Challenges and Strategies for Implementing Enterprise LLM
Challenges and Strategies for Implementing Enterprise LLM
Challenges and Strategies for Implementing Enterprise LLM
Rehan Asif
Apr 20, 2024




Integrating large language models (LLMs) into enterprise applications is becoming a game-changer for many large companies. Substantial investments are flowing into this area, and businesses are eager to harness the power of LLMs for various innovative applications.
From engaging customers in more human-like conversations to detecting fraud and even diagnosing medical conditions, the potential uses are as diverse as they are impactful.
As AI evolves, the corporate world increasingly recognizes the value of implementing advanced LLMs. Companies are investing heavily financially and in the resources needed to integrate these technologies into their operations.
This surge in investment is driven by the promise of significant returns through enhanced efficiencies, improved customer interactions, and new capabilities that were once thought impossible without human intervention.
Key Challenges in Enterprise LLM Implementation
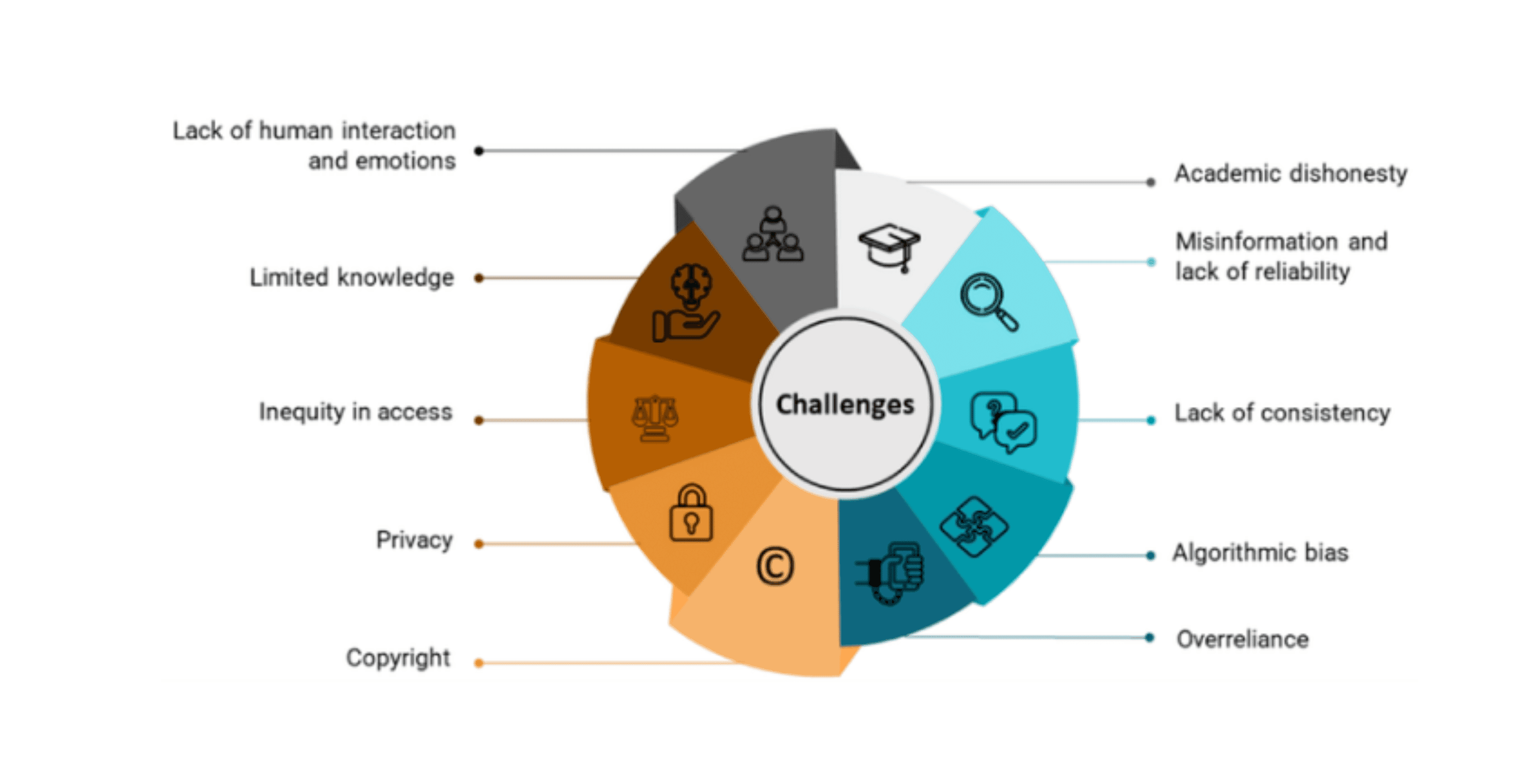
Source: Research Gate
Implementing LLMs in an enterprise setting comes with a set of unique challenges. These range from ensuring the models meet the high accuracy demands of specific applications to overcoming integration hurdles and securing sensitive data.
Generic LLM Limitations for Specific Enterprise Applications
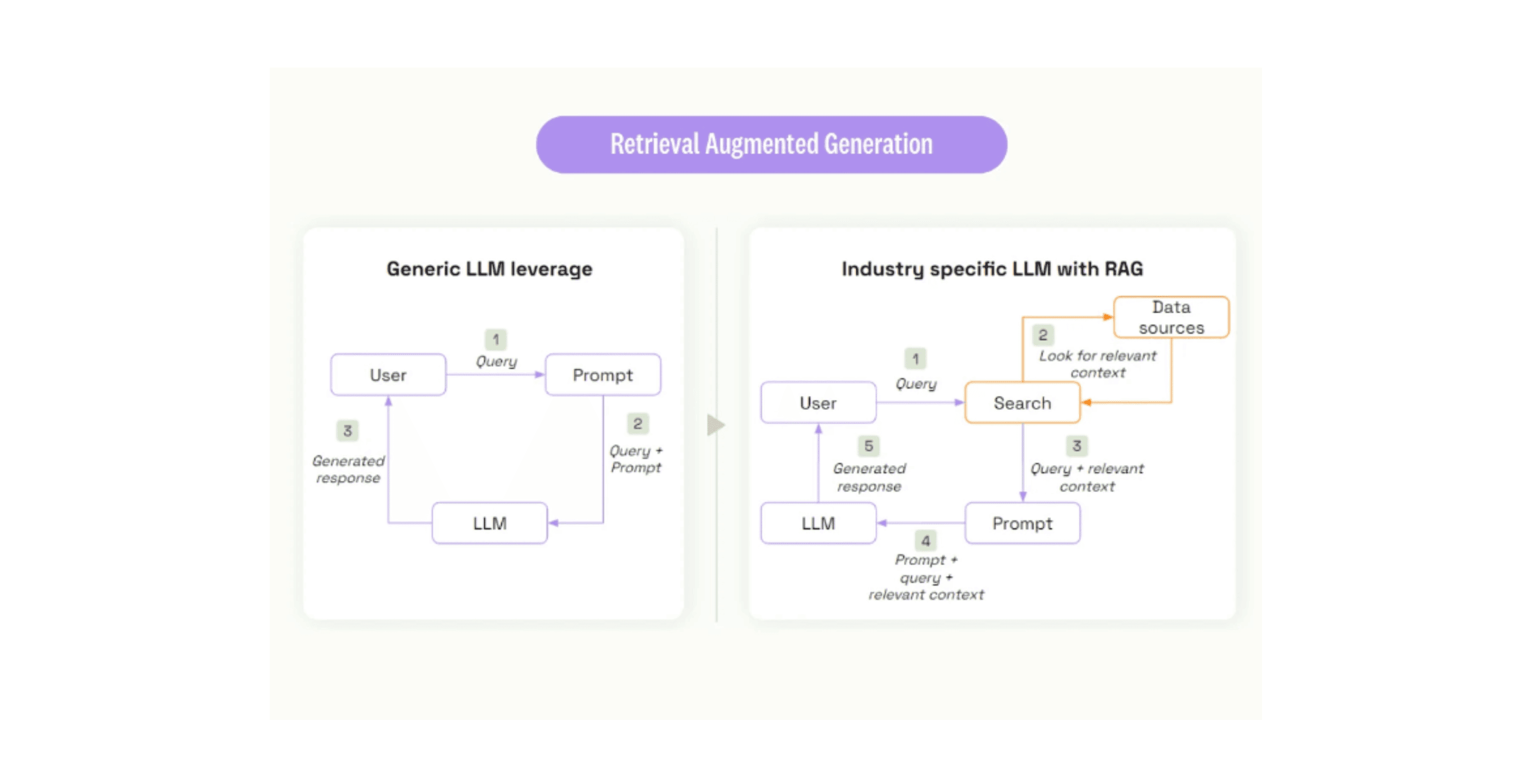
Source: Kili Technology
While LLMs are powerful, their generic training sometimes aligns differently with specific enterprise needs. For example, a model trained on broad data may need to improve in specialized tasks like legal document analysis or technical support for complex products with significant customization.
This misalignment can lead to inefficiencies or inaccuracies in task execution.
Read more on Customizing LLMs for Specific Tasks with RagaAI
High Accuracy Demands in Critical Domains like Banking and Healthcare
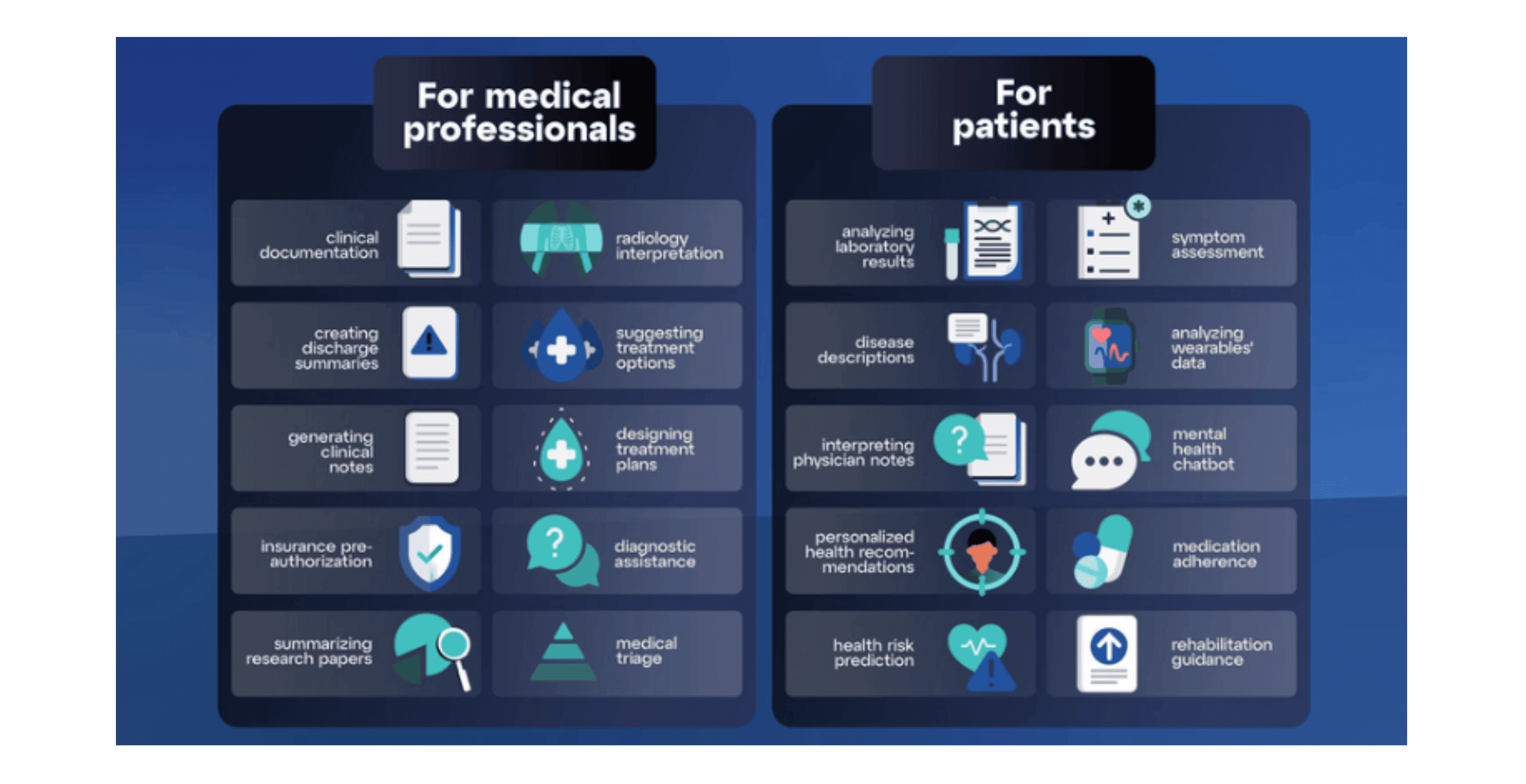
Source: Research Gate
In domains such as banking and healthcare, the stakes for accuracy are exceptionally high. An LLM that assists with diagnosing medical conditions must be precise and reliable, as misdiagnoses can have serious consequences.
Similarly, in banking, inaccuracies in fraud detection can lead to substantial financial losses or regulatory penalties. Ensuring these models meet or exceed human performance standards is a significant challenge.
Read more on Ensuring High Accuracy in Critical Domains with RagaAI
Integration Challenges and the Need for a Solid Data Foundation
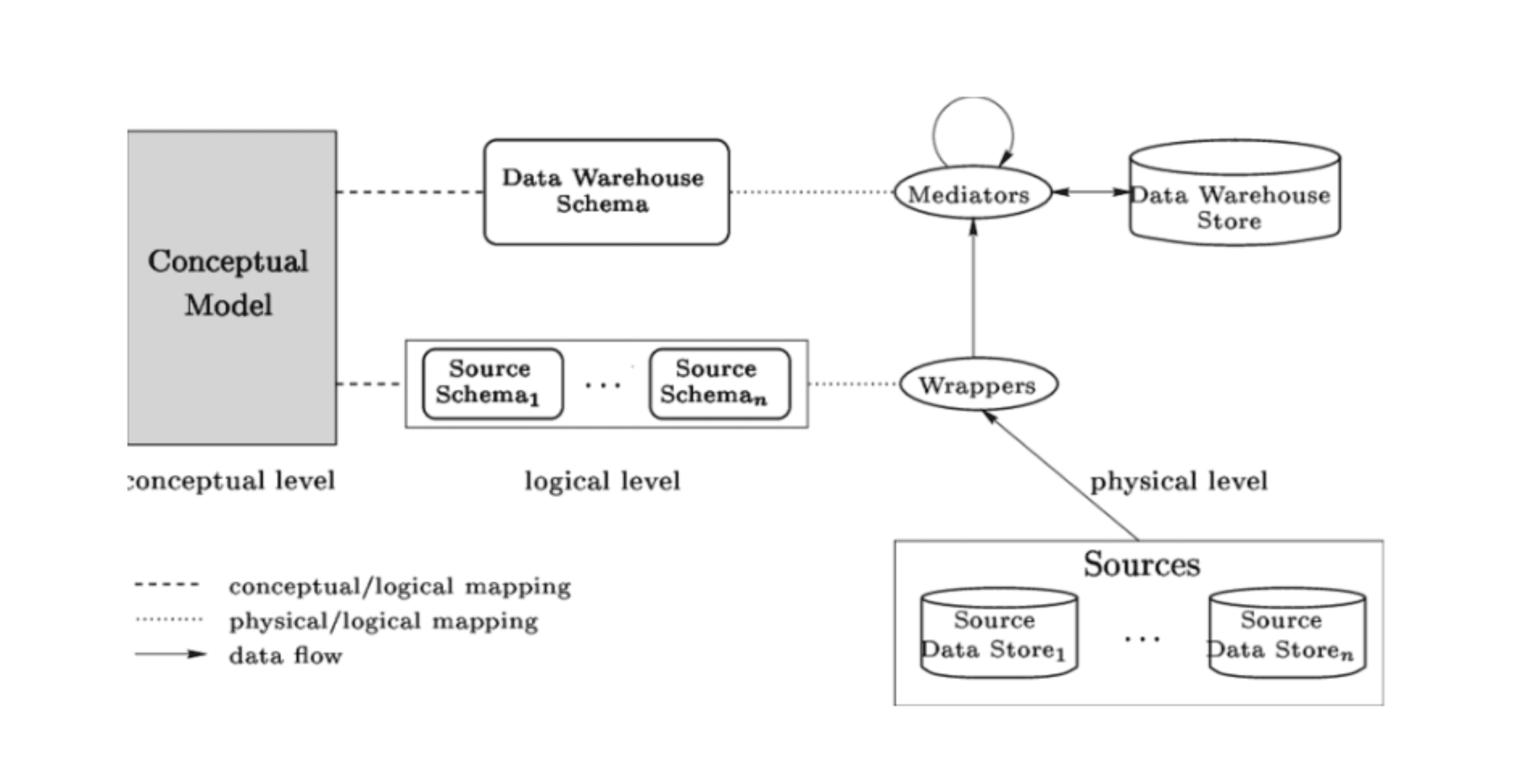
Source: Research Gate
Integrating LLMs into existing IT ecosystems can be daunting. These models often require substantial data inputs to function optimally, which means they must be seamlessly integrated with existing databases and IT infrastructure.
Moreover, the quality of outputs depends heavily on the quality of input data. Therefore, establishing a solid data foundation is critical to successfully deploying LLMs.
Security, Data Privacy, and the Risks of Data Leakage
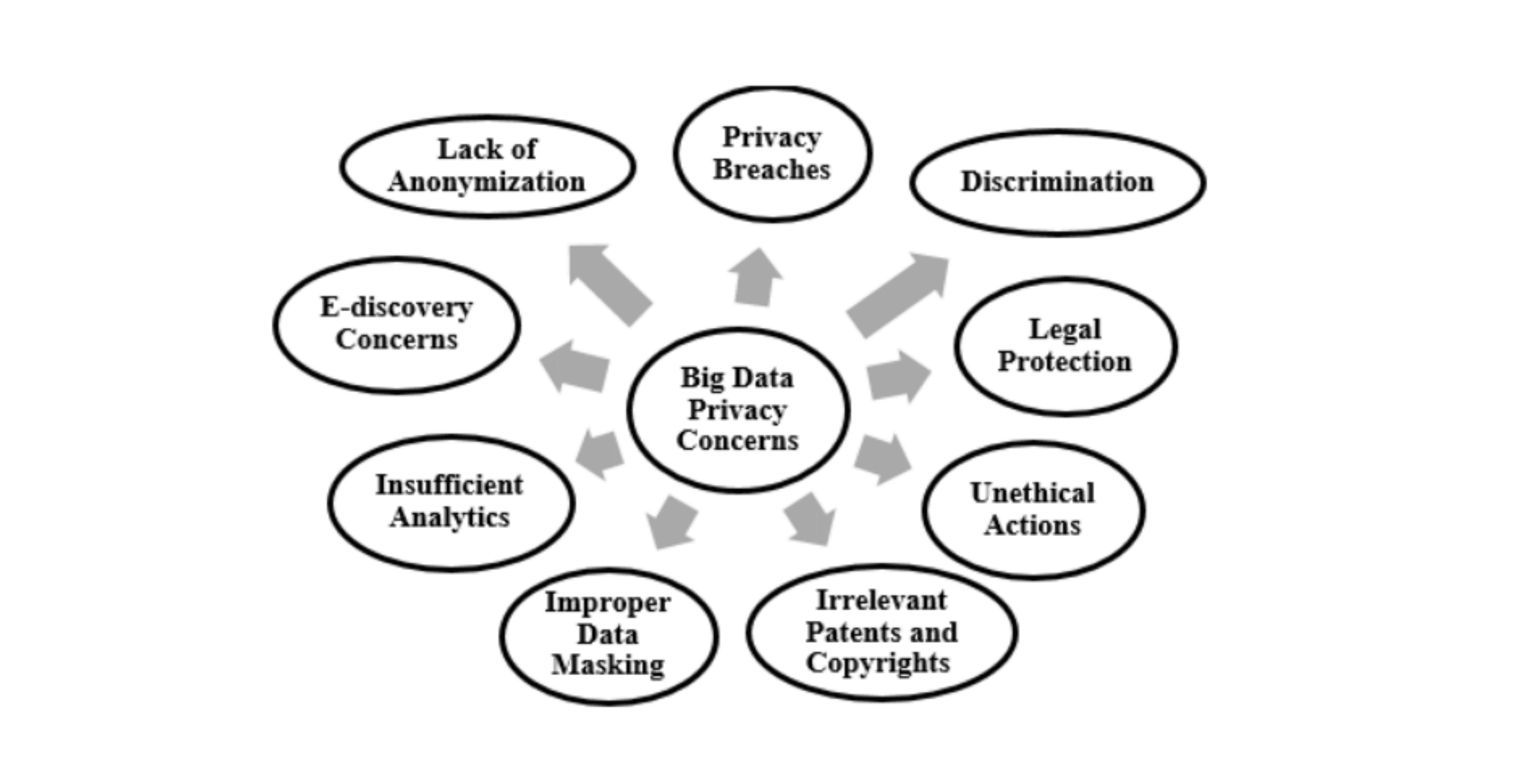
Source: Research Gate
Data security and privacy are major concerns since LLMs often process sensitive information. Ensuring these models comply with data protection regulations like GDPR and HIPAA is crucial.
The risk of data leakage through LLM interactions or training processes also poses a significant threat, necessitating robust security measures to protect data integrity and confidentiality.
These challenges underscore the complexity of deploying LLMs in an enterprise context, where the demands for accuracy, integration, and security are significantly heightened.
As we move forward, we'll explore strategies to overcome these hurdles and ensure that LLM implementations are practical, secure, and compliant with industry standards.
Learn about the Data Security Measures that Raga AI implements to protect sensitive information.
Strategies for Overcoming Challenges
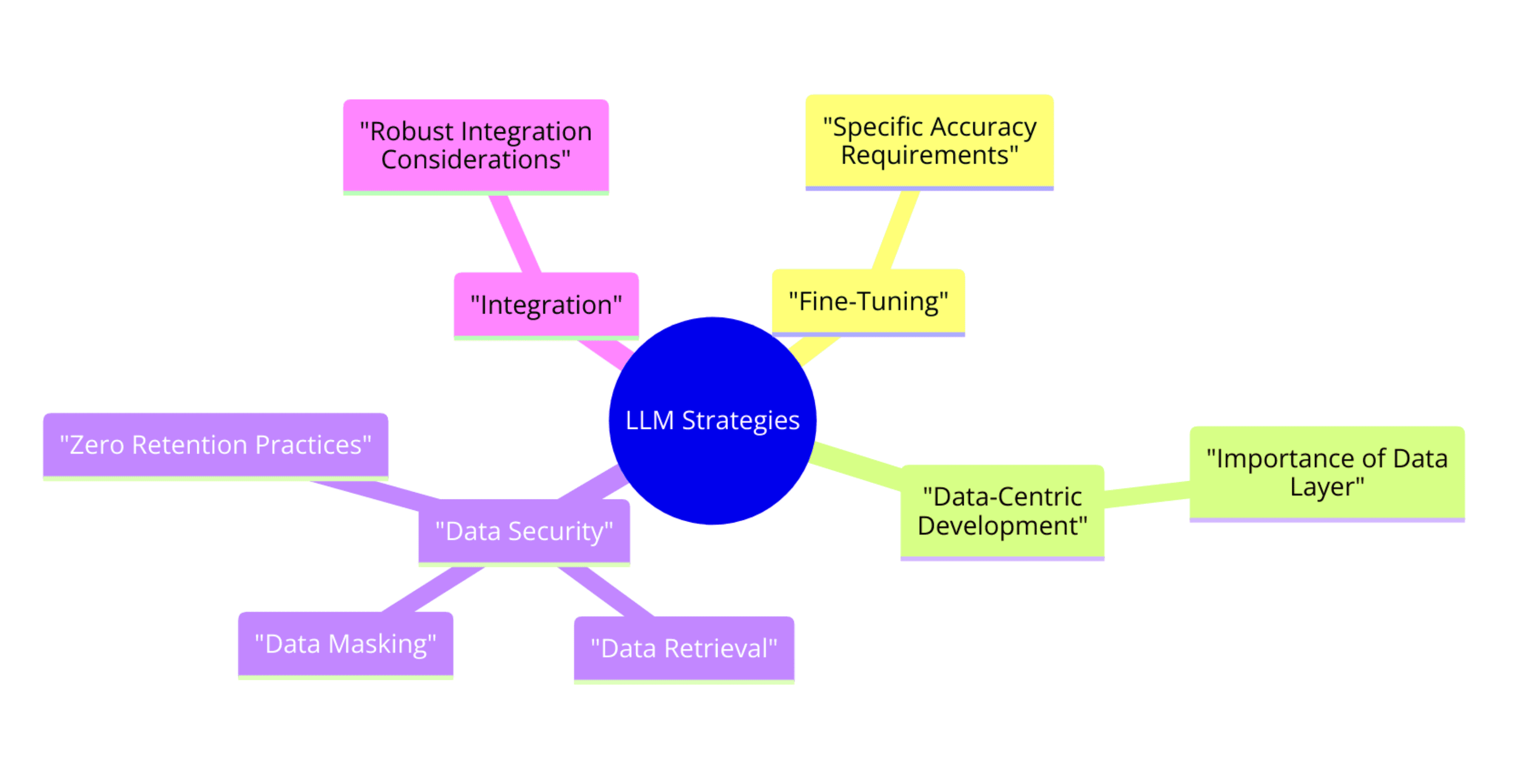
Successfully deploying LLMs in enterprise settings requires a comprehensive strategy that addresses each enterprise's unique challenges. Here are some effective strategies to consider:
Adapting LLMs to Enterprise Needs Through Fine-Tuning for Specific Accuracy Requirements
Fine-tuning the model with specific, high-quality data is essential to ensure that LLMs deliver the high accuracy needed for enterprise applications, particularly in sensitive areas like healthcare and finance.
This involves training the LLM on a dataset that closely mirrors the actual scenarios and data it will encounter in its operational environment.
Example: In healthcare, an LLM used for patient diagnosis can be fine-tuned with anonymized patient records and outcomes to improve its diagnostic accuracy, ensuring it learns from relevant, real-world medical cases.
Utilizing Data-Centric Development and the Importance of the Data Layer
A data-centric approach to LLM implementation focuses on improving the data quality used for training and operating the model rather than just tweaking the model architecture. Ensuring the robust data layer involves curating, cleaning, and continuously updating the dataset to reflect the latest information and contexts.
Example: For a banking LLM used in fraud detection, regularly updating the training dataset with the latest types of fraud cases and transaction profiles can help the model stay relevant and practical.
Securing Data Retrieval, Data Masking, and Zero Retention Practices
Security measures are critical to protect sensitive data used by LLMs. Data retrieval processes should be secure, employing encryption and secure access protocols. Data masking techniques can be used to anonymize sensitive information, ensuring that even if data is accessed inappropriately, it cannot be traced back to anyone.
Example: Implementing zero-knowledge proof systems where the LLM processes information but does not store any personally identifiable information (PII) can help maintain privacy and security.
Additional Considerations for Robust Integration
Another critical strategy is ensuring that LLMs are well-integrated into the existing technological infrastructure without disrupting current operations. This involves API integrations, middleware solutions, or custom adapters that allow LLMs to communicate seamlessly with existing databases and applications.
Example: Using API gateways to manage requests between enterprise applications and LLMs can help regulate data flow and ensure that only necessary information is shared, maintaining performance and security.
Customizing and Building Enterprise LLMs
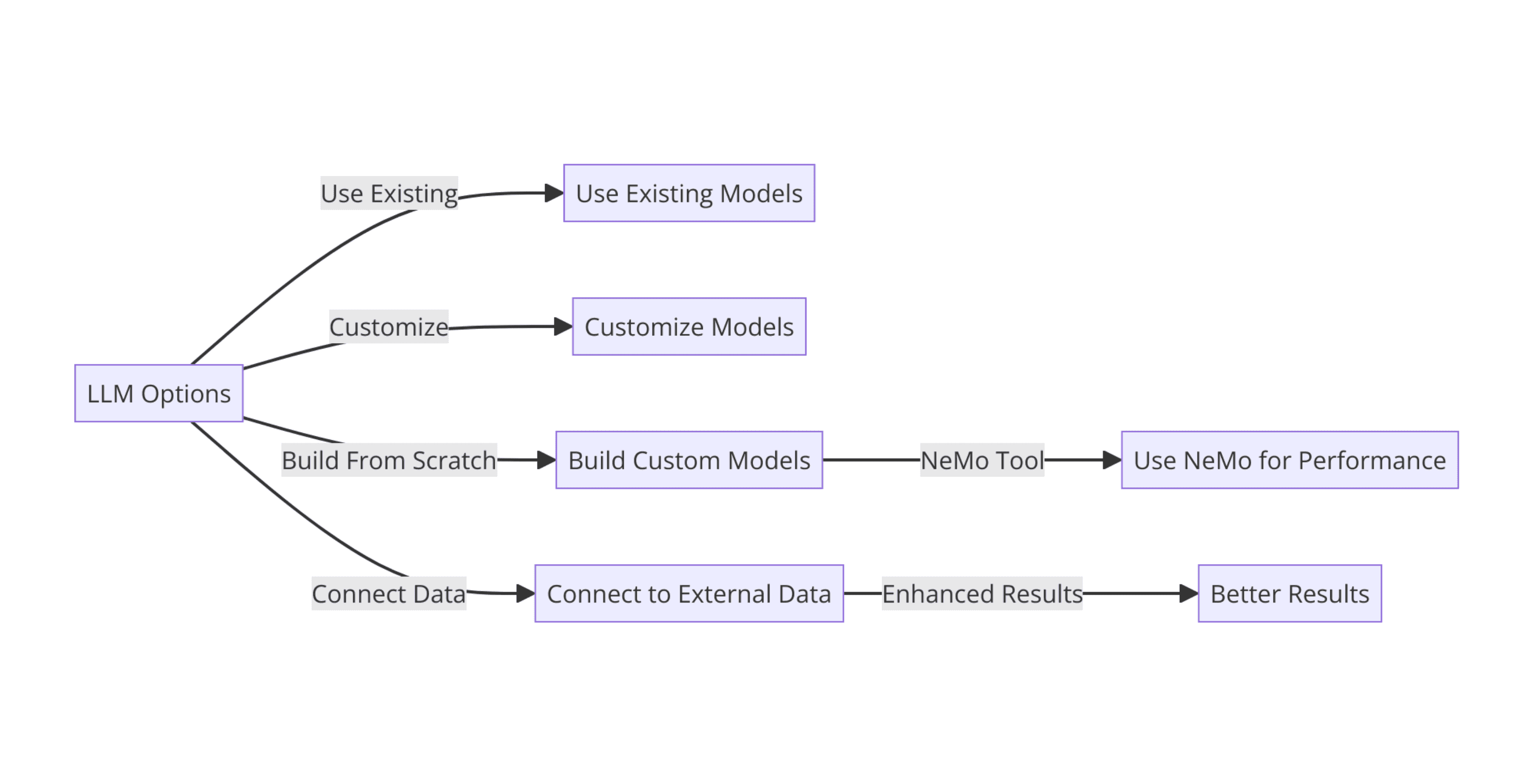
Customizing LLMs or building them from scratch offers enterprises the flexibility to address their unique challenges and objectives. Here’s how businesses can approach these tasks:
Options for Using, Customizing, or Building LLMs from Scratch
Enterprises have several paths to choose from when deploying LLMs: they can use off-the-shelf models, customize them to fit their needs or build entirely new models. Each option has its own set of benefits and considerations:
Using Standard Models: Quick deployment and initial cost savings.
Customizing Existing Models: Better performance in specific tasks while leveraging the foundational strengths of pre-trained models.
Building from Scratch: Complete control over model design, data used, and fine-tuning, but with higher costs and longer development time.
Building Custom Models with Tools like NeMo for Enhanced Performance
For those opting to build or customize models, tools like NVIDIA’s NeMo offer frameworks to create state-of-the-art conversational AI models. NeMo allows developers to fine-tune models on specific datasets, incorporate unique vocabularies, and adjust model architectures to suit particular tasks better.
Example: A telecom company could use NeMo to train a model specifically to understand and generate responses based on telecommunications jargon and customer service interactions, providing more accurate and contextually appropriate customer service.
Connecting an LLM to External Data for Better Results
Enhancing an LLM’s capabilities often involves integrating it with external data sources to enrich the context it has access to. Depending on the application, this might include customer databases, product catalogs, or real-time market data.
Example: An investment firm might integrate its LLM with real-time stock market data and historical investment databases to provide clients with more insightful, data-driven investment advice.
Technical Approach:
import openai
def integrate_external_data(model, data_sources):
enriched_context = ""
for source in data_sources:
enriched_context += extract_data(source)
response = openai.Completion.create(
engine=model,
prompt="Analyze the following market trends: " + enriched_context,
max_tokens=150 )
return response.choices[0].text
def extract_data(source):
# This is a placeholder for a function that fetches and formats data from a
source
return "Latest stock market trends from " + source
# Example of integrating an LLM with external data sources
model = "text-davinci-003"
data_sources = ["NASDAQ updates", "NYSE top movers"]
analysis = integrate_external_data(model, data_sources)
print("Market Analysis: ", analysis)
This example shows how an LLM can be enhanced by connecting it to relevant external data, providing more precise and valuable outputs based on the latest information.
Customizing and building enterprise-specific LLMs allows businesses to meet their unique needs more effectively and maintain a competitive edge by leveraging tailored AI solutions.
Now, let's explore the critical aspects of integrating Large Language Models (LLMs) into enterprise systems and ensuring their security.
This section will cover effectively merging LLMs into existing business processes and safeguarding sensitive information against potential threats.
Read more on Enhancing LLM Reliability with RagaAI
Integration and Security
Integrating LLMs into enterprise environments presents its own set of challenges, especially when it comes to ensuring that these systems can interact seamlessly with existing infrastructure and manage data securely.
Overcoming Integration Challenges for Personalized Customer Responses
Integrating LLMs requires a thoughtful approach to connect these models with existing customer relationship management (CRM) systems, databases, and other enterprise applications. This integration enables LLMs to access the necessary data for personalized responses and support.
Example: By linking an LLM to a CRM system, a business can enable the model to access customer purchase histories, support tickets, and preferences. This integration allows the LLM to deliver highly personalized customer service interactions, such as suggesting products based on past purchases or quickly resolving support issues by referring to previous tickets.
Strategies for Secure Data Retrieval and Protecting Sensitive Information
Ensuring the security of data accessed and generated by LLMs is paramount. Strategies to secure data include using encryption for data in transit and at rest, implementing robust access controls, and applying anonymization techniques where possible.
Technical Insight:
Encryption: Implement SSL/TLS for data transmitted between the LLM and other systems. Use strong encryption standards like AES-256 for data at rest.
Access Controls: Set up role-based access controls (RBAC) to ensure only authorized personnel can interact with the LLM and its data.
Read more on Addressing Integration Challenges with RagaAI
Monitoring and Aligning LLM Behavior with Enterprise Goals
Continuous monitoring of LLM activities is crucial to ensure they perform as intended and adhere to company policies and ethical guidelines. Setting up monitoring tools to track the performance and outputs of LLMs can help quickly identify and address any deviations from expected behaviors.
Example: Use logging and auditing tools to record all interactions with the LLM. Analyze logs regularly to detect any anomalies or unauthorized attempts to access the system. Implement automated alerts to notify the appropriate personnel when irregular patterns are detected.
import logging
# Setup logging configuration
logging.basicConfig(filename='llm_activity.log', level=logging.INFO, format='%(asctime)s:%(levelname)s:%(message)s')
def log_interaction(user_id, query, response):
logging.info(f"User ID: {user_id}, Query: {query}, Response: {response}")
# Example of logging an interaction
user_id = 12345
query = "What products are recommended for someone who bought X?"
response = "Based on previous purchases, we recommend Y and Z."
log_interaction(user_id, query, response)
This script provides a basic framework for logging interactions with an LLM, helping maintain a transparent record that can be audited for compliance and monitoring purposes.
Ensuring LLMs are Kept on Track and Secure Through Monitoring and Guardrails
Setting up continuous monitoring systems and defining clear operational guardrails are essential to maintaining the integrity and effectiveness of LLM deployments. Monitoring tools can track LLMs' performance and behavior, while guardrails ensure that the models operate within predefined ethical and operational boundaries.
Example Implementation:
def monitor_model_performance(metrics):
if metrics['accuracy'] < threshold:
alert("Model accuracy below expected threshold.")
if metrics['response_time'] > max_response_time:
optimize_model_inference()
def optimize_model_inference():
# Implement optimization logic (e.g., load balancing, resource allocation)
print("Optimizing model inference for better performance.")
# Simulated monitoring data
model_metrics = {'accuracy': 92.5, 'response_time': 0.300} # 300 ms
monitor_model_performance(model_metrics)
This example demonstrates an essential monitoring function that checks model performance against certain thresholds and triggers optimization procedures if needed.
Optimizing LLMs for enterprise applications involves technical adjustments and enhancements and ensuring these systems integrate smoothly with existing corporate infrastructure and policies. This holistic approach helps businesses harness the full power of LLMs while maintaining control over their deployment and usage.
Let's wrap up our comprehensive exploration of implementing Large Language Models (LLMs) in the enterprise context. We'll recap the importance of LLMs, highlight key strategies to overcome challenges, and reiterate the value of customization and data-centric approaches.
Conclusion: Embracing LLMs in Enterprise
Integrating and optimizing large language models (LLMs) within enterprise systems is challenging and rewarding.
As we've explored, LLMs offer tremendous potential to revolutionize various aspects of business operations, from enhancing customer interactions to streamlining complex analytical tasks.
Embracing LLMs requires a commitment to continuous learning and adaptation, but the rewards justify the effort.
As AI technology evolves, so will the opportunities for its application in business. Enterprises that stay ahead of these trends, continuously refine their approaches, and invest in cutting-edge solutions will solve complex challenges and gain a significant competitive advantage.
By understanding and addressing the unique challenges of implementing LLMs and by leveraging the right strategies and tools, enterprises can fully realize the potential of these advanced AI models.
Though filled with technical and strategic challenges, this journey opens up a world of possibilities for innovation and efficiency.
Integrating large language models (LLMs) into enterprise applications is becoming a game-changer for many large companies. Substantial investments are flowing into this area, and businesses are eager to harness the power of LLMs for various innovative applications.
From engaging customers in more human-like conversations to detecting fraud and even diagnosing medical conditions, the potential uses are as diverse as they are impactful.
As AI evolves, the corporate world increasingly recognizes the value of implementing advanced LLMs. Companies are investing heavily financially and in the resources needed to integrate these technologies into their operations.
This surge in investment is driven by the promise of significant returns through enhanced efficiencies, improved customer interactions, and new capabilities that were once thought impossible without human intervention.
Key Challenges in Enterprise LLM Implementation
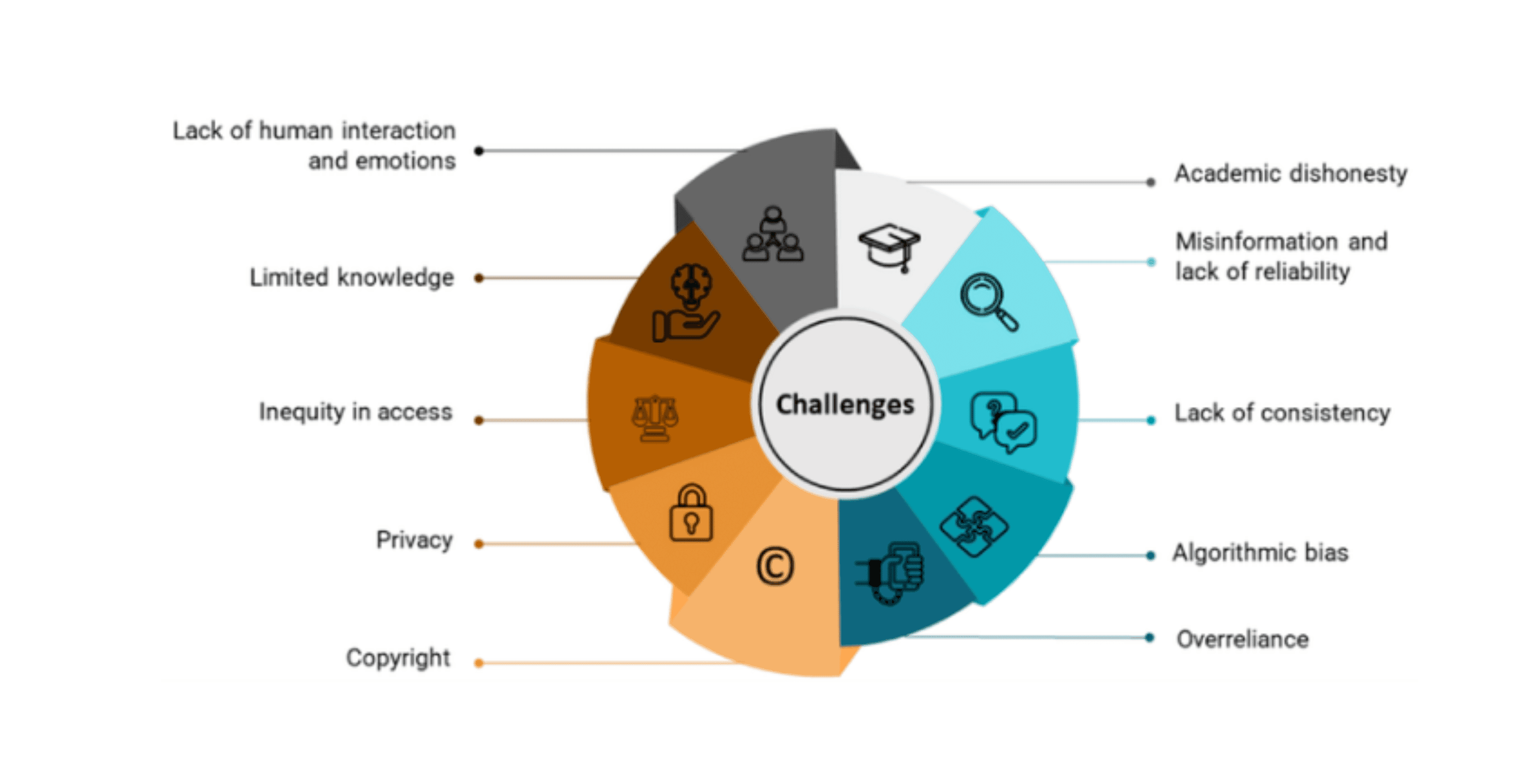
Source: Research Gate
Implementing LLMs in an enterprise setting comes with a set of unique challenges. These range from ensuring the models meet the high accuracy demands of specific applications to overcoming integration hurdles and securing sensitive data.
Generic LLM Limitations for Specific Enterprise Applications
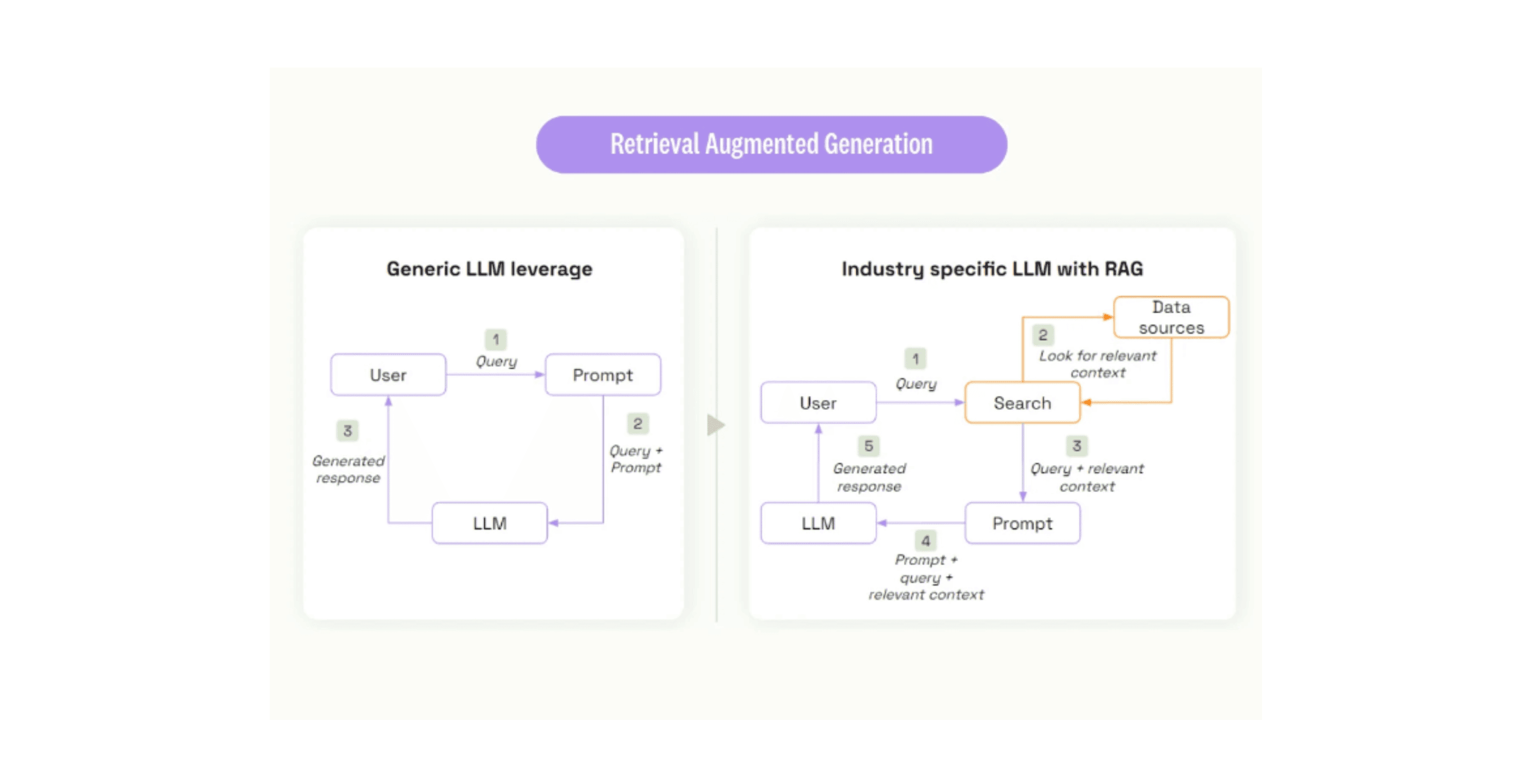
Source: Kili Technology
While LLMs are powerful, their generic training sometimes aligns differently with specific enterprise needs. For example, a model trained on broad data may need to improve in specialized tasks like legal document analysis or technical support for complex products with significant customization.
This misalignment can lead to inefficiencies or inaccuracies in task execution.
Read more on Customizing LLMs for Specific Tasks with RagaAI
High Accuracy Demands in Critical Domains like Banking and Healthcare
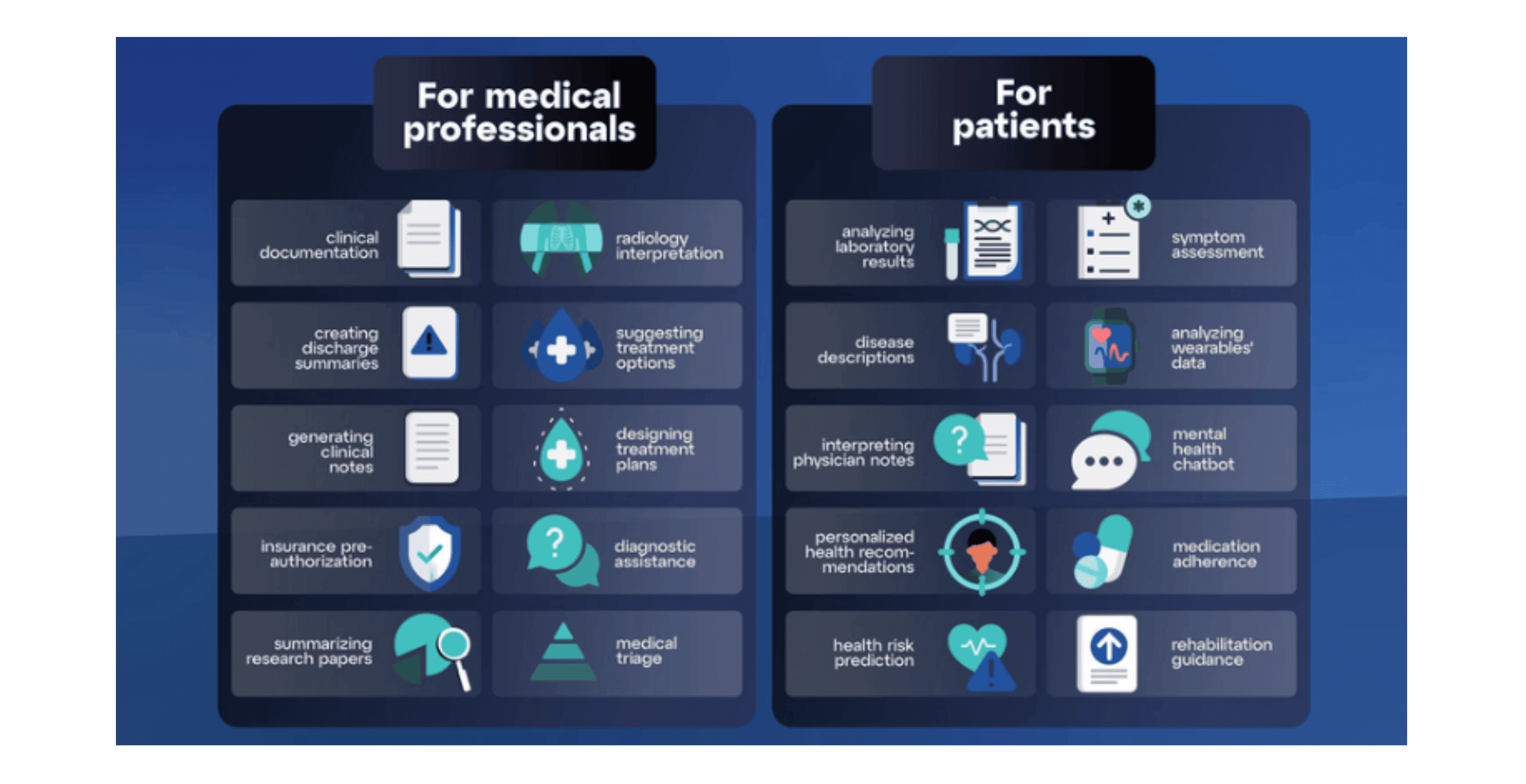
Source: Research Gate
In domains such as banking and healthcare, the stakes for accuracy are exceptionally high. An LLM that assists with diagnosing medical conditions must be precise and reliable, as misdiagnoses can have serious consequences.
Similarly, in banking, inaccuracies in fraud detection can lead to substantial financial losses or regulatory penalties. Ensuring these models meet or exceed human performance standards is a significant challenge.
Read more on Ensuring High Accuracy in Critical Domains with RagaAI
Integration Challenges and the Need for a Solid Data Foundation
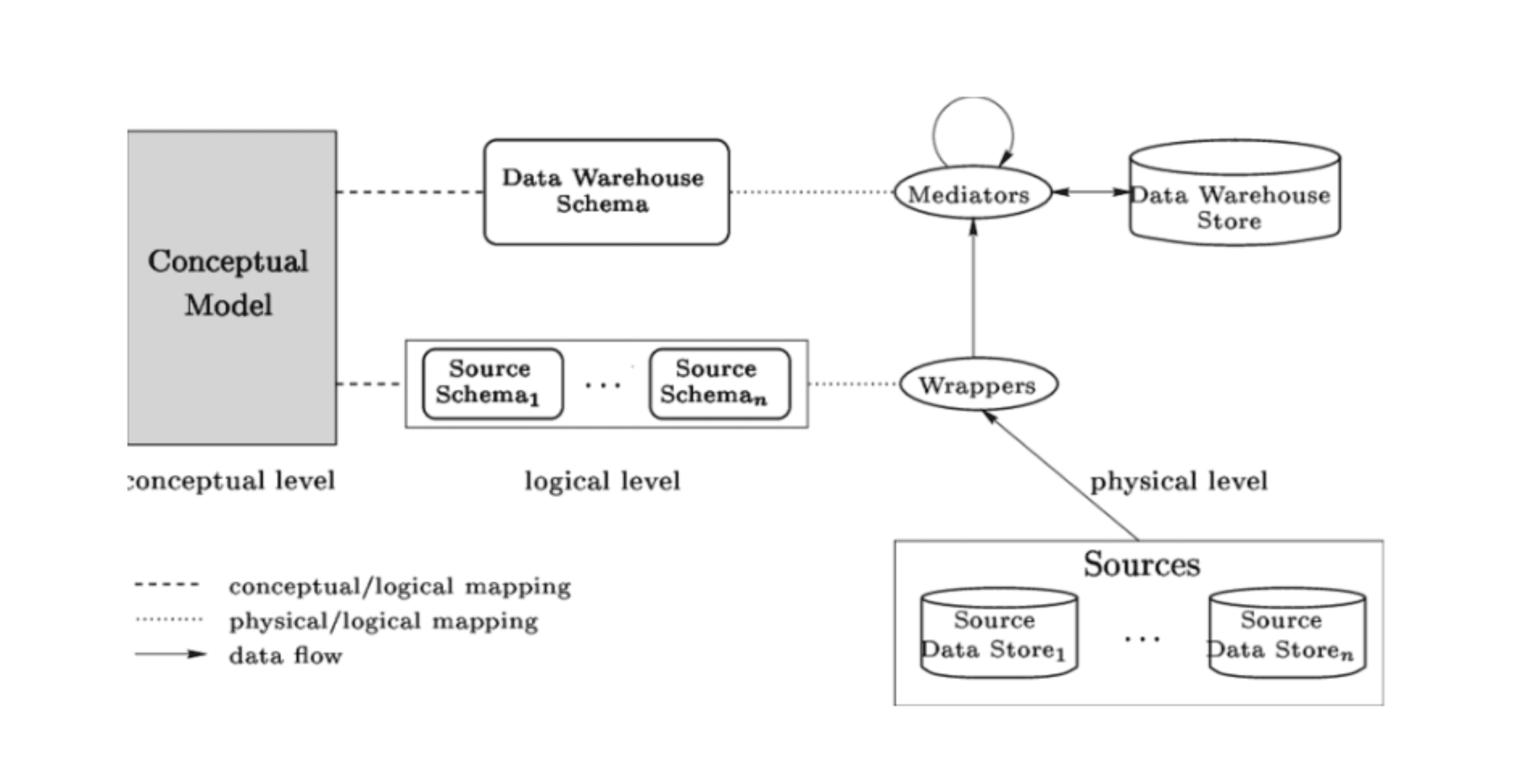
Source: Research Gate
Integrating LLMs into existing IT ecosystems can be daunting. These models often require substantial data inputs to function optimally, which means they must be seamlessly integrated with existing databases and IT infrastructure.
Moreover, the quality of outputs depends heavily on the quality of input data. Therefore, establishing a solid data foundation is critical to successfully deploying LLMs.
Security, Data Privacy, and the Risks of Data Leakage
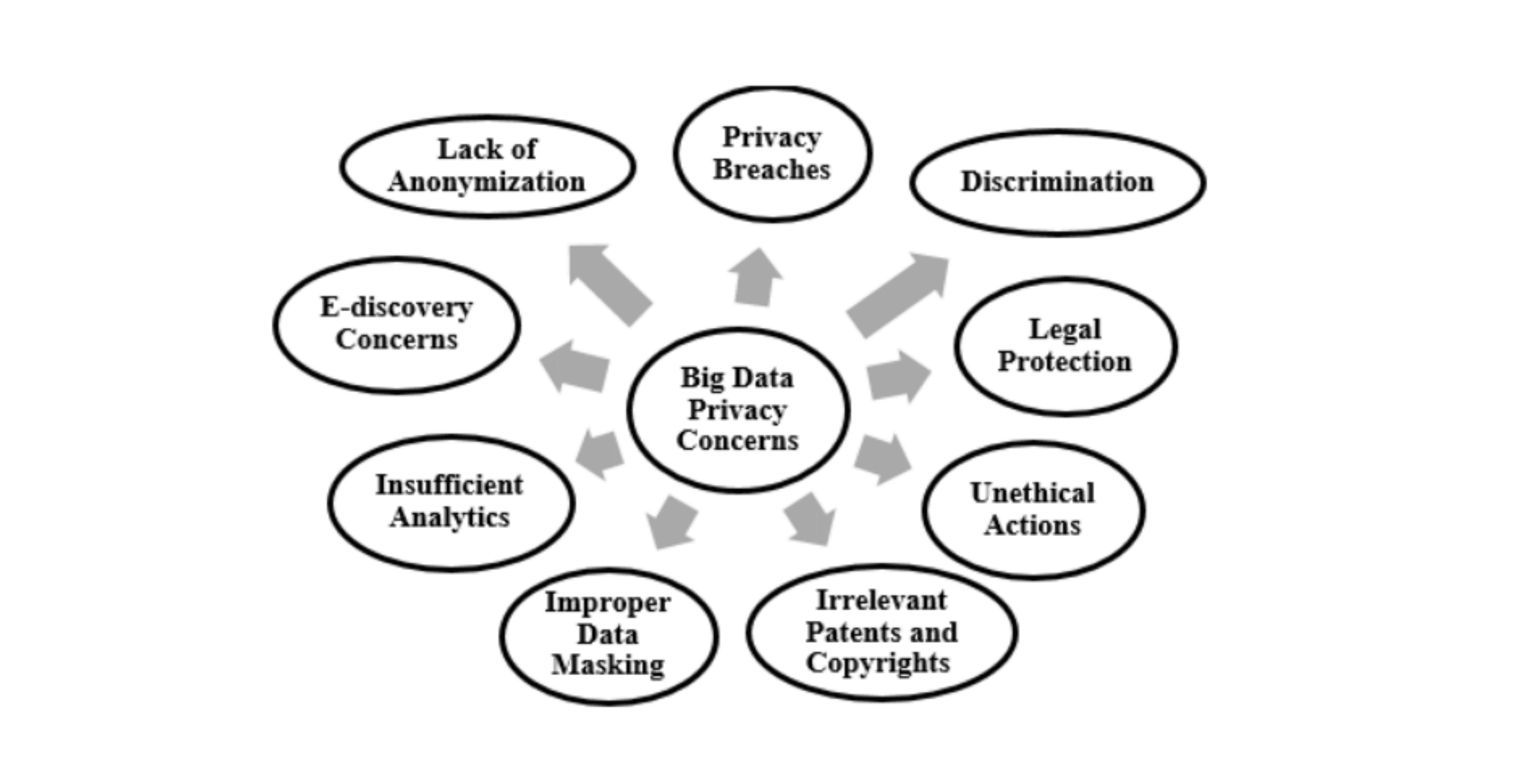
Source: Research Gate
Data security and privacy are major concerns since LLMs often process sensitive information. Ensuring these models comply with data protection regulations like GDPR and HIPAA is crucial.
The risk of data leakage through LLM interactions or training processes also poses a significant threat, necessitating robust security measures to protect data integrity and confidentiality.
These challenges underscore the complexity of deploying LLMs in an enterprise context, where the demands for accuracy, integration, and security are significantly heightened.
As we move forward, we'll explore strategies to overcome these hurdles and ensure that LLM implementations are practical, secure, and compliant with industry standards.
Learn about the Data Security Measures that Raga AI implements to protect sensitive information.
Strategies for Overcoming Challenges
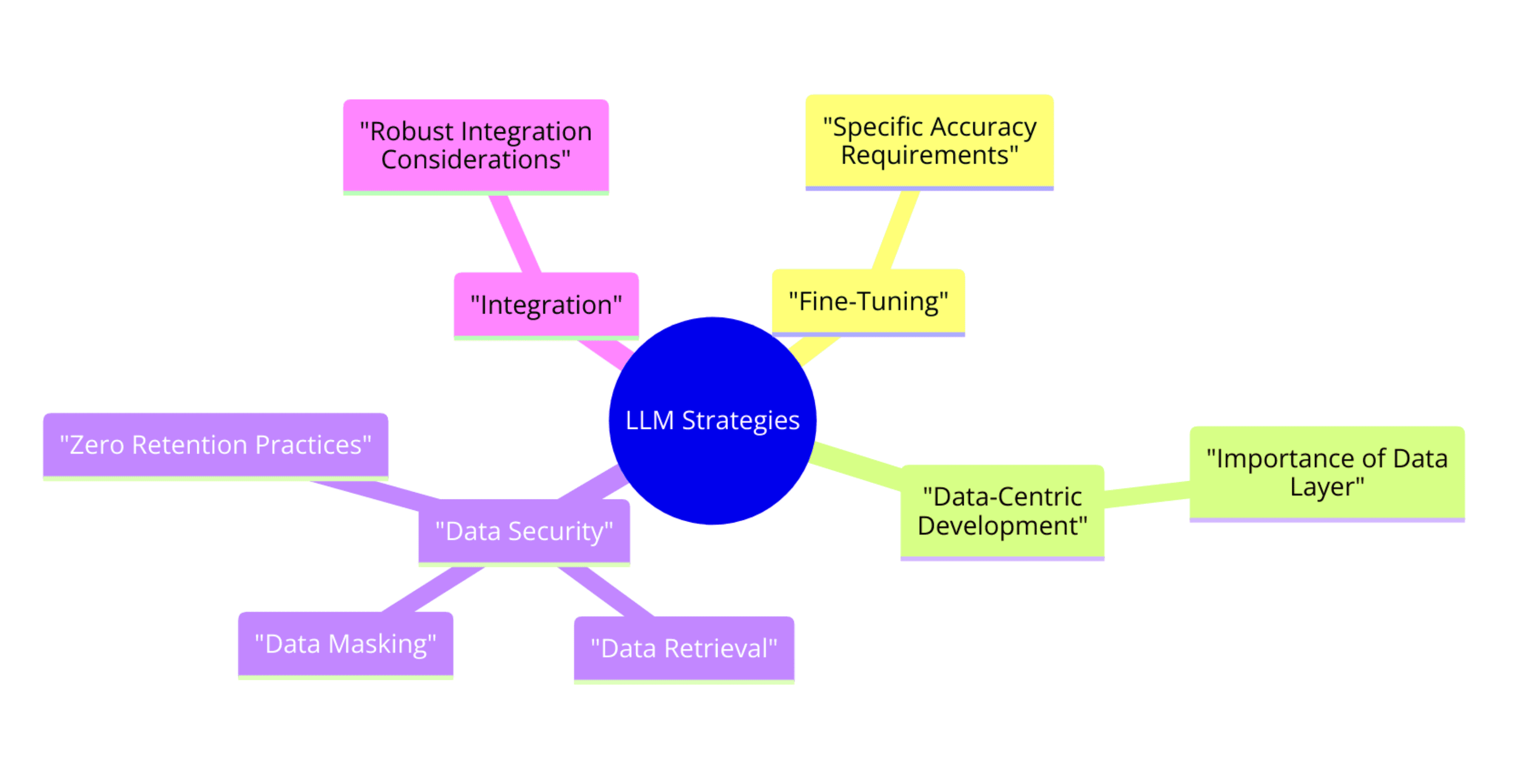
Successfully deploying LLMs in enterprise settings requires a comprehensive strategy that addresses each enterprise's unique challenges. Here are some effective strategies to consider:
Adapting LLMs to Enterprise Needs Through Fine-Tuning for Specific Accuracy Requirements
Fine-tuning the model with specific, high-quality data is essential to ensure that LLMs deliver the high accuracy needed for enterprise applications, particularly in sensitive areas like healthcare and finance.
This involves training the LLM on a dataset that closely mirrors the actual scenarios and data it will encounter in its operational environment.
Example: In healthcare, an LLM used for patient diagnosis can be fine-tuned with anonymized patient records and outcomes to improve its diagnostic accuracy, ensuring it learns from relevant, real-world medical cases.
Utilizing Data-Centric Development and the Importance of the Data Layer
A data-centric approach to LLM implementation focuses on improving the data quality used for training and operating the model rather than just tweaking the model architecture. Ensuring the robust data layer involves curating, cleaning, and continuously updating the dataset to reflect the latest information and contexts.
Example: For a banking LLM used in fraud detection, regularly updating the training dataset with the latest types of fraud cases and transaction profiles can help the model stay relevant and practical.
Securing Data Retrieval, Data Masking, and Zero Retention Practices
Security measures are critical to protect sensitive data used by LLMs. Data retrieval processes should be secure, employing encryption and secure access protocols. Data masking techniques can be used to anonymize sensitive information, ensuring that even if data is accessed inappropriately, it cannot be traced back to anyone.
Example: Implementing zero-knowledge proof systems where the LLM processes information but does not store any personally identifiable information (PII) can help maintain privacy and security.
Additional Considerations for Robust Integration
Another critical strategy is ensuring that LLMs are well-integrated into the existing technological infrastructure without disrupting current operations. This involves API integrations, middleware solutions, or custom adapters that allow LLMs to communicate seamlessly with existing databases and applications.
Example: Using API gateways to manage requests between enterprise applications and LLMs can help regulate data flow and ensure that only necessary information is shared, maintaining performance and security.
Customizing and Building Enterprise LLMs
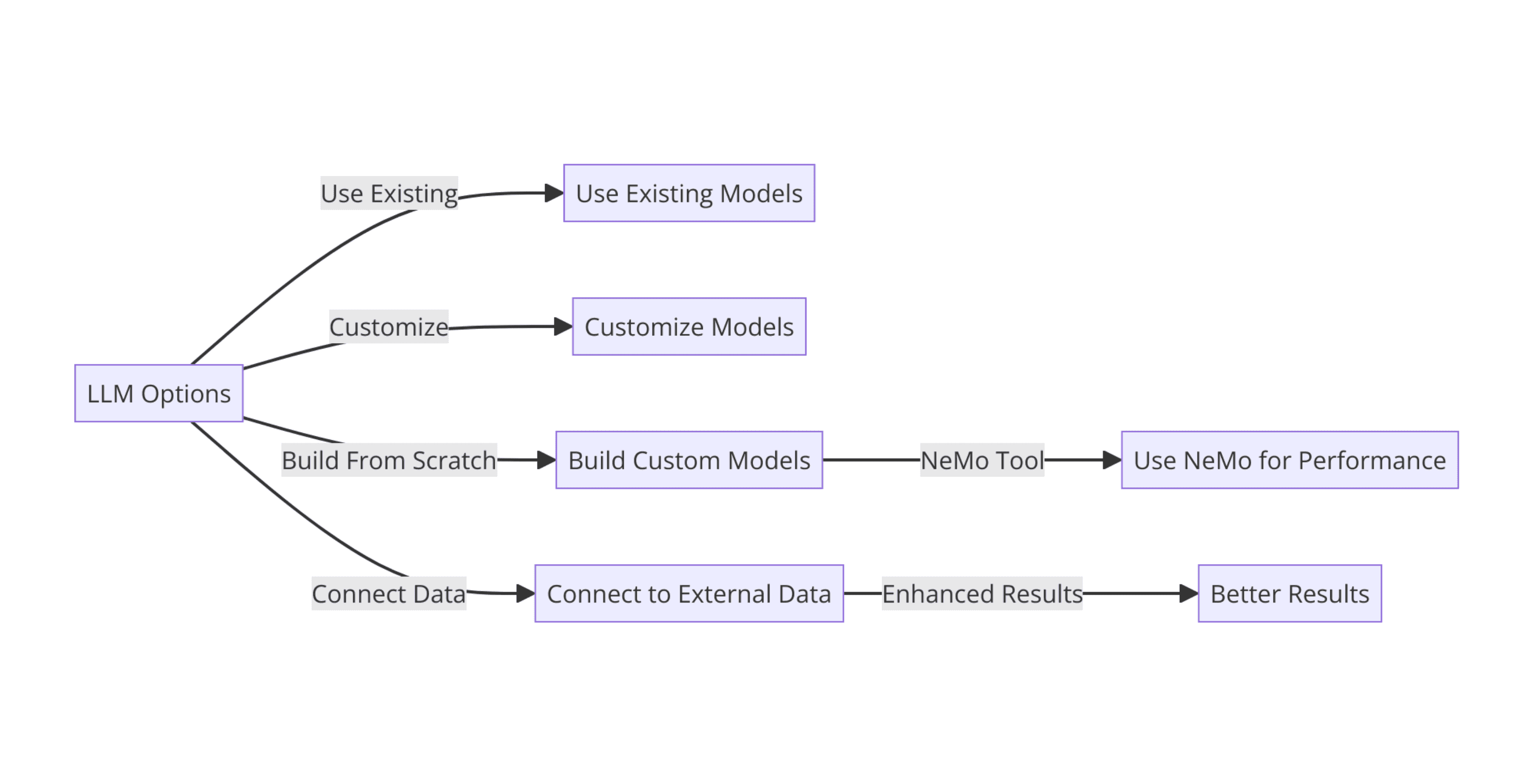
Customizing LLMs or building them from scratch offers enterprises the flexibility to address their unique challenges and objectives. Here’s how businesses can approach these tasks:
Options for Using, Customizing, or Building LLMs from Scratch
Enterprises have several paths to choose from when deploying LLMs: they can use off-the-shelf models, customize them to fit their needs or build entirely new models. Each option has its own set of benefits and considerations:
Using Standard Models: Quick deployment and initial cost savings.
Customizing Existing Models: Better performance in specific tasks while leveraging the foundational strengths of pre-trained models.
Building from Scratch: Complete control over model design, data used, and fine-tuning, but with higher costs and longer development time.
Building Custom Models with Tools like NeMo for Enhanced Performance
For those opting to build or customize models, tools like NVIDIA’s NeMo offer frameworks to create state-of-the-art conversational AI models. NeMo allows developers to fine-tune models on specific datasets, incorporate unique vocabularies, and adjust model architectures to suit particular tasks better.
Example: A telecom company could use NeMo to train a model specifically to understand and generate responses based on telecommunications jargon and customer service interactions, providing more accurate and contextually appropriate customer service.
Connecting an LLM to External Data for Better Results
Enhancing an LLM’s capabilities often involves integrating it with external data sources to enrich the context it has access to. Depending on the application, this might include customer databases, product catalogs, or real-time market data.
Example: An investment firm might integrate its LLM with real-time stock market data and historical investment databases to provide clients with more insightful, data-driven investment advice.
Technical Approach:
import openai
def integrate_external_data(model, data_sources):
enriched_context = ""
for source in data_sources:
enriched_context += extract_data(source)
response = openai.Completion.create(
engine=model,
prompt="Analyze the following market trends: " + enriched_context,
max_tokens=150 )
return response.choices[0].text
def extract_data(source):
# This is a placeholder for a function that fetches and formats data from a
source
return "Latest stock market trends from " + source
# Example of integrating an LLM with external data sources
model = "text-davinci-003"
data_sources = ["NASDAQ updates", "NYSE top movers"]
analysis = integrate_external_data(model, data_sources)
print("Market Analysis: ", analysis)
This example shows how an LLM can be enhanced by connecting it to relevant external data, providing more precise and valuable outputs based on the latest information.
Customizing and building enterprise-specific LLMs allows businesses to meet their unique needs more effectively and maintain a competitive edge by leveraging tailored AI solutions.
Now, let's explore the critical aspects of integrating Large Language Models (LLMs) into enterprise systems and ensuring their security.
This section will cover effectively merging LLMs into existing business processes and safeguarding sensitive information against potential threats.
Read more on Enhancing LLM Reliability with RagaAI
Integration and Security
Integrating LLMs into enterprise environments presents its own set of challenges, especially when it comes to ensuring that these systems can interact seamlessly with existing infrastructure and manage data securely.
Overcoming Integration Challenges for Personalized Customer Responses
Integrating LLMs requires a thoughtful approach to connect these models with existing customer relationship management (CRM) systems, databases, and other enterprise applications. This integration enables LLMs to access the necessary data for personalized responses and support.
Example: By linking an LLM to a CRM system, a business can enable the model to access customer purchase histories, support tickets, and preferences. This integration allows the LLM to deliver highly personalized customer service interactions, such as suggesting products based on past purchases or quickly resolving support issues by referring to previous tickets.
Strategies for Secure Data Retrieval and Protecting Sensitive Information
Ensuring the security of data accessed and generated by LLMs is paramount. Strategies to secure data include using encryption for data in transit and at rest, implementing robust access controls, and applying anonymization techniques where possible.
Technical Insight:
Encryption: Implement SSL/TLS for data transmitted between the LLM and other systems. Use strong encryption standards like AES-256 for data at rest.
Access Controls: Set up role-based access controls (RBAC) to ensure only authorized personnel can interact with the LLM and its data.
Read more on Addressing Integration Challenges with RagaAI
Monitoring and Aligning LLM Behavior with Enterprise Goals
Continuous monitoring of LLM activities is crucial to ensure they perform as intended and adhere to company policies and ethical guidelines. Setting up monitoring tools to track the performance and outputs of LLMs can help quickly identify and address any deviations from expected behaviors.
Example: Use logging and auditing tools to record all interactions with the LLM. Analyze logs regularly to detect any anomalies or unauthorized attempts to access the system. Implement automated alerts to notify the appropriate personnel when irregular patterns are detected.
import logging
# Setup logging configuration
logging.basicConfig(filename='llm_activity.log', level=logging.INFO, format='%(asctime)s:%(levelname)s:%(message)s')
def log_interaction(user_id, query, response):
logging.info(f"User ID: {user_id}, Query: {query}, Response: {response}")
# Example of logging an interaction
user_id = 12345
query = "What products are recommended for someone who bought X?"
response = "Based on previous purchases, we recommend Y and Z."
log_interaction(user_id, query, response)
This script provides a basic framework for logging interactions with an LLM, helping maintain a transparent record that can be audited for compliance and monitoring purposes.
Ensuring LLMs are Kept on Track and Secure Through Monitoring and Guardrails
Setting up continuous monitoring systems and defining clear operational guardrails are essential to maintaining the integrity and effectiveness of LLM deployments. Monitoring tools can track LLMs' performance and behavior, while guardrails ensure that the models operate within predefined ethical and operational boundaries.
Example Implementation:
def monitor_model_performance(metrics):
if metrics['accuracy'] < threshold:
alert("Model accuracy below expected threshold.")
if metrics['response_time'] > max_response_time:
optimize_model_inference()
def optimize_model_inference():
# Implement optimization logic (e.g., load balancing, resource allocation)
print("Optimizing model inference for better performance.")
# Simulated monitoring data
model_metrics = {'accuracy': 92.5, 'response_time': 0.300} # 300 ms
monitor_model_performance(model_metrics)
This example demonstrates an essential monitoring function that checks model performance against certain thresholds and triggers optimization procedures if needed.
Optimizing LLMs for enterprise applications involves technical adjustments and enhancements and ensuring these systems integrate smoothly with existing corporate infrastructure and policies. This holistic approach helps businesses harness the full power of LLMs while maintaining control over their deployment and usage.
Let's wrap up our comprehensive exploration of implementing Large Language Models (LLMs) in the enterprise context. We'll recap the importance of LLMs, highlight key strategies to overcome challenges, and reiterate the value of customization and data-centric approaches.
Conclusion: Embracing LLMs in Enterprise
Integrating and optimizing large language models (LLMs) within enterprise systems is challenging and rewarding.
As we've explored, LLMs offer tremendous potential to revolutionize various aspects of business operations, from enhancing customer interactions to streamlining complex analytical tasks.
Embracing LLMs requires a commitment to continuous learning and adaptation, but the rewards justify the effort.
As AI technology evolves, so will the opportunities for its application in business. Enterprises that stay ahead of these trends, continuously refine their approaches, and invest in cutting-edge solutions will solve complex challenges and gain a significant competitive advantage.
By understanding and addressing the unique challenges of implementing LLMs and by leveraging the right strategies and tools, enterprises can fully realize the potential of these advanced AI models.
Though filled with technical and strategic challenges, this journey opens up a world of possibilities for innovation and efficiency.
Integrating large language models (LLMs) into enterprise applications is becoming a game-changer for many large companies. Substantial investments are flowing into this area, and businesses are eager to harness the power of LLMs for various innovative applications.
From engaging customers in more human-like conversations to detecting fraud and even diagnosing medical conditions, the potential uses are as diverse as they are impactful.
As AI evolves, the corporate world increasingly recognizes the value of implementing advanced LLMs. Companies are investing heavily financially and in the resources needed to integrate these technologies into their operations.
This surge in investment is driven by the promise of significant returns through enhanced efficiencies, improved customer interactions, and new capabilities that were once thought impossible without human intervention.
Key Challenges in Enterprise LLM Implementation
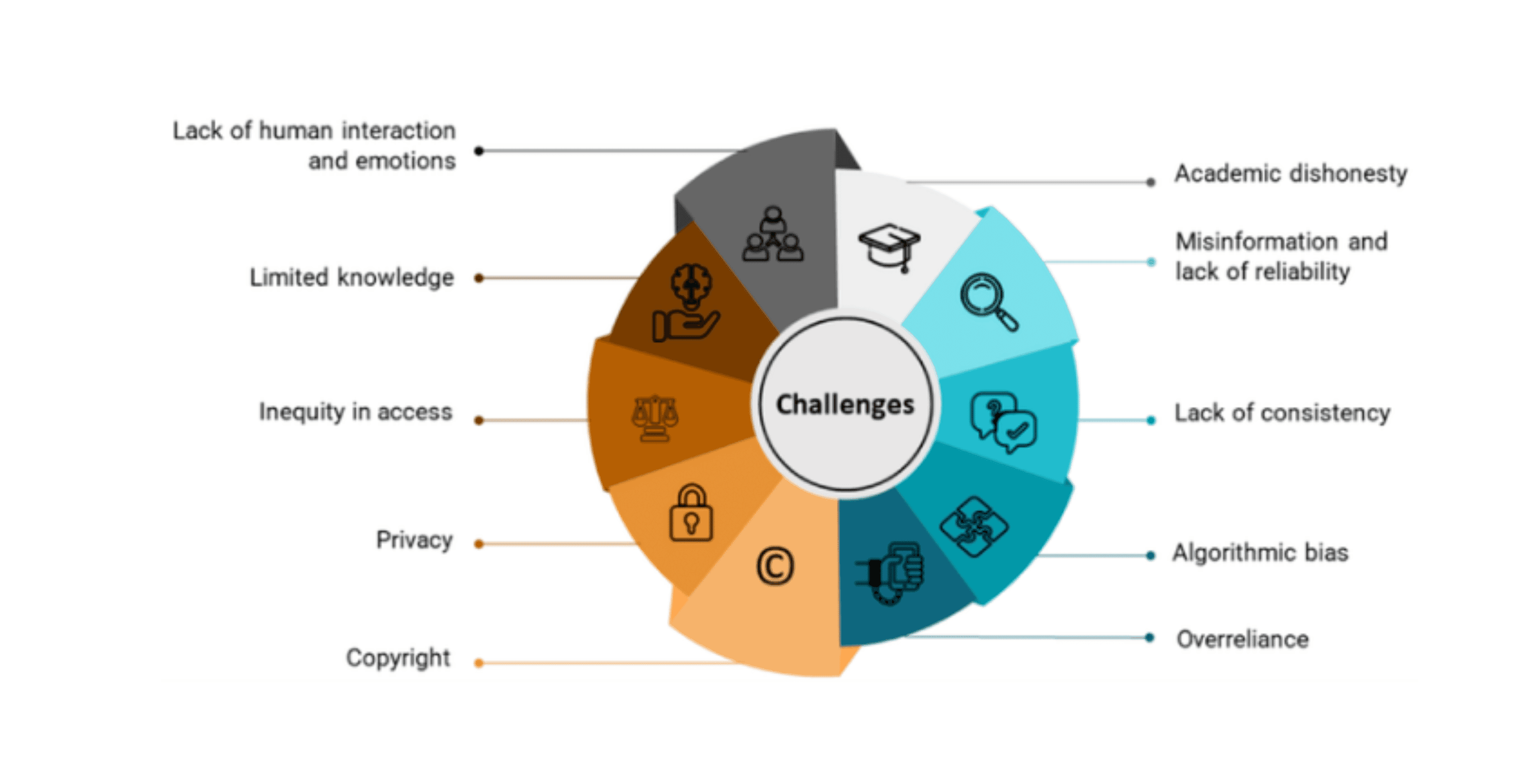
Source: Research Gate
Implementing LLMs in an enterprise setting comes with a set of unique challenges. These range from ensuring the models meet the high accuracy demands of specific applications to overcoming integration hurdles and securing sensitive data.
Generic LLM Limitations for Specific Enterprise Applications
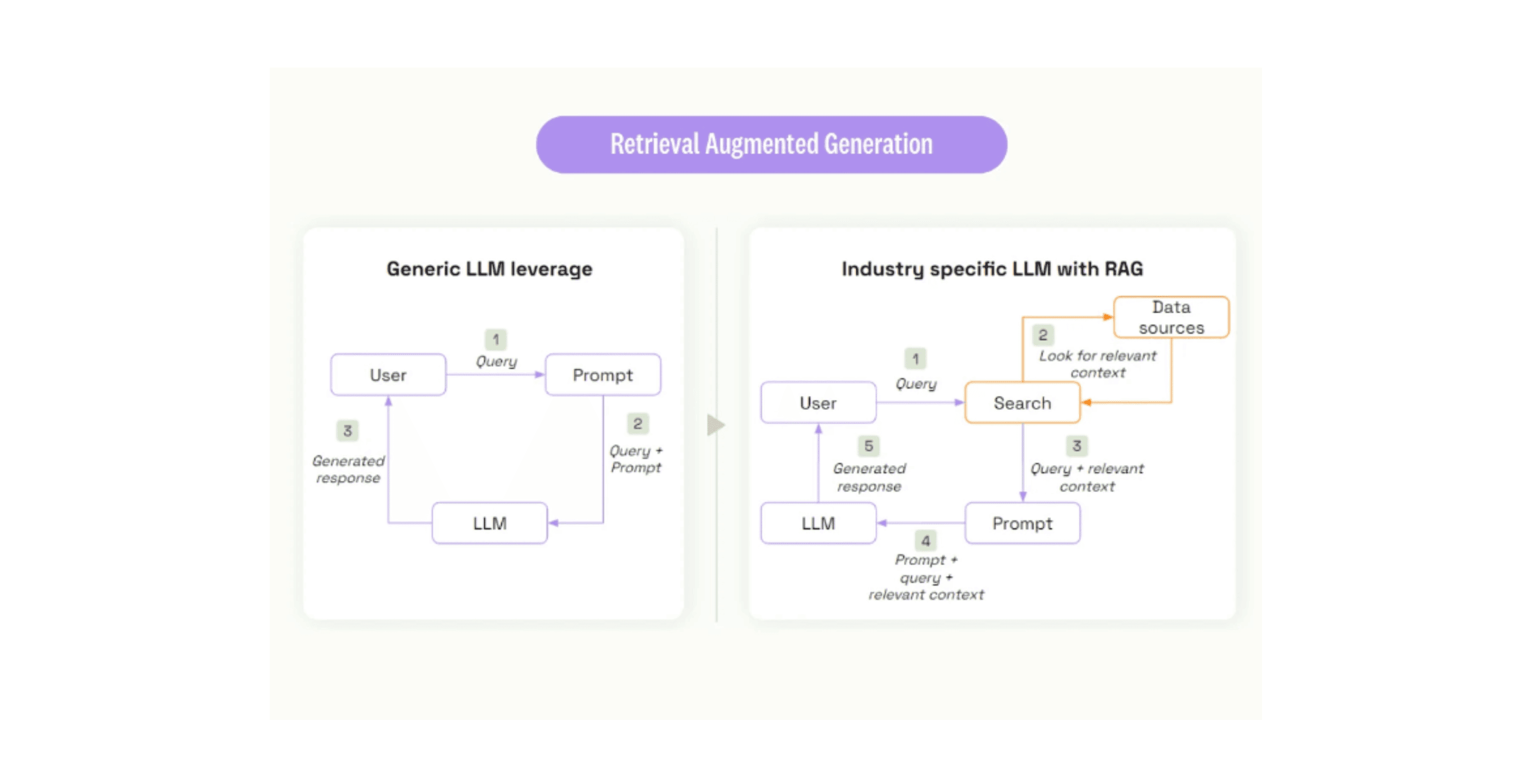
Source: Kili Technology
While LLMs are powerful, their generic training sometimes aligns differently with specific enterprise needs. For example, a model trained on broad data may need to improve in specialized tasks like legal document analysis or technical support for complex products with significant customization.
This misalignment can lead to inefficiencies or inaccuracies in task execution.
Read more on Customizing LLMs for Specific Tasks with RagaAI
High Accuracy Demands in Critical Domains like Banking and Healthcare
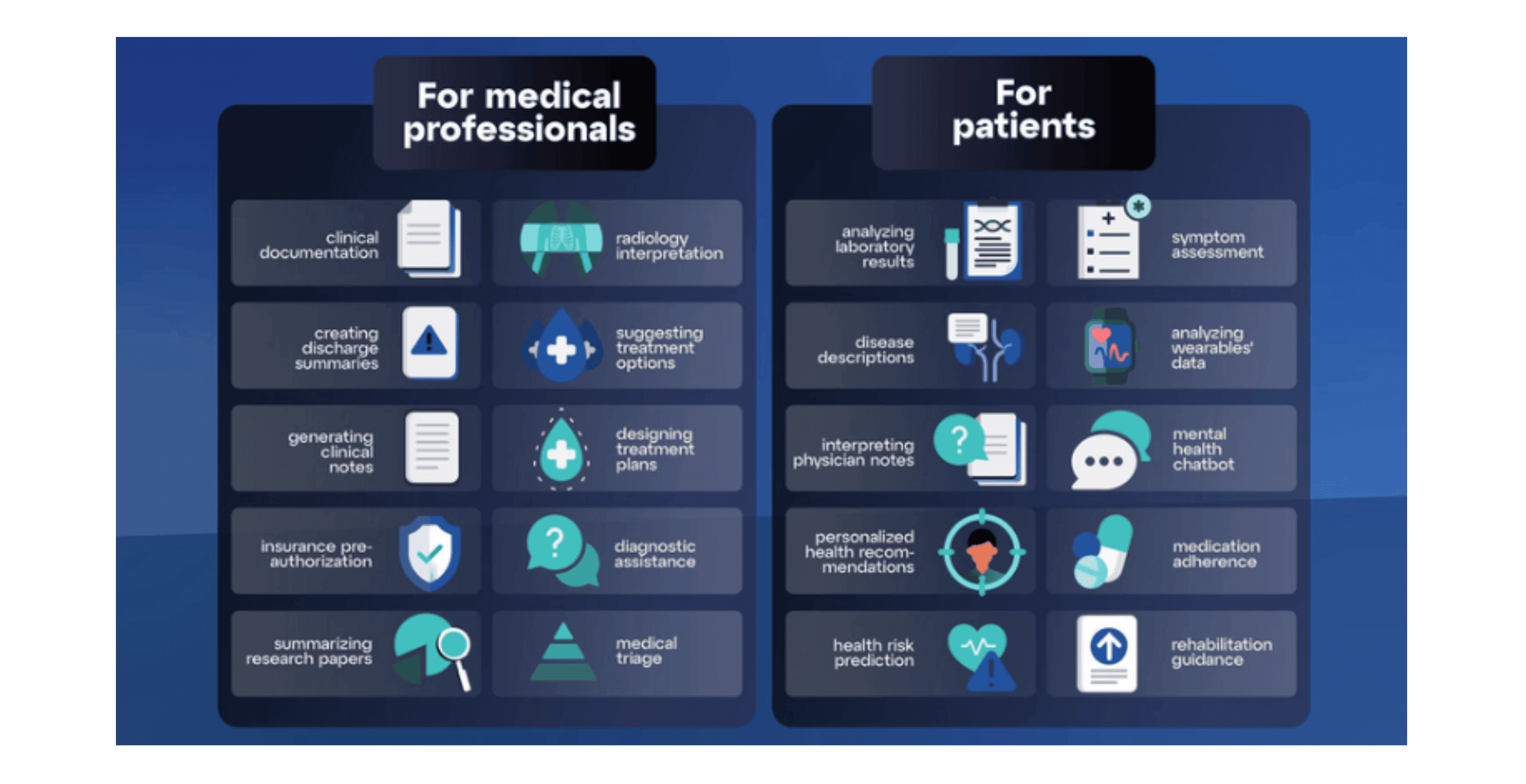
Source: Research Gate
In domains such as banking and healthcare, the stakes for accuracy are exceptionally high. An LLM that assists with diagnosing medical conditions must be precise and reliable, as misdiagnoses can have serious consequences.
Similarly, in banking, inaccuracies in fraud detection can lead to substantial financial losses or regulatory penalties. Ensuring these models meet or exceed human performance standards is a significant challenge.
Read more on Ensuring High Accuracy in Critical Domains with RagaAI
Integration Challenges and the Need for a Solid Data Foundation
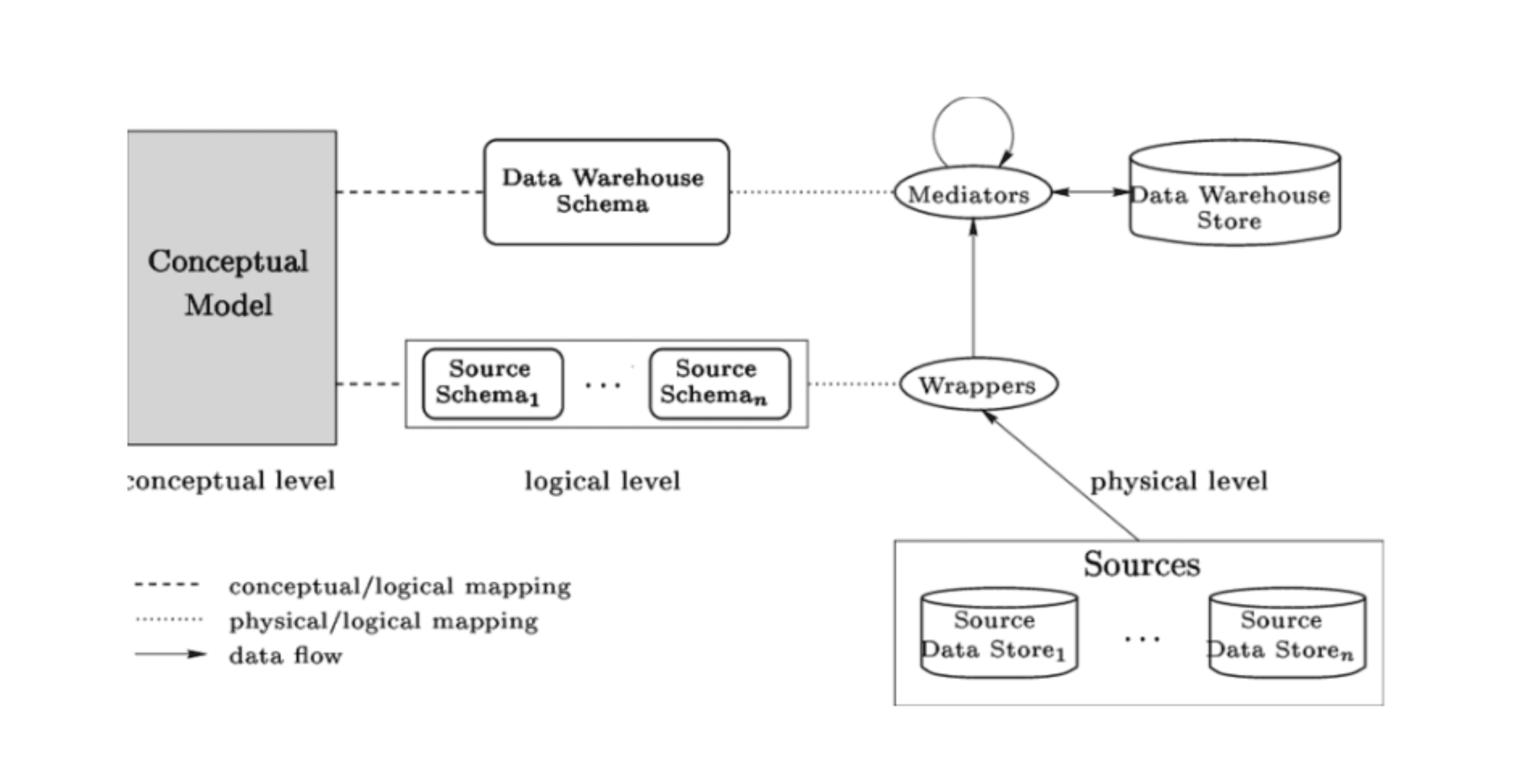
Source: Research Gate
Integrating LLMs into existing IT ecosystems can be daunting. These models often require substantial data inputs to function optimally, which means they must be seamlessly integrated with existing databases and IT infrastructure.
Moreover, the quality of outputs depends heavily on the quality of input data. Therefore, establishing a solid data foundation is critical to successfully deploying LLMs.
Security, Data Privacy, and the Risks of Data Leakage
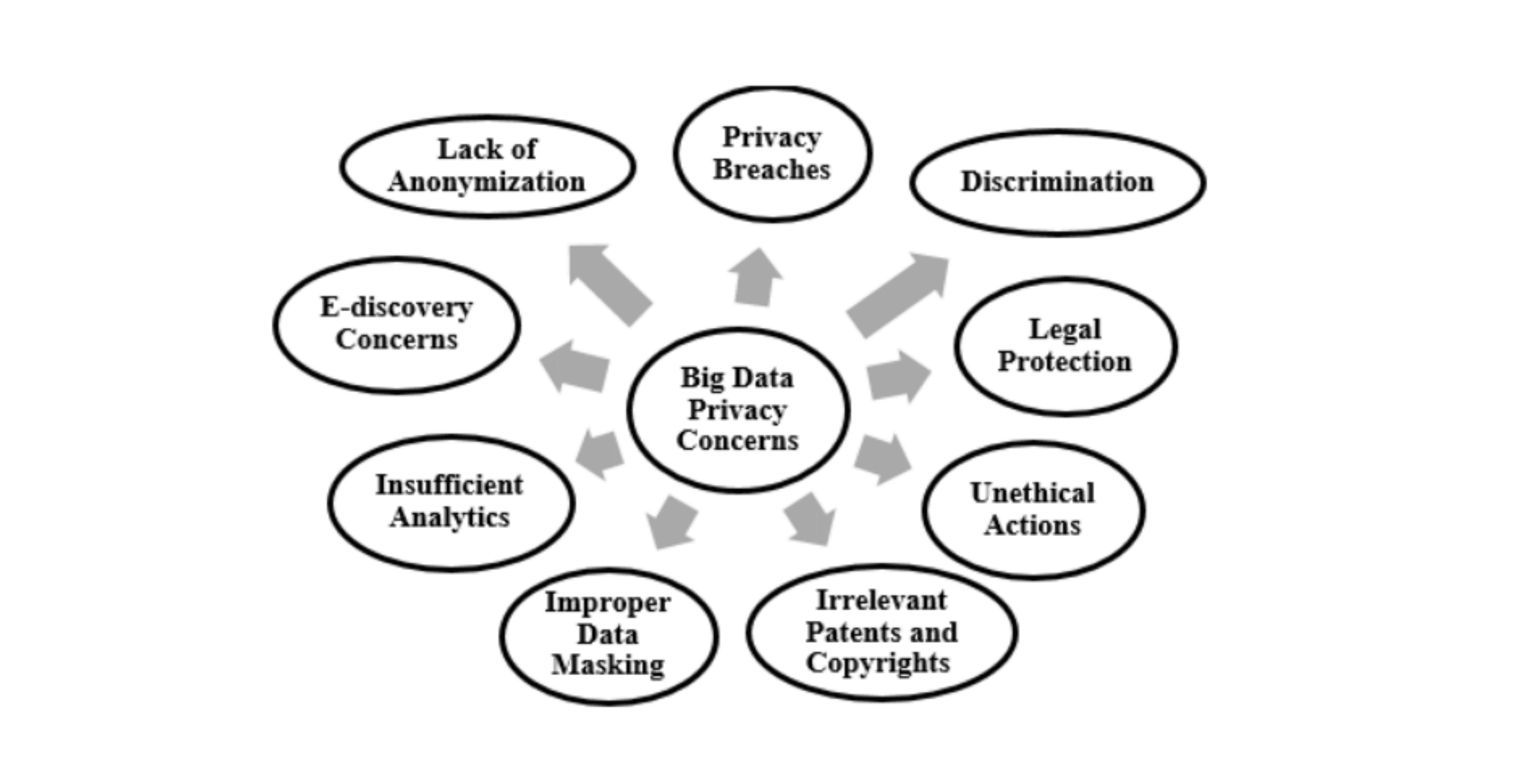
Source: Research Gate
Data security and privacy are major concerns since LLMs often process sensitive information. Ensuring these models comply with data protection regulations like GDPR and HIPAA is crucial.
The risk of data leakage through LLM interactions or training processes also poses a significant threat, necessitating robust security measures to protect data integrity and confidentiality.
These challenges underscore the complexity of deploying LLMs in an enterprise context, where the demands for accuracy, integration, and security are significantly heightened.
As we move forward, we'll explore strategies to overcome these hurdles and ensure that LLM implementations are practical, secure, and compliant with industry standards.
Learn about the Data Security Measures that Raga AI implements to protect sensitive information.
Strategies for Overcoming Challenges
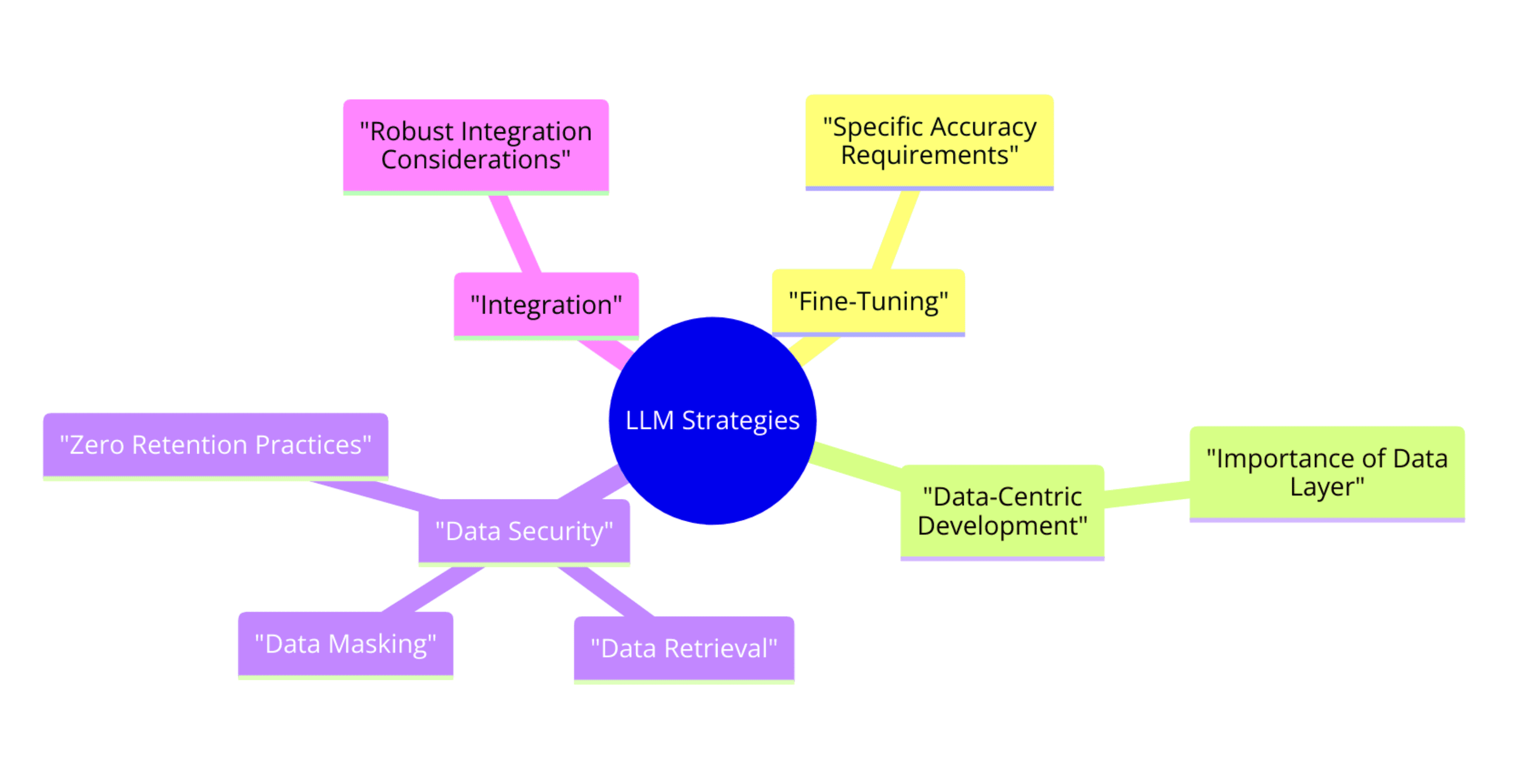
Successfully deploying LLMs in enterprise settings requires a comprehensive strategy that addresses each enterprise's unique challenges. Here are some effective strategies to consider:
Adapting LLMs to Enterprise Needs Through Fine-Tuning for Specific Accuracy Requirements
Fine-tuning the model with specific, high-quality data is essential to ensure that LLMs deliver the high accuracy needed for enterprise applications, particularly in sensitive areas like healthcare and finance.
This involves training the LLM on a dataset that closely mirrors the actual scenarios and data it will encounter in its operational environment.
Example: In healthcare, an LLM used for patient diagnosis can be fine-tuned with anonymized patient records and outcomes to improve its diagnostic accuracy, ensuring it learns from relevant, real-world medical cases.
Utilizing Data-Centric Development and the Importance of the Data Layer
A data-centric approach to LLM implementation focuses on improving the data quality used for training and operating the model rather than just tweaking the model architecture. Ensuring the robust data layer involves curating, cleaning, and continuously updating the dataset to reflect the latest information and contexts.
Example: For a banking LLM used in fraud detection, regularly updating the training dataset with the latest types of fraud cases and transaction profiles can help the model stay relevant and practical.
Securing Data Retrieval, Data Masking, and Zero Retention Practices
Security measures are critical to protect sensitive data used by LLMs. Data retrieval processes should be secure, employing encryption and secure access protocols. Data masking techniques can be used to anonymize sensitive information, ensuring that even if data is accessed inappropriately, it cannot be traced back to anyone.
Example: Implementing zero-knowledge proof systems where the LLM processes information but does not store any personally identifiable information (PII) can help maintain privacy and security.
Additional Considerations for Robust Integration
Another critical strategy is ensuring that LLMs are well-integrated into the existing technological infrastructure without disrupting current operations. This involves API integrations, middleware solutions, or custom adapters that allow LLMs to communicate seamlessly with existing databases and applications.
Example: Using API gateways to manage requests between enterprise applications and LLMs can help regulate data flow and ensure that only necessary information is shared, maintaining performance and security.
Customizing and Building Enterprise LLMs
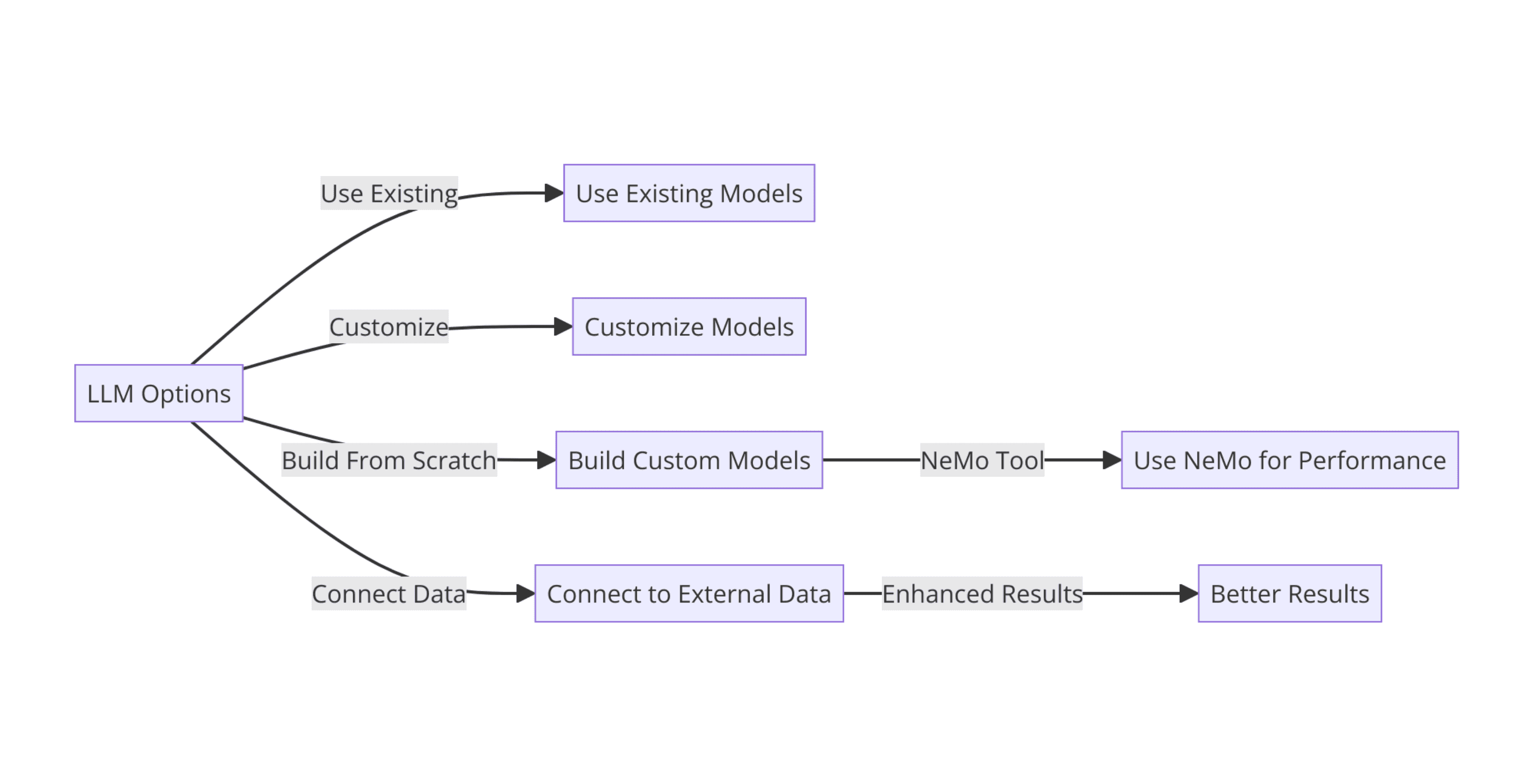
Customizing LLMs or building them from scratch offers enterprises the flexibility to address their unique challenges and objectives. Here’s how businesses can approach these tasks:
Options for Using, Customizing, or Building LLMs from Scratch
Enterprises have several paths to choose from when deploying LLMs: they can use off-the-shelf models, customize them to fit their needs or build entirely new models. Each option has its own set of benefits and considerations:
Using Standard Models: Quick deployment and initial cost savings.
Customizing Existing Models: Better performance in specific tasks while leveraging the foundational strengths of pre-trained models.
Building from Scratch: Complete control over model design, data used, and fine-tuning, but with higher costs and longer development time.
Building Custom Models with Tools like NeMo for Enhanced Performance
For those opting to build or customize models, tools like NVIDIA’s NeMo offer frameworks to create state-of-the-art conversational AI models. NeMo allows developers to fine-tune models on specific datasets, incorporate unique vocabularies, and adjust model architectures to suit particular tasks better.
Example: A telecom company could use NeMo to train a model specifically to understand and generate responses based on telecommunications jargon and customer service interactions, providing more accurate and contextually appropriate customer service.
Connecting an LLM to External Data for Better Results
Enhancing an LLM’s capabilities often involves integrating it with external data sources to enrich the context it has access to. Depending on the application, this might include customer databases, product catalogs, or real-time market data.
Example: An investment firm might integrate its LLM with real-time stock market data and historical investment databases to provide clients with more insightful, data-driven investment advice.
Technical Approach:
import openai
def integrate_external_data(model, data_sources):
enriched_context = ""
for source in data_sources:
enriched_context += extract_data(source)
response = openai.Completion.create(
engine=model,
prompt="Analyze the following market trends: " + enriched_context,
max_tokens=150 )
return response.choices[0].text
def extract_data(source):
# This is a placeholder for a function that fetches and formats data from a
source
return "Latest stock market trends from " + source
# Example of integrating an LLM with external data sources
model = "text-davinci-003"
data_sources = ["NASDAQ updates", "NYSE top movers"]
analysis = integrate_external_data(model, data_sources)
print("Market Analysis: ", analysis)
This example shows how an LLM can be enhanced by connecting it to relevant external data, providing more precise and valuable outputs based on the latest information.
Customizing and building enterprise-specific LLMs allows businesses to meet their unique needs more effectively and maintain a competitive edge by leveraging tailored AI solutions.
Now, let's explore the critical aspects of integrating Large Language Models (LLMs) into enterprise systems and ensuring their security.
This section will cover effectively merging LLMs into existing business processes and safeguarding sensitive information against potential threats.
Read more on Enhancing LLM Reliability with RagaAI
Integration and Security
Integrating LLMs into enterprise environments presents its own set of challenges, especially when it comes to ensuring that these systems can interact seamlessly with existing infrastructure and manage data securely.
Overcoming Integration Challenges for Personalized Customer Responses
Integrating LLMs requires a thoughtful approach to connect these models with existing customer relationship management (CRM) systems, databases, and other enterprise applications. This integration enables LLMs to access the necessary data for personalized responses and support.
Example: By linking an LLM to a CRM system, a business can enable the model to access customer purchase histories, support tickets, and preferences. This integration allows the LLM to deliver highly personalized customer service interactions, such as suggesting products based on past purchases or quickly resolving support issues by referring to previous tickets.
Strategies for Secure Data Retrieval and Protecting Sensitive Information
Ensuring the security of data accessed and generated by LLMs is paramount. Strategies to secure data include using encryption for data in transit and at rest, implementing robust access controls, and applying anonymization techniques where possible.
Technical Insight:
Encryption: Implement SSL/TLS for data transmitted between the LLM and other systems. Use strong encryption standards like AES-256 for data at rest.
Access Controls: Set up role-based access controls (RBAC) to ensure only authorized personnel can interact with the LLM and its data.
Read more on Addressing Integration Challenges with RagaAI
Monitoring and Aligning LLM Behavior with Enterprise Goals
Continuous monitoring of LLM activities is crucial to ensure they perform as intended and adhere to company policies and ethical guidelines. Setting up monitoring tools to track the performance and outputs of LLMs can help quickly identify and address any deviations from expected behaviors.
Example: Use logging and auditing tools to record all interactions with the LLM. Analyze logs regularly to detect any anomalies or unauthorized attempts to access the system. Implement automated alerts to notify the appropriate personnel when irregular patterns are detected.
import logging
# Setup logging configuration
logging.basicConfig(filename='llm_activity.log', level=logging.INFO, format='%(asctime)s:%(levelname)s:%(message)s')
def log_interaction(user_id, query, response):
logging.info(f"User ID: {user_id}, Query: {query}, Response: {response}")
# Example of logging an interaction
user_id = 12345
query = "What products are recommended for someone who bought X?"
response = "Based on previous purchases, we recommend Y and Z."
log_interaction(user_id, query, response)
This script provides a basic framework for logging interactions with an LLM, helping maintain a transparent record that can be audited for compliance and monitoring purposes.
Ensuring LLMs are Kept on Track and Secure Through Monitoring and Guardrails
Setting up continuous monitoring systems and defining clear operational guardrails are essential to maintaining the integrity and effectiveness of LLM deployments. Monitoring tools can track LLMs' performance and behavior, while guardrails ensure that the models operate within predefined ethical and operational boundaries.
Example Implementation:
def monitor_model_performance(metrics):
if metrics['accuracy'] < threshold:
alert("Model accuracy below expected threshold.")
if metrics['response_time'] > max_response_time:
optimize_model_inference()
def optimize_model_inference():
# Implement optimization logic (e.g., load balancing, resource allocation)
print("Optimizing model inference for better performance.")
# Simulated monitoring data
model_metrics = {'accuracy': 92.5, 'response_time': 0.300} # 300 ms
monitor_model_performance(model_metrics)
This example demonstrates an essential monitoring function that checks model performance against certain thresholds and triggers optimization procedures if needed.
Optimizing LLMs for enterprise applications involves technical adjustments and enhancements and ensuring these systems integrate smoothly with existing corporate infrastructure and policies. This holistic approach helps businesses harness the full power of LLMs while maintaining control over their deployment and usage.
Let's wrap up our comprehensive exploration of implementing Large Language Models (LLMs) in the enterprise context. We'll recap the importance of LLMs, highlight key strategies to overcome challenges, and reiterate the value of customization and data-centric approaches.
Conclusion: Embracing LLMs in Enterprise
Integrating and optimizing large language models (LLMs) within enterprise systems is challenging and rewarding.
As we've explored, LLMs offer tremendous potential to revolutionize various aspects of business operations, from enhancing customer interactions to streamlining complex analytical tasks.
Embracing LLMs requires a commitment to continuous learning and adaptation, but the rewards justify the effort.
As AI technology evolves, so will the opportunities for its application in business. Enterprises that stay ahead of these trends, continuously refine their approaches, and invest in cutting-edge solutions will solve complex challenges and gain a significant competitive advantage.
By understanding and addressing the unique challenges of implementing LLMs and by leveraging the right strategies and tools, enterprises can fully realize the potential of these advanced AI models.
Though filled with technical and strategic challenges, this journey opens up a world of possibilities for innovation and efficiency.
Integrating large language models (LLMs) into enterprise applications is becoming a game-changer for many large companies. Substantial investments are flowing into this area, and businesses are eager to harness the power of LLMs for various innovative applications.
From engaging customers in more human-like conversations to detecting fraud and even diagnosing medical conditions, the potential uses are as diverse as they are impactful.
As AI evolves, the corporate world increasingly recognizes the value of implementing advanced LLMs. Companies are investing heavily financially and in the resources needed to integrate these technologies into their operations.
This surge in investment is driven by the promise of significant returns through enhanced efficiencies, improved customer interactions, and new capabilities that were once thought impossible without human intervention.
Key Challenges in Enterprise LLM Implementation
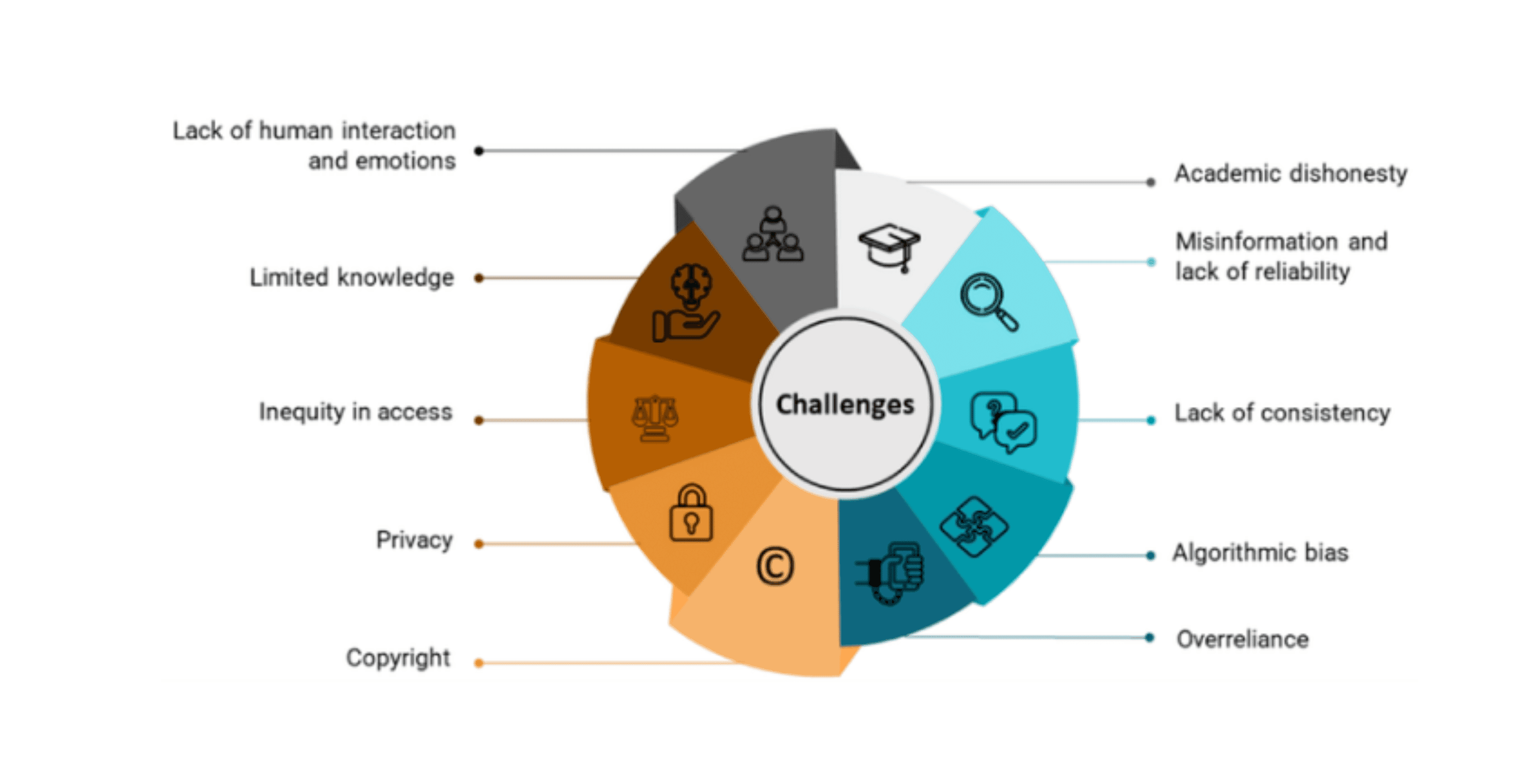
Source: Research Gate
Implementing LLMs in an enterprise setting comes with a set of unique challenges. These range from ensuring the models meet the high accuracy demands of specific applications to overcoming integration hurdles and securing sensitive data.
Generic LLM Limitations for Specific Enterprise Applications
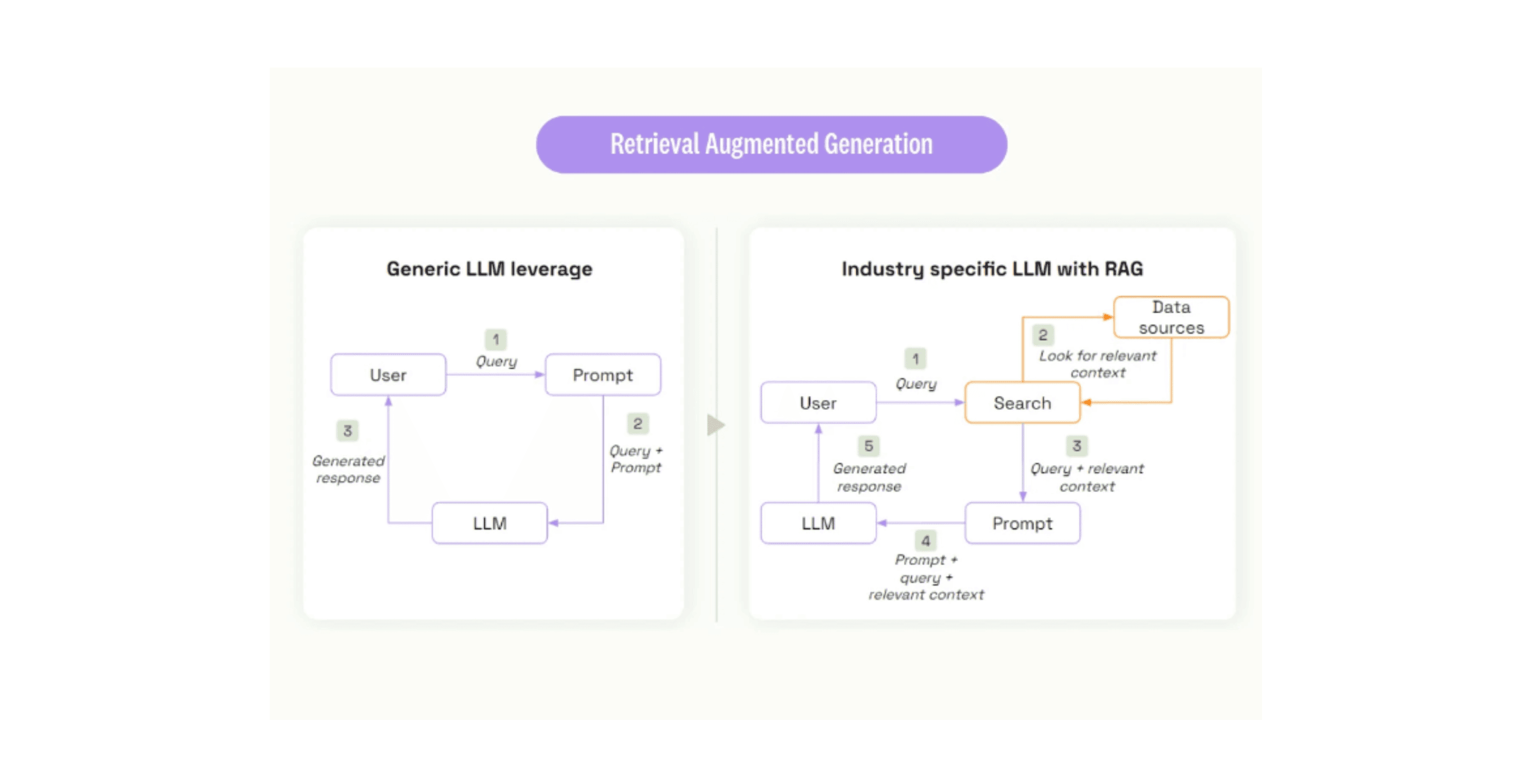
Source: Kili Technology
While LLMs are powerful, their generic training sometimes aligns differently with specific enterprise needs. For example, a model trained on broad data may need to improve in specialized tasks like legal document analysis or technical support for complex products with significant customization.
This misalignment can lead to inefficiencies or inaccuracies in task execution.
Read more on Customizing LLMs for Specific Tasks with RagaAI
High Accuracy Demands in Critical Domains like Banking and Healthcare
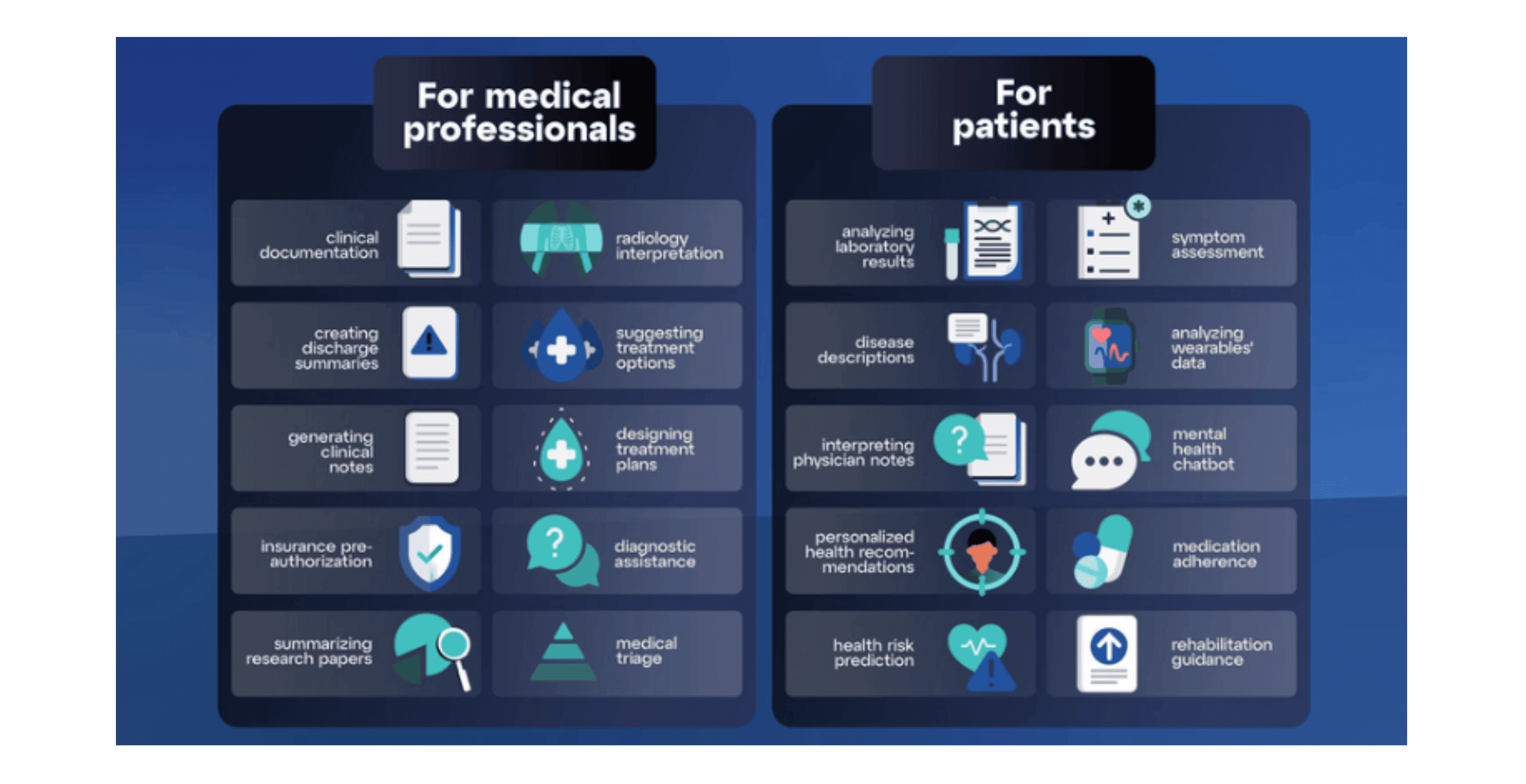
Source: Research Gate
In domains such as banking and healthcare, the stakes for accuracy are exceptionally high. An LLM that assists with diagnosing medical conditions must be precise and reliable, as misdiagnoses can have serious consequences.
Similarly, in banking, inaccuracies in fraud detection can lead to substantial financial losses or regulatory penalties. Ensuring these models meet or exceed human performance standards is a significant challenge.
Read more on Ensuring High Accuracy in Critical Domains with RagaAI
Integration Challenges and the Need for a Solid Data Foundation
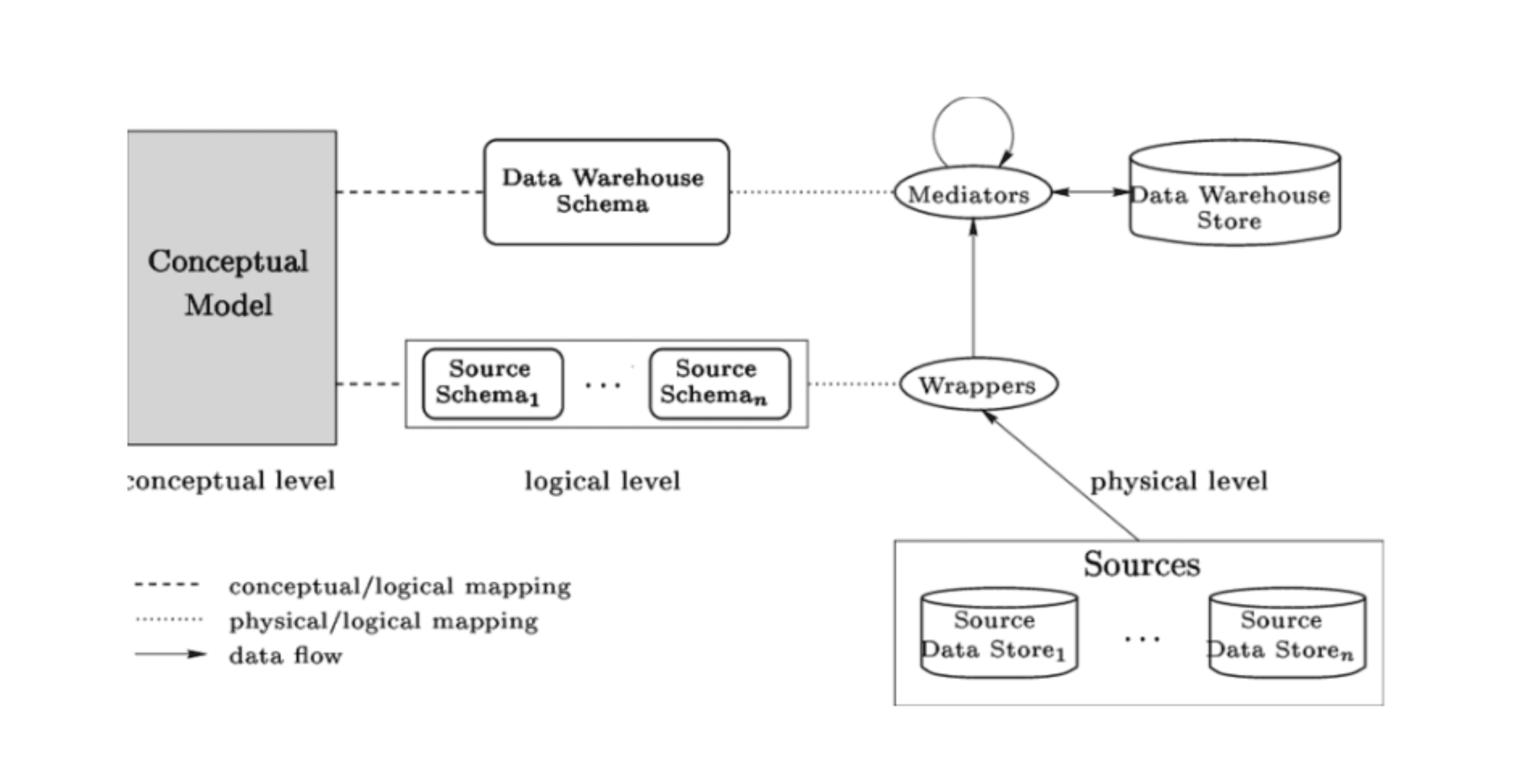
Source: Research Gate
Integrating LLMs into existing IT ecosystems can be daunting. These models often require substantial data inputs to function optimally, which means they must be seamlessly integrated with existing databases and IT infrastructure.
Moreover, the quality of outputs depends heavily on the quality of input data. Therefore, establishing a solid data foundation is critical to successfully deploying LLMs.
Security, Data Privacy, and the Risks of Data Leakage
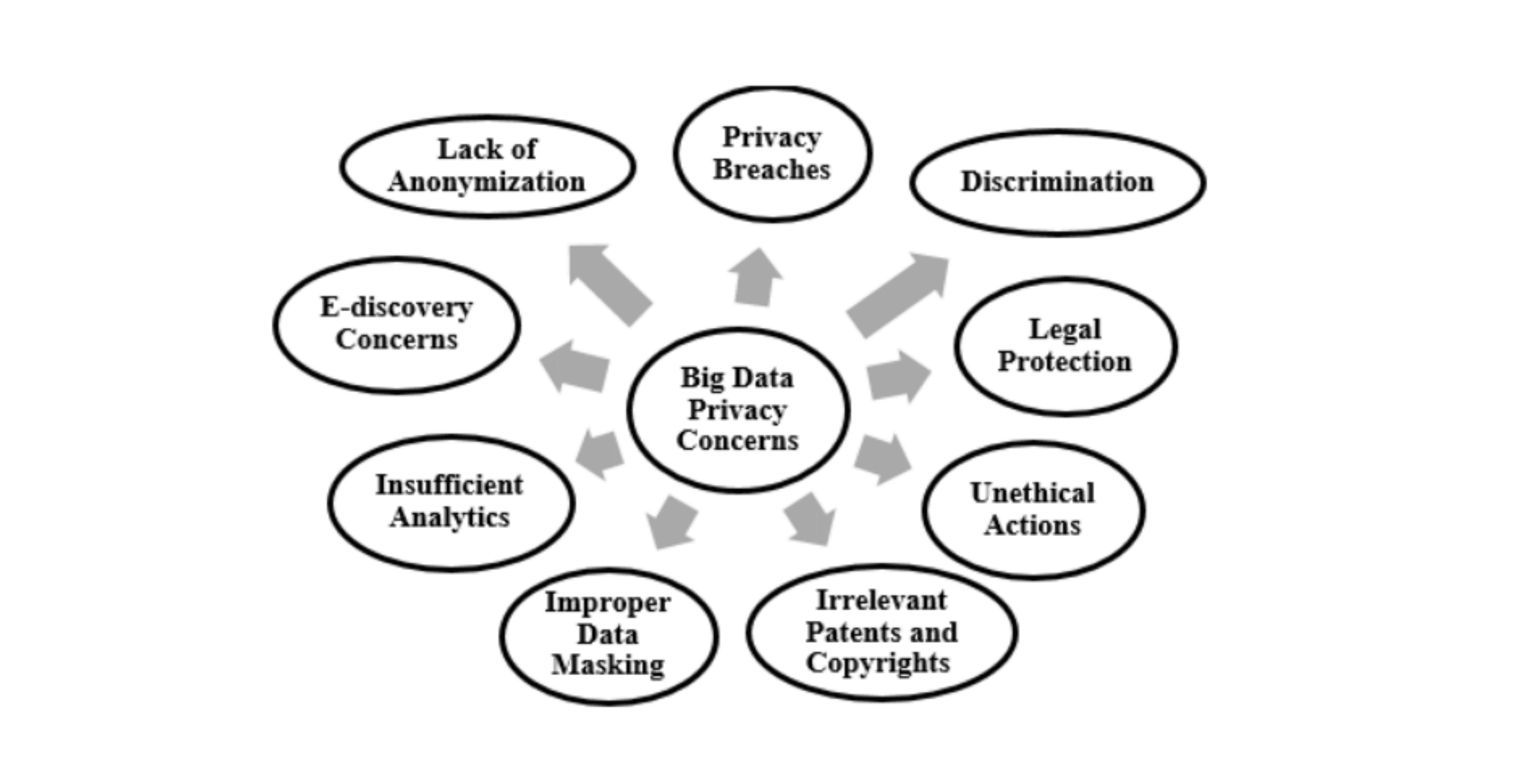
Source: Research Gate
Data security and privacy are major concerns since LLMs often process sensitive information. Ensuring these models comply with data protection regulations like GDPR and HIPAA is crucial.
The risk of data leakage through LLM interactions or training processes also poses a significant threat, necessitating robust security measures to protect data integrity and confidentiality.
These challenges underscore the complexity of deploying LLMs in an enterprise context, where the demands for accuracy, integration, and security are significantly heightened.
As we move forward, we'll explore strategies to overcome these hurdles and ensure that LLM implementations are practical, secure, and compliant with industry standards.
Learn about the Data Security Measures that Raga AI implements to protect sensitive information.
Strategies for Overcoming Challenges
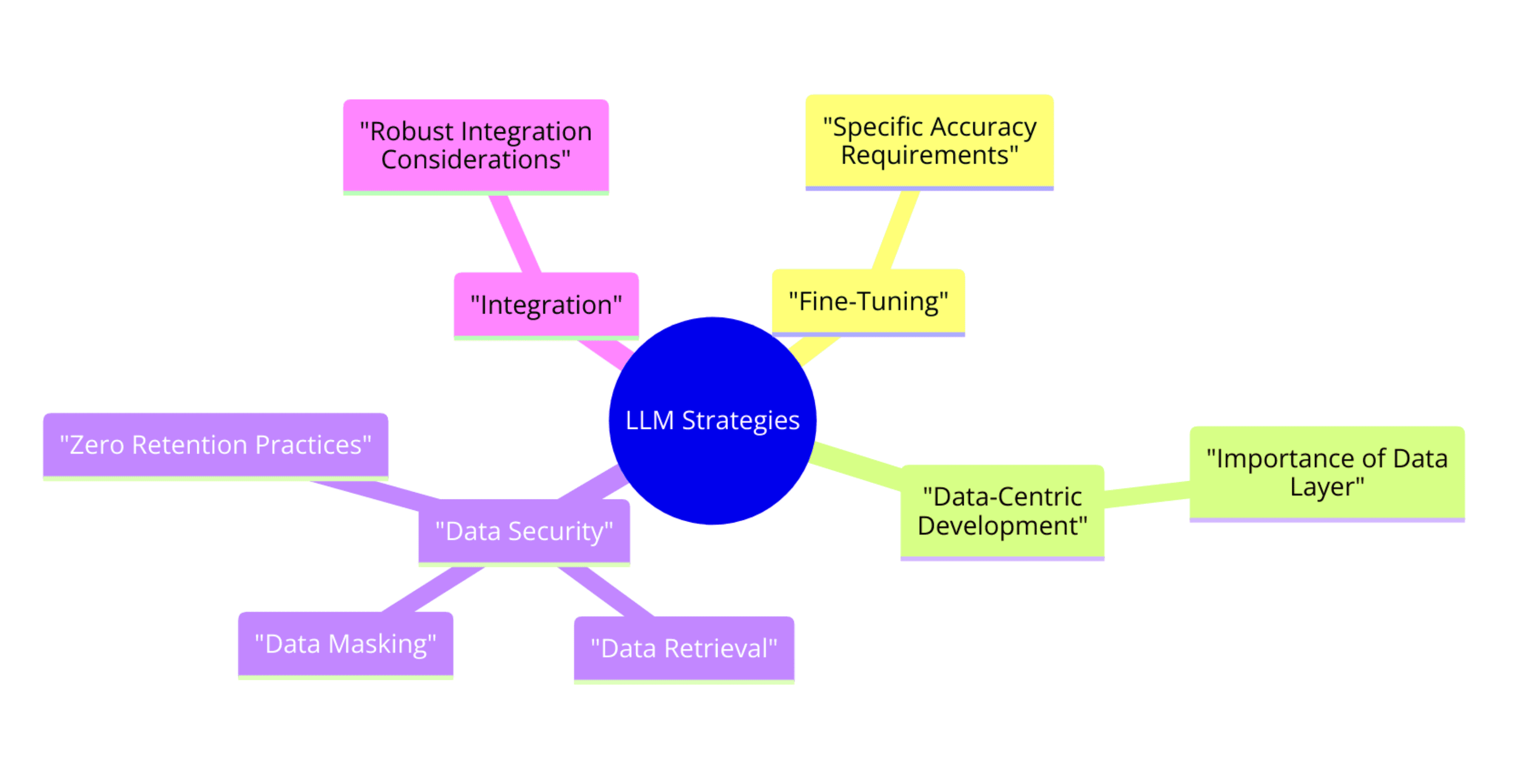
Successfully deploying LLMs in enterprise settings requires a comprehensive strategy that addresses each enterprise's unique challenges. Here are some effective strategies to consider:
Adapting LLMs to Enterprise Needs Through Fine-Tuning for Specific Accuracy Requirements
Fine-tuning the model with specific, high-quality data is essential to ensure that LLMs deliver the high accuracy needed for enterprise applications, particularly in sensitive areas like healthcare and finance.
This involves training the LLM on a dataset that closely mirrors the actual scenarios and data it will encounter in its operational environment.
Example: In healthcare, an LLM used for patient diagnosis can be fine-tuned with anonymized patient records and outcomes to improve its diagnostic accuracy, ensuring it learns from relevant, real-world medical cases.
Utilizing Data-Centric Development and the Importance of the Data Layer
A data-centric approach to LLM implementation focuses on improving the data quality used for training and operating the model rather than just tweaking the model architecture. Ensuring the robust data layer involves curating, cleaning, and continuously updating the dataset to reflect the latest information and contexts.
Example: For a banking LLM used in fraud detection, regularly updating the training dataset with the latest types of fraud cases and transaction profiles can help the model stay relevant and practical.
Securing Data Retrieval, Data Masking, and Zero Retention Practices
Security measures are critical to protect sensitive data used by LLMs. Data retrieval processes should be secure, employing encryption and secure access protocols. Data masking techniques can be used to anonymize sensitive information, ensuring that even if data is accessed inappropriately, it cannot be traced back to anyone.
Example: Implementing zero-knowledge proof systems where the LLM processes information but does not store any personally identifiable information (PII) can help maintain privacy and security.
Additional Considerations for Robust Integration
Another critical strategy is ensuring that LLMs are well-integrated into the existing technological infrastructure without disrupting current operations. This involves API integrations, middleware solutions, or custom adapters that allow LLMs to communicate seamlessly with existing databases and applications.
Example: Using API gateways to manage requests between enterprise applications and LLMs can help regulate data flow and ensure that only necessary information is shared, maintaining performance and security.
Customizing and Building Enterprise LLMs
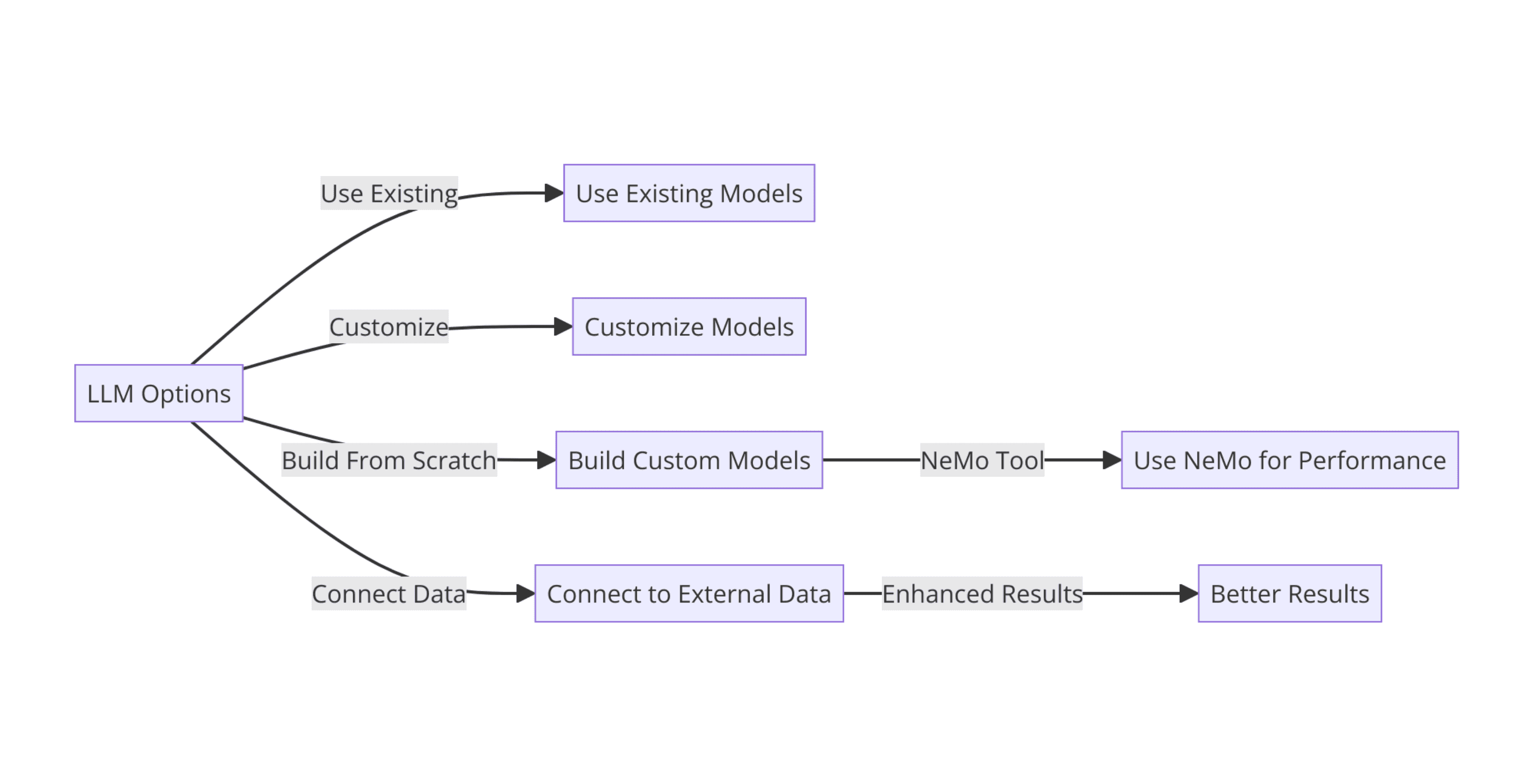
Customizing LLMs or building them from scratch offers enterprises the flexibility to address their unique challenges and objectives. Here’s how businesses can approach these tasks:
Options for Using, Customizing, or Building LLMs from Scratch
Enterprises have several paths to choose from when deploying LLMs: they can use off-the-shelf models, customize them to fit their needs or build entirely new models. Each option has its own set of benefits and considerations:
Using Standard Models: Quick deployment and initial cost savings.
Customizing Existing Models: Better performance in specific tasks while leveraging the foundational strengths of pre-trained models.
Building from Scratch: Complete control over model design, data used, and fine-tuning, but with higher costs and longer development time.
Building Custom Models with Tools like NeMo for Enhanced Performance
For those opting to build or customize models, tools like NVIDIA’s NeMo offer frameworks to create state-of-the-art conversational AI models. NeMo allows developers to fine-tune models on specific datasets, incorporate unique vocabularies, and adjust model architectures to suit particular tasks better.
Example: A telecom company could use NeMo to train a model specifically to understand and generate responses based on telecommunications jargon and customer service interactions, providing more accurate and contextually appropriate customer service.
Connecting an LLM to External Data for Better Results
Enhancing an LLM’s capabilities often involves integrating it with external data sources to enrich the context it has access to. Depending on the application, this might include customer databases, product catalogs, or real-time market data.
Example: An investment firm might integrate its LLM with real-time stock market data and historical investment databases to provide clients with more insightful, data-driven investment advice.
Technical Approach:
import openai
def integrate_external_data(model, data_sources):
enriched_context = ""
for source in data_sources:
enriched_context += extract_data(source)
response = openai.Completion.create(
engine=model,
prompt="Analyze the following market trends: " + enriched_context,
max_tokens=150 )
return response.choices[0].text
def extract_data(source):
# This is a placeholder for a function that fetches and formats data from a
source
return "Latest stock market trends from " + source
# Example of integrating an LLM with external data sources
model = "text-davinci-003"
data_sources = ["NASDAQ updates", "NYSE top movers"]
analysis = integrate_external_data(model, data_sources)
print("Market Analysis: ", analysis)
This example shows how an LLM can be enhanced by connecting it to relevant external data, providing more precise and valuable outputs based on the latest information.
Customizing and building enterprise-specific LLMs allows businesses to meet their unique needs more effectively and maintain a competitive edge by leveraging tailored AI solutions.
Now, let's explore the critical aspects of integrating Large Language Models (LLMs) into enterprise systems and ensuring their security.
This section will cover effectively merging LLMs into existing business processes and safeguarding sensitive information against potential threats.
Read more on Enhancing LLM Reliability with RagaAI
Integration and Security
Integrating LLMs into enterprise environments presents its own set of challenges, especially when it comes to ensuring that these systems can interact seamlessly with existing infrastructure and manage data securely.
Overcoming Integration Challenges for Personalized Customer Responses
Integrating LLMs requires a thoughtful approach to connect these models with existing customer relationship management (CRM) systems, databases, and other enterprise applications. This integration enables LLMs to access the necessary data for personalized responses and support.
Example: By linking an LLM to a CRM system, a business can enable the model to access customer purchase histories, support tickets, and preferences. This integration allows the LLM to deliver highly personalized customer service interactions, such as suggesting products based on past purchases or quickly resolving support issues by referring to previous tickets.
Strategies for Secure Data Retrieval and Protecting Sensitive Information
Ensuring the security of data accessed and generated by LLMs is paramount. Strategies to secure data include using encryption for data in transit and at rest, implementing robust access controls, and applying anonymization techniques where possible.
Technical Insight:
Encryption: Implement SSL/TLS for data transmitted between the LLM and other systems. Use strong encryption standards like AES-256 for data at rest.
Access Controls: Set up role-based access controls (RBAC) to ensure only authorized personnel can interact with the LLM and its data.
Read more on Addressing Integration Challenges with RagaAI
Monitoring and Aligning LLM Behavior with Enterprise Goals
Continuous monitoring of LLM activities is crucial to ensure they perform as intended and adhere to company policies and ethical guidelines. Setting up monitoring tools to track the performance and outputs of LLMs can help quickly identify and address any deviations from expected behaviors.
Example: Use logging and auditing tools to record all interactions with the LLM. Analyze logs regularly to detect any anomalies or unauthorized attempts to access the system. Implement automated alerts to notify the appropriate personnel when irregular patterns are detected.
import logging
# Setup logging configuration
logging.basicConfig(filename='llm_activity.log', level=logging.INFO, format='%(asctime)s:%(levelname)s:%(message)s')
def log_interaction(user_id, query, response):
logging.info(f"User ID: {user_id}, Query: {query}, Response: {response}")
# Example of logging an interaction
user_id = 12345
query = "What products are recommended for someone who bought X?"
response = "Based on previous purchases, we recommend Y and Z."
log_interaction(user_id, query, response)
This script provides a basic framework for logging interactions with an LLM, helping maintain a transparent record that can be audited for compliance and monitoring purposes.
Ensuring LLMs are Kept on Track and Secure Through Monitoring and Guardrails
Setting up continuous monitoring systems and defining clear operational guardrails are essential to maintaining the integrity and effectiveness of LLM deployments. Monitoring tools can track LLMs' performance and behavior, while guardrails ensure that the models operate within predefined ethical and operational boundaries.
Example Implementation:
def monitor_model_performance(metrics):
if metrics['accuracy'] < threshold:
alert("Model accuracy below expected threshold.")
if metrics['response_time'] > max_response_time:
optimize_model_inference()
def optimize_model_inference():
# Implement optimization logic (e.g., load balancing, resource allocation)
print("Optimizing model inference for better performance.")
# Simulated monitoring data
model_metrics = {'accuracy': 92.5, 'response_time': 0.300} # 300 ms
monitor_model_performance(model_metrics)
This example demonstrates an essential monitoring function that checks model performance against certain thresholds and triggers optimization procedures if needed.
Optimizing LLMs for enterprise applications involves technical adjustments and enhancements and ensuring these systems integrate smoothly with existing corporate infrastructure and policies. This holistic approach helps businesses harness the full power of LLMs while maintaining control over their deployment and usage.
Let's wrap up our comprehensive exploration of implementing Large Language Models (LLMs) in the enterprise context. We'll recap the importance of LLMs, highlight key strategies to overcome challenges, and reiterate the value of customization and data-centric approaches.
Conclusion: Embracing LLMs in Enterprise
Integrating and optimizing large language models (LLMs) within enterprise systems is challenging and rewarding.
As we've explored, LLMs offer tremendous potential to revolutionize various aspects of business operations, from enhancing customer interactions to streamlining complex analytical tasks.
Embracing LLMs requires a commitment to continuous learning and adaptation, but the rewards justify the effort.
As AI technology evolves, so will the opportunities for its application in business. Enterprises that stay ahead of these trends, continuously refine their approaches, and invest in cutting-edge solutions will solve complex challenges and gain a significant competitive advantage.
By understanding and addressing the unique challenges of implementing LLMs and by leveraging the right strategies and tools, enterprises can fully realize the potential of these advanced AI models.
Though filled with technical and strategic challenges, this journey opens up a world of possibilities for innovation and efficiency.
Integrating large language models (LLMs) into enterprise applications is becoming a game-changer for many large companies. Substantial investments are flowing into this area, and businesses are eager to harness the power of LLMs for various innovative applications.
From engaging customers in more human-like conversations to detecting fraud and even diagnosing medical conditions, the potential uses are as diverse as they are impactful.
As AI evolves, the corporate world increasingly recognizes the value of implementing advanced LLMs. Companies are investing heavily financially and in the resources needed to integrate these technologies into their operations.
This surge in investment is driven by the promise of significant returns through enhanced efficiencies, improved customer interactions, and new capabilities that were once thought impossible without human intervention.
Key Challenges in Enterprise LLM Implementation
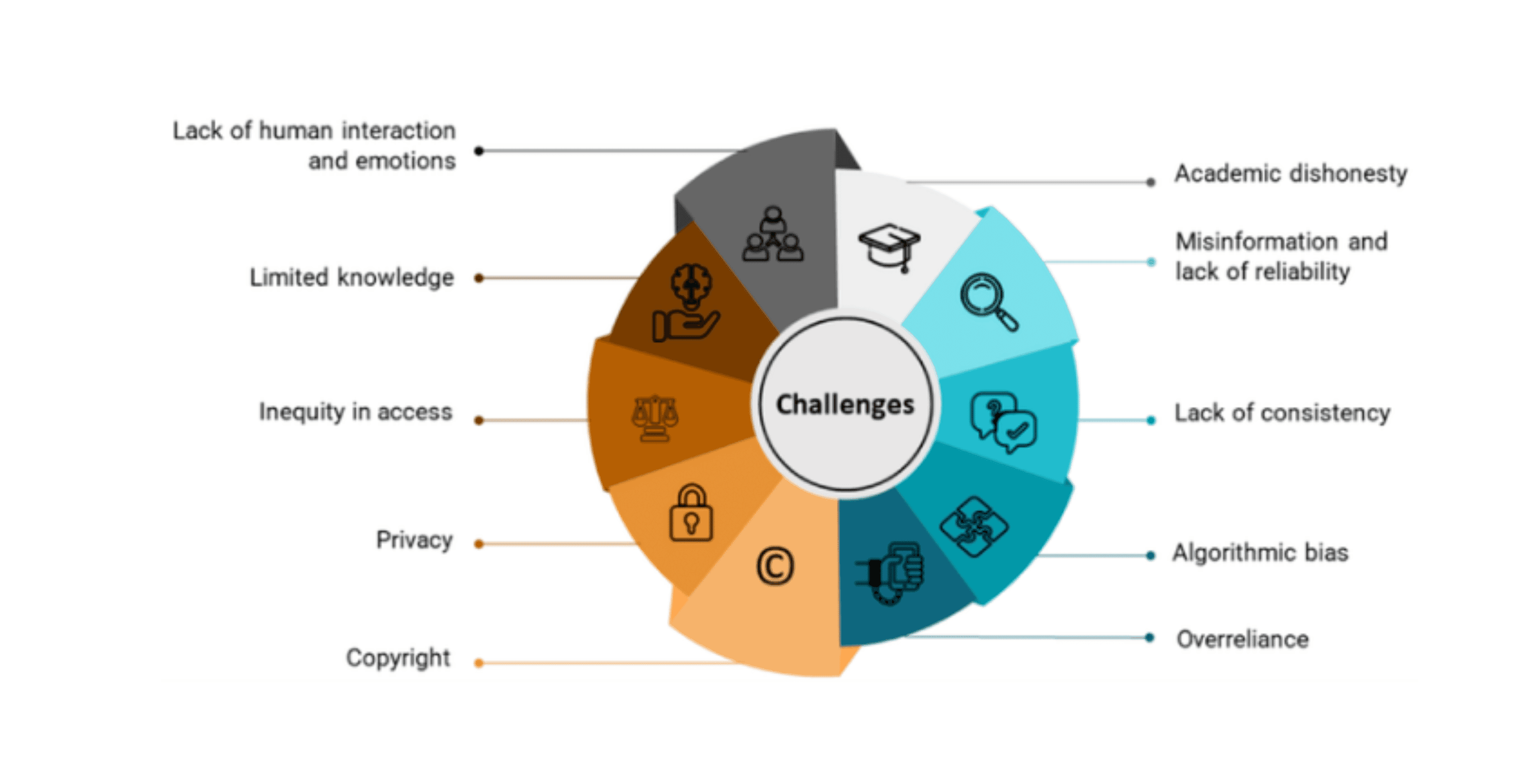
Source: Research Gate
Implementing LLMs in an enterprise setting comes with a set of unique challenges. These range from ensuring the models meet the high accuracy demands of specific applications to overcoming integration hurdles and securing sensitive data.
Generic LLM Limitations for Specific Enterprise Applications
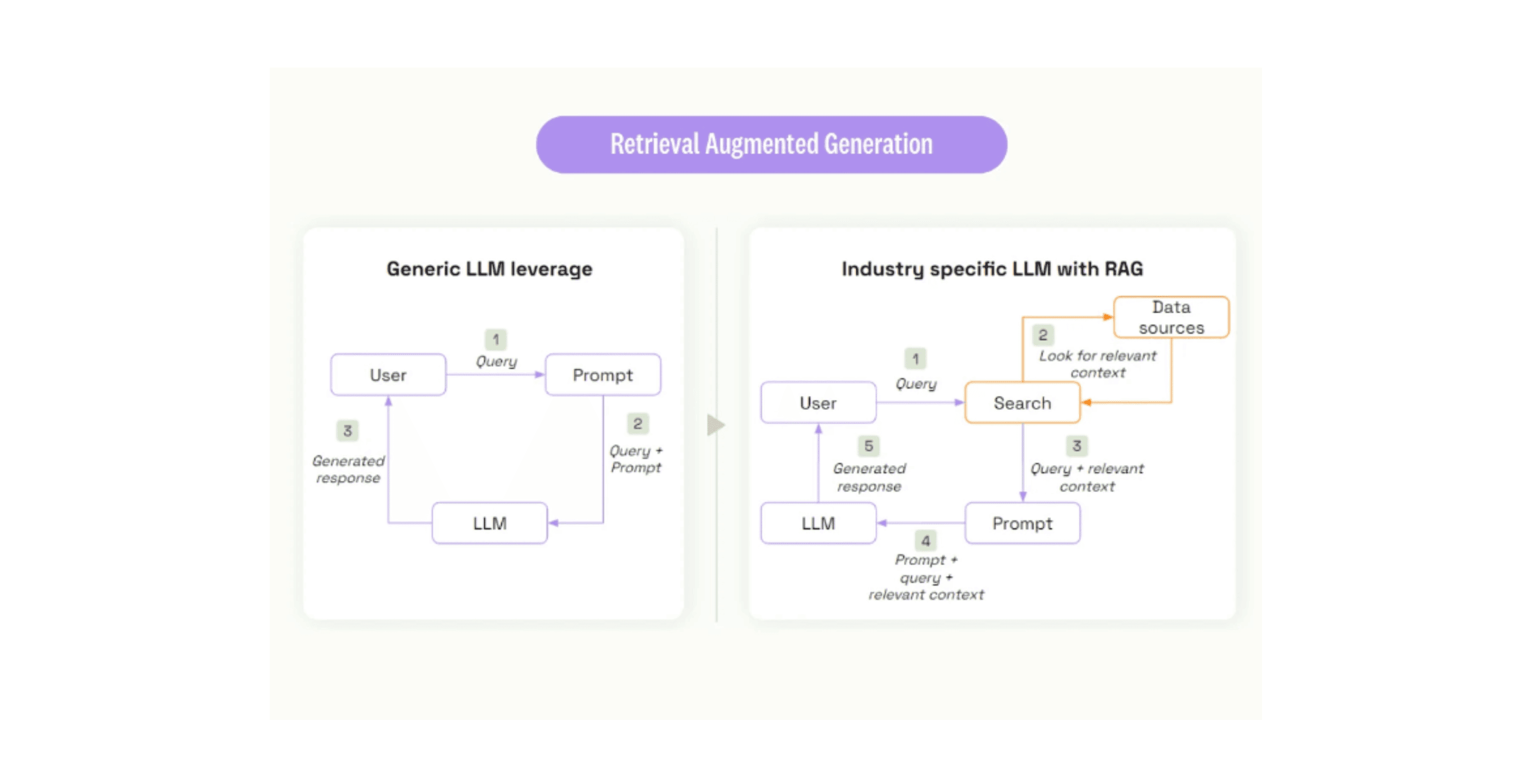
Source: Kili Technology
While LLMs are powerful, their generic training sometimes aligns differently with specific enterprise needs. For example, a model trained on broad data may need to improve in specialized tasks like legal document analysis or technical support for complex products with significant customization.
This misalignment can lead to inefficiencies or inaccuracies in task execution.
Read more on Customizing LLMs for Specific Tasks with RagaAI
High Accuracy Demands in Critical Domains like Banking and Healthcare
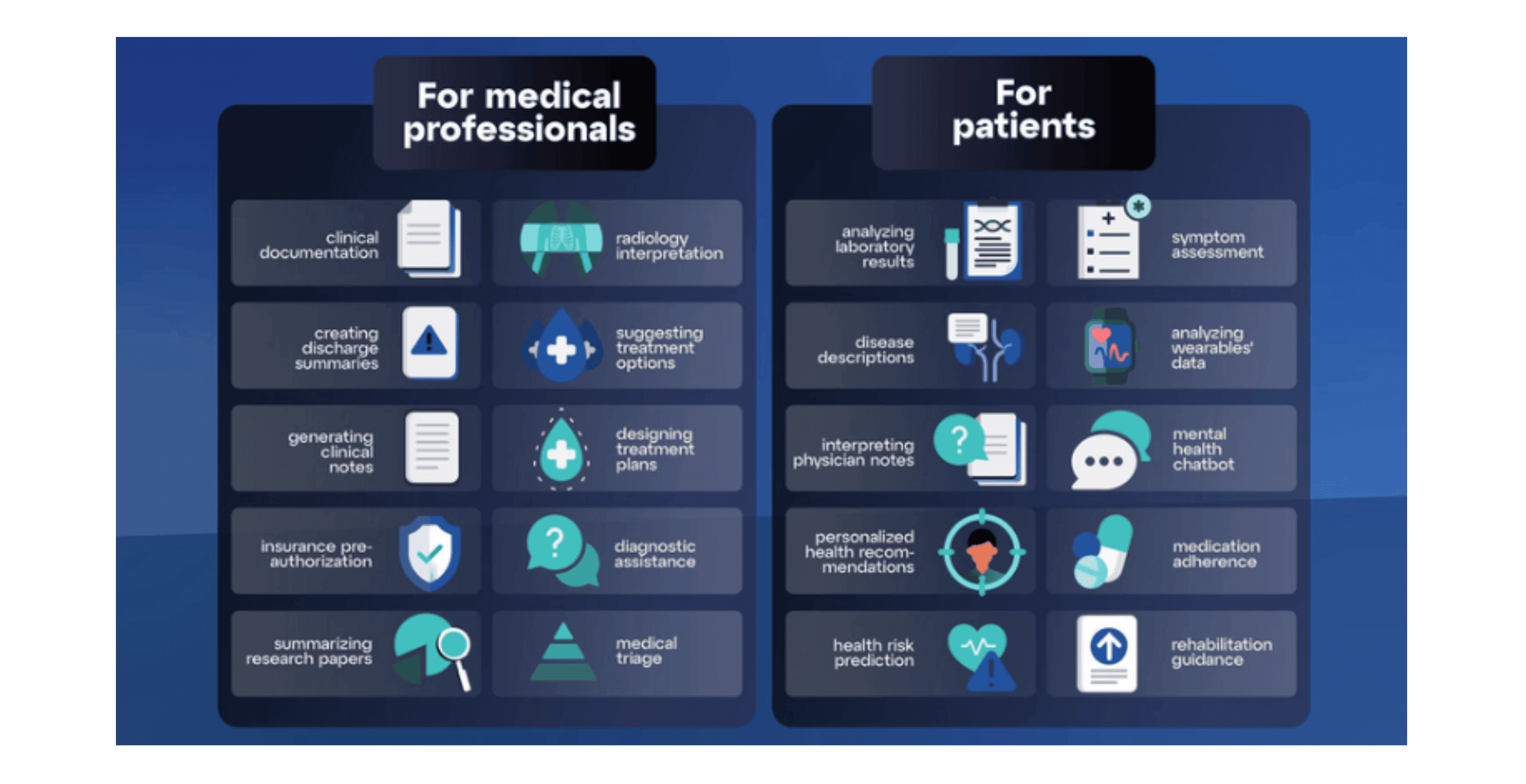
Source: Research Gate
In domains such as banking and healthcare, the stakes for accuracy are exceptionally high. An LLM that assists with diagnosing medical conditions must be precise and reliable, as misdiagnoses can have serious consequences.
Similarly, in banking, inaccuracies in fraud detection can lead to substantial financial losses or regulatory penalties. Ensuring these models meet or exceed human performance standards is a significant challenge.
Read more on Ensuring High Accuracy in Critical Domains with RagaAI
Integration Challenges and the Need for a Solid Data Foundation
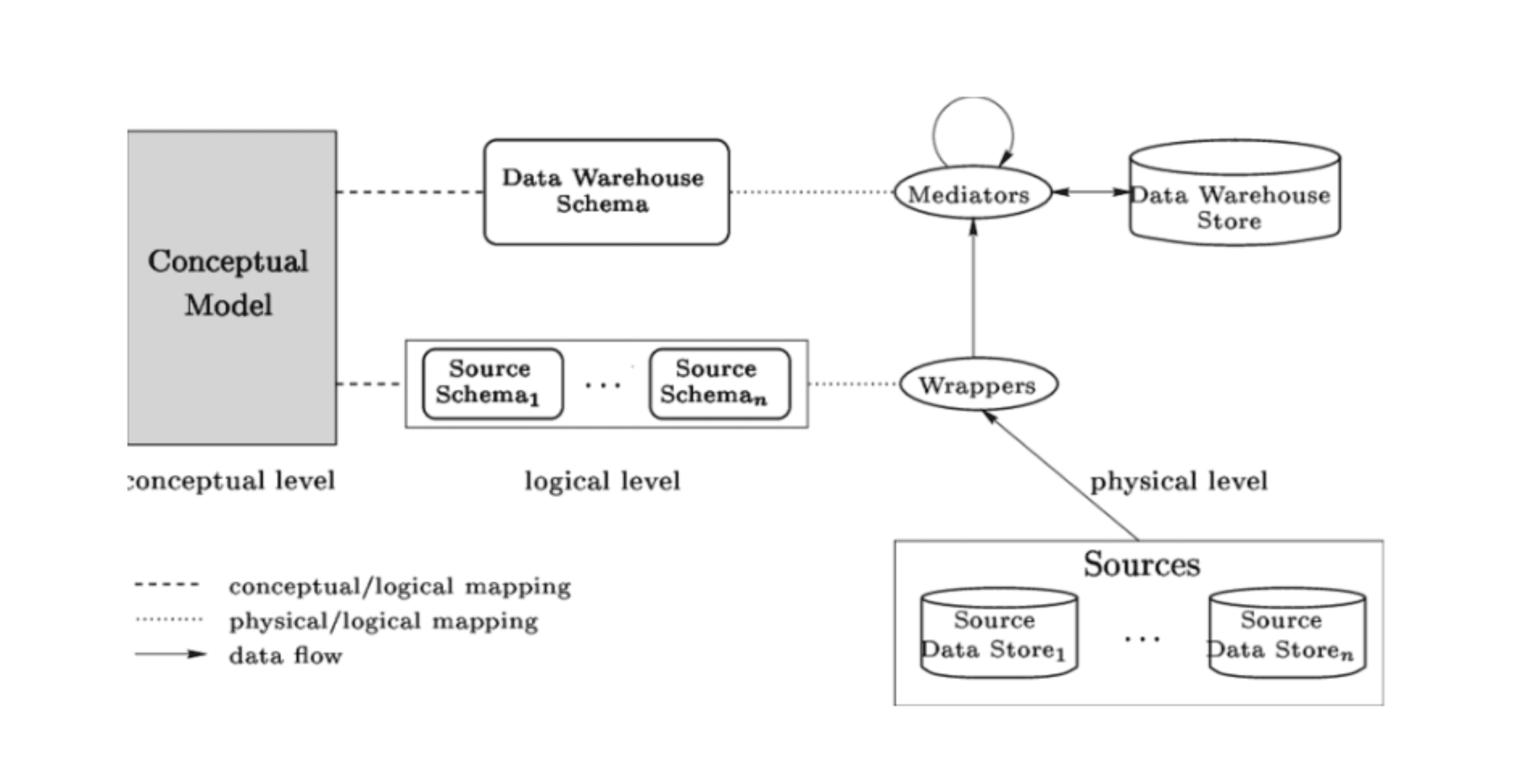
Source: Research Gate
Integrating LLMs into existing IT ecosystems can be daunting. These models often require substantial data inputs to function optimally, which means they must be seamlessly integrated with existing databases and IT infrastructure.
Moreover, the quality of outputs depends heavily on the quality of input data. Therefore, establishing a solid data foundation is critical to successfully deploying LLMs.
Security, Data Privacy, and the Risks of Data Leakage
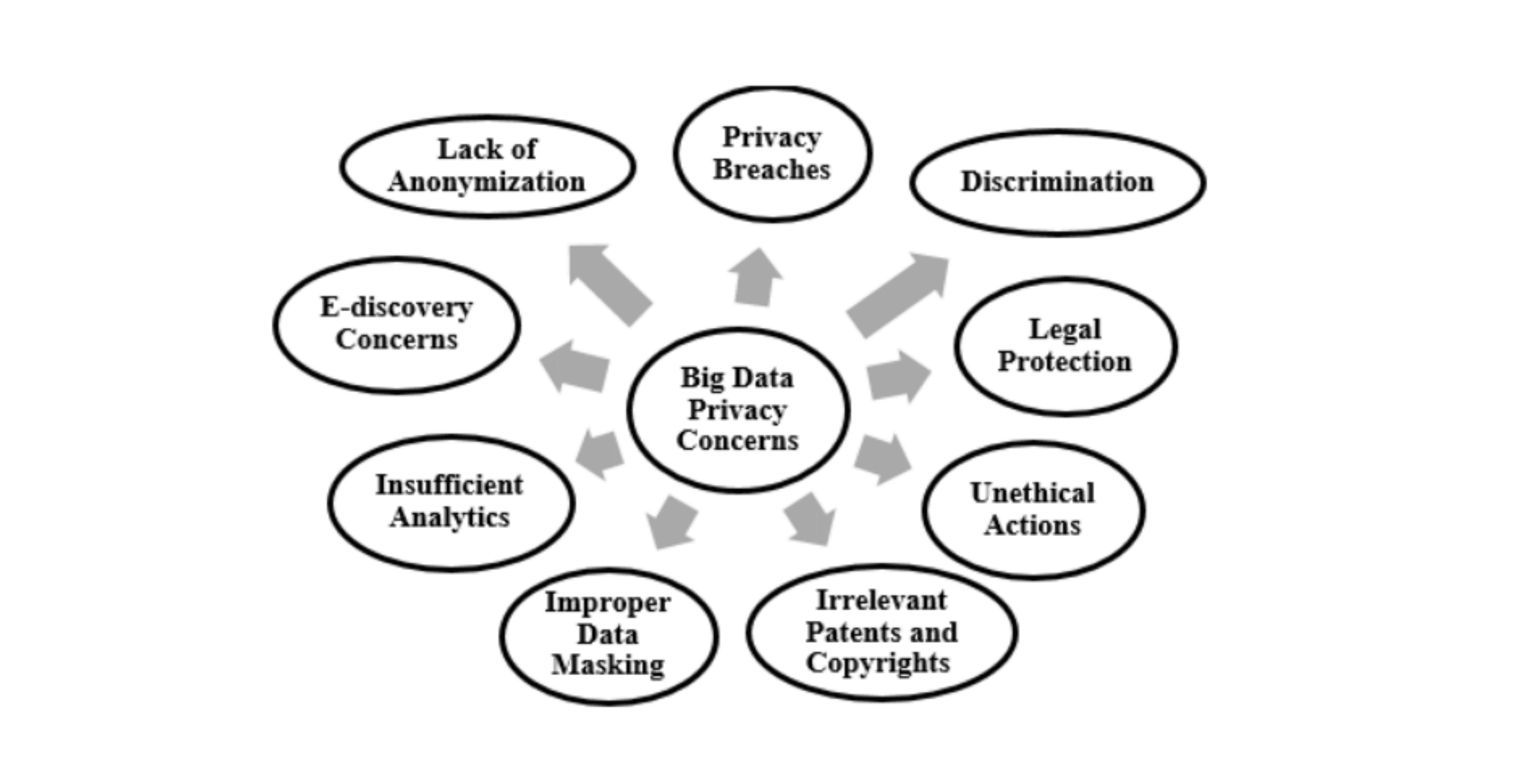
Source: Research Gate
Data security and privacy are major concerns since LLMs often process sensitive information. Ensuring these models comply with data protection regulations like GDPR and HIPAA is crucial.
The risk of data leakage through LLM interactions or training processes also poses a significant threat, necessitating robust security measures to protect data integrity and confidentiality.
These challenges underscore the complexity of deploying LLMs in an enterprise context, where the demands for accuracy, integration, and security are significantly heightened.
As we move forward, we'll explore strategies to overcome these hurdles and ensure that LLM implementations are practical, secure, and compliant with industry standards.
Learn about the Data Security Measures that Raga AI implements to protect sensitive information.
Strategies for Overcoming Challenges
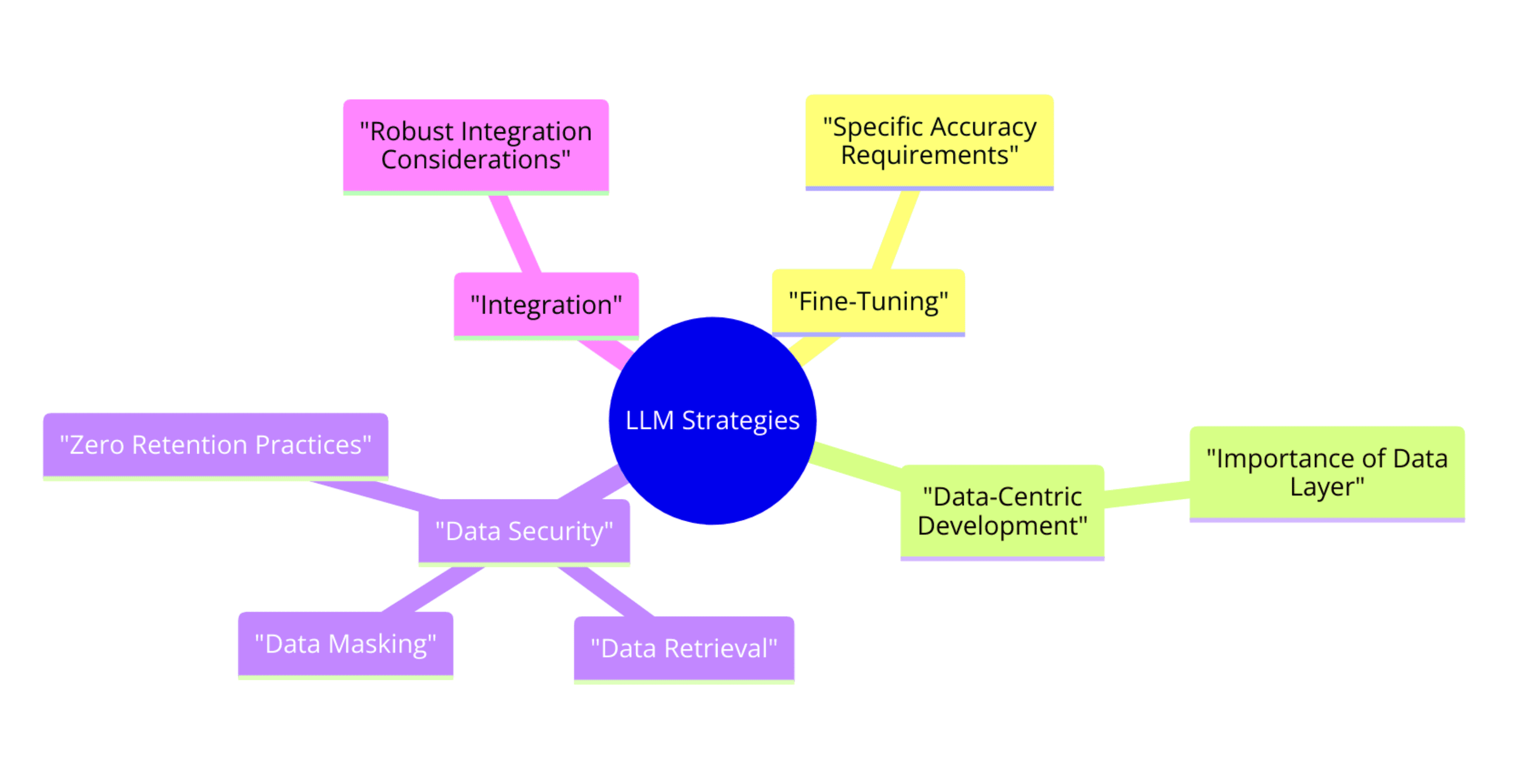
Successfully deploying LLMs in enterprise settings requires a comprehensive strategy that addresses each enterprise's unique challenges. Here are some effective strategies to consider:
Adapting LLMs to Enterprise Needs Through Fine-Tuning for Specific Accuracy Requirements
Fine-tuning the model with specific, high-quality data is essential to ensure that LLMs deliver the high accuracy needed for enterprise applications, particularly in sensitive areas like healthcare and finance.
This involves training the LLM on a dataset that closely mirrors the actual scenarios and data it will encounter in its operational environment.
Example: In healthcare, an LLM used for patient diagnosis can be fine-tuned with anonymized patient records and outcomes to improve its diagnostic accuracy, ensuring it learns from relevant, real-world medical cases.
Utilizing Data-Centric Development and the Importance of the Data Layer
A data-centric approach to LLM implementation focuses on improving the data quality used for training and operating the model rather than just tweaking the model architecture. Ensuring the robust data layer involves curating, cleaning, and continuously updating the dataset to reflect the latest information and contexts.
Example: For a banking LLM used in fraud detection, regularly updating the training dataset with the latest types of fraud cases and transaction profiles can help the model stay relevant and practical.
Securing Data Retrieval, Data Masking, and Zero Retention Practices
Security measures are critical to protect sensitive data used by LLMs. Data retrieval processes should be secure, employing encryption and secure access protocols. Data masking techniques can be used to anonymize sensitive information, ensuring that even if data is accessed inappropriately, it cannot be traced back to anyone.
Example: Implementing zero-knowledge proof systems where the LLM processes information but does not store any personally identifiable information (PII) can help maintain privacy and security.
Additional Considerations for Robust Integration
Another critical strategy is ensuring that LLMs are well-integrated into the existing technological infrastructure without disrupting current operations. This involves API integrations, middleware solutions, or custom adapters that allow LLMs to communicate seamlessly with existing databases and applications.
Example: Using API gateways to manage requests between enterprise applications and LLMs can help regulate data flow and ensure that only necessary information is shared, maintaining performance and security.
Customizing and Building Enterprise LLMs
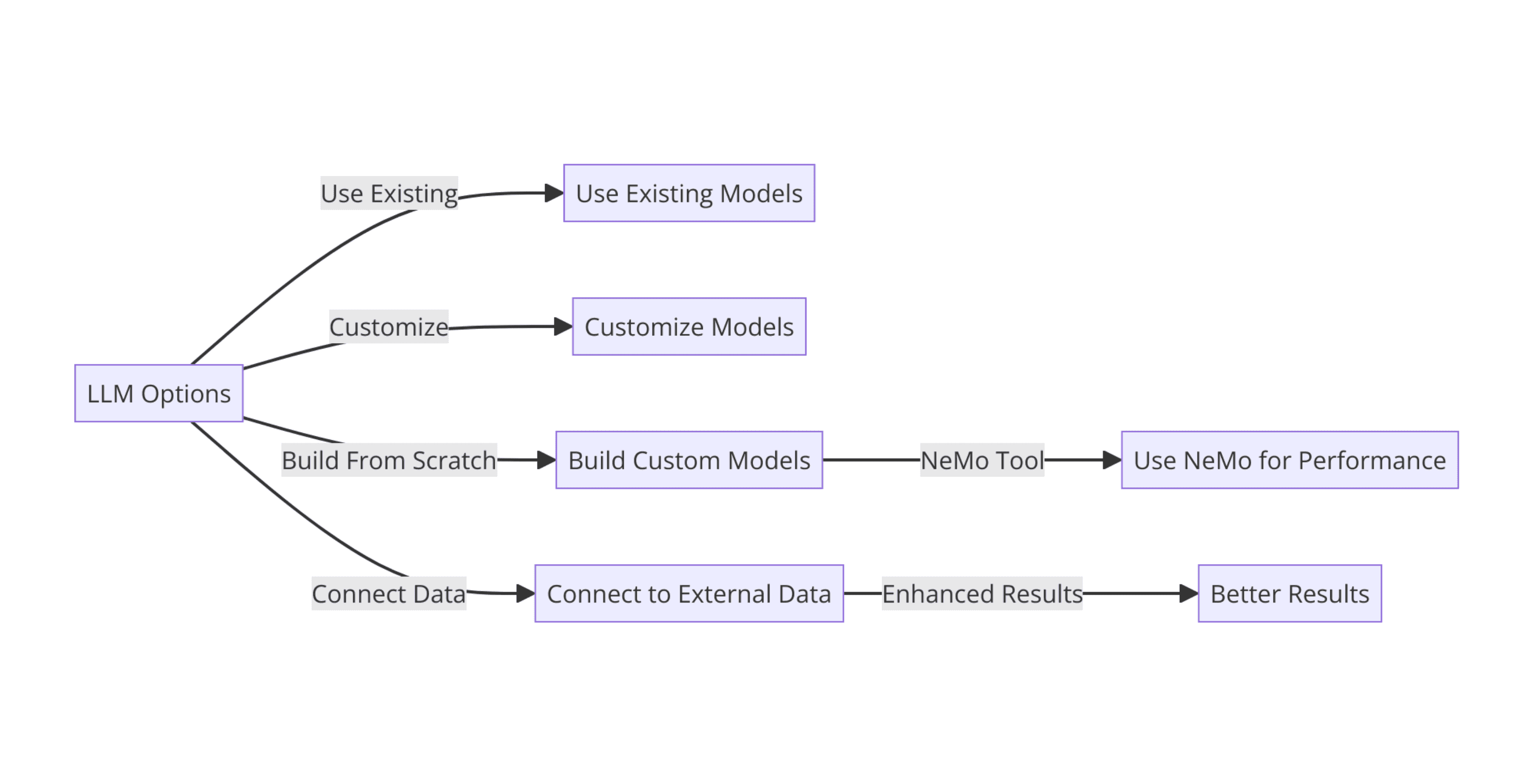
Customizing LLMs or building them from scratch offers enterprises the flexibility to address their unique challenges and objectives. Here’s how businesses can approach these tasks:
Options for Using, Customizing, or Building LLMs from Scratch
Enterprises have several paths to choose from when deploying LLMs: they can use off-the-shelf models, customize them to fit their needs or build entirely new models. Each option has its own set of benefits and considerations:
Using Standard Models: Quick deployment and initial cost savings.
Customizing Existing Models: Better performance in specific tasks while leveraging the foundational strengths of pre-trained models.
Building from Scratch: Complete control over model design, data used, and fine-tuning, but with higher costs and longer development time.
Building Custom Models with Tools like NeMo for Enhanced Performance
For those opting to build or customize models, tools like NVIDIA’s NeMo offer frameworks to create state-of-the-art conversational AI models. NeMo allows developers to fine-tune models on specific datasets, incorporate unique vocabularies, and adjust model architectures to suit particular tasks better.
Example: A telecom company could use NeMo to train a model specifically to understand and generate responses based on telecommunications jargon and customer service interactions, providing more accurate and contextually appropriate customer service.
Connecting an LLM to External Data for Better Results
Enhancing an LLM’s capabilities often involves integrating it with external data sources to enrich the context it has access to. Depending on the application, this might include customer databases, product catalogs, or real-time market data.
Example: An investment firm might integrate its LLM with real-time stock market data and historical investment databases to provide clients with more insightful, data-driven investment advice.
Technical Approach:
import openai
def integrate_external_data(model, data_sources):
enriched_context = ""
for source in data_sources:
enriched_context += extract_data(source)
response = openai.Completion.create(
engine=model,
prompt="Analyze the following market trends: " + enriched_context,
max_tokens=150 )
return response.choices[0].text
def extract_data(source):
# This is a placeholder for a function that fetches and formats data from a
source
return "Latest stock market trends from " + source
# Example of integrating an LLM with external data sources
model = "text-davinci-003"
data_sources = ["NASDAQ updates", "NYSE top movers"]
analysis = integrate_external_data(model, data_sources)
print("Market Analysis: ", analysis)
This example shows how an LLM can be enhanced by connecting it to relevant external data, providing more precise and valuable outputs based on the latest information.
Customizing and building enterprise-specific LLMs allows businesses to meet their unique needs more effectively and maintain a competitive edge by leveraging tailored AI solutions.
Now, let's explore the critical aspects of integrating Large Language Models (LLMs) into enterprise systems and ensuring their security.
This section will cover effectively merging LLMs into existing business processes and safeguarding sensitive information against potential threats.
Read more on Enhancing LLM Reliability with RagaAI
Integration and Security
Integrating LLMs into enterprise environments presents its own set of challenges, especially when it comes to ensuring that these systems can interact seamlessly with existing infrastructure and manage data securely.
Overcoming Integration Challenges for Personalized Customer Responses
Integrating LLMs requires a thoughtful approach to connect these models with existing customer relationship management (CRM) systems, databases, and other enterprise applications. This integration enables LLMs to access the necessary data for personalized responses and support.
Example: By linking an LLM to a CRM system, a business can enable the model to access customer purchase histories, support tickets, and preferences. This integration allows the LLM to deliver highly personalized customer service interactions, such as suggesting products based on past purchases or quickly resolving support issues by referring to previous tickets.
Strategies for Secure Data Retrieval and Protecting Sensitive Information
Ensuring the security of data accessed and generated by LLMs is paramount. Strategies to secure data include using encryption for data in transit and at rest, implementing robust access controls, and applying anonymization techniques where possible.
Technical Insight:
Encryption: Implement SSL/TLS for data transmitted between the LLM and other systems. Use strong encryption standards like AES-256 for data at rest.
Access Controls: Set up role-based access controls (RBAC) to ensure only authorized personnel can interact with the LLM and its data.
Read more on Addressing Integration Challenges with RagaAI
Monitoring and Aligning LLM Behavior with Enterprise Goals
Continuous monitoring of LLM activities is crucial to ensure they perform as intended and adhere to company policies and ethical guidelines. Setting up monitoring tools to track the performance and outputs of LLMs can help quickly identify and address any deviations from expected behaviors.
Example: Use logging and auditing tools to record all interactions with the LLM. Analyze logs regularly to detect any anomalies or unauthorized attempts to access the system. Implement automated alerts to notify the appropriate personnel when irregular patterns are detected.
import logging
# Setup logging configuration
logging.basicConfig(filename='llm_activity.log', level=logging.INFO, format='%(asctime)s:%(levelname)s:%(message)s')
def log_interaction(user_id, query, response):
logging.info(f"User ID: {user_id}, Query: {query}, Response: {response}")
# Example of logging an interaction
user_id = 12345
query = "What products are recommended for someone who bought X?"
response = "Based on previous purchases, we recommend Y and Z."
log_interaction(user_id, query, response)
This script provides a basic framework for logging interactions with an LLM, helping maintain a transparent record that can be audited for compliance and monitoring purposes.
Ensuring LLMs are Kept on Track and Secure Through Monitoring and Guardrails
Setting up continuous monitoring systems and defining clear operational guardrails are essential to maintaining the integrity and effectiveness of LLM deployments. Monitoring tools can track LLMs' performance and behavior, while guardrails ensure that the models operate within predefined ethical and operational boundaries.
Example Implementation:
def monitor_model_performance(metrics):
if metrics['accuracy'] < threshold:
alert("Model accuracy below expected threshold.")
if metrics['response_time'] > max_response_time:
optimize_model_inference()
def optimize_model_inference():
# Implement optimization logic (e.g., load balancing, resource allocation)
print("Optimizing model inference for better performance.")
# Simulated monitoring data
model_metrics = {'accuracy': 92.5, 'response_time': 0.300} # 300 ms
monitor_model_performance(model_metrics)
This example demonstrates an essential monitoring function that checks model performance against certain thresholds and triggers optimization procedures if needed.
Optimizing LLMs for enterprise applications involves technical adjustments and enhancements and ensuring these systems integrate smoothly with existing corporate infrastructure and policies. This holistic approach helps businesses harness the full power of LLMs while maintaining control over their deployment and usage.
Let's wrap up our comprehensive exploration of implementing Large Language Models (LLMs) in the enterprise context. We'll recap the importance of LLMs, highlight key strategies to overcome challenges, and reiterate the value of customization and data-centric approaches.
Conclusion: Embracing LLMs in Enterprise
Integrating and optimizing large language models (LLMs) within enterprise systems is challenging and rewarding.
As we've explored, LLMs offer tremendous potential to revolutionize various aspects of business operations, from enhancing customer interactions to streamlining complex analytical tasks.
Embracing LLMs requires a commitment to continuous learning and adaptation, but the rewards justify the effort.
As AI technology evolves, so will the opportunities for its application in business. Enterprises that stay ahead of these trends, continuously refine their approaches, and invest in cutting-edge solutions will solve complex challenges and gain a significant competitive advantage.
By understanding and addressing the unique challenges of implementing LLMs and by leveraging the right strategies and tools, enterprises can fully realize the potential of these advanced AI models.
Though filled with technical and strategic challenges, this journey opens up a world of possibilities for innovation and efficiency.
Subscribe to our newsletter to never miss an update
Other articles


Agents Talk, Models Think: A2A + MCP for Enterprise Agentic AI

Riya Parikh, Nitai Agarwal
Apr 27, 2025
Read the article
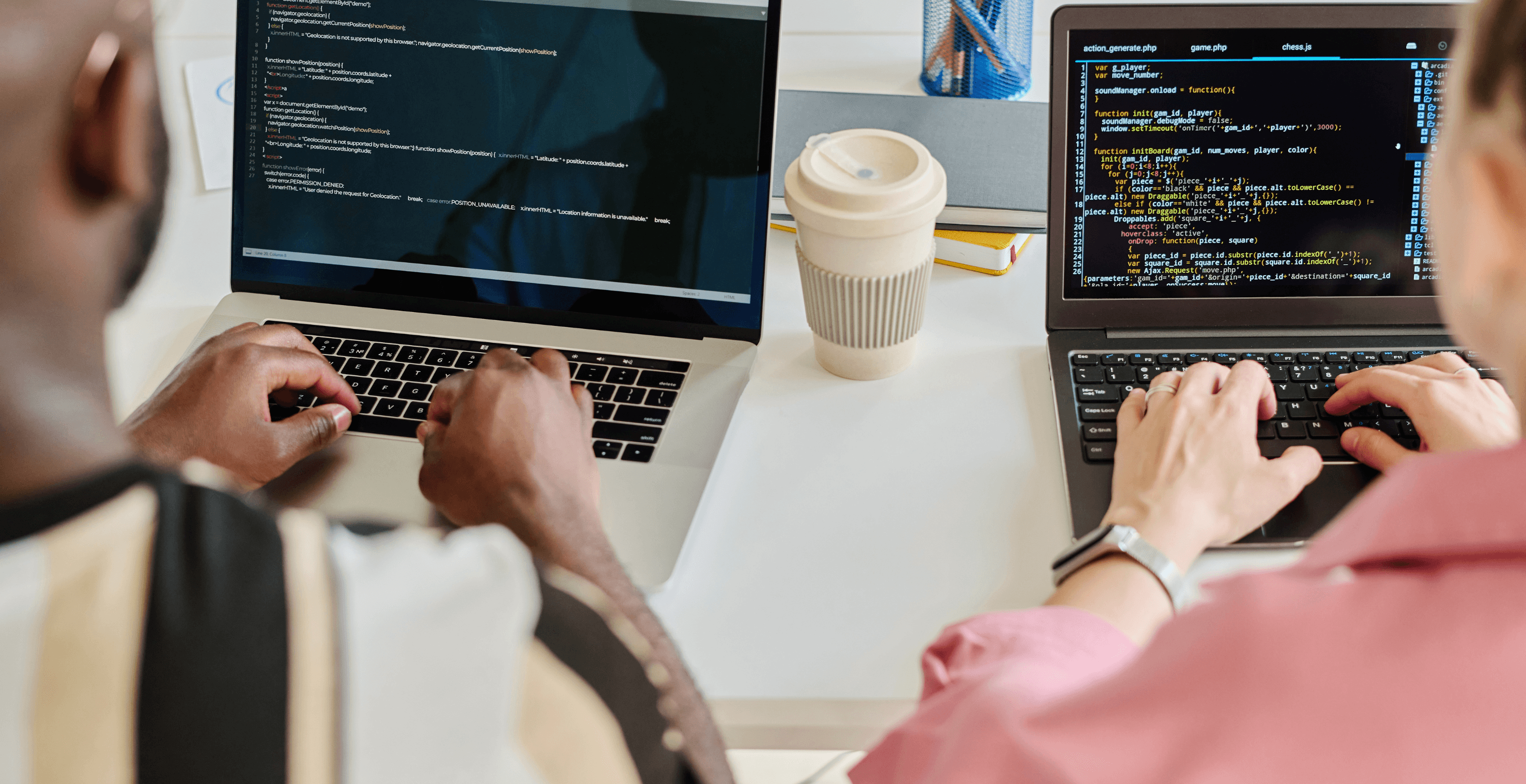
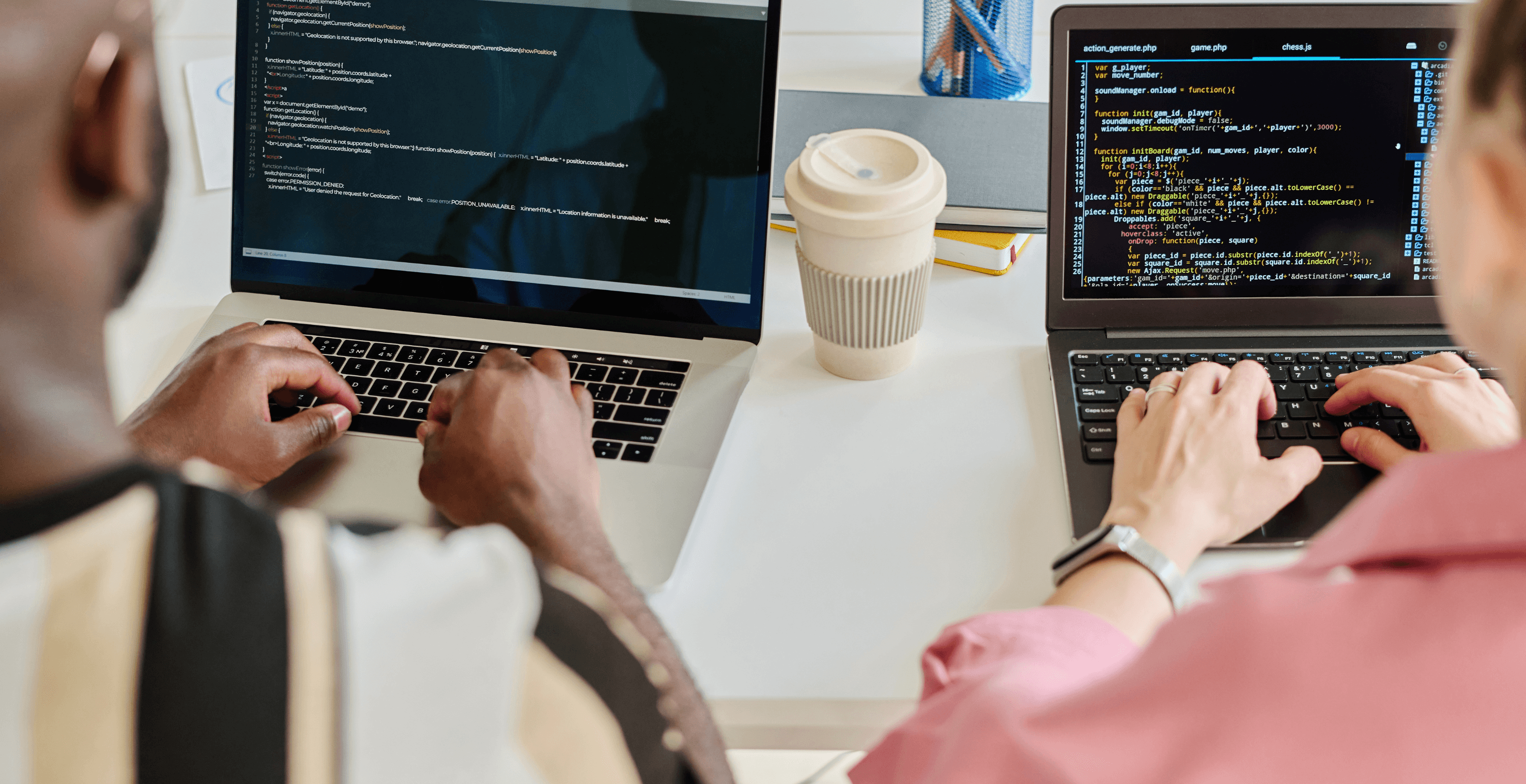
Understanding NeMo Guardrails: A Toolkit for LLM Security

Rehan Asif
Dec 24, 2024
Read the article


Understanding Differences in Large vs Small Language Models (LLM vs SLM)

Rehan Asif
Dec 21, 2024
Read the article