Integration Of RAG Platforms With Existing Enterprise Systems
Integration Of RAG Platforms With Existing Enterprise Systems
Integration Of RAG Platforms With Existing Enterprise Systems
Jigar Gupta
Apr 30, 2024




Integrating RAG platforms with enterprise systems is a bit like trying to put a square peg in a round hole, but with the right tools, anything's possible. Let's dive in and learn about RAG Platform Integration!
Introduction to Integration of RAG Platforms with Enterprise Systems
When you are looking to gear up your enterprise software systems, RAG (Retrieval-Augmented Generation) platforms are gradually becoming important.
By incorporating advanced NLP technologies such as RAG into your existing framework, you not only improve the working but also sleek the procedures to meet the growing demands of the venture landscape.
Overview of RAG Platforms and Their Significance in Enhancing Enterprise Software Systems
RAG platforms (Retrieval-Augmented Generation) are a type of Artificial Intelligence System that improves machine learning models by incorporating recovery abilities directly into the productive process.
This approach leverages a huge database of information, recovering necessary data to accelerate the generation of responses In the conditions of enterprise software systems, RAG platforms offer various important improvements:
Customer Support Systems: RAG Platforms transform customer support systems by giving precise, context-aware answers to customer questions. By recovering pertinent information from comprehensive documentation or knowledge bases, these platforms ensure that support agents and mechanized systems deliver accurate and prompt support, enhancing customer satisfaction and curtailing resolution times.
Decision Support Systems: Integrating RAG platforms into decision support systems improves the coherent abilities of enterprise software. These platforms can recover and consolidate pertinent data points from numerous sources, offering decision-makers with thorough and precise perceptions. This results in more acquainted and efficient planned decisions, boosting the venture growth and diligence.
Document Management and Compliance: RAG platforms simplify document management and compliance processes by effectively recovering relevant regulatory details and documentation. Enterprises can ensure conformity to regulatory needs and industry criterions by using these platforms to access the latest compliance standards and documentation, alleviating the threats of non-compliance and interrelated forfeits.
Research and Development: Enterprise engaged in research and development (R&D) advantage from RAG platforms through improved access to the latest investigation discovery and technical literature. These platforms accelerate the recovery of pertinent studies, trademark, and technical documents, aiding inventiveness, and hastening the expansion of new merchandises and technologies.
Importance of Integrating Advanced NLP Technologies into Existing Enterprise Infrastructure
The advanced NLP technologies integration into enterprise infrastructure is substantially significant for various reasons:
Improved Data Processing and Analysis
Efficient Unstructured Data Handling
Advanced NLP technologies effectively process unstructured data, like emails, reports, social media posts, and customer responses.
By extracting significant information from these disparate data sources, enterprises can gain a pragmatic comprehension of market trends, customer emotions, and internal procedures. This expertise alters the raw data into extravagant perceptions, driving informed decision-making.
Improved Text Mining and Information Recovery
Incorporating NLP technologies improves the text mining and informational recovery procedures within an enterprise.
These technologies can recognize pertinent information from comprehensive document storage, databases, and external sources in real-time. This aptitude ensures that employees and the decision-makers have access to precise and latest information, providing prompt and well-informed decisions.
Optimized Customer Engagement
Advanced Chatbots and Virtual Assistants
Enterprises can position NL–powered chatbots and virtual helpers to offer 24/7 customer support. These intelligent systems comprehend and reciprocate to the queries of the customers in natural language, delivering customized and contextually pertinent support. By managing routine investigation and tasks, NLP technologies free up human support to focus on intricate problems, improving overall customer effectiveness.
Sentiment Analysis and Customer Perceptions
Progressed NLP Technologies permit sentiment analysis, permitting enterprises to gauge customer emotions and beliefs from text data.
By inspecting customer reviews, social media companionship, and support tickets, enterprises can recognize areas for enhancement, observe brand reputation, and tailor their marketing plans to better meet customer requirements.
The Advantages Of RAG Compared to LLMs
Envision this: LLMs are like those know-it-all friends who depend on what they learn in school. On the contrary, RAG Models are like those friends who can also strap out their mobiles and rapidly locate the latest information.
This imperium of RAG models comes from their secret weapon- retrieval. They can access and utilize tons of real-details, from news articles to research papers, to give you the most precise and latest answers.
When it comes to selecting between Retrieval-Augmented Generation (RAG) and standalone Large Language Models (LLMs), it is clear that RAG has some substantial benefits. Let’s know why RAG stands out:
Enhanced Knowledge Access and Accuracy
Real-Time Information Retrieval: RAG Models use real-time retrieval apparatus to access huge external databases or knowledge bases. This expertise ensures that the produced responses are not only relatedly apt but also up-to-date. Unlike LLMs, which are restricted by the stagnant nature of their training data, RAG models can integrate the latest information, substantially improving the accuracy and pertinence of the responses.
Vanquishing Training Data Limitations: LLMs are trained on large but limited sets of data, resulting in intrinsic knowledge cutoff. In comparison, RAG models vigorously recover relevant information from external sources, efficiently skirting the restrictions levied by the stagnant training data. This is specifically advantageous in domains where information swiftly progresses, like technology, medicine and finance.
Enhanced Response Quality and Relevance
Contingent Relevance: By incorporating retrieval mechanisms, RAG models can tailor answers based on precise context of a question. The recovery element pinpoint and provides contingently pertinent documents, which the productive element then uses to craft more accurate and situational cognizant responses. This results in higher-quality yields, contrast to LLMs alone, which might produce credible but not significantly precise answers based on incomplete context.
Fact-Checking and Reduced Hallucination: One of the substantial challenges with LLMs is the tenor to hallucinate-produce genuinely incorrect information positively. RAG models alleviate this by wrecking the produced content in recovered, demonstrable documents. This retrieval-augment approach acts as an annotating mechanism, significantly curtailing the examples of hallucinations and enhancing the dependability of the produced text.
Efficiency and Performance
Computational Efficiency: RAG models can be more arithmetically effective compared to training LLMs on ever-evolving sets of data. The recovery mechanism permits for a more tractable model size since the requirement to entrench all attainable knowledge within the model dimensions is reduced. Instead, pertinent knowledge is retrieved on demand, resulting in better use of computational resources.
Scalability: The flexible nature of RAG models improves scalability. The recovery element can independently scale with the expansion of the knowledge base, without requiring a conforming increase in the produced model. This separation of solicitudes allow for more adaptable and effective scaling plans.
Understanding RAG Platforms
So, what absolutely are RAG platforms? In essence, these systems include 2 main components: a retriever and a generator.
The retriever sieves through a huge database to find pertinent information, while the generator takes this information and crafts replies that are coherent and situationally apt.
This bi-component setup uses natural language processing to improve information recovery and generation, making it a solid tool for any enterprise.
Definition and Components of Retrieval-Augmented Generation Platforms
Retrieval-Augment Generation (RAG) platforms are a hybrid AI approach that merges machine learning models with recovery-based mechanisms to improve language generation tasks. The main components of RAG platforms are:
Retriever: The component’s job is to find appropriate documents from a large collection of data that can help in answering a query. It utilizes information recovery approaches to choose documents or data snippets that are appropriate to the input prompt. The retriever is commonly built on top of search computations and systems of indexing that can effectively manage large volumes of data.
Generator: Once the appropriate information is recovered, the generator utilizes this context to generate a coherent and contextually relevant response. This component is generally a large-scale language model trained on wide datasets to create human-like text based on the input and retrieved details.
Database or Knowledge Base: The retriever standardized or unstandardized data storage. It can indulge a wide range of information, from textual documents to standardized databases, relying on the application.
Integrator or Fusion Component: This part of the system incorporates the output from the retriever with the process of generation, often altering the input to the generator to include the recovered context or influencing the process of generation based strongly on recovered data.
Importance of RAG
Large Language Models (LLMs) are prominent Artificial Intelligence (AI) technology, generating smart chatbots and other NLP apps.
The intention is to generate bots that can respond to user queries in numerous situations by referencing reliable comprehension provenance.
Sadly, the creation of LLM technology acquaints volatility in LLM responses. In addition, LLM training data is stagnant and acquaints a cut-off date on the comprehension it has.
Let’s take a look at the detailed breakdown of why RAG is significant:
Improved Accuracy and Relevance
Incorporation of External Knowledge
RAG Systems uses external data storage, knowledge bases, or documents to recover pertinent data, which is then integrated into the process of generation. This ensures that the produced content is not only coherent but factually precise and latest.
Contextual Awareness
By recovering contextually pertinent information from huge external sources, RAG models can produce responses that are better customized to the precise query or response, resulting in more accurate and contextually pertinent production.
Scalability and Flexibility
Scalability Knowledge Incorporation
RAG models can incorporate enormous and diverse external data sources, making them highly flexible. This adaptability permits them to adjust to distinct domains and applications without requiring comprehensive briefing on domain-specific data.
Adaptive Learning
The recovery element can constantly update with new data, permitting the model to adjust to new data and progressing comprehension without the requirement for constant re-training of the produced element.
Technical Implementation
Dual-Encoder Architecture
RAG usually applies a dual-encoder architecture where one encoder processes the questions and the other encoder processes the recovered documents. This setup permits for effective matching of the questions with pertinent external information, which is then utilized by the produced model to produce the final output.
End-to-End Training
RAG models can be trained end-to-end, upgrading both recovered and generation elements concurrently. This incorporated training approach ensures that the recovered process is impeccably tuned to aid the generation process, resulting in better overall performance.
Latest RAG Pipelines
The latest expansion in Real-Augmented Generation (RAG) pipelines exhibits a spectrum of advanced attributes and incorporations designed to improve the effectiveness and performance of generative AI applications.
R2R Framework: The R2R (RAG to Riches) Framework is a pragmatic tool for rapid expansion, positioning and upgradation of RAG systems. It provides flexible elements like Providers, Pipes and Pipelines, which permits developers to construct and fine-tune high standard RAG applications efficiently. The framework supports assorted use cases from document handling to real-time query responses, improving both adaptability and robustness.
NVIDIA AI Enterprise: NVIDIA’s solution to enterprise-grade RAG applications offers a sturdy infrastructure from moving RAG models from pilot to outputs. The procedure indulges evaluating LLMs, exporting models as api-driven architecture, evolving sample applications, and positioning them in Kubernetes clusters utilizing the NVIDIA RAG LLM Operator. The pragmatic approach ensures that RAG systems are upgraded for performance and smoothly incorporated into existing enterprise landscapes.
Super-RAG: Superagent-ai's Super-RAG is designed for speedy RAG applications, providing a simple APi for encapsulation, recovery and code analysis. It aids multiple document formats vector information, making it adaptable for numerous use cases. The built-in REST API and cloud API alternatives simplify easy incorporation and positioning of RAG pipelines in both expansion and yielding environments.
These expansions in RAG pipelines emphasize the progressing capabilities and applications of productive AI, making it easier for developers to construct, position and handle sophisticated AI systems across numerous domains.
The Role of Generator and Retriever in RAG Platforms
The retriever and generator plays different but supportive roles in RAG platforms:-
Role of the Retriever: The main function of the retriever is to give appropriate context to the generator. By attaining a large repository, it finds information that can augment the abilities of the generator, allowing more precise, thorough and context-aware responses. By guaranteeing that the generation is grounded in real-world information rather than completely constructing replies, the retriever ensures reliability.
Role of the Generator: Integrating the recovered information into a coherent output constitutes the generator’s role. It takes the context given by the retriever and fabricates replies that are not only appropriate but also engaging to the inquiry. The generator must adjust its responses based on the quality and significance of the information recovered.
How RAG Platforms Employ Natural Language Processing for Information Retrieval and Generation?
RAG platforms apply natural language processing in various key ways:
Information Retrieval: To form efficient queries for the retriever, NLP techniques comprehend and process the users' input. This indulges natural language comprehension, query construction, and sometimes explicit search approaches to ensure that the recovery is appropriate.
Contextual Relevance: NLP is crucial in explaining the recovered details to determine its relevance and usefulness in the context of the current task. This often indulges comprehending variations, entity identification and contextual lodging.
Text Generation: NLP is utilized in the generator to create natural language responses. This indulges leveraging models trained on a wide corpora of text that comprehends grammar, syntax and style, permitting for the generation of responses that are not only situationally relevant but also verbally coherent.
Strategies for RAG Platform Integration
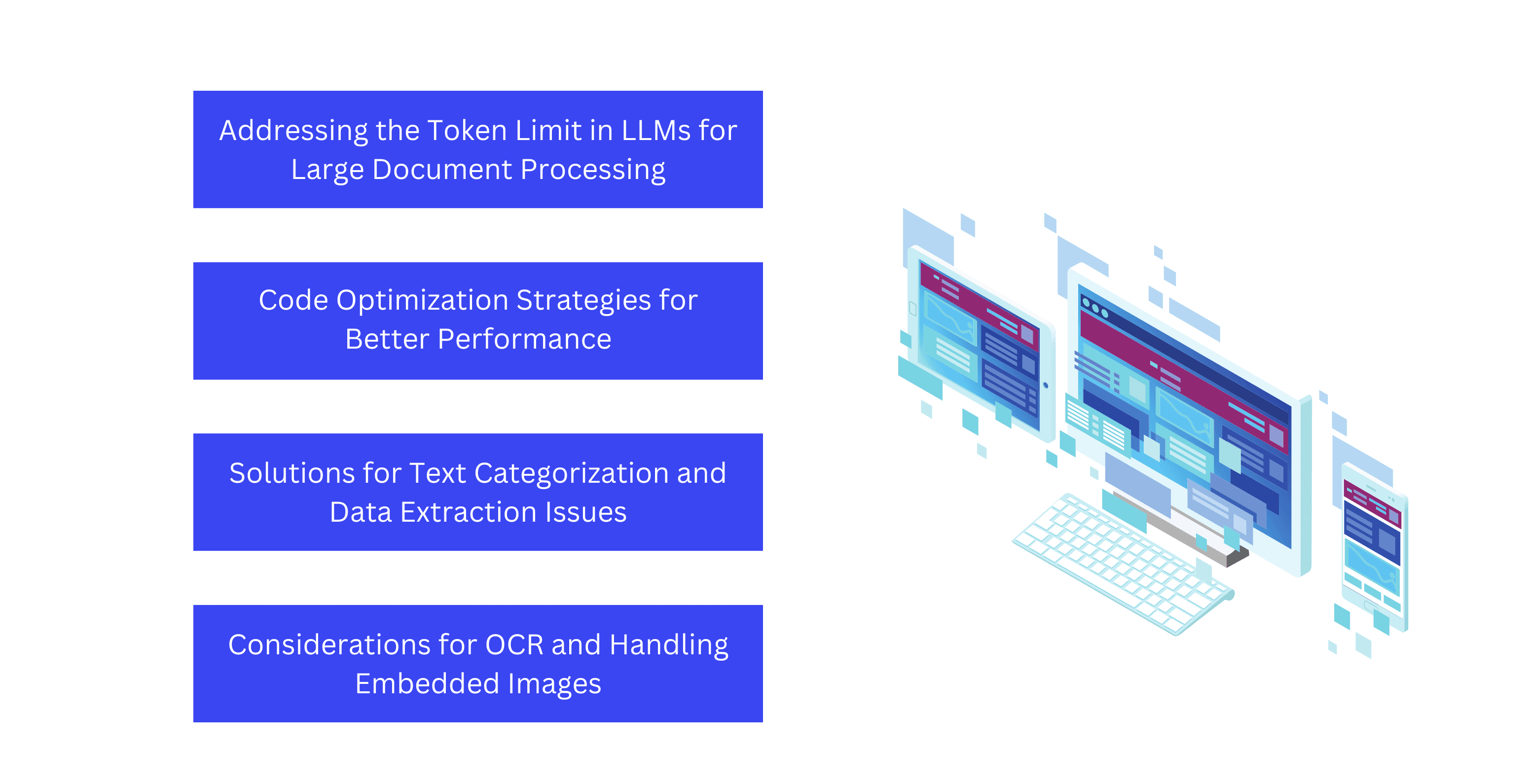
To successfully incorporate a RAG platform into your enterprise systems, you need to follow these strategies:-
Assessing Enterprise Systems for RAG Platform Integration Viability
Assess the existing enterprise systems to judge if they can support the incorporation with RAG platforms. This includes checking the compatibility of the system and receptivity for new technologies.
Integration Planning and Execution
Create a thorough integration plan that includes timelines, resources and milestones. Execute the plan with focus on least disruption to current operations.
Data Management and Compatibility
Ensure that data can be smoothly exchanged between RAG platforms and enterprise systems. Address any congenial problems and set up rules for constant management of data to maintain credibility and attainability.
Use Cases of RAG in Enterprise Systems
RAG can be highly efficient in improving enterprise systems. Here’s how the use cases can be implemented:
Enhancing Knowledge Base and Documentation with RAG for Better Accessibility
As inquiries are being treated, RAG rigorously recovers information from a wide corporate knowledge base. This technique allows the generation of more precise answers, creating internal wikis and documentation more communal and feasible.
Enterprises can ensure that employees find the most appropriate details swiftly, enhancing productivity and decreasing the time spent searching for details, by incorporating RAG.
Automated Code Generation for Software Development within Enterprises
By recovering code snippets and patterns of programming based on the context of the development task, RAG can be used to automate certain aspects of coding.
For instance, when a developer asks how to use a particular feature, RAG can pull instances from internal databases, Stack Overflow, or other documented sources, and create a custom piece that fits the parameters of the projects. This decreases monotonous work and promotes the cycle of development.
Improving Customer Support and Troubleshooting through Efficient Information Retrieval
Using RAG systems can significantly improve customer support. When a support query is received, RAG can promptly recover appropriate troubleshooting guides, part tickets, and solutions from a large repository, helping support agents to give quicker and more precise responses.
The system can also be incorporated into chatbots and virtual assistants that will help to get 24/7 assistance and support to the customers.
Data Management and Vector Data in RAG Integration
Enforcing RAG in enterprise applications indulges careful consideration of the management of data and the efficient use of vector data. Let’s take a look in detail:-
The Importance of Data Quality and Structure for RAG Effectiveness
Data Quality: The quality of data is important for RAG models as it directly affects the model’s capability to recover appropriate information and generate precise text. High-quality data should be precise, up-to-date and bias free.
Data Structure: Assembling data in a way that improves recoverability is important. This means that arranging information in clear, logical formats that a RAG system can easily examine and recover. For instance, forming data in well aligned layouts with compatible fields can significantly enhance the efficacy of data retrieval.
Utilizing Vector Data for Improving Retrieval Functions in Enterprise Applications
Vector Embeddings: RAG models often utilize vector embeddings of the input query and the documents in a knowledge base to find the most appropriate content to accelerate generation.
Improving Retrieval: To improve the functions of retrieval, vector data must be tuned well and upgraded. Approaches like dimensionality reduction can speed up recovery times and enhance the relevance of the retrieved documents.
Strategies for Data Validation and Training to Support RAG Functions
Data Validation: To maintain the integrity and appropriateness of data, regular checks are required.This includes procedures like anomaly detection to spot deviations, coherence checks to ensure data coordination.
Training and Updating: You could constantly train the RAG system on new and updated data to keep the model up-to-date and efficient. This indulges not only initiating the fundamental language models but also updating the document embeddings as new data is integrated.
Challenges and Solutions in Integration
Incorporating RAG platforms into an existing business environment can be a complicated procedure with several challenges that needs to be addressed carefully. So, lets see the challenges and solutions in Integration:-
Common Challenges Faced During RAG Platform Integration
Compatibility Issues: Often, existing IT systems and software may not be directly congenial with new RAG platforms, leading to incorporation challenges.
Data Quality and Integrity: Ensuring the data relocated to or handled by RAG platforms is precise and maintains its credibility is a major challenge.
Resistance to change: Governing resistance from employees usual to existing processes can block the incorporation procedure.
Technical and Organizational Solutions to Overcome Integration Barriers
Middleware solutions: Middleware can help bridge the gap between distinct technologies and provide seamless integration by working as a translator between applications.
Data Cleansing Tools: To ensure that the data being relocated is precise and useful, apply data cleansing tools before incorporation.
Change Management Strategies: Efficient change management strategies, including training of staff and investor management, can help diminish resistance to new systems.
Ensuring Data Security and Privacy Compliance in RAG-enabled Systems
Encryption and Data-Masking: To safeguard sensitive data, both at rest and in movement, utilize encryption and data masking approaches.
Regular Audits: To ensure continuous adherence to appropriate laws and policies, conduct constant security audits and consent reviews.
Access Controls: To limit access to sensitive data and systems, apply rigorous access controls and validation apparatus.
Conclusion
To conclude the guide, the RAG platform integration with existing enterprise systems depicts a crucial growth in improving organizational efficacy and decision-making abilities.
Throughout this guide, we have inspected several methodologies and best practices important for a smooth RAG platform integration.
Main strategies included Assessing enterprise systems for RAG platform integration viability, Planning and executing the integration process and Managing data compatibility and ensuring seamless data flow between RAG platforms and enterprise systems.
By comprehending and implementing RAG platforms in your enterprise systems, you deploy your business at the front line of technological innovation, developed to face the future with the best tools at your disposal.
Integrating RAG platforms with enterprise systems is a bit like trying to put a square peg in a round hole, but with the right tools, anything's possible. Let's dive in and learn about RAG Platform Integration!
Introduction to Integration of RAG Platforms with Enterprise Systems
When you are looking to gear up your enterprise software systems, RAG (Retrieval-Augmented Generation) platforms are gradually becoming important.
By incorporating advanced NLP technologies such as RAG into your existing framework, you not only improve the working but also sleek the procedures to meet the growing demands of the venture landscape.
Overview of RAG Platforms and Their Significance in Enhancing Enterprise Software Systems
RAG platforms (Retrieval-Augmented Generation) are a type of Artificial Intelligence System that improves machine learning models by incorporating recovery abilities directly into the productive process.
This approach leverages a huge database of information, recovering necessary data to accelerate the generation of responses In the conditions of enterprise software systems, RAG platforms offer various important improvements:
Customer Support Systems: RAG Platforms transform customer support systems by giving precise, context-aware answers to customer questions. By recovering pertinent information from comprehensive documentation or knowledge bases, these platforms ensure that support agents and mechanized systems deliver accurate and prompt support, enhancing customer satisfaction and curtailing resolution times.
Decision Support Systems: Integrating RAG platforms into decision support systems improves the coherent abilities of enterprise software. These platforms can recover and consolidate pertinent data points from numerous sources, offering decision-makers with thorough and precise perceptions. This results in more acquainted and efficient planned decisions, boosting the venture growth and diligence.
Document Management and Compliance: RAG platforms simplify document management and compliance processes by effectively recovering relevant regulatory details and documentation. Enterprises can ensure conformity to regulatory needs and industry criterions by using these platforms to access the latest compliance standards and documentation, alleviating the threats of non-compliance and interrelated forfeits.
Research and Development: Enterprise engaged in research and development (R&D) advantage from RAG platforms through improved access to the latest investigation discovery and technical literature. These platforms accelerate the recovery of pertinent studies, trademark, and technical documents, aiding inventiveness, and hastening the expansion of new merchandises and technologies.
Importance of Integrating Advanced NLP Technologies into Existing Enterprise Infrastructure
The advanced NLP technologies integration into enterprise infrastructure is substantially significant for various reasons:
Improved Data Processing and Analysis
Efficient Unstructured Data Handling
Advanced NLP technologies effectively process unstructured data, like emails, reports, social media posts, and customer responses.
By extracting significant information from these disparate data sources, enterprises can gain a pragmatic comprehension of market trends, customer emotions, and internal procedures. This expertise alters the raw data into extravagant perceptions, driving informed decision-making.
Improved Text Mining and Information Recovery
Incorporating NLP technologies improves the text mining and informational recovery procedures within an enterprise.
These technologies can recognize pertinent information from comprehensive document storage, databases, and external sources in real-time. This aptitude ensures that employees and the decision-makers have access to precise and latest information, providing prompt and well-informed decisions.
Optimized Customer Engagement
Advanced Chatbots and Virtual Assistants
Enterprises can position NL–powered chatbots and virtual helpers to offer 24/7 customer support. These intelligent systems comprehend and reciprocate to the queries of the customers in natural language, delivering customized and contextually pertinent support. By managing routine investigation and tasks, NLP technologies free up human support to focus on intricate problems, improving overall customer effectiveness.
Sentiment Analysis and Customer Perceptions
Progressed NLP Technologies permit sentiment analysis, permitting enterprises to gauge customer emotions and beliefs from text data.
By inspecting customer reviews, social media companionship, and support tickets, enterprises can recognize areas for enhancement, observe brand reputation, and tailor their marketing plans to better meet customer requirements.
The Advantages Of RAG Compared to LLMs
Envision this: LLMs are like those know-it-all friends who depend on what they learn in school. On the contrary, RAG Models are like those friends who can also strap out their mobiles and rapidly locate the latest information.
This imperium of RAG models comes from their secret weapon- retrieval. They can access and utilize tons of real-details, from news articles to research papers, to give you the most precise and latest answers.
When it comes to selecting between Retrieval-Augmented Generation (RAG) and standalone Large Language Models (LLMs), it is clear that RAG has some substantial benefits. Let’s know why RAG stands out:
Enhanced Knowledge Access and Accuracy
Real-Time Information Retrieval: RAG Models use real-time retrieval apparatus to access huge external databases or knowledge bases. This expertise ensures that the produced responses are not only relatedly apt but also up-to-date. Unlike LLMs, which are restricted by the stagnant nature of their training data, RAG models can integrate the latest information, substantially improving the accuracy and pertinence of the responses.
Vanquishing Training Data Limitations: LLMs are trained on large but limited sets of data, resulting in intrinsic knowledge cutoff. In comparison, RAG models vigorously recover relevant information from external sources, efficiently skirting the restrictions levied by the stagnant training data. This is specifically advantageous in domains where information swiftly progresses, like technology, medicine and finance.
Enhanced Response Quality and Relevance
Contingent Relevance: By incorporating retrieval mechanisms, RAG models can tailor answers based on precise context of a question. The recovery element pinpoint and provides contingently pertinent documents, which the productive element then uses to craft more accurate and situational cognizant responses. This results in higher-quality yields, contrast to LLMs alone, which might produce credible but not significantly precise answers based on incomplete context.
Fact-Checking and Reduced Hallucination: One of the substantial challenges with LLMs is the tenor to hallucinate-produce genuinely incorrect information positively. RAG models alleviate this by wrecking the produced content in recovered, demonstrable documents. This retrieval-augment approach acts as an annotating mechanism, significantly curtailing the examples of hallucinations and enhancing the dependability of the produced text.
Efficiency and Performance
Computational Efficiency: RAG models can be more arithmetically effective compared to training LLMs on ever-evolving sets of data. The recovery mechanism permits for a more tractable model size since the requirement to entrench all attainable knowledge within the model dimensions is reduced. Instead, pertinent knowledge is retrieved on demand, resulting in better use of computational resources.
Scalability: The flexible nature of RAG models improves scalability. The recovery element can independently scale with the expansion of the knowledge base, without requiring a conforming increase in the produced model. This separation of solicitudes allow for more adaptable and effective scaling plans.
Understanding RAG Platforms
So, what absolutely are RAG platforms? In essence, these systems include 2 main components: a retriever and a generator.
The retriever sieves through a huge database to find pertinent information, while the generator takes this information and crafts replies that are coherent and situationally apt.
This bi-component setup uses natural language processing to improve information recovery and generation, making it a solid tool for any enterprise.
Definition and Components of Retrieval-Augmented Generation Platforms
Retrieval-Augment Generation (RAG) platforms are a hybrid AI approach that merges machine learning models with recovery-based mechanisms to improve language generation tasks. The main components of RAG platforms are:
Retriever: The component’s job is to find appropriate documents from a large collection of data that can help in answering a query. It utilizes information recovery approaches to choose documents or data snippets that are appropriate to the input prompt. The retriever is commonly built on top of search computations and systems of indexing that can effectively manage large volumes of data.
Generator: Once the appropriate information is recovered, the generator utilizes this context to generate a coherent and contextually relevant response. This component is generally a large-scale language model trained on wide datasets to create human-like text based on the input and retrieved details.
Database or Knowledge Base: The retriever standardized or unstandardized data storage. It can indulge a wide range of information, from textual documents to standardized databases, relying on the application.
Integrator or Fusion Component: This part of the system incorporates the output from the retriever with the process of generation, often altering the input to the generator to include the recovered context or influencing the process of generation based strongly on recovered data.
Importance of RAG
Large Language Models (LLMs) are prominent Artificial Intelligence (AI) technology, generating smart chatbots and other NLP apps.
The intention is to generate bots that can respond to user queries in numerous situations by referencing reliable comprehension provenance.
Sadly, the creation of LLM technology acquaints volatility in LLM responses. In addition, LLM training data is stagnant and acquaints a cut-off date on the comprehension it has.
Let’s take a look at the detailed breakdown of why RAG is significant:
Improved Accuracy and Relevance
Incorporation of External Knowledge
RAG Systems uses external data storage, knowledge bases, or documents to recover pertinent data, which is then integrated into the process of generation. This ensures that the produced content is not only coherent but factually precise and latest.
Contextual Awareness
By recovering contextually pertinent information from huge external sources, RAG models can produce responses that are better customized to the precise query or response, resulting in more accurate and contextually pertinent production.
Scalability and Flexibility
Scalability Knowledge Incorporation
RAG models can incorporate enormous and diverse external data sources, making them highly flexible. This adaptability permits them to adjust to distinct domains and applications without requiring comprehensive briefing on domain-specific data.
Adaptive Learning
The recovery element can constantly update with new data, permitting the model to adjust to new data and progressing comprehension without the requirement for constant re-training of the produced element.
Technical Implementation
Dual-Encoder Architecture
RAG usually applies a dual-encoder architecture where one encoder processes the questions and the other encoder processes the recovered documents. This setup permits for effective matching of the questions with pertinent external information, which is then utilized by the produced model to produce the final output.
End-to-End Training
RAG models can be trained end-to-end, upgrading both recovered and generation elements concurrently. This incorporated training approach ensures that the recovered process is impeccably tuned to aid the generation process, resulting in better overall performance.
Latest RAG Pipelines
The latest expansion in Real-Augmented Generation (RAG) pipelines exhibits a spectrum of advanced attributes and incorporations designed to improve the effectiveness and performance of generative AI applications.
R2R Framework: The R2R (RAG to Riches) Framework is a pragmatic tool for rapid expansion, positioning and upgradation of RAG systems. It provides flexible elements like Providers, Pipes and Pipelines, which permits developers to construct and fine-tune high standard RAG applications efficiently. The framework supports assorted use cases from document handling to real-time query responses, improving both adaptability and robustness.
NVIDIA AI Enterprise: NVIDIA’s solution to enterprise-grade RAG applications offers a sturdy infrastructure from moving RAG models from pilot to outputs. The procedure indulges evaluating LLMs, exporting models as api-driven architecture, evolving sample applications, and positioning them in Kubernetes clusters utilizing the NVIDIA RAG LLM Operator. The pragmatic approach ensures that RAG systems are upgraded for performance and smoothly incorporated into existing enterprise landscapes.
Super-RAG: Superagent-ai's Super-RAG is designed for speedy RAG applications, providing a simple APi for encapsulation, recovery and code analysis. It aids multiple document formats vector information, making it adaptable for numerous use cases. The built-in REST API and cloud API alternatives simplify easy incorporation and positioning of RAG pipelines in both expansion and yielding environments.
These expansions in RAG pipelines emphasize the progressing capabilities and applications of productive AI, making it easier for developers to construct, position and handle sophisticated AI systems across numerous domains.
The Role of Generator and Retriever in RAG Platforms
The retriever and generator plays different but supportive roles in RAG platforms:-
Role of the Retriever: The main function of the retriever is to give appropriate context to the generator. By attaining a large repository, it finds information that can augment the abilities of the generator, allowing more precise, thorough and context-aware responses. By guaranteeing that the generation is grounded in real-world information rather than completely constructing replies, the retriever ensures reliability.
Role of the Generator: Integrating the recovered information into a coherent output constitutes the generator’s role. It takes the context given by the retriever and fabricates replies that are not only appropriate but also engaging to the inquiry. The generator must adjust its responses based on the quality and significance of the information recovered.
How RAG Platforms Employ Natural Language Processing for Information Retrieval and Generation?
RAG platforms apply natural language processing in various key ways:
Information Retrieval: To form efficient queries for the retriever, NLP techniques comprehend and process the users' input. This indulges natural language comprehension, query construction, and sometimes explicit search approaches to ensure that the recovery is appropriate.
Contextual Relevance: NLP is crucial in explaining the recovered details to determine its relevance and usefulness in the context of the current task. This often indulges comprehending variations, entity identification and contextual lodging.
Text Generation: NLP is utilized in the generator to create natural language responses. This indulges leveraging models trained on a wide corpora of text that comprehends grammar, syntax and style, permitting for the generation of responses that are not only situationally relevant but also verbally coherent.
Strategies for RAG Platform Integration
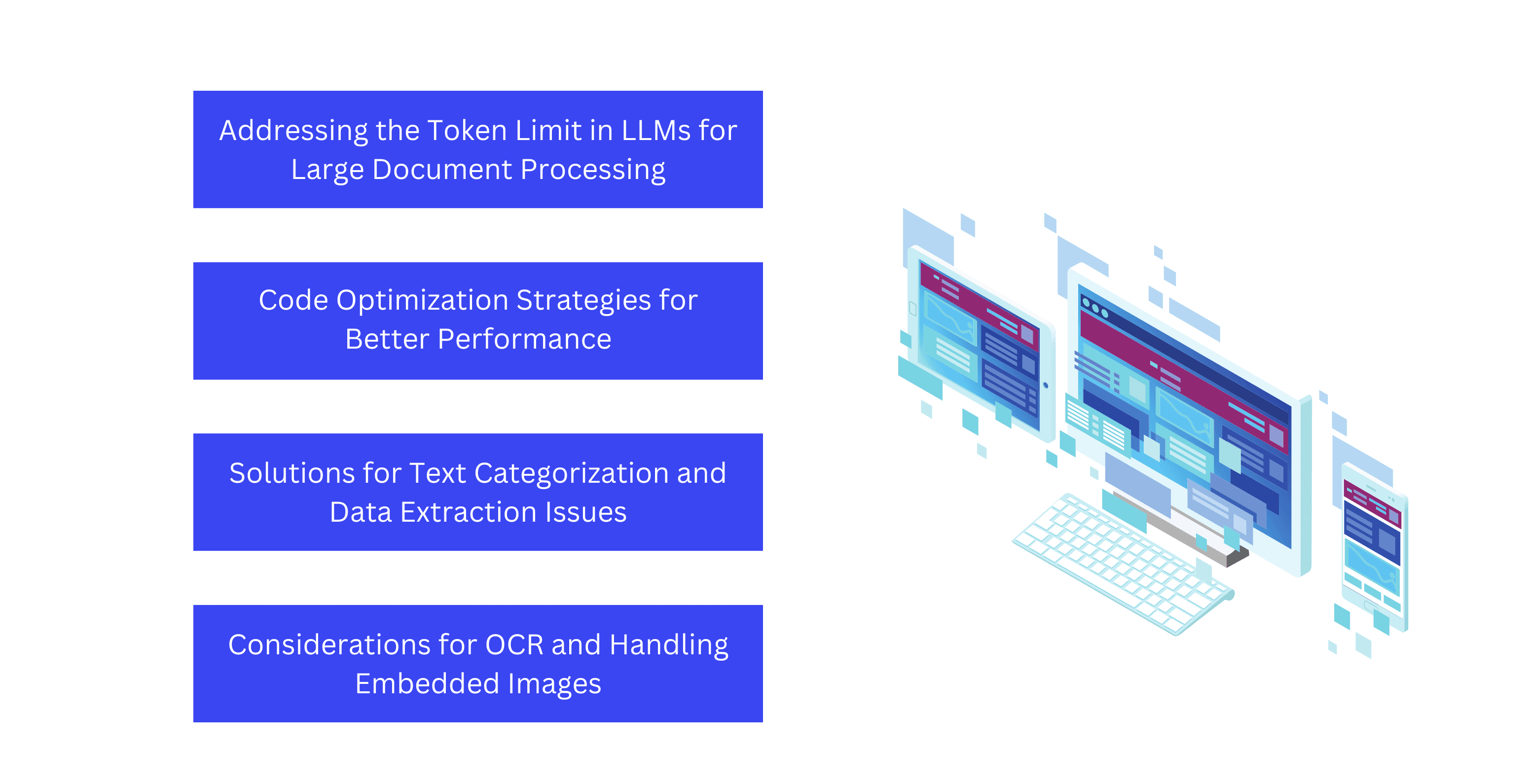
To successfully incorporate a RAG platform into your enterprise systems, you need to follow these strategies:-
Assessing Enterprise Systems for RAG Platform Integration Viability
Assess the existing enterprise systems to judge if they can support the incorporation with RAG platforms. This includes checking the compatibility of the system and receptivity for new technologies.
Integration Planning and Execution
Create a thorough integration plan that includes timelines, resources and milestones. Execute the plan with focus on least disruption to current operations.
Data Management and Compatibility
Ensure that data can be smoothly exchanged between RAG platforms and enterprise systems. Address any congenial problems and set up rules for constant management of data to maintain credibility and attainability.
Use Cases of RAG in Enterprise Systems
RAG can be highly efficient in improving enterprise systems. Here’s how the use cases can be implemented:
Enhancing Knowledge Base and Documentation with RAG for Better Accessibility
As inquiries are being treated, RAG rigorously recovers information from a wide corporate knowledge base. This technique allows the generation of more precise answers, creating internal wikis and documentation more communal and feasible.
Enterprises can ensure that employees find the most appropriate details swiftly, enhancing productivity and decreasing the time spent searching for details, by incorporating RAG.
Automated Code Generation for Software Development within Enterprises
By recovering code snippets and patterns of programming based on the context of the development task, RAG can be used to automate certain aspects of coding.
For instance, when a developer asks how to use a particular feature, RAG can pull instances from internal databases, Stack Overflow, or other documented sources, and create a custom piece that fits the parameters of the projects. This decreases monotonous work and promotes the cycle of development.
Improving Customer Support and Troubleshooting through Efficient Information Retrieval
Using RAG systems can significantly improve customer support. When a support query is received, RAG can promptly recover appropriate troubleshooting guides, part tickets, and solutions from a large repository, helping support agents to give quicker and more precise responses.
The system can also be incorporated into chatbots and virtual assistants that will help to get 24/7 assistance and support to the customers.
Data Management and Vector Data in RAG Integration
Enforcing RAG in enterprise applications indulges careful consideration of the management of data and the efficient use of vector data. Let’s take a look in detail:-
The Importance of Data Quality and Structure for RAG Effectiveness
Data Quality: The quality of data is important for RAG models as it directly affects the model’s capability to recover appropriate information and generate precise text. High-quality data should be precise, up-to-date and bias free.
Data Structure: Assembling data in a way that improves recoverability is important. This means that arranging information in clear, logical formats that a RAG system can easily examine and recover. For instance, forming data in well aligned layouts with compatible fields can significantly enhance the efficacy of data retrieval.
Utilizing Vector Data for Improving Retrieval Functions in Enterprise Applications
Vector Embeddings: RAG models often utilize vector embeddings of the input query and the documents in a knowledge base to find the most appropriate content to accelerate generation.
Improving Retrieval: To improve the functions of retrieval, vector data must be tuned well and upgraded. Approaches like dimensionality reduction can speed up recovery times and enhance the relevance of the retrieved documents.
Strategies for Data Validation and Training to Support RAG Functions
Data Validation: To maintain the integrity and appropriateness of data, regular checks are required.This includes procedures like anomaly detection to spot deviations, coherence checks to ensure data coordination.
Training and Updating: You could constantly train the RAG system on new and updated data to keep the model up-to-date and efficient. This indulges not only initiating the fundamental language models but also updating the document embeddings as new data is integrated.
Challenges and Solutions in Integration
Incorporating RAG platforms into an existing business environment can be a complicated procedure with several challenges that needs to be addressed carefully. So, lets see the challenges and solutions in Integration:-
Common Challenges Faced During RAG Platform Integration
Compatibility Issues: Often, existing IT systems and software may not be directly congenial with new RAG platforms, leading to incorporation challenges.
Data Quality and Integrity: Ensuring the data relocated to or handled by RAG platforms is precise and maintains its credibility is a major challenge.
Resistance to change: Governing resistance from employees usual to existing processes can block the incorporation procedure.
Technical and Organizational Solutions to Overcome Integration Barriers
Middleware solutions: Middleware can help bridge the gap between distinct technologies and provide seamless integration by working as a translator between applications.
Data Cleansing Tools: To ensure that the data being relocated is precise and useful, apply data cleansing tools before incorporation.
Change Management Strategies: Efficient change management strategies, including training of staff and investor management, can help diminish resistance to new systems.
Ensuring Data Security and Privacy Compliance in RAG-enabled Systems
Encryption and Data-Masking: To safeguard sensitive data, both at rest and in movement, utilize encryption and data masking approaches.
Regular Audits: To ensure continuous adherence to appropriate laws and policies, conduct constant security audits and consent reviews.
Access Controls: To limit access to sensitive data and systems, apply rigorous access controls and validation apparatus.
Conclusion
To conclude the guide, the RAG platform integration with existing enterprise systems depicts a crucial growth in improving organizational efficacy and decision-making abilities.
Throughout this guide, we have inspected several methodologies and best practices important for a smooth RAG platform integration.
Main strategies included Assessing enterprise systems for RAG platform integration viability, Planning and executing the integration process and Managing data compatibility and ensuring seamless data flow between RAG platforms and enterprise systems.
By comprehending and implementing RAG platforms in your enterprise systems, you deploy your business at the front line of technological innovation, developed to face the future with the best tools at your disposal.
Integrating RAG platforms with enterprise systems is a bit like trying to put a square peg in a round hole, but with the right tools, anything's possible. Let's dive in and learn about RAG Platform Integration!
Introduction to Integration of RAG Platforms with Enterprise Systems
When you are looking to gear up your enterprise software systems, RAG (Retrieval-Augmented Generation) platforms are gradually becoming important.
By incorporating advanced NLP technologies such as RAG into your existing framework, you not only improve the working but also sleek the procedures to meet the growing demands of the venture landscape.
Overview of RAG Platforms and Their Significance in Enhancing Enterprise Software Systems
RAG platforms (Retrieval-Augmented Generation) are a type of Artificial Intelligence System that improves machine learning models by incorporating recovery abilities directly into the productive process.
This approach leverages a huge database of information, recovering necessary data to accelerate the generation of responses In the conditions of enterprise software systems, RAG platforms offer various important improvements:
Customer Support Systems: RAG Platforms transform customer support systems by giving precise, context-aware answers to customer questions. By recovering pertinent information from comprehensive documentation or knowledge bases, these platforms ensure that support agents and mechanized systems deliver accurate and prompt support, enhancing customer satisfaction and curtailing resolution times.
Decision Support Systems: Integrating RAG platforms into decision support systems improves the coherent abilities of enterprise software. These platforms can recover and consolidate pertinent data points from numerous sources, offering decision-makers with thorough and precise perceptions. This results in more acquainted and efficient planned decisions, boosting the venture growth and diligence.
Document Management and Compliance: RAG platforms simplify document management and compliance processes by effectively recovering relevant regulatory details and documentation. Enterprises can ensure conformity to regulatory needs and industry criterions by using these platforms to access the latest compliance standards and documentation, alleviating the threats of non-compliance and interrelated forfeits.
Research and Development: Enterprise engaged in research and development (R&D) advantage from RAG platforms through improved access to the latest investigation discovery and technical literature. These platforms accelerate the recovery of pertinent studies, trademark, and technical documents, aiding inventiveness, and hastening the expansion of new merchandises and technologies.
Importance of Integrating Advanced NLP Technologies into Existing Enterprise Infrastructure
The advanced NLP technologies integration into enterprise infrastructure is substantially significant for various reasons:
Improved Data Processing and Analysis
Efficient Unstructured Data Handling
Advanced NLP technologies effectively process unstructured data, like emails, reports, social media posts, and customer responses.
By extracting significant information from these disparate data sources, enterprises can gain a pragmatic comprehension of market trends, customer emotions, and internal procedures. This expertise alters the raw data into extravagant perceptions, driving informed decision-making.
Improved Text Mining and Information Recovery
Incorporating NLP technologies improves the text mining and informational recovery procedures within an enterprise.
These technologies can recognize pertinent information from comprehensive document storage, databases, and external sources in real-time. This aptitude ensures that employees and the decision-makers have access to precise and latest information, providing prompt and well-informed decisions.
Optimized Customer Engagement
Advanced Chatbots and Virtual Assistants
Enterprises can position NL–powered chatbots and virtual helpers to offer 24/7 customer support. These intelligent systems comprehend and reciprocate to the queries of the customers in natural language, delivering customized and contextually pertinent support. By managing routine investigation and tasks, NLP technologies free up human support to focus on intricate problems, improving overall customer effectiveness.
Sentiment Analysis and Customer Perceptions
Progressed NLP Technologies permit sentiment analysis, permitting enterprises to gauge customer emotions and beliefs from text data.
By inspecting customer reviews, social media companionship, and support tickets, enterprises can recognize areas for enhancement, observe brand reputation, and tailor their marketing plans to better meet customer requirements.
The Advantages Of RAG Compared to LLMs
Envision this: LLMs are like those know-it-all friends who depend on what they learn in school. On the contrary, RAG Models are like those friends who can also strap out their mobiles and rapidly locate the latest information.
This imperium of RAG models comes from their secret weapon- retrieval. They can access and utilize tons of real-details, from news articles to research papers, to give you the most precise and latest answers.
When it comes to selecting between Retrieval-Augmented Generation (RAG) and standalone Large Language Models (LLMs), it is clear that RAG has some substantial benefits. Let’s know why RAG stands out:
Enhanced Knowledge Access and Accuracy
Real-Time Information Retrieval: RAG Models use real-time retrieval apparatus to access huge external databases or knowledge bases. This expertise ensures that the produced responses are not only relatedly apt but also up-to-date. Unlike LLMs, which are restricted by the stagnant nature of their training data, RAG models can integrate the latest information, substantially improving the accuracy and pertinence of the responses.
Vanquishing Training Data Limitations: LLMs are trained on large but limited sets of data, resulting in intrinsic knowledge cutoff. In comparison, RAG models vigorously recover relevant information from external sources, efficiently skirting the restrictions levied by the stagnant training data. This is specifically advantageous in domains where information swiftly progresses, like technology, medicine and finance.
Enhanced Response Quality and Relevance
Contingent Relevance: By incorporating retrieval mechanisms, RAG models can tailor answers based on precise context of a question. The recovery element pinpoint and provides contingently pertinent documents, which the productive element then uses to craft more accurate and situational cognizant responses. This results in higher-quality yields, contrast to LLMs alone, which might produce credible but not significantly precise answers based on incomplete context.
Fact-Checking and Reduced Hallucination: One of the substantial challenges with LLMs is the tenor to hallucinate-produce genuinely incorrect information positively. RAG models alleviate this by wrecking the produced content in recovered, demonstrable documents. This retrieval-augment approach acts as an annotating mechanism, significantly curtailing the examples of hallucinations and enhancing the dependability of the produced text.
Efficiency and Performance
Computational Efficiency: RAG models can be more arithmetically effective compared to training LLMs on ever-evolving sets of data. The recovery mechanism permits for a more tractable model size since the requirement to entrench all attainable knowledge within the model dimensions is reduced. Instead, pertinent knowledge is retrieved on demand, resulting in better use of computational resources.
Scalability: The flexible nature of RAG models improves scalability. The recovery element can independently scale with the expansion of the knowledge base, without requiring a conforming increase in the produced model. This separation of solicitudes allow for more adaptable and effective scaling plans.
Understanding RAG Platforms
So, what absolutely are RAG platforms? In essence, these systems include 2 main components: a retriever and a generator.
The retriever sieves through a huge database to find pertinent information, while the generator takes this information and crafts replies that are coherent and situationally apt.
This bi-component setup uses natural language processing to improve information recovery and generation, making it a solid tool for any enterprise.
Definition and Components of Retrieval-Augmented Generation Platforms
Retrieval-Augment Generation (RAG) platforms are a hybrid AI approach that merges machine learning models with recovery-based mechanisms to improve language generation tasks. The main components of RAG platforms are:
Retriever: The component’s job is to find appropriate documents from a large collection of data that can help in answering a query. It utilizes information recovery approaches to choose documents or data snippets that are appropriate to the input prompt. The retriever is commonly built on top of search computations and systems of indexing that can effectively manage large volumes of data.
Generator: Once the appropriate information is recovered, the generator utilizes this context to generate a coherent and contextually relevant response. This component is generally a large-scale language model trained on wide datasets to create human-like text based on the input and retrieved details.
Database or Knowledge Base: The retriever standardized or unstandardized data storage. It can indulge a wide range of information, from textual documents to standardized databases, relying on the application.
Integrator or Fusion Component: This part of the system incorporates the output from the retriever with the process of generation, often altering the input to the generator to include the recovered context or influencing the process of generation based strongly on recovered data.
Importance of RAG
Large Language Models (LLMs) are prominent Artificial Intelligence (AI) technology, generating smart chatbots and other NLP apps.
The intention is to generate bots that can respond to user queries in numerous situations by referencing reliable comprehension provenance.
Sadly, the creation of LLM technology acquaints volatility in LLM responses. In addition, LLM training data is stagnant and acquaints a cut-off date on the comprehension it has.
Let’s take a look at the detailed breakdown of why RAG is significant:
Improved Accuracy and Relevance
Incorporation of External Knowledge
RAG Systems uses external data storage, knowledge bases, or documents to recover pertinent data, which is then integrated into the process of generation. This ensures that the produced content is not only coherent but factually precise and latest.
Contextual Awareness
By recovering contextually pertinent information from huge external sources, RAG models can produce responses that are better customized to the precise query or response, resulting in more accurate and contextually pertinent production.
Scalability and Flexibility
Scalability Knowledge Incorporation
RAG models can incorporate enormous and diverse external data sources, making them highly flexible. This adaptability permits them to adjust to distinct domains and applications without requiring comprehensive briefing on domain-specific data.
Adaptive Learning
The recovery element can constantly update with new data, permitting the model to adjust to new data and progressing comprehension without the requirement for constant re-training of the produced element.
Technical Implementation
Dual-Encoder Architecture
RAG usually applies a dual-encoder architecture where one encoder processes the questions and the other encoder processes the recovered documents. This setup permits for effective matching of the questions with pertinent external information, which is then utilized by the produced model to produce the final output.
End-to-End Training
RAG models can be trained end-to-end, upgrading both recovered and generation elements concurrently. This incorporated training approach ensures that the recovered process is impeccably tuned to aid the generation process, resulting in better overall performance.
Latest RAG Pipelines
The latest expansion in Real-Augmented Generation (RAG) pipelines exhibits a spectrum of advanced attributes and incorporations designed to improve the effectiveness and performance of generative AI applications.
R2R Framework: The R2R (RAG to Riches) Framework is a pragmatic tool for rapid expansion, positioning and upgradation of RAG systems. It provides flexible elements like Providers, Pipes and Pipelines, which permits developers to construct and fine-tune high standard RAG applications efficiently. The framework supports assorted use cases from document handling to real-time query responses, improving both adaptability and robustness.
NVIDIA AI Enterprise: NVIDIA’s solution to enterprise-grade RAG applications offers a sturdy infrastructure from moving RAG models from pilot to outputs. The procedure indulges evaluating LLMs, exporting models as api-driven architecture, evolving sample applications, and positioning them in Kubernetes clusters utilizing the NVIDIA RAG LLM Operator. The pragmatic approach ensures that RAG systems are upgraded for performance and smoothly incorporated into existing enterprise landscapes.
Super-RAG: Superagent-ai's Super-RAG is designed for speedy RAG applications, providing a simple APi for encapsulation, recovery and code analysis. It aids multiple document formats vector information, making it adaptable for numerous use cases. The built-in REST API and cloud API alternatives simplify easy incorporation and positioning of RAG pipelines in both expansion and yielding environments.
These expansions in RAG pipelines emphasize the progressing capabilities and applications of productive AI, making it easier for developers to construct, position and handle sophisticated AI systems across numerous domains.
The Role of Generator and Retriever in RAG Platforms
The retriever and generator plays different but supportive roles in RAG platforms:-
Role of the Retriever: The main function of the retriever is to give appropriate context to the generator. By attaining a large repository, it finds information that can augment the abilities of the generator, allowing more precise, thorough and context-aware responses. By guaranteeing that the generation is grounded in real-world information rather than completely constructing replies, the retriever ensures reliability.
Role of the Generator: Integrating the recovered information into a coherent output constitutes the generator’s role. It takes the context given by the retriever and fabricates replies that are not only appropriate but also engaging to the inquiry. The generator must adjust its responses based on the quality and significance of the information recovered.
How RAG Platforms Employ Natural Language Processing for Information Retrieval and Generation?
RAG platforms apply natural language processing in various key ways:
Information Retrieval: To form efficient queries for the retriever, NLP techniques comprehend and process the users' input. This indulges natural language comprehension, query construction, and sometimes explicit search approaches to ensure that the recovery is appropriate.
Contextual Relevance: NLP is crucial in explaining the recovered details to determine its relevance and usefulness in the context of the current task. This often indulges comprehending variations, entity identification and contextual lodging.
Text Generation: NLP is utilized in the generator to create natural language responses. This indulges leveraging models trained on a wide corpora of text that comprehends grammar, syntax and style, permitting for the generation of responses that are not only situationally relevant but also verbally coherent.
Strategies for RAG Platform Integration
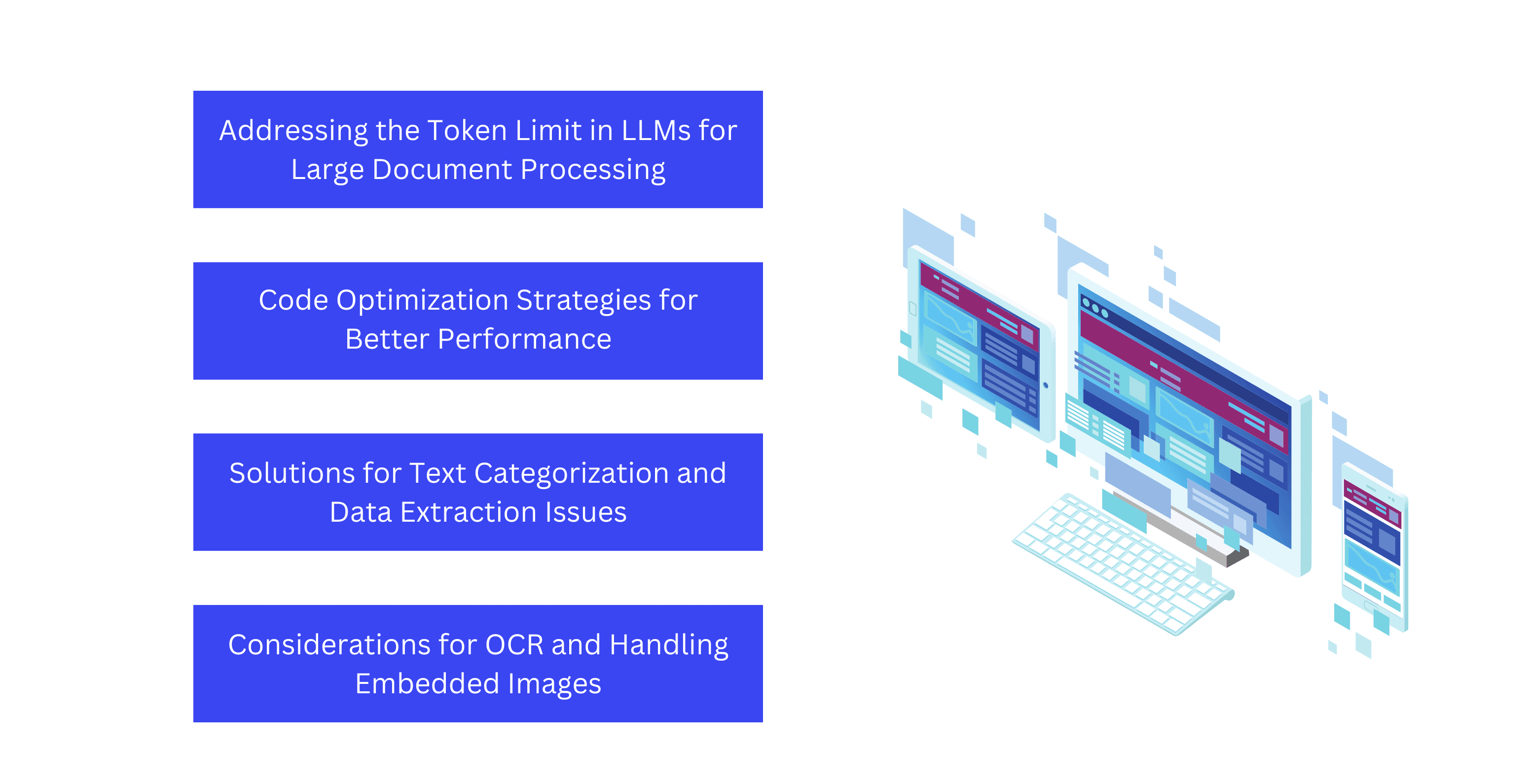
To successfully incorporate a RAG platform into your enterprise systems, you need to follow these strategies:-
Assessing Enterprise Systems for RAG Platform Integration Viability
Assess the existing enterprise systems to judge if they can support the incorporation with RAG platforms. This includes checking the compatibility of the system and receptivity for new technologies.
Integration Planning and Execution
Create a thorough integration plan that includes timelines, resources and milestones. Execute the plan with focus on least disruption to current operations.
Data Management and Compatibility
Ensure that data can be smoothly exchanged between RAG platforms and enterprise systems. Address any congenial problems and set up rules for constant management of data to maintain credibility and attainability.
Use Cases of RAG in Enterprise Systems
RAG can be highly efficient in improving enterprise systems. Here’s how the use cases can be implemented:
Enhancing Knowledge Base and Documentation with RAG for Better Accessibility
As inquiries are being treated, RAG rigorously recovers information from a wide corporate knowledge base. This technique allows the generation of more precise answers, creating internal wikis and documentation more communal and feasible.
Enterprises can ensure that employees find the most appropriate details swiftly, enhancing productivity and decreasing the time spent searching for details, by incorporating RAG.
Automated Code Generation for Software Development within Enterprises
By recovering code snippets and patterns of programming based on the context of the development task, RAG can be used to automate certain aspects of coding.
For instance, when a developer asks how to use a particular feature, RAG can pull instances from internal databases, Stack Overflow, or other documented sources, and create a custom piece that fits the parameters of the projects. This decreases monotonous work and promotes the cycle of development.
Improving Customer Support and Troubleshooting through Efficient Information Retrieval
Using RAG systems can significantly improve customer support. When a support query is received, RAG can promptly recover appropriate troubleshooting guides, part tickets, and solutions from a large repository, helping support agents to give quicker and more precise responses.
The system can also be incorporated into chatbots and virtual assistants that will help to get 24/7 assistance and support to the customers.
Data Management and Vector Data in RAG Integration
Enforcing RAG in enterprise applications indulges careful consideration of the management of data and the efficient use of vector data. Let’s take a look in detail:-
The Importance of Data Quality and Structure for RAG Effectiveness
Data Quality: The quality of data is important for RAG models as it directly affects the model’s capability to recover appropriate information and generate precise text. High-quality data should be precise, up-to-date and bias free.
Data Structure: Assembling data in a way that improves recoverability is important. This means that arranging information in clear, logical formats that a RAG system can easily examine and recover. For instance, forming data in well aligned layouts with compatible fields can significantly enhance the efficacy of data retrieval.
Utilizing Vector Data for Improving Retrieval Functions in Enterprise Applications
Vector Embeddings: RAG models often utilize vector embeddings of the input query and the documents in a knowledge base to find the most appropriate content to accelerate generation.
Improving Retrieval: To improve the functions of retrieval, vector data must be tuned well and upgraded. Approaches like dimensionality reduction can speed up recovery times and enhance the relevance of the retrieved documents.
Strategies for Data Validation and Training to Support RAG Functions
Data Validation: To maintain the integrity and appropriateness of data, regular checks are required.This includes procedures like anomaly detection to spot deviations, coherence checks to ensure data coordination.
Training and Updating: You could constantly train the RAG system on new and updated data to keep the model up-to-date and efficient. This indulges not only initiating the fundamental language models but also updating the document embeddings as new data is integrated.
Challenges and Solutions in Integration
Incorporating RAG platforms into an existing business environment can be a complicated procedure with several challenges that needs to be addressed carefully. So, lets see the challenges and solutions in Integration:-
Common Challenges Faced During RAG Platform Integration
Compatibility Issues: Often, existing IT systems and software may not be directly congenial with new RAG platforms, leading to incorporation challenges.
Data Quality and Integrity: Ensuring the data relocated to or handled by RAG platforms is precise and maintains its credibility is a major challenge.
Resistance to change: Governing resistance from employees usual to existing processes can block the incorporation procedure.
Technical and Organizational Solutions to Overcome Integration Barriers
Middleware solutions: Middleware can help bridge the gap between distinct technologies and provide seamless integration by working as a translator between applications.
Data Cleansing Tools: To ensure that the data being relocated is precise and useful, apply data cleansing tools before incorporation.
Change Management Strategies: Efficient change management strategies, including training of staff and investor management, can help diminish resistance to new systems.
Ensuring Data Security and Privacy Compliance in RAG-enabled Systems
Encryption and Data-Masking: To safeguard sensitive data, both at rest and in movement, utilize encryption and data masking approaches.
Regular Audits: To ensure continuous adherence to appropriate laws and policies, conduct constant security audits and consent reviews.
Access Controls: To limit access to sensitive data and systems, apply rigorous access controls and validation apparatus.
Conclusion
To conclude the guide, the RAG platform integration with existing enterprise systems depicts a crucial growth in improving organizational efficacy and decision-making abilities.
Throughout this guide, we have inspected several methodologies and best practices important for a smooth RAG platform integration.
Main strategies included Assessing enterprise systems for RAG platform integration viability, Planning and executing the integration process and Managing data compatibility and ensuring seamless data flow between RAG platforms and enterprise systems.
By comprehending and implementing RAG platforms in your enterprise systems, you deploy your business at the front line of technological innovation, developed to face the future with the best tools at your disposal.
Integrating RAG platforms with enterprise systems is a bit like trying to put a square peg in a round hole, but with the right tools, anything's possible. Let's dive in and learn about RAG Platform Integration!
Introduction to Integration of RAG Platforms with Enterprise Systems
When you are looking to gear up your enterprise software systems, RAG (Retrieval-Augmented Generation) platforms are gradually becoming important.
By incorporating advanced NLP technologies such as RAG into your existing framework, you not only improve the working but also sleek the procedures to meet the growing demands of the venture landscape.
Overview of RAG Platforms and Their Significance in Enhancing Enterprise Software Systems
RAG platforms (Retrieval-Augmented Generation) are a type of Artificial Intelligence System that improves machine learning models by incorporating recovery abilities directly into the productive process.
This approach leverages a huge database of information, recovering necessary data to accelerate the generation of responses In the conditions of enterprise software systems, RAG platforms offer various important improvements:
Customer Support Systems: RAG Platforms transform customer support systems by giving precise, context-aware answers to customer questions. By recovering pertinent information from comprehensive documentation or knowledge bases, these platforms ensure that support agents and mechanized systems deliver accurate and prompt support, enhancing customer satisfaction and curtailing resolution times.
Decision Support Systems: Integrating RAG platforms into decision support systems improves the coherent abilities of enterprise software. These platforms can recover and consolidate pertinent data points from numerous sources, offering decision-makers with thorough and precise perceptions. This results in more acquainted and efficient planned decisions, boosting the venture growth and diligence.
Document Management and Compliance: RAG platforms simplify document management and compliance processes by effectively recovering relevant regulatory details and documentation. Enterprises can ensure conformity to regulatory needs and industry criterions by using these platforms to access the latest compliance standards and documentation, alleviating the threats of non-compliance and interrelated forfeits.
Research and Development: Enterprise engaged in research and development (R&D) advantage from RAG platforms through improved access to the latest investigation discovery and technical literature. These platforms accelerate the recovery of pertinent studies, trademark, and technical documents, aiding inventiveness, and hastening the expansion of new merchandises and technologies.
Importance of Integrating Advanced NLP Technologies into Existing Enterprise Infrastructure
The advanced NLP technologies integration into enterprise infrastructure is substantially significant for various reasons:
Improved Data Processing and Analysis
Efficient Unstructured Data Handling
Advanced NLP technologies effectively process unstructured data, like emails, reports, social media posts, and customer responses.
By extracting significant information from these disparate data sources, enterprises can gain a pragmatic comprehension of market trends, customer emotions, and internal procedures. This expertise alters the raw data into extravagant perceptions, driving informed decision-making.
Improved Text Mining and Information Recovery
Incorporating NLP technologies improves the text mining and informational recovery procedures within an enterprise.
These technologies can recognize pertinent information from comprehensive document storage, databases, and external sources in real-time. This aptitude ensures that employees and the decision-makers have access to precise and latest information, providing prompt and well-informed decisions.
Optimized Customer Engagement
Advanced Chatbots and Virtual Assistants
Enterprises can position NL–powered chatbots and virtual helpers to offer 24/7 customer support. These intelligent systems comprehend and reciprocate to the queries of the customers in natural language, delivering customized and contextually pertinent support. By managing routine investigation and tasks, NLP technologies free up human support to focus on intricate problems, improving overall customer effectiveness.
Sentiment Analysis and Customer Perceptions
Progressed NLP Technologies permit sentiment analysis, permitting enterprises to gauge customer emotions and beliefs from text data.
By inspecting customer reviews, social media companionship, and support tickets, enterprises can recognize areas for enhancement, observe brand reputation, and tailor their marketing plans to better meet customer requirements.
The Advantages Of RAG Compared to LLMs
Envision this: LLMs are like those know-it-all friends who depend on what they learn in school. On the contrary, RAG Models are like those friends who can also strap out their mobiles and rapidly locate the latest information.
This imperium of RAG models comes from their secret weapon- retrieval. They can access and utilize tons of real-details, from news articles to research papers, to give you the most precise and latest answers.
When it comes to selecting between Retrieval-Augmented Generation (RAG) and standalone Large Language Models (LLMs), it is clear that RAG has some substantial benefits. Let’s know why RAG stands out:
Enhanced Knowledge Access and Accuracy
Real-Time Information Retrieval: RAG Models use real-time retrieval apparatus to access huge external databases or knowledge bases. This expertise ensures that the produced responses are not only relatedly apt but also up-to-date. Unlike LLMs, which are restricted by the stagnant nature of their training data, RAG models can integrate the latest information, substantially improving the accuracy and pertinence of the responses.
Vanquishing Training Data Limitations: LLMs are trained on large but limited sets of data, resulting in intrinsic knowledge cutoff. In comparison, RAG models vigorously recover relevant information from external sources, efficiently skirting the restrictions levied by the stagnant training data. This is specifically advantageous in domains where information swiftly progresses, like technology, medicine and finance.
Enhanced Response Quality and Relevance
Contingent Relevance: By incorporating retrieval mechanisms, RAG models can tailor answers based on precise context of a question. The recovery element pinpoint and provides contingently pertinent documents, which the productive element then uses to craft more accurate and situational cognizant responses. This results in higher-quality yields, contrast to LLMs alone, which might produce credible but not significantly precise answers based on incomplete context.
Fact-Checking and Reduced Hallucination: One of the substantial challenges with LLMs is the tenor to hallucinate-produce genuinely incorrect information positively. RAG models alleviate this by wrecking the produced content in recovered, demonstrable documents. This retrieval-augment approach acts as an annotating mechanism, significantly curtailing the examples of hallucinations and enhancing the dependability of the produced text.
Efficiency and Performance
Computational Efficiency: RAG models can be more arithmetically effective compared to training LLMs on ever-evolving sets of data. The recovery mechanism permits for a more tractable model size since the requirement to entrench all attainable knowledge within the model dimensions is reduced. Instead, pertinent knowledge is retrieved on demand, resulting in better use of computational resources.
Scalability: The flexible nature of RAG models improves scalability. The recovery element can independently scale with the expansion of the knowledge base, without requiring a conforming increase in the produced model. This separation of solicitudes allow for more adaptable and effective scaling plans.
Understanding RAG Platforms
So, what absolutely are RAG platforms? In essence, these systems include 2 main components: a retriever and a generator.
The retriever sieves through a huge database to find pertinent information, while the generator takes this information and crafts replies that are coherent and situationally apt.
This bi-component setup uses natural language processing to improve information recovery and generation, making it a solid tool for any enterprise.
Definition and Components of Retrieval-Augmented Generation Platforms
Retrieval-Augment Generation (RAG) platforms are a hybrid AI approach that merges machine learning models with recovery-based mechanisms to improve language generation tasks. The main components of RAG platforms are:
Retriever: The component’s job is to find appropriate documents from a large collection of data that can help in answering a query. It utilizes information recovery approaches to choose documents or data snippets that are appropriate to the input prompt. The retriever is commonly built on top of search computations and systems of indexing that can effectively manage large volumes of data.
Generator: Once the appropriate information is recovered, the generator utilizes this context to generate a coherent and contextually relevant response. This component is generally a large-scale language model trained on wide datasets to create human-like text based on the input and retrieved details.
Database or Knowledge Base: The retriever standardized or unstandardized data storage. It can indulge a wide range of information, from textual documents to standardized databases, relying on the application.
Integrator or Fusion Component: This part of the system incorporates the output from the retriever with the process of generation, often altering the input to the generator to include the recovered context or influencing the process of generation based strongly on recovered data.
Importance of RAG
Large Language Models (LLMs) are prominent Artificial Intelligence (AI) technology, generating smart chatbots and other NLP apps.
The intention is to generate bots that can respond to user queries in numerous situations by referencing reliable comprehension provenance.
Sadly, the creation of LLM technology acquaints volatility in LLM responses. In addition, LLM training data is stagnant and acquaints a cut-off date on the comprehension it has.
Let’s take a look at the detailed breakdown of why RAG is significant:
Improved Accuracy and Relevance
Incorporation of External Knowledge
RAG Systems uses external data storage, knowledge bases, or documents to recover pertinent data, which is then integrated into the process of generation. This ensures that the produced content is not only coherent but factually precise and latest.
Contextual Awareness
By recovering contextually pertinent information from huge external sources, RAG models can produce responses that are better customized to the precise query or response, resulting in more accurate and contextually pertinent production.
Scalability and Flexibility
Scalability Knowledge Incorporation
RAG models can incorporate enormous and diverse external data sources, making them highly flexible. This adaptability permits them to adjust to distinct domains and applications without requiring comprehensive briefing on domain-specific data.
Adaptive Learning
The recovery element can constantly update with new data, permitting the model to adjust to new data and progressing comprehension without the requirement for constant re-training of the produced element.
Technical Implementation
Dual-Encoder Architecture
RAG usually applies a dual-encoder architecture where one encoder processes the questions and the other encoder processes the recovered documents. This setup permits for effective matching of the questions with pertinent external information, which is then utilized by the produced model to produce the final output.
End-to-End Training
RAG models can be trained end-to-end, upgrading both recovered and generation elements concurrently. This incorporated training approach ensures that the recovered process is impeccably tuned to aid the generation process, resulting in better overall performance.
Latest RAG Pipelines
The latest expansion in Real-Augmented Generation (RAG) pipelines exhibits a spectrum of advanced attributes and incorporations designed to improve the effectiveness and performance of generative AI applications.
R2R Framework: The R2R (RAG to Riches) Framework is a pragmatic tool for rapid expansion, positioning and upgradation of RAG systems. It provides flexible elements like Providers, Pipes and Pipelines, which permits developers to construct and fine-tune high standard RAG applications efficiently. The framework supports assorted use cases from document handling to real-time query responses, improving both adaptability and robustness.
NVIDIA AI Enterprise: NVIDIA’s solution to enterprise-grade RAG applications offers a sturdy infrastructure from moving RAG models from pilot to outputs. The procedure indulges evaluating LLMs, exporting models as api-driven architecture, evolving sample applications, and positioning them in Kubernetes clusters utilizing the NVIDIA RAG LLM Operator. The pragmatic approach ensures that RAG systems are upgraded for performance and smoothly incorporated into existing enterprise landscapes.
Super-RAG: Superagent-ai's Super-RAG is designed for speedy RAG applications, providing a simple APi for encapsulation, recovery and code analysis. It aids multiple document formats vector information, making it adaptable for numerous use cases. The built-in REST API and cloud API alternatives simplify easy incorporation and positioning of RAG pipelines in both expansion and yielding environments.
These expansions in RAG pipelines emphasize the progressing capabilities and applications of productive AI, making it easier for developers to construct, position and handle sophisticated AI systems across numerous domains.
The Role of Generator and Retriever in RAG Platforms
The retriever and generator plays different but supportive roles in RAG platforms:-
Role of the Retriever: The main function of the retriever is to give appropriate context to the generator. By attaining a large repository, it finds information that can augment the abilities of the generator, allowing more precise, thorough and context-aware responses. By guaranteeing that the generation is grounded in real-world information rather than completely constructing replies, the retriever ensures reliability.
Role of the Generator: Integrating the recovered information into a coherent output constitutes the generator’s role. It takes the context given by the retriever and fabricates replies that are not only appropriate but also engaging to the inquiry. The generator must adjust its responses based on the quality and significance of the information recovered.
How RAG Platforms Employ Natural Language Processing for Information Retrieval and Generation?
RAG platforms apply natural language processing in various key ways:
Information Retrieval: To form efficient queries for the retriever, NLP techniques comprehend and process the users' input. This indulges natural language comprehension, query construction, and sometimes explicit search approaches to ensure that the recovery is appropriate.
Contextual Relevance: NLP is crucial in explaining the recovered details to determine its relevance and usefulness in the context of the current task. This often indulges comprehending variations, entity identification and contextual lodging.
Text Generation: NLP is utilized in the generator to create natural language responses. This indulges leveraging models trained on a wide corpora of text that comprehends grammar, syntax and style, permitting for the generation of responses that are not only situationally relevant but also verbally coherent.
Strategies for RAG Platform Integration
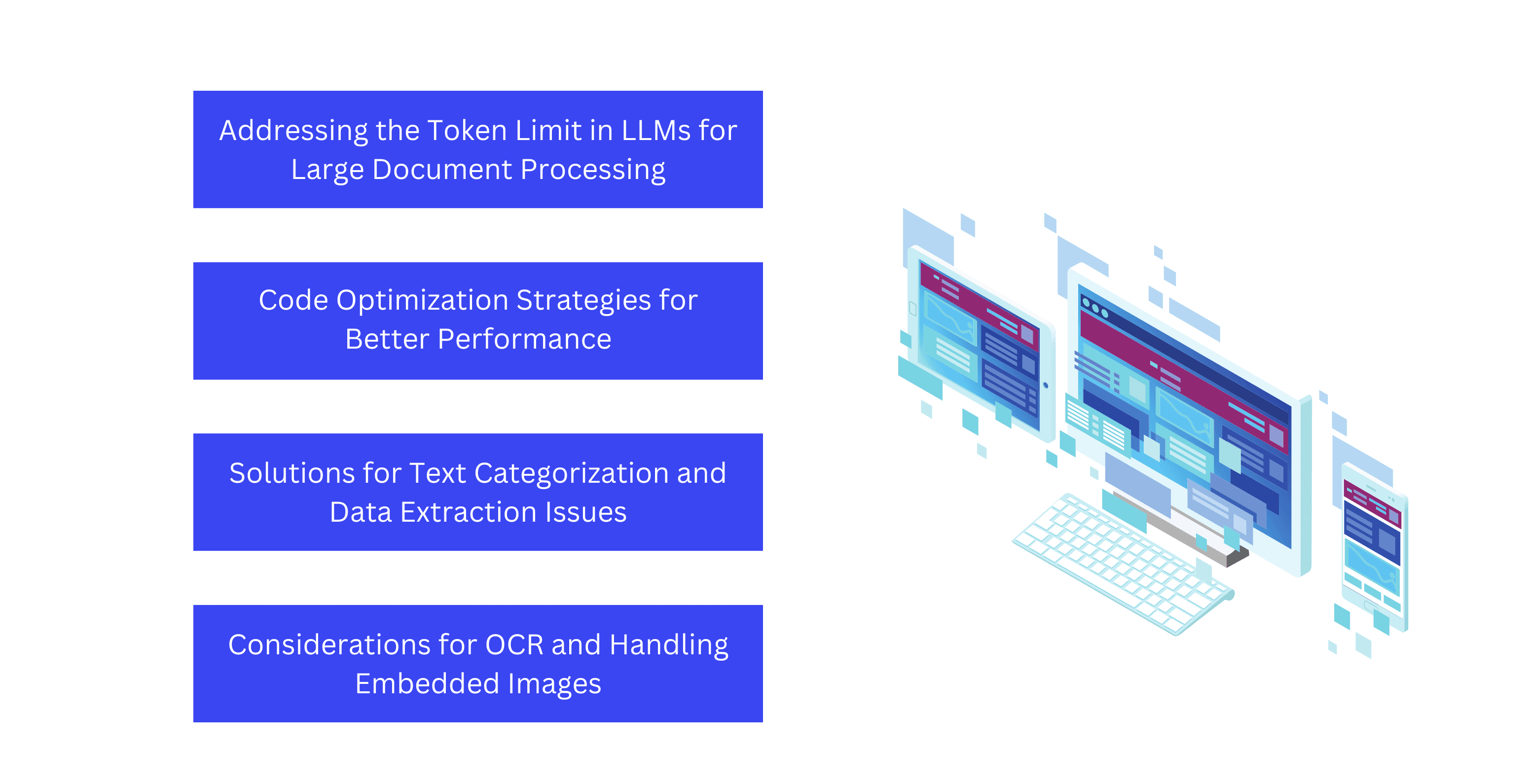
To successfully incorporate a RAG platform into your enterprise systems, you need to follow these strategies:-
Assessing Enterprise Systems for RAG Platform Integration Viability
Assess the existing enterprise systems to judge if they can support the incorporation with RAG platforms. This includes checking the compatibility of the system and receptivity for new technologies.
Integration Planning and Execution
Create a thorough integration plan that includes timelines, resources and milestones. Execute the plan with focus on least disruption to current operations.
Data Management and Compatibility
Ensure that data can be smoothly exchanged between RAG platforms and enterprise systems. Address any congenial problems and set up rules for constant management of data to maintain credibility and attainability.
Use Cases of RAG in Enterprise Systems
RAG can be highly efficient in improving enterprise systems. Here’s how the use cases can be implemented:
Enhancing Knowledge Base and Documentation with RAG for Better Accessibility
As inquiries are being treated, RAG rigorously recovers information from a wide corporate knowledge base. This technique allows the generation of more precise answers, creating internal wikis and documentation more communal and feasible.
Enterprises can ensure that employees find the most appropriate details swiftly, enhancing productivity and decreasing the time spent searching for details, by incorporating RAG.
Automated Code Generation for Software Development within Enterprises
By recovering code snippets and patterns of programming based on the context of the development task, RAG can be used to automate certain aspects of coding.
For instance, when a developer asks how to use a particular feature, RAG can pull instances from internal databases, Stack Overflow, or other documented sources, and create a custom piece that fits the parameters of the projects. This decreases monotonous work and promotes the cycle of development.
Improving Customer Support and Troubleshooting through Efficient Information Retrieval
Using RAG systems can significantly improve customer support. When a support query is received, RAG can promptly recover appropriate troubleshooting guides, part tickets, and solutions from a large repository, helping support agents to give quicker and more precise responses.
The system can also be incorporated into chatbots and virtual assistants that will help to get 24/7 assistance and support to the customers.
Data Management and Vector Data in RAG Integration
Enforcing RAG in enterprise applications indulges careful consideration of the management of data and the efficient use of vector data. Let’s take a look in detail:-
The Importance of Data Quality and Structure for RAG Effectiveness
Data Quality: The quality of data is important for RAG models as it directly affects the model’s capability to recover appropriate information and generate precise text. High-quality data should be precise, up-to-date and bias free.
Data Structure: Assembling data in a way that improves recoverability is important. This means that arranging information in clear, logical formats that a RAG system can easily examine and recover. For instance, forming data in well aligned layouts with compatible fields can significantly enhance the efficacy of data retrieval.
Utilizing Vector Data for Improving Retrieval Functions in Enterprise Applications
Vector Embeddings: RAG models often utilize vector embeddings of the input query and the documents in a knowledge base to find the most appropriate content to accelerate generation.
Improving Retrieval: To improve the functions of retrieval, vector data must be tuned well and upgraded. Approaches like dimensionality reduction can speed up recovery times and enhance the relevance of the retrieved documents.
Strategies for Data Validation and Training to Support RAG Functions
Data Validation: To maintain the integrity and appropriateness of data, regular checks are required.This includes procedures like anomaly detection to spot deviations, coherence checks to ensure data coordination.
Training and Updating: You could constantly train the RAG system on new and updated data to keep the model up-to-date and efficient. This indulges not only initiating the fundamental language models but also updating the document embeddings as new data is integrated.
Challenges and Solutions in Integration
Incorporating RAG platforms into an existing business environment can be a complicated procedure with several challenges that needs to be addressed carefully. So, lets see the challenges and solutions in Integration:-
Common Challenges Faced During RAG Platform Integration
Compatibility Issues: Often, existing IT systems and software may not be directly congenial with new RAG platforms, leading to incorporation challenges.
Data Quality and Integrity: Ensuring the data relocated to or handled by RAG platforms is precise and maintains its credibility is a major challenge.
Resistance to change: Governing resistance from employees usual to existing processes can block the incorporation procedure.
Technical and Organizational Solutions to Overcome Integration Barriers
Middleware solutions: Middleware can help bridge the gap between distinct technologies and provide seamless integration by working as a translator between applications.
Data Cleansing Tools: To ensure that the data being relocated is precise and useful, apply data cleansing tools before incorporation.
Change Management Strategies: Efficient change management strategies, including training of staff and investor management, can help diminish resistance to new systems.
Ensuring Data Security and Privacy Compliance in RAG-enabled Systems
Encryption and Data-Masking: To safeguard sensitive data, both at rest and in movement, utilize encryption and data masking approaches.
Regular Audits: To ensure continuous adherence to appropriate laws and policies, conduct constant security audits and consent reviews.
Access Controls: To limit access to sensitive data and systems, apply rigorous access controls and validation apparatus.
Conclusion
To conclude the guide, the RAG platform integration with existing enterprise systems depicts a crucial growth in improving organizational efficacy and decision-making abilities.
Throughout this guide, we have inspected several methodologies and best practices important for a smooth RAG platform integration.
Main strategies included Assessing enterprise systems for RAG platform integration viability, Planning and executing the integration process and Managing data compatibility and ensuring seamless data flow between RAG platforms and enterprise systems.
By comprehending and implementing RAG platforms in your enterprise systems, you deploy your business at the front line of technological innovation, developed to face the future with the best tools at your disposal.
Integrating RAG platforms with enterprise systems is a bit like trying to put a square peg in a round hole, but with the right tools, anything's possible. Let's dive in and learn about RAG Platform Integration!
Introduction to Integration of RAG Platforms with Enterprise Systems
When you are looking to gear up your enterprise software systems, RAG (Retrieval-Augmented Generation) platforms are gradually becoming important.
By incorporating advanced NLP technologies such as RAG into your existing framework, you not only improve the working but also sleek the procedures to meet the growing demands of the venture landscape.
Overview of RAG Platforms and Their Significance in Enhancing Enterprise Software Systems
RAG platforms (Retrieval-Augmented Generation) are a type of Artificial Intelligence System that improves machine learning models by incorporating recovery abilities directly into the productive process.
This approach leverages a huge database of information, recovering necessary data to accelerate the generation of responses In the conditions of enterprise software systems, RAG platforms offer various important improvements:
Customer Support Systems: RAG Platforms transform customer support systems by giving precise, context-aware answers to customer questions. By recovering pertinent information from comprehensive documentation or knowledge bases, these platforms ensure that support agents and mechanized systems deliver accurate and prompt support, enhancing customer satisfaction and curtailing resolution times.
Decision Support Systems: Integrating RAG platforms into decision support systems improves the coherent abilities of enterprise software. These platforms can recover and consolidate pertinent data points from numerous sources, offering decision-makers with thorough and precise perceptions. This results in more acquainted and efficient planned decisions, boosting the venture growth and diligence.
Document Management and Compliance: RAG platforms simplify document management and compliance processes by effectively recovering relevant regulatory details and documentation. Enterprises can ensure conformity to regulatory needs and industry criterions by using these platforms to access the latest compliance standards and documentation, alleviating the threats of non-compliance and interrelated forfeits.
Research and Development: Enterprise engaged in research and development (R&D) advantage from RAG platforms through improved access to the latest investigation discovery and technical literature. These platforms accelerate the recovery of pertinent studies, trademark, and technical documents, aiding inventiveness, and hastening the expansion of new merchandises and technologies.
Importance of Integrating Advanced NLP Technologies into Existing Enterprise Infrastructure
The advanced NLP technologies integration into enterprise infrastructure is substantially significant for various reasons:
Improved Data Processing and Analysis
Efficient Unstructured Data Handling
Advanced NLP technologies effectively process unstructured data, like emails, reports, social media posts, and customer responses.
By extracting significant information from these disparate data sources, enterprises can gain a pragmatic comprehension of market trends, customer emotions, and internal procedures. This expertise alters the raw data into extravagant perceptions, driving informed decision-making.
Improved Text Mining and Information Recovery
Incorporating NLP technologies improves the text mining and informational recovery procedures within an enterprise.
These technologies can recognize pertinent information from comprehensive document storage, databases, and external sources in real-time. This aptitude ensures that employees and the decision-makers have access to precise and latest information, providing prompt and well-informed decisions.
Optimized Customer Engagement
Advanced Chatbots and Virtual Assistants
Enterprises can position NL–powered chatbots and virtual helpers to offer 24/7 customer support. These intelligent systems comprehend and reciprocate to the queries of the customers in natural language, delivering customized and contextually pertinent support. By managing routine investigation and tasks, NLP technologies free up human support to focus on intricate problems, improving overall customer effectiveness.
Sentiment Analysis and Customer Perceptions
Progressed NLP Technologies permit sentiment analysis, permitting enterprises to gauge customer emotions and beliefs from text data.
By inspecting customer reviews, social media companionship, and support tickets, enterprises can recognize areas for enhancement, observe brand reputation, and tailor their marketing plans to better meet customer requirements.
The Advantages Of RAG Compared to LLMs
Envision this: LLMs are like those know-it-all friends who depend on what they learn in school. On the contrary, RAG Models are like those friends who can also strap out their mobiles and rapidly locate the latest information.
This imperium of RAG models comes from their secret weapon- retrieval. They can access and utilize tons of real-details, from news articles to research papers, to give you the most precise and latest answers.
When it comes to selecting between Retrieval-Augmented Generation (RAG) and standalone Large Language Models (LLMs), it is clear that RAG has some substantial benefits. Let’s know why RAG stands out:
Enhanced Knowledge Access and Accuracy
Real-Time Information Retrieval: RAG Models use real-time retrieval apparatus to access huge external databases or knowledge bases. This expertise ensures that the produced responses are not only relatedly apt but also up-to-date. Unlike LLMs, which are restricted by the stagnant nature of their training data, RAG models can integrate the latest information, substantially improving the accuracy and pertinence of the responses.
Vanquishing Training Data Limitations: LLMs are trained on large but limited sets of data, resulting in intrinsic knowledge cutoff. In comparison, RAG models vigorously recover relevant information from external sources, efficiently skirting the restrictions levied by the stagnant training data. This is specifically advantageous in domains where information swiftly progresses, like technology, medicine and finance.
Enhanced Response Quality and Relevance
Contingent Relevance: By incorporating retrieval mechanisms, RAG models can tailor answers based on precise context of a question. The recovery element pinpoint and provides contingently pertinent documents, which the productive element then uses to craft more accurate and situational cognizant responses. This results in higher-quality yields, contrast to LLMs alone, which might produce credible but not significantly precise answers based on incomplete context.
Fact-Checking and Reduced Hallucination: One of the substantial challenges with LLMs is the tenor to hallucinate-produce genuinely incorrect information positively. RAG models alleviate this by wrecking the produced content in recovered, demonstrable documents. This retrieval-augment approach acts as an annotating mechanism, significantly curtailing the examples of hallucinations and enhancing the dependability of the produced text.
Efficiency and Performance
Computational Efficiency: RAG models can be more arithmetically effective compared to training LLMs on ever-evolving sets of data. The recovery mechanism permits for a more tractable model size since the requirement to entrench all attainable knowledge within the model dimensions is reduced. Instead, pertinent knowledge is retrieved on demand, resulting in better use of computational resources.
Scalability: The flexible nature of RAG models improves scalability. The recovery element can independently scale with the expansion of the knowledge base, without requiring a conforming increase in the produced model. This separation of solicitudes allow for more adaptable and effective scaling plans.
Understanding RAG Platforms
So, what absolutely are RAG platforms? In essence, these systems include 2 main components: a retriever and a generator.
The retriever sieves through a huge database to find pertinent information, while the generator takes this information and crafts replies that are coherent and situationally apt.
This bi-component setup uses natural language processing to improve information recovery and generation, making it a solid tool for any enterprise.
Definition and Components of Retrieval-Augmented Generation Platforms
Retrieval-Augment Generation (RAG) platforms are a hybrid AI approach that merges machine learning models with recovery-based mechanisms to improve language generation tasks. The main components of RAG platforms are:
Retriever: The component’s job is to find appropriate documents from a large collection of data that can help in answering a query. It utilizes information recovery approaches to choose documents or data snippets that are appropriate to the input prompt. The retriever is commonly built on top of search computations and systems of indexing that can effectively manage large volumes of data.
Generator: Once the appropriate information is recovered, the generator utilizes this context to generate a coherent and contextually relevant response. This component is generally a large-scale language model trained on wide datasets to create human-like text based on the input and retrieved details.
Database or Knowledge Base: The retriever standardized or unstandardized data storage. It can indulge a wide range of information, from textual documents to standardized databases, relying on the application.
Integrator or Fusion Component: This part of the system incorporates the output from the retriever with the process of generation, often altering the input to the generator to include the recovered context or influencing the process of generation based strongly on recovered data.
Importance of RAG
Large Language Models (LLMs) are prominent Artificial Intelligence (AI) technology, generating smart chatbots and other NLP apps.
The intention is to generate bots that can respond to user queries in numerous situations by referencing reliable comprehension provenance.
Sadly, the creation of LLM technology acquaints volatility in LLM responses. In addition, LLM training data is stagnant and acquaints a cut-off date on the comprehension it has.
Let’s take a look at the detailed breakdown of why RAG is significant:
Improved Accuracy and Relevance
Incorporation of External Knowledge
RAG Systems uses external data storage, knowledge bases, or documents to recover pertinent data, which is then integrated into the process of generation. This ensures that the produced content is not only coherent but factually precise and latest.
Contextual Awareness
By recovering contextually pertinent information from huge external sources, RAG models can produce responses that are better customized to the precise query or response, resulting in more accurate and contextually pertinent production.
Scalability and Flexibility
Scalability Knowledge Incorporation
RAG models can incorporate enormous and diverse external data sources, making them highly flexible. This adaptability permits them to adjust to distinct domains and applications without requiring comprehensive briefing on domain-specific data.
Adaptive Learning
The recovery element can constantly update with new data, permitting the model to adjust to new data and progressing comprehension without the requirement for constant re-training of the produced element.
Technical Implementation
Dual-Encoder Architecture
RAG usually applies a dual-encoder architecture where one encoder processes the questions and the other encoder processes the recovered documents. This setup permits for effective matching of the questions with pertinent external information, which is then utilized by the produced model to produce the final output.
End-to-End Training
RAG models can be trained end-to-end, upgrading both recovered and generation elements concurrently. This incorporated training approach ensures that the recovered process is impeccably tuned to aid the generation process, resulting in better overall performance.
Latest RAG Pipelines
The latest expansion in Real-Augmented Generation (RAG) pipelines exhibits a spectrum of advanced attributes and incorporations designed to improve the effectiveness and performance of generative AI applications.
R2R Framework: The R2R (RAG to Riches) Framework is a pragmatic tool for rapid expansion, positioning and upgradation of RAG systems. It provides flexible elements like Providers, Pipes and Pipelines, which permits developers to construct and fine-tune high standard RAG applications efficiently. The framework supports assorted use cases from document handling to real-time query responses, improving both adaptability and robustness.
NVIDIA AI Enterprise: NVIDIA’s solution to enterprise-grade RAG applications offers a sturdy infrastructure from moving RAG models from pilot to outputs. The procedure indulges evaluating LLMs, exporting models as api-driven architecture, evolving sample applications, and positioning them in Kubernetes clusters utilizing the NVIDIA RAG LLM Operator. The pragmatic approach ensures that RAG systems are upgraded for performance and smoothly incorporated into existing enterprise landscapes.
Super-RAG: Superagent-ai's Super-RAG is designed for speedy RAG applications, providing a simple APi for encapsulation, recovery and code analysis. It aids multiple document formats vector information, making it adaptable for numerous use cases. The built-in REST API and cloud API alternatives simplify easy incorporation and positioning of RAG pipelines in both expansion and yielding environments.
These expansions in RAG pipelines emphasize the progressing capabilities and applications of productive AI, making it easier for developers to construct, position and handle sophisticated AI systems across numerous domains.
The Role of Generator and Retriever in RAG Platforms
The retriever and generator plays different but supportive roles in RAG platforms:-
Role of the Retriever: The main function of the retriever is to give appropriate context to the generator. By attaining a large repository, it finds information that can augment the abilities of the generator, allowing more precise, thorough and context-aware responses. By guaranteeing that the generation is grounded in real-world information rather than completely constructing replies, the retriever ensures reliability.
Role of the Generator: Integrating the recovered information into a coherent output constitutes the generator’s role. It takes the context given by the retriever and fabricates replies that are not only appropriate but also engaging to the inquiry. The generator must adjust its responses based on the quality and significance of the information recovered.
How RAG Platforms Employ Natural Language Processing for Information Retrieval and Generation?
RAG platforms apply natural language processing in various key ways:
Information Retrieval: To form efficient queries for the retriever, NLP techniques comprehend and process the users' input. This indulges natural language comprehension, query construction, and sometimes explicit search approaches to ensure that the recovery is appropriate.
Contextual Relevance: NLP is crucial in explaining the recovered details to determine its relevance and usefulness in the context of the current task. This often indulges comprehending variations, entity identification and contextual lodging.
Text Generation: NLP is utilized in the generator to create natural language responses. This indulges leveraging models trained on a wide corpora of text that comprehends grammar, syntax and style, permitting for the generation of responses that are not only situationally relevant but also verbally coherent.
Strategies for RAG Platform Integration
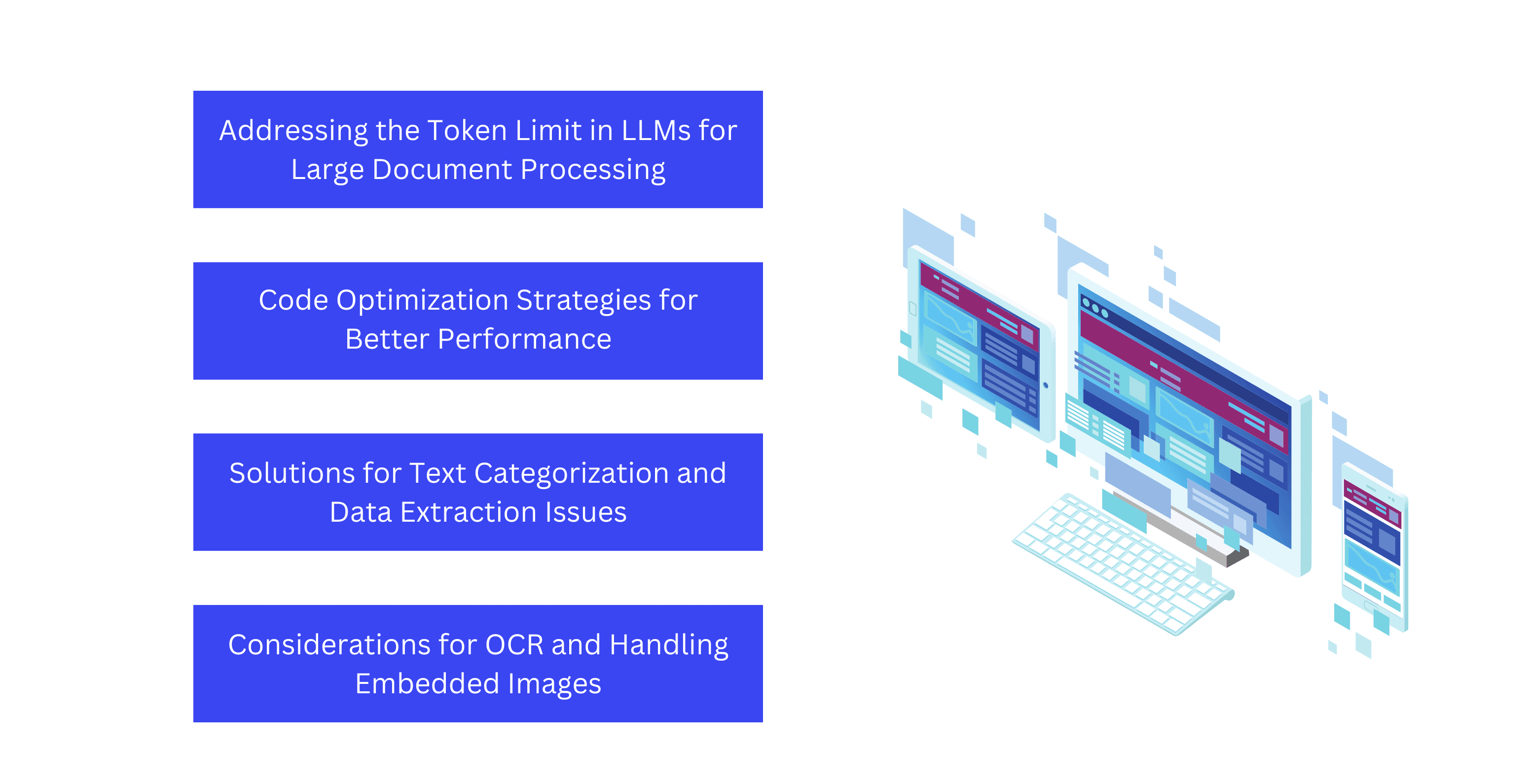
To successfully incorporate a RAG platform into your enterprise systems, you need to follow these strategies:-
Assessing Enterprise Systems for RAG Platform Integration Viability
Assess the existing enterprise systems to judge if they can support the incorporation with RAG platforms. This includes checking the compatibility of the system and receptivity for new technologies.
Integration Planning and Execution
Create a thorough integration plan that includes timelines, resources and milestones. Execute the plan with focus on least disruption to current operations.
Data Management and Compatibility
Ensure that data can be smoothly exchanged between RAG platforms and enterprise systems. Address any congenial problems and set up rules for constant management of data to maintain credibility and attainability.
Use Cases of RAG in Enterprise Systems
RAG can be highly efficient in improving enterprise systems. Here’s how the use cases can be implemented:
Enhancing Knowledge Base and Documentation with RAG for Better Accessibility
As inquiries are being treated, RAG rigorously recovers information from a wide corporate knowledge base. This technique allows the generation of more precise answers, creating internal wikis and documentation more communal and feasible.
Enterprises can ensure that employees find the most appropriate details swiftly, enhancing productivity and decreasing the time spent searching for details, by incorporating RAG.
Automated Code Generation for Software Development within Enterprises
By recovering code snippets and patterns of programming based on the context of the development task, RAG can be used to automate certain aspects of coding.
For instance, when a developer asks how to use a particular feature, RAG can pull instances from internal databases, Stack Overflow, or other documented sources, and create a custom piece that fits the parameters of the projects. This decreases monotonous work and promotes the cycle of development.
Improving Customer Support and Troubleshooting through Efficient Information Retrieval
Using RAG systems can significantly improve customer support. When a support query is received, RAG can promptly recover appropriate troubleshooting guides, part tickets, and solutions from a large repository, helping support agents to give quicker and more precise responses.
The system can also be incorporated into chatbots and virtual assistants that will help to get 24/7 assistance and support to the customers.
Data Management and Vector Data in RAG Integration
Enforcing RAG in enterprise applications indulges careful consideration of the management of data and the efficient use of vector data. Let’s take a look in detail:-
The Importance of Data Quality and Structure for RAG Effectiveness
Data Quality: The quality of data is important for RAG models as it directly affects the model’s capability to recover appropriate information and generate precise text. High-quality data should be precise, up-to-date and bias free.
Data Structure: Assembling data in a way that improves recoverability is important. This means that arranging information in clear, logical formats that a RAG system can easily examine and recover. For instance, forming data in well aligned layouts with compatible fields can significantly enhance the efficacy of data retrieval.
Utilizing Vector Data for Improving Retrieval Functions in Enterprise Applications
Vector Embeddings: RAG models often utilize vector embeddings of the input query and the documents in a knowledge base to find the most appropriate content to accelerate generation.
Improving Retrieval: To improve the functions of retrieval, vector data must be tuned well and upgraded. Approaches like dimensionality reduction can speed up recovery times and enhance the relevance of the retrieved documents.
Strategies for Data Validation and Training to Support RAG Functions
Data Validation: To maintain the integrity and appropriateness of data, regular checks are required.This includes procedures like anomaly detection to spot deviations, coherence checks to ensure data coordination.
Training and Updating: You could constantly train the RAG system on new and updated data to keep the model up-to-date and efficient. This indulges not only initiating the fundamental language models but also updating the document embeddings as new data is integrated.
Challenges and Solutions in Integration
Incorporating RAG platforms into an existing business environment can be a complicated procedure with several challenges that needs to be addressed carefully. So, lets see the challenges and solutions in Integration:-
Common Challenges Faced During RAG Platform Integration
Compatibility Issues: Often, existing IT systems and software may not be directly congenial with new RAG platforms, leading to incorporation challenges.
Data Quality and Integrity: Ensuring the data relocated to or handled by RAG platforms is precise and maintains its credibility is a major challenge.
Resistance to change: Governing resistance from employees usual to existing processes can block the incorporation procedure.
Technical and Organizational Solutions to Overcome Integration Barriers
Middleware solutions: Middleware can help bridge the gap between distinct technologies and provide seamless integration by working as a translator between applications.
Data Cleansing Tools: To ensure that the data being relocated is precise and useful, apply data cleansing tools before incorporation.
Change Management Strategies: Efficient change management strategies, including training of staff and investor management, can help diminish resistance to new systems.
Ensuring Data Security and Privacy Compliance in RAG-enabled Systems
Encryption and Data-Masking: To safeguard sensitive data, both at rest and in movement, utilize encryption and data masking approaches.
Regular Audits: To ensure continuous adherence to appropriate laws and policies, conduct constant security audits and consent reviews.
Access Controls: To limit access to sensitive data and systems, apply rigorous access controls and validation apparatus.
Conclusion
To conclude the guide, the RAG platform integration with existing enterprise systems depicts a crucial growth in improving organizational efficacy and decision-making abilities.
Throughout this guide, we have inspected several methodologies and best practices important for a smooth RAG platform integration.
Main strategies included Assessing enterprise systems for RAG platform integration viability, Planning and executing the integration process and Managing data compatibility and ensuring seamless data flow between RAG platforms and enterprise systems.
By comprehending and implementing RAG platforms in your enterprise systems, you deploy your business at the front line of technological innovation, developed to face the future with the best tools at your disposal.
Subscribe to our newsletter to never miss an update
Other articles


Agents Talk, Models Think: A2A + MCP for Enterprise Agentic AI

Riya Parikh, Nitai Agarwal
Apr 27, 2025
Read the article
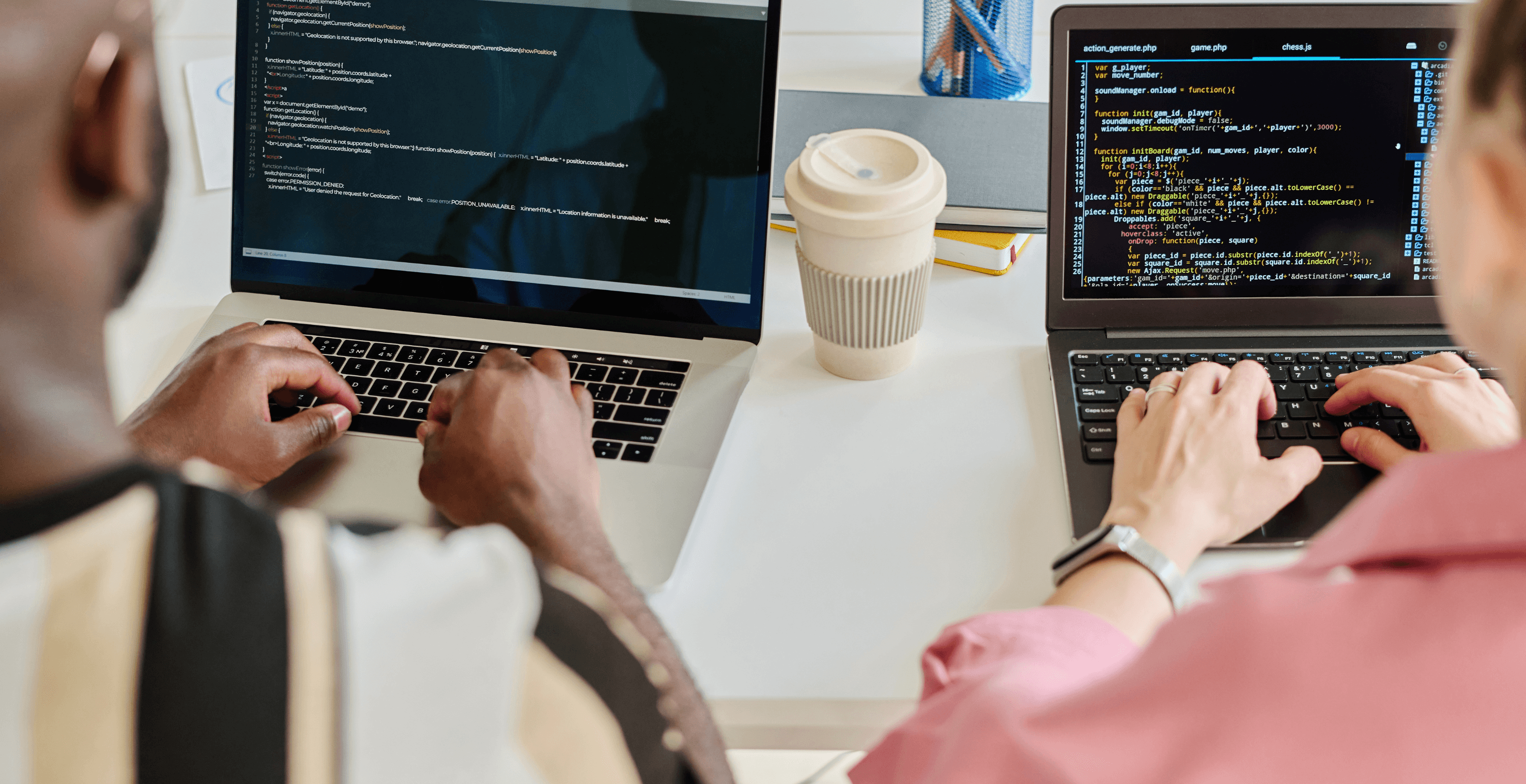
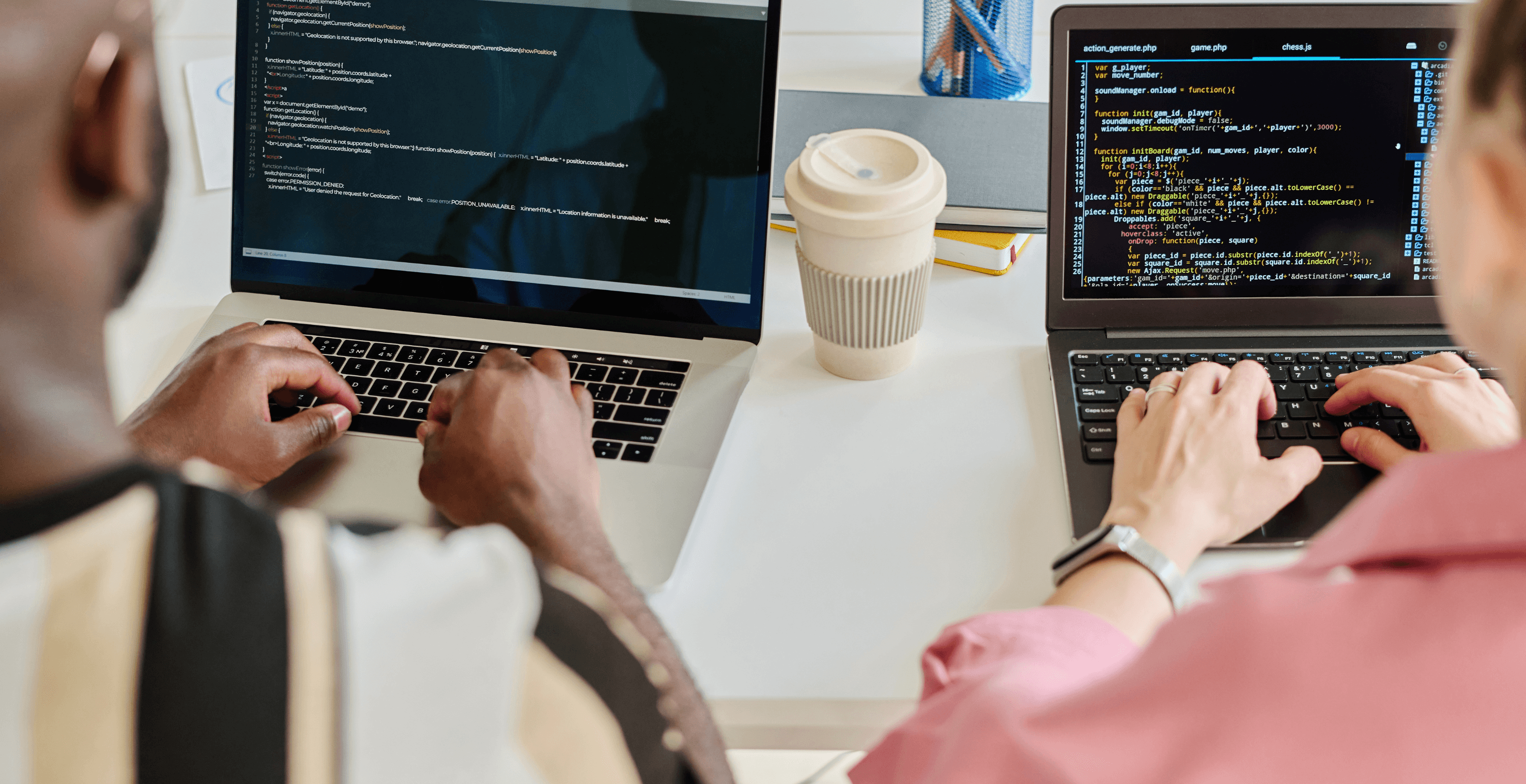
Understanding NeMo Guardrails: A Toolkit for LLM Security

Rehan Asif
Dec 24, 2024
Read the article


Understanding Differences in Large vs Small Language Models (LLM vs SLM)

Rehan Asif
Dec 21, 2024
Read the article