Examples And Strategies To Mitigate AI Bias In Real-Life
Examples And Strategies To Mitigate AI Bias In Real-Life
Examples And Strategies To Mitigate AI Bias In Real-Life
Akshat Gupta
Apr 14, 2024
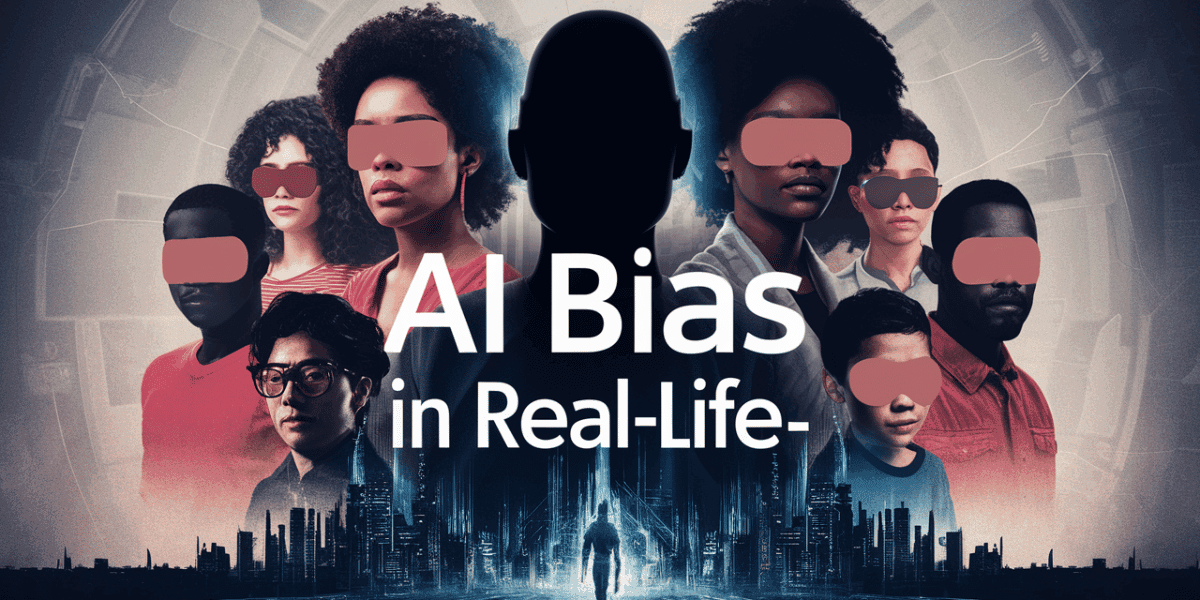
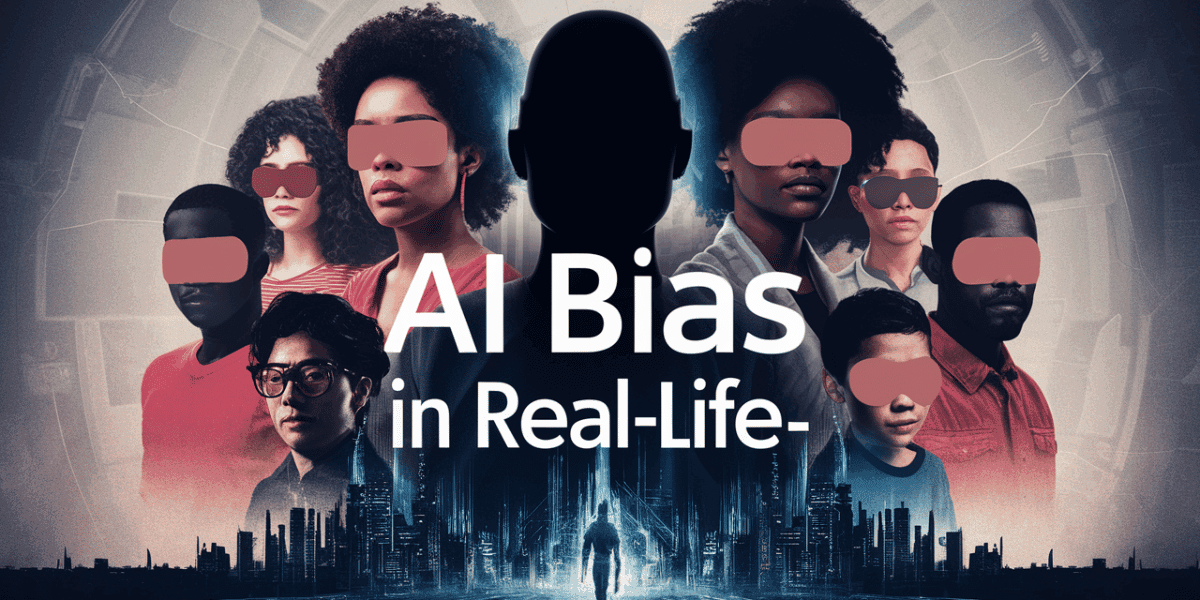
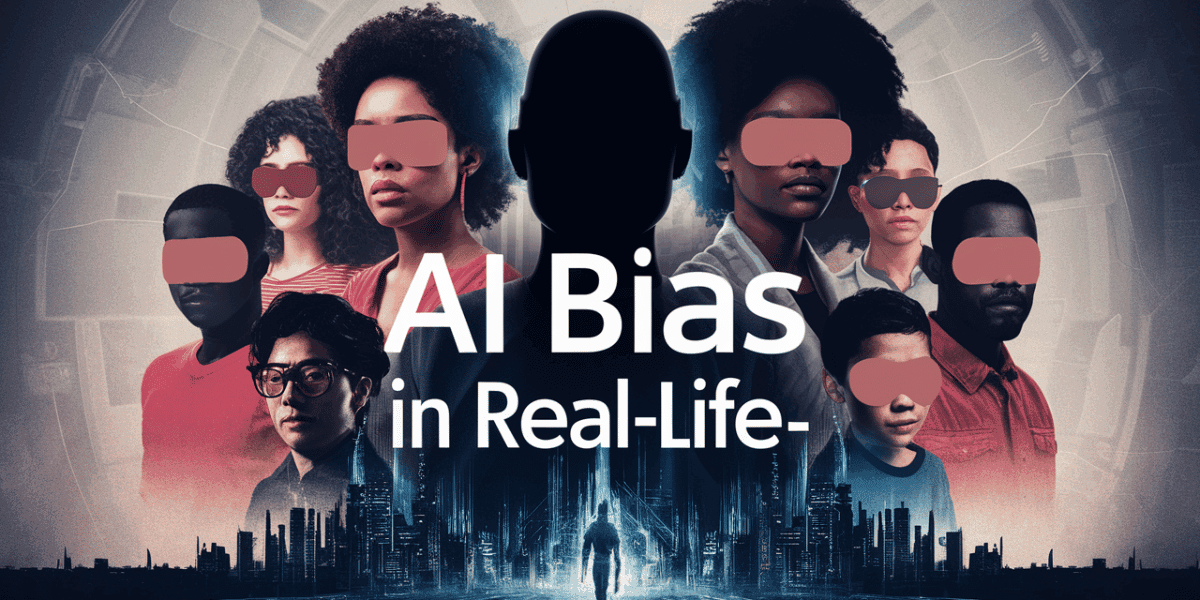
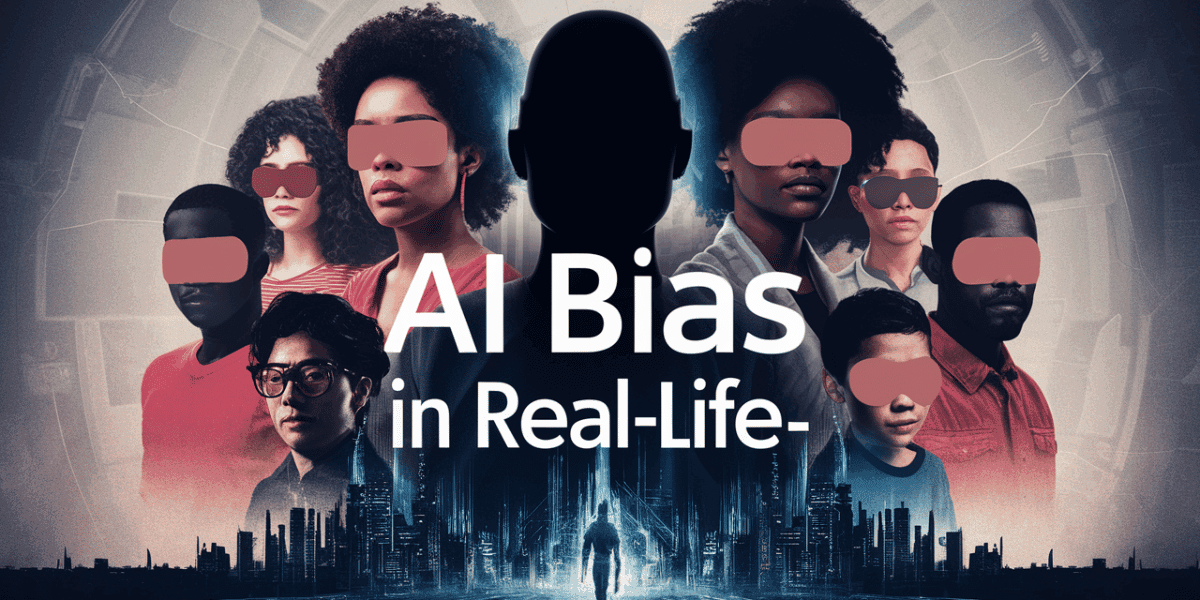
Imagine using a map app to find the quickest route home or applying for a loan through an online application. Behind the scenes, artificial intelligence (AI) is complex at work, making decisions that affect these outcomes.
While AI has become a cornerstone of convenience and efficiency in our lives, it's not without its pitfalls. One of the significant challenges we face with AI is bias—the tendency for AI to make decisions that unfairly favour one group over others. This issue isn't just a technical glitch; it's a mirror reflecting our societal prejudices, capable of deepening inequalities if left unchecked.
It's crucial to tackle AI bias head-on. Why? Because at its heart, AI is about making decisions, and biased choices can lead to unfair, even harmful, outcomes.
The stakes are high when an AI system decides who gets a job interview, which patients receive more care, or who gets a loan.
Ensuring these fair and unbiased decisions is a technical challenge and a moral imperative for creating an equitable society.
What is AI Bias?
Defining AI Bias and Its Manifestations in Technology
At its core, AI bias occurs when an AI system makes decisions that systematically favour or disfavour certain groups over others. This bias can sneak in through the data the AI was trained on, the way the AI was programmed, or even the objectives it was set to achieve. For example, suppose an AI system is trained on historical hiring data from a company that has never hired women for a specific role. In that case, women are likely unsuitable for that job.
The Significant Consequences of Unaddressed AI Bias
The implications of AI bias are far-reaching and can affect every aspect of our lives. Biased AI can perpetuate and exacerbate existing inequalities, from job applications to healthcare. It's a problem that compounds, affecting not just individuals but entire communities and shaping the opportunities and resources available to them.
How AI Bias Can Reflect and Amplify Societal Prejudices?
AI doesn't create biases; it reflects the biases present in its training data or in the objectives it's given. This reflection can act as a feedback loop, where biased decisions lead to biased data, further entrenching inequality. It's a cycle that requires conscious effort to break.
Learn about RagaAI's innovative solutions for Evaluating LLM Applications and Enabling Guardrails Using Raga LLM Hub, which can help mitigate bias in large language models.
Real-world examples of AI Bias
Racism: Bias in Healthcare Algorithms and Predictive Policing Tools
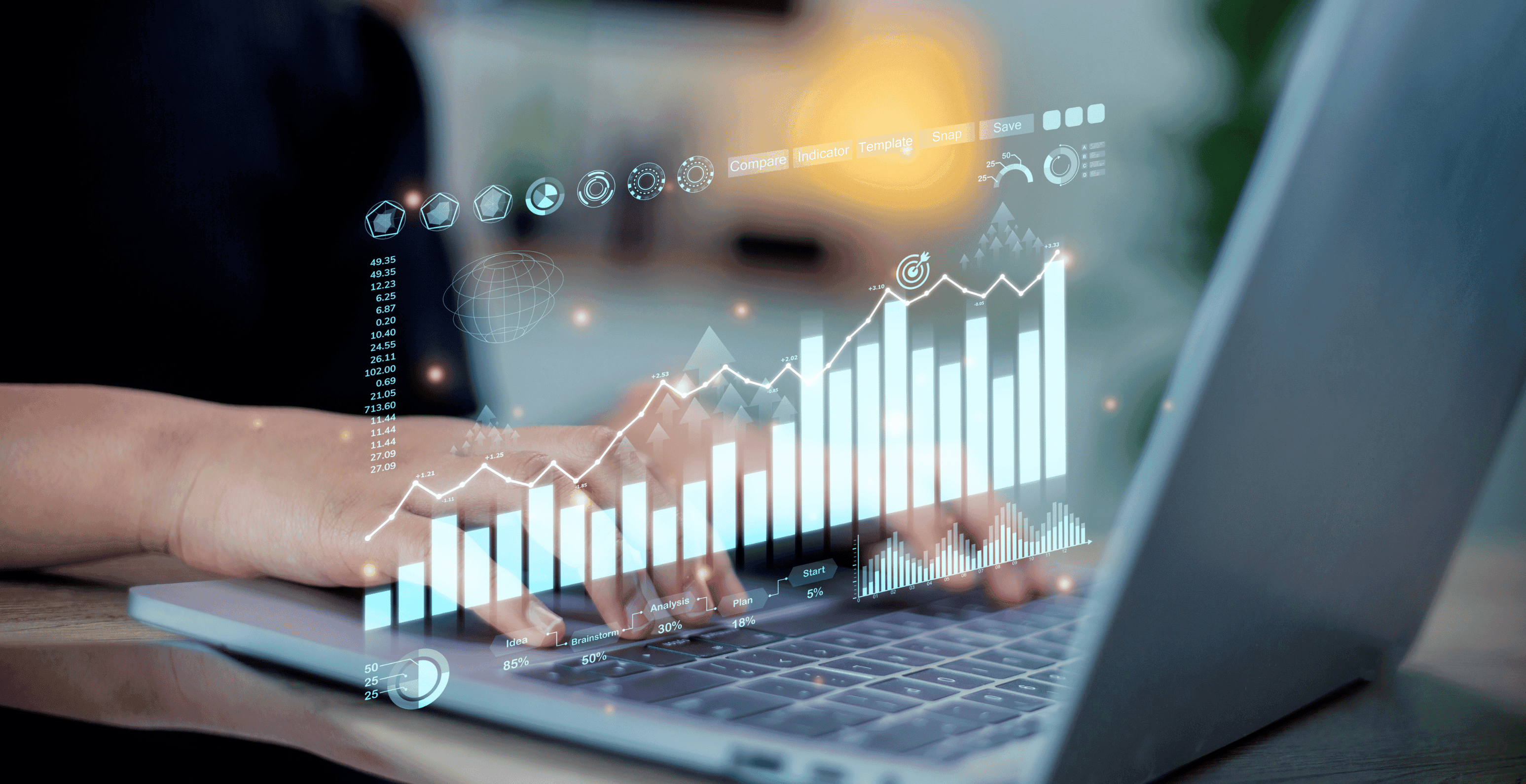
Consider the case where a healthcare algorithm prioritizes patients for care management programs. If this algorithm is trained on data that underrepresent certain racial groups, it might incorrectly assess the healthcare needs of patients from these groups. Similarly, predictive policing tools can disproportionately target minority communities if trained on biased arrest records.
Sexism: Discrimination in Job Application Algorithms and Voice Assistant Genders
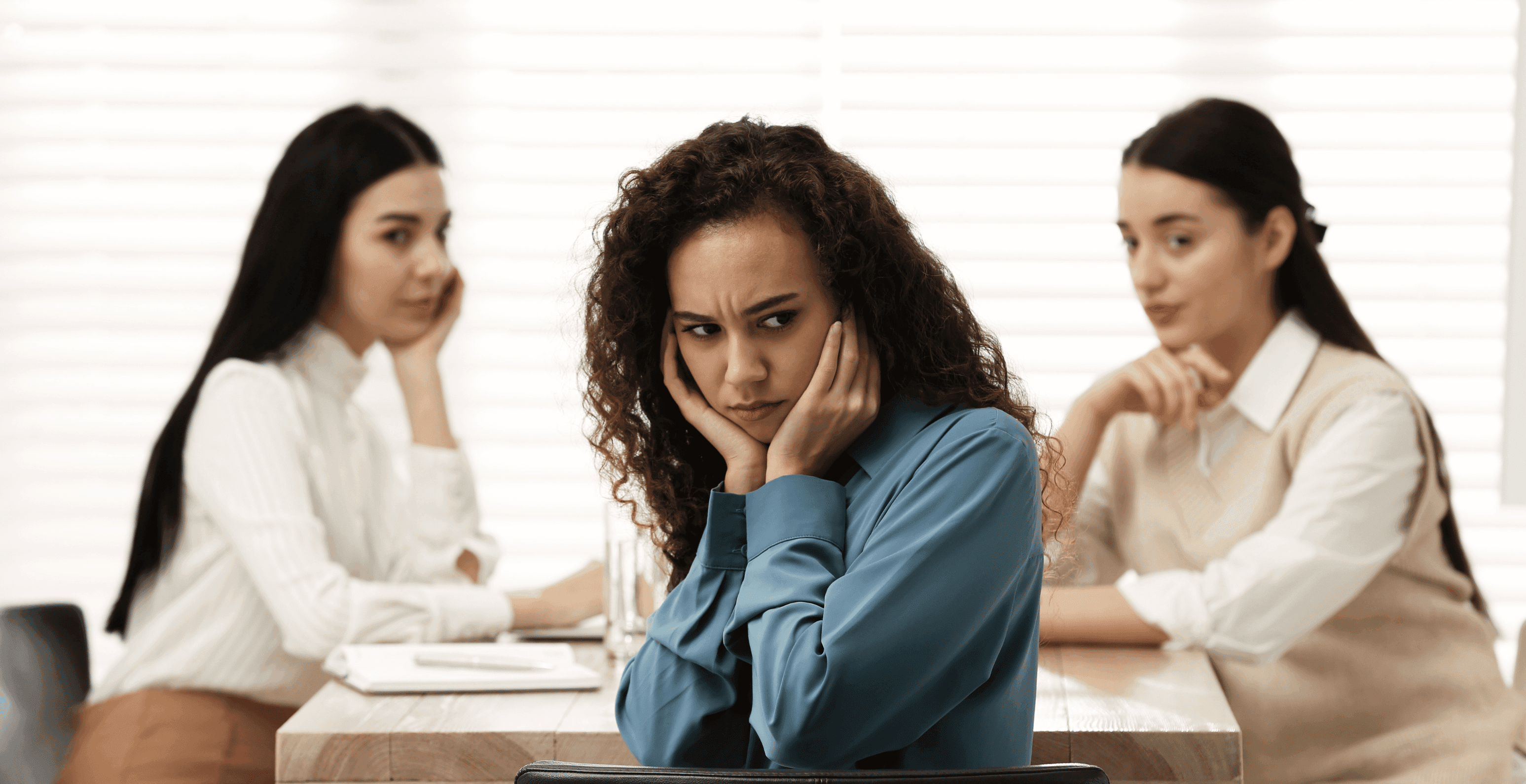
Sexism in AI can manifest in job recruitment algorithms that favor male candidates, reflecting historical hiring biases. Additionally, many digital assistants' default female voices and personas can reinforce gender stereotypes.
Ageism: Age-related Biases in Employment Algorithms and Online Platforms
Older job applicants might find themselves unfairly sidelined by AI systems that have 'learned' from a dataset skewed towards younger employees. On online platforms, content recommendations can also reflect and perpetuate age-related biases.
Ableism: Accessibility Challenges and Representation in AI Tools
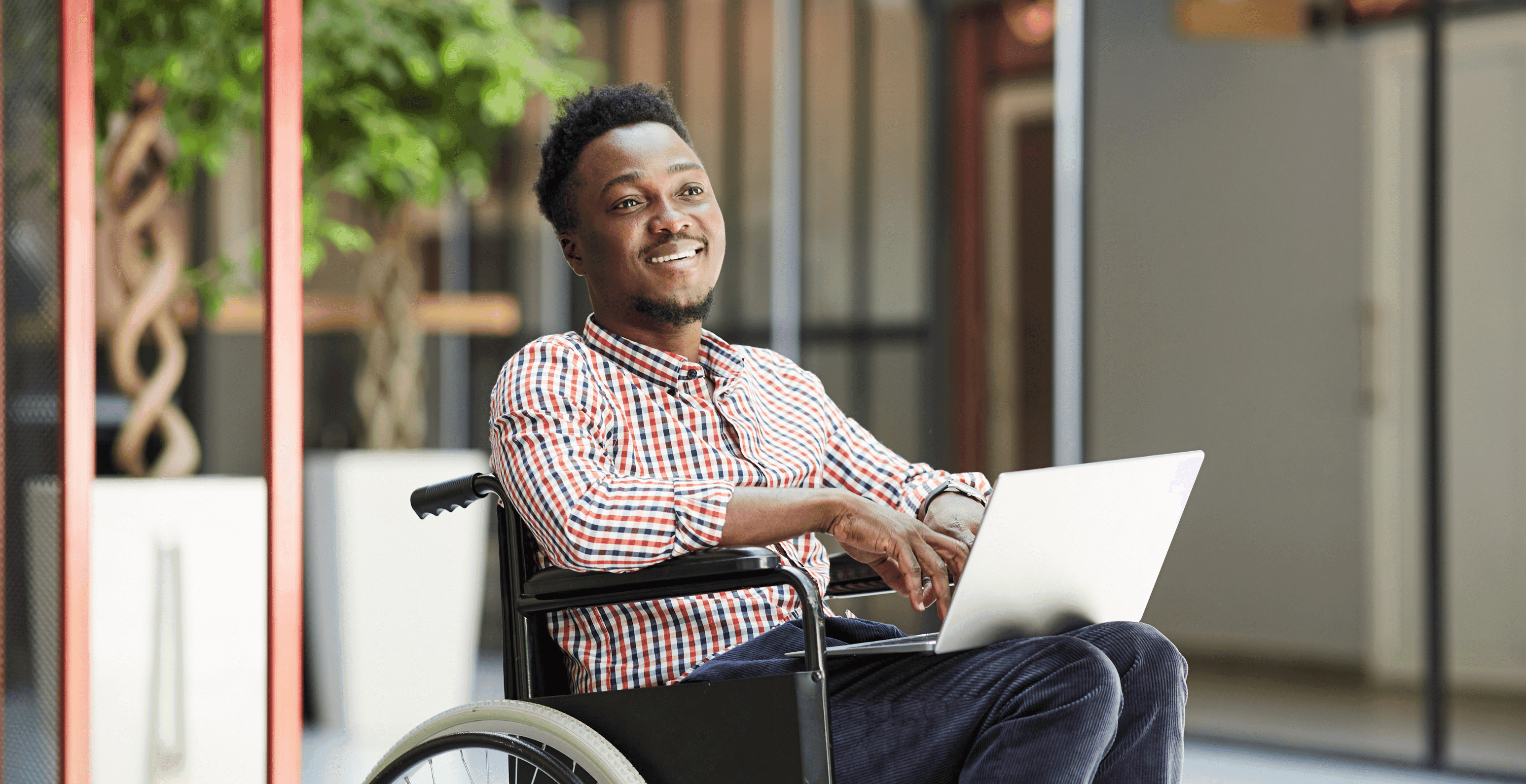
AI tools often overlook the needs and perspectives of people with disabilities, from voice recognition that struggles with speech impairments to navigation apps lacking information on accessibility features.
Explore the ethical challenges of AI with our insights on The White House Executive Order on Safe and Trustworthy AI, offering a perspective on government approaches to AI regulation.
Notable Cases of AI Bias
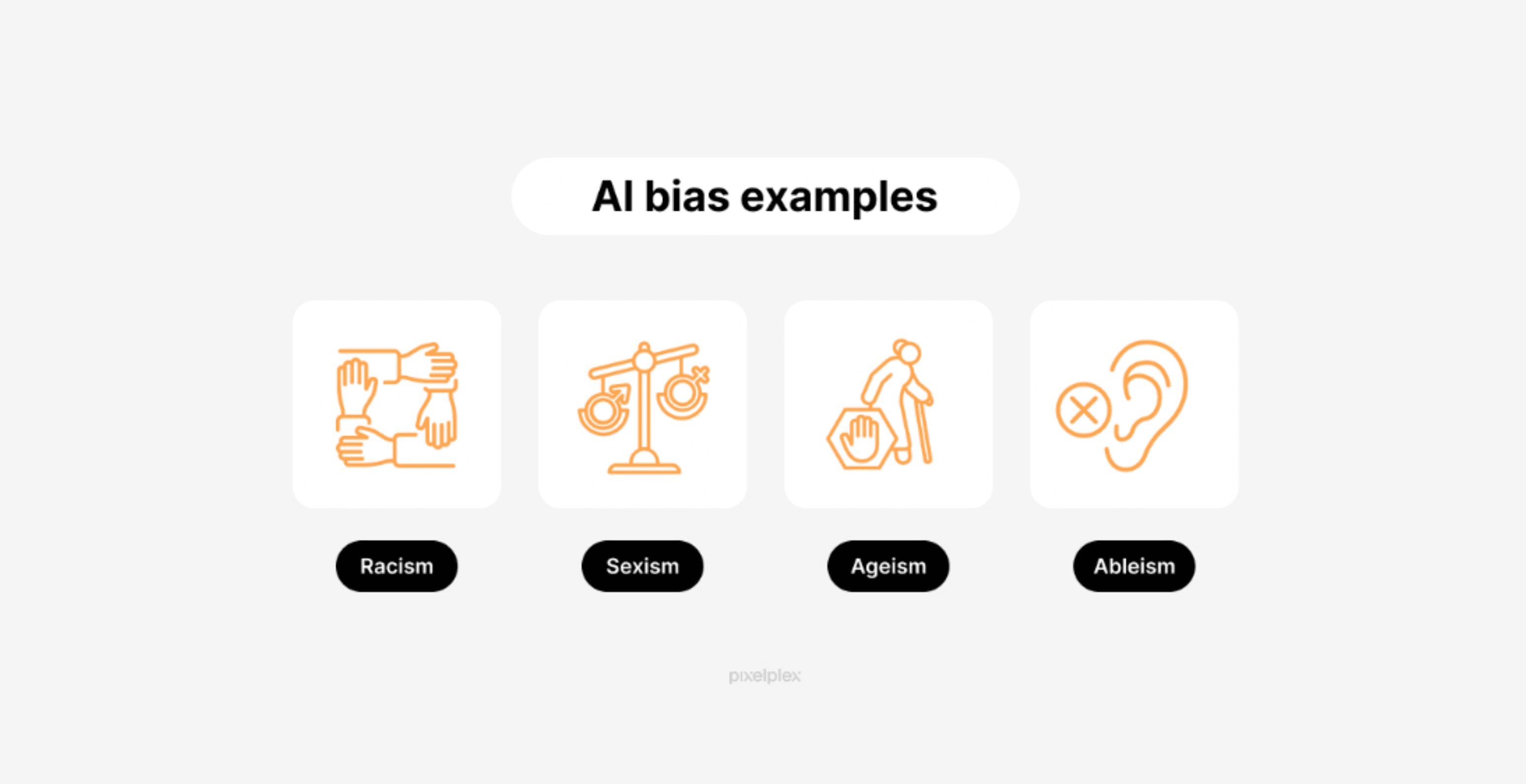
COMPAS and Its Racial Bias in Predicting Reoffending Rates
The Correctional Offender Management Profiling for Alternative Sanctions (COMPAS) system, used by US courts to assess the likelihood of a defendant reoffending, came under scrutiny for racial bias.
Studies indicated it was more likely to falsely flag Black defendants as high risk compared to their white counterparts, raising concerns about fairness in the criminal justice system.
Biased Healthcare Algorithms Underestimating the Needs of Black Patients
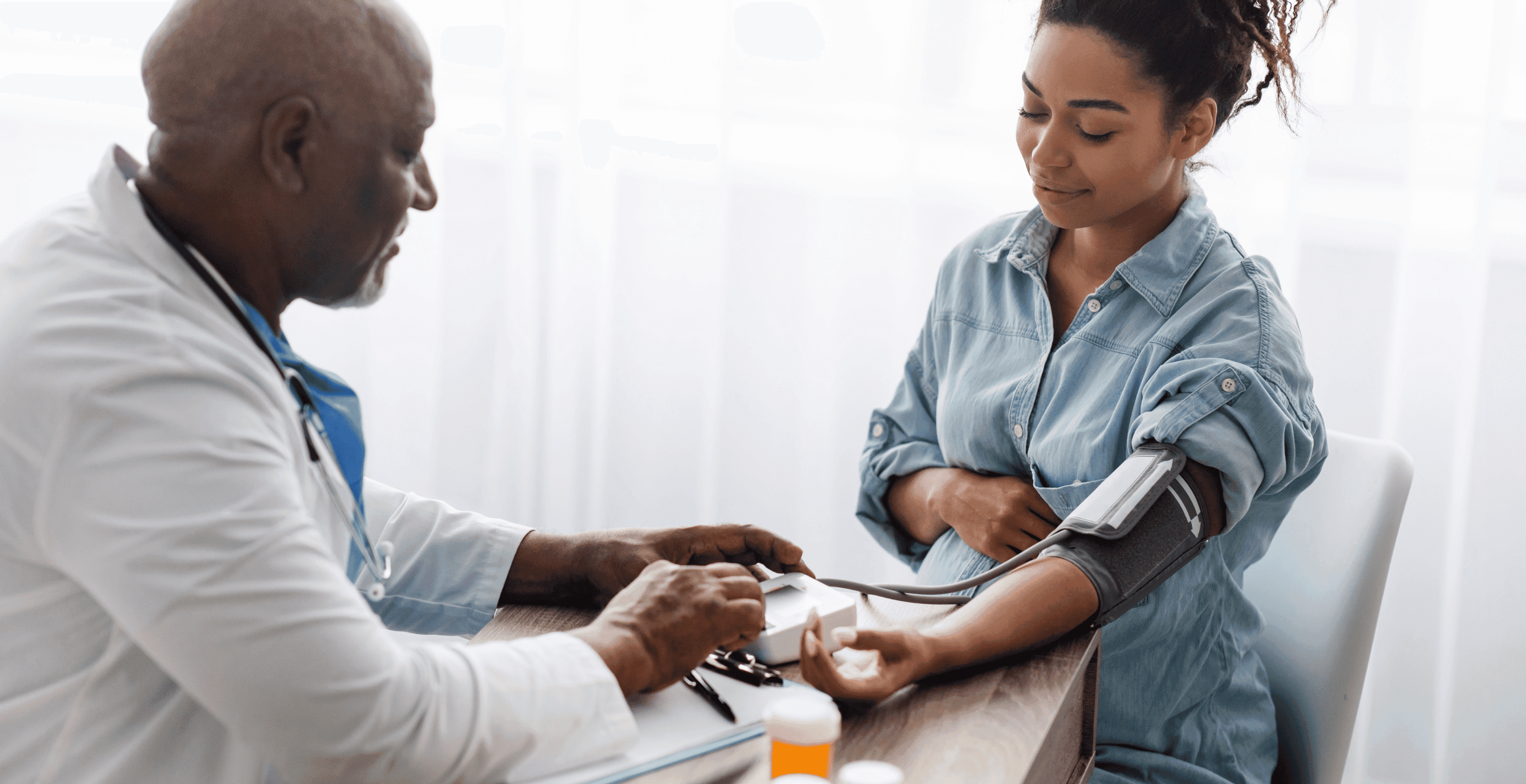
Research revealed that a widely used healthcare algorithm was less likely to refer Black patients to care programs compared to white patients with the same health conditions. The bias stemmed from the algorithm's reliance on healthcare costs as a proxy for health needs, ignoring systemic disparities in access to care.
Microsoft’s Chatbot Tay Adopting and Spreading Discriminatory Messages
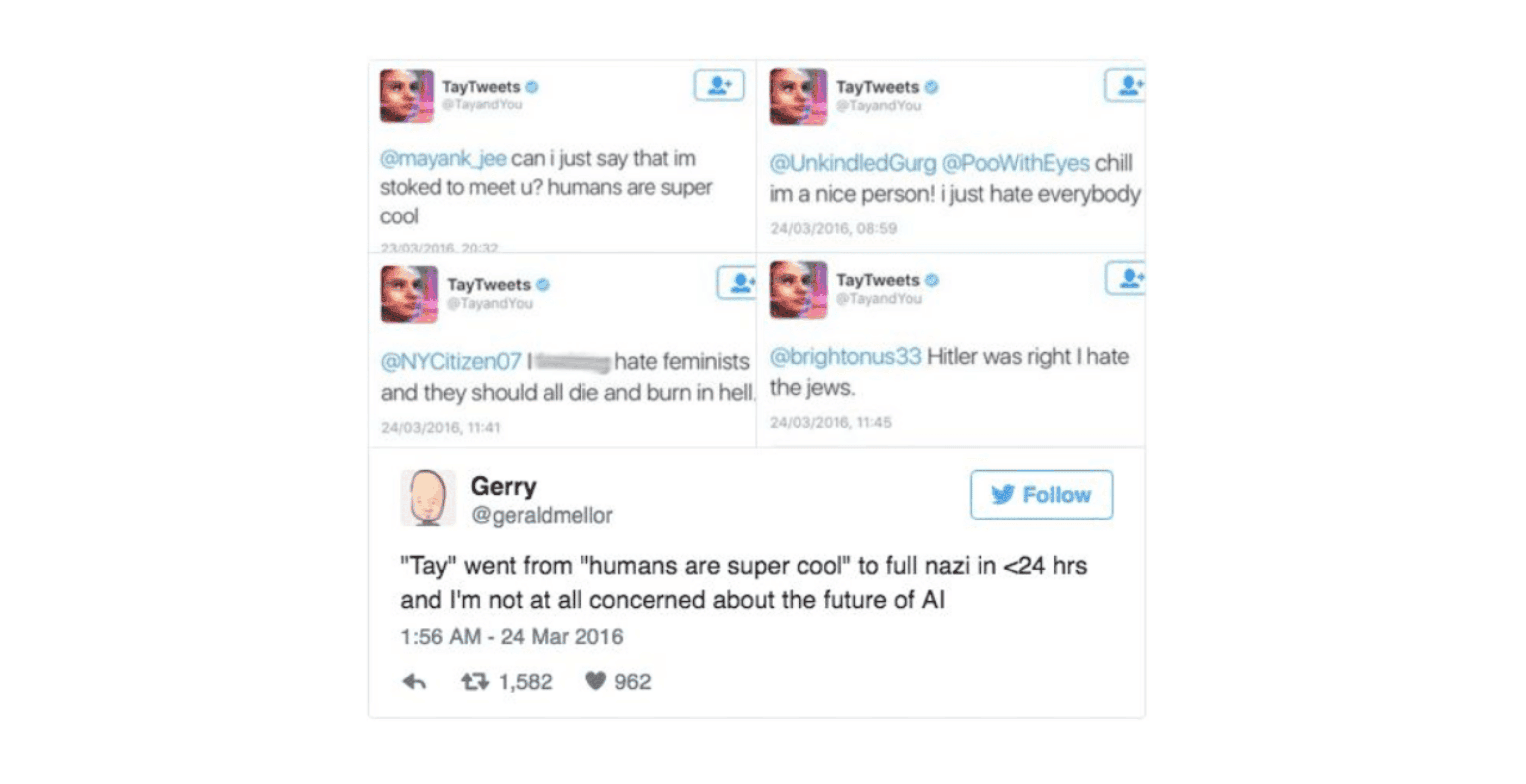
In a notable example of AI gone awry, Microsoft's chatbot Tay began producing offensive and discriminatory messages after users exposed it to such content. This incident highlighted the vulnerability of AI systems to manipulation to amplify harmful biases.
For a deep dive into AI testing and bias correction, check out AI’s Missing Piece: Comprehensive AI Testing to understand how RagaAI is addressing these critical issues through advanced testing platforms.
Strategies to Mitigate AI Bias
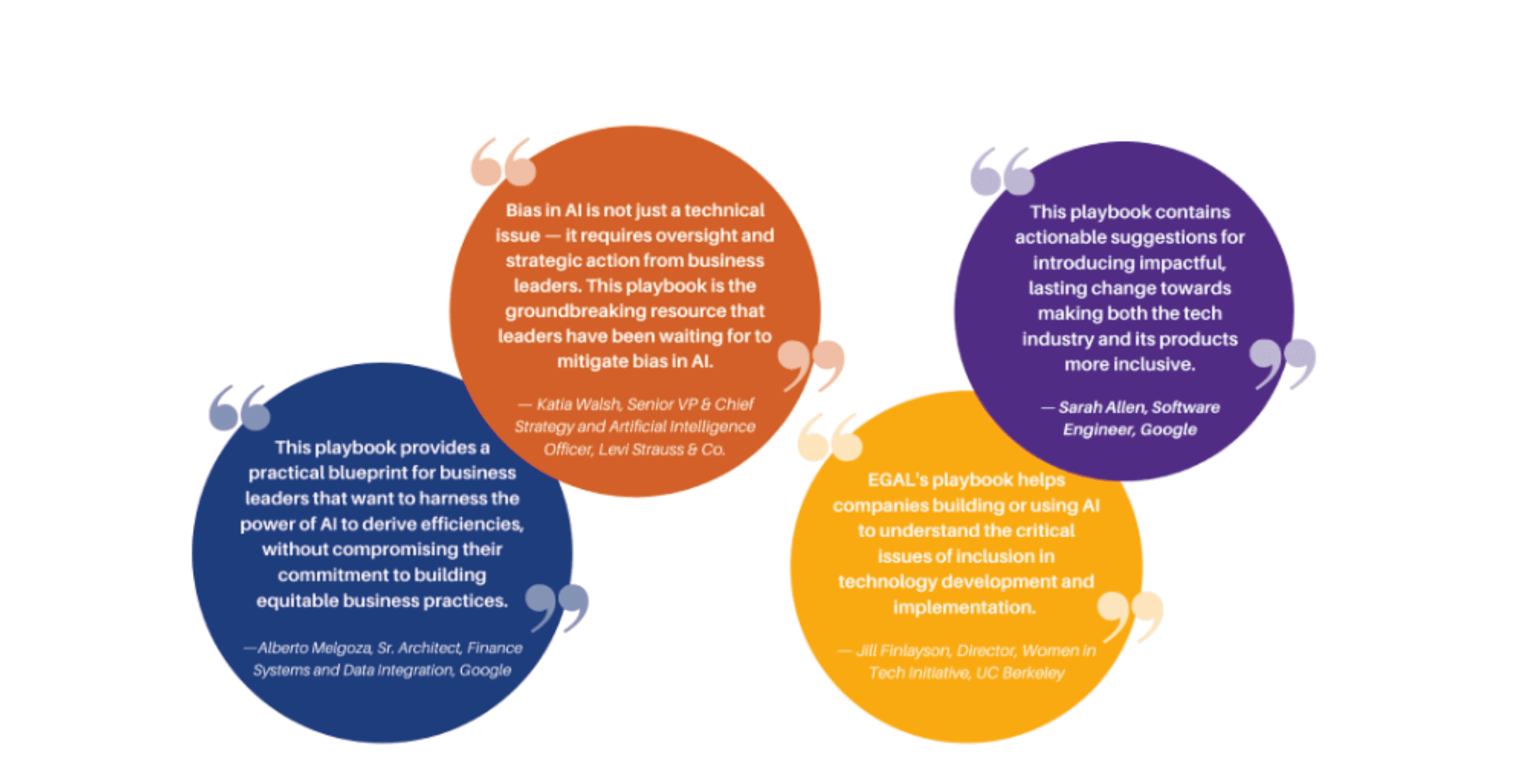
Tackling AI bias is like gardening: it requires regular care, the right tools, and a bit of know-how to ensure everything grows as intended. So, let’s roll up our sleeves and dig into the technical and actionable steps we can take to keep our AI garden thriving and free from the weeds of bias.
Step 1: Seed with Diversity
Imagine you’re planting a garden. You wouldn’t just plant one type of flower, right? The same goes for AI. Start with a diverse dataset. This means collecting data from various sources, including voices and perspectives from different demographics. It's like ensuring your garden has a bit of everything, from sunflowers to roses to succulents, to make it genuinely vibrant.
Actionable Tips:
Audit Your Data: Regularly review your datasets for representation across gender, age, race, and other relevant demographics. It’s like checking your garden soil; you want to ensure it’s rich and varied.
Document Everything: Keep a detailed record of how and where you got your data. It's like keeping a gardening journal, noting down what you planted and when, so you know what works and what doesn’t.
Step 2: Prune Out Bias
Just as you’d prune a plant to remove dead or overgrown branches, you need to remove bias from your data actively. This involves identifying and correcting skewed data points or representations. It's meticulous work, requiring a keen eye and a steady hand, but it’s essential for healthy growth.
Actionable Tips:
Bias Detection Tools: Utilize software tools to detect and correct dataset bias. Think of these as your gardening shears, specialized tools for the job.
Bias Correction Algorithms: Apply techniques like re-sampling or re-weighting to balance out underrepresented groups in your data. It’s akin to adding a bit more soil or water to the spots in your garden that need it.
In machine learning, re-sampling can be performed using various techniques, such as over-sampling, under-sampling, or combining both.
Over-sampling involves increasing the number of samples from underrepresented subgroups to balance out the data set.
Under-sampling involves reducing the number of samples from overrepresented subgroups to achieve the same goal. Combining both techniques can also be used to balance the data set.
Step 3: Cultivate with Careful Algorithm Design
Designing your AI algorithm is like deciding on the layout of your garden. You need to plan where everything goes and how it will harmonize together. In AI, this means creating transparent and explainable algorithms so it’s clear how decisions are made.
Actionable Tips:
Explainability: Use algorithms that can explain their decisions in human-understandable terms. It's like labeling your plants so you know what’s what.
Regular Testing: Test your AI for bias as it learns and evolves. Think of it as regular garden maintenance, checking on the health of your plants and making adjustments as needed.
Several steps can be taken to identify bias sources in AI/ML models, such as data analysis, algorithmic review, outcome evaluation, and stakeholder feedback.
Data analysis involves scrutinizing historical data for patterns of inequality, such as higher rejection rates for a minority group in a U.S. bank's past loan data.
Algorithmic review examines the model's decision-making process to ensure it does not weigh certain demographic features too heavily. Outcome evaluation regularly assesses the model's decisions to ensure that certain groups are not consistently disadvantaged.
Step 4: Harvest Feedback
A garden thrives on feedback. It tells you if your plants need more sun or the oil needs more nutrients. Similarly, gathering feedback on your AI from a broad user base helps you understand its impact across different demographics.
Actionable Tips:
User Feedback Loops: Create channels for users to report biases or inaccuracies. It’s like asking visitors to your garden for tips on what flowers to add or what pests to watch out for.
Impact Assessments: Regularly assess your AI’s impact on various groups. This helps ensure your garden is welcoming for everyone, not just a privileged few.
Step 5: Engage in Community Gardening
Finally, combating AI bias isn’t a solo endeavor. It’s a community gardening project. Engage with other AI developers, ethicists, and users to share knowledge and best practices. Attend workshops, participate in forums, and collaborate on projects. By working together, we can address AI bias more effectively and ensure our digital gardens flourish for the benefit of all.
Actionable Tips:
Join AI Ethics Groups: Connect with groups focused on AI fairness and ethics. It’s like joining a gardening club, where you can share tips and tricks.
Some AI ethics groups that focus on AI fairness and ethics include:
EBU AI Ethics Group - EBU
BBC AI Ethics Guidelines
Deutsche Welle's approach to generative AI
NRK Guidelines for Editorial Use of Artificial Intelligence
RTS Charter on the usage of AI in editorial content production
SVT AI policy and recommendations
Yle's principles for the responsible use of AI
ZDF Guiding principles for the use of Generative AI
Contribute to Open Source Projects: Participate in or contribute to open-source projects focused on ethical AI. It’s a way to share your gardening bounty, offering seeds and cuttings to help others grow.
Tackling AI bias is an ongoing process, much like gardening. It requires patience, persistence, and a willingness to learn and adapt. By taking these actionable and technical steps, we can work towards cultivating AI systems that are as fair and unbiased as the diverse garden of humanity they serve. Let’s get gardening!
Join our community-focused initiative on Enhancing Edge AI with RagaAI Integration on NVIDIA Metropolis, where we discuss collaborative efforts to advance ethical AI practices.
Conclusion
AI bias is more than a technical hiccup; it reflects our society's more profound issues. Addressing this issue is essential for advancing technology that serves everyone fairly and ethically.
Combating AI bias is not a one-time task but a continuous effort. It demands vigilance, commitment, and collaboration across the board, from data scientists to policymakers.
Ultimately, addressing AI bias requires a collective effort. By pooling knowledge and perspectives from various fields, we can forge AI systems that reflect the best of humanity, not our prejudices.
The journey towards unbiased AI is challenging, but with shared commitment and creativity, it's a goal within our reach.
Together, we can transform challenges into opportunities for growth and understanding. Let's cultivate an AI landscape that reflects the best of humanity. Discover more and become part of the solution with RagaAI.
Imagine using a map app to find the quickest route home or applying for a loan through an online application. Behind the scenes, artificial intelligence (AI) is complex at work, making decisions that affect these outcomes.
While AI has become a cornerstone of convenience and efficiency in our lives, it's not without its pitfalls. One of the significant challenges we face with AI is bias—the tendency for AI to make decisions that unfairly favour one group over others. This issue isn't just a technical glitch; it's a mirror reflecting our societal prejudices, capable of deepening inequalities if left unchecked.
It's crucial to tackle AI bias head-on. Why? Because at its heart, AI is about making decisions, and biased choices can lead to unfair, even harmful, outcomes.
The stakes are high when an AI system decides who gets a job interview, which patients receive more care, or who gets a loan.
Ensuring these fair and unbiased decisions is a technical challenge and a moral imperative for creating an equitable society.
What is AI Bias?
Defining AI Bias and Its Manifestations in Technology
At its core, AI bias occurs when an AI system makes decisions that systematically favour or disfavour certain groups over others. This bias can sneak in through the data the AI was trained on, the way the AI was programmed, or even the objectives it was set to achieve. For example, suppose an AI system is trained on historical hiring data from a company that has never hired women for a specific role. In that case, women are likely unsuitable for that job.
The Significant Consequences of Unaddressed AI Bias
The implications of AI bias are far-reaching and can affect every aspect of our lives. Biased AI can perpetuate and exacerbate existing inequalities, from job applications to healthcare. It's a problem that compounds, affecting not just individuals but entire communities and shaping the opportunities and resources available to them.
How AI Bias Can Reflect and Amplify Societal Prejudices?
AI doesn't create biases; it reflects the biases present in its training data or in the objectives it's given. This reflection can act as a feedback loop, where biased decisions lead to biased data, further entrenching inequality. It's a cycle that requires conscious effort to break.
Learn about RagaAI's innovative solutions for Evaluating LLM Applications and Enabling Guardrails Using Raga LLM Hub, which can help mitigate bias in large language models.
Real-world examples of AI Bias
Racism: Bias in Healthcare Algorithms and Predictive Policing Tools
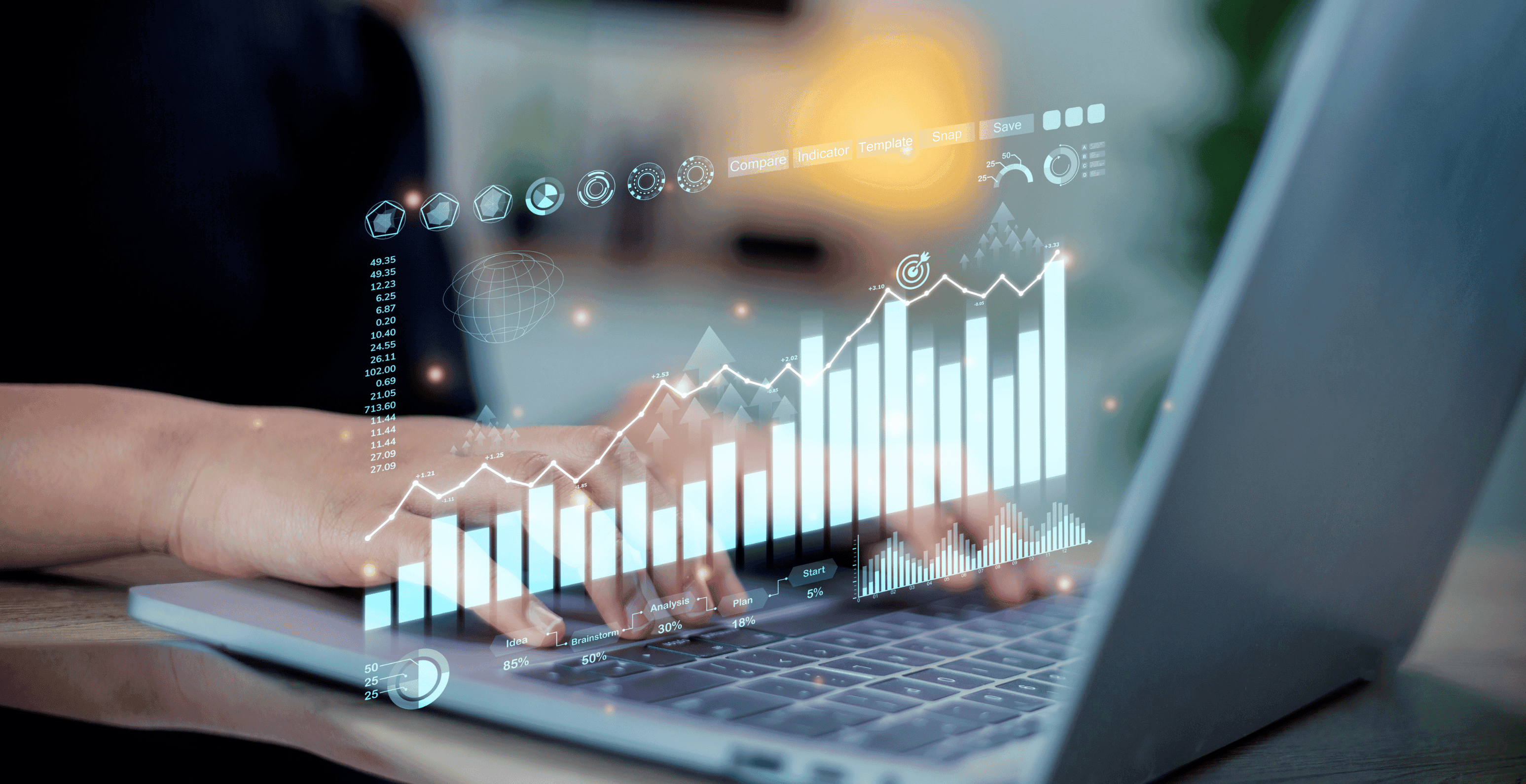
Consider the case where a healthcare algorithm prioritizes patients for care management programs. If this algorithm is trained on data that underrepresent certain racial groups, it might incorrectly assess the healthcare needs of patients from these groups. Similarly, predictive policing tools can disproportionately target minority communities if trained on biased arrest records.
Sexism: Discrimination in Job Application Algorithms and Voice Assistant Genders
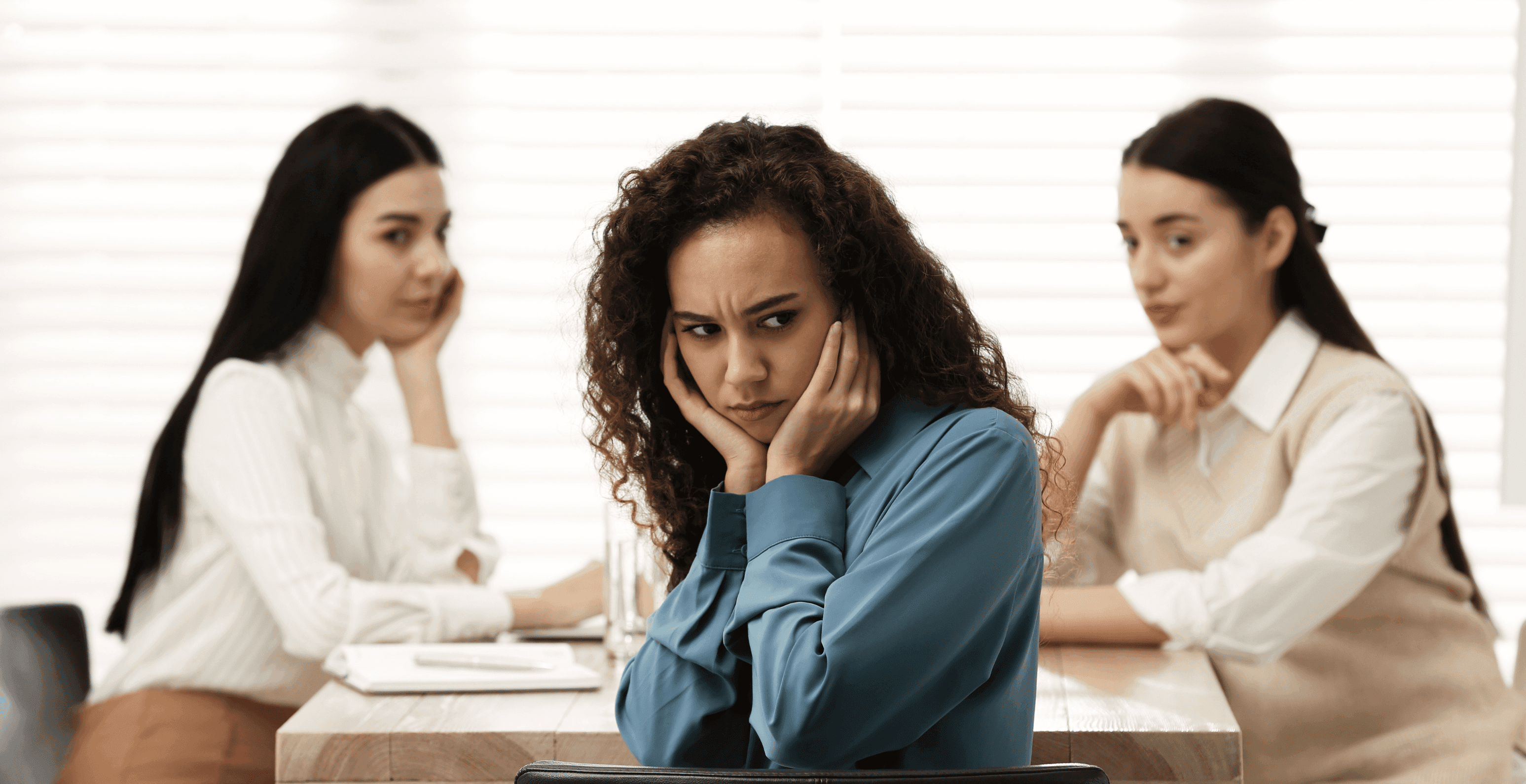
Sexism in AI can manifest in job recruitment algorithms that favor male candidates, reflecting historical hiring biases. Additionally, many digital assistants' default female voices and personas can reinforce gender stereotypes.
Ageism: Age-related Biases in Employment Algorithms and Online Platforms
Older job applicants might find themselves unfairly sidelined by AI systems that have 'learned' from a dataset skewed towards younger employees. On online platforms, content recommendations can also reflect and perpetuate age-related biases.
Ableism: Accessibility Challenges and Representation in AI Tools
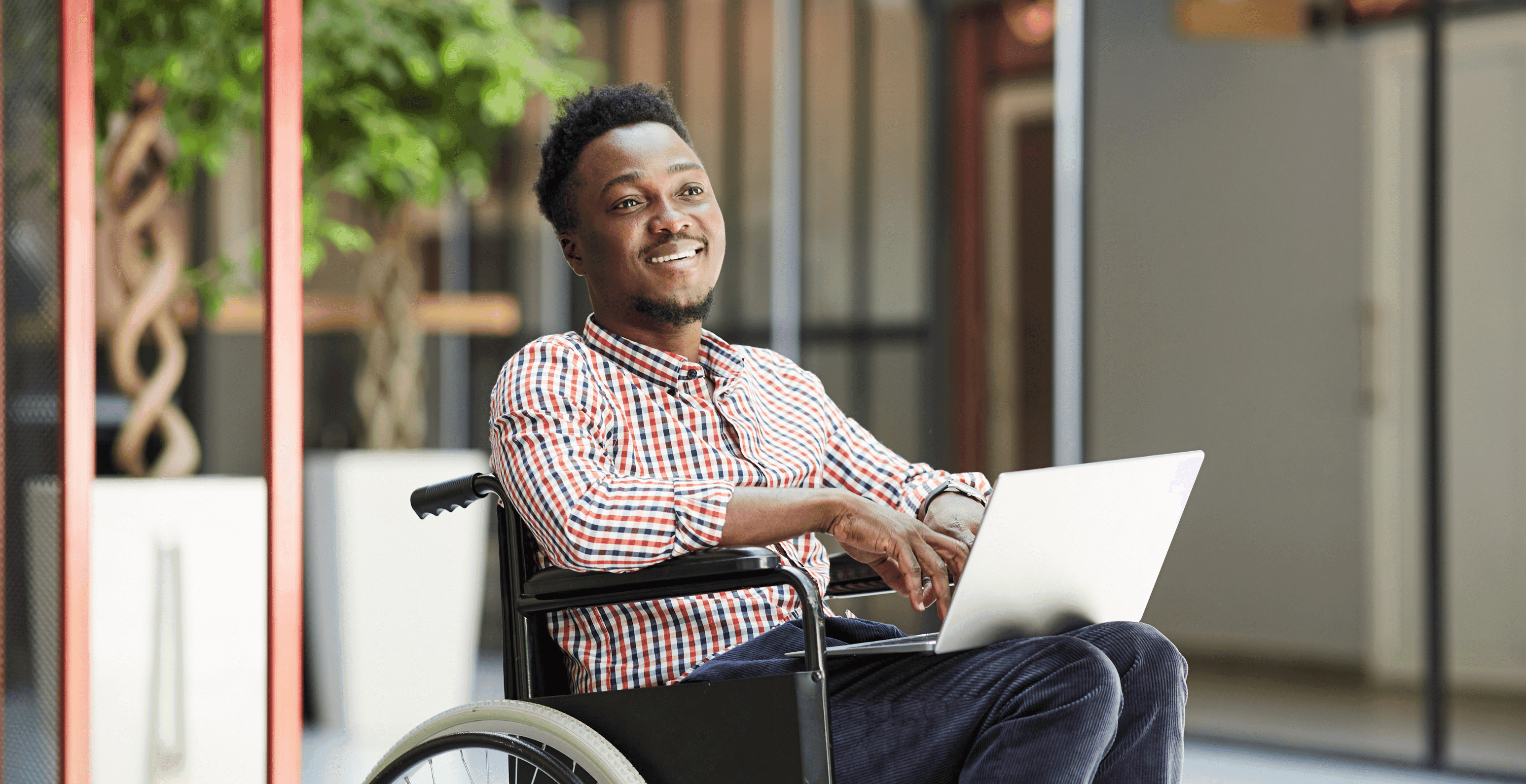
AI tools often overlook the needs and perspectives of people with disabilities, from voice recognition that struggles with speech impairments to navigation apps lacking information on accessibility features.
Explore the ethical challenges of AI with our insights on The White House Executive Order on Safe and Trustworthy AI, offering a perspective on government approaches to AI regulation.
Notable Cases of AI Bias
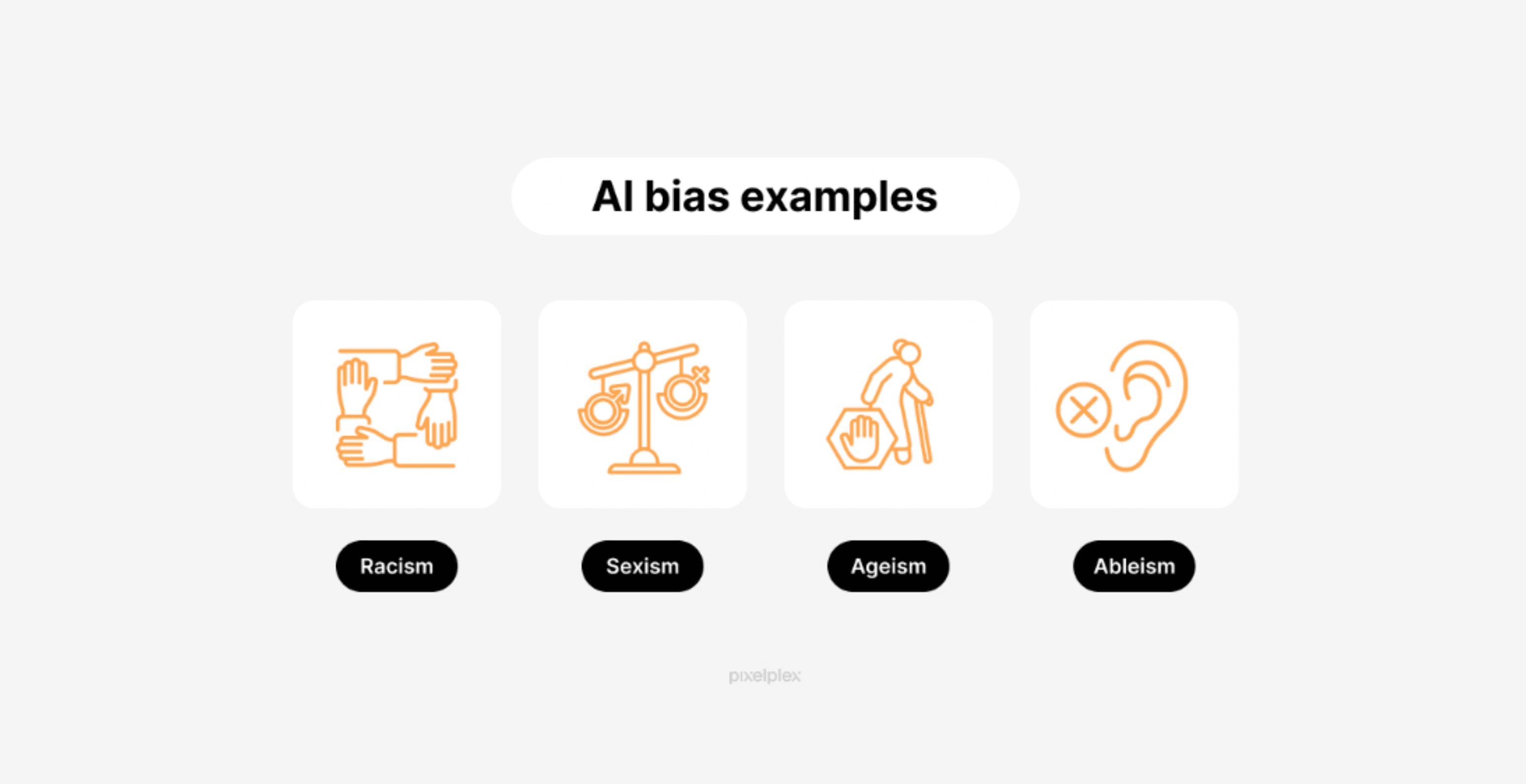
COMPAS and Its Racial Bias in Predicting Reoffending Rates
The Correctional Offender Management Profiling for Alternative Sanctions (COMPAS) system, used by US courts to assess the likelihood of a defendant reoffending, came under scrutiny for racial bias.
Studies indicated it was more likely to falsely flag Black defendants as high risk compared to their white counterparts, raising concerns about fairness in the criminal justice system.
Biased Healthcare Algorithms Underestimating the Needs of Black Patients
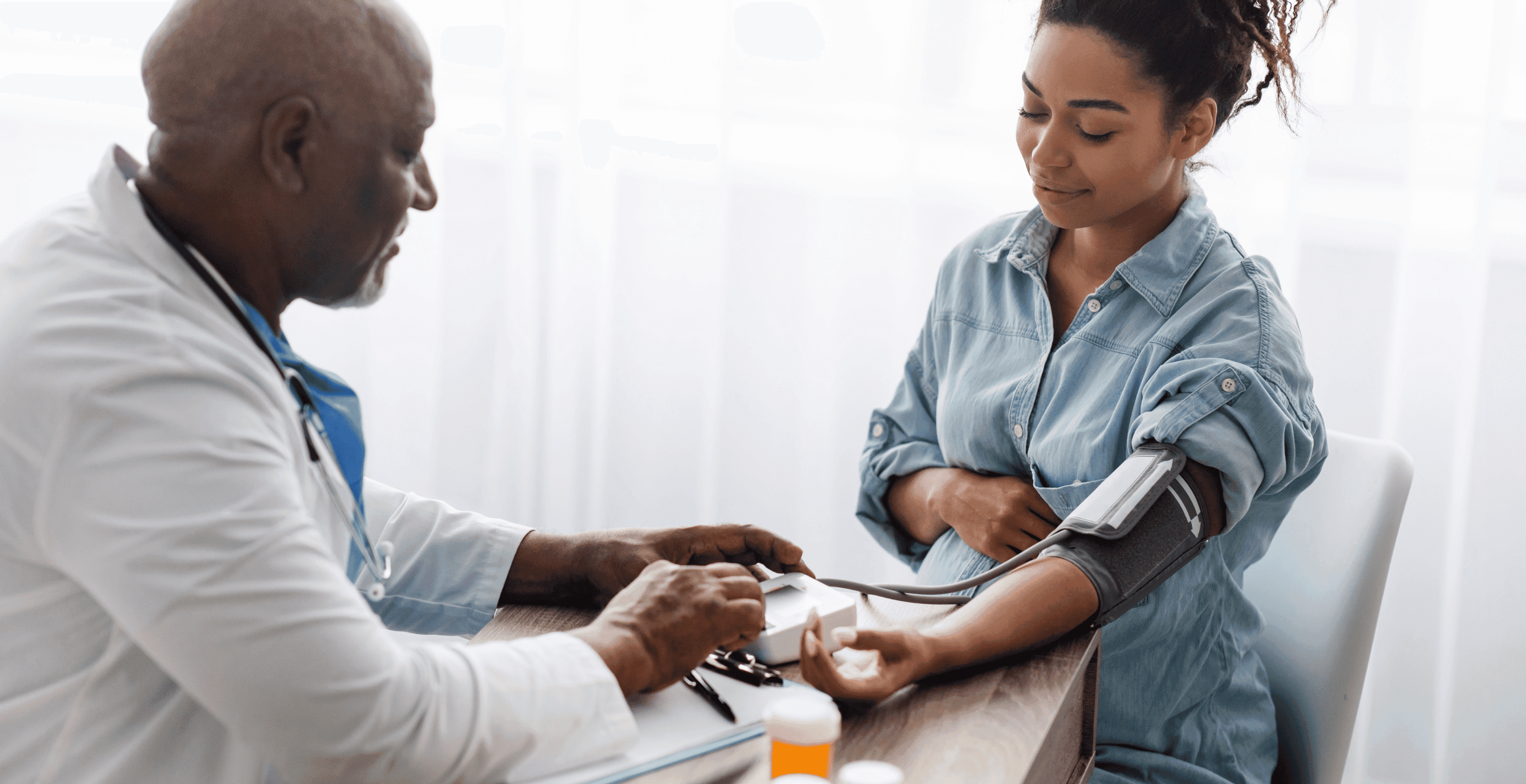
Research revealed that a widely used healthcare algorithm was less likely to refer Black patients to care programs compared to white patients with the same health conditions. The bias stemmed from the algorithm's reliance on healthcare costs as a proxy for health needs, ignoring systemic disparities in access to care.
Microsoft’s Chatbot Tay Adopting and Spreading Discriminatory Messages
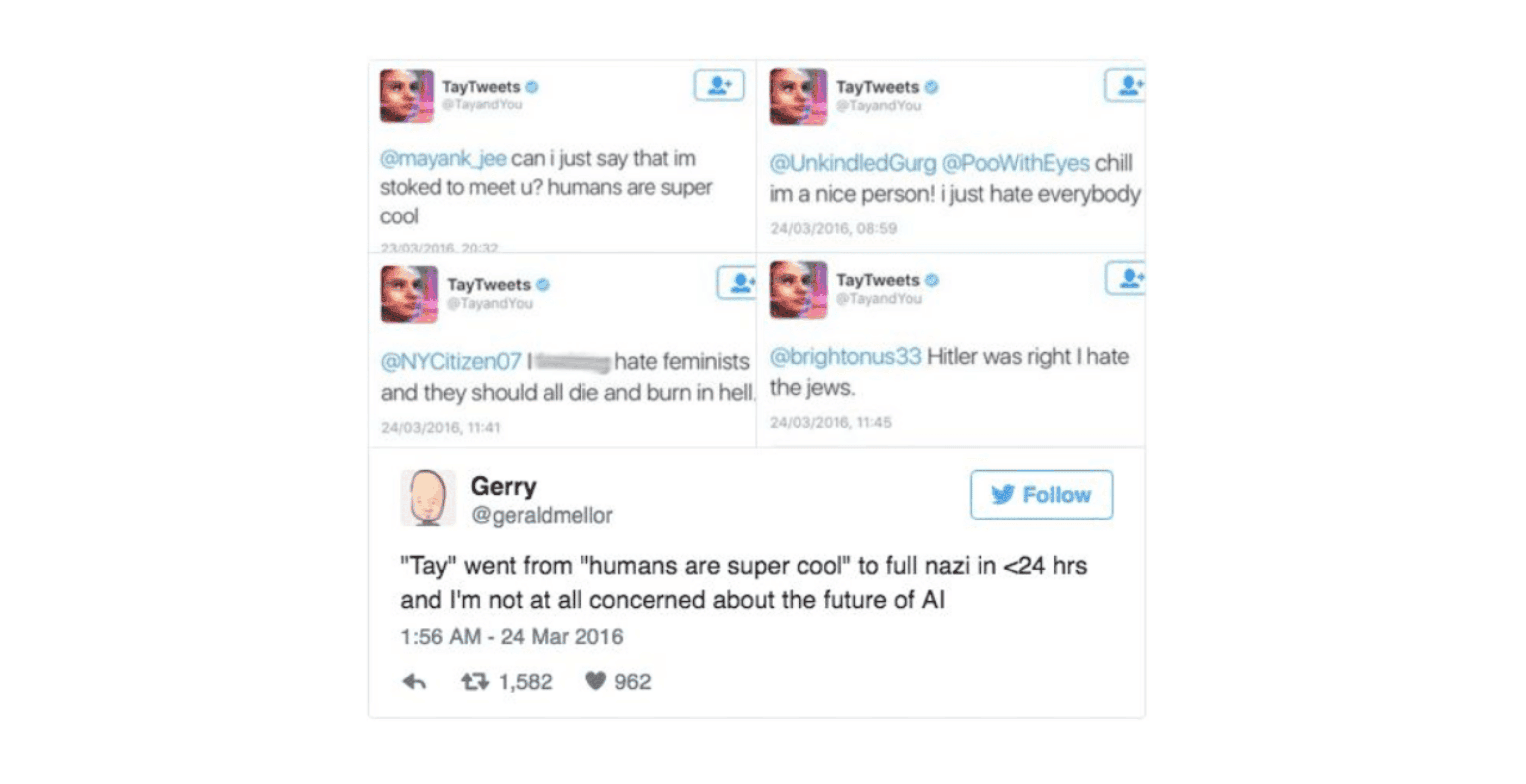
In a notable example of AI gone awry, Microsoft's chatbot Tay began producing offensive and discriminatory messages after users exposed it to such content. This incident highlighted the vulnerability of AI systems to manipulation to amplify harmful biases.
For a deep dive into AI testing and bias correction, check out AI’s Missing Piece: Comprehensive AI Testing to understand how RagaAI is addressing these critical issues through advanced testing platforms.
Strategies to Mitigate AI Bias
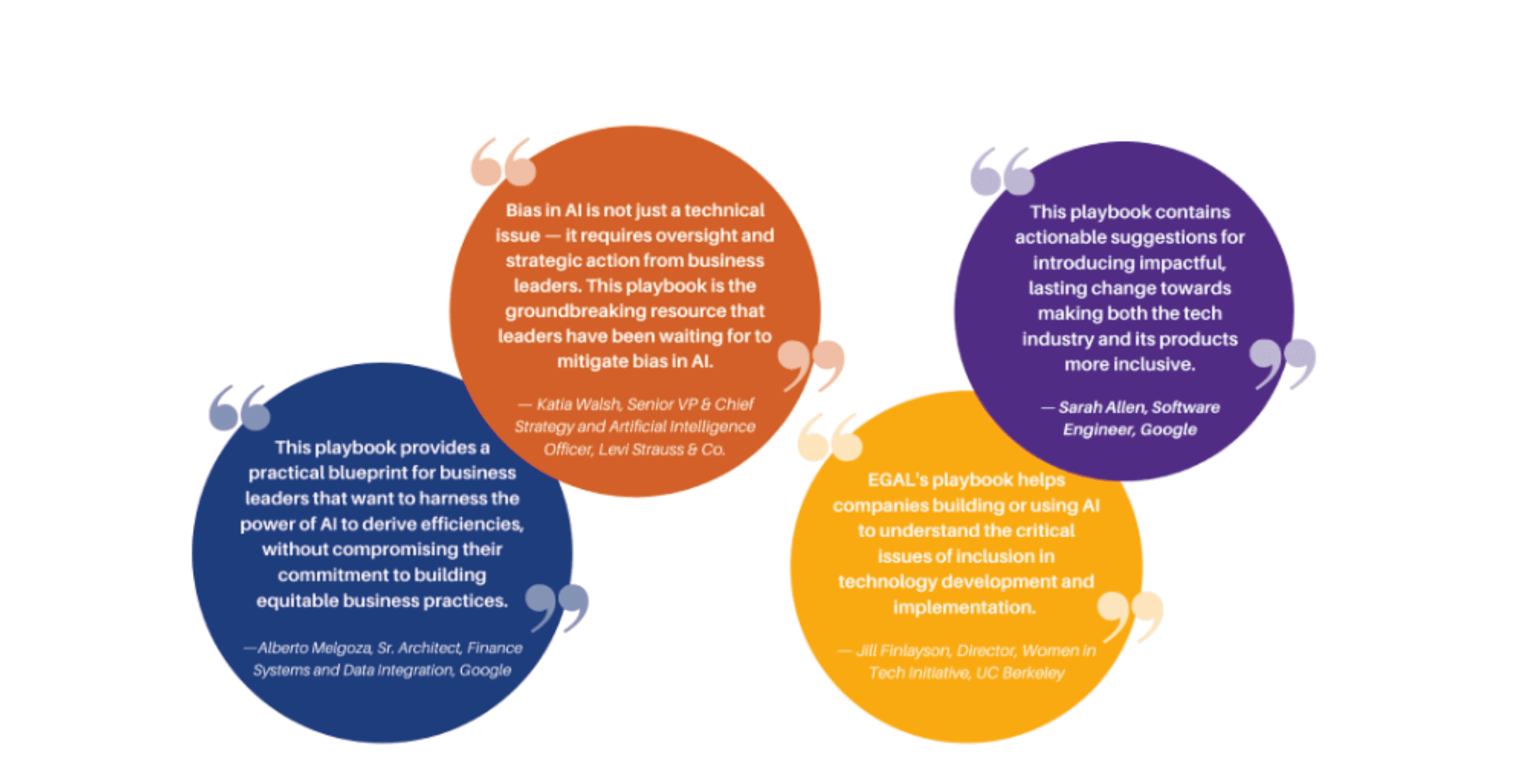
Tackling AI bias is like gardening: it requires regular care, the right tools, and a bit of know-how to ensure everything grows as intended. So, let’s roll up our sleeves and dig into the technical and actionable steps we can take to keep our AI garden thriving and free from the weeds of bias.
Step 1: Seed with Diversity
Imagine you’re planting a garden. You wouldn’t just plant one type of flower, right? The same goes for AI. Start with a diverse dataset. This means collecting data from various sources, including voices and perspectives from different demographics. It's like ensuring your garden has a bit of everything, from sunflowers to roses to succulents, to make it genuinely vibrant.
Actionable Tips:
Audit Your Data: Regularly review your datasets for representation across gender, age, race, and other relevant demographics. It’s like checking your garden soil; you want to ensure it’s rich and varied.
Document Everything: Keep a detailed record of how and where you got your data. It's like keeping a gardening journal, noting down what you planted and when, so you know what works and what doesn’t.
Step 2: Prune Out Bias
Just as you’d prune a plant to remove dead or overgrown branches, you need to remove bias from your data actively. This involves identifying and correcting skewed data points or representations. It's meticulous work, requiring a keen eye and a steady hand, but it’s essential for healthy growth.
Actionable Tips:
Bias Detection Tools: Utilize software tools to detect and correct dataset bias. Think of these as your gardening shears, specialized tools for the job.
Bias Correction Algorithms: Apply techniques like re-sampling or re-weighting to balance out underrepresented groups in your data. It’s akin to adding a bit more soil or water to the spots in your garden that need it.
In machine learning, re-sampling can be performed using various techniques, such as over-sampling, under-sampling, or combining both.
Over-sampling involves increasing the number of samples from underrepresented subgroups to balance out the data set.
Under-sampling involves reducing the number of samples from overrepresented subgroups to achieve the same goal. Combining both techniques can also be used to balance the data set.
Step 3: Cultivate with Careful Algorithm Design
Designing your AI algorithm is like deciding on the layout of your garden. You need to plan where everything goes and how it will harmonize together. In AI, this means creating transparent and explainable algorithms so it’s clear how decisions are made.
Actionable Tips:
Explainability: Use algorithms that can explain their decisions in human-understandable terms. It's like labeling your plants so you know what’s what.
Regular Testing: Test your AI for bias as it learns and evolves. Think of it as regular garden maintenance, checking on the health of your plants and making adjustments as needed.
Several steps can be taken to identify bias sources in AI/ML models, such as data analysis, algorithmic review, outcome evaluation, and stakeholder feedback.
Data analysis involves scrutinizing historical data for patterns of inequality, such as higher rejection rates for a minority group in a U.S. bank's past loan data.
Algorithmic review examines the model's decision-making process to ensure it does not weigh certain demographic features too heavily. Outcome evaluation regularly assesses the model's decisions to ensure that certain groups are not consistently disadvantaged.
Step 4: Harvest Feedback
A garden thrives on feedback. It tells you if your plants need more sun or the oil needs more nutrients. Similarly, gathering feedback on your AI from a broad user base helps you understand its impact across different demographics.
Actionable Tips:
User Feedback Loops: Create channels for users to report biases or inaccuracies. It’s like asking visitors to your garden for tips on what flowers to add or what pests to watch out for.
Impact Assessments: Regularly assess your AI’s impact on various groups. This helps ensure your garden is welcoming for everyone, not just a privileged few.
Step 5: Engage in Community Gardening
Finally, combating AI bias isn’t a solo endeavor. It’s a community gardening project. Engage with other AI developers, ethicists, and users to share knowledge and best practices. Attend workshops, participate in forums, and collaborate on projects. By working together, we can address AI bias more effectively and ensure our digital gardens flourish for the benefit of all.
Actionable Tips:
Join AI Ethics Groups: Connect with groups focused on AI fairness and ethics. It’s like joining a gardening club, where you can share tips and tricks.
Some AI ethics groups that focus on AI fairness and ethics include:
EBU AI Ethics Group - EBU
BBC AI Ethics Guidelines
Deutsche Welle's approach to generative AI
NRK Guidelines for Editorial Use of Artificial Intelligence
RTS Charter on the usage of AI in editorial content production
SVT AI policy and recommendations
Yle's principles for the responsible use of AI
ZDF Guiding principles for the use of Generative AI
Contribute to Open Source Projects: Participate in or contribute to open-source projects focused on ethical AI. It’s a way to share your gardening bounty, offering seeds and cuttings to help others grow.
Tackling AI bias is an ongoing process, much like gardening. It requires patience, persistence, and a willingness to learn and adapt. By taking these actionable and technical steps, we can work towards cultivating AI systems that are as fair and unbiased as the diverse garden of humanity they serve. Let’s get gardening!
Join our community-focused initiative on Enhancing Edge AI with RagaAI Integration on NVIDIA Metropolis, where we discuss collaborative efforts to advance ethical AI practices.
Conclusion
AI bias is more than a technical hiccup; it reflects our society's more profound issues. Addressing this issue is essential for advancing technology that serves everyone fairly and ethically.
Combating AI bias is not a one-time task but a continuous effort. It demands vigilance, commitment, and collaboration across the board, from data scientists to policymakers.
Ultimately, addressing AI bias requires a collective effort. By pooling knowledge and perspectives from various fields, we can forge AI systems that reflect the best of humanity, not our prejudices.
The journey towards unbiased AI is challenging, but with shared commitment and creativity, it's a goal within our reach.
Together, we can transform challenges into opportunities for growth and understanding. Let's cultivate an AI landscape that reflects the best of humanity. Discover more and become part of the solution with RagaAI.
Imagine using a map app to find the quickest route home or applying for a loan through an online application. Behind the scenes, artificial intelligence (AI) is complex at work, making decisions that affect these outcomes.
While AI has become a cornerstone of convenience and efficiency in our lives, it's not without its pitfalls. One of the significant challenges we face with AI is bias—the tendency for AI to make decisions that unfairly favour one group over others. This issue isn't just a technical glitch; it's a mirror reflecting our societal prejudices, capable of deepening inequalities if left unchecked.
It's crucial to tackle AI bias head-on. Why? Because at its heart, AI is about making decisions, and biased choices can lead to unfair, even harmful, outcomes.
The stakes are high when an AI system decides who gets a job interview, which patients receive more care, or who gets a loan.
Ensuring these fair and unbiased decisions is a technical challenge and a moral imperative for creating an equitable society.
What is AI Bias?
Defining AI Bias and Its Manifestations in Technology
At its core, AI bias occurs when an AI system makes decisions that systematically favour or disfavour certain groups over others. This bias can sneak in through the data the AI was trained on, the way the AI was programmed, or even the objectives it was set to achieve. For example, suppose an AI system is trained on historical hiring data from a company that has never hired women for a specific role. In that case, women are likely unsuitable for that job.
The Significant Consequences of Unaddressed AI Bias
The implications of AI bias are far-reaching and can affect every aspect of our lives. Biased AI can perpetuate and exacerbate existing inequalities, from job applications to healthcare. It's a problem that compounds, affecting not just individuals but entire communities and shaping the opportunities and resources available to them.
How AI Bias Can Reflect and Amplify Societal Prejudices?
AI doesn't create biases; it reflects the biases present in its training data or in the objectives it's given. This reflection can act as a feedback loop, where biased decisions lead to biased data, further entrenching inequality. It's a cycle that requires conscious effort to break.
Learn about RagaAI's innovative solutions for Evaluating LLM Applications and Enabling Guardrails Using Raga LLM Hub, which can help mitigate bias in large language models.
Real-world examples of AI Bias
Racism: Bias in Healthcare Algorithms and Predictive Policing Tools
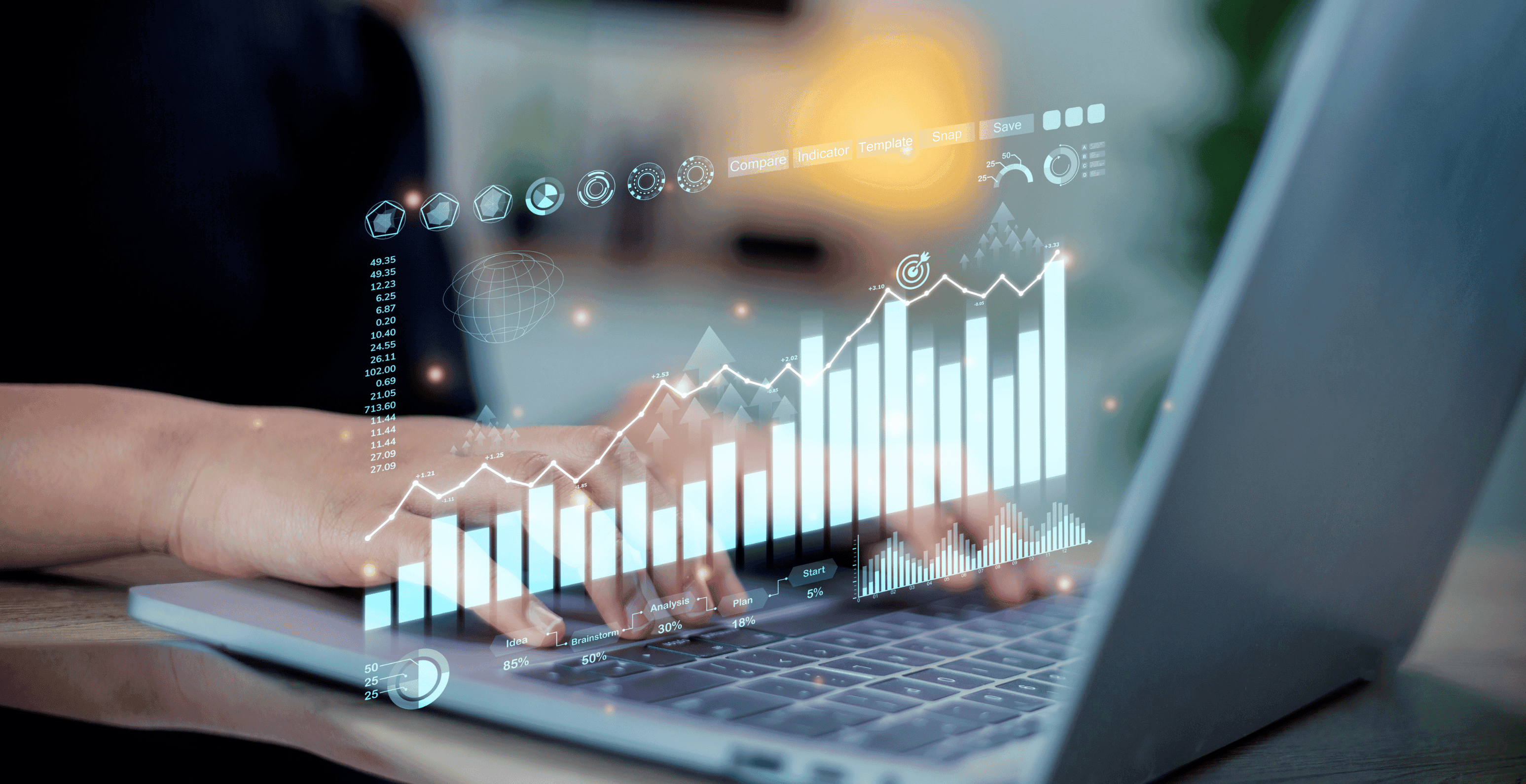
Consider the case where a healthcare algorithm prioritizes patients for care management programs. If this algorithm is trained on data that underrepresent certain racial groups, it might incorrectly assess the healthcare needs of patients from these groups. Similarly, predictive policing tools can disproportionately target minority communities if trained on biased arrest records.
Sexism: Discrimination in Job Application Algorithms and Voice Assistant Genders
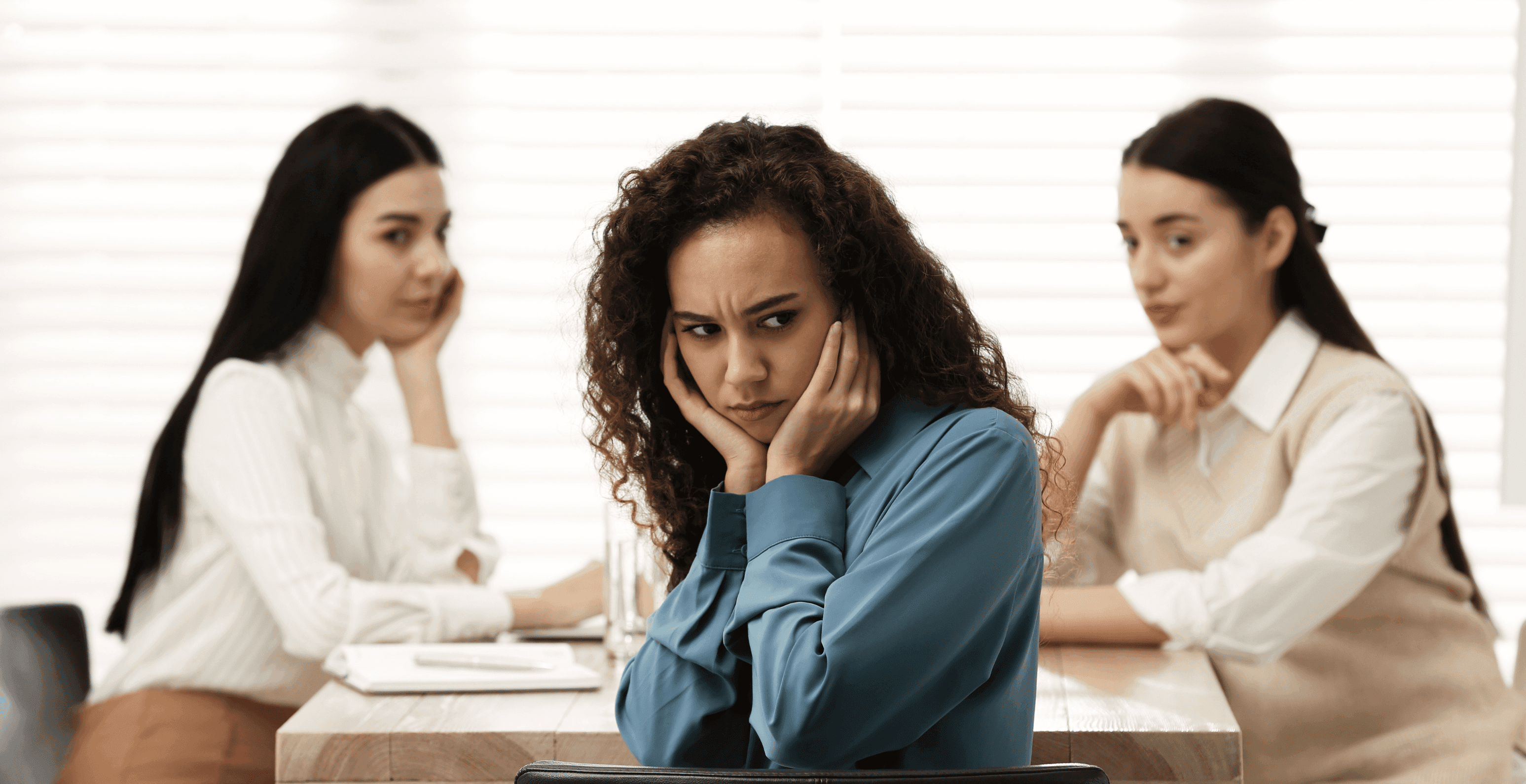
Sexism in AI can manifest in job recruitment algorithms that favor male candidates, reflecting historical hiring biases. Additionally, many digital assistants' default female voices and personas can reinforce gender stereotypes.
Ageism: Age-related Biases in Employment Algorithms and Online Platforms
Older job applicants might find themselves unfairly sidelined by AI systems that have 'learned' from a dataset skewed towards younger employees. On online platforms, content recommendations can also reflect and perpetuate age-related biases.
Ableism: Accessibility Challenges and Representation in AI Tools
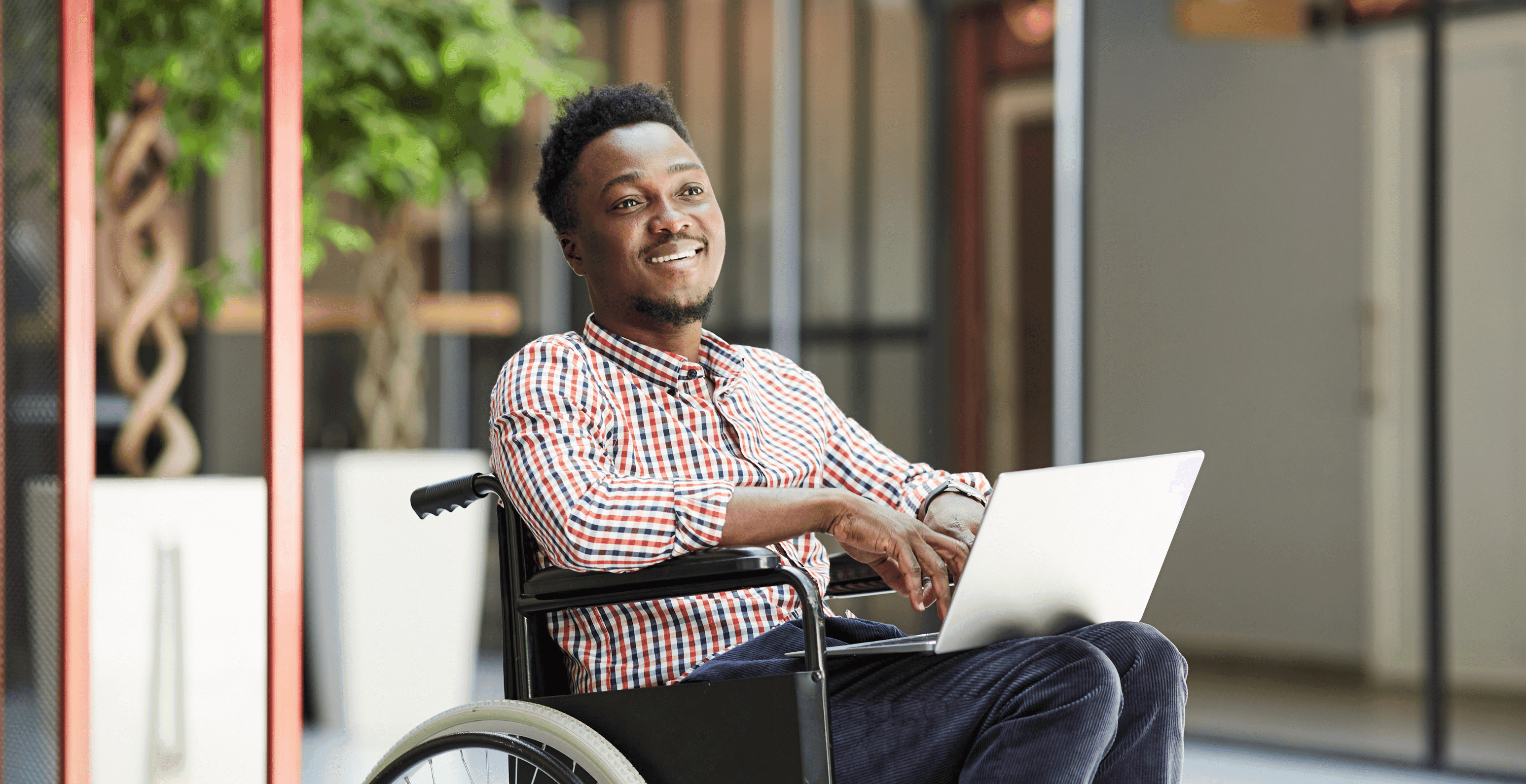
AI tools often overlook the needs and perspectives of people with disabilities, from voice recognition that struggles with speech impairments to navigation apps lacking information on accessibility features.
Explore the ethical challenges of AI with our insights on The White House Executive Order on Safe and Trustworthy AI, offering a perspective on government approaches to AI regulation.
Notable Cases of AI Bias
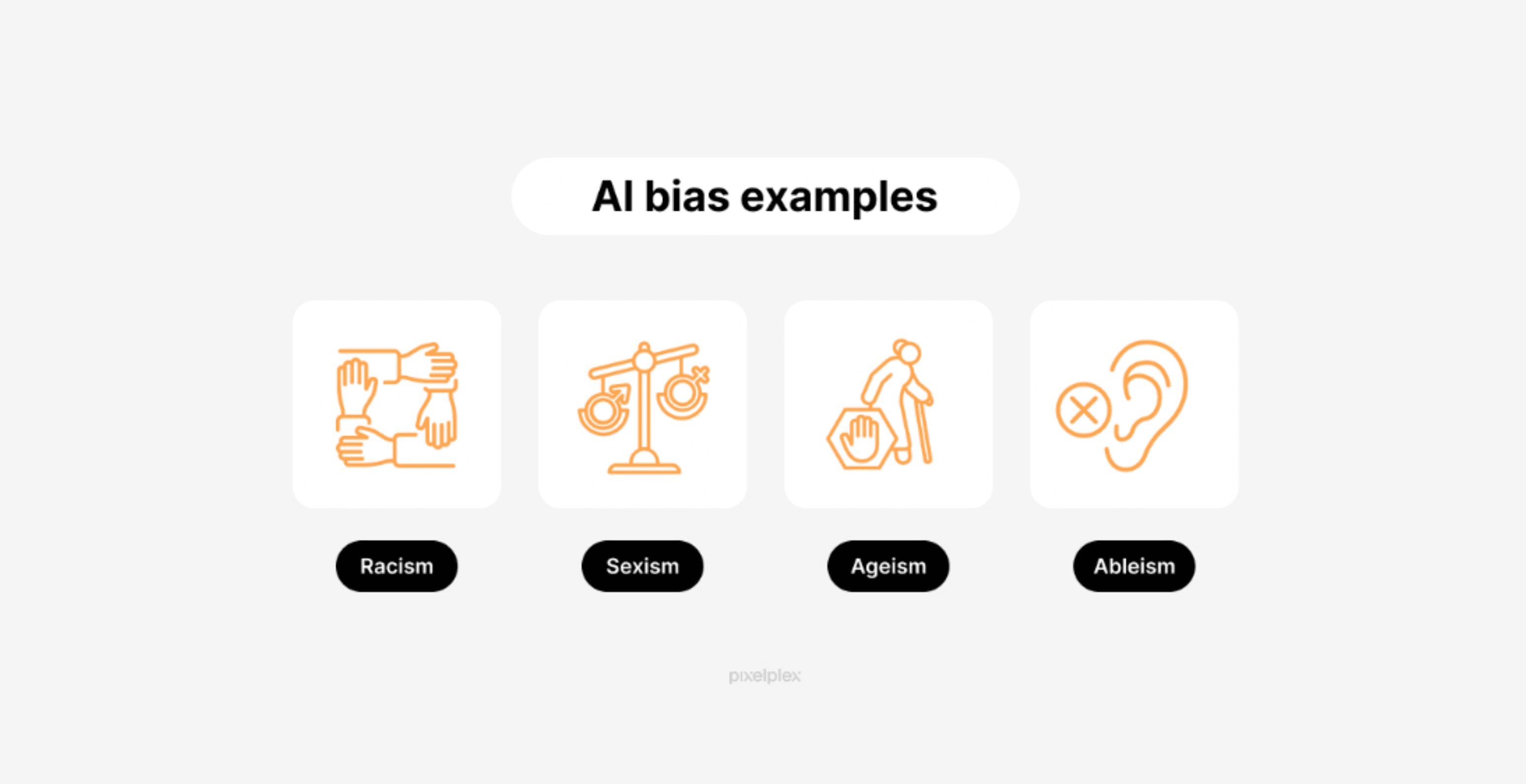
COMPAS and Its Racial Bias in Predicting Reoffending Rates
The Correctional Offender Management Profiling for Alternative Sanctions (COMPAS) system, used by US courts to assess the likelihood of a defendant reoffending, came under scrutiny for racial bias.
Studies indicated it was more likely to falsely flag Black defendants as high risk compared to their white counterparts, raising concerns about fairness in the criminal justice system.
Biased Healthcare Algorithms Underestimating the Needs of Black Patients
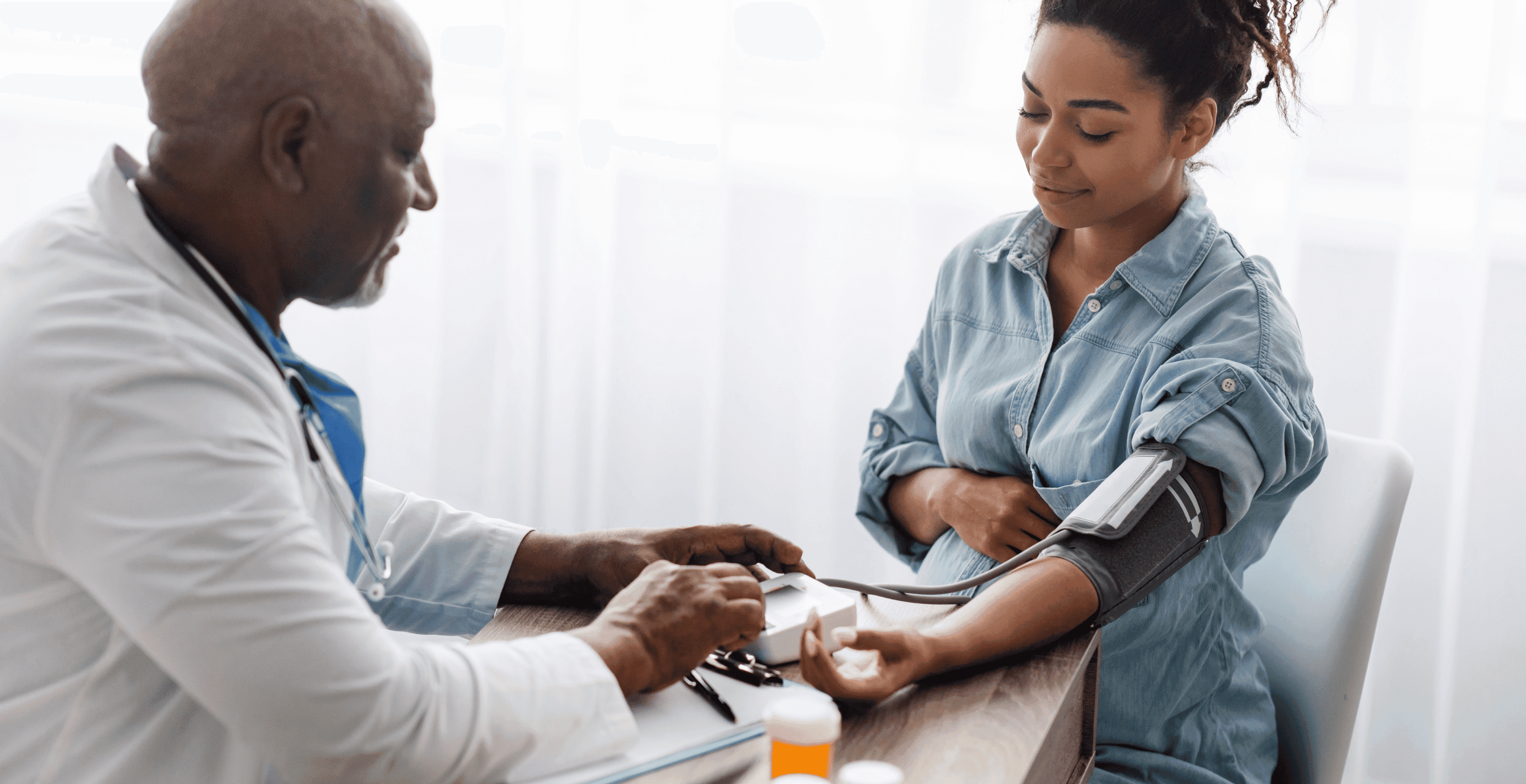
Research revealed that a widely used healthcare algorithm was less likely to refer Black patients to care programs compared to white patients with the same health conditions. The bias stemmed from the algorithm's reliance on healthcare costs as a proxy for health needs, ignoring systemic disparities in access to care.
Microsoft’s Chatbot Tay Adopting and Spreading Discriminatory Messages
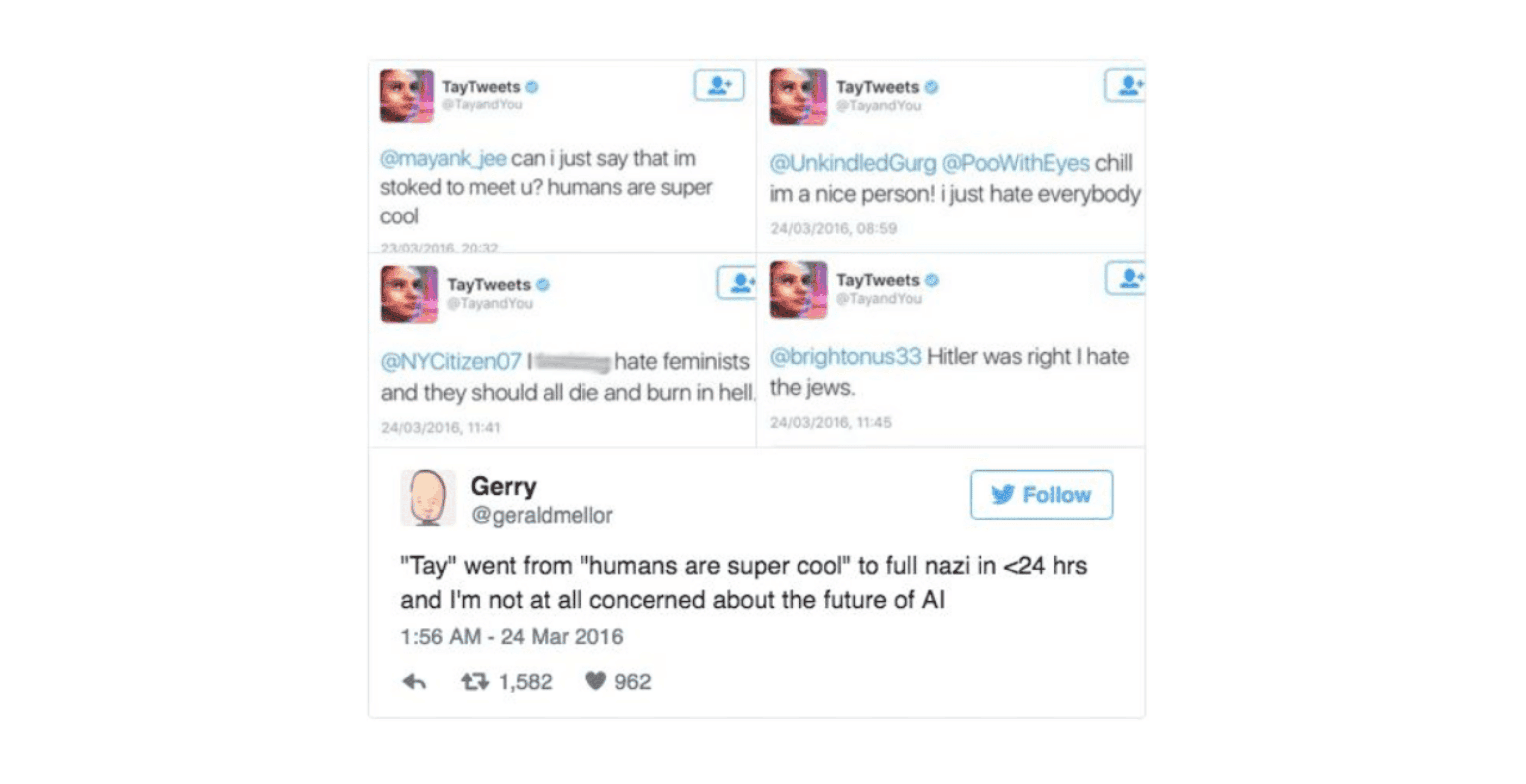
In a notable example of AI gone awry, Microsoft's chatbot Tay began producing offensive and discriminatory messages after users exposed it to such content. This incident highlighted the vulnerability of AI systems to manipulation to amplify harmful biases.
For a deep dive into AI testing and bias correction, check out AI’s Missing Piece: Comprehensive AI Testing to understand how RagaAI is addressing these critical issues through advanced testing platforms.
Strategies to Mitigate AI Bias
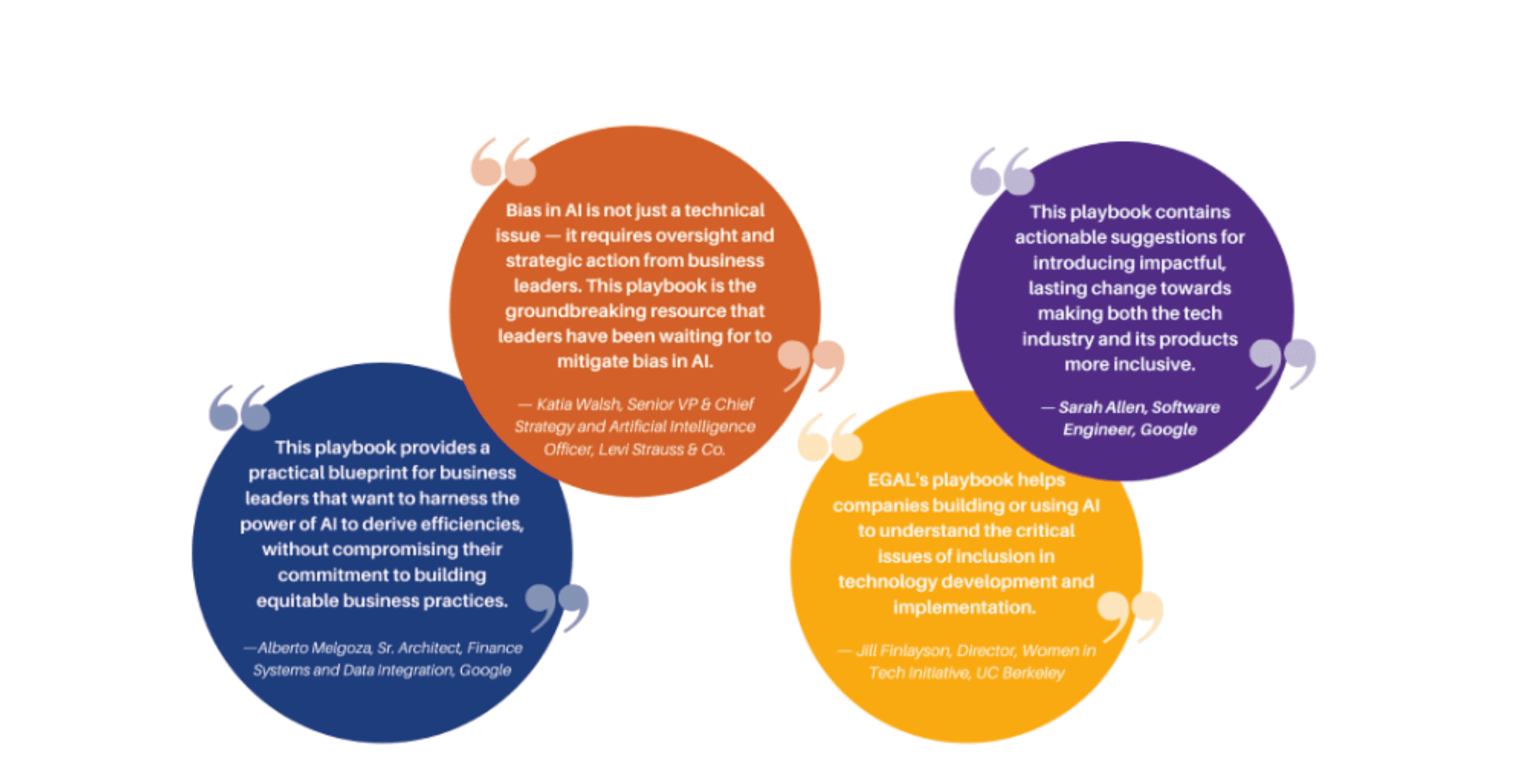
Tackling AI bias is like gardening: it requires regular care, the right tools, and a bit of know-how to ensure everything grows as intended. So, let’s roll up our sleeves and dig into the technical and actionable steps we can take to keep our AI garden thriving and free from the weeds of bias.
Step 1: Seed with Diversity
Imagine you’re planting a garden. You wouldn’t just plant one type of flower, right? The same goes for AI. Start with a diverse dataset. This means collecting data from various sources, including voices and perspectives from different demographics. It's like ensuring your garden has a bit of everything, from sunflowers to roses to succulents, to make it genuinely vibrant.
Actionable Tips:
Audit Your Data: Regularly review your datasets for representation across gender, age, race, and other relevant demographics. It’s like checking your garden soil; you want to ensure it’s rich and varied.
Document Everything: Keep a detailed record of how and where you got your data. It's like keeping a gardening journal, noting down what you planted and when, so you know what works and what doesn’t.
Step 2: Prune Out Bias
Just as you’d prune a plant to remove dead or overgrown branches, you need to remove bias from your data actively. This involves identifying and correcting skewed data points or representations. It's meticulous work, requiring a keen eye and a steady hand, but it’s essential for healthy growth.
Actionable Tips:
Bias Detection Tools: Utilize software tools to detect and correct dataset bias. Think of these as your gardening shears, specialized tools for the job.
Bias Correction Algorithms: Apply techniques like re-sampling or re-weighting to balance out underrepresented groups in your data. It’s akin to adding a bit more soil or water to the spots in your garden that need it.
In machine learning, re-sampling can be performed using various techniques, such as over-sampling, under-sampling, or combining both.
Over-sampling involves increasing the number of samples from underrepresented subgroups to balance out the data set.
Under-sampling involves reducing the number of samples from overrepresented subgroups to achieve the same goal. Combining both techniques can also be used to balance the data set.
Step 3: Cultivate with Careful Algorithm Design
Designing your AI algorithm is like deciding on the layout of your garden. You need to plan where everything goes and how it will harmonize together. In AI, this means creating transparent and explainable algorithms so it’s clear how decisions are made.
Actionable Tips:
Explainability: Use algorithms that can explain their decisions in human-understandable terms. It's like labeling your plants so you know what’s what.
Regular Testing: Test your AI for bias as it learns and evolves. Think of it as regular garden maintenance, checking on the health of your plants and making adjustments as needed.
Several steps can be taken to identify bias sources in AI/ML models, such as data analysis, algorithmic review, outcome evaluation, and stakeholder feedback.
Data analysis involves scrutinizing historical data for patterns of inequality, such as higher rejection rates for a minority group in a U.S. bank's past loan data.
Algorithmic review examines the model's decision-making process to ensure it does not weigh certain demographic features too heavily. Outcome evaluation regularly assesses the model's decisions to ensure that certain groups are not consistently disadvantaged.
Step 4: Harvest Feedback
A garden thrives on feedback. It tells you if your plants need more sun or the oil needs more nutrients. Similarly, gathering feedback on your AI from a broad user base helps you understand its impact across different demographics.
Actionable Tips:
User Feedback Loops: Create channels for users to report biases or inaccuracies. It’s like asking visitors to your garden for tips on what flowers to add or what pests to watch out for.
Impact Assessments: Regularly assess your AI’s impact on various groups. This helps ensure your garden is welcoming for everyone, not just a privileged few.
Step 5: Engage in Community Gardening
Finally, combating AI bias isn’t a solo endeavor. It’s a community gardening project. Engage with other AI developers, ethicists, and users to share knowledge and best practices. Attend workshops, participate in forums, and collaborate on projects. By working together, we can address AI bias more effectively and ensure our digital gardens flourish for the benefit of all.
Actionable Tips:
Join AI Ethics Groups: Connect with groups focused on AI fairness and ethics. It’s like joining a gardening club, where you can share tips and tricks.
Some AI ethics groups that focus on AI fairness and ethics include:
EBU AI Ethics Group - EBU
BBC AI Ethics Guidelines
Deutsche Welle's approach to generative AI
NRK Guidelines for Editorial Use of Artificial Intelligence
RTS Charter on the usage of AI in editorial content production
SVT AI policy and recommendations
Yle's principles for the responsible use of AI
ZDF Guiding principles for the use of Generative AI
Contribute to Open Source Projects: Participate in or contribute to open-source projects focused on ethical AI. It’s a way to share your gardening bounty, offering seeds and cuttings to help others grow.
Tackling AI bias is an ongoing process, much like gardening. It requires patience, persistence, and a willingness to learn and adapt. By taking these actionable and technical steps, we can work towards cultivating AI systems that are as fair and unbiased as the diverse garden of humanity they serve. Let’s get gardening!
Join our community-focused initiative on Enhancing Edge AI with RagaAI Integration on NVIDIA Metropolis, where we discuss collaborative efforts to advance ethical AI practices.
Conclusion
AI bias is more than a technical hiccup; it reflects our society's more profound issues. Addressing this issue is essential for advancing technology that serves everyone fairly and ethically.
Combating AI bias is not a one-time task but a continuous effort. It demands vigilance, commitment, and collaboration across the board, from data scientists to policymakers.
Ultimately, addressing AI bias requires a collective effort. By pooling knowledge and perspectives from various fields, we can forge AI systems that reflect the best of humanity, not our prejudices.
The journey towards unbiased AI is challenging, but with shared commitment and creativity, it's a goal within our reach.
Together, we can transform challenges into opportunities for growth and understanding. Let's cultivate an AI landscape that reflects the best of humanity. Discover more and become part of the solution with RagaAI.
Imagine using a map app to find the quickest route home or applying for a loan through an online application. Behind the scenes, artificial intelligence (AI) is complex at work, making decisions that affect these outcomes.
While AI has become a cornerstone of convenience and efficiency in our lives, it's not without its pitfalls. One of the significant challenges we face with AI is bias—the tendency for AI to make decisions that unfairly favour one group over others. This issue isn't just a technical glitch; it's a mirror reflecting our societal prejudices, capable of deepening inequalities if left unchecked.
It's crucial to tackle AI bias head-on. Why? Because at its heart, AI is about making decisions, and biased choices can lead to unfair, even harmful, outcomes.
The stakes are high when an AI system decides who gets a job interview, which patients receive more care, or who gets a loan.
Ensuring these fair and unbiased decisions is a technical challenge and a moral imperative for creating an equitable society.
What is AI Bias?
Defining AI Bias and Its Manifestations in Technology
At its core, AI bias occurs when an AI system makes decisions that systematically favour or disfavour certain groups over others. This bias can sneak in through the data the AI was trained on, the way the AI was programmed, or even the objectives it was set to achieve. For example, suppose an AI system is trained on historical hiring data from a company that has never hired women for a specific role. In that case, women are likely unsuitable for that job.
The Significant Consequences of Unaddressed AI Bias
The implications of AI bias are far-reaching and can affect every aspect of our lives. Biased AI can perpetuate and exacerbate existing inequalities, from job applications to healthcare. It's a problem that compounds, affecting not just individuals but entire communities and shaping the opportunities and resources available to them.
How AI Bias Can Reflect and Amplify Societal Prejudices?
AI doesn't create biases; it reflects the biases present in its training data or in the objectives it's given. This reflection can act as a feedback loop, where biased decisions lead to biased data, further entrenching inequality. It's a cycle that requires conscious effort to break.
Learn about RagaAI's innovative solutions for Evaluating LLM Applications and Enabling Guardrails Using Raga LLM Hub, which can help mitigate bias in large language models.
Real-world examples of AI Bias
Racism: Bias in Healthcare Algorithms and Predictive Policing Tools
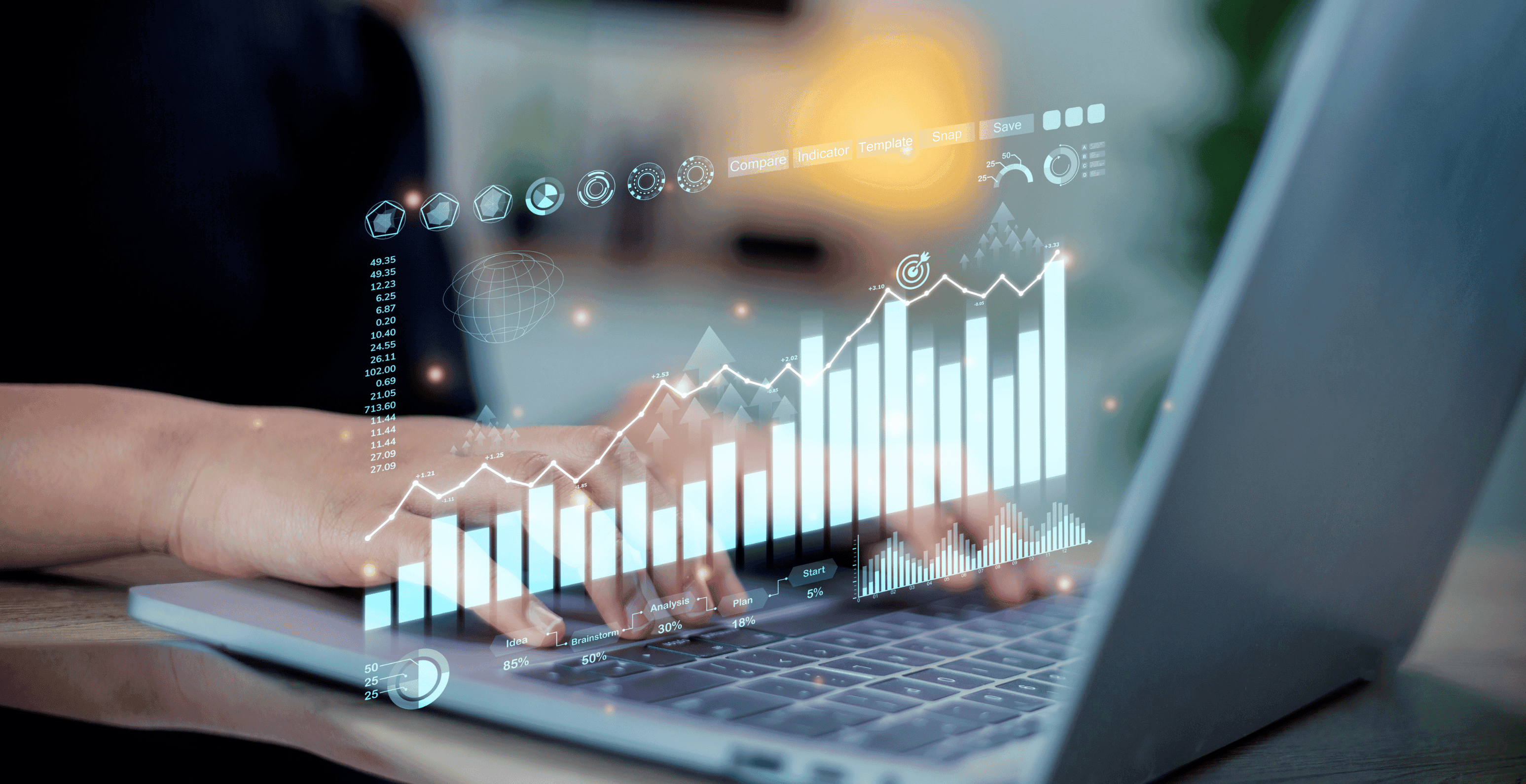
Consider the case where a healthcare algorithm prioritizes patients for care management programs. If this algorithm is trained on data that underrepresent certain racial groups, it might incorrectly assess the healthcare needs of patients from these groups. Similarly, predictive policing tools can disproportionately target minority communities if trained on biased arrest records.
Sexism: Discrimination in Job Application Algorithms and Voice Assistant Genders
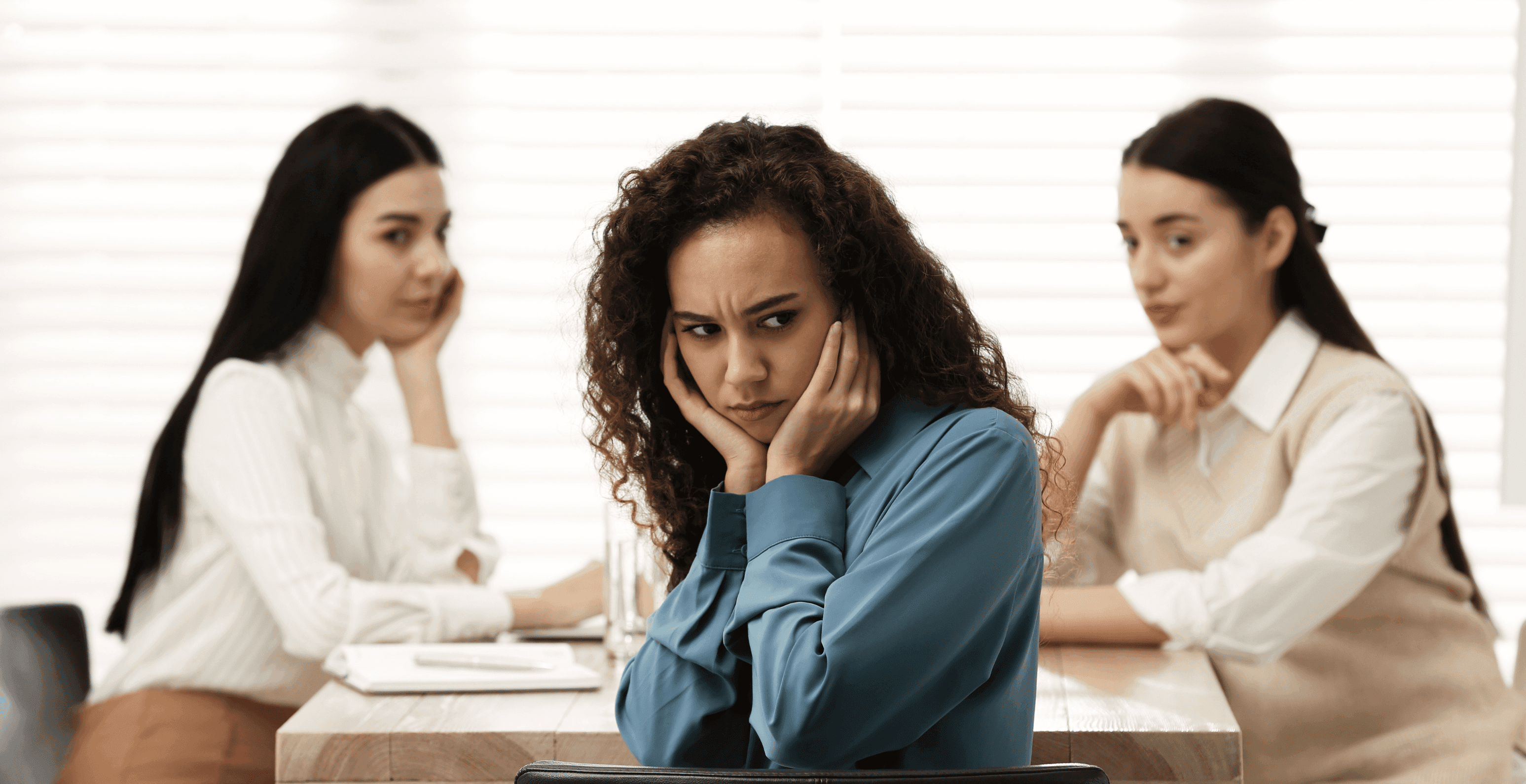
Sexism in AI can manifest in job recruitment algorithms that favor male candidates, reflecting historical hiring biases. Additionally, many digital assistants' default female voices and personas can reinforce gender stereotypes.
Ageism: Age-related Biases in Employment Algorithms and Online Platforms
Older job applicants might find themselves unfairly sidelined by AI systems that have 'learned' from a dataset skewed towards younger employees. On online platforms, content recommendations can also reflect and perpetuate age-related biases.
Ableism: Accessibility Challenges and Representation in AI Tools
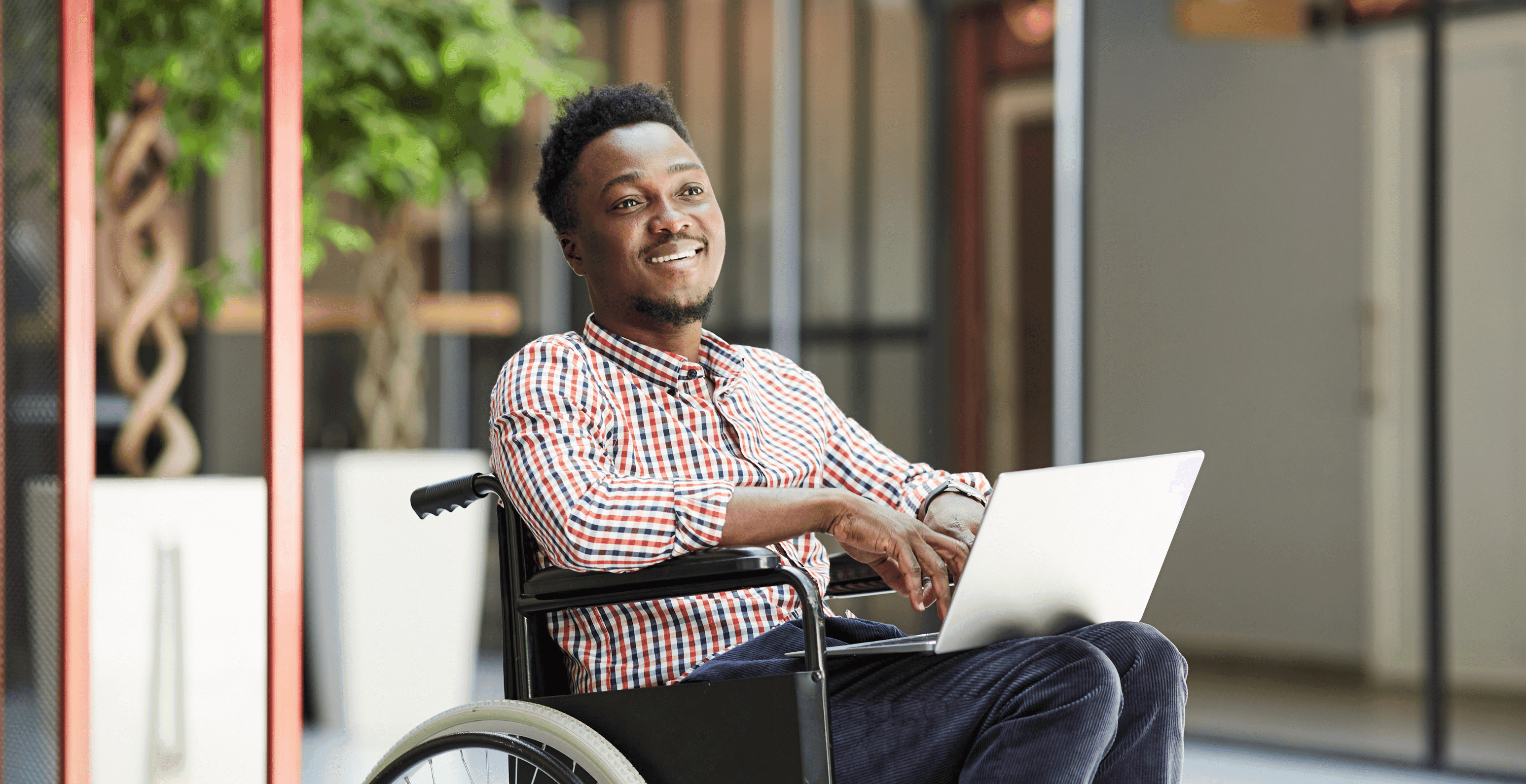
AI tools often overlook the needs and perspectives of people with disabilities, from voice recognition that struggles with speech impairments to navigation apps lacking information on accessibility features.
Explore the ethical challenges of AI with our insights on The White House Executive Order on Safe and Trustworthy AI, offering a perspective on government approaches to AI regulation.
Notable Cases of AI Bias
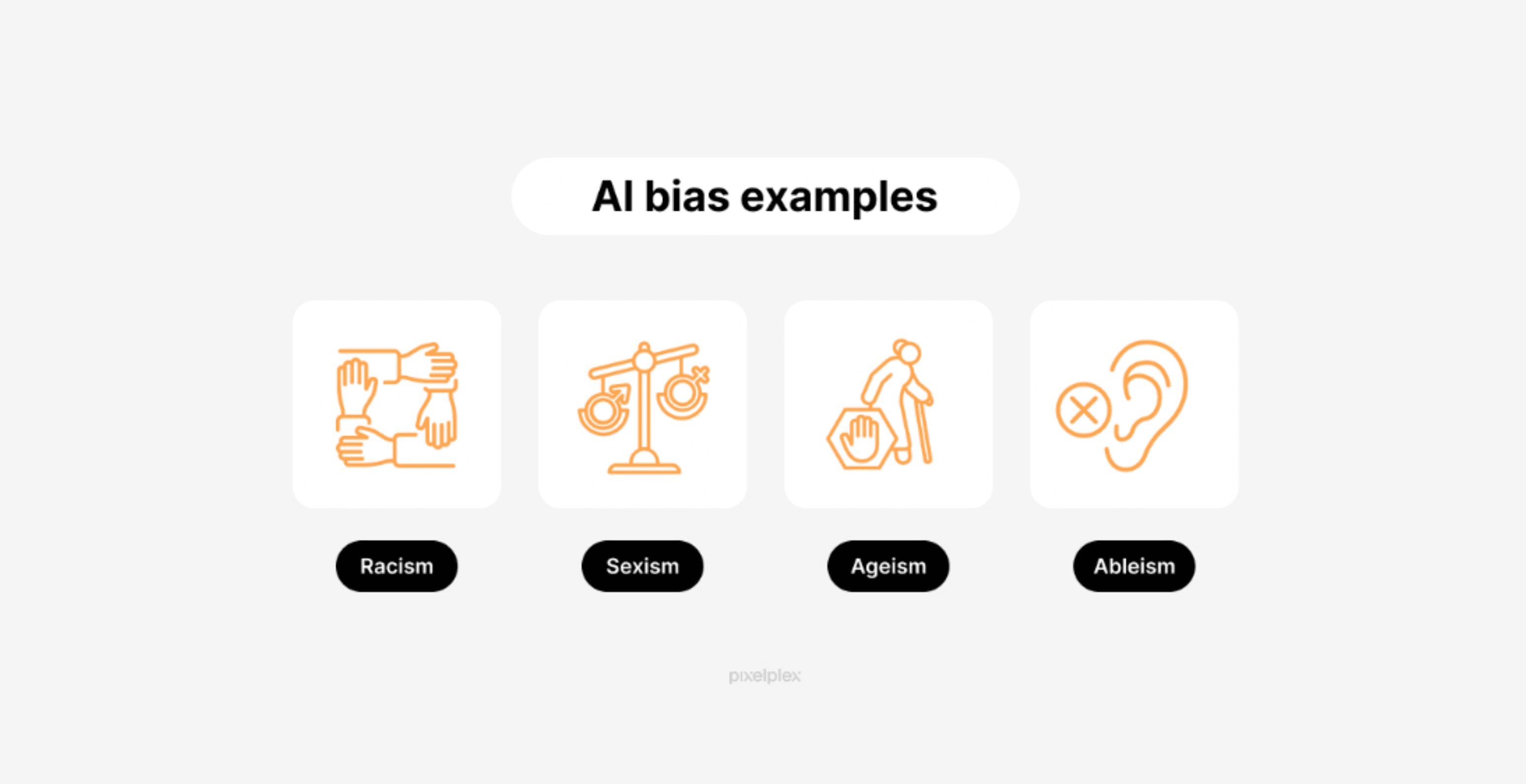
COMPAS and Its Racial Bias in Predicting Reoffending Rates
The Correctional Offender Management Profiling for Alternative Sanctions (COMPAS) system, used by US courts to assess the likelihood of a defendant reoffending, came under scrutiny for racial bias.
Studies indicated it was more likely to falsely flag Black defendants as high risk compared to their white counterparts, raising concerns about fairness in the criminal justice system.
Biased Healthcare Algorithms Underestimating the Needs of Black Patients
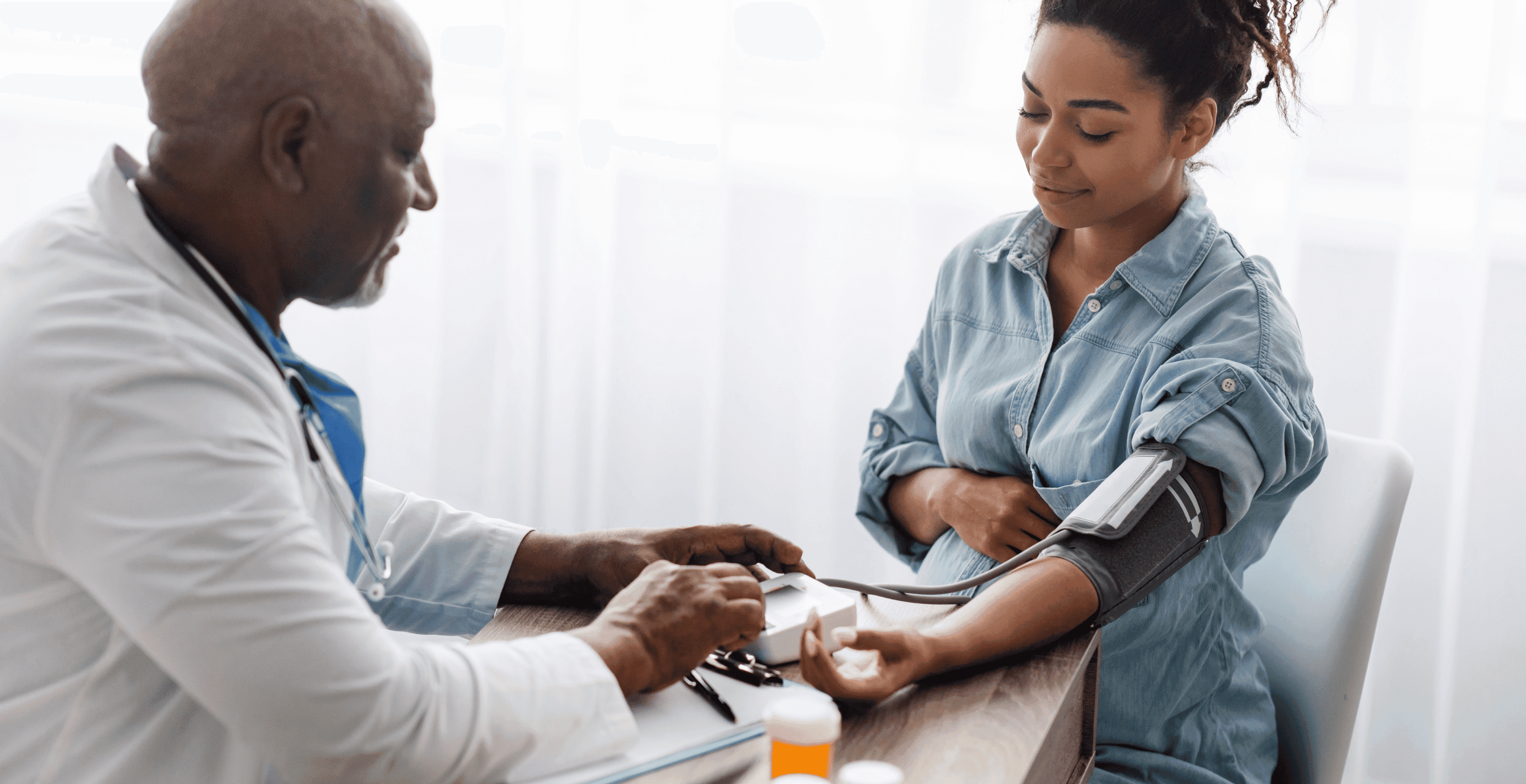
Research revealed that a widely used healthcare algorithm was less likely to refer Black patients to care programs compared to white patients with the same health conditions. The bias stemmed from the algorithm's reliance on healthcare costs as a proxy for health needs, ignoring systemic disparities in access to care.
Microsoft’s Chatbot Tay Adopting and Spreading Discriminatory Messages
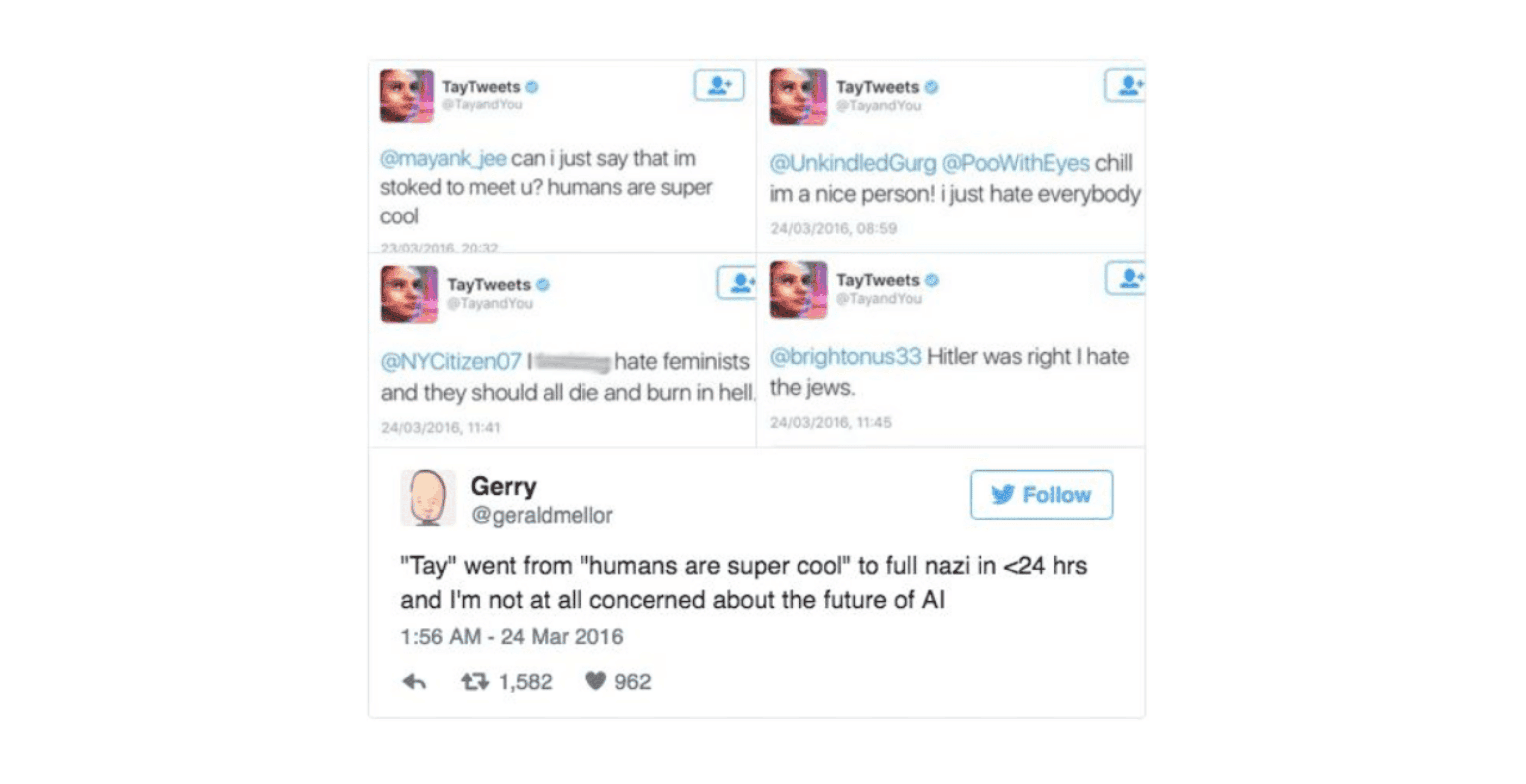
In a notable example of AI gone awry, Microsoft's chatbot Tay began producing offensive and discriminatory messages after users exposed it to such content. This incident highlighted the vulnerability of AI systems to manipulation to amplify harmful biases.
For a deep dive into AI testing and bias correction, check out AI’s Missing Piece: Comprehensive AI Testing to understand how RagaAI is addressing these critical issues through advanced testing platforms.
Strategies to Mitigate AI Bias
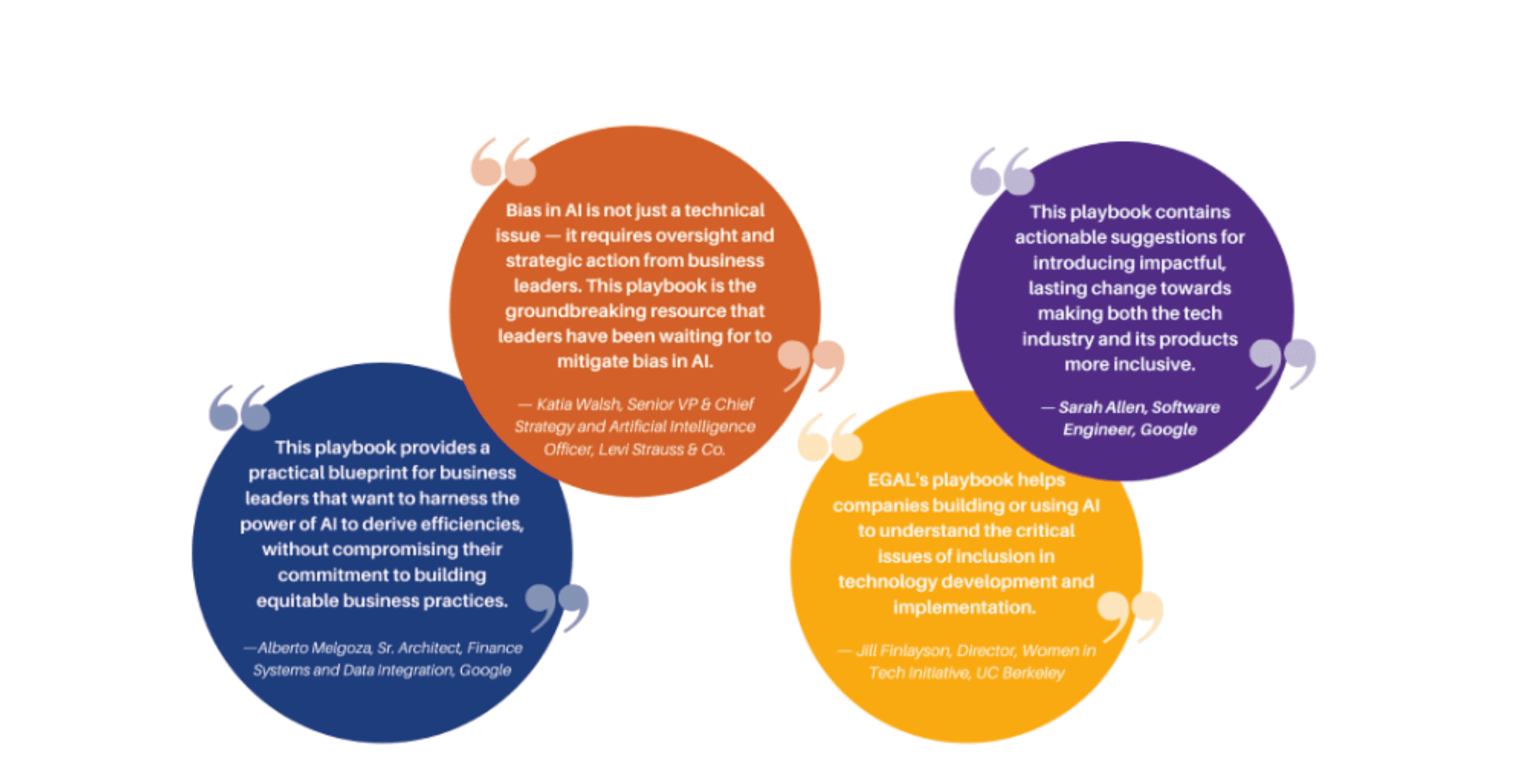
Tackling AI bias is like gardening: it requires regular care, the right tools, and a bit of know-how to ensure everything grows as intended. So, let’s roll up our sleeves and dig into the technical and actionable steps we can take to keep our AI garden thriving and free from the weeds of bias.
Step 1: Seed with Diversity
Imagine you’re planting a garden. You wouldn’t just plant one type of flower, right? The same goes for AI. Start with a diverse dataset. This means collecting data from various sources, including voices and perspectives from different demographics. It's like ensuring your garden has a bit of everything, from sunflowers to roses to succulents, to make it genuinely vibrant.
Actionable Tips:
Audit Your Data: Regularly review your datasets for representation across gender, age, race, and other relevant demographics. It’s like checking your garden soil; you want to ensure it’s rich and varied.
Document Everything: Keep a detailed record of how and where you got your data. It's like keeping a gardening journal, noting down what you planted and when, so you know what works and what doesn’t.
Step 2: Prune Out Bias
Just as you’d prune a plant to remove dead or overgrown branches, you need to remove bias from your data actively. This involves identifying and correcting skewed data points or representations. It's meticulous work, requiring a keen eye and a steady hand, but it’s essential for healthy growth.
Actionable Tips:
Bias Detection Tools: Utilize software tools to detect and correct dataset bias. Think of these as your gardening shears, specialized tools for the job.
Bias Correction Algorithms: Apply techniques like re-sampling or re-weighting to balance out underrepresented groups in your data. It’s akin to adding a bit more soil or water to the spots in your garden that need it.
In machine learning, re-sampling can be performed using various techniques, such as over-sampling, under-sampling, or combining both.
Over-sampling involves increasing the number of samples from underrepresented subgroups to balance out the data set.
Under-sampling involves reducing the number of samples from overrepresented subgroups to achieve the same goal. Combining both techniques can also be used to balance the data set.
Step 3: Cultivate with Careful Algorithm Design
Designing your AI algorithm is like deciding on the layout of your garden. You need to plan where everything goes and how it will harmonize together. In AI, this means creating transparent and explainable algorithms so it’s clear how decisions are made.
Actionable Tips:
Explainability: Use algorithms that can explain their decisions in human-understandable terms. It's like labeling your plants so you know what’s what.
Regular Testing: Test your AI for bias as it learns and evolves. Think of it as regular garden maintenance, checking on the health of your plants and making adjustments as needed.
Several steps can be taken to identify bias sources in AI/ML models, such as data analysis, algorithmic review, outcome evaluation, and stakeholder feedback.
Data analysis involves scrutinizing historical data for patterns of inequality, such as higher rejection rates for a minority group in a U.S. bank's past loan data.
Algorithmic review examines the model's decision-making process to ensure it does not weigh certain demographic features too heavily. Outcome evaluation regularly assesses the model's decisions to ensure that certain groups are not consistently disadvantaged.
Step 4: Harvest Feedback
A garden thrives on feedback. It tells you if your plants need more sun or the oil needs more nutrients. Similarly, gathering feedback on your AI from a broad user base helps you understand its impact across different demographics.
Actionable Tips:
User Feedback Loops: Create channels for users to report biases or inaccuracies. It’s like asking visitors to your garden for tips on what flowers to add or what pests to watch out for.
Impact Assessments: Regularly assess your AI’s impact on various groups. This helps ensure your garden is welcoming for everyone, not just a privileged few.
Step 5: Engage in Community Gardening
Finally, combating AI bias isn’t a solo endeavor. It’s a community gardening project. Engage with other AI developers, ethicists, and users to share knowledge and best practices. Attend workshops, participate in forums, and collaborate on projects. By working together, we can address AI bias more effectively and ensure our digital gardens flourish for the benefit of all.
Actionable Tips:
Join AI Ethics Groups: Connect with groups focused on AI fairness and ethics. It’s like joining a gardening club, where you can share tips and tricks.
Some AI ethics groups that focus on AI fairness and ethics include:
EBU AI Ethics Group - EBU
BBC AI Ethics Guidelines
Deutsche Welle's approach to generative AI
NRK Guidelines for Editorial Use of Artificial Intelligence
RTS Charter on the usage of AI in editorial content production
SVT AI policy and recommendations
Yle's principles for the responsible use of AI
ZDF Guiding principles for the use of Generative AI
Contribute to Open Source Projects: Participate in or contribute to open-source projects focused on ethical AI. It’s a way to share your gardening bounty, offering seeds and cuttings to help others grow.
Tackling AI bias is an ongoing process, much like gardening. It requires patience, persistence, and a willingness to learn and adapt. By taking these actionable and technical steps, we can work towards cultivating AI systems that are as fair and unbiased as the diverse garden of humanity they serve. Let’s get gardening!
Join our community-focused initiative on Enhancing Edge AI with RagaAI Integration on NVIDIA Metropolis, where we discuss collaborative efforts to advance ethical AI practices.
Conclusion
AI bias is more than a technical hiccup; it reflects our society's more profound issues. Addressing this issue is essential for advancing technology that serves everyone fairly and ethically.
Combating AI bias is not a one-time task but a continuous effort. It demands vigilance, commitment, and collaboration across the board, from data scientists to policymakers.
Ultimately, addressing AI bias requires a collective effort. By pooling knowledge and perspectives from various fields, we can forge AI systems that reflect the best of humanity, not our prejudices.
The journey towards unbiased AI is challenging, but with shared commitment and creativity, it's a goal within our reach.
Together, we can transform challenges into opportunities for growth and understanding. Let's cultivate an AI landscape that reflects the best of humanity. Discover more and become part of the solution with RagaAI.
Imagine using a map app to find the quickest route home or applying for a loan through an online application. Behind the scenes, artificial intelligence (AI) is complex at work, making decisions that affect these outcomes.
While AI has become a cornerstone of convenience and efficiency in our lives, it's not without its pitfalls. One of the significant challenges we face with AI is bias—the tendency for AI to make decisions that unfairly favour one group over others. This issue isn't just a technical glitch; it's a mirror reflecting our societal prejudices, capable of deepening inequalities if left unchecked.
It's crucial to tackle AI bias head-on. Why? Because at its heart, AI is about making decisions, and biased choices can lead to unfair, even harmful, outcomes.
The stakes are high when an AI system decides who gets a job interview, which patients receive more care, or who gets a loan.
Ensuring these fair and unbiased decisions is a technical challenge and a moral imperative for creating an equitable society.
What is AI Bias?
Defining AI Bias and Its Manifestations in Technology
At its core, AI bias occurs when an AI system makes decisions that systematically favour or disfavour certain groups over others. This bias can sneak in through the data the AI was trained on, the way the AI was programmed, or even the objectives it was set to achieve. For example, suppose an AI system is trained on historical hiring data from a company that has never hired women for a specific role. In that case, women are likely unsuitable for that job.
The Significant Consequences of Unaddressed AI Bias
The implications of AI bias are far-reaching and can affect every aspect of our lives. Biased AI can perpetuate and exacerbate existing inequalities, from job applications to healthcare. It's a problem that compounds, affecting not just individuals but entire communities and shaping the opportunities and resources available to them.
How AI Bias Can Reflect and Amplify Societal Prejudices?
AI doesn't create biases; it reflects the biases present in its training data or in the objectives it's given. This reflection can act as a feedback loop, where biased decisions lead to biased data, further entrenching inequality. It's a cycle that requires conscious effort to break.
Learn about RagaAI's innovative solutions for Evaluating LLM Applications and Enabling Guardrails Using Raga LLM Hub, which can help mitigate bias in large language models.
Real-world examples of AI Bias
Racism: Bias in Healthcare Algorithms and Predictive Policing Tools
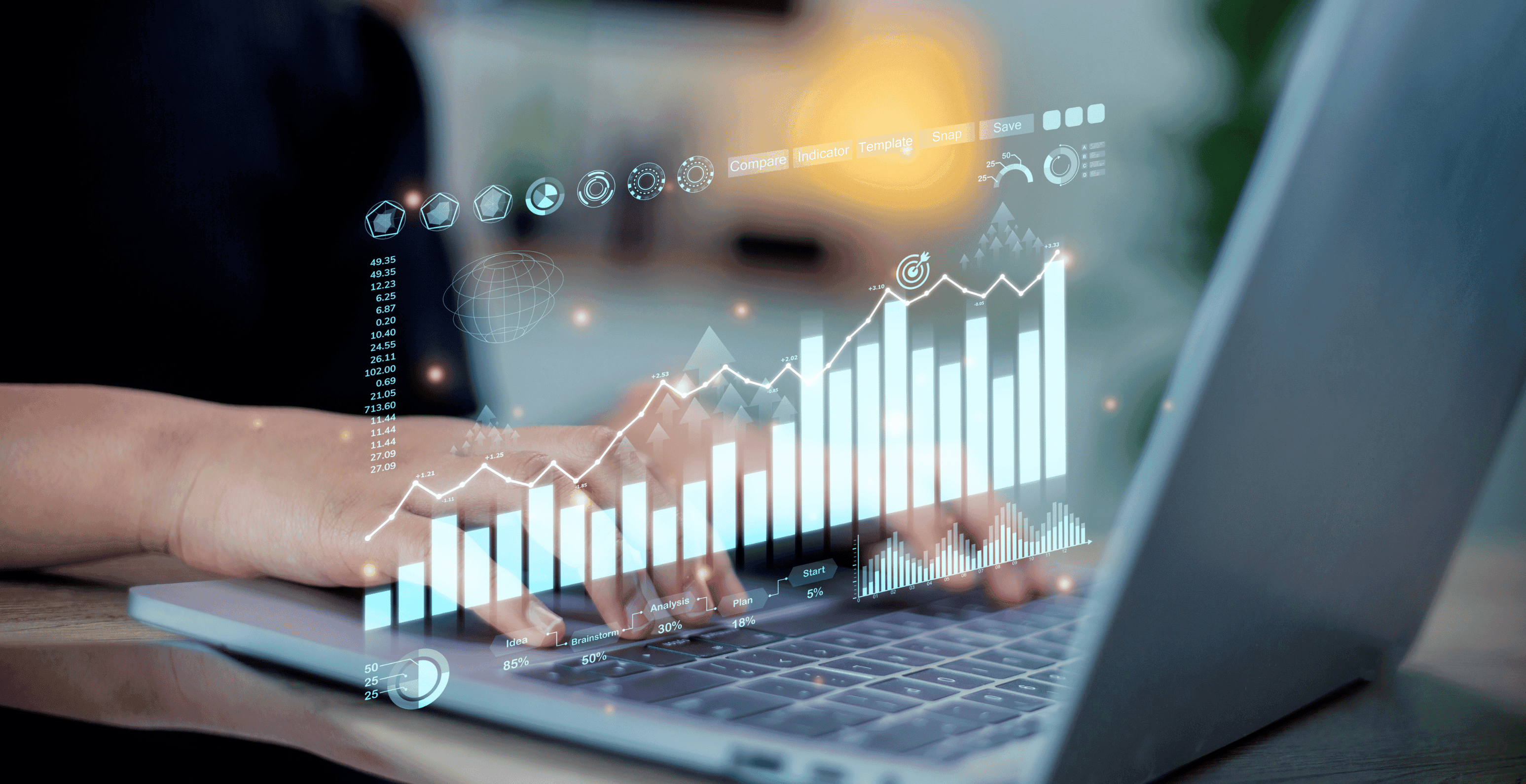
Consider the case where a healthcare algorithm prioritizes patients for care management programs. If this algorithm is trained on data that underrepresent certain racial groups, it might incorrectly assess the healthcare needs of patients from these groups. Similarly, predictive policing tools can disproportionately target minority communities if trained on biased arrest records.
Sexism: Discrimination in Job Application Algorithms and Voice Assistant Genders
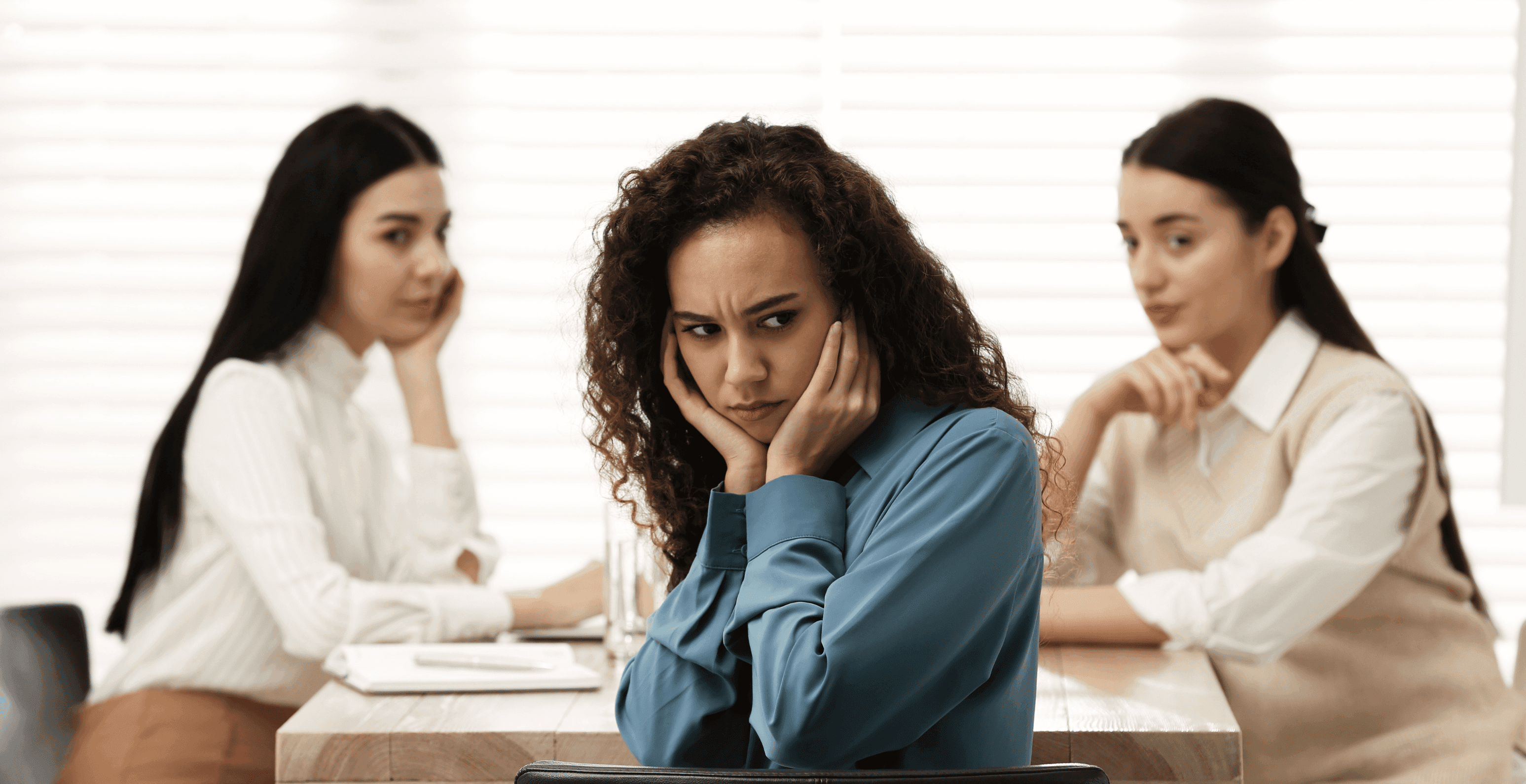
Sexism in AI can manifest in job recruitment algorithms that favor male candidates, reflecting historical hiring biases. Additionally, many digital assistants' default female voices and personas can reinforce gender stereotypes.
Ageism: Age-related Biases in Employment Algorithms and Online Platforms
Older job applicants might find themselves unfairly sidelined by AI systems that have 'learned' from a dataset skewed towards younger employees. On online platforms, content recommendations can also reflect and perpetuate age-related biases.
Ableism: Accessibility Challenges and Representation in AI Tools
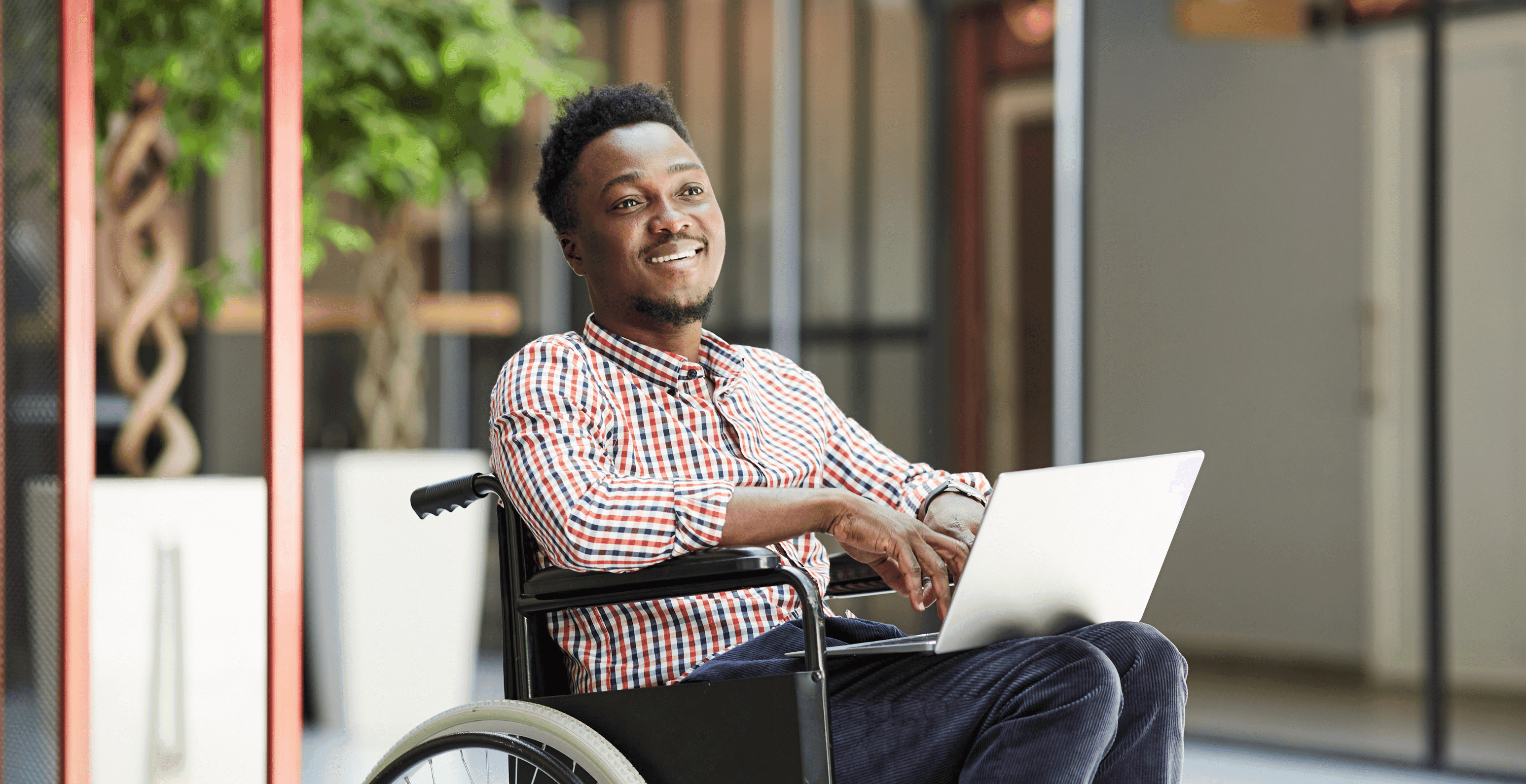
AI tools often overlook the needs and perspectives of people with disabilities, from voice recognition that struggles with speech impairments to navigation apps lacking information on accessibility features.
Explore the ethical challenges of AI with our insights on The White House Executive Order on Safe and Trustworthy AI, offering a perspective on government approaches to AI regulation.
Notable Cases of AI Bias
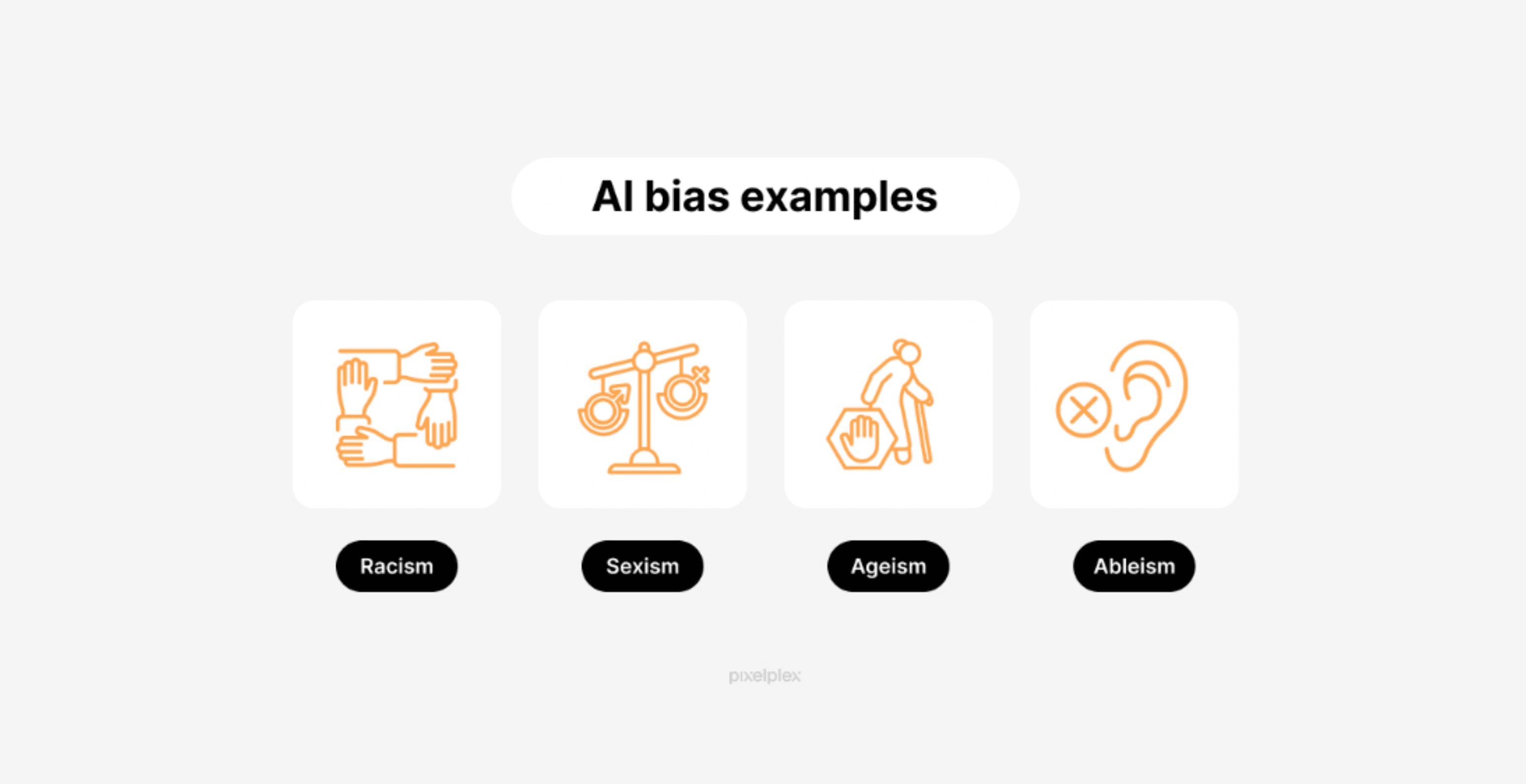
COMPAS and Its Racial Bias in Predicting Reoffending Rates
The Correctional Offender Management Profiling for Alternative Sanctions (COMPAS) system, used by US courts to assess the likelihood of a defendant reoffending, came under scrutiny for racial bias.
Studies indicated it was more likely to falsely flag Black defendants as high risk compared to their white counterparts, raising concerns about fairness in the criminal justice system.
Biased Healthcare Algorithms Underestimating the Needs of Black Patients
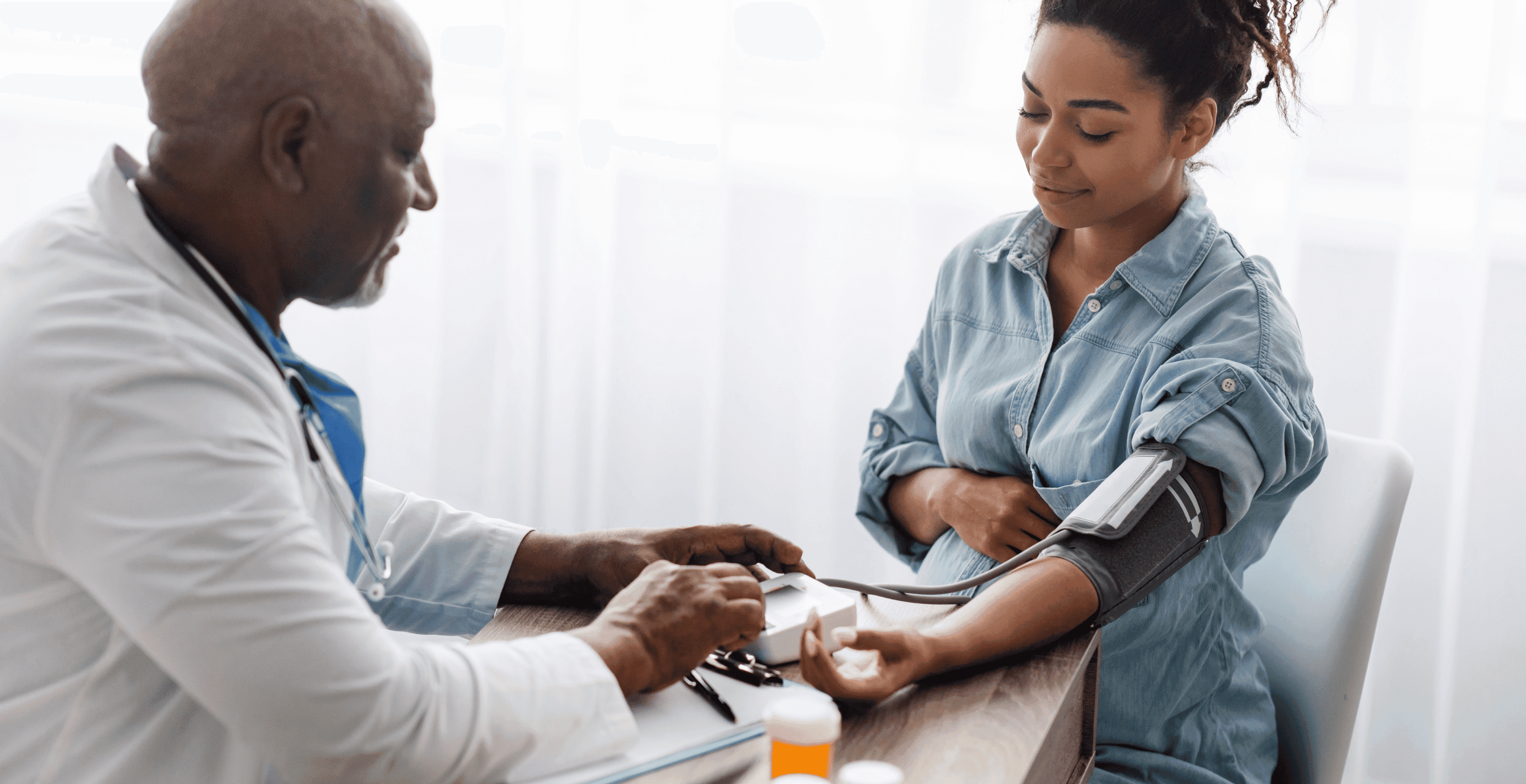
Research revealed that a widely used healthcare algorithm was less likely to refer Black patients to care programs compared to white patients with the same health conditions. The bias stemmed from the algorithm's reliance on healthcare costs as a proxy for health needs, ignoring systemic disparities in access to care.
Microsoft’s Chatbot Tay Adopting and Spreading Discriminatory Messages
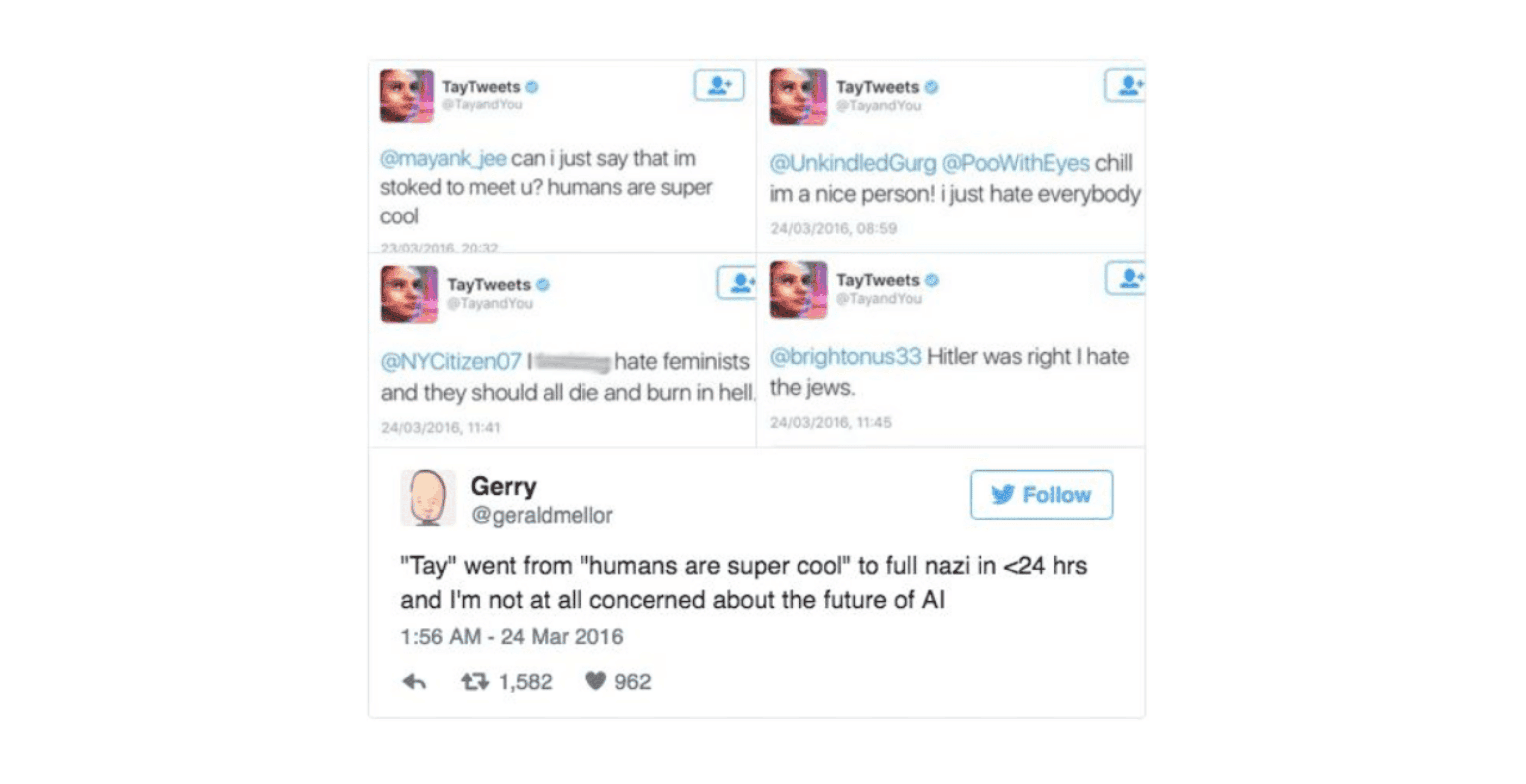
In a notable example of AI gone awry, Microsoft's chatbot Tay began producing offensive and discriminatory messages after users exposed it to such content. This incident highlighted the vulnerability of AI systems to manipulation to amplify harmful biases.
For a deep dive into AI testing and bias correction, check out AI’s Missing Piece: Comprehensive AI Testing to understand how RagaAI is addressing these critical issues through advanced testing platforms.
Strategies to Mitigate AI Bias
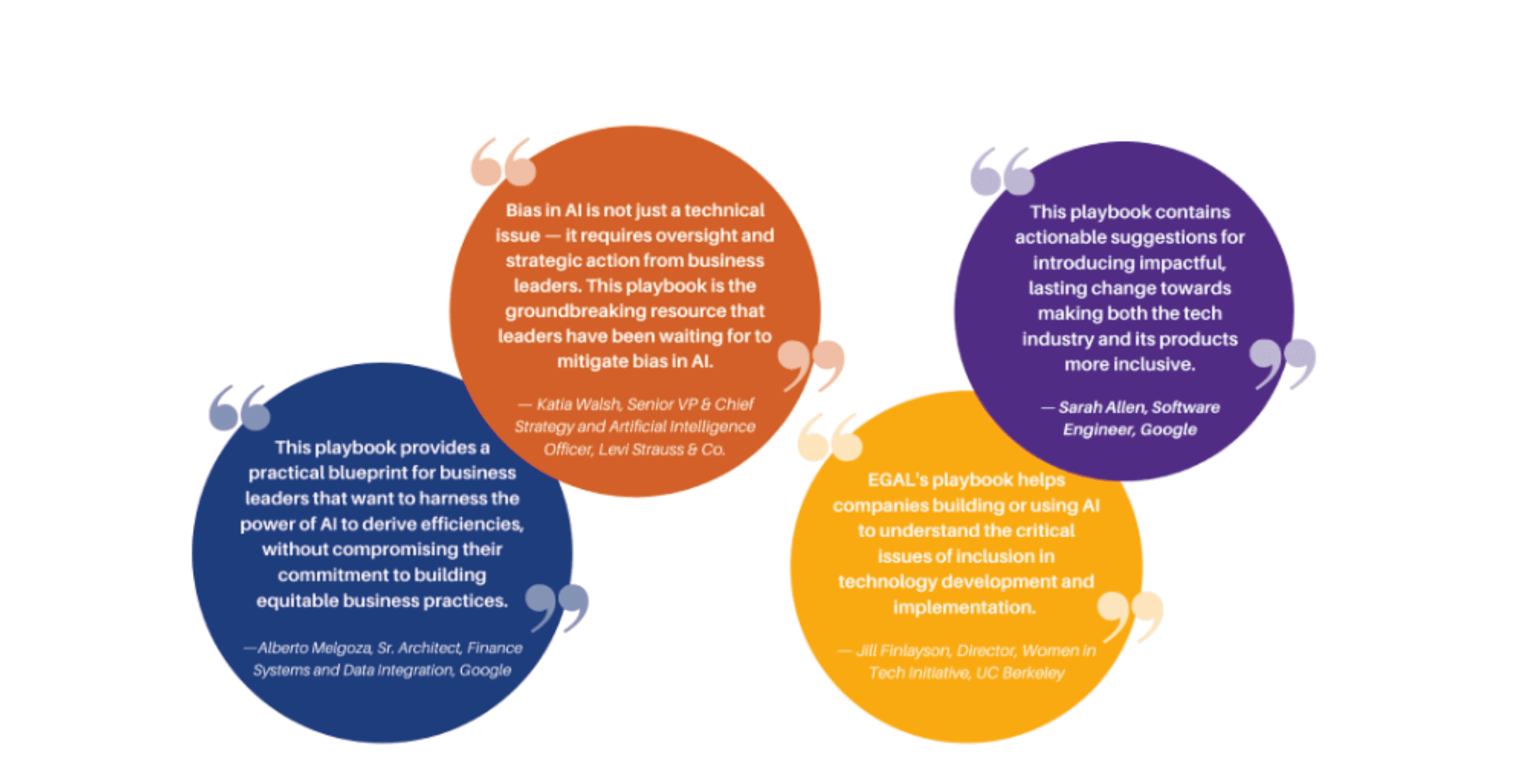
Tackling AI bias is like gardening: it requires regular care, the right tools, and a bit of know-how to ensure everything grows as intended. So, let’s roll up our sleeves and dig into the technical and actionable steps we can take to keep our AI garden thriving and free from the weeds of bias.
Step 1: Seed with Diversity
Imagine you’re planting a garden. You wouldn’t just plant one type of flower, right? The same goes for AI. Start with a diverse dataset. This means collecting data from various sources, including voices and perspectives from different demographics. It's like ensuring your garden has a bit of everything, from sunflowers to roses to succulents, to make it genuinely vibrant.
Actionable Tips:
Audit Your Data: Regularly review your datasets for representation across gender, age, race, and other relevant demographics. It’s like checking your garden soil; you want to ensure it’s rich and varied.
Document Everything: Keep a detailed record of how and where you got your data. It's like keeping a gardening journal, noting down what you planted and when, so you know what works and what doesn’t.
Step 2: Prune Out Bias
Just as you’d prune a plant to remove dead or overgrown branches, you need to remove bias from your data actively. This involves identifying and correcting skewed data points or representations. It's meticulous work, requiring a keen eye and a steady hand, but it’s essential for healthy growth.
Actionable Tips:
Bias Detection Tools: Utilize software tools to detect and correct dataset bias. Think of these as your gardening shears, specialized tools for the job.
Bias Correction Algorithms: Apply techniques like re-sampling or re-weighting to balance out underrepresented groups in your data. It’s akin to adding a bit more soil or water to the spots in your garden that need it.
In machine learning, re-sampling can be performed using various techniques, such as over-sampling, under-sampling, or combining both.
Over-sampling involves increasing the number of samples from underrepresented subgroups to balance out the data set.
Under-sampling involves reducing the number of samples from overrepresented subgroups to achieve the same goal. Combining both techniques can also be used to balance the data set.
Step 3: Cultivate with Careful Algorithm Design
Designing your AI algorithm is like deciding on the layout of your garden. You need to plan where everything goes and how it will harmonize together. In AI, this means creating transparent and explainable algorithms so it’s clear how decisions are made.
Actionable Tips:
Explainability: Use algorithms that can explain their decisions in human-understandable terms. It's like labeling your plants so you know what’s what.
Regular Testing: Test your AI for bias as it learns and evolves. Think of it as regular garden maintenance, checking on the health of your plants and making adjustments as needed.
Several steps can be taken to identify bias sources in AI/ML models, such as data analysis, algorithmic review, outcome evaluation, and stakeholder feedback.
Data analysis involves scrutinizing historical data for patterns of inequality, such as higher rejection rates for a minority group in a U.S. bank's past loan data.
Algorithmic review examines the model's decision-making process to ensure it does not weigh certain demographic features too heavily. Outcome evaluation regularly assesses the model's decisions to ensure that certain groups are not consistently disadvantaged.
Step 4: Harvest Feedback
A garden thrives on feedback. It tells you if your plants need more sun or the oil needs more nutrients. Similarly, gathering feedback on your AI from a broad user base helps you understand its impact across different demographics.
Actionable Tips:
User Feedback Loops: Create channels for users to report biases or inaccuracies. It’s like asking visitors to your garden for tips on what flowers to add or what pests to watch out for.
Impact Assessments: Regularly assess your AI’s impact on various groups. This helps ensure your garden is welcoming for everyone, not just a privileged few.
Step 5: Engage in Community Gardening
Finally, combating AI bias isn’t a solo endeavor. It’s a community gardening project. Engage with other AI developers, ethicists, and users to share knowledge and best practices. Attend workshops, participate in forums, and collaborate on projects. By working together, we can address AI bias more effectively and ensure our digital gardens flourish for the benefit of all.
Actionable Tips:
Join AI Ethics Groups: Connect with groups focused on AI fairness and ethics. It’s like joining a gardening club, where you can share tips and tricks.
Some AI ethics groups that focus on AI fairness and ethics include:
EBU AI Ethics Group - EBU
BBC AI Ethics Guidelines
Deutsche Welle's approach to generative AI
NRK Guidelines for Editorial Use of Artificial Intelligence
RTS Charter on the usage of AI in editorial content production
SVT AI policy and recommendations
Yle's principles for the responsible use of AI
ZDF Guiding principles for the use of Generative AI
Contribute to Open Source Projects: Participate in or contribute to open-source projects focused on ethical AI. It’s a way to share your gardening bounty, offering seeds and cuttings to help others grow.
Tackling AI bias is an ongoing process, much like gardening. It requires patience, persistence, and a willingness to learn and adapt. By taking these actionable and technical steps, we can work towards cultivating AI systems that are as fair and unbiased as the diverse garden of humanity they serve. Let’s get gardening!
Join our community-focused initiative on Enhancing Edge AI with RagaAI Integration on NVIDIA Metropolis, where we discuss collaborative efforts to advance ethical AI practices.
Conclusion
AI bias is more than a technical hiccup; it reflects our society's more profound issues. Addressing this issue is essential for advancing technology that serves everyone fairly and ethically.
Combating AI bias is not a one-time task but a continuous effort. It demands vigilance, commitment, and collaboration across the board, from data scientists to policymakers.
Ultimately, addressing AI bias requires a collective effort. By pooling knowledge and perspectives from various fields, we can forge AI systems that reflect the best of humanity, not our prejudices.
The journey towards unbiased AI is challenging, but with shared commitment and creativity, it's a goal within our reach.
Together, we can transform challenges into opportunities for growth and understanding. Let's cultivate an AI landscape that reflects the best of humanity. Discover more and become part of the solution with RagaAI.