Creating Apps with Large Language Models
Creating Apps with Large Language Models
Creating Apps with Large Language Models
Rehan Asif
Nov 21, 2024
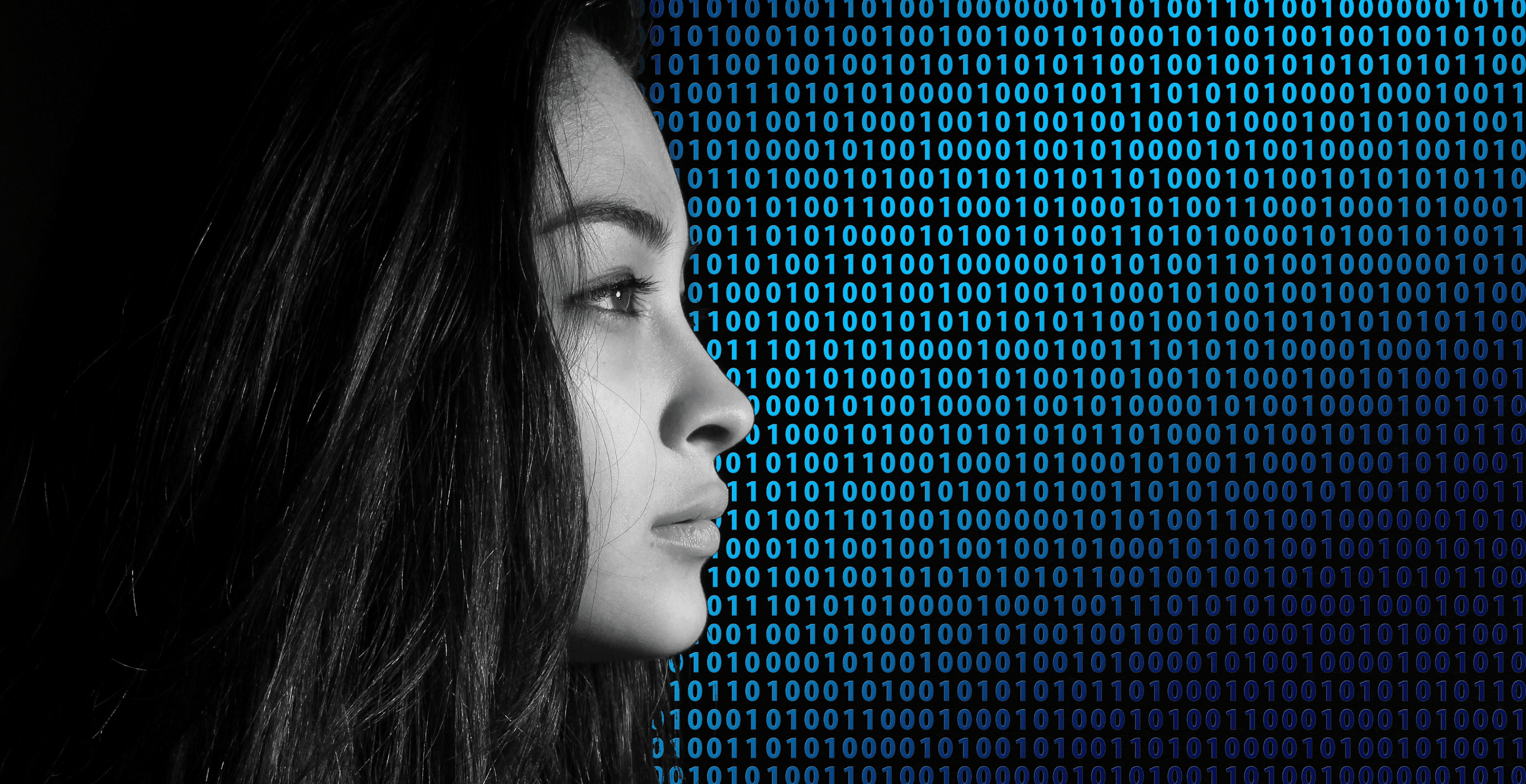
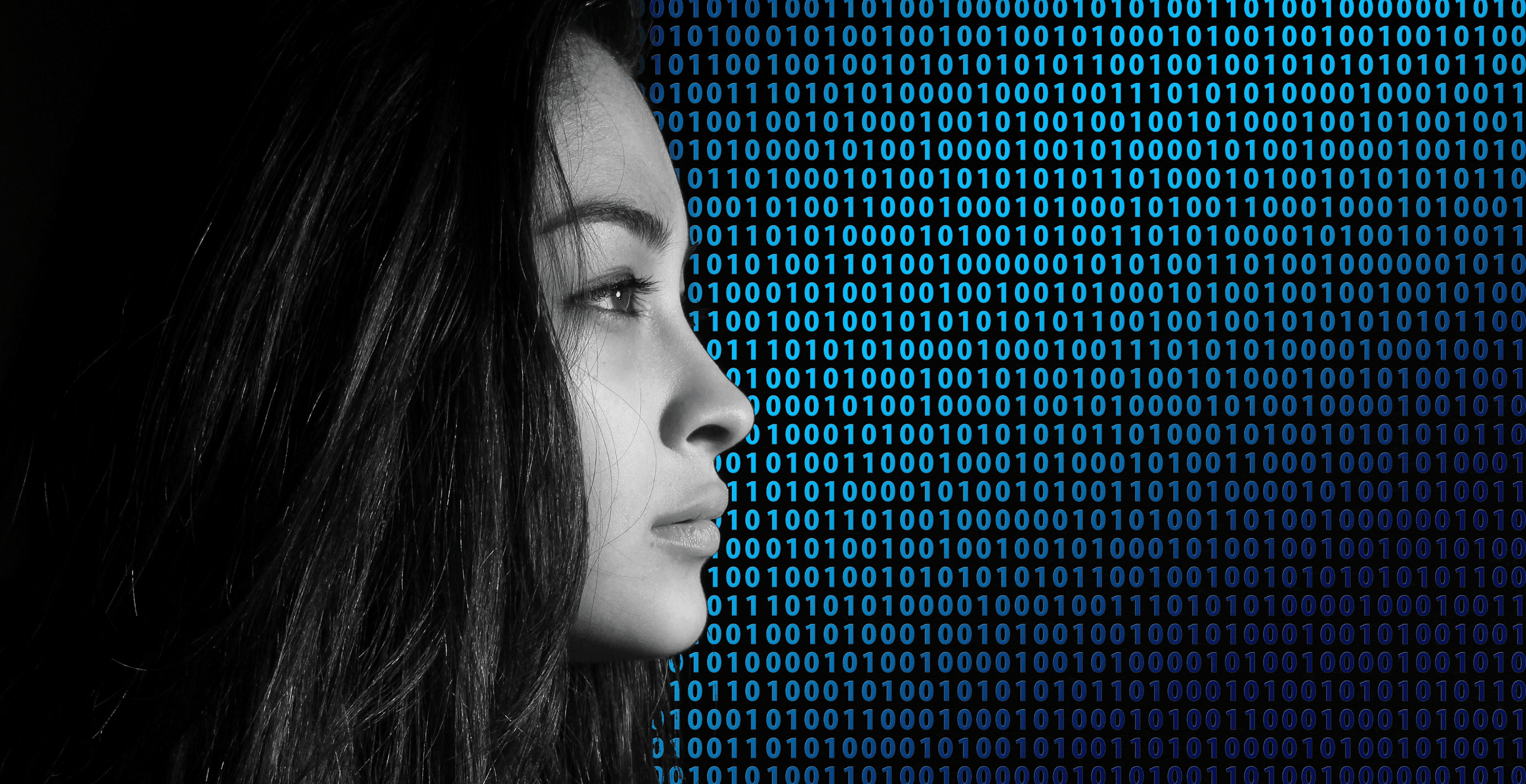
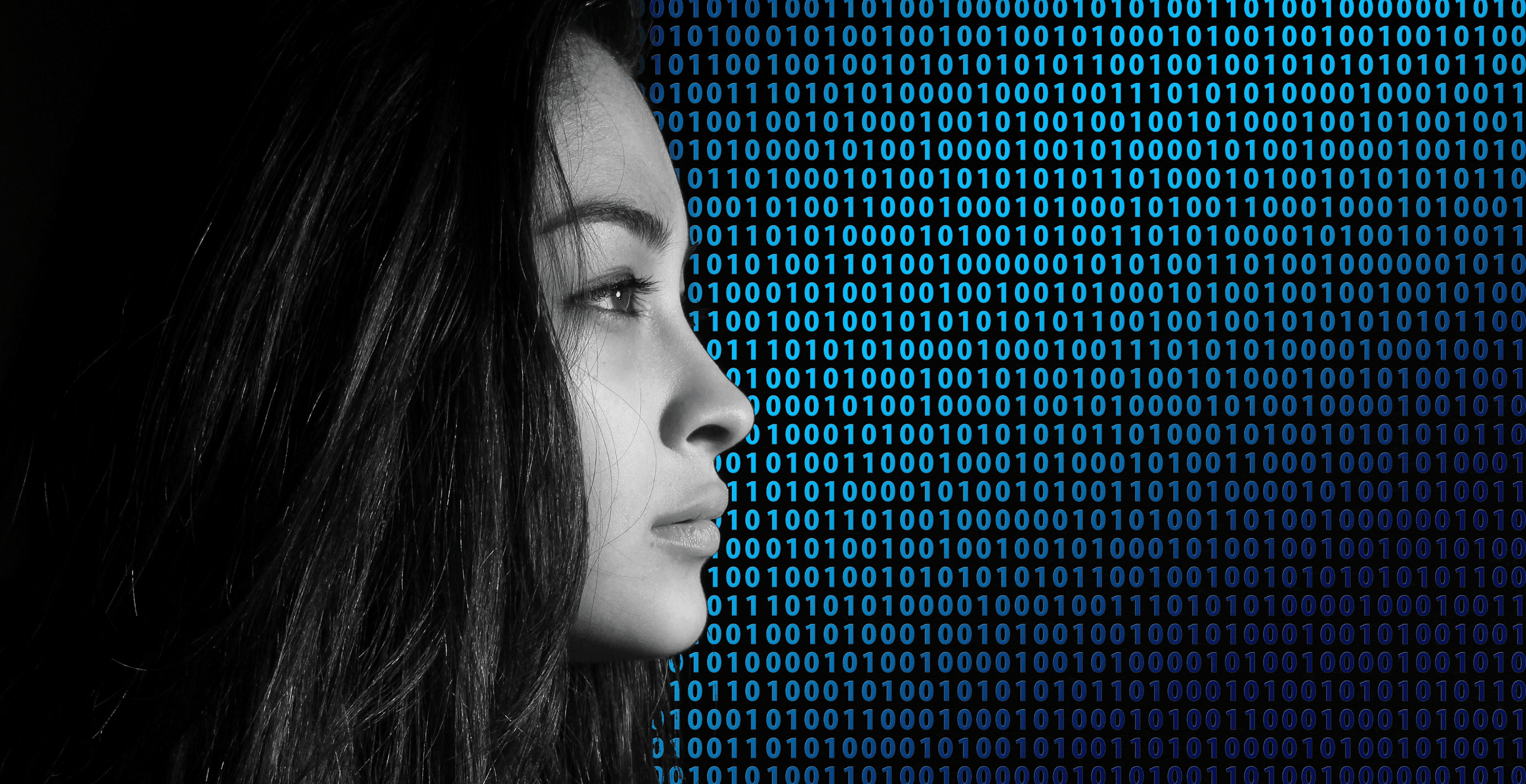
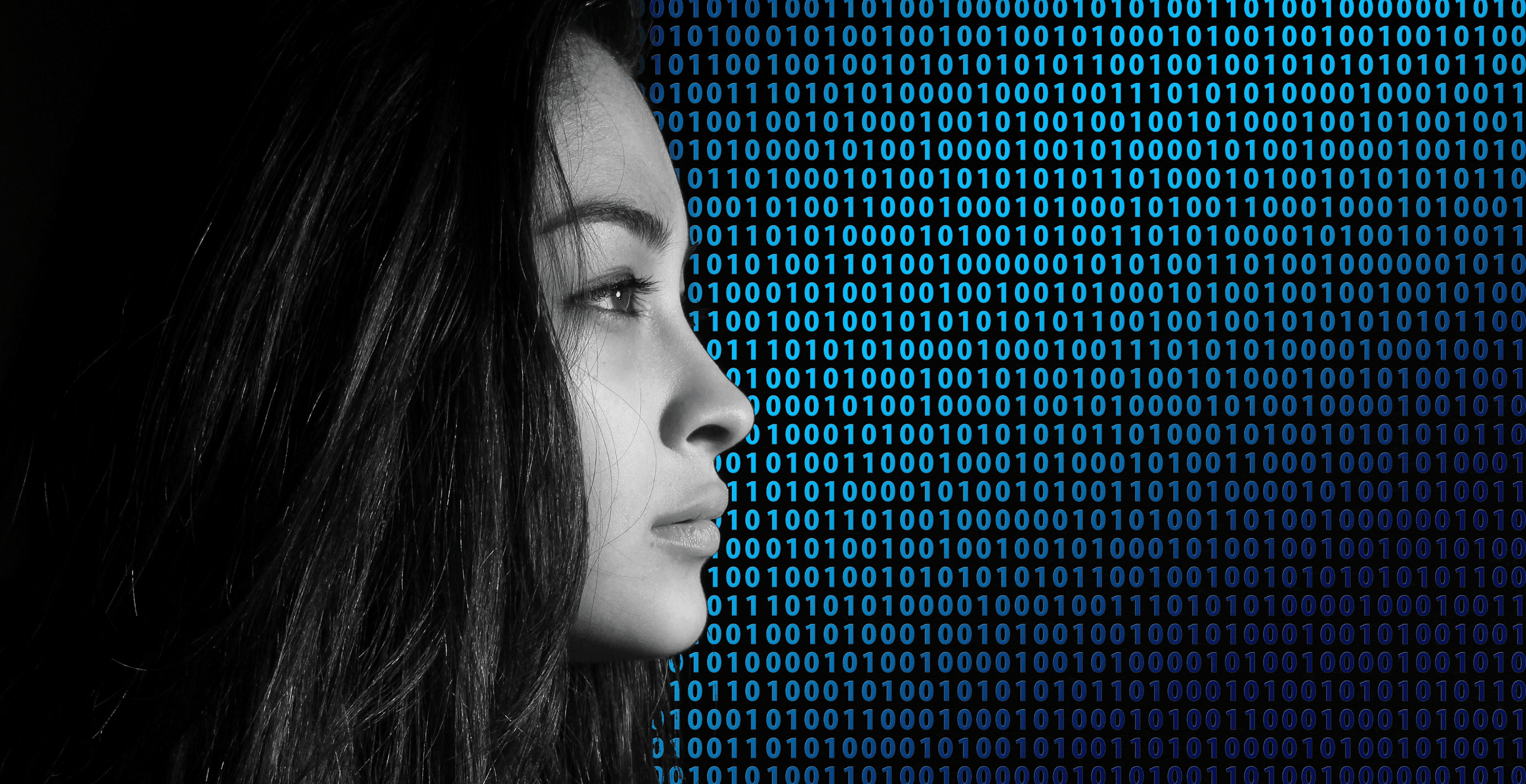
Large language models application through production has become a game-changer in the tech world. These advanced models, like GPT-4 and BERT, have transformed how developers approach app creation, offering new possibilities and efficiencies.
Integrating large language models into your apps can significantly enhance user experience and functionality. Imagine building a chatbot that understands nuanced customer queries or an app that generates content tailored to individual preferences. The Large language models application through production are vast and continually evolving, making this an exciting frontier for developers. Now, let's dive deeper into understanding large language models and their remarkable capabilities.
Overview of Large Language Models (LLMs)
Large Language Models (LLMs) have revolutionized the field of artificial intelligence. These models, like GPT-4 and BERT, are designed to understand and generate human-like text by predicting the next word in a sequence. Their ability to process and produce text with remarkable coherence makes them invaluable tools for a wide range of applications.
Definition
LLMs are advanced AI systems trained on vast datasets to predict and generate text. These models utilize deep learning techniques to analyze and understand the structure of language, allowing them to produce coherent and contextually relevant text.
Historical development and evolution:
Early Models: The journey began with simpler models like n-grams and statistical methods that could only handle limited contexts.
ntroduction of Neural Networks: The use of neural networks marked a significant improvement, allowing models to understand more complex patterns in language.
Transformer Architecture: The advent of transformer models, such as the Transformer by Vaswani et al., revolutionized the field, enabling more efficient processing of text and better handling of context.
Modern LLMs: Today, models like GPT-4 and Gemini represent the pinnacle of this evolution, capable of generating highly coherent and contextually relevant text across a wide range of applications.
Basic Understanding
By analyzing context and word patterns, LLMs can create responses that are contextually relevant and meaningful. They are capable of understanding complex language structures, idiomatic expressions, and the nuances of human communication, making them incredibly versatile.
Examples of popular LLMs:
GPT-4: OpenAI's GPT-4 is renowned for its capacity to produce precisely contextualized text, which makes it perfect for chatbots and other applications. GPT-4 can handle a wide range of tasks, from answering questions to writing essays, demonstrating a high level of linguistic competence.
Gemini: Created by Google DeepMind, Gemini excels in integrating various AI capabilities, including language understanding and generation. Gemini's advanced architecture allows it to perform a wide range of tasks, from conversational AI to complex problem-solving, showcasing its versatility in various applications.
Key differences and use cases:
GPT-4 vs. Gemini: While both models are highly capable, GPT-4 is particularly noted for its text generation prowess, whereas Gemini is praised for its integration of multiple AI functionalities.
Gemini: Unlike GPT-4, Gemini is primarily designed for tasks that require a deep understanding of context, making it ideal for search engines and other query-based applications.
Use Cases: GPT-4 is used for creating conversational agents and automated writing tools; Gemini is suitable for complex AI integrations and is optimal for improving search and retrieval systems.
Rise of LLMs in Application Development
The integration of LLMs into applications is rapidly becoming a standard in the tech industry. Their ability to enhance user interaction and automate complex tasks makes them highly relevant in today's digital landscape.
Growing trend of integrating LLMs into apps:
Increased Adoption: More businesses are incorporating LLMs into their apps to stay competitive and improve user experience. From startups to large enterprises, companies are leveraging LLMs to streamline operations, provide better customer support, and innovate their product offerings.
Technological Advancements: Continuous improvements in LLM technology are driving their widespread adoption across various industries. Enhanced computational power, access to larger datasets, and advancements in AI research have made LLMs more powerful and accessible than ever before.
Potential benefits and applications:
Enhanced User Interaction: LLMs can power chatbots and virtual assistants, providing users with more intuitive and responsive interactions. These models can understand and respond to user queries naturally and conversationally, improving overall user satisfaction.
Automated Content Creation: These models can generate high-quality content, from articles to social media posts, reducing the need for manual effort. Businesses can use LLMs to produce consistent and engaging content quickly, saving time and resources.
Data Analysis and Insights: LLMs can analyze large datasets to extract meaningful insights, aiding in decision-making processes. They can process and interpret complex data sets, identify patterns, and provide actionable insights, making them valuable tools for business intelligence.
Understanding the foundational aspects of LLMs and their growing importance sets the stage for exploring their practical applications in app development.
In the next section, we'll delve deeper into what makes large language models unique and how they work.
Working of Large Language Model
To appreciate the capabilities of LLMs, it's crucial to understand their underlying architecture and the training processes that enable them to perform complex tasks.
Basic architecture and training process:
Transformer Architecture: LLMs typically use transformer architecture, which relies on self-attention mechanisms to weigh the importance of different words in a sentence.
Training Process: Large volumes of text data are used to train these models, which then use the context given by the words before them to predict the word that will appear next in a sequence. For this training, billions of parameters need to be tuned using techniques like gradient descent.
Key features and functionalities:
Contextual Understanding: LLMs excel at understanding the context of words and sentences, allowing them to generate coherent and relevant text.
Text Generation: They can produce high-quality text that mimics human writing, useful for tasks like content creation and automated communication.
Versatility: LLMs are very flexible to a wide range of applications, from data analysis to customer support, since they can be tailored for certain tasks and use scenarios.
With this foundational knowledge, we can now explore how LLMs are applied in real-world scenarios and their impact on various industries.
Applications of LLMs in App Development
With a broad range of applications in app development, Large Language Models (LLMs) greatly improve the capabilities of different software solutions. From improving natural language processing to creating sophisticated chatbots and virtual assistants, LLMs are transforming how developers build and manage applications.
This section explores the diverse uses of LLMs in app development and their impact on user experience and functionality.
Natural Language Processing (NLP)
LLMs are a cornerstone of natural language processing, enabling apps to understand and generate human language with remarkable accuracy.
Text generation and completion:
Text Generation: LLMs can create coherent and contextually relevant text based on a given prompt. This is useful in applications such as automated writing tools, creative content generation, and scriptwriting.
Text Completion: These models can predict and complete sentences or paragraphs, enhancing user productivity in writing apps, email clients, and other text-heavy applications. For instance, they can help users draft emails by suggesting how to complete sentences based on the initial input.
Sentiment analysis and text classification:
Sentiment Analysis: LLMs can analyze text to determine the sentiment behind it, identifying whether the content is positive, negative, or neutral. This capability is essential for customer feedback analysis, social media monitoring, and market research.
Text Classification: They can categorize text into predefined labels, such as spam detection, topic categorization, and content moderation. This helps in organizing large volumes of text data efficiently.
Chatbots and Virtual Assistants
LLMs enhance the functionality and user experience of chatbots and virtual assistants, making them more interactive and helpful.
Enhancing user interaction and support:
Natural Conversations: LLMs enable chatbots to engage in natural, human-like conversations, improving user satisfaction and engagement. They can understand context, manage dialogue flow, and provide accurate responses.
Support Automation: These models can automate customer support tasks, answering frequently asked questions, resolving common issues, and directing users to appropriate resources without human intervention.
Examples of successful implementations:
Customer Service Bots: Companies like Amazon and Apple use LLM-powered chatbots to provide instant customer support, reducing wait times and improving service quality.
Virtual Assistants: Assistants like Google Assistant and Apple's Siri leverage LLMs to perform tasks such as setting reminders, providing weather updates, and answering queries, showcasing the practical applications of these models in daily life.
Content Creation and Management
LLMs streamline content creation and management processes, making it easier to produce and organize large volumes of content.
Automated content generation:
Content Creation: LLMs can generate articles, blog posts, social media updates, and more tailored to specific topics and styles. This capability helps businesses maintain a consistent content output without requiring extensive manual effort.
Personalized Content: They can create personalized content for different user segments, enhancing engagement by catering to individual preferences and needs.
Managing and curating content at scale:
Content Management: LLMs can assist in managing large content repositories by tagging, categorizing, and summarizing content, making it easier to retrieve and utilize.
Content Curation: These models can curate relevant content from various sources, providing users with up-to-date and personalized information feeds and enhancing the value of content platforms.
Data Analysis and Insights
LLMs offer powerful tools for analyzing data and extracting actionable insights, automating many of the processes traditionally handled by data scientists.
Extracting meaningful insights from data:
Data Interpretation: LLMs can analyze complex datasets to identify trends, patterns, and anomalies, helping businesses make informed decisions based on comprehensive data analysis.
Report Generation: Their ability to produce comprehensive reports and summaries from unprocessed data facilitates stakeholders' comprehension and application of the findings.
Automating data processing tasks:
Data Cleaning and Preparation: Time-consuming chores of cleaning and preparing data for analysis can be automated by LLMs, which also lowers the risk of error.
Automated Analytics: These models may analyze data in real time and deliver immediate insights, allowing businesses to respond swiftly to changing circumstances and make more effective decisions based on data.
By leveraging the capabilities of LLMs, developers can create more intelligent, responsive, and efficient applications that enhance user experience and drive business value.
Next, we'll explore the steps to integrate these powerful models into your app development process.
Steps to Integrate LLMs into Apps
Integrating Large Language Models (LLMs) into your applications can significantly enhance their capabilities and user experience. To deploy and manage the software, among other crucial processes, the process must first identify use cases.
The necessary actions to guarantee a seamless and successful integration of LLMs into your applications are described in this section.
Identifying Use Cases and Requirements
Before integrating an LLM, it's crucial to understand the specific needs of your application and define clear objectives and goals.
Understanding the specific needs of the App:
User Requirements: Determine what users need from the app and how an LLM can fulfill those needs. This involves analyzing user interactions, feedback, and common tasks that the app should support.
Functional Requirements: Identify the specific functionalities that the LLM will enhance or automate, such as natural language understanding, text generation, or sentiment analysis.
Defining clear objectives and goals:
Objectives: Clearly define your goals and assign metrics for achieving the LLM integration. For example, improving customer service response times or automating content generation.
Goals: Establish long-term goals that align with your business strategy, such as increasing user engagement, reducing operational costs, or expanding service offerings.
Choosing the Right LLM
Selecting the appropriate LLM is critical for achieving the desired outcomes and ensuring compatibility with your app.
Evaluating different models based on the use case:
Model Comparison: Compare various LLMs like GPT-4, Gemini, and others to understand their strengths and limitations. Consider how well each model addresses your specific use cases.
Performance Metrics: Evaluate models based on performance metrics such as accuracy, correctness, response time, and ability to handle specific tasks.
Considering factors like performance, cost, and compatibility:
Performance: Assess the performance of the LLM in real-world scenarios relevant to your application. Ensure it can handle the expected workload efficiently.
Cost: Consider the cost implications of using different LLMs, including licensing fees, computational resources, and ongoing maintenance.
Compatibility: Make sure the LLM you select can be easily incorporated into your development environment and is compatible with the technology stack you currently have.
Setting Up the Development Environment
A well-prepared development environment is essential for integrating and testing LLMs effectively.
Tools and frameworks for integration:
Development Tools: Utilize tools like TensorFlow, PyTorch, or specific APIs provided by LLM developers to integrate the models into your app.
TensorFlow Example
import tensorflow as tf
# Set up the computational environment
tf.config.set_visible_devices([tf.config.list_physical_devices('GPU')[0]])
PyTorch Example
import torch
import torch.nn as nn
# Set up the computational environment
device = torch.device("cuda:0" if torch.cuda.is_available() else "cpu")
Frameworks: Consider using frameworks like Hugging Face's Transformers or LangChain to simplify the integration process and manage model interactions.
Preparing the necessary infrastructure:
Computational Resources: Ensure you have adequate computational resources, such as GPUs or cloud-based services, to handle the demands of training and running LLMs.
Data Management: Set up robust data management practices to handle the large volumes of data required for training and fine-tuning the models.
Training and Fine-Tuning the Model
Customizing the LLM to suit your specific needs involves training and fine-tuning the model.
Customizing the Model for specific tasks:
Task-Specific Training: Train the model on datasets relevant to your application to improve its performance in specific tasks like customer support or content generation.
Domain Adaptation: Adapt the model to understand and generate text specific to your industry or domain, enhancing its relevance and accuracy.
Techniques for effective training and fine-tuning:
Supervised Learning: Use supervised learning techniques to train the model on labeled datasets, ensuring it learns to perform tasks accurately.
Supervised Learning Example (TensorFlow)
import tensorflow as tf
from tensorflow.keras.preprocessing.text import Tokenizer
from tensorflow.keras.preprocessing.sequence import pad_sequences
# Load the dataset
train_texts, train_labels = ...
# Tokenize the text data
tokenizer = Tokenizer(num_words=5000)
train_sequences = tokenizer.texts_to_sequences(train_texts)
# Pad the sequences
max_length = 128
padded_train_sequences = pad_sequences(train_sequences, maxlen=max_length)
# Define the model
model = tf.keras.Sequential([
tf.keras.layers.Embedding(input_dim=5000, output_dim=128, input_length=max_length),
tf.keras.layers.LSTM(64, return_sequences=True),
tf.keras.layers.Dense(1, activation='sigmoid')
])
# Compile the model
model.compile(optimizer='adam', loss='binary_crossentropy', metrics=['accuracy'])
# Train the model
model.fit(padded_train_sequences, train_labels, epochs=10, batch_size=32)
Fine-Tuning Example (PyTorch)
import torch
import torch.nn as nn
import torch.optim as optim
# Load the pre-trained model
model = torch.load('pretrained_model.pt')
# Define the fine-tuning model
fine_tuning_model = nn.Sequential(
nn.Linear(128, 64),
nn.ReLU(),
nn.Linear(64, 1),
nn.Sigmoid()
)
# Define the optimizer and loss fhttps://docs.google.com/document/d/1SFJpqYWCqufiQ7HpkOxTVF5gEI0uIFLjrtZjf4hIRvg/edit#heading=h.vwj13hlpd57function
optimizer = optim.Adam(fine_tuning_model.parameters(), lr=0.001)
criterion = nn.BCELoss()
# Train the fine-tuning model
for epoch in range(10):
for batch in train_loader:
inputs, labels = batch
outputs = fine_tuning_model(inputs)
loss = criterion(outputs, labels)
optimizer.zero_grad()
loss.backward()
optimizer.step()
Hyperparameter Tuning: Optimize hyperparameters to improve model performance, such as adjusting learning rates, batch sizes, and training epochs.
Implementing the Model into the App
The implementation phase involves coding and integrating the LLM into your app, followed by thorough testing and debugging.
Coding and integration processes:
API Integration: Implement the LLM using APIs, ensuring smooth communication between the model and your application.
Example of API Integration:
import requests
# Define the API endpoint
api_endpoint = 'https://api.example.com/llm'
# Define the request payload
payload = {'text': 'Hello, world'}
# Send the request
response = requests.post(api_endpoint, json=payload)
# Process the response
if response.status_code == 200:
response_data = response.json()
print(response_data)
Modular Design: Use a modular design approach to integrate the LLM, allowing for easier updates and maintenance.
Example of Modular Design:
class LLMIntegration:
def __init__(self):
self.llm = None
def load_llm(self):
self.llm = torch.load('pretrained_model.pt')
def generate_text(self, prompt):
if self.llm is None:
self.load_llm()
return self.llm.generate_text(prompt)
# Create an instance of the LLMIntegration class
llm_integration = LLMIntegration()
# Generate text using the LLM
prompt = 'Write a short story about a cat'
text = llm_integration.generate_text(prompt)
print(text)
Testing and debugging:
Unit Testing: Conduct unit tests to verify that individual components of the integration work as expected.
End-to-end Testing: Perform end-to-end testing to ensure the entire application functions correctly with the integrated LLM.
Debugging: Identify and fix any issues that arise during testing, ensuring the model operates smoothly within the app.
Deployment and Maintenance
Deploying the app in a production environment and maintaining it ensures long-term success and optimal performance.
Deploying the App in a production environment:
Scalability: Ensure the app can scale to handle increased usage and data volumes, utilizing cloud services or on-premises solutions as needed.
Scalability Example (Docker)
FROM python:3.9-slim
# Install required libraries
RUN pip install torch torchvision
# Copy the application code
COPY app.py .
# Define the environment variables
ENV LLM_MODEL=pretrained_model.pt
# Define the command to run the application
CMD ["python", "app.py"]
Security: Implement robust security measures to protect user data and ensure compliance with relevant regulations.
Ongoing monitoring and updates:
Performance Monitoring: Continuously monitor the performance of the LLM within the app, using tools to track metrics and identify potential issues.
Performance Monitoring Example (Python)
import logging
import time
# Set up the logging configuration
logging.basicConfig(level=logging.INFO)
# Define the performance monitoring function
def monitor_performance():
# Log the performance metrics
logging.info(f"Model accuracy: {model.accuracy:.2f}")
logging.info(f"Model latency: {model.latency:.2f} ms")
# Run the performance monitoring function periodically
while True:
monitor_performance()
time.sleep(60
# Run the performance monitoring function periodicallywhile True: monitor_performance() time.sleep(60)
Regular Updates: Keep the model and app updated with the latest features and improvements, addressing any bugs or vulnerabilities promptly.
User Feedback: Collect and analyze user feedback to make iterative improvements, ensuring the app remains relevant and effective.
By following these steps, you can successfully integrate LLMs into your applications, enhancing their functionality and providing a superior user experience.
Next, we'll explore the challenges and considerations involved in working with LLMs.
Challenges and Considerations
Integrating Large Language Models (LLMs) into applications brings significant benefits, but it also presents several challenges and considerations. Addressing these effectively is crucial for the successful deployment and operation of LLM-powered apps.
This section covers the ethical and privacy concerns, technical challenges, and user experience considerations that developers must navigate.
Ethical and Privacy Concerns
As powerful as LLMs are, their deployment must be handled with a keen awareness of ethical and privacy issues.
Addressing data privacy and security issues:
Data Protection: Achieve secure processing and storage of user data. To protect sensitive data, this entails putting access limits, encryption, and frequent security assessments in place.
Compliance: Adhere to data protection regulations such as GDPR, CCPA, and other relevant laws. This includes obtaining user consent for data collection and providing options for data deletion upon request.
Ensuring ethical use of LLMs:
Bias Mitigation: Actively work to identify and mitigate biases in the training data and the model’s outputs. This helps prevent the perpetuation of harmful stereotypes or unfair treatment of certain user groups.
Transparency: Maintain transparency about how the LLM is used within the app. Inform users about the data being collected, how it is used, and the role of the AI in generating responses or making decisions.
Technical Challenges
The technical aspects of integrating LLMs pose significant challenges, especially concerning data and computational requirements.
Handling large datasets and computational requirements:
Data Management: Managing and processing large datasets required for training LLMs can be complex and resource-intensive. Effective data management practices, including data cleaning, preprocessing, and storage solutions, are essential.
Computational Resources: Training and deploying LLMs require substantial computational power. Leveraging cloud-based solutions or investing in high-performance hardware can help meet these demands.
Ensuring scalability and performance:
Scalability: Design the system to scale efficiently with increasing user loads and data volumes. This involves using scalable cloud infrastructure, load balancing, and efficient data handling practices.
Performance Optimization: Continuously optimize the performance of the LLM and the application. This includes reducing latency, improving response times, and ensuring that the system can handle peak loads without degradation in performance.
User Experience
The success of an LLM-integrated app heavily relies on providing a seamless and intuitive user experience.
Designing intuitive and user-friendly interfaces:
User-Centric Design: Focus on creating interfaces that are easy to navigate and understand. This involves using clear language, intuitive navigation, and providing helpful prompts or suggestions.
Accessibility: Ensure that the app is accessible to all users, including those with disabilities. Implement features like text-to-speech, screen reader compatibility, and adjustable text sizes.
Managing user expectations and feedback:
Expectation Management: Clearly communicate the capabilities and limitations of the LLM to users. Setting realistic expectations helps prevent frustration and enhances user satisfaction.
Feedback Mechanisms: Implement robust feedback mechanisms to gather user insights and suggestions. Regularly analyze this feedback to make iterative improvements to the app, ensuring it meets user needs and preferences.
Understanding and addressing these challenges is crucial for leveraging the full potential of LLMs while maintaining high ethical standards, technical robustness, and a positive user experience.
In the next section, we will delve into real-world case studies and examples of successful LLM implementations.
Case Studies and Examples
Understanding real-world implementations and future trends of Large Language Models (LLMs) can provide valuable insights and inspiration for leveraging these powerful tools in app development.
This section highlights successful implementations, including those from RAGA AI, and explores innovative uses and future trends.
Successful Implementations
LLMs have been successfully integrated into various applications across different industries, showcasing their versatility and effectiveness.
Real-world examples of apps using LLMs:
Grammarly: Uses LLMs to enhance its grammar and style correction capabilities, helping users write more clearly and effectively.
Netflix: Utilizes sentiment analysis powered by LLMs to understand viewer reactions and refine content recommendations.
Duolingo: Implements LLMs to provide personalized language learning experiences, adapting lessons to the user's proficiency level.
Shopify: Uses LLMs to power its customer support chatbots, improving response times and user satisfaction.
Case studies from RAGA AI:
Safeguarding Enterprise LLM Applications: RAGA AI implemented guardrails to enhance the reliability of an enterprise LLM application, significantly reducing error rates and improving user trust.
Evaluating and Monitoring LLM Applications: A financial services firm used RAGA AI to monitor and evaluate their LLM application, leading to a 30% increase in efficiency and a 20% reduction in operational costs.
Advancing Geospatial Semantic Segmentation: RAGA AI's automated testing platform improved the accuracy of a geospatial semantic segmentation model, enabling faster and more reliable data processing for a government agency.
Key takeaways and lessons learned:
User-Centric Design: Focus on designing applications that meet specific user needs and enhance user experience.
Continuous Improvement: Regularly update and fine-tune the LLM to keep up with evolving user expectations and new data.
Scalability: Ensure that the infrastructure can scale efficiently to handle increased user loads and data volumes.
Ethical Considerations: Actively work to identify and mitigate biases in the LLM to ensure fair and ethical use.
Innovative Uses and Future Trends
LLMs are being used in innovative ways, pushing the boundaries of what is possible with AI in app development.
.
Cutting-edge applications and developments:
Healthcare: LLMs are used to analyze patient data, assist in diagnostics, and provide personalized health recommendations.
Finance: Financial institutions use LLMs to detect fraudulent activities, automate customer service, and provide investment advice.
Education: Educational platforms leverage LLMs to create interactive tutoring systems, personalized learning plans, and automated grading systems.
Creative Arts: LLMs are being used to generate music, art, and literature, assisting artists in the creative process.
Predictions for the future of LLMs in app development:
Increased Personalization: LLMs will enable more personalized user experiences, tailoring content and interactions to individual preferences and behaviours.
Enhanced Interactivity: Future apps will feature more interactive and conversational interfaces, making user interactions more natural and engaging.
Cross-Industry Integration: The use of LLMs will expand across various industries, from legal to entertainment, offering innovative solutions to complex problems.
Ethical AI: There will be a stronger focus on developing ethical AI practices, ensuring that LLMs are used responsibly and fairly.
By examining these successful implementations and exploring future trends, developers can gain valuable insights into the potential of LLMs and how to integrate them into their applications effectively.
Additional Resources
For those looking to delve deeper into the world of Large Language Models (LLMs) and their applications in app development, there are numerous resources available.
This section provides recommended readings, tools, libraries, and communities for support.
Recommended Reading and Tutorials
Enhance your understanding of LLMs with these valuable books, articles, and online courses.
Books:
"Deep Learning" by Ian Goodfellow, Yoshua Bengio, and Aaron Courville: A comprehensive resource on deep learning principles, including the foundations of LLMs. Get the book
"Natural Language Processing with PyTorch" by Delip Rao and Brian McMahan: A practical guide to NLP, with applications of LLMs using PyTorch. Get the book
Articles:
"Attention is All You Need" by Vaswani et al.: The seminal paper introducing the transformer architecture that underpins modern LLMs. Read it here
"The Illustrated Transformer" by Jay Alammar: A visual and intuitive guide to understanding the transformer model. Read it here
Online Courses:
"Deep Learning Specialization" by Andrew Ng on Coursera: A series of courses covering the basics of deep learning, including NLP applications. Enroll here
"Natural Language Processing with Deep Learning" by Stanford University: A course focusing on advanced NLP techniques and LLMs. Access the course
Tools and Libraries
Utilize these essential tools and libraries to work effectively with LLMs.
Transformers by Hugging Face: A library providing thousands of pre-trained models for NLP tasks, including GPT-4 and other LLMs. Explore Transformers
TensorFlow: An open-source platform for machine learning, offering tools for building and training LLMs. Visit TensorFlow
PyTorch: A flexible deep learning framework used for developing and training LLMs. Visit PyTorch
OpenAI API: Access powerful models like GPT-4 via a simple API, enabling easy integration into applications. Learn more
Communities and Support
Engage with online forums and communities to seek support, share knowledge, and stay updated with the latest developments in LLMs.
Reddit - r/MachineLearning: A popular community for discussing machine learning topics, sharing research, and asking questions. Join the conversation
Hugging Face Forums: A supportive community focused on the Hugging Face ecosystem, including transformers and other NLP tools. Join the forums
AI Alignment Forum: A platform dedicated to discussing AI alignment and safety, relevant for ethical considerations in LLM development. Participate here
Stack Overflow: A valuable resource for developers to ask questions and share solutions related to LLMs and other programming topics. Ask a question
Conclusion
Large Language Models (LLMs) have revolutionized app development, enhancing NLP, chatbots, content creation, and data insights. Despite integration challenges, their benefits in scalability, performance, and user experience are immense. As technology advances, LLMs will drive further innovation and personalization. Developers should continue exploring these models to create more intuitive and impactful applications with a promising future across various industries.
RAGA AI provides tools for developing and deploying LLM-powered applications, ensuring model accuracy, ethical usage, and streamlined integration. By leveraging RAGA AI's capabilities, developers can enhance model accuracy, ensure ethical usage, and streamline integration processes. This results in more robust, scalable, and user-friendly applications tailored to meet diverse industry needs.
Sign up for RAGA and get your LLM application ready 3x faster!
Large language models application through production has become a game-changer in the tech world. These advanced models, like GPT-4 and BERT, have transformed how developers approach app creation, offering new possibilities and efficiencies.
Integrating large language models into your apps can significantly enhance user experience and functionality. Imagine building a chatbot that understands nuanced customer queries or an app that generates content tailored to individual preferences. The Large language models application through production are vast and continually evolving, making this an exciting frontier for developers. Now, let's dive deeper into understanding large language models and their remarkable capabilities.
Overview of Large Language Models (LLMs)
Large Language Models (LLMs) have revolutionized the field of artificial intelligence. These models, like GPT-4 and BERT, are designed to understand and generate human-like text by predicting the next word in a sequence. Their ability to process and produce text with remarkable coherence makes them invaluable tools for a wide range of applications.
Definition
LLMs are advanced AI systems trained on vast datasets to predict and generate text. These models utilize deep learning techniques to analyze and understand the structure of language, allowing them to produce coherent and contextually relevant text.
Historical development and evolution:
Early Models: The journey began with simpler models like n-grams and statistical methods that could only handle limited contexts.
ntroduction of Neural Networks: The use of neural networks marked a significant improvement, allowing models to understand more complex patterns in language.
Transformer Architecture: The advent of transformer models, such as the Transformer by Vaswani et al., revolutionized the field, enabling more efficient processing of text and better handling of context.
Modern LLMs: Today, models like GPT-4 and Gemini represent the pinnacle of this evolution, capable of generating highly coherent and contextually relevant text across a wide range of applications.
Basic Understanding
By analyzing context and word patterns, LLMs can create responses that are contextually relevant and meaningful. They are capable of understanding complex language structures, idiomatic expressions, and the nuances of human communication, making them incredibly versatile.
Examples of popular LLMs:
GPT-4: OpenAI's GPT-4 is renowned for its capacity to produce precisely contextualized text, which makes it perfect for chatbots and other applications. GPT-4 can handle a wide range of tasks, from answering questions to writing essays, demonstrating a high level of linguistic competence.
Gemini: Created by Google DeepMind, Gemini excels in integrating various AI capabilities, including language understanding and generation. Gemini's advanced architecture allows it to perform a wide range of tasks, from conversational AI to complex problem-solving, showcasing its versatility in various applications.
Key differences and use cases:
GPT-4 vs. Gemini: While both models are highly capable, GPT-4 is particularly noted for its text generation prowess, whereas Gemini is praised for its integration of multiple AI functionalities.
Gemini: Unlike GPT-4, Gemini is primarily designed for tasks that require a deep understanding of context, making it ideal for search engines and other query-based applications.
Use Cases: GPT-4 is used for creating conversational agents and automated writing tools; Gemini is suitable for complex AI integrations and is optimal for improving search and retrieval systems.
Rise of LLMs in Application Development
The integration of LLMs into applications is rapidly becoming a standard in the tech industry. Their ability to enhance user interaction and automate complex tasks makes them highly relevant in today's digital landscape.
Growing trend of integrating LLMs into apps:
Increased Adoption: More businesses are incorporating LLMs into their apps to stay competitive and improve user experience. From startups to large enterprises, companies are leveraging LLMs to streamline operations, provide better customer support, and innovate their product offerings.
Technological Advancements: Continuous improvements in LLM technology are driving their widespread adoption across various industries. Enhanced computational power, access to larger datasets, and advancements in AI research have made LLMs more powerful and accessible than ever before.
Potential benefits and applications:
Enhanced User Interaction: LLMs can power chatbots and virtual assistants, providing users with more intuitive and responsive interactions. These models can understand and respond to user queries naturally and conversationally, improving overall user satisfaction.
Automated Content Creation: These models can generate high-quality content, from articles to social media posts, reducing the need for manual effort. Businesses can use LLMs to produce consistent and engaging content quickly, saving time and resources.
Data Analysis and Insights: LLMs can analyze large datasets to extract meaningful insights, aiding in decision-making processes. They can process and interpret complex data sets, identify patterns, and provide actionable insights, making them valuable tools for business intelligence.
Understanding the foundational aspects of LLMs and their growing importance sets the stage for exploring their practical applications in app development.
In the next section, we'll delve deeper into what makes large language models unique and how they work.
Working of Large Language Model
To appreciate the capabilities of LLMs, it's crucial to understand their underlying architecture and the training processes that enable them to perform complex tasks.
Basic architecture and training process:
Transformer Architecture: LLMs typically use transformer architecture, which relies on self-attention mechanisms to weigh the importance of different words in a sentence.
Training Process: Large volumes of text data are used to train these models, which then use the context given by the words before them to predict the word that will appear next in a sequence. For this training, billions of parameters need to be tuned using techniques like gradient descent.
Key features and functionalities:
Contextual Understanding: LLMs excel at understanding the context of words and sentences, allowing them to generate coherent and relevant text.
Text Generation: They can produce high-quality text that mimics human writing, useful for tasks like content creation and automated communication.
Versatility: LLMs are very flexible to a wide range of applications, from data analysis to customer support, since they can be tailored for certain tasks and use scenarios.
With this foundational knowledge, we can now explore how LLMs are applied in real-world scenarios and their impact on various industries.
Applications of LLMs in App Development
With a broad range of applications in app development, Large Language Models (LLMs) greatly improve the capabilities of different software solutions. From improving natural language processing to creating sophisticated chatbots and virtual assistants, LLMs are transforming how developers build and manage applications.
This section explores the diverse uses of LLMs in app development and their impact on user experience and functionality.
Natural Language Processing (NLP)
LLMs are a cornerstone of natural language processing, enabling apps to understand and generate human language with remarkable accuracy.
Text generation and completion:
Text Generation: LLMs can create coherent and contextually relevant text based on a given prompt. This is useful in applications such as automated writing tools, creative content generation, and scriptwriting.
Text Completion: These models can predict and complete sentences or paragraphs, enhancing user productivity in writing apps, email clients, and other text-heavy applications. For instance, they can help users draft emails by suggesting how to complete sentences based on the initial input.
Sentiment analysis and text classification:
Sentiment Analysis: LLMs can analyze text to determine the sentiment behind it, identifying whether the content is positive, negative, or neutral. This capability is essential for customer feedback analysis, social media monitoring, and market research.
Text Classification: They can categorize text into predefined labels, such as spam detection, topic categorization, and content moderation. This helps in organizing large volumes of text data efficiently.
Chatbots and Virtual Assistants
LLMs enhance the functionality and user experience of chatbots and virtual assistants, making them more interactive and helpful.
Enhancing user interaction and support:
Natural Conversations: LLMs enable chatbots to engage in natural, human-like conversations, improving user satisfaction and engagement. They can understand context, manage dialogue flow, and provide accurate responses.
Support Automation: These models can automate customer support tasks, answering frequently asked questions, resolving common issues, and directing users to appropriate resources without human intervention.
Examples of successful implementations:
Customer Service Bots: Companies like Amazon and Apple use LLM-powered chatbots to provide instant customer support, reducing wait times and improving service quality.
Virtual Assistants: Assistants like Google Assistant and Apple's Siri leverage LLMs to perform tasks such as setting reminders, providing weather updates, and answering queries, showcasing the practical applications of these models in daily life.
Content Creation and Management
LLMs streamline content creation and management processes, making it easier to produce and organize large volumes of content.
Automated content generation:
Content Creation: LLMs can generate articles, blog posts, social media updates, and more tailored to specific topics and styles. This capability helps businesses maintain a consistent content output without requiring extensive manual effort.
Personalized Content: They can create personalized content for different user segments, enhancing engagement by catering to individual preferences and needs.
Managing and curating content at scale:
Content Management: LLMs can assist in managing large content repositories by tagging, categorizing, and summarizing content, making it easier to retrieve and utilize.
Content Curation: These models can curate relevant content from various sources, providing users with up-to-date and personalized information feeds and enhancing the value of content platforms.
Data Analysis and Insights
LLMs offer powerful tools for analyzing data and extracting actionable insights, automating many of the processes traditionally handled by data scientists.
Extracting meaningful insights from data:
Data Interpretation: LLMs can analyze complex datasets to identify trends, patterns, and anomalies, helping businesses make informed decisions based on comprehensive data analysis.
Report Generation: Their ability to produce comprehensive reports and summaries from unprocessed data facilitates stakeholders' comprehension and application of the findings.
Automating data processing tasks:
Data Cleaning and Preparation: Time-consuming chores of cleaning and preparing data for analysis can be automated by LLMs, which also lowers the risk of error.
Automated Analytics: These models may analyze data in real time and deliver immediate insights, allowing businesses to respond swiftly to changing circumstances and make more effective decisions based on data.
By leveraging the capabilities of LLMs, developers can create more intelligent, responsive, and efficient applications that enhance user experience and drive business value.
Next, we'll explore the steps to integrate these powerful models into your app development process.
Steps to Integrate LLMs into Apps
Integrating Large Language Models (LLMs) into your applications can significantly enhance their capabilities and user experience. To deploy and manage the software, among other crucial processes, the process must first identify use cases.
The necessary actions to guarantee a seamless and successful integration of LLMs into your applications are described in this section.
Identifying Use Cases and Requirements
Before integrating an LLM, it's crucial to understand the specific needs of your application and define clear objectives and goals.
Understanding the specific needs of the App:
User Requirements: Determine what users need from the app and how an LLM can fulfill those needs. This involves analyzing user interactions, feedback, and common tasks that the app should support.
Functional Requirements: Identify the specific functionalities that the LLM will enhance or automate, such as natural language understanding, text generation, or sentiment analysis.
Defining clear objectives and goals:
Objectives: Clearly define your goals and assign metrics for achieving the LLM integration. For example, improving customer service response times or automating content generation.
Goals: Establish long-term goals that align with your business strategy, such as increasing user engagement, reducing operational costs, or expanding service offerings.
Choosing the Right LLM
Selecting the appropriate LLM is critical for achieving the desired outcomes and ensuring compatibility with your app.
Evaluating different models based on the use case:
Model Comparison: Compare various LLMs like GPT-4, Gemini, and others to understand their strengths and limitations. Consider how well each model addresses your specific use cases.
Performance Metrics: Evaluate models based on performance metrics such as accuracy, correctness, response time, and ability to handle specific tasks.
Considering factors like performance, cost, and compatibility:
Performance: Assess the performance of the LLM in real-world scenarios relevant to your application. Ensure it can handle the expected workload efficiently.
Cost: Consider the cost implications of using different LLMs, including licensing fees, computational resources, and ongoing maintenance.
Compatibility: Make sure the LLM you select can be easily incorporated into your development environment and is compatible with the technology stack you currently have.
Setting Up the Development Environment
A well-prepared development environment is essential for integrating and testing LLMs effectively.
Tools and frameworks for integration:
Development Tools: Utilize tools like TensorFlow, PyTorch, or specific APIs provided by LLM developers to integrate the models into your app.
TensorFlow Example
import tensorflow as tf
# Set up the computational environment
tf.config.set_visible_devices([tf.config.list_physical_devices('GPU')[0]])
PyTorch Example
import torch
import torch.nn as nn
# Set up the computational environment
device = torch.device("cuda:0" if torch.cuda.is_available() else "cpu")
Frameworks: Consider using frameworks like Hugging Face's Transformers or LangChain to simplify the integration process and manage model interactions.
Preparing the necessary infrastructure:
Computational Resources: Ensure you have adequate computational resources, such as GPUs or cloud-based services, to handle the demands of training and running LLMs.
Data Management: Set up robust data management practices to handle the large volumes of data required for training and fine-tuning the models.
Training and Fine-Tuning the Model
Customizing the LLM to suit your specific needs involves training and fine-tuning the model.
Customizing the Model for specific tasks:
Task-Specific Training: Train the model on datasets relevant to your application to improve its performance in specific tasks like customer support or content generation.
Domain Adaptation: Adapt the model to understand and generate text specific to your industry or domain, enhancing its relevance and accuracy.
Techniques for effective training and fine-tuning:
Supervised Learning: Use supervised learning techniques to train the model on labeled datasets, ensuring it learns to perform tasks accurately.
Supervised Learning Example (TensorFlow)
import tensorflow as tf
from tensorflow.keras.preprocessing.text import Tokenizer
from tensorflow.keras.preprocessing.sequence import pad_sequences
# Load the dataset
train_texts, train_labels = ...
# Tokenize the text data
tokenizer = Tokenizer(num_words=5000)
train_sequences = tokenizer.texts_to_sequences(train_texts)
# Pad the sequences
max_length = 128
padded_train_sequences = pad_sequences(train_sequences, maxlen=max_length)
# Define the model
model = tf.keras.Sequential([
tf.keras.layers.Embedding(input_dim=5000, output_dim=128, input_length=max_length),
tf.keras.layers.LSTM(64, return_sequences=True),
tf.keras.layers.Dense(1, activation='sigmoid')
])
# Compile the model
model.compile(optimizer='adam', loss='binary_crossentropy', metrics=['accuracy'])
# Train the model
model.fit(padded_train_sequences, train_labels, epochs=10, batch_size=32)
Fine-Tuning Example (PyTorch)
import torch
import torch.nn as nn
import torch.optim as optim
# Load the pre-trained model
model = torch.load('pretrained_model.pt')
# Define the fine-tuning model
fine_tuning_model = nn.Sequential(
nn.Linear(128, 64),
nn.ReLU(),
nn.Linear(64, 1),
nn.Sigmoid()
)
# Define the optimizer and loss fhttps://docs.google.com/document/d/1SFJpqYWCqufiQ7HpkOxTVF5gEI0uIFLjrtZjf4hIRvg/edit#heading=h.vwj13hlpd57function
optimizer = optim.Adam(fine_tuning_model.parameters(), lr=0.001)
criterion = nn.BCELoss()
# Train the fine-tuning model
for epoch in range(10):
for batch in train_loader:
inputs, labels = batch
outputs = fine_tuning_model(inputs)
loss = criterion(outputs, labels)
optimizer.zero_grad()
loss.backward()
optimizer.step()
Hyperparameter Tuning: Optimize hyperparameters to improve model performance, such as adjusting learning rates, batch sizes, and training epochs.
Implementing the Model into the App
The implementation phase involves coding and integrating the LLM into your app, followed by thorough testing and debugging.
Coding and integration processes:
API Integration: Implement the LLM using APIs, ensuring smooth communication between the model and your application.
Example of API Integration:
import requests
# Define the API endpoint
api_endpoint = 'https://api.example.com/llm'
# Define the request payload
payload = {'text': 'Hello, world'}
# Send the request
response = requests.post(api_endpoint, json=payload)
# Process the response
if response.status_code == 200:
response_data = response.json()
print(response_data)
Modular Design: Use a modular design approach to integrate the LLM, allowing for easier updates and maintenance.
Example of Modular Design:
class LLMIntegration:
def __init__(self):
self.llm = None
def load_llm(self):
self.llm = torch.load('pretrained_model.pt')
def generate_text(self, prompt):
if self.llm is None:
self.load_llm()
return self.llm.generate_text(prompt)
# Create an instance of the LLMIntegration class
llm_integration = LLMIntegration()
# Generate text using the LLM
prompt = 'Write a short story about a cat'
text = llm_integration.generate_text(prompt)
print(text)
Testing and debugging:
Unit Testing: Conduct unit tests to verify that individual components of the integration work as expected.
End-to-end Testing: Perform end-to-end testing to ensure the entire application functions correctly with the integrated LLM.
Debugging: Identify and fix any issues that arise during testing, ensuring the model operates smoothly within the app.
Deployment and Maintenance
Deploying the app in a production environment and maintaining it ensures long-term success and optimal performance.
Deploying the App in a production environment:
Scalability: Ensure the app can scale to handle increased usage and data volumes, utilizing cloud services or on-premises solutions as needed.
Scalability Example (Docker)
FROM python:3.9-slim
# Install required libraries
RUN pip install torch torchvision
# Copy the application code
COPY app.py .
# Define the environment variables
ENV LLM_MODEL=pretrained_model.pt
# Define the command to run the application
CMD ["python", "app.py"]
Security: Implement robust security measures to protect user data and ensure compliance with relevant regulations.
Ongoing monitoring and updates:
Performance Monitoring: Continuously monitor the performance of the LLM within the app, using tools to track metrics and identify potential issues.
Performance Monitoring Example (Python)
import logging
import time
# Set up the logging configuration
logging.basicConfig(level=logging.INFO)
# Define the performance monitoring function
def monitor_performance():
# Log the performance metrics
logging.info(f"Model accuracy: {model.accuracy:.2f}")
logging.info(f"Model latency: {model.latency:.2f} ms")
# Run the performance monitoring function periodically
while True:
monitor_performance()
time.sleep(60
# Run the performance monitoring function periodicallywhile True: monitor_performance() time.sleep(60)
Regular Updates: Keep the model and app updated with the latest features and improvements, addressing any bugs or vulnerabilities promptly.
User Feedback: Collect and analyze user feedback to make iterative improvements, ensuring the app remains relevant and effective.
By following these steps, you can successfully integrate LLMs into your applications, enhancing their functionality and providing a superior user experience.
Next, we'll explore the challenges and considerations involved in working with LLMs.
Challenges and Considerations
Integrating Large Language Models (LLMs) into applications brings significant benefits, but it also presents several challenges and considerations. Addressing these effectively is crucial for the successful deployment and operation of LLM-powered apps.
This section covers the ethical and privacy concerns, technical challenges, and user experience considerations that developers must navigate.
Ethical and Privacy Concerns
As powerful as LLMs are, their deployment must be handled with a keen awareness of ethical and privacy issues.
Addressing data privacy and security issues:
Data Protection: Achieve secure processing and storage of user data. To protect sensitive data, this entails putting access limits, encryption, and frequent security assessments in place.
Compliance: Adhere to data protection regulations such as GDPR, CCPA, and other relevant laws. This includes obtaining user consent for data collection and providing options for data deletion upon request.
Ensuring ethical use of LLMs:
Bias Mitigation: Actively work to identify and mitigate biases in the training data and the model’s outputs. This helps prevent the perpetuation of harmful stereotypes or unfair treatment of certain user groups.
Transparency: Maintain transparency about how the LLM is used within the app. Inform users about the data being collected, how it is used, and the role of the AI in generating responses or making decisions.
Technical Challenges
The technical aspects of integrating LLMs pose significant challenges, especially concerning data and computational requirements.
Handling large datasets and computational requirements:
Data Management: Managing and processing large datasets required for training LLMs can be complex and resource-intensive. Effective data management practices, including data cleaning, preprocessing, and storage solutions, are essential.
Computational Resources: Training and deploying LLMs require substantial computational power. Leveraging cloud-based solutions or investing in high-performance hardware can help meet these demands.
Ensuring scalability and performance:
Scalability: Design the system to scale efficiently with increasing user loads and data volumes. This involves using scalable cloud infrastructure, load balancing, and efficient data handling practices.
Performance Optimization: Continuously optimize the performance of the LLM and the application. This includes reducing latency, improving response times, and ensuring that the system can handle peak loads without degradation in performance.
User Experience
The success of an LLM-integrated app heavily relies on providing a seamless and intuitive user experience.
Designing intuitive and user-friendly interfaces:
User-Centric Design: Focus on creating interfaces that are easy to navigate and understand. This involves using clear language, intuitive navigation, and providing helpful prompts or suggestions.
Accessibility: Ensure that the app is accessible to all users, including those with disabilities. Implement features like text-to-speech, screen reader compatibility, and adjustable text sizes.
Managing user expectations and feedback:
Expectation Management: Clearly communicate the capabilities and limitations of the LLM to users. Setting realistic expectations helps prevent frustration and enhances user satisfaction.
Feedback Mechanisms: Implement robust feedback mechanisms to gather user insights and suggestions. Regularly analyze this feedback to make iterative improvements to the app, ensuring it meets user needs and preferences.
Understanding and addressing these challenges is crucial for leveraging the full potential of LLMs while maintaining high ethical standards, technical robustness, and a positive user experience.
In the next section, we will delve into real-world case studies and examples of successful LLM implementations.
Case Studies and Examples
Understanding real-world implementations and future trends of Large Language Models (LLMs) can provide valuable insights and inspiration for leveraging these powerful tools in app development.
This section highlights successful implementations, including those from RAGA AI, and explores innovative uses and future trends.
Successful Implementations
LLMs have been successfully integrated into various applications across different industries, showcasing their versatility and effectiveness.
Real-world examples of apps using LLMs:
Grammarly: Uses LLMs to enhance its grammar and style correction capabilities, helping users write more clearly and effectively.
Netflix: Utilizes sentiment analysis powered by LLMs to understand viewer reactions and refine content recommendations.
Duolingo: Implements LLMs to provide personalized language learning experiences, adapting lessons to the user's proficiency level.
Shopify: Uses LLMs to power its customer support chatbots, improving response times and user satisfaction.
Case studies from RAGA AI:
Safeguarding Enterprise LLM Applications: RAGA AI implemented guardrails to enhance the reliability of an enterprise LLM application, significantly reducing error rates and improving user trust.
Evaluating and Monitoring LLM Applications: A financial services firm used RAGA AI to monitor and evaluate their LLM application, leading to a 30% increase in efficiency and a 20% reduction in operational costs.
Advancing Geospatial Semantic Segmentation: RAGA AI's automated testing platform improved the accuracy of a geospatial semantic segmentation model, enabling faster and more reliable data processing for a government agency.
Key takeaways and lessons learned:
User-Centric Design: Focus on designing applications that meet specific user needs and enhance user experience.
Continuous Improvement: Regularly update and fine-tune the LLM to keep up with evolving user expectations and new data.
Scalability: Ensure that the infrastructure can scale efficiently to handle increased user loads and data volumes.
Ethical Considerations: Actively work to identify and mitigate biases in the LLM to ensure fair and ethical use.
Innovative Uses and Future Trends
LLMs are being used in innovative ways, pushing the boundaries of what is possible with AI in app development.
.
Cutting-edge applications and developments:
Healthcare: LLMs are used to analyze patient data, assist in diagnostics, and provide personalized health recommendations.
Finance: Financial institutions use LLMs to detect fraudulent activities, automate customer service, and provide investment advice.
Education: Educational platforms leverage LLMs to create interactive tutoring systems, personalized learning plans, and automated grading systems.
Creative Arts: LLMs are being used to generate music, art, and literature, assisting artists in the creative process.
Predictions for the future of LLMs in app development:
Increased Personalization: LLMs will enable more personalized user experiences, tailoring content and interactions to individual preferences and behaviours.
Enhanced Interactivity: Future apps will feature more interactive and conversational interfaces, making user interactions more natural and engaging.
Cross-Industry Integration: The use of LLMs will expand across various industries, from legal to entertainment, offering innovative solutions to complex problems.
Ethical AI: There will be a stronger focus on developing ethical AI practices, ensuring that LLMs are used responsibly and fairly.
By examining these successful implementations and exploring future trends, developers can gain valuable insights into the potential of LLMs and how to integrate them into their applications effectively.
Additional Resources
For those looking to delve deeper into the world of Large Language Models (LLMs) and their applications in app development, there are numerous resources available.
This section provides recommended readings, tools, libraries, and communities for support.
Recommended Reading and Tutorials
Enhance your understanding of LLMs with these valuable books, articles, and online courses.
Books:
"Deep Learning" by Ian Goodfellow, Yoshua Bengio, and Aaron Courville: A comprehensive resource on deep learning principles, including the foundations of LLMs. Get the book
"Natural Language Processing with PyTorch" by Delip Rao and Brian McMahan: A practical guide to NLP, with applications of LLMs using PyTorch. Get the book
Articles:
"Attention is All You Need" by Vaswani et al.: The seminal paper introducing the transformer architecture that underpins modern LLMs. Read it here
"The Illustrated Transformer" by Jay Alammar: A visual and intuitive guide to understanding the transformer model. Read it here
Online Courses:
"Deep Learning Specialization" by Andrew Ng on Coursera: A series of courses covering the basics of deep learning, including NLP applications. Enroll here
"Natural Language Processing with Deep Learning" by Stanford University: A course focusing on advanced NLP techniques and LLMs. Access the course
Tools and Libraries
Utilize these essential tools and libraries to work effectively with LLMs.
Transformers by Hugging Face: A library providing thousands of pre-trained models for NLP tasks, including GPT-4 and other LLMs. Explore Transformers
TensorFlow: An open-source platform for machine learning, offering tools for building and training LLMs. Visit TensorFlow
PyTorch: A flexible deep learning framework used for developing and training LLMs. Visit PyTorch
OpenAI API: Access powerful models like GPT-4 via a simple API, enabling easy integration into applications. Learn more
Communities and Support
Engage with online forums and communities to seek support, share knowledge, and stay updated with the latest developments in LLMs.
Reddit - r/MachineLearning: A popular community for discussing machine learning topics, sharing research, and asking questions. Join the conversation
Hugging Face Forums: A supportive community focused on the Hugging Face ecosystem, including transformers and other NLP tools. Join the forums
AI Alignment Forum: A platform dedicated to discussing AI alignment and safety, relevant for ethical considerations in LLM development. Participate here
Stack Overflow: A valuable resource for developers to ask questions and share solutions related to LLMs and other programming topics. Ask a question
Conclusion
Large Language Models (LLMs) have revolutionized app development, enhancing NLP, chatbots, content creation, and data insights. Despite integration challenges, their benefits in scalability, performance, and user experience are immense. As technology advances, LLMs will drive further innovation and personalization. Developers should continue exploring these models to create more intuitive and impactful applications with a promising future across various industries.
RAGA AI provides tools for developing and deploying LLM-powered applications, ensuring model accuracy, ethical usage, and streamlined integration. By leveraging RAGA AI's capabilities, developers can enhance model accuracy, ensure ethical usage, and streamline integration processes. This results in more robust, scalable, and user-friendly applications tailored to meet diverse industry needs.
Sign up for RAGA and get your LLM application ready 3x faster!
Large language models application through production has become a game-changer in the tech world. These advanced models, like GPT-4 and BERT, have transformed how developers approach app creation, offering new possibilities and efficiencies.
Integrating large language models into your apps can significantly enhance user experience and functionality. Imagine building a chatbot that understands nuanced customer queries or an app that generates content tailored to individual preferences. The Large language models application through production are vast and continually evolving, making this an exciting frontier for developers. Now, let's dive deeper into understanding large language models and their remarkable capabilities.
Overview of Large Language Models (LLMs)
Large Language Models (LLMs) have revolutionized the field of artificial intelligence. These models, like GPT-4 and BERT, are designed to understand and generate human-like text by predicting the next word in a sequence. Their ability to process and produce text with remarkable coherence makes them invaluable tools for a wide range of applications.
Definition
LLMs are advanced AI systems trained on vast datasets to predict and generate text. These models utilize deep learning techniques to analyze and understand the structure of language, allowing them to produce coherent and contextually relevant text.
Historical development and evolution:
Early Models: The journey began with simpler models like n-grams and statistical methods that could only handle limited contexts.
ntroduction of Neural Networks: The use of neural networks marked a significant improvement, allowing models to understand more complex patterns in language.
Transformer Architecture: The advent of transformer models, such as the Transformer by Vaswani et al., revolutionized the field, enabling more efficient processing of text and better handling of context.
Modern LLMs: Today, models like GPT-4 and Gemini represent the pinnacle of this evolution, capable of generating highly coherent and contextually relevant text across a wide range of applications.
Basic Understanding
By analyzing context and word patterns, LLMs can create responses that are contextually relevant and meaningful. They are capable of understanding complex language structures, idiomatic expressions, and the nuances of human communication, making them incredibly versatile.
Examples of popular LLMs:
GPT-4: OpenAI's GPT-4 is renowned for its capacity to produce precisely contextualized text, which makes it perfect for chatbots and other applications. GPT-4 can handle a wide range of tasks, from answering questions to writing essays, demonstrating a high level of linguistic competence.
Gemini: Created by Google DeepMind, Gemini excels in integrating various AI capabilities, including language understanding and generation. Gemini's advanced architecture allows it to perform a wide range of tasks, from conversational AI to complex problem-solving, showcasing its versatility in various applications.
Key differences and use cases:
GPT-4 vs. Gemini: While both models are highly capable, GPT-4 is particularly noted for its text generation prowess, whereas Gemini is praised for its integration of multiple AI functionalities.
Gemini: Unlike GPT-4, Gemini is primarily designed for tasks that require a deep understanding of context, making it ideal for search engines and other query-based applications.
Use Cases: GPT-4 is used for creating conversational agents and automated writing tools; Gemini is suitable for complex AI integrations and is optimal for improving search and retrieval systems.
Rise of LLMs in Application Development
The integration of LLMs into applications is rapidly becoming a standard in the tech industry. Their ability to enhance user interaction and automate complex tasks makes them highly relevant in today's digital landscape.
Growing trend of integrating LLMs into apps:
Increased Adoption: More businesses are incorporating LLMs into their apps to stay competitive and improve user experience. From startups to large enterprises, companies are leveraging LLMs to streamline operations, provide better customer support, and innovate their product offerings.
Technological Advancements: Continuous improvements in LLM technology are driving their widespread adoption across various industries. Enhanced computational power, access to larger datasets, and advancements in AI research have made LLMs more powerful and accessible than ever before.
Potential benefits and applications:
Enhanced User Interaction: LLMs can power chatbots and virtual assistants, providing users with more intuitive and responsive interactions. These models can understand and respond to user queries naturally and conversationally, improving overall user satisfaction.
Automated Content Creation: These models can generate high-quality content, from articles to social media posts, reducing the need for manual effort. Businesses can use LLMs to produce consistent and engaging content quickly, saving time and resources.
Data Analysis and Insights: LLMs can analyze large datasets to extract meaningful insights, aiding in decision-making processes. They can process and interpret complex data sets, identify patterns, and provide actionable insights, making them valuable tools for business intelligence.
Understanding the foundational aspects of LLMs and their growing importance sets the stage for exploring their practical applications in app development.
In the next section, we'll delve deeper into what makes large language models unique and how they work.
Working of Large Language Model
To appreciate the capabilities of LLMs, it's crucial to understand their underlying architecture and the training processes that enable them to perform complex tasks.
Basic architecture and training process:
Transformer Architecture: LLMs typically use transformer architecture, which relies on self-attention mechanisms to weigh the importance of different words in a sentence.
Training Process: Large volumes of text data are used to train these models, which then use the context given by the words before them to predict the word that will appear next in a sequence. For this training, billions of parameters need to be tuned using techniques like gradient descent.
Key features and functionalities:
Contextual Understanding: LLMs excel at understanding the context of words and sentences, allowing them to generate coherent and relevant text.
Text Generation: They can produce high-quality text that mimics human writing, useful for tasks like content creation and automated communication.
Versatility: LLMs are very flexible to a wide range of applications, from data analysis to customer support, since they can be tailored for certain tasks and use scenarios.
With this foundational knowledge, we can now explore how LLMs are applied in real-world scenarios and their impact on various industries.
Applications of LLMs in App Development
With a broad range of applications in app development, Large Language Models (LLMs) greatly improve the capabilities of different software solutions. From improving natural language processing to creating sophisticated chatbots and virtual assistants, LLMs are transforming how developers build and manage applications.
This section explores the diverse uses of LLMs in app development and their impact on user experience and functionality.
Natural Language Processing (NLP)
LLMs are a cornerstone of natural language processing, enabling apps to understand and generate human language with remarkable accuracy.
Text generation and completion:
Text Generation: LLMs can create coherent and contextually relevant text based on a given prompt. This is useful in applications such as automated writing tools, creative content generation, and scriptwriting.
Text Completion: These models can predict and complete sentences or paragraphs, enhancing user productivity in writing apps, email clients, and other text-heavy applications. For instance, they can help users draft emails by suggesting how to complete sentences based on the initial input.
Sentiment analysis and text classification:
Sentiment Analysis: LLMs can analyze text to determine the sentiment behind it, identifying whether the content is positive, negative, or neutral. This capability is essential for customer feedback analysis, social media monitoring, and market research.
Text Classification: They can categorize text into predefined labels, such as spam detection, topic categorization, and content moderation. This helps in organizing large volumes of text data efficiently.
Chatbots and Virtual Assistants
LLMs enhance the functionality and user experience of chatbots and virtual assistants, making them more interactive and helpful.
Enhancing user interaction and support:
Natural Conversations: LLMs enable chatbots to engage in natural, human-like conversations, improving user satisfaction and engagement. They can understand context, manage dialogue flow, and provide accurate responses.
Support Automation: These models can automate customer support tasks, answering frequently asked questions, resolving common issues, and directing users to appropriate resources without human intervention.
Examples of successful implementations:
Customer Service Bots: Companies like Amazon and Apple use LLM-powered chatbots to provide instant customer support, reducing wait times and improving service quality.
Virtual Assistants: Assistants like Google Assistant and Apple's Siri leverage LLMs to perform tasks such as setting reminders, providing weather updates, and answering queries, showcasing the practical applications of these models in daily life.
Content Creation and Management
LLMs streamline content creation and management processes, making it easier to produce and organize large volumes of content.
Automated content generation:
Content Creation: LLMs can generate articles, blog posts, social media updates, and more tailored to specific topics and styles. This capability helps businesses maintain a consistent content output without requiring extensive manual effort.
Personalized Content: They can create personalized content for different user segments, enhancing engagement by catering to individual preferences and needs.
Managing and curating content at scale:
Content Management: LLMs can assist in managing large content repositories by tagging, categorizing, and summarizing content, making it easier to retrieve and utilize.
Content Curation: These models can curate relevant content from various sources, providing users with up-to-date and personalized information feeds and enhancing the value of content platforms.
Data Analysis and Insights
LLMs offer powerful tools for analyzing data and extracting actionable insights, automating many of the processes traditionally handled by data scientists.
Extracting meaningful insights from data:
Data Interpretation: LLMs can analyze complex datasets to identify trends, patterns, and anomalies, helping businesses make informed decisions based on comprehensive data analysis.
Report Generation: Their ability to produce comprehensive reports and summaries from unprocessed data facilitates stakeholders' comprehension and application of the findings.
Automating data processing tasks:
Data Cleaning and Preparation: Time-consuming chores of cleaning and preparing data for analysis can be automated by LLMs, which also lowers the risk of error.
Automated Analytics: These models may analyze data in real time and deliver immediate insights, allowing businesses to respond swiftly to changing circumstances and make more effective decisions based on data.
By leveraging the capabilities of LLMs, developers can create more intelligent, responsive, and efficient applications that enhance user experience and drive business value.
Next, we'll explore the steps to integrate these powerful models into your app development process.
Steps to Integrate LLMs into Apps
Integrating Large Language Models (LLMs) into your applications can significantly enhance their capabilities and user experience. To deploy and manage the software, among other crucial processes, the process must first identify use cases.
The necessary actions to guarantee a seamless and successful integration of LLMs into your applications are described in this section.
Identifying Use Cases and Requirements
Before integrating an LLM, it's crucial to understand the specific needs of your application and define clear objectives and goals.
Understanding the specific needs of the App:
User Requirements: Determine what users need from the app and how an LLM can fulfill those needs. This involves analyzing user interactions, feedback, and common tasks that the app should support.
Functional Requirements: Identify the specific functionalities that the LLM will enhance or automate, such as natural language understanding, text generation, or sentiment analysis.
Defining clear objectives and goals:
Objectives: Clearly define your goals and assign metrics for achieving the LLM integration. For example, improving customer service response times or automating content generation.
Goals: Establish long-term goals that align with your business strategy, such as increasing user engagement, reducing operational costs, or expanding service offerings.
Choosing the Right LLM
Selecting the appropriate LLM is critical for achieving the desired outcomes and ensuring compatibility with your app.
Evaluating different models based on the use case:
Model Comparison: Compare various LLMs like GPT-4, Gemini, and others to understand their strengths and limitations. Consider how well each model addresses your specific use cases.
Performance Metrics: Evaluate models based on performance metrics such as accuracy, correctness, response time, and ability to handle specific tasks.
Considering factors like performance, cost, and compatibility:
Performance: Assess the performance of the LLM in real-world scenarios relevant to your application. Ensure it can handle the expected workload efficiently.
Cost: Consider the cost implications of using different LLMs, including licensing fees, computational resources, and ongoing maintenance.
Compatibility: Make sure the LLM you select can be easily incorporated into your development environment and is compatible with the technology stack you currently have.
Setting Up the Development Environment
A well-prepared development environment is essential for integrating and testing LLMs effectively.
Tools and frameworks for integration:
Development Tools: Utilize tools like TensorFlow, PyTorch, or specific APIs provided by LLM developers to integrate the models into your app.
TensorFlow Example
import tensorflow as tf
# Set up the computational environment
tf.config.set_visible_devices([tf.config.list_physical_devices('GPU')[0]])
PyTorch Example
import torch
import torch.nn as nn
# Set up the computational environment
device = torch.device("cuda:0" if torch.cuda.is_available() else "cpu")
Frameworks: Consider using frameworks like Hugging Face's Transformers or LangChain to simplify the integration process and manage model interactions.
Preparing the necessary infrastructure:
Computational Resources: Ensure you have adequate computational resources, such as GPUs or cloud-based services, to handle the demands of training and running LLMs.
Data Management: Set up robust data management practices to handle the large volumes of data required for training and fine-tuning the models.
Training and Fine-Tuning the Model
Customizing the LLM to suit your specific needs involves training and fine-tuning the model.
Customizing the Model for specific tasks:
Task-Specific Training: Train the model on datasets relevant to your application to improve its performance in specific tasks like customer support or content generation.
Domain Adaptation: Adapt the model to understand and generate text specific to your industry or domain, enhancing its relevance and accuracy.
Techniques for effective training and fine-tuning:
Supervised Learning: Use supervised learning techniques to train the model on labeled datasets, ensuring it learns to perform tasks accurately.
Supervised Learning Example (TensorFlow)
import tensorflow as tf
from tensorflow.keras.preprocessing.text import Tokenizer
from tensorflow.keras.preprocessing.sequence import pad_sequences
# Load the dataset
train_texts, train_labels = ...
# Tokenize the text data
tokenizer = Tokenizer(num_words=5000)
train_sequences = tokenizer.texts_to_sequences(train_texts)
# Pad the sequences
max_length = 128
padded_train_sequences = pad_sequences(train_sequences, maxlen=max_length)
# Define the model
model = tf.keras.Sequential([
tf.keras.layers.Embedding(input_dim=5000, output_dim=128, input_length=max_length),
tf.keras.layers.LSTM(64, return_sequences=True),
tf.keras.layers.Dense(1, activation='sigmoid')
])
# Compile the model
model.compile(optimizer='adam', loss='binary_crossentropy', metrics=['accuracy'])
# Train the model
model.fit(padded_train_sequences, train_labels, epochs=10, batch_size=32)
Fine-Tuning Example (PyTorch)
import torch
import torch.nn as nn
import torch.optim as optim
# Load the pre-trained model
model = torch.load('pretrained_model.pt')
# Define the fine-tuning model
fine_tuning_model = nn.Sequential(
nn.Linear(128, 64),
nn.ReLU(),
nn.Linear(64, 1),
nn.Sigmoid()
)
# Define the optimizer and loss fhttps://docs.google.com/document/d/1SFJpqYWCqufiQ7HpkOxTVF5gEI0uIFLjrtZjf4hIRvg/edit#heading=h.vwj13hlpd57function
optimizer = optim.Adam(fine_tuning_model.parameters(), lr=0.001)
criterion = nn.BCELoss()
# Train the fine-tuning model
for epoch in range(10):
for batch in train_loader:
inputs, labels = batch
outputs = fine_tuning_model(inputs)
loss = criterion(outputs, labels)
optimizer.zero_grad()
loss.backward()
optimizer.step()
Hyperparameter Tuning: Optimize hyperparameters to improve model performance, such as adjusting learning rates, batch sizes, and training epochs.
Implementing the Model into the App
The implementation phase involves coding and integrating the LLM into your app, followed by thorough testing and debugging.
Coding and integration processes:
API Integration: Implement the LLM using APIs, ensuring smooth communication between the model and your application.
Example of API Integration:
import requests
# Define the API endpoint
api_endpoint = 'https://api.example.com/llm'
# Define the request payload
payload = {'text': 'Hello, world'}
# Send the request
response = requests.post(api_endpoint, json=payload)
# Process the response
if response.status_code == 200:
response_data = response.json()
print(response_data)
Modular Design: Use a modular design approach to integrate the LLM, allowing for easier updates and maintenance.
Example of Modular Design:
class LLMIntegration:
def __init__(self):
self.llm = None
def load_llm(self):
self.llm = torch.load('pretrained_model.pt')
def generate_text(self, prompt):
if self.llm is None:
self.load_llm()
return self.llm.generate_text(prompt)
# Create an instance of the LLMIntegration class
llm_integration = LLMIntegration()
# Generate text using the LLM
prompt = 'Write a short story about a cat'
text = llm_integration.generate_text(prompt)
print(text)
Testing and debugging:
Unit Testing: Conduct unit tests to verify that individual components of the integration work as expected.
End-to-end Testing: Perform end-to-end testing to ensure the entire application functions correctly with the integrated LLM.
Debugging: Identify and fix any issues that arise during testing, ensuring the model operates smoothly within the app.
Deployment and Maintenance
Deploying the app in a production environment and maintaining it ensures long-term success and optimal performance.
Deploying the App in a production environment:
Scalability: Ensure the app can scale to handle increased usage and data volumes, utilizing cloud services or on-premises solutions as needed.
Scalability Example (Docker)
FROM python:3.9-slim
# Install required libraries
RUN pip install torch torchvision
# Copy the application code
COPY app.py .
# Define the environment variables
ENV LLM_MODEL=pretrained_model.pt
# Define the command to run the application
CMD ["python", "app.py"]
Security: Implement robust security measures to protect user data and ensure compliance with relevant regulations.
Ongoing monitoring and updates:
Performance Monitoring: Continuously monitor the performance of the LLM within the app, using tools to track metrics and identify potential issues.
Performance Monitoring Example (Python)
import logging
import time
# Set up the logging configuration
logging.basicConfig(level=logging.INFO)
# Define the performance monitoring function
def monitor_performance():
# Log the performance metrics
logging.info(f"Model accuracy: {model.accuracy:.2f}")
logging.info(f"Model latency: {model.latency:.2f} ms")
# Run the performance monitoring function periodically
while True:
monitor_performance()
time.sleep(60
# Run the performance monitoring function periodicallywhile True: monitor_performance() time.sleep(60)
Regular Updates: Keep the model and app updated with the latest features and improvements, addressing any bugs or vulnerabilities promptly.
User Feedback: Collect and analyze user feedback to make iterative improvements, ensuring the app remains relevant and effective.
By following these steps, you can successfully integrate LLMs into your applications, enhancing their functionality and providing a superior user experience.
Next, we'll explore the challenges and considerations involved in working with LLMs.
Challenges and Considerations
Integrating Large Language Models (LLMs) into applications brings significant benefits, but it also presents several challenges and considerations. Addressing these effectively is crucial for the successful deployment and operation of LLM-powered apps.
This section covers the ethical and privacy concerns, technical challenges, and user experience considerations that developers must navigate.
Ethical and Privacy Concerns
As powerful as LLMs are, their deployment must be handled with a keen awareness of ethical and privacy issues.
Addressing data privacy and security issues:
Data Protection: Achieve secure processing and storage of user data. To protect sensitive data, this entails putting access limits, encryption, and frequent security assessments in place.
Compliance: Adhere to data protection regulations such as GDPR, CCPA, and other relevant laws. This includes obtaining user consent for data collection and providing options for data deletion upon request.
Ensuring ethical use of LLMs:
Bias Mitigation: Actively work to identify and mitigate biases in the training data and the model’s outputs. This helps prevent the perpetuation of harmful stereotypes or unfair treatment of certain user groups.
Transparency: Maintain transparency about how the LLM is used within the app. Inform users about the data being collected, how it is used, and the role of the AI in generating responses or making decisions.
Technical Challenges
The technical aspects of integrating LLMs pose significant challenges, especially concerning data and computational requirements.
Handling large datasets and computational requirements:
Data Management: Managing and processing large datasets required for training LLMs can be complex and resource-intensive. Effective data management practices, including data cleaning, preprocessing, and storage solutions, are essential.
Computational Resources: Training and deploying LLMs require substantial computational power. Leveraging cloud-based solutions or investing in high-performance hardware can help meet these demands.
Ensuring scalability and performance:
Scalability: Design the system to scale efficiently with increasing user loads and data volumes. This involves using scalable cloud infrastructure, load balancing, and efficient data handling practices.
Performance Optimization: Continuously optimize the performance of the LLM and the application. This includes reducing latency, improving response times, and ensuring that the system can handle peak loads without degradation in performance.
User Experience
The success of an LLM-integrated app heavily relies on providing a seamless and intuitive user experience.
Designing intuitive and user-friendly interfaces:
User-Centric Design: Focus on creating interfaces that are easy to navigate and understand. This involves using clear language, intuitive navigation, and providing helpful prompts or suggestions.
Accessibility: Ensure that the app is accessible to all users, including those with disabilities. Implement features like text-to-speech, screen reader compatibility, and adjustable text sizes.
Managing user expectations and feedback:
Expectation Management: Clearly communicate the capabilities and limitations of the LLM to users. Setting realistic expectations helps prevent frustration and enhances user satisfaction.
Feedback Mechanisms: Implement robust feedback mechanisms to gather user insights and suggestions. Regularly analyze this feedback to make iterative improvements to the app, ensuring it meets user needs and preferences.
Understanding and addressing these challenges is crucial for leveraging the full potential of LLMs while maintaining high ethical standards, technical robustness, and a positive user experience.
In the next section, we will delve into real-world case studies and examples of successful LLM implementations.
Case Studies and Examples
Understanding real-world implementations and future trends of Large Language Models (LLMs) can provide valuable insights and inspiration for leveraging these powerful tools in app development.
This section highlights successful implementations, including those from RAGA AI, and explores innovative uses and future trends.
Successful Implementations
LLMs have been successfully integrated into various applications across different industries, showcasing their versatility and effectiveness.
Real-world examples of apps using LLMs:
Grammarly: Uses LLMs to enhance its grammar and style correction capabilities, helping users write more clearly and effectively.
Netflix: Utilizes sentiment analysis powered by LLMs to understand viewer reactions and refine content recommendations.
Duolingo: Implements LLMs to provide personalized language learning experiences, adapting lessons to the user's proficiency level.
Shopify: Uses LLMs to power its customer support chatbots, improving response times and user satisfaction.
Case studies from RAGA AI:
Safeguarding Enterprise LLM Applications: RAGA AI implemented guardrails to enhance the reliability of an enterprise LLM application, significantly reducing error rates and improving user trust.
Evaluating and Monitoring LLM Applications: A financial services firm used RAGA AI to monitor and evaluate their LLM application, leading to a 30% increase in efficiency and a 20% reduction in operational costs.
Advancing Geospatial Semantic Segmentation: RAGA AI's automated testing platform improved the accuracy of a geospatial semantic segmentation model, enabling faster and more reliable data processing for a government agency.
Key takeaways and lessons learned:
User-Centric Design: Focus on designing applications that meet specific user needs and enhance user experience.
Continuous Improvement: Regularly update and fine-tune the LLM to keep up with evolving user expectations and new data.
Scalability: Ensure that the infrastructure can scale efficiently to handle increased user loads and data volumes.
Ethical Considerations: Actively work to identify and mitigate biases in the LLM to ensure fair and ethical use.
Innovative Uses and Future Trends
LLMs are being used in innovative ways, pushing the boundaries of what is possible with AI in app development.
.
Cutting-edge applications and developments:
Healthcare: LLMs are used to analyze patient data, assist in diagnostics, and provide personalized health recommendations.
Finance: Financial institutions use LLMs to detect fraudulent activities, automate customer service, and provide investment advice.
Education: Educational platforms leverage LLMs to create interactive tutoring systems, personalized learning plans, and automated grading systems.
Creative Arts: LLMs are being used to generate music, art, and literature, assisting artists in the creative process.
Predictions for the future of LLMs in app development:
Increased Personalization: LLMs will enable more personalized user experiences, tailoring content and interactions to individual preferences and behaviours.
Enhanced Interactivity: Future apps will feature more interactive and conversational interfaces, making user interactions more natural and engaging.
Cross-Industry Integration: The use of LLMs will expand across various industries, from legal to entertainment, offering innovative solutions to complex problems.
Ethical AI: There will be a stronger focus on developing ethical AI practices, ensuring that LLMs are used responsibly and fairly.
By examining these successful implementations and exploring future trends, developers can gain valuable insights into the potential of LLMs and how to integrate them into their applications effectively.
Additional Resources
For those looking to delve deeper into the world of Large Language Models (LLMs) and their applications in app development, there are numerous resources available.
This section provides recommended readings, tools, libraries, and communities for support.
Recommended Reading and Tutorials
Enhance your understanding of LLMs with these valuable books, articles, and online courses.
Books:
"Deep Learning" by Ian Goodfellow, Yoshua Bengio, and Aaron Courville: A comprehensive resource on deep learning principles, including the foundations of LLMs. Get the book
"Natural Language Processing with PyTorch" by Delip Rao and Brian McMahan: A practical guide to NLP, with applications of LLMs using PyTorch. Get the book
Articles:
"Attention is All You Need" by Vaswani et al.: The seminal paper introducing the transformer architecture that underpins modern LLMs. Read it here
"The Illustrated Transformer" by Jay Alammar: A visual and intuitive guide to understanding the transformer model. Read it here
Online Courses:
"Deep Learning Specialization" by Andrew Ng on Coursera: A series of courses covering the basics of deep learning, including NLP applications. Enroll here
"Natural Language Processing with Deep Learning" by Stanford University: A course focusing on advanced NLP techniques and LLMs. Access the course
Tools and Libraries
Utilize these essential tools and libraries to work effectively with LLMs.
Transformers by Hugging Face: A library providing thousands of pre-trained models for NLP tasks, including GPT-4 and other LLMs. Explore Transformers
TensorFlow: An open-source platform for machine learning, offering tools for building and training LLMs. Visit TensorFlow
PyTorch: A flexible deep learning framework used for developing and training LLMs. Visit PyTorch
OpenAI API: Access powerful models like GPT-4 via a simple API, enabling easy integration into applications. Learn more
Communities and Support
Engage with online forums and communities to seek support, share knowledge, and stay updated with the latest developments in LLMs.
Reddit - r/MachineLearning: A popular community for discussing machine learning topics, sharing research, and asking questions. Join the conversation
Hugging Face Forums: A supportive community focused on the Hugging Face ecosystem, including transformers and other NLP tools. Join the forums
AI Alignment Forum: A platform dedicated to discussing AI alignment and safety, relevant for ethical considerations in LLM development. Participate here
Stack Overflow: A valuable resource for developers to ask questions and share solutions related to LLMs and other programming topics. Ask a question
Conclusion
Large Language Models (LLMs) have revolutionized app development, enhancing NLP, chatbots, content creation, and data insights. Despite integration challenges, their benefits in scalability, performance, and user experience are immense. As technology advances, LLMs will drive further innovation and personalization. Developers should continue exploring these models to create more intuitive and impactful applications with a promising future across various industries.
RAGA AI provides tools for developing and deploying LLM-powered applications, ensuring model accuracy, ethical usage, and streamlined integration. By leveraging RAGA AI's capabilities, developers can enhance model accuracy, ensure ethical usage, and streamline integration processes. This results in more robust, scalable, and user-friendly applications tailored to meet diverse industry needs.
Sign up for RAGA and get your LLM application ready 3x faster!
Large language models application through production has become a game-changer in the tech world. These advanced models, like GPT-4 and BERT, have transformed how developers approach app creation, offering new possibilities and efficiencies.
Integrating large language models into your apps can significantly enhance user experience and functionality. Imagine building a chatbot that understands nuanced customer queries or an app that generates content tailored to individual preferences. The Large language models application through production are vast and continually evolving, making this an exciting frontier for developers. Now, let's dive deeper into understanding large language models and their remarkable capabilities.
Overview of Large Language Models (LLMs)
Large Language Models (LLMs) have revolutionized the field of artificial intelligence. These models, like GPT-4 and BERT, are designed to understand and generate human-like text by predicting the next word in a sequence. Their ability to process and produce text with remarkable coherence makes them invaluable tools for a wide range of applications.
Definition
LLMs are advanced AI systems trained on vast datasets to predict and generate text. These models utilize deep learning techniques to analyze and understand the structure of language, allowing them to produce coherent and contextually relevant text.
Historical development and evolution:
Early Models: The journey began with simpler models like n-grams and statistical methods that could only handle limited contexts.
ntroduction of Neural Networks: The use of neural networks marked a significant improvement, allowing models to understand more complex patterns in language.
Transformer Architecture: The advent of transformer models, such as the Transformer by Vaswani et al., revolutionized the field, enabling more efficient processing of text and better handling of context.
Modern LLMs: Today, models like GPT-4 and Gemini represent the pinnacle of this evolution, capable of generating highly coherent and contextually relevant text across a wide range of applications.
Basic Understanding
By analyzing context and word patterns, LLMs can create responses that are contextually relevant and meaningful. They are capable of understanding complex language structures, idiomatic expressions, and the nuances of human communication, making them incredibly versatile.
Examples of popular LLMs:
GPT-4: OpenAI's GPT-4 is renowned for its capacity to produce precisely contextualized text, which makes it perfect for chatbots and other applications. GPT-4 can handle a wide range of tasks, from answering questions to writing essays, demonstrating a high level of linguistic competence.
Gemini: Created by Google DeepMind, Gemini excels in integrating various AI capabilities, including language understanding and generation. Gemini's advanced architecture allows it to perform a wide range of tasks, from conversational AI to complex problem-solving, showcasing its versatility in various applications.
Key differences and use cases:
GPT-4 vs. Gemini: While both models are highly capable, GPT-4 is particularly noted for its text generation prowess, whereas Gemini is praised for its integration of multiple AI functionalities.
Gemini: Unlike GPT-4, Gemini is primarily designed for tasks that require a deep understanding of context, making it ideal for search engines and other query-based applications.
Use Cases: GPT-4 is used for creating conversational agents and automated writing tools; Gemini is suitable for complex AI integrations and is optimal for improving search and retrieval systems.
Rise of LLMs in Application Development
The integration of LLMs into applications is rapidly becoming a standard in the tech industry. Their ability to enhance user interaction and automate complex tasks makes them highly relevant in today's digital landscape.
Growing trend of integrating LLMs into apps:
Increased Adoption: More businesses are incorporating LLMs into their apps to stay competitive and improve user experience. From startups to large enterprises, companies are leveraging LLMs to streamline operations, provide better customer support, and innovate their product offerings.
Technological Advancements: Continuous improvements in LLM technology are driving their widespread adoption across various industries. Enhanced computational power, access to larger datasets, and advancements in AI research have made LLMs more powerful and accessible than ever before.
Potential benefits and applications:
Enhanced User Interaction: LLMs can power chatbots and virtual assistants, providing users with more intuitive and responsive interactions. These models can understand and respond to user queries naturally and conversationally, improving overall user satisfaction.
Automated Content Creation: These models can generate high-quality content, from articles to social media posts, reducing the need for manual effort. Businesses can use LLMs to produce consistent and engaging content quickly, saving time and resources.
Data Analysis and Insights: LLMs can analyze large datasets to extract meaningful insights, aiding in decision-making processes. They can process and interpret complex data sets, identify patterns, and provide actionable insights, making them valuable tools for business intelligence.
Understanding the foundational aspects of LLMs and their growing importance sets the stage for exploring their practical applications in app development.
In the next section, we'll delve deeper into what makes large language models unique and how they work.
Working of Large Language Model
To appreciate the capabilities of LLMs, it's crucial to understand their underlying architecture and the training processes that enable them to perform complex tasks.
Basic architecture and training process:
Transformer Architecture: LLMs typically use transformer architecture, which relies on self-attention mechanisms to weigh the importance of different words in a sentence.
Training Process: Large volumes of text data are used to train these models, which then use the context given by the words before them to predict the word that will appear next in a sequence. For this training, billions of parameters need to be tuned using techniques like gradient descent.
Key features and functionalities:
Contextual Understanding: LLMs excel at understanding the context of words and sentences, allowing them to generate coherent and relevant text.
Text Generation: They can produce high-quality text that mimics human writing, useful for tasks like content creation and automated communication.
Versatility: LLMs are very flexible to a wide range of applications, from data analysis to customer support, since they can be tailored for certain tasks and use scenarios.
With this foundational knowledge, we can now explore how LLMs are applied in real-world scenarios and their impact on various industries.
Applications of LLMs in App Development
With a broad range of applications in app development, Large Language Models (LLMs) greatly improve the capabilities of different software solutions. From improving natural language processing to creating sophisticated chatbots and virtual assistants, LLMs are transforming how developers build and manage applications.
This section explores the diverse uses of LLMs in app development and their impact on user experience and functionality.
Natural Language Processing (NLP)
LLMs are a cornerstone of natural language processing, enabling apps to understand and generate human language with remarkable accuracy.
Text generation and completion:
Text Generation: LLMs can create coherent and contextually relevant text based on a given prompt. This is useful in applications such as automated writing tools, creative content generation, and scriptwriting.
Text Completion: These models can predict and complete sentences or paragraphs, enhancing user productivity in writing apps, email clients, and other text-heavy applications. For instance, they can help users draft emails by suggesting how to complete sentences based on the initial input.
Sentiment analysis and text classification:
Sentiment Analysis: LLMs can analyze text to determine the sentiment behind it, identifying whether the content is positive, negative, or neutral. This capability is essential for customer feedback analysis, social media monitoring, and market research.
Text Classification: They can categorize text into predefined labels, such as spam detection, topic categorization, and content moderation. This helps in organizing large volumes of text data efficiently.
Chatbots and Virtual Assistants
LLMs enhance the functionality and user experience of chatbots and virtual assistants, making them more interactive and helpful.
Enhancing user interaction and support:
Natural Conversations: LLMs enable chatbots to engage in natural, human-like conversations, improving user satisfaction and engagement. They can understand context, manage dialogue flow, and provide accurate responses.
Support Automation: These models can automate customer support tasks, answering frequently asked questions, resolving common issues, and directing users to appropriate resources without human intervention.
Examples of successful implementations:
Customer Service Bots: Companies like Amazon and Apple use LLM-powered chatbots to provide instant customer support, reducing wait times and improving service quality.
Virtual Assistants: Assistants like Google Assistant and Apple's Siri leverage LLMs to perform tasks such as setting reminders, providing weather updates, and answering queries, showcasing the practical applications of these models in daily life.
Content Creation and Management
LLMs streamline content creation and management processes, making it easier to produce and organize large volumes of content.
Automated content generation:
Content Creation: LLMs can generate articles, blog posts, social media updates, and more tailored to specific topics and styles. This capability helps businesses maintain a consistent content output without requiring extensive manual effort.
Personalized Content: They can create personalized content for different user segments, enhancing engagement by catering to individual preferences and needs.
Managing and curating content at scale:
Content Management: LLMs can assist in managing large content repositories by tagging, categorizing, and summarizing content, making it easier to retrieve and utilize.
Content Curation: These models can curate relevant content from various sources, providing users with up-to-date and personalized information feeds and enhancing the value of content platforms.
Data Analysis and Insights
LLMs offer powerful tools for analyzing data and extracting actionable insights, automating many of the processes traditionally handled by data scientists.
Extracting meaningful insights from data:
Data Interpretation: LLMs can analyze complex datasets to identify trends, patterns, and anomalies, helping businesses make informed decisions based on comprehensive data analysis.
Report Generation: Their ability to produce comprehensive reports and summaries from unprocessed data facilitates stakeholders' comprehension and application of the findings.
Automating data processing tasks:
Data Cleaning and Preparation: Time-consuming chores of cleaning and preparing data for analysis can be automated by LLMs, which also lowers the risk of error.
Automated Analytics: These models may analyze data in real time and deliver immediate insights, allowing businesses to respond swiftly to changing circumstances and make more effective decisions based on data.
By leveraging the capabilities of LLMs, developers can create more intelligent, responsive, and efficient applications that enhance user experience and drive business value.
Next, we'll explore the steps to integrate these powerful models into your app development process.
Steps to Integrate LLMs into Apps
Integrating Large Language Models (LLMs) into your applications can significantly enhance their capabilities and user experience. To deploy and manage the software, among other crucial processes, the process must first identify use cases.
The necessary actions to guarantee a seamless and successful integration of LLMs into your applications are described in this section.
Identifying Use Cases and Requirements
Before integrating an LLM, it's crucial to understand the specific needs of your application and define clear objectives and goals.
Understanding the specific needs of the App:
User Requirements: Determine what users need from the app and how an LLM can fulfill those needs. This involves analyzing user interactions, feedback, and common tasks that the app should support.
Functional Requirements: Identify the specific functionalities that the LLM will enhance or automate, such as natural language understanding, text generation, or sentiment analysis.
Defining clear objectives and goals:
Objectives: Clearly define your goals and assign metrics for achieving the LLM integration. For example, improving customer service response times or automating content generation.
Goals: Establish long-term goals that align with your business strategy, such as increasing user engagement, reducing operational costs, or expanding service offerings.
Choosing the Right LLM
Selecting the appropriate LLM is critical for achieving the desired outcomes and ensuring compatibility with your app.
Evaluating different models based on the use case:
Model Comparison: Compare various LLMs like GPT-4, Gemini, and others to understand their strengths and limitations. Consider how well each model addresses your specific use cases.
Performance Metrics: Evaluate models based on performance metrics such as accuracy, correctness, response time, and ability to handle specific tasks.
Considering factors like performance, cost, and compatibility:
Performance: Assess the performance of the LLM in real-world scenarios relevant to your application. Ensure it can handle the expected workload efficiently.
Cost: Consider the cost implications of using different LLMs, including licensing fees, computational resources, and ongoing maintenance.
Compatibility: Make sure the LLM you select can be easily incorporated into your development environment and is compatible with the technology stack you currently have.
Setting Up the Development Environment
A well-prepared development environment is essential for integrating and testing LLMs effectively.
Tools and frameworks for integration:
Development Tools: Utilize tools like TensorFlow, PyTorch, or specific APIs provided by LLM developers to integrate the models into your app.
TensorFlow Example
import tensorflow as tf
# Set up the computational environment
tf.config.set_visible_devices([tf.config.list_physical_devices('GPU')[0]])
PyTorch Example
import torch
import torch.nn as nn
# Set up the computational environment
device = torch.device("cuda:0" if torch.cuda.is_available() else "cpu")
Frameworks: Consider using frameworks like Hugging Face's Transformers or LangChain to simplify the integration process and manage model interactions.
Preparing the necessary infrastructure:
Computational Resources: Ensure you have adequate computational resources, such as GPUs or cloud-based services, to handle the demands of training and running LLMs.
Data Management: Set up robust data management practices to handle the large volumes of data required for training and fine-tuning the models.
Training and Fine-Tuning the Model
Customizing the LLM to suit your specific needs involves training and fine-tuning the model.
Customizing the Model for specific tasks:
Task-Specific Training: Train the model on datasets relevant to your application to improve its performance in specific tasks like customer support or content generation.
Domain Adaptation: Adapt the model to understand and generate text specific to your industry or domain, enhancing its relevance and accuracy.
Techniques for effective training and fine-tuning:
Supervised Learning: Use supervised learning techniques to train the model on labeled datasets, ensuring it learns to perform tasks accurately.
Supervised Learning Example (TensorFlow)
import tensorflow as tf
from tensorflow.keras.preprocessing.text import Tokenizer
from tensorflow.keras.preprocessing.sequence import pad_sequences
# Load the dataset
train_texts, train_labels = ...
# Tokenize the text data
tokenizer = Tokenizer(num_words=5000)
train_sequences = tokenizer.texts_to_sequences(train_texts)
# Pad the sequences
max_length = 128
padded_train_sequences = pad_sequences(train_sequences, maxlen=max_length)
# Define the model
model = tf.keras.Sequential([
tf.keras.layers.Embedding(input_dim=5000, output_dim=128, input_length=max_length),
tf.keras.layers.LSTM(64, return_sequences=True),
tf.keras.layers.Dense(1, activation='sigmoid')
])
# Compile the model
model.compile(optimizer='adam', loss='binary_crossentropy', metrics=['accuracy'])
# Train the model
model.fit(padded_train_sequences, train_labels, epochs=10, batch_size=32)
Fine-Tuning Example (PyTorch)
import torch
import torch.nn as nn
import torch.optim as optim
# Load the pre-trained model
model = torch.load('pretrained_model.pt')
# Define the fine-tuning model
fine_tuning_model = nn.Sequential(
nn.Linear(128, 64),
nn.ReLU(),
nn.Linear(64, 1),
nn.Sigmoid()
)
# Define the optimizer and loss fhttps://docs.google.com/document/d/1SFJpqYWCqufiQ7HpkOxTVF5gEI0uIFLjrtZjf4hIRvg/edit#heading=h.vwj13hlpd57function
optimizer = optim.Adam(fine_tuning_model.parameters(), lr=0.001)
criterion = nn.BCELoss()
# Train the fine-tuning model
for epoch in range(10):
for batch in train_loader:
inputs, labels = batch
outputs = fine_tuning_model(inputs)
loss = criterion(outputs, labels)
optimizer.zero_grad()
loss.backward()
optimizer.step()
Hyperparameter Tuning: Optimize hyperparameters to improve model performance, such as adjusting learning rates, batch sizes, and training epochs.
Implementing the Model into the App
The implementation phase involves coding and integrating the LLM into your app, followed by thorough testing and debugging.
Coding and integration processes:
API Integration: Implement the LLM using APIs, ensuring smooth communication between the model and your application.
Example of API Integration:
import requests
# Define the API endpoint
api_endpoint = 'https://api.example.com/llm'
# Define the request payload
payload = {'text': 'Hello, world'}
# Send the request
response = requests.post(api_endpoint, json=payload)
# Process the response
if response.status_code == 200:
response_data = response.json()
print(response_data)
Modular Design: Use a modular design approach to integrate the LLM, allowing for easier updates and maintenance.
Example of Modular Design:
class LLMIntegration:
def __init__(self):
self.llm = None
def load_llm(self):
self.llm = torch.load('pretrained_model.pt')
def generate_text(self, prompt):
if self.llm is None:
self.load_llm()
return self.llm.generate_text(prompt)
# Create an instance of the LLMIntegration class
llm_integration = LLMIntegration()
# Generate text using the LLM
prompt = 'Write a short story about a cat'
text = llm_integration.generate_text(prompt)
print(text)
Testing and debugging:
Unit Testing: Conduct unit tests to verify that individual components of the integration work as expected.
End-to-end Testing: Perform end-to-end testing to ensure the entire application functions correctly with the integrated LLM.
Debugging: Identify and fix any issues that arise during testing, ensuring the model operates smoothly within the app.
Deployment and Maintenance
Deploying the app in a production environment and maintaining it ensures long-term success and optimal performance.
Deploying the App in a production environment:
Scalability: Ensure the app can scale to handle increased usage and data volumes, utilizing cloud services or on-premises solutions as needed.
Scalability Example (Docker)
FROM python:3.9-slim
# Install required libraries
RUN pip install torch torchvision
# Copy the application code
COPY app.py .
# Define the environment variables
ENV LLM_MODEL=pretrained_model.pt
# Define the command to run the application
CMD ["python", "app.py"]
Security: Implement robust security measures to protect user data and ensure compliance with relevant regulations.
Ongoing monitoring and updates:
Performance Monitoring: Continuously monitor the performance of the LLM within the app, using tools to track metrics and identify potential issues.
Performance Monitoring Example (Python)
import logging
import time
# Set up the logging configuration
logging.basicConfig(level=logging.INFO)
# Define the performance monitoring function
def monitor_performance():
# Log the performance metrics
logging.info(f"Model accuracy: {model.accuracy:.2f}")
logging.info(f"Model latency: {model.latency:.2f} ms")
# Run the performance monitoring function periodically
while True:
monitor_performance()
time.sleep(60
# Run the performance monitoring function periodicallywhile True: monitor_performance() time.sleep(60)
Regular Updates: Keep the model and app updated with the latest features and improvements, addressing any bugs or vulnerabilities promptly.
User Feedback: Collect and analyze user feedback to make iterative improvements, ensuring the app remains relevant and effective.
By following these steps, you can successfully integrate LLMs into your applications, enhancing their functionality and providing a superior user experience.
Next, we'll explore the challenges and considerations involved in working with LLMs.
Challenges and Considerations
Integrating Large Language Models (LLMs) into applications brings significant benefits, but it also presents several challenges and considerations. Addressing these effectively is crucial for the successful deployment and operation of LLM-powered apps.
This section covers the ethical and privacy concerns, technical challenges, and user experience considerations that developers must navigate.
Ethical and Privacy Concerns
As powerful as LLMs are, their deployment must be handled with a keen awareness of ethical and privacy issues.
Addressing data privacy and security issues:
Data Protection: Achieve secure processing and storage of user data. To protect sensitive data, this entails putting access limits, encryption, and frequent security assessments in place.
Compliance: Adhere to data protection regulations such as GDPR, CCPA, and other relevant laws. This includes obtaining user consent for data collection and providing options for data deletion upon request.
Ensuring ethical use of LLMs:
Bias Mitigation: Actively work to identify and mitigate biases in the training data and the model’s outputs. This helps prevent the perpetuation of harmful stereotypes or unfair treatment of certain user groups.
Transparency: Maintain transparency about how the LLM is used within the app. Inform users about the data being collected, how it is used, and the role of the AI in generating responses or making decisions.
Technical Challenges
The technical aspects of integrating LLMs pose significant challenges, especially concerning data and computational requirements.
Handling large datasets and computational requirements:
Data Management: Managing and processing large datasets required for training LLMs can be complex and resource-intensive. Effective data management practices, including data cleaning, preprocessing, and storage solutions, are essential.
Computational Resources: Training and deploying LLMs require substantial computational power. Leveraging cloud-based solutions or investing in high-performance hardware can help meet these demands.
Ensuring scalability and performance:
Scalability: Design the system to scale efficiently with increasing user loads and data volumes. This involves using scalable cloud infrastructure, load balancing, and efficient data handling practices.
Performance Optimization: Continuously optimize the performance of the LLM and the application. This includes reducing latency, improving response times, and ensuring that the system can handle peak loads without degradation in performance.
User Experience
The success of an LLM-integrated app heavily relies on providing a seamless and intuitive user experience.
Designing intuitive and user-friendly interfaces:
User-Centric Design: Focus on creating interfaces that are easy to navigate and understand. This involves using clear language, intuitive navigation, and providing helpful prompts or suggestions.
Accessibility: Ensure that the app is accessible to all users, including those with disabilities. Implement features like text-to-speech, screen reader compatibility, and adjustable text sizes.
Managing user expectations and feedback:
Expectation Management: Clearly communicate the capabilities and limitations of the LLM to users. Setting realistic expectations helps prevent frustration and enhances user satisfaction.
Feedback Mechanisms: Implement robust feedback mechanisms to gather user insights and suggestions. Regularly analyze this feedback to make iterative improvements to the app, ensuring it meets user needs and preferences.
Understanding and addressing these challenges is crucial for leveraging the full potential of LLMs while maintaining high ethical standards, technical robustness, and a positive user experience.
In the next section, we will delve into real-world case studies and examples of successful LLM implementations.
Case Studies and Examples
Understanding real-world implementations and future trends of Large Language Models (LLMs) can provide valuable insights and inspiration for leveraging these powerful tools in app development.
This section highlights successful implementations, including those from RAGA AI, and explores innovative uses and future trends.
Successful Implementations
LLMs have been successfully integrated into various applications across different industries, showcasing their versatility and effectiveness.
Real-world examples of apps using LLMs:
Grammarly: Uses LLMs to enhance its grammar and style correction capabilities, helping users write more clearly and effectively.
Netflix: Utilizes sentiment analysis powered by LLMs to understand viewer reactions and refine content recommendations.
Duolingo: Implements LLMs to provide personalized language learning experiences, adapting lessons to the user's proficiency level.
Shopify: Uses LLMs to power its customer support chatbots, improving response times and user satisfaction.
Case studies from RAGA AI:
Safeguarding Enterprise LLM Applications: RAGA AI implemented guardrails to enhance the reliability of an enterprise LLM application, significantly reducing error rates and improving user trust.
Evaluating and Monitoring LLM Applications: A financial services firm used RAGA AI to monitor and evaluate their LLM application, leading to a 30% increase in efficiency and a 20% reduction in operational costs.
Advancing Geospatial Semantic Segmentation: RAGA AI's automated testing platform improved the accuracy of a geospatial semantic segmentation model, enabling faster and more reliable data processing for a government agency.
Key takeaways and lessons learned:
User-Centric Design: Focus on designing applications that meet specific user needs and enhance user experience.
Continuous Improvement: Regularly update and fine-tune the LLM to keep up with evolving user expectations and new data.
Scalability: Ensure that the infrastructure can scale efficiently to handle increased user loads and data volumes.
Ethical Considerations: Actively work to identify and mitigate biases in the LLM to ensure fair and ethical use.
Innovative Uses and Future Trends
LLMs are being used in innovative ways, pushing the boundaries of what is possible with AI in app development.
.
Cutting-edge applications and developments:
Healthcare: LLMs are used to analyze patient data, assist in diagnostics, and provide personalized health recommendations.
Finance: Financial institutions use LLMs to detect fraudulent activities, automate customer service, and provide investment advice.
Education: Educational platforms leverage LLMs to create interactive tutoring systems, personalized learning plans, and automated grading systems.
Creative Arts: LLMs are being used to generate music, art, and literature, assisting artists in the creative process.
Predictions for the future of LLMs in app development:
Increased Personalization: LLMs will enable more personalized user experiences, tailoring content and interactions to individual preferences and behaviours.
Enhanced Interactivity: Future apps will feature more interactive and conversational interfaces, making user interactions more natural and engaging.
Cross-Industry Integration: The use of LLMs will expand across various industries, from legal to entertainment, offering innovative solutions to complex problems.
Ethical AI: There will be a stronger focus on developing ethical AI practices, ensuring that LLMs are used responsibly and fairly.
By examining these successful implementations and exploring future trends, developers can gain valuable insights into the potential of LLMs and how to integrate them into their applications effectively.
Additional Resources
For those looking to delve deeper into the world of Large Language Models (LLMs) and their applications in app development, there are numerous resources available.
This section provides recommended readings, tools, libraries, and communities for support.
Recommended Reading and Tutorials
Enhance your understanding of LLMs with these valuable books, articles, and online courses.
Books:
"Deep Learning" by Ian Goodfellow, Yoshua Bengio, and Aaron Courville: A comprehensive resource on deep learning principles, including the foundations of LLMs. Get the book
"Natural Language Processing with PyTorch" by Delip Rao and Brian McMahan: A practical guide to NLP, with applications of LLMs using PyTorch. Get the book
Articles:
"Attention is All You Need" by Vaswani et al.: The seminal paper introducing the transformer architecture that underpins modern LLMs. Read it here
"The Illustrated Transformer" by Jay Alammar: A visual and intuitive guide to understanding the transformer model. Read it here
Online Courses:
"Deep Learning Specialization" by Andrew Ng on Coursera: A series of courses covering the basics of deep learning, including NLP applications. Enroll here
"Natural Language Processing with Deep Learning" by Stanford University: A course focusing on advanced NLP techniques and LLMs. Access the course
Tools and Libraries
Utilize these essential tools and libraries to work effectively with LLMs.
Transformers by Hugging Face: A library providing thousands of pre-trained models for NLP tasks, including GPT-4 and other LLMs. Explore Transformers
TensorFlow: An open-source platform for machine learning, offering tools for building and training LLMs. Visit TensorFlow
PyTorch: A flexible deep learning framework used for developing and training LLMs. Visit PyTorch
OpenAI API: Access powerful models like GPT-4 via a simple API, enabling easy integration into applications. Learn more
Communities and Support
Engage with online forums and communities to seek support, share knowledge, and stay updated with the latest developments in LLMs.
Reddit - r/MachineLearning: A popular community for discussing machine learning topics, sharing research, and asking questions. Join the conversation
Hugging Face Forums: A supportive community focused on the Hugging Face ecosystem, including transformers and other NLP tools. Join the forums
AI Alignment Forum: A platform dedicated to discussing AI alignment and safety, relevant for ethical considerations in LLM development. Participate here
Stack Overflow: A valuable resource for developers to ask questions and share solutions related to LLMs and other programming topics. Ask a question
Conclusion
Large Language Models (LLMs) have revolutionized app development, enhancing NLP, chatbots, content creation, and data insights. Despite integration challenges, their benefits in scalability, performance, and user experience are immense. As technology advances, LLMs will drive further innovation and personalization. Developers should continue exploring these models to create more intuitive and impactful applications with a promising future across various industries.
RAGA AI provides tools for developing and deploying LLM-powered applications, ensuring model accuracy, ethical usage, and streamlined integration. By leveraging RAGA AI's capabilities, developers can enhance model accuracy, ensure ethical usage, and streamline integration processes. This results in more robust, scalable, and user-friendly applications tailored to meet diverse industry needs.
Sign up for RAGA and get your LLM application ready 3x faster!
Large language models application through production has become a game-changer in the tech world. These advanced models, like GPT-4 and BERT, have transformed how developers approach app creation, offering new possibilities and efficiencies.
Integrating large language models into your apps can significantly enhance user experience and functionality. Imagine building a chatbot that understands nuanced customer queries or an app that generates content tailored to individual preferences. The Large language models application through production are vast and continually evolving, making this an exciting frontier for developers. Now, let's dive deeper into understanding large language models and their remarkable capabilities.
Overview of Large Language Models (LLMs)
Large Language Models (LLMs) have revolutionized the field of artificial intelligence. These models, like GPT-4 and BERT, are designed to understand and generate human-like text by predicting the next word in a sequence. Their ability to process and produce text with remarkable coherence makes them invaluable tools for a wide range of applications.
Definition
LLMs are advanced AI systems trained on vast datasets to predict and generate text. These models utilize deep learning techniques to analyze and understand the structure of language, allowing them to produce coherent and contextually relevant text.
Historical development and evolution:
Early Models: The journey began with simpler models like n-grams and statistical methods that could only handle limited contexts.
ntroduction of Neural Networks: The use of neural networks marked a significant improvement, allowing models to understand more complex patterns in language.
Transformer Architecture: The advent of transformer models, such as the Transformer by Vaswani et al., revolutionized the field, enabling more efficient processing of text and better handling of context.
Modern LLMs: Today, models like GPT-4 and Gemini represent the pinnacle of this evolution, capable of generating highly coherent and contextually relevant text across a wide range of applications.
Basic Understanding
By analyzing context and word patterns, LLMs can create responses that are contextually relevant and meaningful. They are capable of understanding complex language structures, idiomatic expressions, and the nuances of human communication, making them incredibly versatile.
Examples of popular LLMs:
GPT-4: OpenAI's GPT-4 is renowned for its capacity to produce precisely contextualized text, which makes it perfect for chatbots and other applications. GPT-4 can handle a wide range of tasks, from answering questions to writing essays, demonstrating a high level of linguistic competence.
Gemini: Created by Google DeepMind, Gemini excels in integrating various AI capabilities, including language understanding and generation. Gemini's advanced architecture allows it to perform a wide range of tasks, from conversational AI to complex problem-solving, showcasing its versatility in various applications.
Key differences and use cases:
GPT-4 vs. Gemini: While both models are highly capable, GPT-4 is particularly noted for its text generation prowess, whereas Gemini is praised for its integration of multiple AI functionalities.
Gemini: Unlike GPT-4, Gemini is primarily designed for tasks that require a deep understanding of context, making it ideal for search engines and other query-based applications.
Use Cases: GPT-4 is used for creating conversational agents and automated writing tools; Gemini is suitable for complex AI integrations and is optimal for improving search and retrieval systems.
Rise of LLMs in Application Development
The integration of LLMs into applications is rapidly becoming a standard in the tech industry. Their ability to enhance user interaction and automate complex tasks makes them highly relevant in today's digital landscape.
Growing trend of integrating LLMs into apps:
Increased Adoption: More businesses are incorporating LLMs into their apps to stay competitive and improve user experience. From startups to large enterprises, companies are leveraging LLMs to streamline operations, provide better customer support, and innovate their product offerings.
Technological Advancements: Continuous improvements in LLM technology are driving their widespread adoption across various industries. Enhanced computational power, access to larger datasets, and advancements in AI research have made LLMs more powerful and accessible than ever before.
Potential benefits and applications:
Enhanced User Interaction: LLMs can power chatbots and virtual assistants, providing users with more intuitive and responsive interactions. These models can understand and respond to user queries naturally and conversationally, improving overall user satisfaction.
Automated Content Creation: These models can generate high-quality content, from articles to social media posts, reducing the need for manual effort. Businesses can use LLMs to produce consistent and engaging content quickly, saving time and resources.
Data Analysis and Insights: LLMs can analyze large datasets to extract meaningful insights, aiding in decision-making processes. They can process and interpret complex data sets, identify patterns, and provide actionable insights, making them valuable tools for business intelligence.
Understanding the foundational aspects of LLMs and their growing importance sets the stage for exploring their practical applications in app development.
In the next section, we'll delve deeper into what makes large language models unique and how they work.
Working of Large Language Model
To appreciate the capabilities of LLMs, it's crucial to understand their underlying architecture and the training processes that enable them to perform complex tasks.
Basic architecture and training process:
Transformer Architecture: LLMs typically use transformer architecture, which relies on self-attention mechanisms to weigh the importance of different words in a sentence.
Training Process: Large volumes of text data are used to train these models, which then use the context given by the words before them to predict the word that will appear next in a sequence. For this training, billions of parameters need to be tuned using techniques like gradient descent.
Key features and functionalities:
Contextual Understanding: LLMs excel at understanding the context of words and sentences, allowing them to generate coherent and relevant text.
Text Generation: They can produce high-quality text that mimics human writing, useful for tasks like content creation and automated communication.
Versatility: LLMs are very flexible to a wide range of applications, from data analysis to customer support, since they can be tailored for certain tasks and use scenarios.
With this foundational knowledge, we can now explore how LLMs are applied in real-world scenarios and their impact on various industries.
Applications of LLMs in App Development
With a broad range of applications in app development, Large Language Models (LLMs) greatly improve the capabilities of different software solutions. From improving natural language processing to creating sophisticated chatbots and virtual assistants, LLMs are transforming how developers build and manage applications.
This section explores the diverse uses of LLMs in app development and their impact on user experience and functionality.
Natural Language Processing (NLP)
LLMs are a cornerstone of natural language processing, enabling apps to understand and generate human language with remarkable accuracy.
Text generation and completion:
Text Generation: LLMs can create coherent and contextually relevant text based on a given prompt. This is useful in applications such as automated writing tools, creative content generation, and scriptwriting.
Text Completion: These models can predict and complete sentences or paragraphs, enhancing user productivity in writing apps, email clients, and other text-heavy applications. For instance, they can help users draft emails by suggesting how to complete sentences based on the initial input.
Sentiment analysis and text classification:
Sentiment Analysis: LLMs can analyze text to determine the sentiment behind it, identifying whether the content is positive, negative, or neutral. This capability is essential for customer feedback analysis, social media monitoring, and market research.
Text Classification: They can categorize text into predefined labels, such as spam detection, topic categorization, and content moderation. This helps in organizing large volumes of text data efficiently.
Chatbots and Virtual Assistants
LLMs enhance the functionality and user experience of chatbots and virtual assistants, making them more interactive and helpful.
Enhancing user interaction and support:
Natural Conversations: LLMs enable chatbots to engage in natural, human-like conversations, improving user satisfaction and engagement. They can understand context, manage dialogue flow, and provide accurate responses.
Support Automation: These models can automate customer support tasks, answering frequently asked questions, resolving common issues, and directing users to appropriate resources without human intervention.
Examples of successful implementations:
Customer Service Bots: Companies like Amazon and Apple use LLM-powered chatbots to provide instant customer support, reducing wait times and improving service quality.
Virtual Assistants: Assistants like Google Assistant and Apple's Siri leverage LLMs to perform tasks such as setting reminders, providing weather updates, and answering queries, showcasing the practical applications of these models in daily life.
Content Creation and Management
LLMs streamline content creation and management processes, making it easier to produce and organize large volumes of content.
Automated content generation:
Content Creation: LLMs can generate articles, blog posts, social media updates, and more tailored to specific topics and styles. This capability helps businesses maintain a consistent content output without requiring extensive manual effort.
Personalized Content: They can create personalized content for different user segments, enhancing engagement by catering to individual preferences and needs.
Managing and curating content at scale:
Content Management: LLMs can assist in managing large content repositories by tagging, categorizing, and summarizing content, making it easier to retrieve and utilize.
Content Curation: These models can curate relevant content from various sources, providing users with up-to-date and personalized information feeds and enhancing the value of content platforms.
Data Analysis and Insights
LLMs offer powerful tools for analyzing data and extracting actionable insights, automating many of the processes traditionally handled by data scientists.
Extracting meaningful insights from data:
Data Interpretation: LLMs can analyze complex datasets to identify trends, patterns, and anomalies, helping businesses make informed decisions based on comprehensive data analysis.
Report Generation: Their ability to produce comprehensive reports and summaries from unprocessed data facilitates stakeholders' comprehension and application of the findings.
Automating data processing tasks:
Data Cleaning and Preparation: Time-consuming chores of cleaning and preparing data for analysis can be automated by LLMs, which also lowers the risk of error.
Automated Analytics: These models may analyze data in real time and deliver immediate insights, allowing businesses to respond swiftly to changing circumstances and make more effective decisions based on data.
By leveraging the capabilities of LLMs, developers can create more intelligent, responsive, and efficient applications that enhance user experience and drive business value.
Next, we'll explore the steps to integrate these powerful models into your app development process.
Steps to Integrate LLMs into Apps
Integrating Large Language Models (LLMs) into your applications can significantly enhance their capabilities and user experience. To deploy and manage the software, among other crucial processes, the process must first identify use cases.
The necessary actions to guarantee a seamless and successful integration of LLMs into your applications are described in this section.
Identifying Use Cases and Requirements
Before integrating an LLM, it's crucial to understand the specific needs of your application and define clear objectives and goals.
Understanding the specific needs of the App:
User Requirements: Determine what users need from the app and how an LLM can fulfill those needs. This involves analyzing user interactions, feedback, and common tasks that the app should support.
Functional Requirements: Identify the specific functionalities that the LLM will enhance or automate, such as natural language understanding, text generation, or sentiment analysis.
Defining clear objectives and goals:
Objectives: Clearly define your goals and assign metrics for achieving the LLM integration. For example, improving customer service response times or automating content generation.
Goals: Establish long-term goals that align with your business strategy, such as increasing user engagement, reducing operational costs, or expanding service offerings.
Choosing the Right LLM
Selecting the appropriate LLM is critical for achieving the desired outcomes and ensuring compatibility with your app.
Evaluating different models based on the use case:
Model Comparison: Compare various LLMs like GPT-4, Gemini, and others to understand their strengths and limitations. Consider how well each model addresses your specific use cases.
Performance Metrics: Evaluate models based on performance metrics such as accuracy, correctness, response time, and ability to handle specific tasks.
Considering factors like performance, cost, and compatibility:
Performance: Assess the performance of the LLM in real-world scenarios relevant to your application. Ensure it can handle the expected workload efficiently.
Cost: Consider the cost implications of using different LLMs, including licensing fees, computational resources, and ongoing maintenance.
Compatibility: Make sure the LLM you select can be easily incorporated into your development environment and is compatible with the technology stack you currently have.
Setting Up the Development Environment
A well-prepared development environment is essential for integrating and testing LLMs effectively.
Tools and frameworks for integration:
Development Tools: Utilize tools like TensorFlow, PyTorch, or specific APIs provided by LLM developers to integrate the models into your app.
TensorFlow Example
import tensorflow as tf
# Set up the computational environment
tf.config.set_visible_devices([tf.config.list_physical_devices('GPU')[0]])
PyTorch Example
import torch
import torch.nn as nn
# Set up the computational environment
device = torch.device("cuda:0" if torch.cuda.is_available() else "cpu")
Frameworks: Consider using frameworks like Hugging Face's Transformers or LangChain to simplify the integration process and manage model interactions.
Preparing the necessary infrastructure:
Computational Resources: Ensure you have adequate computational resources, such as GPUs or cloud-based services, to handle the demands of training and running LLMs.
Data Management: Set up robust data management practices to handle the large volumes of data required for training and fine-tuning the models.
Training and Fine-Tuning the Model
Customizing the LLM to suit your specific needs involves training and fine-tuning the model.
Customizing the Model for specific tasks:
Task-Specific Training: Train the model on datasets relevant to your application to improve its performance in specific tasks like customer support or content generation.
Domain Adaptation: Adapt the model to understand and generate text specific to your industry or domain, enhancing its relevance and accuracy.
Techniques for effective training and fine-tuning:
Supervised Learning: Use supervised learning techniques to train the model on labeled datasets, ensuring it learns to perform tasks accurately.
Supervised Learning Example (TensorFlow)
import tensorflow as tf
from tensorflow.keras.preprocessing.text import Tokenizer
from tensorflow.keras.preprocessing.sequence import pad_sequences
# Load the dataset
train_texts, train_labels = ...
# Tokenize the text data
tokenizer = Tokenizer(num_words=5000)
train_sequences = tokenizer.texts_to_sequences(train_texts)
# Pad the sequences
max_length = 128
padded_train_sequences = pad_sequences(train_sequences, maxlen=max_length)
# Define the model
model = tf.keras.Sequential([
tf.keras.layers.Embedding(input_dim=5000, output_dim=128, input_length=max_length),
tf.keras.layers.LSTM(64, return_sequences=True),
tf.keras.layers.Dense(1, activation='sigmoid')
])
# Compile the model
model.compile(optimizer='adam', loss='binary_crossentropy', metrics=['accuracy'])
# Train the model
model.fit(padded_train_sequences, train_labels, epochs=10, batch_size=32)
Fine-Tuning Example (PyTorch)
import torch
import torch.nn as nn
import torch.optim as optim
# Load the pre-trained model
model = torch.load('pretrained_model.pt')
# Define the fine-tuning model
fine_tuning_model = nn.Sequential(
nn.Linear(128, 64),
nn.ReLU(),
nn.Linear(64, 1),
nn.Sigmoid()
)
# Define the optimizer and loss fhttps://docs.google.com/document/d/1SFJpqYWCqufiQ7HpkOxTVF5gEI0uIFLjrtZjf4hIRvg/edit#heading=h.vwj13hlpd57function
optimizer = optim.Adam(fine_tuning_model.parameters(), lr=0.001)
criterion = nn.BCELoss()
# Train the fine-tuning model
for epoch in range(10):
for batch in train_loader:
inputs, labels = batch
outputs = fine_tuning_model(inputs)
loss = criterion(outputs, labels)
optimizer.zero_grad()
loss.backward()
optimizer.step()
Hyperparameter Tuning: Optimize hyperparameters to improve model performance, such as adjusting learning rates, batch sizes, and training epochs.
Implementing the Model into the App
The implementation phase involves coding and integrating the LLM into your app, followed by thorough testing and debugging.
Coding and integration processes:
API Integration: Implement the LLM using APIs, ensuring smooth communication between the model and your application.
Example of API Integration:
import requests
# Define the API endpoint
api_endpoint = 'https://api.example.com/llm'
# Define the request payload
payload = {'text': 'Hello, world'}
# Send the request
response = requests.post(api_endpoint, json=payload)
# Process the response
if response.status_code == 200:
response_data = response.json()
print(response_data)
Modular Design: Use a modular design approach to integrate the LLM, allowing for easier updates and maintenance.
Example of Modular Design:
class LLMIntegration:
def __init__(self):
self.llm = None
def load_llm(self):
self.llm = torch.load('pretrained_model.pt')
def generate_text(self, prompt):
if self.llm is None:
self.load_llm()
return self.llm.generate_text(prompt)
# Create an instance of the LLMIntegration class
llm_integration = LLMIntegration()
# Generate text using the LLM
prompt = 'Write a short story about a cat'
text = llm_integration.generate_text(prompt)
print(text)
Testing and debugging:
Unit Testing: Conduct unit tests to verify that individual components of the integration work as expected.
End-to-end Testing: Perform end-to-end testing to ensure the entire application functions correctly with the integrated LLM.
Debugging: Identify and fix any issues that arise during testing, ensuring the model operates smoothly within the app.
Deployment and Maintenance
Deploying the app in a production environment and maintaining it ensures long-term success and optimal performance.
Deploying the App in a production environment:
Scalability: Ensure the app can scale to handle increased usage and data volumes, utilizing cloud services or on-premises solutions as needed.
Scalability Example (Docker)
FROM python:3.9-slim
# Install required libraries
RUN pip install torch torchvision
# Copy the application code
COPY app.py .
# Define the environment variables
ENV LLM_MODEL=pretrained_model.pt
# Define the command to run the application
CMD ["python", "app.py"]
Security: Implement robust security measures to protect user data and ensure compliance with relevant regulations.
Ongoing monitoring and updates:
Performance Monitoring: Continuously monitor the performance of the LLM within the app, using tools to track metrics and identify potential issues.
Performance Monitoring Example (Python)
import logging
import time
# Set up the logging configuration
logging.basicConfig(level=logging.INFO)
# Define the performance monitoring function
def monitor_performance():
# Log the performance metrics
logging.info(f"Model accuracy: {model.accuracy:.2f}")
logging.info(f"Model latency: {model.latency:.2f} ms")
# Run the performance monitoring function periodically
while True:
monitor_performance()
time.sleep(60
# Run the performance monitoring function periodicallywhile True: monitor_performance() time.sleep(60)
Regular Updates: Keep the model and app updated with the latest features and improvements, addressing any bugs or vulnerabilities promptly.
User Feedback: Collect and analyze user feedback to make iterative improvements, ensuring the app remains relevant and effective.
By following these steps, you can successfully integrate LLMs into your applications, enhancing their functionality and providing a superior user experience.
Next, we'll explore the challenges and considerations involved in working with LLMs.
Challenges and Considerations
Integrating Large Language Models (LLMs) into applications brings significant benefits, but it also presents several challenges and considerations. Addressing these effectively is crucial for the successful deployment and operation of LLM-powered apps.
This section covers the ethical and privacy concerns, technical challenges, and user experience considerations that developers must navigate.
Ethical and Privacy Concerns
As powerful as LLMs are, their deployment must be handled with a keen awareness of ethical and privacy issues.
Addressing data privacy and security issues:
Data Protection: Achieve secure processing and storage of user data. To protect sensitive data, this entails putting access limits, encryption, and frequent security assessments in place.
Compliance: Adhere to data protection regulations such as GDPR, CCPA, and other relevant laws. This includes obtaining user consent for data collection and providing options for data deletion upon request.
Ensuring ethical use of LLMs:
Bias Mitigation: Actively work to identify and mitigate biases in the training data and the model’s outputs. This helps prevent the perpetuation of harmful stereotypes or unfair treatment of certain user groups.
Transparency: Maintain transparency about how the LLM is used within the app. Inform users about the data being collected, how it is used, and the role of the AI in generating responses or making decisions.
Technical Challenges
The technical aspects of integrating LLMs pose significant challenges, especially concerning data and computational requirements.
Handling large datasets and computational requirements:
Data Management: Managing and processing large datasets required for training LLMs can be complex and resource-intensive. Effective data management practices, including data cleaning, preprocessing, and storage solutions, are essential.
Computational Resources: Training and deploying LLMs require substantial computational power. Leveraging cloud-based solutions or investing in high-performance hardware can help meet these demands.
Ensuring scalability and performance:
Scalability: Design the system to scale efficiently with increasing user loads and data volumes. This involves using scalable cloud infrastructure, load balancing, and efficient data handling practices.
Performance Optimization: Continuously optimize the performance of the LLM and the application. This includes reducing latency, improving response times, and ensuring that the system can handle peak loads without degradation in performance.
User Experience
The success of an LLM-integrated app heavily relies on providing a seamless and intuitive user experience.
Designing intuitive and user-friendly interfaces:
User-Centric Design: Focus on creating interfaces that are easy to navigate and understand. This involves using clear language, intuitive navigation, and providing helpful prompts or suggestions.
Accessibility: Ensure that the app is accessible to all users, including those with disabilities. Implement features like text-to-speech, screen reader compatibility, and adjustable text sizes.
Managing user expectations and feedback:
Expectation Management: Clearly communicate the capabilities and limitations of the LLM to users. Setting realistic expectations helps prevent frustration and enhances user satisfaction.
Feedback Mechanisms: Implement robust feedback mechanisms to gather user insights and suggestions. Regularly analyze this feedback to make iterative improvements to the app, ensuring it meets user needs and preferences.
Understanding and addressing these challenges is crucial for leveraging the full potential of LLMs while maintaining high ethical standards, technical robustness, and a positive user experience.
In the next section, we will delve into real-world case studies and examples of successful LLM implementations.
Case Studies and Examples
Understanding real-world implementations and future trends of Large Language Models (LLMs) can provide valuable insights and inspiration for leveraging these powerful tools in app development.
This section highlights successful implementations, including those from RAGA AI, and explores innovative uses and future trends.
Successful Implementations
LLMs have been successfully integrated into various applications across different industries, showcasing their versatility and effectiveness.
Real-world examples of apps using LLMs:
Grammarly: Uses LLMs to enhance its grammar and style correction capabilities, helping users write more clearly and effectively.
Netflix: Utilizes sentiment analysis powered by LLMs to understand viewer reactions and refine content recommendations.
Duolingo: Implements LLMs to provide personalized language learning experiences, adapting lessons to the user's proficiency level.
Shopify: Uses LLMs to power its customer support chatbots, improving response times and user satisfaction.
Case studies from RAGA AI:
Safeguarding Enterprise LLM Applications: RAGA AI implemented guardrails to enhance the reliability of an enterprise LLM application, significantly reducing error rates and improving user trust.
Evaluating and Monitoring LLM Applications: A financial services firm used RAGA AI to monitor and evaluate their LLM application, leading to a 30% increase in efficiency and a 20% reduction in operational costs.
Advancing Geospatial Semantic Segmentation: RAGA AI's automated testing platform improved the accuracy of a geospatial semantic segmentation model, enabling faster and more reliable data processing for a government agency.
Key takeaways and lessons learned:
User-Centric Design: Focus on designing applications that meet specific user needs and enhance user experience.
Continuous Improvement: Regularly update and fine-tune the LLM to keep up with evolving user expectations and new data.
Scalability: Ensure that the infrastructure can scale efficiently to handle increased user loads and data volumes.
Ethical Considerations: Actively work to identify and mitigate biases in the LLM to ensure fair and ethical use.
Innovative Uses and Future Trends
LLMs are being used in innovative ways, pushing the boundaries of what is possible with AI in app development.
.
Cutting-edge applications and developments:
Healthcare: LLMs are used to analyze patient data, assist in diagnostics, and provide personalized health recommendations.
Finance: Financial institutions use LLMs to detect fraudulent activities, automate customer service, and provide investment advice.
Education: Educational platforms leverage LLMs to create interactive tutoring systems, personalized learning plans, and automated grading systems.
Creative Arts: LLMs are being used to generate music, art, and literature, assisting artists in the creative process.
Predictions for the future of LLMs in app development:
Increased Personalization: LLMs will enable more personalized user experiences, tailoring content and interactions to individual preferences and behaviours.
Enhanced Interactivity: Future apps will feature more interactive and conversational interfaces, making user interactions more natural and engaging.
Cross-Industry Integration: The use of LLMs will expand across various industries, from legal to entertainment, offering innovative solutions to complex problems.
Ethical AI: There will be a stronger focus on developing ethical AI practices, ensuring that LLMs are used responsibly and fairly.
By examining these successful implementations and exploring future trends, developers can gain valuable insights into the potential of LLMs and how to integrate them into their applications effectively.
Additional Resources
For those looking to delve deeper into the world of Large Language Models (LLMs) and their applications in app development, there are numerous resources available.
This section provides recommended readings, tools, libraries, and communities for support.
Recommended Reading and Tutorials
Enhance your understanding of LLMs with these valuable books, articles, and online courses.
Books:
"Deep Learning" by Ian Goodfellow, Yoshua Bengio, and Aaron Courville: A comprehensive resource on deep learning principles, including the foundations of LLMs. Get the book
"Natural Language Processing with PyTorch" by Delip Rao and Brian McMahan: A practical guide to NLP, with applications of LLMs using PyTorch. Get the book
Articles:
"Attention is All You Need" by Vaswani et al.: The seminal paper introducing the transformer architecture that underpins modern LLMs. Read it here
"The Illustrated Transformer" by Jay Alammar: A visual and intuitive guide to understanding the transformer model. Read it here
Online Courses:
"Deep Learning Specialization" by Andrew Ng on Coursera: A series of courses covering the basics of deep learning, including NLP applications. Enroll here
"Natural Language Processing with Deep Learning" by Stanford University: A course focusing on advanced NLP techniques and LLMs. Access the course
Tools and Libraries
Utilize these essential tools and libraries to work effectively with LLMs.
Transformers by Hugging Face: A library providing thousands of pre-trained models for NLP tasks, including GPT-4 and other LLMs. Explore Transformers
TensorFlow: An open-source platform for machine learning, offering tools for building and training LLMs. Visit TensorFlow
PyTorch: A flexible deep learning framework used for developing and training LLMs. Visit PyTorch
OpenAI API: Access powerful models like GPT-4 via a simple API, enabling easy integration into applications. Learn more
Communities and Support
Engage with online forums and communities to seek support, share knowledge, and stay updated with the latest developments in LLMs.
Reddit - r/MachineLearning: A popular community for discussing machine learning topics, sharing research, and asking questions. Join the conversation
Hugging Face Forums: A supportive community focused on the Hugging Face ecosystem, including transformers and other NLP tools. Join the forums
AI Alignment Forum: A platform dedicated to discussing AI alignment and safety, relevant for ethical considerations in LLM development. Participate here
Stack Overflow: A valuable resource for developers to ask questions and share solutions related to LLMs and other programming topics. Ask a question
Conclusion
Large Language Models (LLMs) have revolutionized app development, enhancing NLP, chatbots, content creation, and data insights. Despite integration challenges, their benefits in scalability, performance, and user experience are immense. As technology advances, LLMs will drive further innovation and personalization. Developers should continue exploring these models to create more intuitive and impactful applications with a promising future across various industries.
RAGA AI provides tools for developing and deploying LLM-powered applications, ensuring model accuracy, ethical usage, and streamlined integration. By leveraging RAGA AI's capabilities, developers can enhance model accuracy, ensure ethical usage, and streamline integration processes. This results in more robust, scalable, and user-friendly applications tailored to meet diverse industry needs.
Sign up for RAGA and get your LLM application ready 3x faster!
Subscribe to our newsletter to never miss an update
Other articles


Agents Talk, Models Think: A2A + MCP for Enterprise Agentic AI

Riya Parikh, Nitai Agarwal
Apr 27, 2025
Read the article
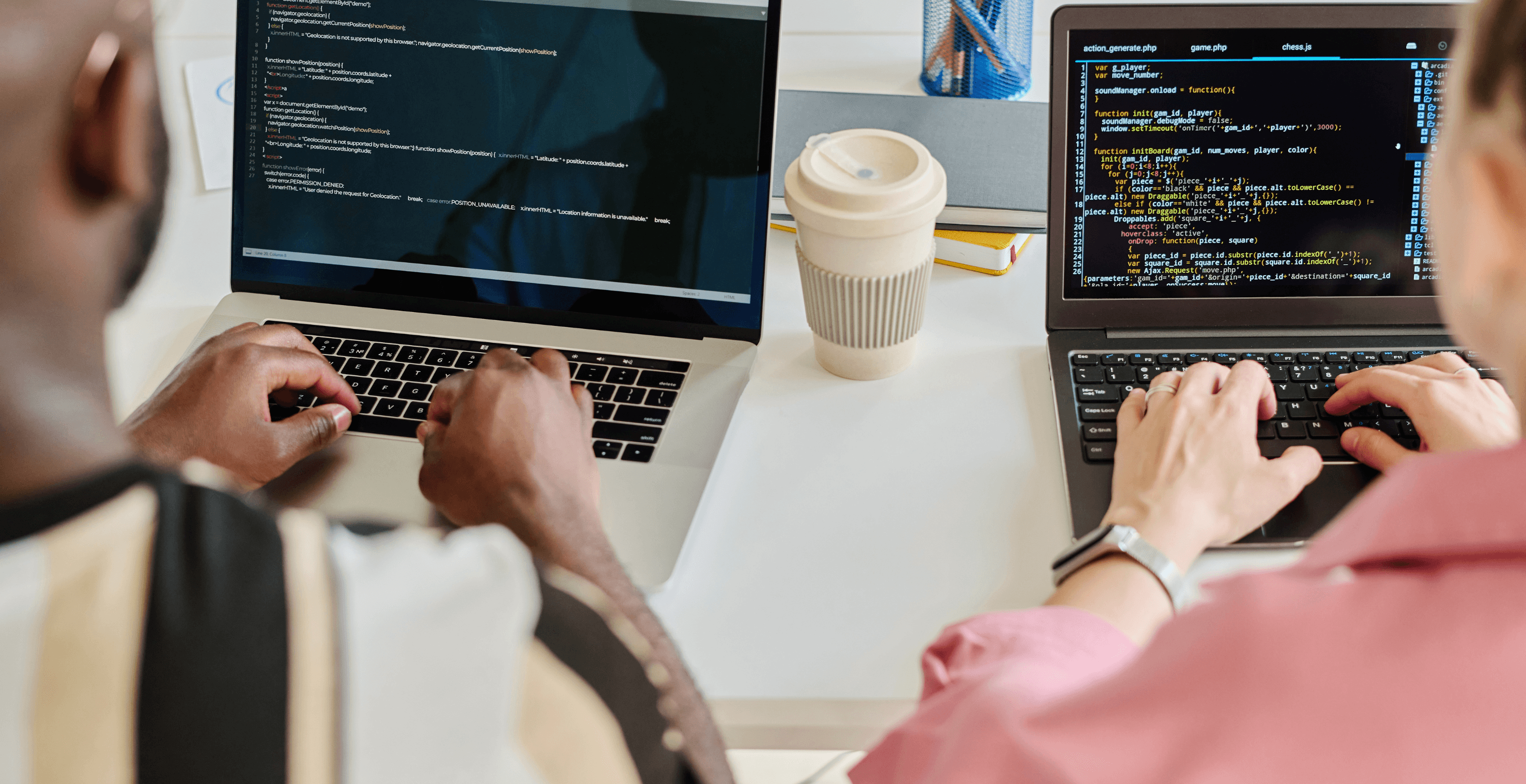
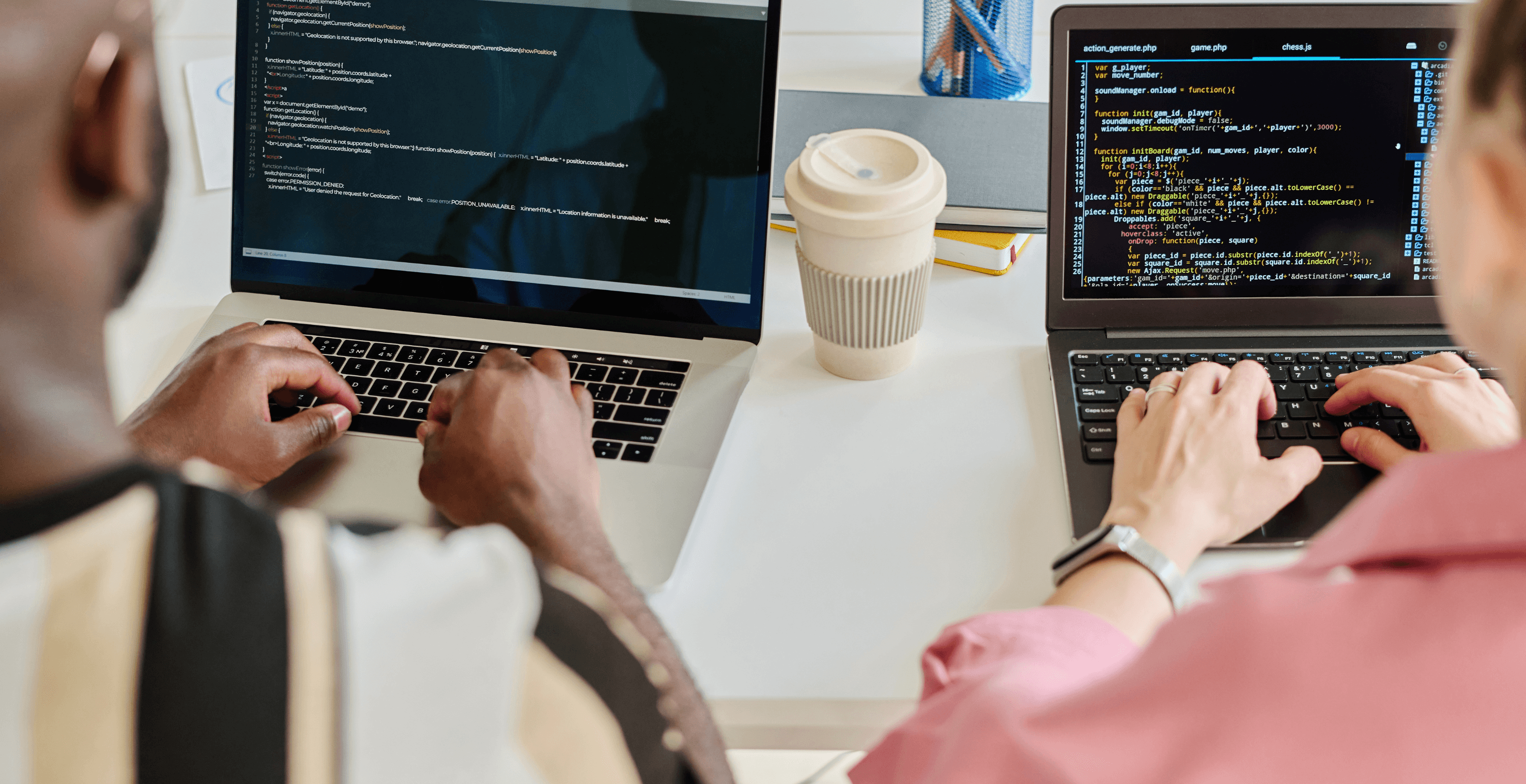
Understanding NeMo Guardrails: A Toolkit for LLM Security

Rehan Asif
Dec 24, 2024
Read the article


Understanding Differences in Large vs Small Language Models (LLM vs SLM)

Rehan Asif
Dec 21, 2024
Read the article