive systems marks a substantial leap in how we use the power of AI to enhance daily communications and productivity.
By clasping these future guidance, you can use RAG to create more smart, receptive and flexible applications, pushing the boundaries of what’s possible in technology today.
To cap things off, let’s look at how you can seamlessly integrate these advanced RAG platforms into your existing systems to elevate productivity and effectiveness.
Conclusion
Retrieval-Augmented Generation is a revolutionary technology assured to transform numerous industries. By using real-time information and context-aware refining, RAG ensures that AI systems deliver the most precise and pertinent information, paving the way for innovative applications and improved user experiences.
Whether it’s healthcare, legitimate research, or customer support, RAG is set to make a substantial impact, driving effectiveness and precision across disparate domains.
Explore how you can smoothly integrate advanced RAG platforms into your current enterprise systems for improved effectiveness and productivity in our thorough guide on Integration Of RAG Platforms With Existing Enterprise Systems.
Ever wondered if your AI assistant could fetch real-time data while still making clever quips? Welcome to the world of Retrieval-Augmented Generation (RAG) where your wish is its command!
Envision a world where AI systems can attain and incorporate real-time data from the web to offer you up-to-date, precise and contextually pertinent details. This is the power of Retrieval-Augmented Generation (RAG), an innovative approach transforming the abilities of language models.
By smoothly merging data retrieval with context-aware refining, RAG ensures that large language models (LLMs) are not just producing text from stagnant training data but are dynamically pulling in current, dependable facts from external databases.
This innovation clarifies data sources and alleviates concerns about bias in LLM yields, making it a groundbreaker in numerous fields. Let’s dive into what makes RAG so important and explore its disparate use cases.
Want to get a detailed analysis on distinct LLMs, check out our, “Comparing Different Large Language Models (LLMs)” article.
The Basics of Retrieval Augmented Generation
How RAG Works: Merging Dynamic Retrieval with Context-Aware Processing
Envisage a system that can retrieve the most pertinent data from a vast database and produce context-aware, coherent responses in real-time.
That’s Retrieval-Augmented Generation (RAG) in action. It merges 2 strong AI capabilities: dynamic information retrieval and context-aware processing. When you ask a question, RAG first retrieves relevant information from a knowledge base.
Then, it uses this data to produce a response that is both precise and contextually pertinent. This dual-step procedure ensures that the answers you get are not only pertinent but also pragmatic.
Distinction Between Two Types of RAG Models
There are two predominant RAG models: RAG-Token and RAG Sequence.
RAG-Token: This model recovers details at the token level. The model produces each word (token) of the response by actively pulling pertinent pieces of information. This approach permits highly correct and precise responses, as each token is informed by the latest information.
RAG-Sequence: Unlike RAG-Token, the RAG-Sequence model recovers information for the whole sequence of text before producing the responses. This technique ensures that the response is coherent and contextually congruous, as the entire sequence is based on a pragmatic comprehension of the recovered data.
Key Components of RAG
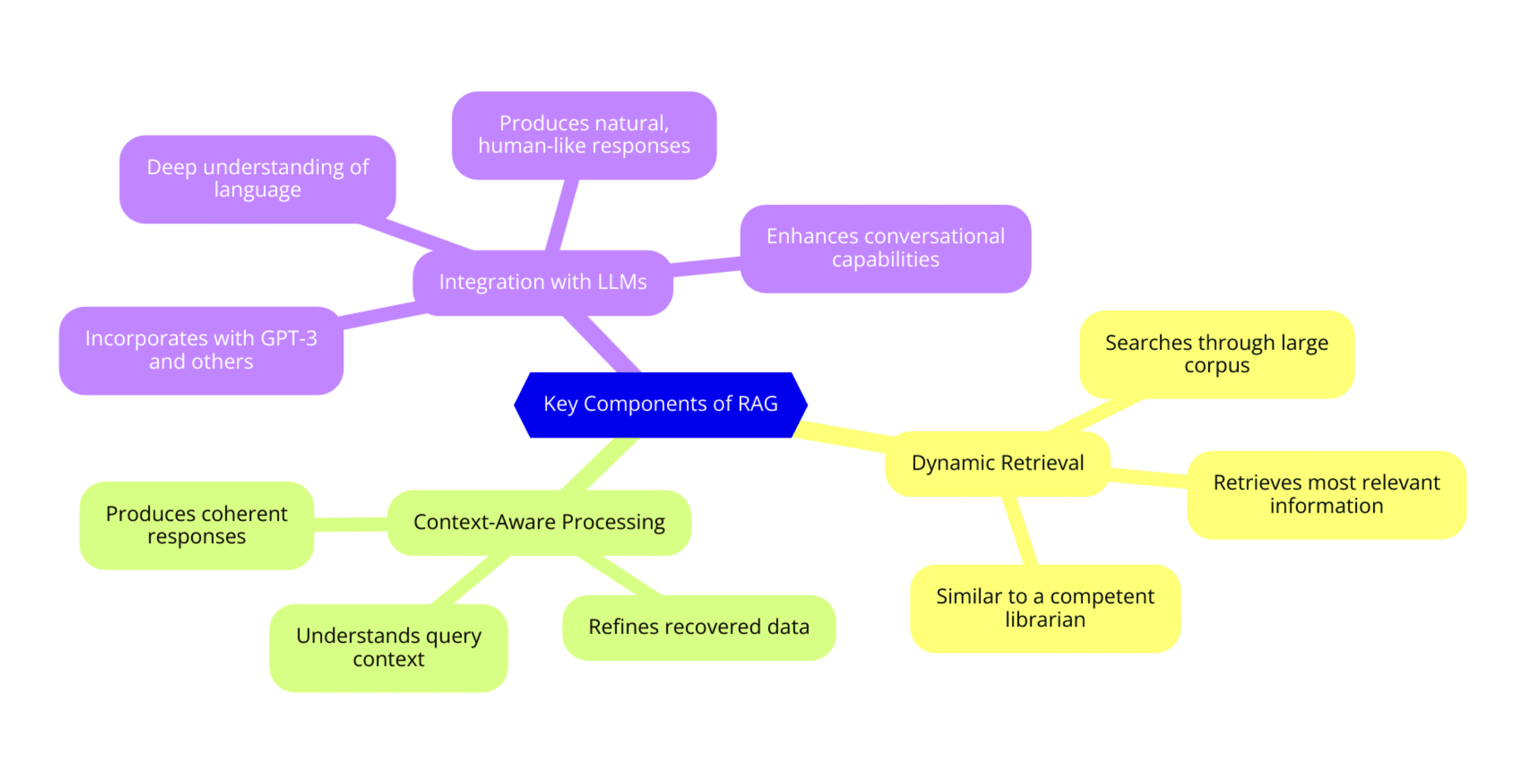
To entirely learn how RAG works, let’s break down its key components:
Dynamic Retrieval: This is the initial step, where the system searches through a huge corpus of information to retrieve the most relevant information. It resembles a competent librarian promptly locating the exact book or documents you need.
Context-Aware Processing: Once the pertinent data is recovered, the system refines it in a way that comprehends the context of your query. It ensures that the produced response is not just an arbitrarily collection of facts but a coherent and contextually apt response.
Integration with Large Language Models (LLMs): RAG incorporates smoothly with LLMs such as GPT-3. These models have a deep comprehension of language, enabling them to produce natural, human-like responses. The incorporation with LLMs permits RAG to use their conversational capabilities, improving the overall quality of the produced content.
Path of Development Since Its Creation by Meta Research in 2020
RAG’s expedition began in 2020, established by Meta Research (formerly Facebook AI). Since then, it has developed substantially. Initially, Meta Research designed RAG to improve the precision and pertinence of AI-produced responses, and it has since undergone numerous iterations to enhance its effectiveness and reliability.
Early versions concentrated on fine-tuning the dynamic recovery procedure, ensuring that the system could retrieve the most relevant information rapidly. Subsequent evolutions aimed to improve the context-aware refining abilities, making the responses more coherent and contextually felicitous.
Today, RAG stands as a sturdy structure that incorporates smoothly with LLMs, providing exceptional precision and pertinence in data retrieval and generation.
As you traverse the potential of RAG, you will find its applications in several fields, from customer assistance and education to investigation and content creation. Its capability to merge real-time data retrieval with advanced language generation makes it a groundbreaker in the AI world.
Now that we have a firm grasp on how RAG functions, let's dive into some real-world applications that showcase its true impact.
Practical Use Cases of RAG (Retrieval-Augmented Generation)
Document Question Answering Systems: Enhancing Access to Proprietary Documents
Envisage having an enormous base of proprietary documents and requiring precise details from them swiftly. Document Question Answering System, powered by RAG, can transform this process. By asking queries in natural language, you can promptly recover specific responses from your documents, saving time and enhancing effectiveness.
Conversational Agents: Customizing LLMs to Specific Guidelines or Manuals
Conversational agents can become even more efficient when customized to precise instructions or manuals. With RAG, you can tailor language models to adhere to concrete conventions and industry standards. This ensures that the AI interacts with precision while complying with specific needs.
Real-time Event Commentary with Live Data and LLMs
For live events, giving real-time commentary is critical. RAG can connect language models to live data feeds, permitting you to produce up-to-minute reports that improve the virtual experience. Whether it’s a sports game, a meeting, or a breaking news story, RAG keeps your audience engaged with the newest updates.
Content Generation: Personalizing Content and Ensuring Contextual Relevance
Generating customized content that reverberates with your audience can be challenging. RAG helps by using real-time data to create content that is not only pertinent but also gradually customized. This ensures that your readers find your content appealing and valuable, elevating your content’s efficiency.
Personalized Recommendation: Evolving Content Recommendations through LLMs
RAG can revolutionize how you provide customized suggestions. By incorporating retrieval mechanisms and language models, you can offer suggestions that develop based on user interactions and choices. This dynamic approach ensures that your suggestions remain pertinent and customized over time.
Virtual Assistants: Creating More Personalized User Experiences
Virtual Assistants equipped with RAG abilities can provide gradually customized user experiences. They can recover pertinent details and produce answers that serve specifically to the user’s requirement and context. This makes interactions more relevant and improves user contentment.
Customer Support Chatbots: Providing Up-to-date and Accurate Responses
Customer support chatbots need to deliver precise and prompt responses. With RAG, your chatbots can attain the most latest information, ensuring they give dependable and up-to-date details. This enhances customer service standards and decreases answering duration.
Business Intelligence and Analysis: Delivering Domain-specific, Relevant Insights
In the scenario of Business Intelligence, RAG can be a groundbreaker. By delivering domain-specific perceptions, RAG enables you to make informed decisions based on the newest and most pertinent information. This improves your inquisitive abilities and helps you stay ahead in your industry.
Healthcare Information Systems: Accessing Medical Research and Patient Data for Better Care
Healthcare professionals can take advantage of RAG by attaining medical investigation and patient records efficiently. RAG permits for swift recovery of relevant details, helping in better curing and treatment plans, eventually enhancing patient care.
Legal Research and Compliance: Assisting in the Analysis of Legal Documents and Regulatory Compliance
Legitimate professionals can use RAG to sleek the inspection of legitimate documents and ensure regulatory compliance. By recovering and producing pertinent legitimate data, RAG helps in comprehensive investigation and compliance checks, making legitimate processes more effective and precise.
And that's not all—RAG's utility is expanding into even more advanced, specialized areas. Check out some next-level use cases.
Advanced RAG Use Cases
Gaining Insights from Sales Rep Feedback
Imagine turning your sales representative’ remarks into gold mines of applicable insights. You can use Retrieval-Augmented Generation (RAG) to dissect sales feedback. By involuntarily classifying and amalgamating responses, you can pinpoint trends, common problems and opportunities.
This permits you to cautiously acknowledge concerns, customize your approach to customer requirements, and eventually drive better customer success results. It’s like having a 24/7 annotator that turns every piece of response into planned insights.
Medical Insights Miner: Enhancing Research with Real-Time PubMed Data
Stay ahead in medical investigation by pounding into real-time information from PubMed using RAG. This tool permits you to constantly observe and extract pertinent research discoveries, keeping you updated with the newest evolutions.
By incorporating these perceptions into your research process, you can improve the quality and promptness of your studies. This approach boosts discovery, helps in pinpointing emerging trends, and ensures that your work stays at the cutting edge of medical science.
L1/L2 Customer Support Assistant: Improving Customer Support Experiences
Elevate your customer support experience by using RAG to assist your L1 and L2 support teams. This tool can rapidly recover and present pertinent solutions from a wide knowledge base, ensuring that your support agents always have the correct data at their fingertips. By doing so, you can decrease response duration, increase solution rates, and improve overall customer contentment. It’s like giving your support team a significant support that never sleeps and always has the answers.
Compliance in Customer Contact Centers: Ensuring Behavior Analysis in Regulated Industries
Ensure your customer centers follow regulatory requirements using RAG. This tool can dissect interactions for compliance, discerning any divergence required conventions.
By giving real-time responses and recommendations, you can acknowledge problems instantly, ensuring that your functioning remains within the bounds of industry regulations. This proactive approach not only helps in sustaining compliance but also builds trust with your customers and investors.
Employee Knowledge Training Assessment: Enhancing Training Effectiveness Across Roles
Revolutionize your employee training programs with RAG. By inspecting training materials and employee responses, you can pinpoint gaps in knowledge and areas for enhancement.
This tool helps in customizing training sessions to acknowledge precise requirements, ensuring that employees across all roles receive the most efficient and pertinent training. By constantly evaluating and processing your training programs, you can elevate workflow, improve expertise, and ensure that your employees are always prepared to meet new challenges.
Global SOP Standardization: Analyzing and Improving Standard Operating Procedures
Sleek your worldwide operations by homogenizing your Standard Operating Procedures (SOPs) with RAG. This tool can dissect SOPs from distinct regions, dissect inconsistencies, and recommend enhancements.
By ensuring that all your SOPs are aligned and upgraded, you can improve functioning effectiveness, reduce errors, and ensure congruous quality across your organization. It’s like having a universal process examiner that ensures every process is up to par.
Operations Support Assistant in Manufacturing: Assisting Technical Productivity with Complex Machinery Maintenance
Improve your manufacturing operations with an RAG-powered support assistant. This tool can aid in sustaining intricate machinery by offering real-time troubleshooting and preserving data.
By rapidly recovering and presenting pertinent technical data, you can reduce interruption, enhance workflow, and lengthen the lifespan of your equipment. This approach ensures that your technical workforce always has the details they need to keep your operations running sleekly.
Of course, implementing RAG comes with its own set of best practices and considerations, and we'll explore those next.
Implementing RAG - Best Practices and Considerations
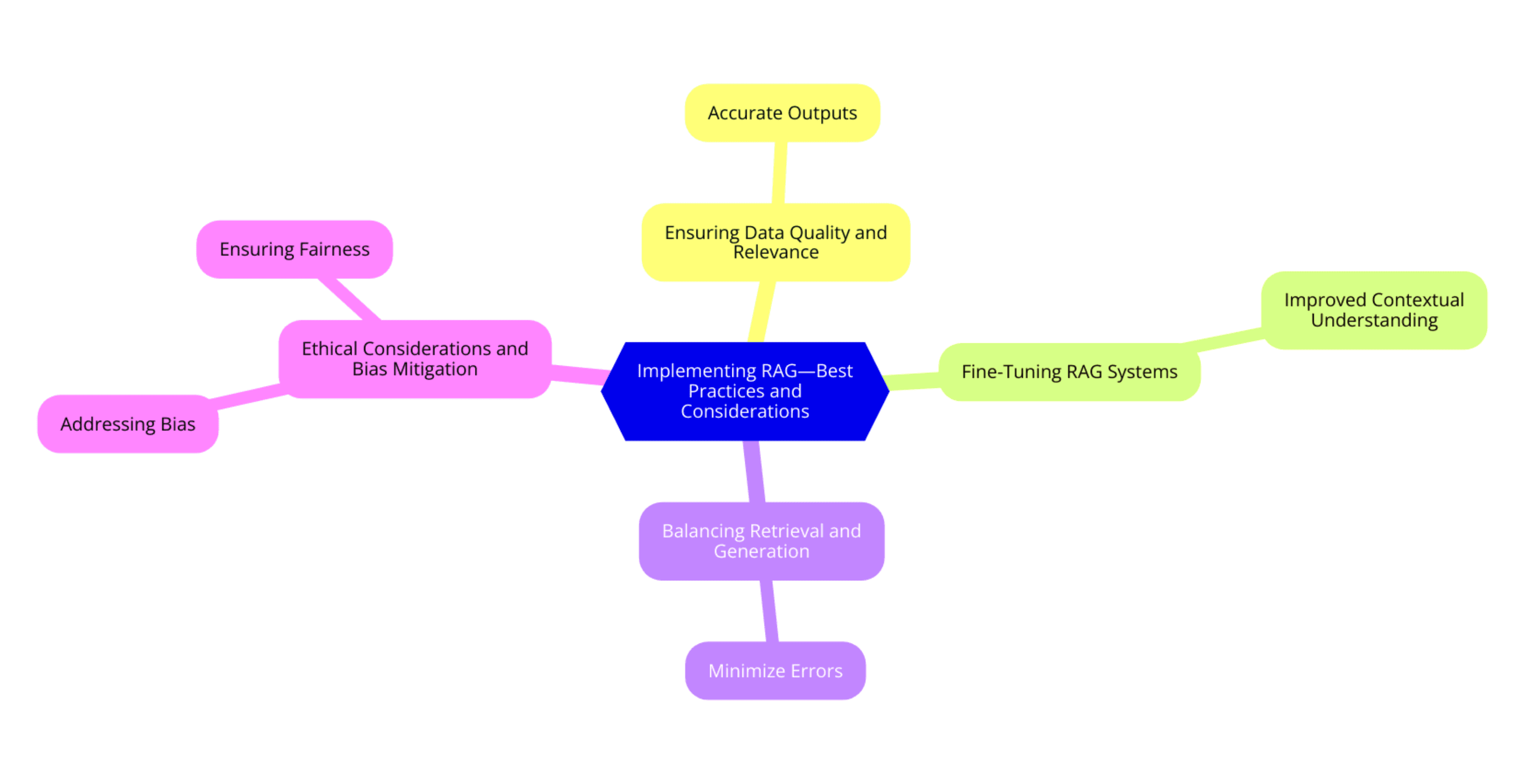
Ensuring Data Quality and Relevance for Accurate Outputs
When enforcing RAG (Retrieval-Augmented Generation), data quality and pertinence are your top priorities. You require to begin by consolidating a high-quality data set that is relevant to your domain.
This means using dependable sources, updating your data frequently, and removing any outdated or irrelevant data. High-quality information ensures that your RAG model recovers the most precise and helpful information, which results in more accurate and dependable yields.
Fine-Tuning RAG Systems for Improved Contextual Understanding
To get the most out of your RAG system, refining is important. This involves instructing your model on domain-specific data so it can comprehend the variations and context of your queries better. Use methods such as supervised refining with interpreted information to help your model grasp the correct answers.
This step is critical for improving the contextual comprehension of your system, making its yields more pertinent and precise.
Balancing Retrieval and Generation to Minimize Errors
Locating the right balance between retrieval and generation is key to minimizing mistakes and hallucinations. Too much dependency on generation can result in fabricated data, while over-dependence on recovery can restrict the creativity and profundity of the responses. You should adapt the emphasis of recovery and generation elements based on the nature of your application. Frequently assess the yields and modify the system to ensure it gives illuminating and dependable responses.
Ethical Considerations and Bias Mitigation in RAG Implementations
Ethics and bias mitigation should be essential to your RAG enforcement procedure. Begin by inspecting your dataset for biases and ensuring a disparate range of sources. Enforcing fairness-aware algorithms can help in lessening bias in the retrieval process. In addition, it’s important to sustain clarity with users about how your system operates and the sources it uses. By concentrating on ethical contemplations, you can build trust and ensure that your RAG system provides impartial and fair data.
So, what does the future hold for RAG? Let's explore the exciting advancements and potential impacts on various industries.
Future Directions and Impact of RAG
Exploration of Multimodal Capabilities and API Access
Envisage a globe where your applications can refine not just text, but pictures, audio and video smoothly. That’s where the probing of multimodal abilities in RAG (Retrieval-Augmented Generation) is heading. By incorporating numerous data types, you improve the opulence and context of data your application can handle.
Connected with API access, you can pull real-time information from disparate sources, making your solutions more robust and receptive. This means more reciprocal and captivating user experience, where your app can describe an image, condense a video, or even communicate through voice.
Broader Applications and Enhanced User Experiences
RAGs potential isn’t restricted to a single field. You can apply it across education, finance, healthcare and beyond. Picture an informational platform that not only answers student queries but also gives comprehensive elucidations, suggests further reading, and even interrogates students based on their learning growth. In healthcare, RAG can assist doctors by recovering the latest investigation and patient record, helping them make informed decisions.
The key is the enriched user experience, where communications feel more natural and private, making technology an inherent augmentation of human abilities.
Data Integration and Innovations in Multimodal Models
Incorporating distinct types of data such as text, images, and standardized data opens new frontiers in RAG applications. This incorporation permits for more sophisticated data refining and insights generation. Innovations in multimodal models mean you can evolve applications that comprehend and produce content across multiple formats.
For example, a customer service bot could dissect a customer’s voice tone and the content of their texts to offer more compassionate and precise answers. Such innovations drive forward a more associated and perceptive digital ecosystem.
LangChain and LLM RAG: Generative Search, Data Chat, and Next-Gen Customer Service
LanChain and large language model (LLM) RAG applications are revolutionizing how you communicate with data. With generative search, your doubts return not just pertinent documents, but integrated, brief responses drawn from numerous sources.
Chatting with data means engaging in a conversation with your datasets, asking intricate questions and getting comprehensible, applicable perceptions. In customer service, next-generation applications can handle more complex queries, giving prompt, precise support and freeing up human agents for more intricate tasks. This shift towards savvy, more receptive systems marks a substantial leap in how we use the power of AI to enhance daily communications and productivity.
By clasping these future guidance, you can use RAG to create more smart, receptive and flexible applications, pushing the boundaries of what’s possible in technology today.
To cap things off, let’s look at how you can seamlessly integrate these advanced RAG platforms into your existing systems to elevate productivity and effectiveness.
Conclusion
Retrieval-Augmented Generation is a revolutionary technology assured to transform numerous industries. By using real-time information and context-aware refining, RAG ensures that AI systems deliver the most precise and pertinent information, paving the way for innovative applications and improved user experiences.
Whether it’s healthcare, legitimate research, or customer support, RAG is set to make a substantial impact, driving effectiveness and precision across disparate domains.
Explore how you can smoothly integrate advanced RAG platforms into your current enterprise systems for improved effectiveness and productivity in our thorough guide on Integration Of RAG Platforms With Existing Enterprise Systems.
Subscribe to our newsletter to never miss an update
Subscribe to our newsletter to never miss an update
Other articles
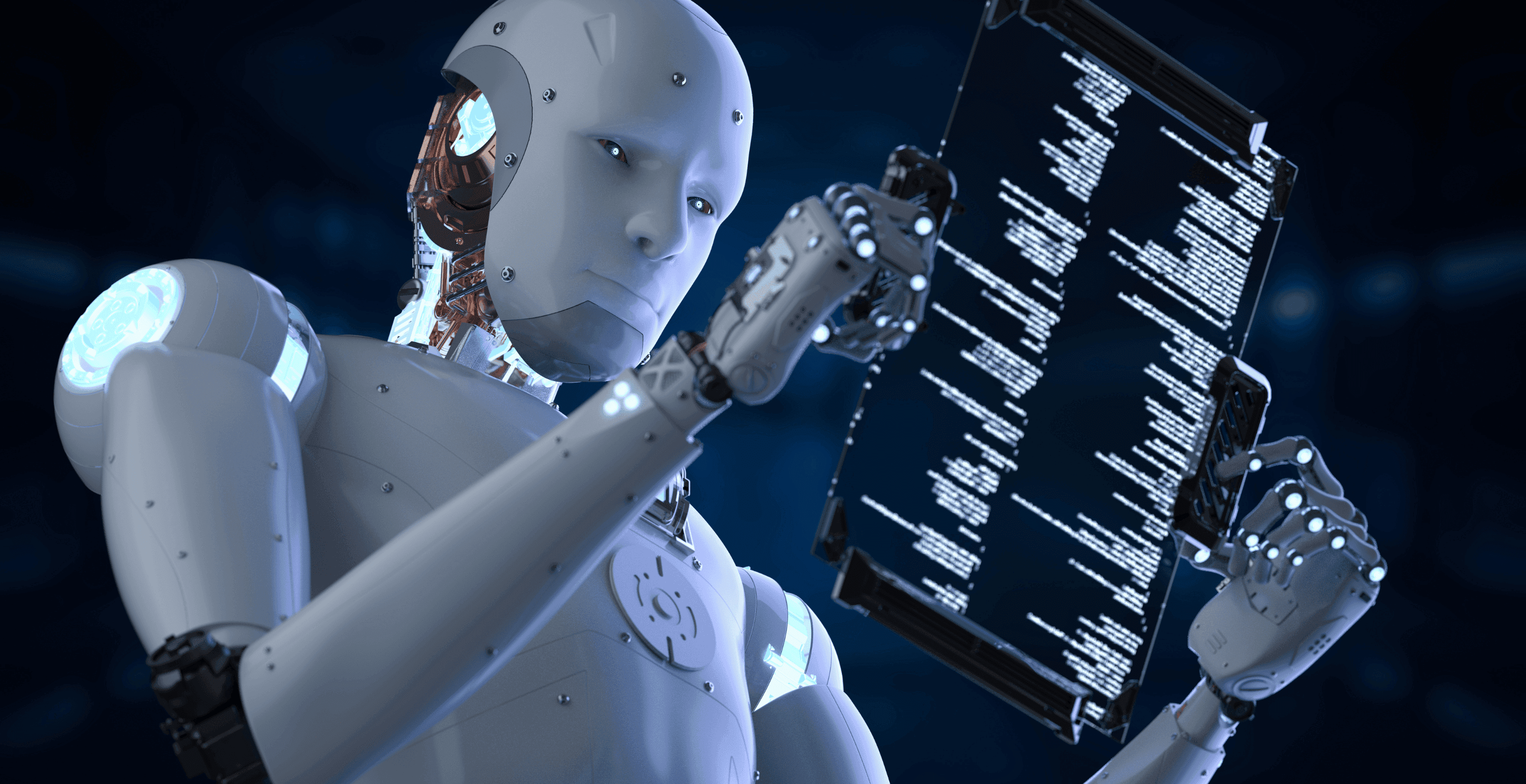
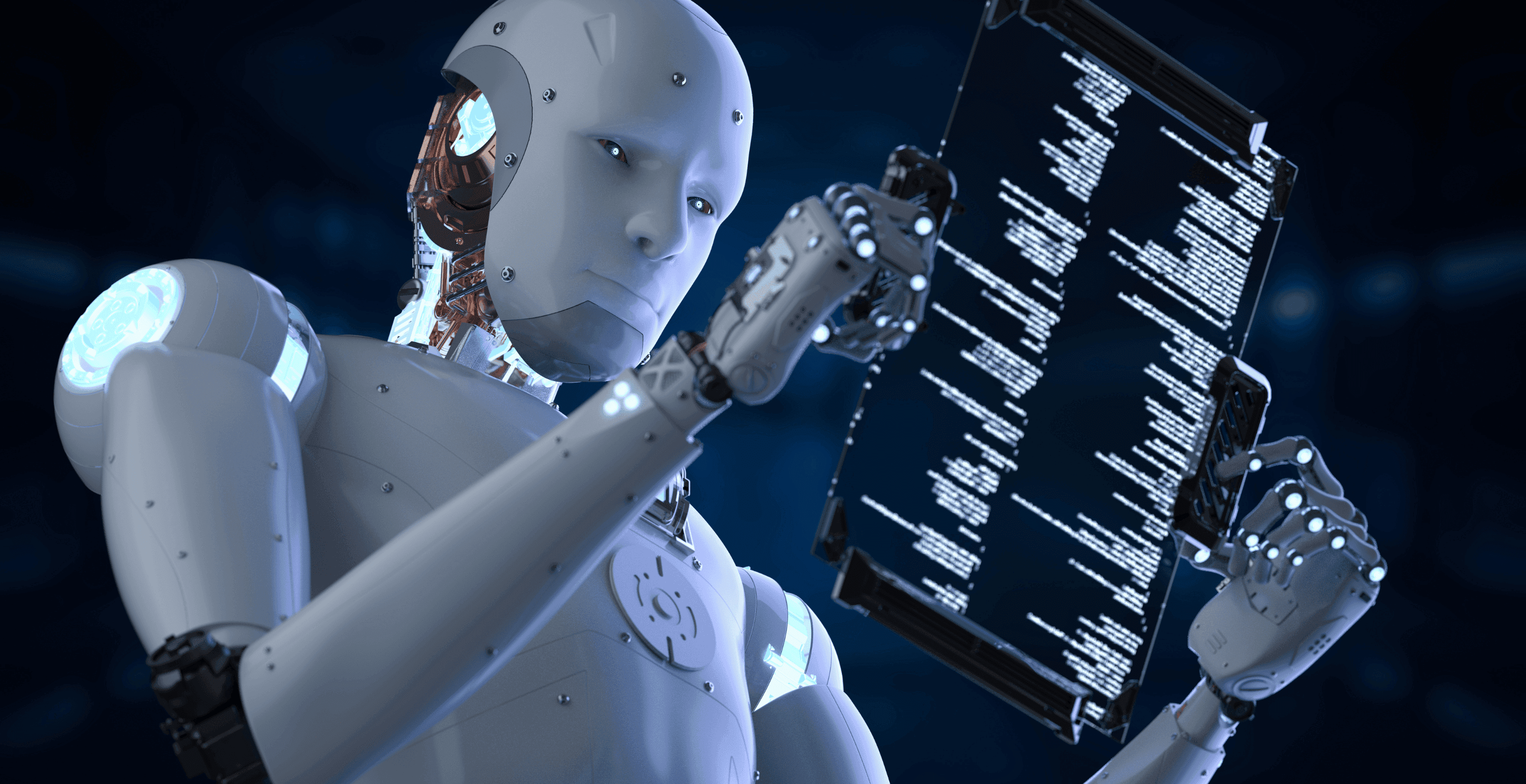
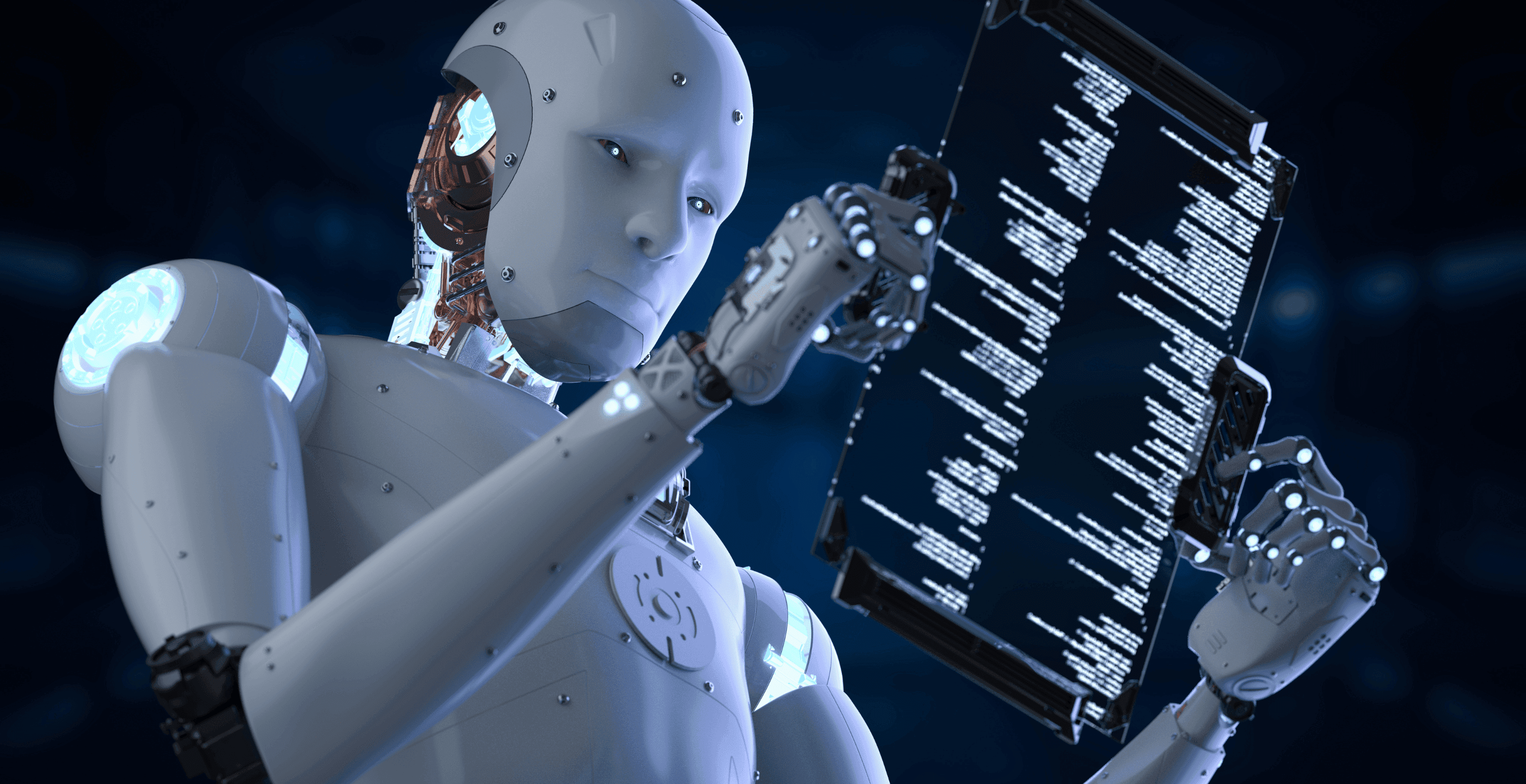
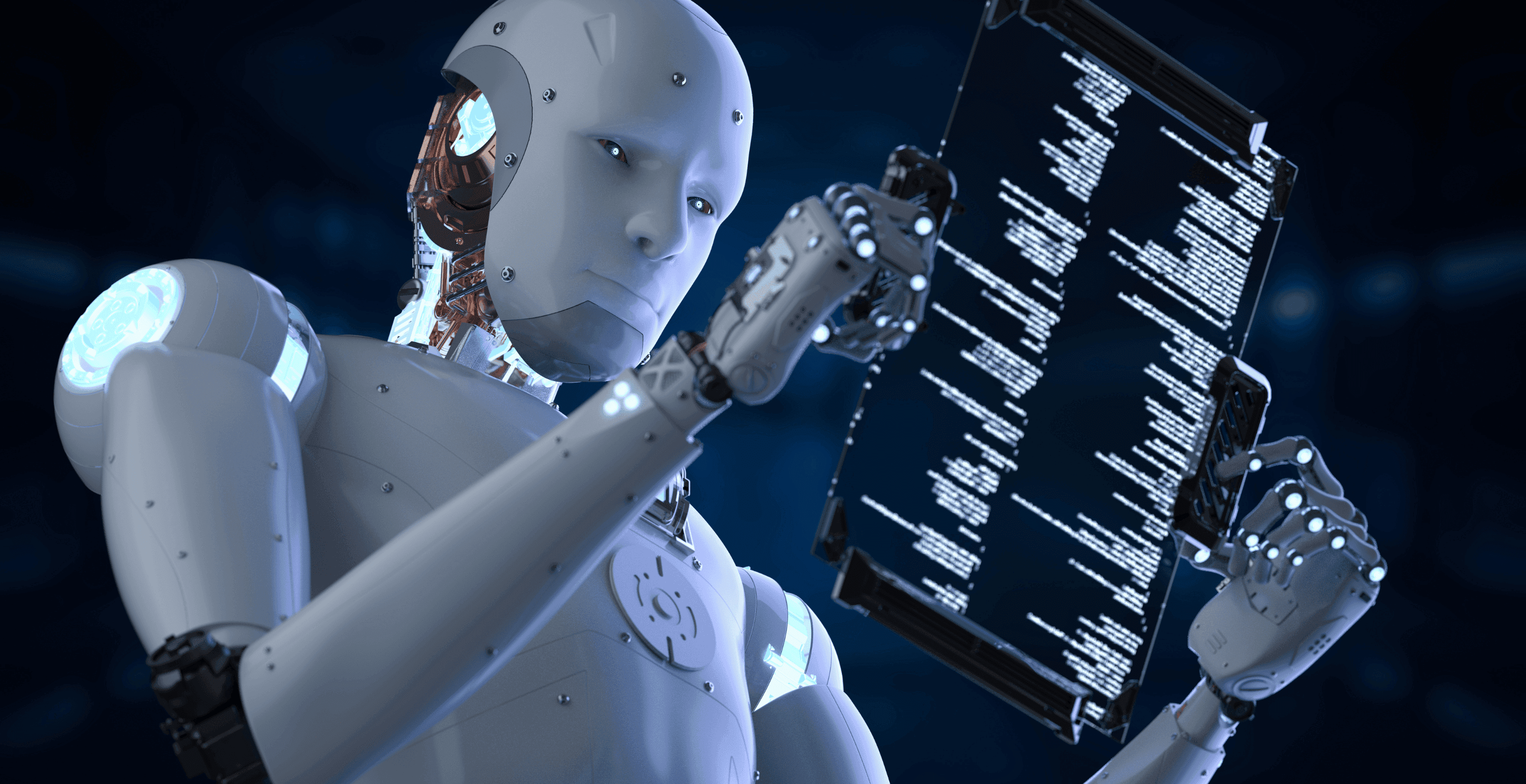
Exploring Intelligent Agents in AI

Rehan Asif
Jan 3, 2025
Read the article
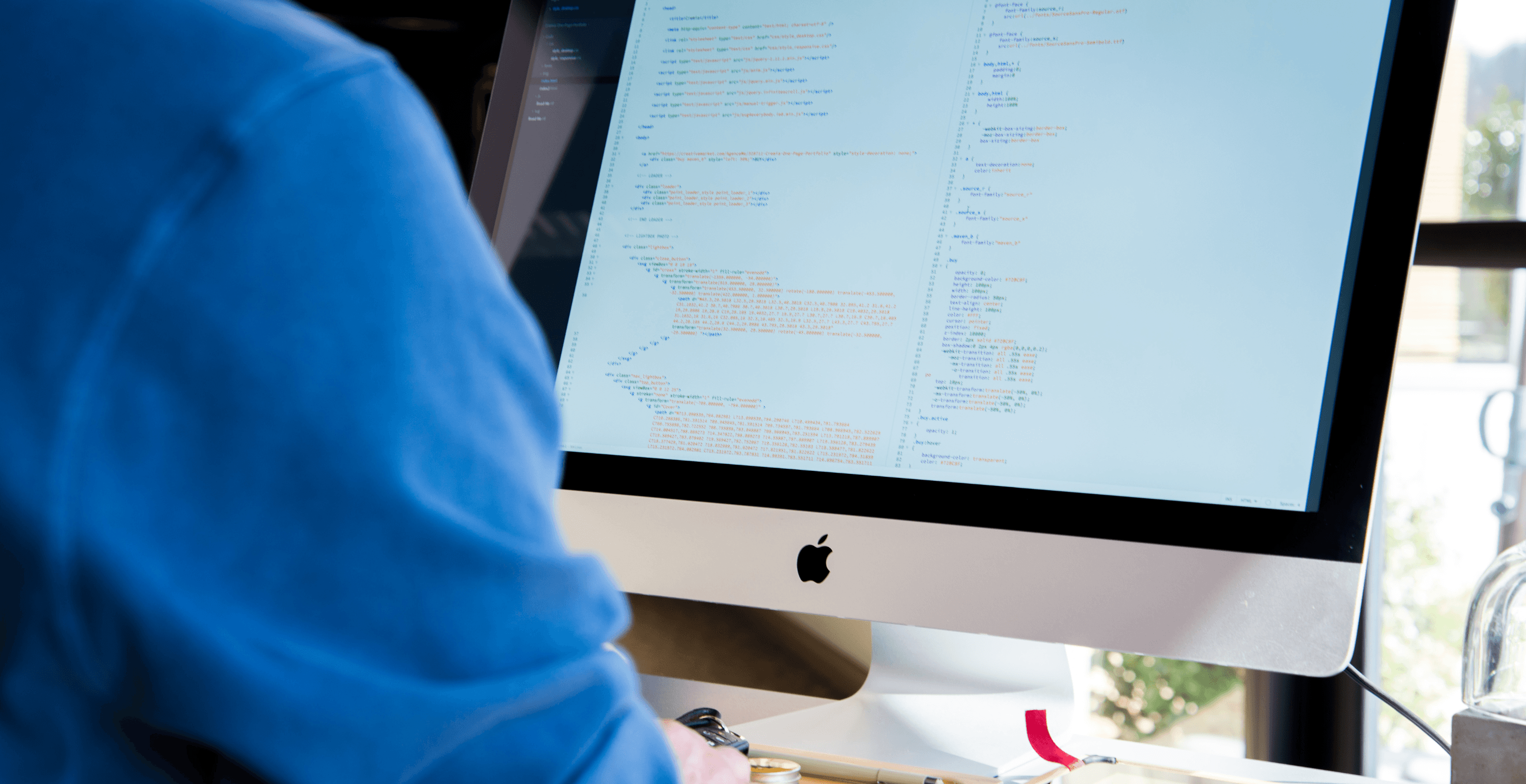
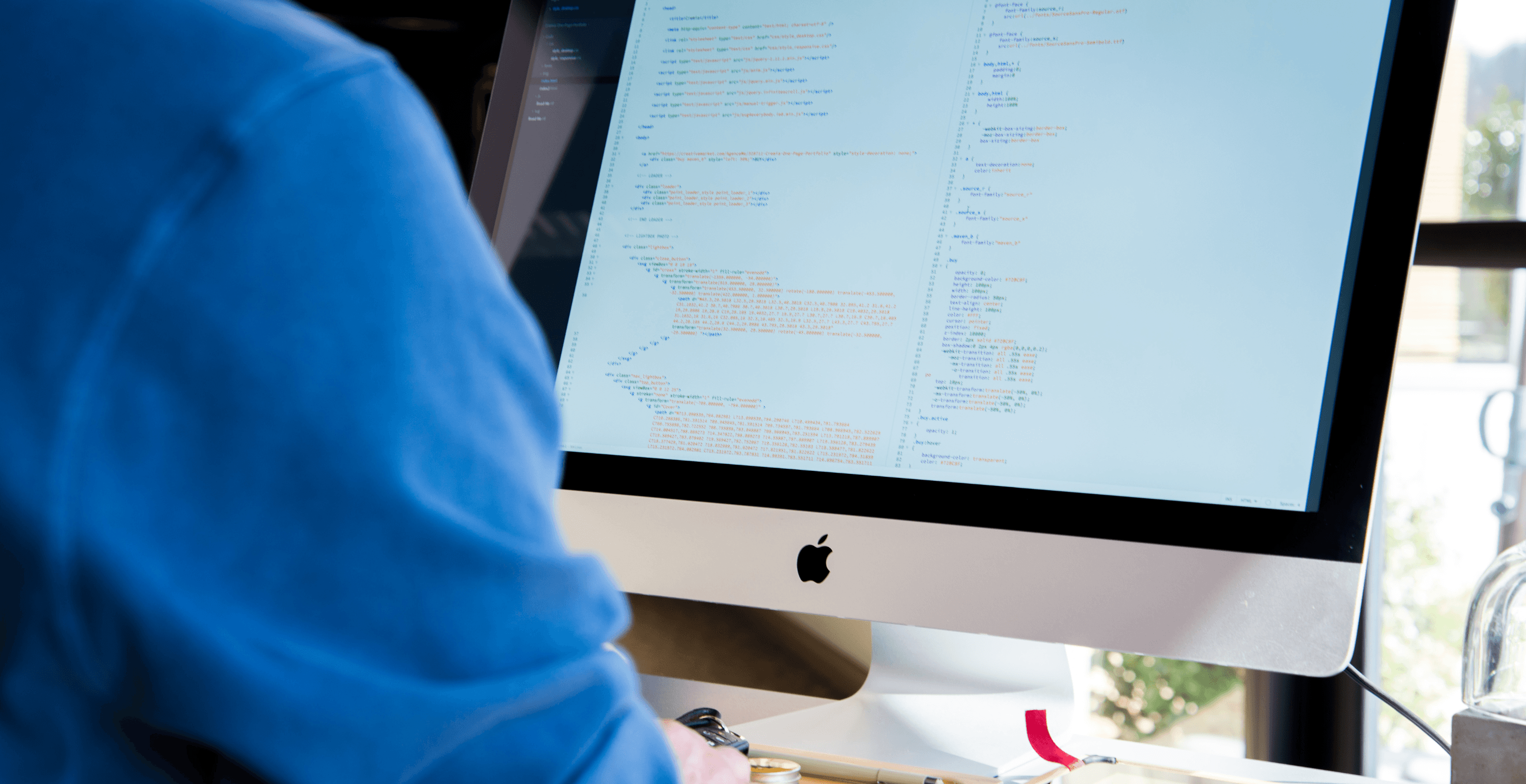
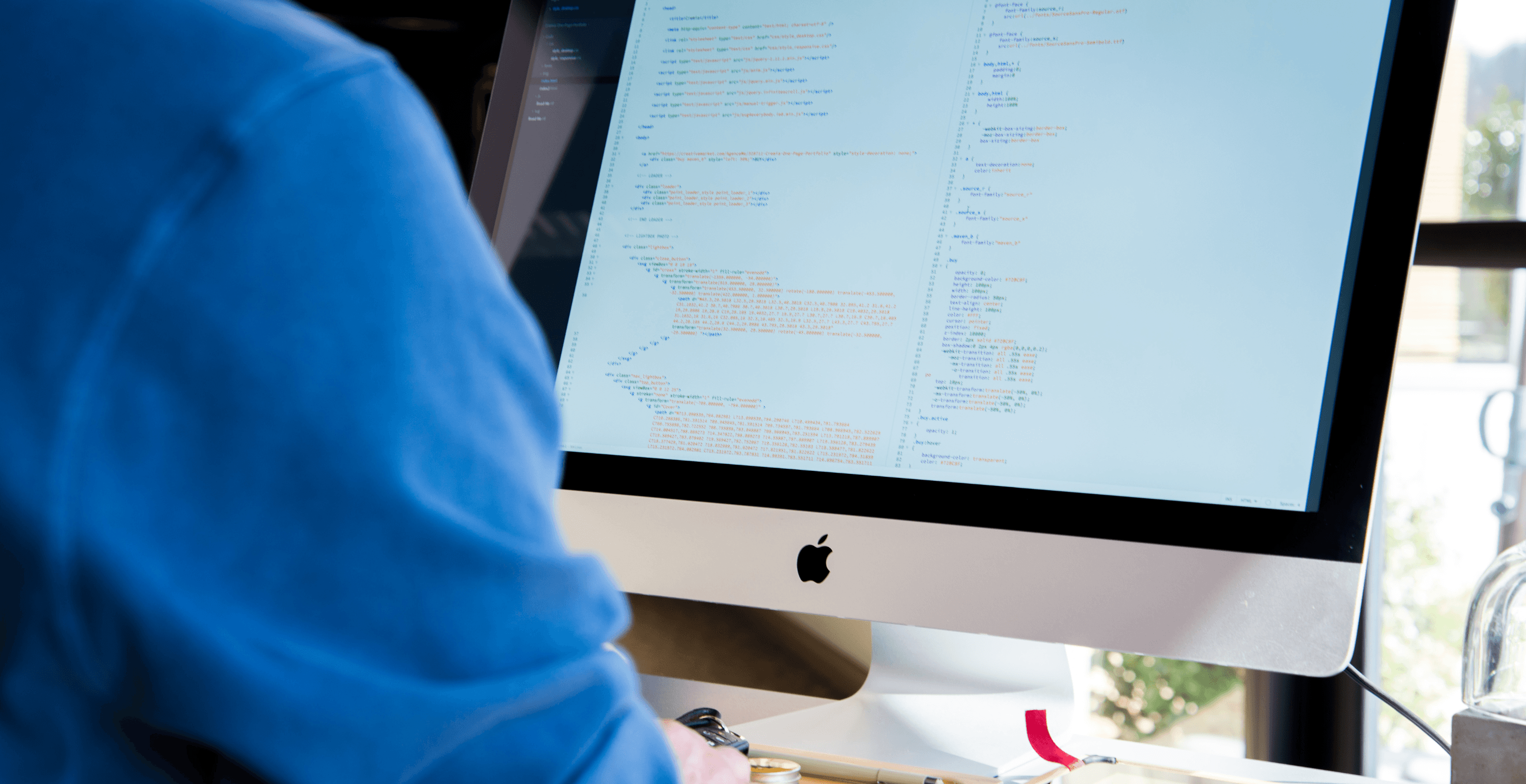
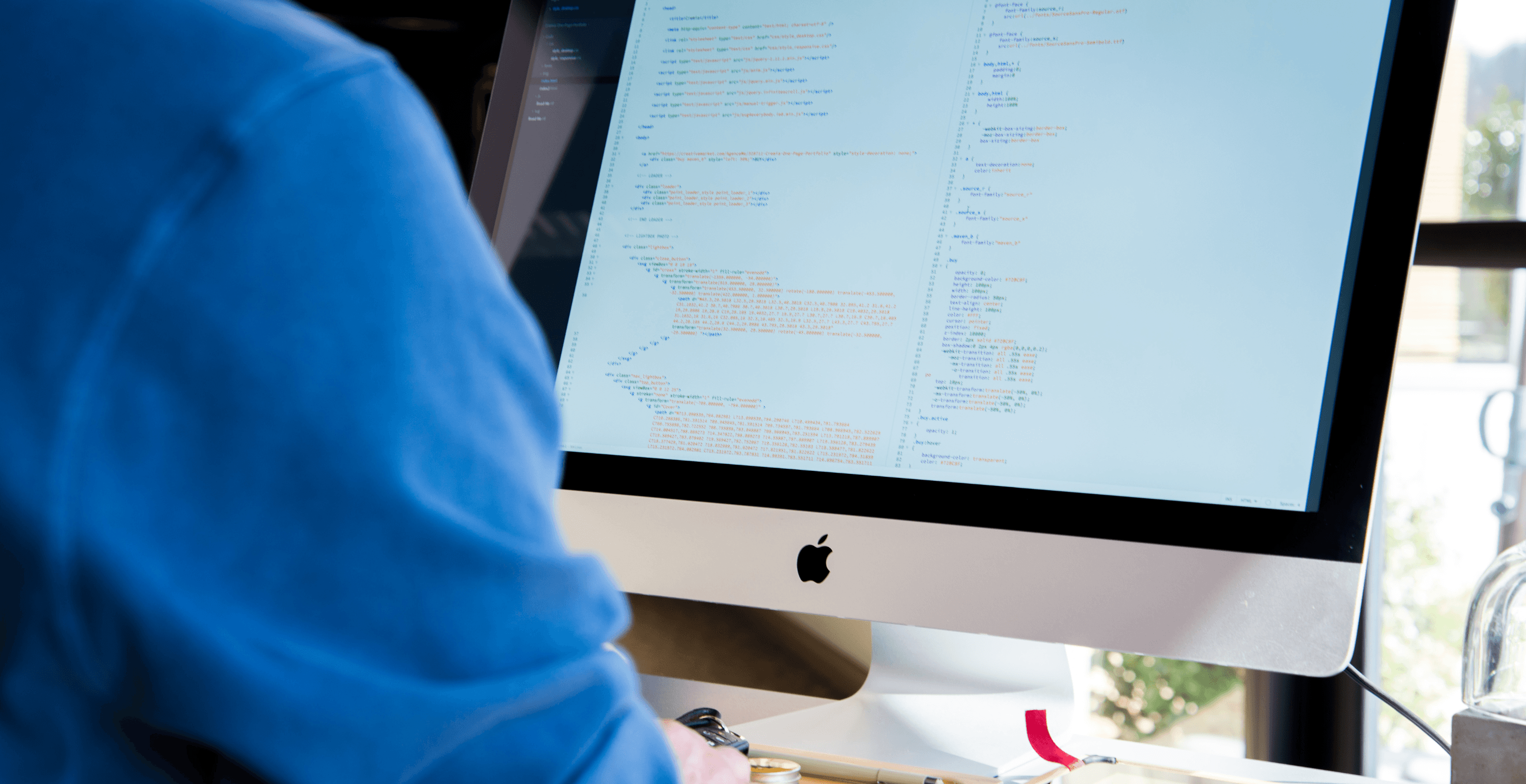
Understanding What AI Red Teaming Means for Generative Models
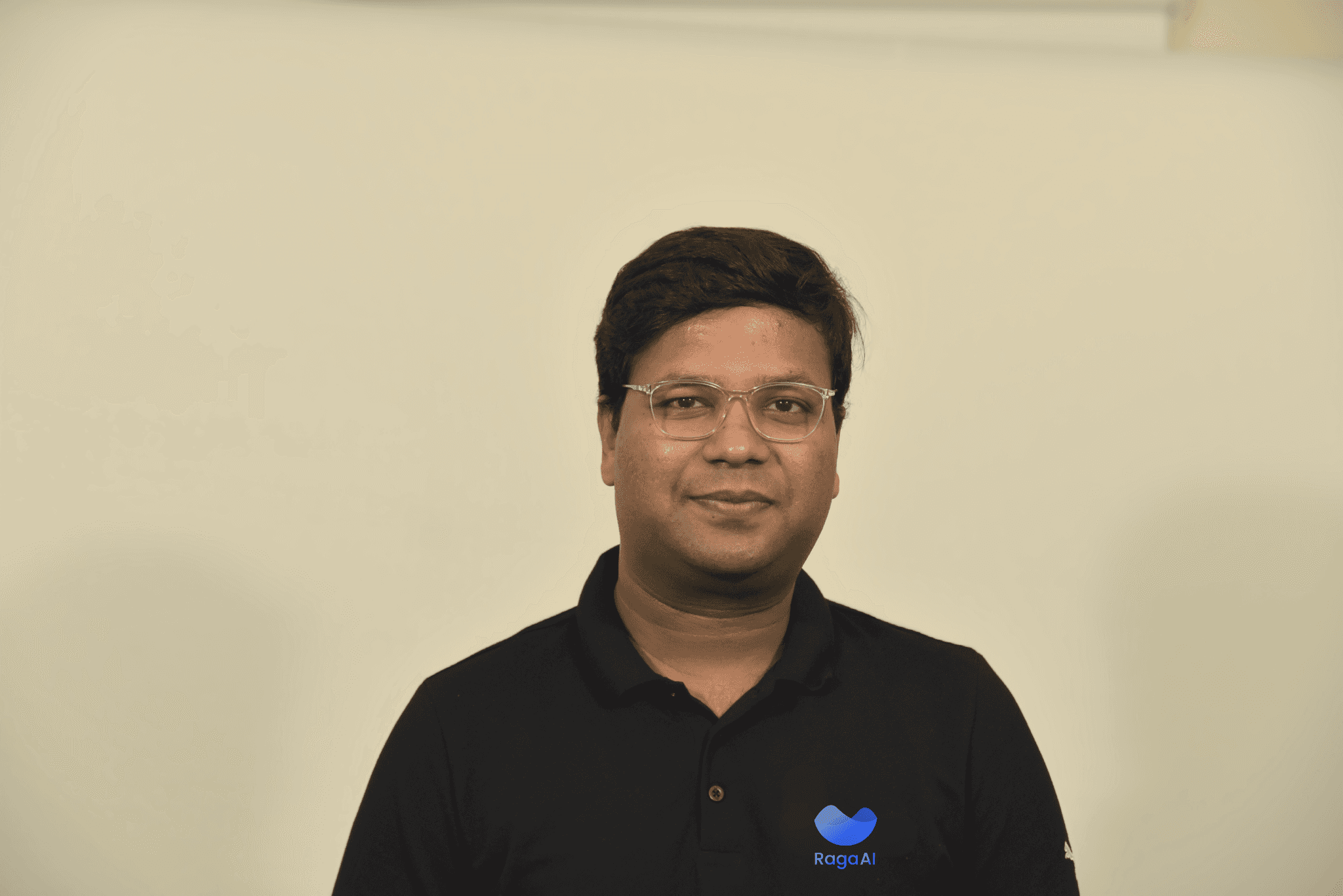
Jigar Gupta
Dec 30, 2024
Read the article
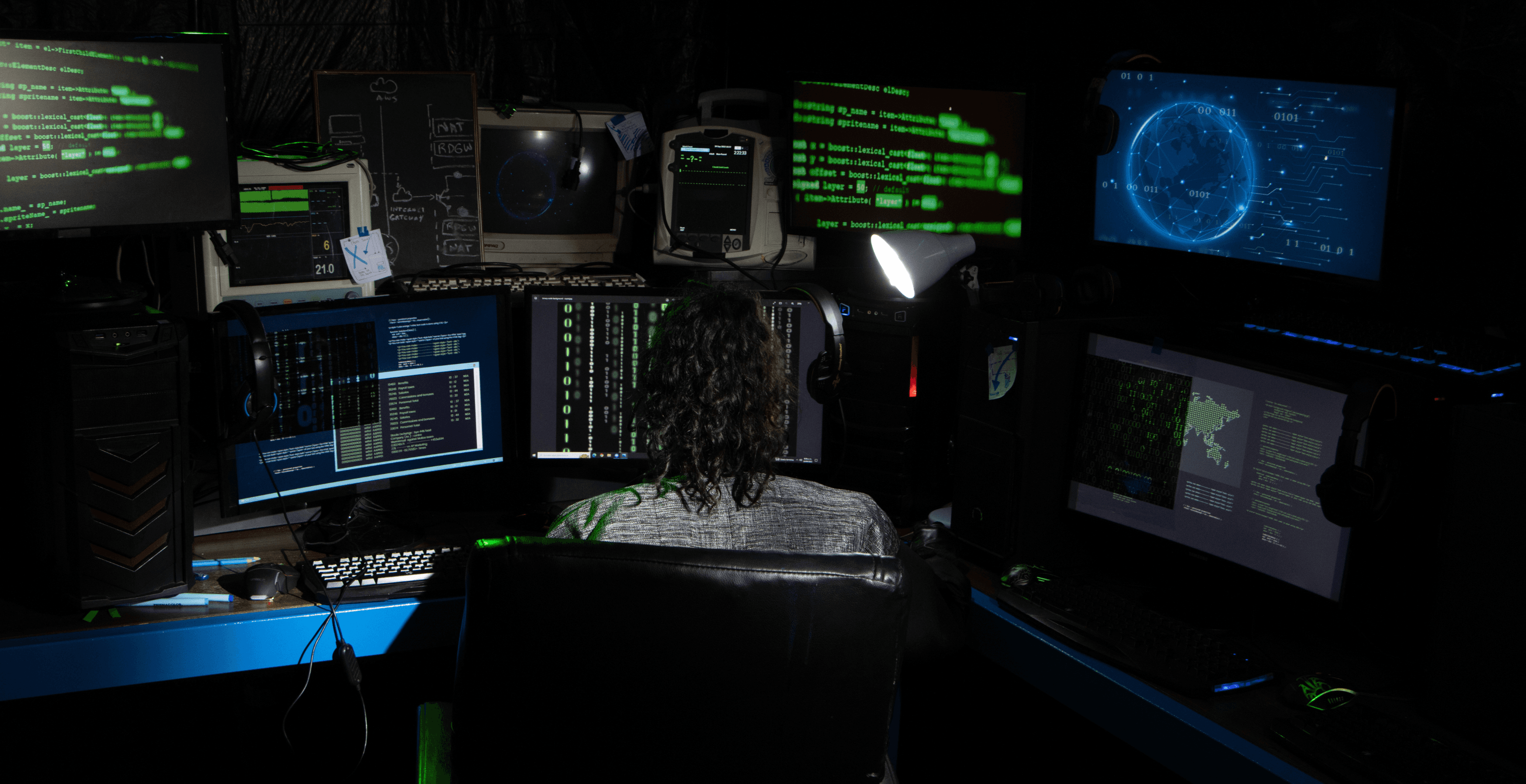
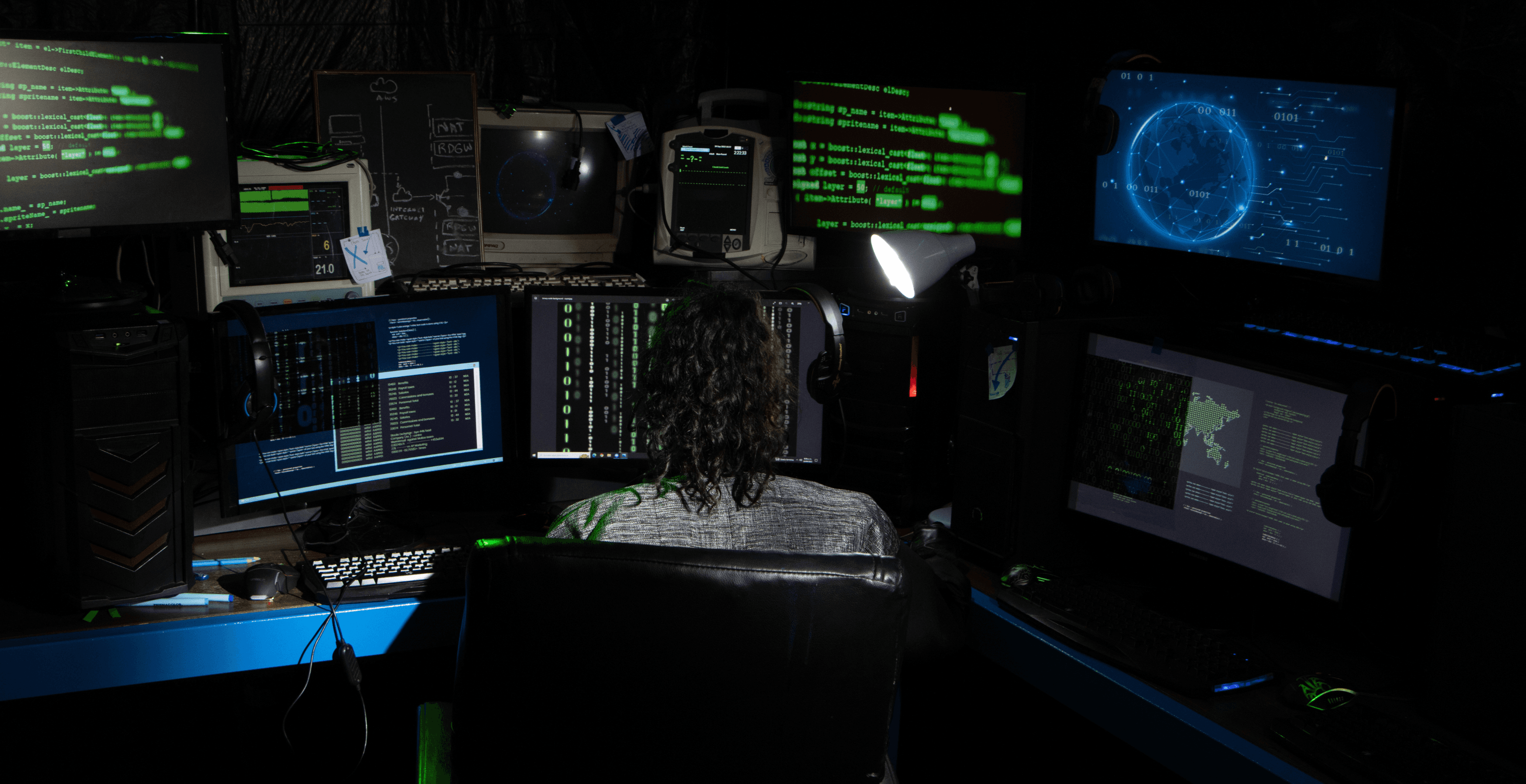
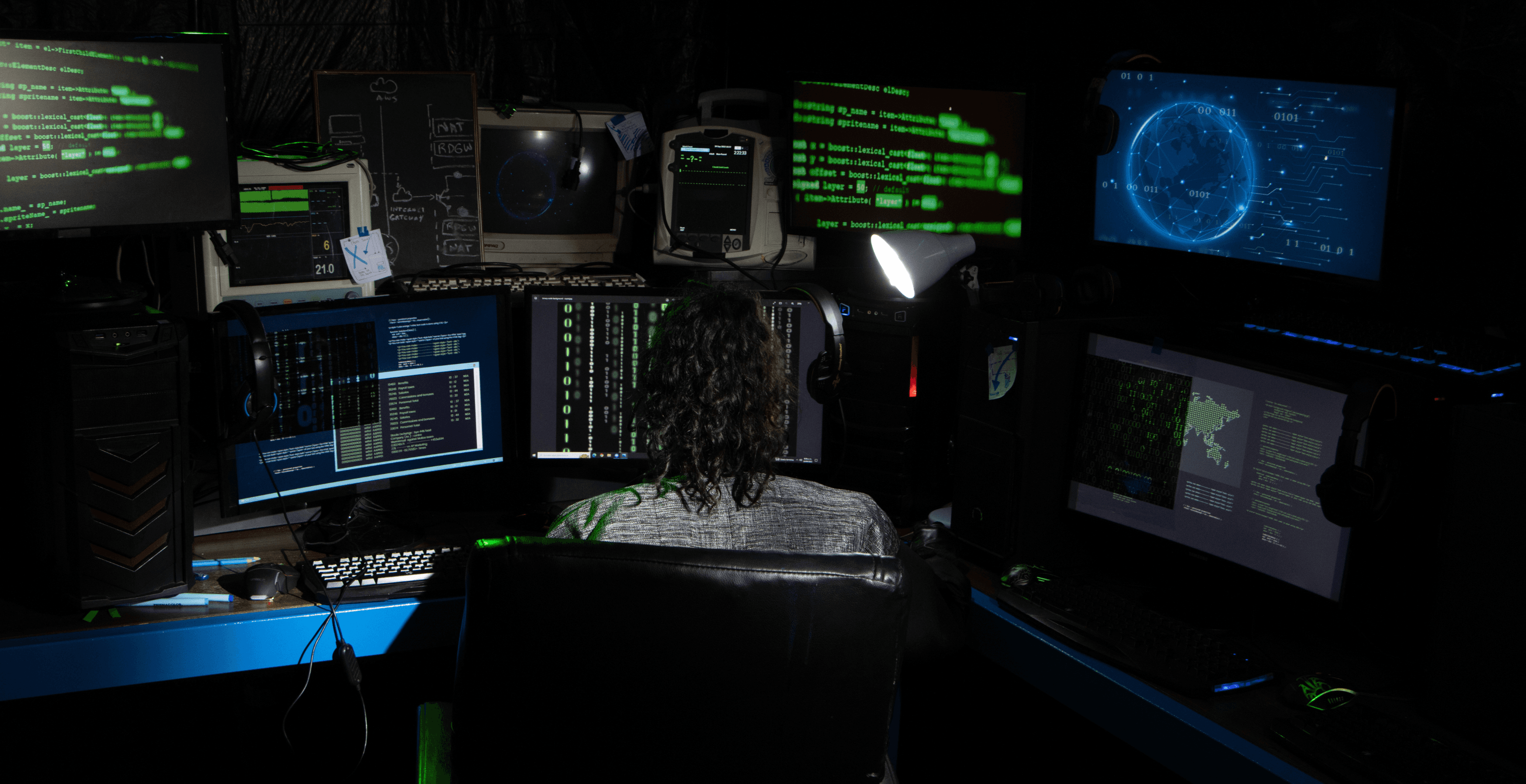
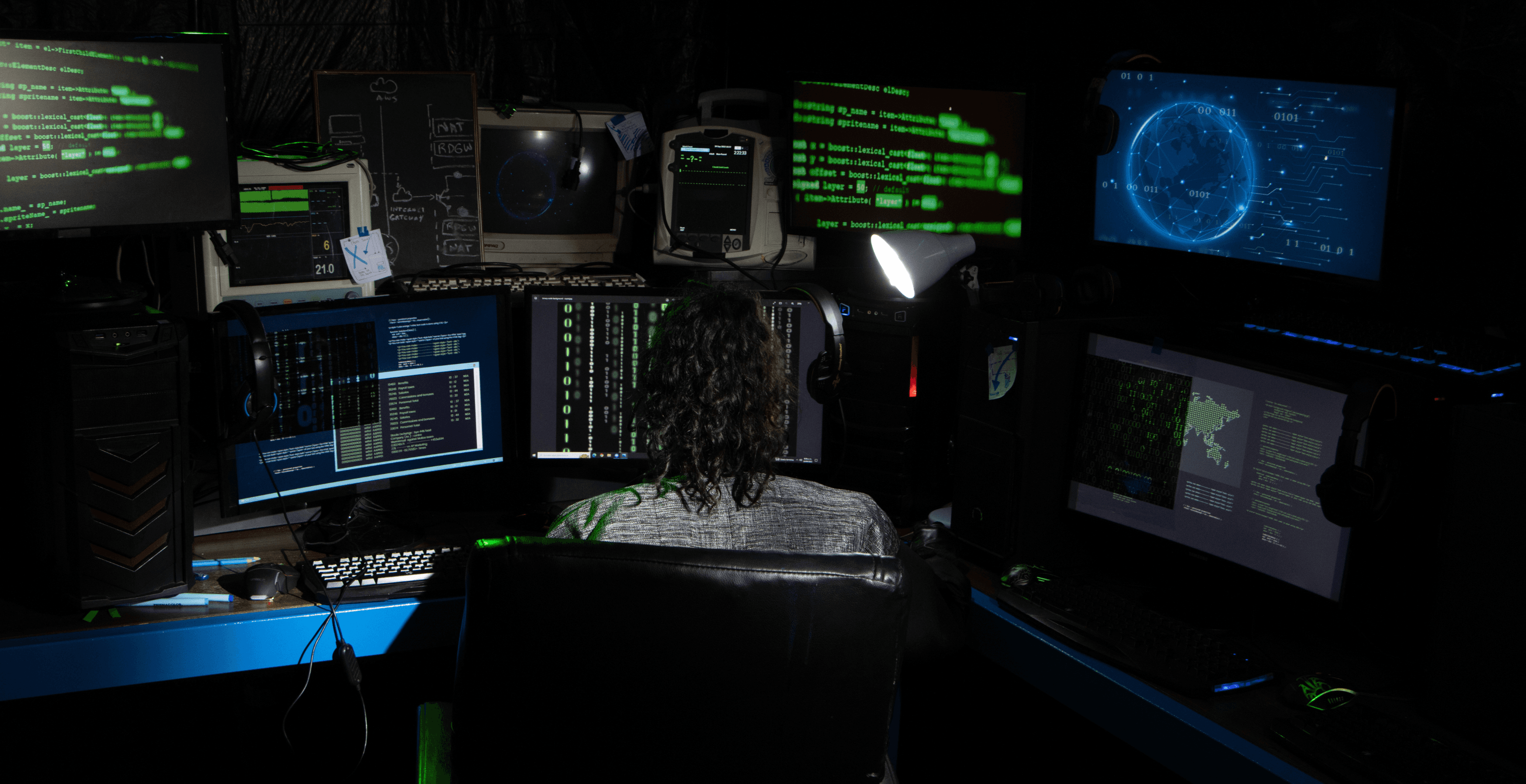
RAG vs Fine-Tuning: Choosing the Best AI Learning Technique
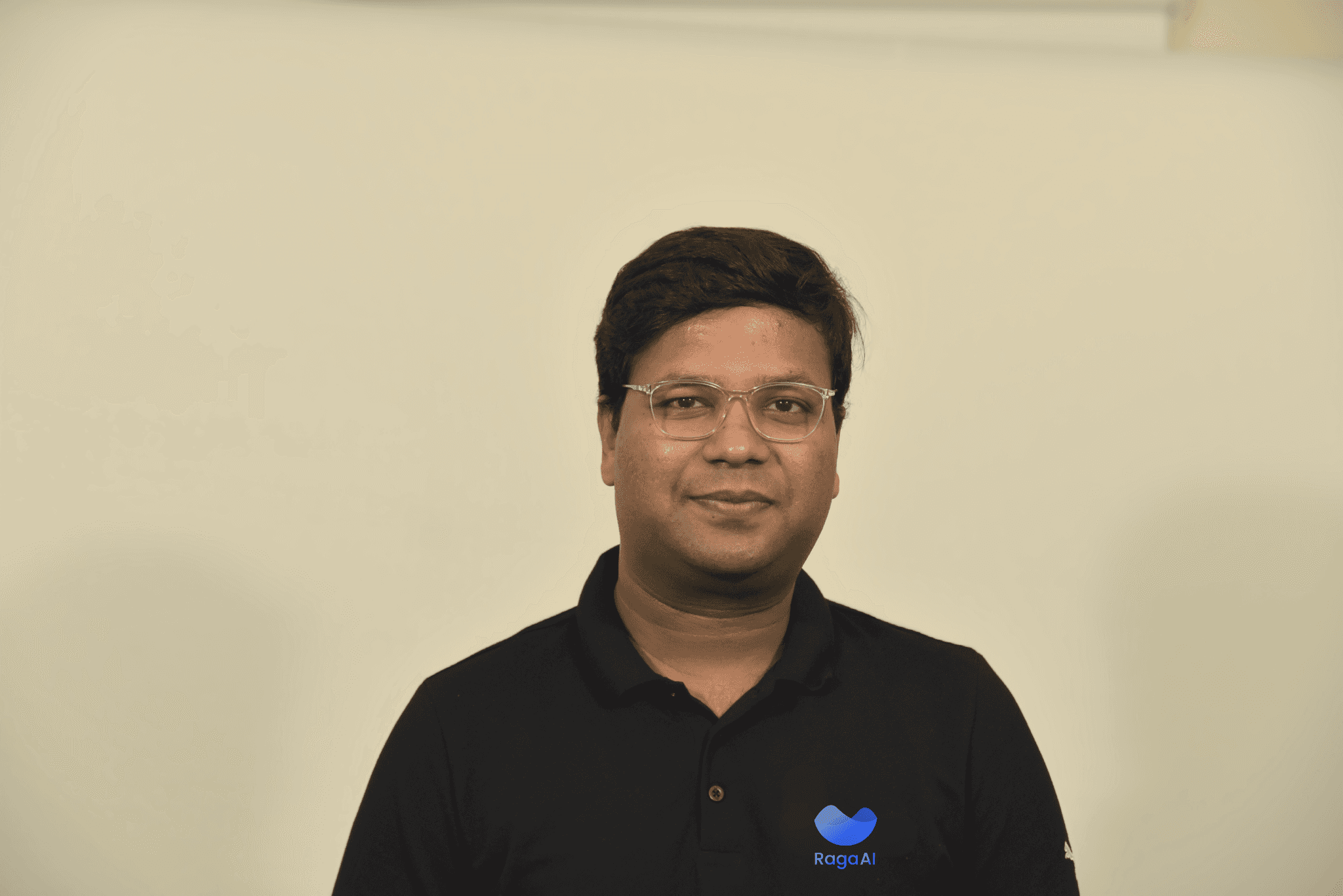
Jigar Gupta
Dec 27, 2024
Read the article
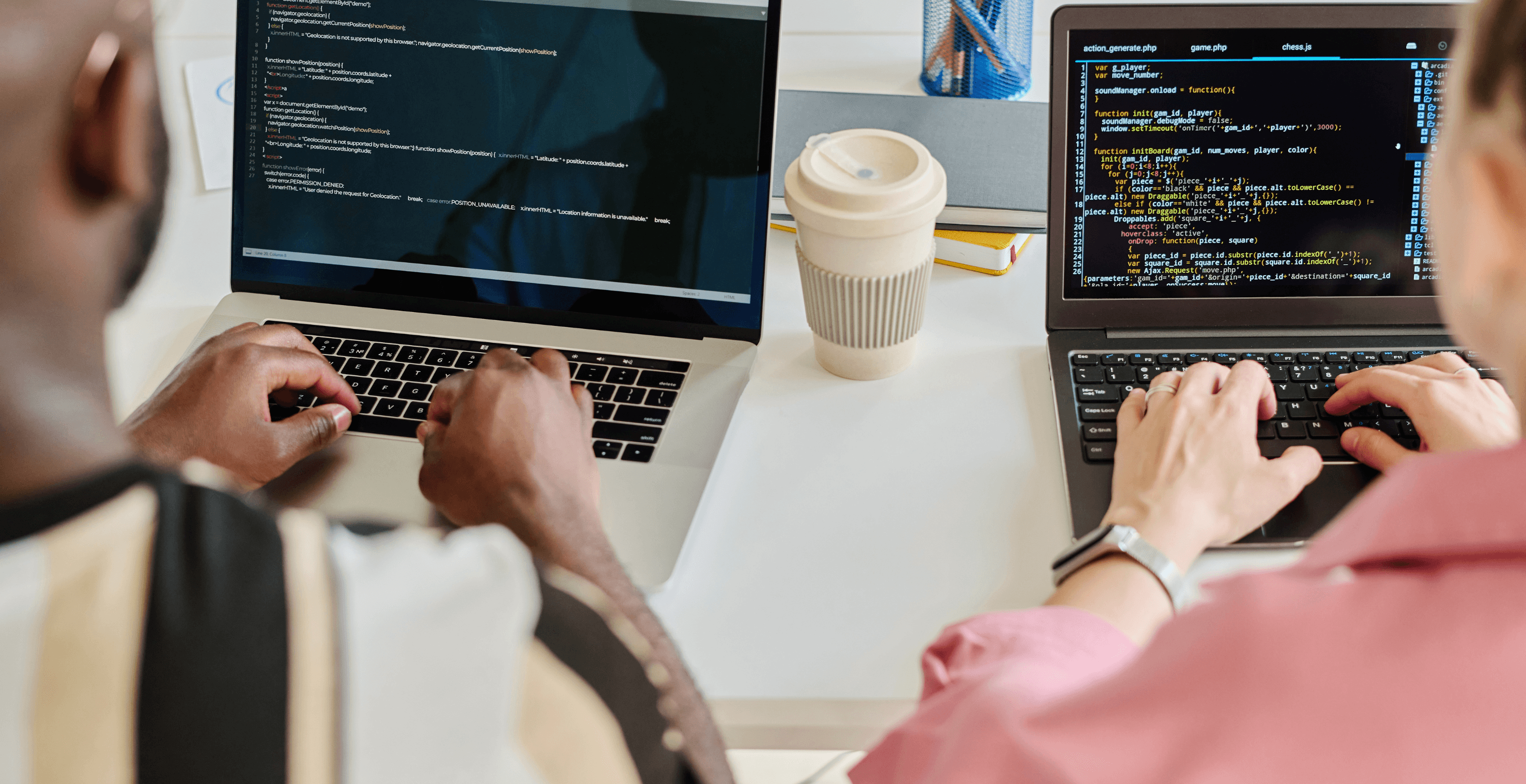
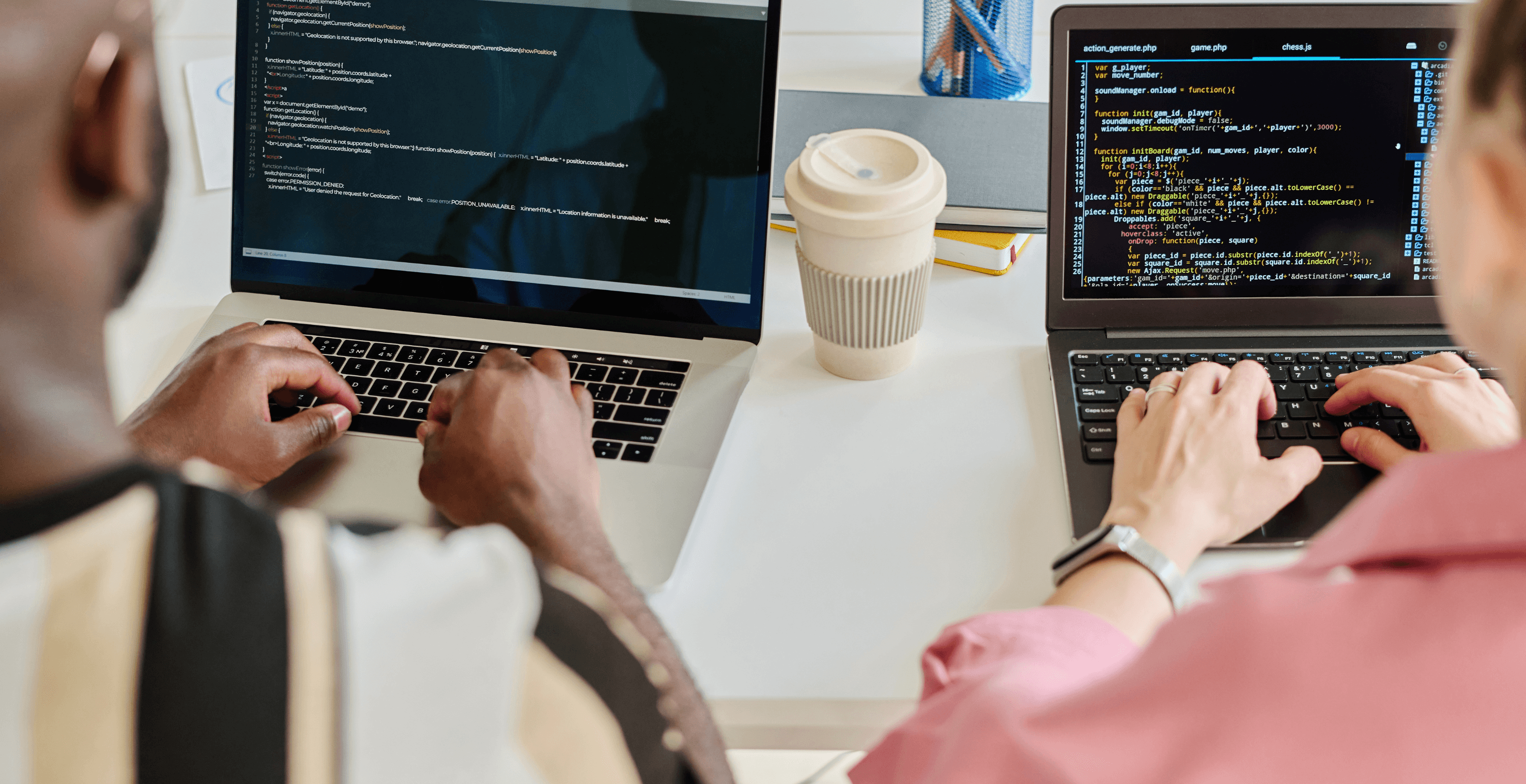
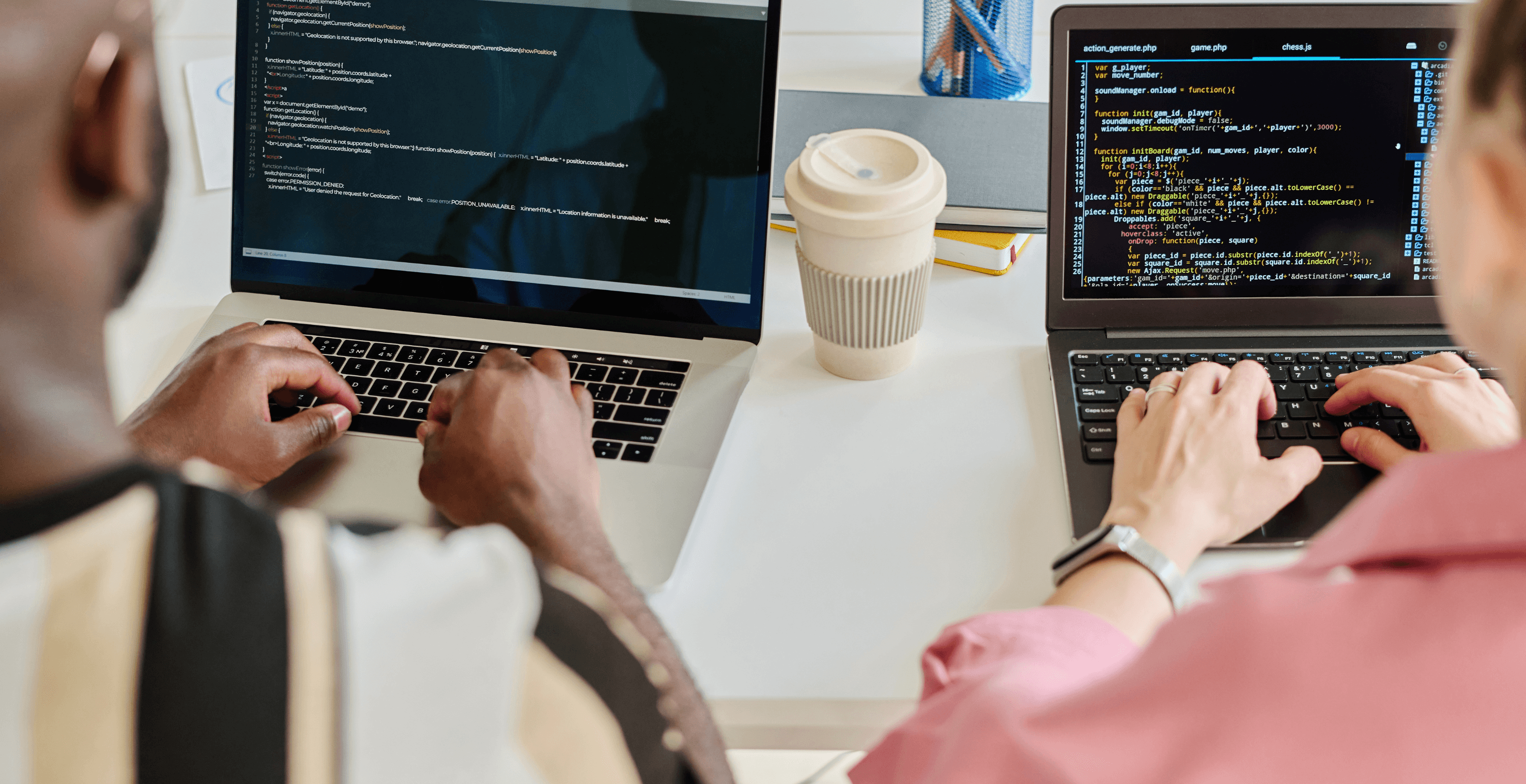
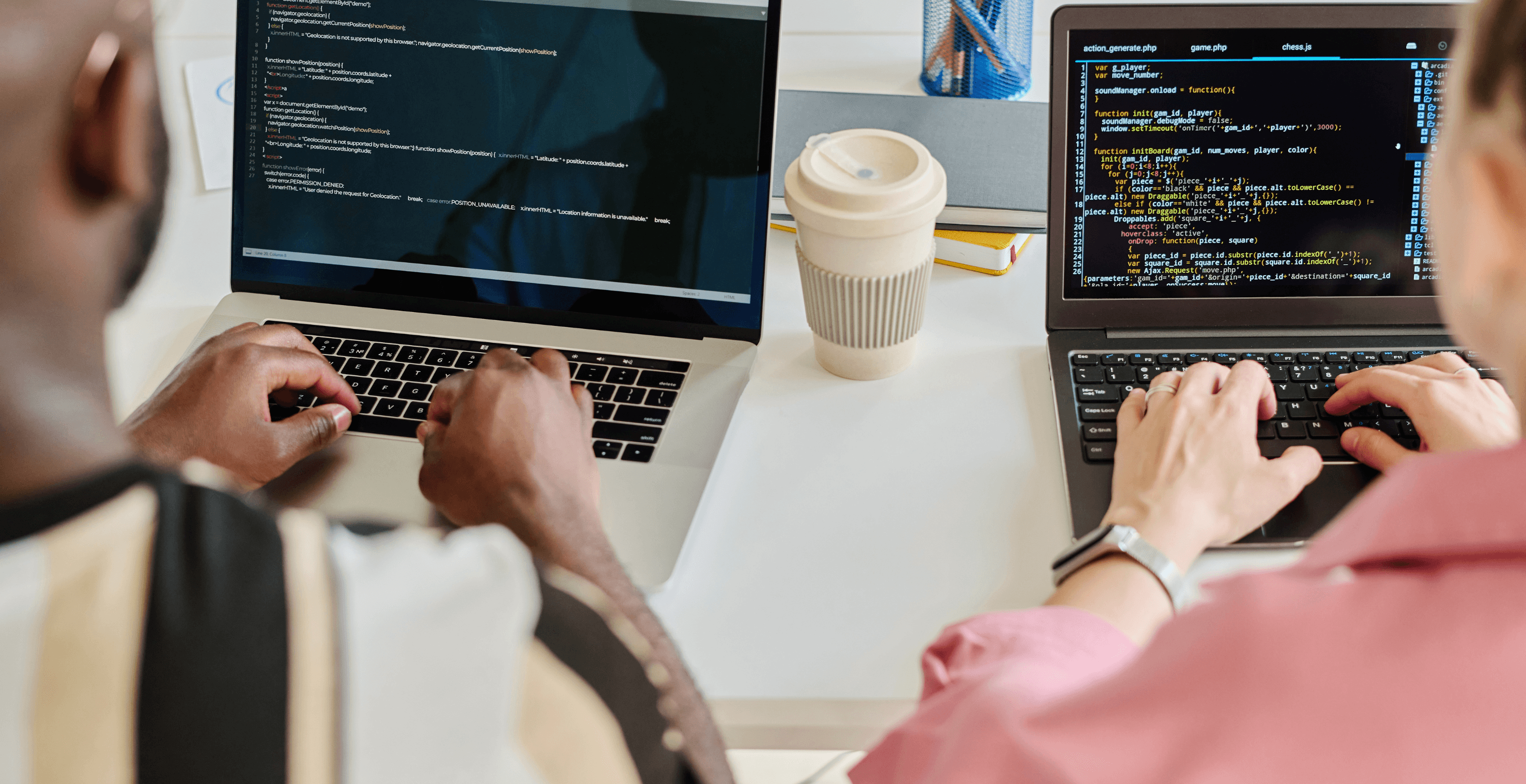
Understanding NeMo Guardrails: A Toolkit for LLM Security

Rehan Asif
Dec 24, 2024
Read the article




Understanding Differences in Large vs Small Language Models (LLM vs SLM)

Rehan Asif
Dec 21, 2024
Read the article




Understanding What an AI Agent is: Key Applications and Examples
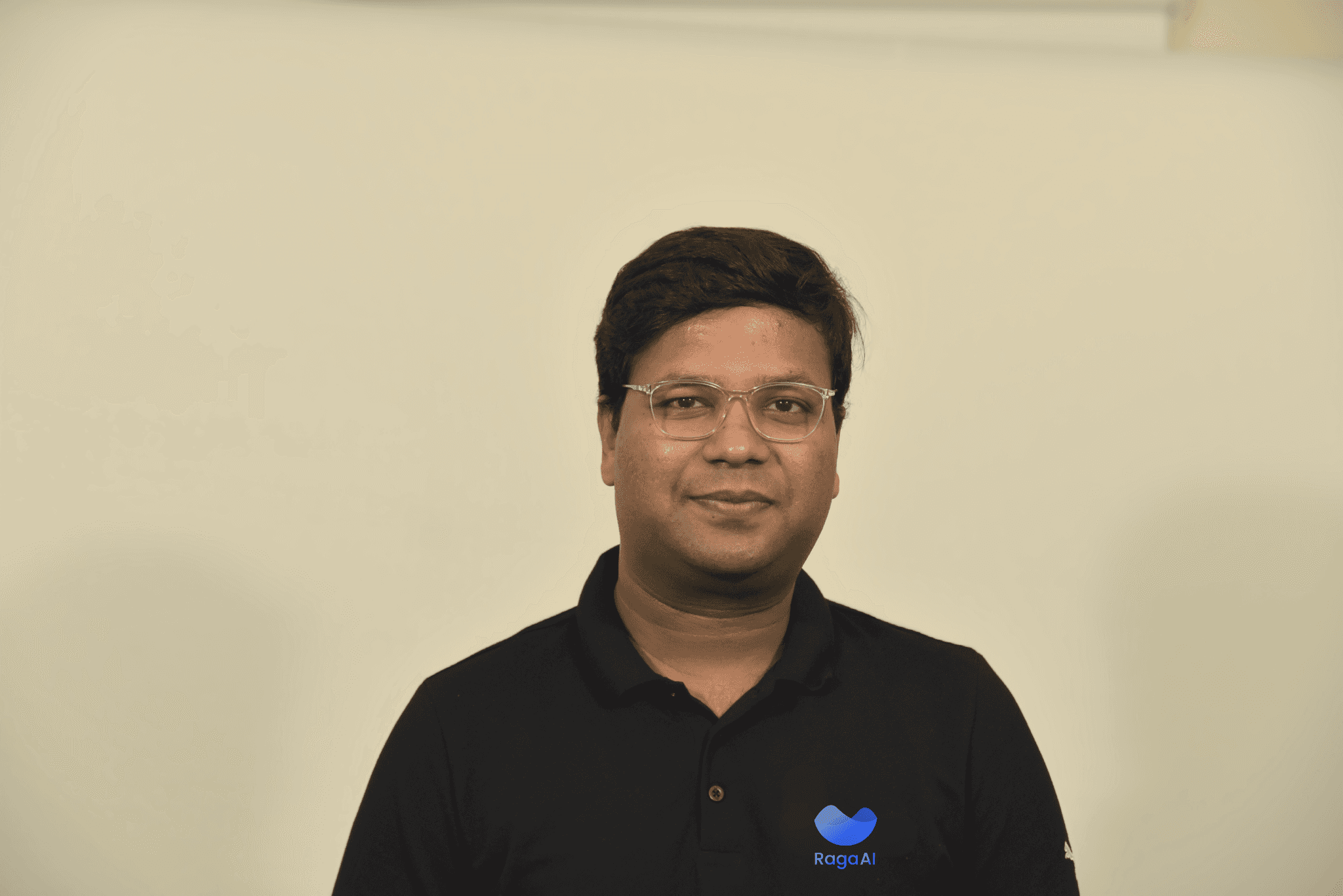
Jigar Gupta
Dec 17, 2024
Read the article




Prompt Engineering and Retrieval Augmented Generation (RAG)
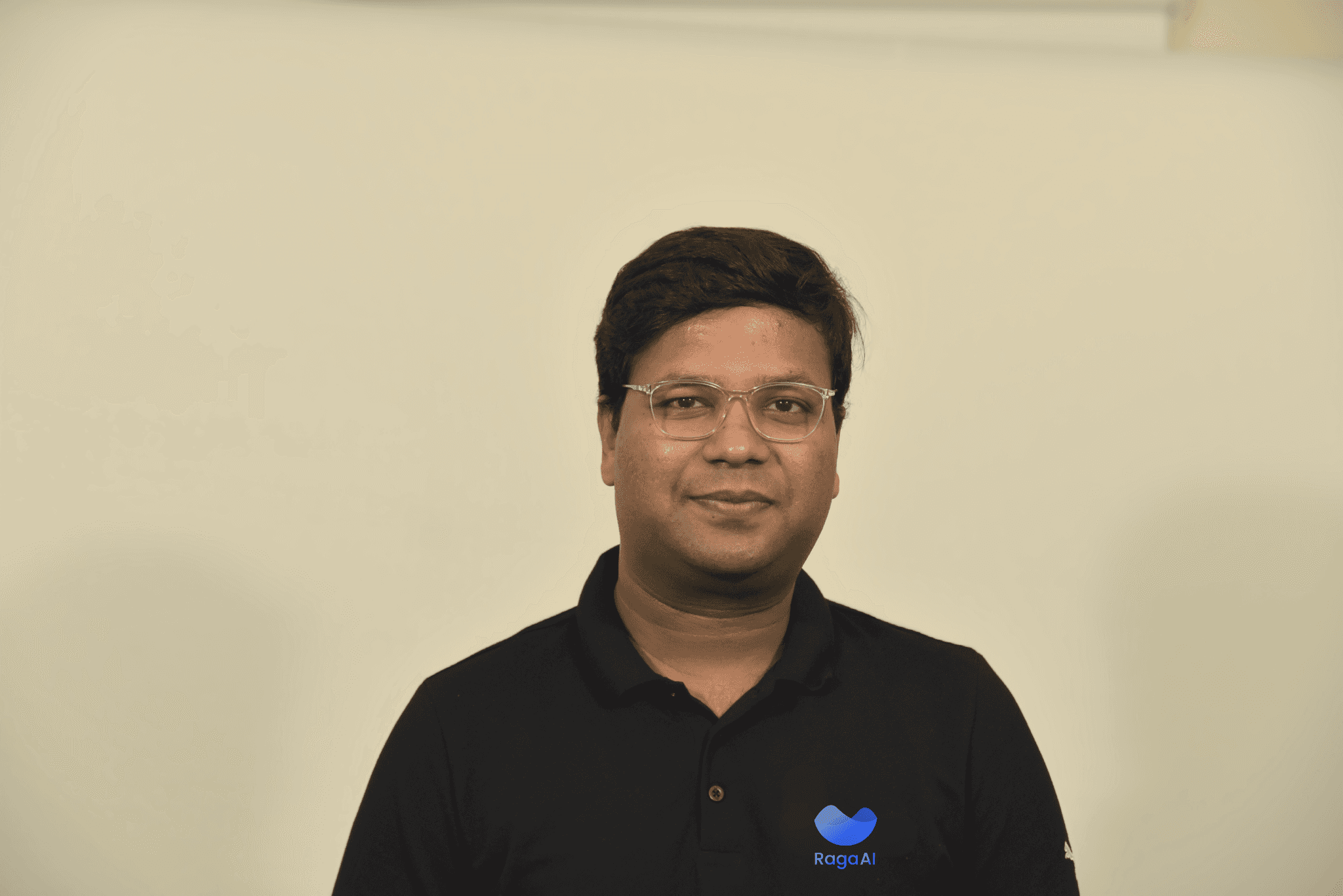
Jigar Gupta
Dec 12, 2024
Read the article




Exploring How Multimodal Large Language Models Work

Rehan Asif
Dec 9, 2024
Read the article
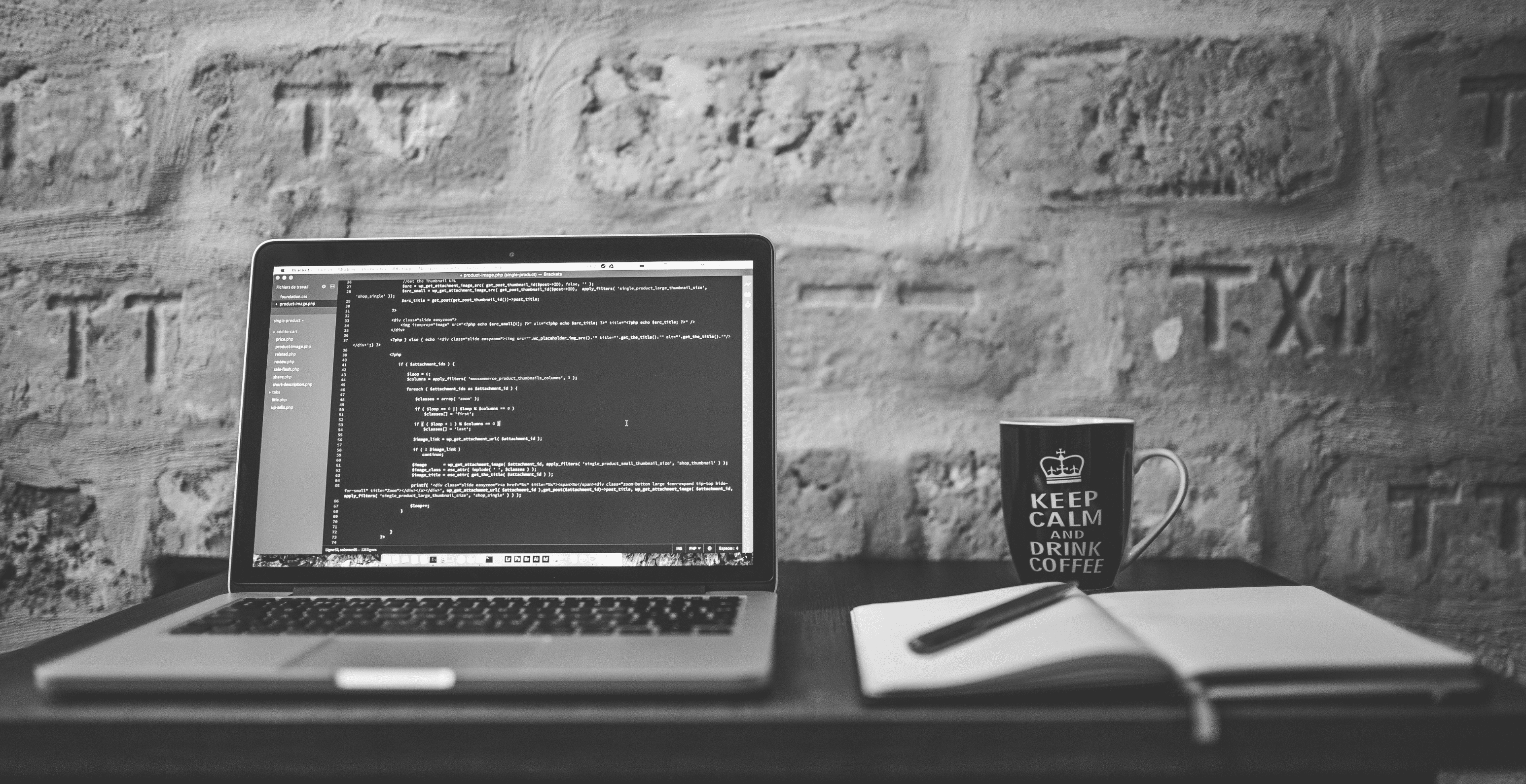
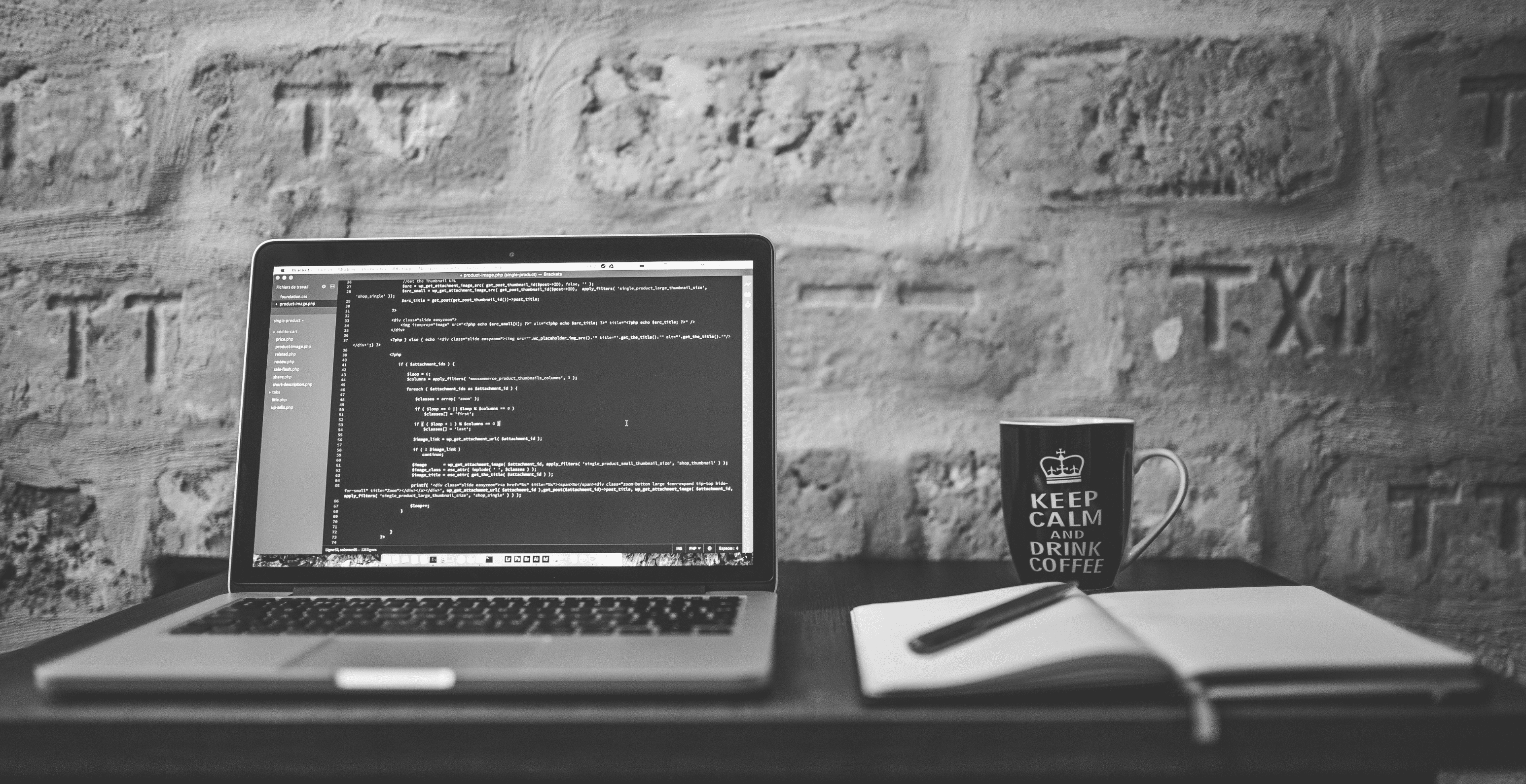
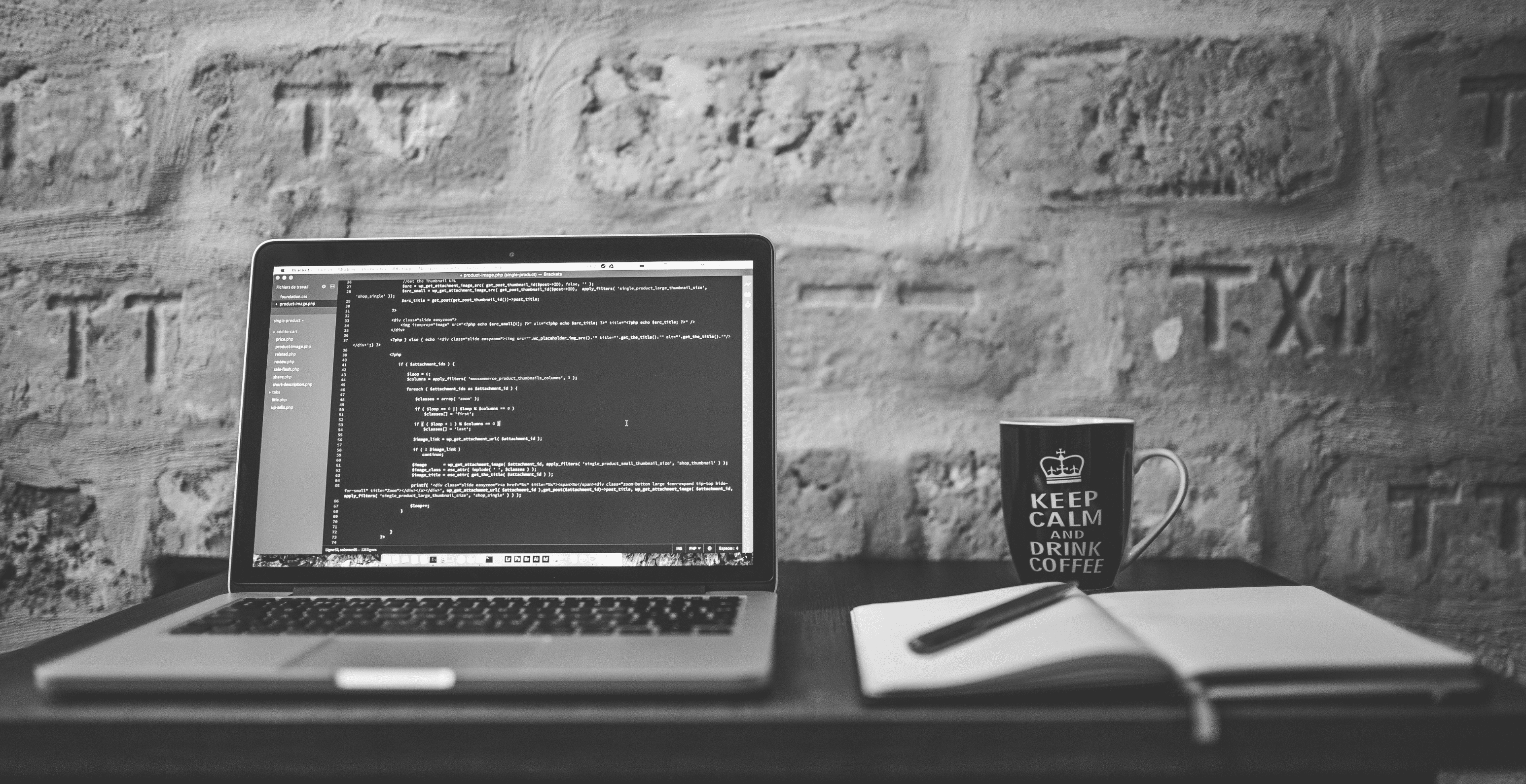
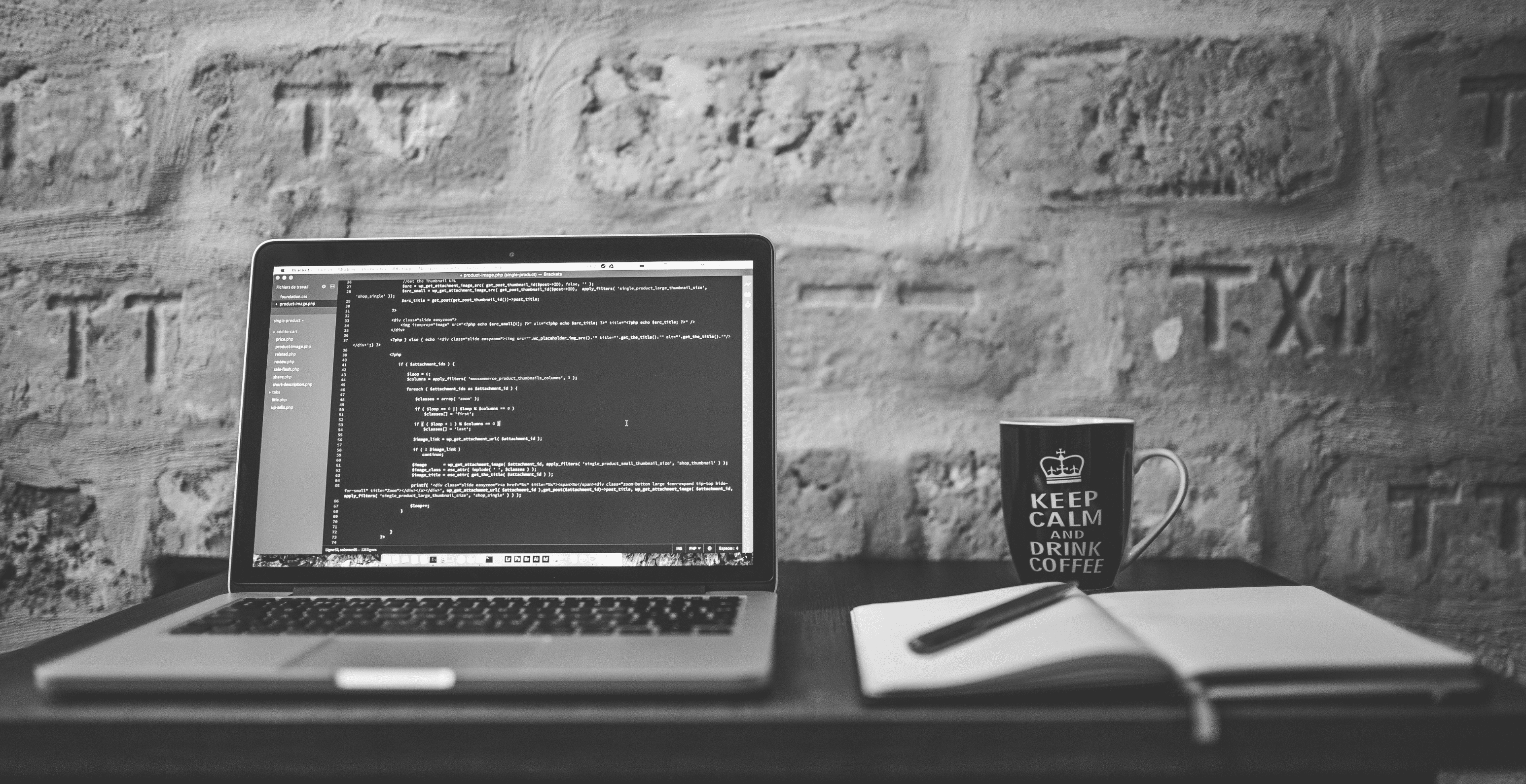
Evaluating and Enhancing LLM-as-a-Judge with Automated Tools

Rehan Asif
Dec 6, 2024
Read the article




Optimizing Performance and Cost by Caching LLM Queries

Rehan Asif
Dec 3, 2024
Read the article




LoRA vs RAG: Full Model Fine-Tuning in Large Language Models
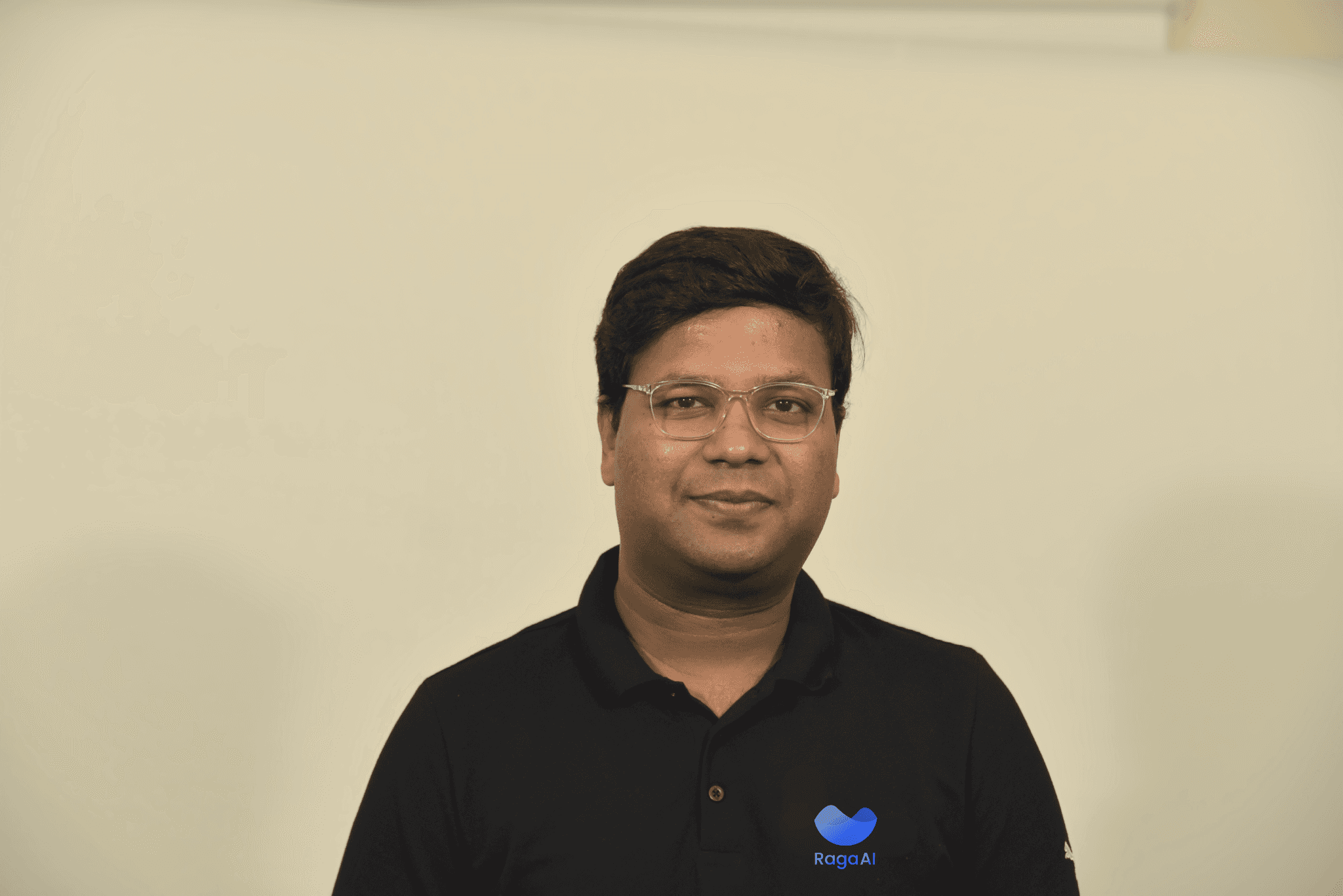
Jigar Gupta
Nov 30, 2024
Read the article
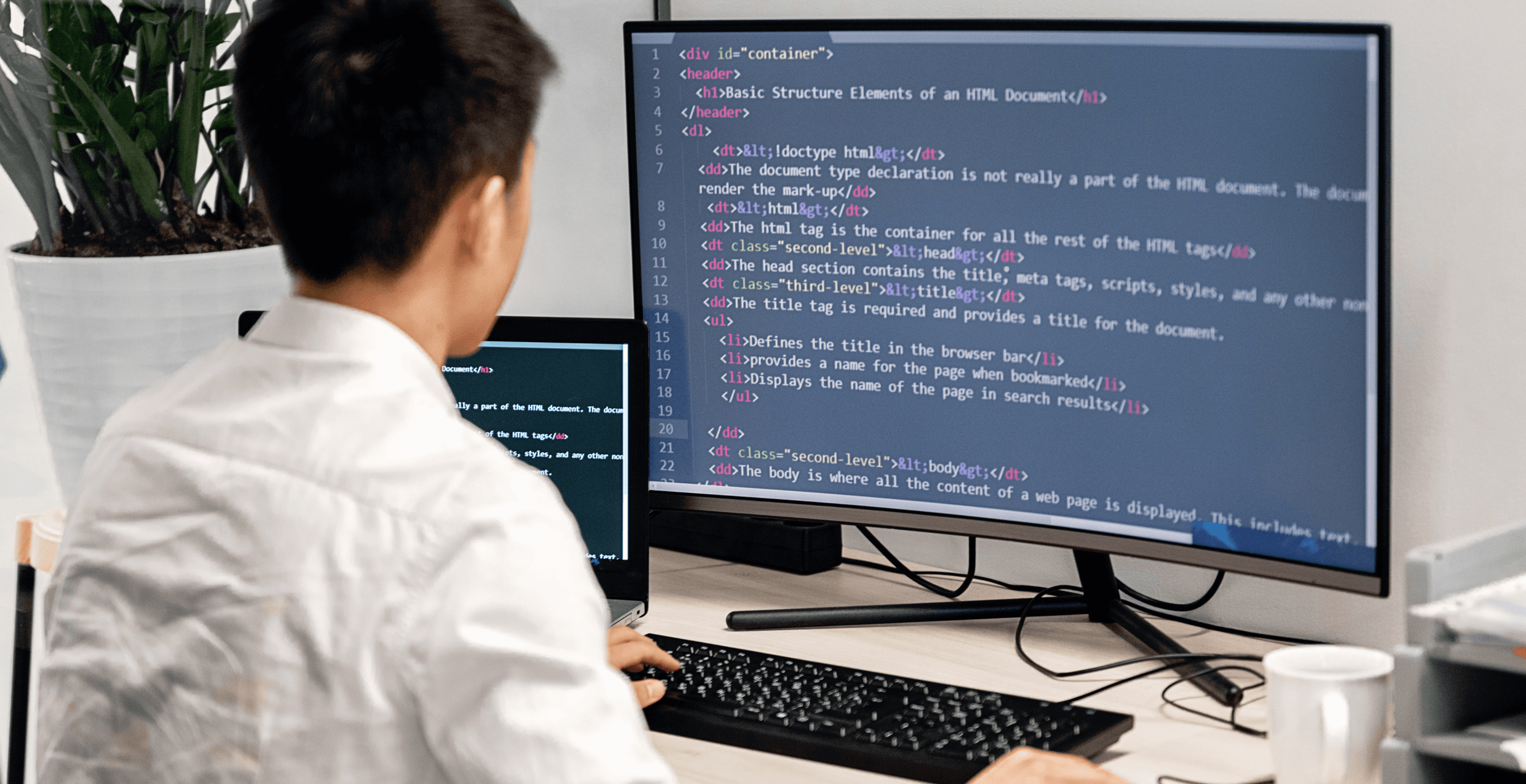
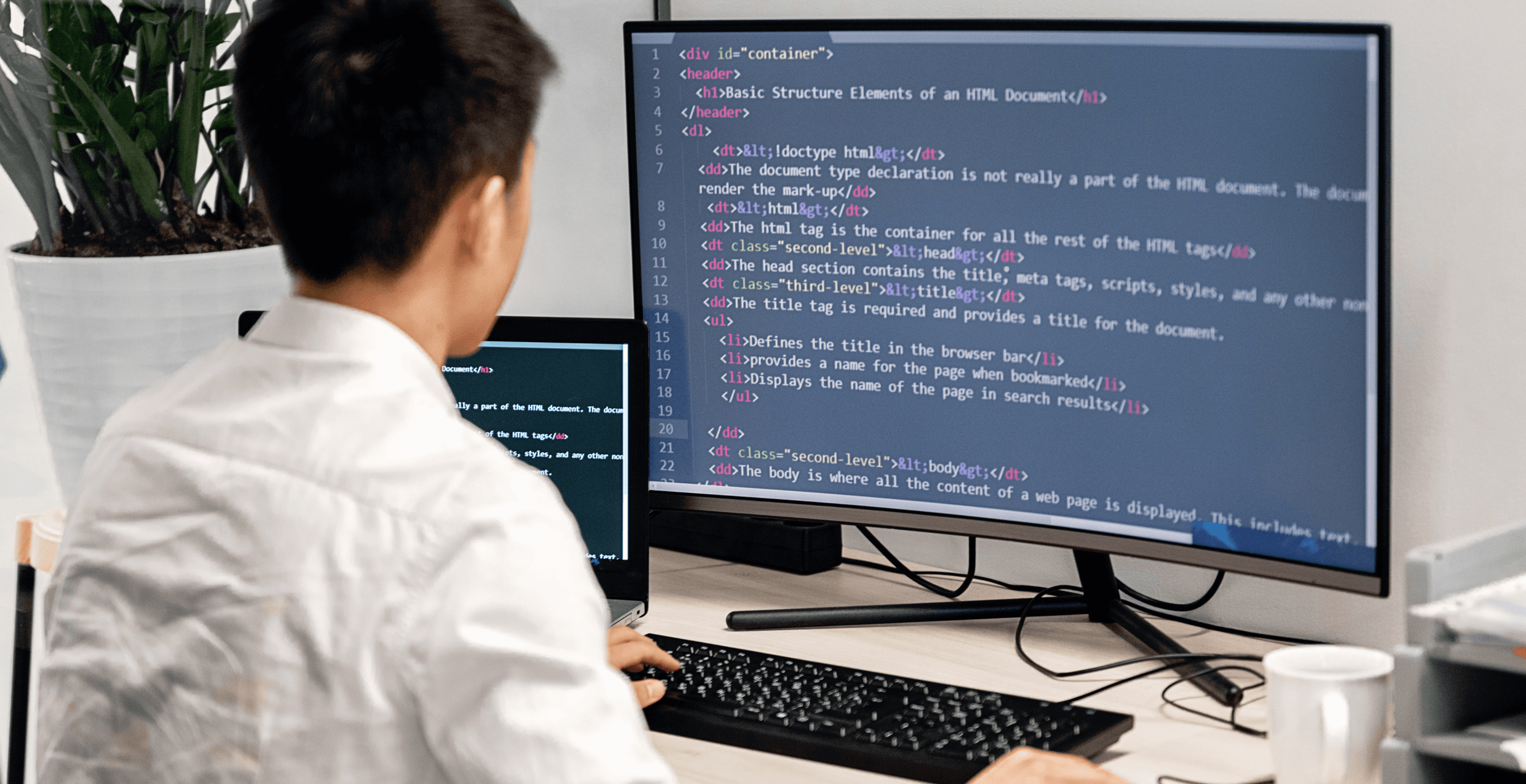
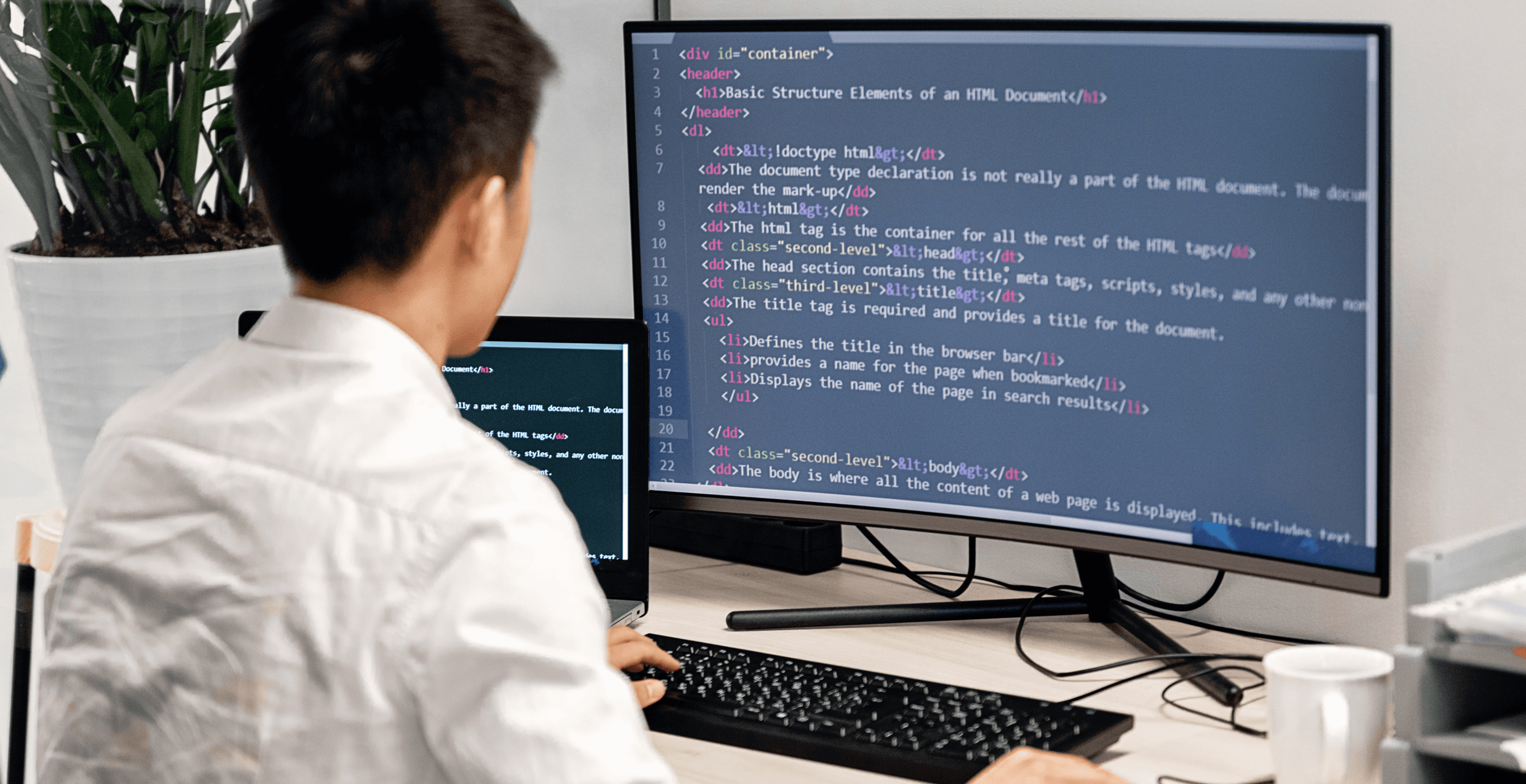
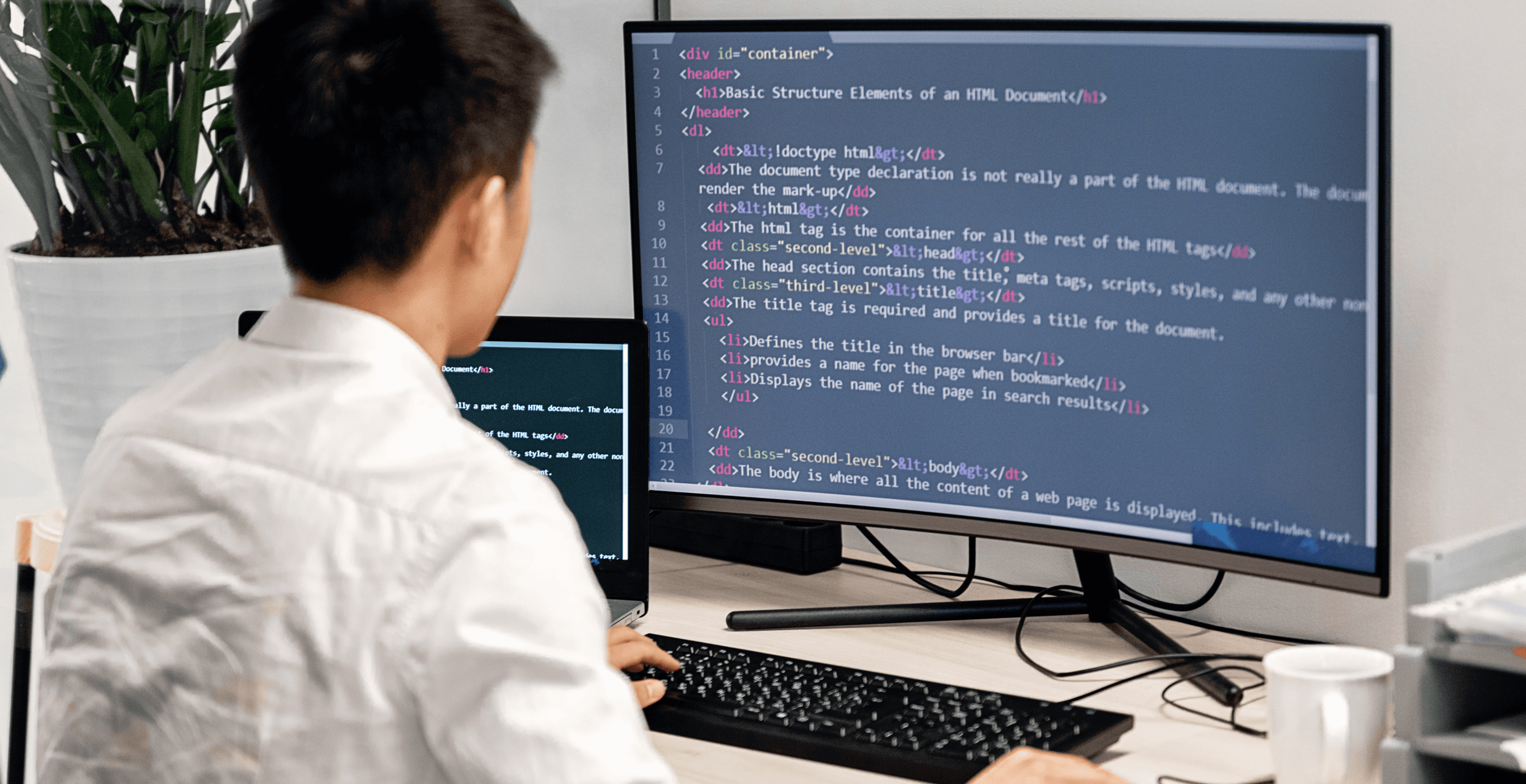
Steps to Train LLM on Personal Data

Rehan Asif
Nov 28, 2024
Read the article




Step by Step Guide to Building RAG-based LLM Applications with Examples

Rehan Asif
Nov 27, 2024
Read the article
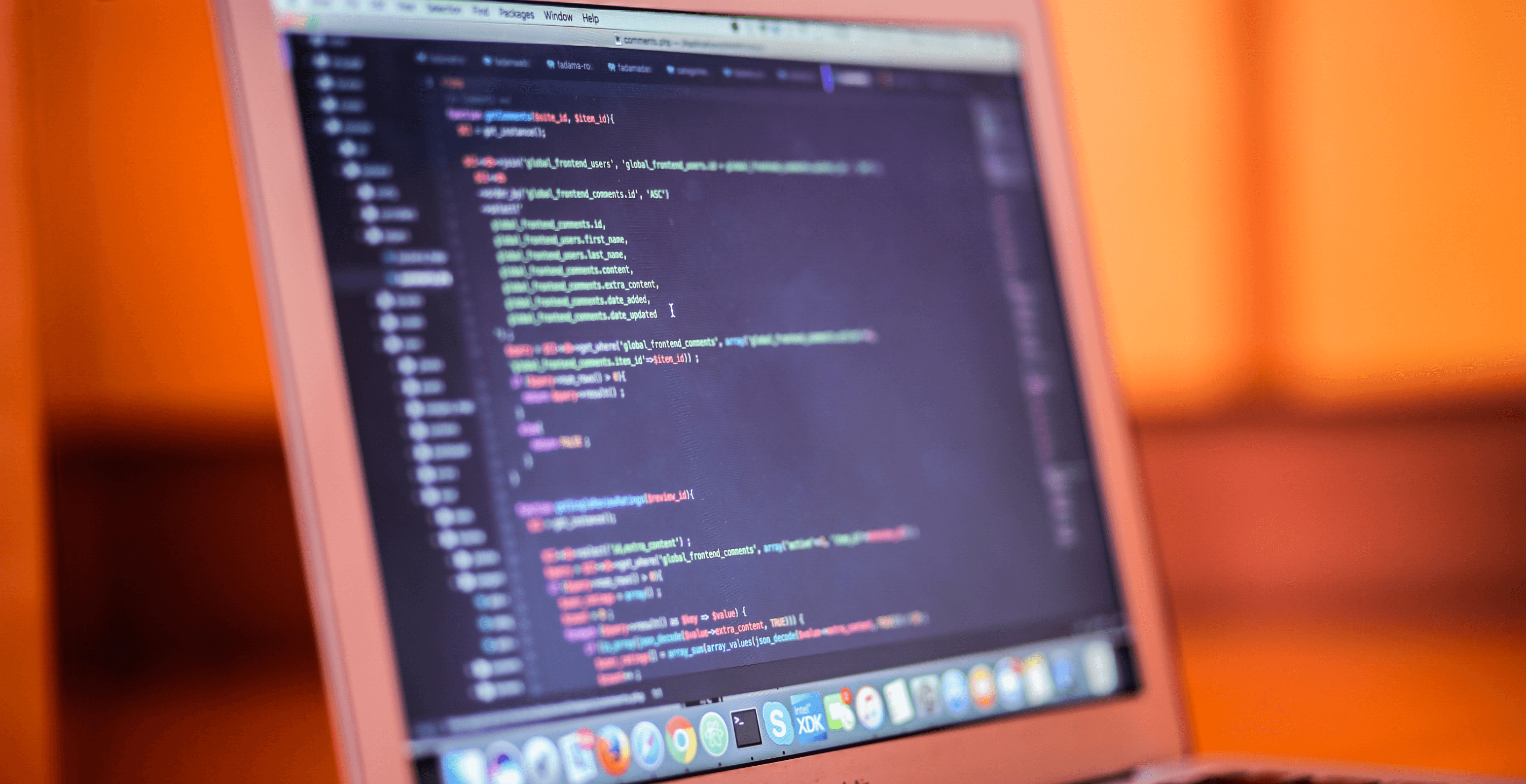
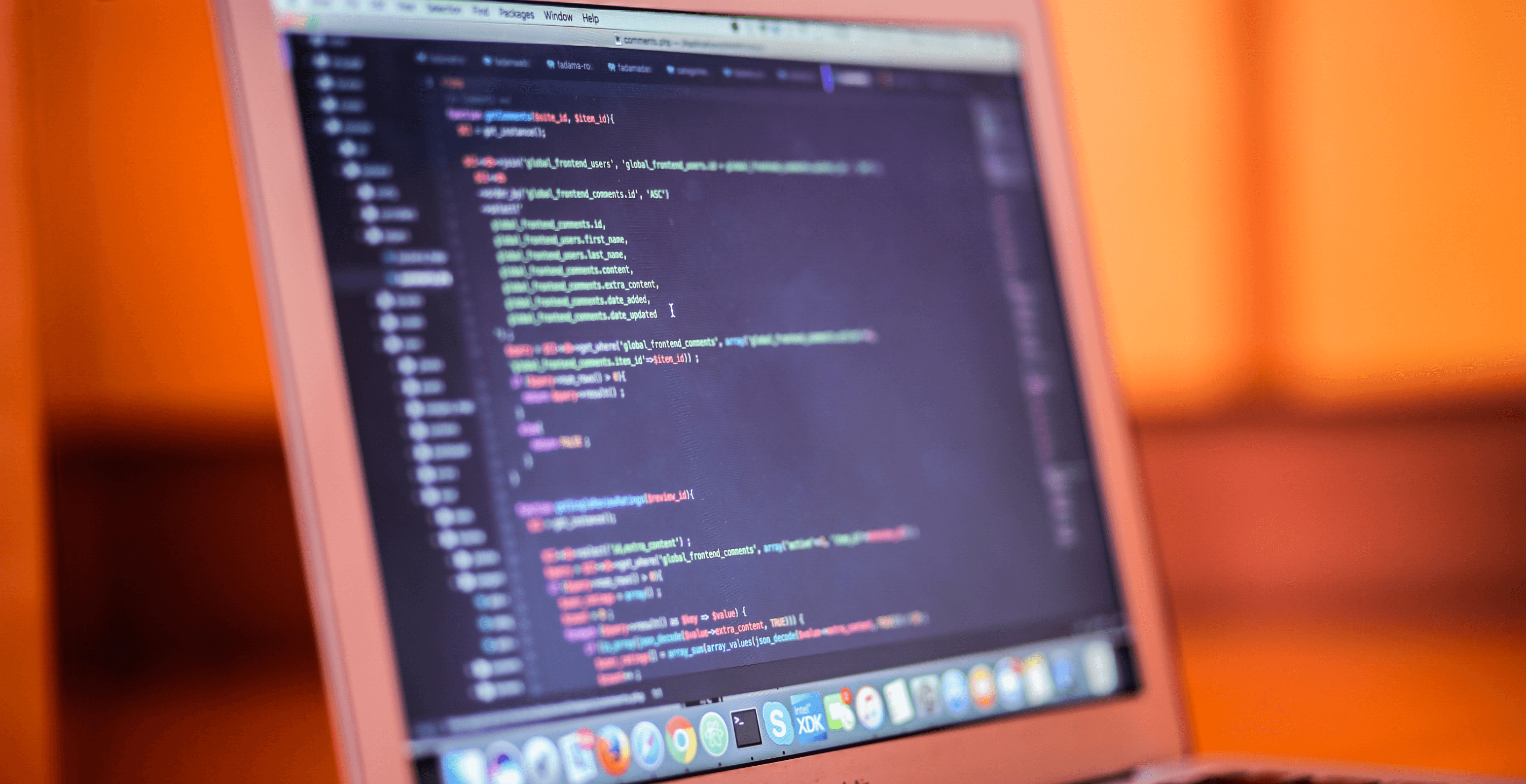
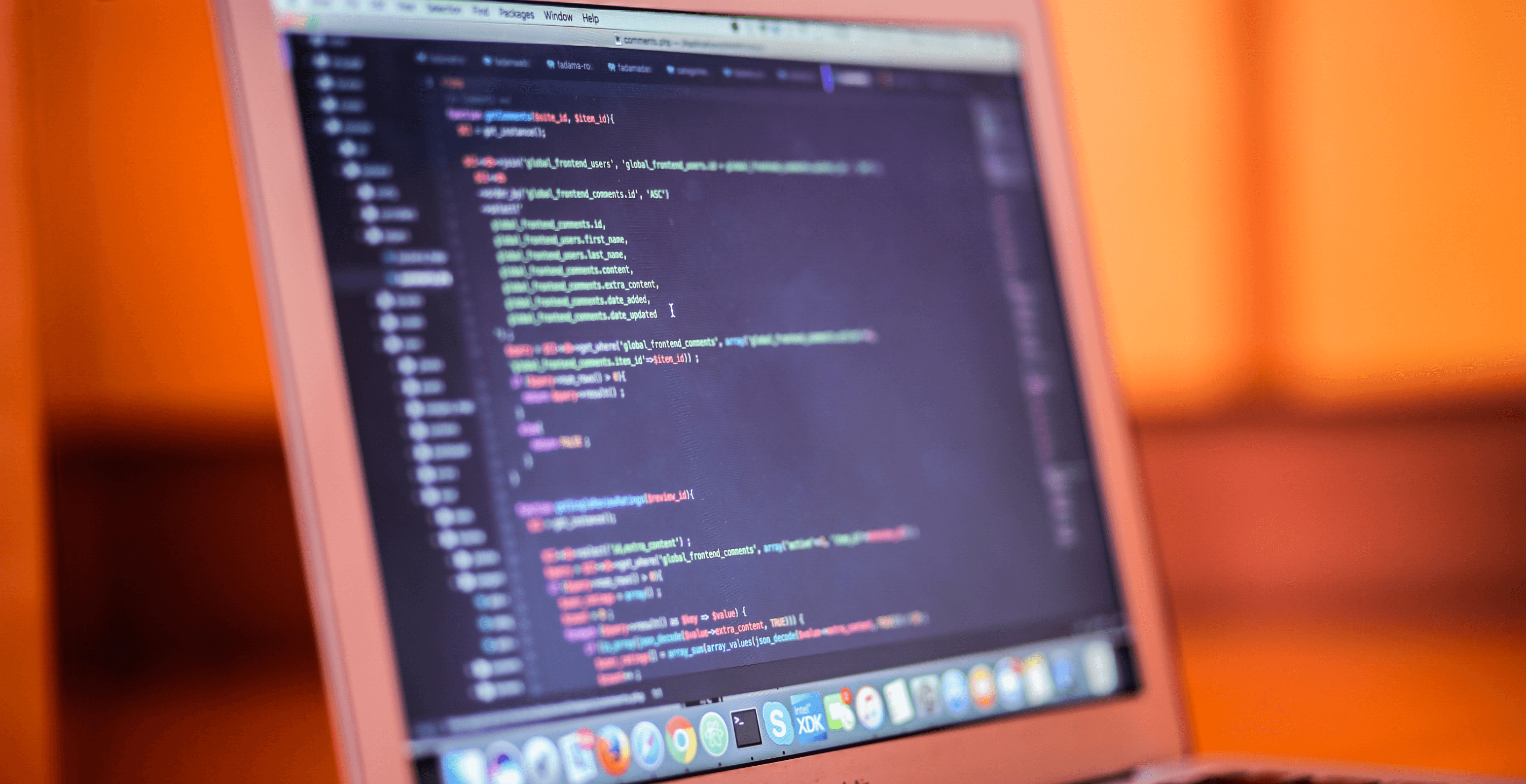
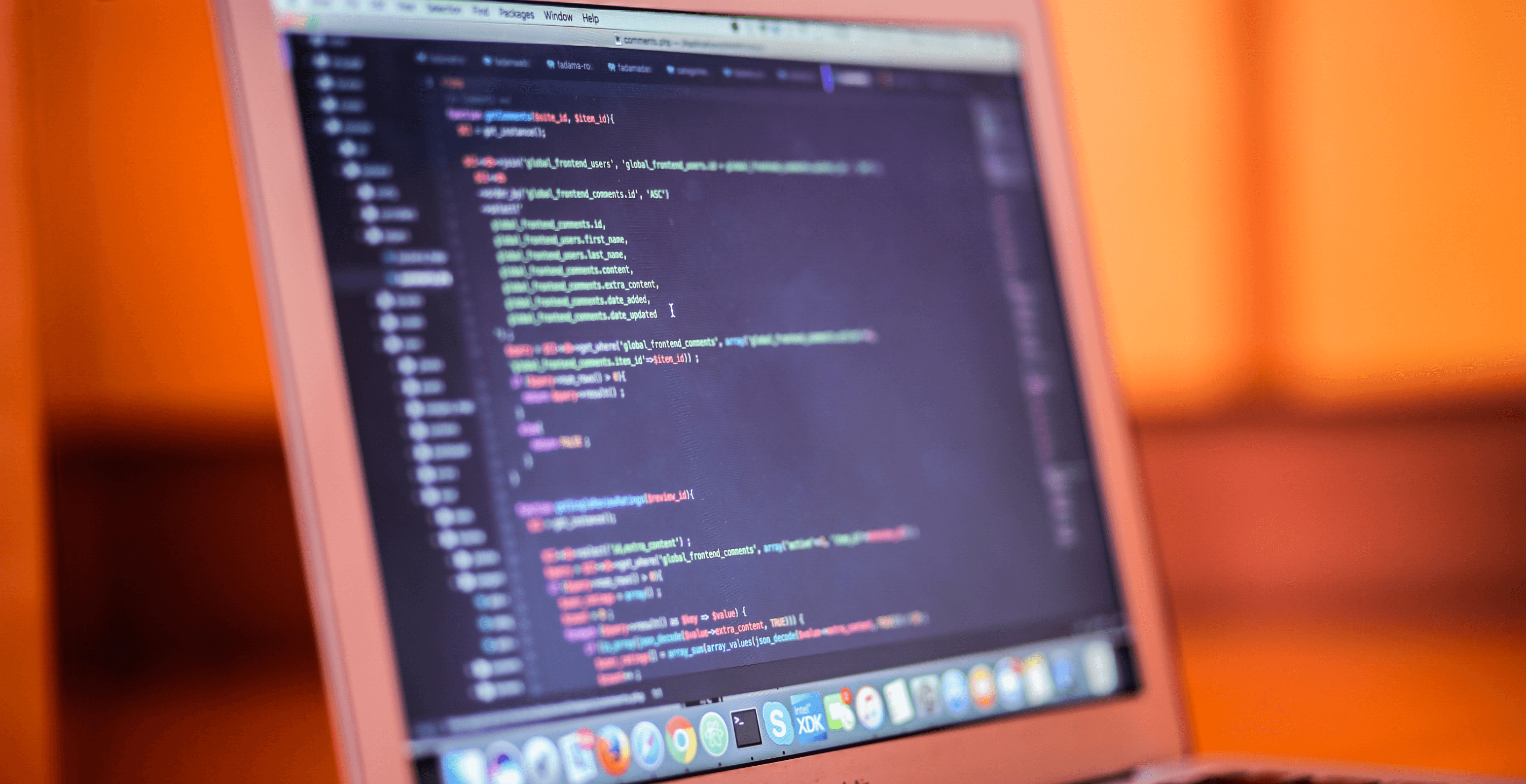
Building AI Agentic Workflows with Multi-Agent Collaboration
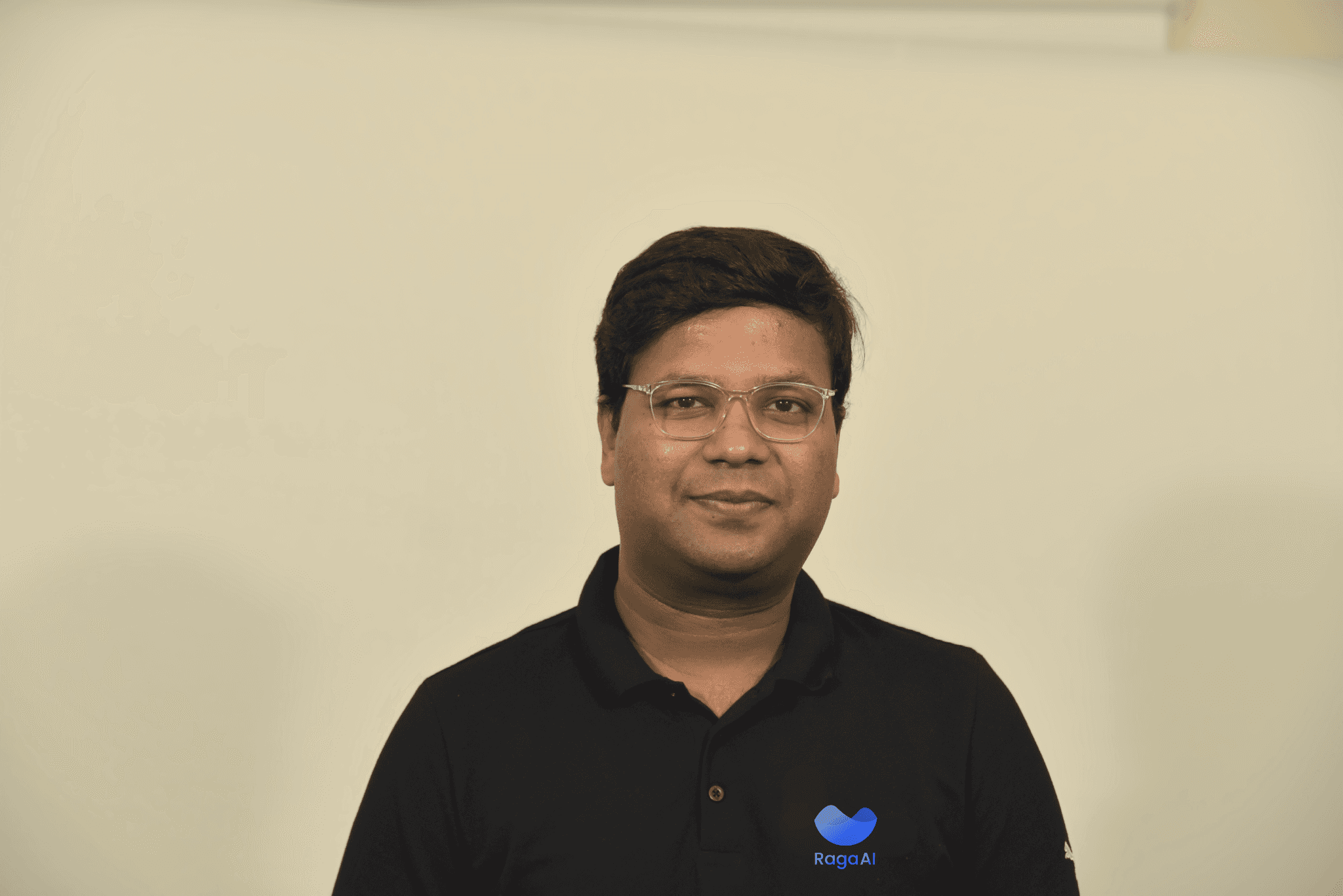
Jigar Gupta
Nov 25, 2024
Read the article




Top Large Language Models (LLMs) in 2024

Rehan Asif
Nov 22, 2024
Read the article
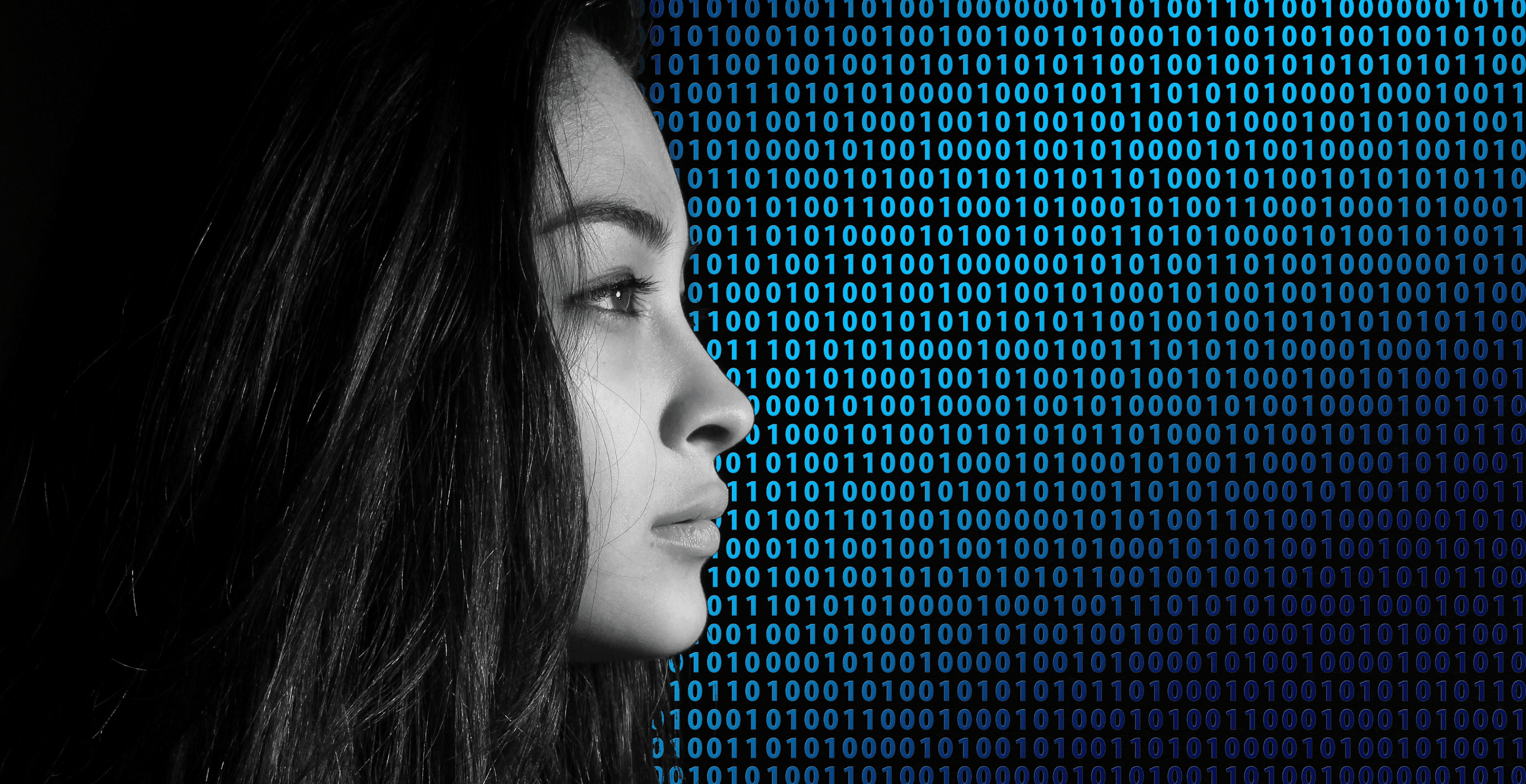
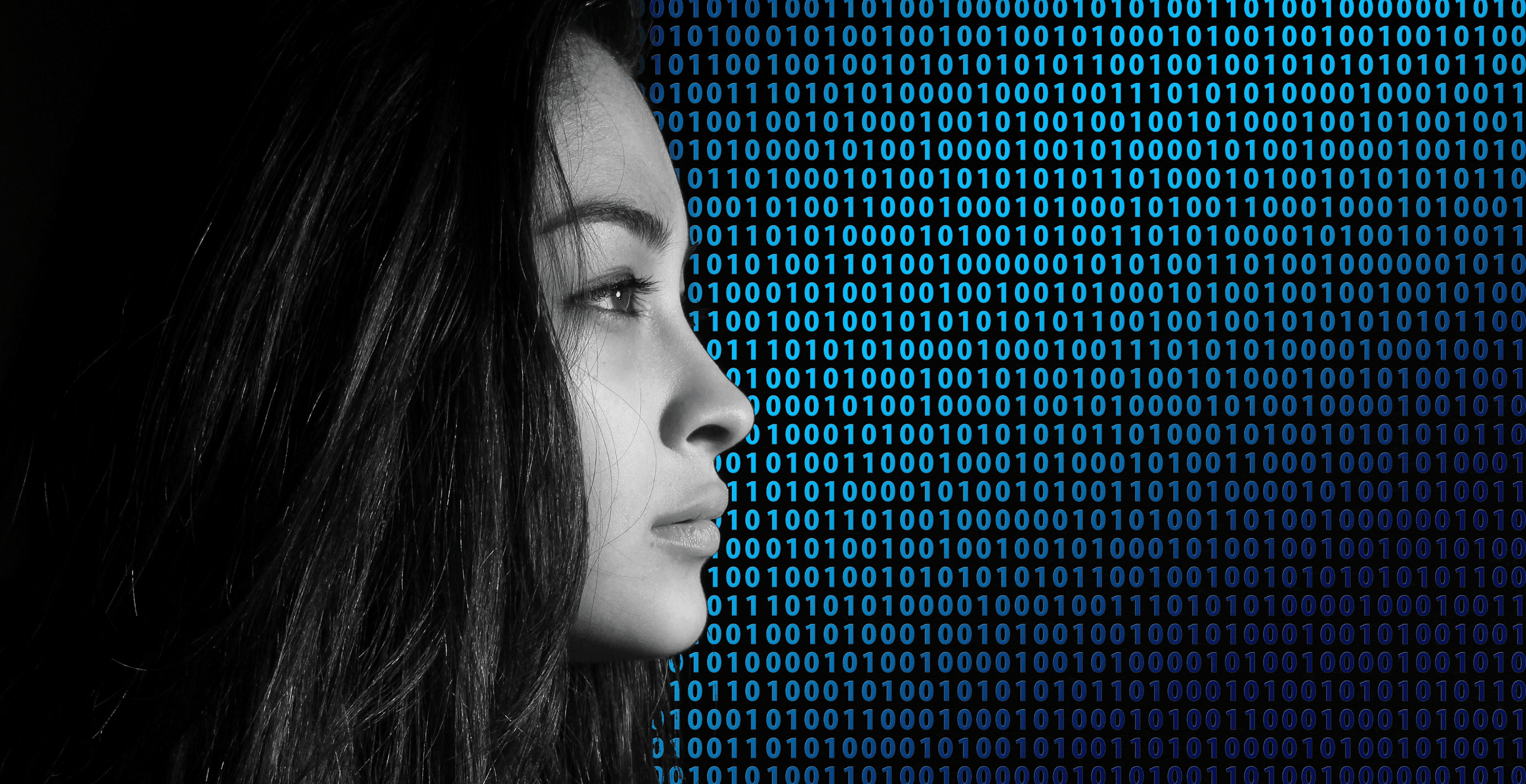
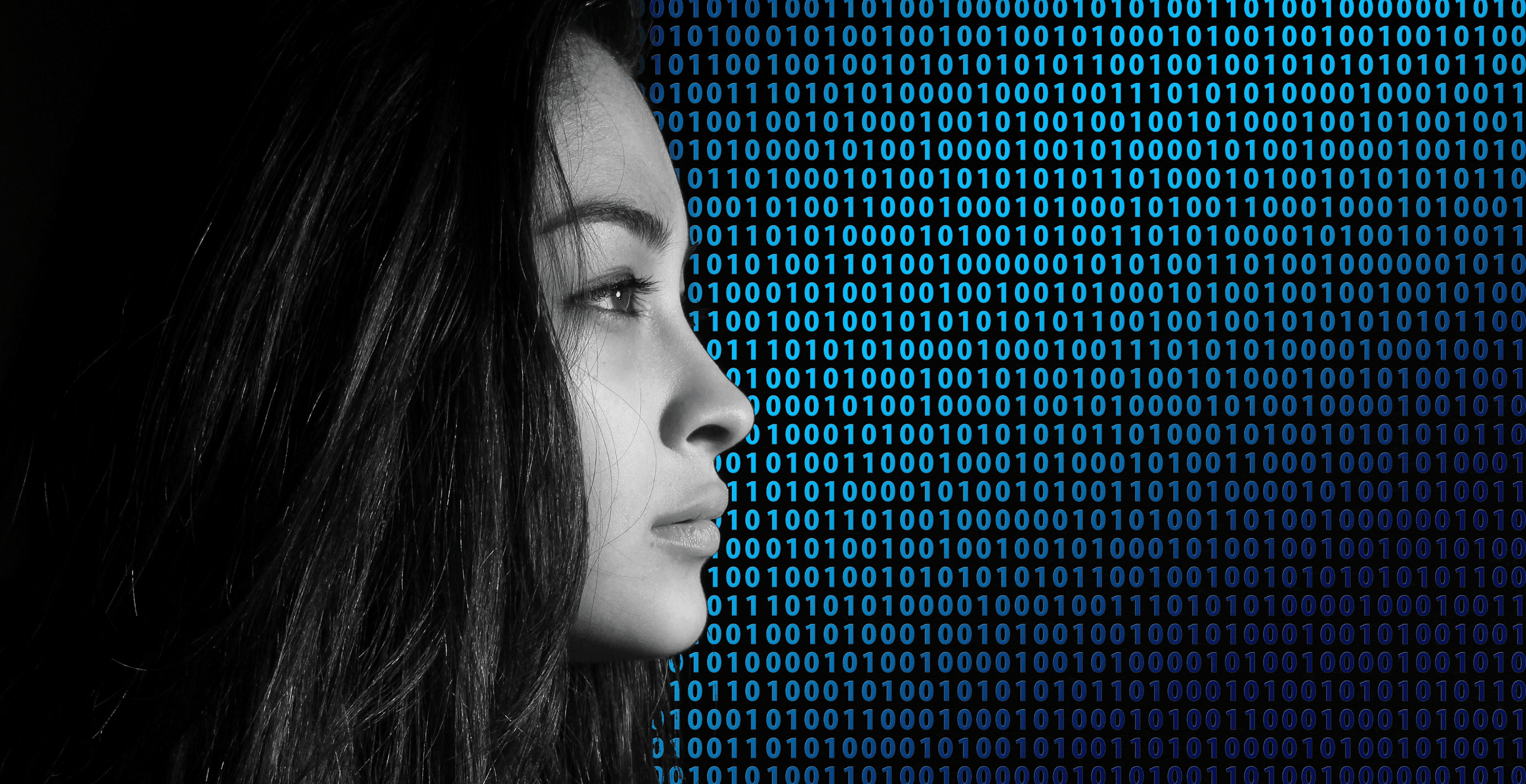
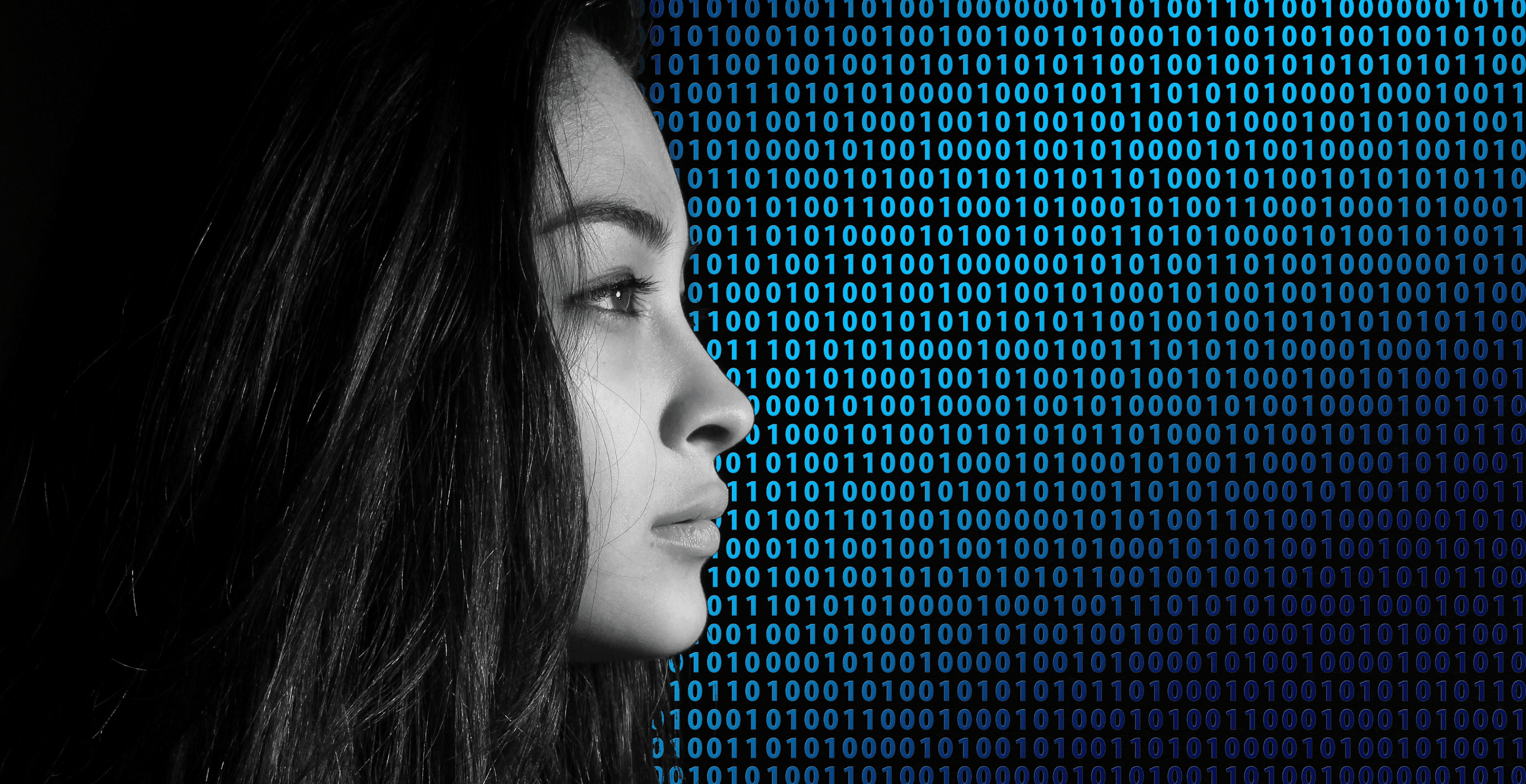
Creating Apps with Large Language Models

Rehan Asif
Nov 21, 2024
Read the article
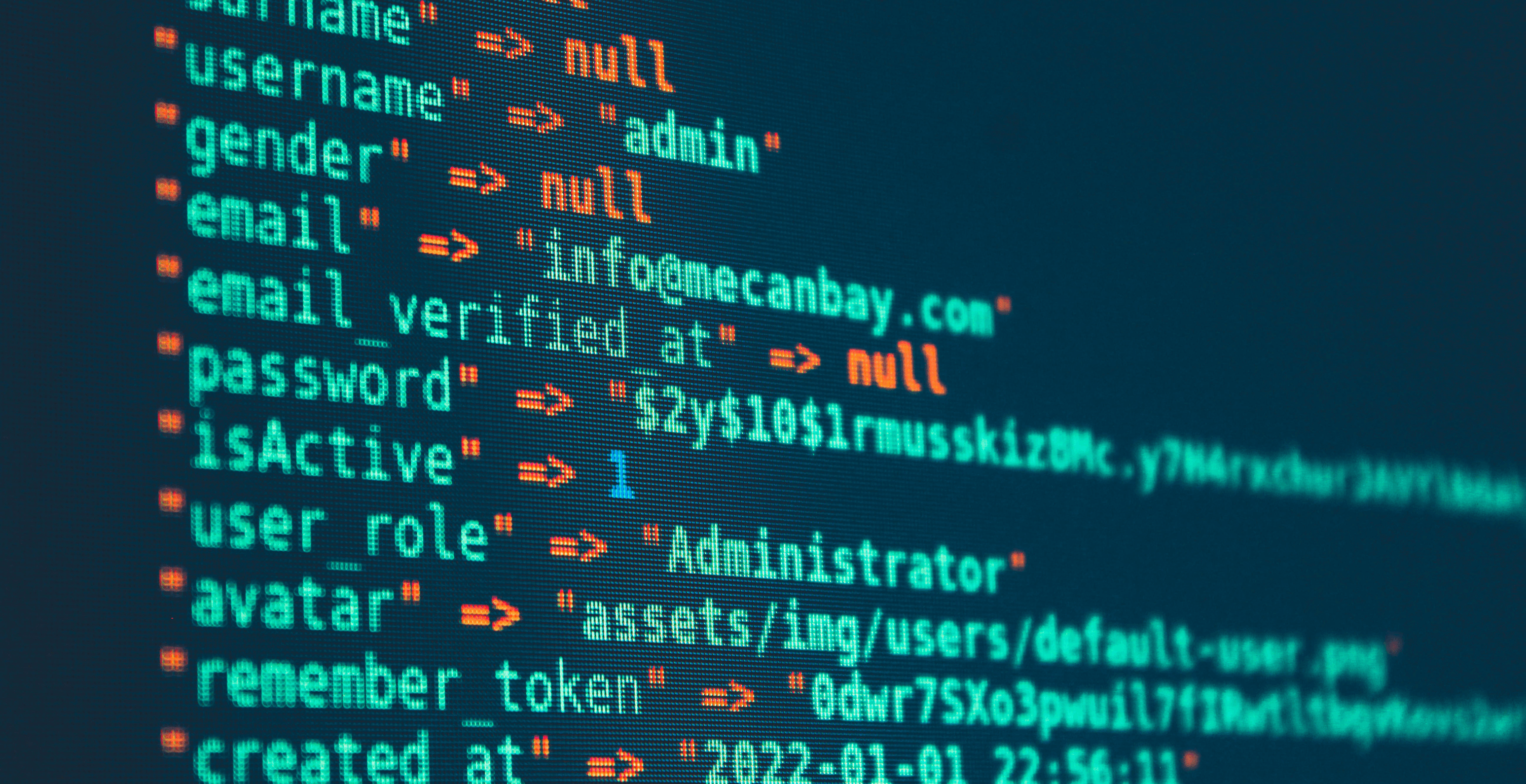
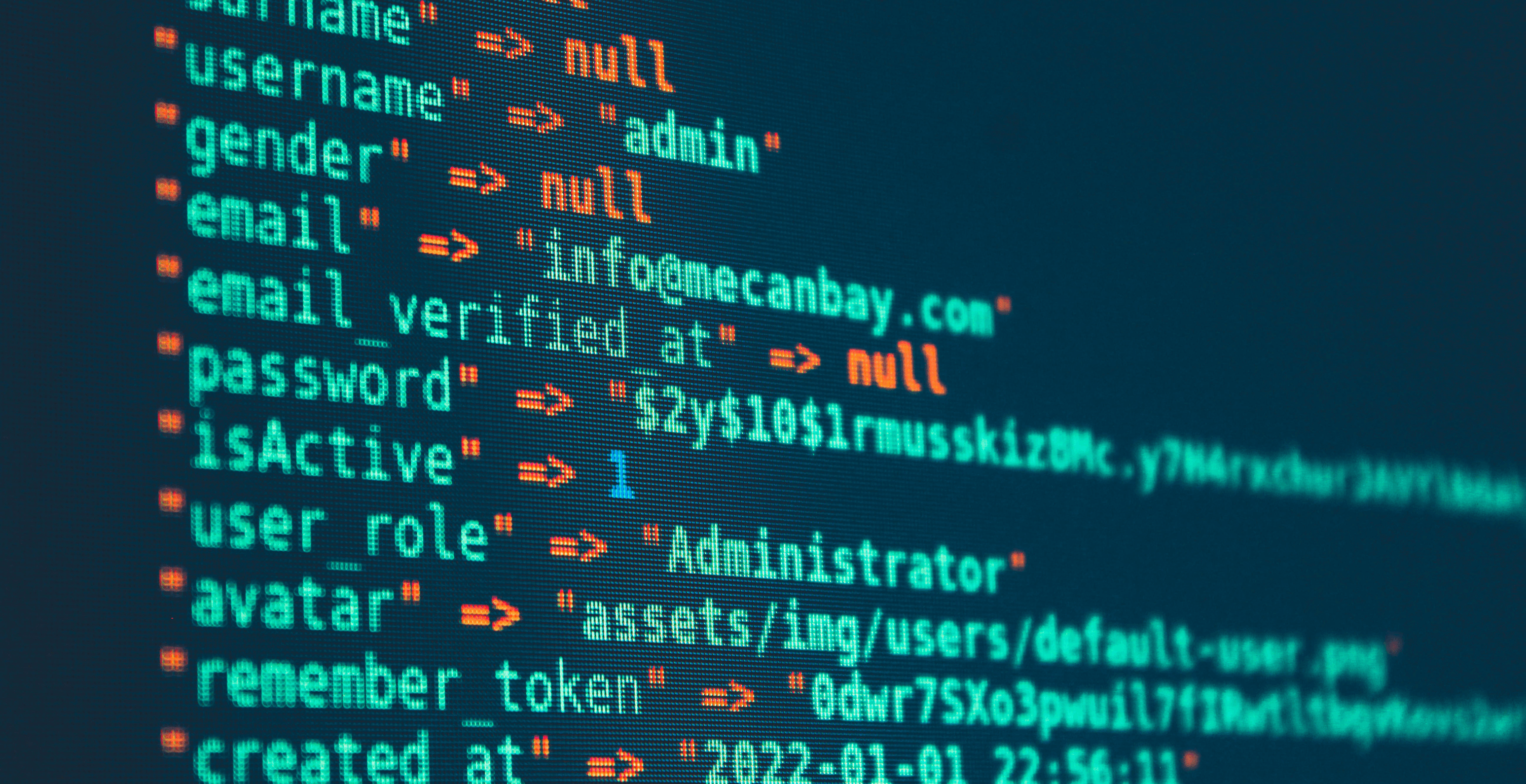
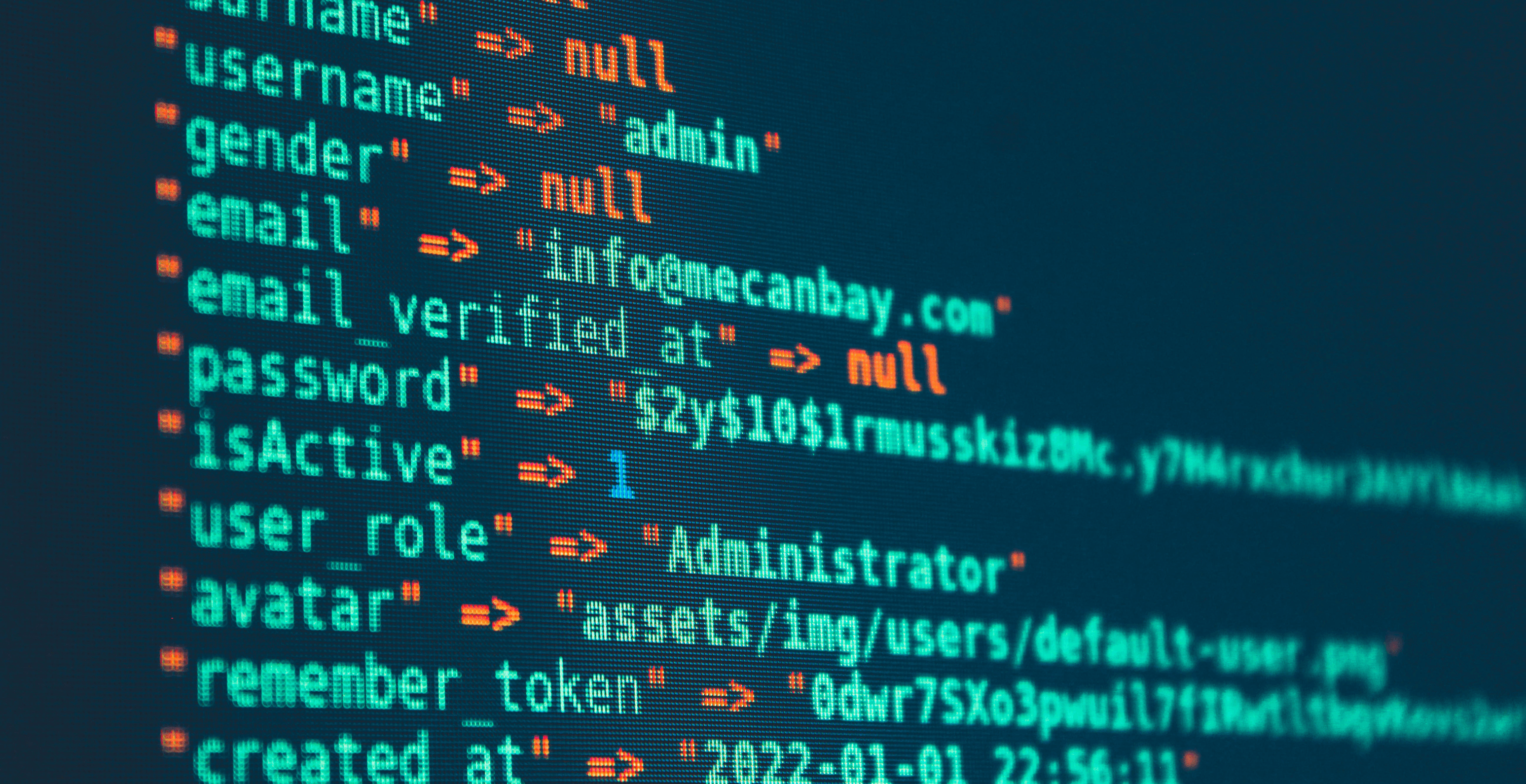
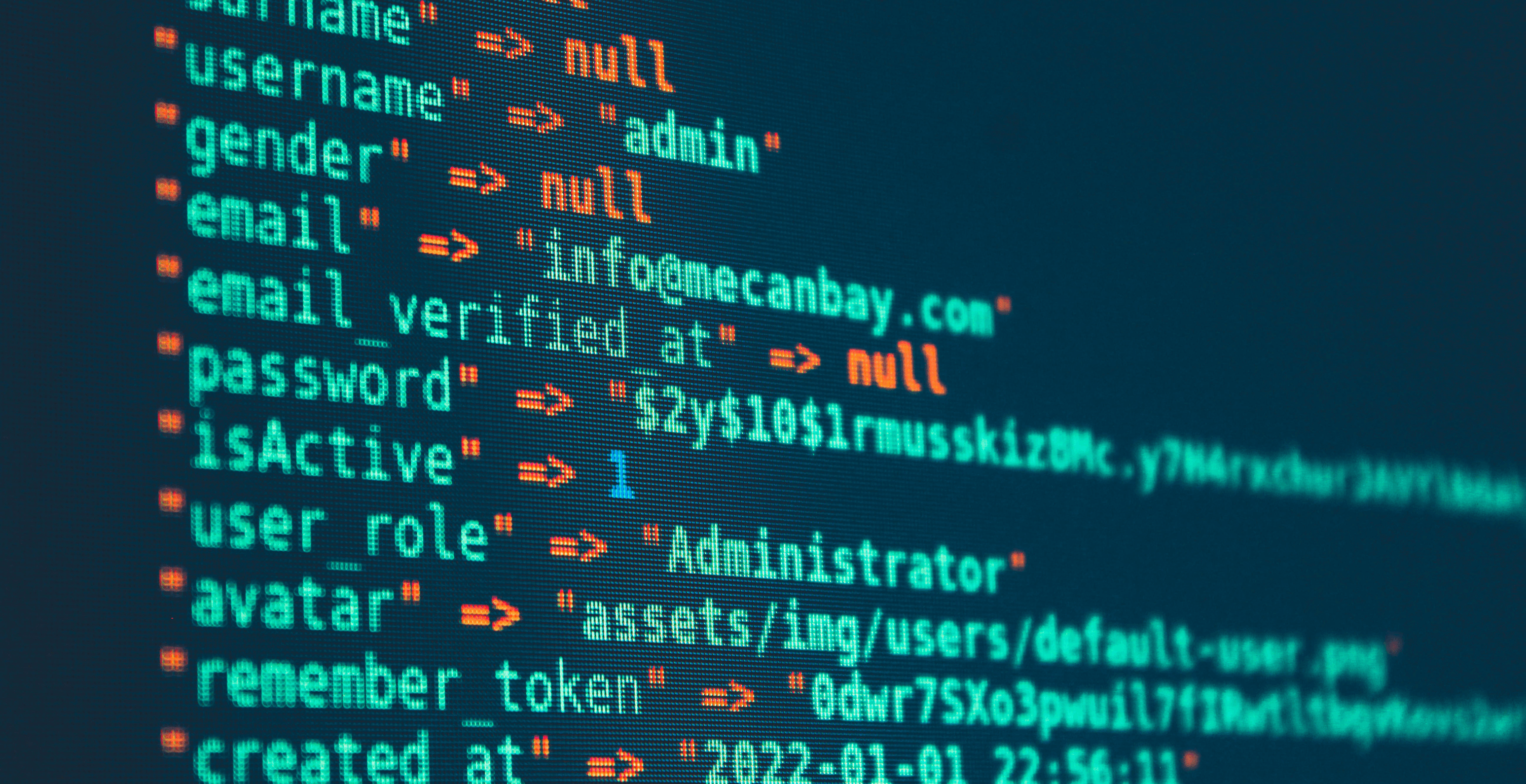
Best Practices In Data Governance For AI
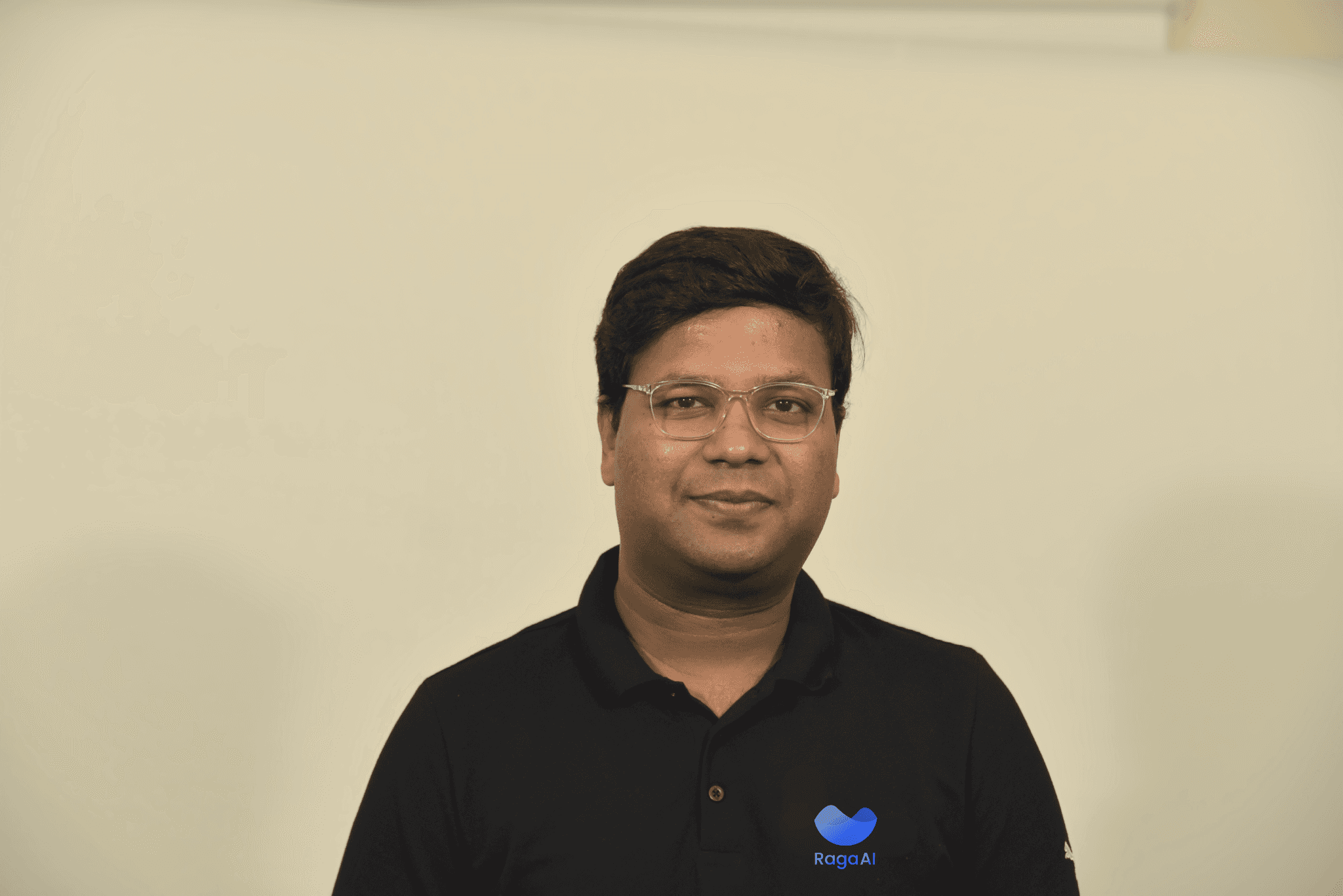
Jigar Gupta
Nov 17, 2024
Read the article
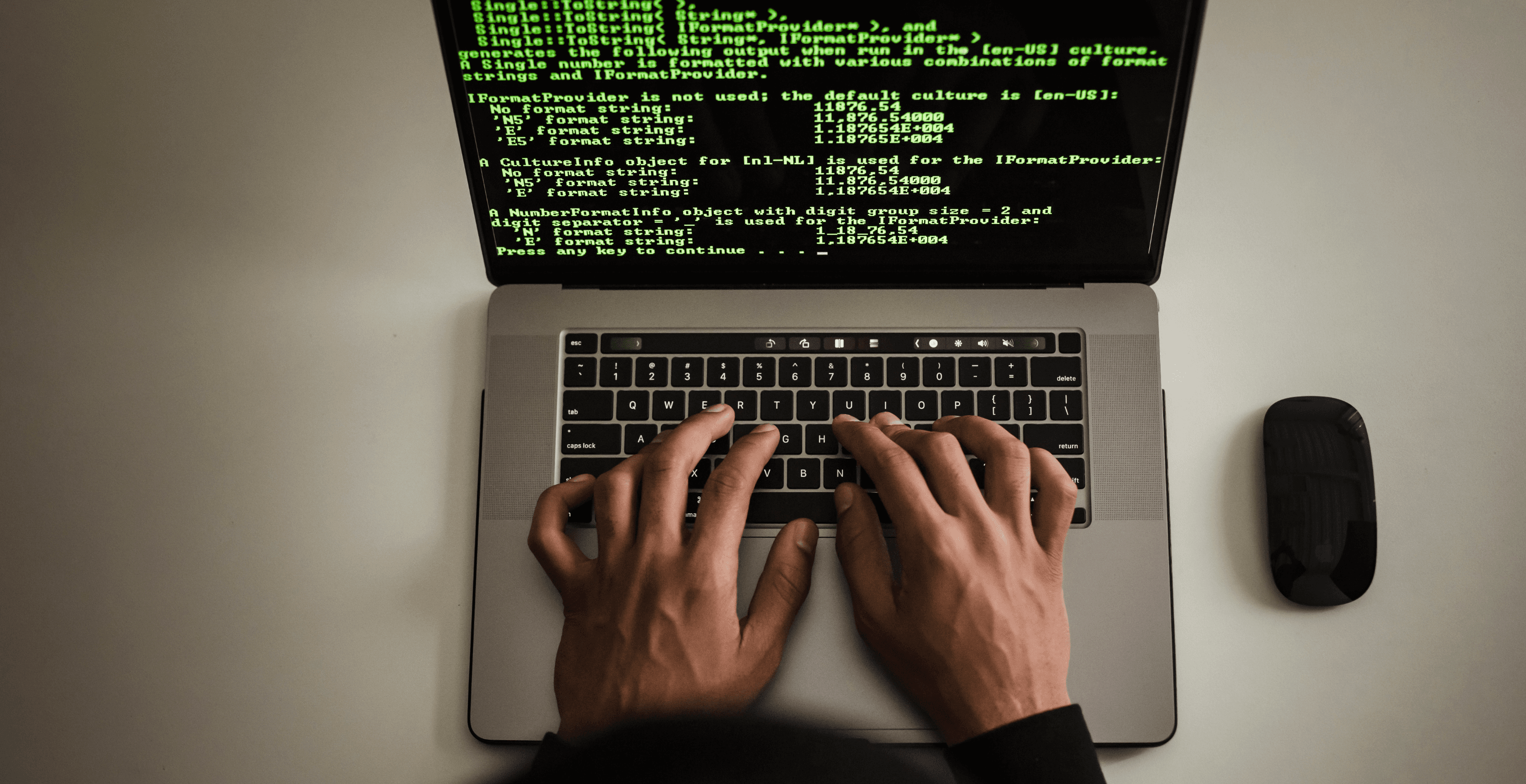
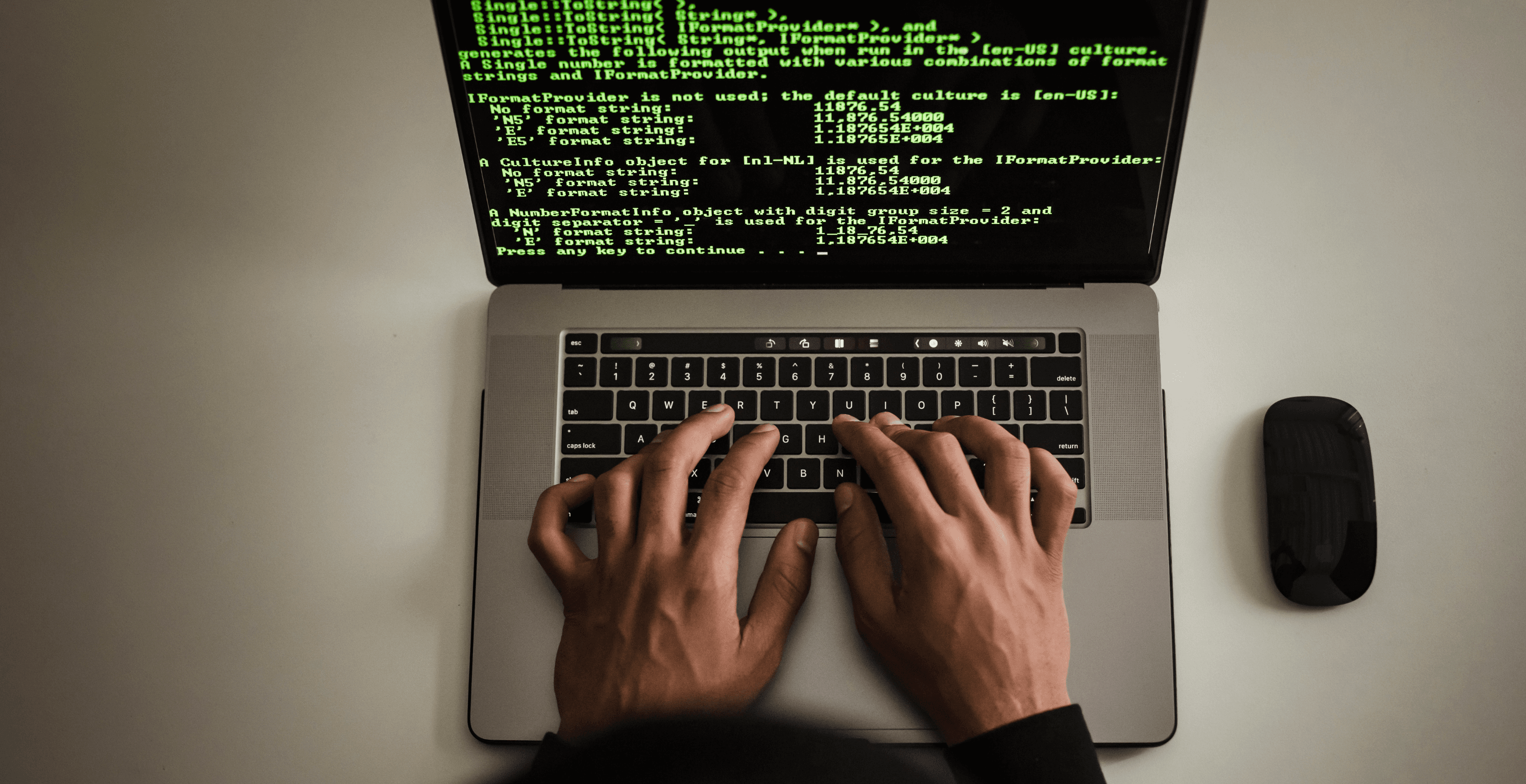
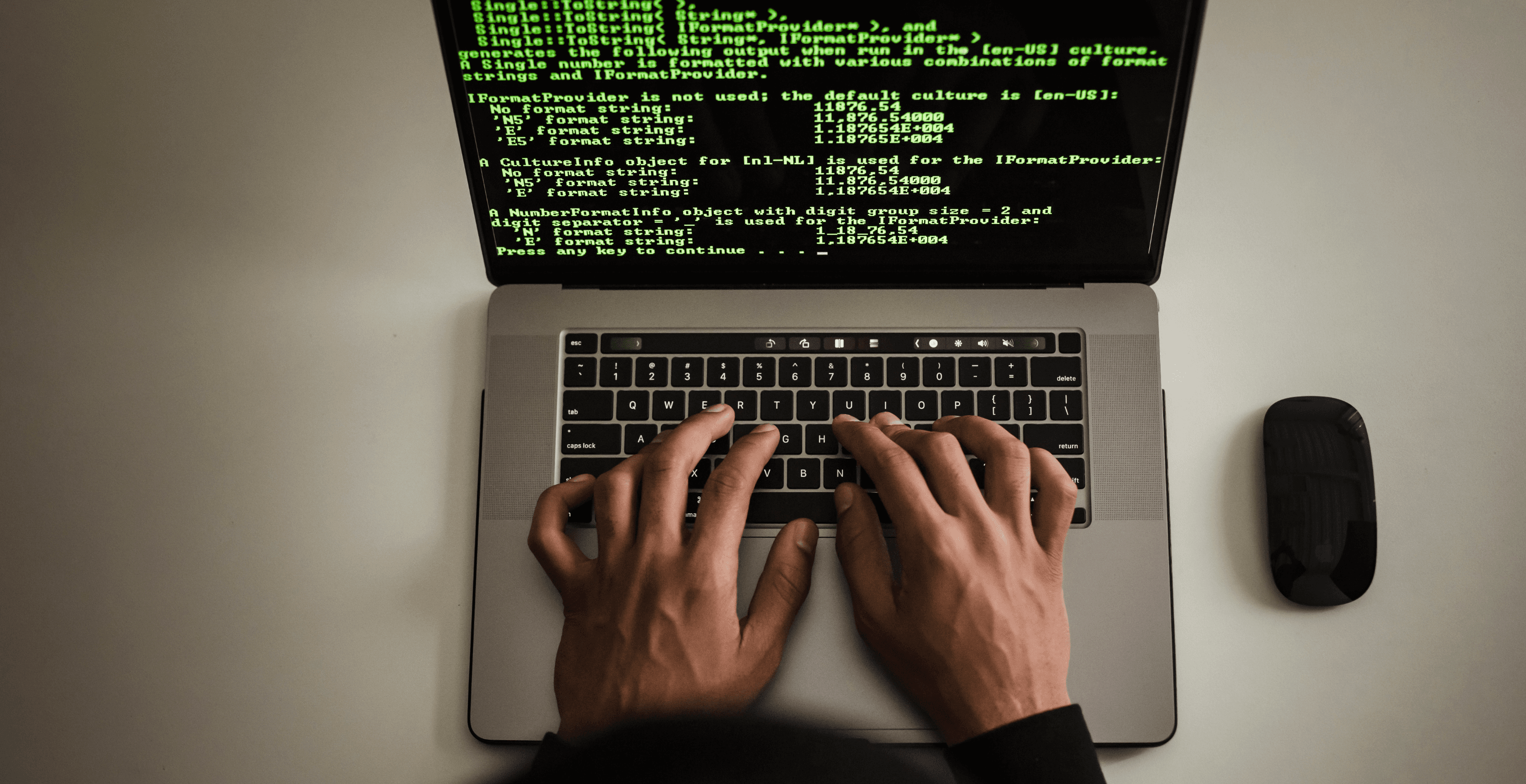
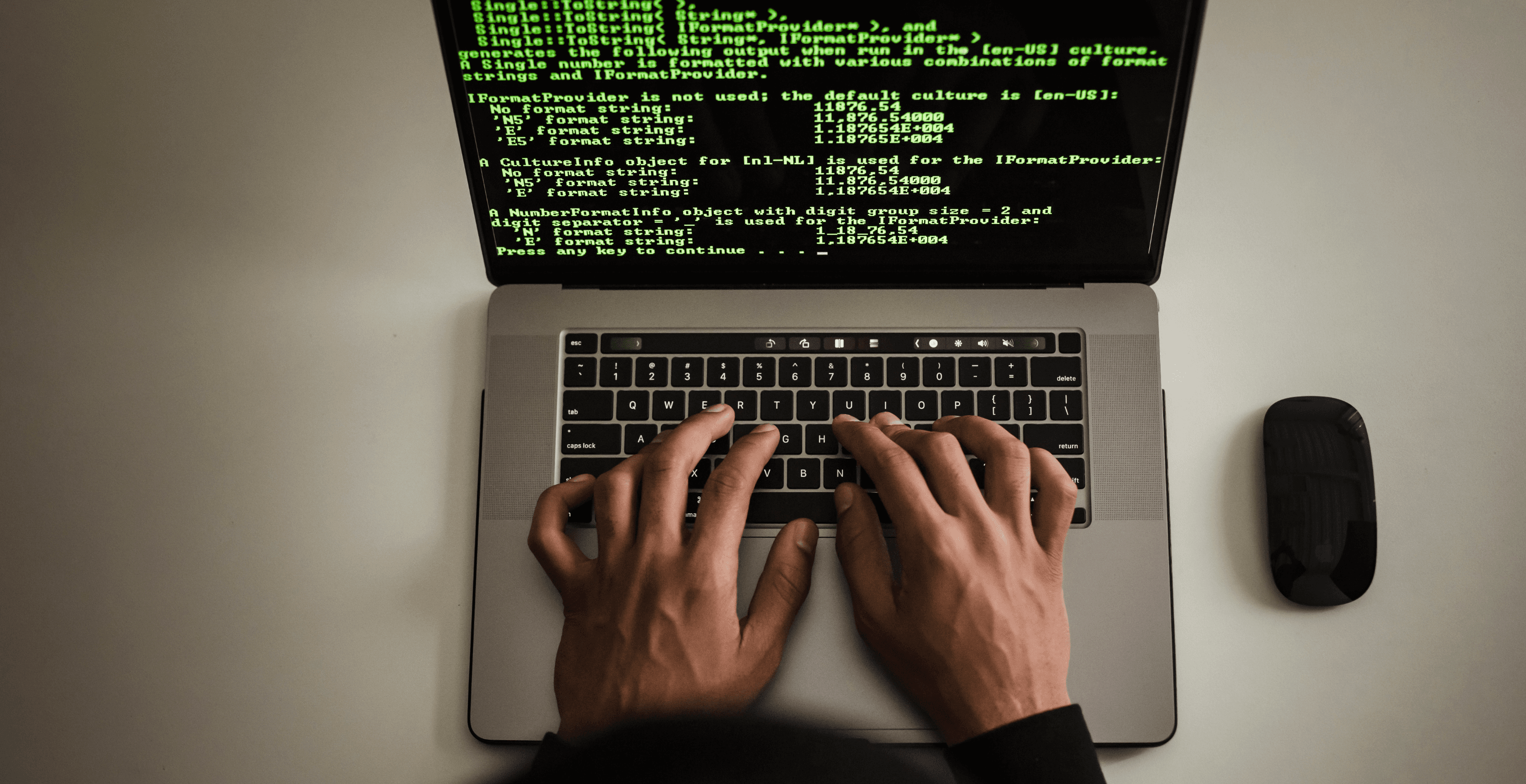
Transforming Conversational AI with Large Language Models

Rehan Asif
Nov 15, 2024
Read the article
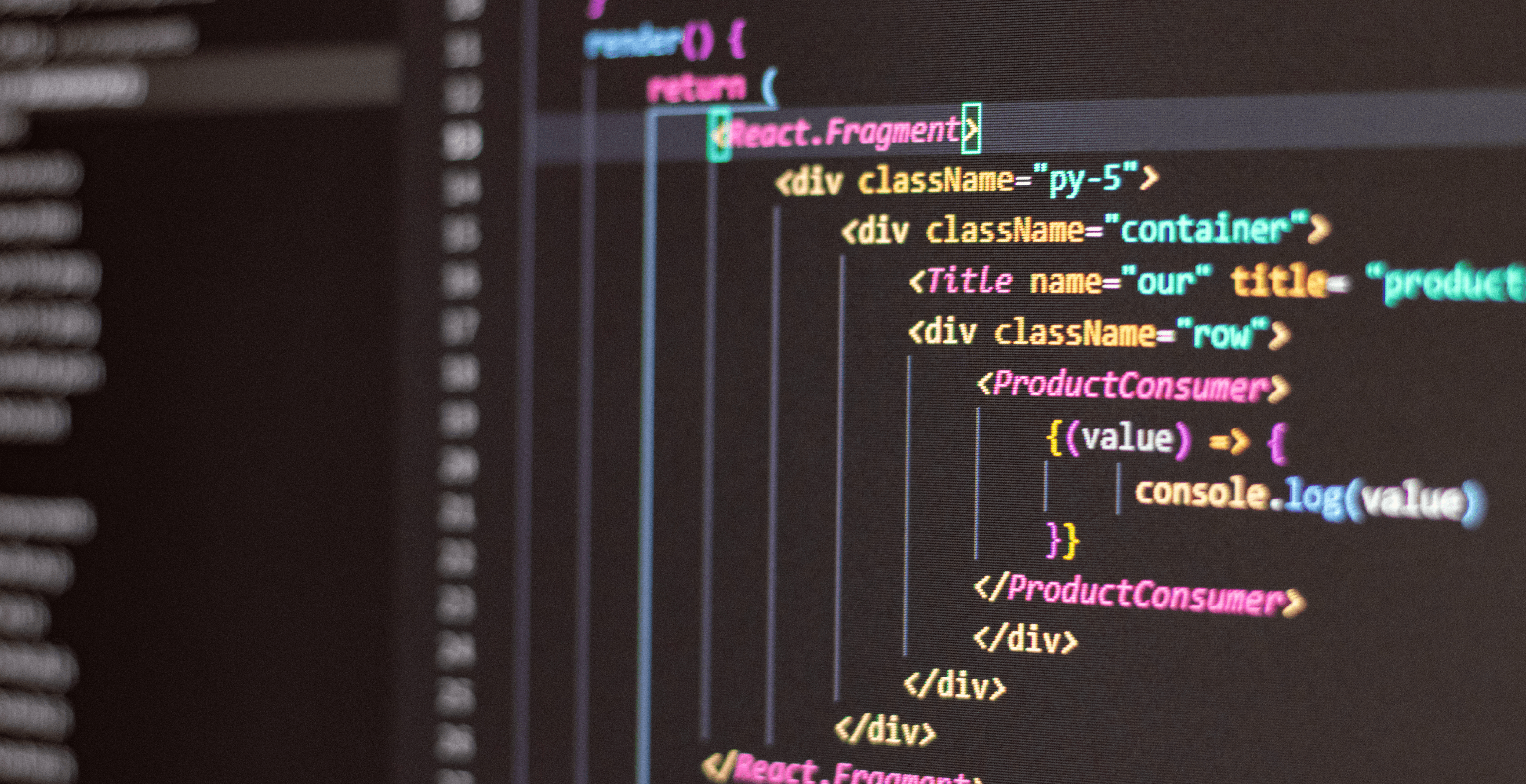
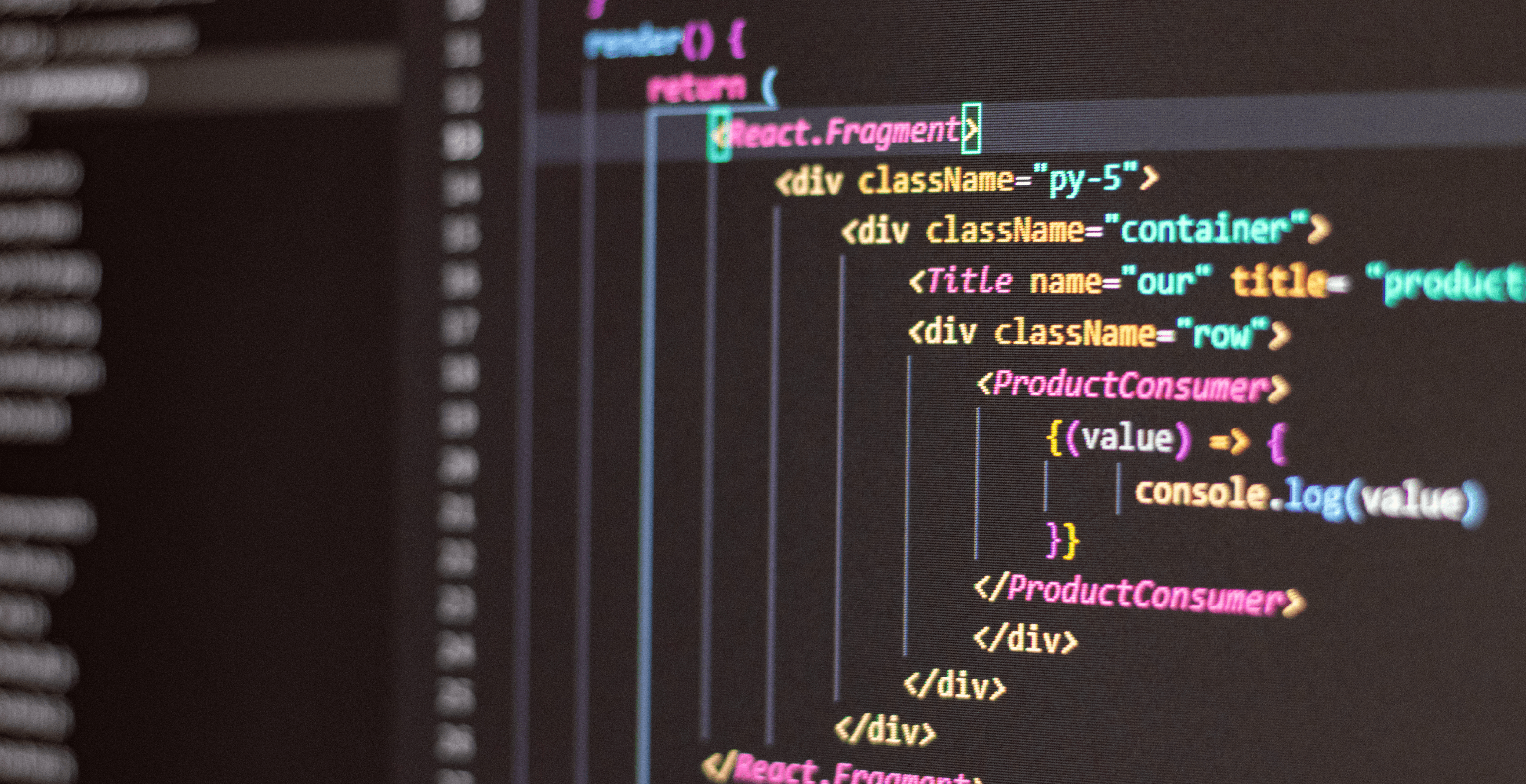
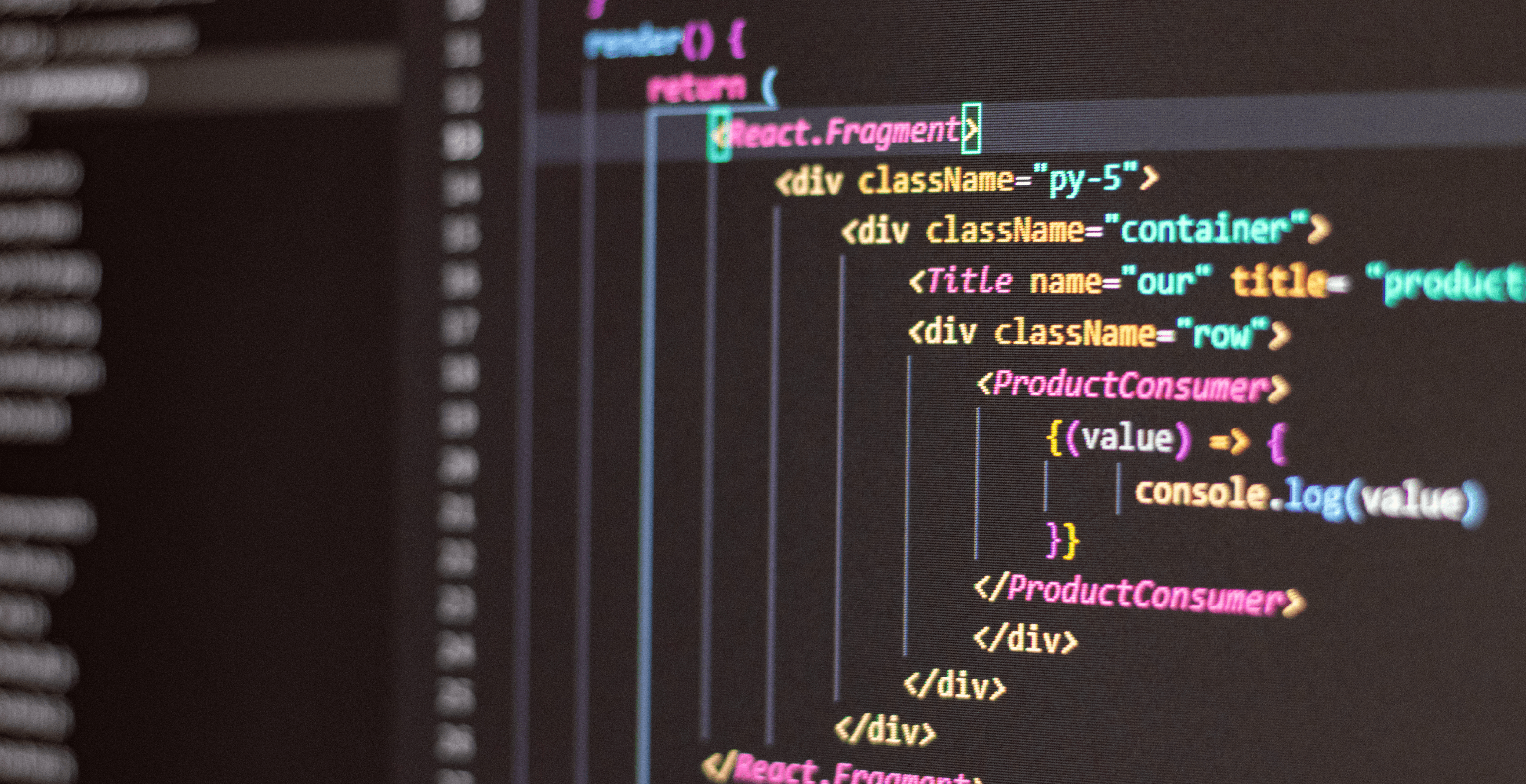
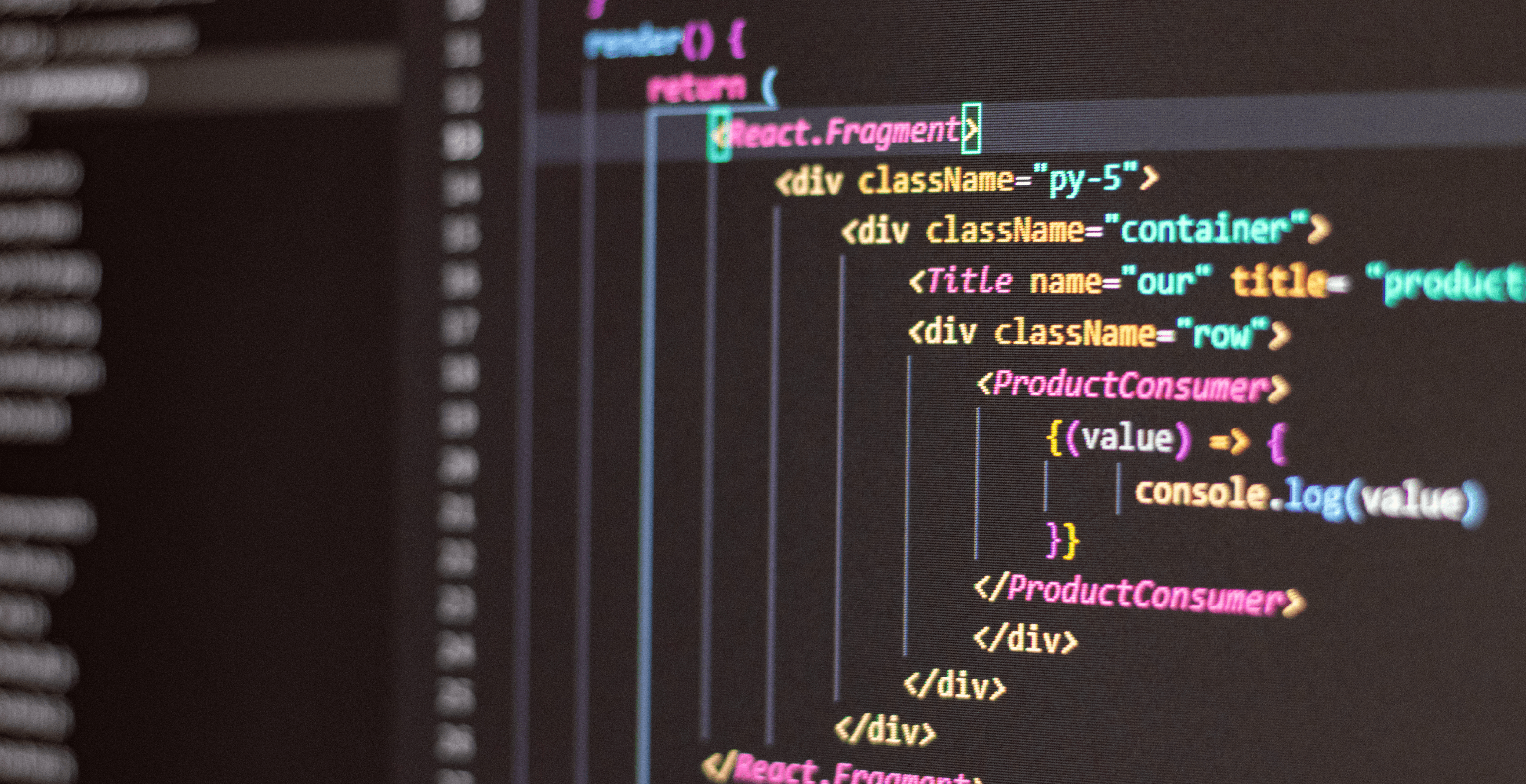
Deploying Generative AI Agents with Local LLMs

Rehan Asif
Nov 13, 2024
Read the article




Exploring Different Types of AI Agents with Key Examples
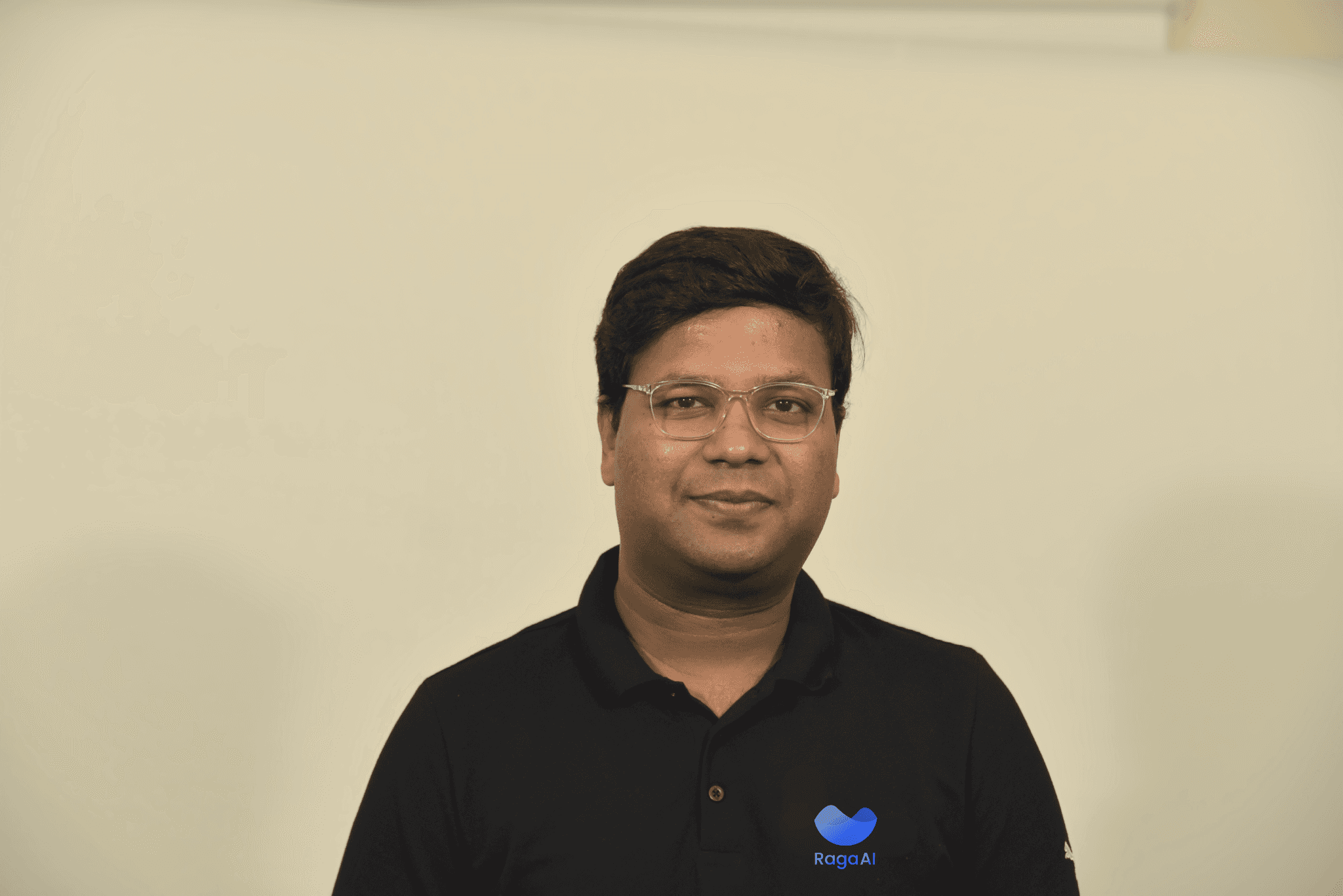
Jigar Gupta
Nov 11, 2024
Read the article




Creating Your Own Personal LLM Agents: Introduction to Implementation

Rehan Asif
Nov 8, 2024
Read the article




Exploring Agentic AI Architecture and Design Patterns
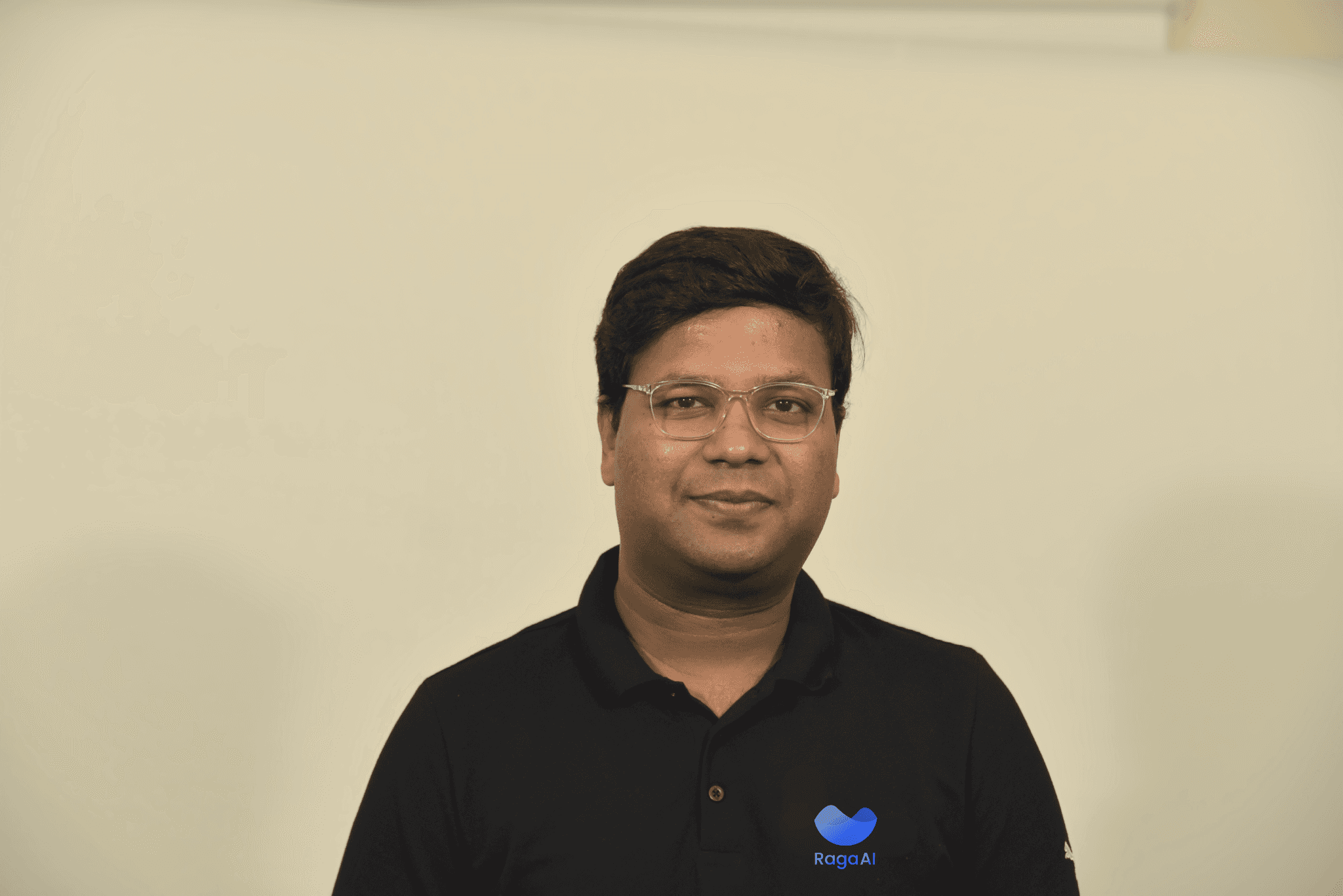
Jigar Gupta
Nov 6, 2024
Read the article
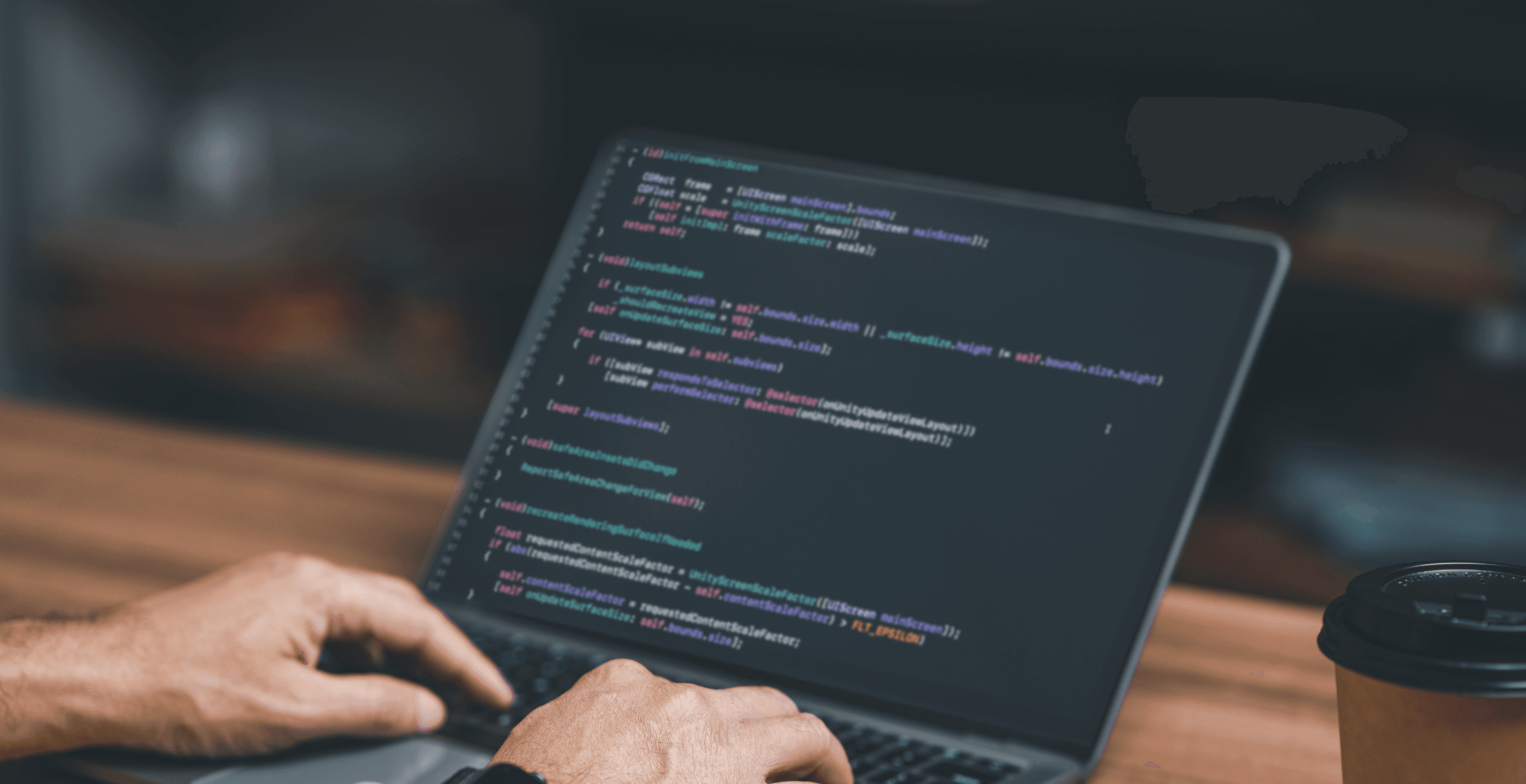
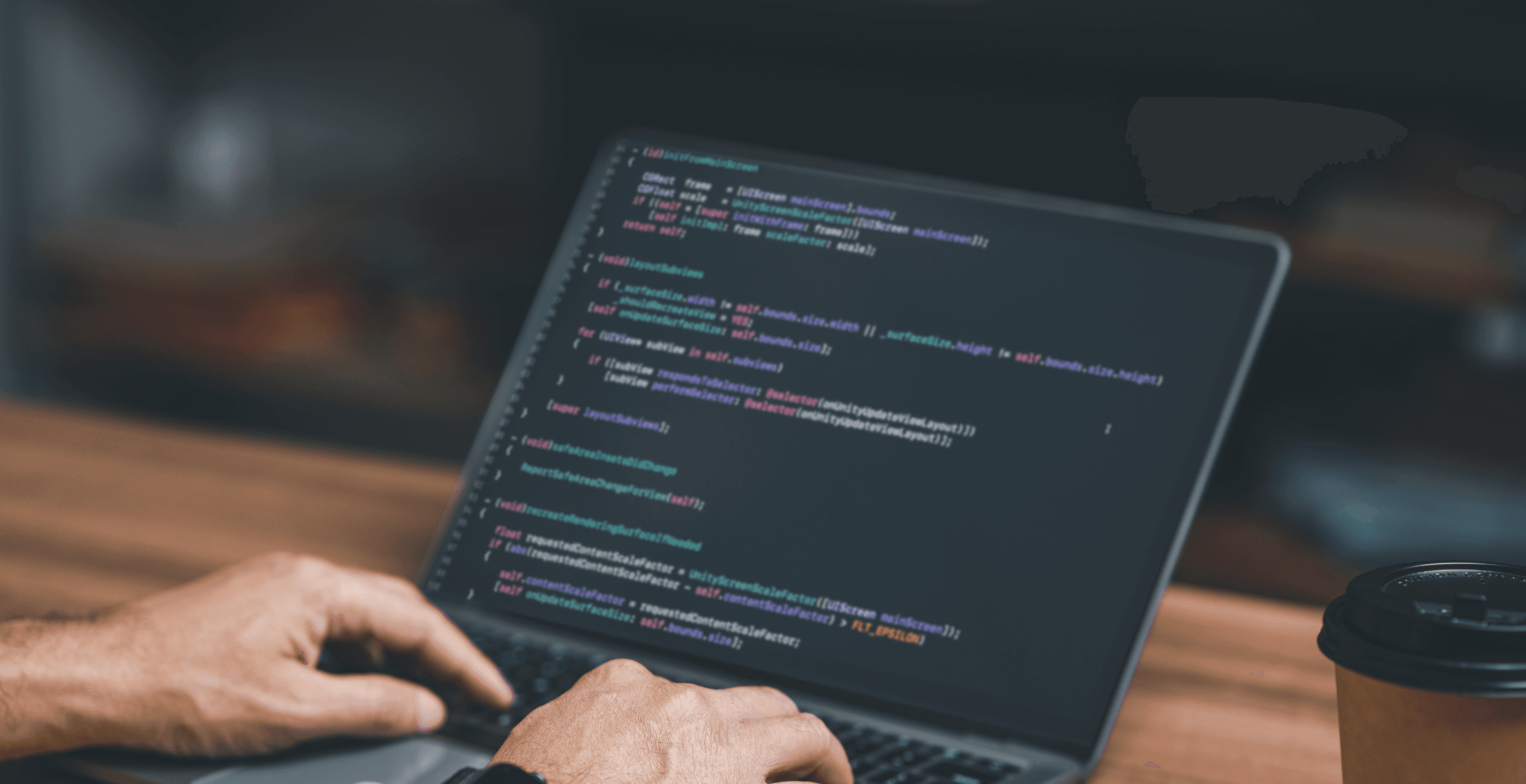
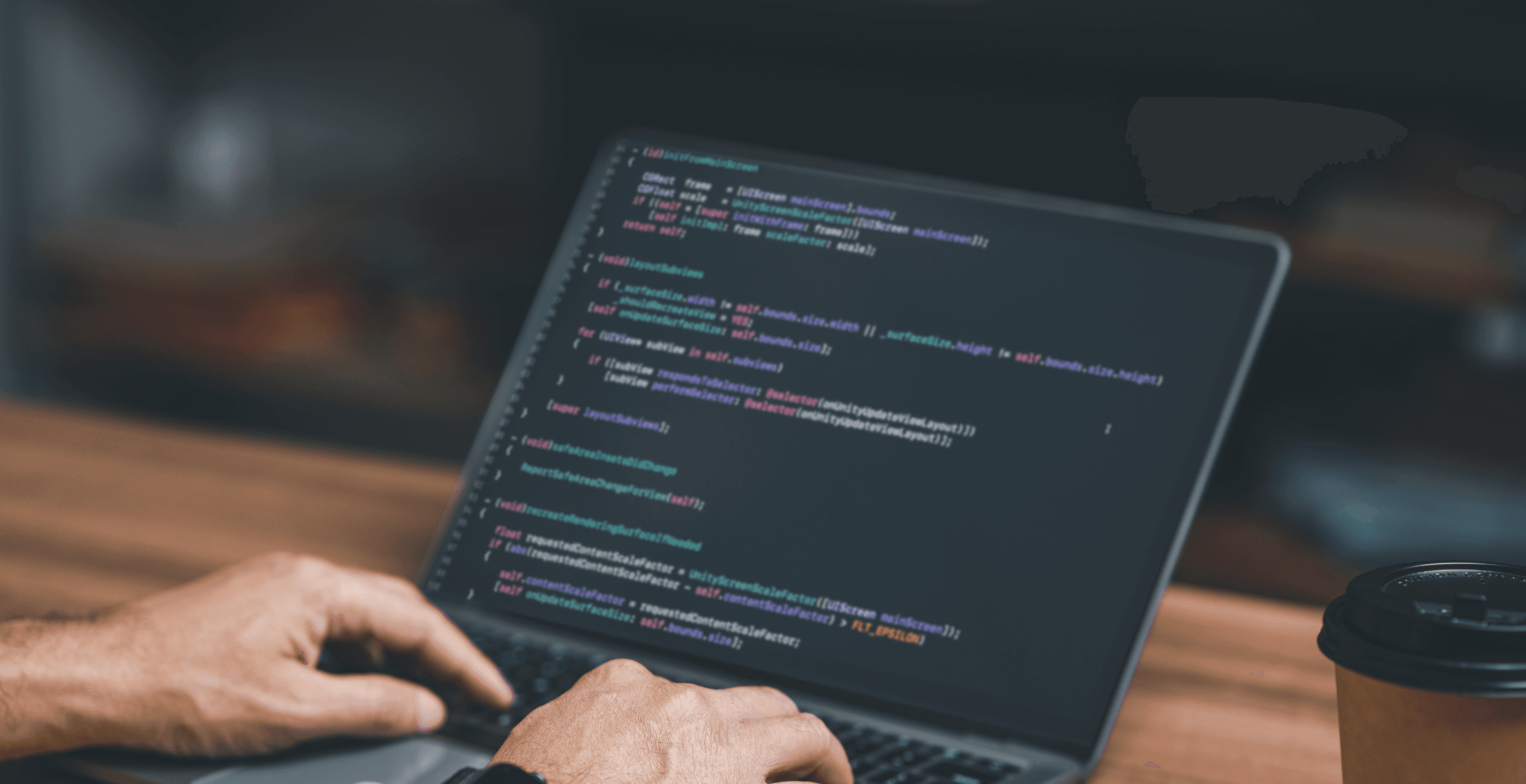
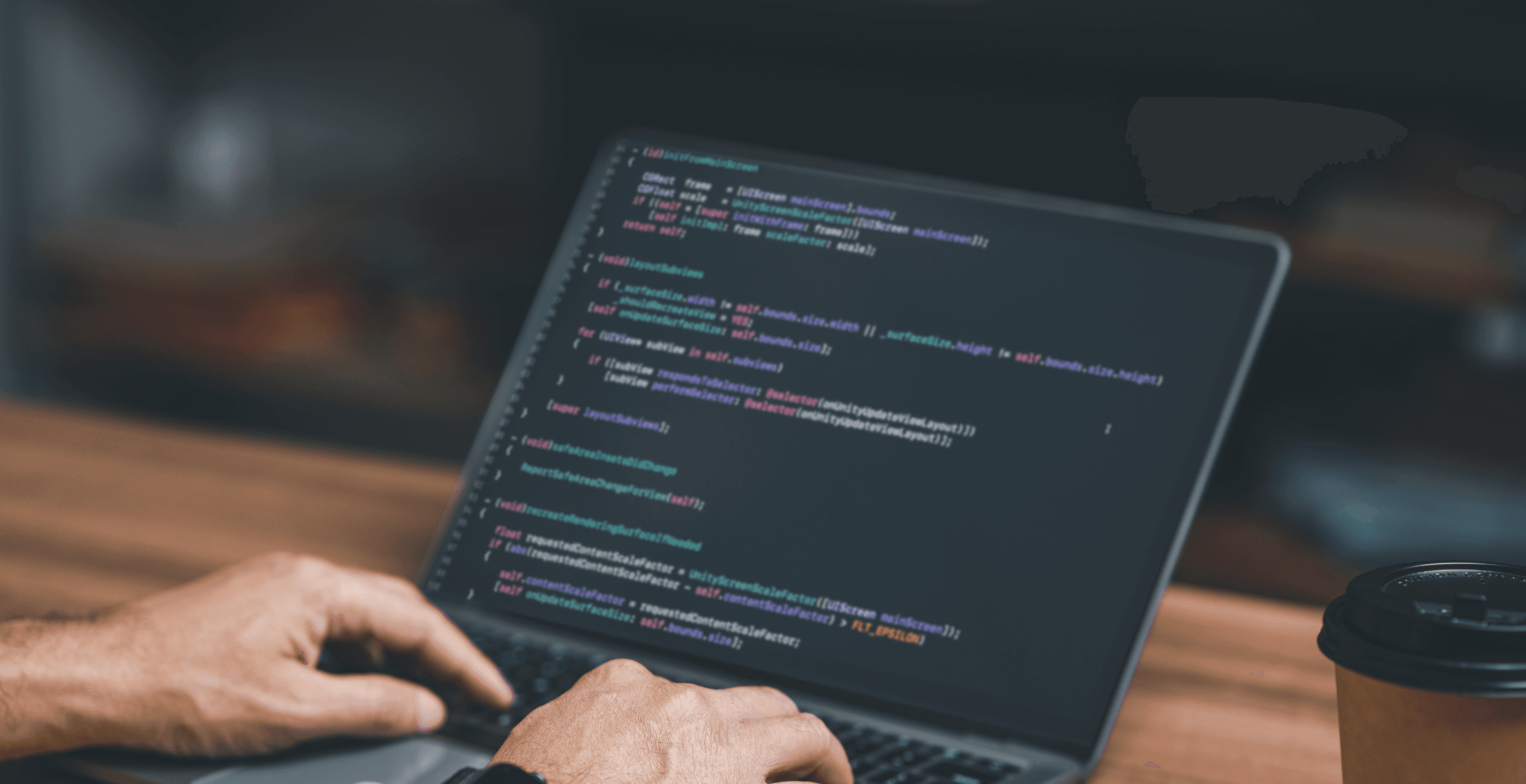
Building Your First LLM Agent Framework Application

Rehan Asif
Nov 4, 2024
Read the article




Multi-Agent Design and Collaboration Patterns

Rehan Asif
Nov 1, 2024
Read the article




Creating Your Own LLM Agent Application from Scratch

Rehan Asif
Oct 30, 2024
Read the article




Solving LLM Token Limit Issues: Understanding and Approaches

Rehan Asif
Oct 27, 2024
Read the article
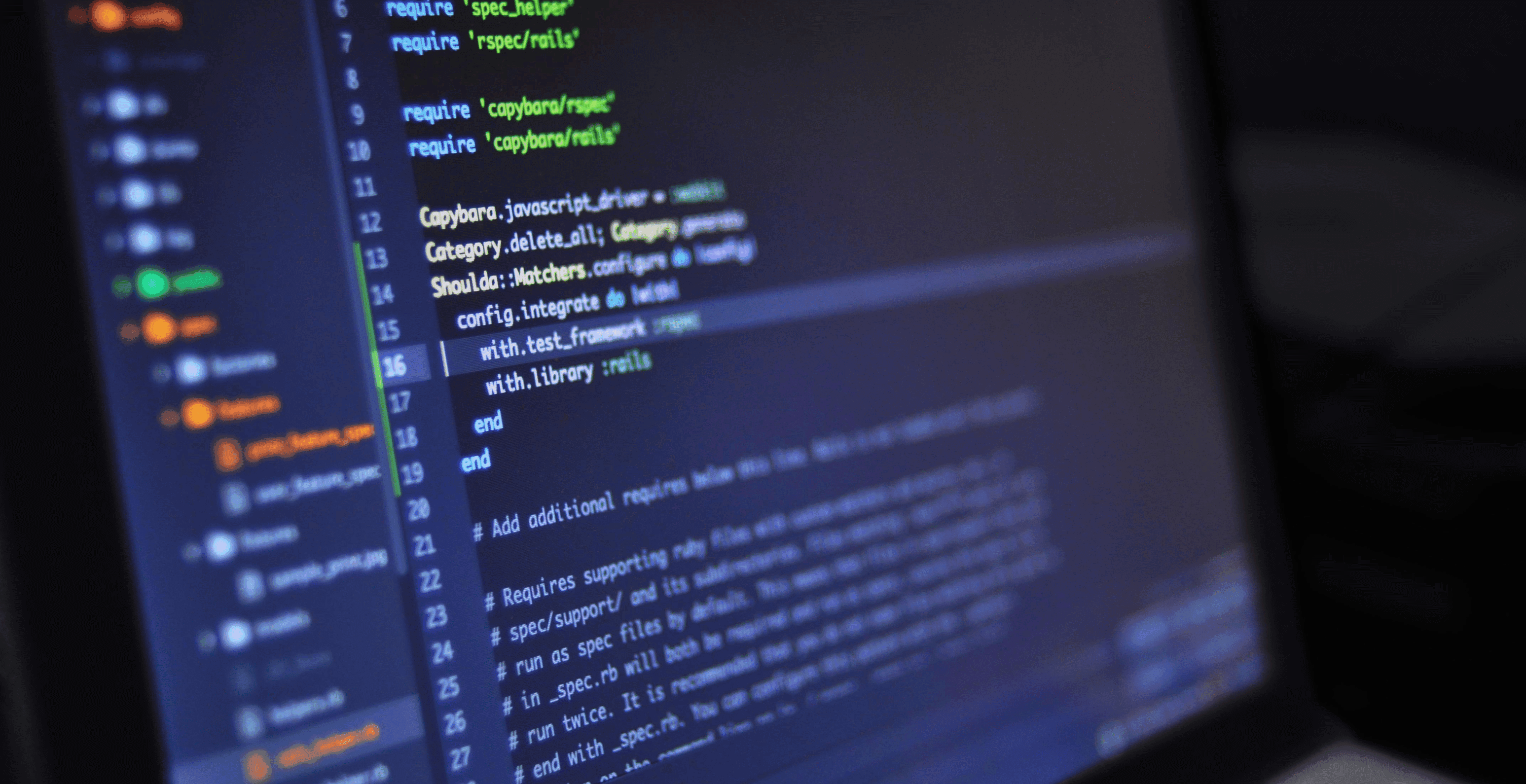
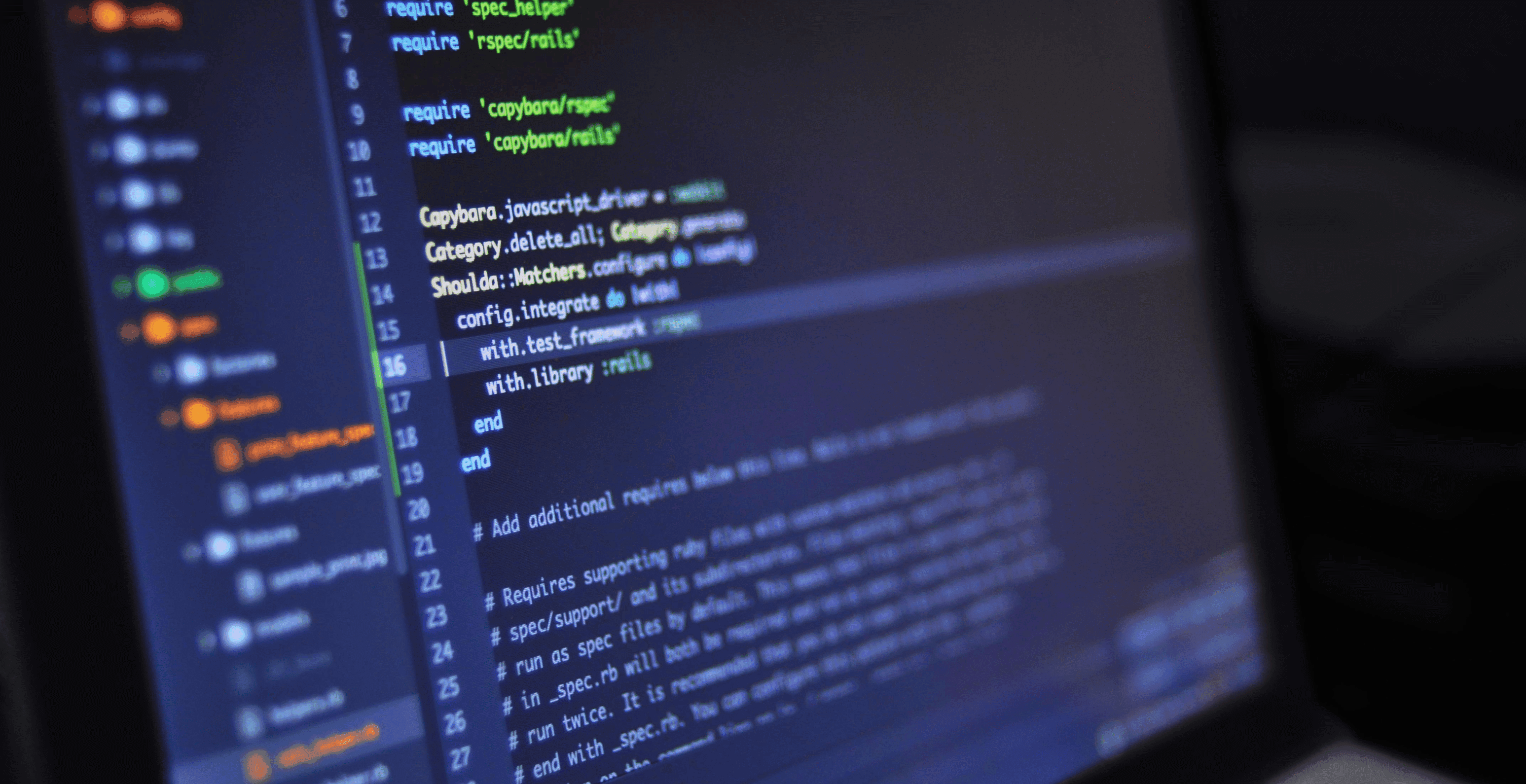
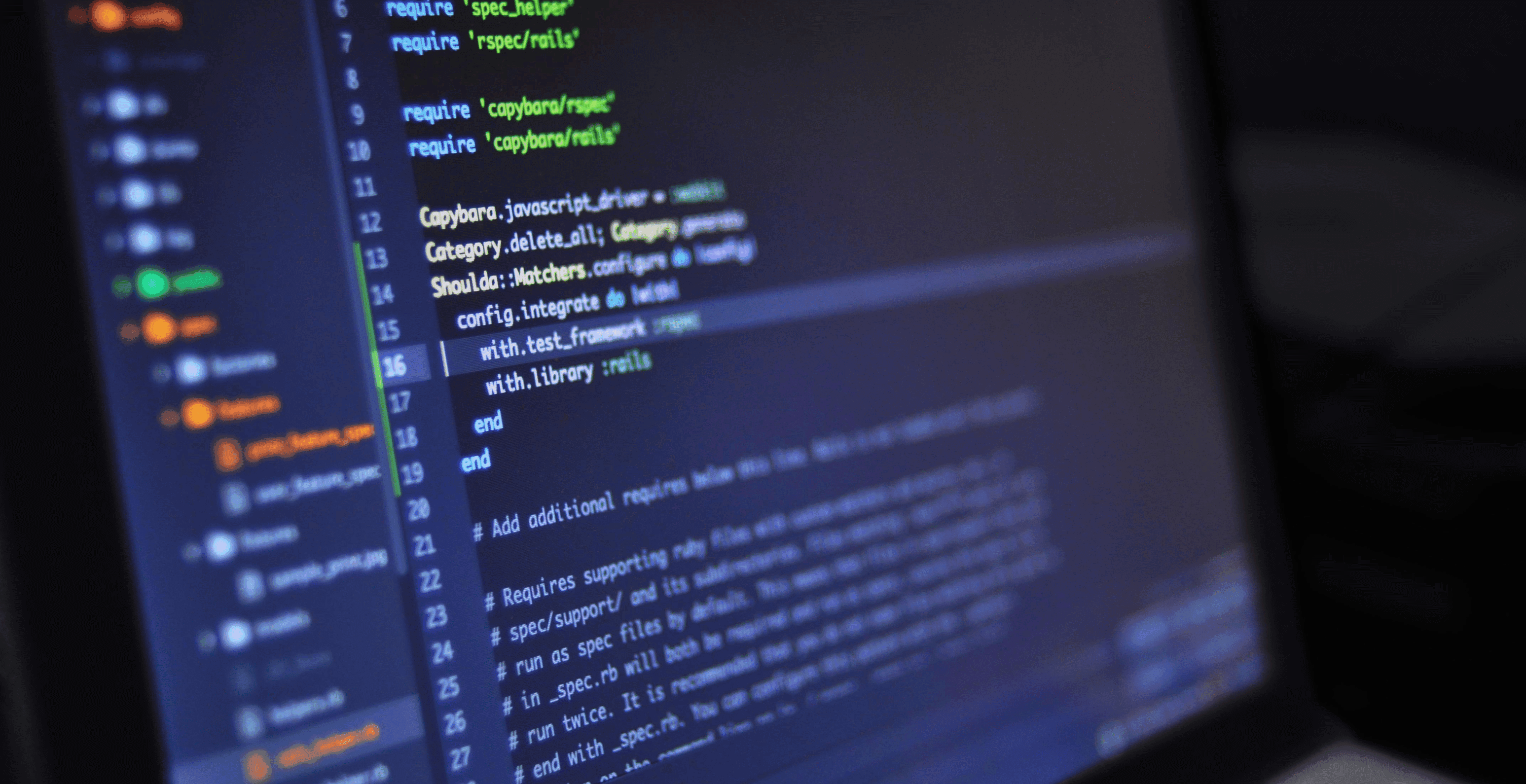
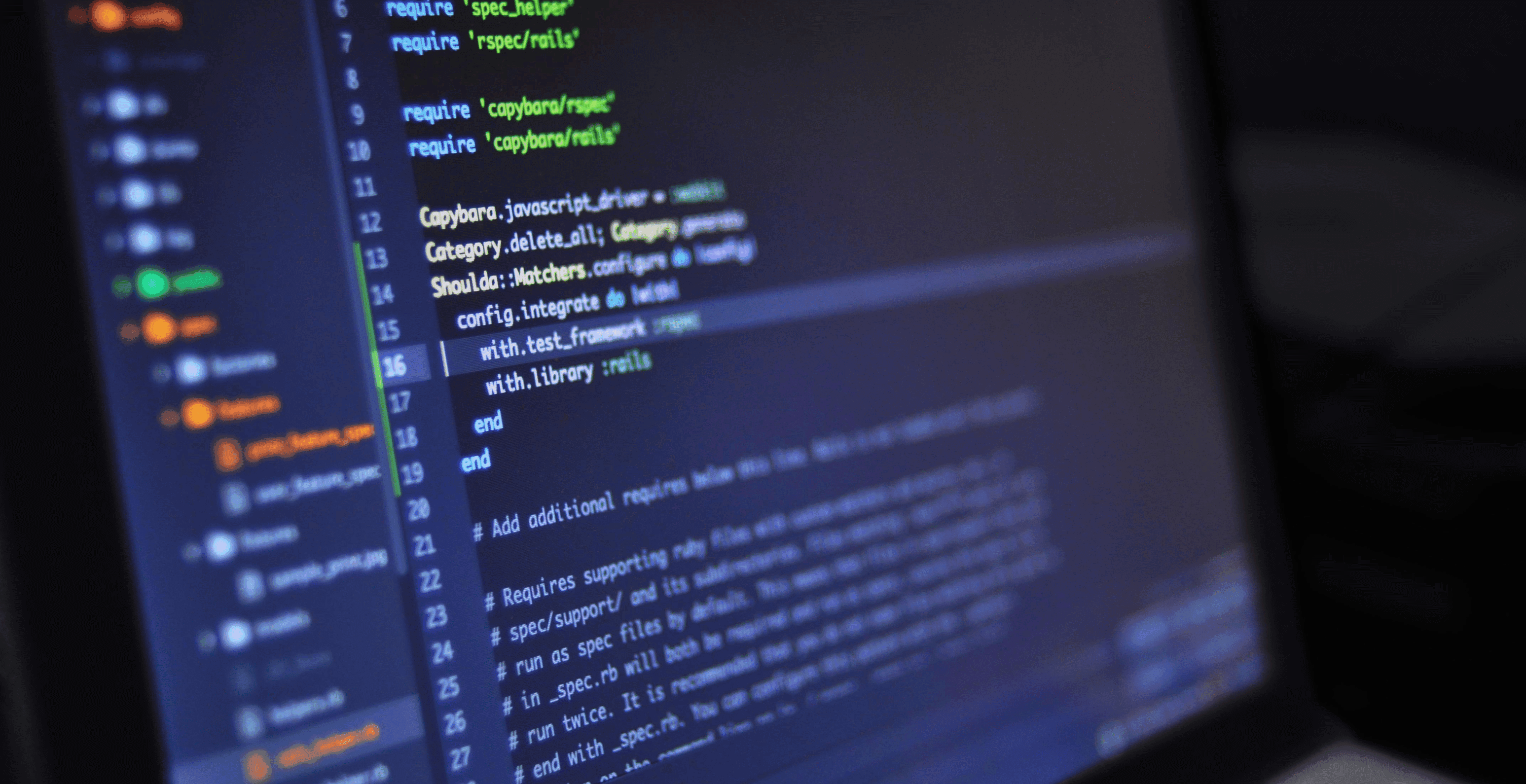
Understanding the Impact of Inference Cost on Generative AI Adoption
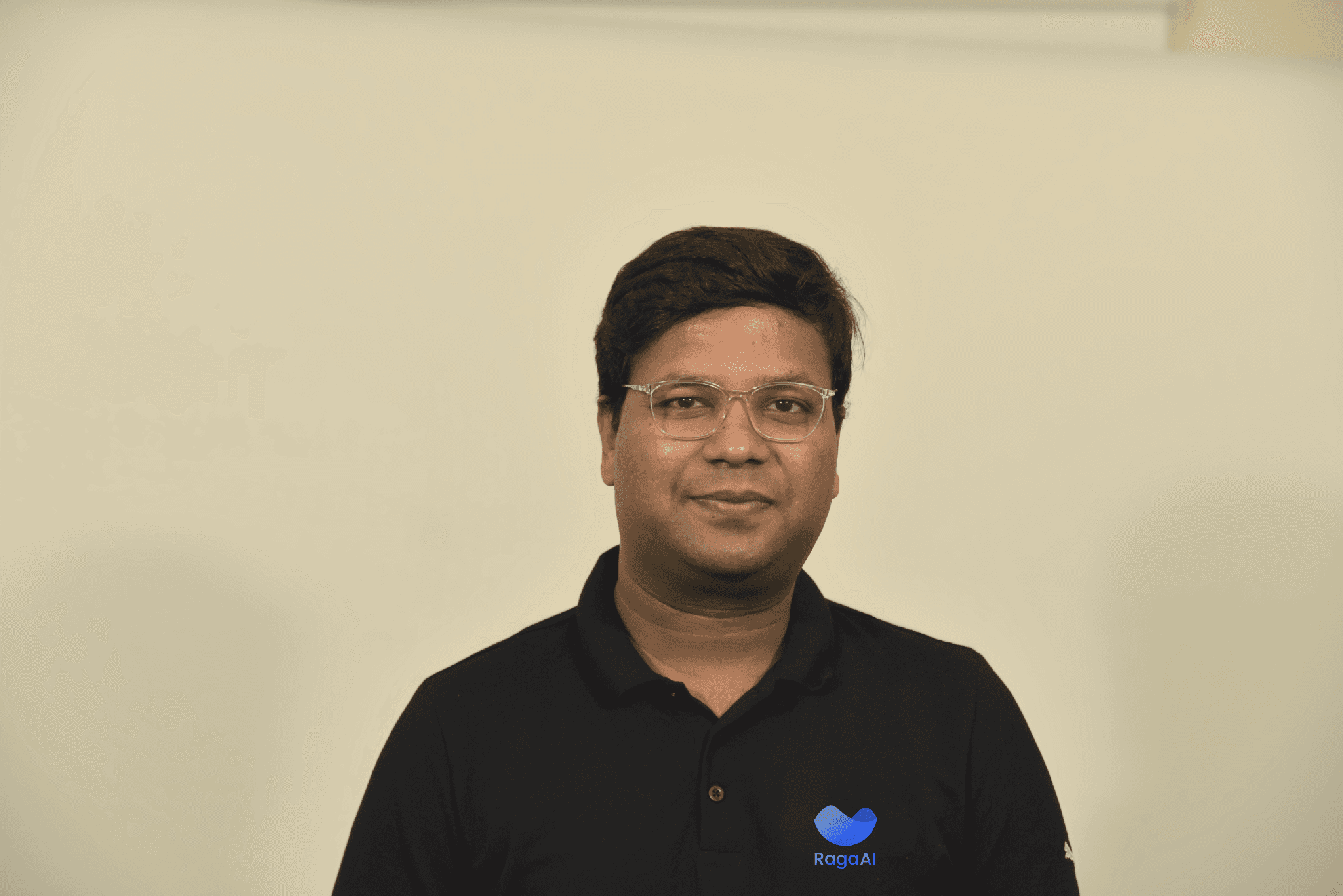
Jigar Gupta
Oct 24, 2024
Read the article




Data Security: Risks, Solutions, Types and Best Practices
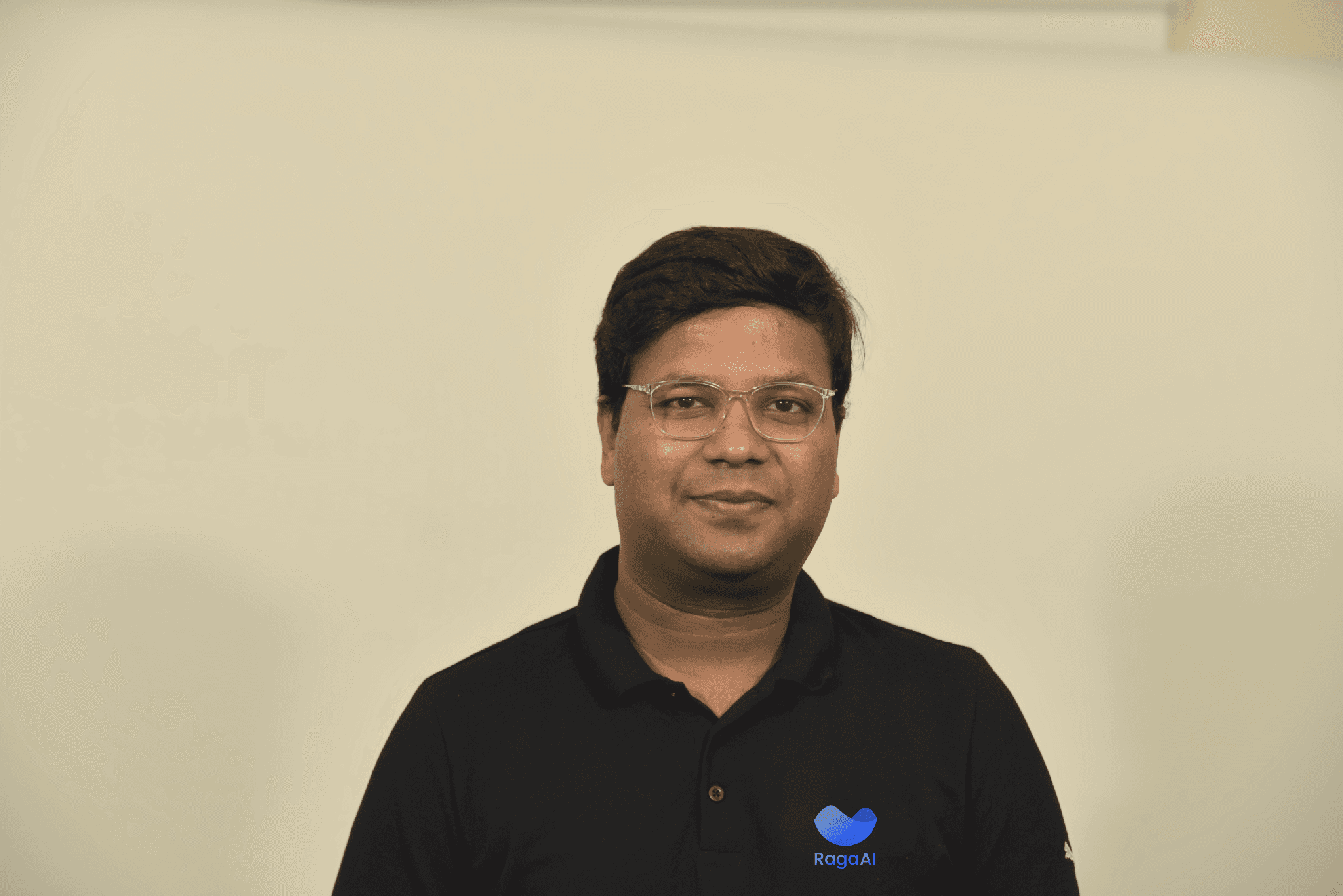
Jigar Gupta
Oct 21, 2024
Read the article
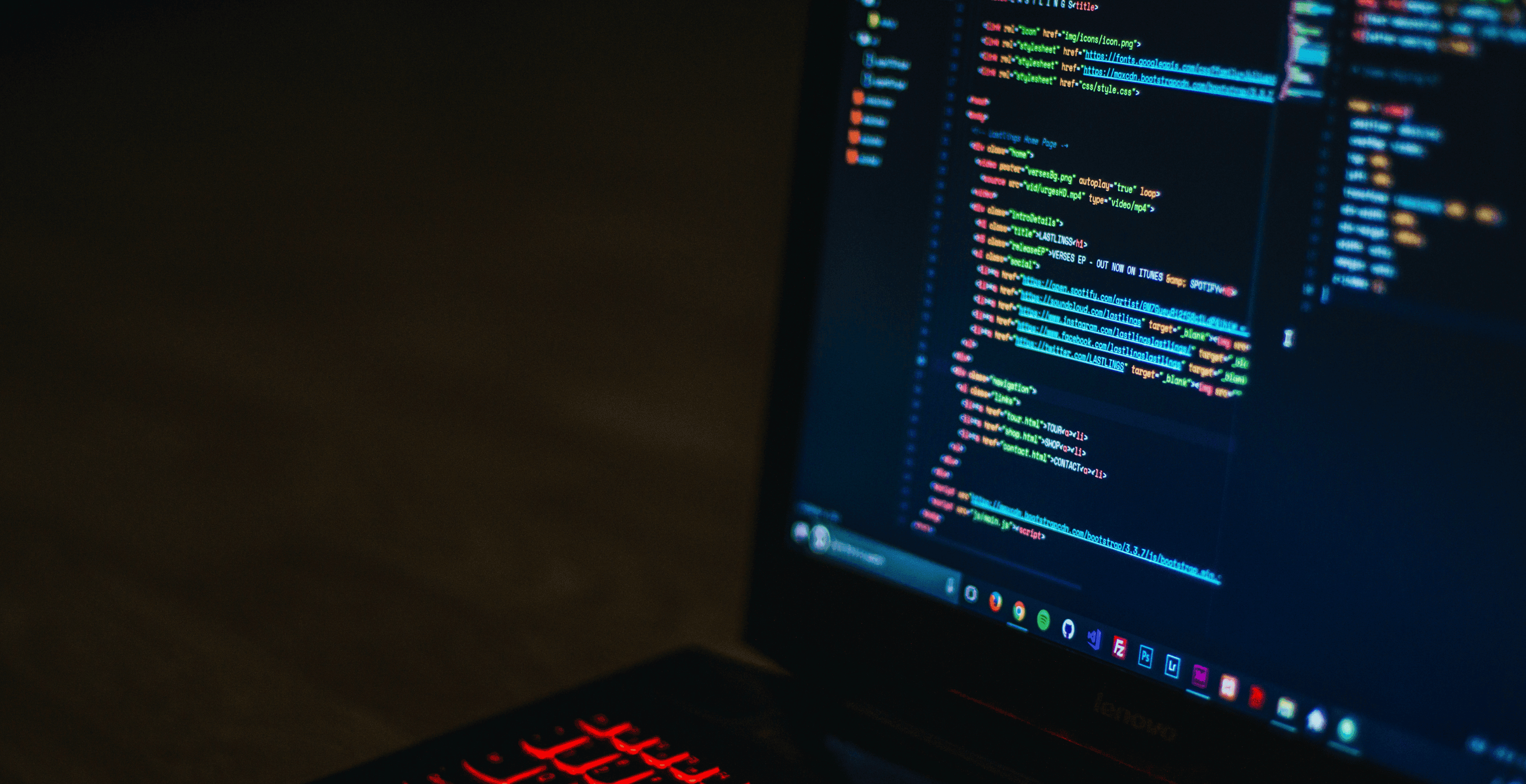
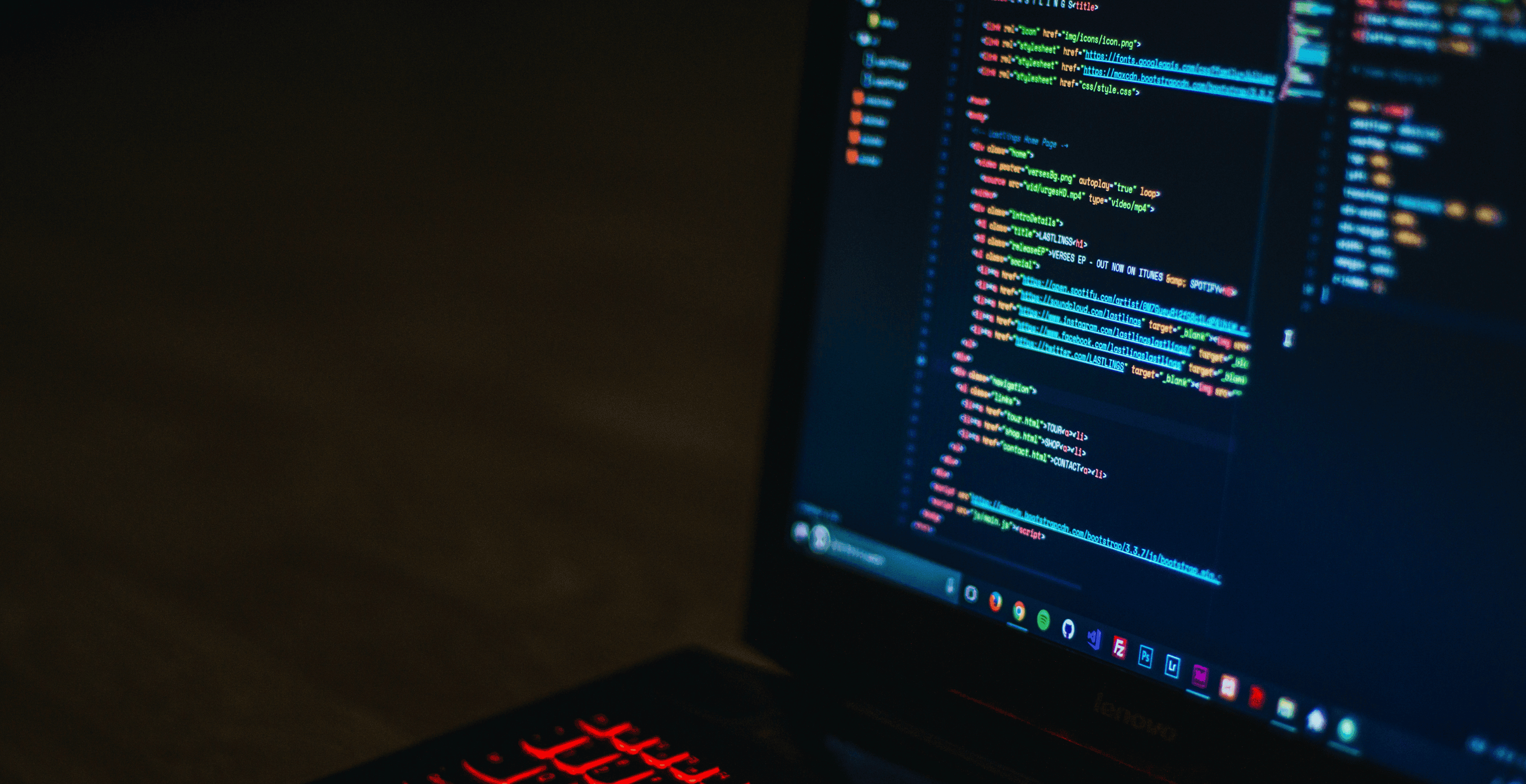
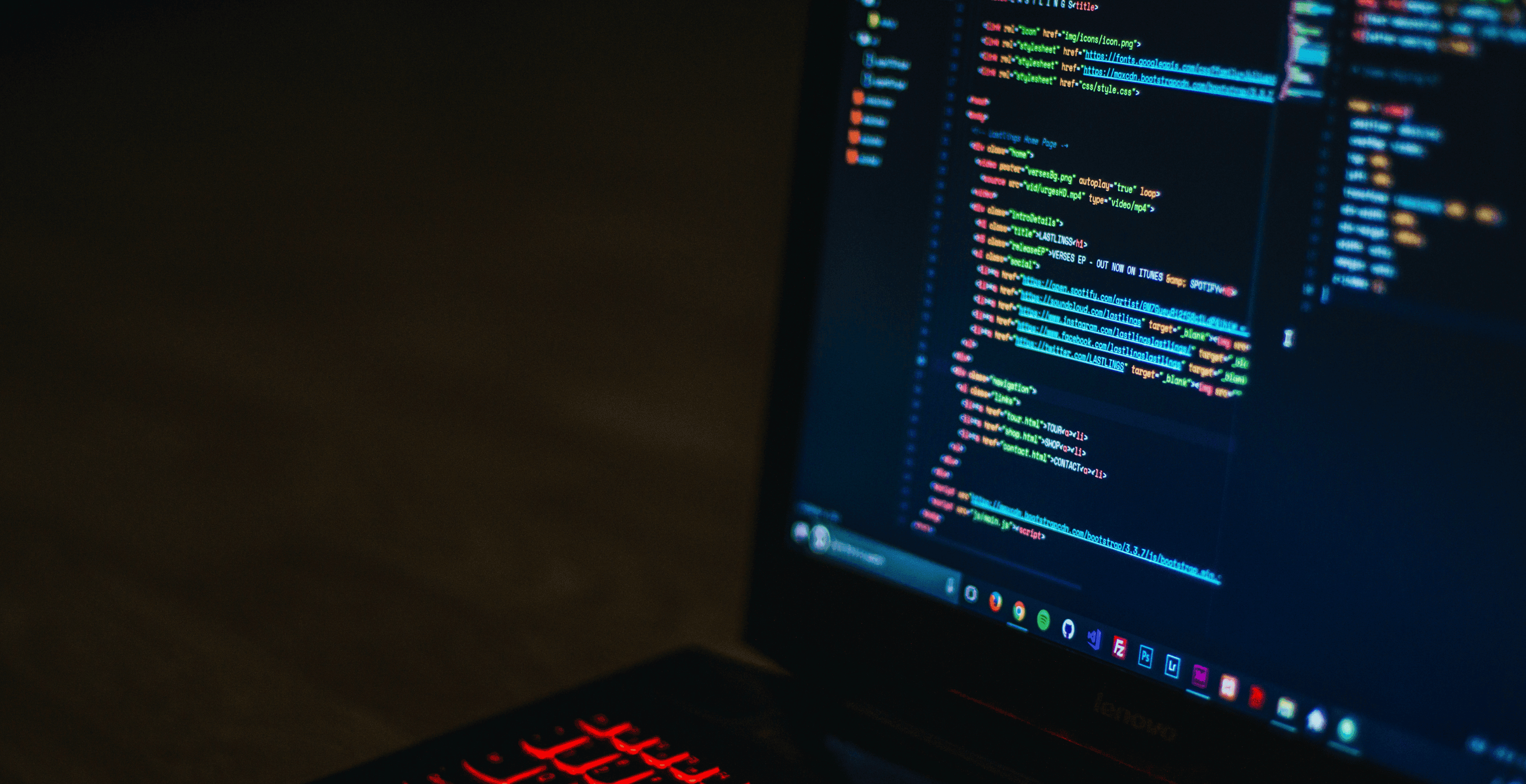
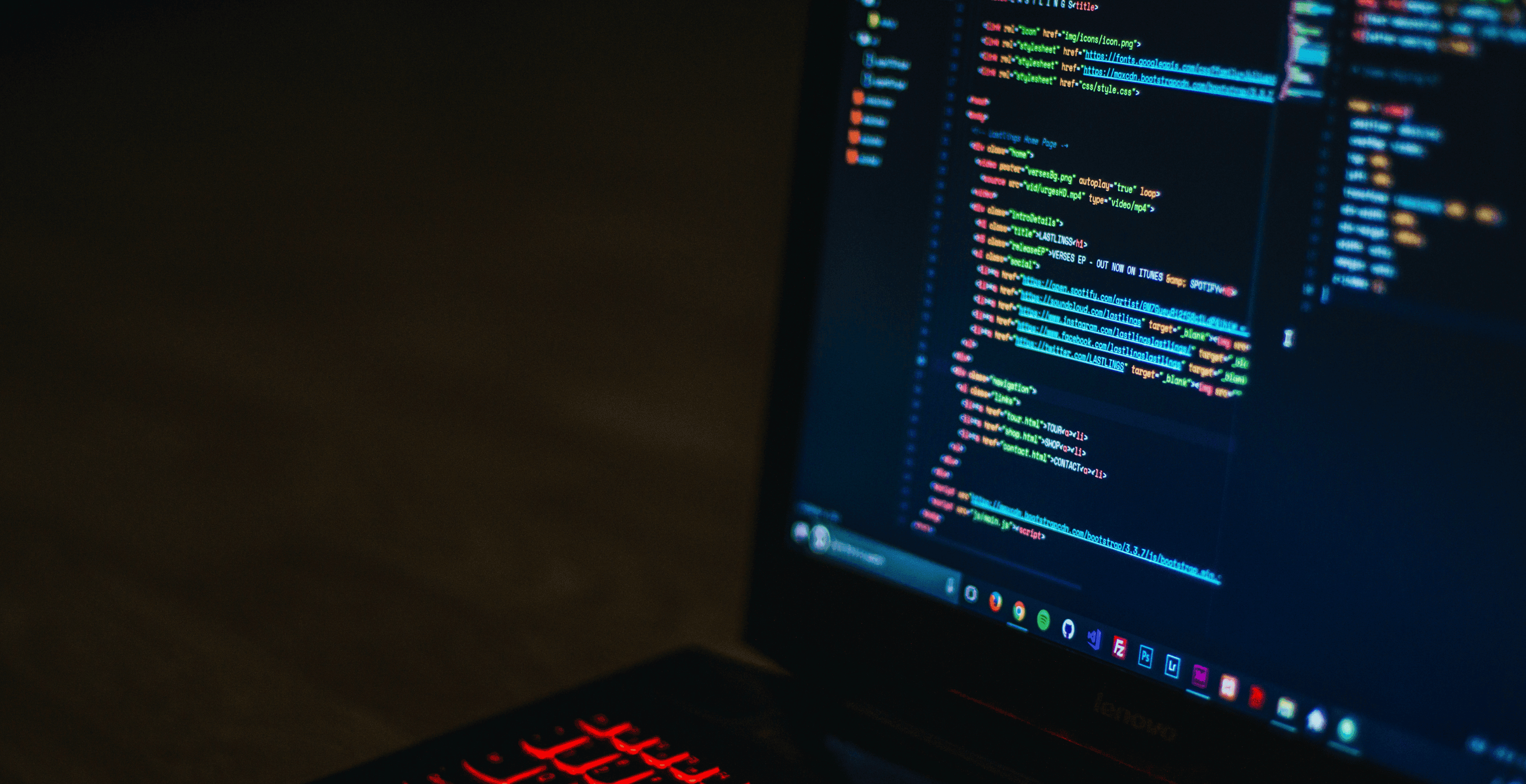
Getting Contextual Understanding Right for RAG Applications
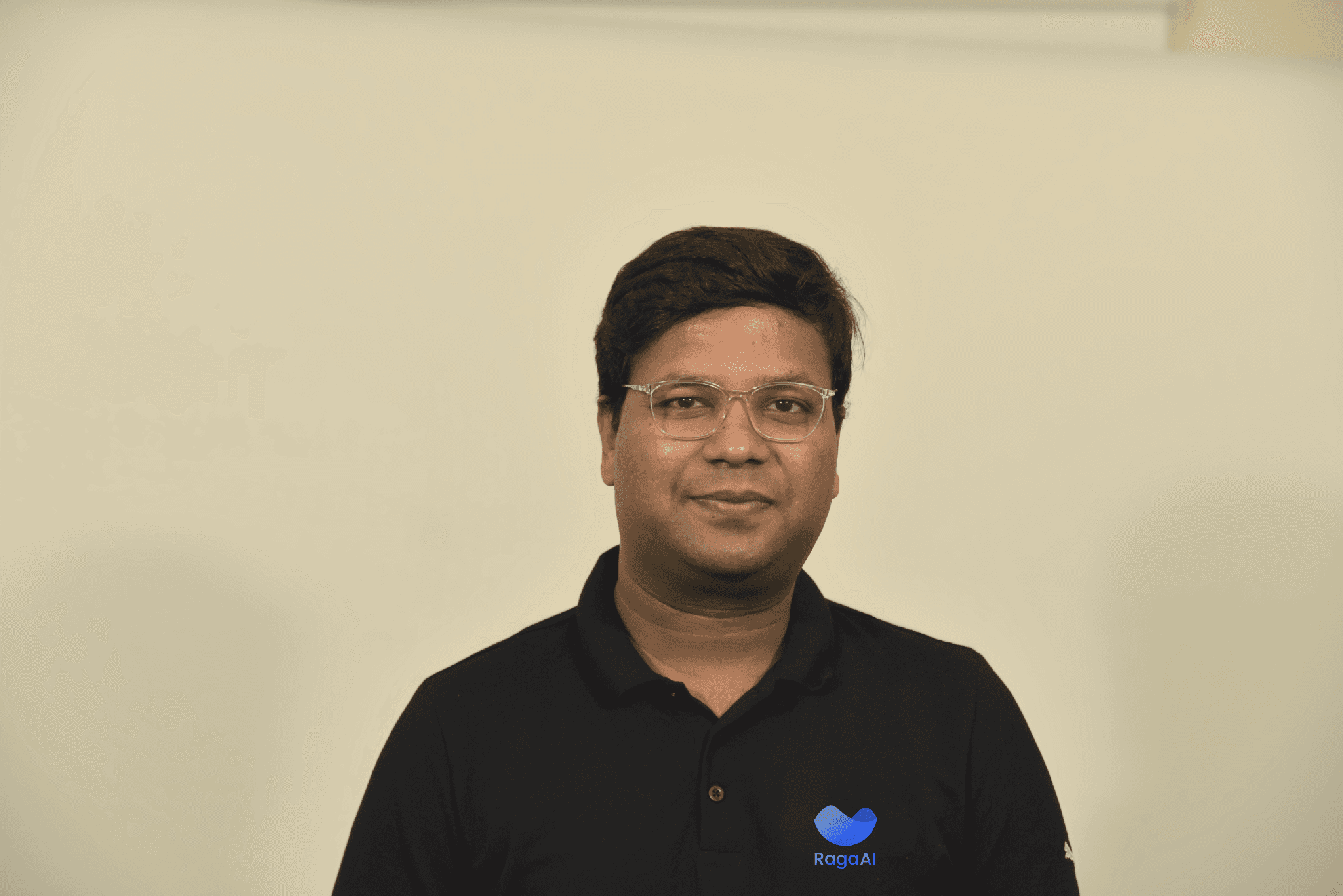
Jigar Gupta
Oct 19, 2024
Read the article
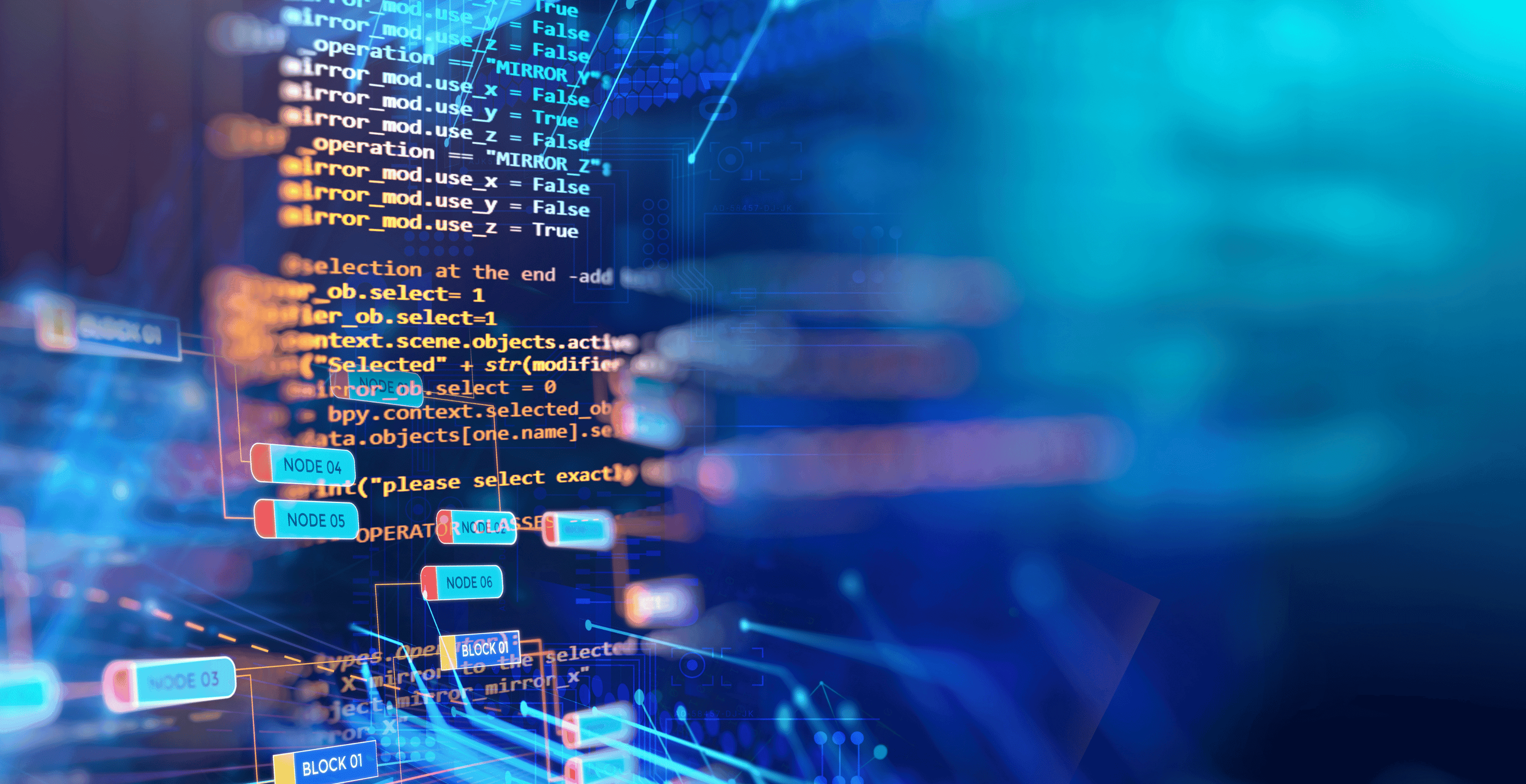
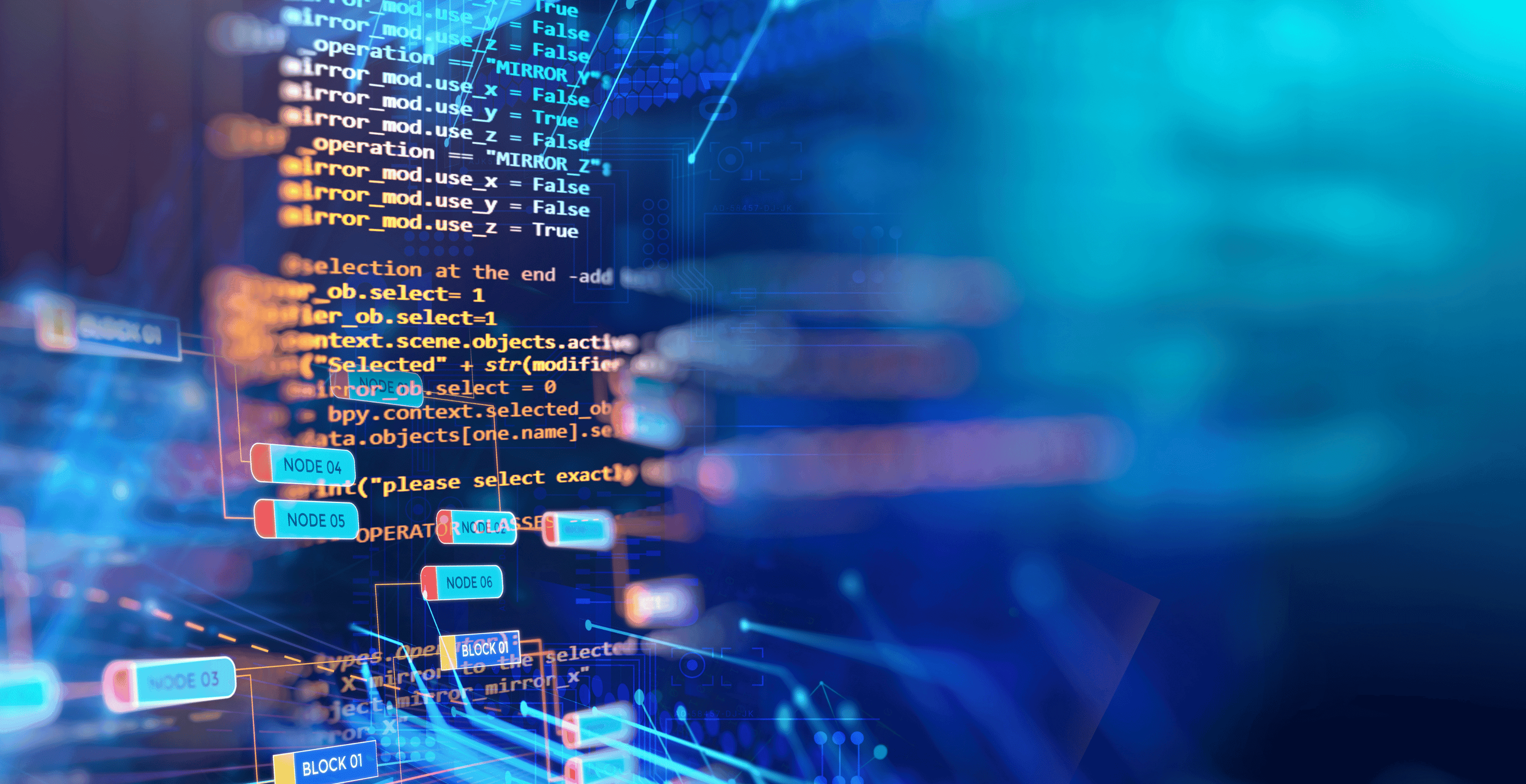
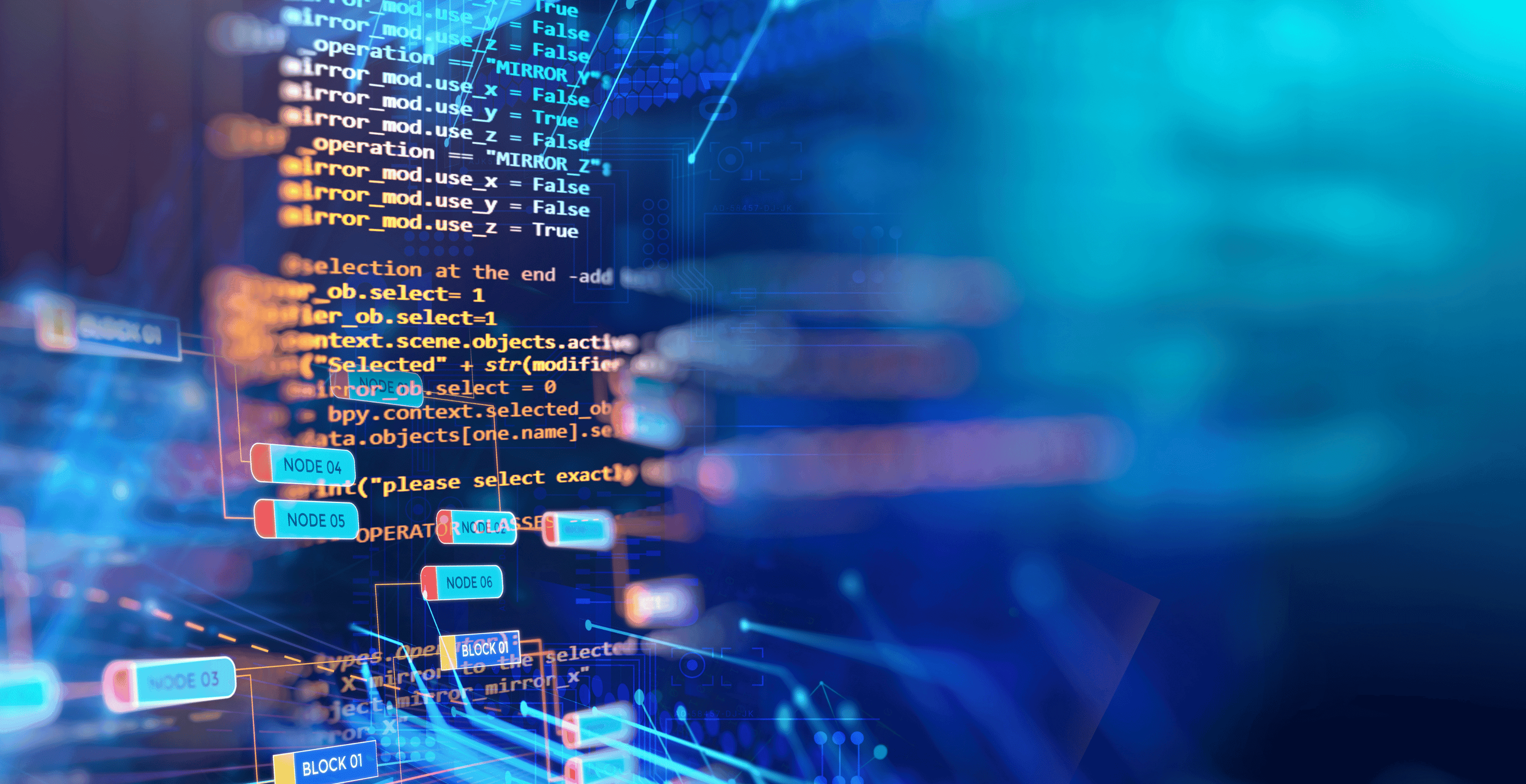
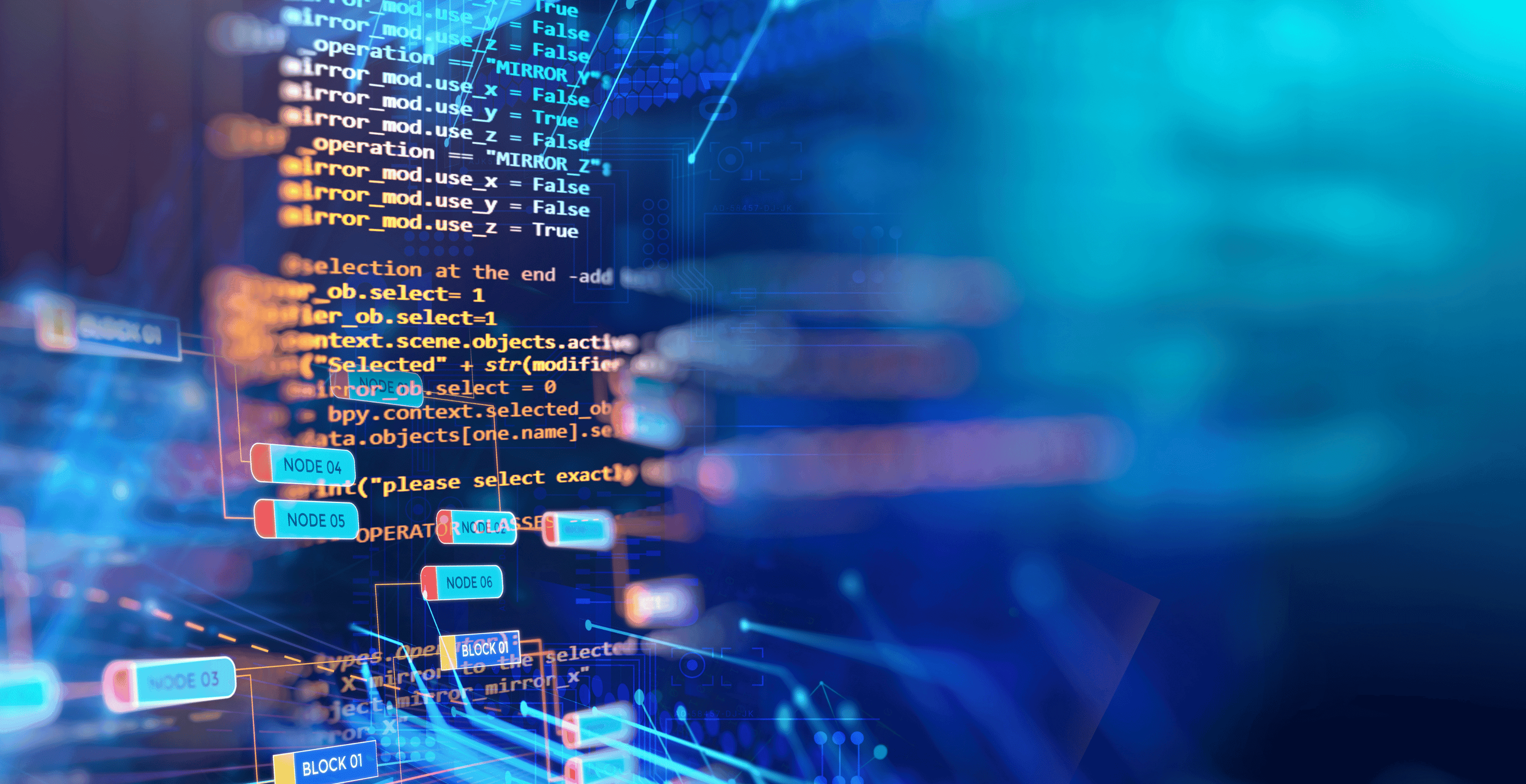
Understanding Data Fragmentation and Strategies to Overcome It
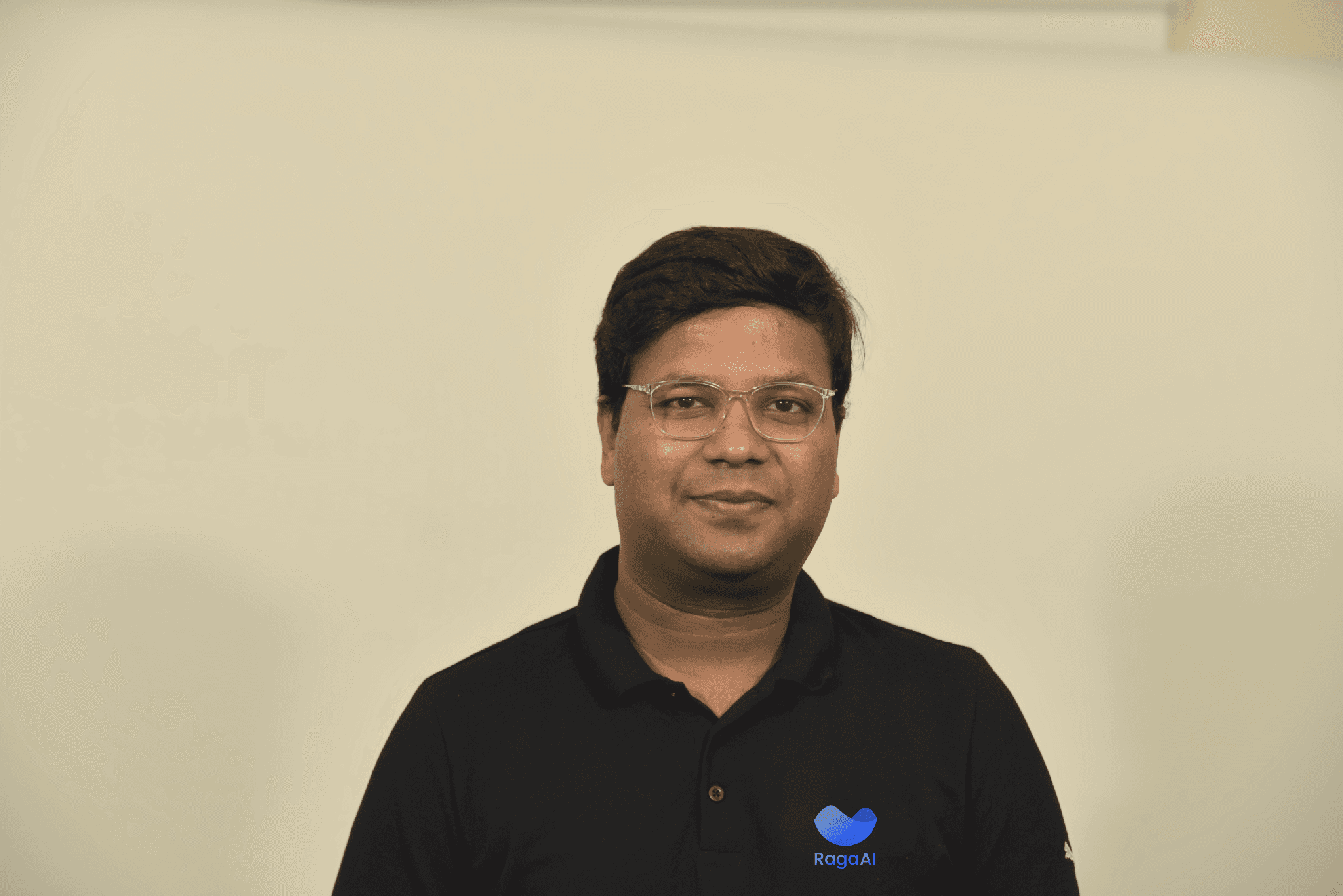
Jigar Gupta
Oct 16, 2024
Read the article
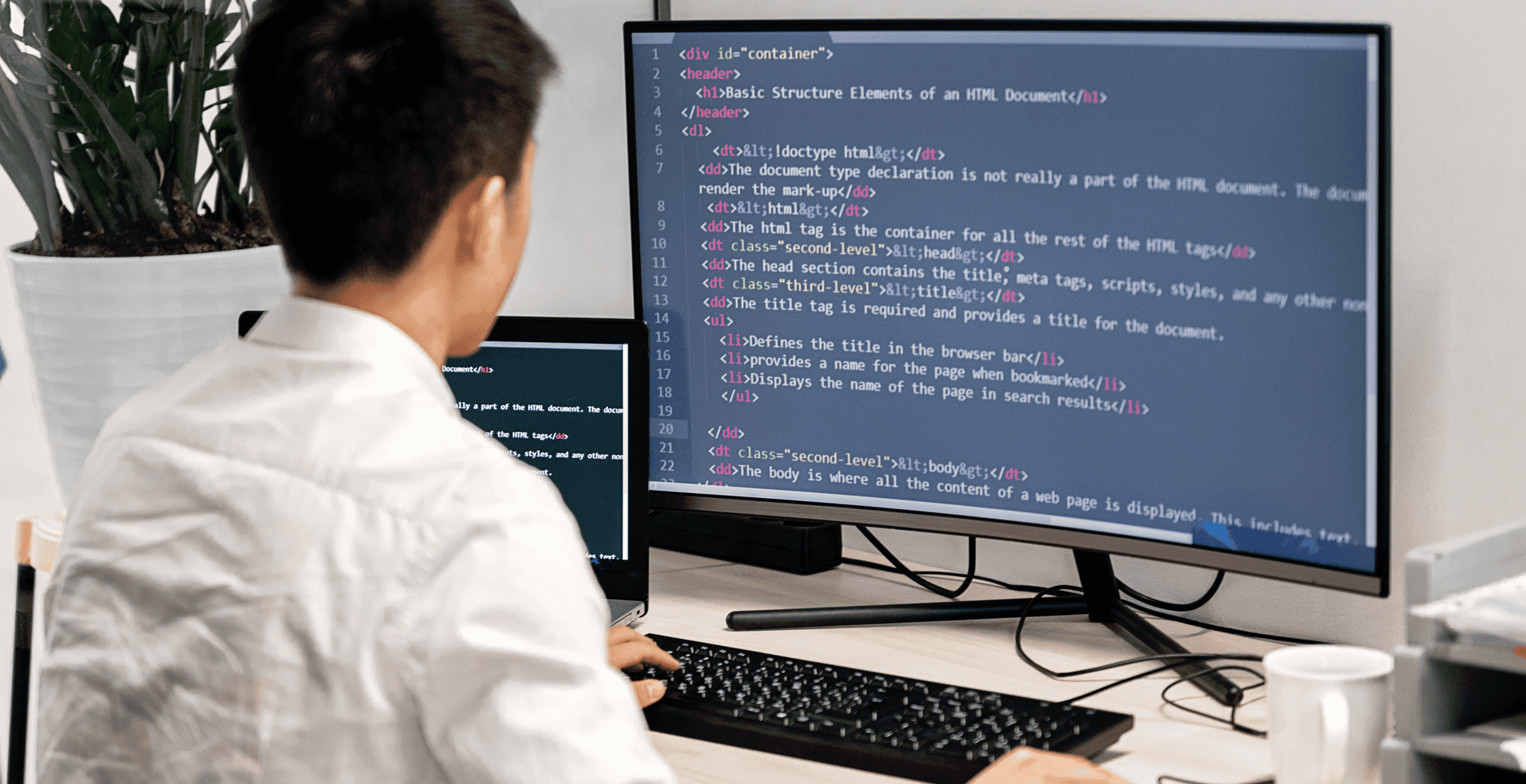
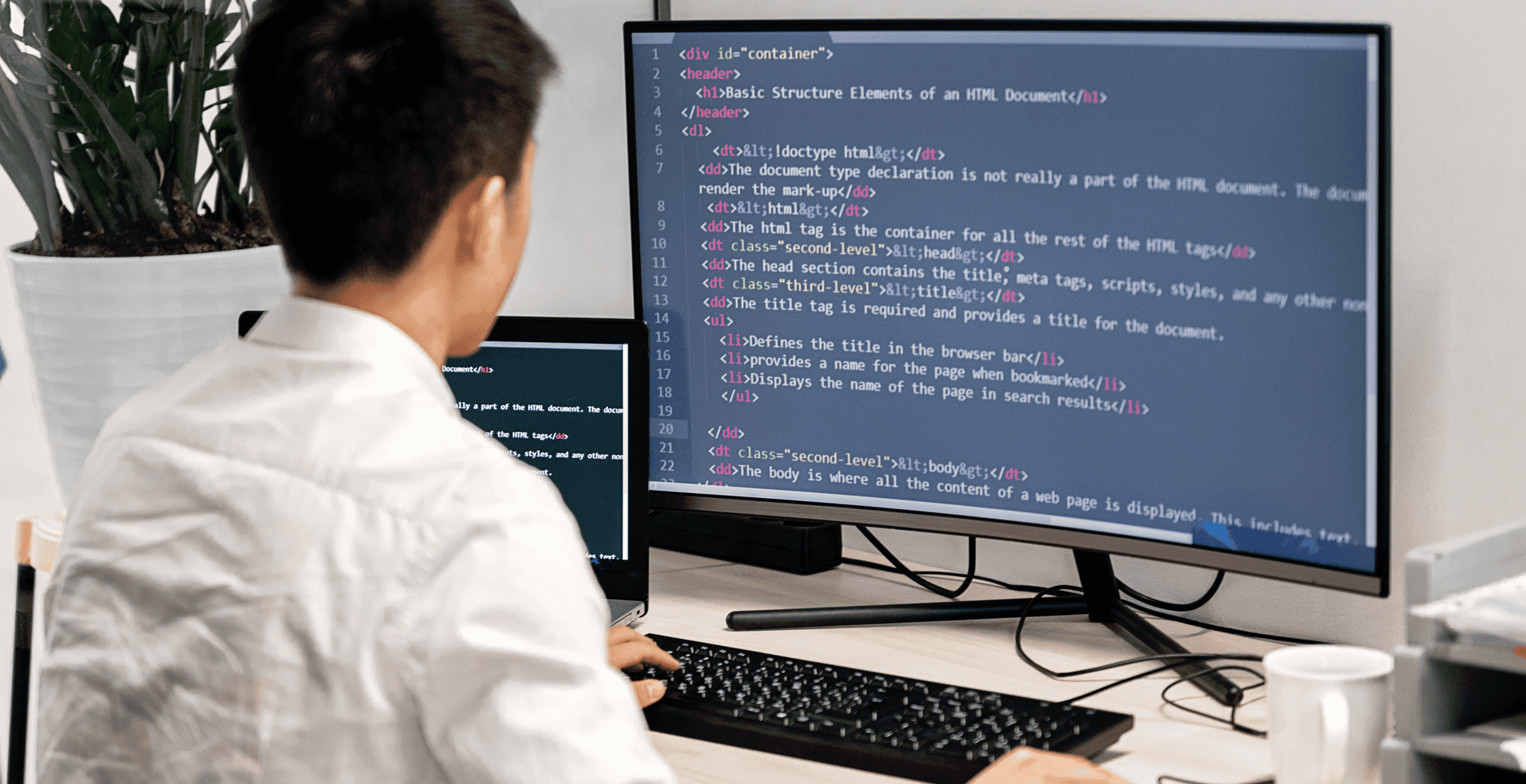
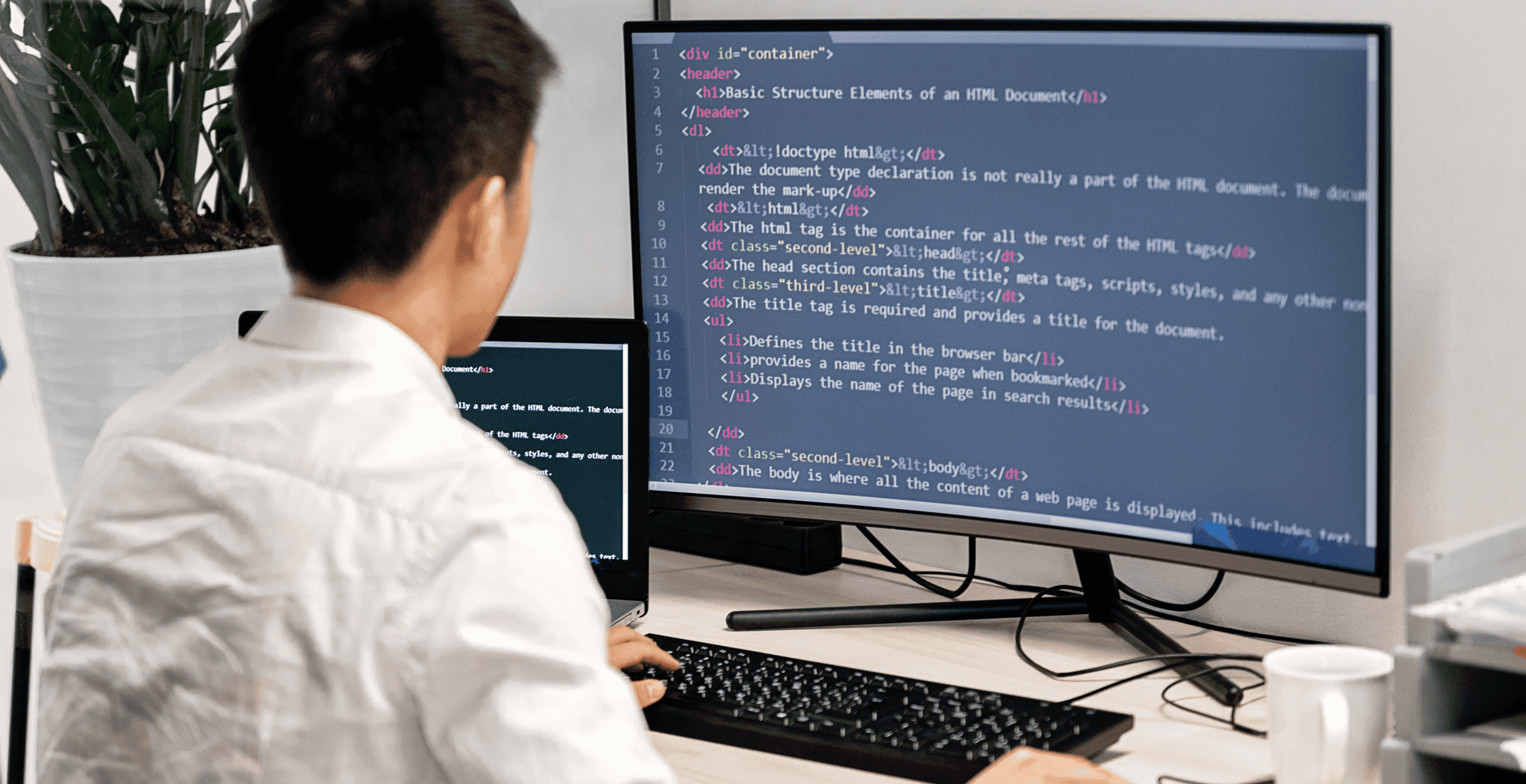
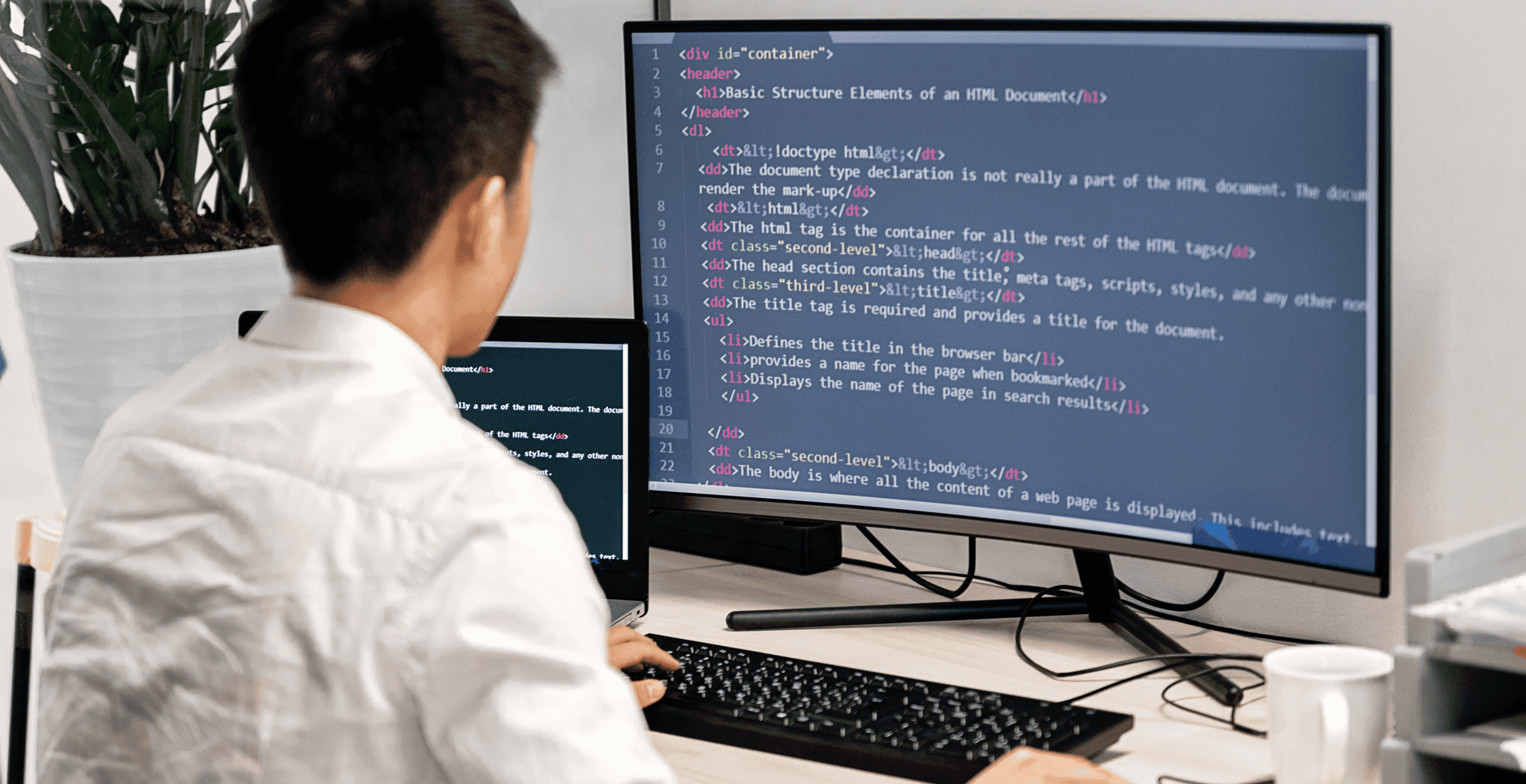
Understanding Techniques and Applications for Grounding LLMs in Data

Rehan Asif
Oct 13, 2024
Read the article




Advantages Of Using LLMs For Rapid Application Development

Rehan Asif
Oct 10, 2024
Read the article
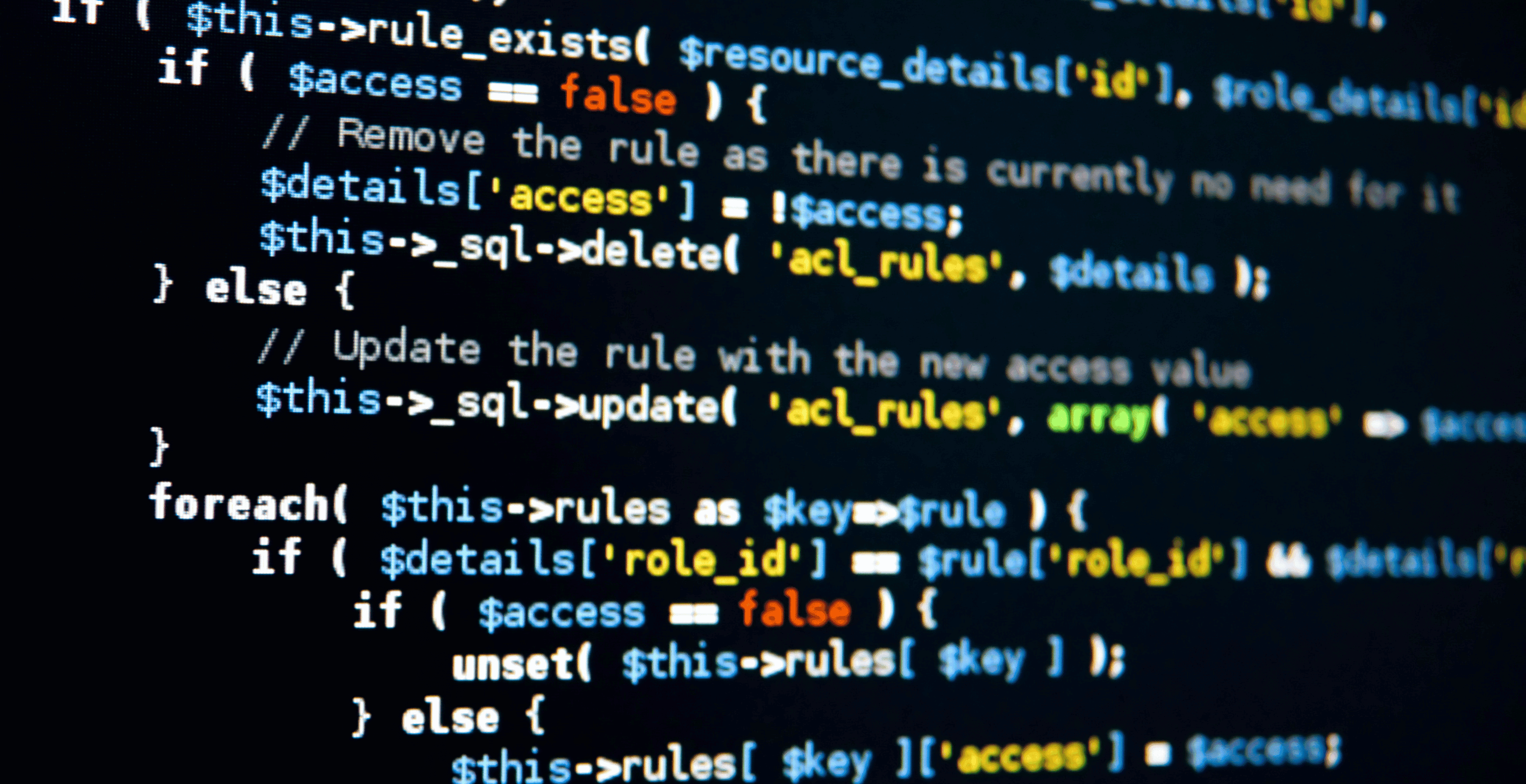
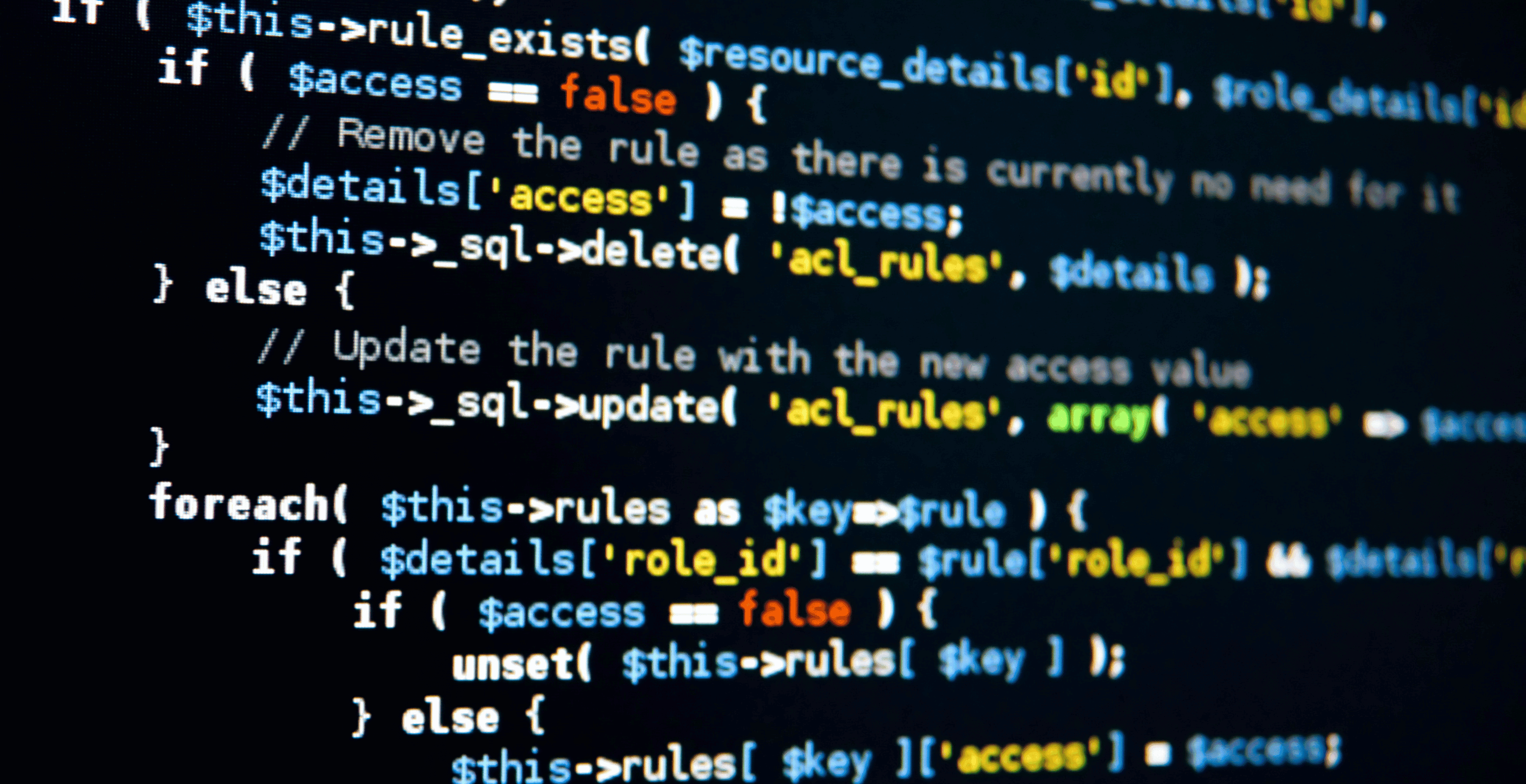
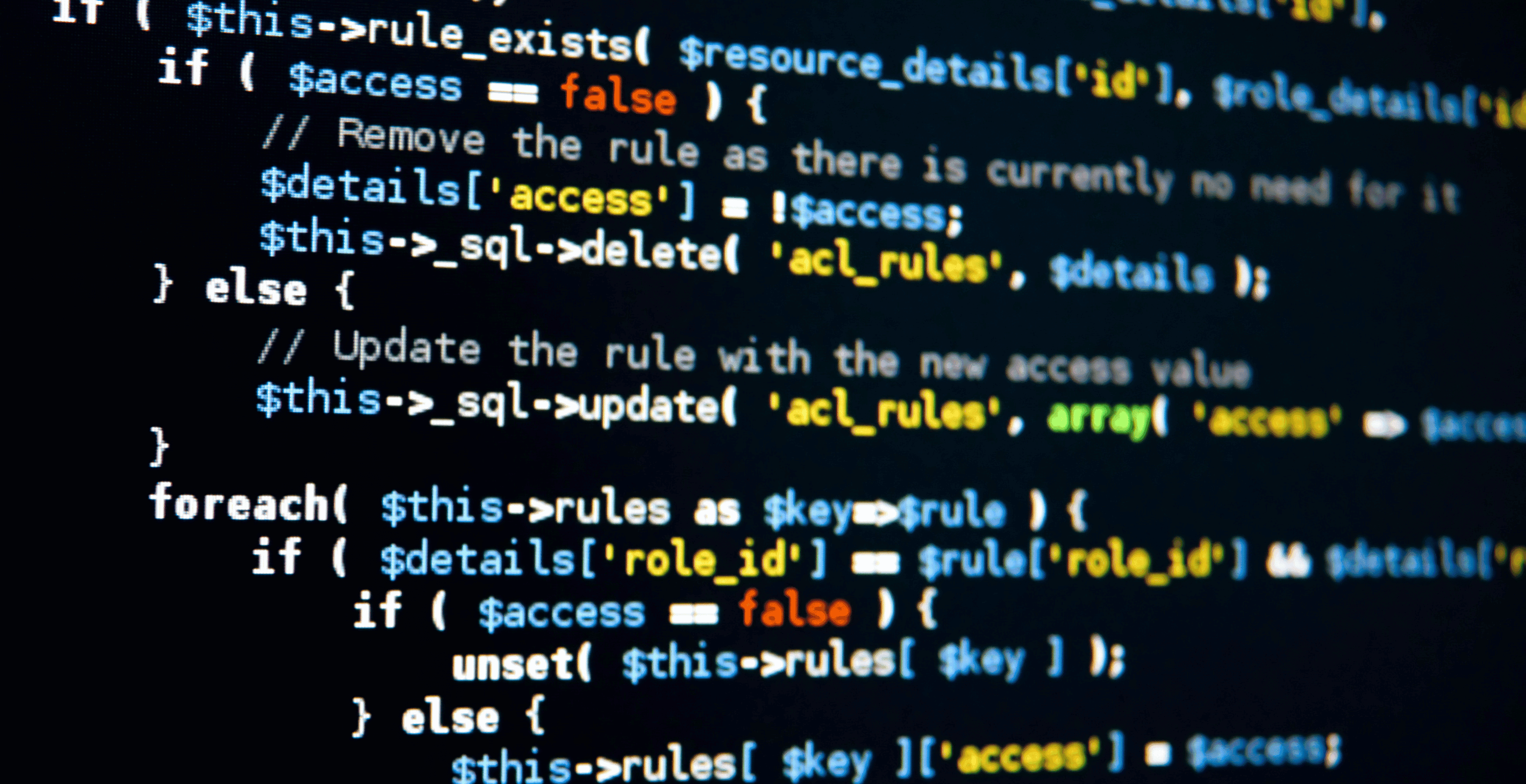
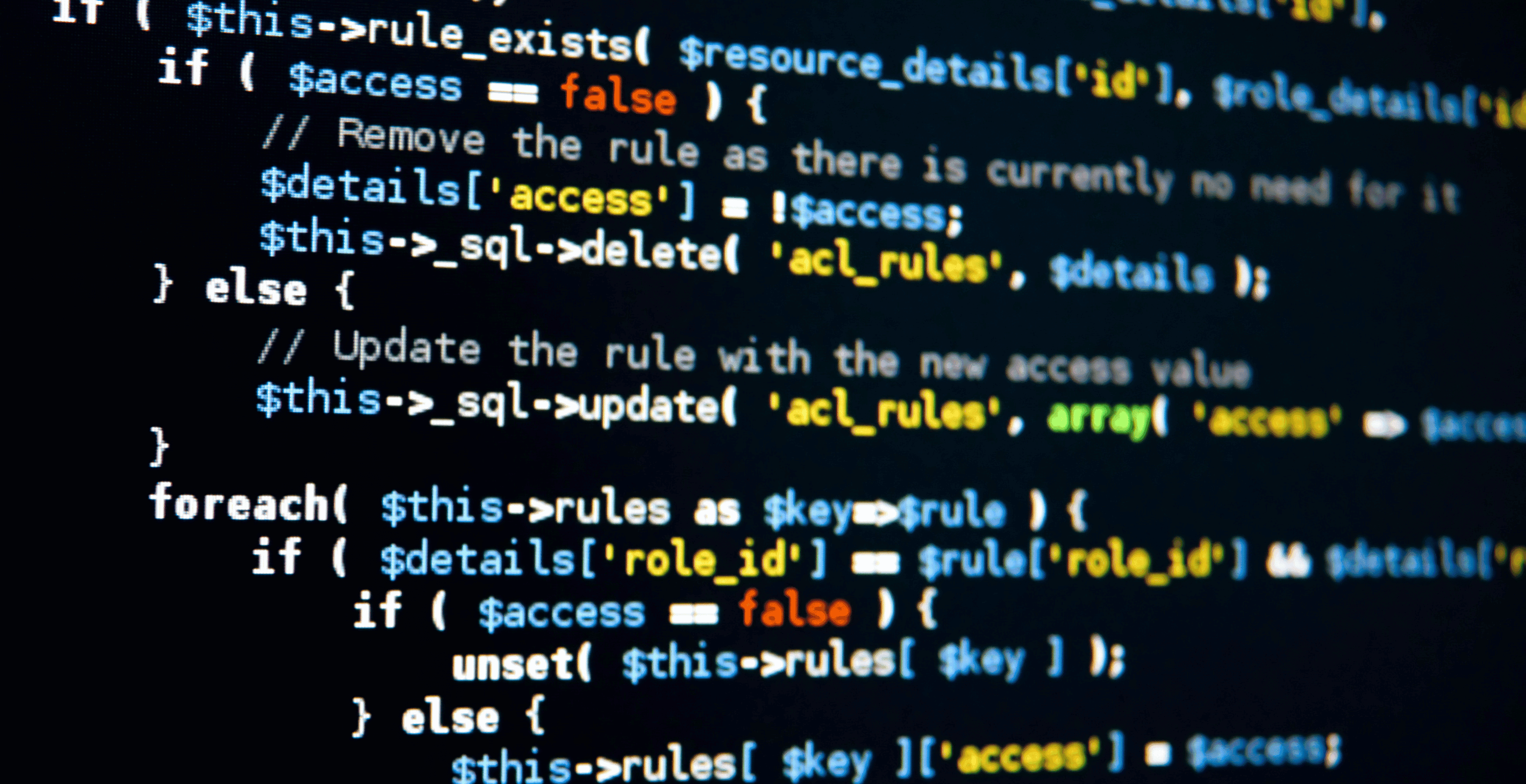
Understanding React Agent in LangChain Engineering

Rehan Asif
Oct 7, 2024
Read the article
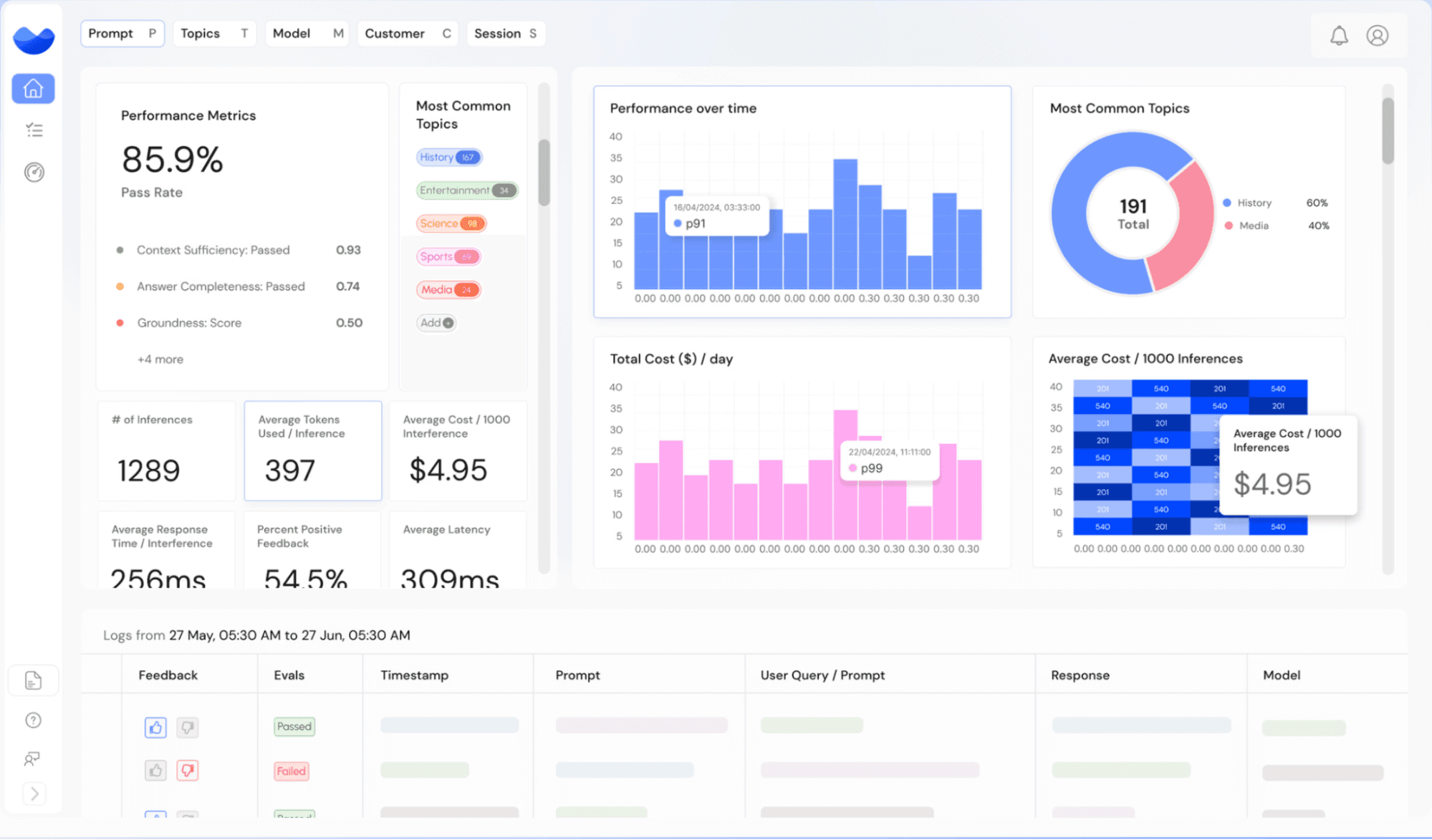
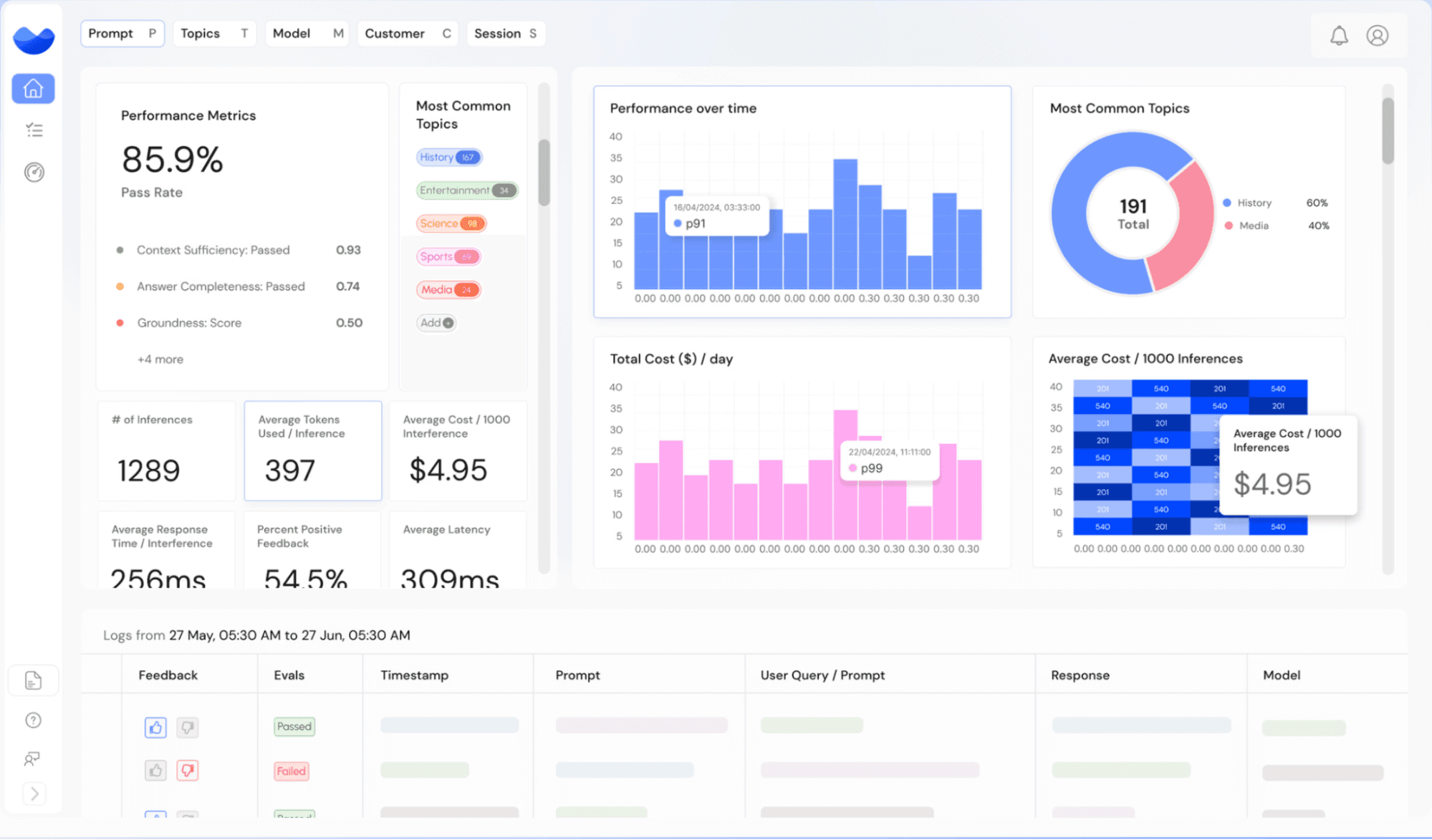
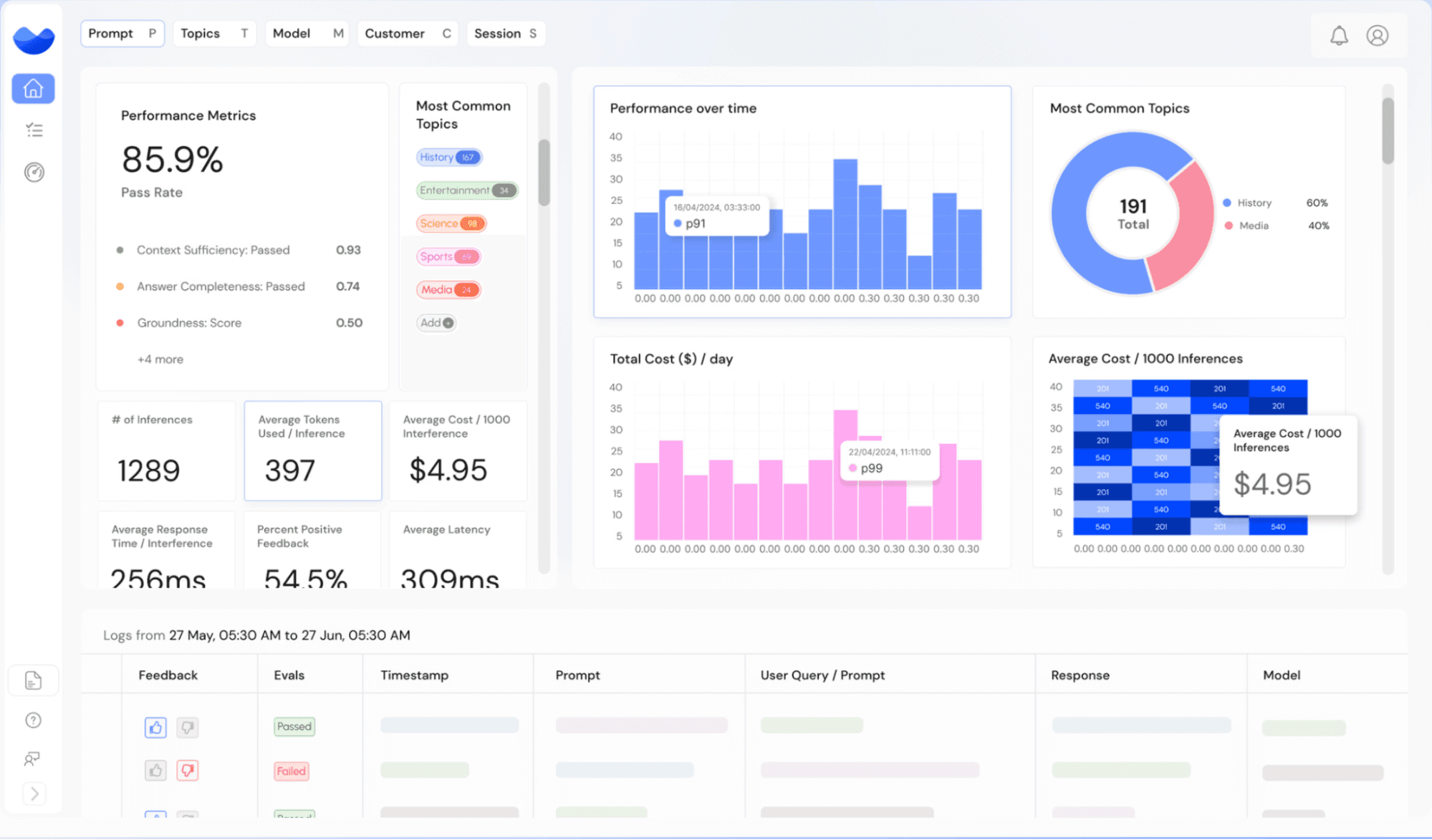
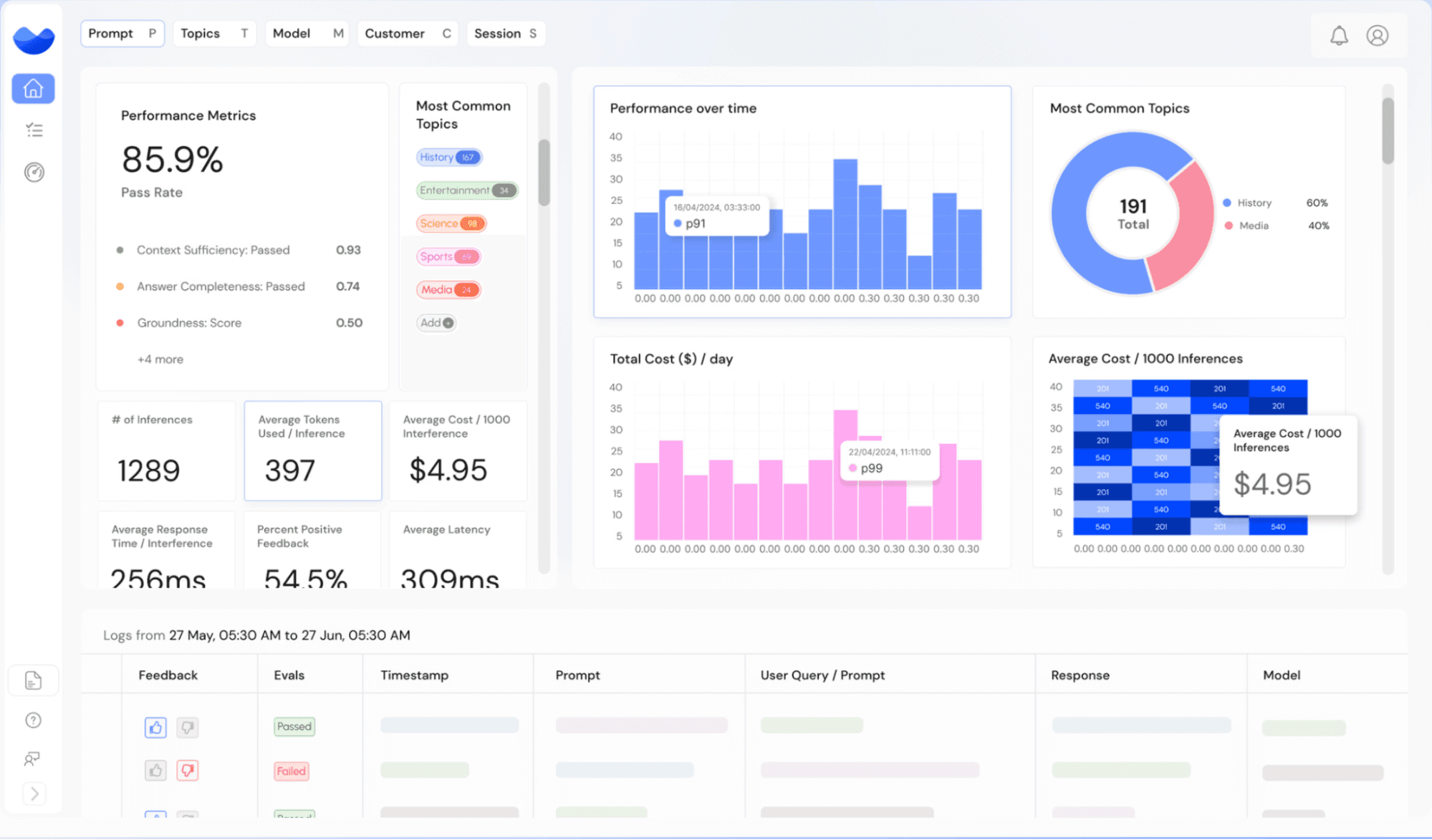
Using RagaAI Catalyst to Evaluate LLM Applications

Gaurav Agarwal
Oct 4, 2024
Read the article




Step-by-Step Guide on Training Large Language Models

Rehan Asif
Oct 1, 2024
Read the article
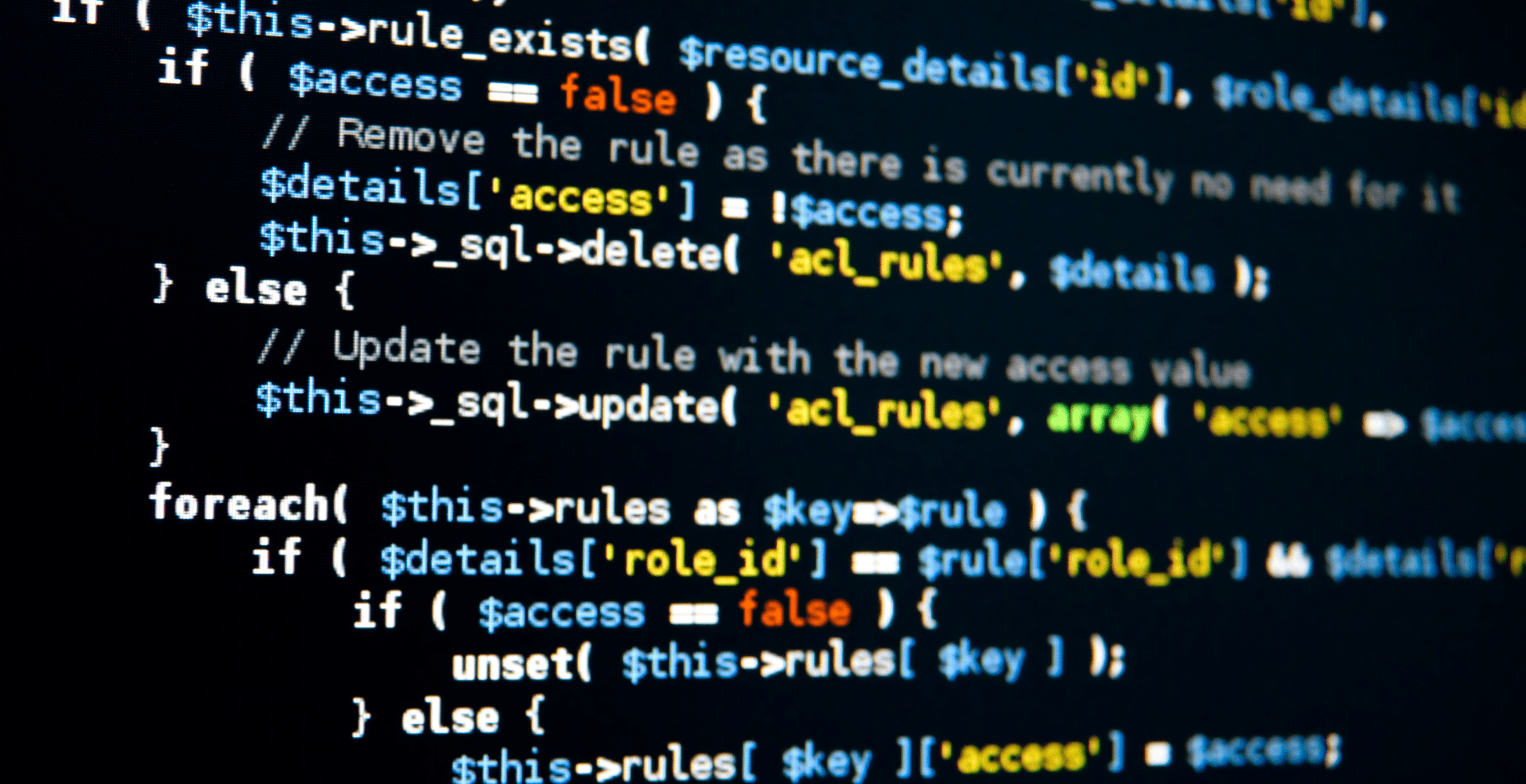
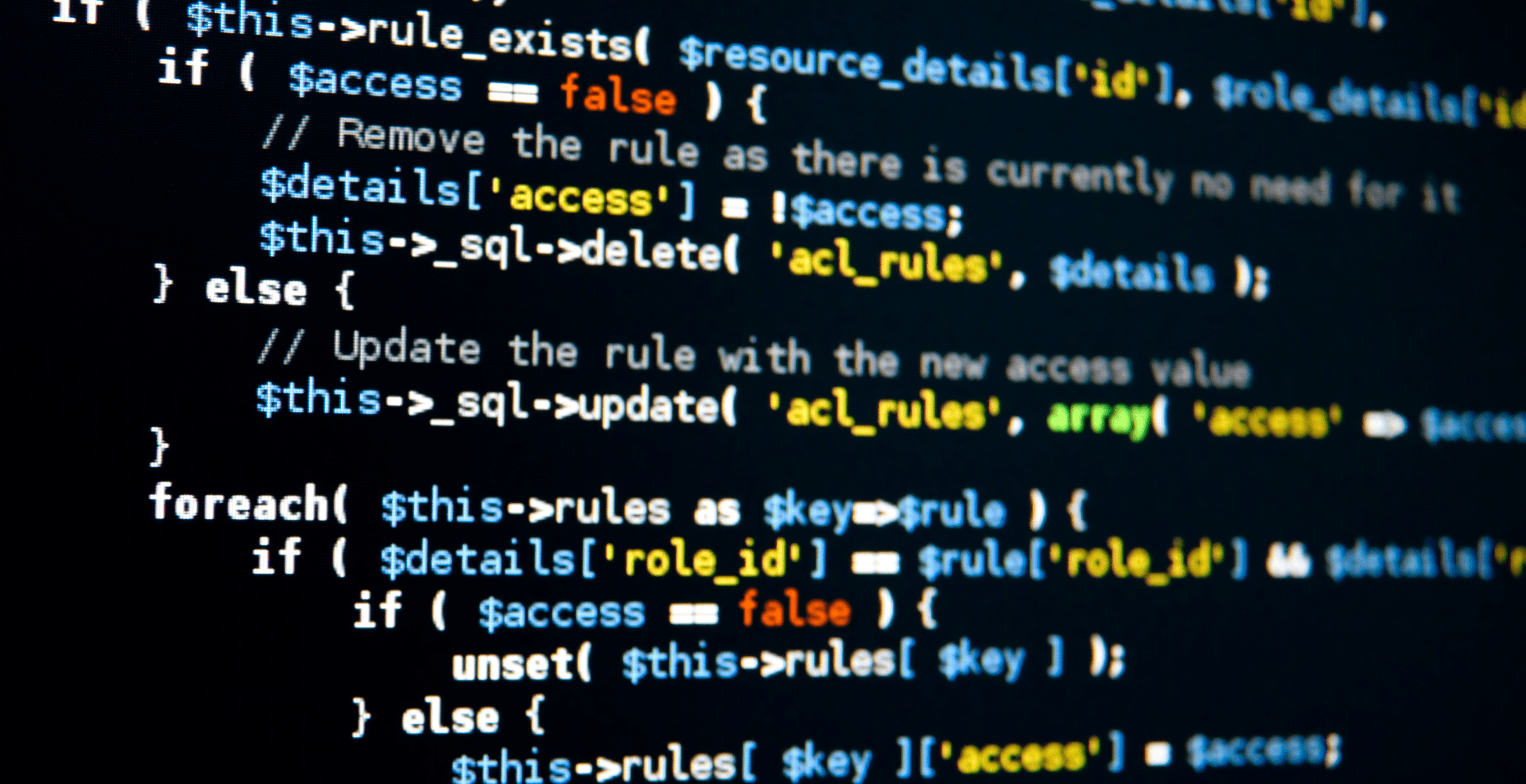
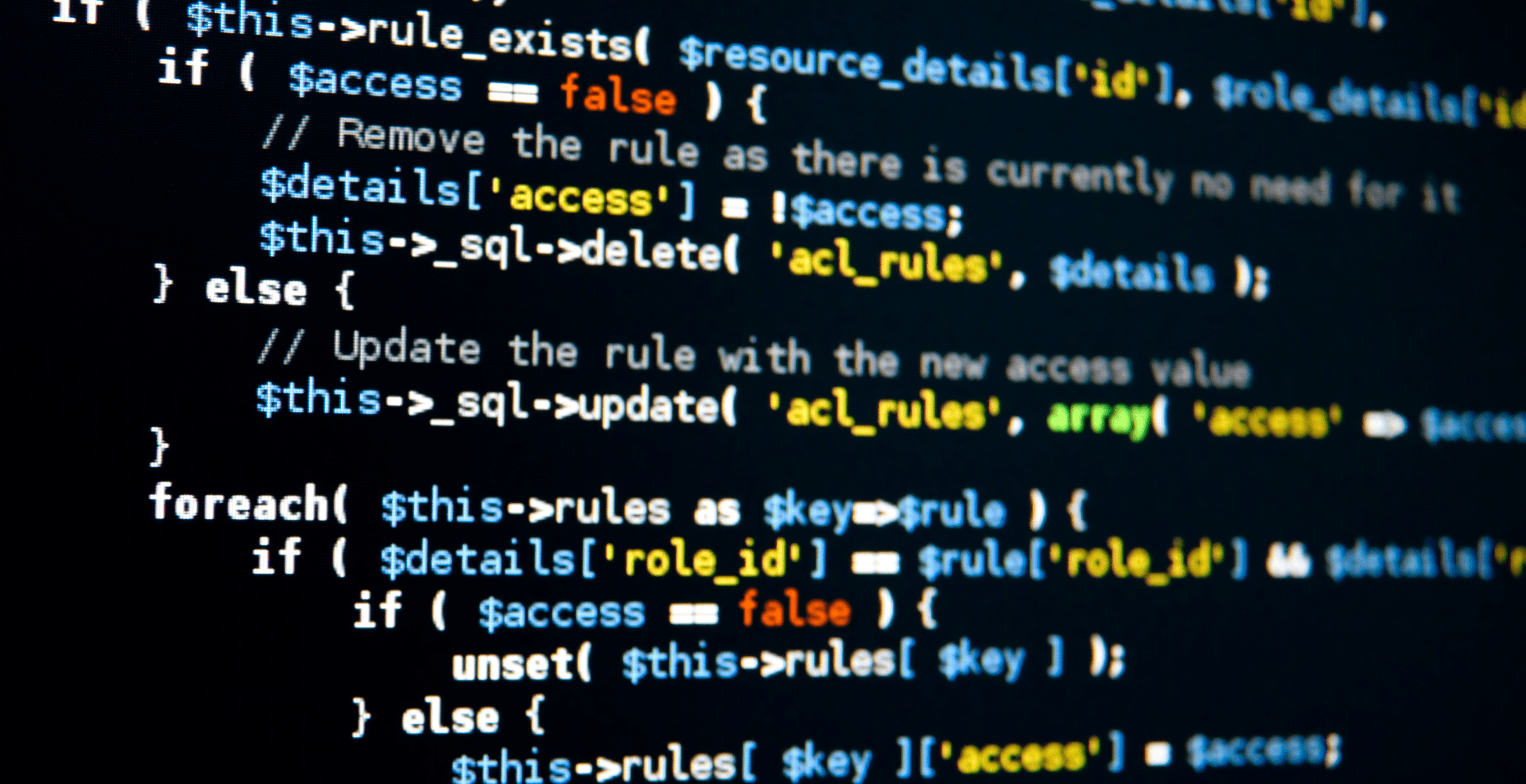
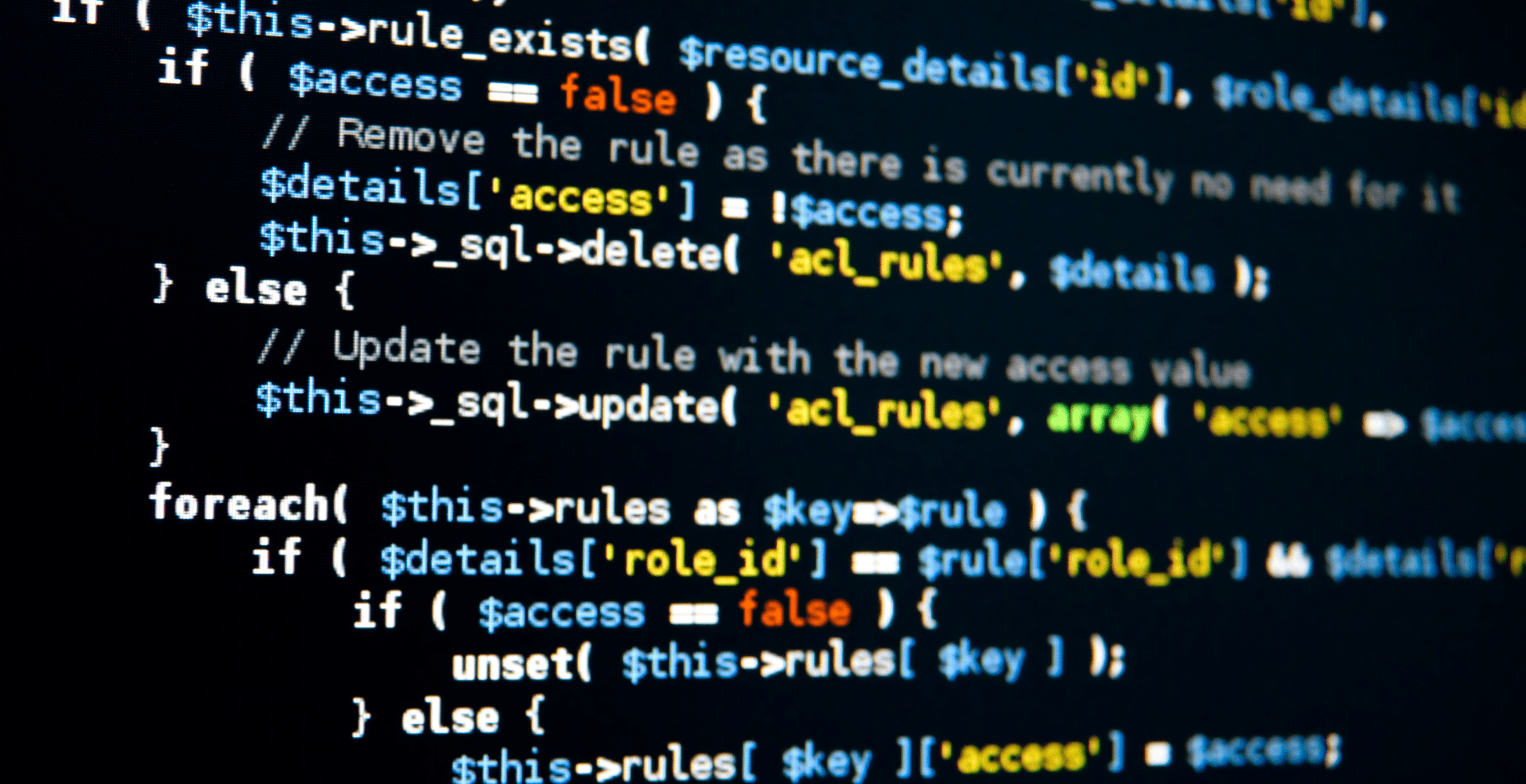
Understanding LLM Agent Architecture

Rehan Asif
Aug 19, 2024
Read the article




Understanding the Need and Possibilities of AI Guardrails Today
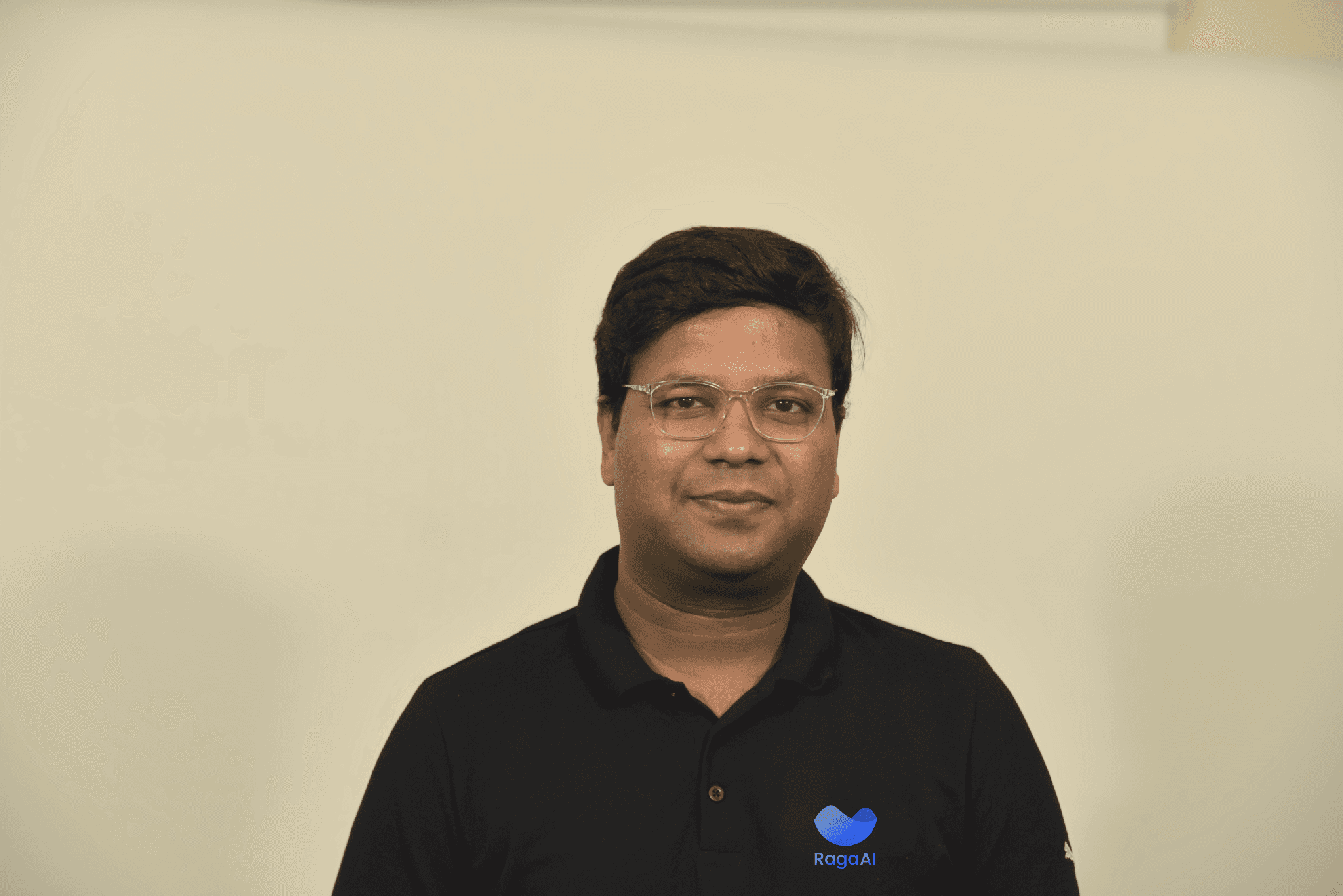
Jigar Gupta
Aug 19, 2024
Read the article




How to Prepare Quality Dataset for LLM Training

Rehan Asif
Aug 14, 2024
Read the article




Understanding Multi-Agent LLM Framework and Its Performance Scaling

Rehan Asif
Aug 15, 2024
Read the article
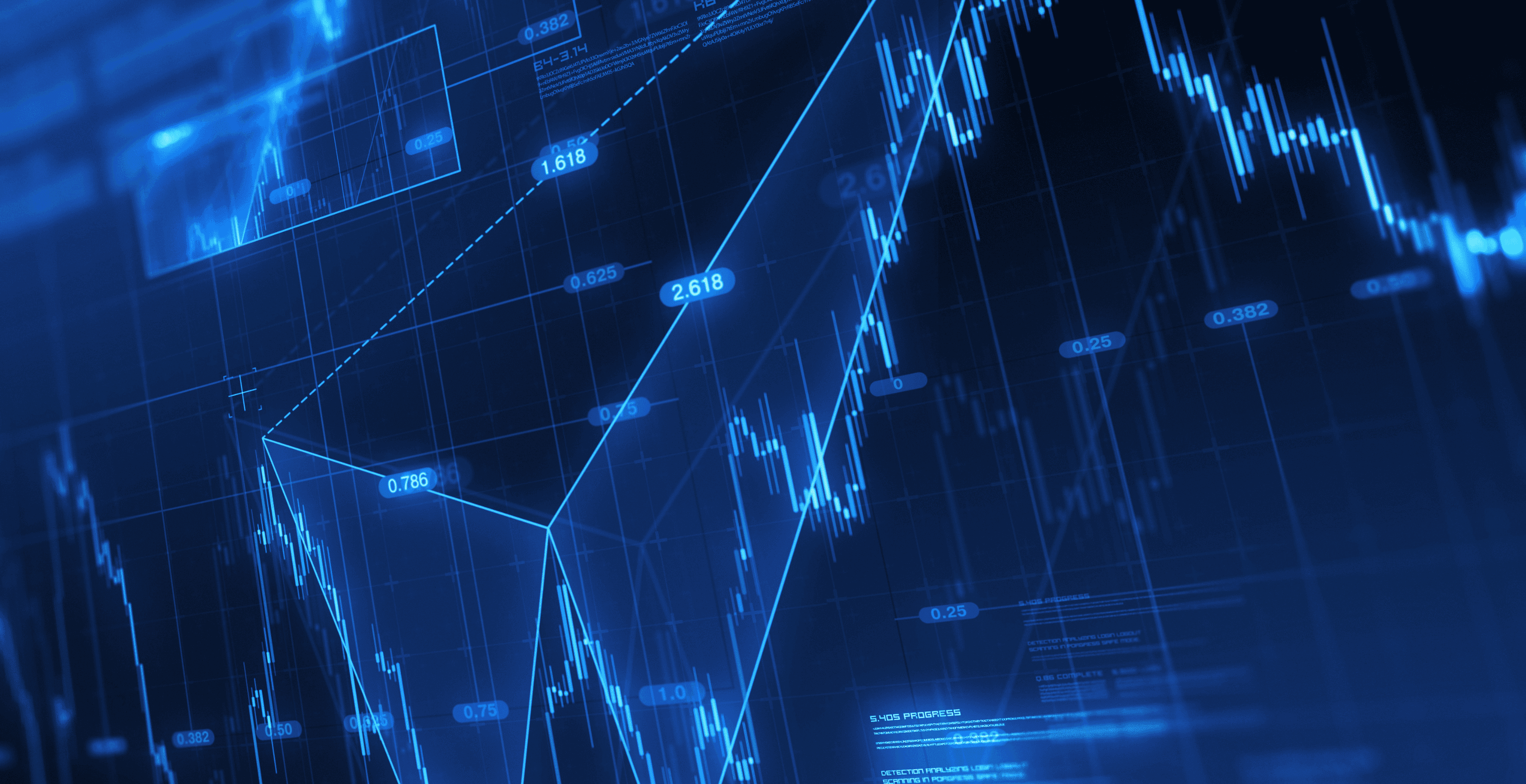
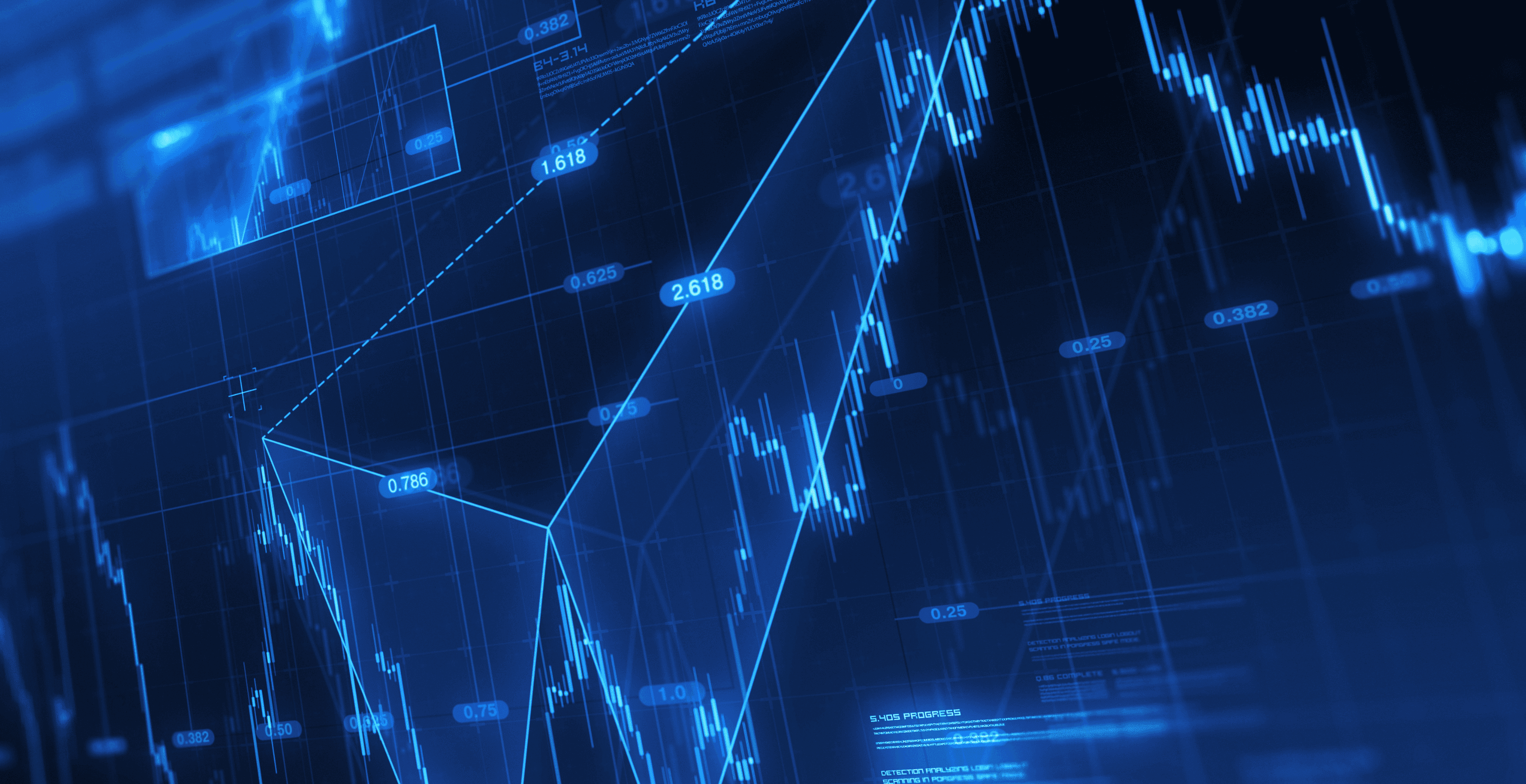
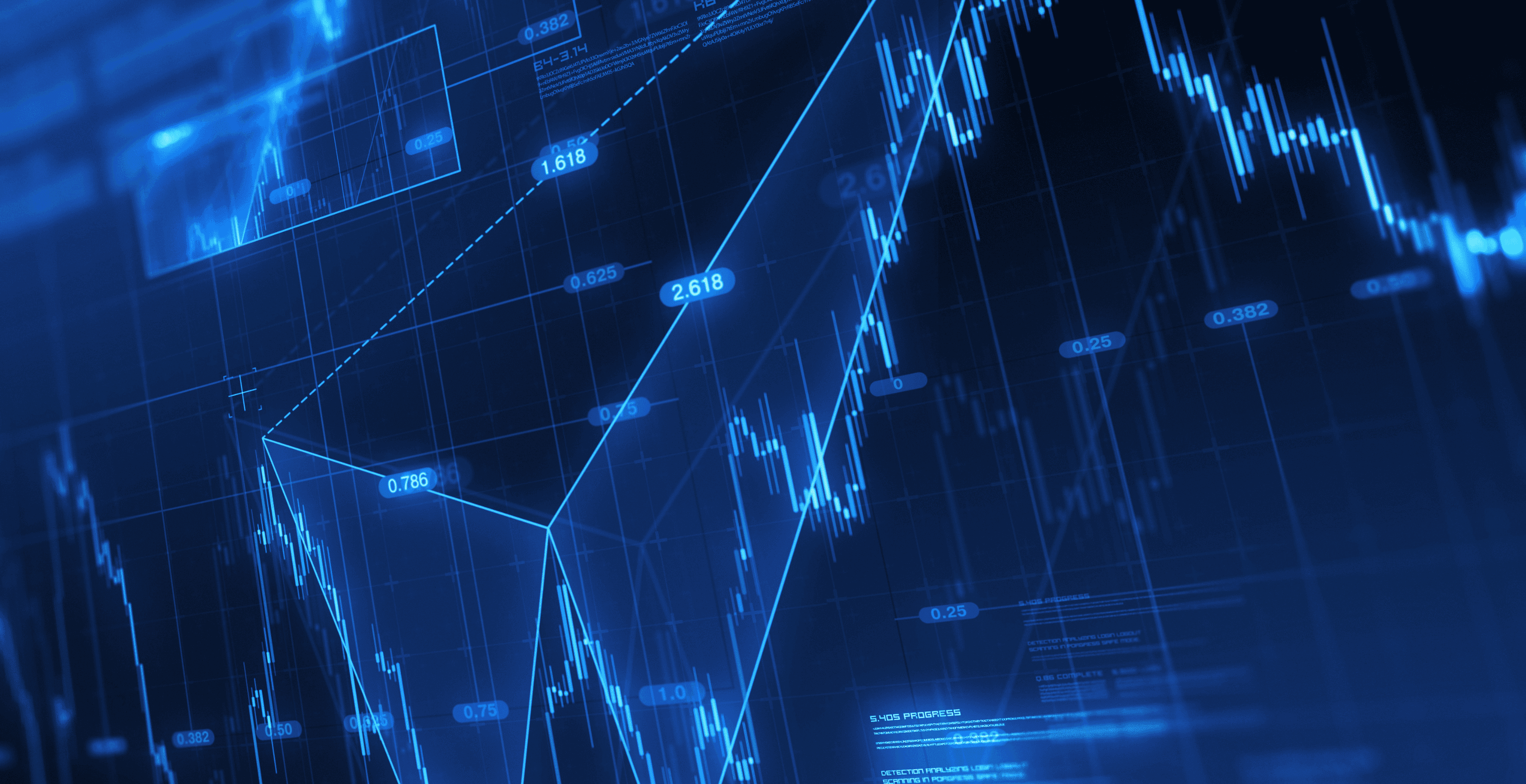
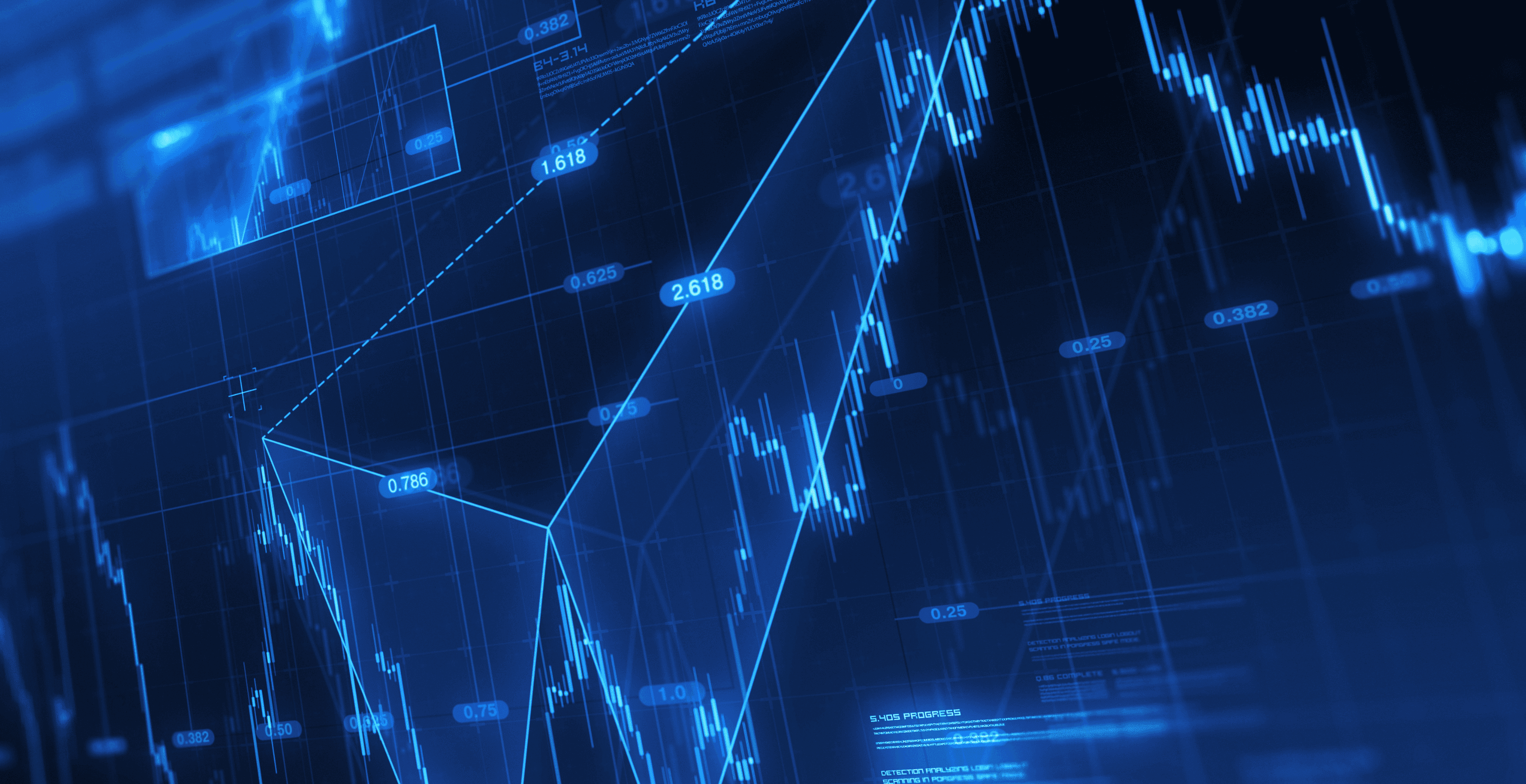
Understanding and Tackling Data Drift: Causes, Impact, and Automation Strategies
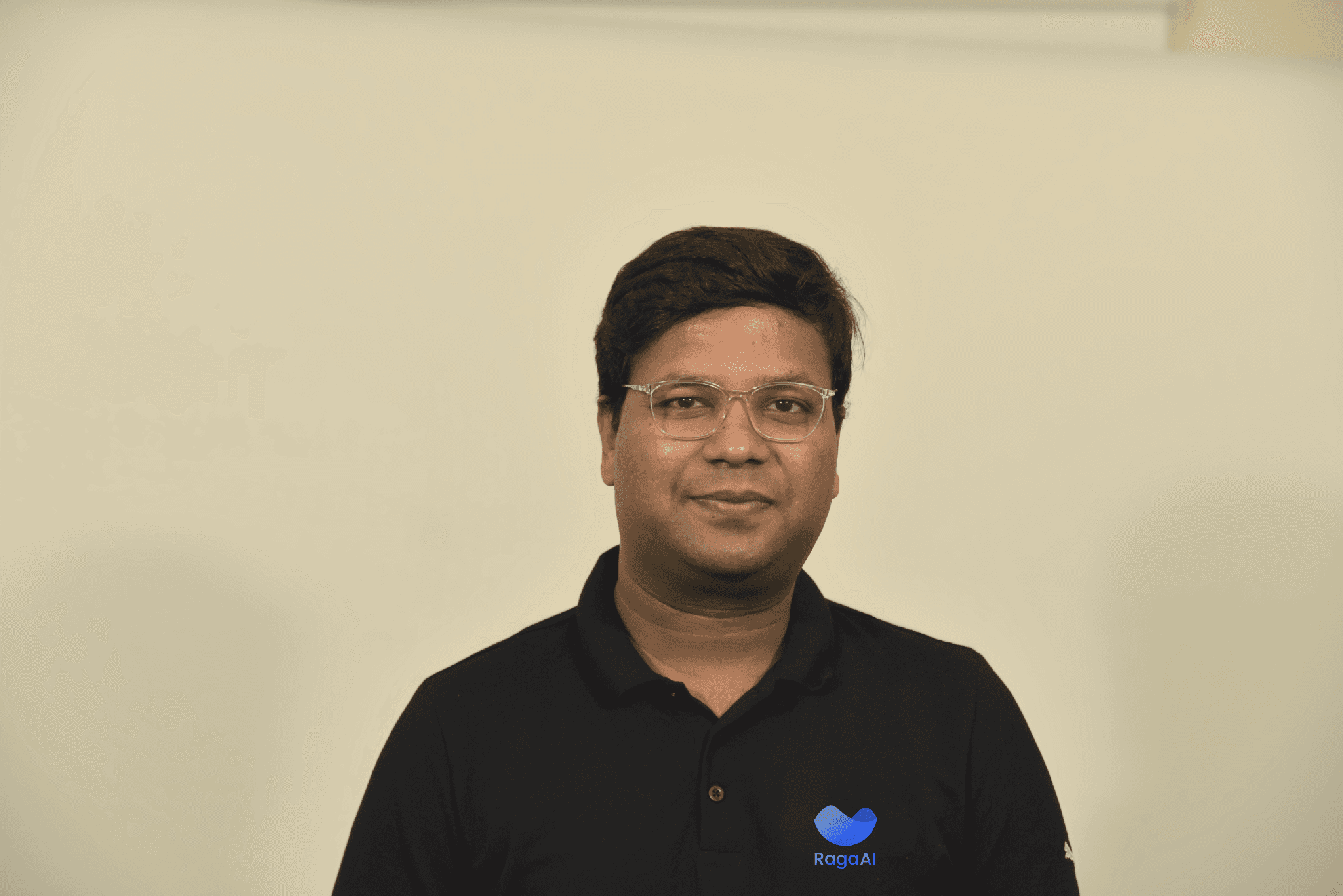
Jigar Gupta
Aug 14, 2024
Read the article




Introducing RagaAI Catalyst: Best in class automated LLM evaluation with 93% Human Alignment
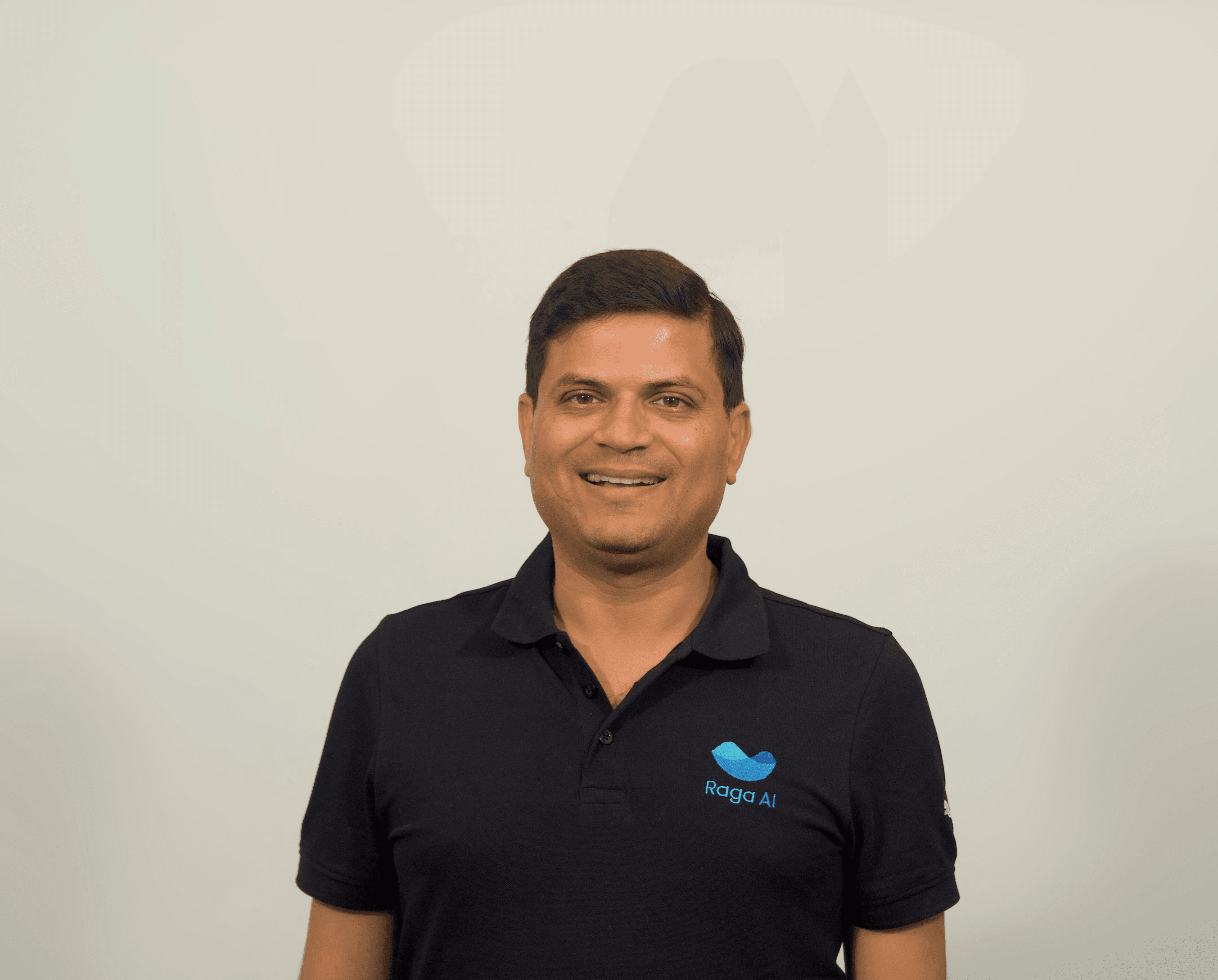
Gaurav Agarwal
Jul 15, 2024
Read the article




Key Pillars and Techniques for LLM Observability and Monitoring

Rehan Asif
Jul 24, 2024
Read the article
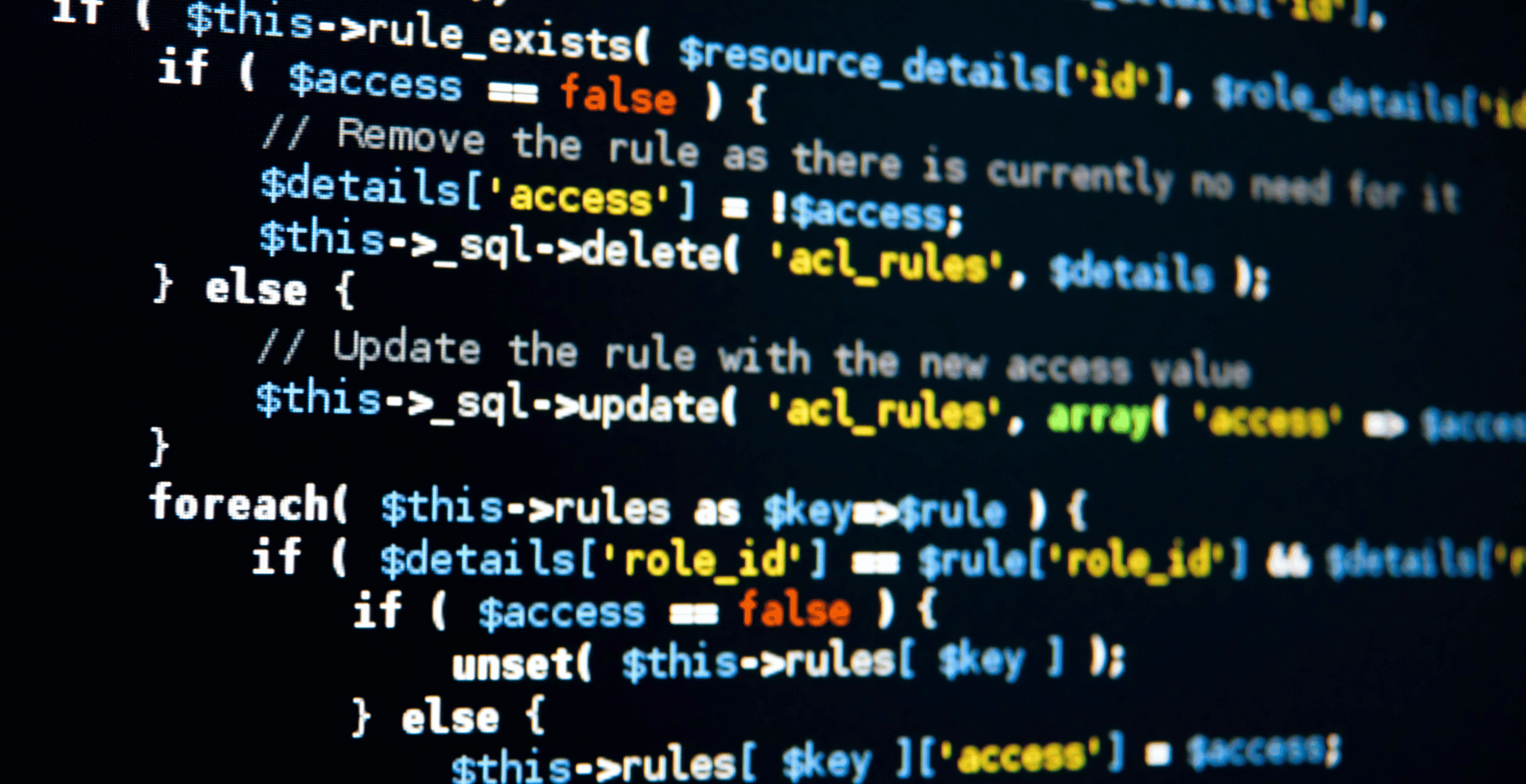
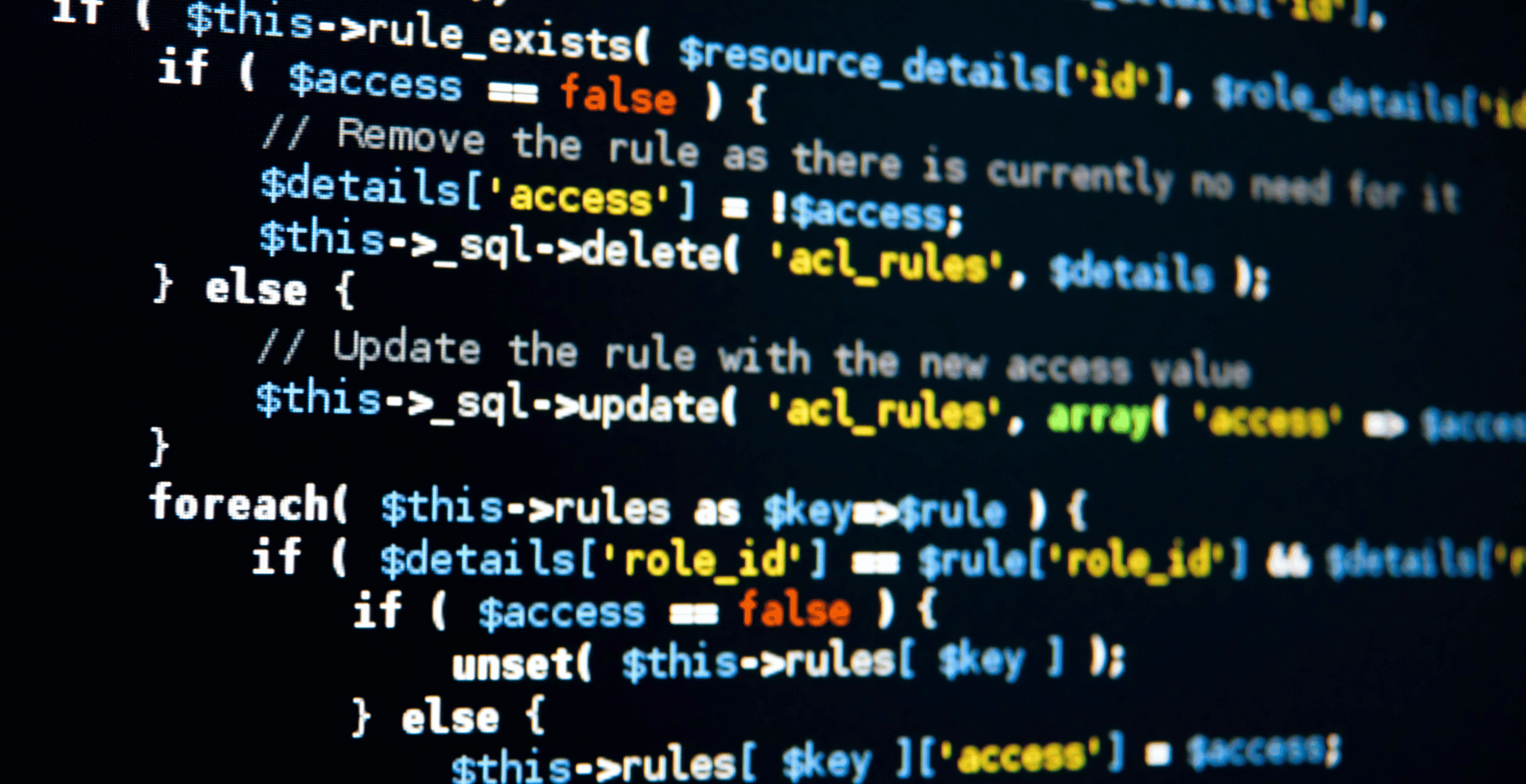
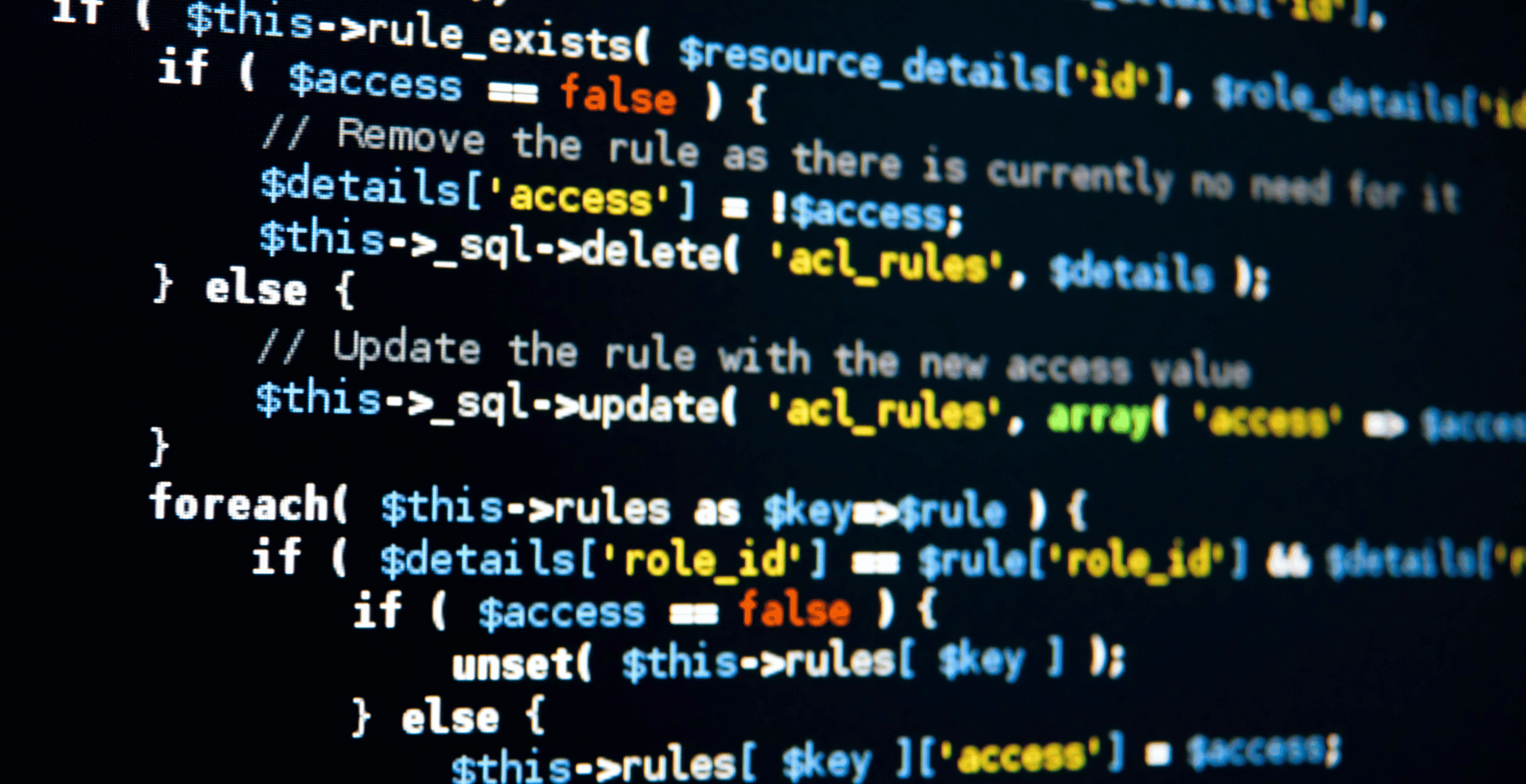
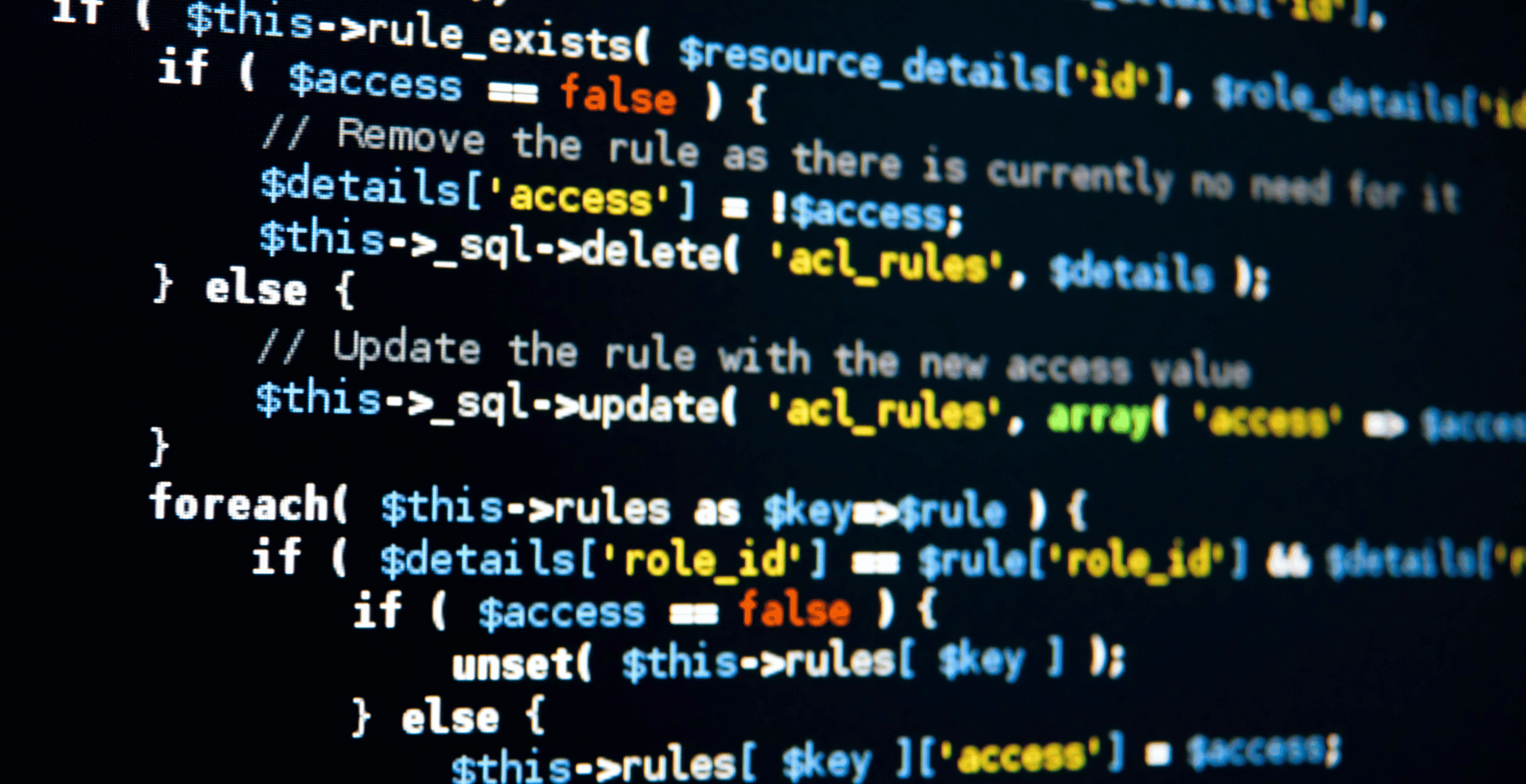
Introduction to What is LLM Agents and How They Work?

Rehan Asif
Jul 24, 2024
Read the article




Analysis of the Large Language Model Landscape Evolution

Rehan Asif
Jul 24, 2024
Read the article




Marketing Success With Retrieval Augmented Generation (RAG) Platforms
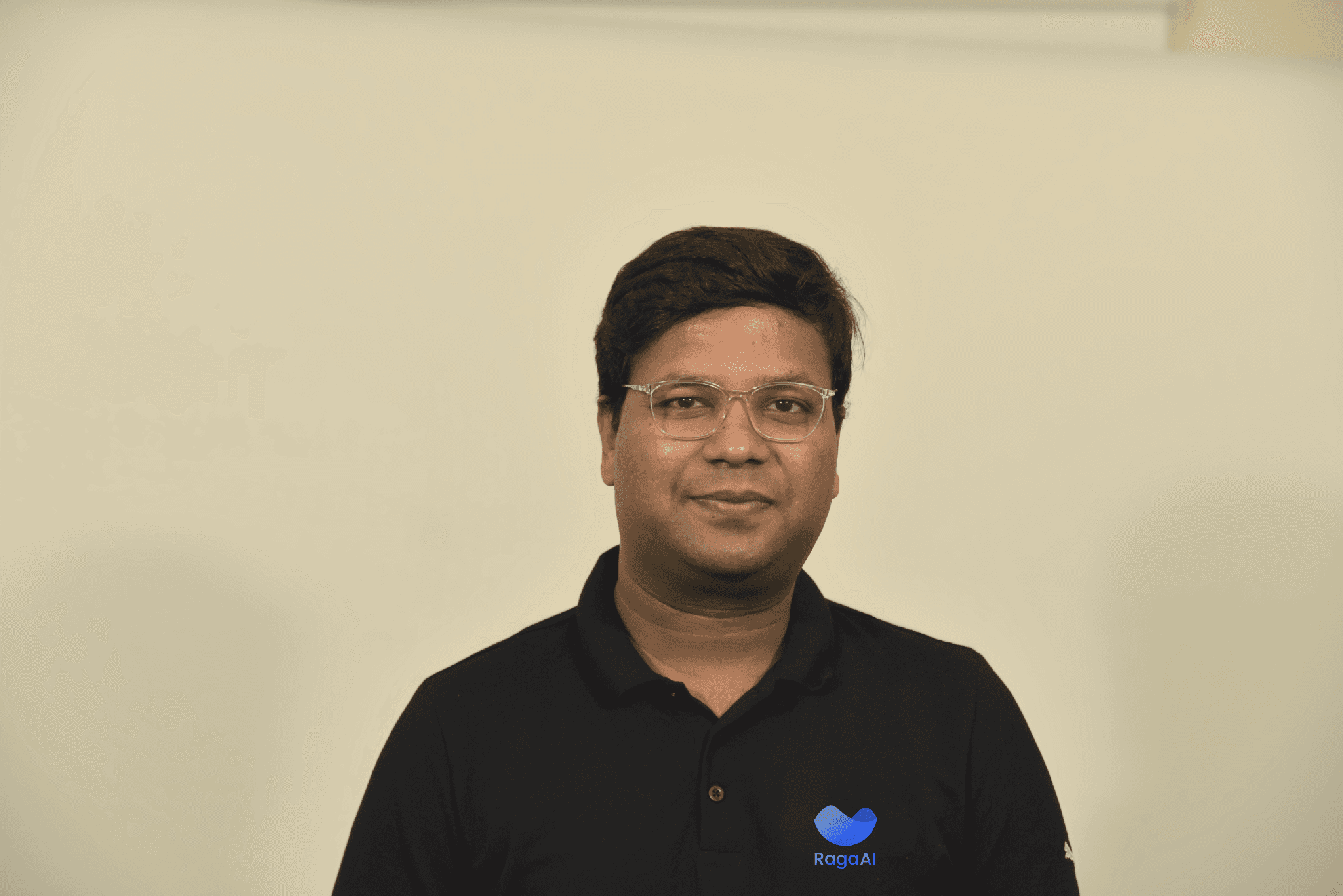
Jigar Gupta
Jul 24, 2024
Read the article




Developing AI Agent Strategies Using GPT
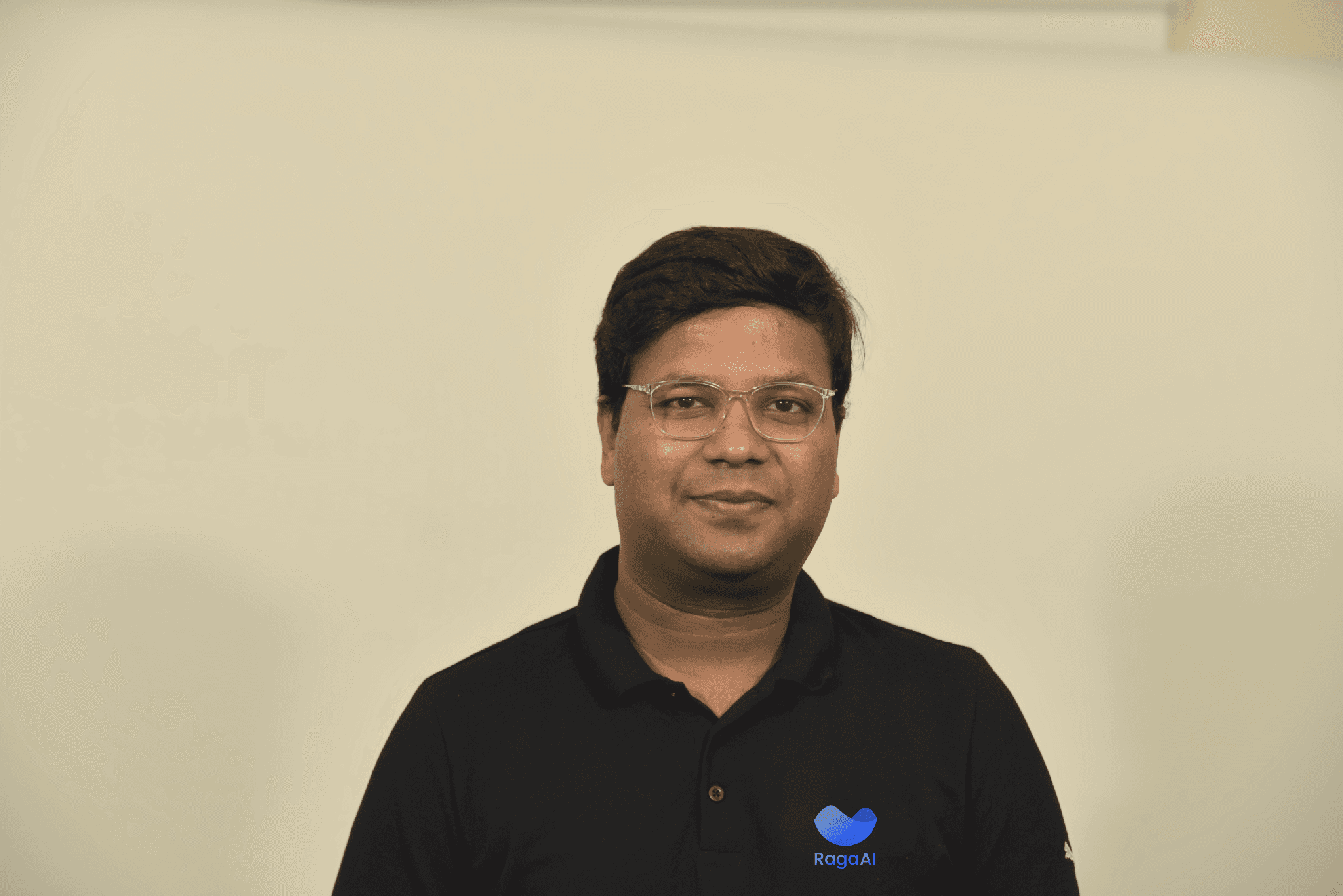
Jigar Gupta
Jul 24, 2024
Read the article




Identifying Triggers for Retraining AI Models to Maintain Performance
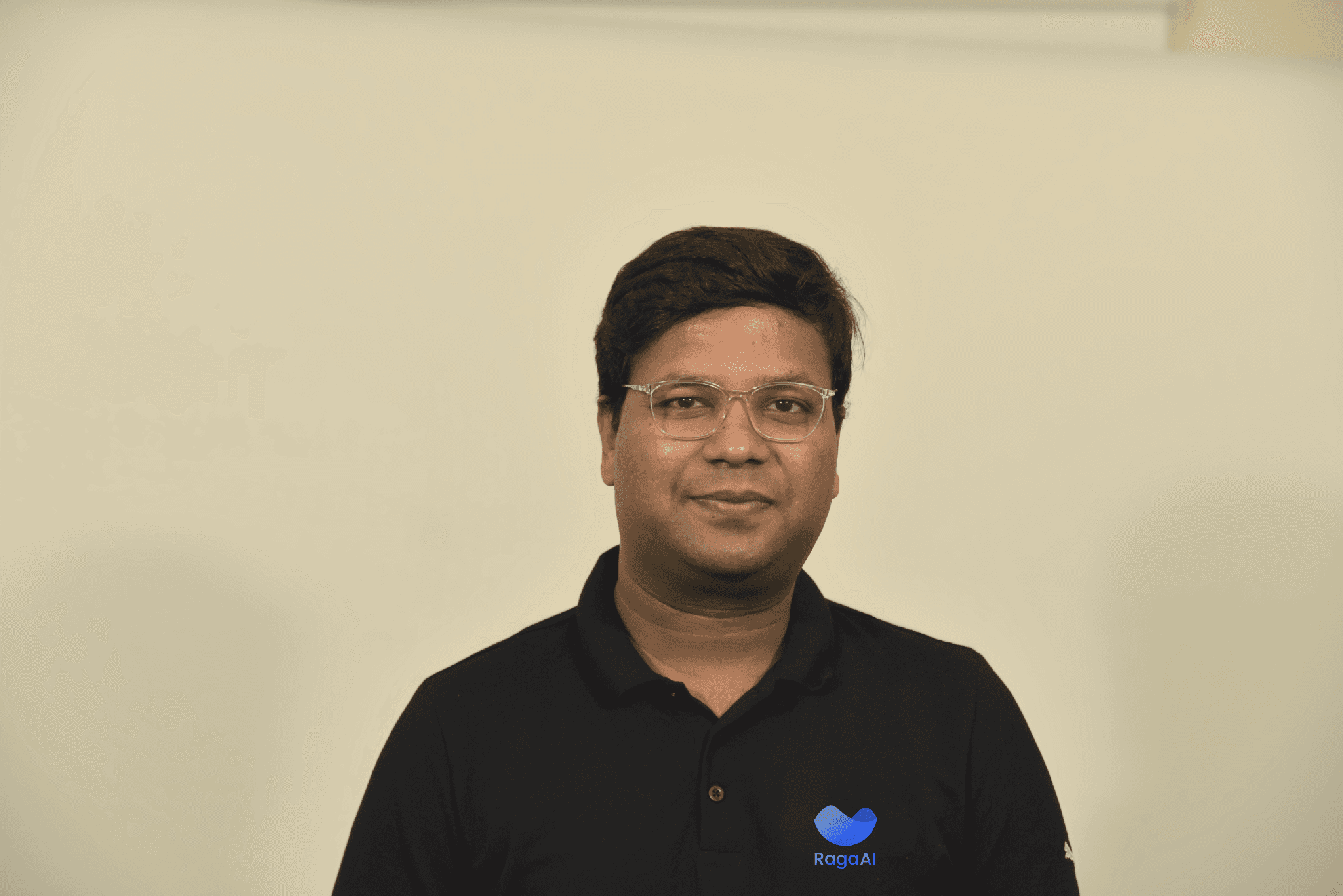
Jigar Gupta
Jul 16, 2024
Read the article
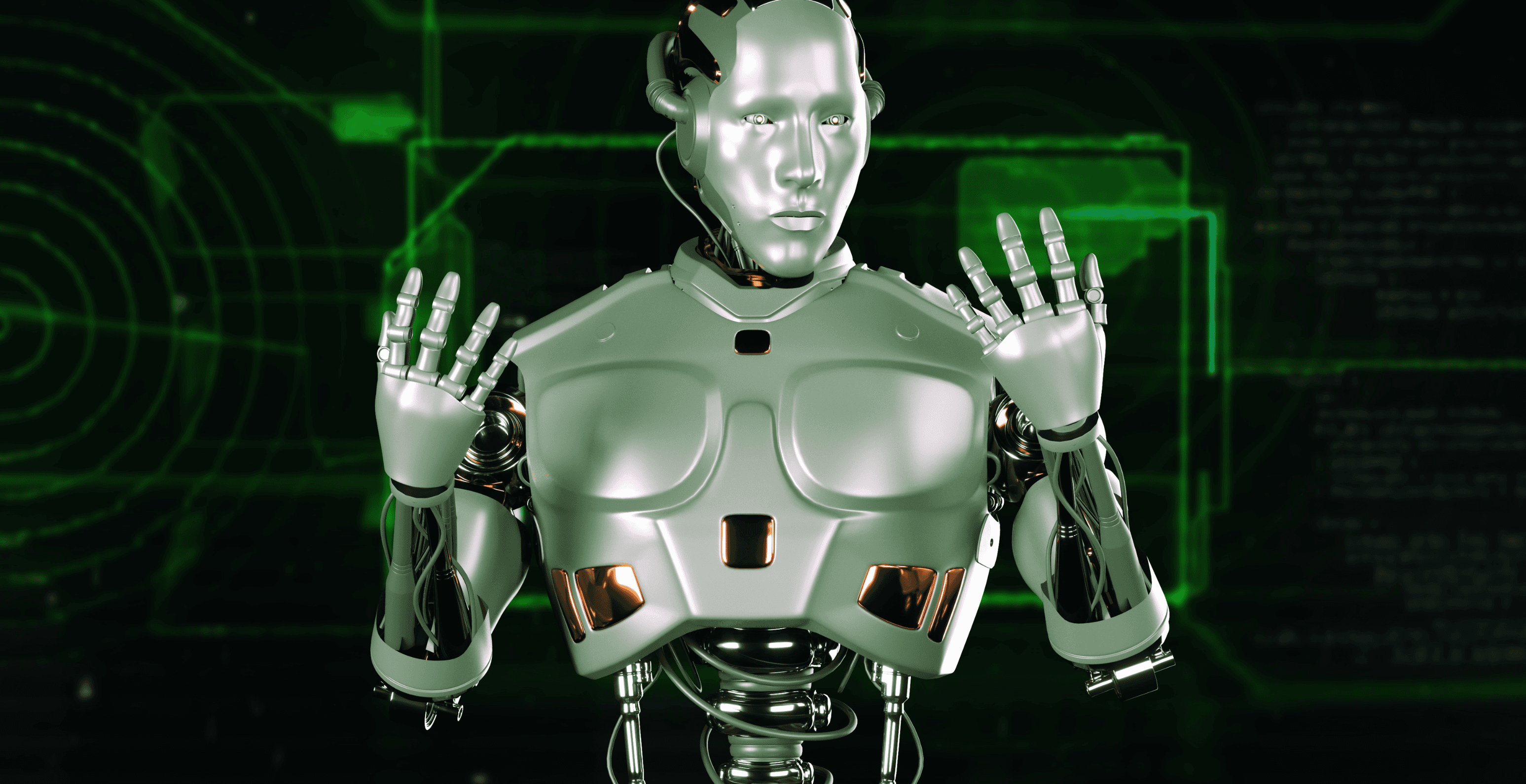
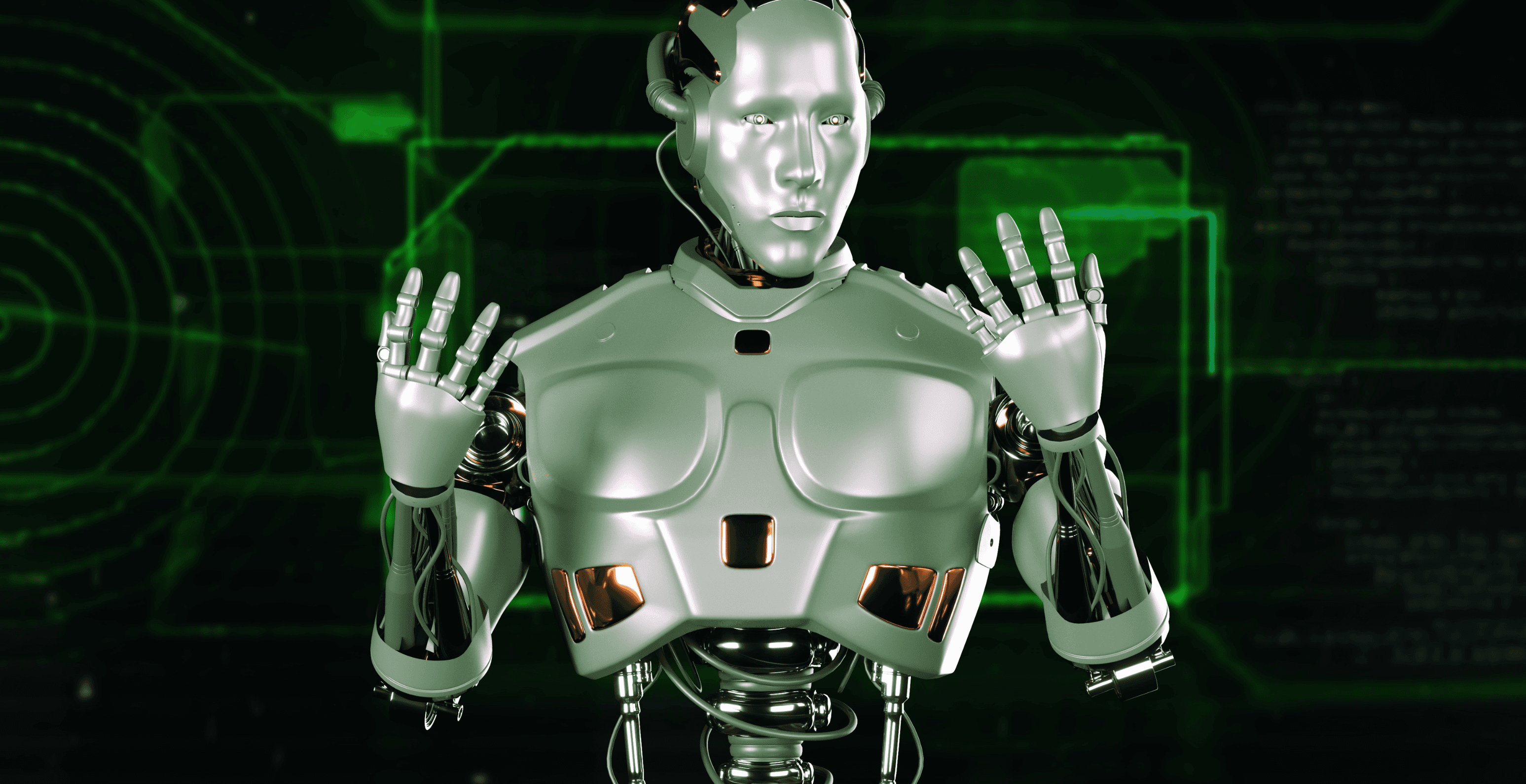
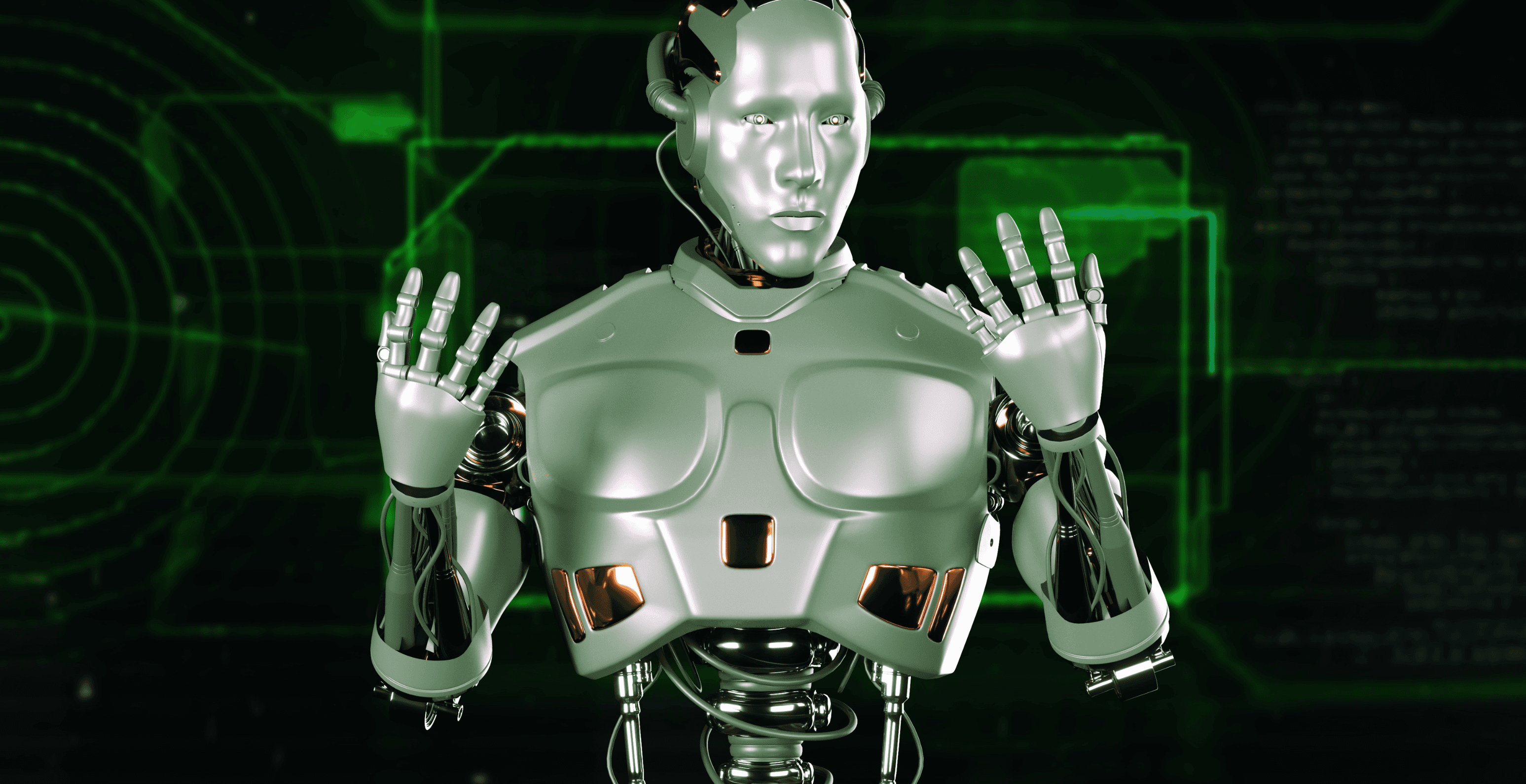
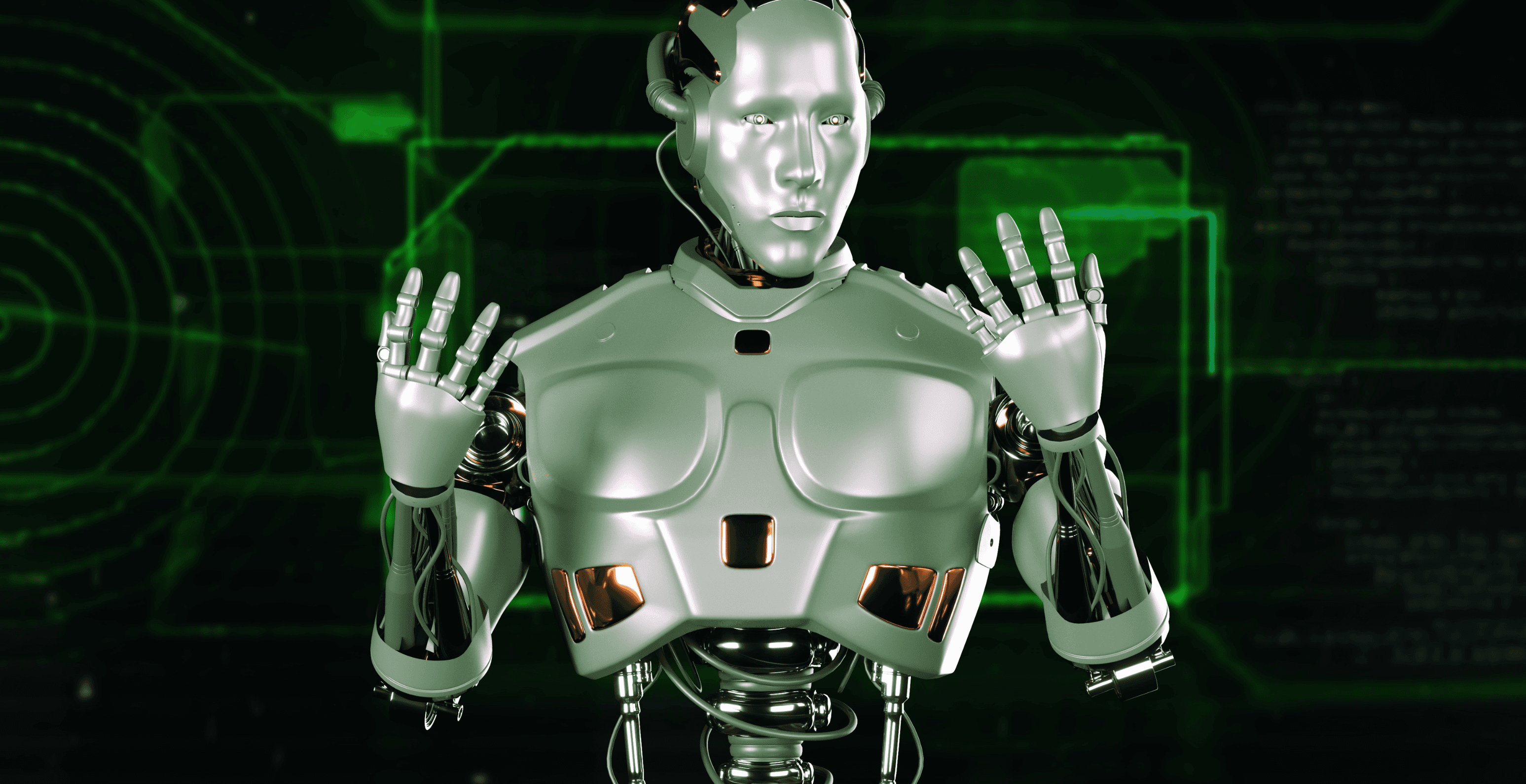
Agentic Design Patterns In LLM-Based Applications

Rehan Asif
Jul 16, 2024
Read the article
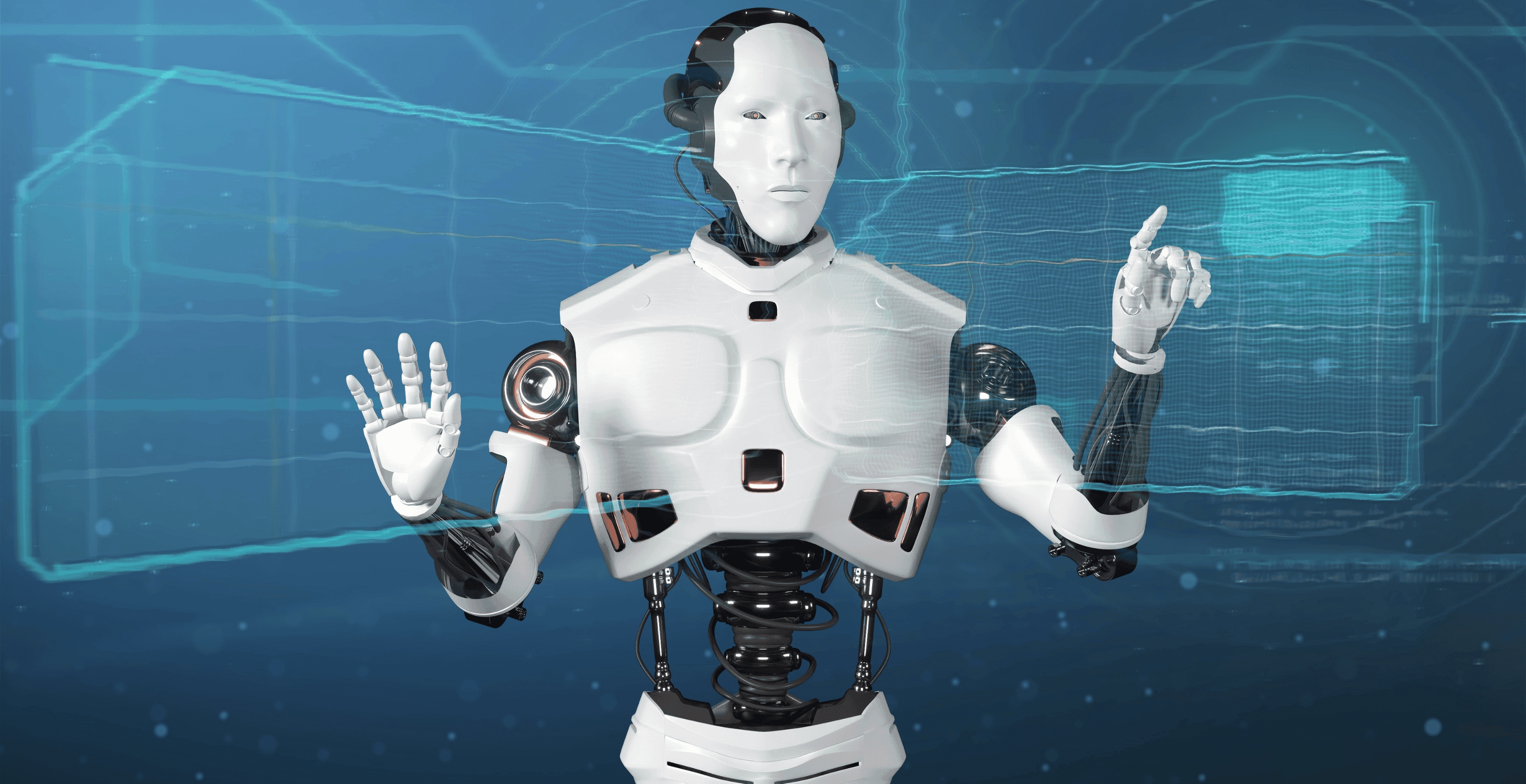
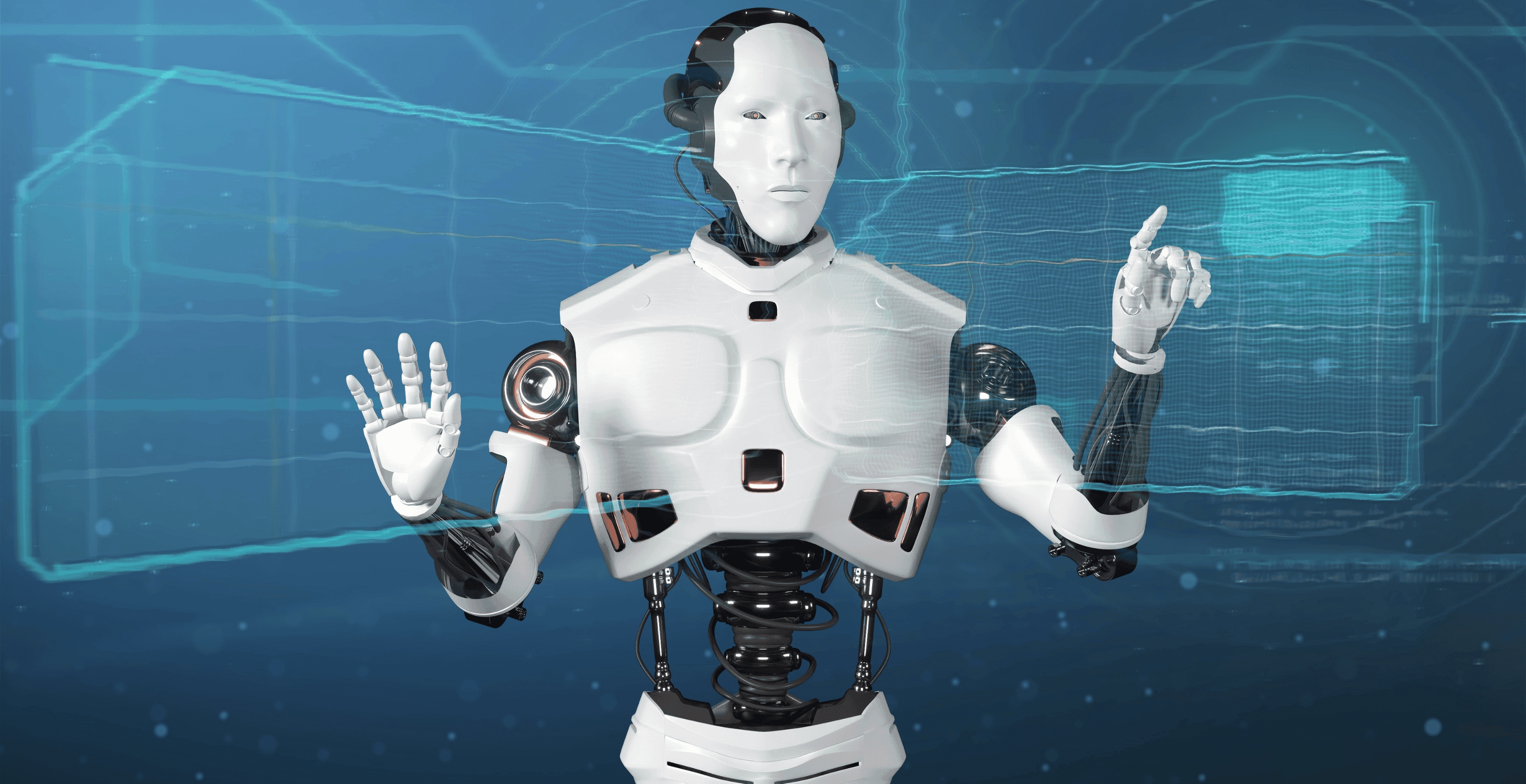
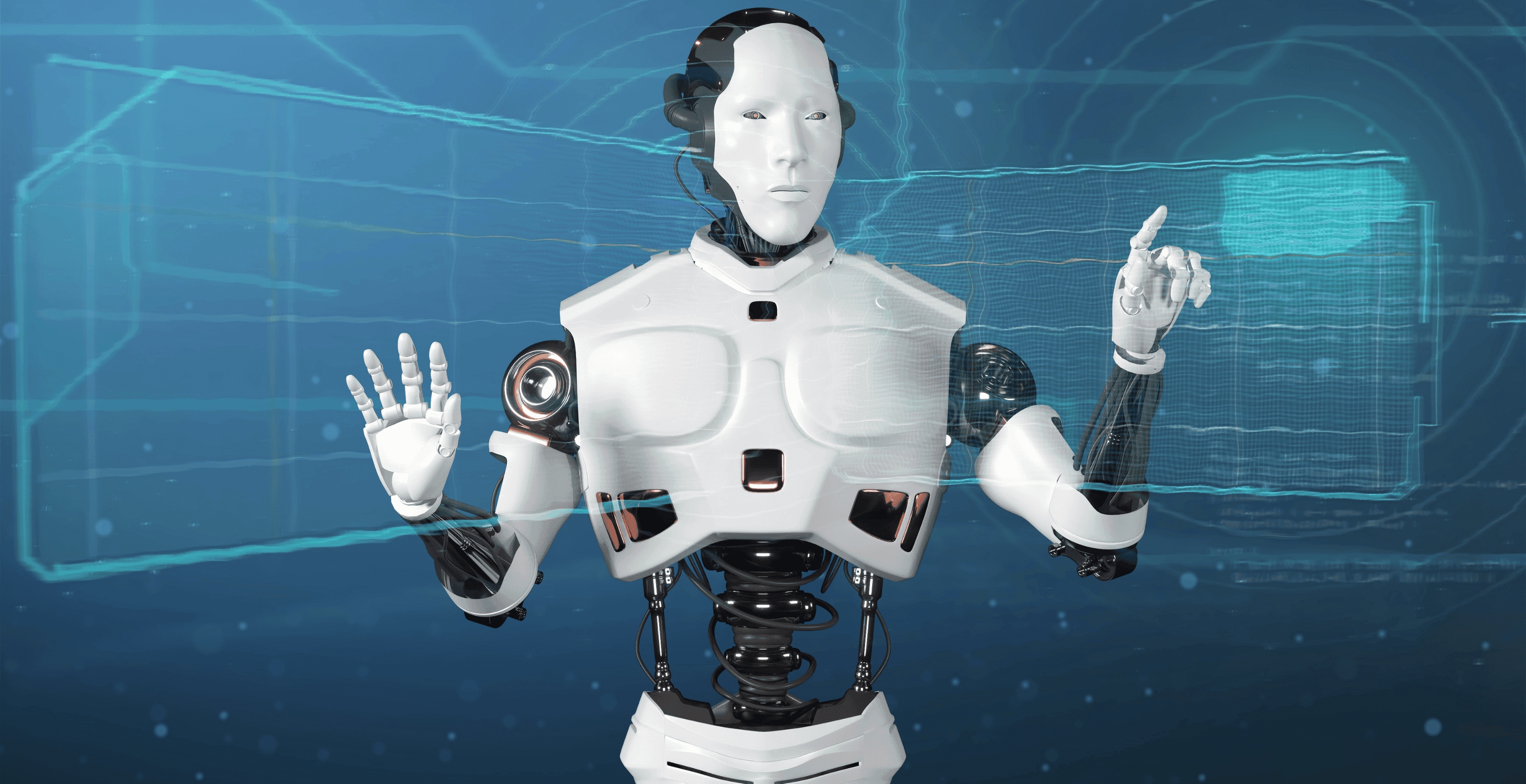
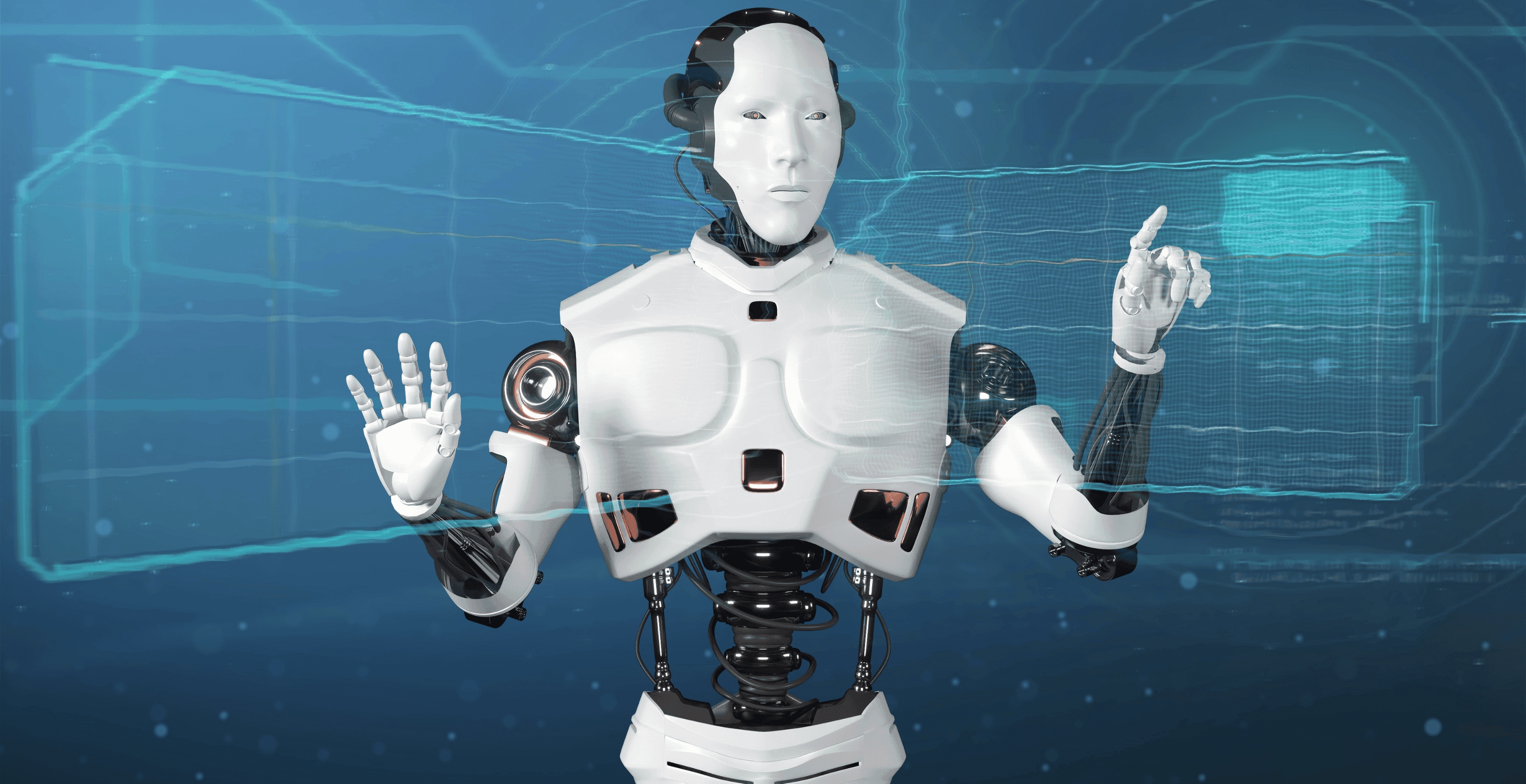
Generative AI And Document Question Answering With LLMs
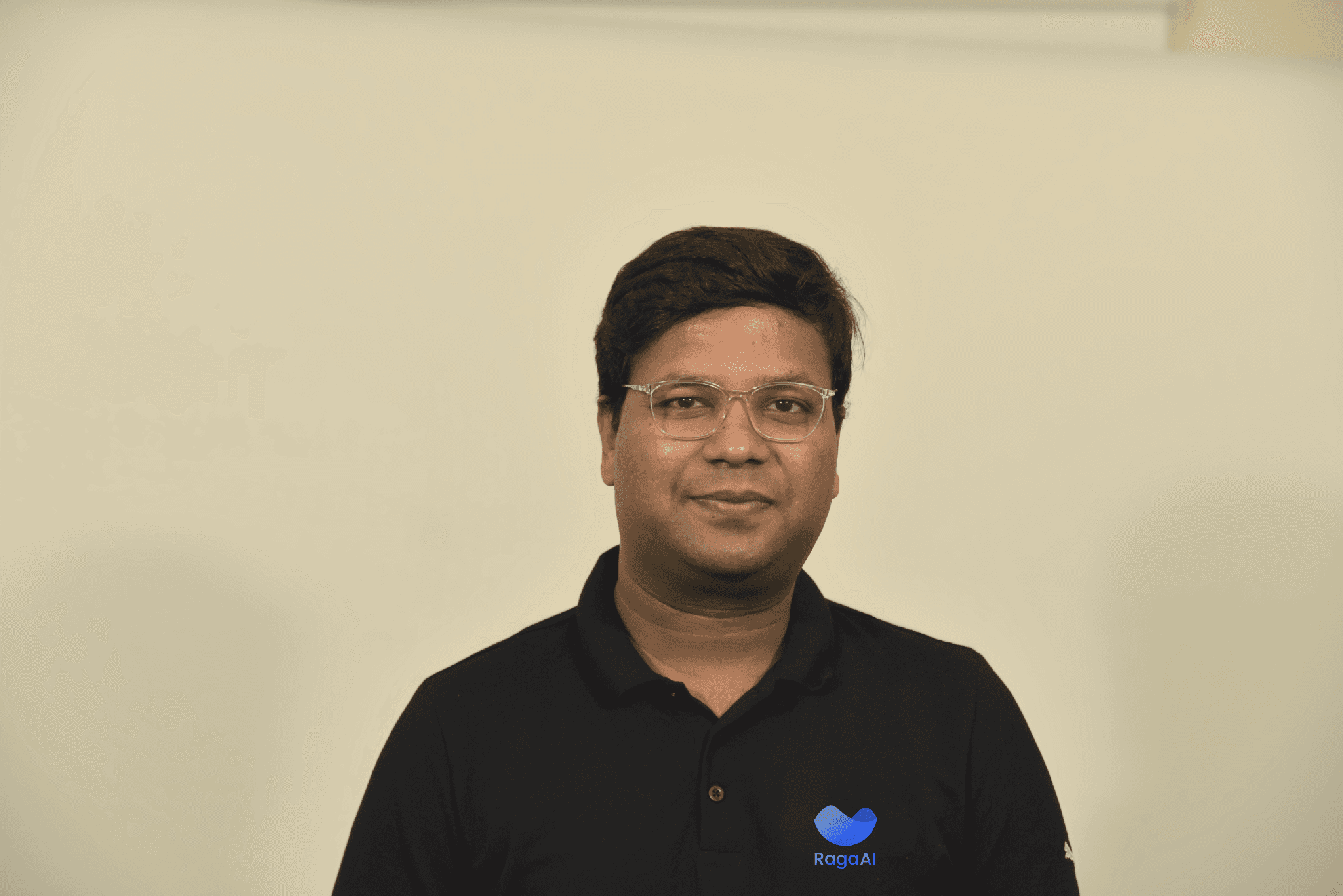
Jigar Gupta
Jul 15, 2024
Read the article




How to Fine-Tune ChatGPT for Your Use Case - Step by Step Guide
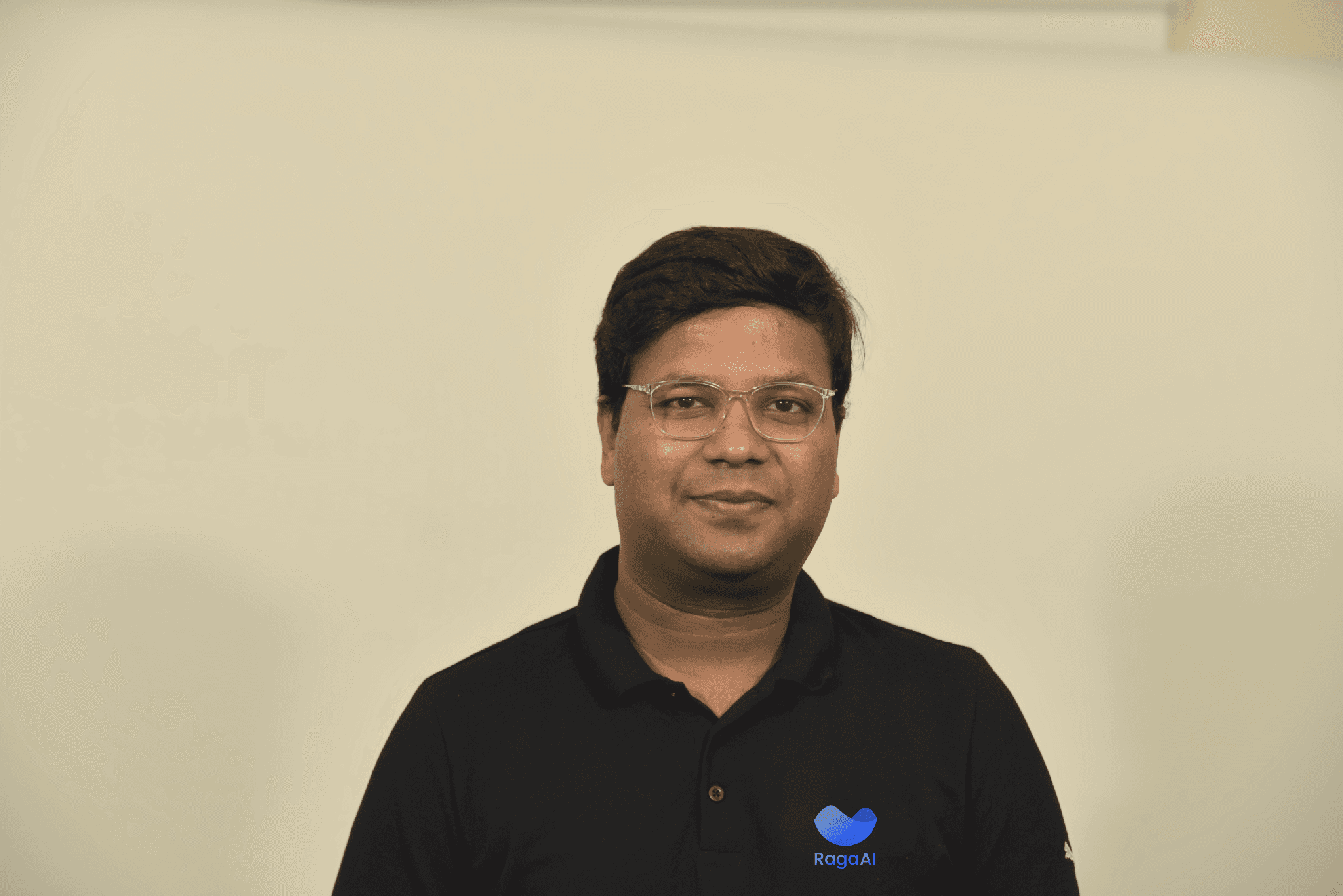
Jigar Gupta
Jul 15, 2024
Read the article




Security and LLM Firewall Controls

Rehan Asif
Jul 15, 2024
Read the article
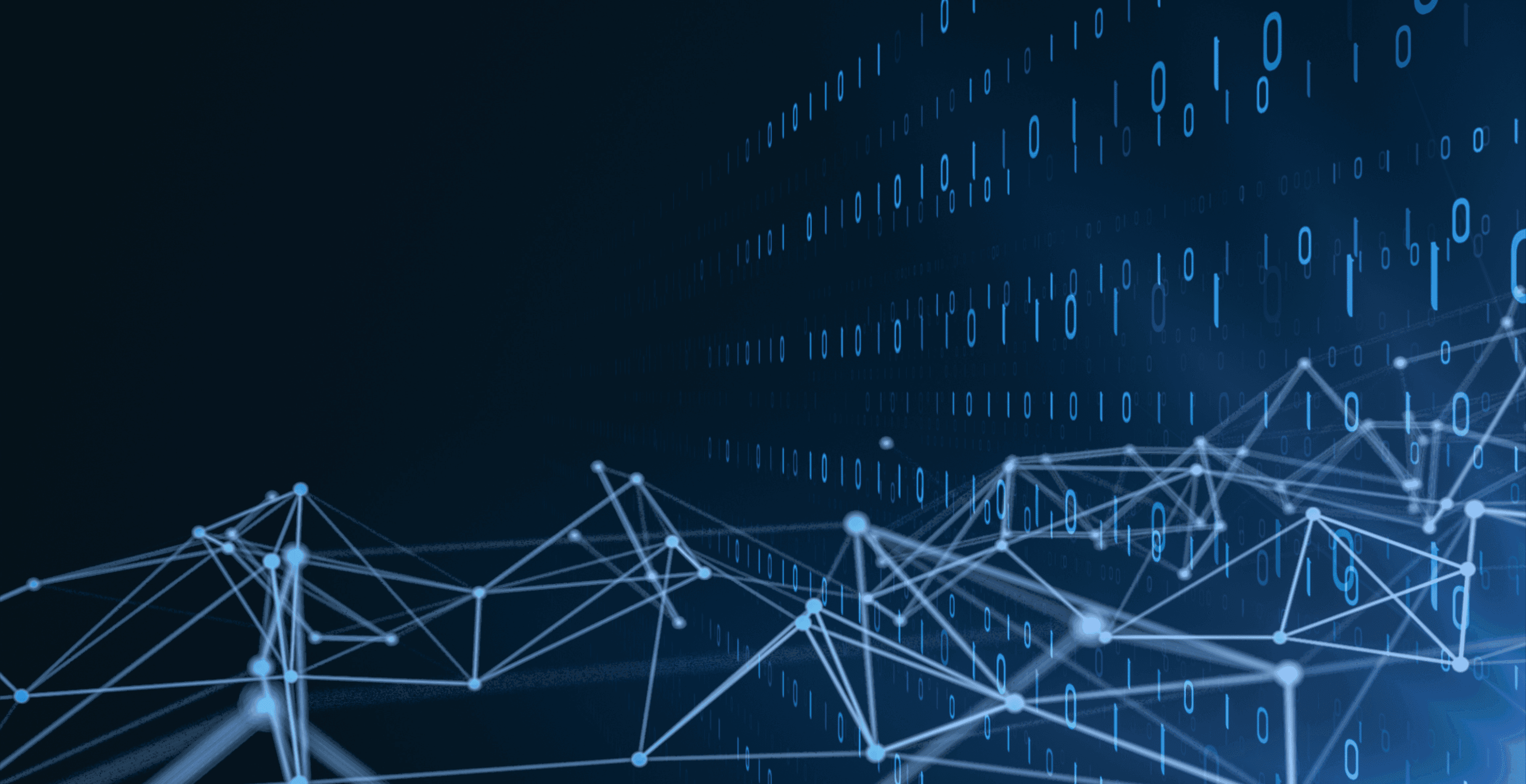
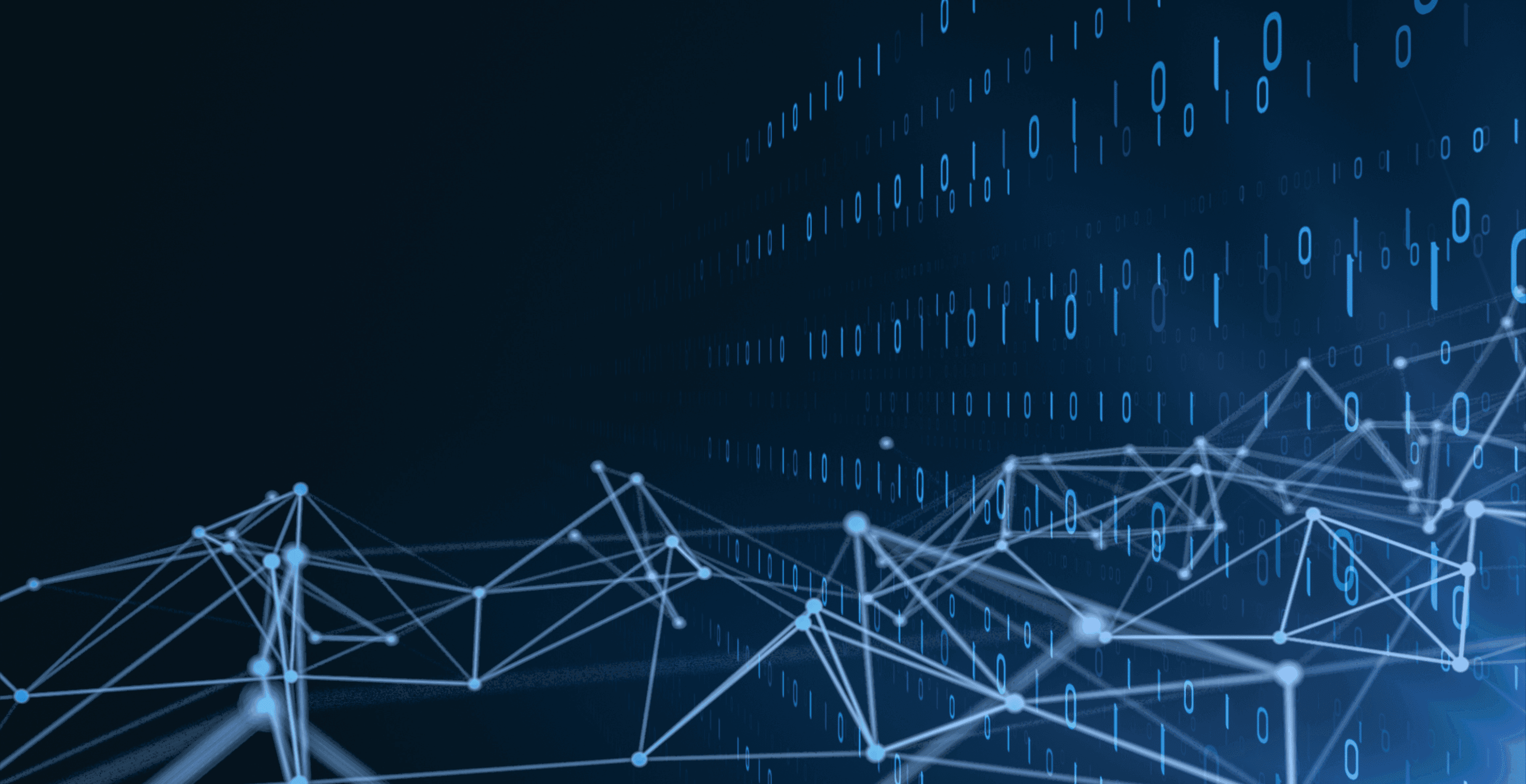
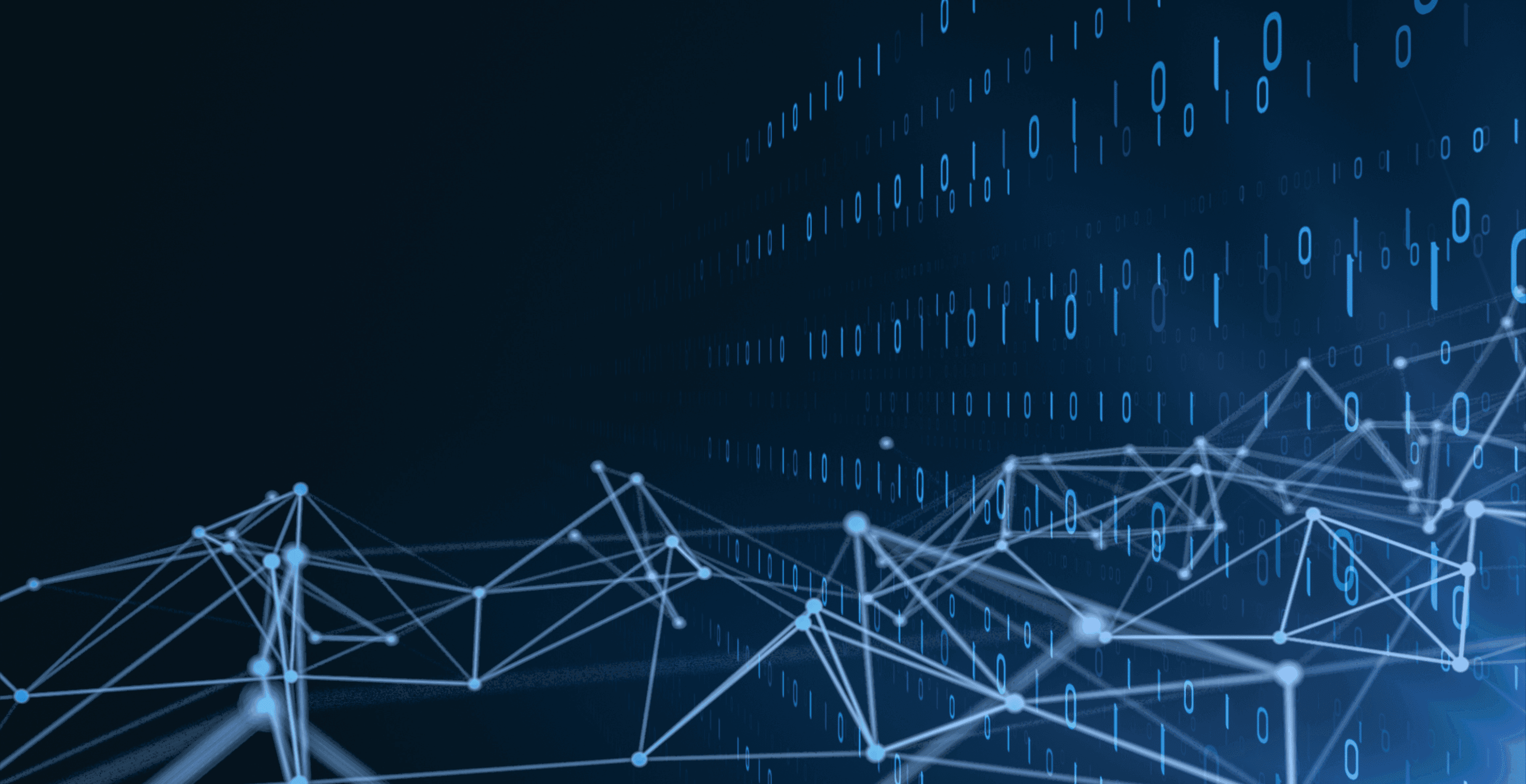
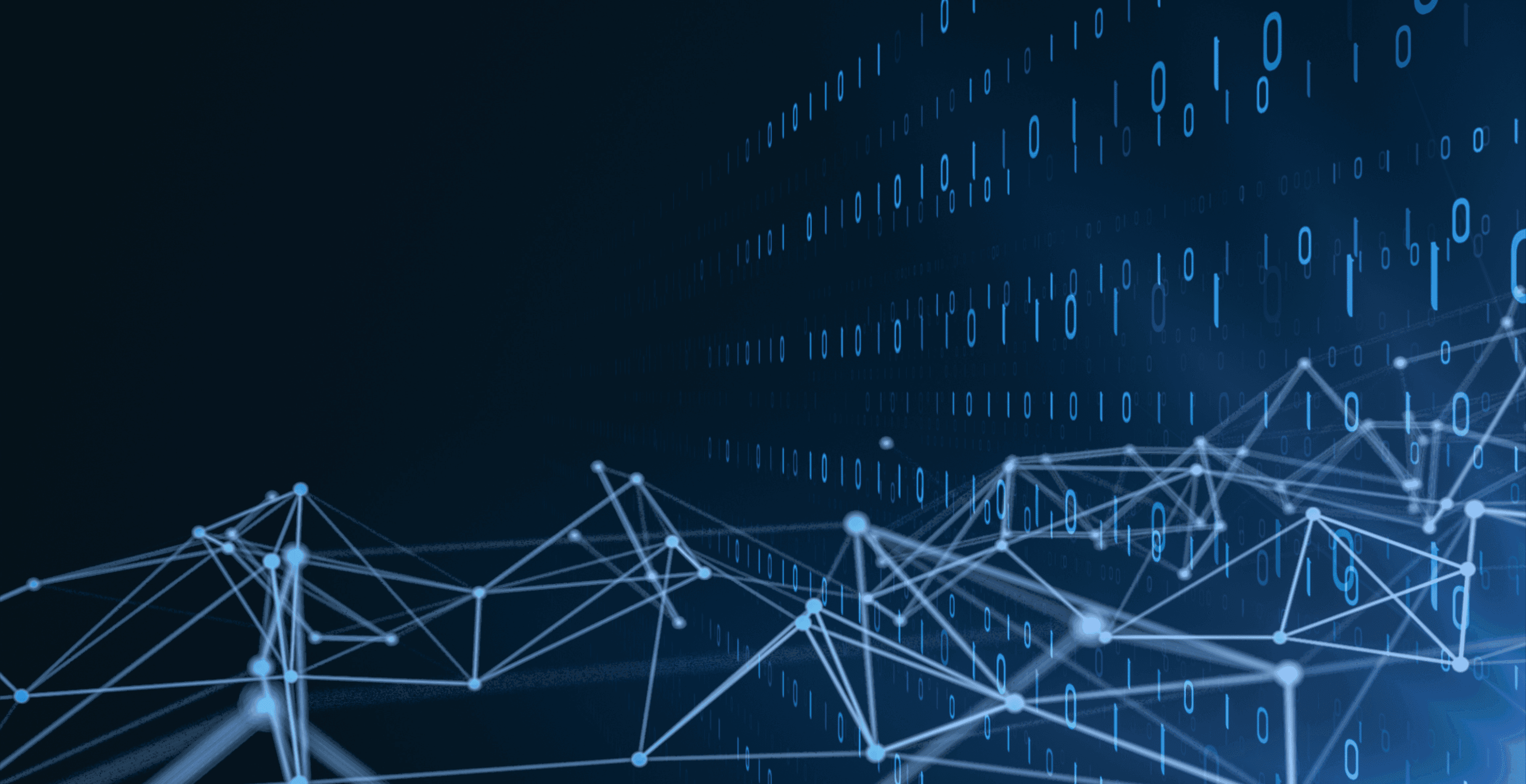
Understanding the Use of Guardrail Metrics in Ensuring LLM Safety

Rehan Asif
Jul 13, 2024
Read the article
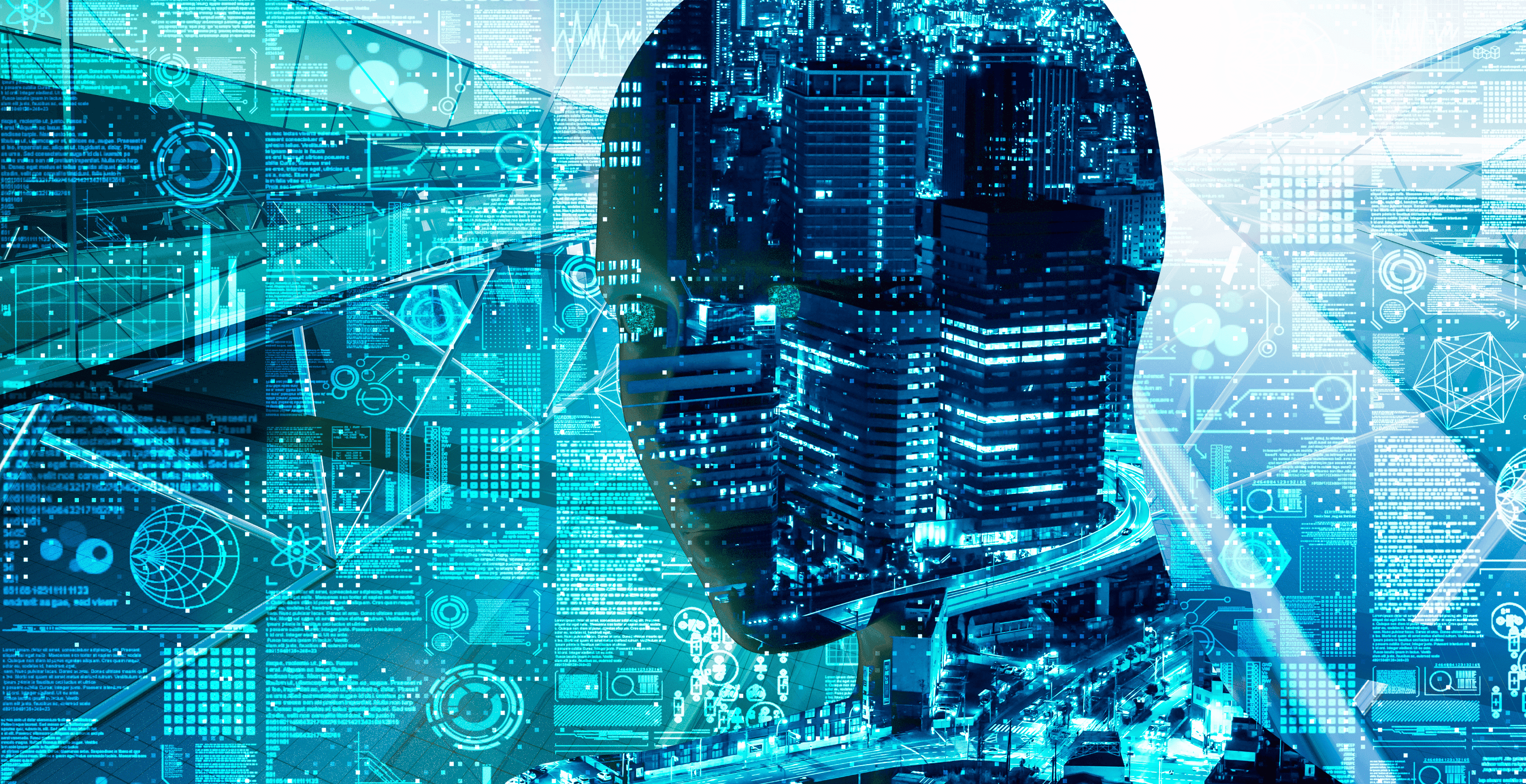
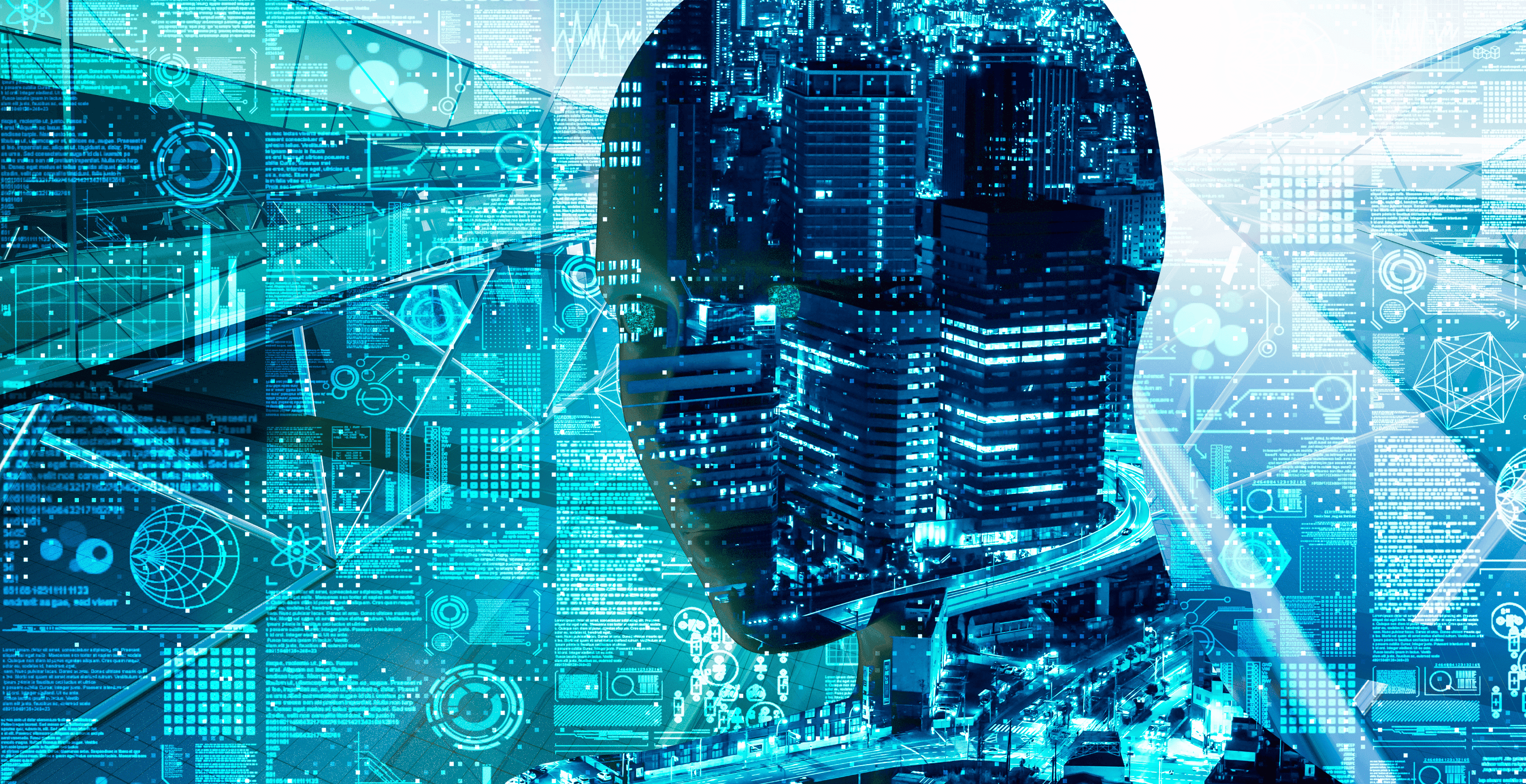
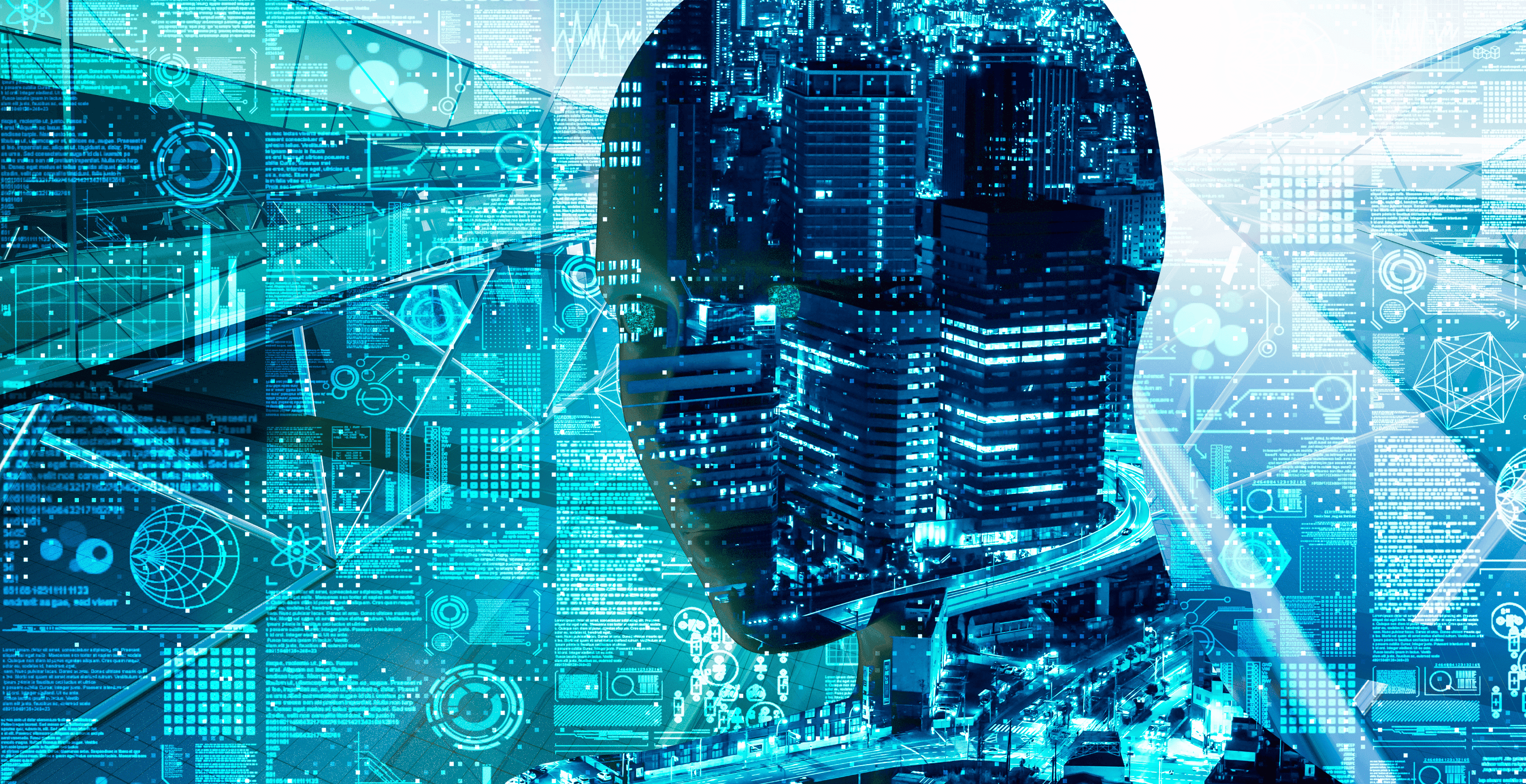
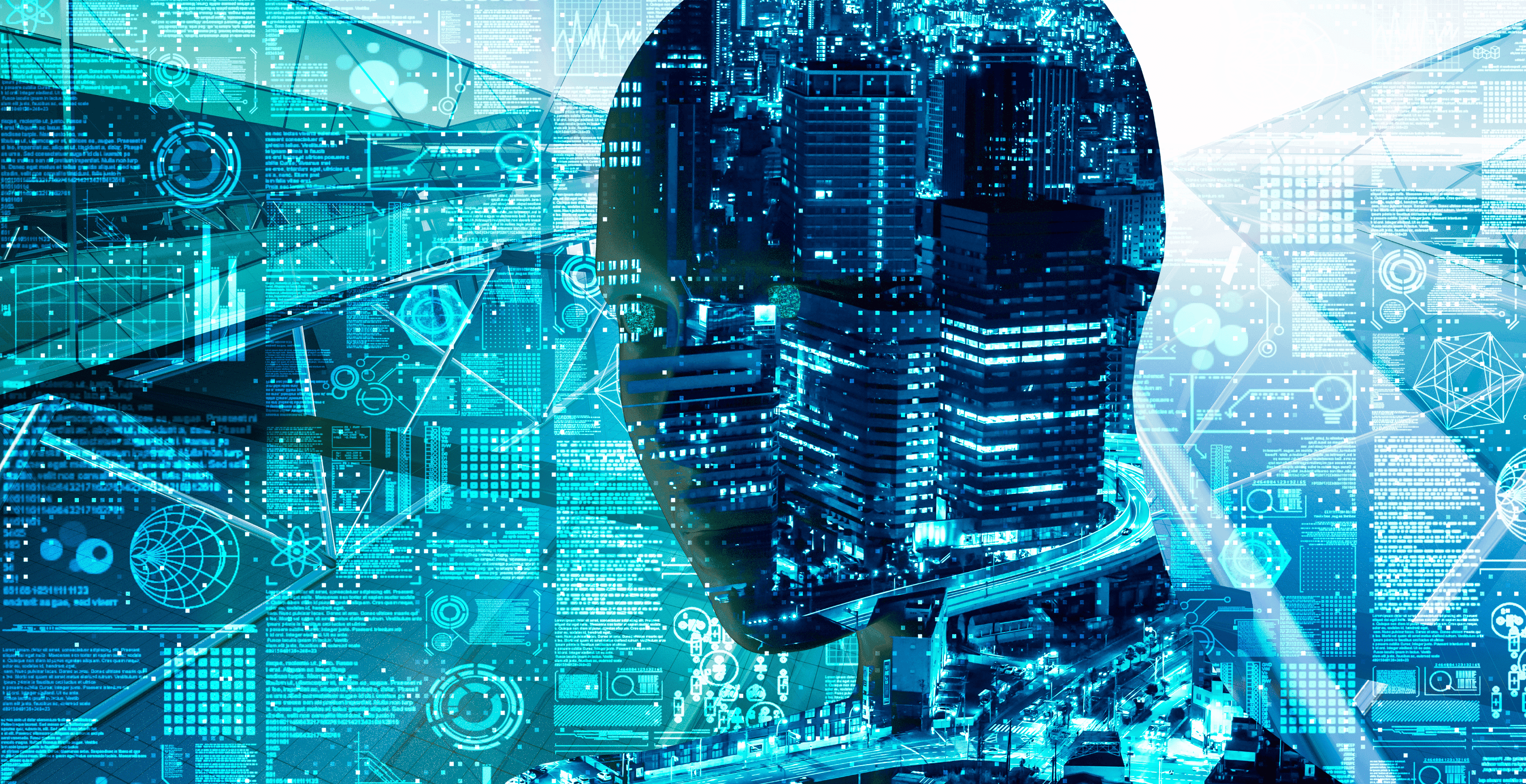
Exploring the Future of LLM and Generative AI Infrastructure

Rehan Asif
Jul 13, 2024
Read the article




Comprehensive Guide to RLHF and Fine Tuning LLMs from Scratch

Rehan Asif
Jul 13, 2024
Read the article
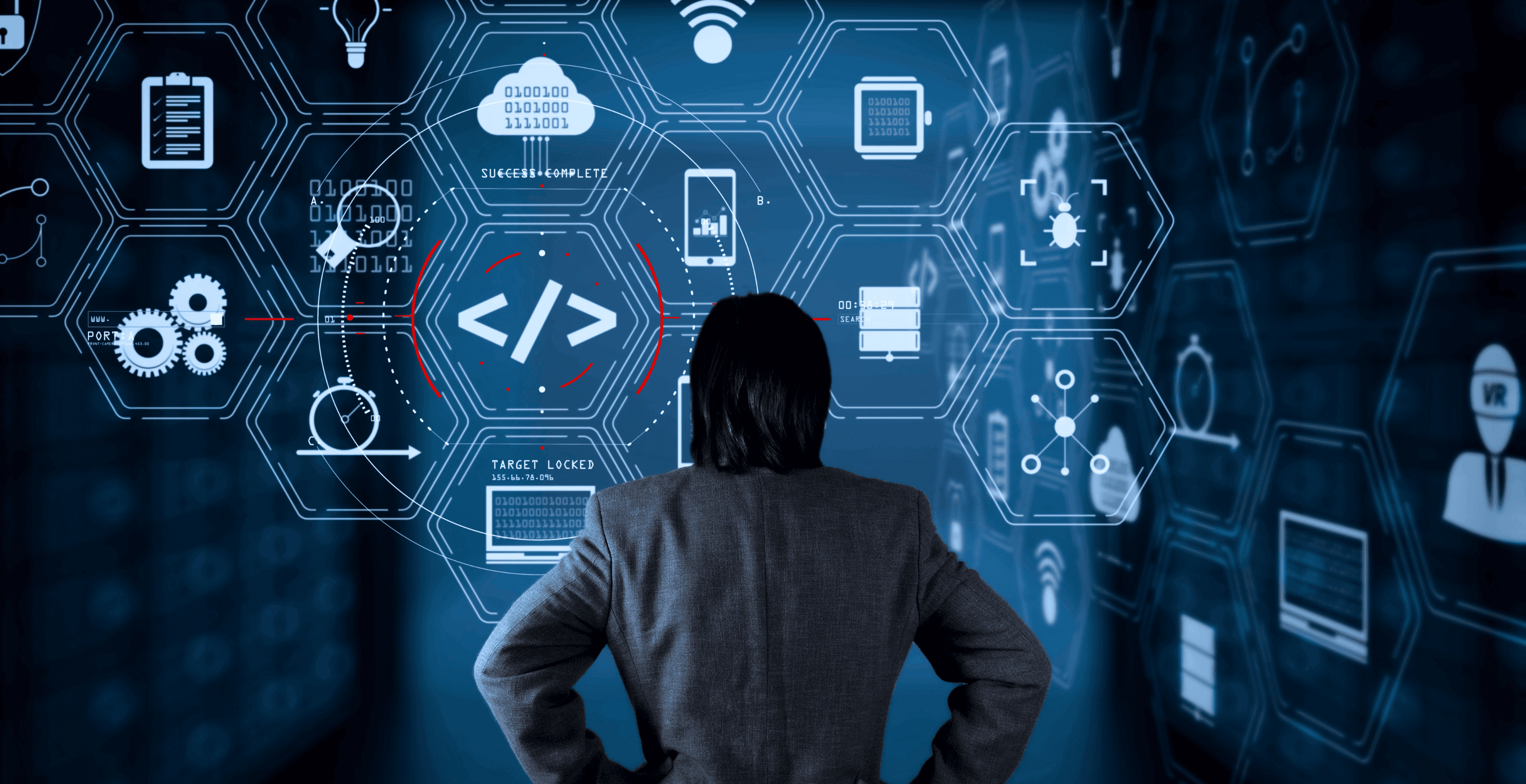
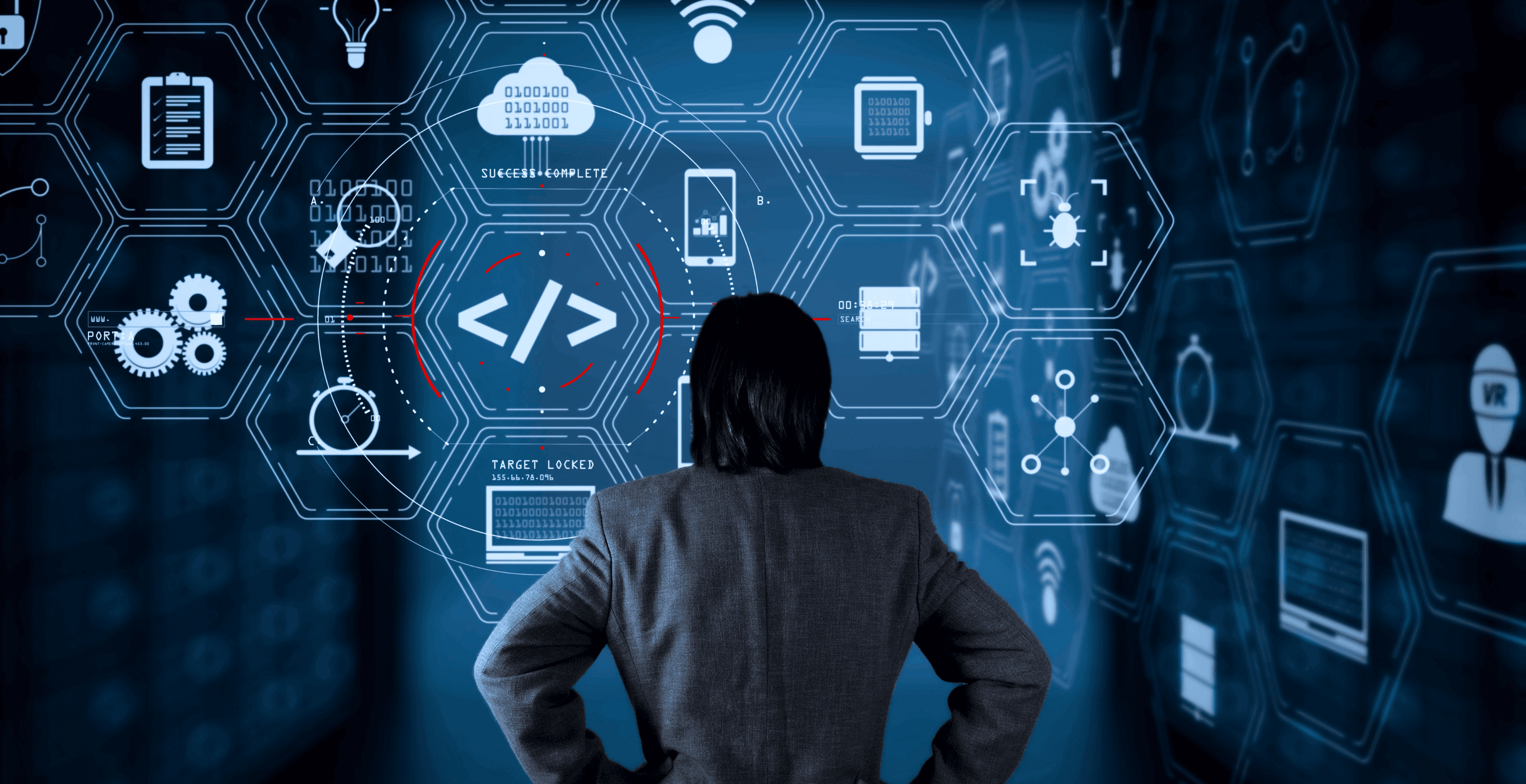
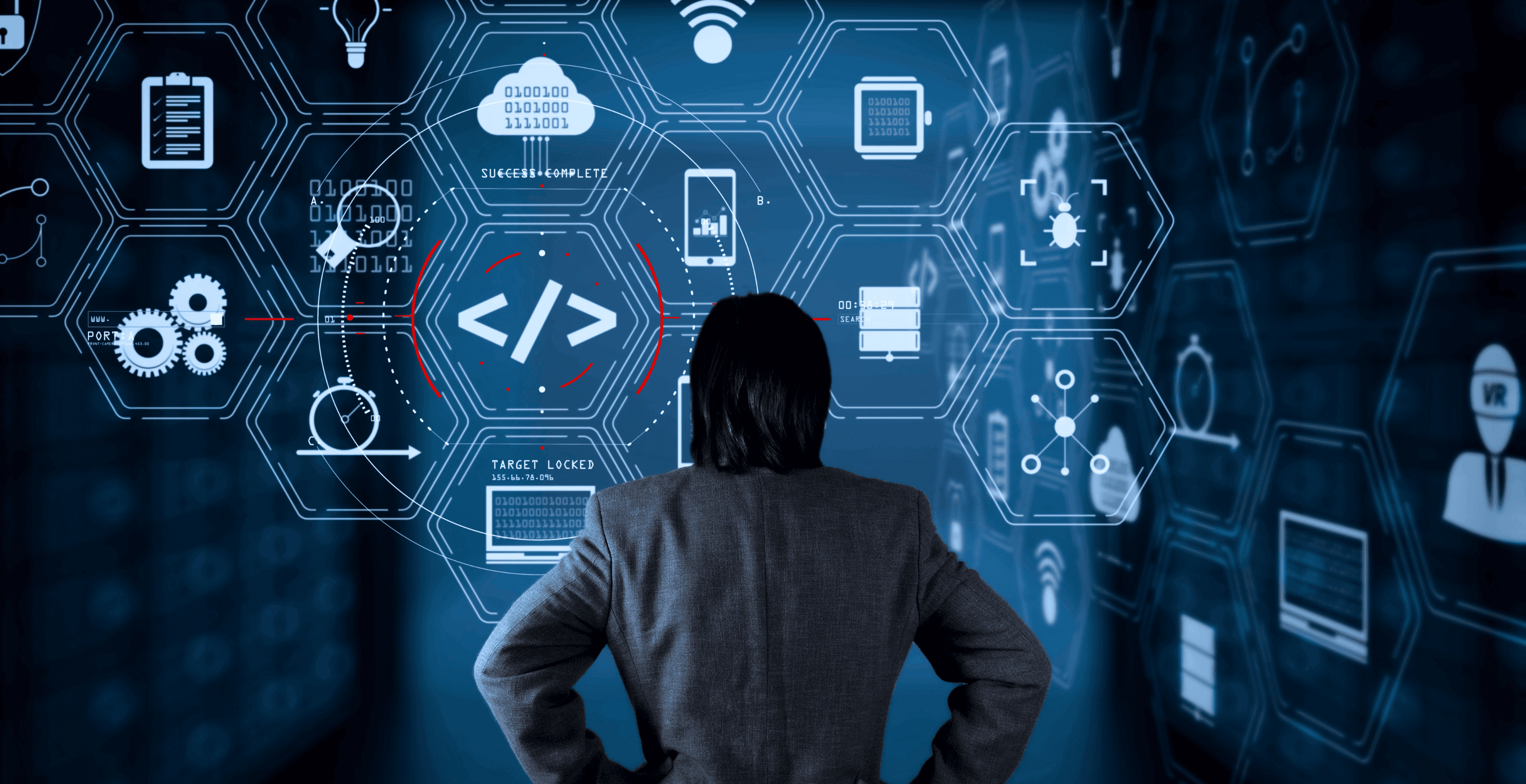
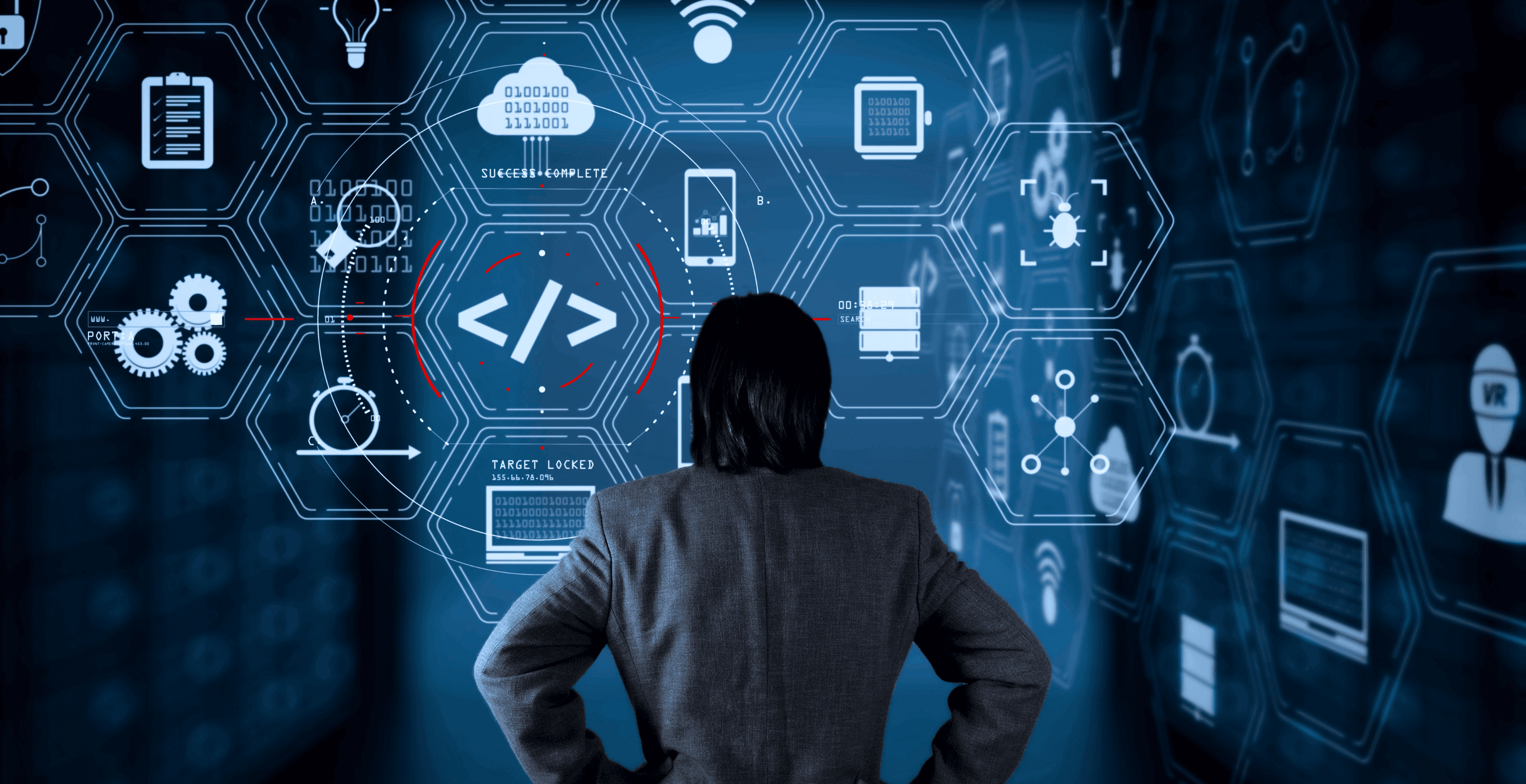
Using Synthetic Data To Enrich RAG Applications
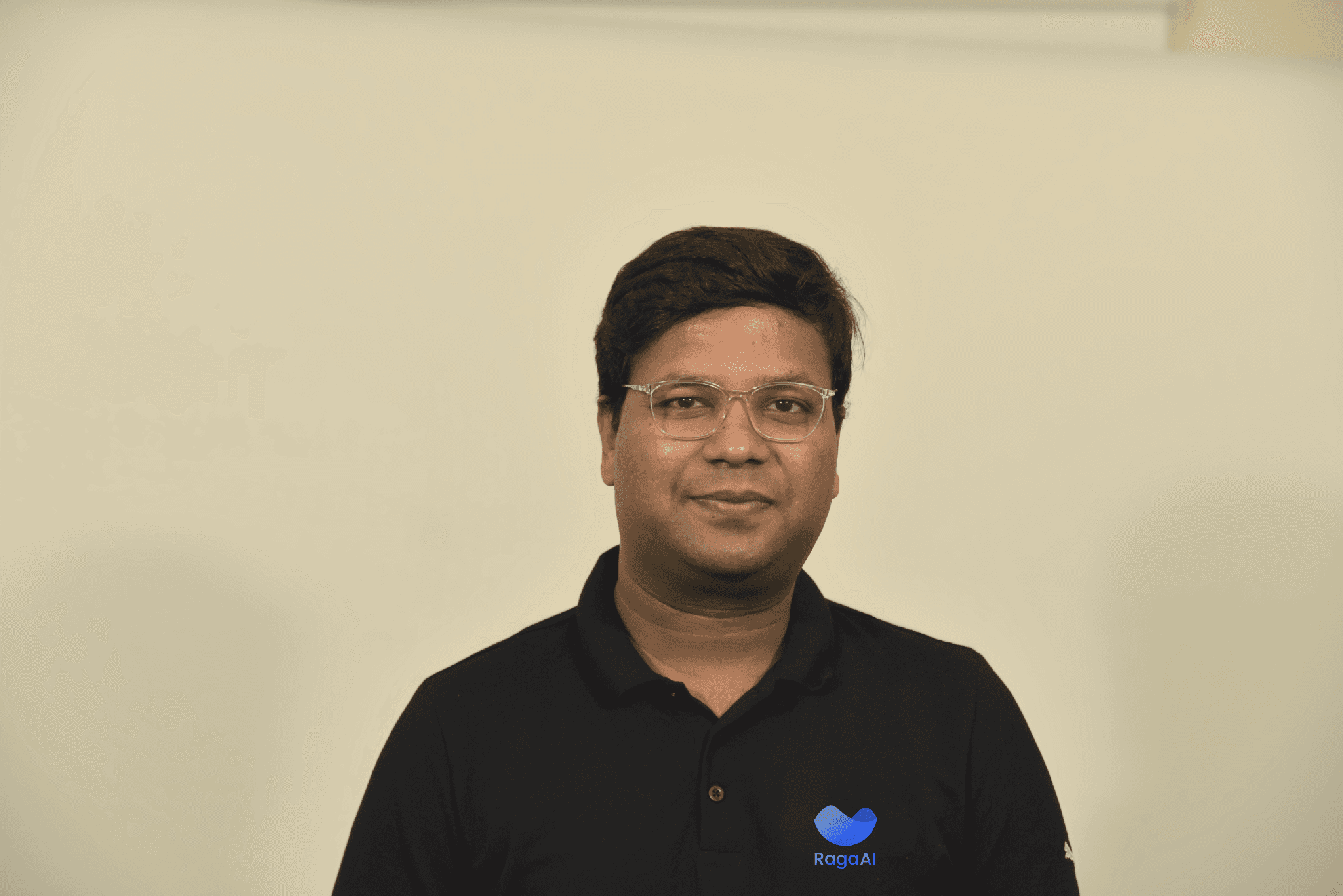
Jigar Gupta
Jul 13, 2024
Read the article




Comparing Different Large Language Model (LLM) Frameworks

Rehan Asif
Jul 12, 2024
Read the article
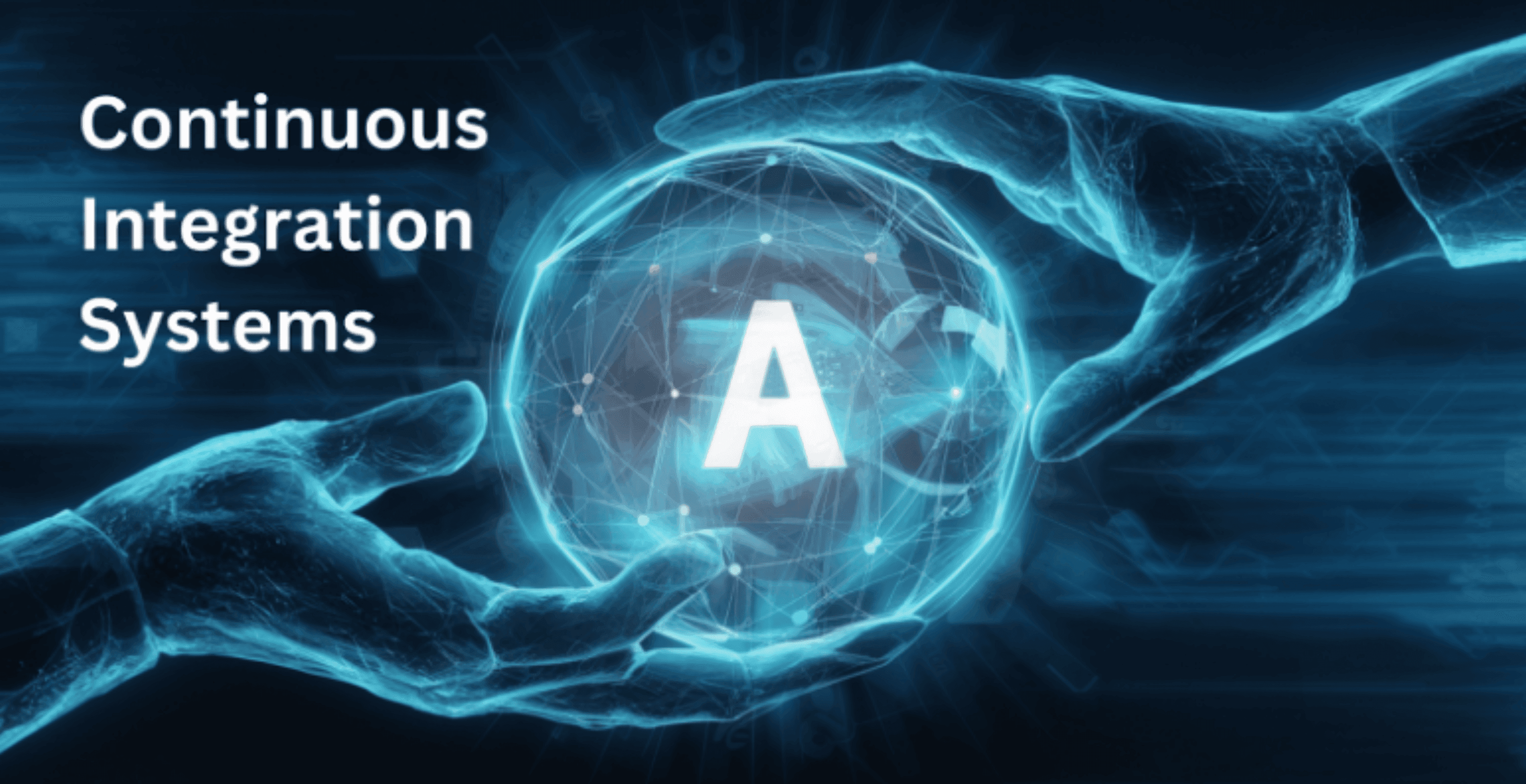
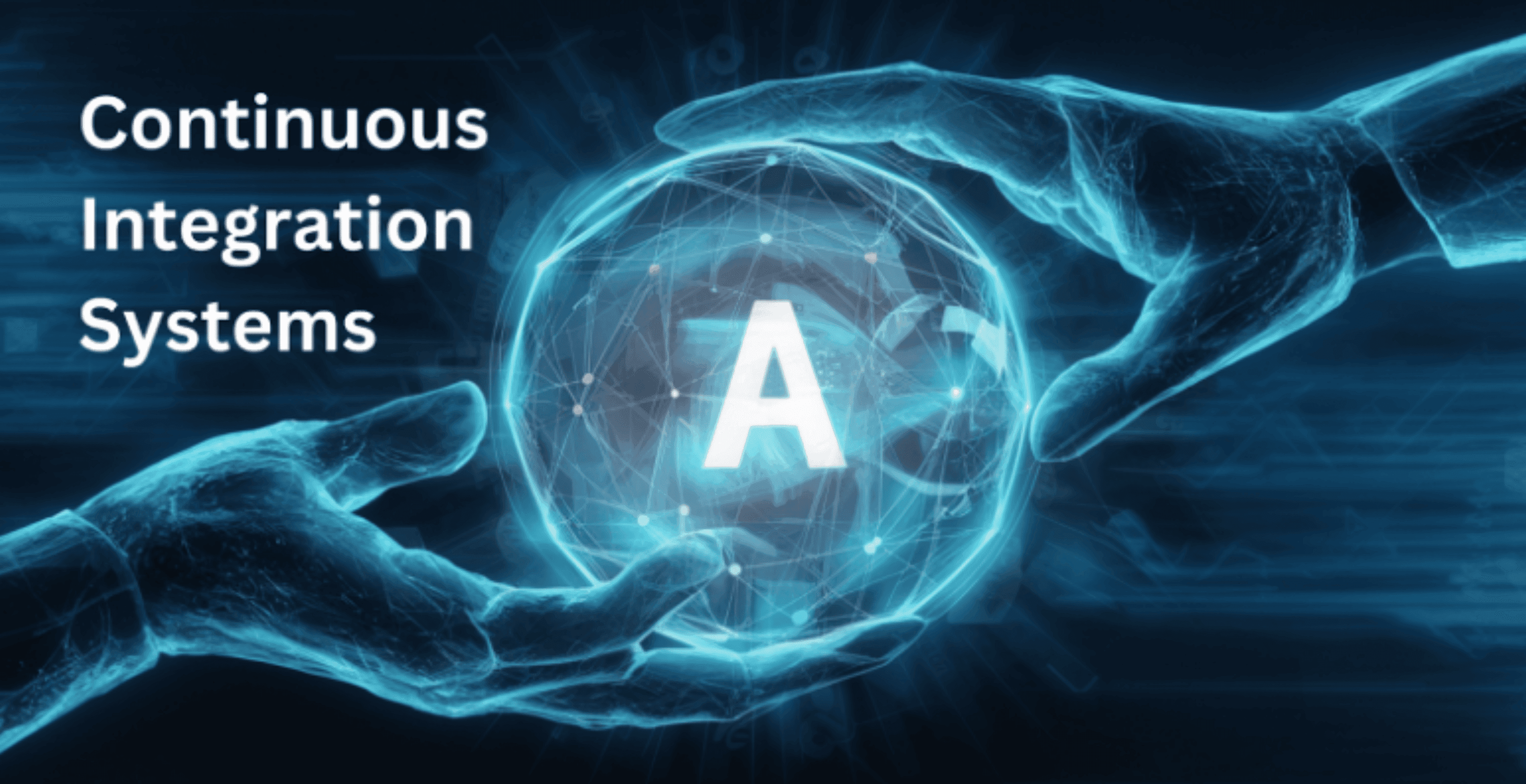
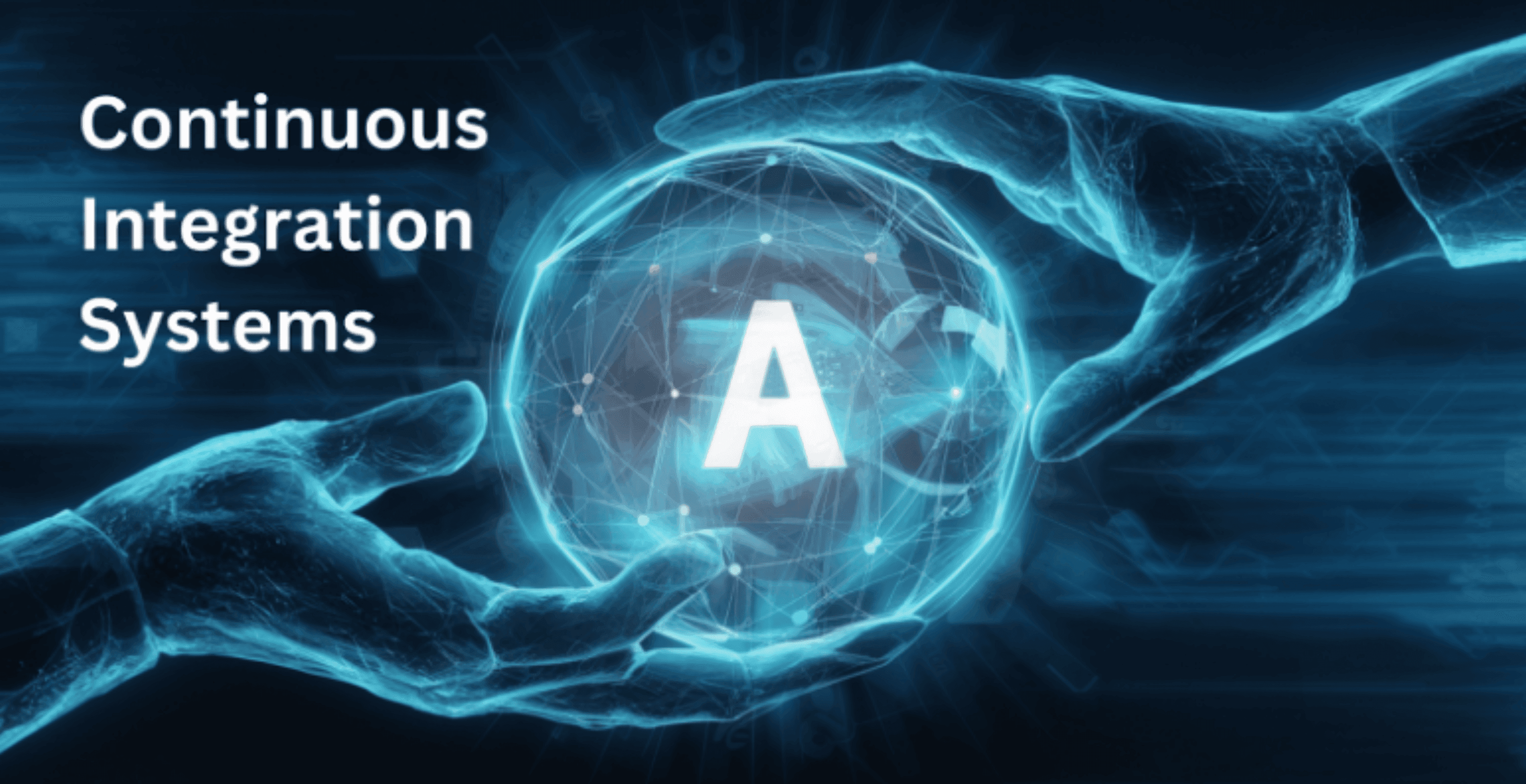
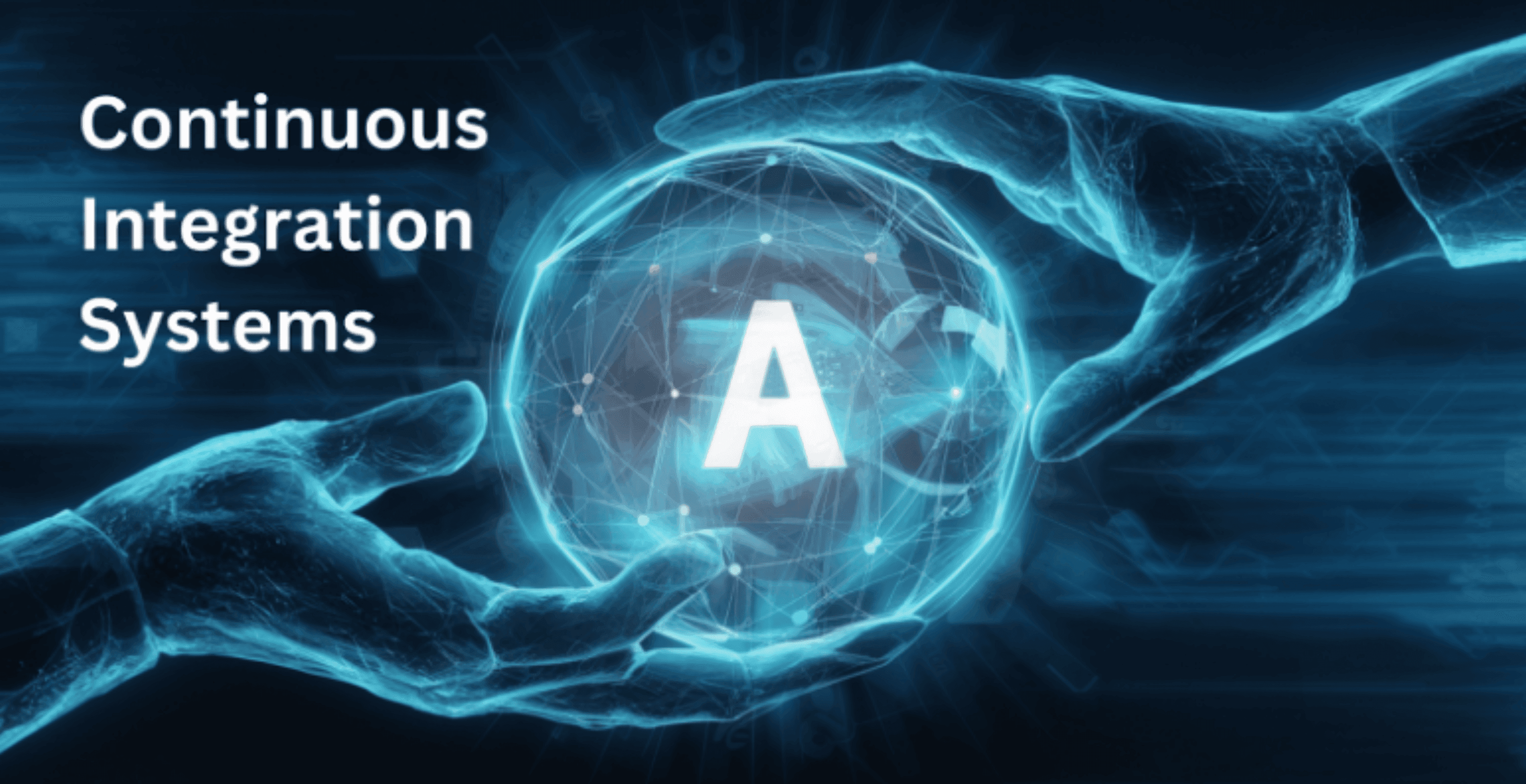
Integrating AI Models with Continuous Integration Systems
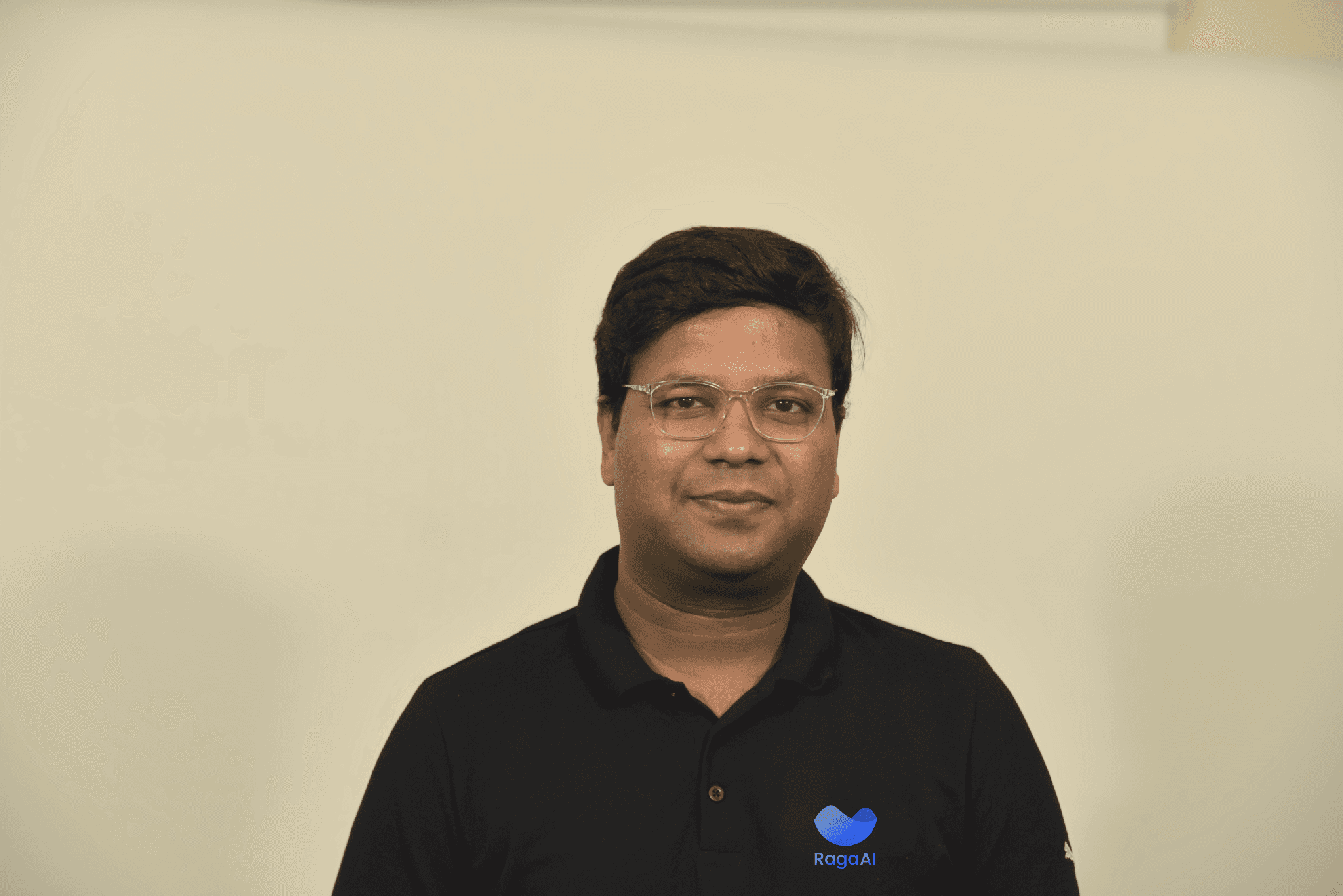
Jigar Gupta
Jul 12, 2024
Read the article




Understanding Retrieval Augmented Generation for Large Language Models: A Survey
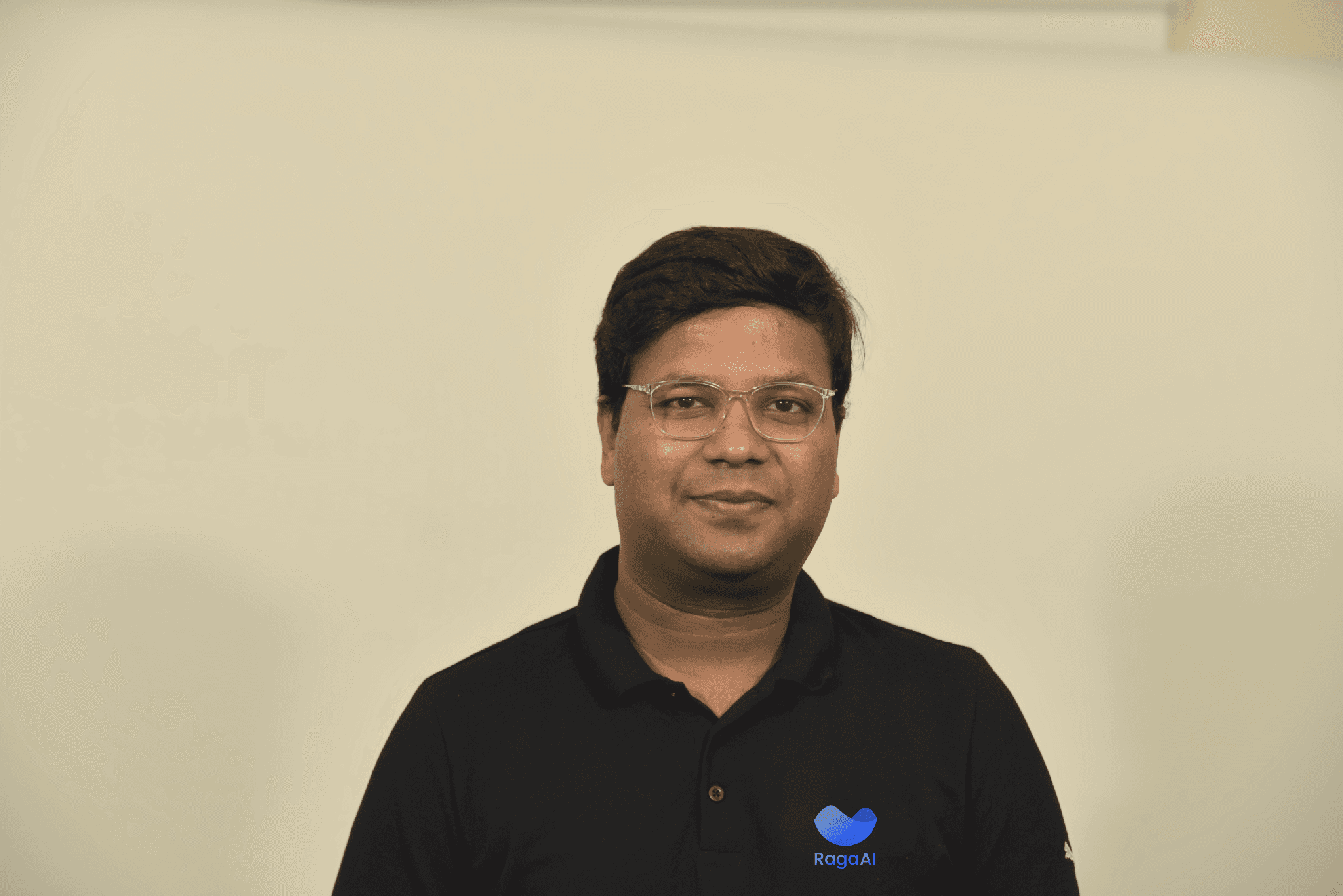
Jigar Gupta
Jul 12, 2024
Read the article




Leveraging AI For Enhanced Retail Customer Experiences
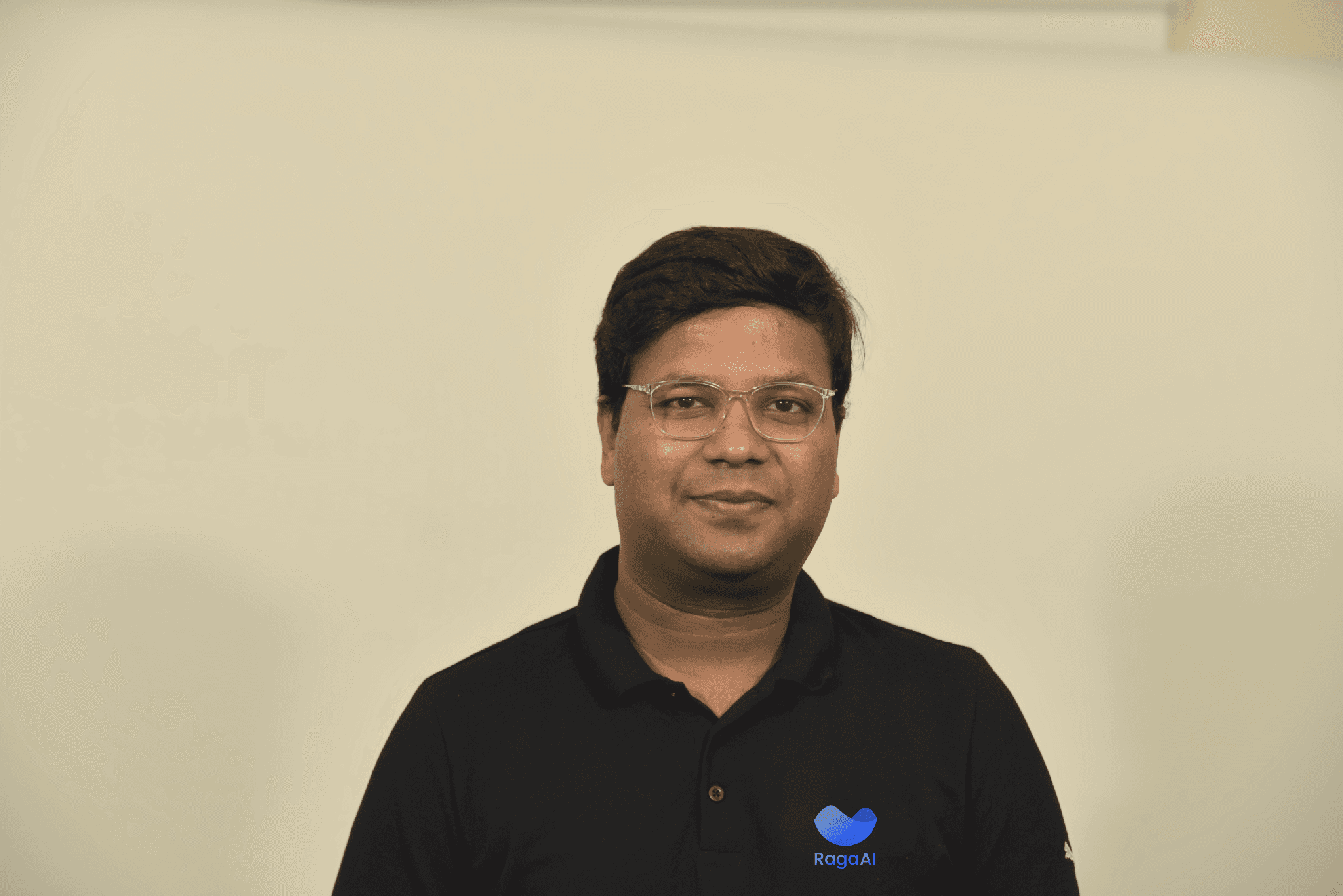
Jigar Gupta
Jul 1, 2024
Read the article
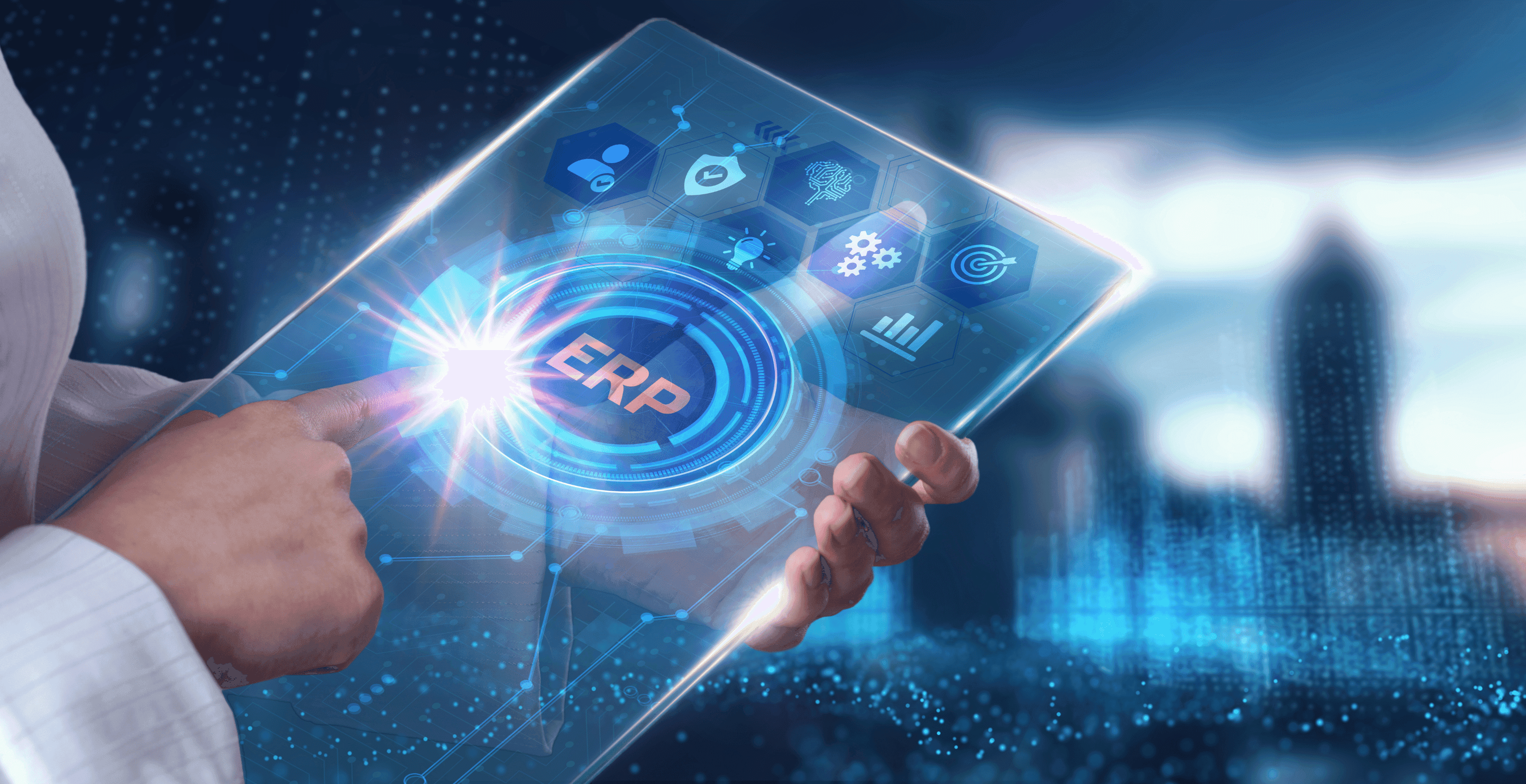
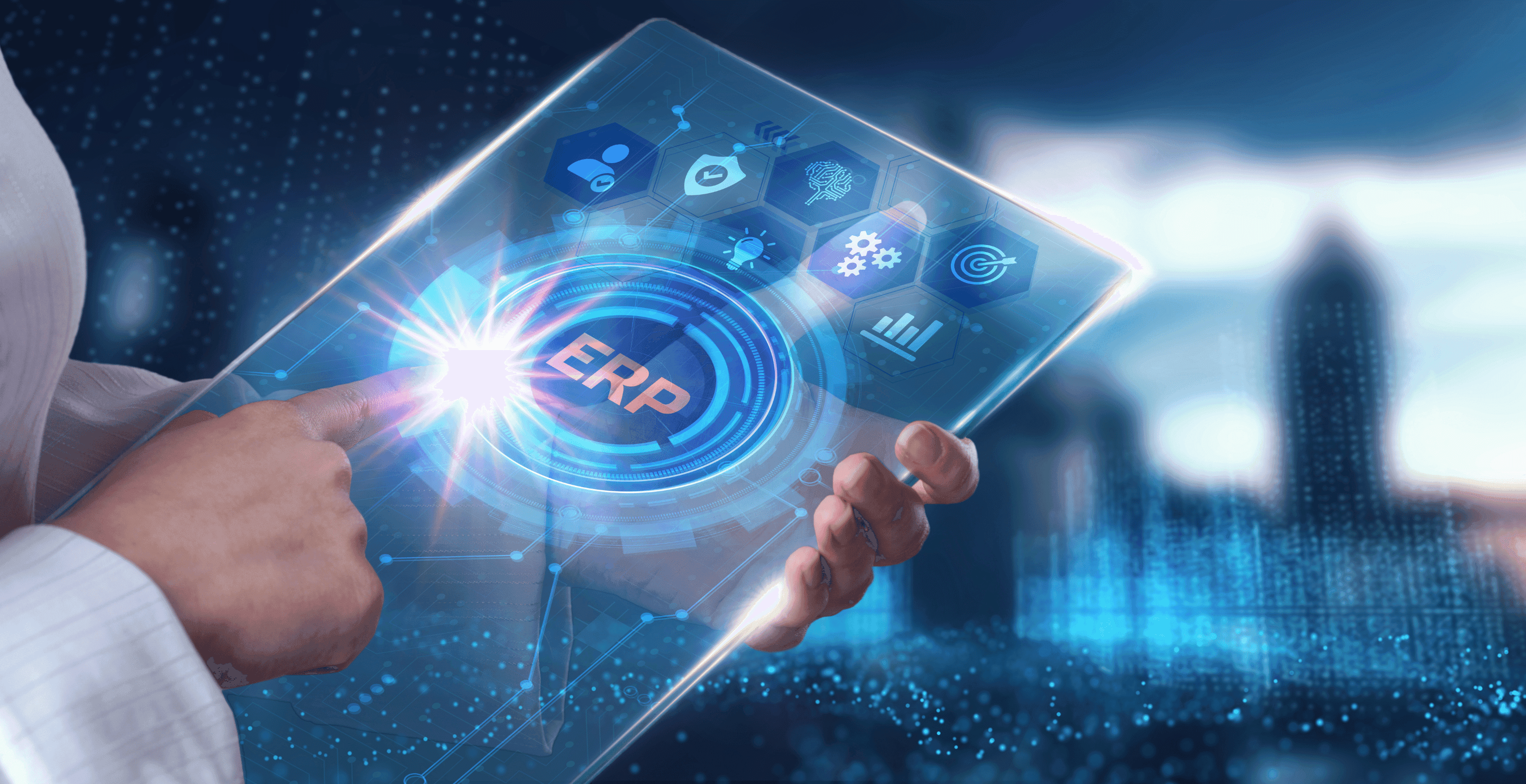
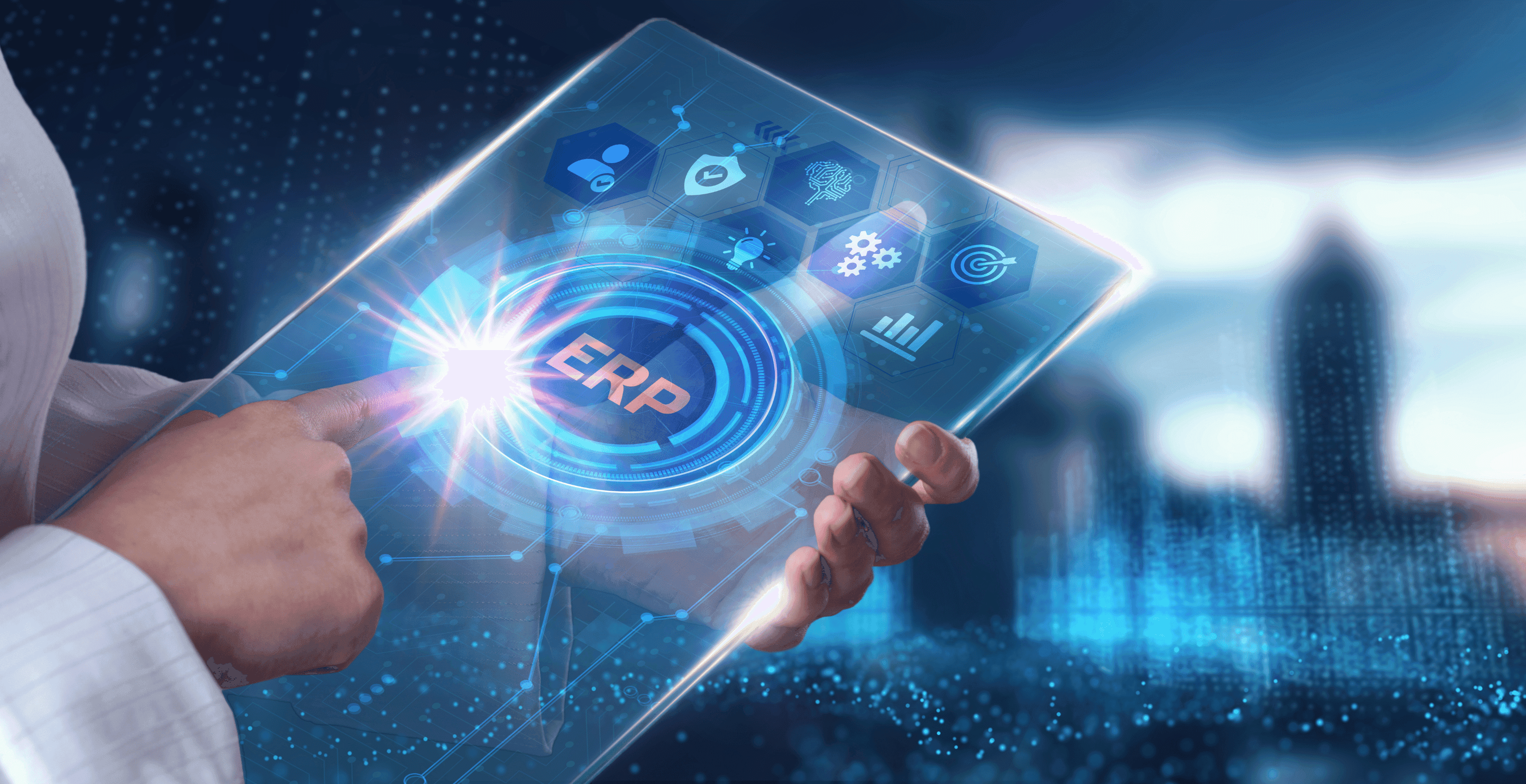
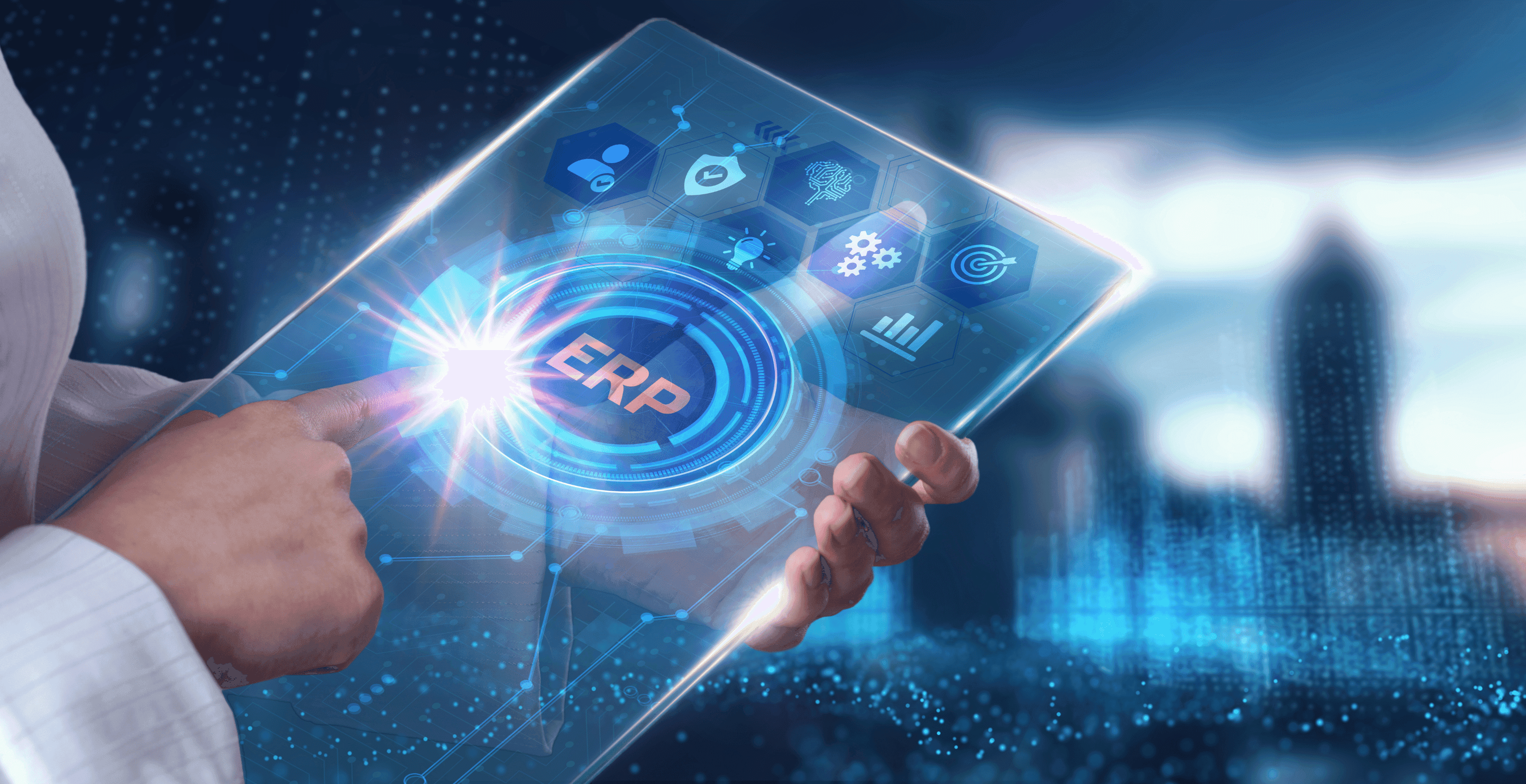
Enhancing Enterprise Search Using RAG and LLMs

Rehan Asif
Jul 1, 2024
Read the article
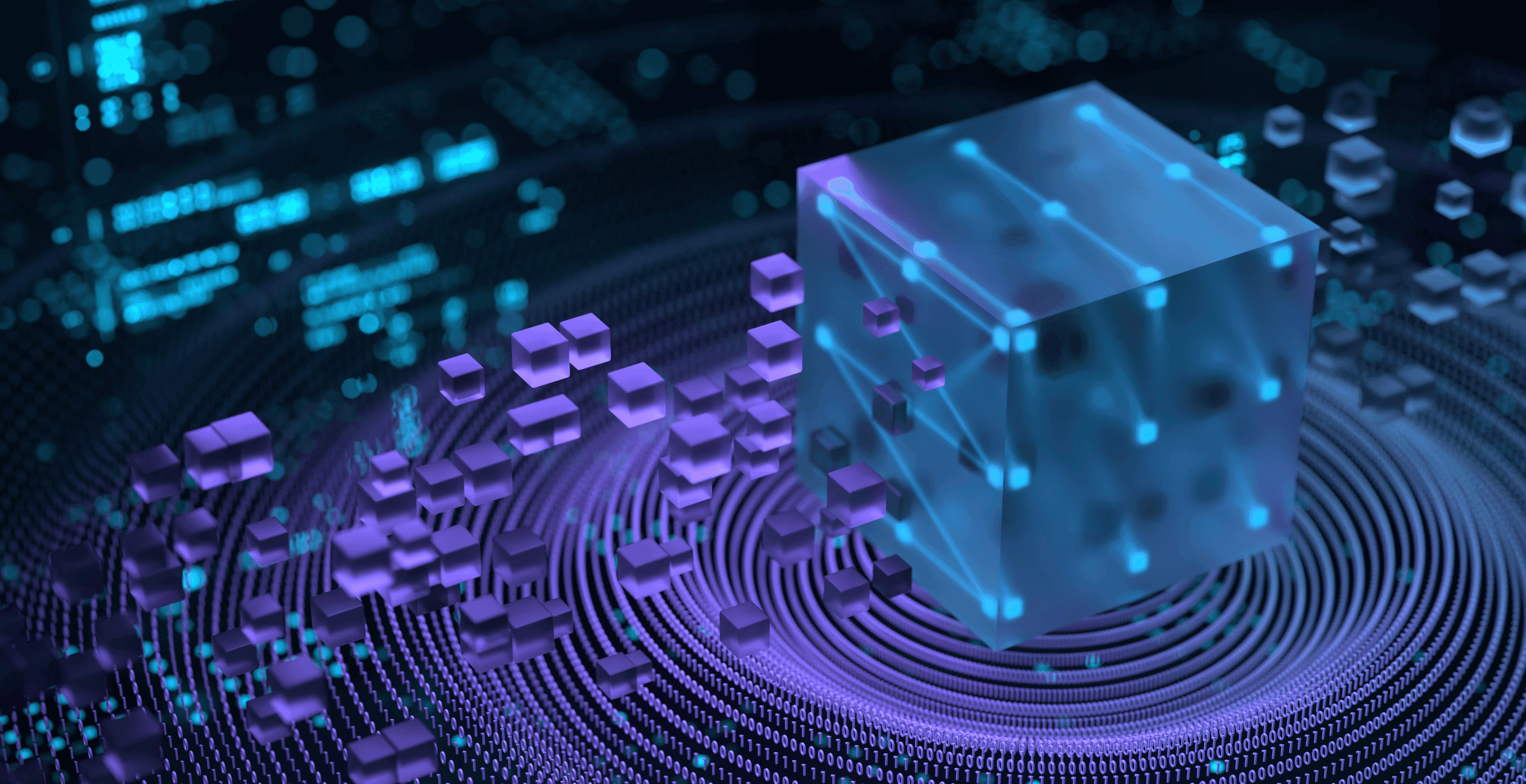
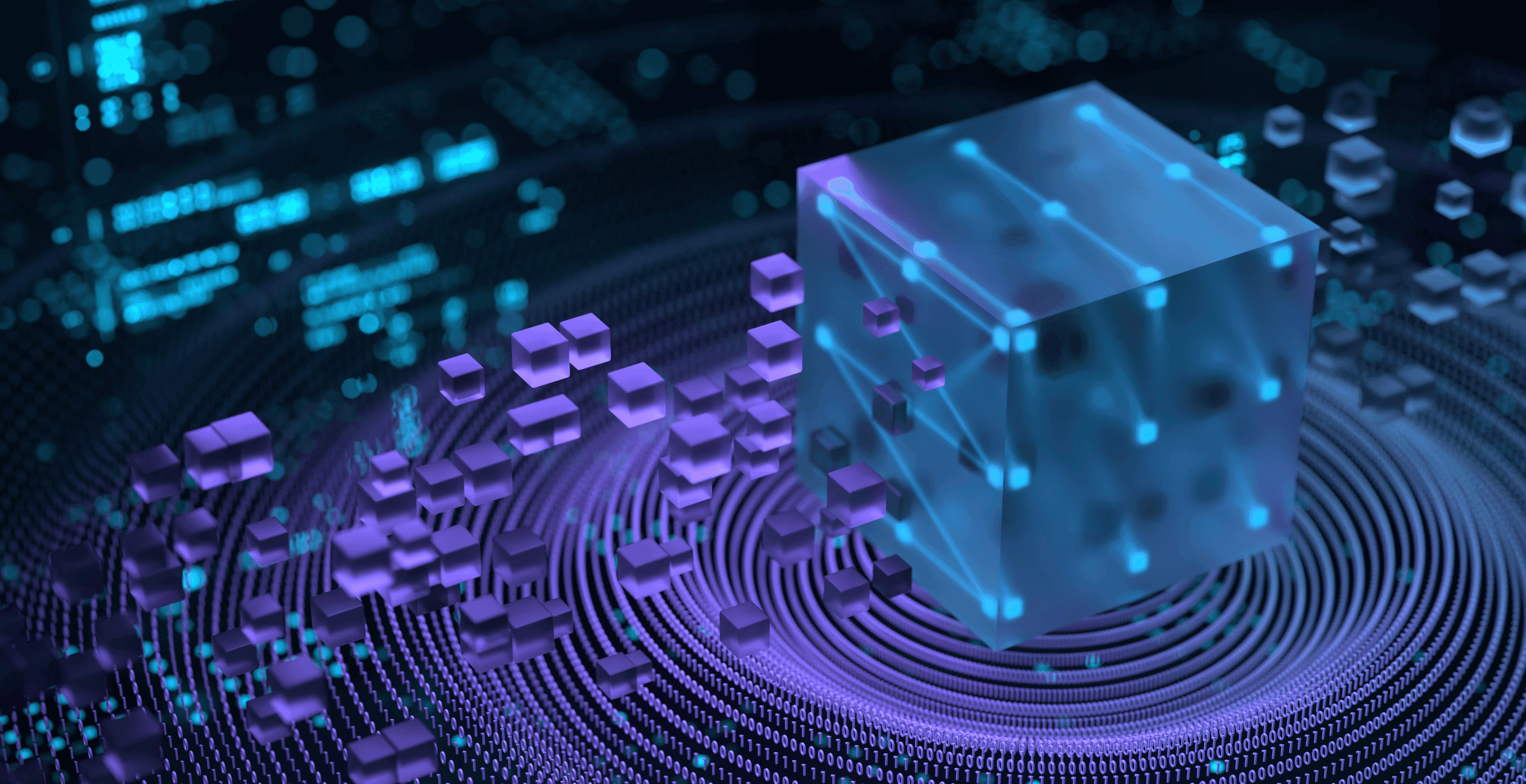
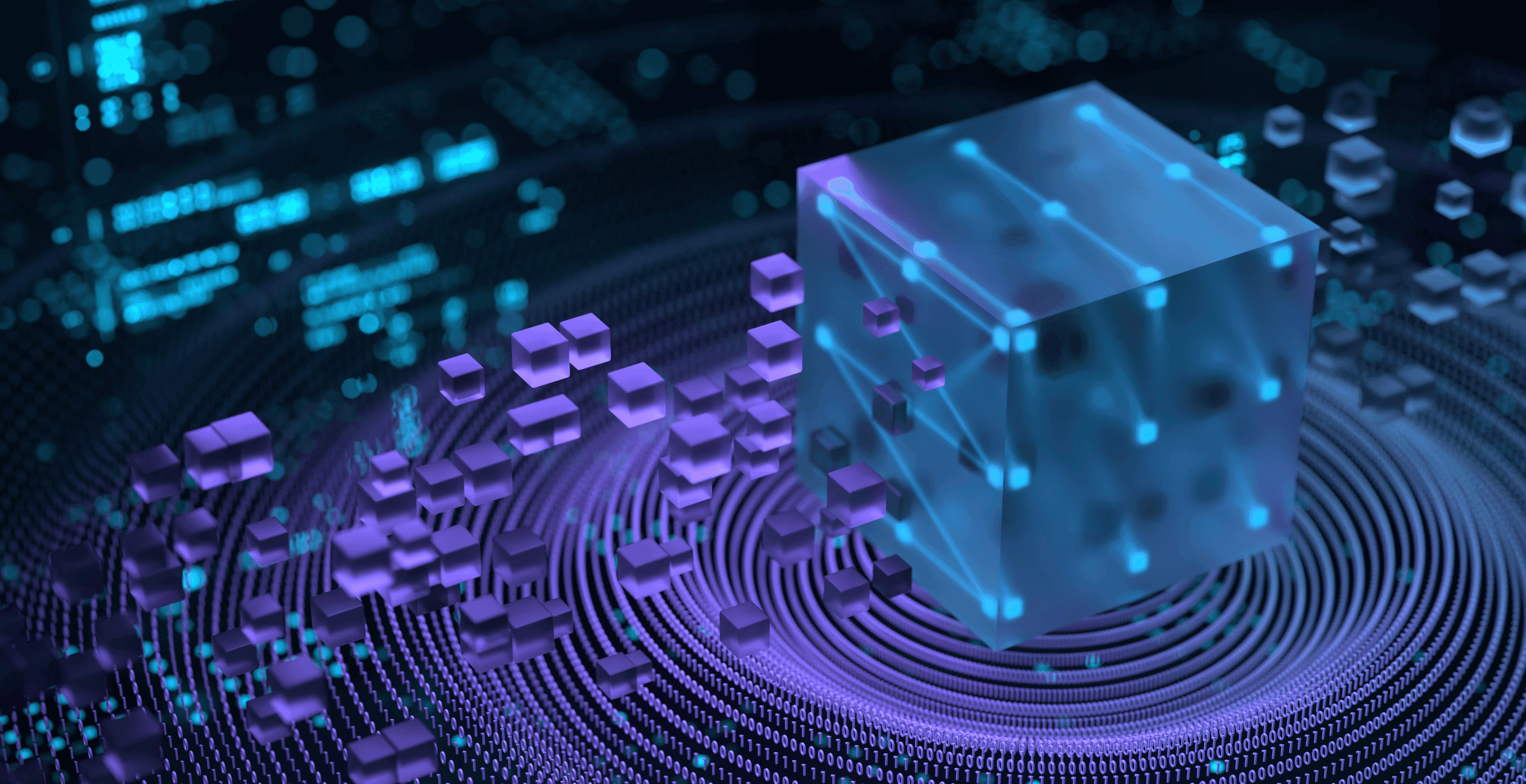
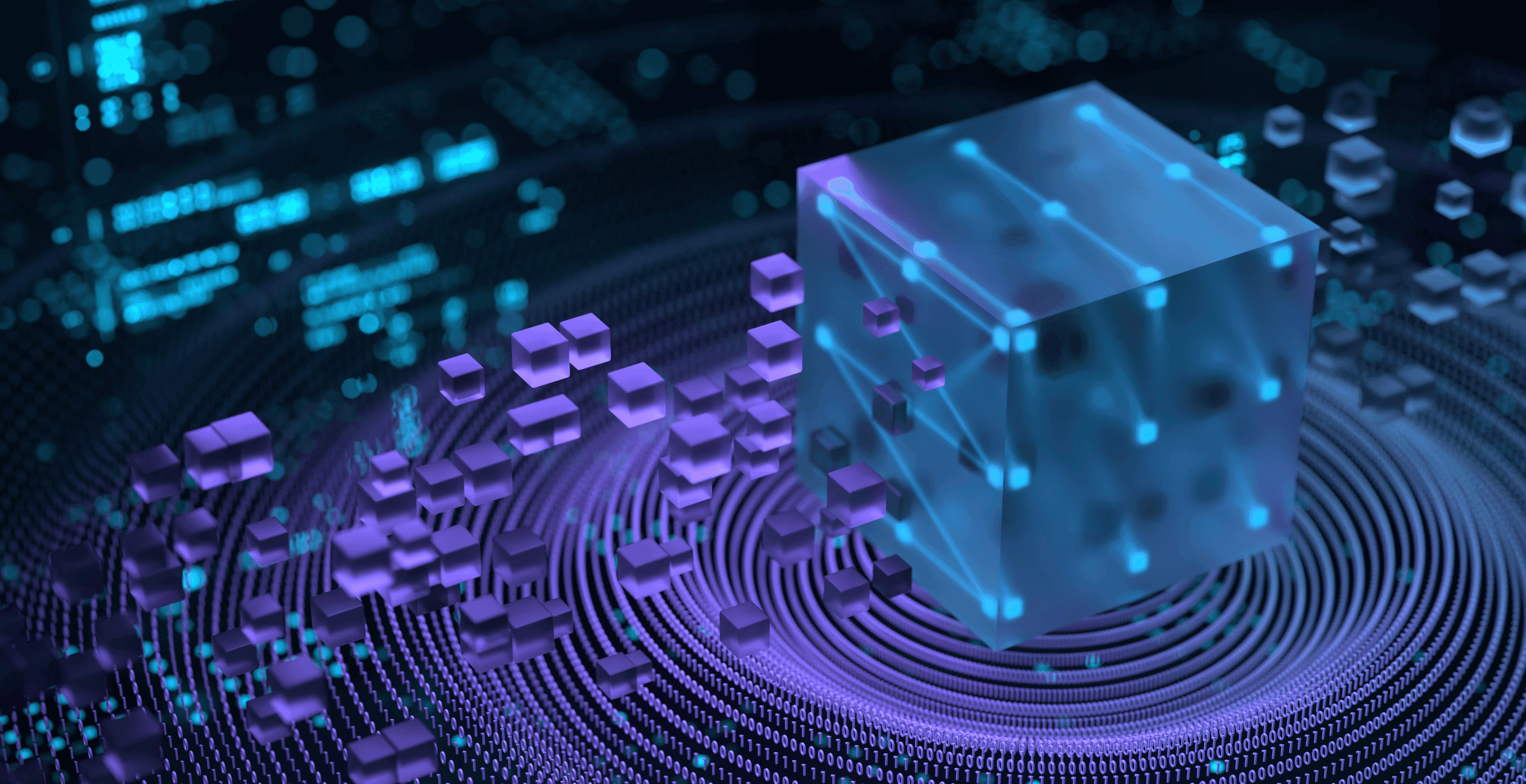
Importance of Accuracy and Reliability in Tabular Data Models
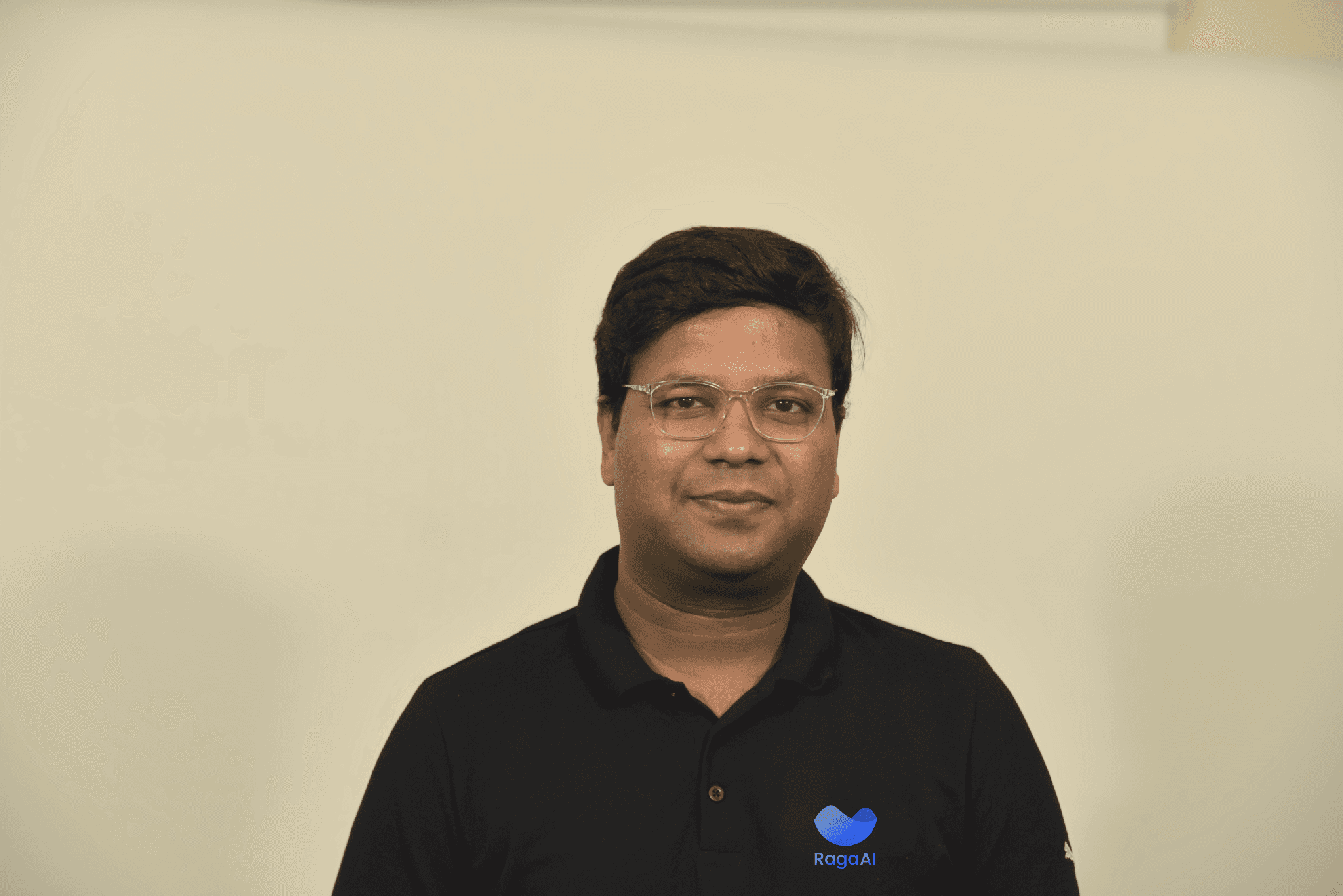
Jigar Gupta
Jul 1, 2024
Read the article
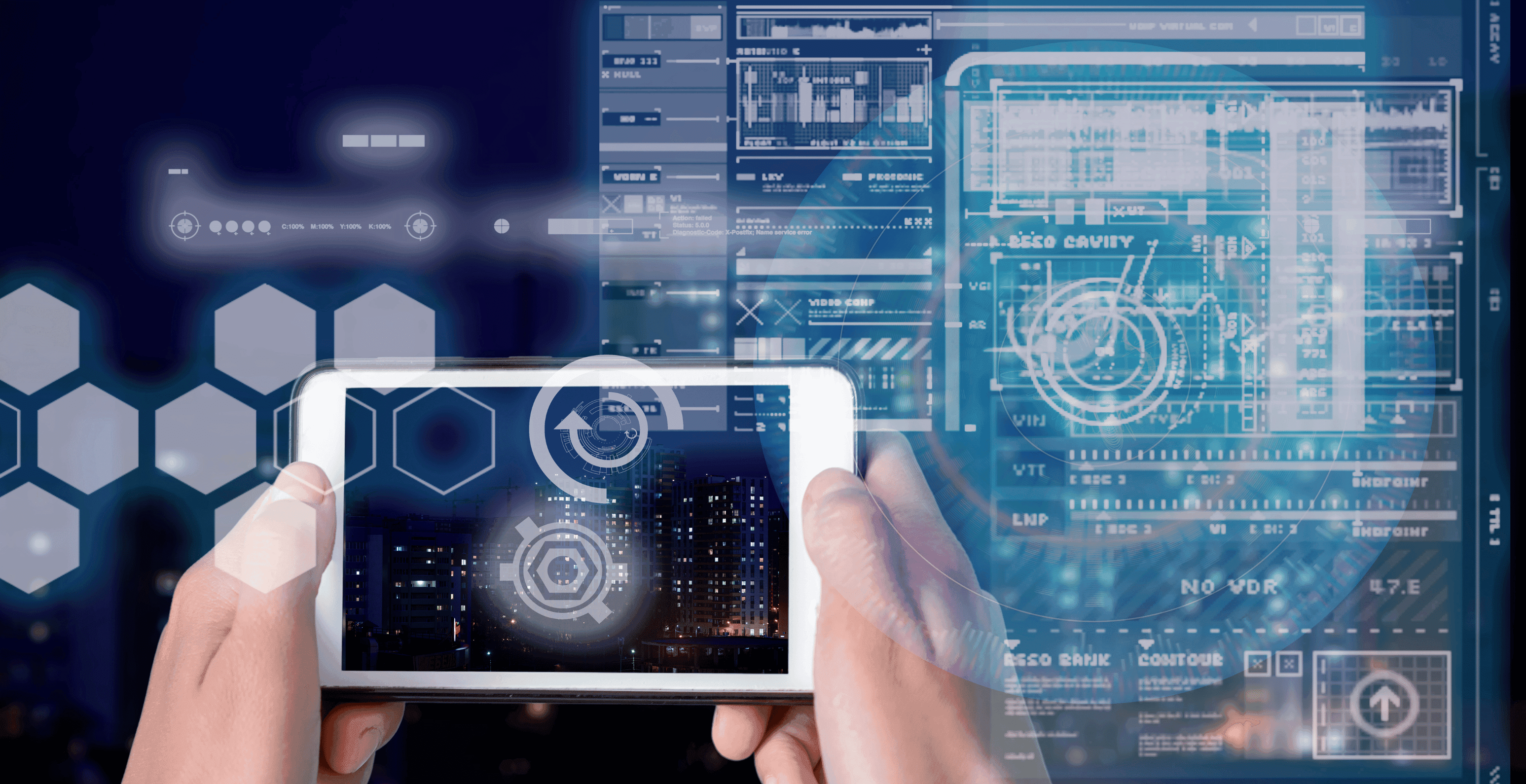
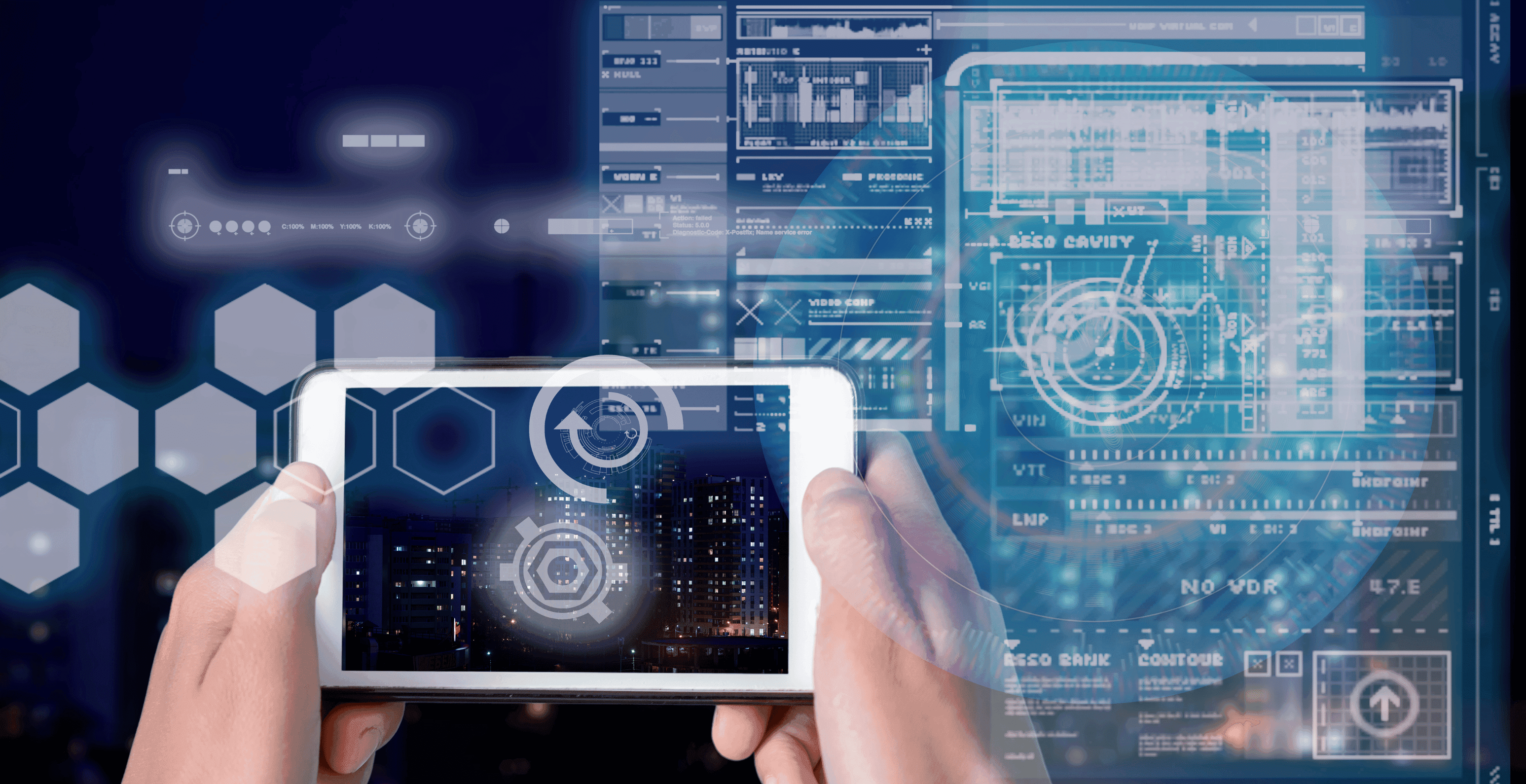
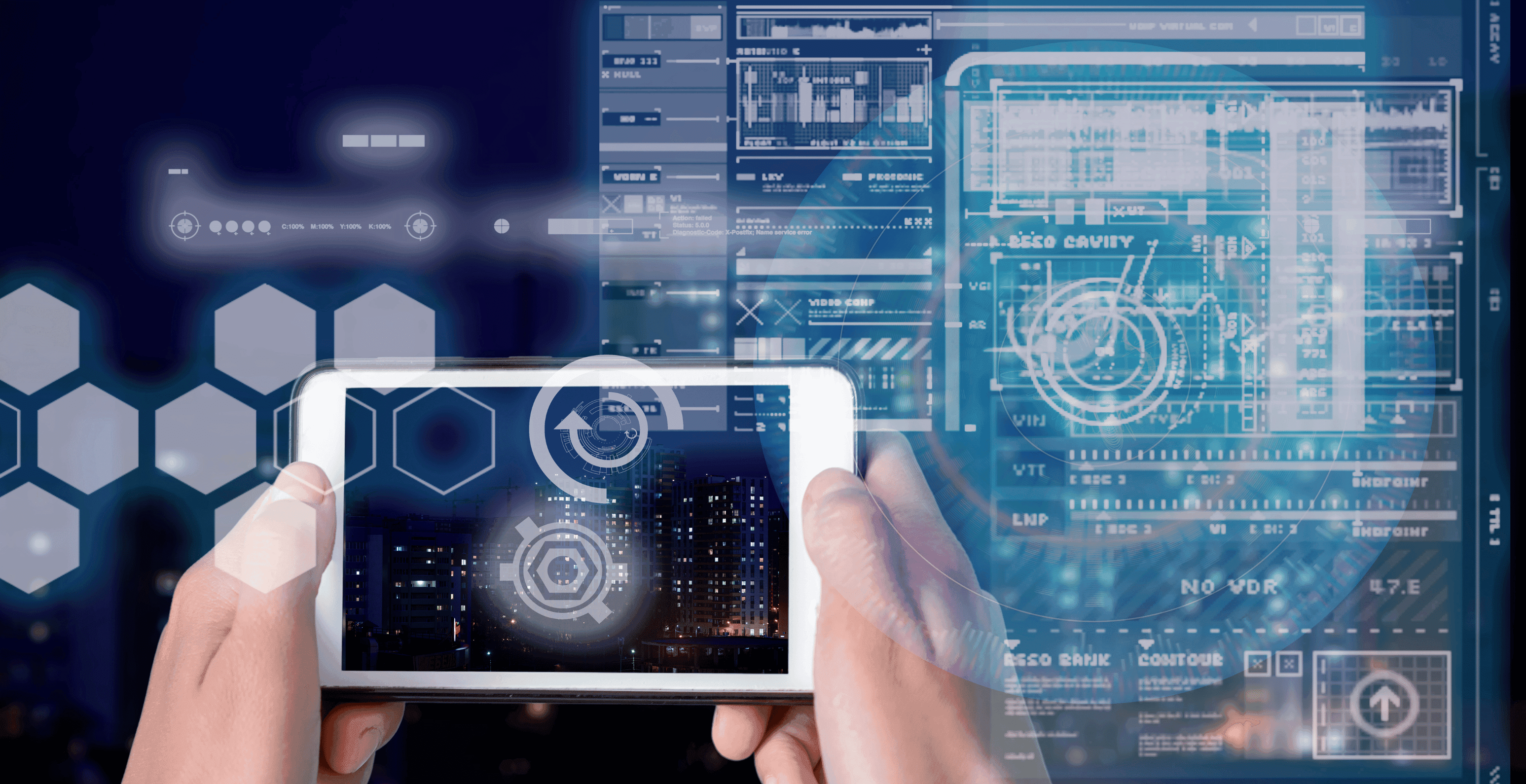
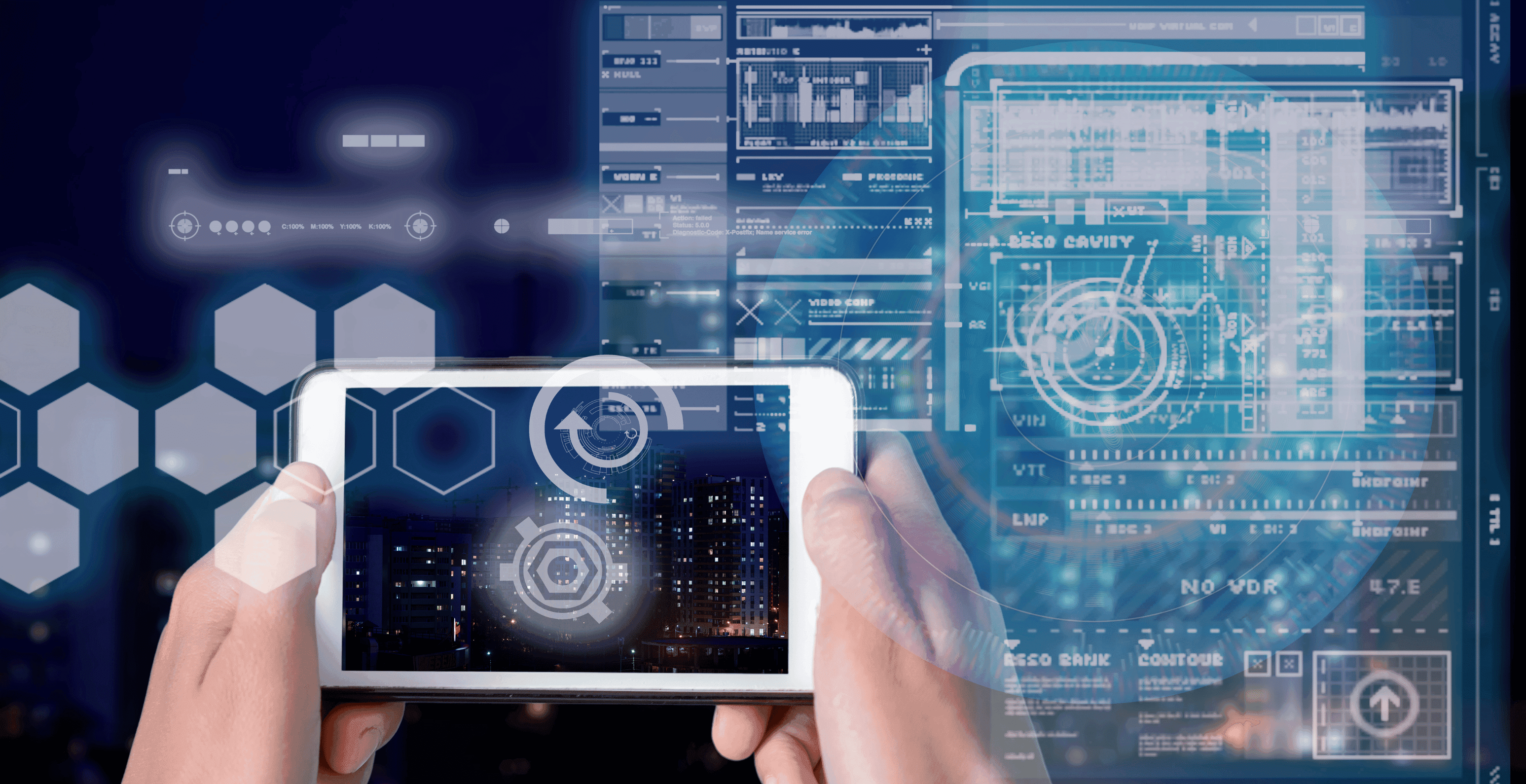
Information Retrieval And LLMs: RAG Explained

Rehan Asif
Jul 1, 2024
Read the article




Introduction to LLM Powered Autonomous Agents

Rehan Asif
Jul 1, 2024
Read the article
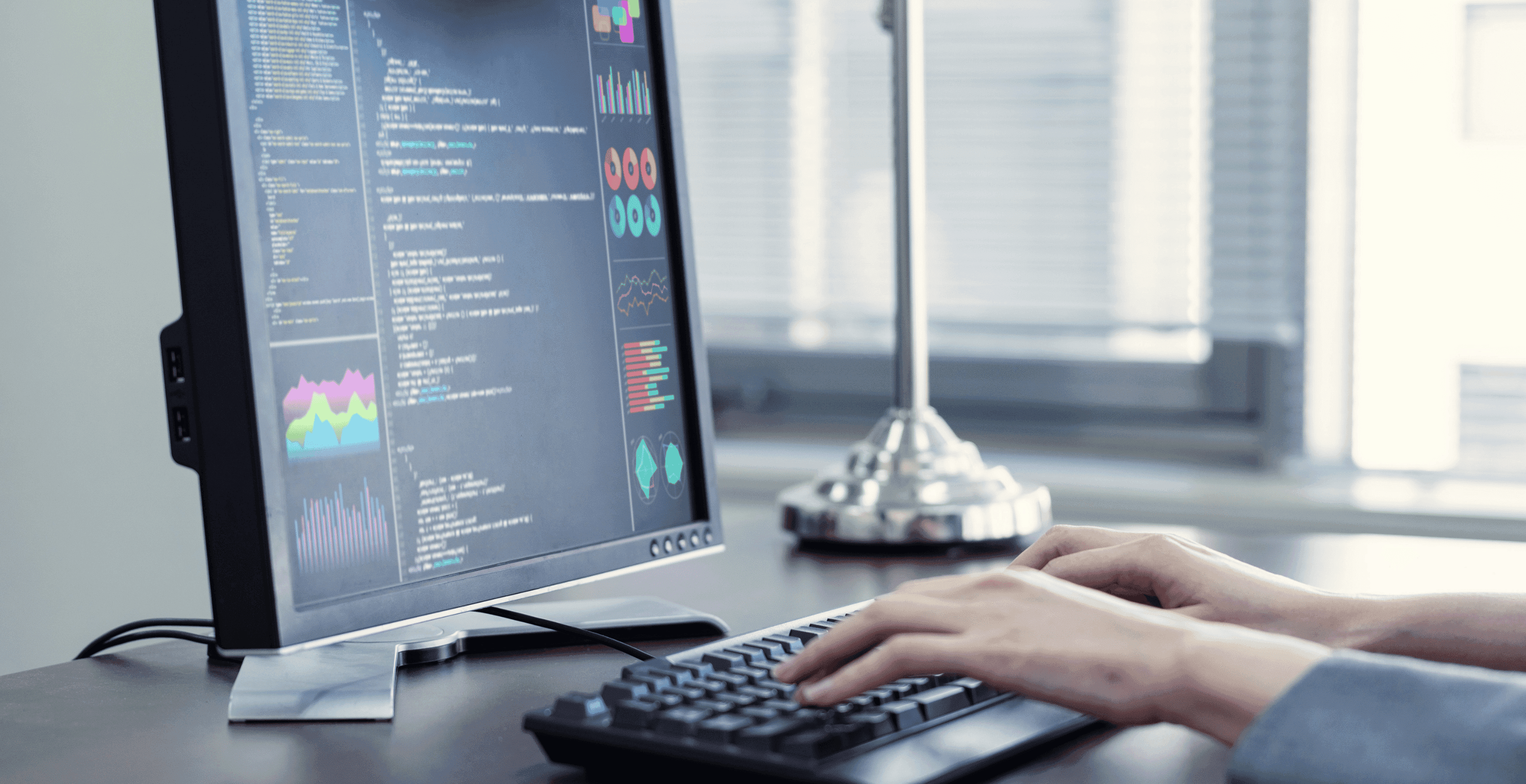
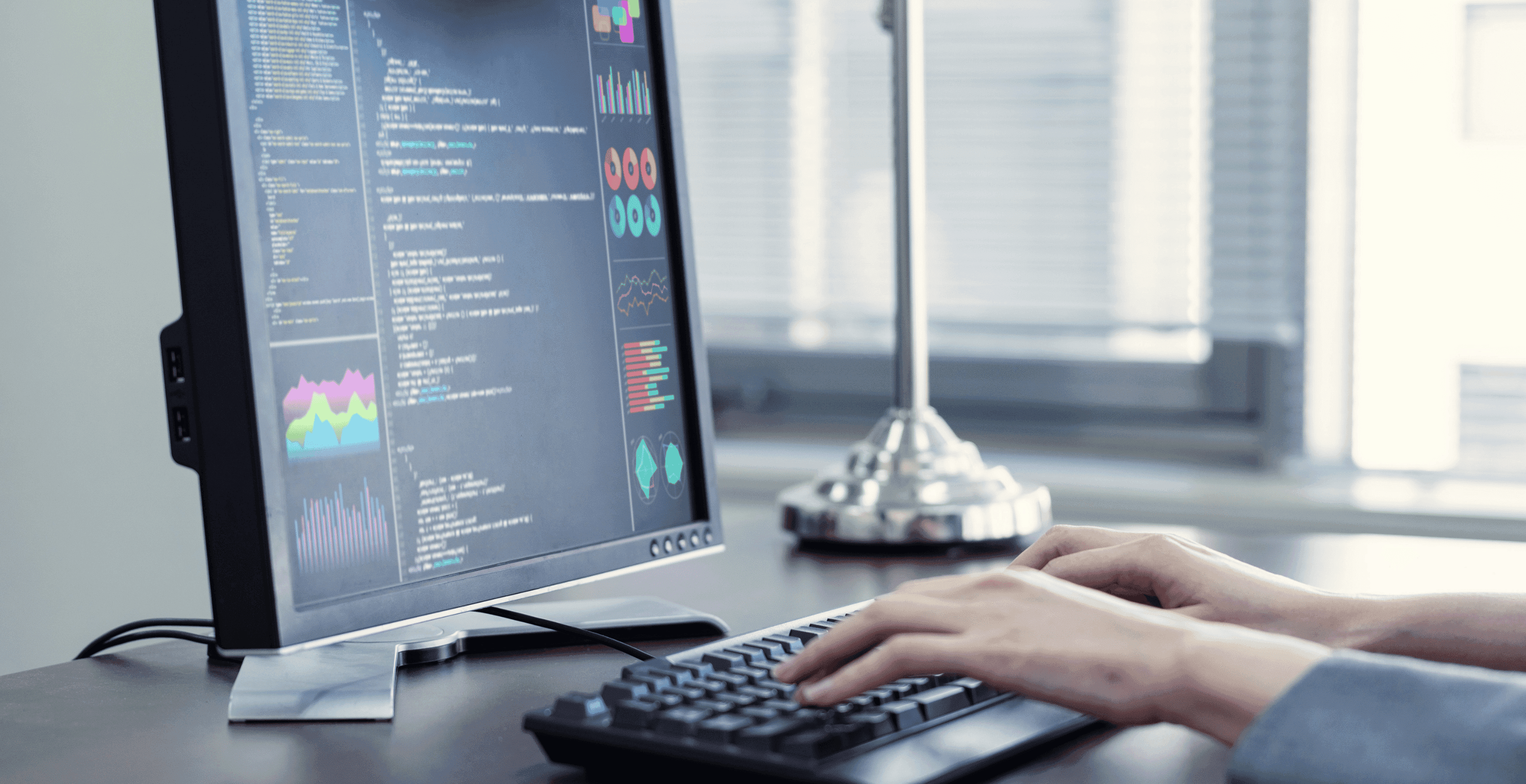
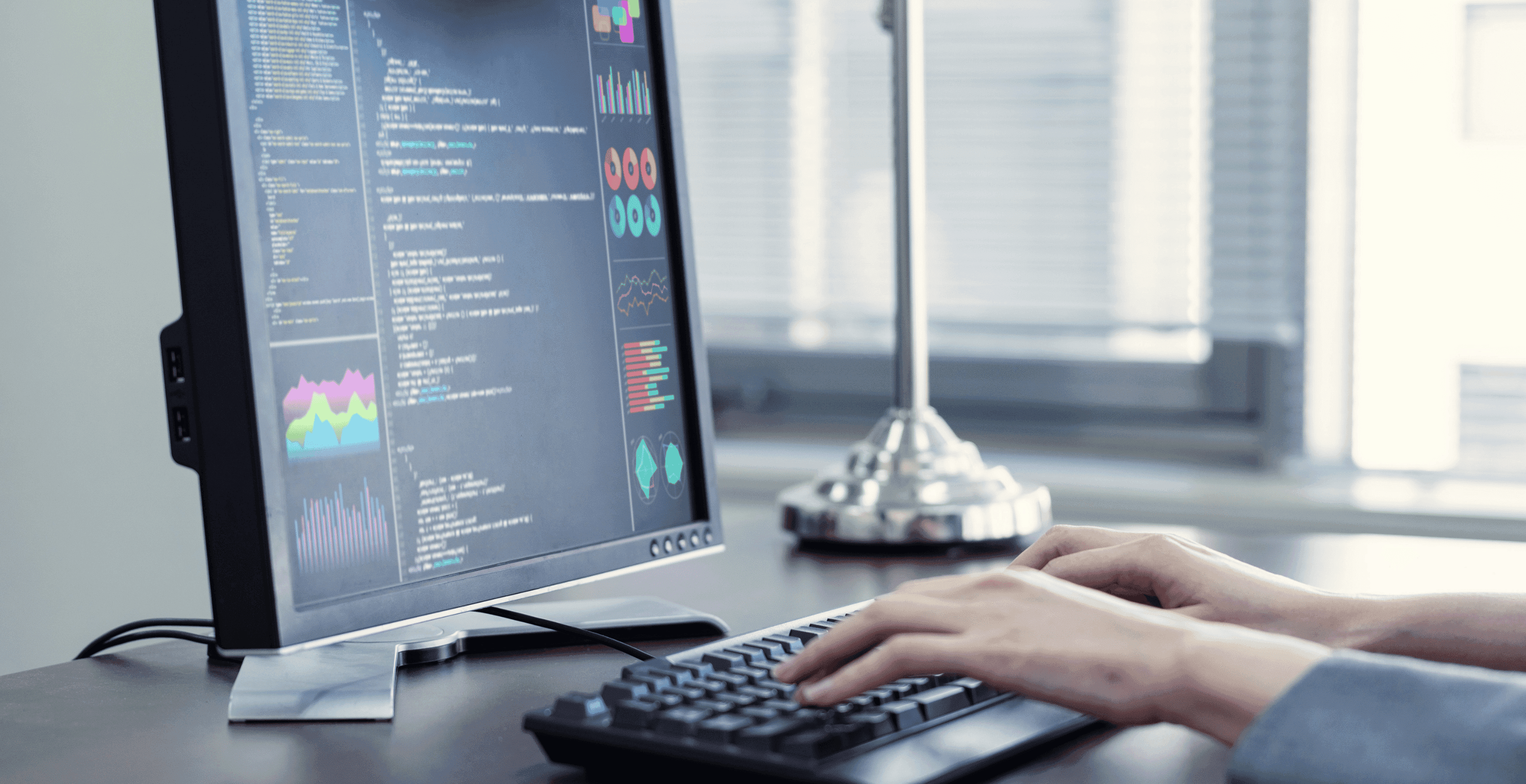
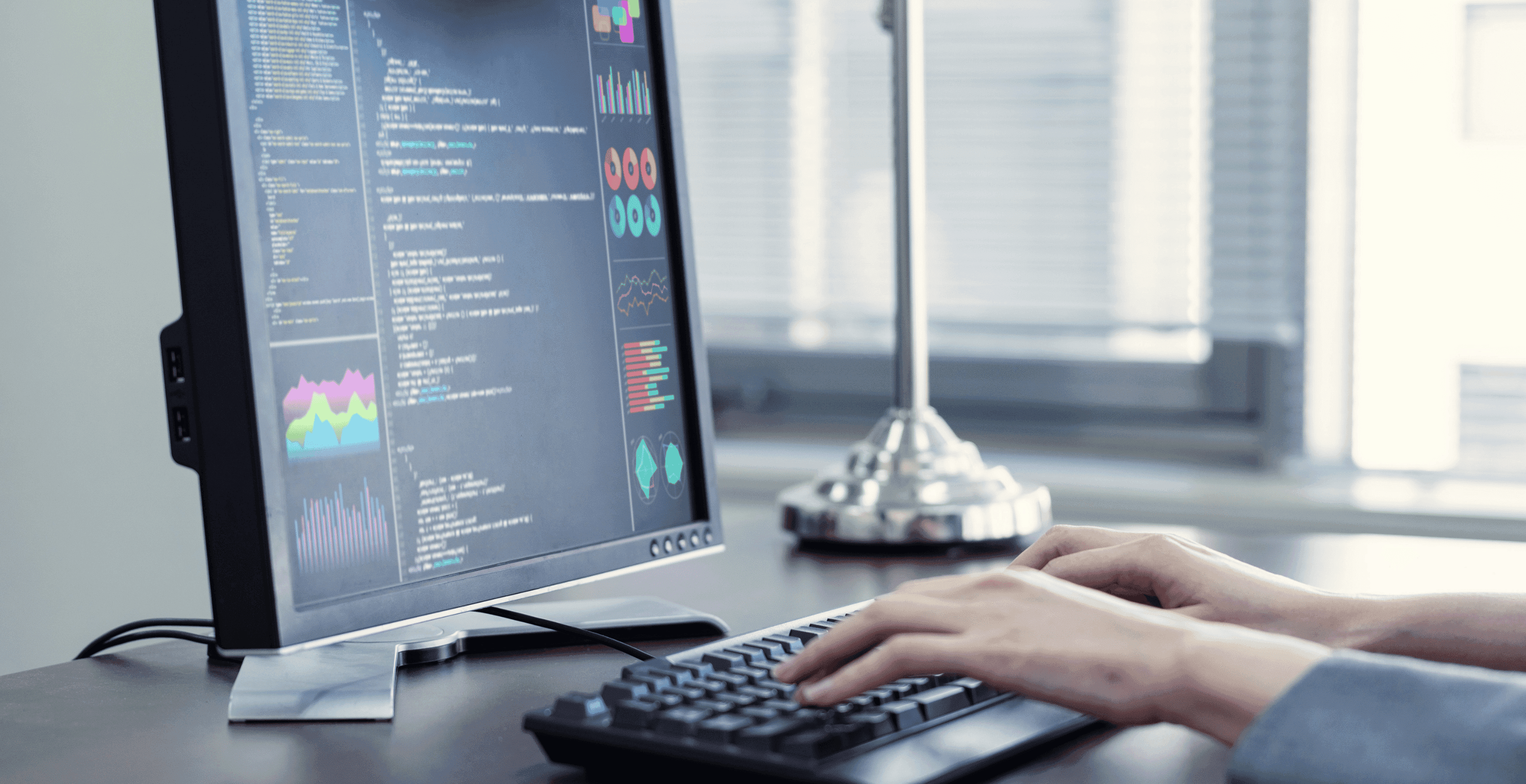
Guide on Unified Multi-Dimensional LLM Evaluation and Benchmark Metrics

Rehan Asif
Jul 1, 2024
Read the article




Innovations In AI For Healthcare
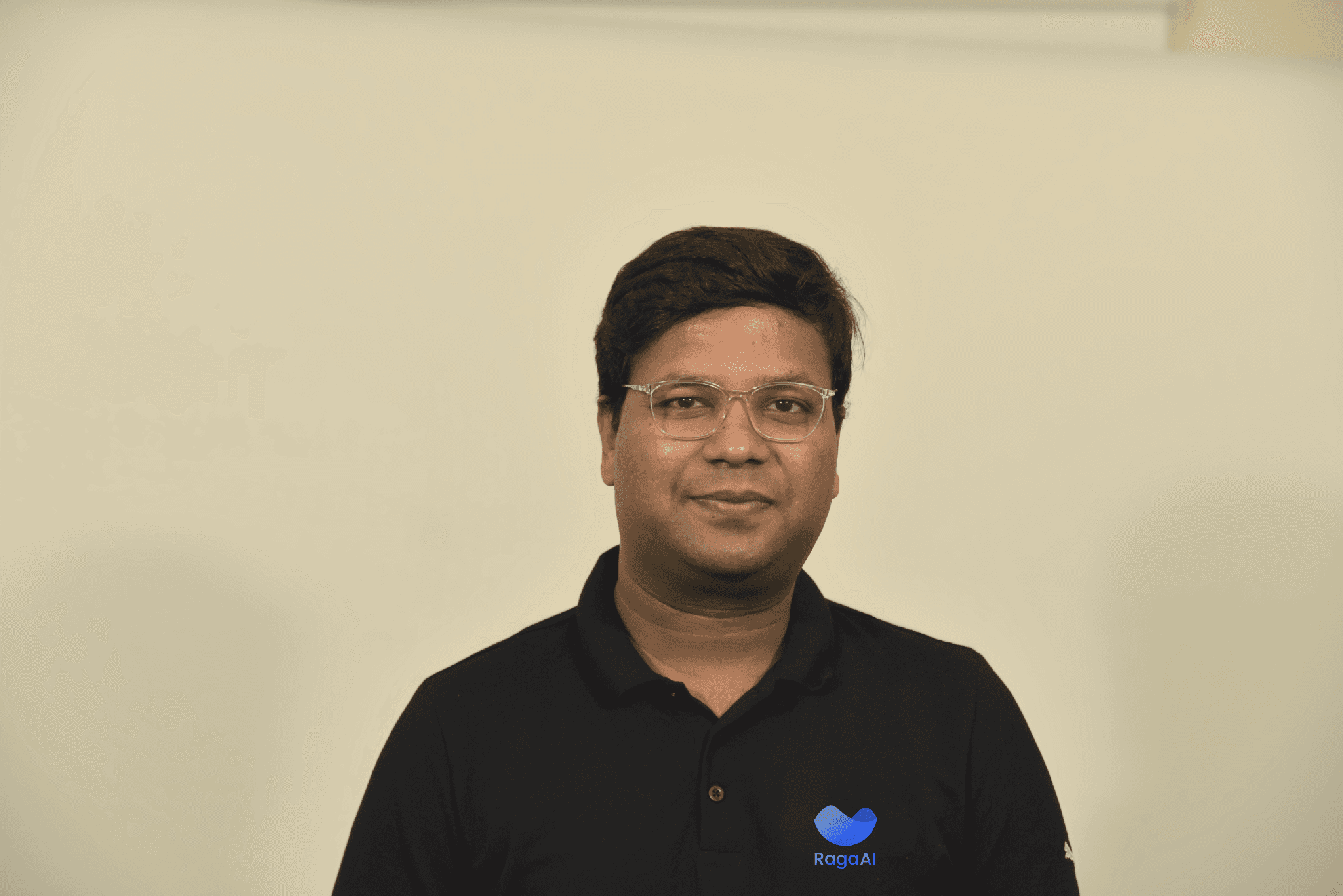
Jigar Gupta
Jun 24, 2024
Read the article




Implementing AI-Driven Inventory Management For The Retail Industry
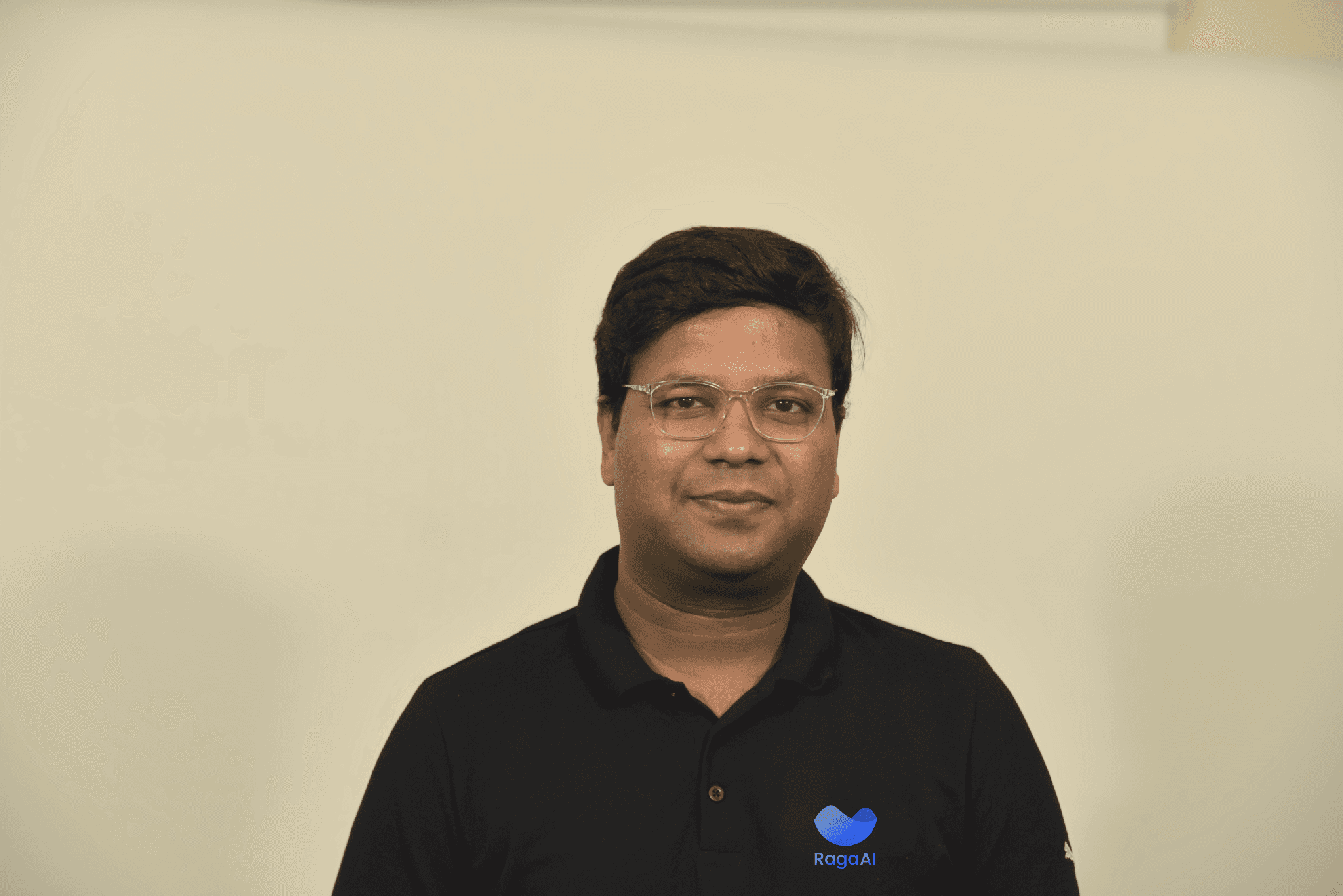
Jigar Gupta
Jun 24, 2024
Read the article




Practical Retrieval Augmented Generation: Use Cases And Impact
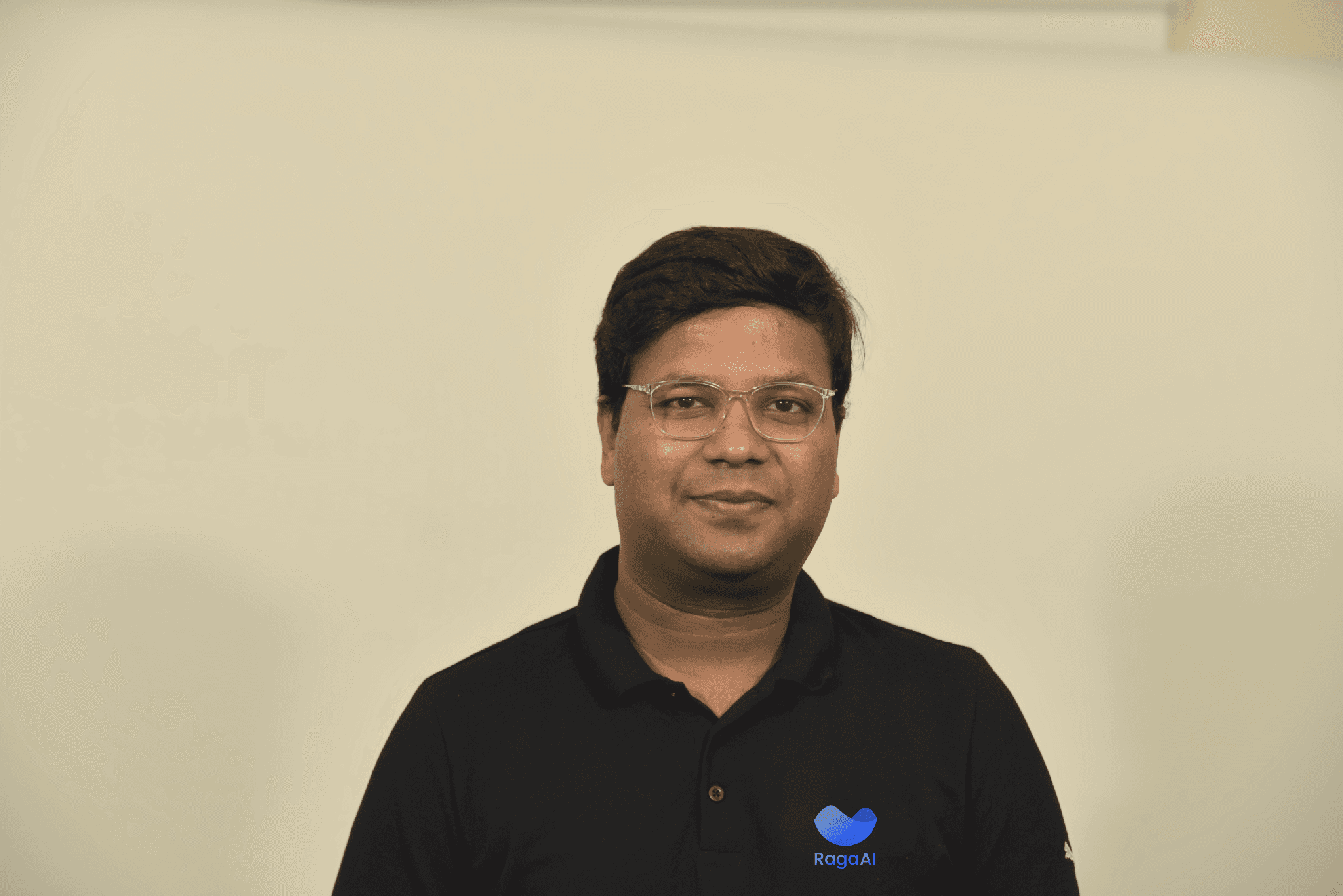
Jigar Gupta
Jun 24, 2024
Read the article




LLM Pre-Training and Fine-Tuning Differences

Rehan Asif
Jun 23, 2024
Read the article




20 LLM Project Ideas For Beginners Using Large Language Models

Rehan Asif
Jun 23, 2024
Read the article




Understanding LLM Parameters: Tuning Top-P, Temperature And Tokens

Rehan Asif
Jun 23, 2024
Read the article




Understanding Large Action Models In AI

Rehan Asif
Jun 23, 2024
Read the article




Building And Implementing Custom LLM Guardrails

Rehan Asif
Jun 12, 2024
Read the article




Understanding LLM Alignment: A Simple Guide

Rehan Asif
Jun 12, 2024
Read the article
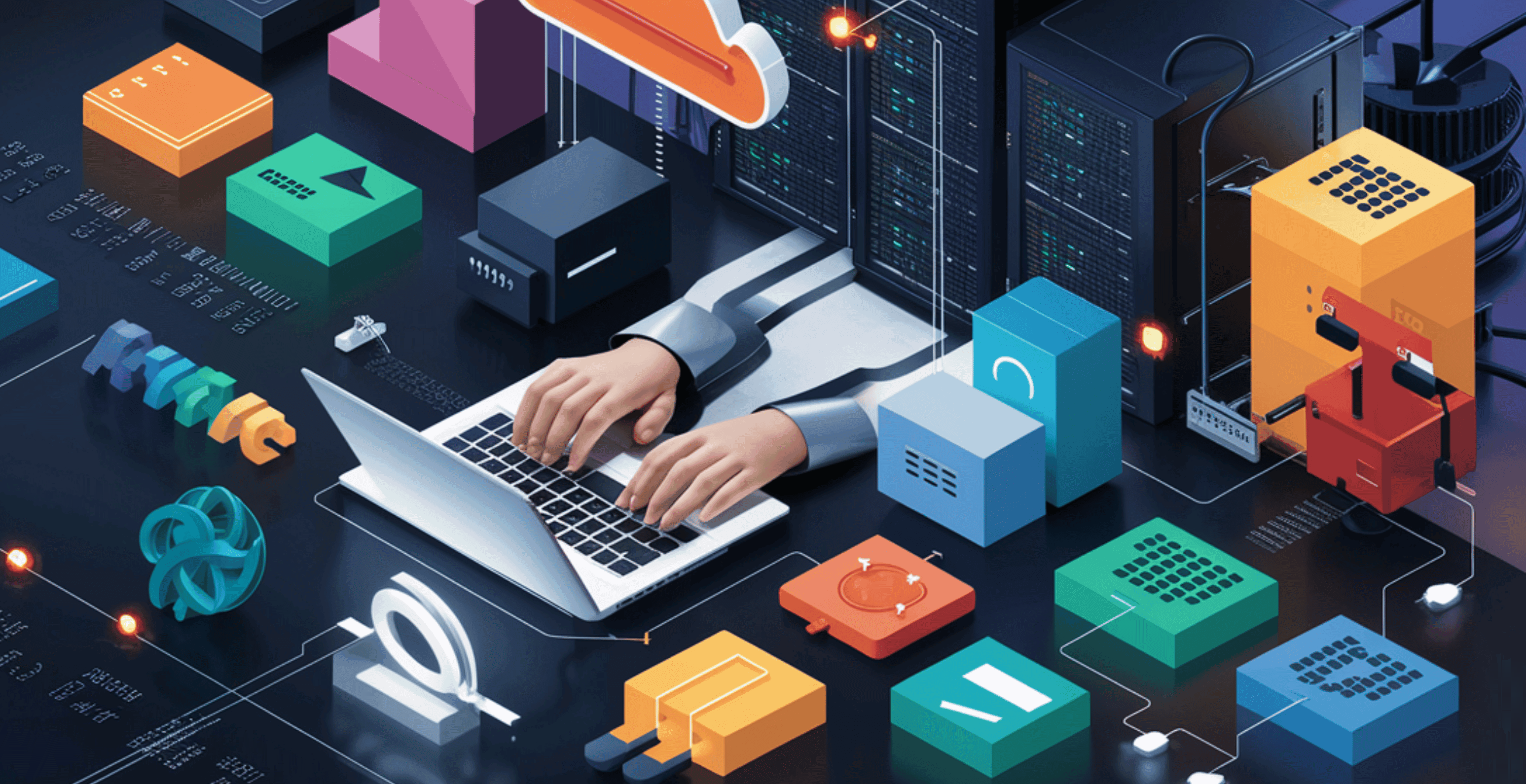
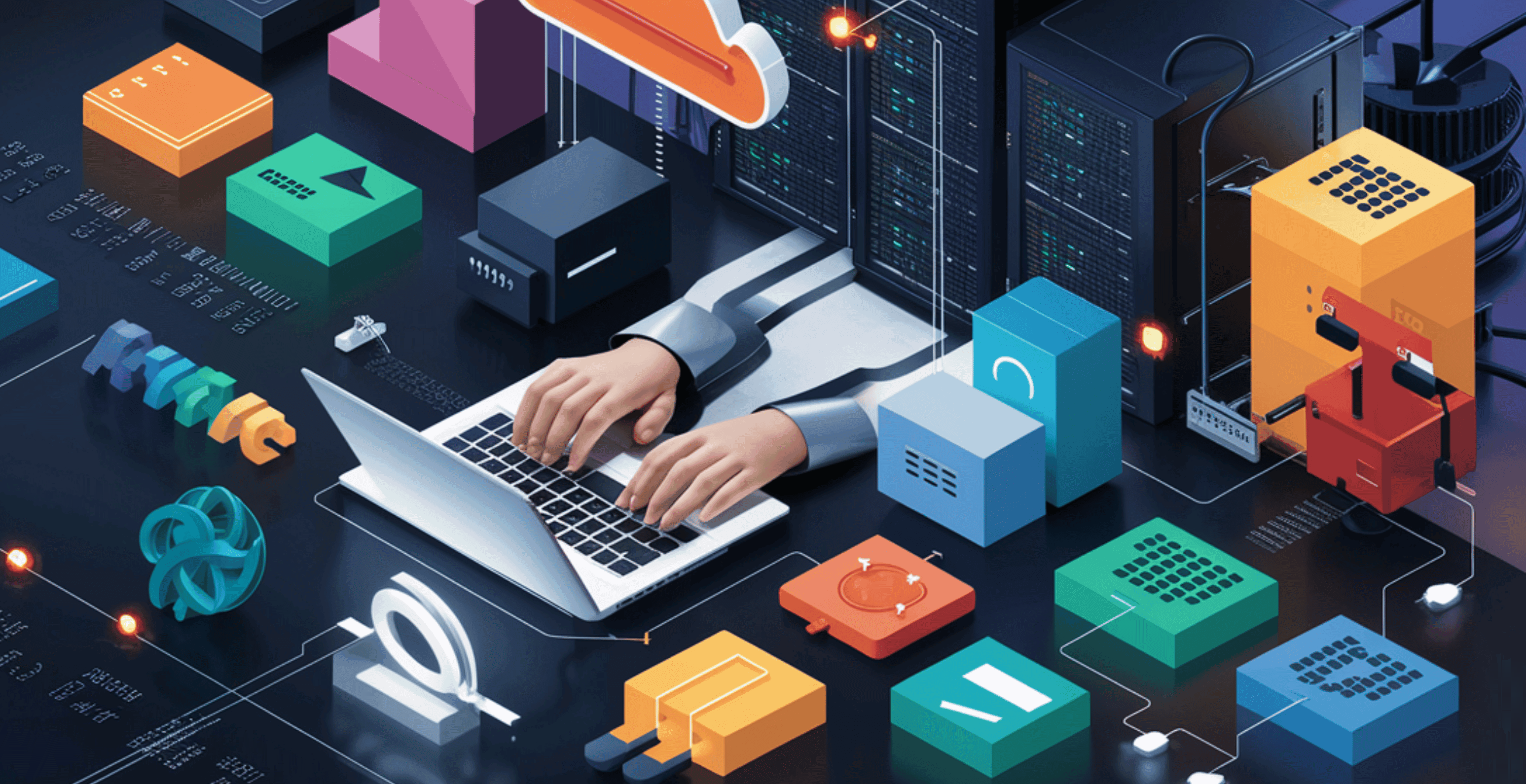
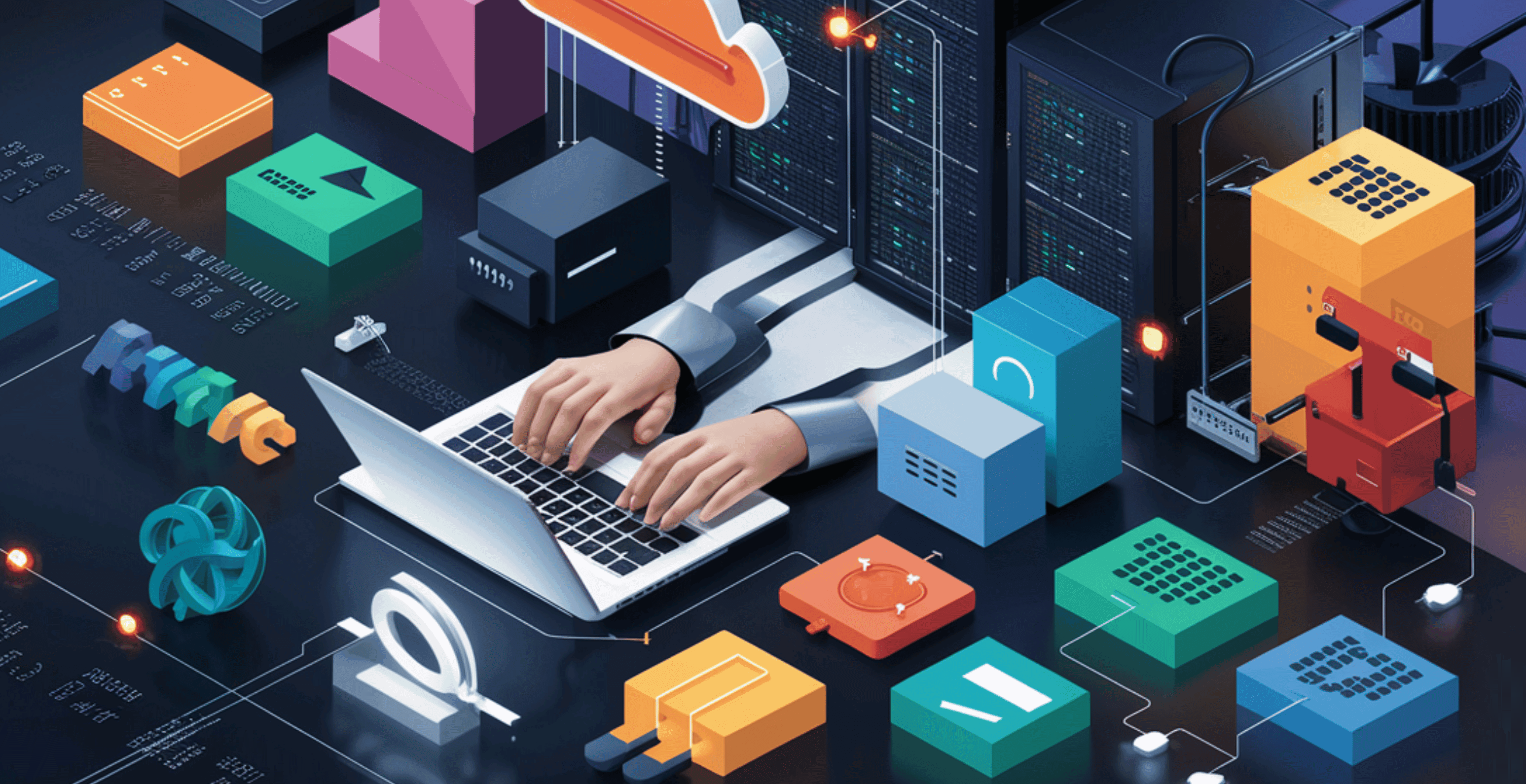
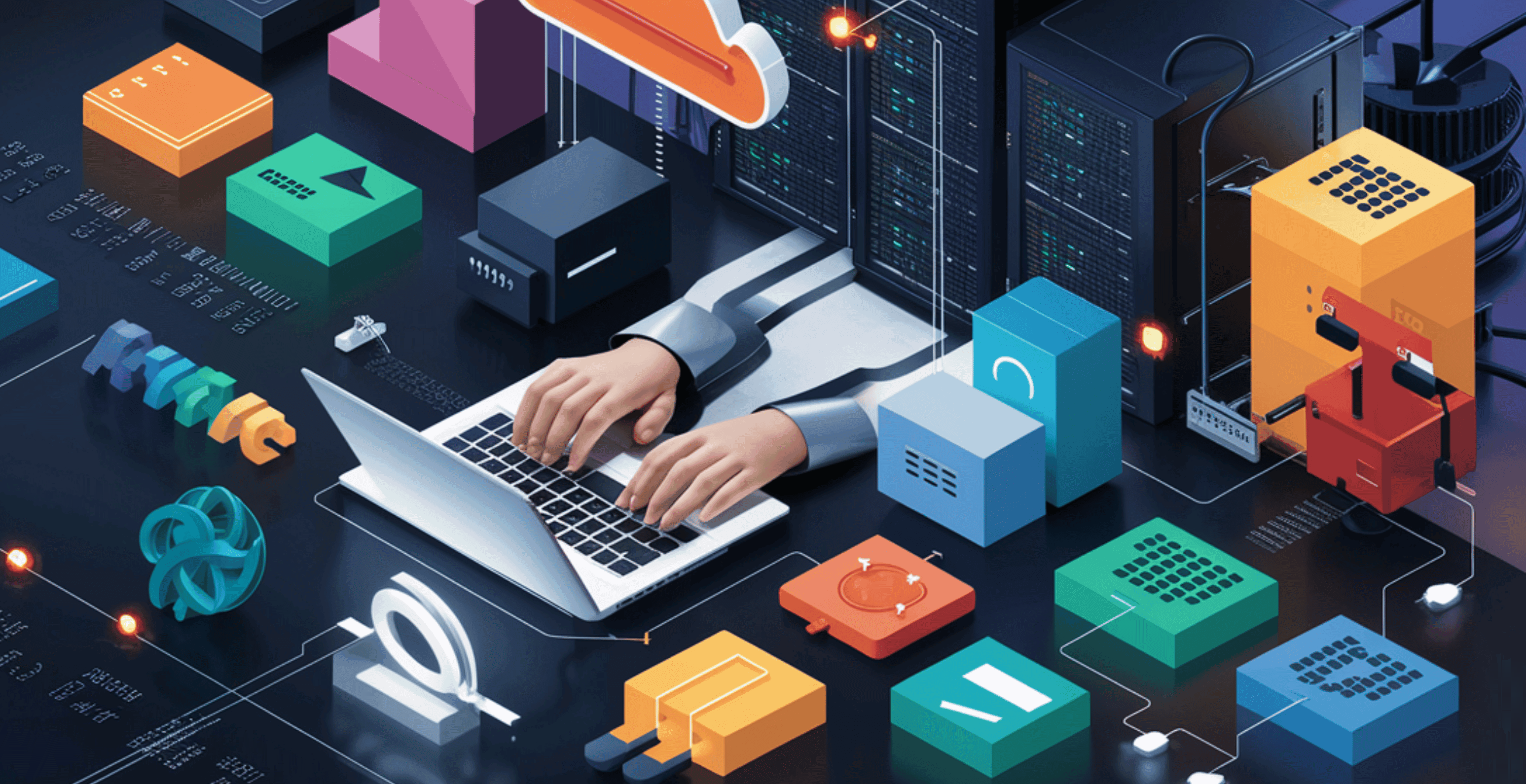
Practical Strategies For Self-Hosting Large Language Models

Rehan Asif
Jun 12, 2024
Read the article
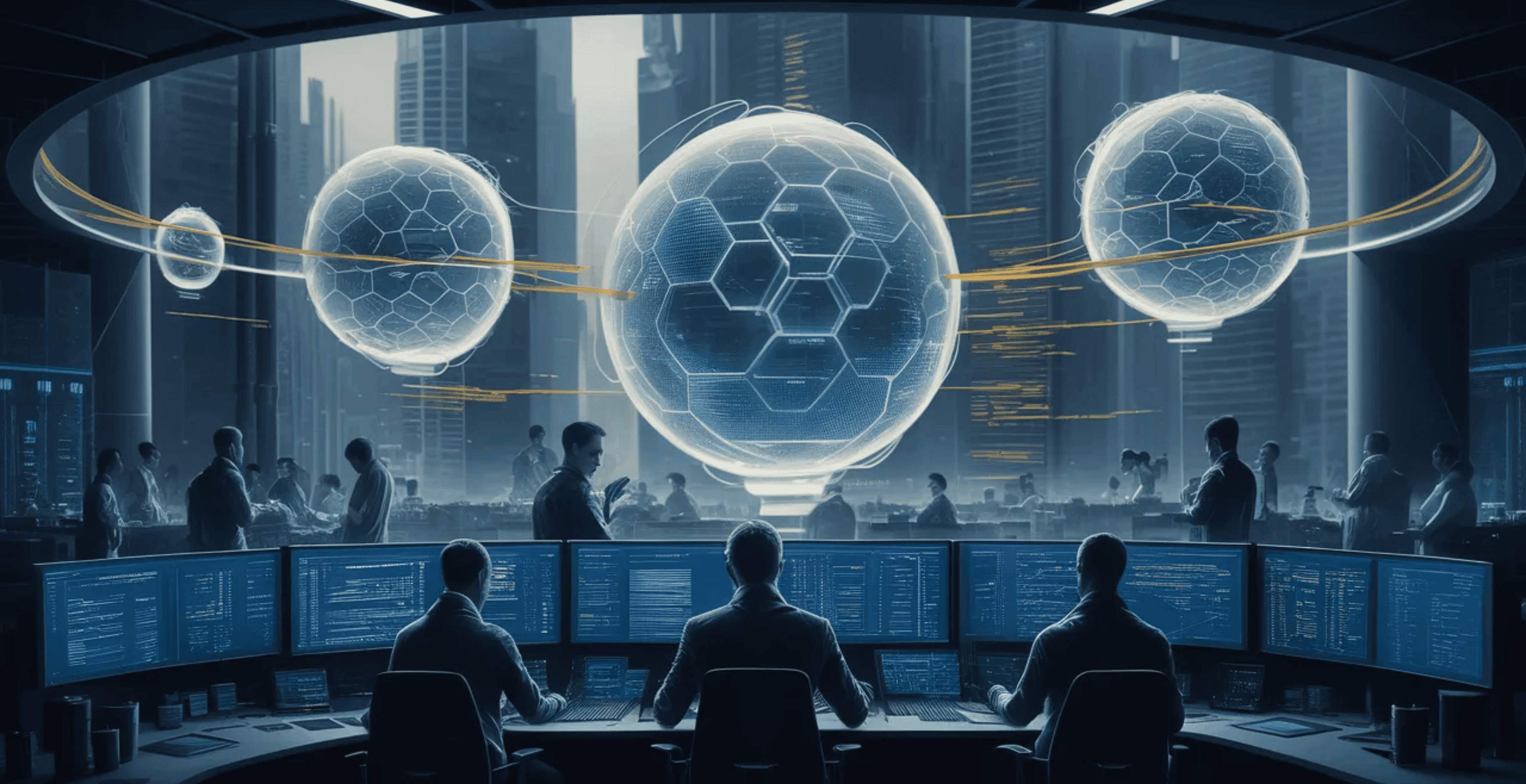
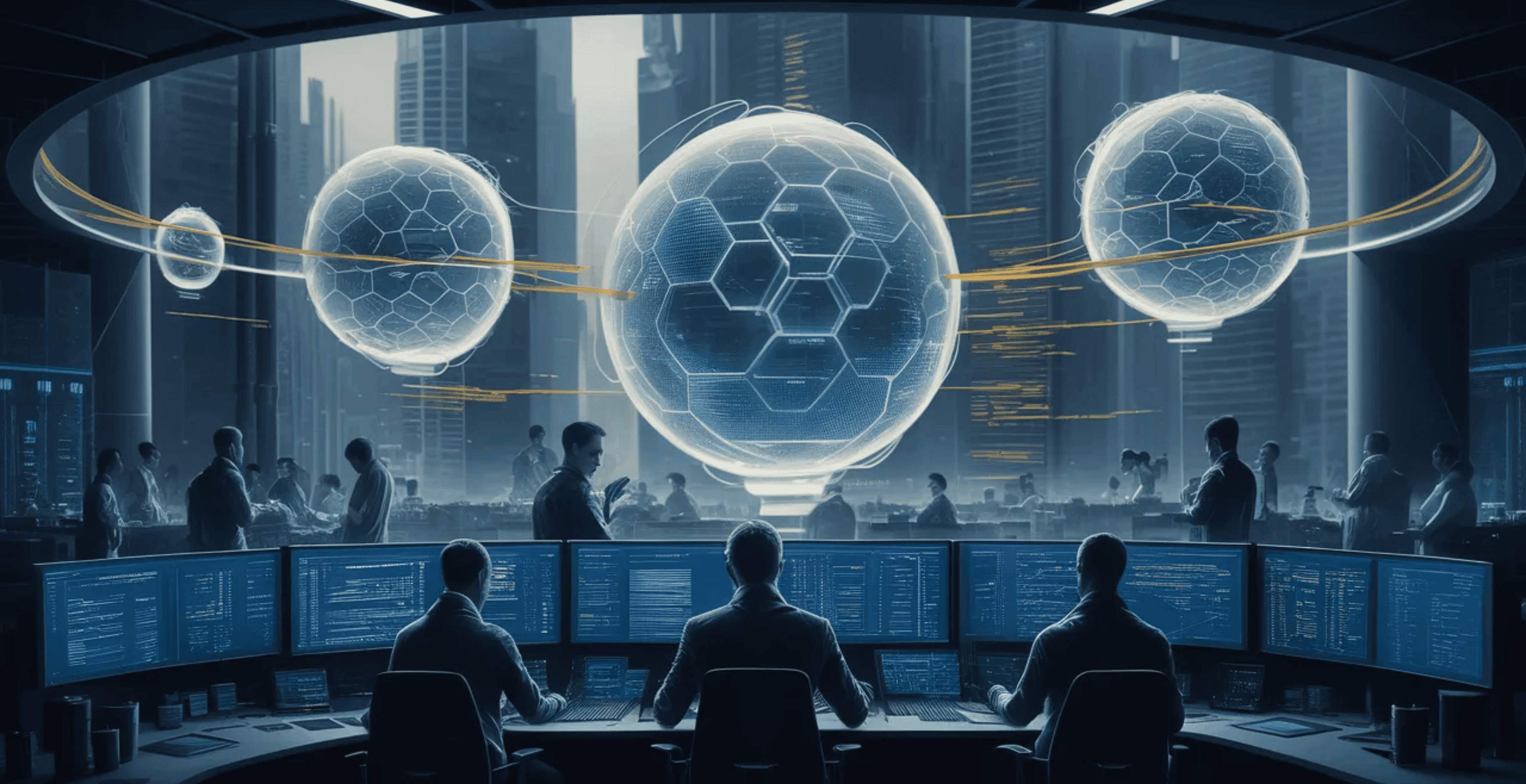
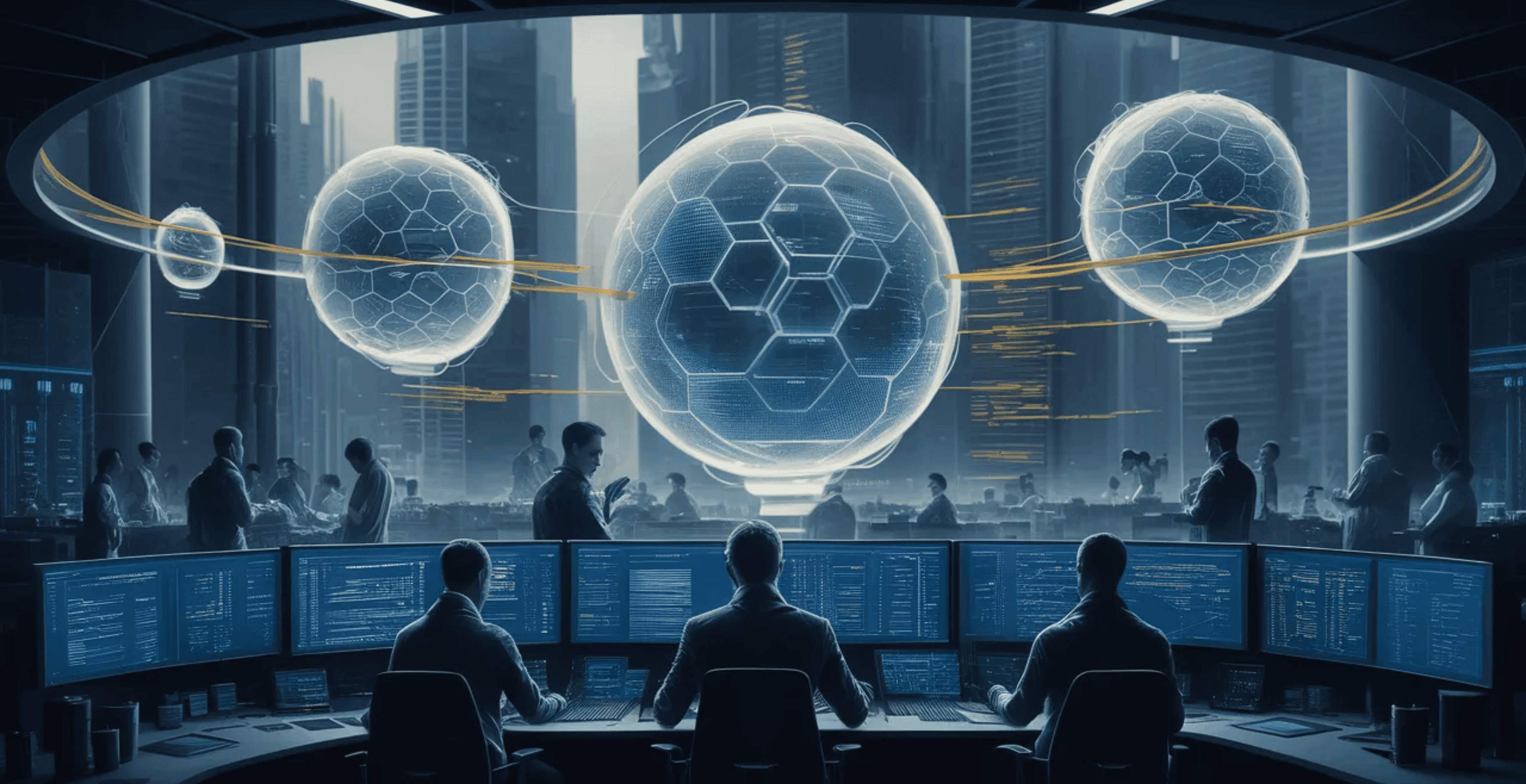
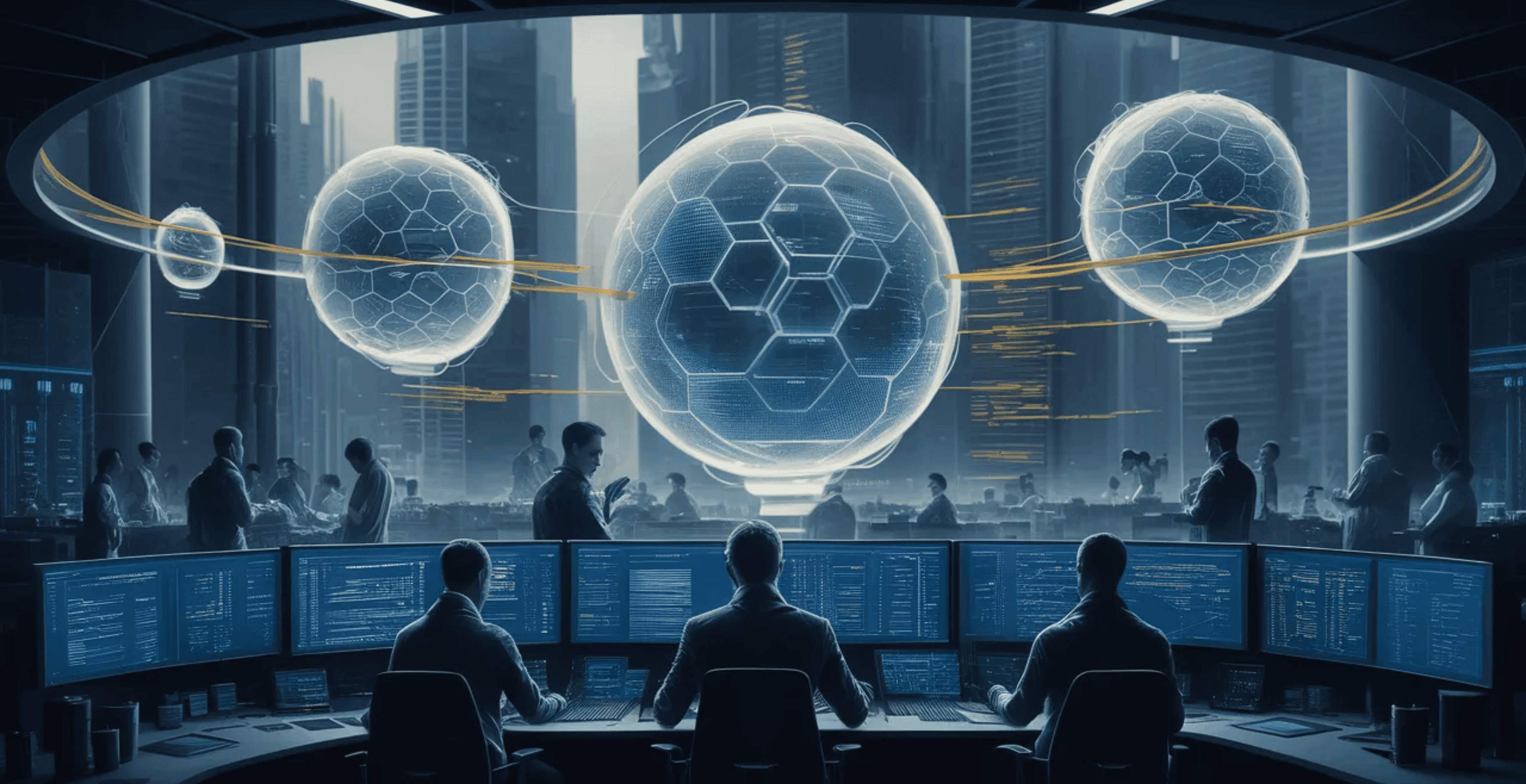
Practical Guide For Deploying LLMs In Production

Rehan Asif
Jun 12, 2024
Read the article
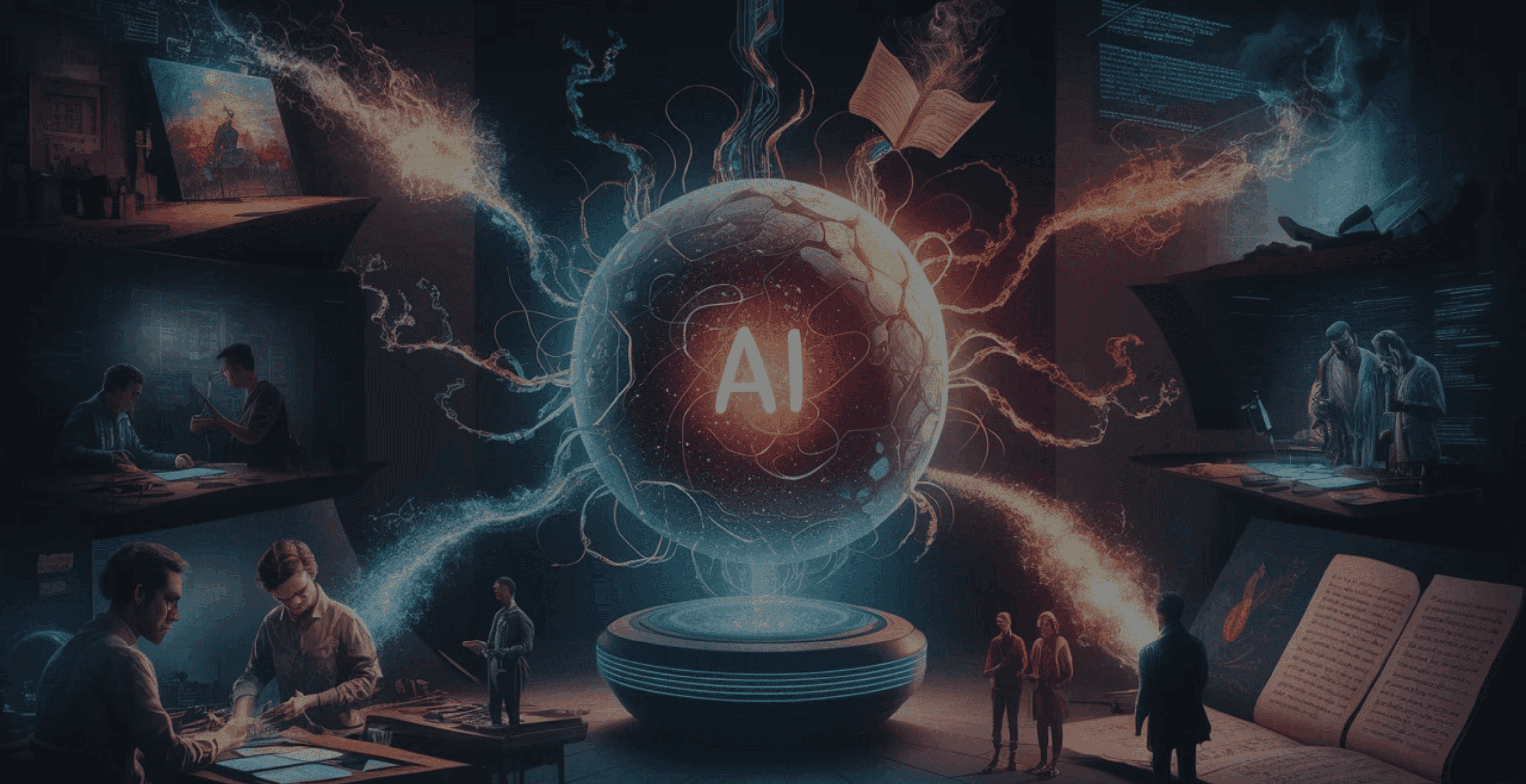
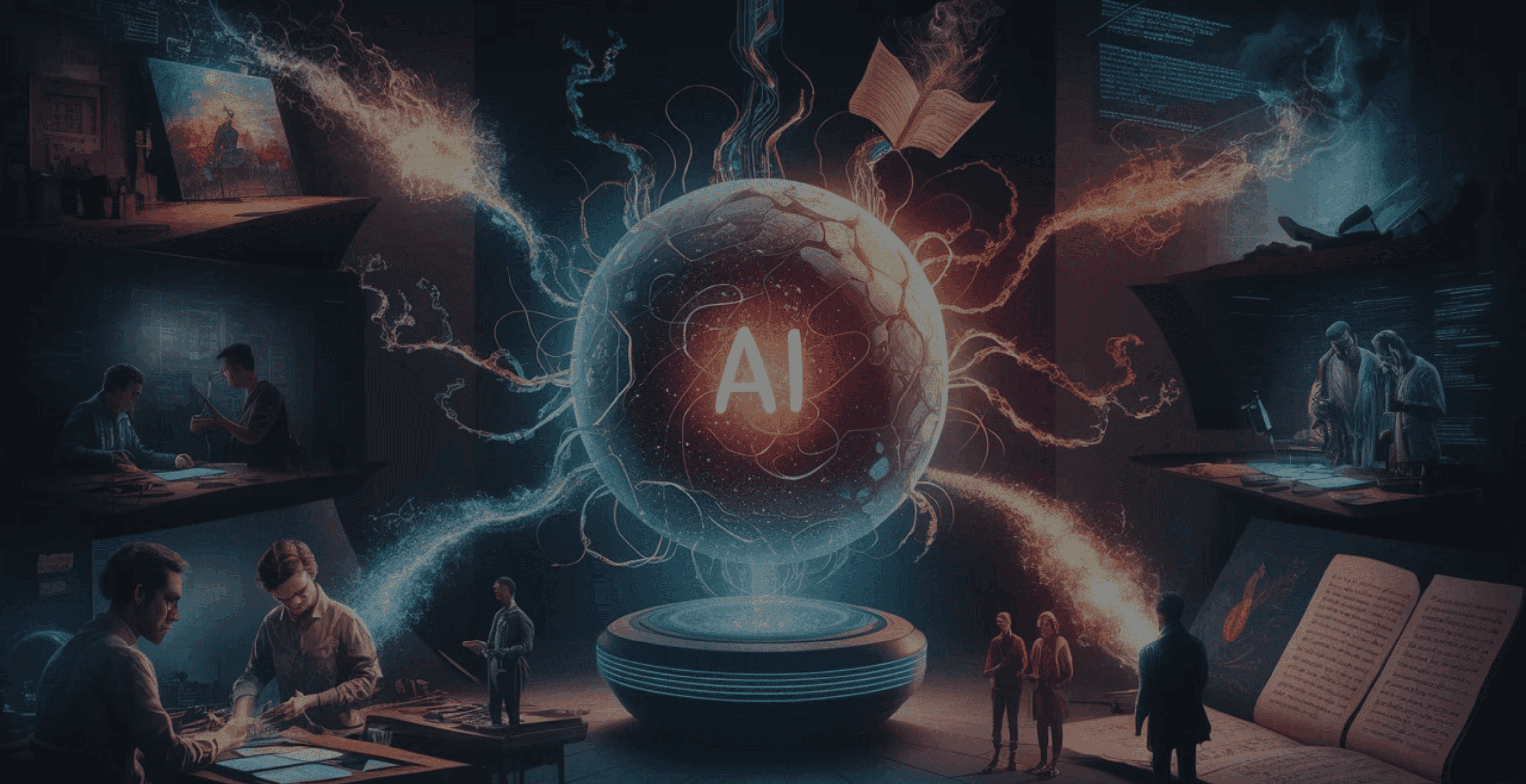
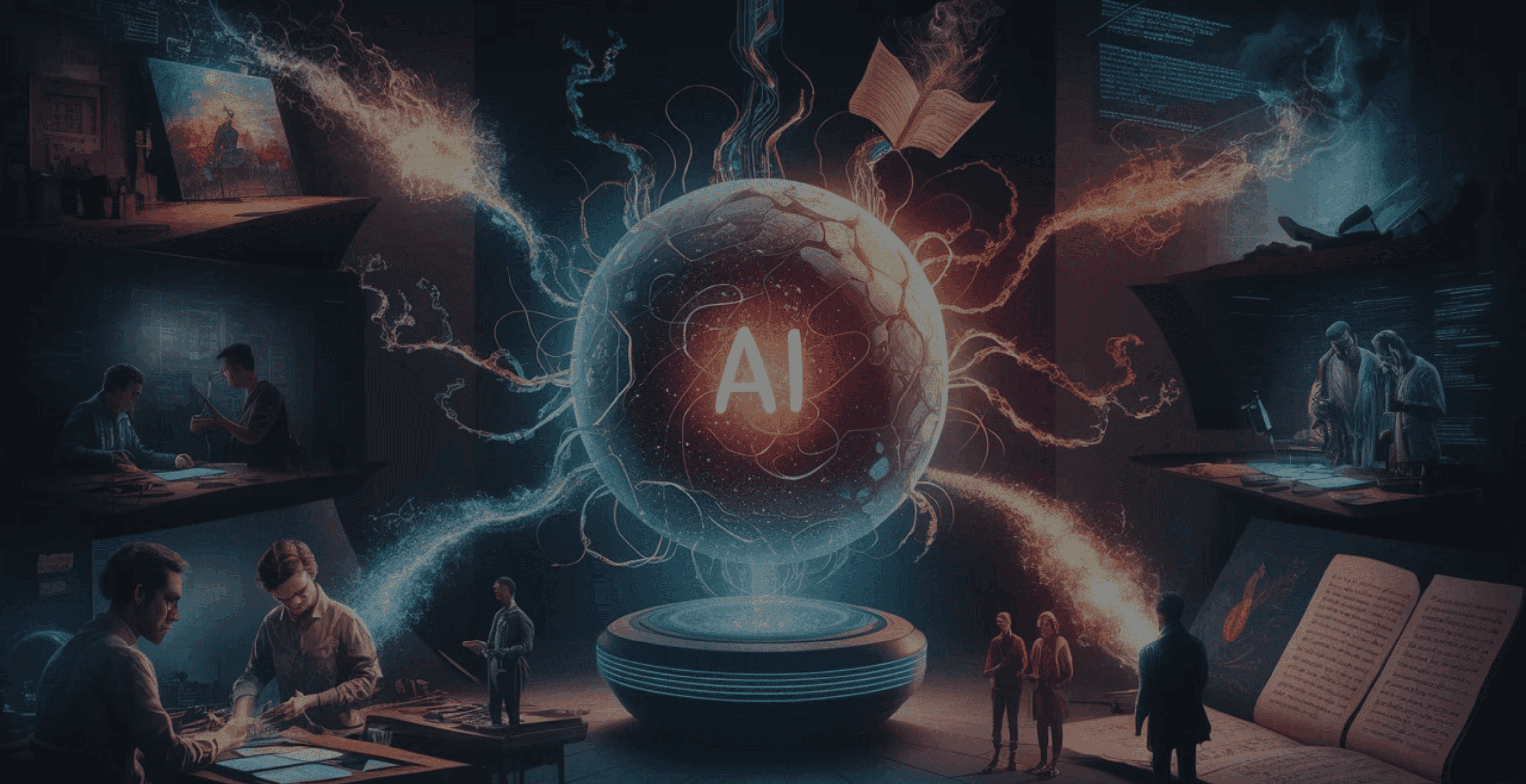
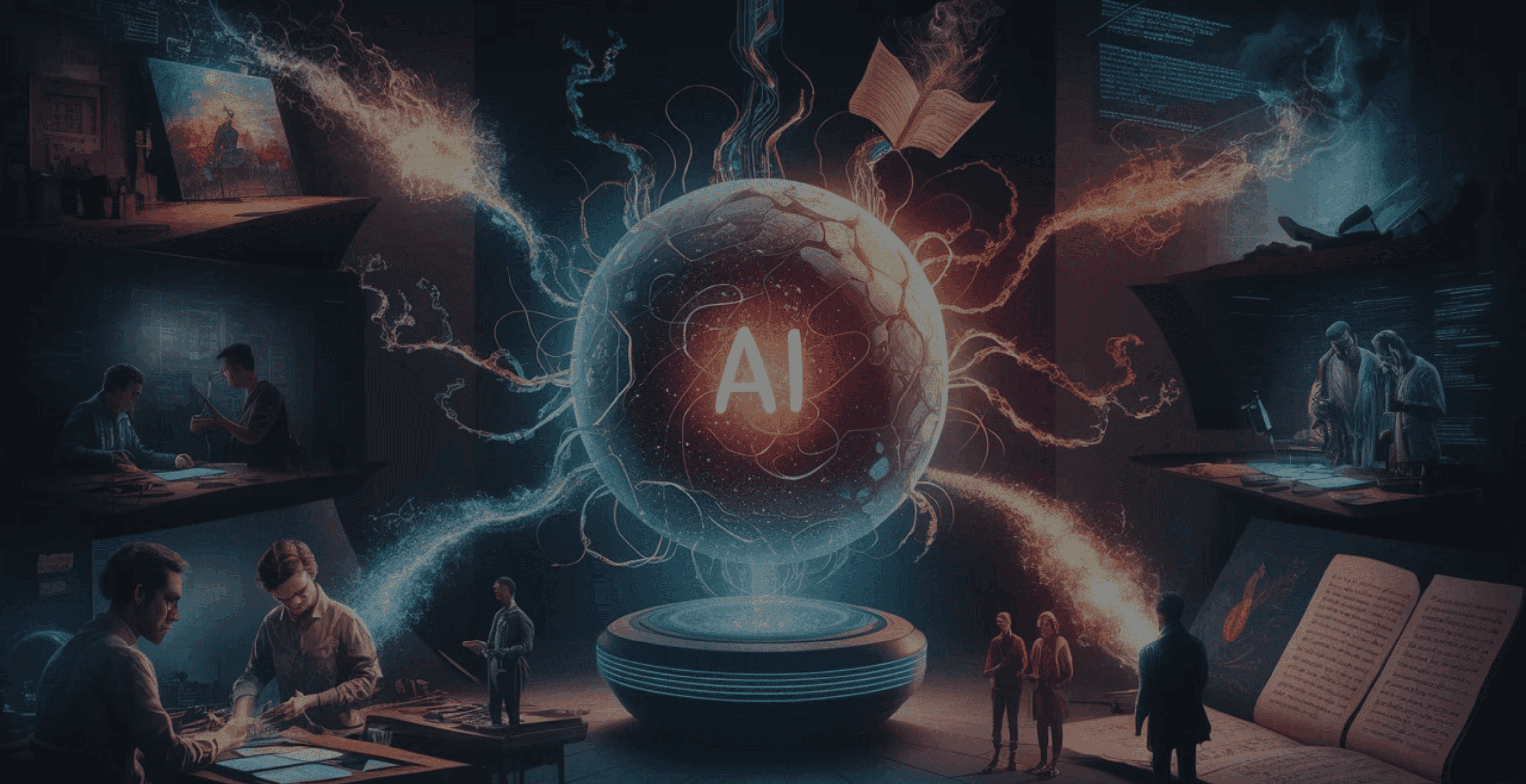
The Impact Of Generative Models On Content Creation
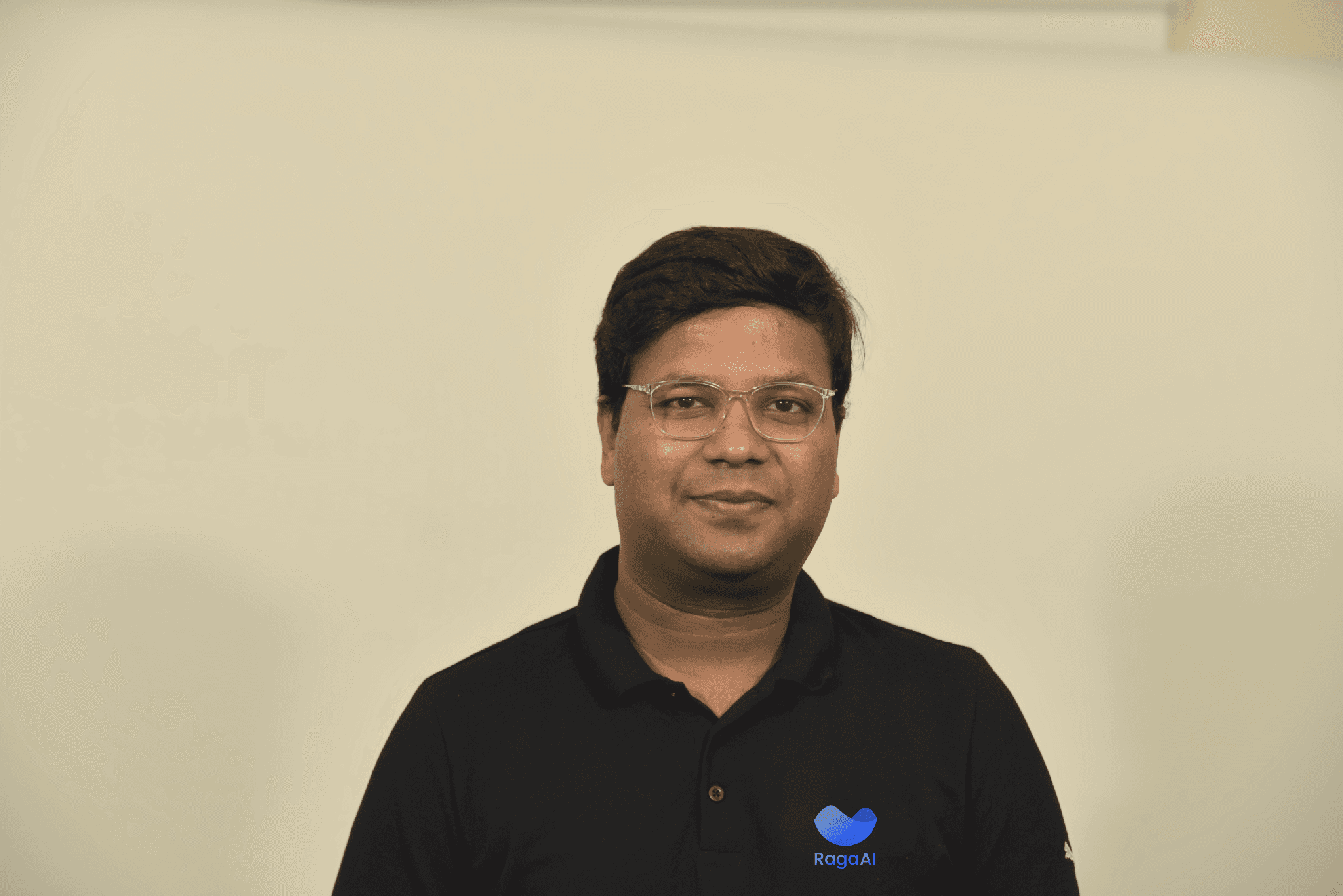
Jigar Gupta
Jun 12, 2024
Read the article




Implementing Regression Tests In AI Development
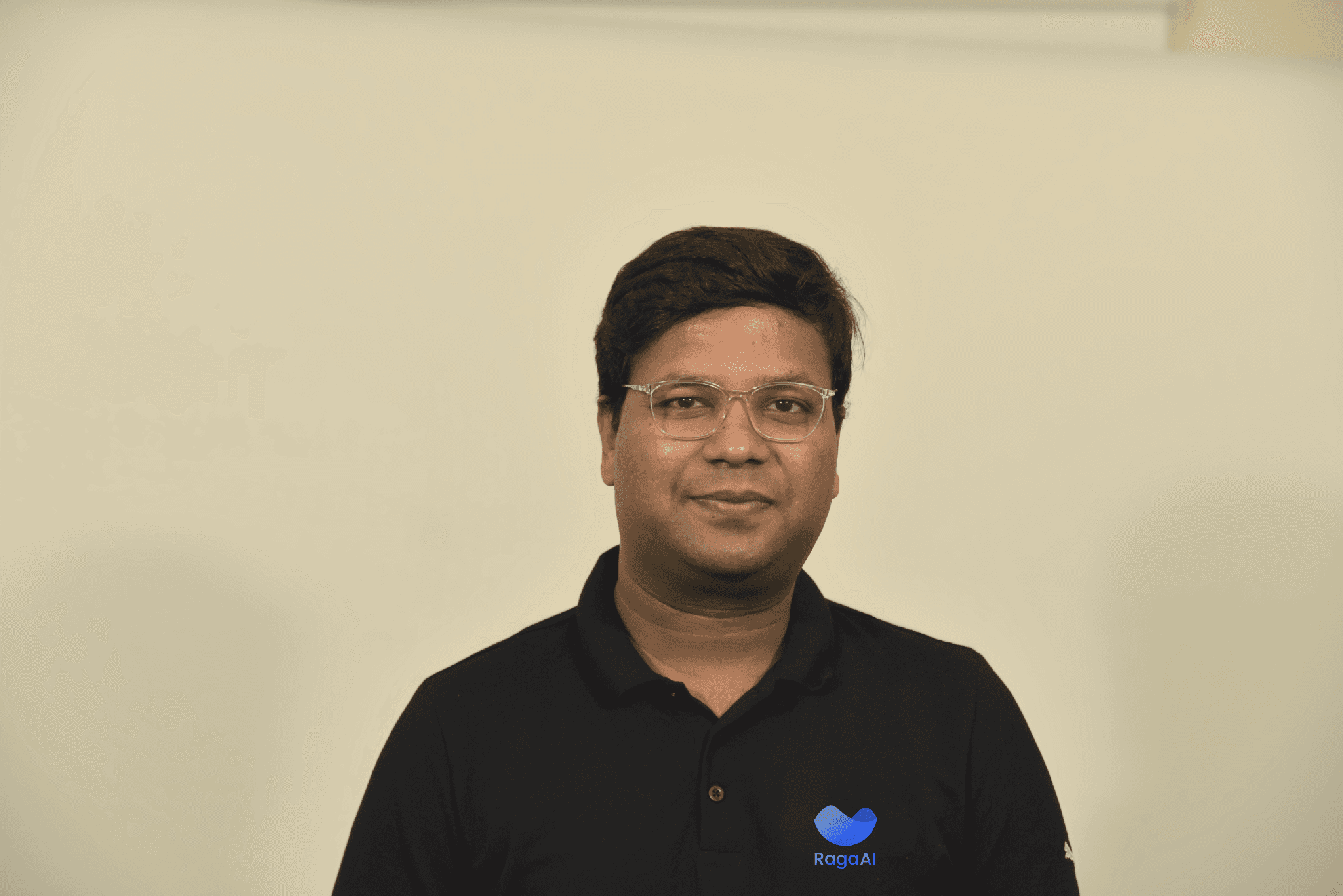
Jigar Gupta
Jun 12, 2024
Read the article
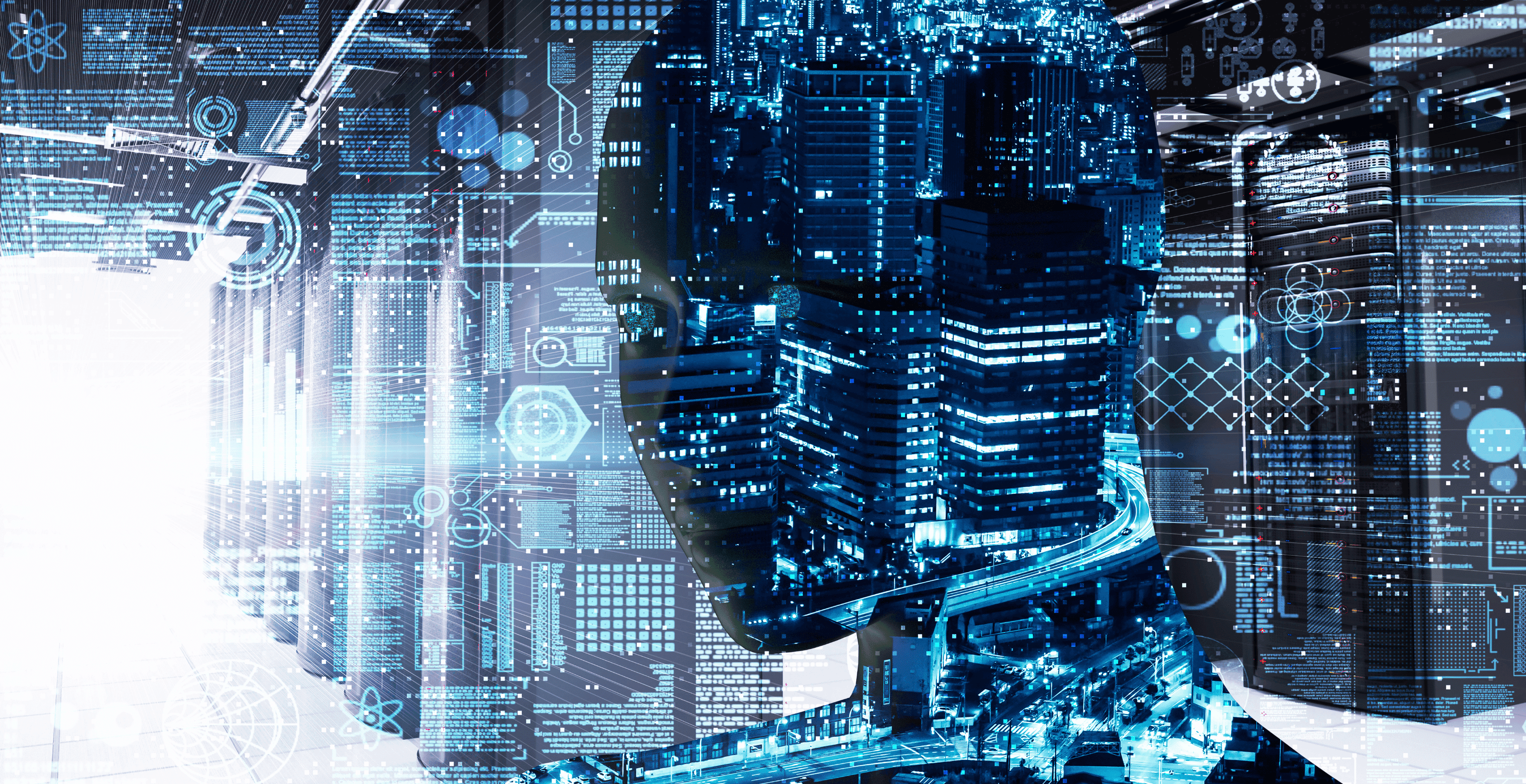
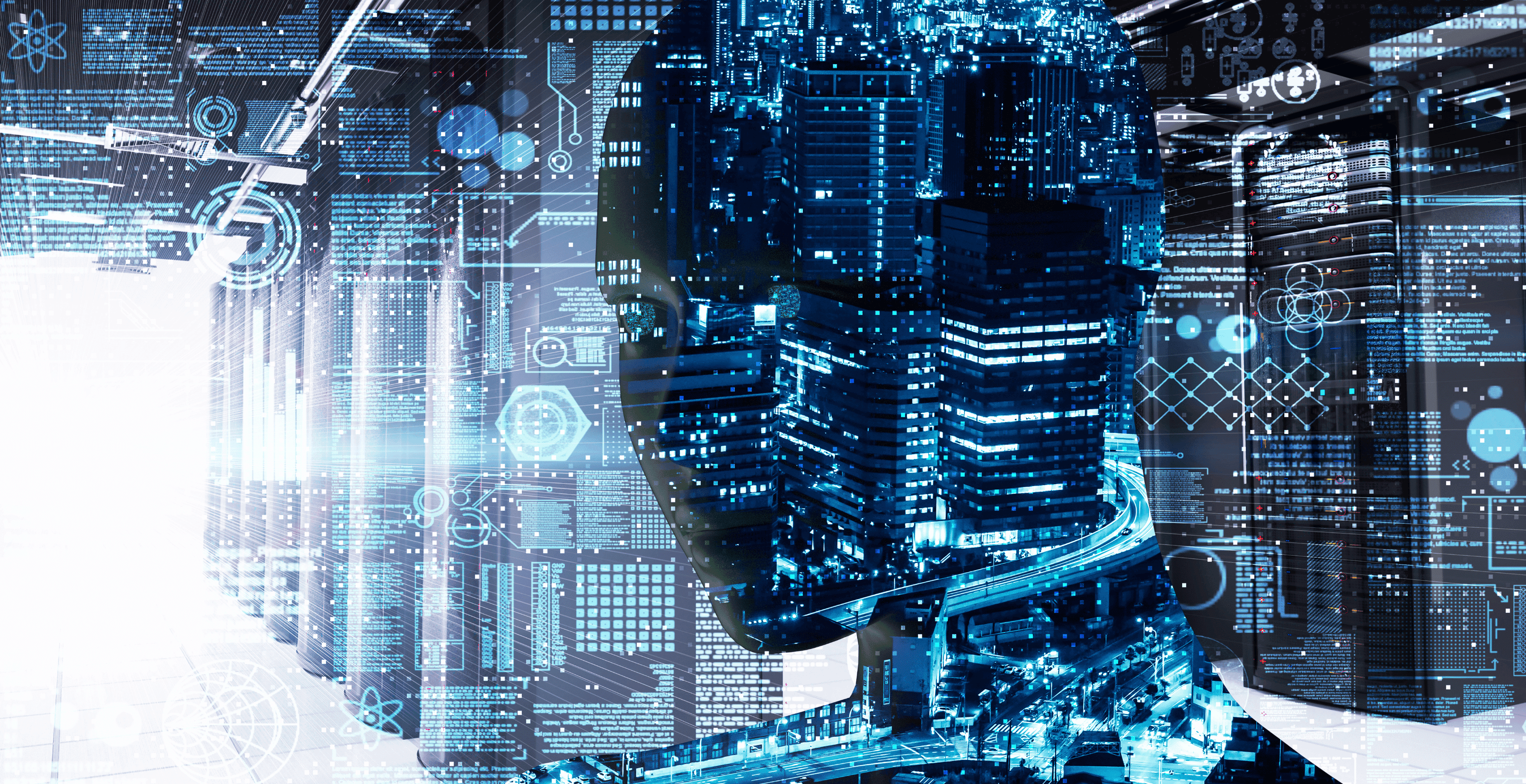
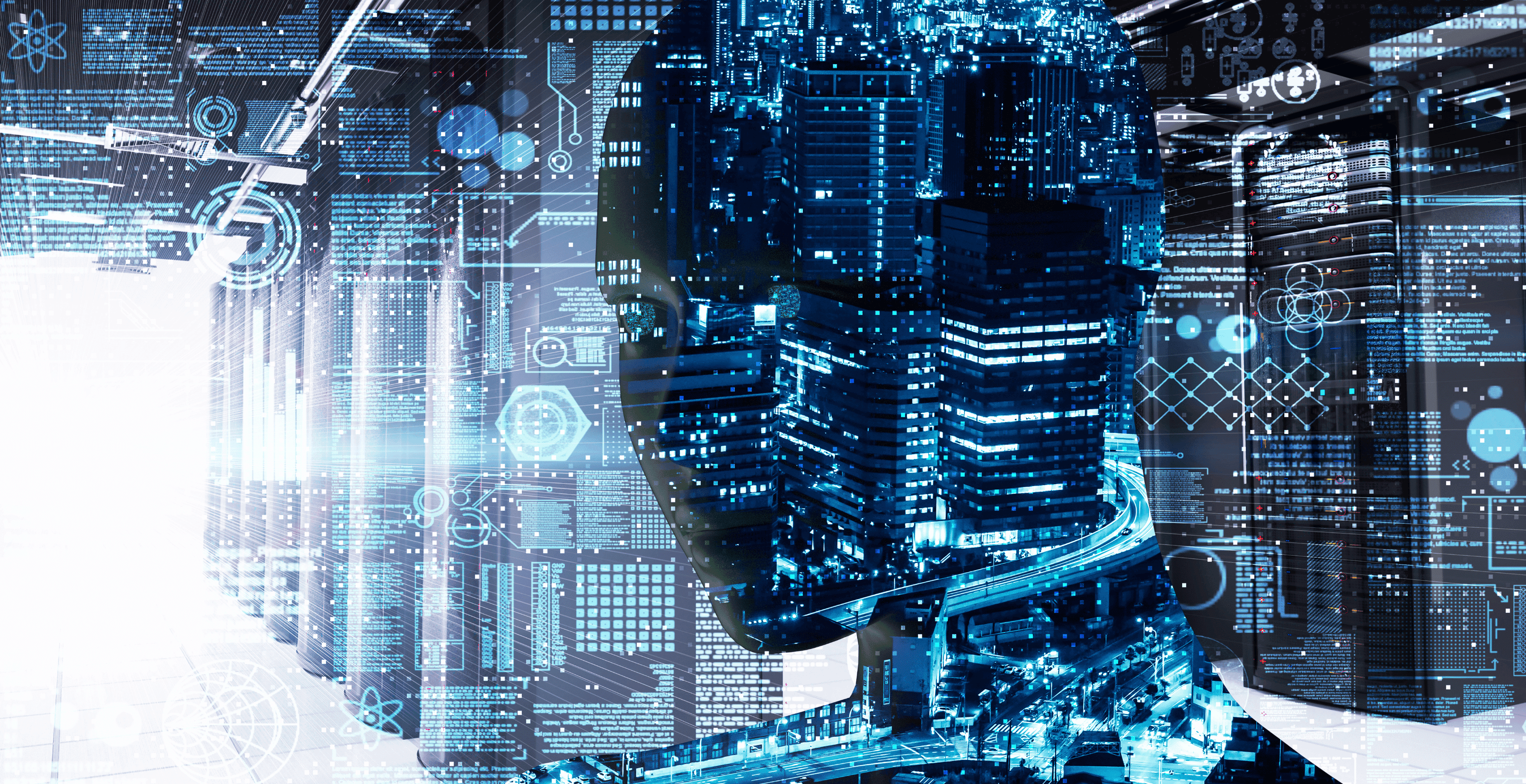
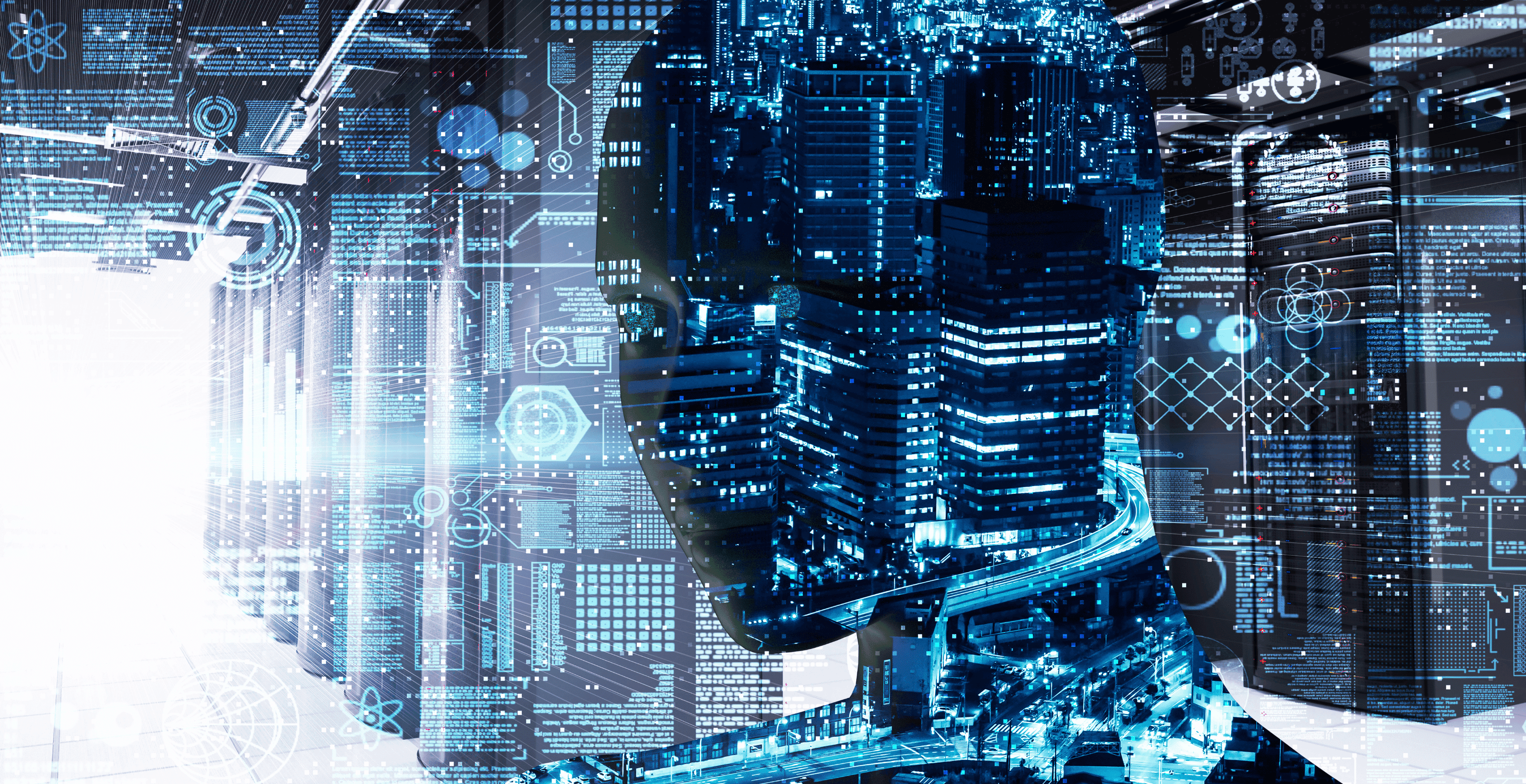
In-Depth Case Studies in AI Model Testing: Exploring Real-World Applications and Insights
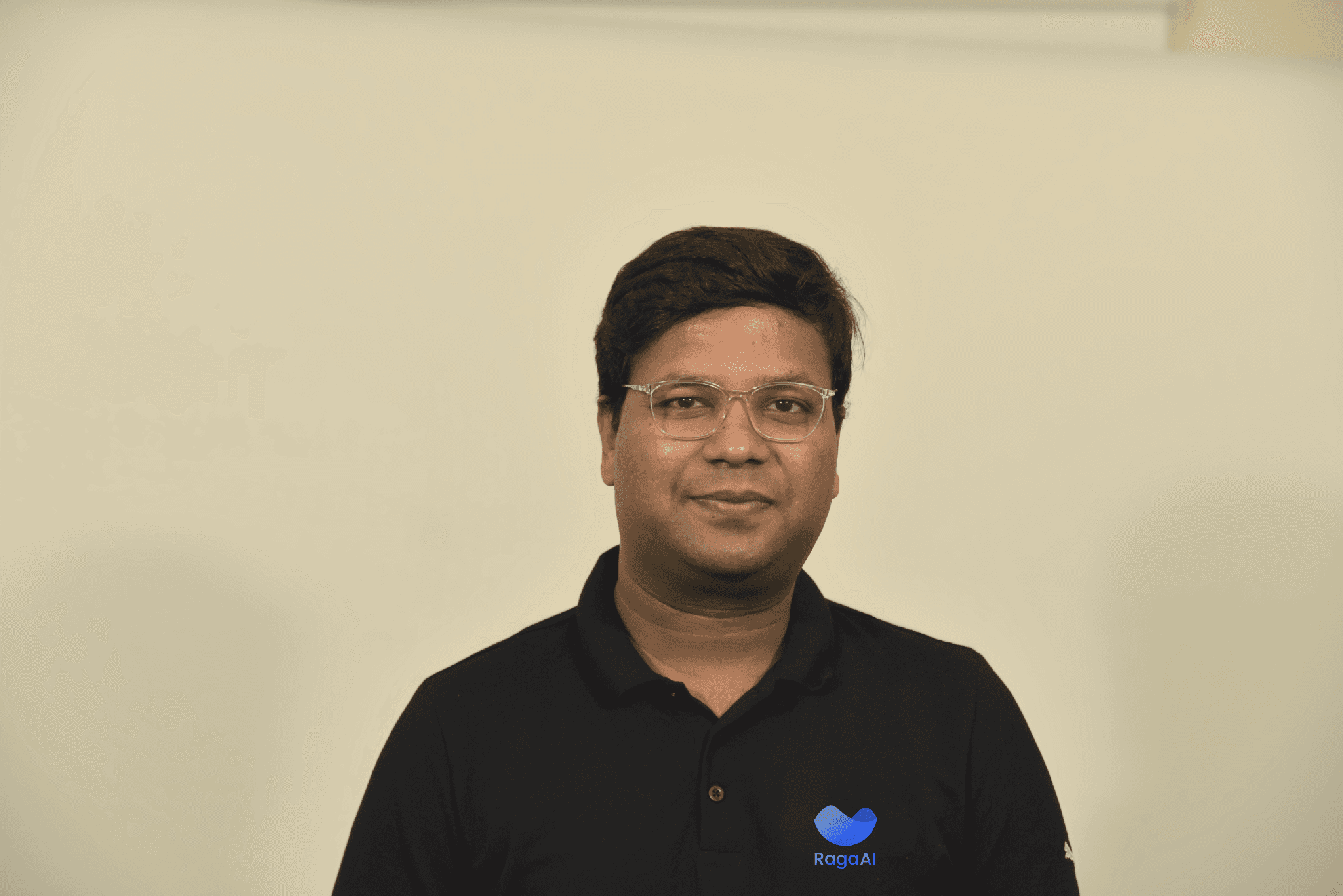
Jigar Gupta
Jun 11, 2024
Read the article




Techniques and Importance of Stress Testing AI Systems
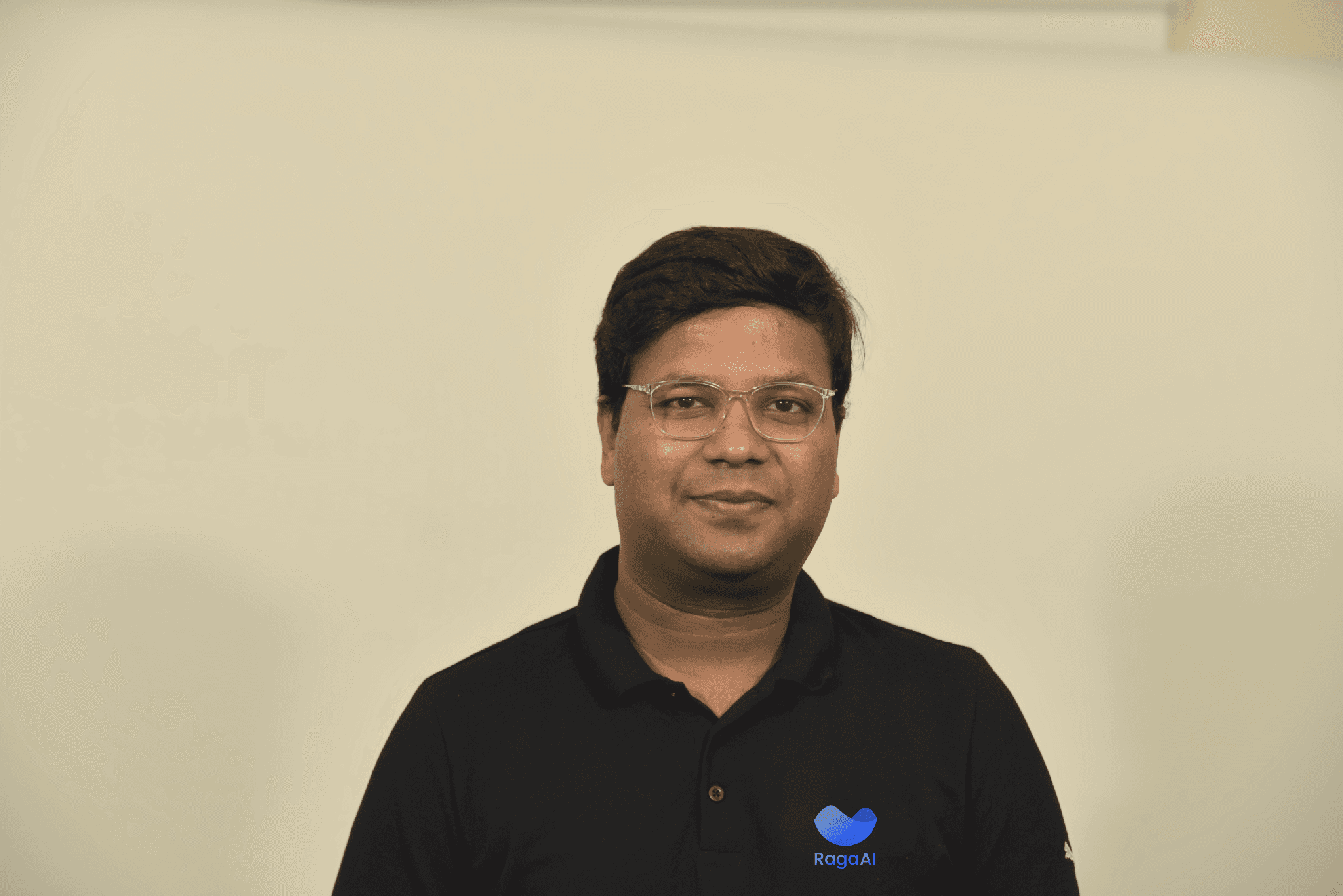
Jigar Gupta
Jun 11, 2024
Read the article
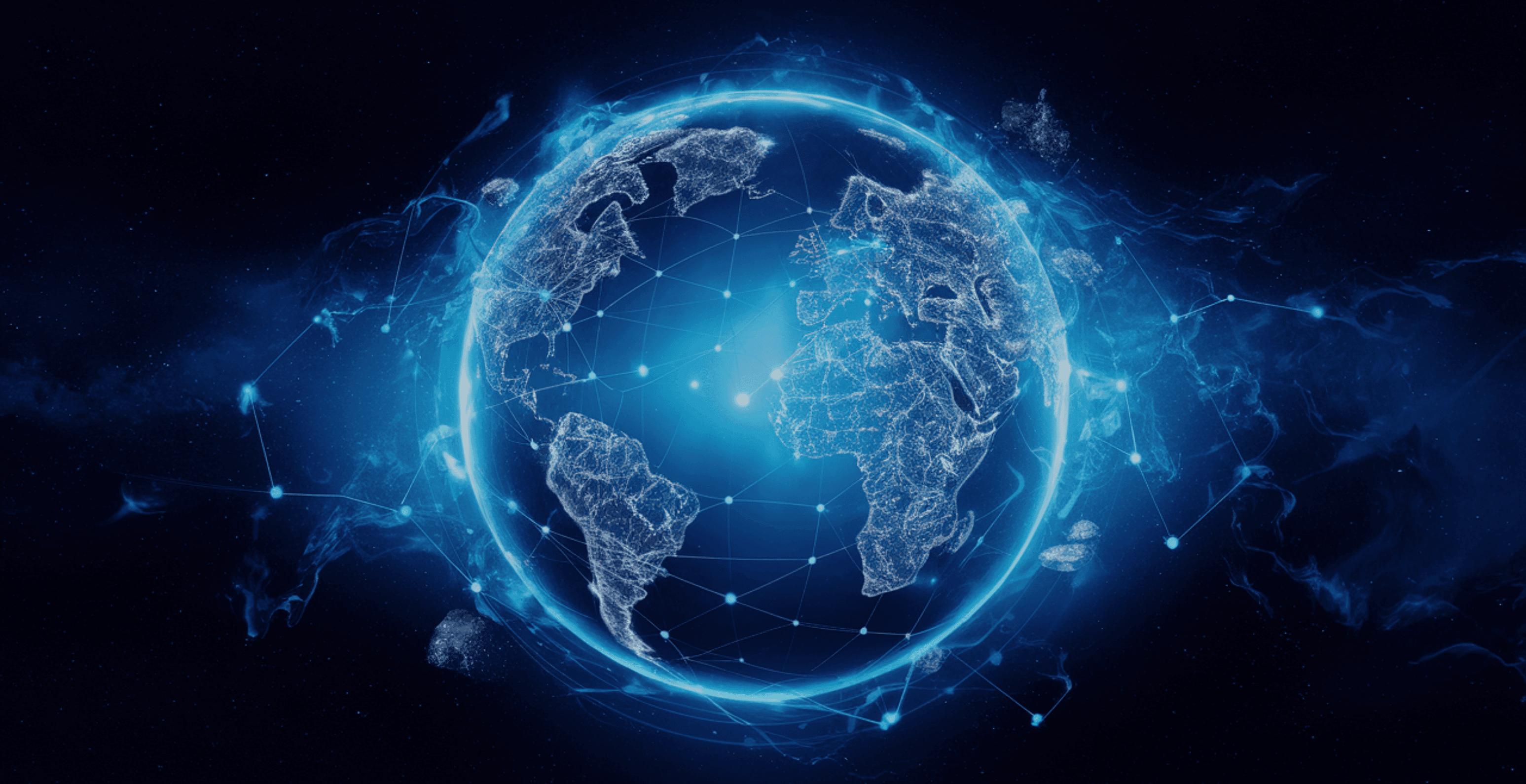
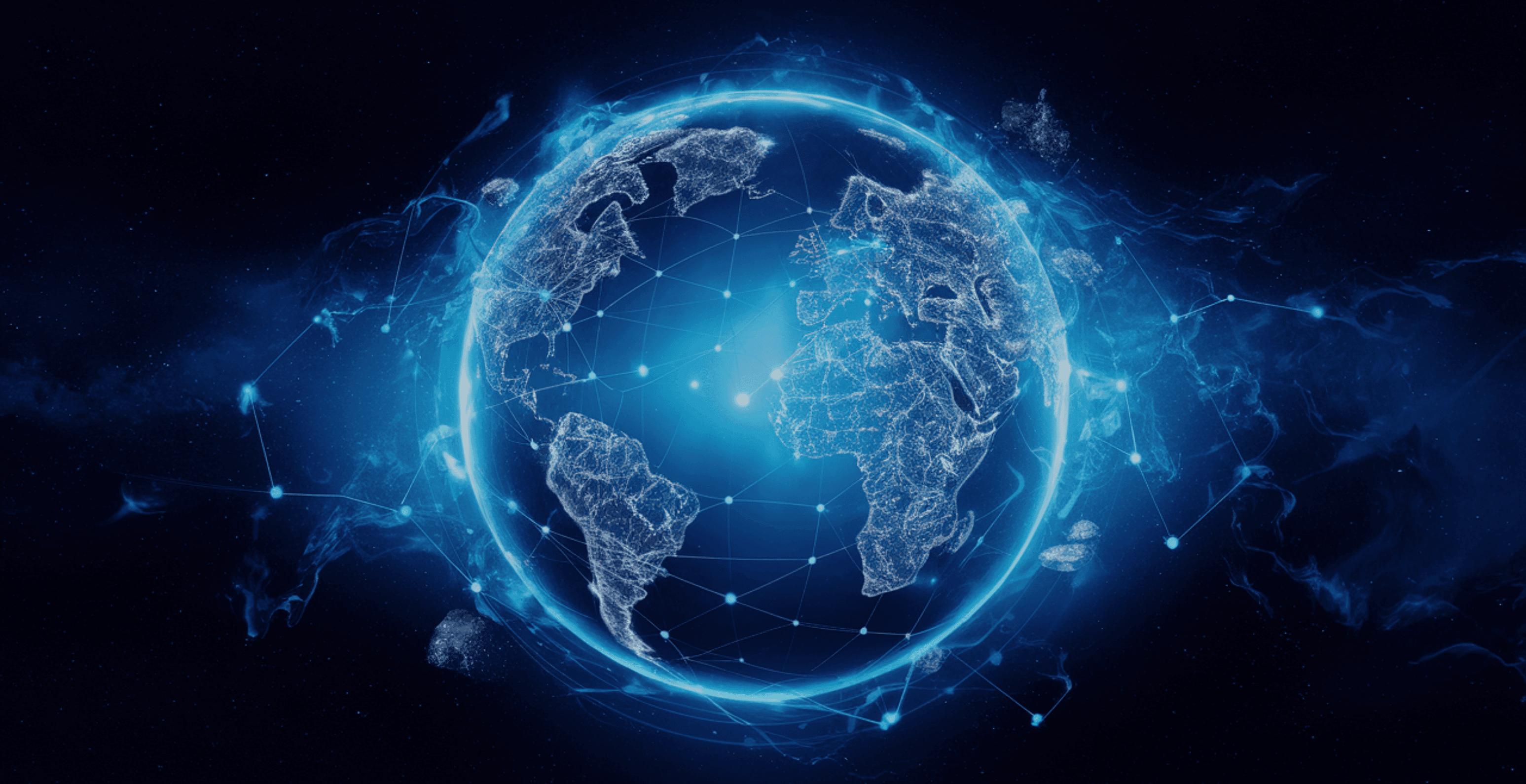
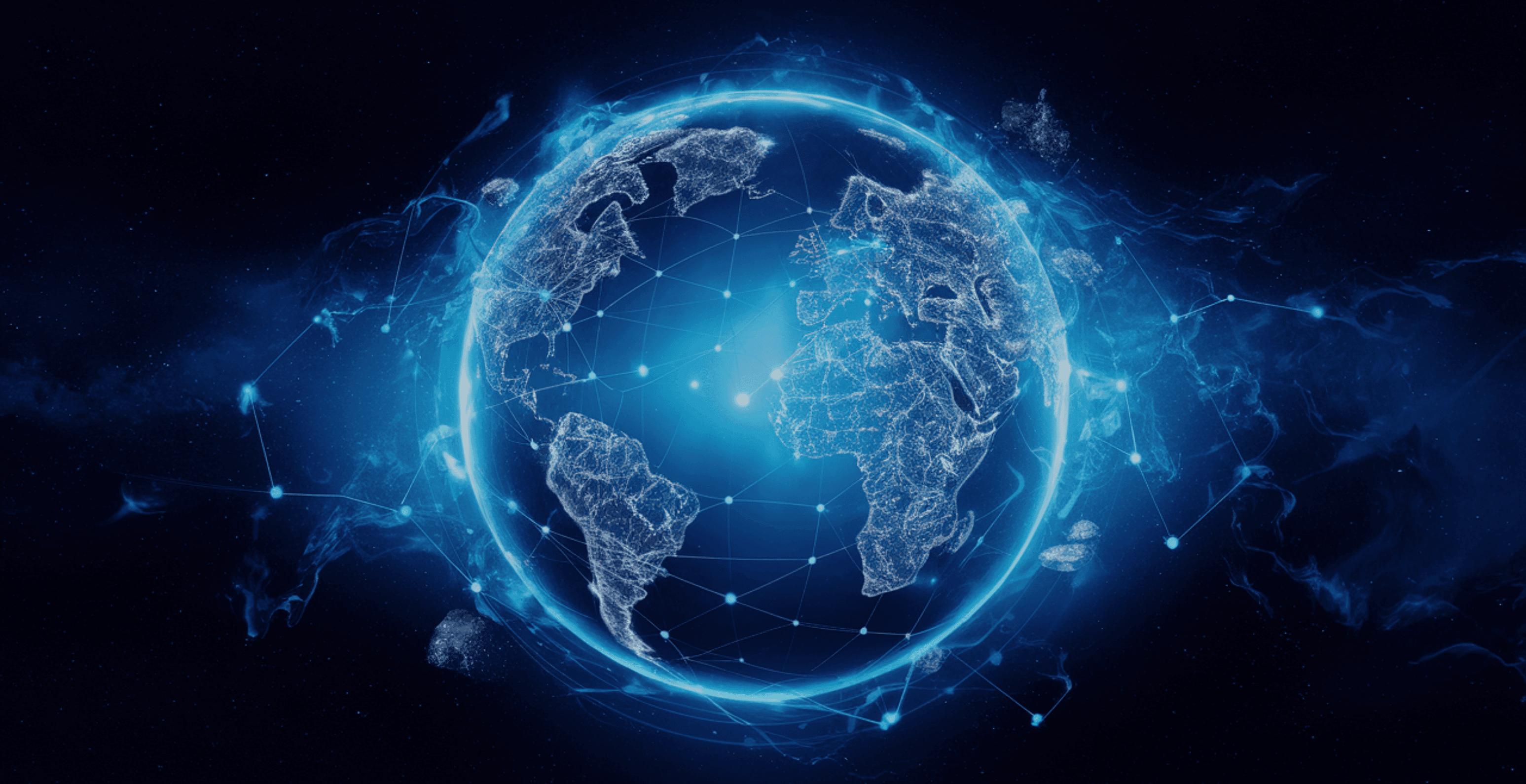
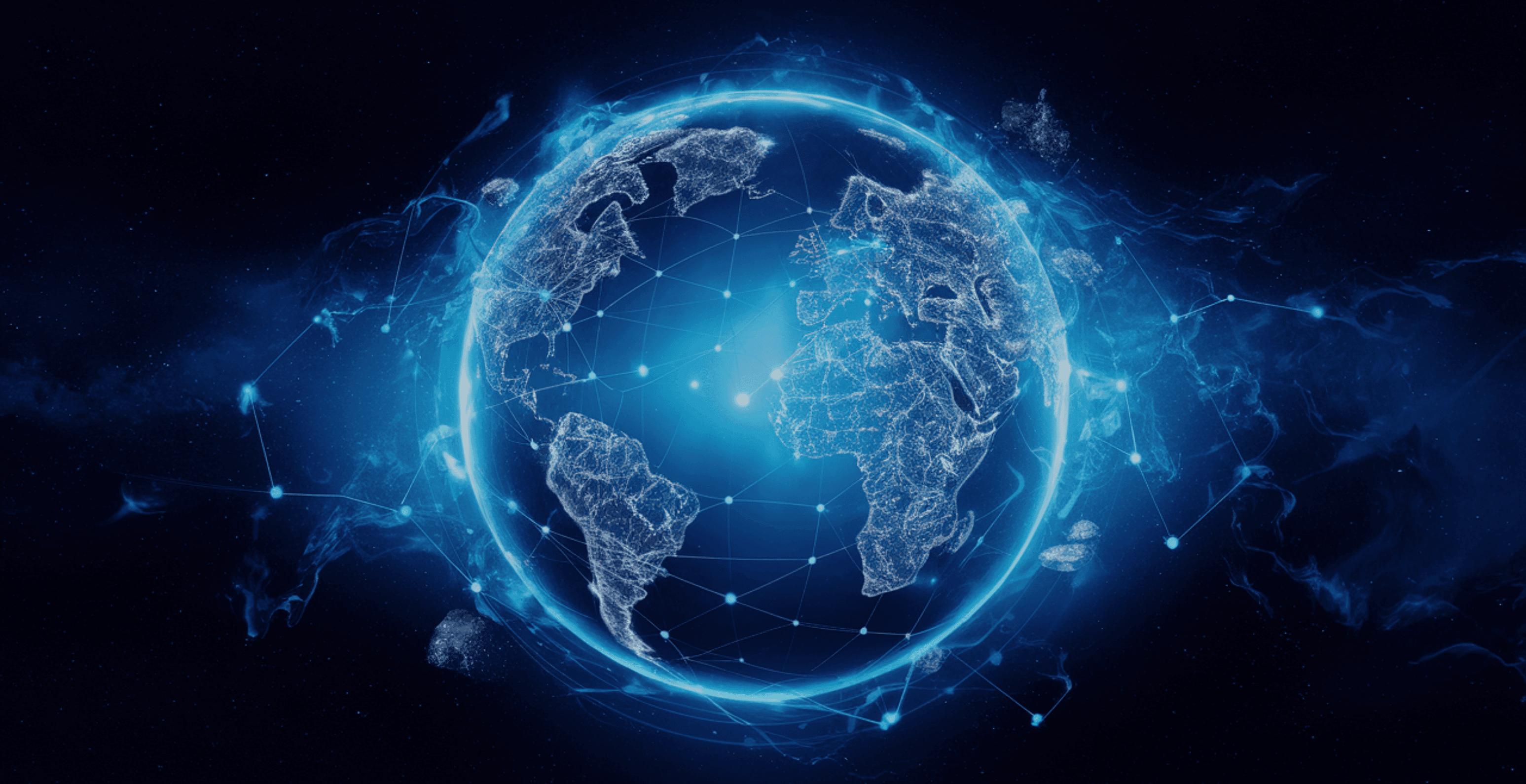
Navigating Global AI Regulations and Standards

Rehan Asif
Jun 10, 2024
Read the article




The Cost of Errors In AI Application Development

Rehan Asif
Jun 10, 2024
Read the article
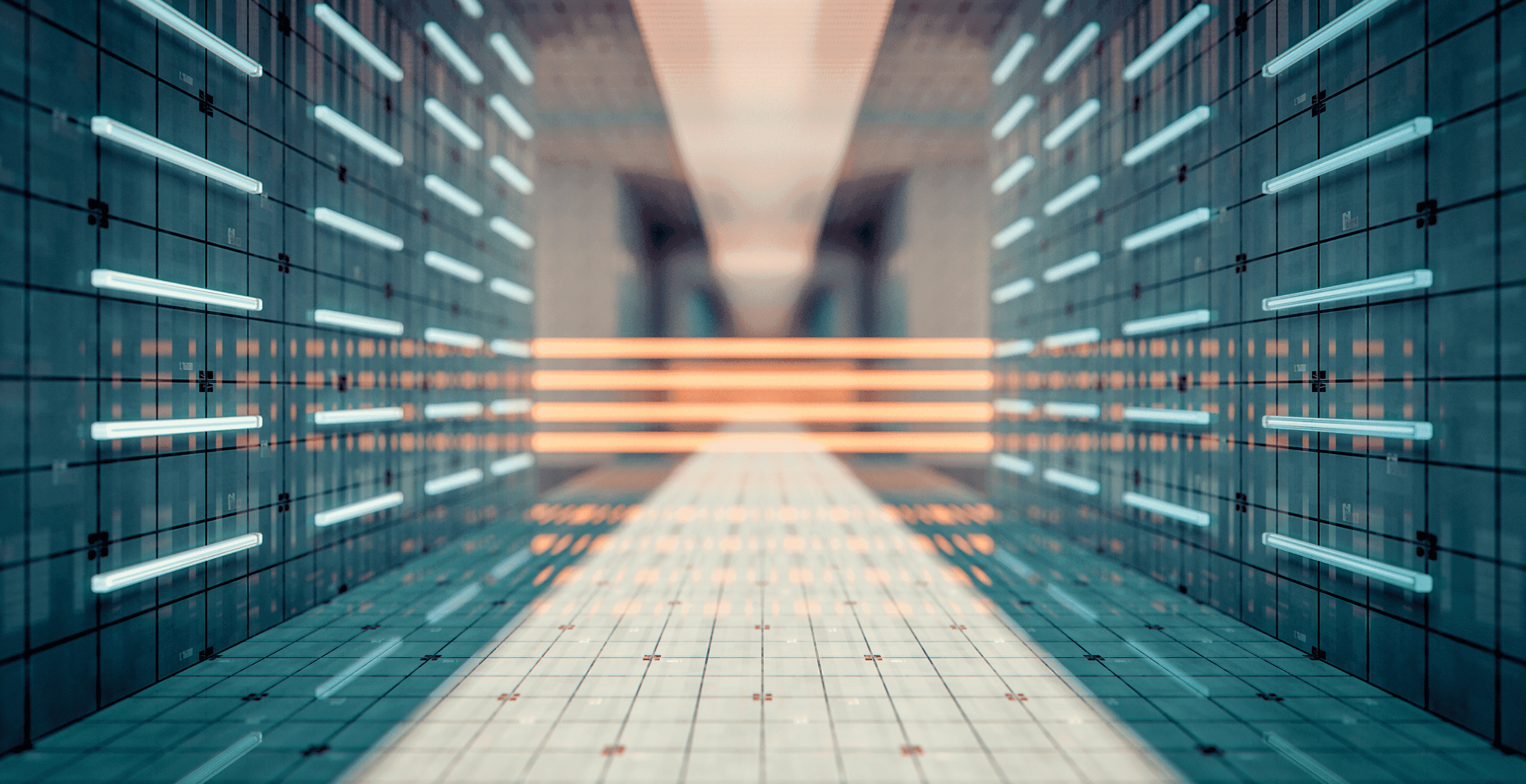
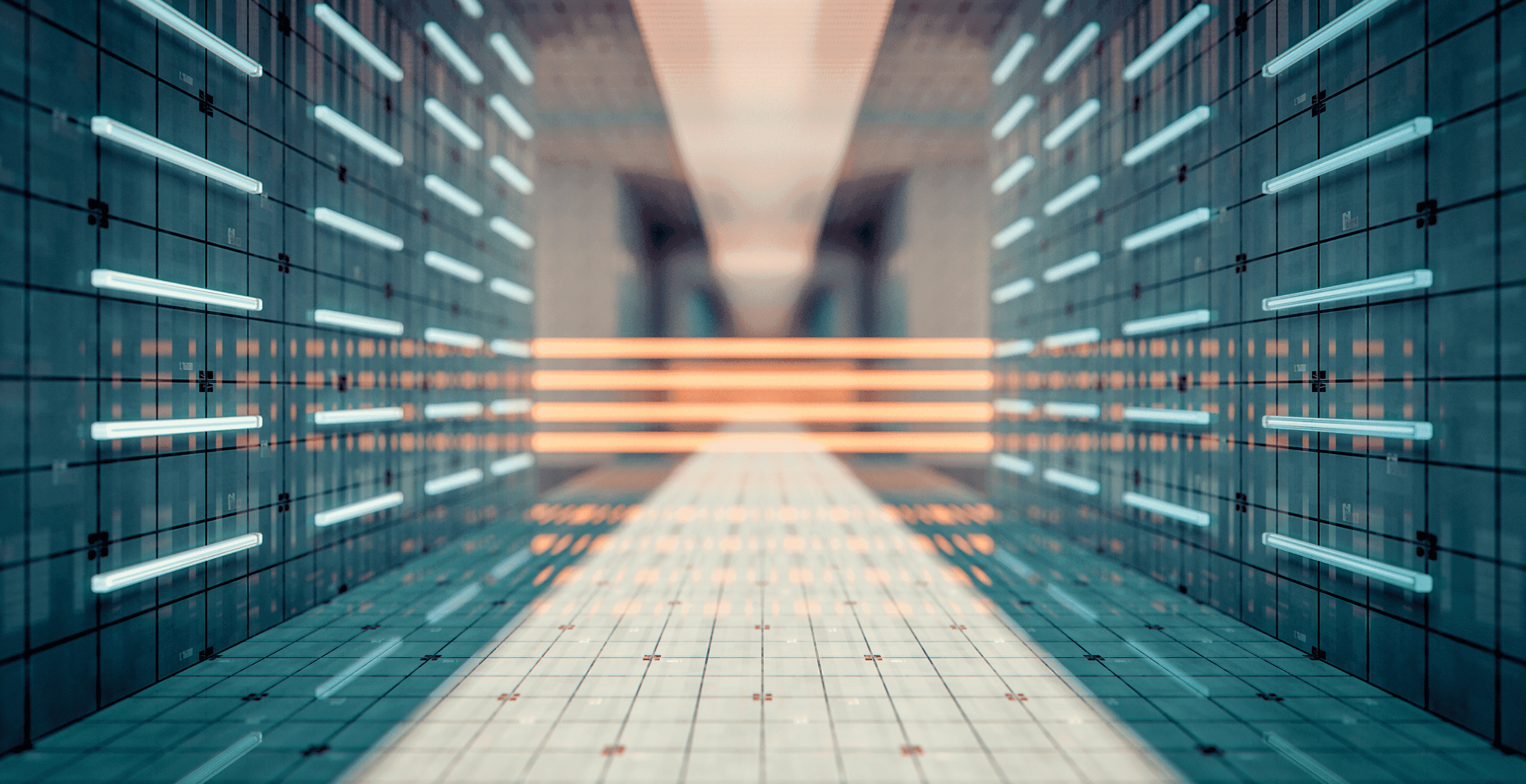
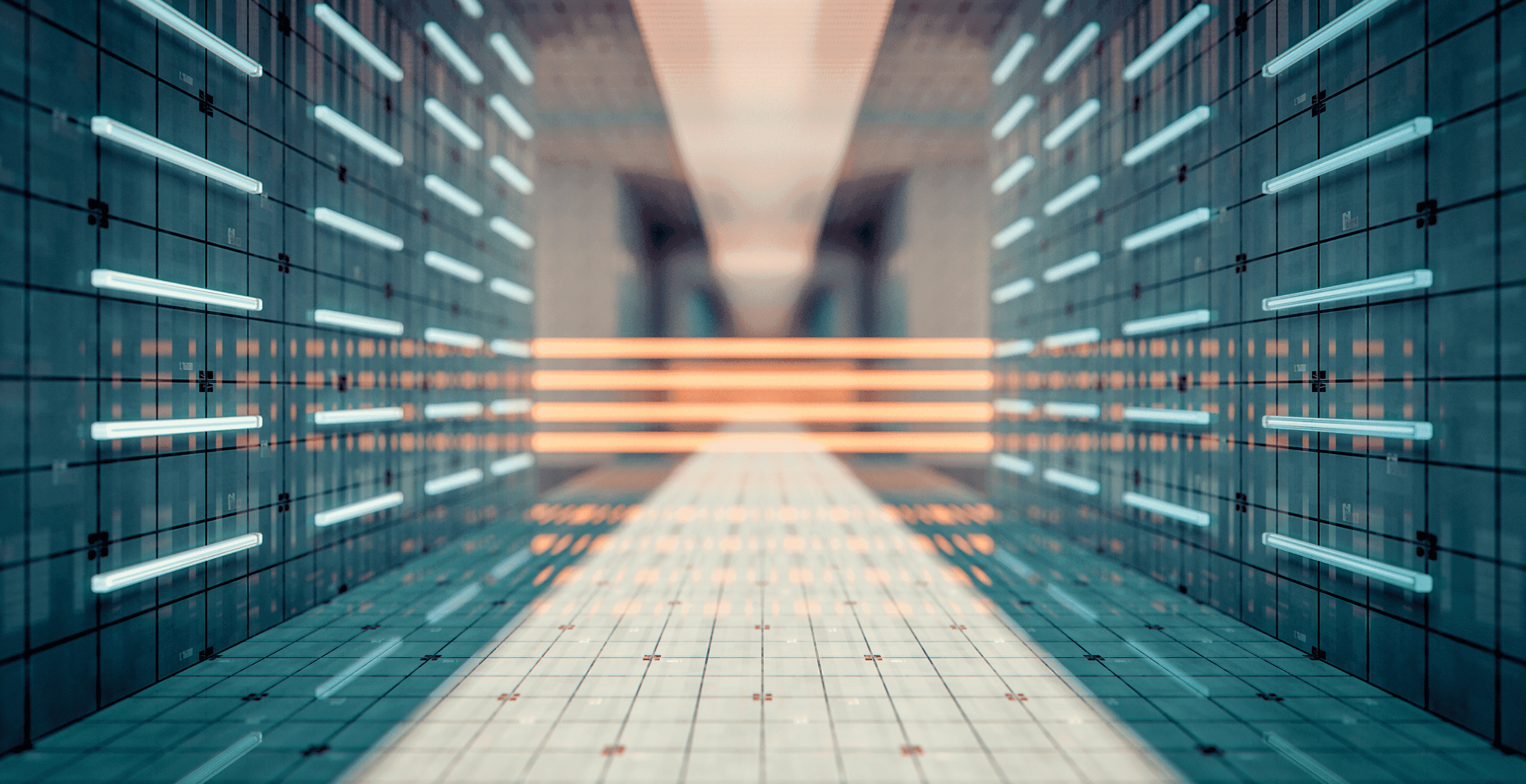
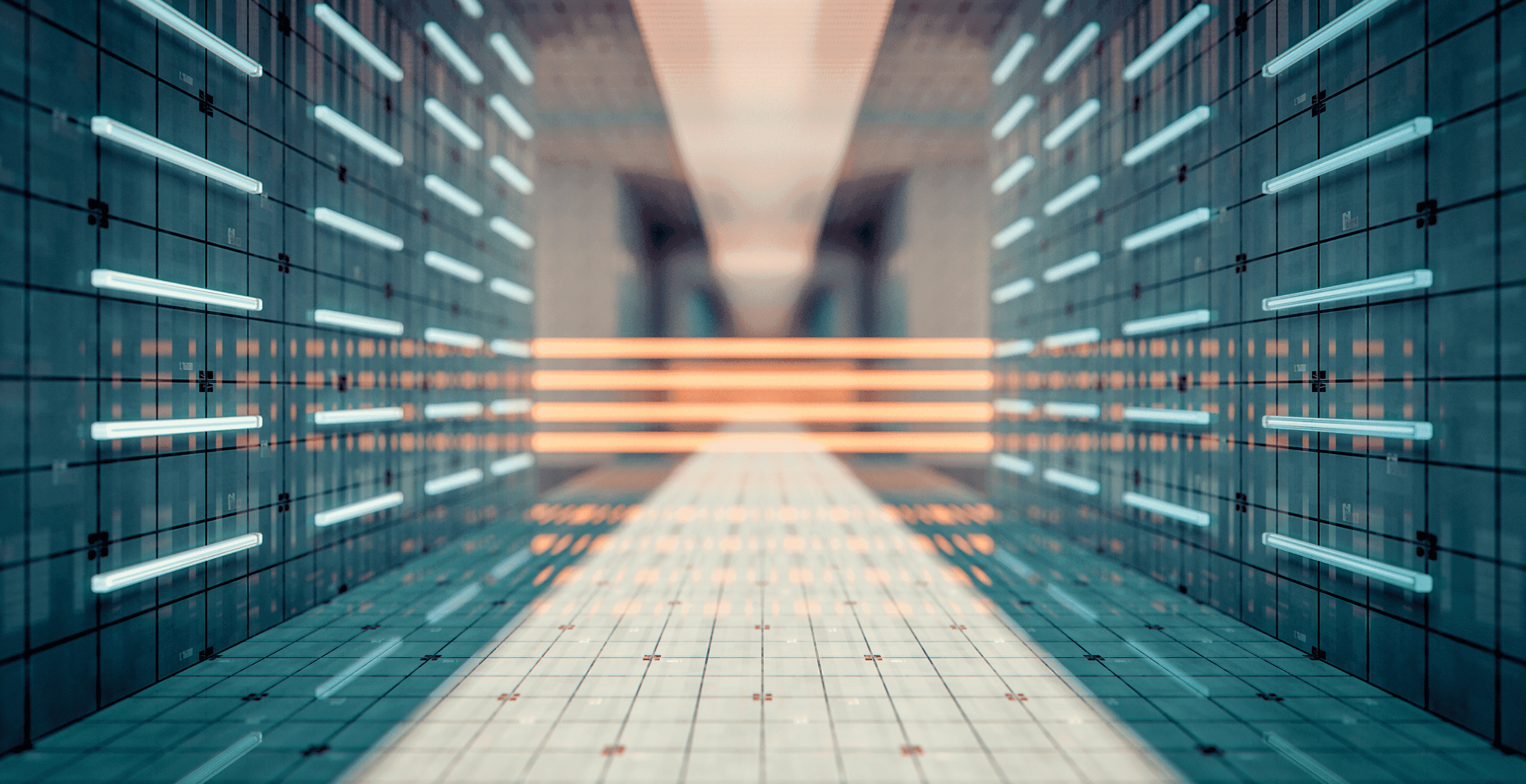
Best Practices In Data Governance For AI

Rehan Asif
Jun 10, 2024
Read the article




Success Stories And Case Studies Of AI Adoption Across Industries
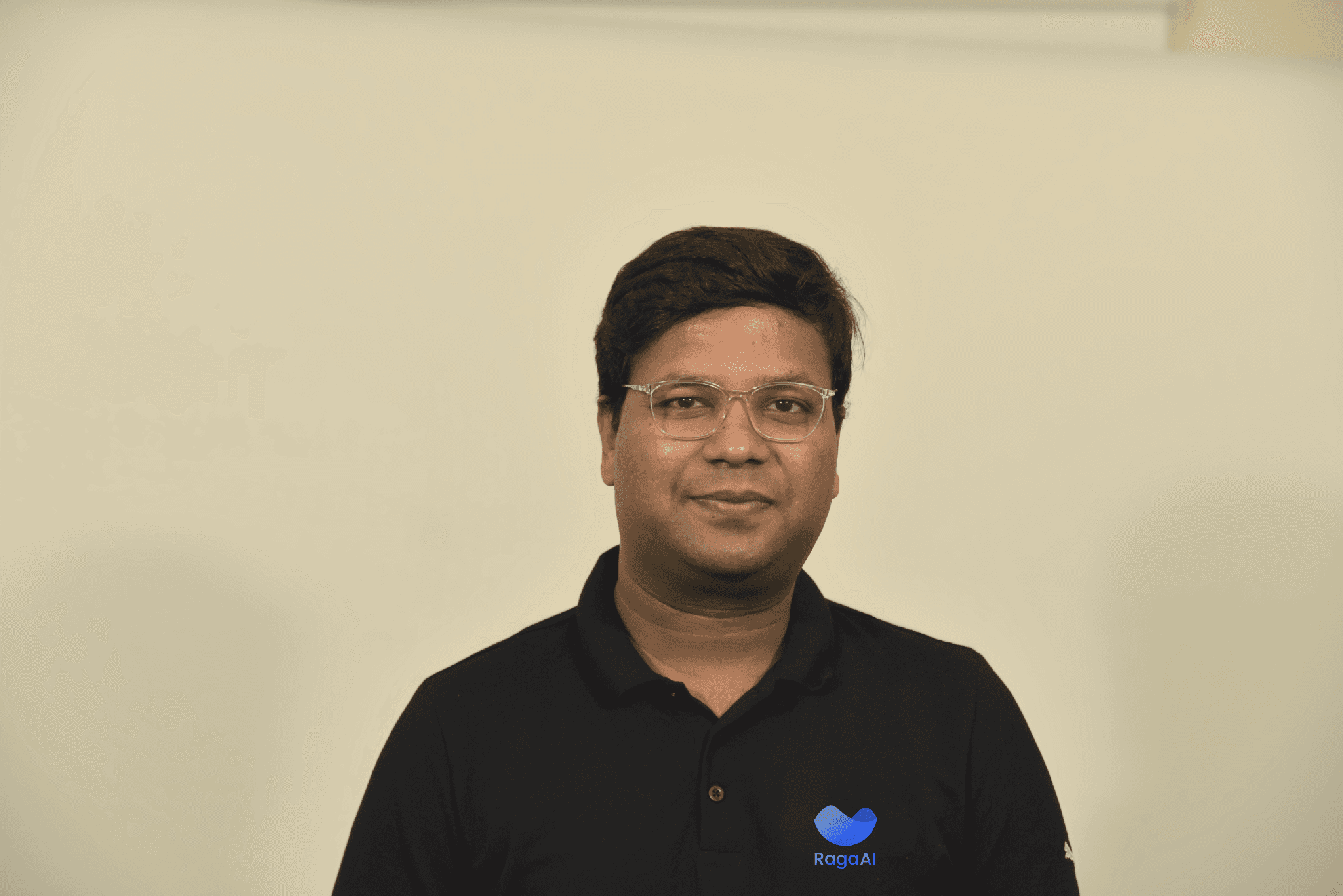
Jigar Gupta
May 1, 2024
Read the article
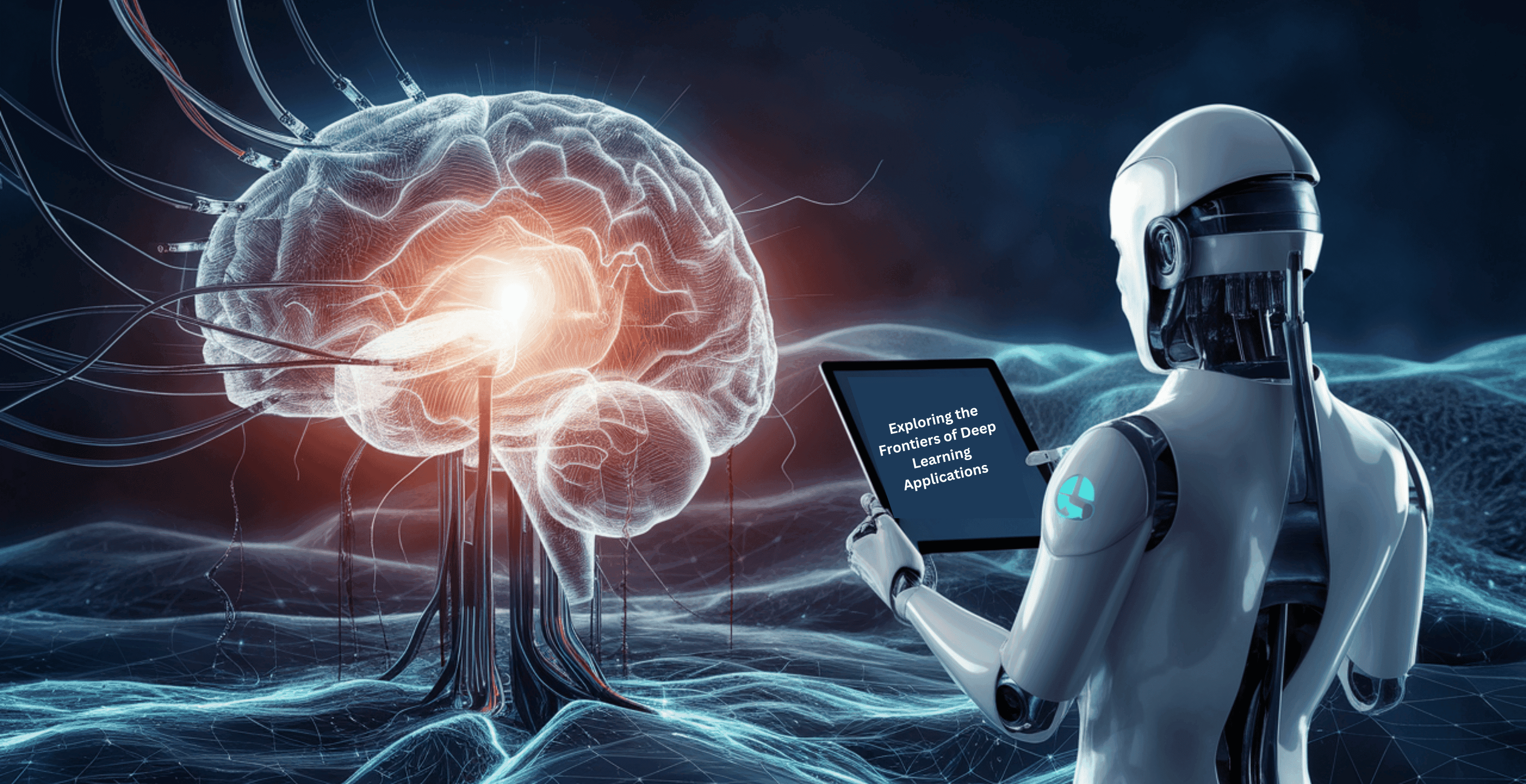
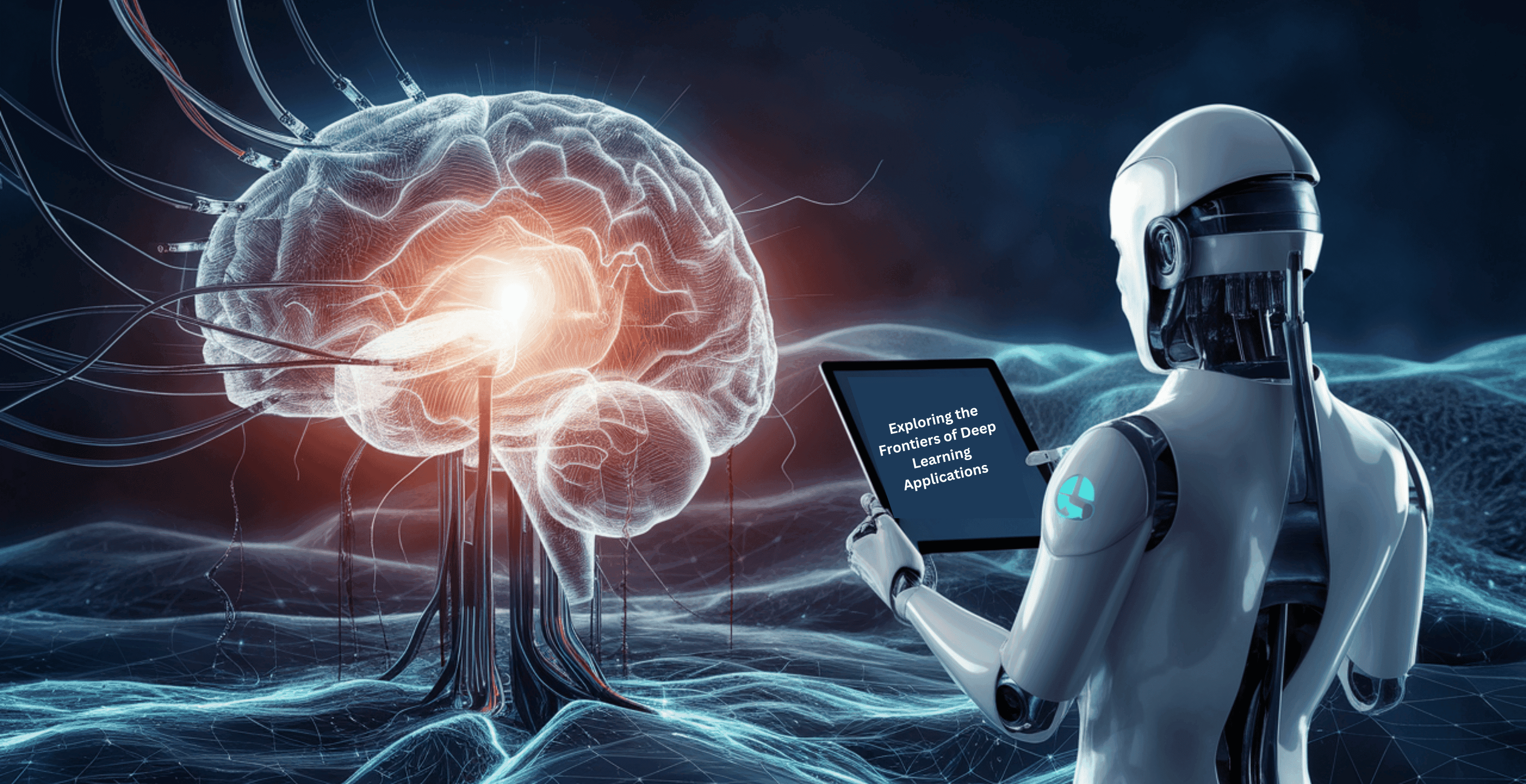
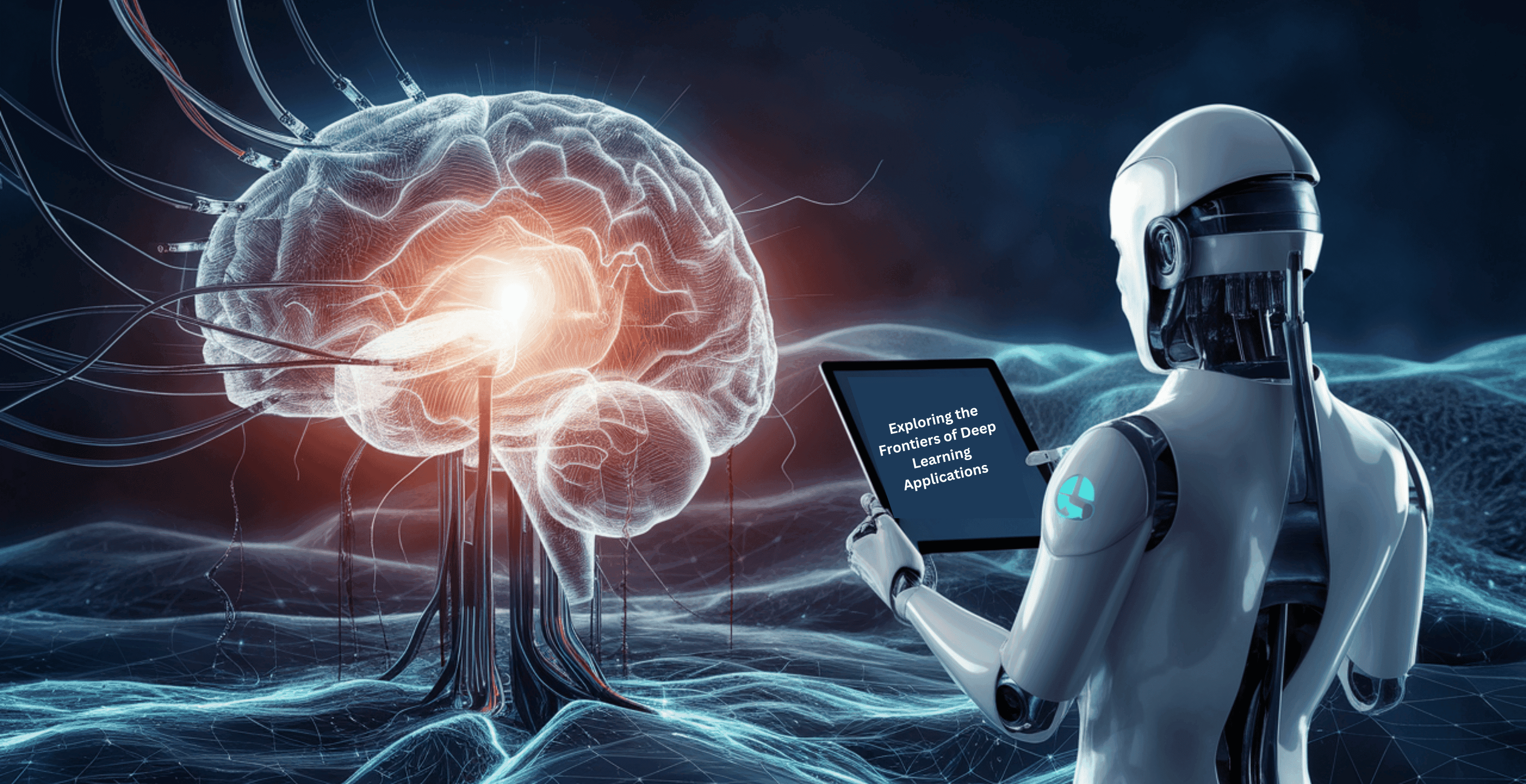
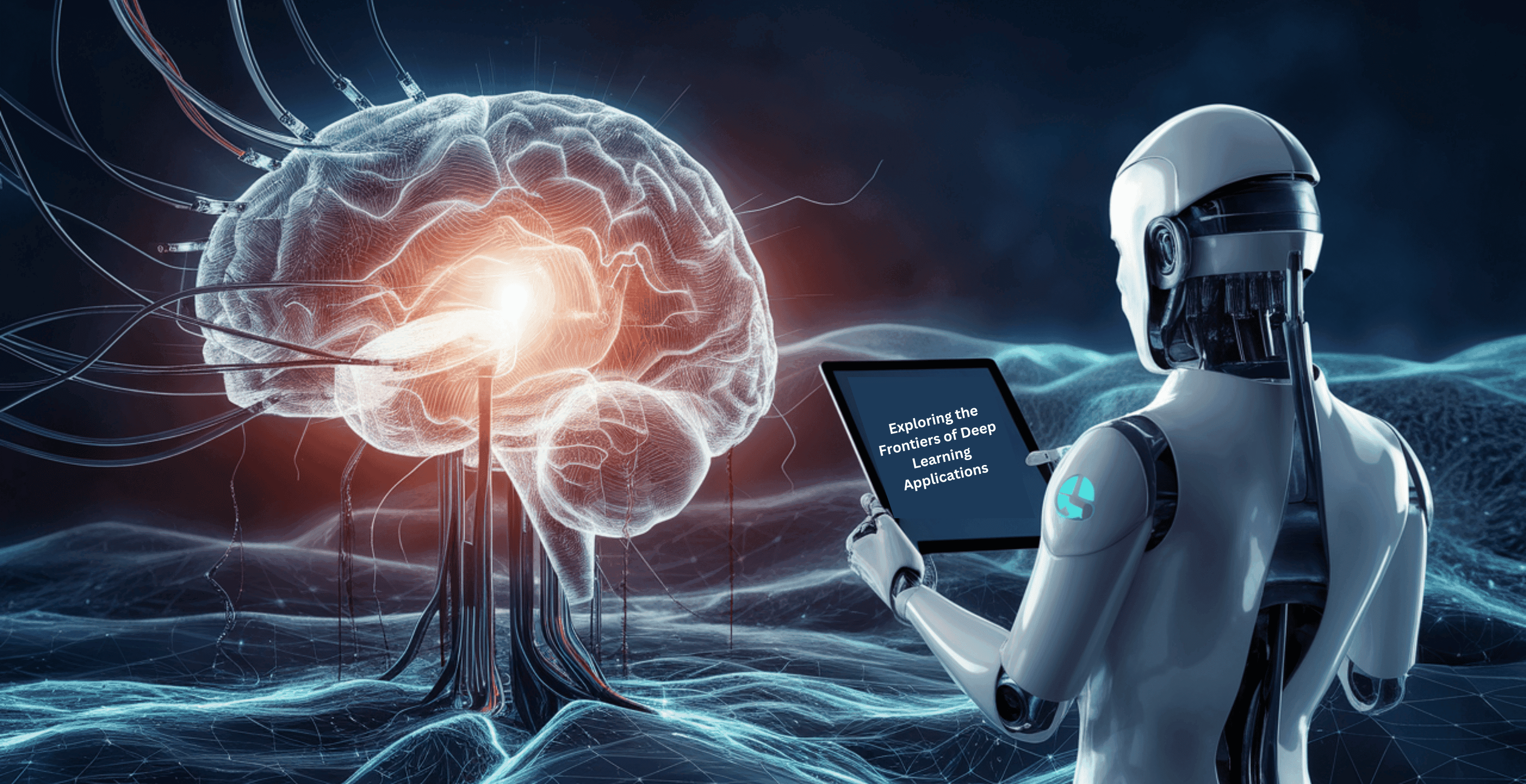
Exploring The Frontiers Of Deep Learning Applications
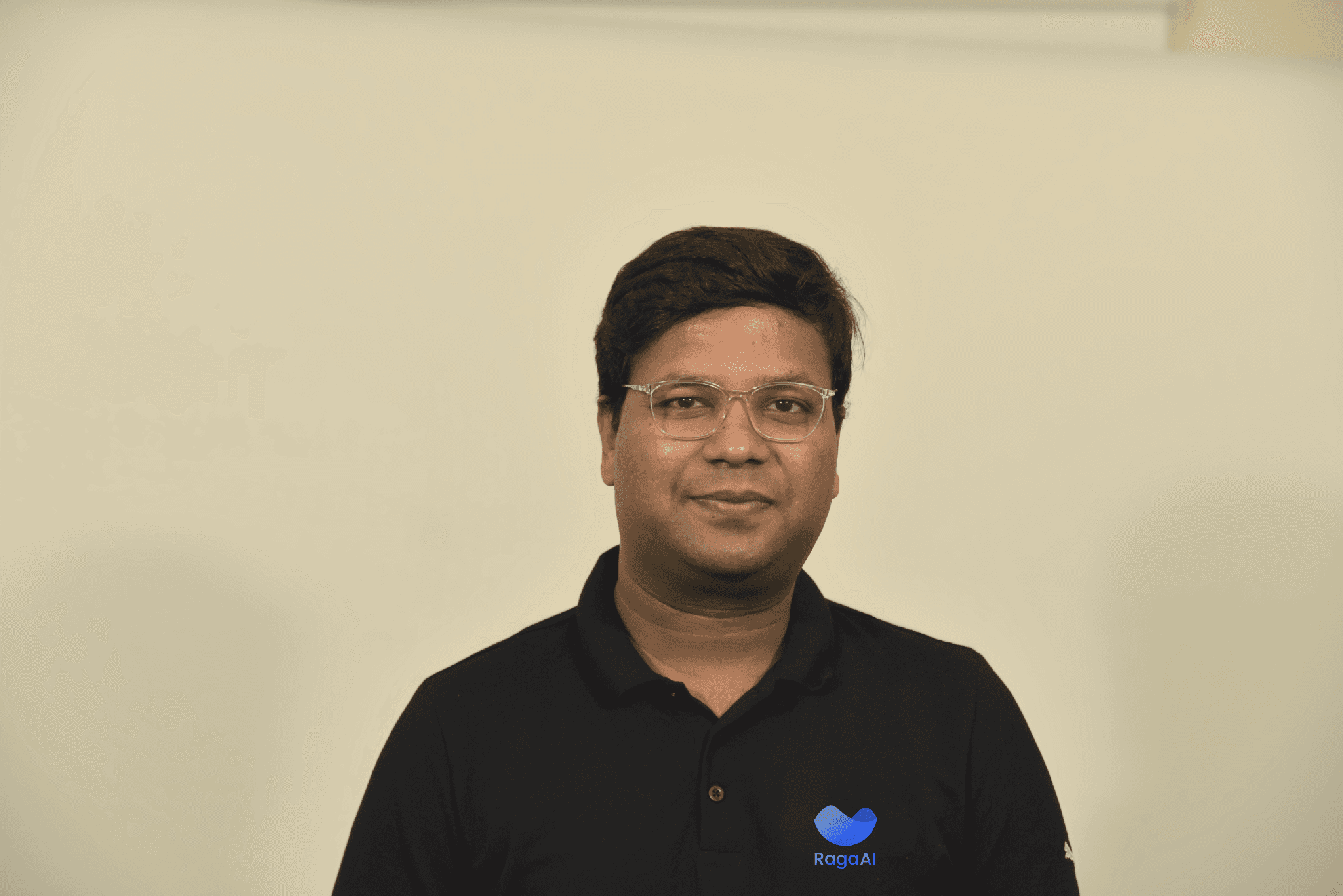
Jigar Gupta
May 1, 2024
Read the article




Integration Of RAG Platforms With Existing Enterprise Systems
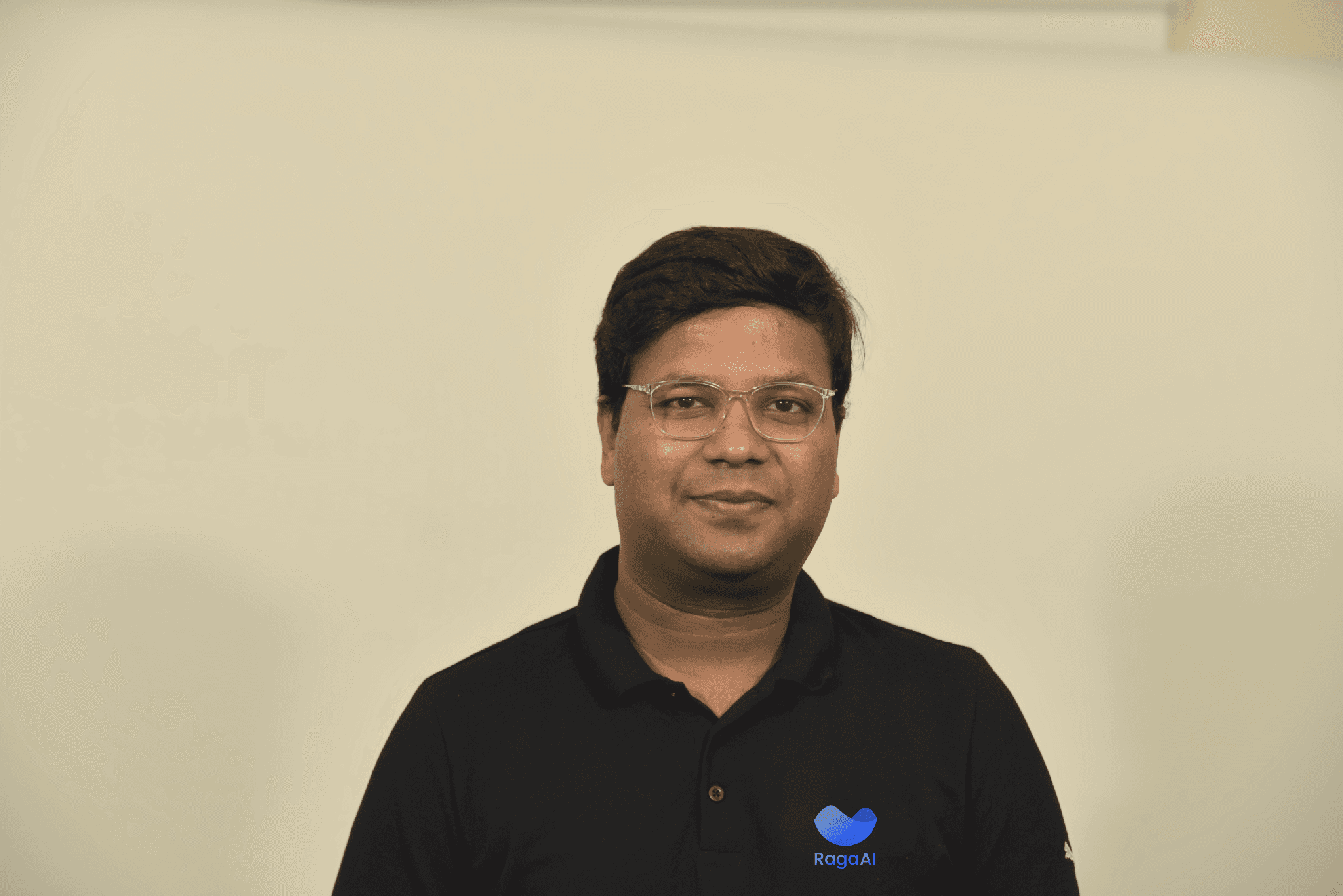
Jigar Gupta
Apr 30, 2024
Read the article




Multimodal LLMS Using Image And Text

Rehan Asif
Apr 30, 2024
Read the article




Understanding ML Model Monitoring In Production

Rehan Asif
Apr 30, 2024
Read the article
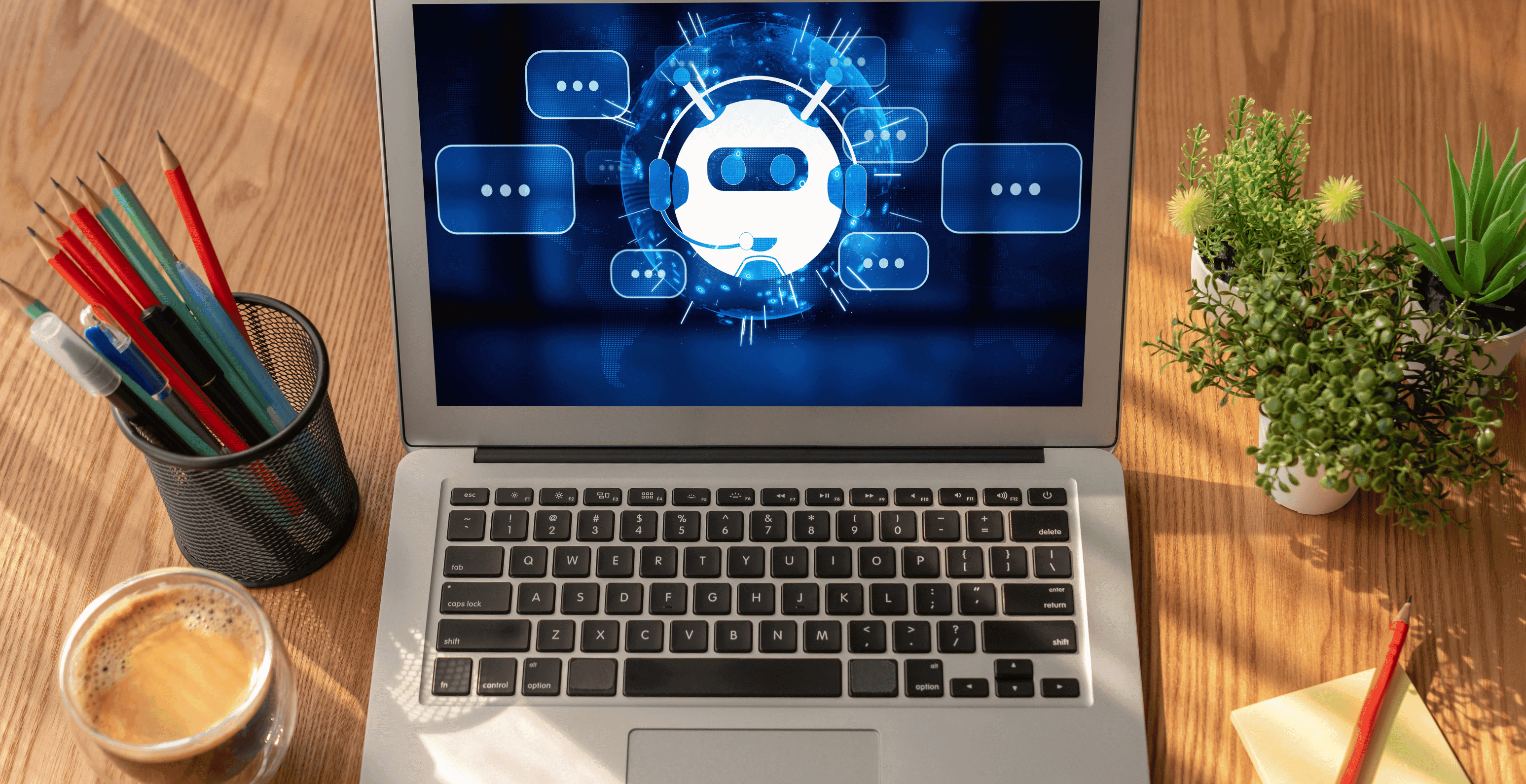
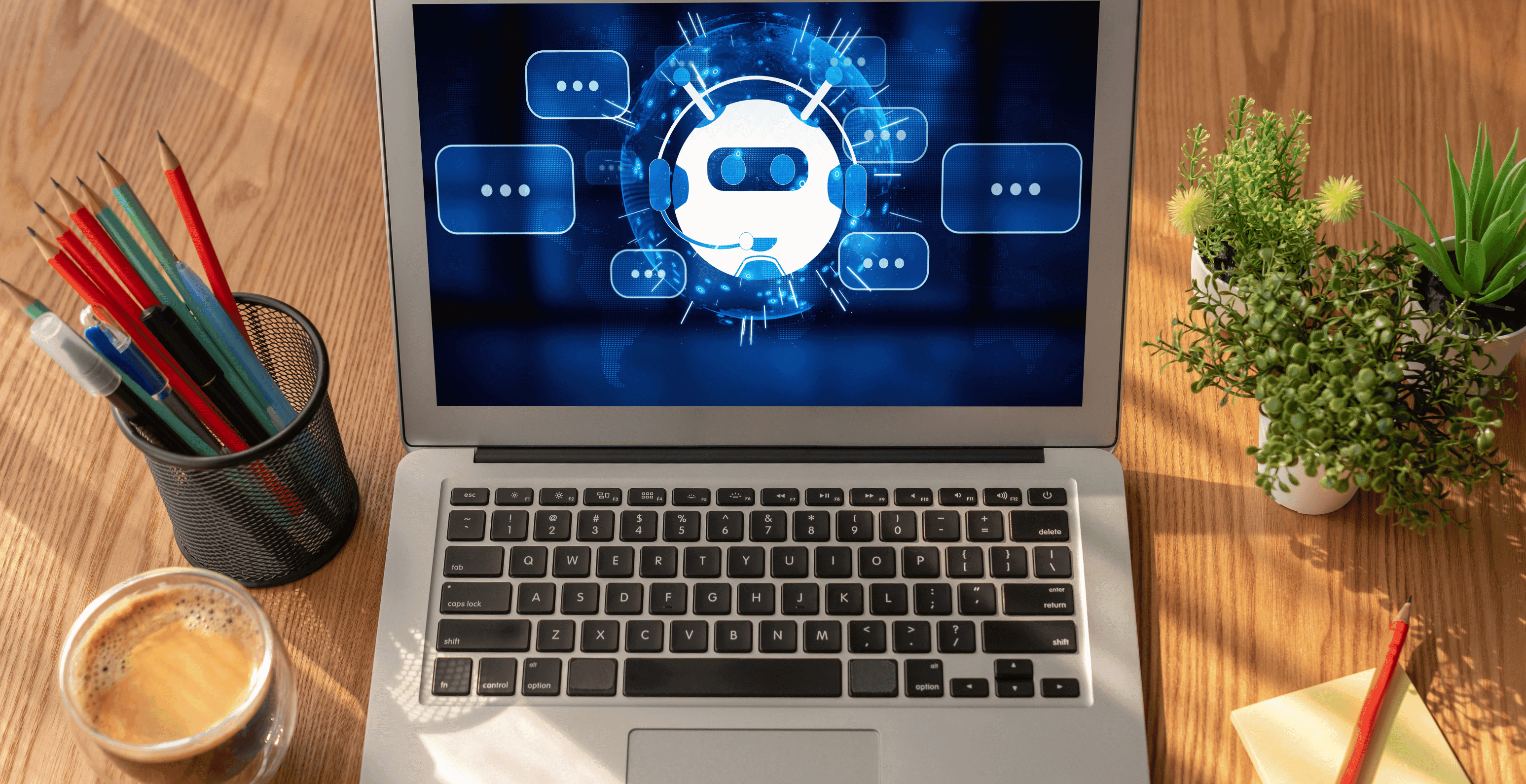
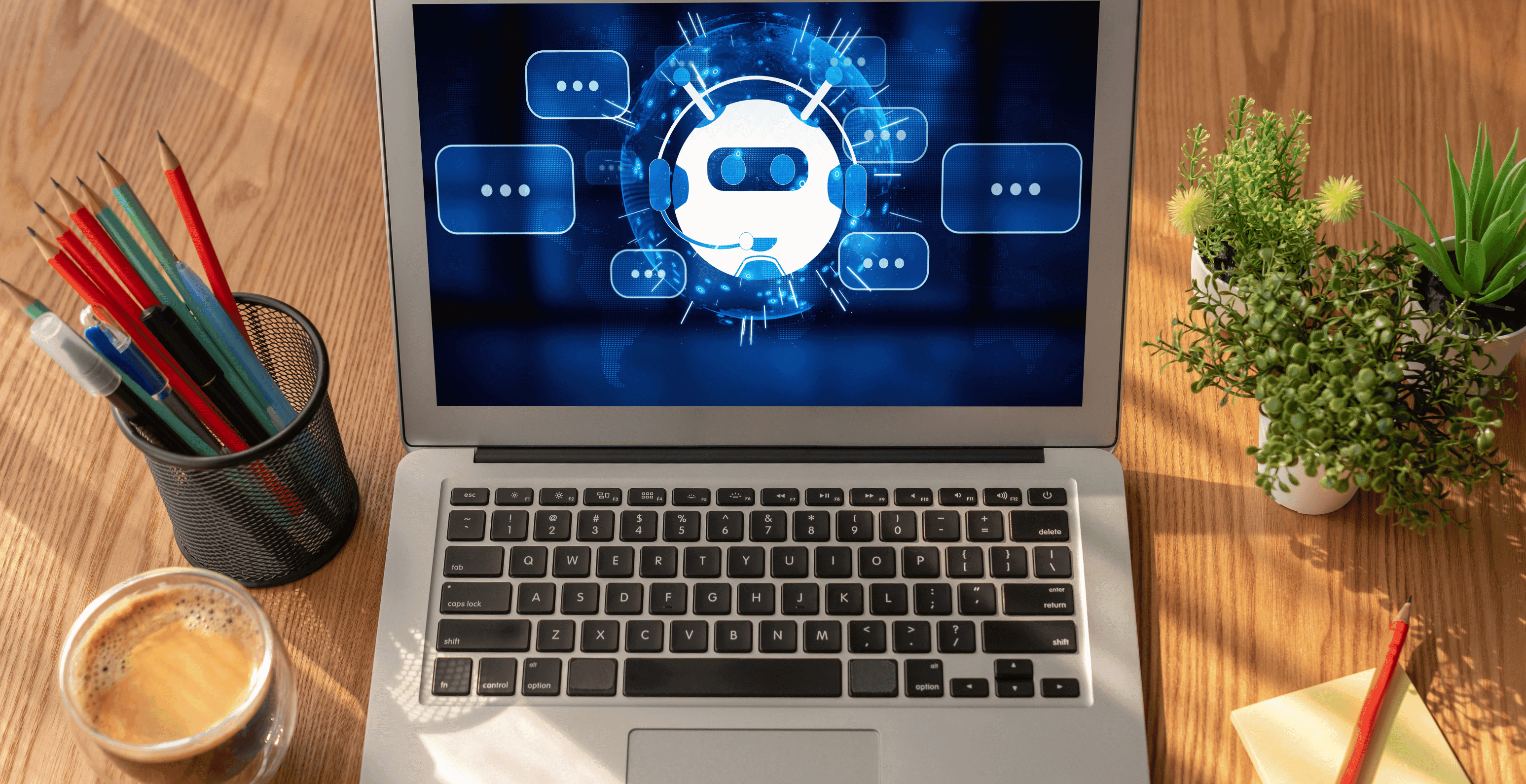
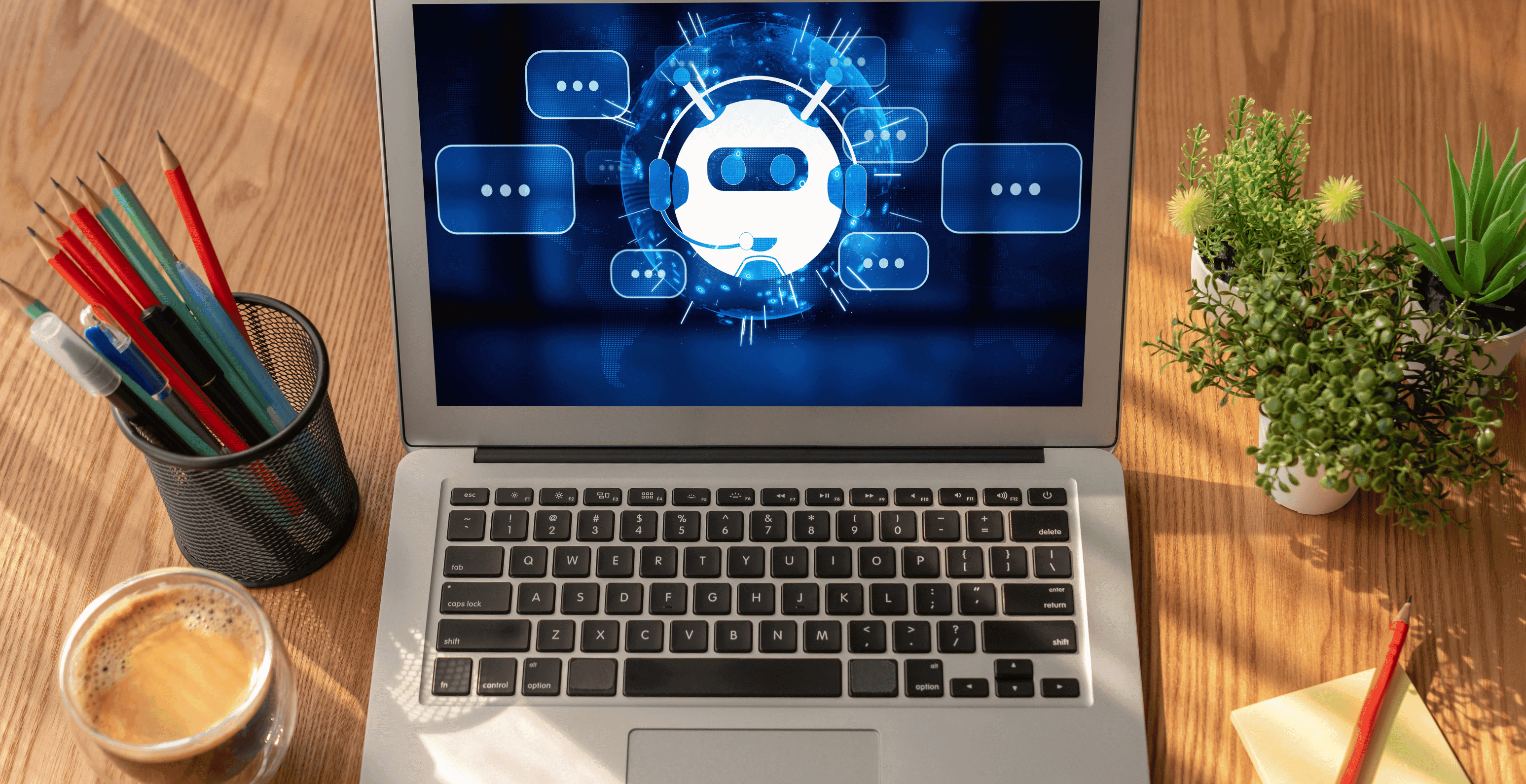
Strategic Approach To Testing AI-Powered Applications And Systems

Rehan Asif
Apr 30, 2024
Read the article




Navigating GDPR Compliance for AI Applications

Rehan Asif
Apr 26, 2024
Read the article
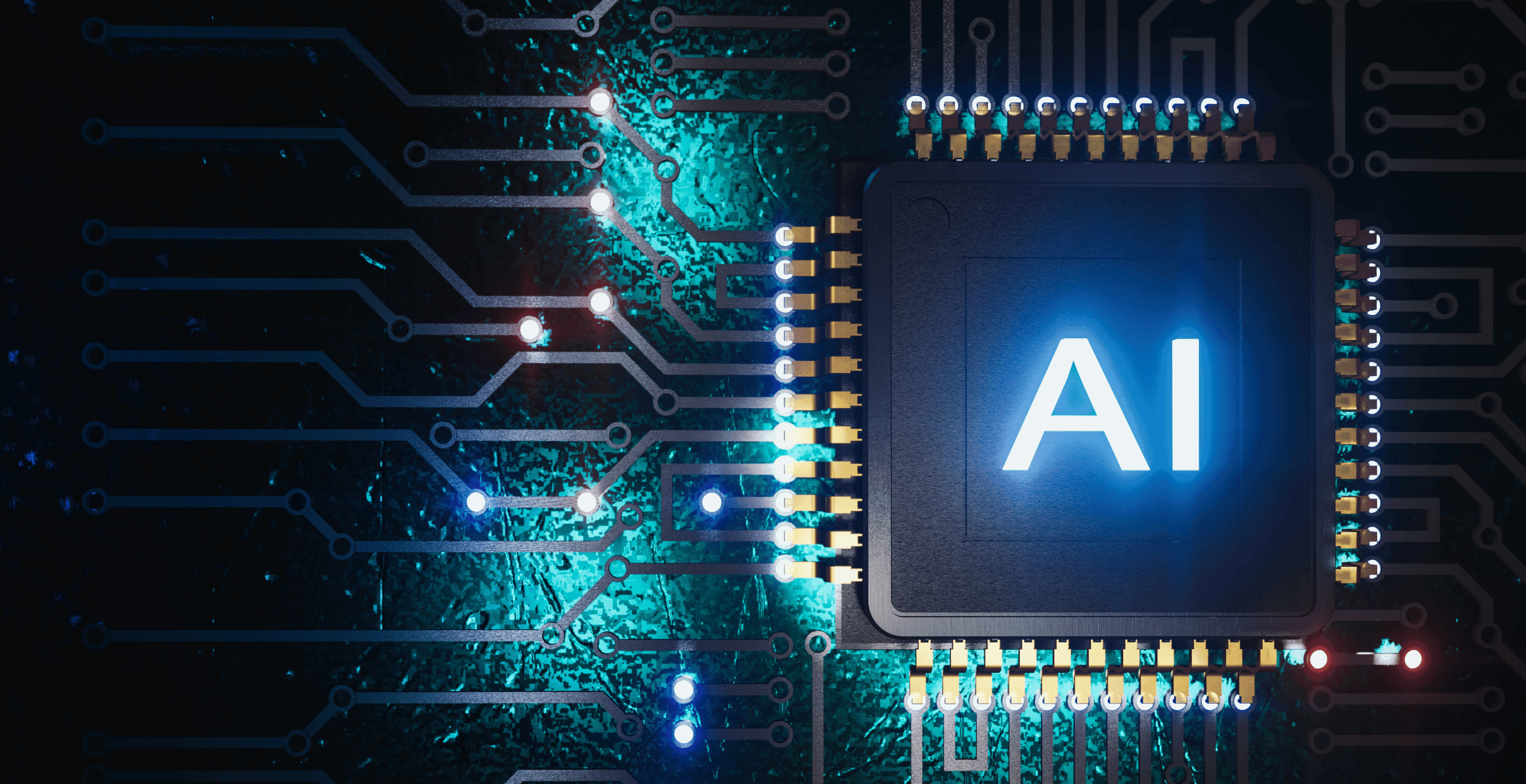
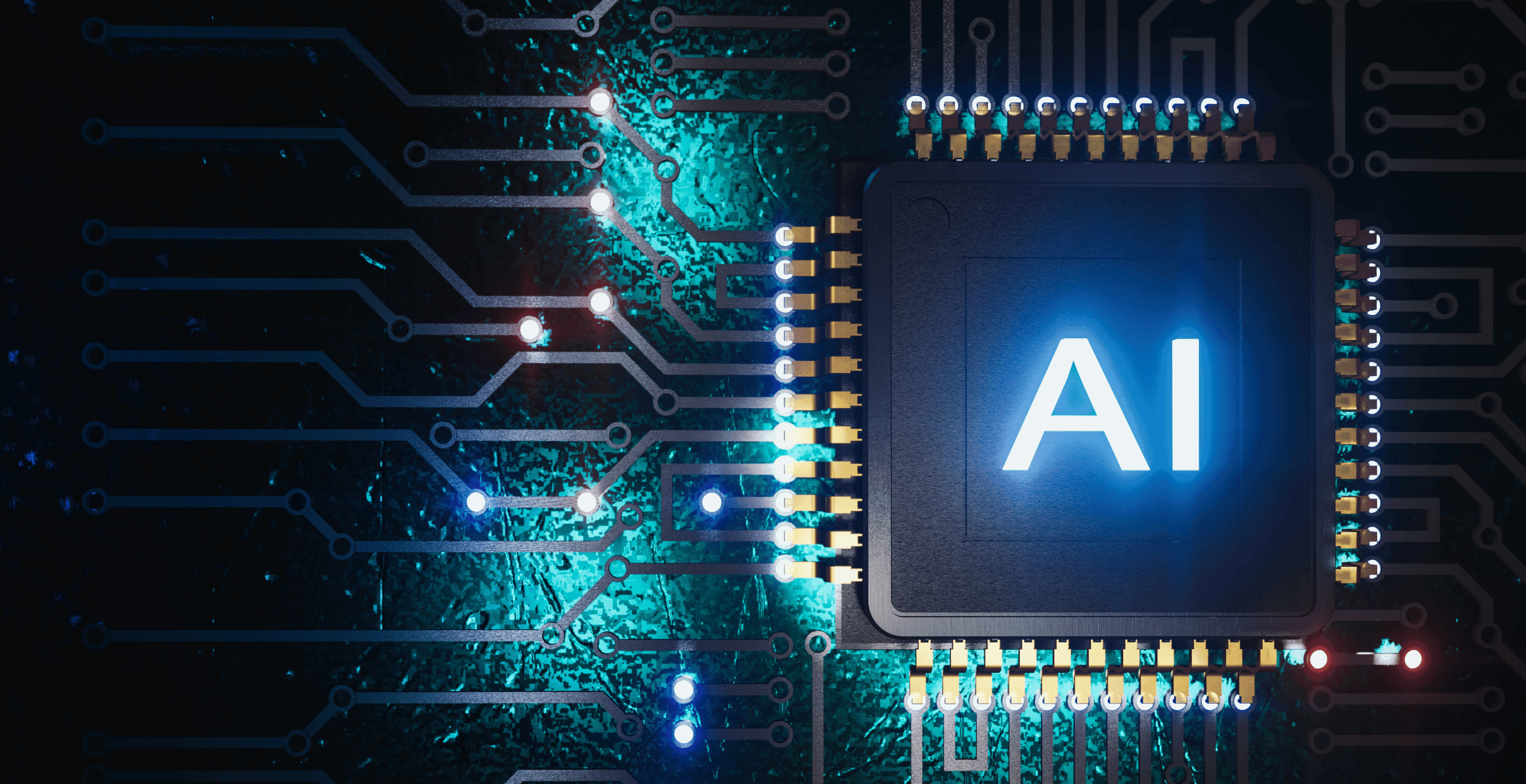
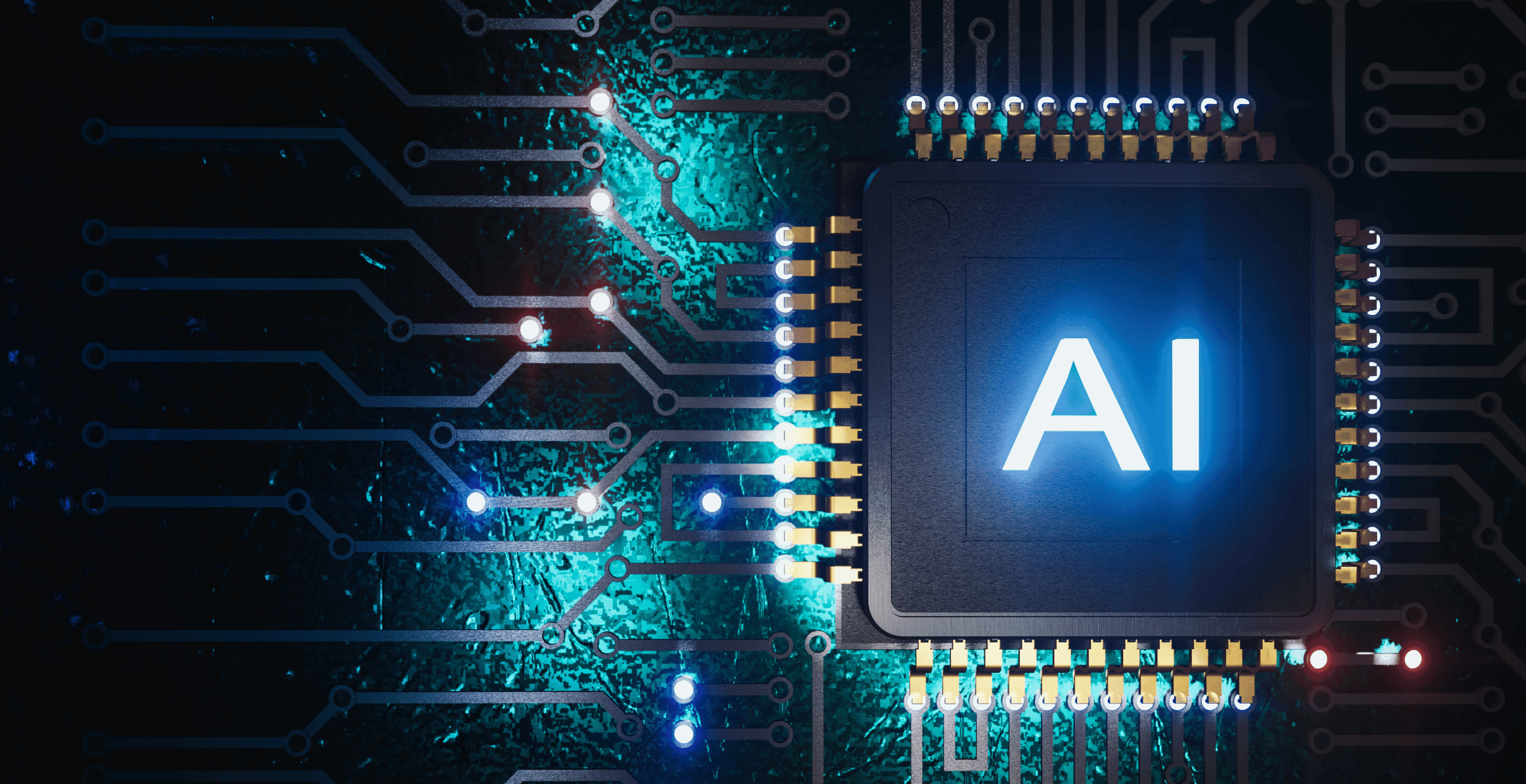
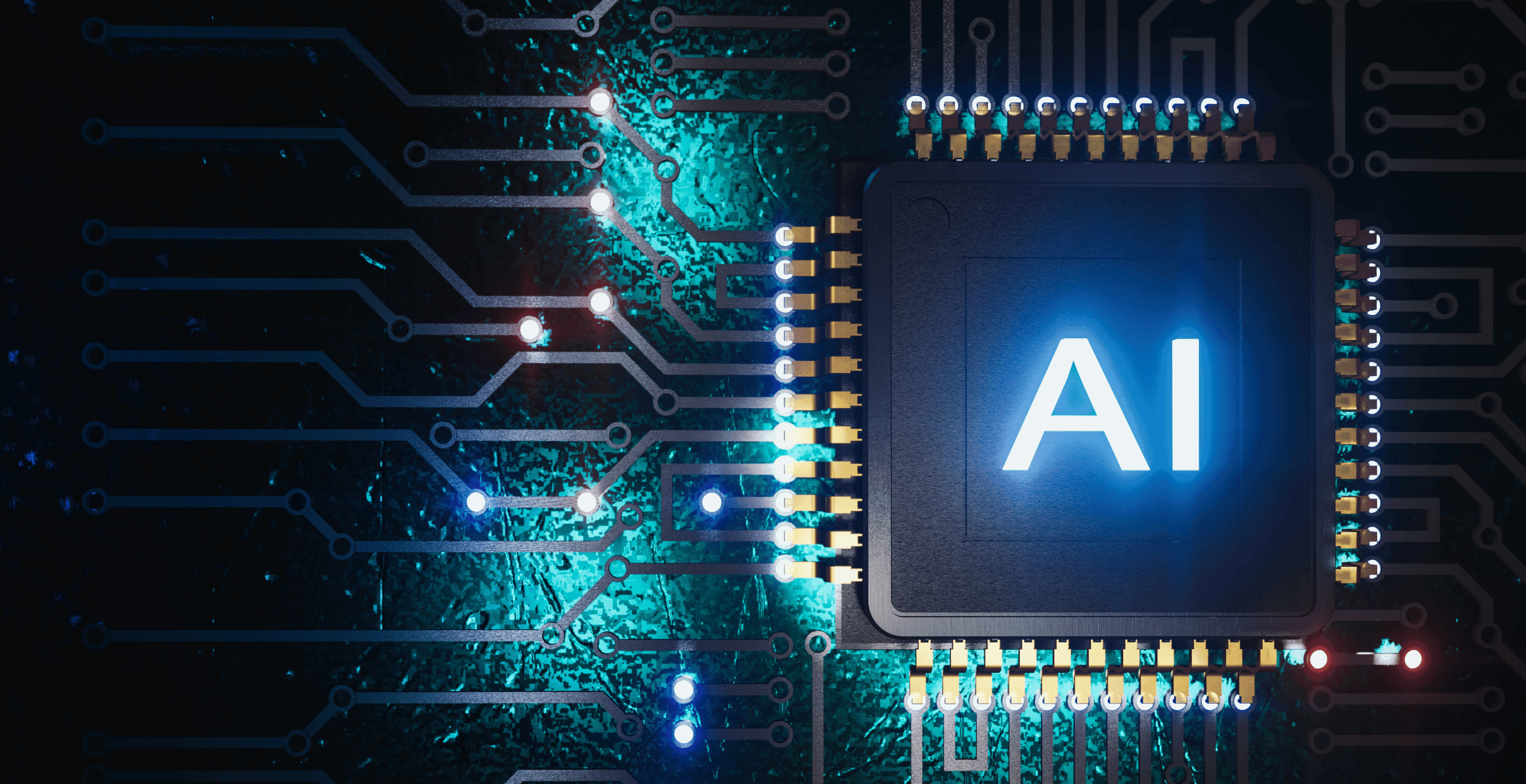
The Impact of AI Governance on Innovation and Development Speed

Rehan Asif
Apr 26, 2024
Read the article
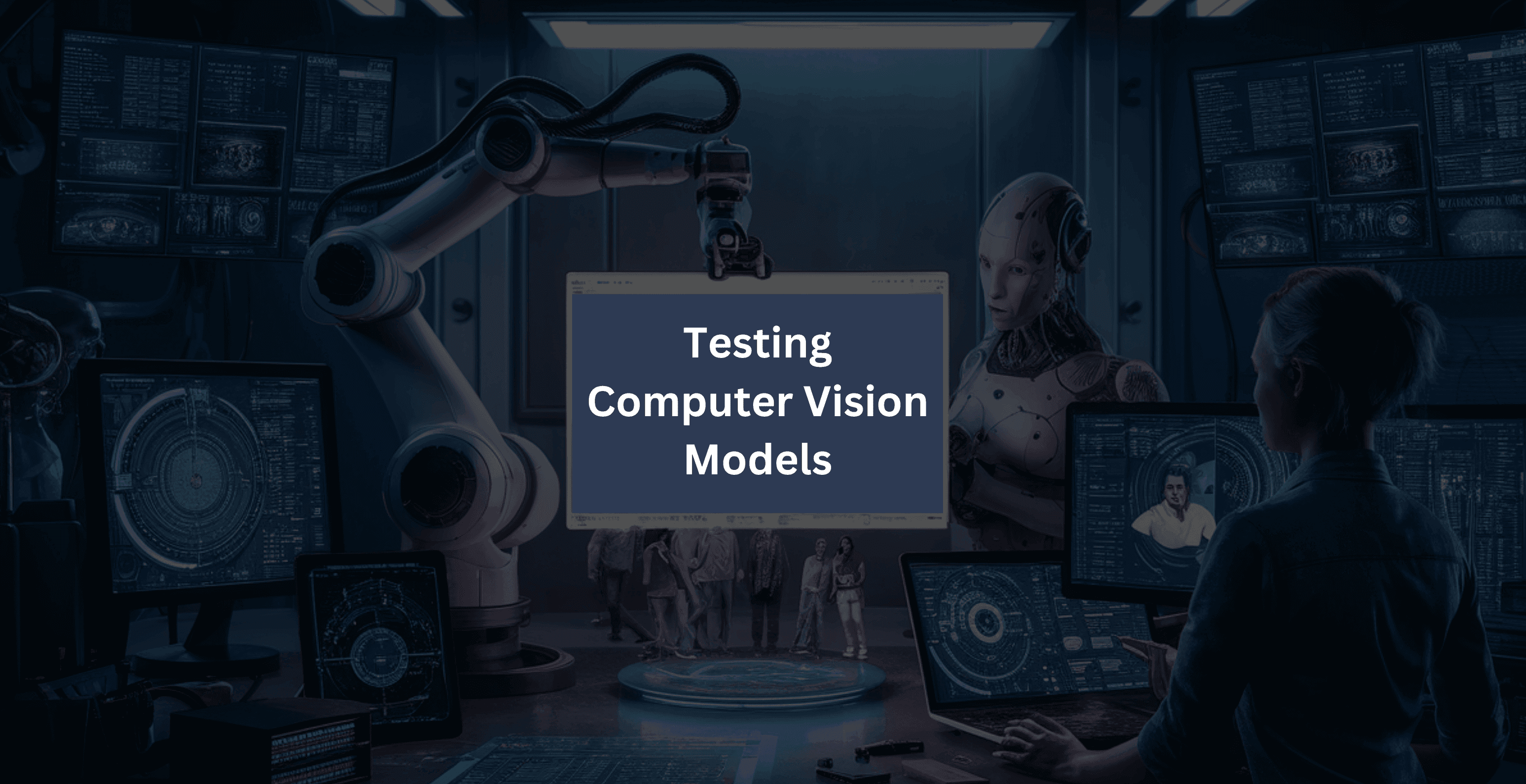
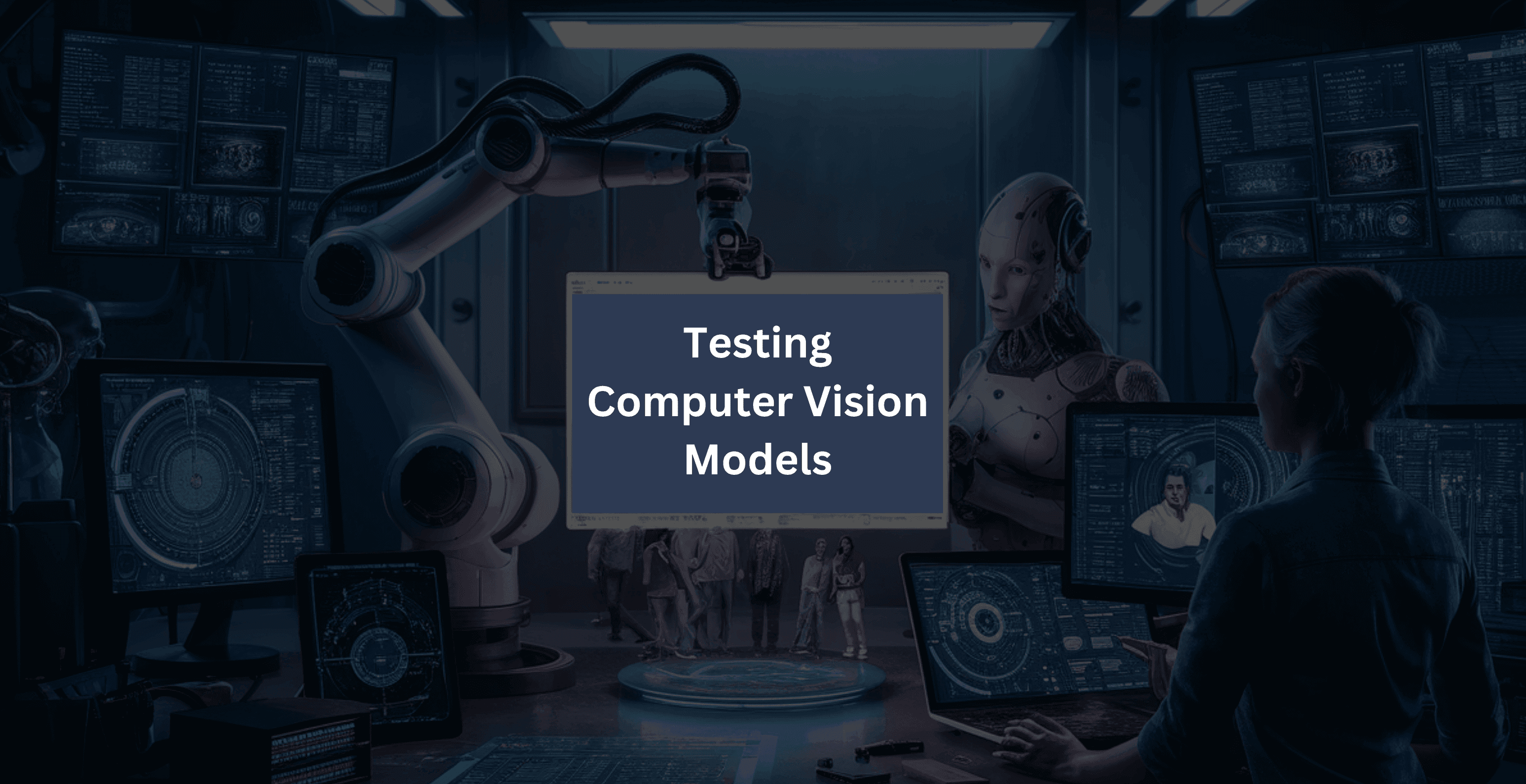
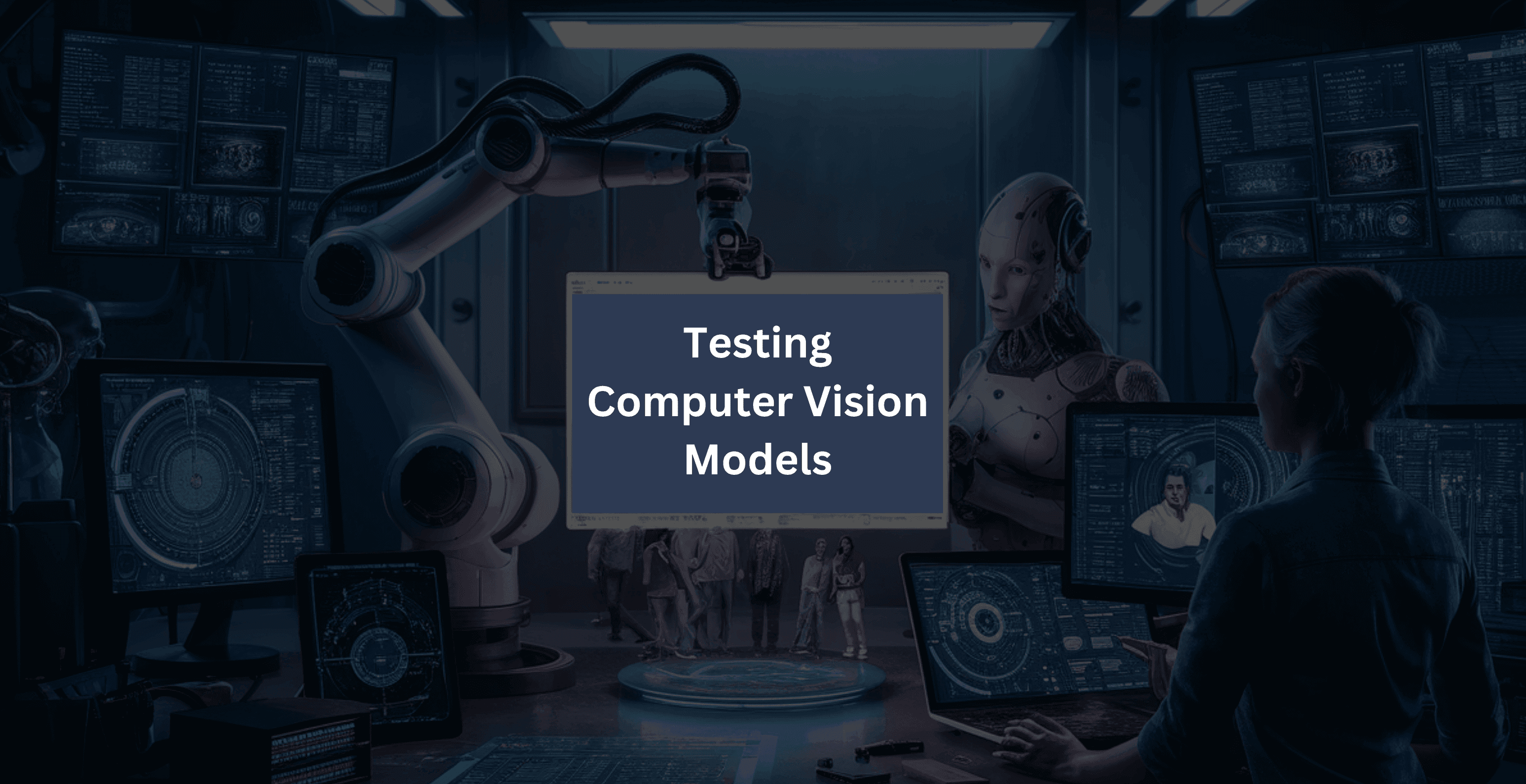
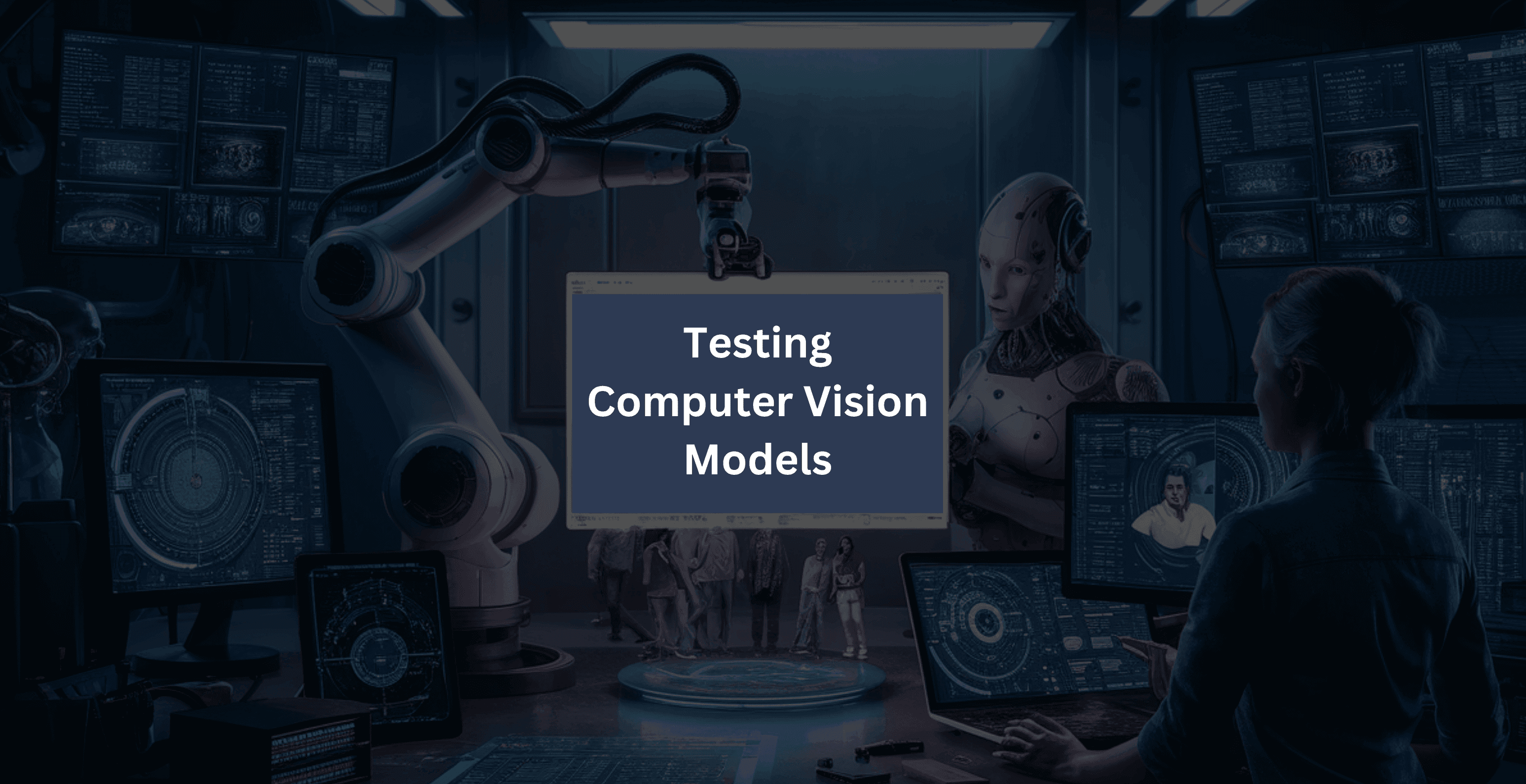
Best Practices For Testing Computer Vision Models
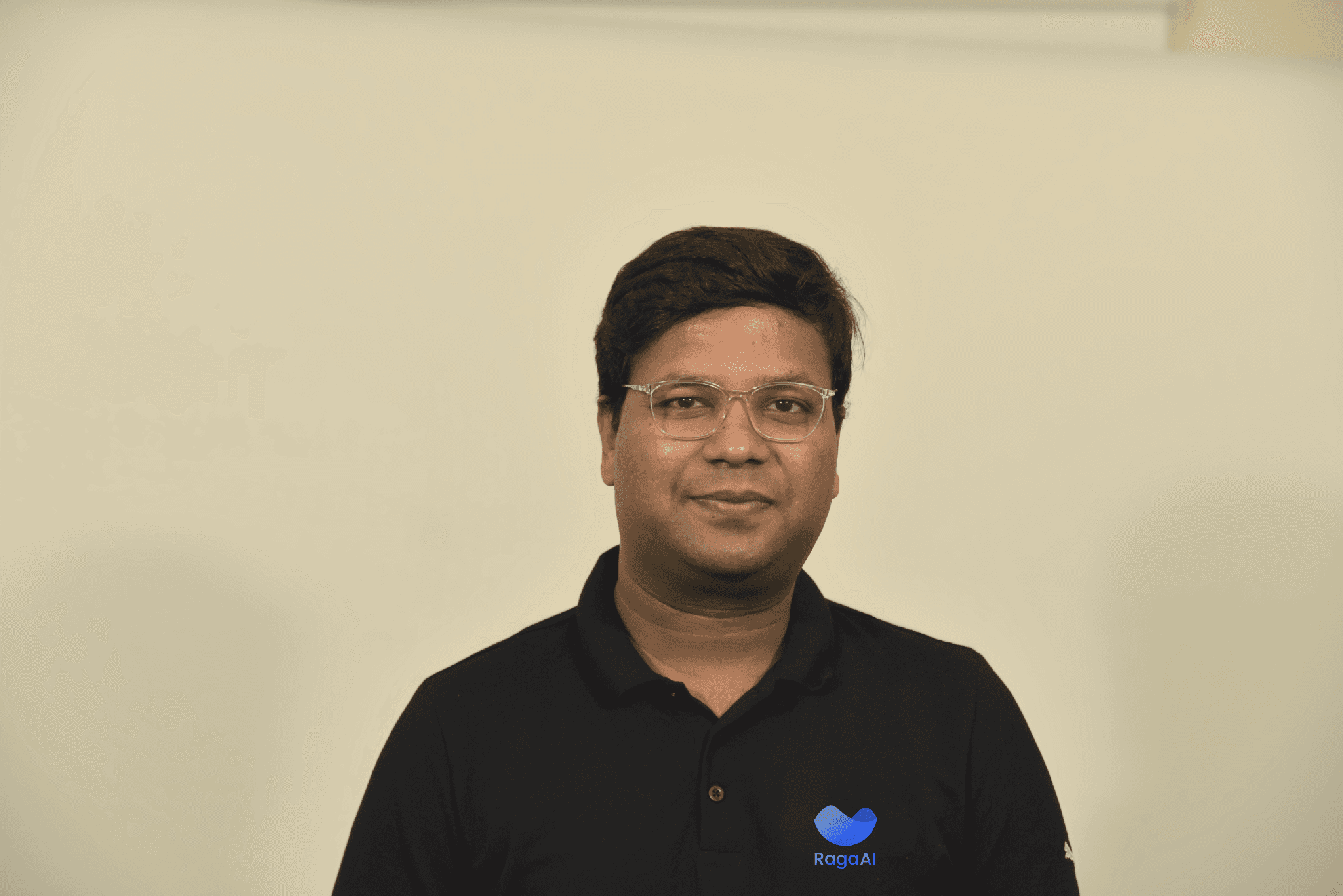
Jigar Gupta
Apr 25, 2024
Read the article
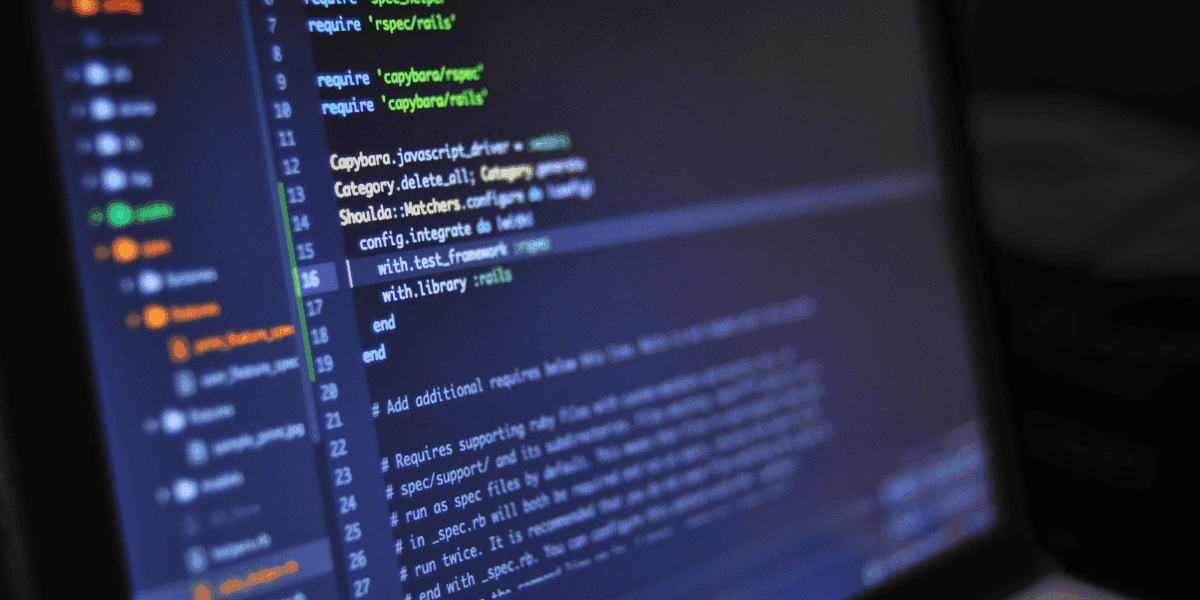
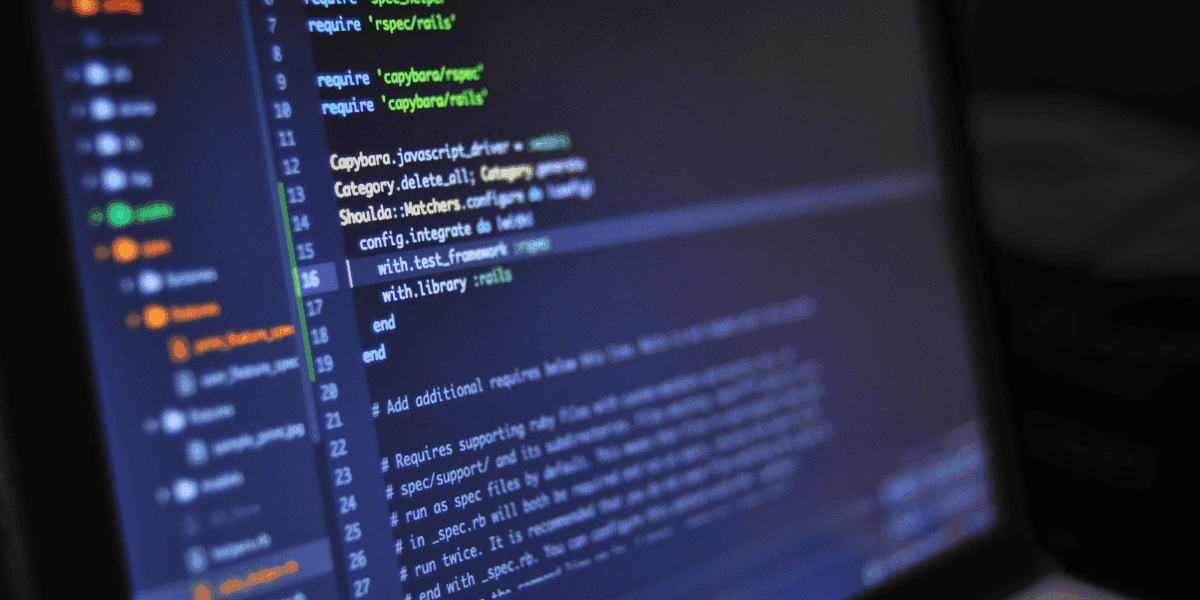
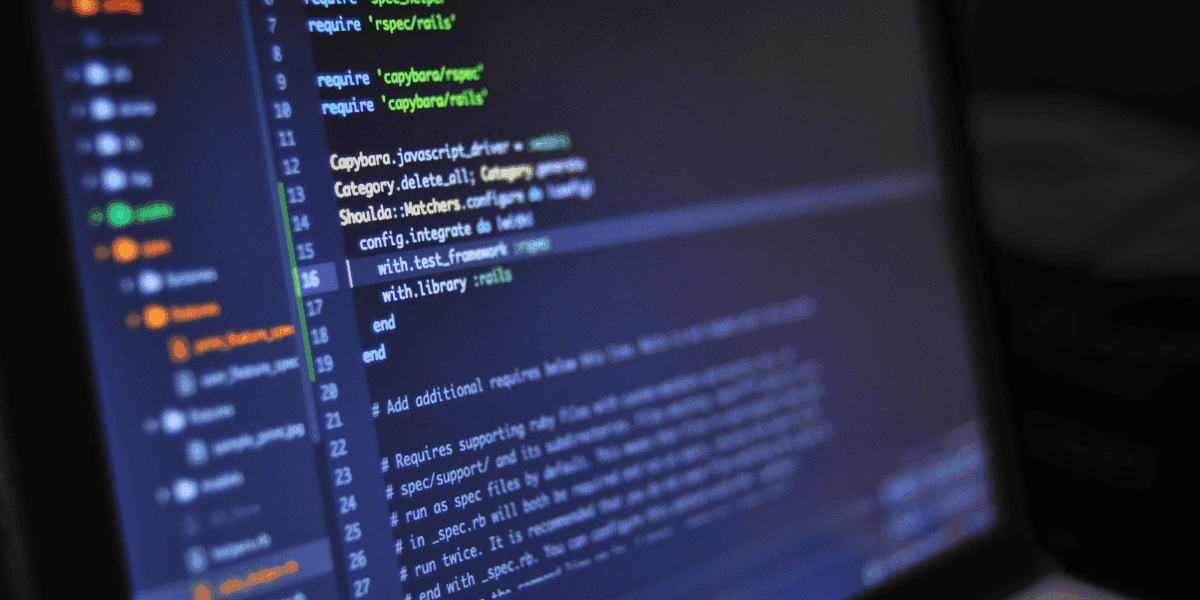
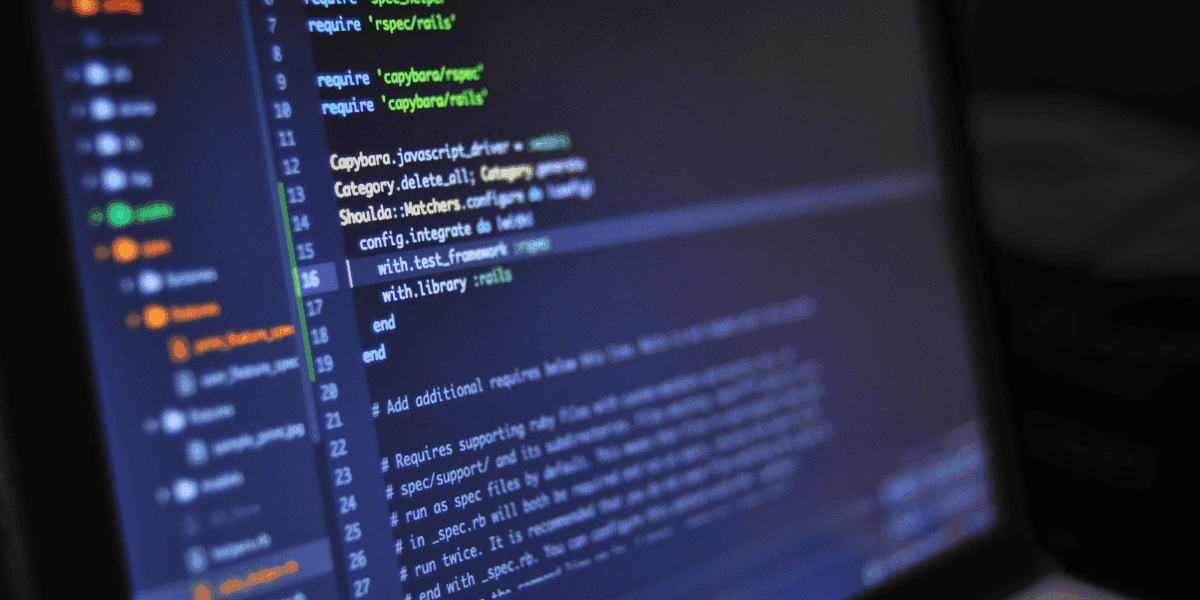
Building Low-Code LLM Apps with Visual Programming

Rehan Asif
Apr 26, 2024
Read the article




Understanding AI regulations In Finance

Akshat Gupta
Apr 26, 2024
Read the article




Compliance Automation: Getting Started with Regulatory Management

Akshat Gupta
Apr 25, 2024
Read the article
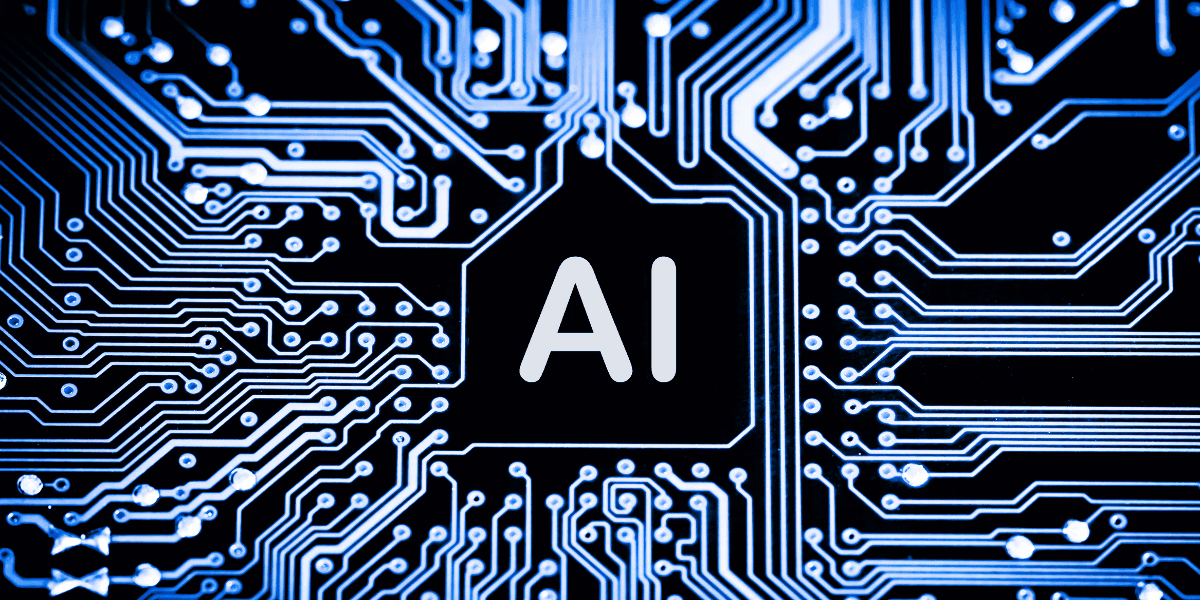
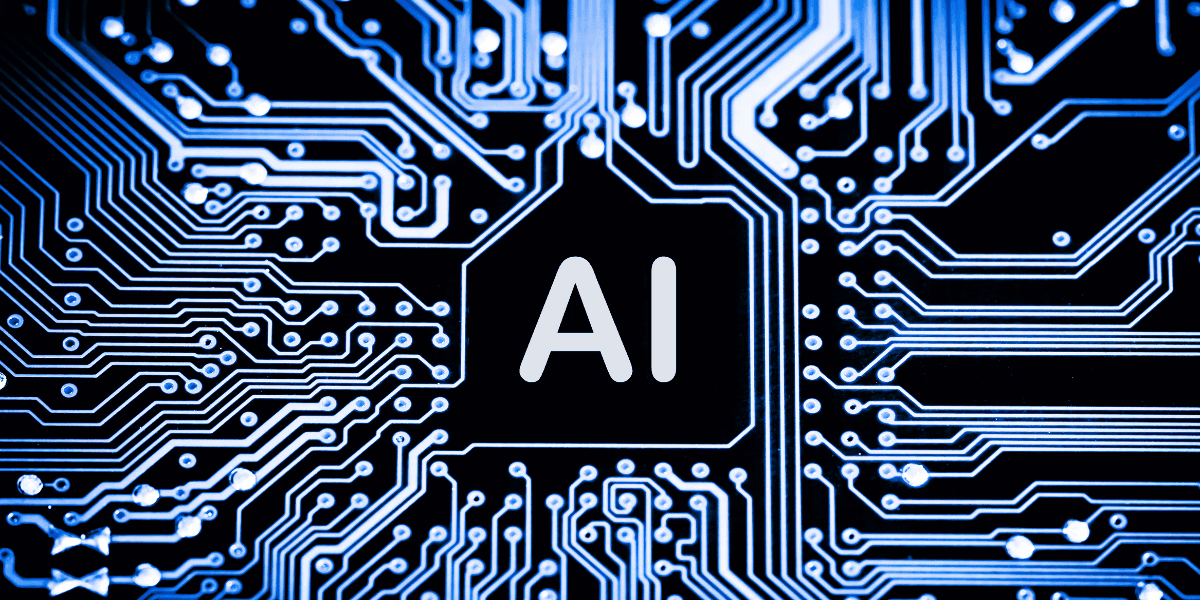
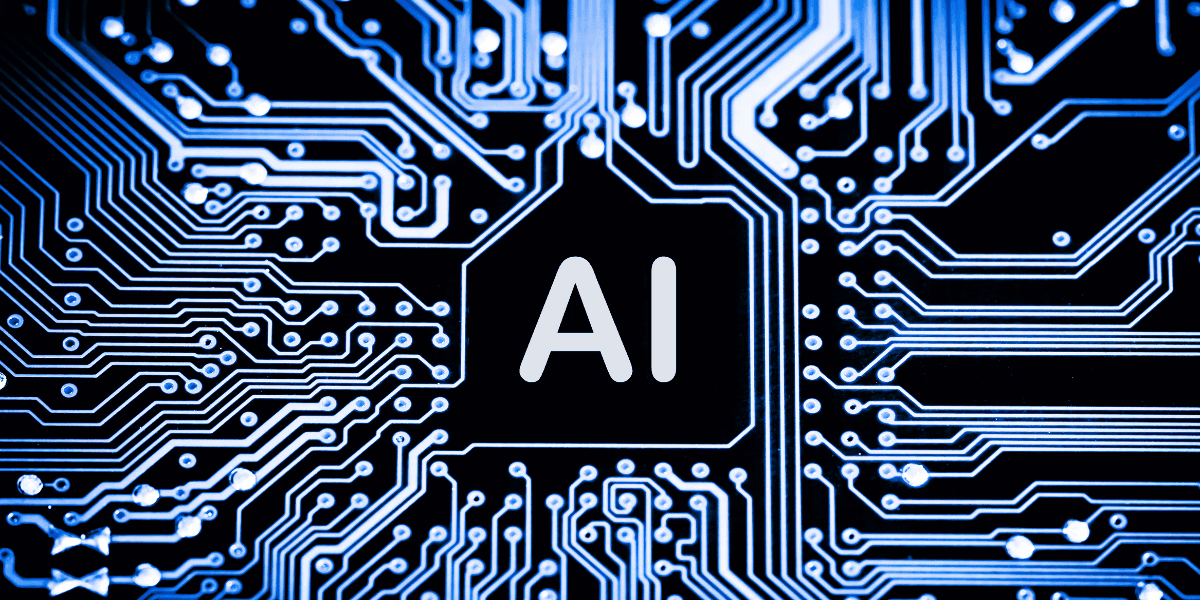
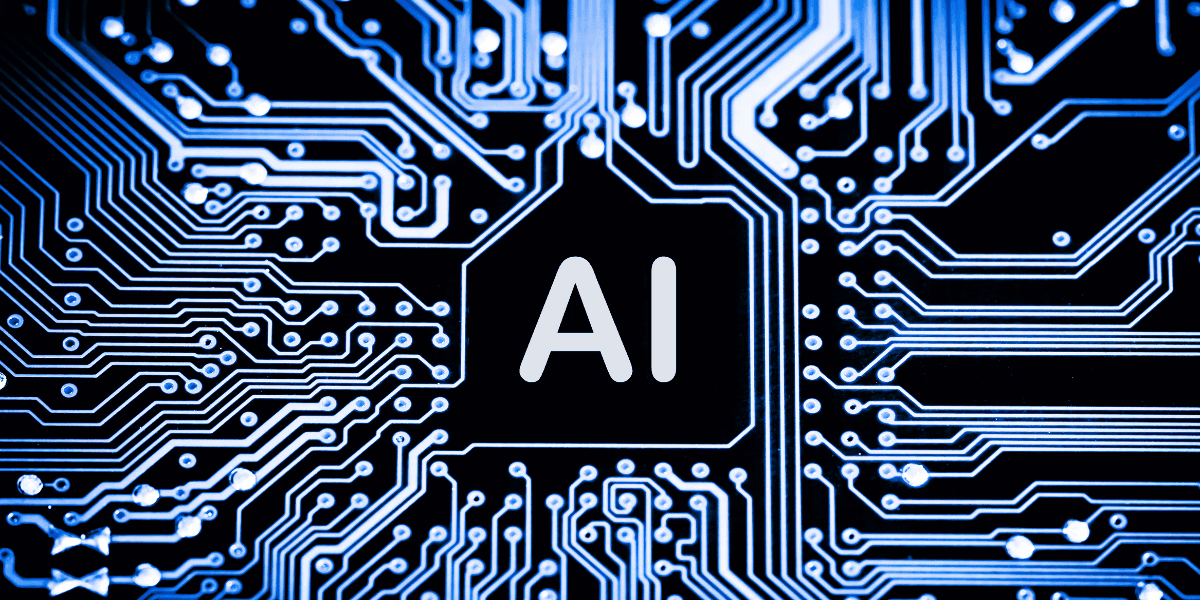
Practical Guide to Fine-Tuning OpenAI GPT Models Using Python

Rehan Asif
Apr 24, 2024
Read the article




Comparing Different Large Language Models (LLM)

Rehan Asif
Apr 23, 2024
Read the article




Evaluating Large Language Models: Methods And Metrics

Rehan Asif
Apr 22, 2024
Read the article




Significant AI Errors, Mistakes, Failures, and Flaws Companies Encounter

Akshat Gupta
Apr 21, 2024
Read the article




Challenges and Strategies for Implementing Enterprise LLM

Rehan Asif
Apr 20, 2024
Read the article




Enhancing Computer Vision with Synthetic Data: Advantages and Generation Techniques
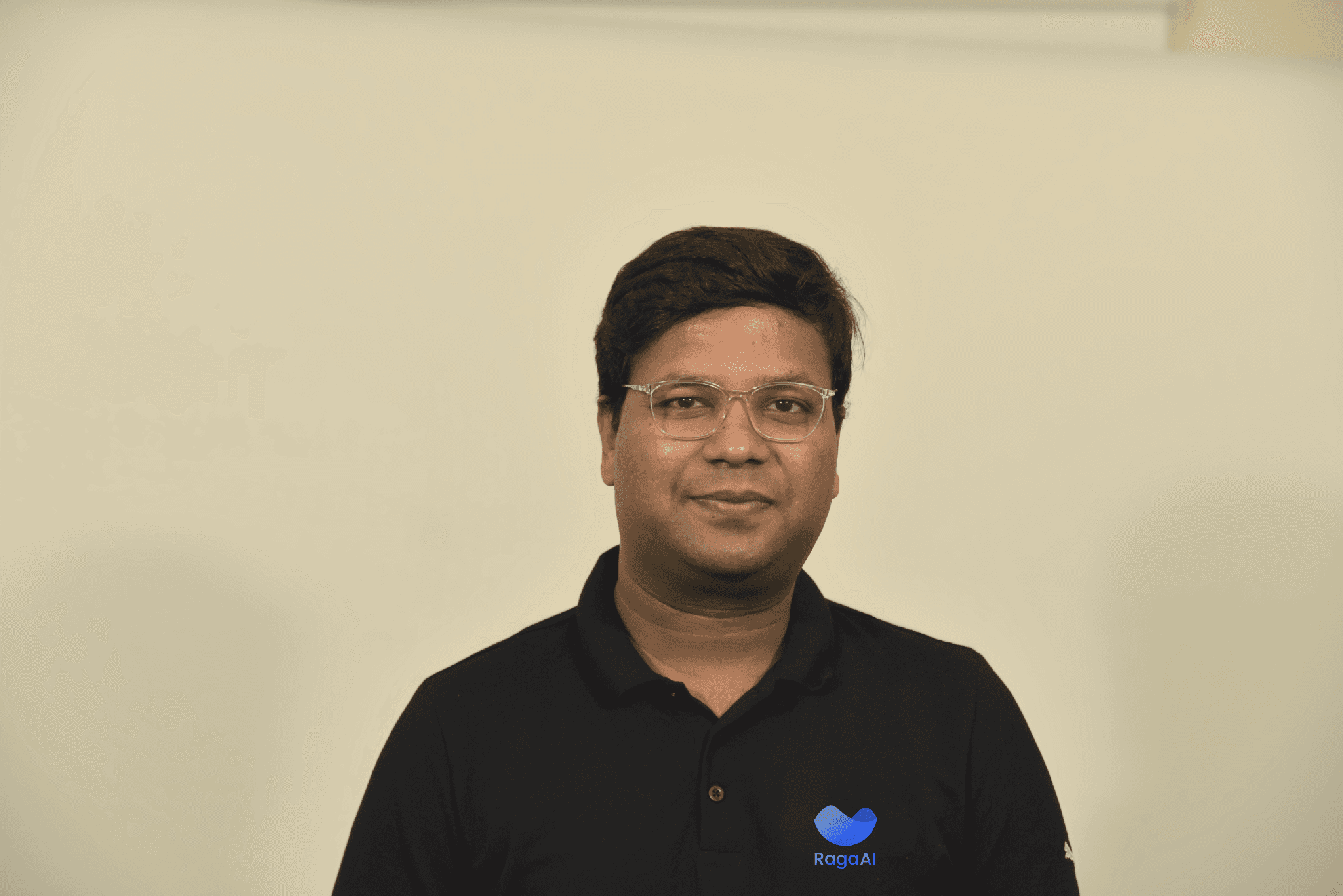
Jigar Gupta
Apr 20, 2024
Read the article
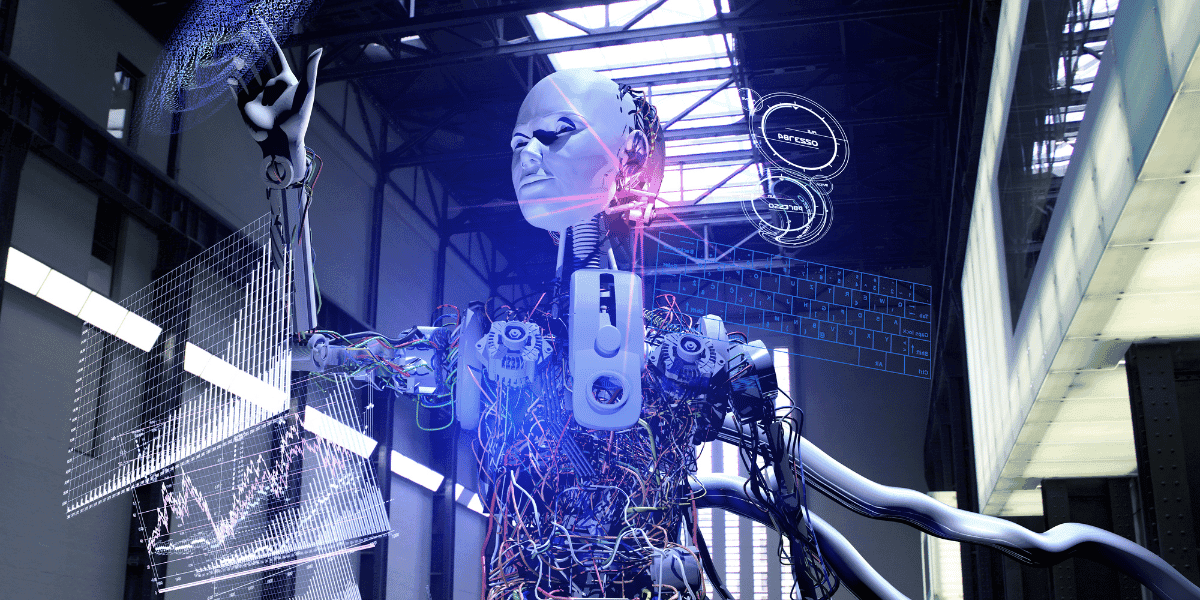
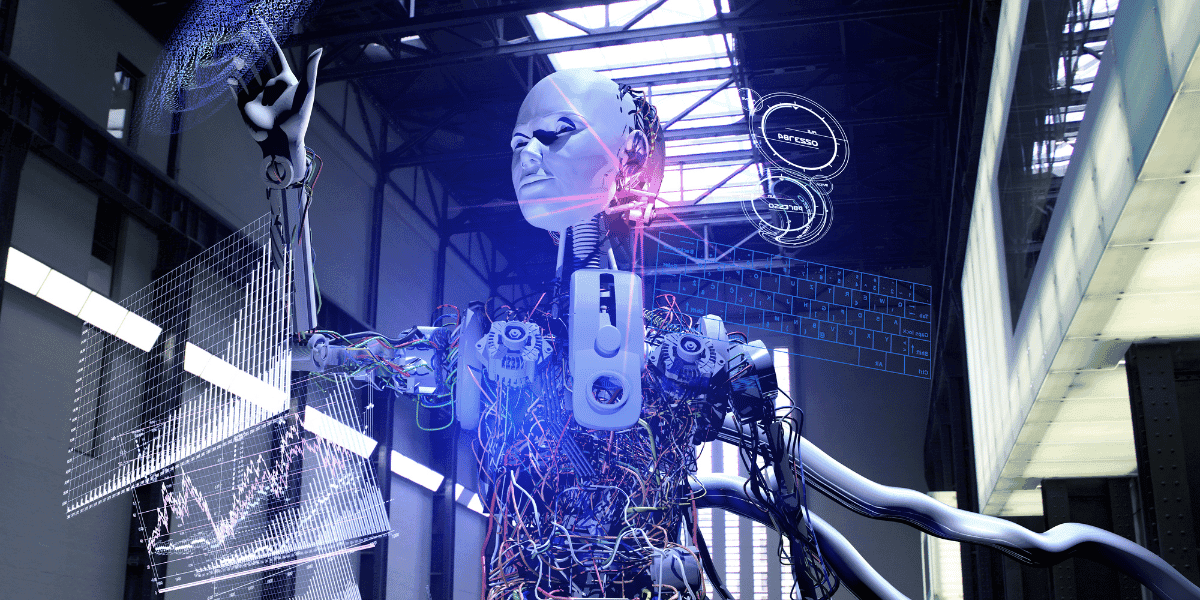
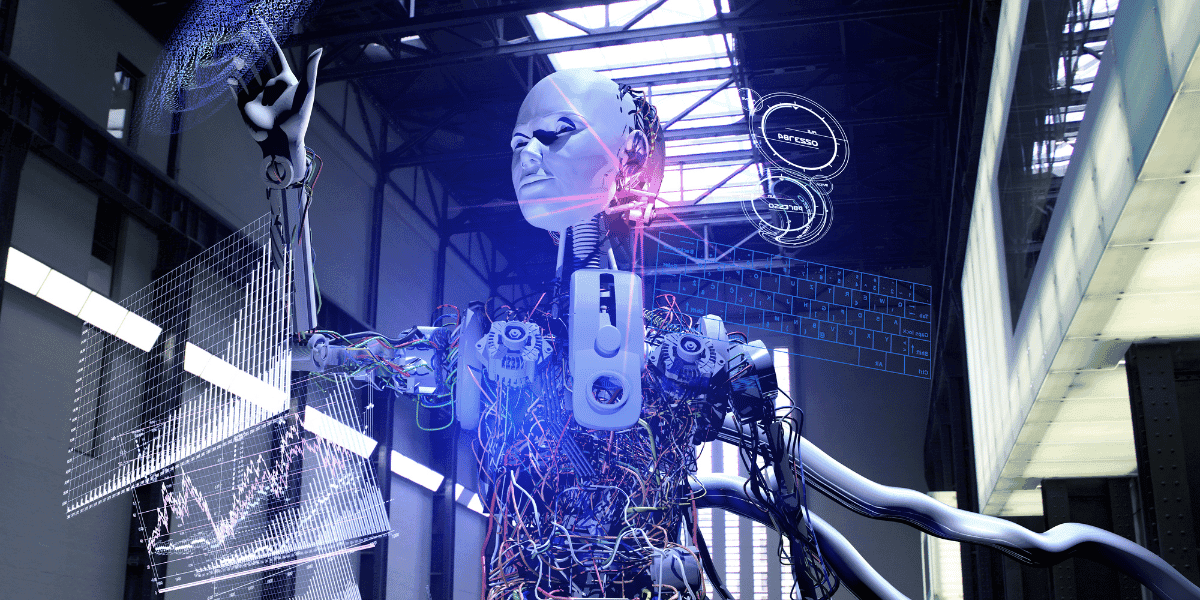
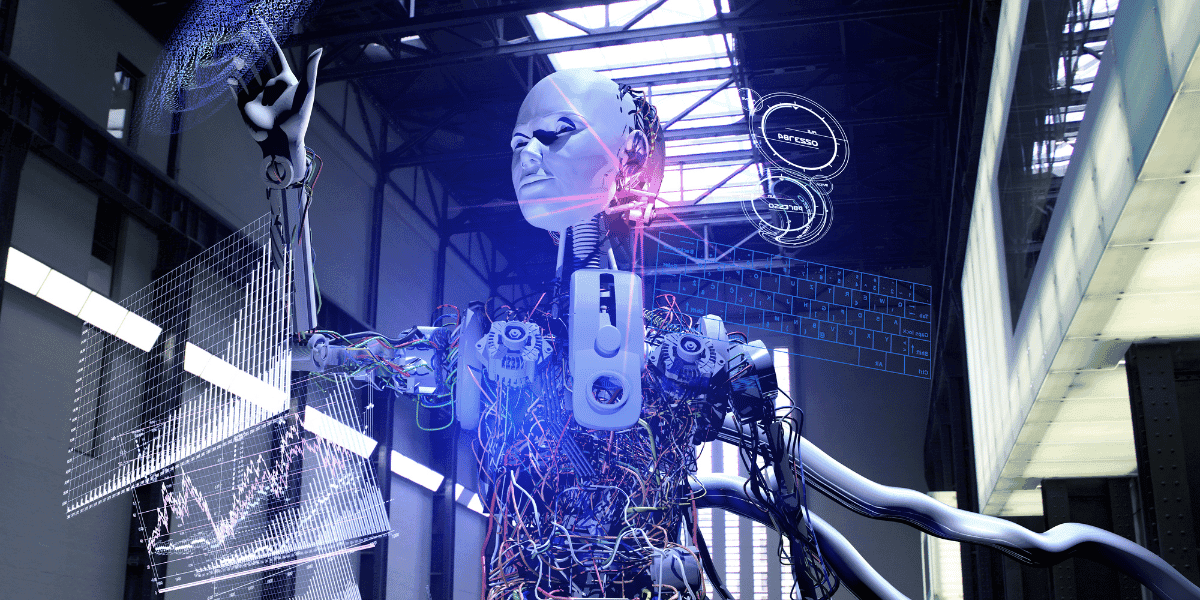
Building Trust In Artificial Intelligence Systems

Akshat Gupta
Apr 19, 2024
Read the article




A Brief Guide To LLM Parameters: Tuning and Optimization

Rehan Asif
Apr 18, 2024
Read the article




Unlocking The Potential Of Computer Vision Testing: Key Techniques And Tools
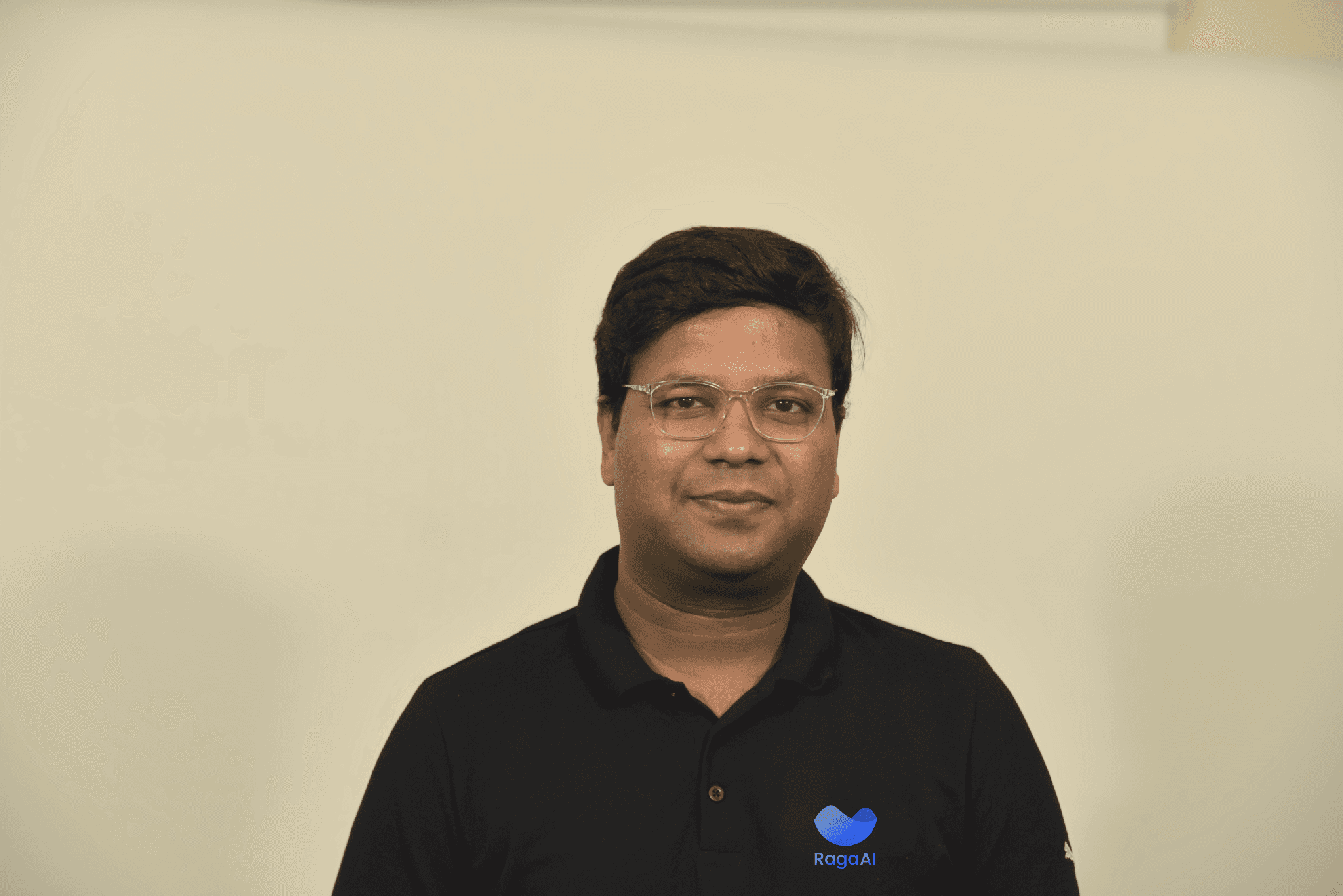
Jigar Gupta
Apr 17, 2024
Read the article




Understanding AI Regulatory Compliance And Its Importance

Akshat Gupta
Apr 16, 2024
Read the article
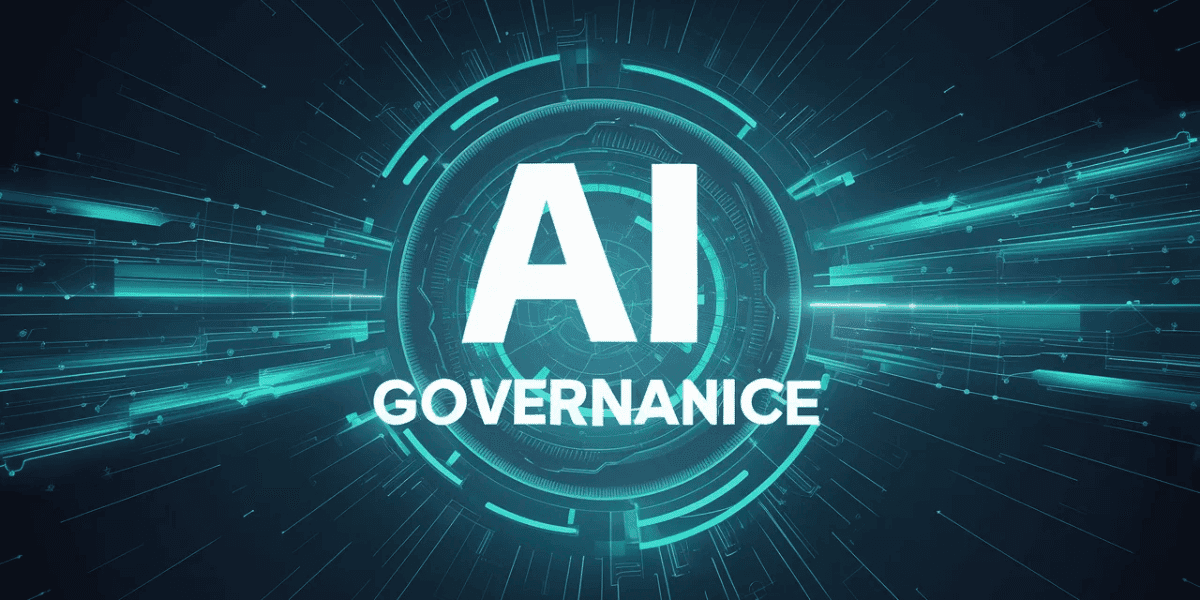
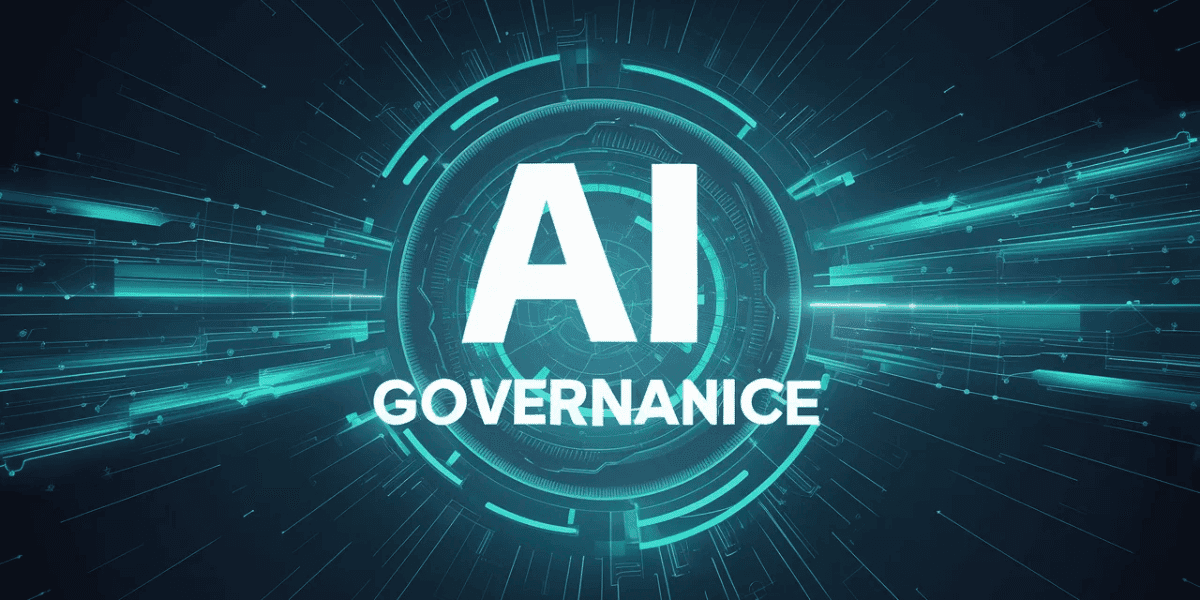
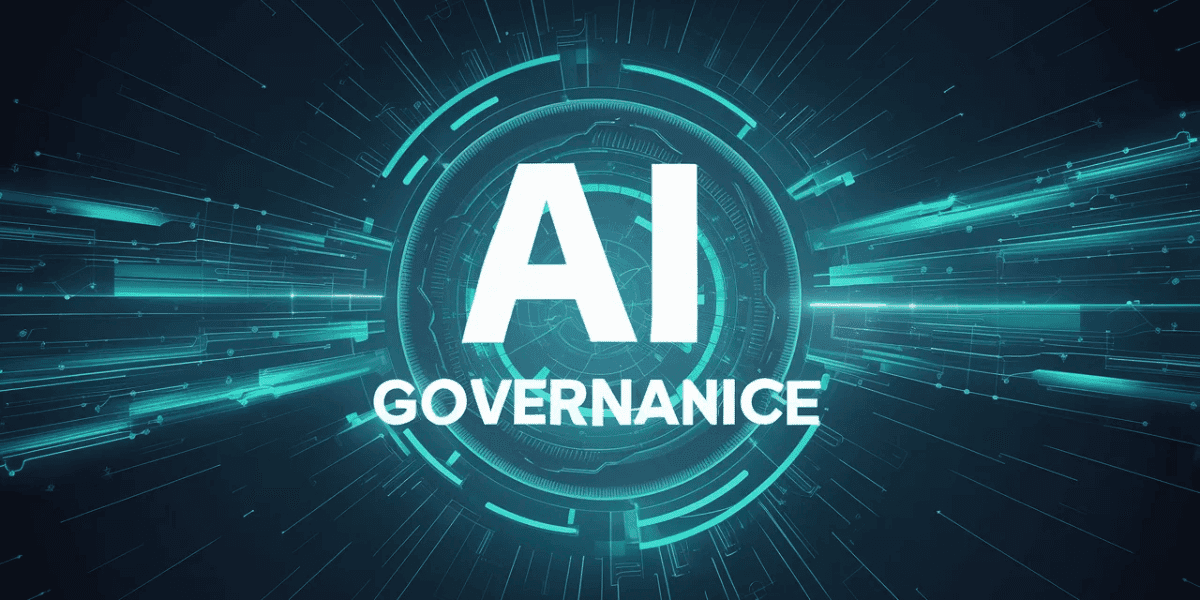
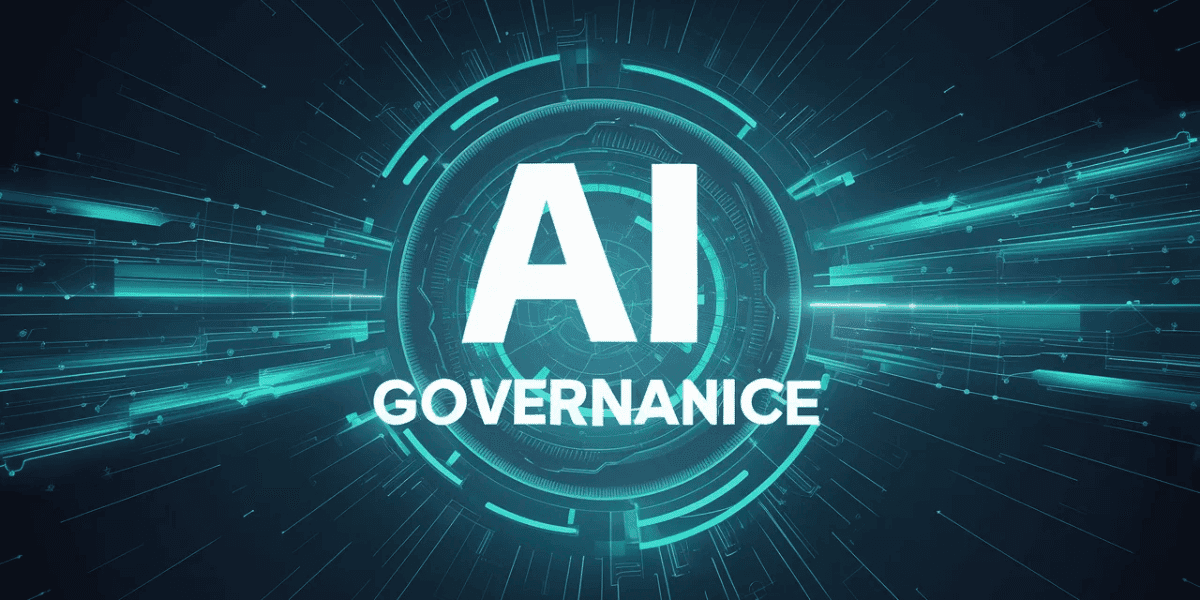
Understanding The Basics Of AI Governance

Akshat Gupta
Apr 15, 2024
Read the article




Understanding Prompt Engineering: A Guide

Rehan Asif
Apr 15, 2024
Read the article
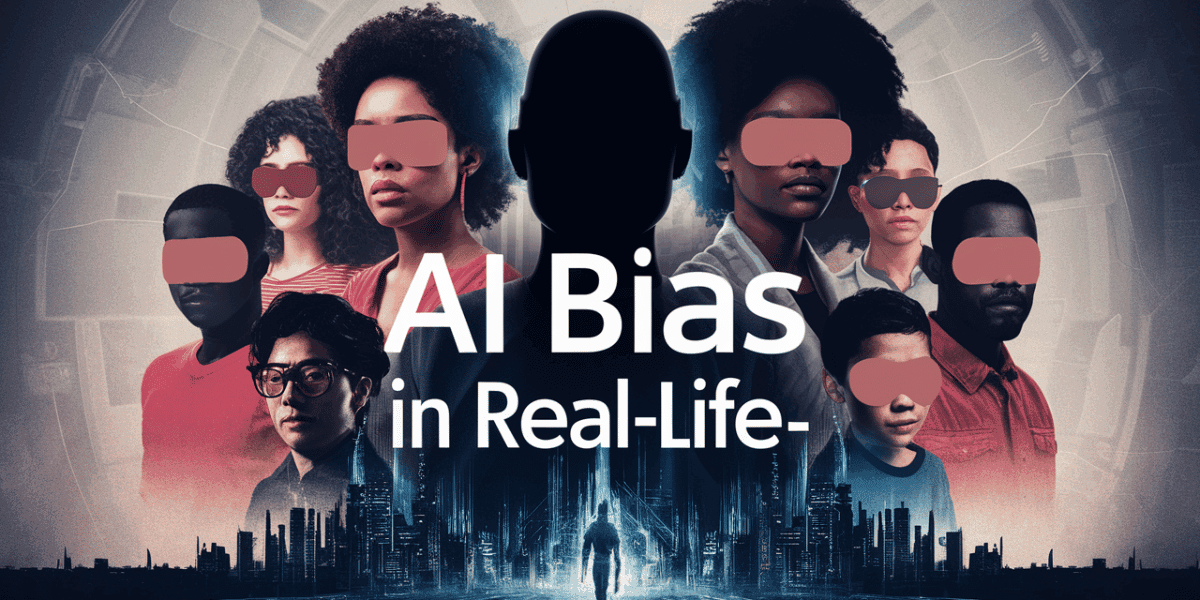
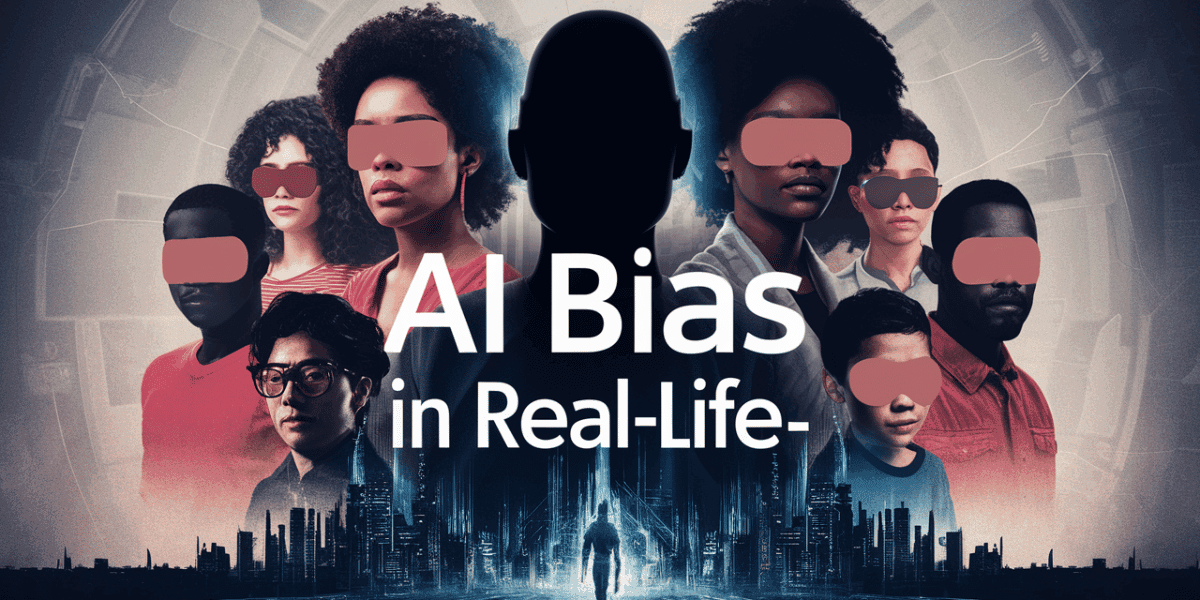
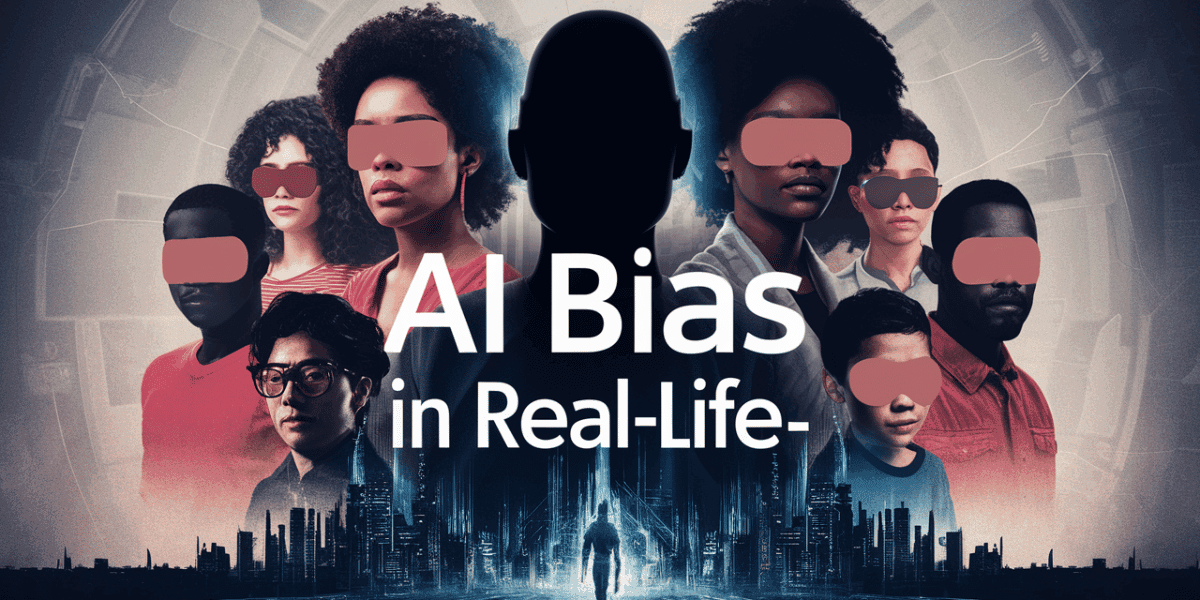
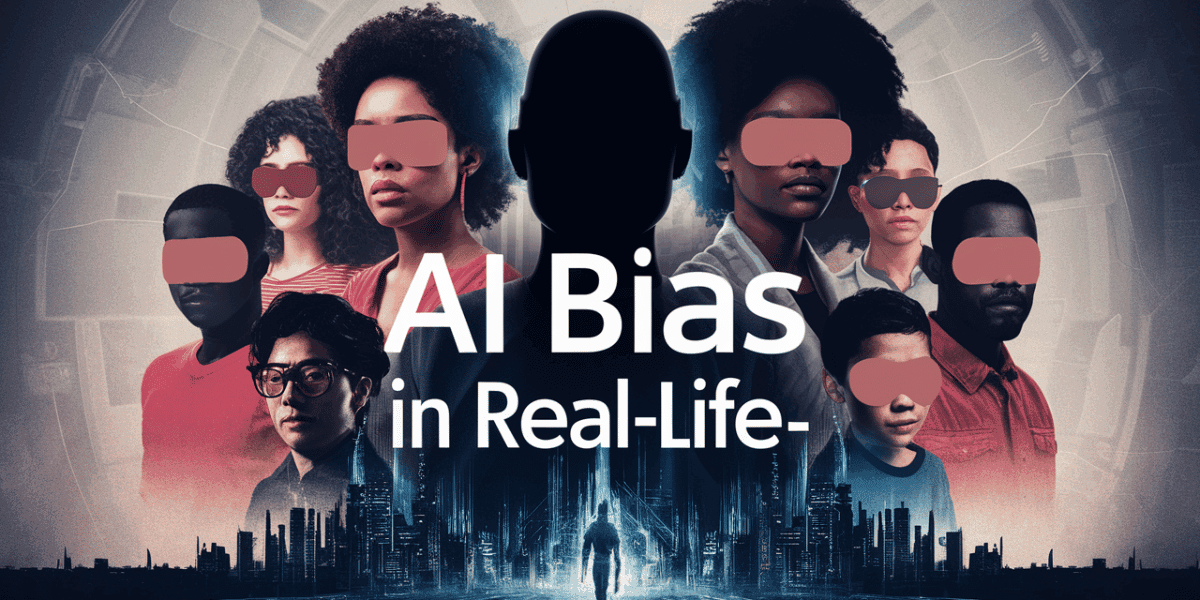
Examples And Strategies To Mitigate AI Bias In Real-Life

Akshat Gupta
Apr 14, 2024
Read the article




Understanding The Basics Of LLM Fine-tuning With Custom Data

Rehan Asif
Apr 13, 2024
Read the article
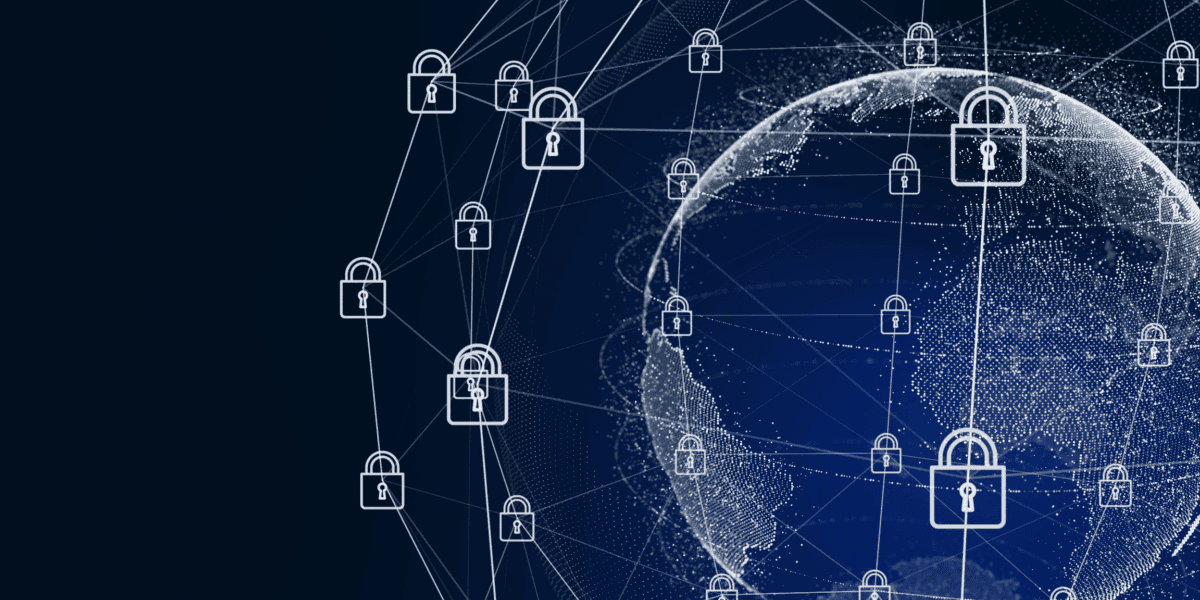
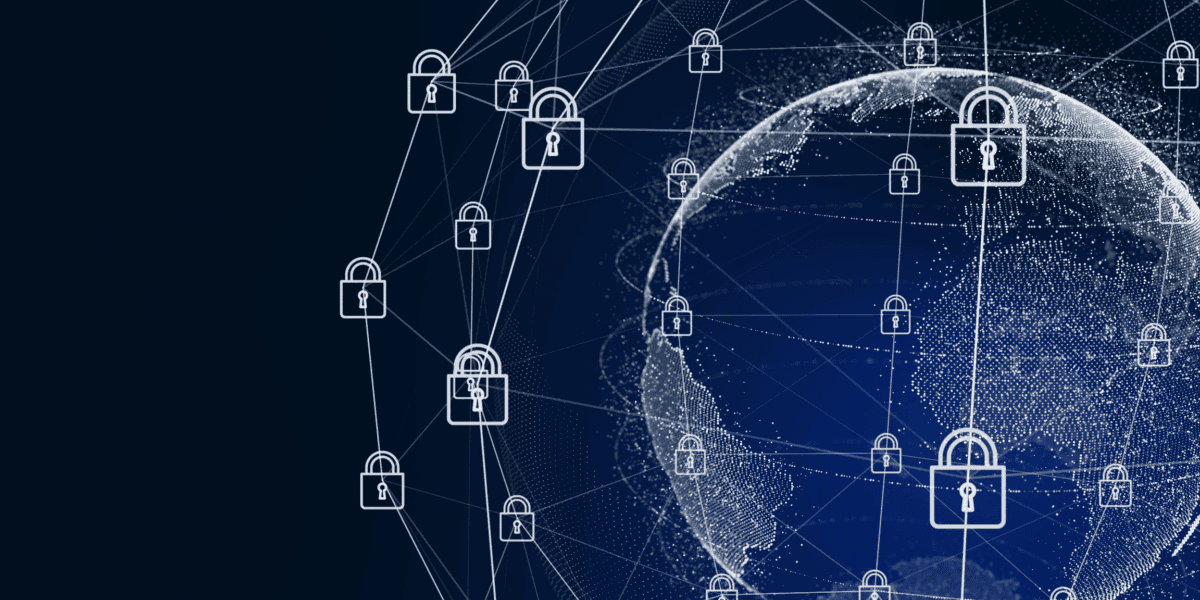
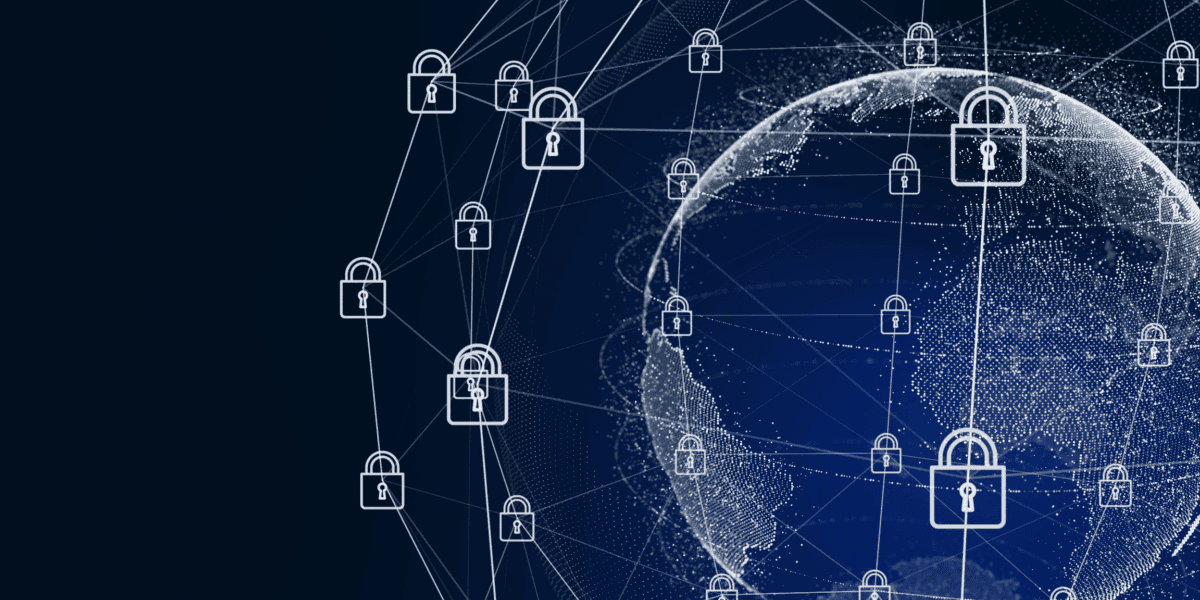
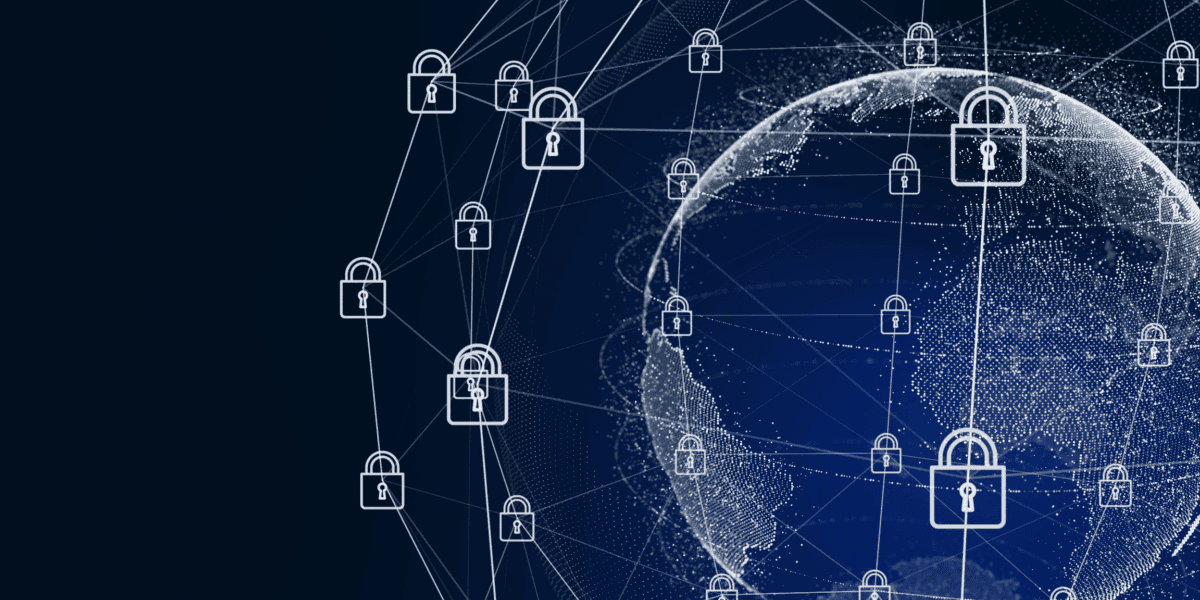
Overview Of Key Concepts In AI Safety And Security
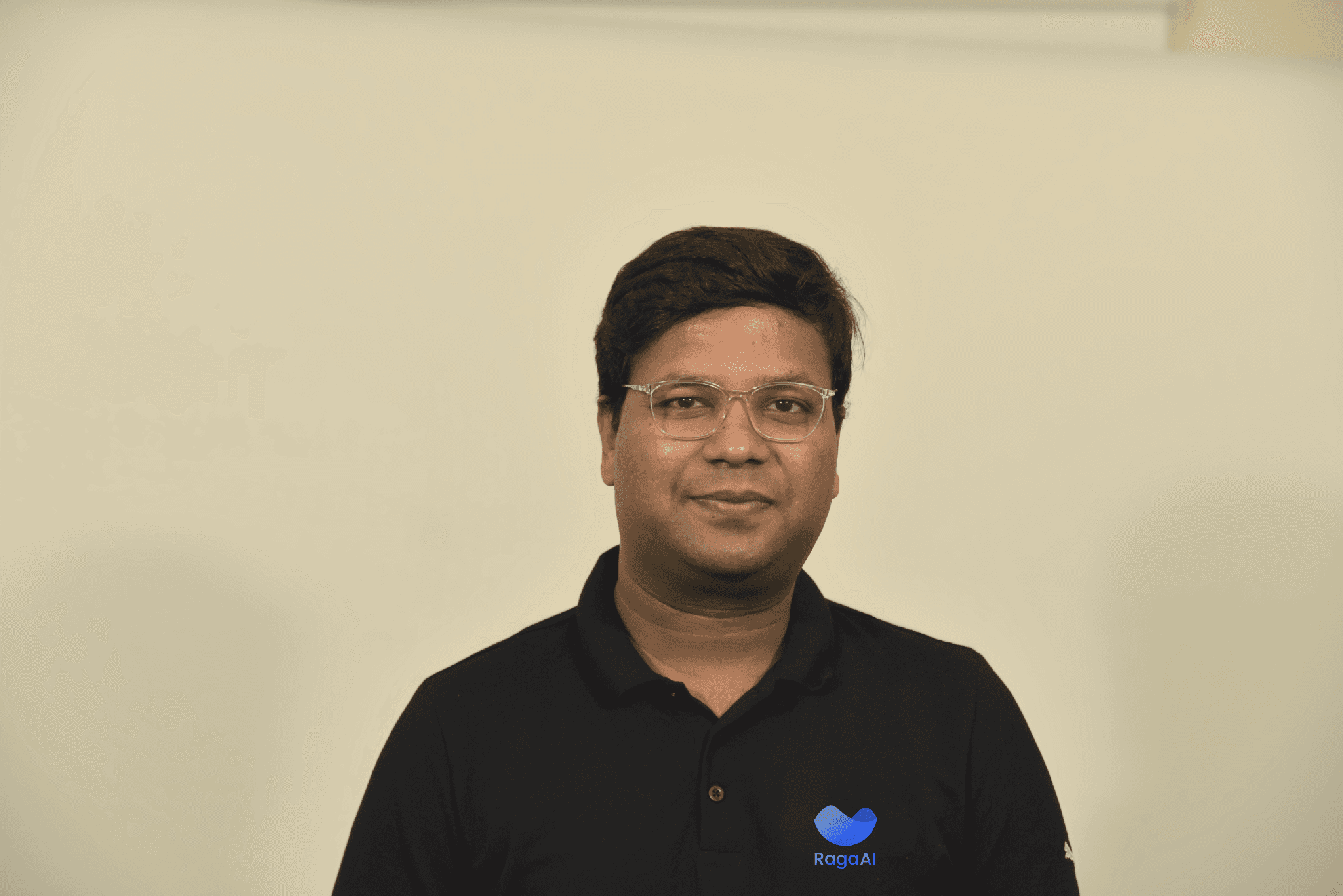
Jigar Gupta
Apr 12, 2024
Read the article




Understanding Hallucinations In LLMs

Rehan Asif
Apr 7, 2024
Read the article




Demystifying FDA's Approach to AI/ML in Healthcare: Your Ultimate Guide
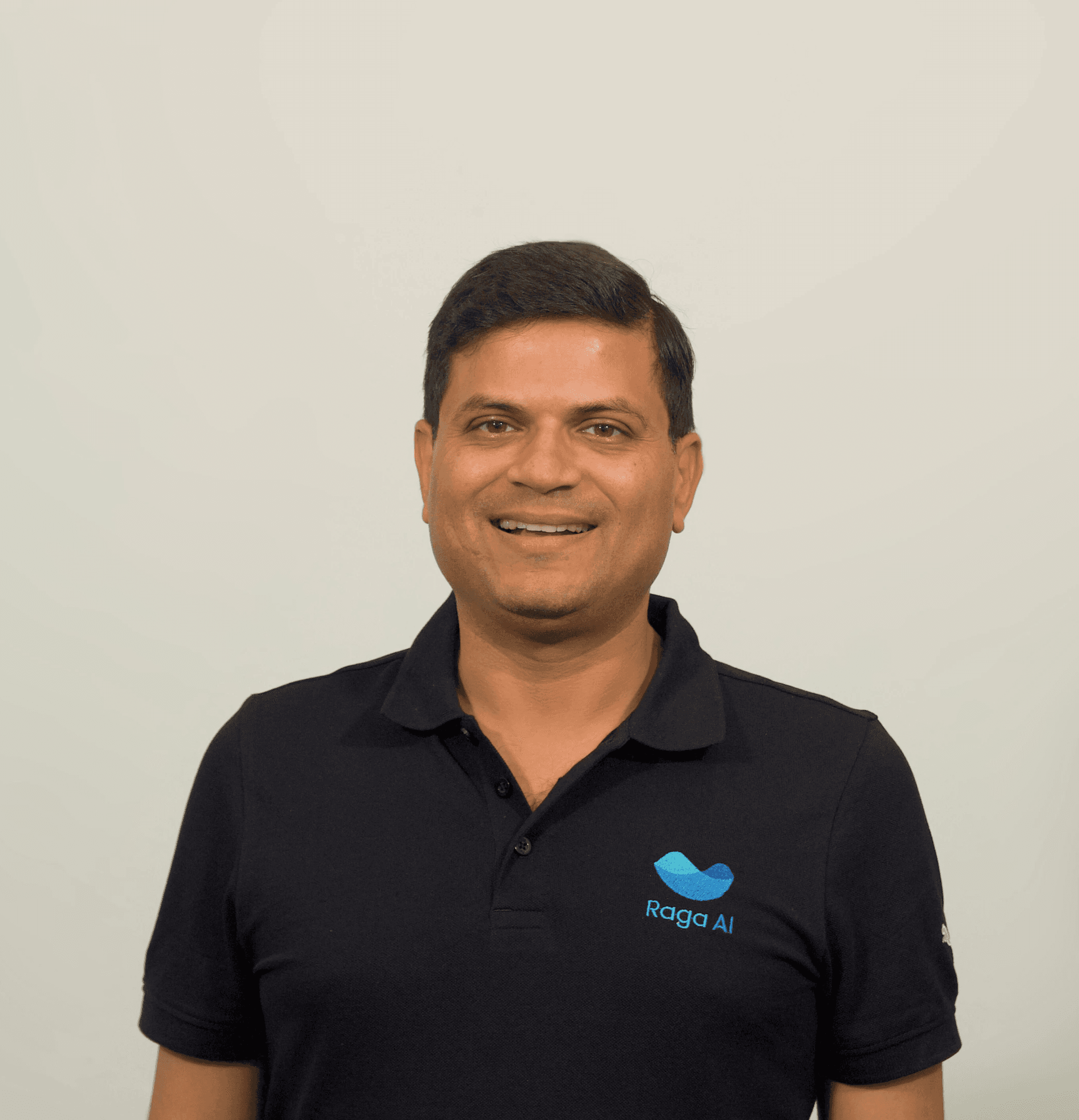
Gaurav Agarwal
Apr 4, 2024
Read the article




Navigating AI Governance in Aerospace Industry

Akshat Gupta
Apr 3, 2024
Read the article




The White House Executive Order on Safe and Trustworthy AI

Jigar Gupta
Mar 29, 2024
Read the article




The EU AI Act - All you need to know
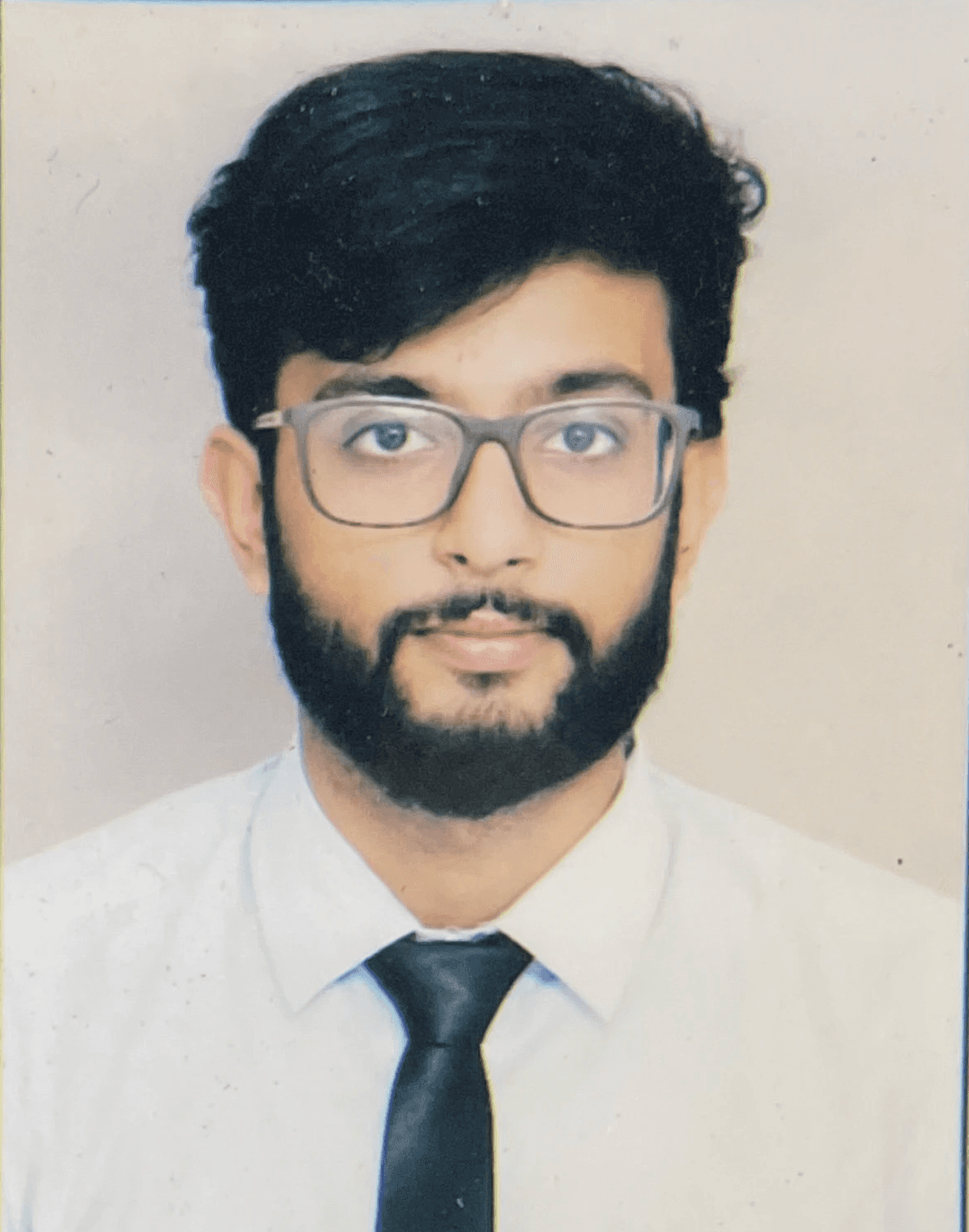
Akshat Gupta
Mar 27, 2024
Read the article
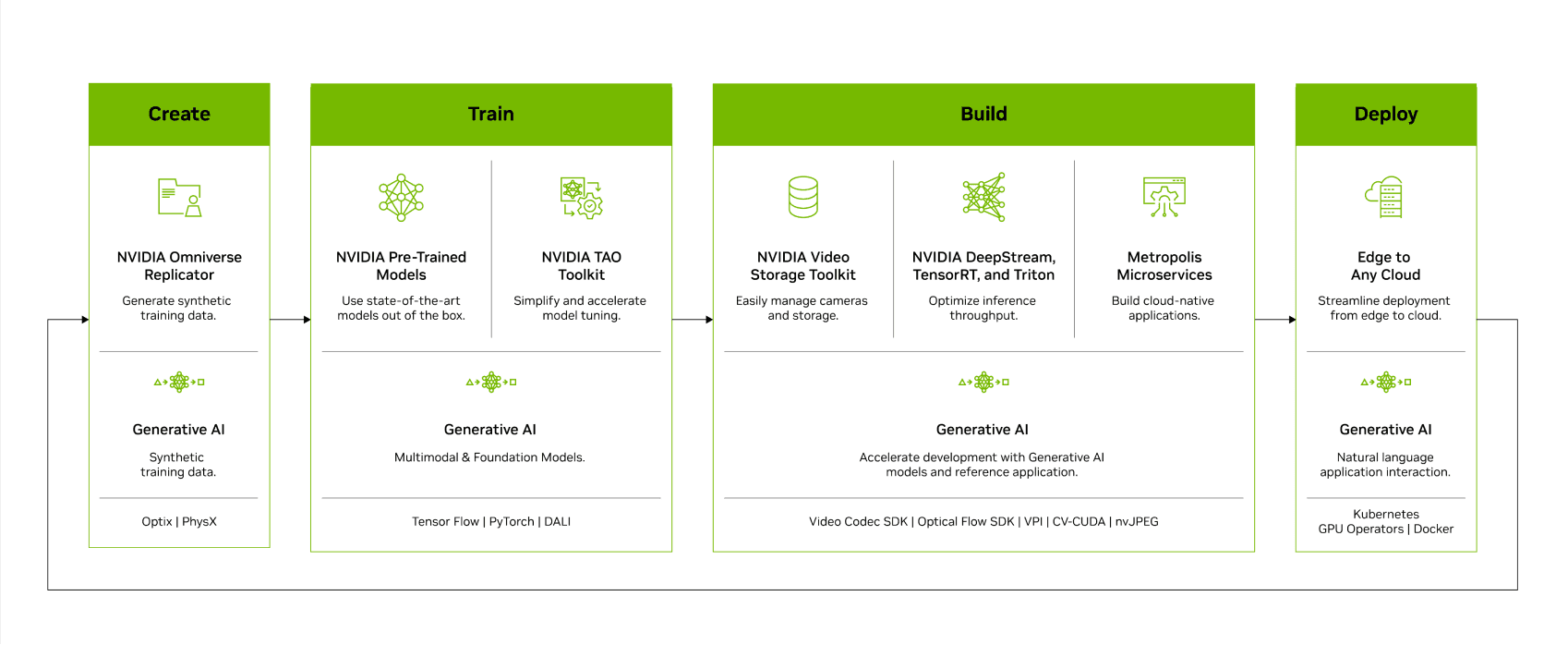
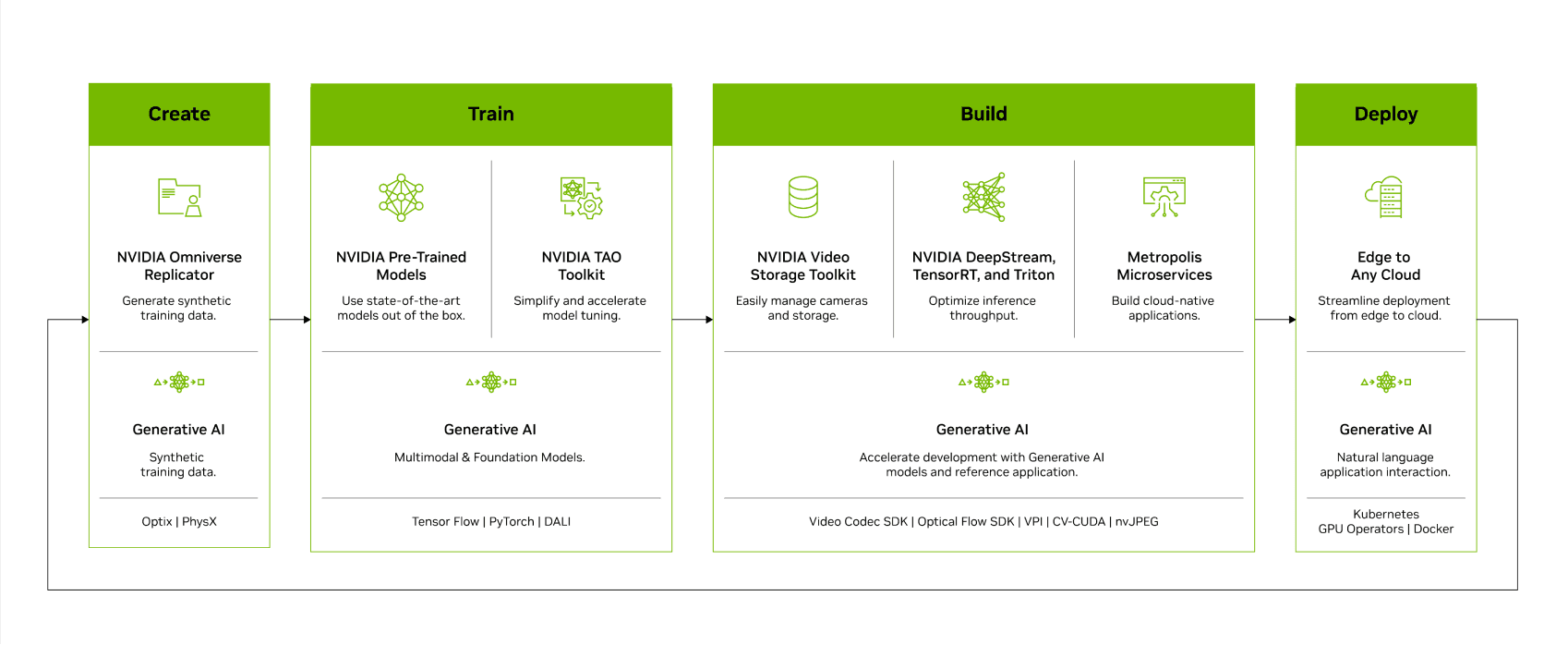
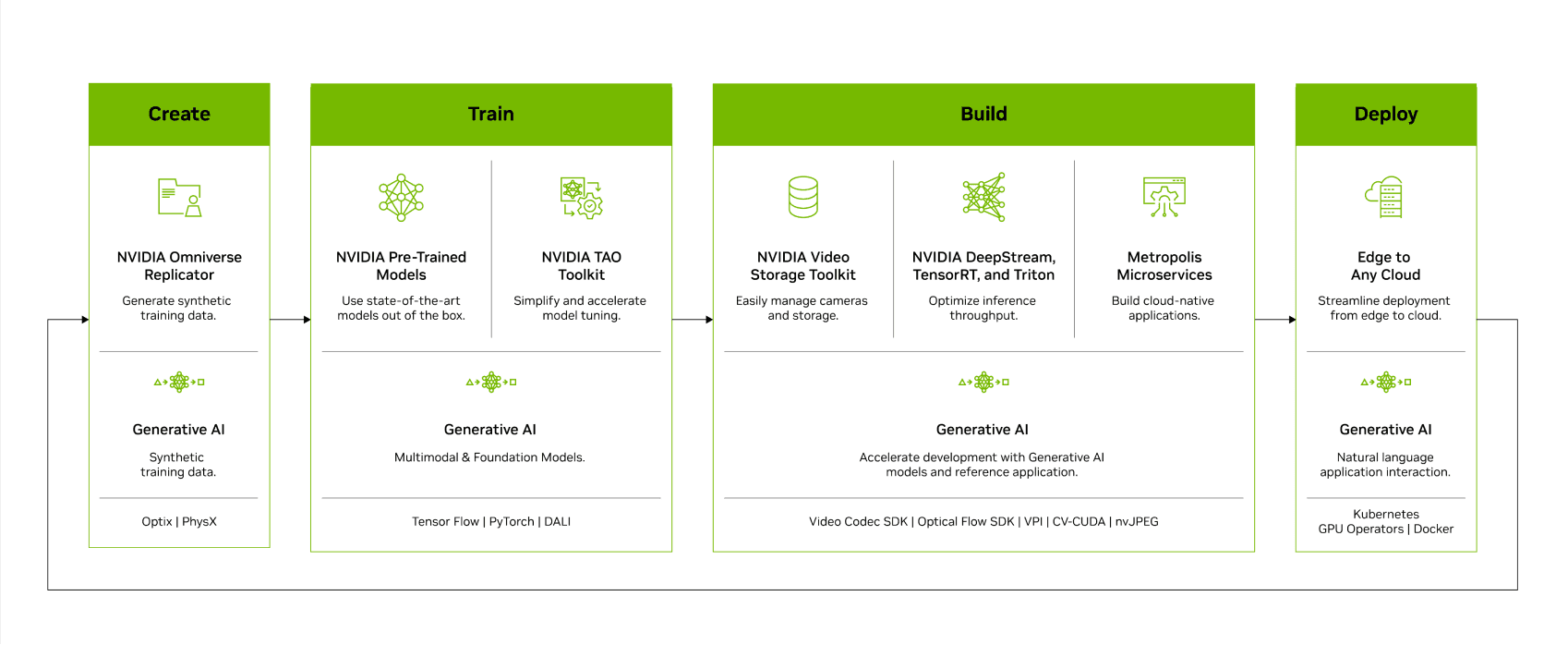
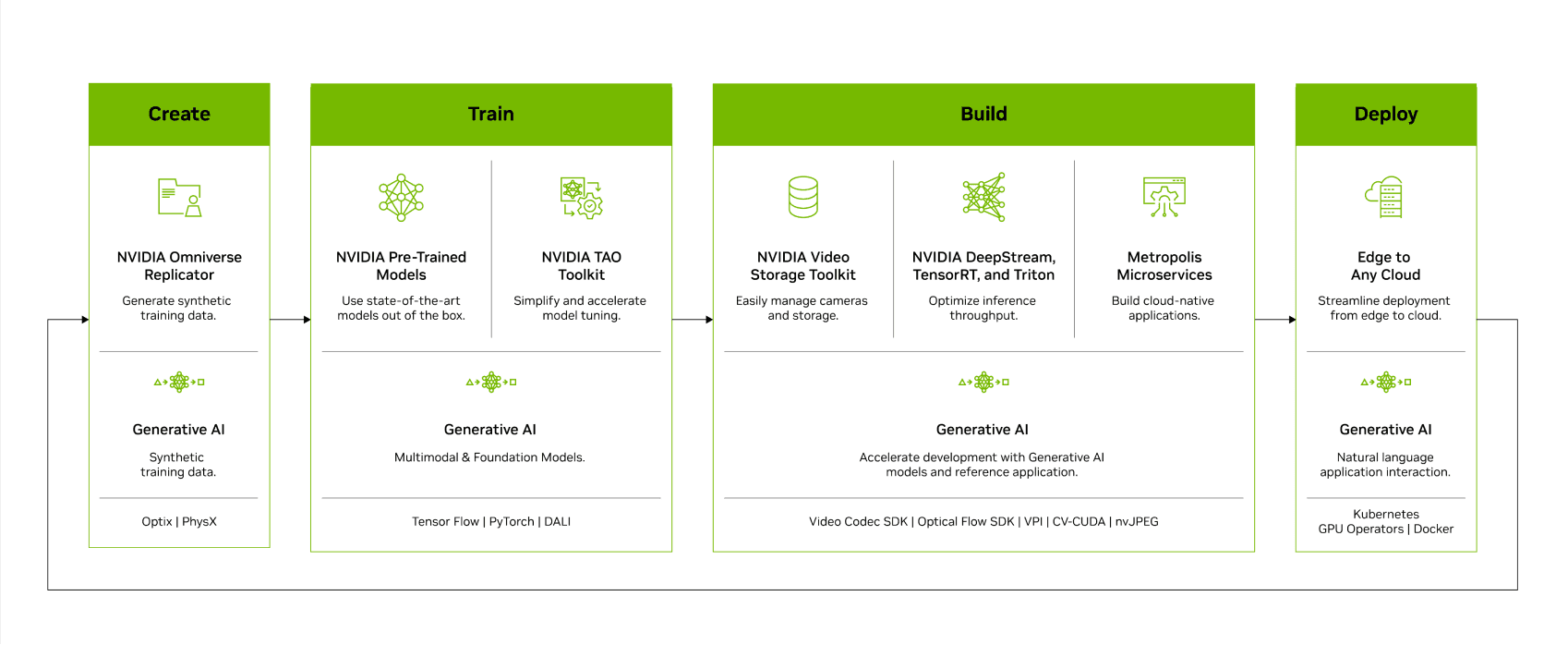
Enhancing Edge AI with RagaAI Integration on NVIDIA Metropolis

Siddharth Jain
Mar 15, 2024
Read the article




RagaAI releases the most comprehensive open-source LLM Evaluation and Guardrails package

Gaurav Agarwal
Mar 7, 2024
Read the article
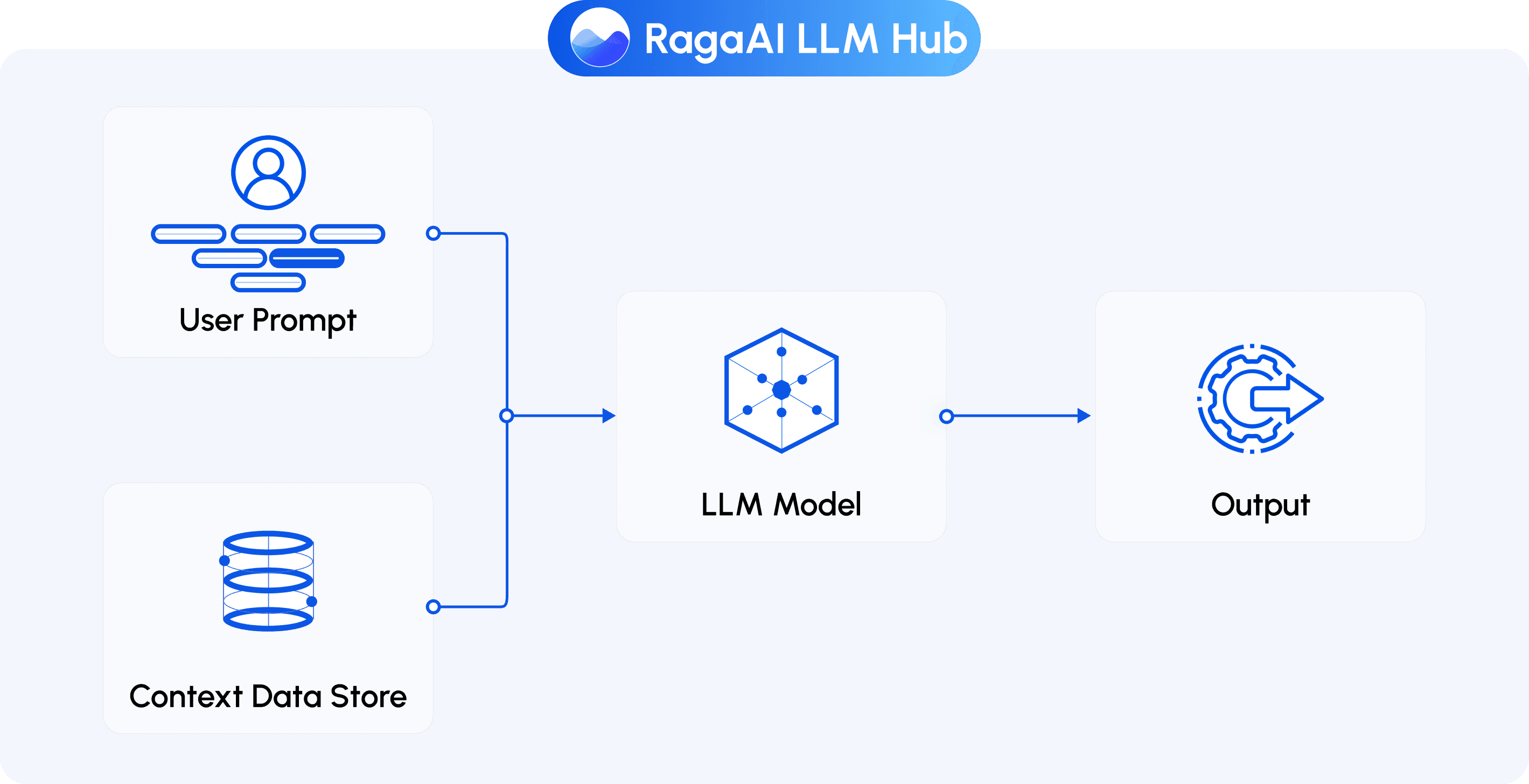
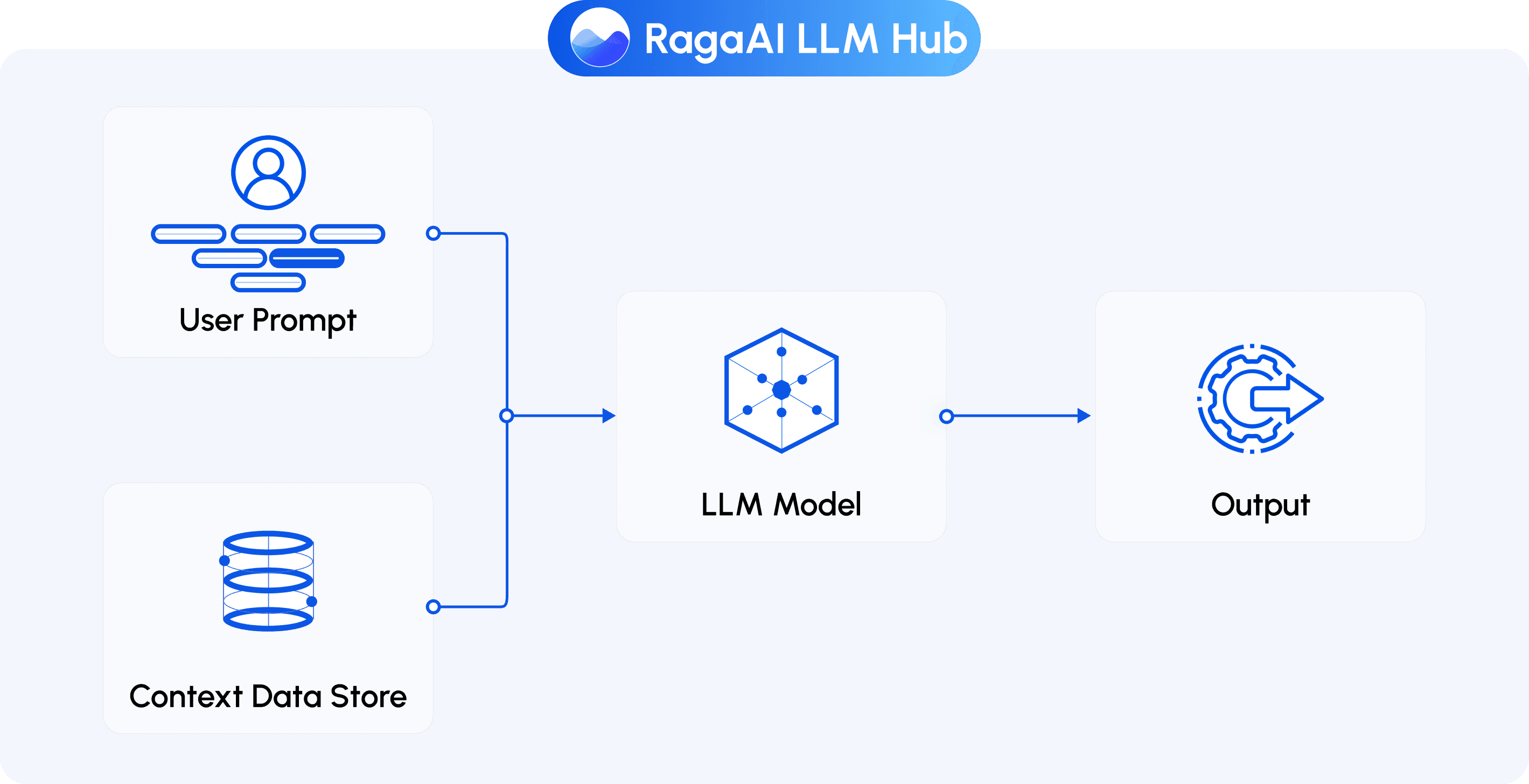
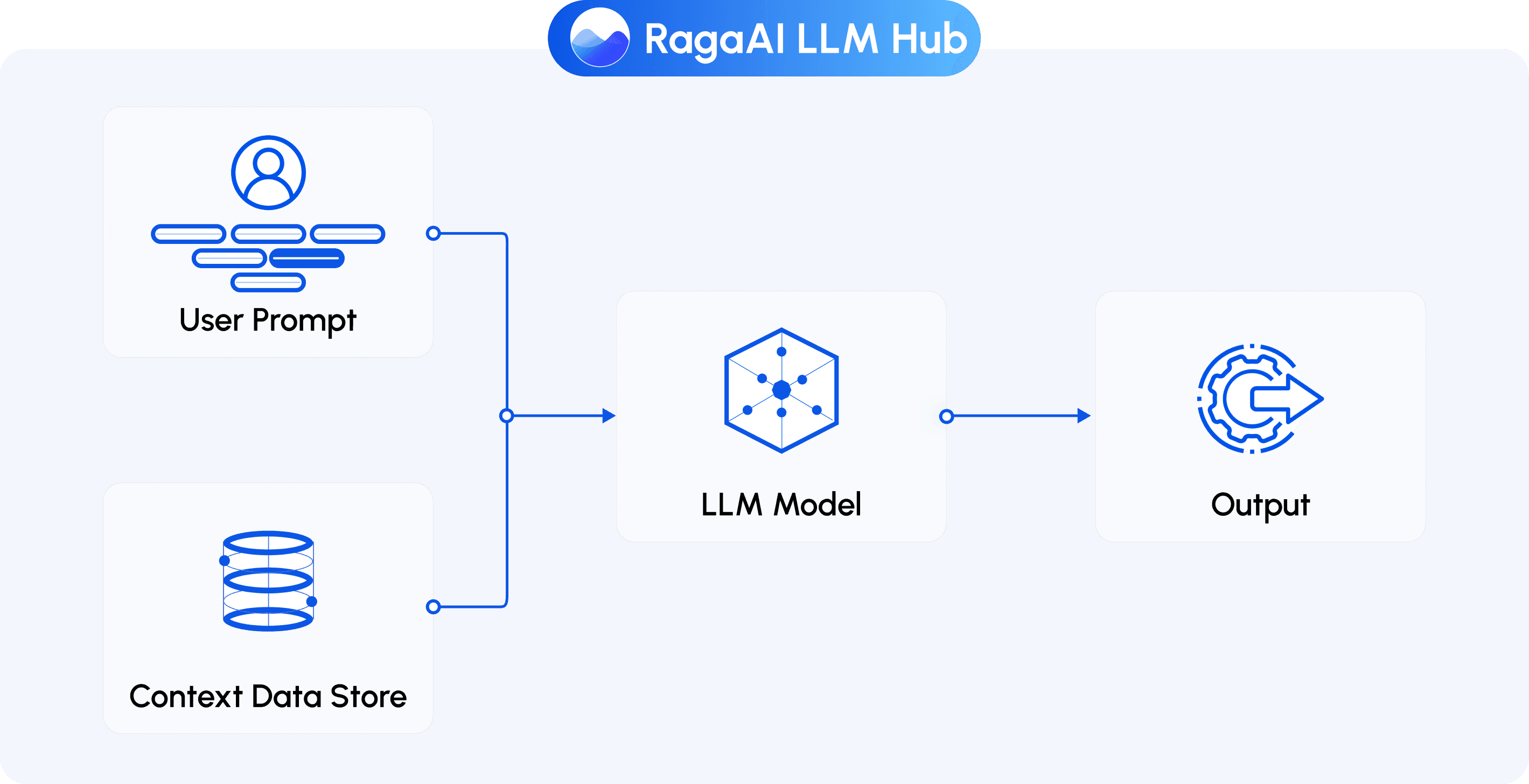
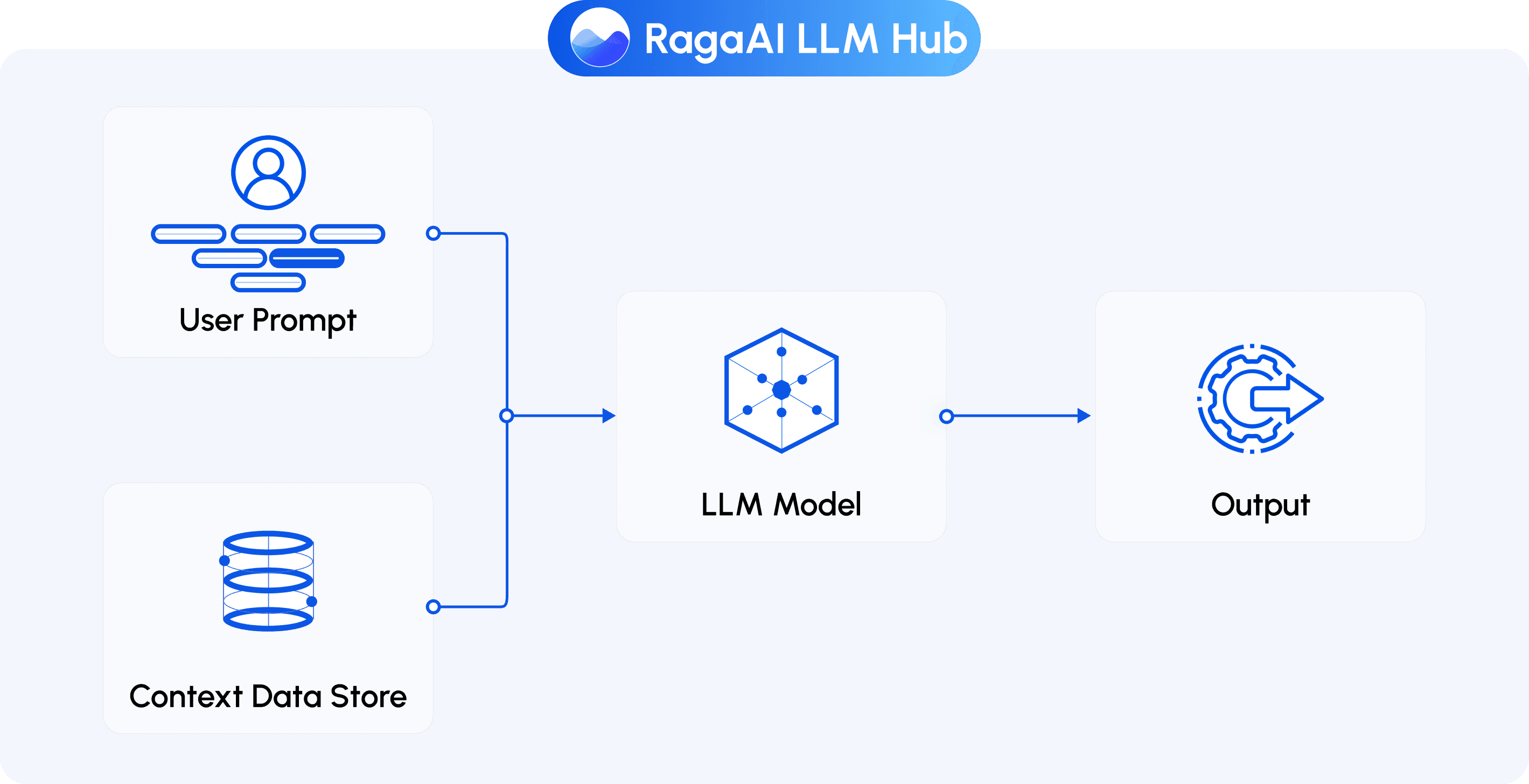
A Guide to Evaluating LLM Applications and enabling Guardrails using Raga-LLM-Hub

Rehan Asif
Mar 7, 2024
Read the article




Identifying edge cases within CelebA Dataset using RagaAI testing Platform

Rehan Asif
Feb 15, 2024
Read the article
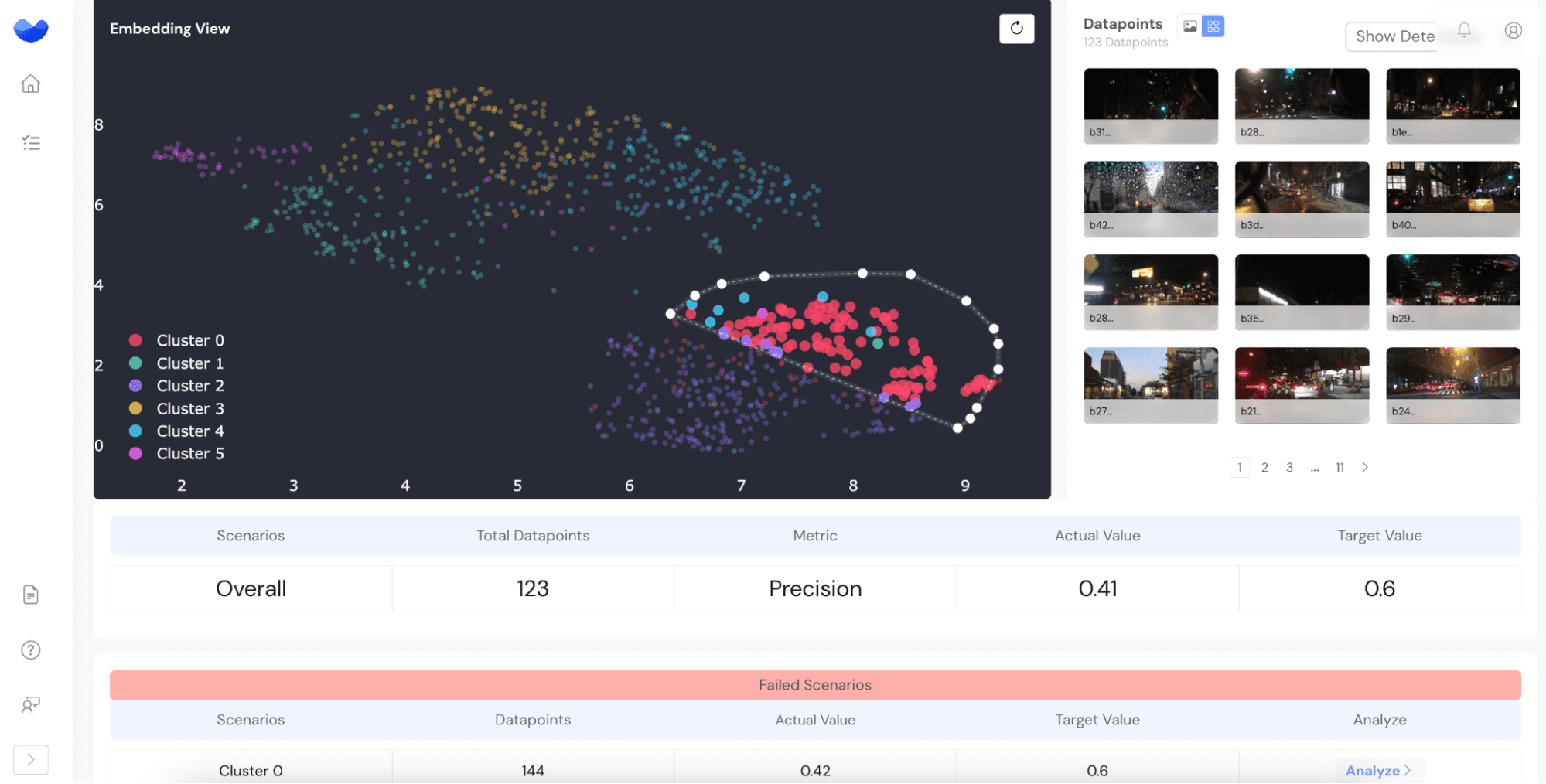
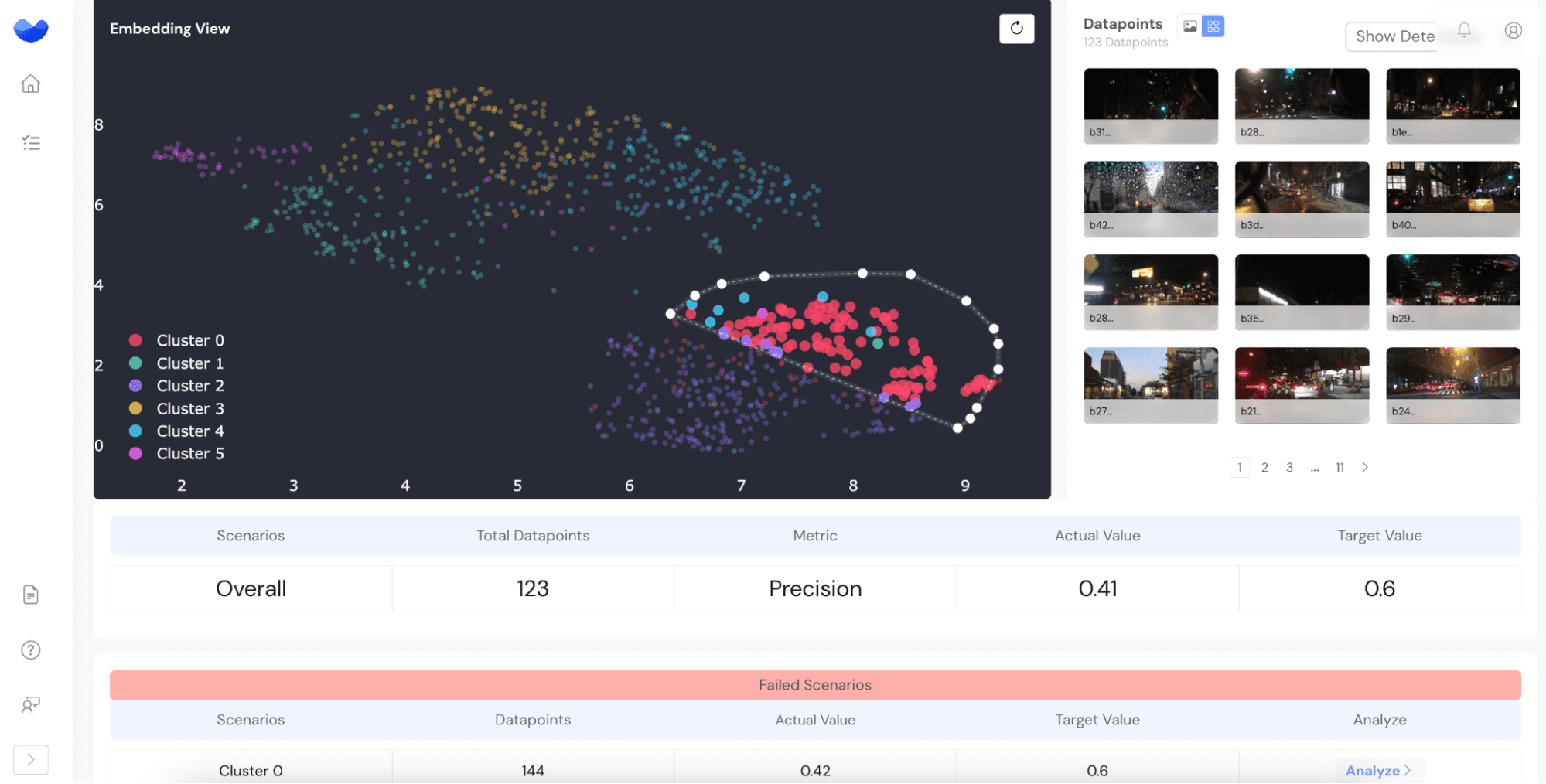
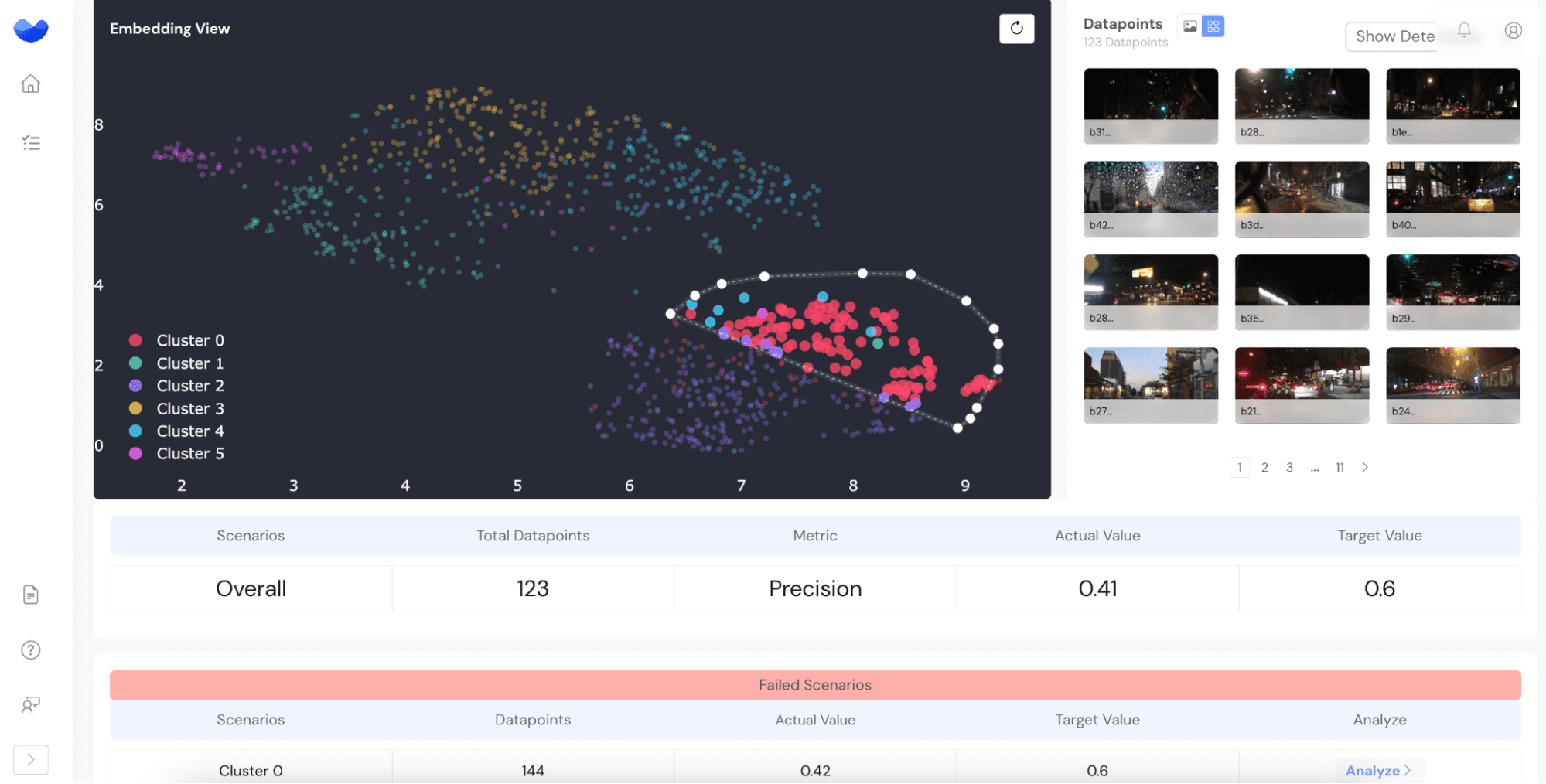
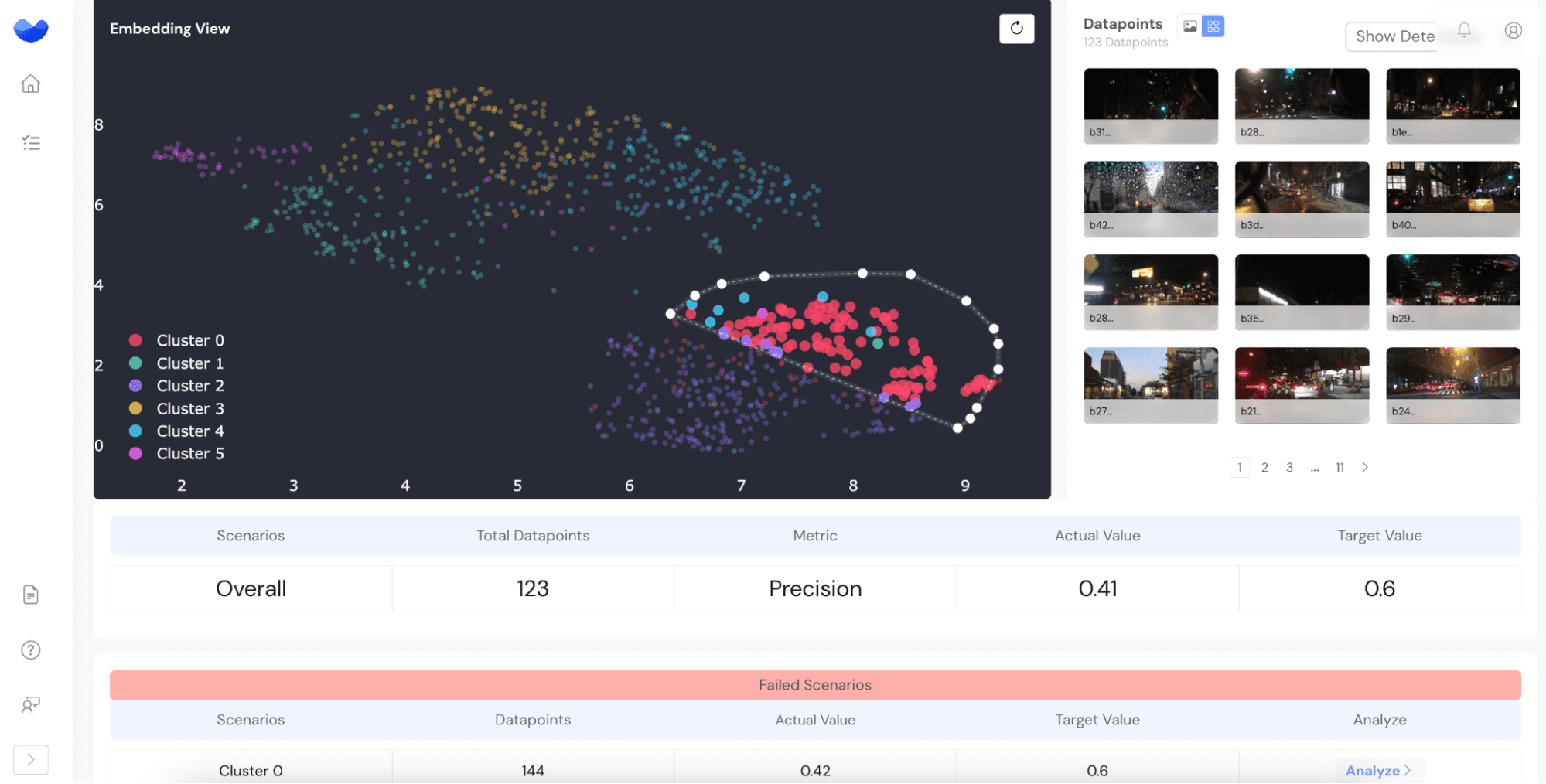
How to Detect and Fix AI Issues with RagaAI
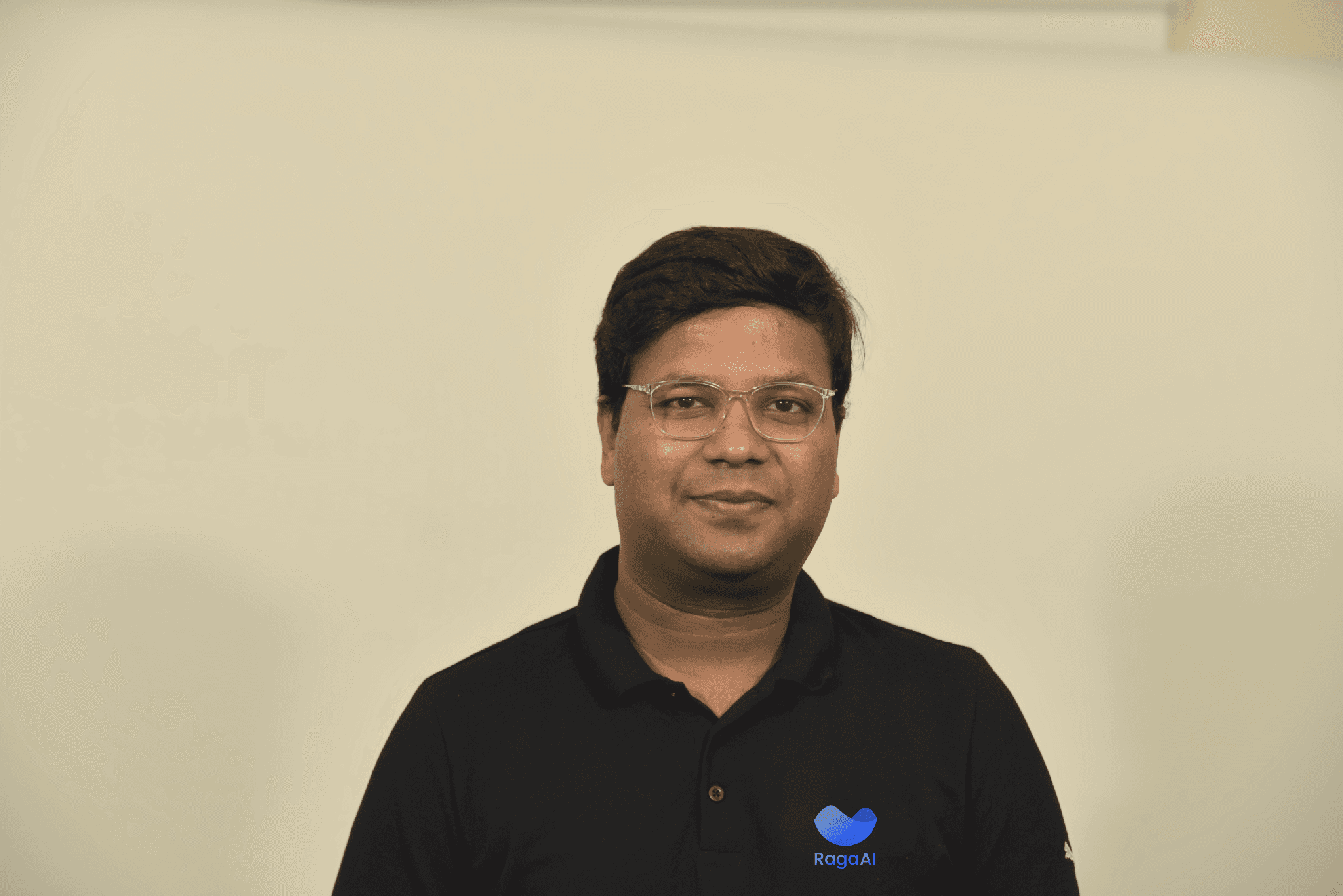
Jigar Gupta
Feb 16, 2024
Read the article
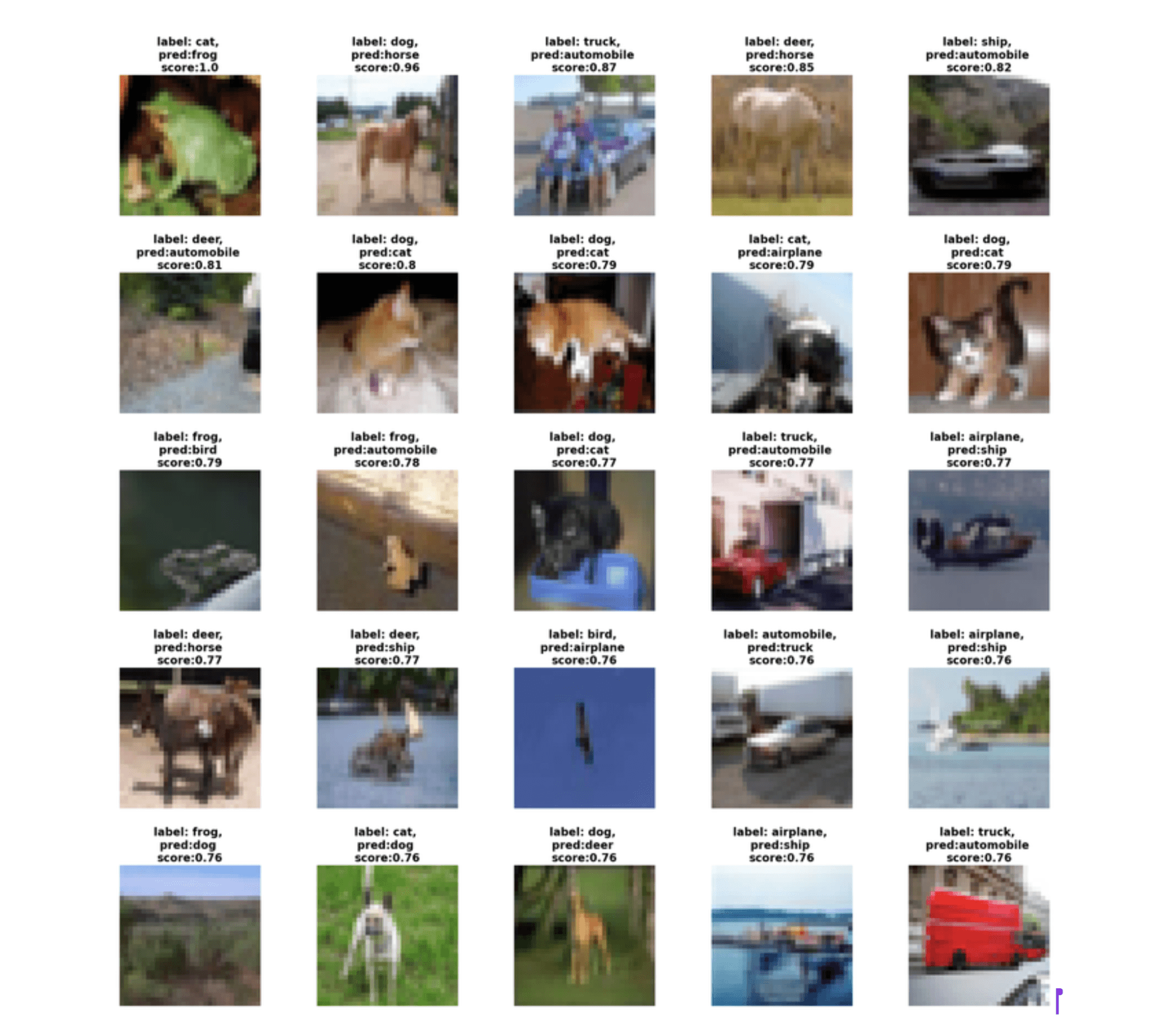
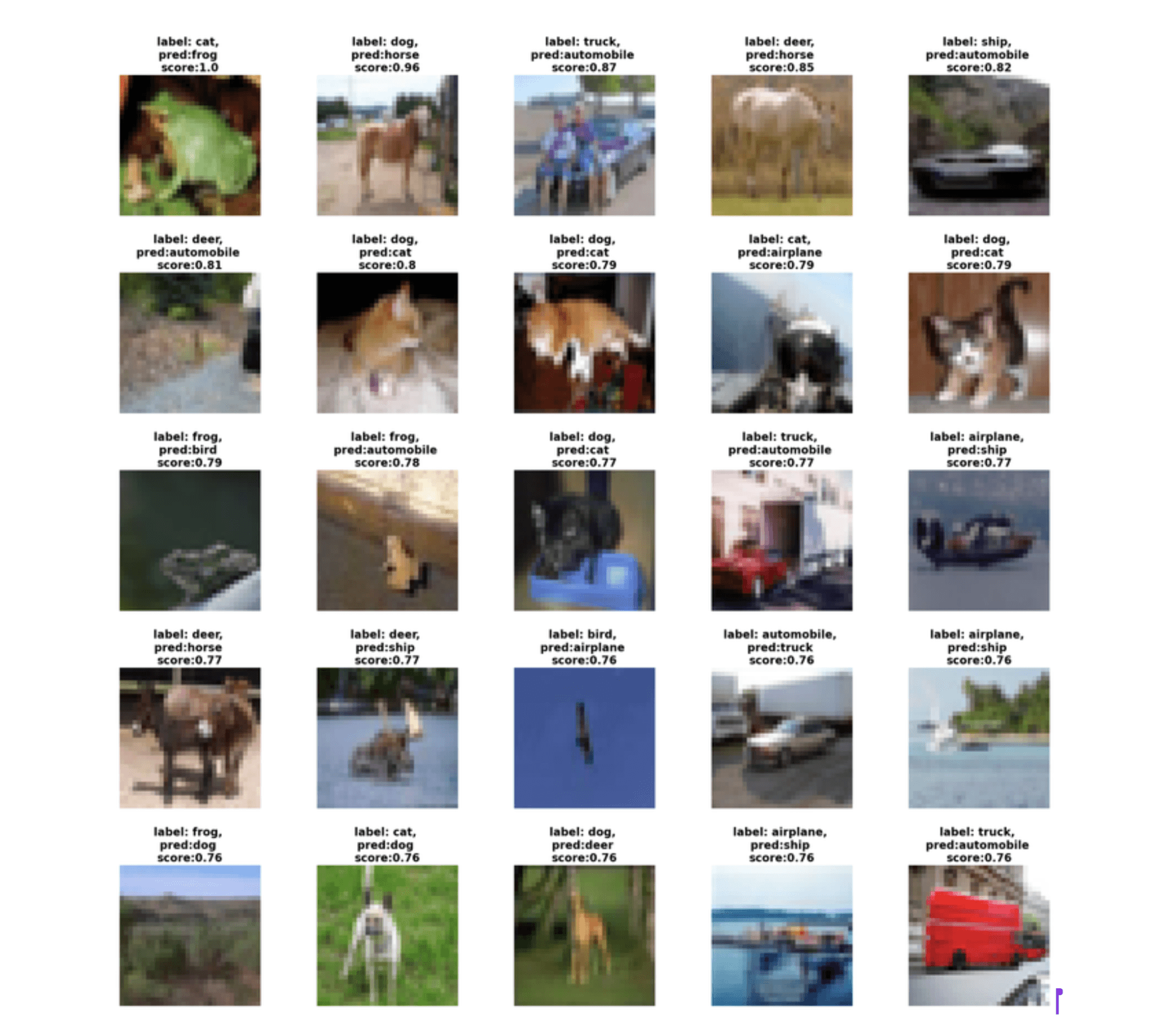
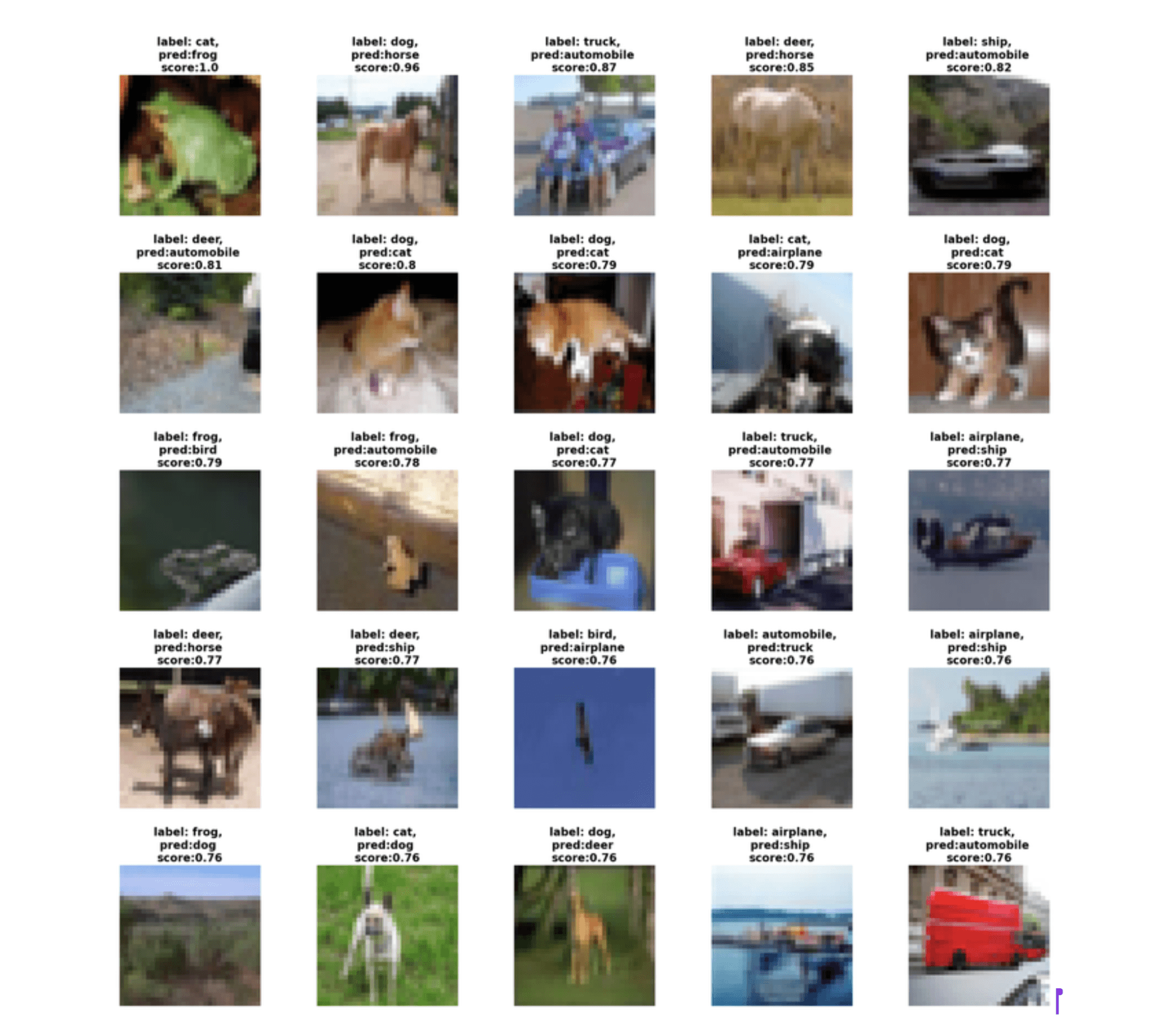
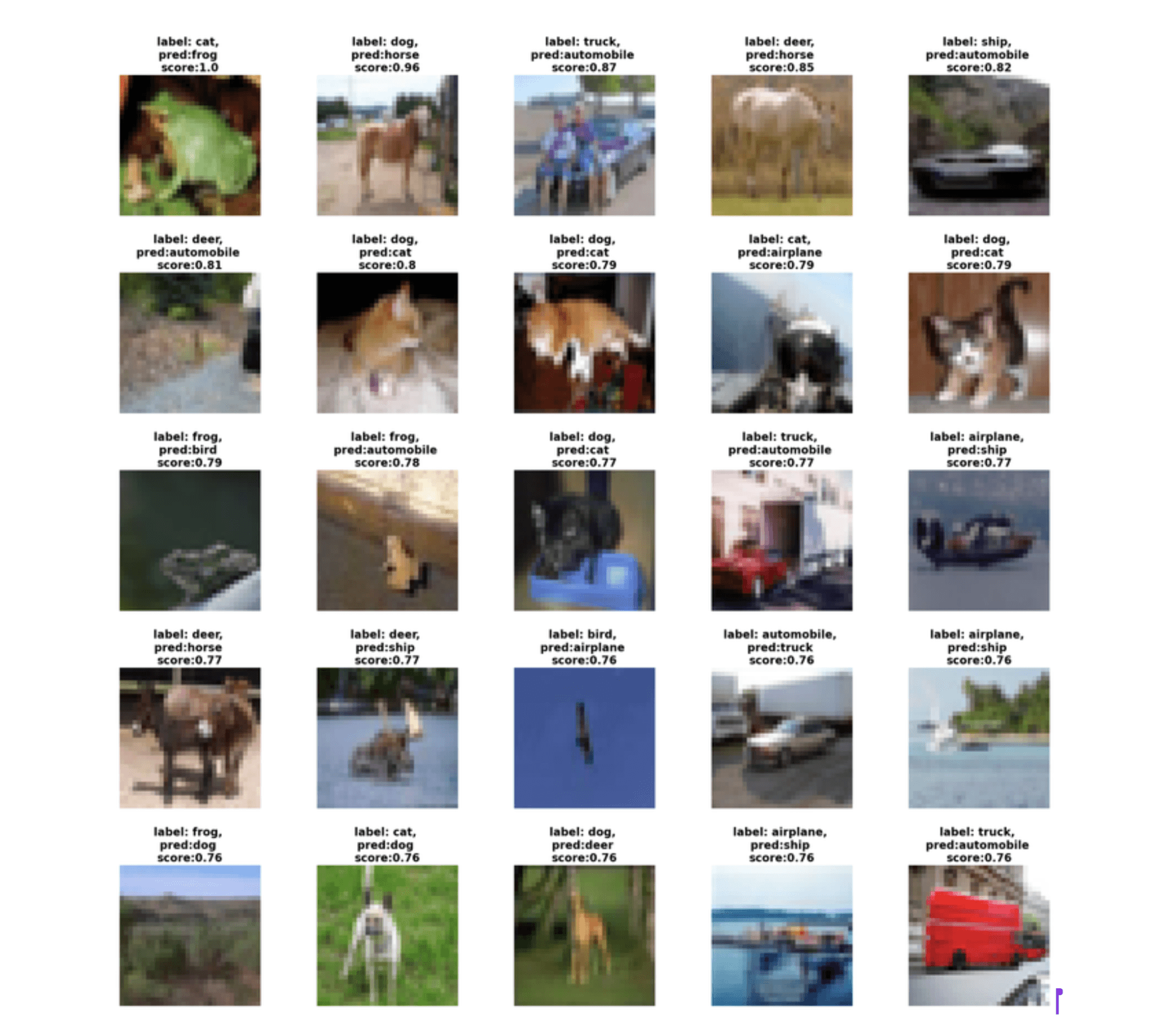
Detection of Labelling Issue in CIFAR-10 Dataset using RagaAI Platform
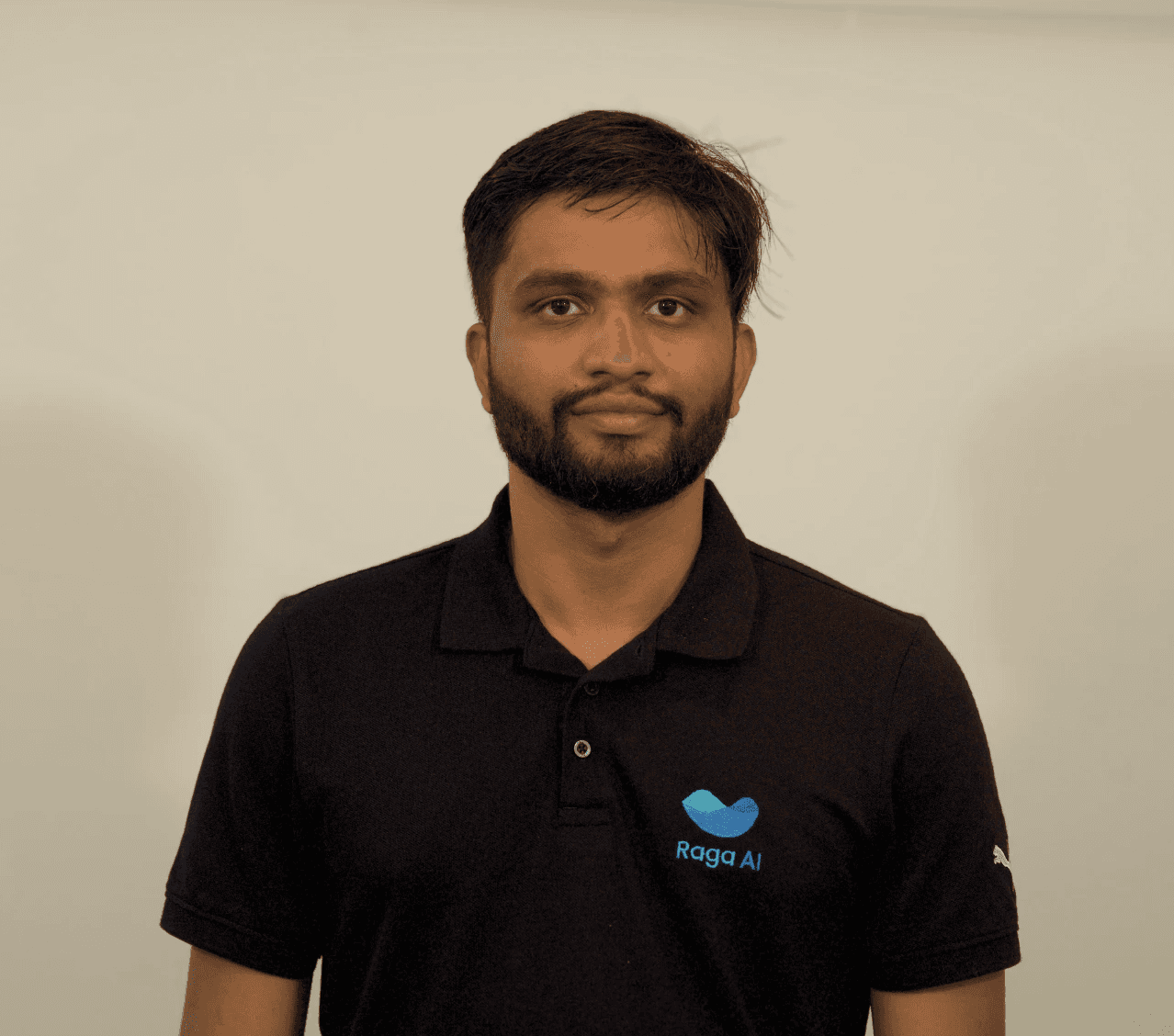
Rehan Asif
Feb 5, 2024
Read the article
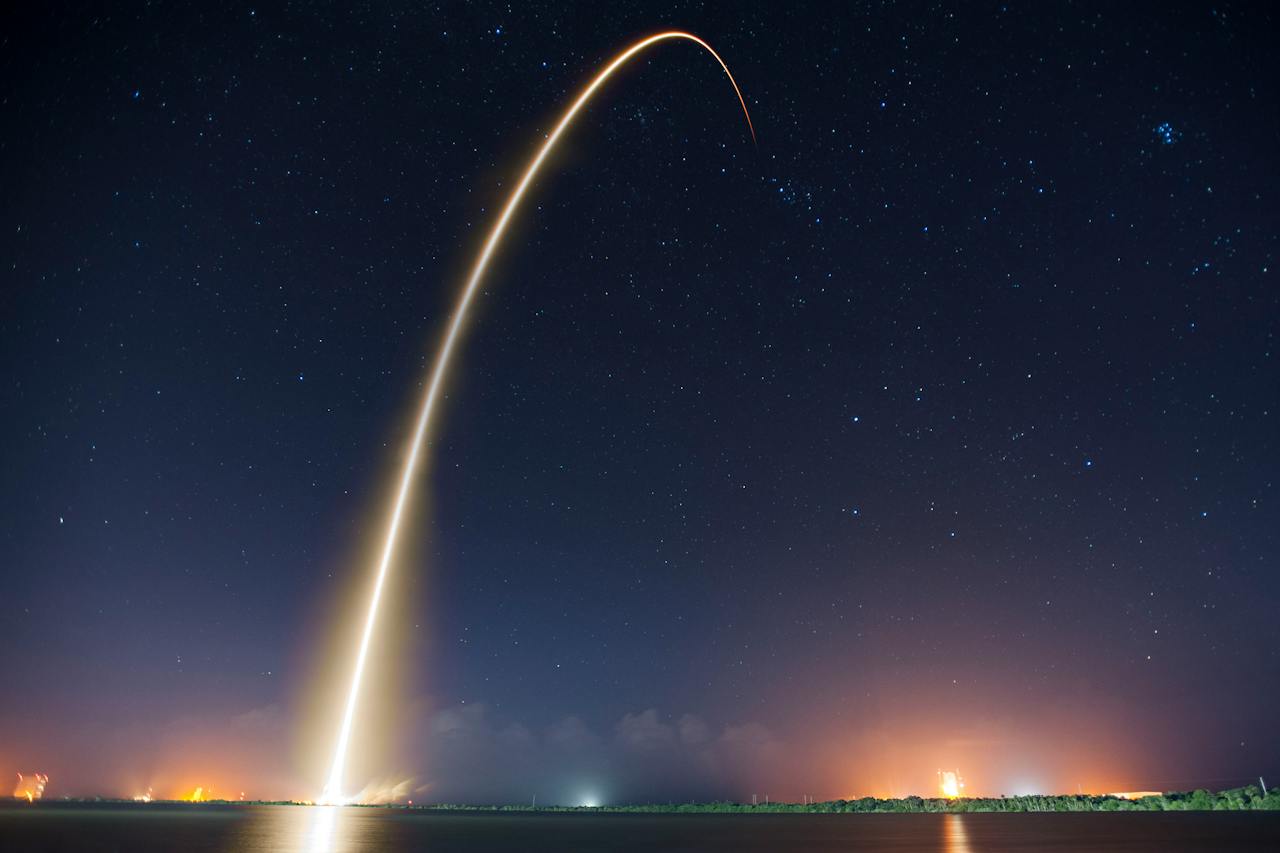
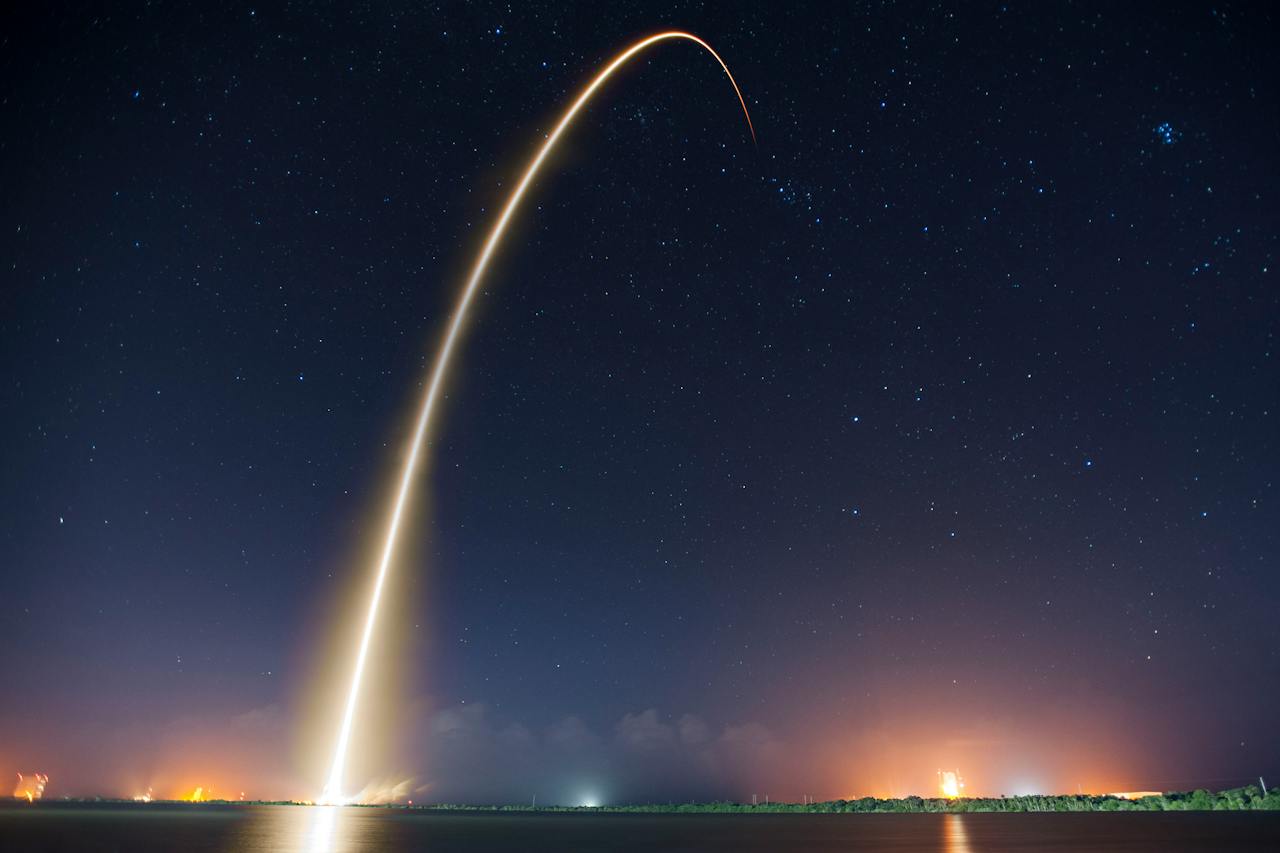
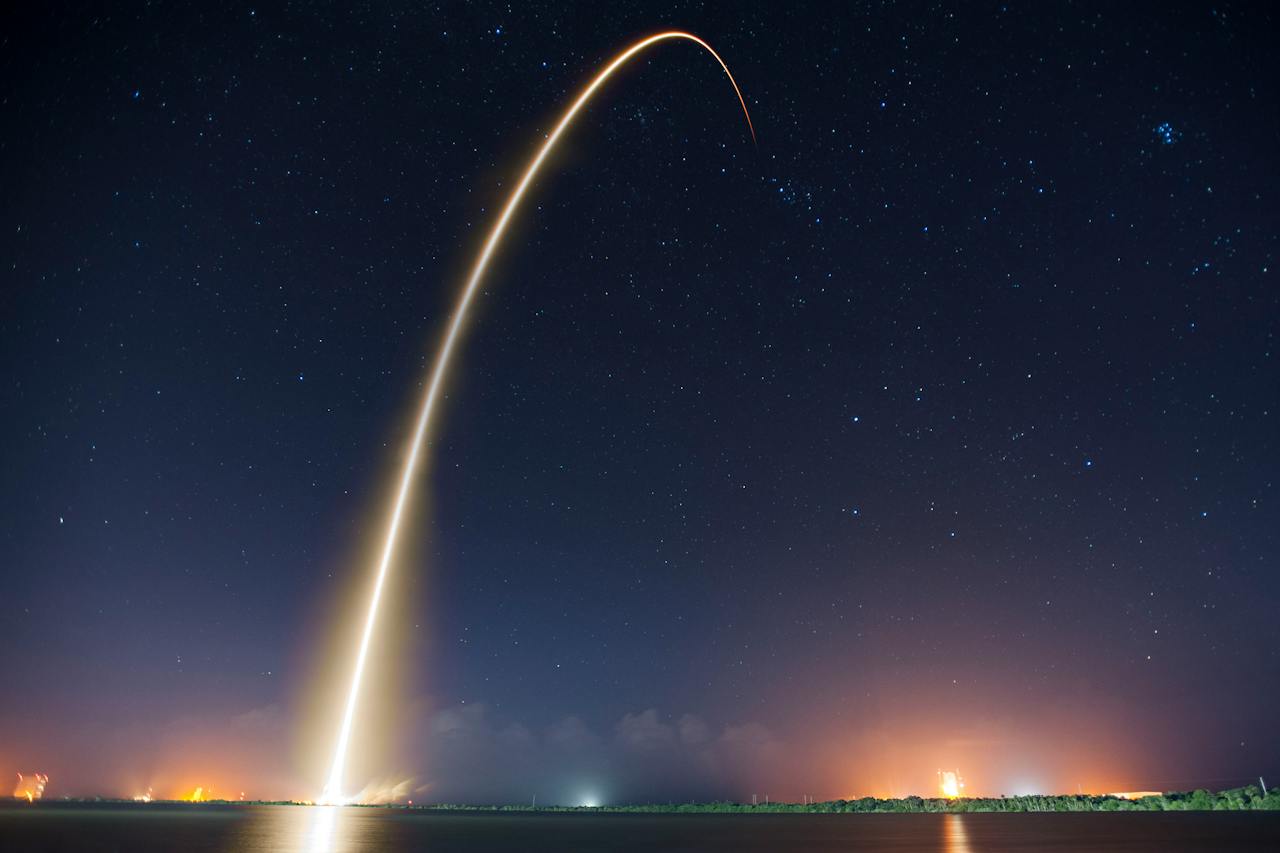
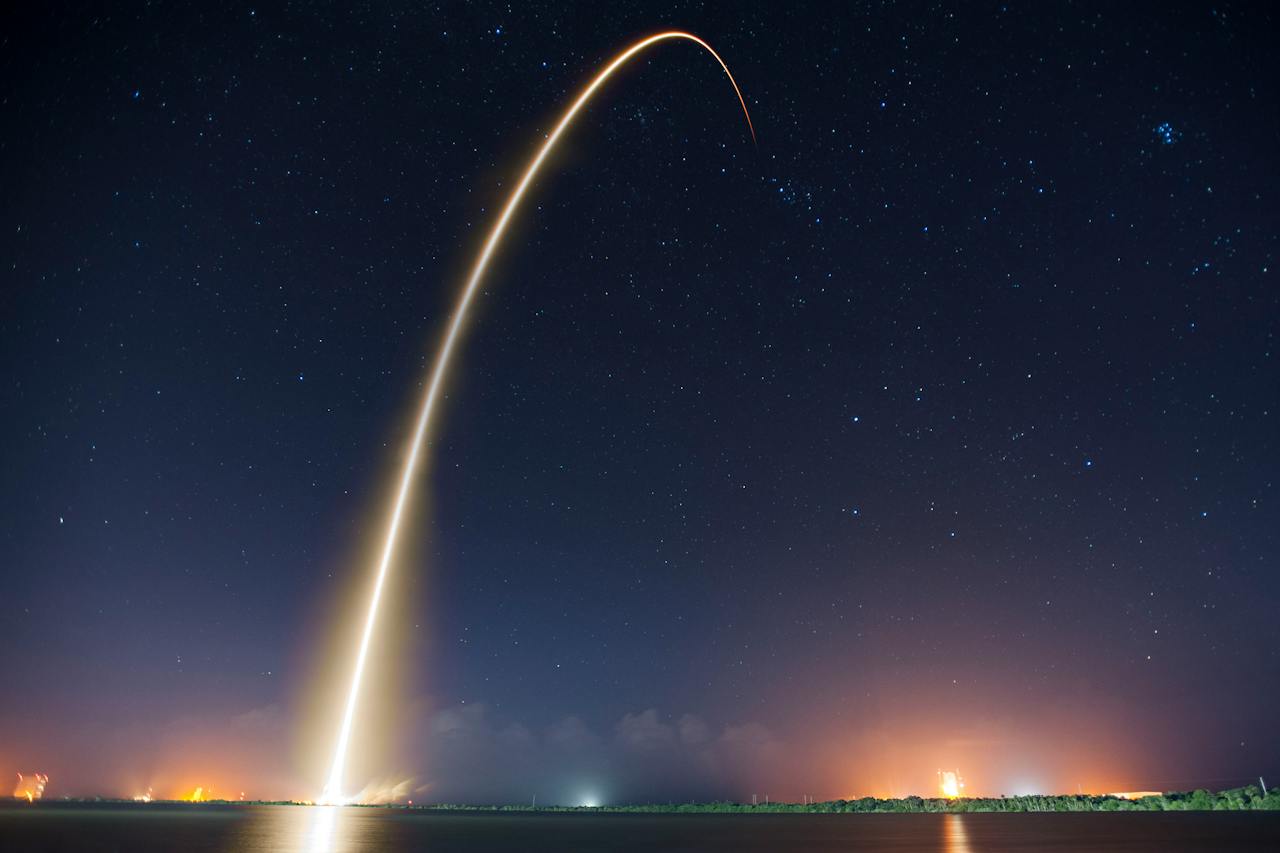
RagaAI emerges from Stealth with the most Comprehensive Testing Platform for AI
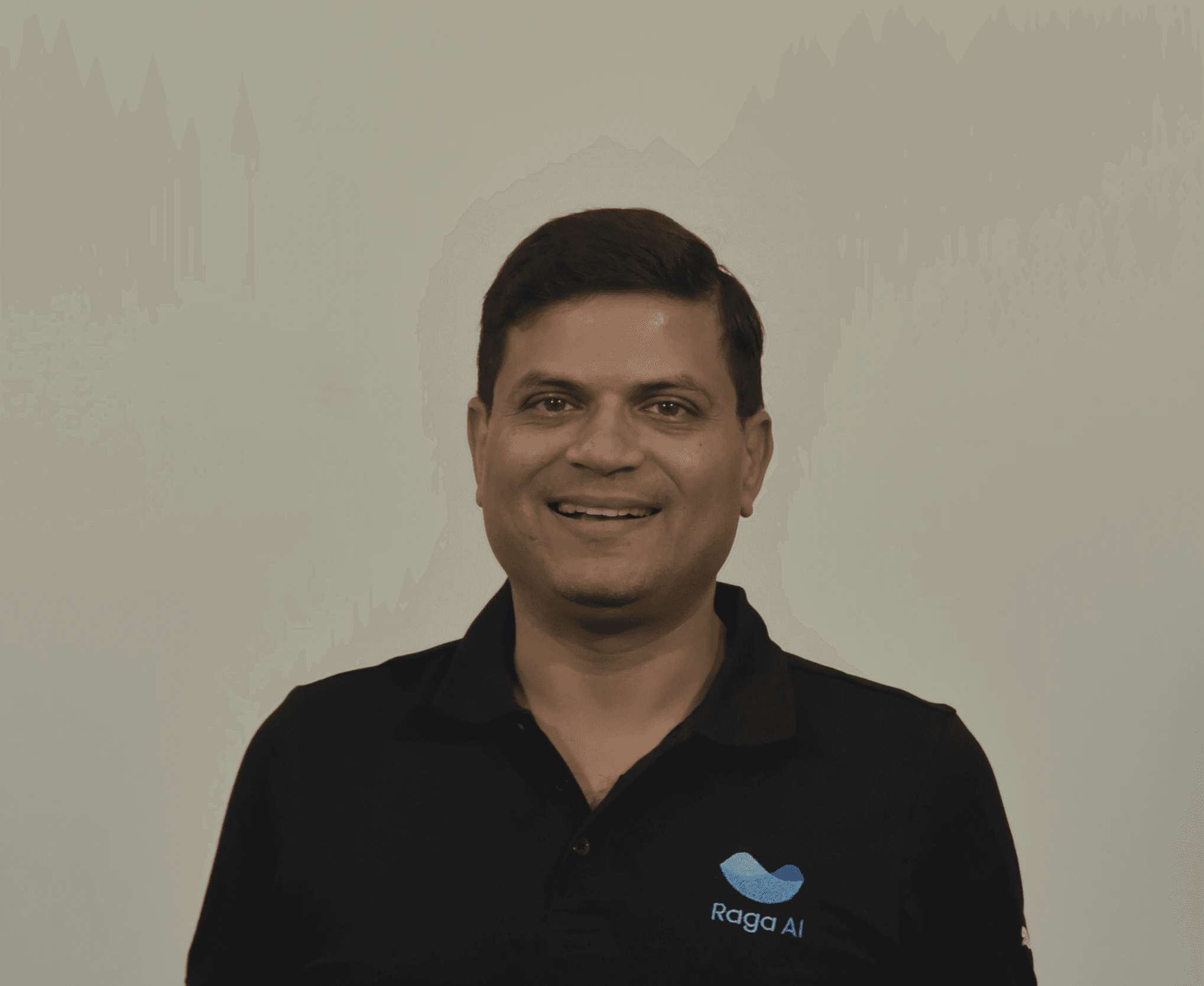
Gaurav Agarwal
Jan 23, 2024
Read the article




AI’s Missing Piece: Comprehensive AI Testing

Gaurav Agarwal
Jan 11, 2024
Read the article




Introducing RagaAI - The Future of AI Testing
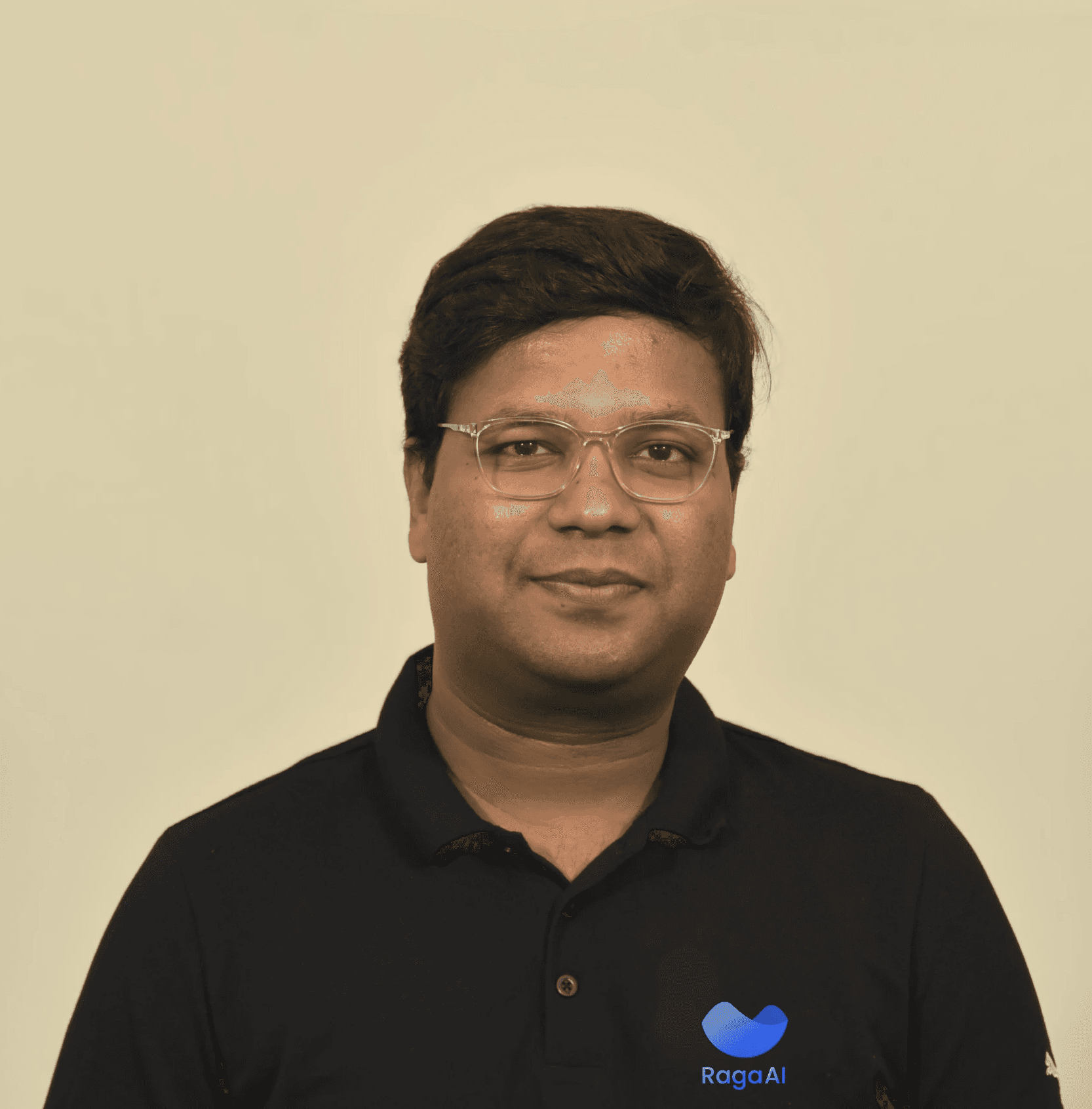
Jigar Gupta
Jan 14, 2024
Read the article




Introducing RagaAI DNA: The Multi-modal Foundation Model for AI Testing
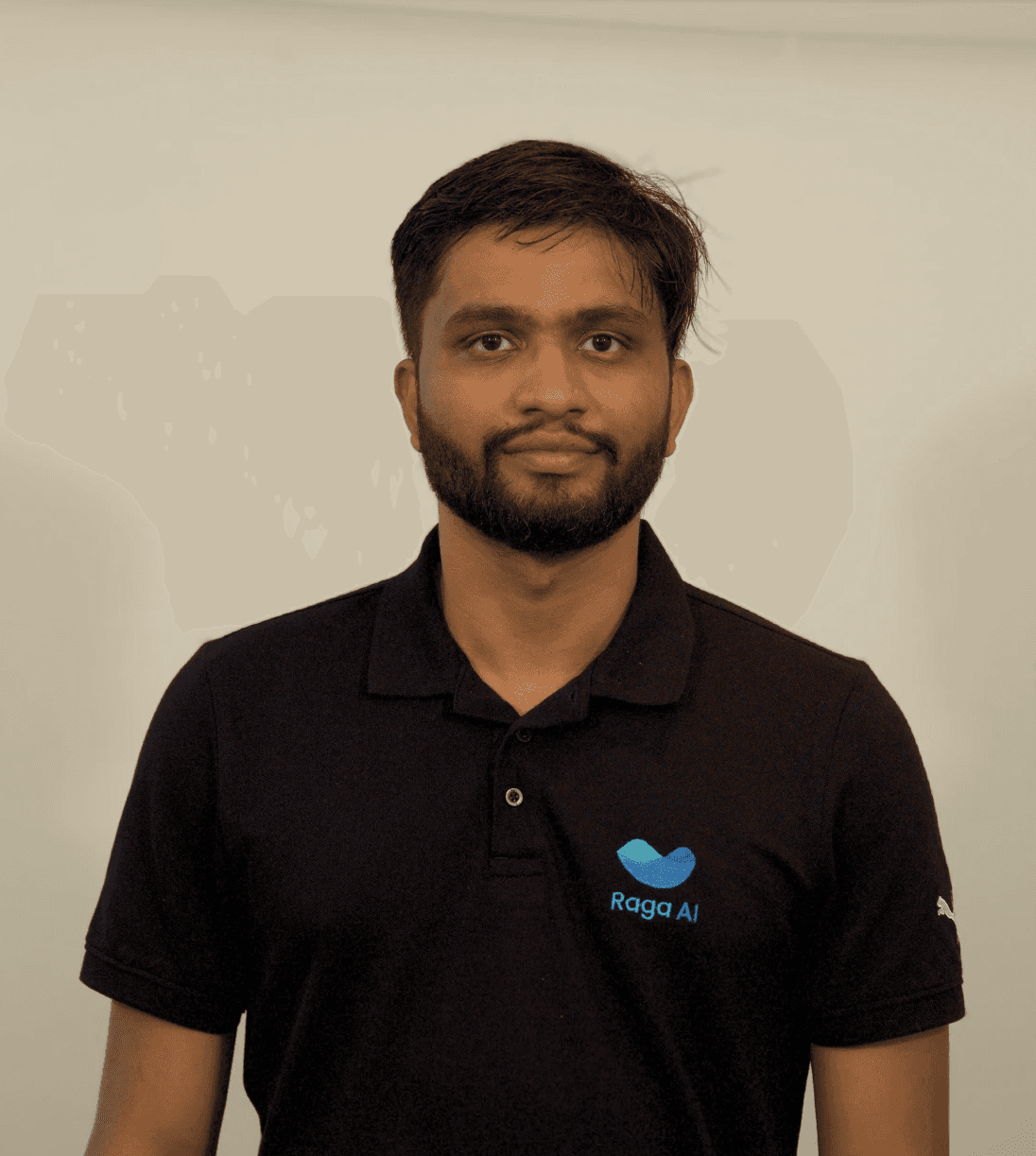
Rehan Asif
Jan 13, 2024
Read the article
Get Started With RagaAI®
Book a Demo
Schedule a call with AI Testing Experts

Get Started With RagaAI®
Book a Demo
Schedule a call with AI Testing Experts
