Introduction to What is LLM Agents and How They Work?
Introduction to What is LLM Agents and How They Work?
Introduction to What is LLM Agents and How They Work?
Rehan Asif
Jul 24, 2024
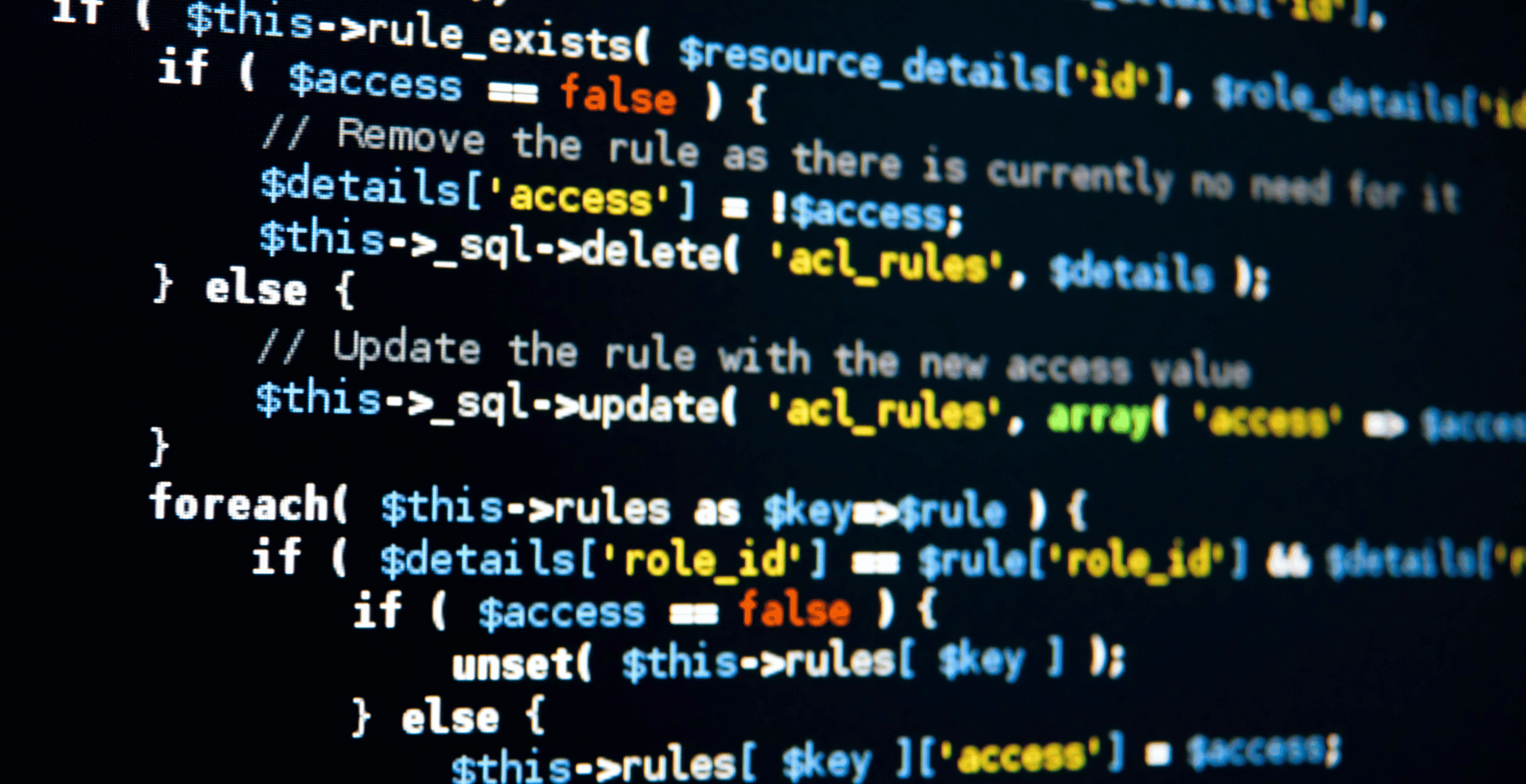
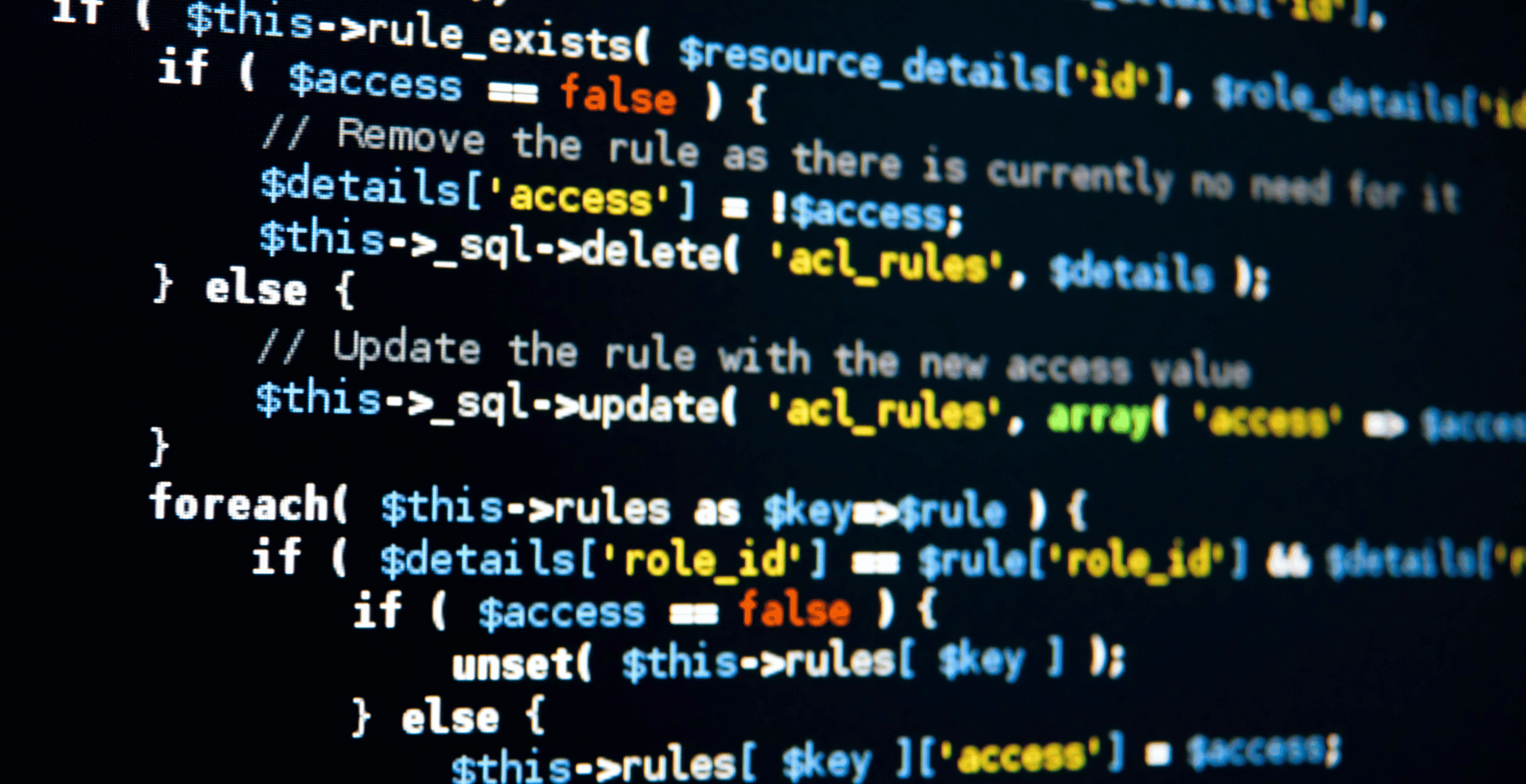
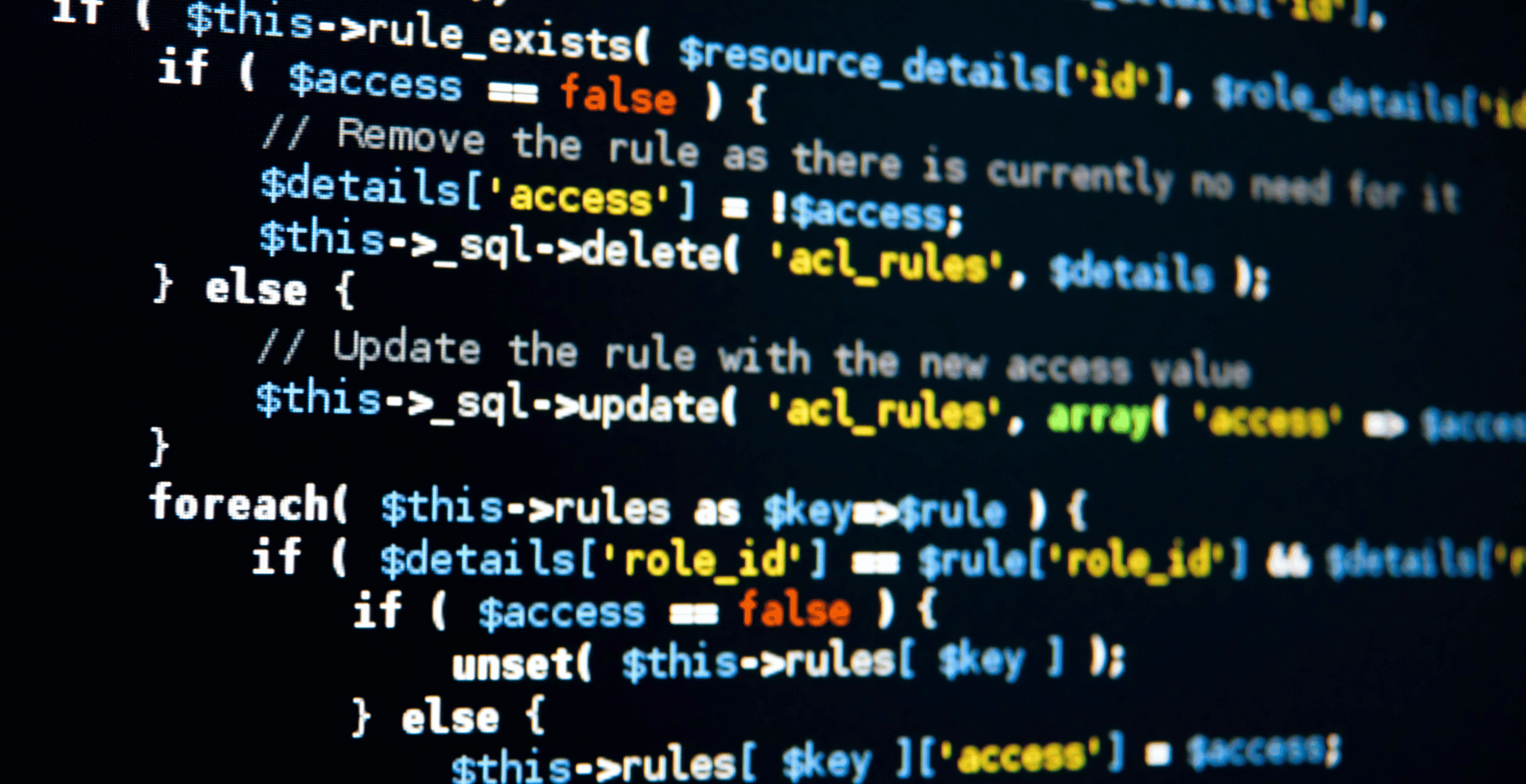
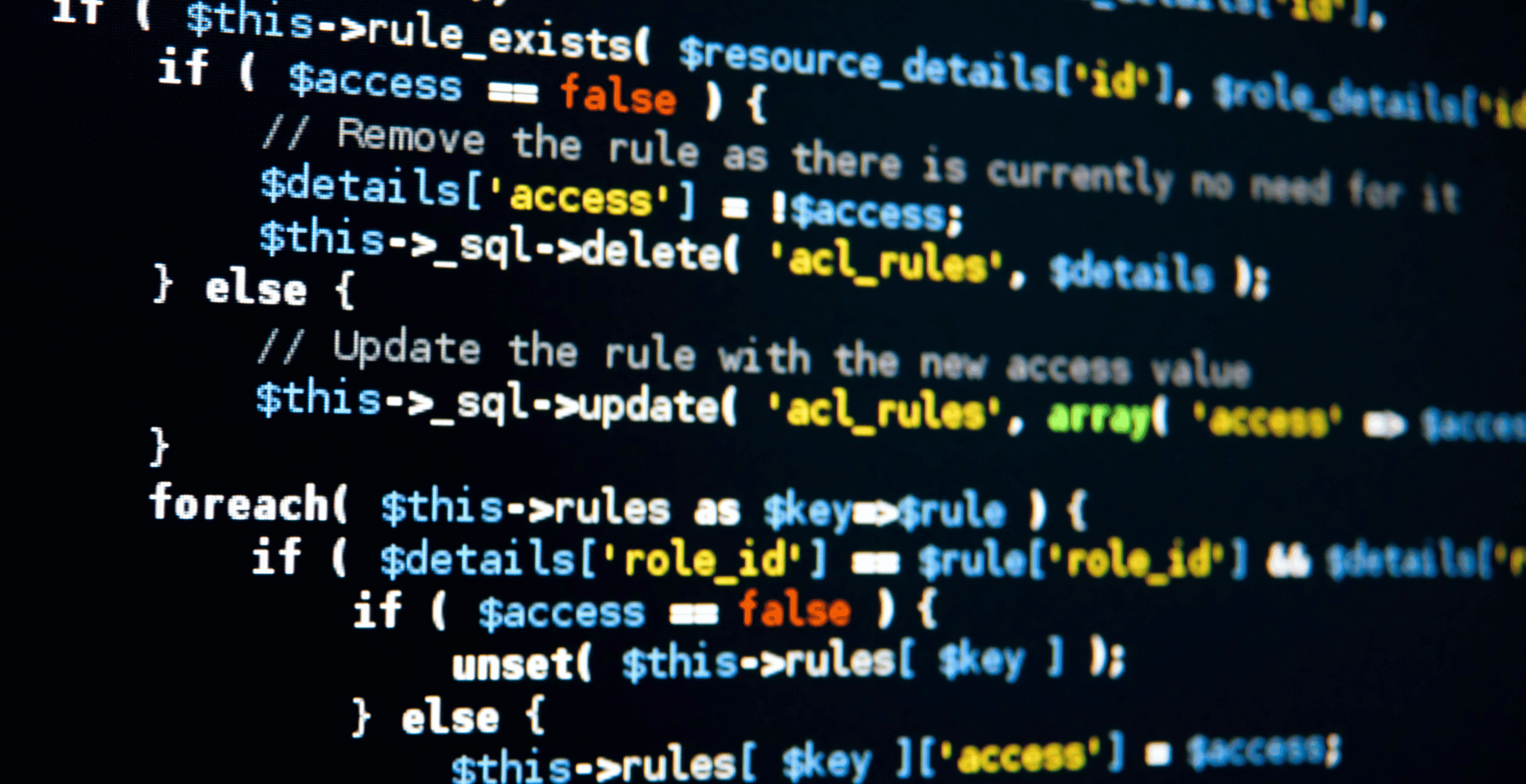
In the swiftly developing field of Artificial Intelligence, LLM agents are the latest expansions, able to solve intricate, multi-step real-globe tasks. You might have heard AI systems producing text or answering questions, but LLM agents take it a step further. They can execute a series of tasks that need sequential reasoning, making them indispensable in numerous applications.
Components of LLM Agents
Large Language Models (LLMs) are built from numerous key components that enable them to refine, comprehend, and produce human language efficiently. Here are the main components of LLM agents:
Agent Core
The agent core is very special to the heart of every LLM agent, acting as the fundamental coordination component. Researchers train this core on enormous datasets, enabling it to refine and produce human-like text. Its predominant function is to sustain the communications and functioning of the whole system, ensuring smooth communication between distinct components.
Memory
LLM agents have a sophisticated Memory system, which is important for controlling tasks and sustaining conversational records. Engineers divide this system into short-term and long-term memory components.
Short-term Memory: This memory manages prompt, context-specific data. It acts as a booklet where it swiftly writes down significant information during an interaction. This memory component traces the information of the ongoing interaction, helping the model acknowledge sensibly to the instant context. At the same time, Short-term memory is provisional, dispersing once the task is finished.
Long-Term Memory: On the contrary, this memory retains information over extended periods, providing consistency and pertinence in enduring interactions. It stores the information from past communications over weeks.
Planning
Efficient planning is important for the functionality of LLM agents. This module concentrates on the agent forms plans by fermenting tasks and queries followed by reflective analysis. By breaking down intricate questions into tractable parts, the agent can produce pragmatic strategies to acknowledge user requirements precisely. Let’s take a look at the two main phases of planning:
Plan Formulation
Plan formulation is the process through which an LLM agent develops a plan to accomplish a precise aim. This involves numerous key aspects:
Understanding the Goal: The agent first needs to clearly comprehend the intention. This could be anything from producing a comprehensive report to responding to an intricate query.
Collecting information: The agents gather pertinent data from their knowledge base or external sources. This may indulge data recovery, contextual comprehension, and determining key variables.
Generating Steps: The agent articulates a sequence of actions needed to accomplish the goal. The agent breaks down the task into tractable steps, considering reliability and reasonable order.
Evaluating Feasibility: The agent evaluates the feasibility of the plan, ensuring it applies to each step and that the overall strategy is likely to flourish.
Adapting for Constraints: The agent alters the strategy to account for any limitations, like time restrictions, resource attainability, or precise user needs.
For instance, if the aim is to draft a guide on the advantages of renewable energy, the LLM agent would:
Comprehend the scope and objective of the guide.
Collect information on distinct types of renewable energy sources, their advantages, and recent progressions.
Produce an outline with sections such as introduction, types of renewable energy, advantages, and conclusion.
Assess the data to ensure precision and pertinence.
Adapt the content to fit the needed word count and tone.
Plan Reflection
Plan reflection is the process by which an LLM agent assesses the result of its actions and grasps from its experiences. This indulges:
Retrospecting Actions: The agent retrospects the steps it took and the verdicts made during plan origination and enforcement.
Evaluating Results: The agent assesses the success of the strategy by contrasting the actual results with the desired goals.
Determining Errors: The agent determines any errors or areas where the plan did not perform as anticipated.
Learning and Adjusting: The agent uses the perceptions gained from reflection to enhance future performance. This could include updating its knowledge base, adapting algorithms, or altering plans.
Feedback Incorporation: The agent integrates responses from users or external analysts to process its approach and improve its capabilities.
Continuing with the renewable energy article instance, after finishing the draft, the agent would:
Retrospect the content to ensure all key points were coated.
Contrast the draft against the foremost aim to ensure it meets the needs.
Determine the gaps or inaccuracies in the information provided.
Grasp from any mistakes, like incorrect information or unclear elucidations to enhance future guides.
Incorporate feedback from editors or readers to process the writing style and content quality.
Tools
LLM agents use numerous tools to enforce their tasks. These tools include APIs and services specially customized for distinct duties, like data retrieval, refining, and content generation. By incorporating these tools, the agent improves its capabilities and delivers more accurate and effective outcomes.
Knowledge
The knowledge component is important for understanding and resolving tasks. This includes not only the enormous amount of data the agent has been instructed on but also the ability to recover auxiliary information as required. Refining LLMs and using tool-aided data retrieval are key aspects of this component, ensuring the agent can provide precise and pertinent responses.
Prompts
Eventually, Prompts are the guidelines that guide the LLM agent’s aims, behaviors, and strategies. These prompts are crucial for controlling the agent’s responses and actions, ensuring they follow the desired intentions and contexts explicit by the user.
Comprehending these components helps you learn the complex functioning of LLM agents and their prospective applications across numerous domains.
Alright, now that we’ve got a grasp of the pieces that make up an LLM agent, let’s dive into how they actually work.
Want to gain deeper perceptions into evaluating the performance of Large Language Models? Check out our thorough article on Evaluating Large Language Models: Methods and Metrics.
How LLM Agents Work
LLM agents work by using prompts to guide their actions and answers, using memory for coherent communication, deliberately planning through task fermentation and reflection, employing tools and APIs for precise tasks, and constantly enhancing based on feedback and flexible learning.
Using Prompts for Actions and Responses
You communicate with LLM agents predominantly through prompts. Think of prompts as guidelines or queries you give to Artificial Intelligence. These prompts guide the AI on what actions to take or answers to produce. For instance, if you ask an LLM agent to draft an email, your prompt might include information about the email’s objective, the recipient, and key points to cover. The AI then refines this input and crafts a response customized to your requirements. This prompt-driven interaction is what makes LLM agents incredibly adaptable and able to handle a wide range of tasks.
Memory Utilization for Effective Decision-Making
LLM agents use memory to ensure coherent interactions and efficient decision-making. When you are involved in a conversation with an LLM agent, it reminds you of previous interactions and uses that data to offer pertinent and congruous responses. This memory ability permits the agent to sustain context over multiple exchanges, making the communication more natural and useful. For example, if you’re discussing a project over numerous sessions, the AI can remember information from past conversations, helping you pick up right where you left off.
Task Decomposition and Reflective Analysis in Strategic Planning
Strategic planning is a key strength of LLM agents. They break down intricate tasks into inferior, tractable components, a process called task decomposition. This approach enables the AI to tackle complex issues in an orderly. Moreover, LLM agents use reflective analysis to assess their actions and results. By reflecting on what functioned well and what didn’t, they can adapt their plans to enhance future performance. This amalgamation of task decomposition and reflection helps LLM agents effectively regulate and finish multifaceted tasks.
Tools and APIs for Task Execution
To implement precise tasks, LLM agents often employ several tools and APIs (Application Programming Interfaces). These external resources improve the AI's capabilities by offering specialized functions that fundamental models may not innately possess. For instance, an LLM agent might use a translation API to convert text from one language to another or a weather API to retrieve current weather information. By incorporating these tools, LLM agents can provide more accurate and thorough solutions customized to your precise needs.
Feedback-Driven Continuous Improvement
LLM agents constantly enhance through feedback and flexible grasping. When you give feedback on their performance, whether positive or negative, the AI uses this data to process its future responses. This flexible learning process permits LLM agents to become more precise and efficient over time. For example, suppose you fix an error or recommend a better way to execute a task. In that case, the AI learns from this input, improving its capability to meet your anticipations in ensuing communications. This constant enhancement cycle ensures that LLM agents remain robust and receptive to your growing requirements.
So, you've seen the building blocks–now, let’s see them in action!
Explore how to smoothly incorporate low-code and visual programming into your tasks by reading our pragmatic guide on Building Low-Code LLM Apps with Visual Programming.
Applications and Use Cases of LLM Agents
LLM Agents are flexible tools that can transform several fields with their advanced capabilities. Here’s how you can use the power of LLM agents across distinct domains:
Customer Support, Content Creation, Language Translation
Envision having a support that never tries and can handle multiple questions concurrently. LLM agents can sleek your customer support by giving rapid, precise responses, ensuring customer contentment. When it comes to creating content, these agents can produce high-quality guides, blog posts, or social media updates, saving you precious time. Need to interact in multiple languages? LLM agents can translate text effectively, breaking down language obstacles and enabling smooth universal interactions.
Education and Tutoring, Programming, Research
In education, LLM agents can cater as personal tutors, providing elucidations, responding to queries, and offering personalized learning experiences. For programming, they can aid by producing code snippets, debugging, and elucidating intricate concepts. Researchers take advantage of these agents through their capability to sum up enormous amounts of data, determine pertinent studies, and even recommend new research directions.
Healthcare Assistance, Personal Assistants
Healthcare professionals can use LLM agents for preliminary diagnosis, patient records inspection, and medical transcription, improving effectiveness and precision. As personal assistants, these agents can sustain your schedules, set reminders, and manage daily tasks, permitting you to concentrate on more crucial activities.
Legal and Compliance Support, Accessibility Tools
Going through the legitimate scenario can be daunting. LLM agents can help by drafting legitimate documents, performing contract inspections, and ensuring compliance with regulations. For individuals with impairments, these agents can offer attainability tools, like real-time transcription and text-to-speech services, making digital content more attainable.
Interactive Entertainment, Marketing, Social Media Management
In entertainment, LLM agents can create communicative stories, counterfeit conversations, and improve gaming experiences. Marketers can use these agents to determine market trends, produce persuasive content, and sustain campaigns. For social media management, LLM agents can automate posts, engage with followers, and trace performance metrics, elevating your online presence.
Human Resources Management
The Human Resources department can aid incredibly from LLM agents. They can automate recruitment procedures, screen resumes, schedule interviews, and even supervise preliminary candidate evaluation. This not only saves time but also helps in locating the best talent more effectively.
By incorporating LLM agents into your productivity, you can improve workflow, enhance customer experiences, and stay ahead in a gradually advanced globe. Whether you’re in customer support, education, healthcare, or any other field, LLM agents provide inventive solutions customized to your requirements.
But hold on—it’s not all smooth sailing—there are also some challenges you need to be aware of.
Want to get details about self-hosting LLMs? Then, read our pragmatic article on Practical Strategies For Self-Hosting Large Language Models.
Challenges and Limitations of LLM Agents
When learning about the globe of Large Language Model (LLM) agents, you’ll locate they come with their fair share of challenges and restrictions. While these advanced AI systems have splendid abilities, it’s critical to comprehend where they might fall short.
Limited Context and Long-Term Planning Challenges
First off, LLM agents tussle with sustaining context over long chats. They might shine at comprehending and answering instant prompts, but keeping track of information across an elongated interaction is not their powerful suit. These restrictions make it hard for them to implement long-term planning efficiently, often causing them to miss the colossal picture.
Adapting to Specific Roles and Ensuring Consistent Outputs
You will also spot that their yields can be inconsistent. While they can produce coherent and contextually pertinent text, they might not always hit the mark, specifically when adjusting to precise roles or tones. Their inconsistency can be frustrating when you need dependable and accurate data.
Prompt Dependence and Accurate Knowledge Management
LLM agents heavily rely on the quality and clarity of prompts. If your prompt is ambiguous, the answer will likely reflect that. In addition, sustaining knowledge precisely is a congruous battle. They might have to attain enormous amounts of data, but ensuring they apply it properly in numerous contexts can be problematic.
Concerns Over Bias, Misinformation, and Privacy Leaks
Bias and misinformation are substantial concerns. These models grasp from enormous datasets, which may include partial or false data. As an outcome, they can accidentally commemorate stereotypes or spread inaccuracies. Privacy leaks are another crucial problem. Since LLM agents refine large amounts of information, ensuring they don’t accidentally disclose sensitive data is chief.
Environmental Impact Due to Computational Demands
Lastly, the environmental impact of these models can’t be overlooked. Training and running LLM agents need significant computational power, resulting in high energy consumption. This demand has a noticeable environmental footprint, raising queries about the imperishable nature of such technologies.
Comprehending these challenges and restrictions helps you better explore the use of LLM agents, set pragmatic anticipations, and acknowledge potential problems proactively.
Check out our pragmatic article on Comparing Different Large Language Models (LLMs) to discover the strengths and applications of each.
Conclusion
To conclude the article, LLM agents represent a substantial leap forward in AI, providing a colossal potential across various fields. By comprehending their components, how they operate, and their applications, you can better appreciate their abilities and the effect they can have on our lives. While challenges remain, perpetual innovations vow to make LLM agents even stronger and more adaptable in the future.
Improve your LLM performance with RagaAI! Sign up today and experience our inventive LLM solutions designed to deliver exceptional outcomes across any application. Optimize effortlessly and accomplish outstanding results. Don’t miss out– Join the AI revolution now!
In the swiftly developing field of Artificial Intelligence, LLM agents are the latest expansions, able to solve intricate, multi-step real-globe tasks. You might have heard AI systems producing text or answering questions, but LLM agents take it a step further. They can execute a series of tasks that need sequential reasoning, making them indispensable in numerous applications.
Components of LLM Agents
Large Language Models (LLMs) are built from numerous key components that enable them to refine, comprehend, and produce human language efficiently. Here are the main components of LLM agents:
Agent Core
The agent core is very special to the heart of every LLM agent, acting as the fundamental coordination component. Researchers train this core on enormous datasets, enabling it to refine and produce human-like text. Its predominant function is to sustain the communications and functioning of the whole system, ensuring smooth communication between distinct components.
Memory
LLM agents have a sophisticated Memory system, which is important for controlling tasks and sustaining conversational records. Engineers divide this system into short-term and long-term memory components.
Short-term Memory: This memory manages prompt, context-specific data. It acts as a booklet where it swiftly writes down significant information during an interaction. This memory component traces the information of the ongoing interaction, helping the model acknowledge sensibly to the instant context. At the same time, Short-term memory is provisional, dispersing once the task is finished.
Long-Term Memory: On the contrary, this memory retains information over extended periods, providing consistency and pertinence in enduring interactions. It stores the information from past communications over weeks.
Planning
Efficient planning is important for the functionality of LLM agents. This module concentrates on the agent forms plans by fermenting tasks and queries followed by reflective analysis. By breaking down intricate questions into tractable parts, the agent can produce pragmatic strategies to acknowledge user requirements precisely. Let’s take a look at the two main phases of planning:
Plan Formulation
Plan formulation is the process through which an LLM agent develops a plan to accomplish a precise aim. This involves numerous key aspects:
Understanding the Goal: The agent first needs to clearly comprehend the intention. This could be anything from producing a comprehensive report to responding to an intricate query.
Collecting information: The agents gather pertinent data from their knowledge base or external sources. This may indulge data recovery, contextual comprehension, and determining key variables.
Generating Steps: The agent articulates a sequence of actions needed to accomplish the goal. The agent breaks down the task into tractable steps, considering reliability and reasonable order.
Evaluating Feasibility: The agent evaluates the feasibility of the plan, ensuring it applies to each step and that the overall strategy is likely to flourish.
Adapting for Constraints: The agent alters the strategy to account for any limitations, like time restrictions, resource attainability, or precise user needs.
For instance, if the aim is to draft a guide on the advantages of renewable energy, the LLM agent would:
Comprehend the scope and objective of the guide.
Collect information on distinct types of renewable energy sources, their advantages, and recent progressions.
Produce an outline with sections such as introduction, types of renewable energy, advantages, and conclusion.
Assess the data to ensure precision and pertinence.
Adapt the content to fit the needed word count and tone.
Plan Reflection
Plan reflection is the process by which an LLM agent assesses the result of its actions and grasps from its experiences. This indulges:
Retrospecting Actions: The agent retrospects the steps it took and the verdicts made during plan origination and enforcement.
Evaluating Results: The agent assesses the success of the strategy by contrasting the actual results with the desired goals.
Determining Errors: The agent determines any errors or areas where the plan did not perform as anticipated.
Learning and Adjusting: The agent uses the perceptions gained from reflection to enhance future performance. This could include updating its knowledge base, adapting algorithms, or altering plans.
Feedback Incorporation: The agent integrates responses from users or external analysts to process its approach and improve its capabilities.
Continuing with the renewable energy article instance, after finishing the draft, the agent would:
Retrospect the content to ensure all key points were coated.
Contrast the draft against the foremost aim to ensure it meets the needs.
Determine the gaps or inaccuracies in the information provided.
Grasp from any mistakes, like incorrect information or unclear elucidations to enhance future guides.
Incorporate feedback from editors or readers to process the writing style and content quality.
Tools
LLM agents use numerous tools to enforce their tasks. These tools include APIs and services specially customized for distinct duties, like data retrieval, refining, and content generation. By incorporating these tools, the agent improves its capabilities and delivers more accurate and effective outcomes.
Knowledge
The knowledge component is important for understanding and resolving tasks. This includes not only the enormous amount of data the agent has been instructed on but also the ability to recover auxiliary information as required. Refining LLMs and using tool-aided data retrieval are key aspects of this component, ensuring the agent can provide precise and pertinent responses.
Prompts
Eventually, Prompts are the guidelines that guide the LLM agent’s aims, behaviors, and strategies. These prompts are crucial for controlling the agent’s responses and actions, ensuring they follow the desired intentions and contexts explicit by the user.
Comprehending these components helps you learn the complex functioning of LLM agents and their prospective applications across numerous domains.
Alright, now that we’ve got a grasp of the pieces that make up an LLM agent, let’s dive into how they actually work.
Want to gain deeper perceptions into evaluating the performance of Large Language Models? Check out our thorough article on Evaluating Large Language Models: Methods and Metrics.
How LLM Agents Work
LLM agents work by using prompts to guide their actions and answers, using memory for coherent communication, deliberately planning through task fermentation and reflection, employing tools and APIs for precise tasks, and constantly enhancing based on feedback and flexible learning.
Using Prompts for Actions and Responses
You communicate with LLM agents predominantly through prompts. Think of prompts as guidelines or queries you give to Artificial Intelligence. These prompts guide the AI on what actions to take or answers to produce. For instance, if you ask an LLM agent to draft an email, your prompt might include information about the email’s objective, the recipient, and key points to cover. The AI then refines this input and crafts a response customized to your requirements. This prompt-driven interaction is what makes LLM agents incredibly adaptable and able to handle a wide range of tasks.
Memory Utilization for Effective Decision-Making
LLM agents use memory to ensure coherent interactions and efficient decision-making. When you are involved in a conversation with an LLM agent, it reminds you of previous interactions and uses that data to offer pertinent and congruous responses. This memory ability permits the agent to sustain context over multiple exchanges, making the communication more natural and useful. For example, if you’re discussing a project over numerous sessions, the AI can remember information from past conversations, helping you pick up right where you left off.
Task Decomposition and Reflective Analysis in Strategic Planning
Strategic planning is a key strength of LLM agents. They break down intricate tasks into inferior, tractable components, a process called task decomposition. This approach enables the AI to tackle complex issues in an orderly. Moreover, LLM agents use reflective analysis to assess their actions and results. By reflecting on what functioned well and what didn’t, they can adapt their plans to enhance future performance. This amalgamation of task decomposition and reflection helps LLM agents effectively regulate and finish multifaceted tasks.
Tools and APIs for Task Execution
To implement precise tasks, LLM agents often employ several tools and APIs (Application Programming Interfaces). These external resources improve the AI's capabilities by offering specialized functions that fundamental models may not innately possess. For instance, an LLM agent might use a translation API to convert text from one language to another or a weather API to retrieve current weather information. By incorporating these tools, LLM agents can provide more accurate and thorough solutions customized to your precise needs.
Feedback-Driven Continuous Improvement
LLM agents constantly enhance through feedback and flexible grasping. When you give feedback on their performance, whether positive or negative, the AI uses this data to process its future responses. This flexible learning process permits LLM agents to become more precise and efficient over time. For example, suppose you fix an error or recommend a better way to execute a task. In that case, the AI learns from this input, improving its capability to meet your anticipations in ensuing communications. This constant enhancement cycle ensures that LLM agents remain robust and receptive to your growing requirements.
So, you've seen the building blocks–now, let’s see them in action!
Explore how to smoothly incorporate low-code and visual programming into your tasks by reading our pragmatic guide on Building Low-Code LLM Apps with Visual Programming.
Applications and Use Cases of LLM Agents
LLM Agents are flexible tools that can transform several fields with their advanced capabilities. Here’s how you can use the power of LLM agents across distinct domains:
Customer Support, Content Creation, Language Translation
Envision having a support that never tries and can handle multiple questions concurrently. LLM agents can sleek your customer support by giving rapid, precise responses, ensuring customer contentment. When it comes to creating content, these agents can produce high-quality guides, blog posts, or social media updates, saving you precious time. Need to interact in multiple languages? LLM agents can translate text effectively, breaking down language obstacles and enabling smooth universal interactions.
Education and Tutoring, Programming, Research
In education, LLM agents can cater as personal tutors, providing elucidations, responding to queries, and offering personalized learning experiences. For programming, they can aid by producing code snippets, debugging, and elucidating intricate concepts. Researchers take advantage of these agents through their capability to sum up enormous amounts of data, determine pertinent studies, and even recommend new research directions.
Healthcare Assistance, Personal Assistants
Healthcare professionals can use LLM agents for preliminary diagnosis, patient records inspection, and medical transcription, improving effectiveness and precision. As personal assistants, these agents can sustain your schedules, set reminders, and manage daily tasks, permitting you to concentrate on more crucial activities.
Legal and Compliance Support, Accessibility Tools
Going through the legitimate scenario can be daunting. LLM agents can help by drafting legitimate documents, performing contract inspections, and ensuring compliance with regulations. For individuals with impairments, these agents can offer attainability tools, like real-time transcription and text-to-speech services, making digital content more attainable.
Interactive Entertainment, Marketing, Social Media Management
In entertainment, LLM agents can create communicative stories, counterfeit conversations, and improve gaming experiences. Marketers can use these agents to determine market trends, produce persuasive content, and sustain campaigns. For social media management, LLM agents can automate posts, engage with followers, and trace performance metrics, elevating your online presence.
Human Resources Management
The Human Resources department can aid incredibly from LLM agents. They can automate recruitment procedures, screen resumes, schedule interviews, and even supervise preliminary candidate evaluation. This not only saves time but also helps in locating the best talent more effectively.
By incorporating LLM agents into your productivity, you can improve workflow, enhance customer experiences, and stay ahead in a gradually advanced globe. Whether you’re in customer support, education, healthcare, or any other field, LLM agents provide inventive solutions customized to your requirements.
But hold on—it’s not all smooth sailing—there are also some challenges you need to be aware of.
Want to get details about self-hosting LLMs? Then, read our pragmatic article on Practical Strategies For Self-Hosting Large Language Models.
Challenges and Limitations of LLM Agents
When learning about the globe of Large Language Model (LLM) agents, you’ll locate they come with their fair share of challenges and restrictions. While these advanced AI systems have splendid abilities, it’s critical to comprehend where they might fall short.
Limited Context and Long-Term Planning Challenges
First off, LLM agents tussle with sustaining context over long chats. They might shine at comprehending and answering instant prompts, but keeping track of information across an elongated interaction is not their powerful suit. These restrictions make it hard for them to implement long-term planning efficiently, often causing them to miss the colossal picture.
Adapting to Specific Roles and Ensuring Consistent Outputs
You will also spot that their yields can be inconsistent. While they can produce coherent and contextually pertinent text, they might not always hit the mark, specifically when adjusting to precise roles or tones. Their inconsistency can be frustrating when you need dependable and accurate data.
Prompt Dependence and Accurate Knowledge Management
LLM agents heavily rely on the quality and clarity of prompts. If your prompt is ambiguous, the answer will likely reflect that. In addition, sustaining knowledge precisely is a congruous battle. They might have to attain enormous amounts of data, but ensuring they apply it properly in numerous contexts can be problematic.
Concerns Over Bias, Misinformation, and Privacy Leaks
Bias and misinformation are substantial concerns. These models grasp from enormous datasets, which may include partial or false data. As an outcome, they can accidentally commemorate stereotypes or spread inaccuracies. Privacy leaks are another crucial problem. Since LLM agents refine large amounts of information, ensuring they don’t accidentally disclose sensitive data is chief.
Environmental Impact Due to Computational Demands
Lastly, the environmental impact of these models can’t be overlooked. Training and running LLM agents need significant computational power, resulting in high energy consumption. This demand has a noticeable environmental footprint, raising queries about the imperishable nature of such technologies.
Comprehending these challenges and restrictions helps you better explore the use of LLM agents, set pragmatic anticipations, and acknowledge potential problems proactively.
Check out our pragmatic article on Comparing Different Large Language Models (LLMs) to discover the strengths and applications of each.
Conclusion
To conclude the article, LLM agents represent a substantial leap forward in AI, providing a colossal potential across various fields. By comprehending their components, how they operate, and their applications, you can better appreciate their abilities and the effect they can have on our lives. While challenges remain, perpetual innovations vow to make LLM agents even stronger and more adaptable in the future.
Improve your LLM performance with RagaAI! Sign up today and experience our inventive LLM solutions designed to deliver exceptional outcomes across any application. Optimize effortlessly and accomplish outstanding results. Don’t miss out– Join the AI revolution now!
In the swiftly developing field of Artificial Intelligence, LLM agents are the latest expansions, able to solve intricate, multi-step real-globe tasks. You might have heard AI systems producing text or answering questions, but LLM agents take it a step further. They can execute a series of tasks that need sequential reasoning, making them indispensable in numerous applications.
Components of LLM Agents
Large Language Models (LLMs) are built from numerous key components that enable them to refine, comprehend, and produce human language efficiently. Here are the main components of LLM agents:
Agent Core
The agent core is very special to the heart of every LLM agent, acting as the fundamental coordination component. Researchers train this core on enormous datasets, enabling it to refine and produce human-like text. Its predominant function is to sustain the communications and functioning of the whole system, ensuring smooth communication between distinct components.
Memory
LLM agents have a sophisticated Memory system, which is important for controlling tasks and sustaining conversational records. Engineers divide this system into short-term and long-term memory components.
Short-term Memory: This memory manages prompt, context-specific data. It acts as a booklet where it swiftly writes down significant information during an interaction. This memory component traces the information of the ongoing interaction, helping the model acknowledge sensibly to the instant context. At the same time, Short-term memory is provisional, dispersing once the task is finished.
Long-Term Memory: On the contrary, this memory retains information over extended periods, providing consistency and pertinence in enduring interactions. It stores the information from past communications over weeks.
Planning
Efficient planning is important for the functionality of LLM agents. This module concentrates on the agent forms plans by fermenting tasks and queries followed by reflective analysis. By breaking down intricate questions into tractable parts, the agent can produce pragmatic strategies to acknowledge user requirements precisely. Let’s take a look at the two main phases of planning:
Plan Formulation
Plan formulation is the process through which an LLM agent develops a plan to accomplish a precise aim. This involves numerous key aspects:
Understanding the Goal: The agent first needs to clearly comprehend the intention. This could be anything from producing a comprehensive report to responding to an intricate query.
Collecting information: The agents gather pertinent data from their knowledge base or external sources. This may indulge data recovery, contextual comprehension, and determining key variables.
Generating Steps: The agent articulates a sequence of actions needed to accomplish the goal. The agent breaks down the task into tractable steps, considering reliability and reasonable order.
Evaluating Feasibility: The agent evaluates the feasibility of the plan, ensuring it applies to each step and that the overall strategy is likely to flourish.
Adapting for Constraints: The agent alters the strategy to account for any limitations, like time restrictions, resource attainability, or precise user needs.
For instance, if the aim is to draft a guide on the advantages of renewable energy, the LLM agent would:
Comprehend the scope and objective of the guide.
Collect information on distinct types of renewable energy sources, their advantages, and recent progressions.
Produce an outline with sections such as introduction, types of renewable energy, advantages, and conclusion.
Assess the data to ensure precision and pertinence.
Adapt the content to fit the needed word count and tone.
Plan Reflection
Plan reflection is the process by which an LLM agent assesses the result of its actions and grasps from its experiences. This indulges:
Retrospecting Actions: The agent retrospects the steps it took and the verdicts made during plan origination and enforcement.
Evaluating Results: The agent assesses the success of the strategy by contrasting the actual results with the desired goals.
Determining Errors: The agent determines any errors or areas where the plan did not perform as anticipated.
Learning and Adjusting: The agent uses the perceptions gained from reflection to enhance future performance. This could include updating its knowledge base, adapting algorithms, or altering plans.
Feedback Incorporation: The agent integrates responses from users or external analysts to process its approach and improve its capabilities.
Continuing with the renewable energy article instance, after finishing the draft, the agent would:
Retrospect the content to ensure all key points were coated.
Contrast the draft against the foremost aim to ensure it meets the needs.
Determine the gaps or inaccuracies in the information provided.
Grasp from any mistakes, like incorrect information or unclear elucidations to enhance future guides.
Incorporate feedback from editors or readers to process the writing style and content quality.
Tools
LLM agents use numerous tools to enforce their tasks. These tools include APIs and services specially customized for distinct duties, like data retrieval, refining, and content generation. By incorporating these tools, the agent improves its capabilities and delivers more accurate and effective outcomes.
Knowledge
The knowledge component is important for understanding and resolving tasks. This includes not only the enormous amount of data the agent has been instructed on but also the ability to recover auxiliary information as required. Refining LLMs and using tool-aided data retrieval are key aspects of this component, ensuring the agent can provide precise and pertinent responses.
Prompts
Eventually, Prompts are the guidelines that guide the LLM agent’s aims, behaviors, and strategies. These prompts are crucial for controlling the agent’s responses and actions, ensuring they follow the desired intentions and contexts explicit by the user.
Comprehending these components helps you learn the complex functioning of LLM agents and their prospective applications across numerous domains.
Alright, now that we’ve got a grasp of the pieces that make up an LLM agent, let’s dive into how they actually work.
Want to gain deeper perceptions into evaluating the performance of Large Language Models? Check out our thorough article on Evaluating Large Language Models: Methods and Metrics.
How LLM Agents Work
LLM agents work by using prompts to guide their actions and answers, using memory for coherent communication, deliberately planning through task fermentation and reflection, employing tools and APIs for precise tasks, and constantly enhancing based on feedback and flexible learning.
Using Prompts for Actions and Responses
You communicate with LLM agents predominantly through prompts. Think of prompts as guidelines or queries you give to Artificial Intelligence. These prompts guide the AI on what actions to take or answers to produce. For instance, if you ask an LLM agent to draft an email, your prompt might include information about the email’s objective, the recipient, and key points to cover. The AI then refines this input and crafts a response customized to your requirements. This prompt-driven interaction is what makes LLM agents incredibly adaptable and able to handle a wide range of tasks.
Memory Utilization for Effective Decision-Making
LLM agents use memory to ensure coherent interactions and efficient decision-making. When you are involved in a conversation with an LLM agent, it reminds you of previous interactions and uses that data to offer pertinent and congruous responses. This memory ability permits the agent to sustain context over multiple exchanges, making the communication more natural and useful. For example, if you’re discussing a project over numerous sessions, the AI can remember information from past conversations, helping you pick up right where you left off.
Task Decomposition and Reflective Analysis in Strategic Planning
Strategic planning is a key strength of LLM agents. They break down intricate tasks into inferior, tractable components, a process called task decomposition. This approach enables the AI to tackle complex issues in an orderly. Moreover, LLM agents use reflective analysis to assess their actions and results. By reflecting on what functioned well and what didn’t, they can adapt their plans to enhance future performance. This amalgamation of task decomposition and reflection helps LLM agents effectively regulate and finish multifaceted tasks.
Tools and APIs for Task Execution
To implement precise tasks, LLM agents often employ several tools and APIs (Application Programming Interfaces). These external resources improve the AI's capabilities by offering specialized functions that fundamental models may not innately possess. For instance, an LLM agent might use a translation API to convert text from one language to another or a weather API to retrieve current weather information. By incorporating these tools, LLM agents can provide more accurate and thorough solutions customized to your precise needs.
Feedback-Driven Continuous Improvement
LLM agents constantly enhance through feedback and flexible grasping. When you give feedback on their performance, whether positive or negative, the AI uses this data to process its future responses. This flexible learning process permits LLM agents to become more precise and efficient over time. For example, suppose you fix an error or recommend a better way to execute a task. In that case, the AI learns from this input, improving its capability to meet your anticipations in ensuing communications. This constant enhancement cycle ensures that LLM agents remain robust and receptive to your growing requirements.
So, you've seen the building blocks–now, let’s see them in action!
Explore how to smoothly incorporate low-code and visual programming into your tasks by reading our pragmatic guide on Building Low-Code LLM Apps with Visual Programming.
Applications and Use Cases of LLM Agents
LLM Agents are flexible tools that can transform several fields with their advanced capabilities. Here’s how you can use the power of LLM agents across distinct domains:
Customer Support, Content Creation, Language Translation
Envision having a support that never tries and can handle multiple questions concurrently. LLM agents can sleek your customer support by giving rapid, precise responses, ensuring customer contentment. When it comes to creating content, these agents can produce high-quality guides, blog posts, or social media updates, saving you precious time. Need to interact in multiple languages? LLM agents can translate text effectively, breaking down language obstacles and enabling smooth universal interactions.
Education and Tutoring, Programming, Research
In education, LLM agents can cater as personal tutors, providing elucidations, responding to queries, and offering personalized learning experiences. For programming, they can aid by producing code snippets, debugging, and elucidating intricate concepts. Researchers take advantage of these agents through their capability to sum up enormous amounts of data, determine pertinent studies, and even recommend new research directions.
Healthcare Assistance, Personal Assistants
Healthcare professionals can use LLM agents for preliminary diagnosis, patient records inspection, and medical transcription, improving effectiveness and precision. As personal assistants, these agents can sustain your schedules, set reminders, and manage daily tasks, permitting you to concentrate on more crucial activities.
Legal and Compliance Support, Accessibility Tools
Going through the legitimate scenario can be daunting. LLM agents can help by drafting legitimate documents, performing contract inspections, and ensuring compliance with regulations. For individuals with impairments, these agents can offer attainability tools, like real-time transcription and text-to-speech services, making digital content more attainable.
Interactive Entertainment, Marketing, Social Media Management
In entertainment, LLM agents can create communicative stories, counterfeit conversations, and improve gaming experiences. Marketers can use these agents to determine market trends, produce persuasive content, and sustain campaigns. For social media management, LLM agents can automate posts, engage with followers, and trace performance metrics, elevating your online presence.
Human Resources Management
The Human Resources department can aid incredibly from LLM agents. They can automate recruitment procedures, screen resumes, schedule interviews, and even supervise preliminary candidate evaluation. This not only saves time but also helps in locating the best talent more effectively.
By incorporating LLM agents into your productivity, you can improve workflow, enhance customer experiences, and stay ahead in a gradually advanced globe. Whether you’re in customer support, education, healthcare, or any other field, LLM agents provide inventive solutions customized to your requirements.
But hold on—it’s not all smooth sailing—there are also some challenges you need to be aware of.
Want to get details about self-hosting LLMs? Then, read our pragmatic article on Practical Strategies For Self-Hosting Large Language Models.
Challenges and Limitations of LLM Agents
When learning about the globe of Large Language Model (LLM) agents, you’ll locate they come with their fair share of challenges and restrictions. While these advanced AI systems have splendid abilities, it’s critical to comprehend where they might fall short.
Limited Context and Long-Term Planning Challenges
First off, LLM agents tussle with sustaining context over long chats. They might shine at comprehending and answering instant prompts, but keeping track of information across an elongated interaction is not their powerful suit. These restrictions make it hard for them to implement long-term planning efficiently, often causing them to miss the colossal picture.
Adapting to Specific Roles and Ensuring Consistent Outputs
You will also spot that their yields can be inconsistent. While they can produce coherent and contextually pertinent text, they might not always hit the mark, specifically when adjusting to precise roles or tones. Their inconsistency can be frustrating when you need dependable and accurate data.
Prompt Dependence and Accurate Knowledge Management
LLM agents heavily rely on the quality and clarity of prompts. If your prompt is ambiguous, the answer will likely reflect that. In addition, sustaining knowledge precisely is a congruous battle. They might have to attain enormous amounts of data, but ensuring they apply it properly in numerous contexts can be problematic.
Concerns Over Bias, Misinformation, and Privacy Leaks
Bias and misinformation are substantial concerns. These models grasp from enormous datasets, which may include partial or false data. As an outcome, they can accidentally commemorate stereotypes or spread inaccuracies. Privacy leaks are another crucial problem. Since LLM agents refine large amounts of information, ensuring they don’t accidentally disclose sensitive data is chief.
Environmental Impact Due to Computational Demands
Lastly, the environmental impact of these models can’t be overlooked. Training and running LLM agents need significant computational power, resulting in high energy consumption. This demand has a noticeable environmental footprint, raising queries about the imperishable nature of such technologies.
Comprehending these challenges and restrictions helps you better explore the use of LLM agents, set pragmatic anticipations, and acknowledge potential problems proactively.
Check out our pragmatic article on Comparing Different Large Language Models (LLMs) to discover the strengths and applications of each.
Conclusion
To conclude the article, LLM agents represent a substantial leap forward in AI, providing a colossal potential across various fields. By comprehending their components, how they operate, and their applications, you can better appreciate their abilities and the effect they can have on our lives. While challenges remain, perpetual innovations vow to make LLM agents even stronger and more adaptable in the future.
Improve your LLM performance with RagaAI! Sign up today and experience our inventive LLM solutions designed to deliver exceptional outcomes across any application. Optimize effortlessly and accomplish outstanding results. Don’t miss out– Join the AI revolution now!
In the swiftly developing field of Artificial Intelligence, LLM agents are the latest expansions, able to solve intricate, multi-step real-globe tasks. You might have heard AI systems producing text or answering questions, but LLM agents take it a step further. They can execute a series of tasks that need sequential reasoning, making them indispensable in numerous applications.
Components of LLM Agents
Large Language Models (LLMs) are built from numerous key components that enable them to refine, comprehend, and produce human language efficiently. Here are the main components of LLM agents:
Agent Core
The agent core is very special to the heart of every LLM agent, acting as the fundamental coordination component. Researchers train this core on enormous datasets, enabling it to refine and produce human-like text. Its predominant function is to sustain the communications and functioning of the whole system, ensuring smooth communication between distinct components.
Memory
LLM agents have a sophisticated Memory system, which is important for controlling tasks and sustaining conversational records. Engineers divide this system into short-term and long-term memory components.
Short-term Memory: This memory manages prompt, context-specific data. It acts as a booklet where it swiftly writes down significant information during an interaction. This memory component traces the information of the ongoing interaction, helping the model acknowledge sensibly to the instant context. At the same time, Short-term memory is provisional, dispersing once the task is finished.
Long-Term Memory: On the contrary, this memory retains information over extended periods, providing consistency and pertinence in enduring interactions. It stores the information from past communications over weeks.
Planning
Efficient planning is important for the functionality of LLM agents. This module concentrates on the agent forms plans by fermenting tasks and queries followed by reflective analysis. By breaking down intricate questions into tractable parts, the agent can produce pragmatic strategies to acknowledge user requirements precisely. Let’s take a look at the two main phases of planning:
Plan Formulation
Plan formulation is the process through which an LLM agent develops a plan to accomplish a precise aim. This involves numerous key aspects:
Understanding the Goal: The agent first needs to clearly comprehend the intention. This could be anything from producing a comprehensive report to responding to an intricate query.
Collecting information: The agents gather pertinent data from their knowledge base or external sources. This may indulge data recovery, contextual comprehension, and determining key variables.
Generating Steps: The agent articulates a sequence of actions needed to accomplish the goal. The agent breaks down the task into tractable steps, considering reliability and reasonable order.
Evaluating Feasibility: The agent evaluates the feasibility of the plan, ensuring it applies to each step and that the overall strategy is likely to flourish.
Adapting for Constraints: The agent alters the strategy to account for any limitations, like time restrictions, resource attainability, or precise user needs.
For instance, if the aim is to draft a guide on the advantages of renewable energy, the LLM agent would:
Comprehend the scope and objective of the guide.
Collect information on distinct types of renewable energy sources, their advantages, and recent progressions.
Produce an outline with sections such as introduction, types of renewable energy, advantages, and conclusion.
Assess the data to ensure precision and pertinence.
Adapt the content to fit the needed word count and tone.
Plan Reflection
Plan reflection is the process by which an LLM agent assesses the result of its actions and grasps from its experiences. This indulges:
Retrospecting Actions: The agent retrospects the steps it took and the verdicts made during plan origination and enforcement.
Evaluating Results: The agent assesses the success of the strategy by contrasting the actual results with the desired goals.
Determining Errors: The agent determines any errors or areas where the plan did not perform as anticipated.
Learning and Adjusting: The agent uses the perceptions gained from reflection to enhance future performance. This could include updating its knowledge base, adapting algorithms, or altering plans.
Feedback Incorporation: The agent integrates responses from users or external analysts to process its approach and improve its capabilities.
Continuing with the renewable energy article instance, after finishing the draft, the agent would:
Retrospect the content to ensure all key points were coated.
Contrast the draft against the foremost aim to ensure it meets the needs.
Determine the gaps or inaccuracies in the information provided.
Grasp from any mistakes, like incorrect information or unclear elucidations to enhance future guides.
Incorporate feedback from editors or readers to process the writing style and content quality.
Tools
LLM agents use numerous tools to enforce their tasks. These tools include APIs and services specially customized for distinct duties, like data retrieval, refining, and content generation. By incorporating these tools, the agent improves its capabilities and delivers more accurate and effective outcomes.
Knowledge
The knowledge component is important for understanding and resolving tasks. This includes not only the enormous amount of data the agent has been instructed on but also the ability to recover auxiliary information as required. Refining LLMs and using tool-aided data retrieval are key aspects of this component, ensuring the agent can provide precise and pertinent responses.
Prompts
Eventually, Prompts are the guidelines that guide the LLM agent’s aims, behaviors, and strategies. These prompts are crucial for controlling the agent’s responses and actions, ensuring they follow the desired intentions and contexts explicit by the user.
Comprehending these components helps you learn the complex functioning of LLM agents and their prospective applications across numerous domains.
Alright, now that we’ve got a grasp of the pieces that make up an LLM agent, let’s dive into how they actually work.
Want to gain deeper perceptions into evaluating the performance of Large Language Models? Check out our thorough article on Evaluating Large Language Models: Methods and Metrics.
How LLM Agents Work
LLM agents work by using prompts to guide their actions and answers, using memory for coherent communication, deliberately planning through task fermentation and reflection, employing tools and APIs for precise tasks, and constantly enhancing based on feedback and flexible learning.
Using Prompts for Actions and Responses
You communicate with LLM agents predominantly through prompts. Think of prompts as guidelines or queries you give to Artificial Intelligence. These prompts guide the AI on what actions to take or answers to produce. For instance, if you ask an LLM agent to draft an email, your prompt might include information about the email’s objective, the recipient, and key points to cover. The AI then refines this input and crafts a response customized to your requirements. This prompt-driven interaction is what makes LLM agents incredibly adaptable and able to handle a wide range of tasks.
Memory Utilization for Effective Decision-Making
LLM agents use memory to ensure coherent interactions and efficient decision-making. When you are involved in a conversation with an LLM agent, it reminds you of previous interactions and uses that data to offer pertinent and congruous responses. This memory ability permits the agent to sustain context over multiple exchanges, making the communication more natural and useful. For example, if you’re discussing a project over numerous sessions, the AI can remember information from past conversations, helping you pick up right where you left off.
Task Decomposition and Reflective Analysis in Strategic Planning
Strategic planning is a key strength of LLM agents. They break down intricate tasks into inferior, tractable components, a process called task decomposition. This approach enables the AI to tackle complex issues in an orderly. Moreover, LLM agents use reflective analysis to assess their actions and results. By reflecting on what functioned well and what didn’t, they can adapt their plans to enhance future performance. This amalgamation of task decomposition and reflection helps LLM agents effectively regulate and finish multifaceted tasks.
Tools and APIs for Task Execution
To implement precise tasks, LLM agents often employ several tools and APIs (Application Programming Interfaces). These external resources improve the AI's capabilities by offering specialized functions that fundamental models may not innately possess. For instance, an LLM agent might use a translation API to convert text from one language to another or a weather API to retrieve current weather information. By incorporating these tools, LLM agents can provide more accurate and thorough solutions customized to your precise needs.
Feedback-Driven Continuous Improvement
LLM agents constantly enhance through feedback and flexible grasping. When you give feedback on their performance, whether positive or negative, the AI uses this data to process its future responses. This flexible learning process permits LLM agents to become more precise and efficient over time. For example, suppose you fix an error or recommend a better way to execute a task. In that case, the AI learns from this input, improving its capability to meet your anticipations in ensuing communications. This constant enhancement cycle ensures that LLM agents remain robust and receptive to your growing requirements.
So, you've seen the building blocks–now, let’s see them in action!
Explore how to smoothly incorporate low-code and visual programming into your tasks by reading our pragmatic guide on Building Low-Code LLM Apps with Visual Programming.
Applications and Use Cases of LLM Agents
LLM Agents are flexible tools that can transform several fields with their advanced capabilities. Here’s how you can use the power of LLM agents across distinct domains:
Customer Support, Content Creation, Language Translation
Envision having a support that never tries and can handle multiple questions concurrently. LLM agents can sleek your customer support by giving rapid, precise responses, ensuring customer contentment. When it comes to creating content, these agents can produce high-quality guides, blog posts, or social media updates, saving you precious time. Need to interact in multiple languages? LLM agents can translate text effectively, breaking down language obstacles and enabling smooth universal interactions.
Education and Tutoring, Programming, Research
In education, LLM agents can cater as personal tutors, providing elucidations, responding to queries, and offering personalized learning experiences. For programming, they can aid by producing code snippets, debugging, and elucidating intricate concepts. Researchers take advantage of these agents through their capability to sum up enormous amounts of data, determine pertinent studies, and even recommend new research directions.
Healthcare Assistance, Personal Assistants
Healthcare professionals can use LLM agents for preliminary diagnosis, patient records inspection, and medical transcription, improving effectiveness and precision. As personal assistants, these agents can sustain your schedules, set reminders, and manage daily tasks, permitting you to concentrate on more crucial activities.
Legal and Compliance Support, Accessibility Tools
Going through the legitimate scenario can be daunting. LLM agents can help by drafting legitimate documents, performing contract inspections, and ensuring compliance with regulations. For individuals with impairments, these agents can offer attainability tools, like real-time transcription and text-to-speech services, making digital content more attainable.
Interactive Entertainment, Marketing, Social Media Management
In entertainment, LLM agents can create communicative stories, counterfeit conversations, and improve gaming experiences. Marketers can use these agents to determine market trends, produce persuasive content, and sustain campaigns. For social media management, LLM agents can automate posts, engage with followers, and trace performance metrics, elevating your online presence.
Human Resources Management
The Human Resources department can aid incredibly from LLM agents. They can automate recruitment procedures, screen resumes, schedule interviews, and even supervise preliminary candidate evaluation. This not only saves time but also helps in locating the best talent more effectively.
By incorporating LLM agents into your productivity, you can improve workflow, enhance customer experiences, and stay ahead in a gradually advanced globe. Whether you’re in customer support, education, healthcare, or any other field, LLM agents provide inventive solutions customized to your requirements.
But hold on—it’s not all smooth sailing—there are also some challenges you need to be aware of.
Want to get details about self-hosting LLMs? Then, read our pragmatic article on Practical Strategies For Self-Hosting Large Language Models.
Challenges and Limitations of LLM Agents
When learning about the globe of Large Language Model (LLM) agents, you’ll locate they come with their fair share of challenges and restrictions. While these advanced AI systems have splendid abilities, it’s critical to comprehend where they might fall short.
Limited Context and Long-Term Planning Challenges
First off, LLM agents tussle with sustaining context over long chats. They might shine at comprehending and answering instant prompts, but keeping track of information across an elongated interaction is not their powerful suit. These restrictions make it hard for them to implement long-term planning efficiently, often causing them to miss the colossal picture.
Adapting to Specific Roles and Ensuring Consistent Outputs
You will also spot that their yields can be inconsistent. While they can produce coherent and contextually pertinent text, they might not always hit the mark, specifically when adjusting to precise roles or tones. Their inconsistency can be frustrating when you need dependable and accurate data.
Prompt Dependence and Accurate Knowledge Management
LLM agents heavily rely on the quality and clarity of prompts. If your prompt is ambiguous, the answer will likely reflect that. In addition, sustaining knowledge precisely is a congruous battle. They might have to attain enormous amounts of data, but ensuring they apply it properly in numerous contexts can be problematic.
Concerns Over Bias, Misinformation, and Privacy Leaks
Bias and misinformation are substantial concerns. These models grasp from enormous datasets, which may include partial or false data. As an outcome, they can accidentally commemorate stereotypes or spread inaccuracies. Privacy leaks are another crucial problem. Since LLM agents refine large amounts of information, ensuring they don’t accidentally disclose sensitive data is chief.
Environmental Impact Due to Computational Demands
Lastly, the environmental impact of these models can’t be overlooked. Training and running LLM agents need significant computational power, resulting in high energy consumption. This demand has a noticeable environmental footprint, raising queries about the imperishable nature of such technologies.
Comprehending these challenges and restrictions helps you better explore the use of LLM agents, set pragmatic anticipations, and acknowledge potential problems proactively.
Check out our pragmatic article on Comparing Different Large Language Models (LLMs) to discover the strengths and applications of each.
Conclusion
To conclude the article, LLM agents represent a substantial leap forward in AI, providing a colossal potential across various fields. By comprehending their components, how they operate, and their applications, you can better appreciate their abilities and the effect they can have on our lives. While challenges remain, perpetual innovations vow to make LLM agents even stronger and more adaptable in the future.
Improve your LLM performance with RagaAI! Sign up today and experience our inventive LLM solutions designed to deliver exceptional outcomes across any application. Optimize effortlessly and accomplish outstanding results. Don’t miss out– Join the AI revolution now!
In the swiftly developing field of Artificial Intelligence, LLM agents are the latest expansions, able to solve intricate, multi-step real-globe tasks. You might have heard AI systems producing text or answering questions, but LLM agents take it a step further. They can execute a series of tasks that need sequential reasoning, making them indispensable in numerous applications.
Components of LLM Agents
Large Language Models (LLMs) are built from numerous key components that enable them to refine, comprehend, and produce human language efficiently. Here are the main components of LLM agents:
Agent Core
The agent core is very special to the heart of every LLM agent, acting as the fundamental coordination component. Researchers train this core on enormous datasets, enabling it to refine and produce human-like text. Its predominant function is to sustain the communications and functioning of the whole system, ensuring smooth communication between distinct components.
Memory
LLM agents have a sophisticated Memory system, which is important for controlling tasks and sustaining conversational records. Engineers divide this system into short-term and long-term memory components.
Short-term Memory: This memory manages prompt, context-specific data. It acts as a booklet where it swiftly writes down significant information during an interaction. This memory component traces the information of the ongoing interaction, helping the model acknowledge sensibly to the instant context. At the same time, Short-term memory is provisional, dispersing once the task is finished.
Long-Term Memory: On the contrary, this memory retains information over extended periods, providing consistency and pertinence in enduring interactions. It stores the information from past communications over weeks.
Planning
Efficient planning is important for the functionality of LLM agents. This module concentrates on the agent forms plans by fermenting tasks and queries followed by reflective analysis. By breaking down intricate questions into tractable parts, the agent can produce pragmatic strategies to acknowledge user requirements precisely. Let’s take a look at the two main phases of planning:
Plan Formulation
Plan formulation is the process through which an LLM agent develops a plan to accomplish a precise aim. This involves numerous key aspects:
Understanding the Goal: The agent first needs to clearly comprehend the intention. This could be anything from producing a comprehensive report to responding to an intricate query.
Collecting information: The agents gather pertinent data from their knowledge base or external sources. This may indulge data recovery, contextual comprehension, and determining key variables.
Generating Steps: The agent articulates a sequence of actions needed to accomplish the goal. The agent breaks down the task into tractable steps, considering reliability and reasonable order.
Evaluating Feasibility: The agent evaluates the feasibility of the plan, ensuring it applies to each step and that the overall strategy is likely to flourish.
Adapting for Constraints: The agent alters the strategy to account for any limitations, like time restrictions, resource attainability, or precise user needs.
For instance, if the aim is to draft a guide on the advantages of renewable energy, the LLM agent would:
Comprehend the scope and objective of the guide.
Collect information on distinct types of renewable energy sources, their advantages, and recent progressions.
Produce an outline with sections such as introduction, types of renewable energy, advantages, and conclusion.
Assess the data to ensure precision and pertinence.
Adapt the content to fit the needed word count and tone.
Plan Reflection
Plan reflection is the process by which an LLM agent assesses the result of its actions and grasps from its experiences. This indulges:
Retrospecting Actions: The agent retrospects the steps it took and the verdicts made during plan origination and enforcement.
Evaluating Results: The agent assesses the success of the strategy by contrasting the actual results with the desired goals.
Determining Errors: The agent determines any errors or areas where the plan did not perform as anticipated.
Learning and Adjusting: The agent uses the perceptions gained from reflection to enhance future performance. This could include updating its knowledge base, adapting algorithms, or altering plans.
Feedback Incorporation: The agent integrates responses from users or external analysts to process its approach and improve its capabilities.
Continuing with the renewable energy article instance, after finishing the draft, the agent would:
Retrospect the content to ensure all key points were coated.
Contrast the draft against the foremost aim to ensure it meets the needs.
Determine the gaps or inaccuracies in the information provided.
Grasp from any mistakes, like incorrect information or unclear elucidations to enhance future guides.
Incorporate feedback from editors or readers to process the writing style and content quality.
Tools
LLM agents use numerous tools to enforce their tasks. These tools include APIs and services specially customized for distinct duties, like data retrieval, refining, and content generation. By incorporating these tools, the agent improves its capabilities and delivers more accurate and effective outcomes.
Knowledge
The knowledge component is important for understanding and resolving tasks. This includes not only the enormous amount of data the agent has been instructed on but also the ability to recover auxiliary information as required. Refining LLMs and using tool-aided data retrieval are key aspects of this component, ensuring the agent can provide precise and pertinent responses.
Prompts
Eventually, Prompts are the guidelines that guide the LLM agent’s aims, behaviors, and strategies. These prompts are crucial for controlling the agent’s responses and actions, ensuring they follow the desired intentions and contexts explicit by the user.
Comprehending these components helps you learn the complex functioning of LLM agents and their prospective applications across numerous domains.
Alright, now that we’ve got a grasp of the pieces that make up an LLM agent, let’s dive into how they actually work.
Want to gain deeper perceptions into evaluating the performance of Large Language Models? Check out our thorough article on Evaluating Large Language Models: Methods and Metrics.
How LLM Agents Work
LLM agents work by using prompts to guide their actions and answers, using memory for coherent communication, deliberately planning through task fermentation and reflection, employing tools and APIs for precise tasks, and constantly enhancing based on feedback and flexible learning.
Using Prompts for Actions and Responses
You communicate with LLM agents predominantly through prompts. Think of prompts as guidelines or queries you give to Artificial Intelligence. These prompts guide the AI on what actions to take or answers to produce. For instance, if you ask an LLM agent to draft an email, your prompt might include information about the email’s objective, the recipient, and key points to cover. The AI then refines this input and crafts a response customized to your requirements. This prompt-driven interaction is what makes LLM agents incredibly adaptable and able to handle a wide range of tasks.
Memory Utilization for Effective Decision-Making
LLM agents use memory to ensure coherent interactions and efficient decision-making. When you are involved in a conversation with an LLM agent, it reminds you of previous interactions and uses that data to offer pertinent and congruous responses. This memory ability permits the agent to sustain context over multiple exchanges, making the communication more natural and useful. For example, if you’re discussing a project over numerous sessions, the AI can remember information from past conversations, helping you pick up right where you left off.
Task Decomposition and Reflective Analysis in Strategic Planning
Strategic planning is a key strength of LLM agents. They break down intricate tasks into inferior, tractable components, a process called task decomposition. This approach enables the AI to tackle complex issues in an orderly. Moreover, LLM agents use reflective analysis to assess their actions and results. By reflecting on what functioned well and what didn’t, they can adapt their plans to enhance future performance. This amalgamation of task decomposition and reflection helps LLM agents effectively regulate and finish multifaceted tasks.
Tools and APIs for Task Execution
To implement precise tasks, LLM agents often employ several tools and APIs (Application Programming Interfaces). These external resources improve the AI's capabilities by offering specialized functions that fundamental models may not innately possess. For instance, an LLM agent might use a translation API to convert text from one language to another or a weather API to retrieve current weather information. By incorporating these tools, LLM agents can provide more accurate and thorough solutions customized to your precise needs.
Feedback-Driven Continuous Improvement
LLM agents constantly enhance through feedback and flexible grasping. When you give feedback on their performance, whether positive or negative, the AI uses this data to process its future responses. This flexible learning process permits LLM agents to become more precise and efficient over time. For example, suppose you fix an error or recommend a better way to execute a task. In that case, the AI learns from this input, improving its capability to meet your anticipations in ensuing communications. This constant enhancement cycle ensures that LLM agents remain robust and receptive to your growing requirements.
So, you've seen the building blocks–now, let’s see them in action!
Explore how to smoothly incorporate low-code and visual programming into your tasks by reading our pragmatic guide on Building Low-Code LLM Apps with Visual Programming.
Applications and Use Cases of LLM Agents
LLM Agents are flexible tools that can transform several fields with their advanced capabilities. Here’s how you can use the power of LLM agents across distinct domains:
Customer Support, Content Creation, Language Translation
Envision having a support that never tries and can handle multiple questions concurrently. LLM agents can sleek your customer support by giving rapid, precise responses, ensuring customer contentment. When it comes to creating content, these agents can produce high-quality guides, blog posts, or social media updates, saving you precious time. Need to interact in multiple languages? LLM agents can translate text effectively, breaking down language obstacles and enabling smooth universal interactions.
Education and Tutoring, Programming, Research
In education, LLM agents can cater as personal tutors, providing elucidations, responding to queries, and offering personalized learning experiences. For programming, they can aid by producing code snippets, debugging, and elucidating intricate concepts. Researchers take advantage of these agents through their capability to sum up enormous amounts of data, determine pertinent studies, and even recommend new research directions.
Healthcare Assistance, Personal Assistants
Healthcare professionals can use LLM agents for preliminary diagnosis, patient records inspection, and medical transcription, improving effectiveness and precision. As personal assistants, these agents can sustain your schedules, set reminders, and manage daily tasks, permitting you to concentrate on more crucial activities.
Legal and Compliance Support, Accessibility Tools
Going through the legitimate scenario can be daunting. LLM agents can help by drafting legitimate documents, performing contract inspections, and ensuring compliance with regulations. For individuals with impairments, these agents can offer attainability tools, like real-time transcription and text-to-speech services, making digital content more attainable.
Interactive Entertainment, Marketing, Social Media Management
In entertainment, LLM agents can create communicative stories, counterfeit conversations, and improve gaming experiences. Marketers can use these agents to determine market trends, produce persuasive content, and sustain campaigns. For social media management, LLM agents can automate posts, engage with followers, and trace performance metrics, elevating your online presence.
Human Resources Management
The Human Resources department can aid incredibly from LLM agents. They can automate recruitment procedures, screen resumes, schedule interviews, and even supervise preliminary candidate evaluation. This not only saves time but also helps in locating the best talent more effectively.
By incorporating LLM agents into your productivity, you can improve workflow, enhance customer experiences, and stay ahead in a gradually advanced globe. Whether you’re in customer support, education, healthcare, or any other field, LLM agents provide inventive solutions customized to your requirements.
But hold on—it’s not all smooth sailing—there are also some challenges you need to be aware of.
Want to get details about self-hosting LLMs? Then, read our pragmatic article on Practical Strategies For Self-Hosting Large Language Models.
Challenges and Limitations of LLM Agents
When learning about the globe of Large Language Model (LLM) agents, you’ll locate they come with their fair share of challenges and restrictions. While these advanced AI systems have splendid abilities, it’s critical to comprehend where they might fall short.
Limited Context and Long-Term Planning Challenges
First off, LLM agents tussle with sustaining context over long chats. They might shine at comprehending and answering instant prompts, but keeping track of information across an elongated interaction is not their powerful suit. These restrictions make it hard for them to implement long-term planning efficiently, often causing them to miss the colossal picture.
Adapting to Specific Roles and Ensuring Consistent Outputs
You will also spot that their yields can be inconsistent. While they can produce coherent and contextually pertinent text, they might not always hit the mark, specifically when adjusting to precise roles or tones. Their inconsistency can be frustrating when you need dependable and accurate data.
Prompt Dependence and Accurate Knowledge Management
LLM agents heavily rely on the quality and clarity of prompts. If your prompt is ambiguous, the answer will likely reflect that. In addition, sustaining knowledge precisely is a congruous battle. They might have to attain enormous amounts of data, but ensuring they apply it properly in numerous contexts can be problematic.
Concerns Over Bias, Misinformation, and Privacy Leaks
Bias and misinformation are substantial concerns. These models grasp from enormous datasets, which may include partial or false data. As an outcome, they can accidentally commemorate stereotypes or spread inaccuracies. Privacy leaks are another crucial problem. Since LLM agents refine large amounts of information, ensuring they don’t accidentally disclose sensitive data is chief.
Environmental Impact Due to Computational Demands
Lastly, the environmental impact of these models can’t be overlooked. Training and running LLM agents need significant computational power, resulting in high energy consumption. This demand has a noticeable environmental footprint, raising queries about the imperishable nature of such technologies.
Comprehending these challenges and restrictions helps you better explore the use of LLM agents, set pragmatic anticipations, and acknowledge potential problems proactively.
Check out our pragmatic article on Comparing Different Large Language Models (LLMs) to discover the strengths and applications of each.
Conclusion
To conclude the article, LLM agents represent a substantial leap forward in AI, providing a colossal potential across various fields. By comprehending their components, how they operate, and their applications, you can better appreciate their abilities and the effect they can have on our lives. While challenges remain, perpetual innovations vow to make LLM agents even stronger and more adaptable in the future.
Improve your LLM performance with RagaAI! Sign up today and experience our inventive LLM solutions designed to deliver exceptional outcomes across any application. Optimize effortlessly and accomplish outstanding results. Don’t miss out– Join the AI revolution now!