Using RagaAI Catalyst to Evaluate LLM Applications
Gaurav Agarwal
Aug 20, 2024
Other articles
Exploring Intelligent Agents in AI
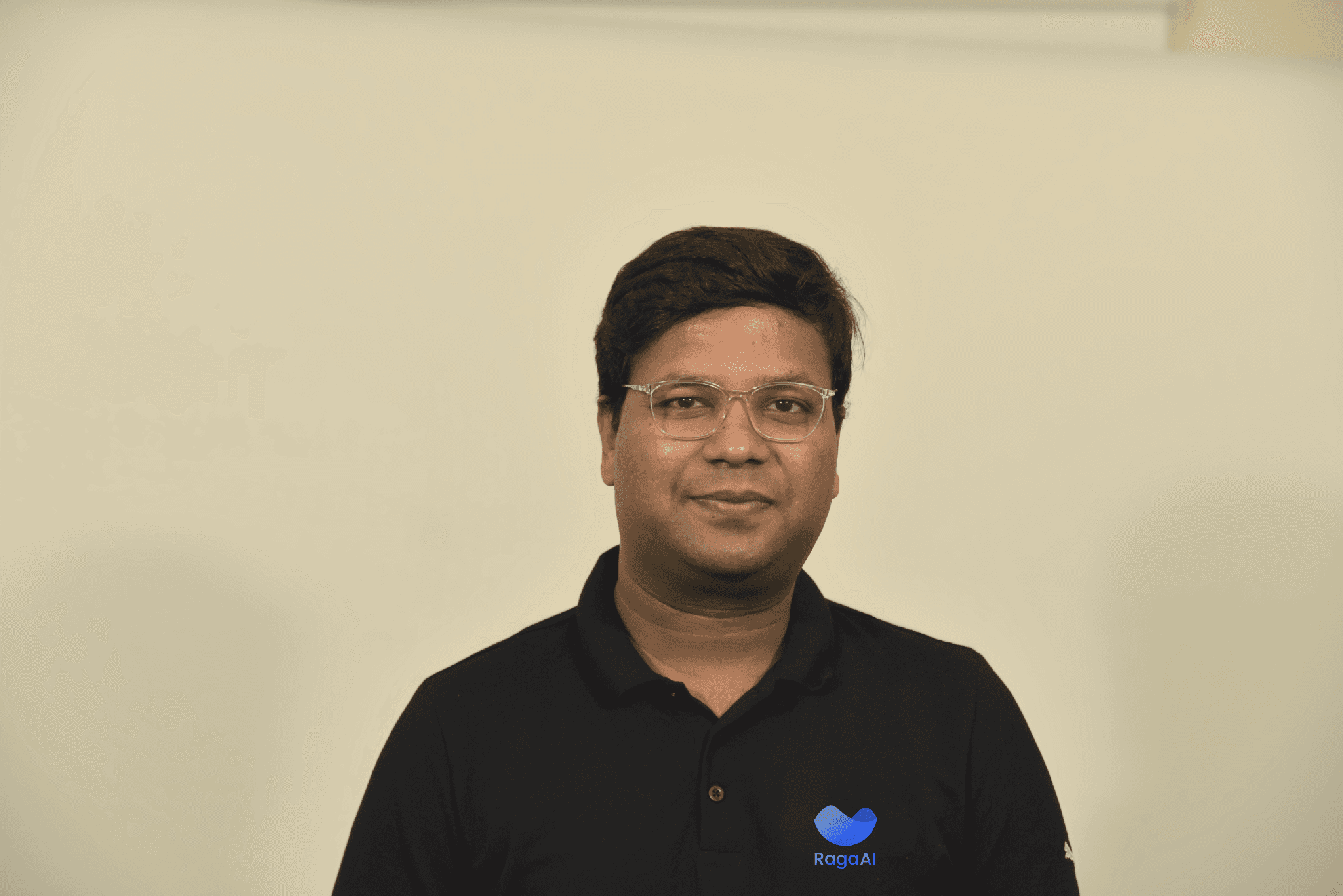
Jigar Gupta
Sep 6, 2024
Read the article
Understanding What AI Red Teaming Means for Generative Models
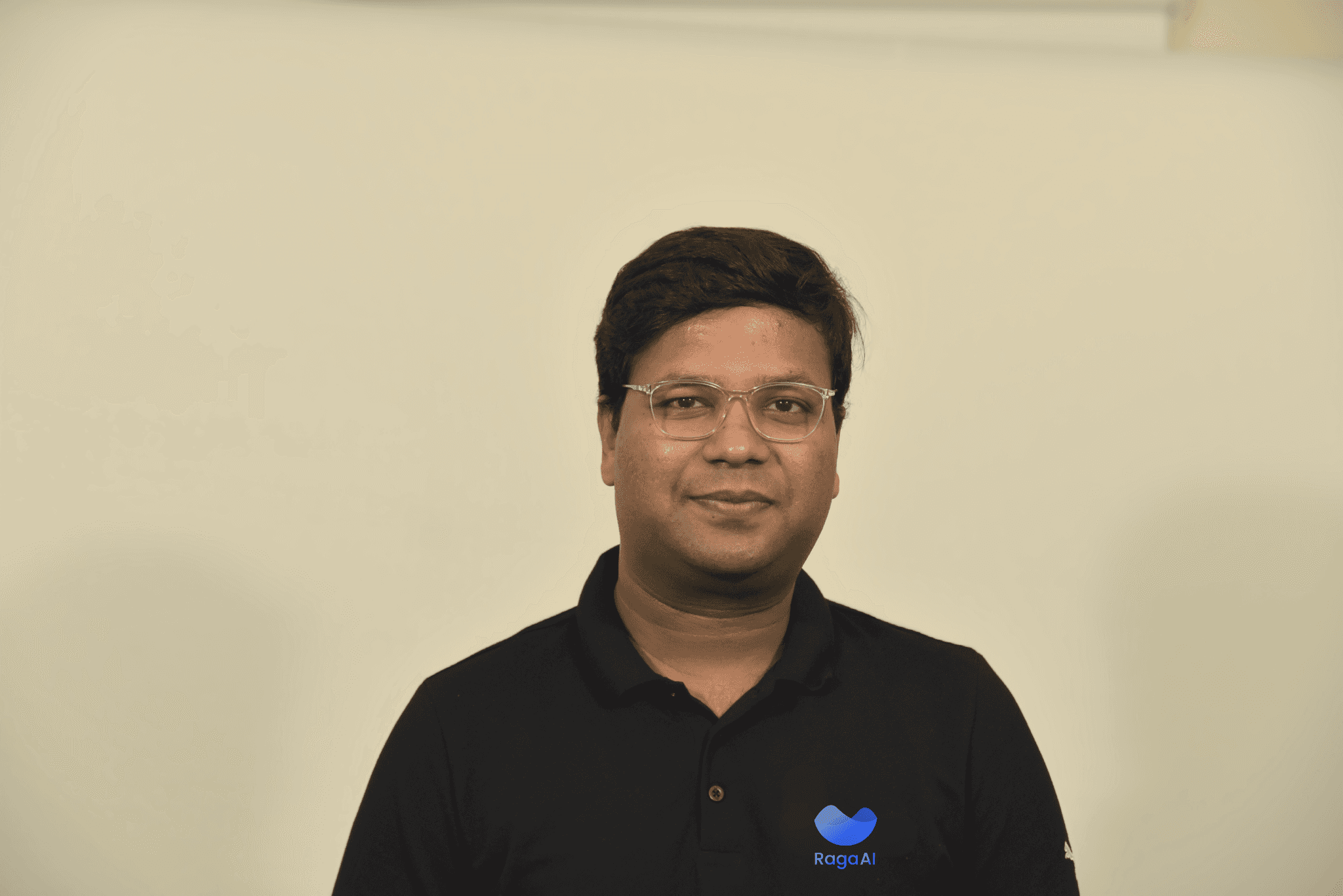
Jigar Gupta
Sep 4, 2024
Read the article
RAG vs Fine-Tuning: Choosing the Best AI Learning Technique
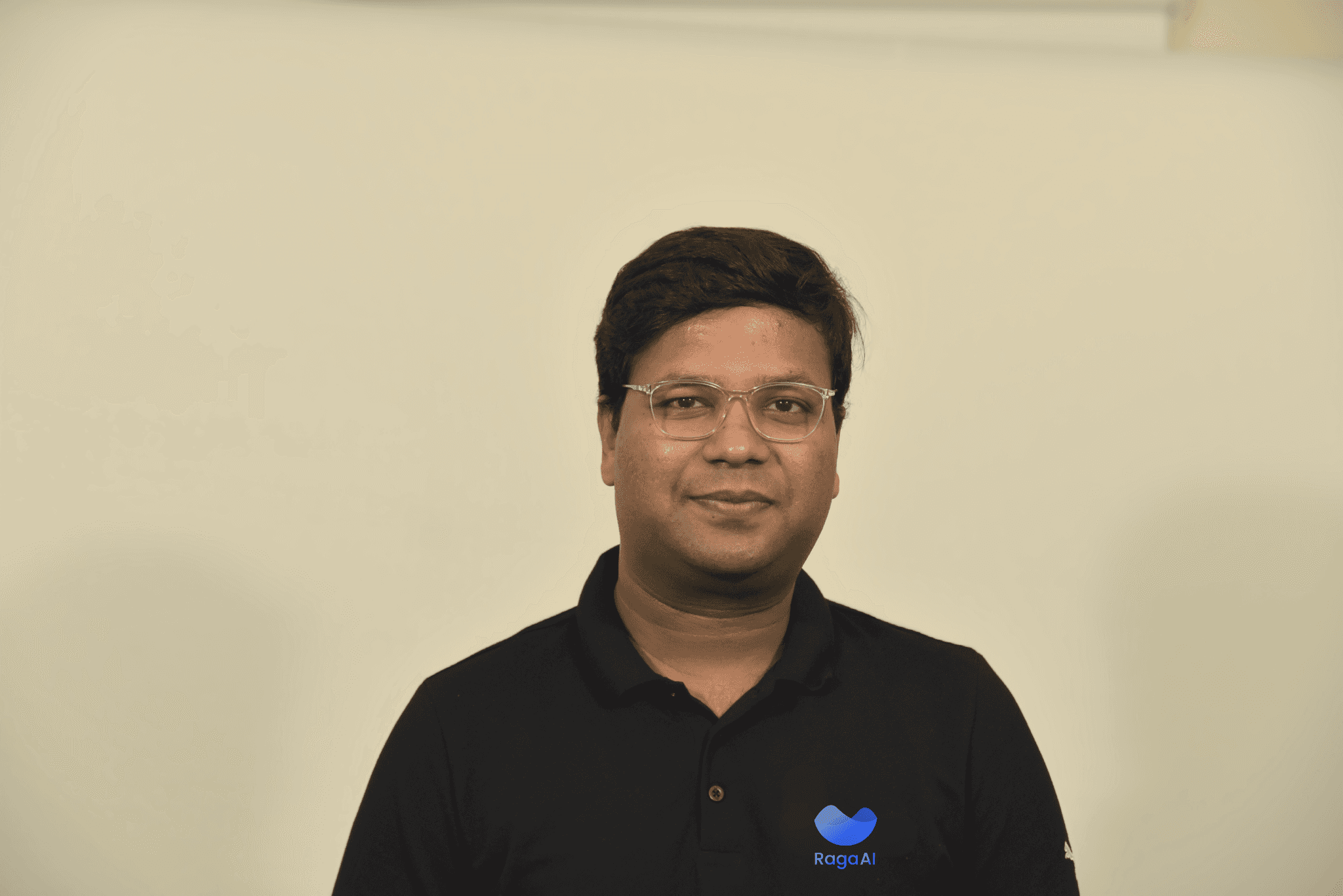
Jigar Gupta
Sep 4, 2024
Read the article
Understanding NeMo Guardrails: A Toolkit for LLM Security

Rehan Asif
Sep 4, 2024
Read the article
Understanding Differences in Large vs Small Language Models (LLM vs SLM)

Rehan Asif
Sep 4, 2024
Read the article
Understanding What an AI Agent is: Key Applications and Examples
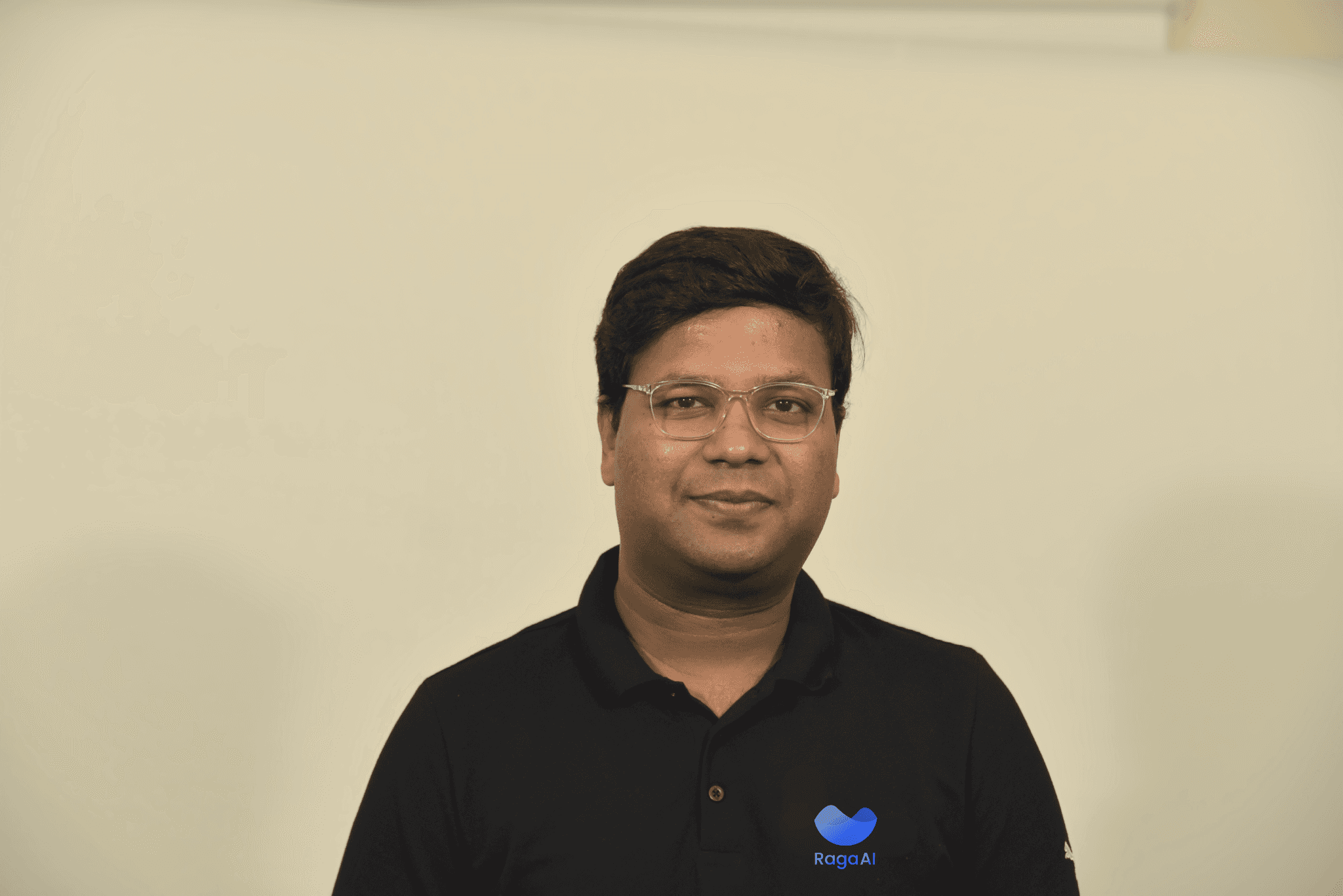
Jigar Gupta
Sep 4, 2024
Read the article
Prompt Engineering and Retrieval Augmented Generation (RAG)
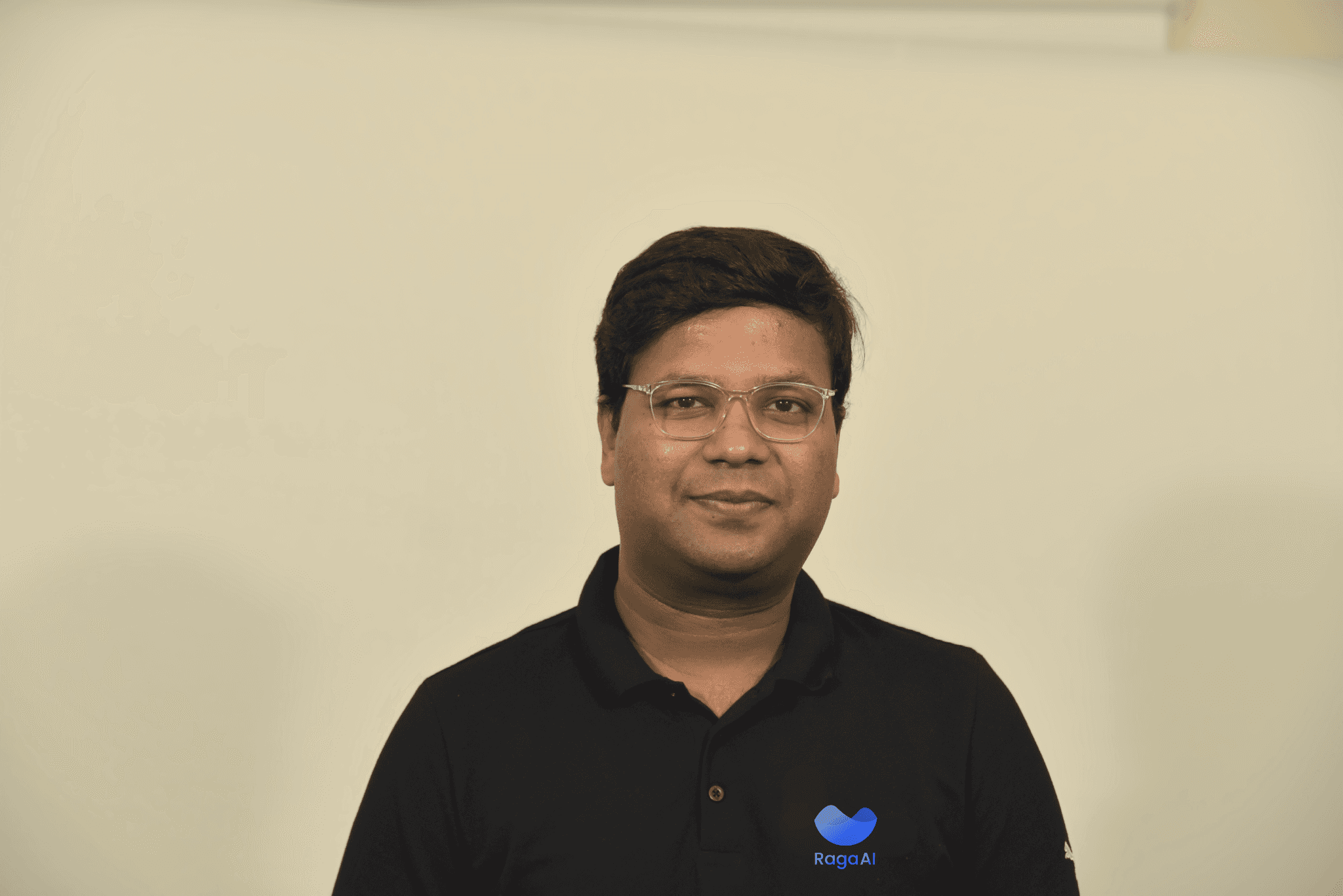
Jigar Gupta
Sep 4, 2024
Read the article
Exploring How Multimodal Large Language Models Work

Rehan Asif
Sep 3, 2024
Read the article
Evaluating and Enhancing LLM-as-a-Judge with Automated Tools

Rehan Asif
Sep 3, 2024
Read the article
Optimizing Performance and Cost by Caching LLM Queries

Rehan Asif
Sep 3, 3034
Read the article
LoRA vs RAG: Full Model Fine-Tuning in Large Language Models
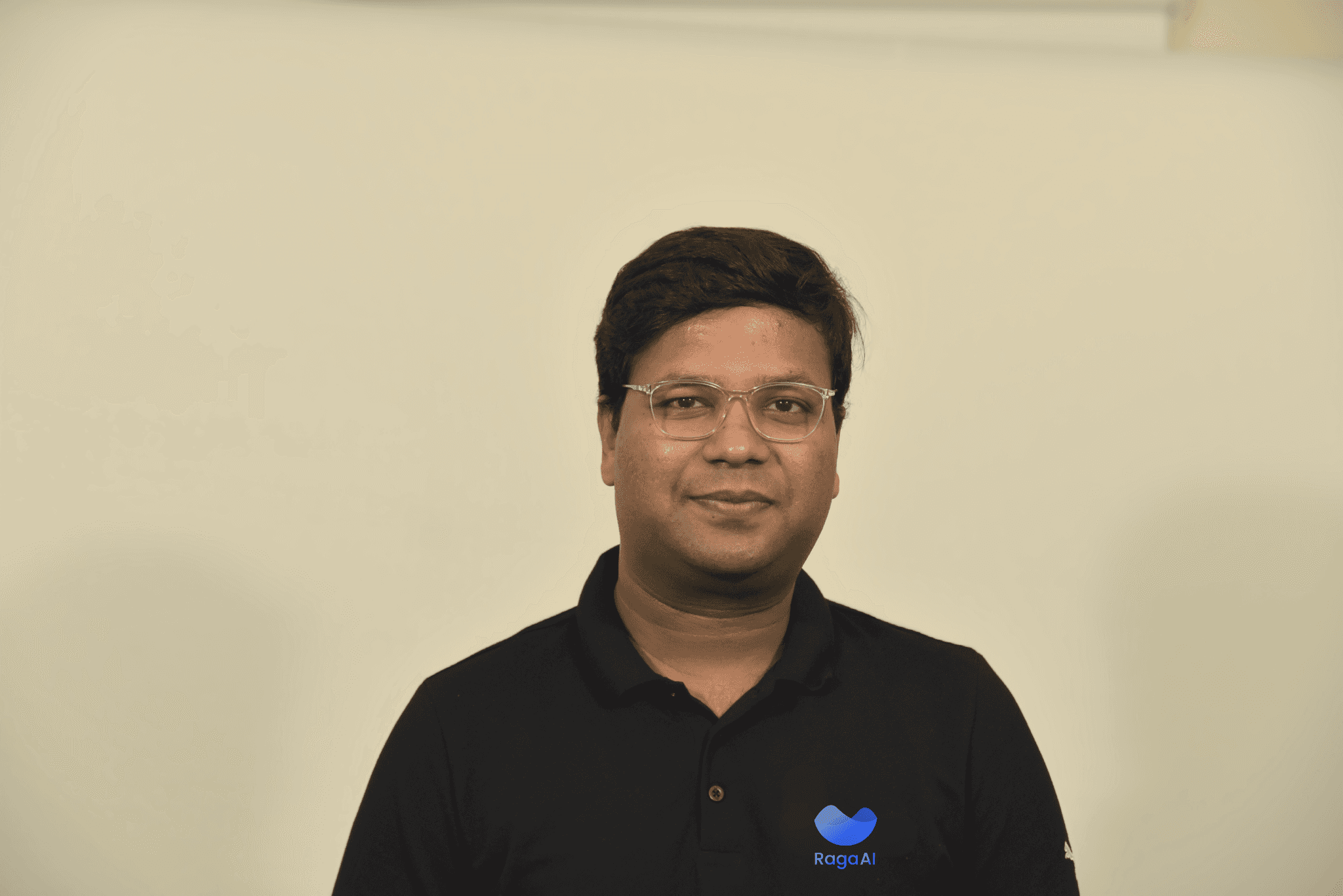
Jigar Gupta
Sep 3, 2024
Read the article
Steps to Train LLM on Personal Data

Rehan Asif
Sep 3, 2024
Read the article
Step by Step Guide to Building RAG-based LLM Applications with Examples

Rehan Asif
Sep 2, 2024
Read the article
Building AI Agentic Workflows with Multi-Agent Collaboration
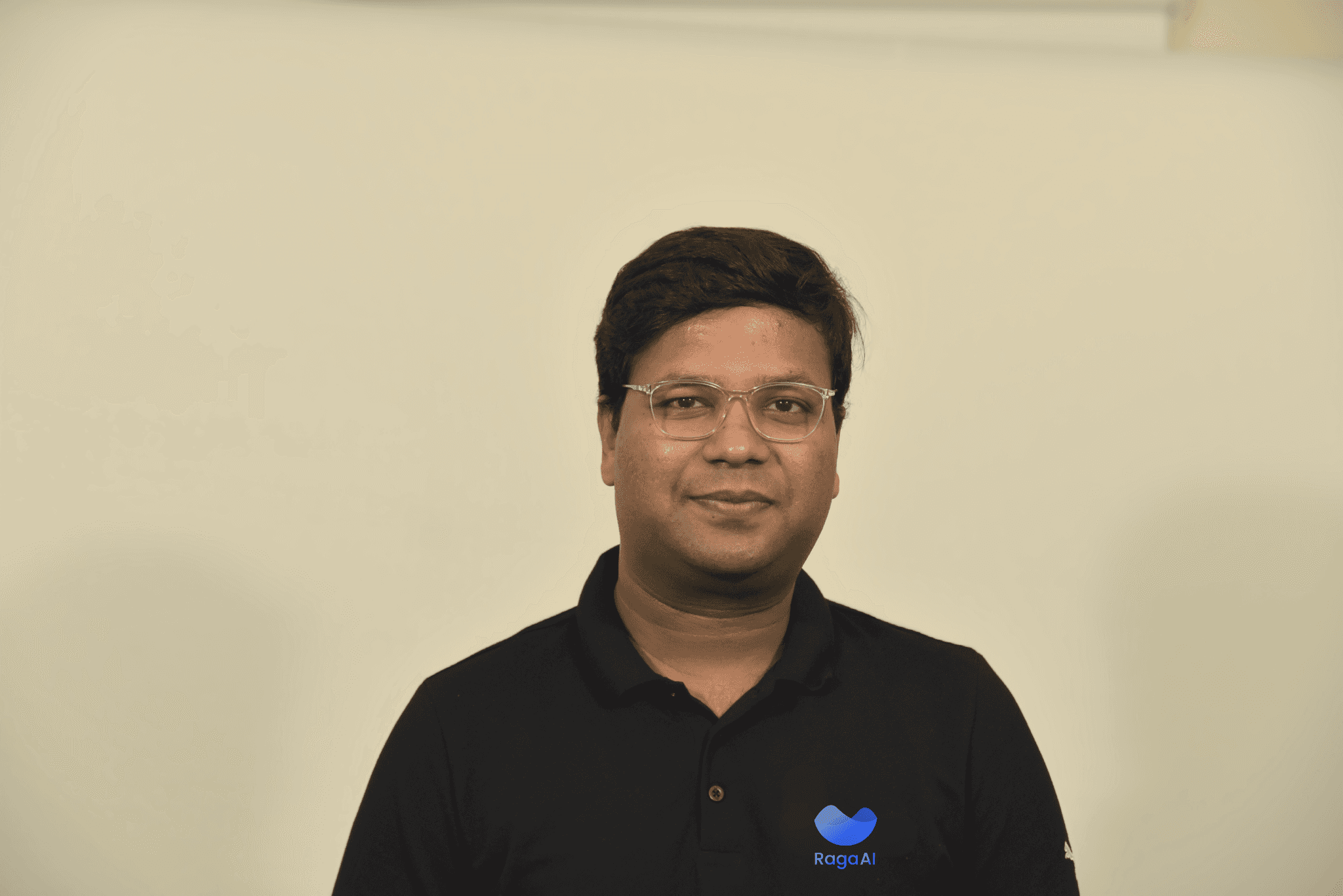
Jigar Gupta
Sep 2, 2024
Read the article
Top Large Language Models (LLMs) in 2024

Rehan Asif
Sep 2, 2024
Read the article
Creating Apps with Large Language Models

Rehan Asif
Sep 2, 2024
Read the article
Best Practices In Data Governance For AI
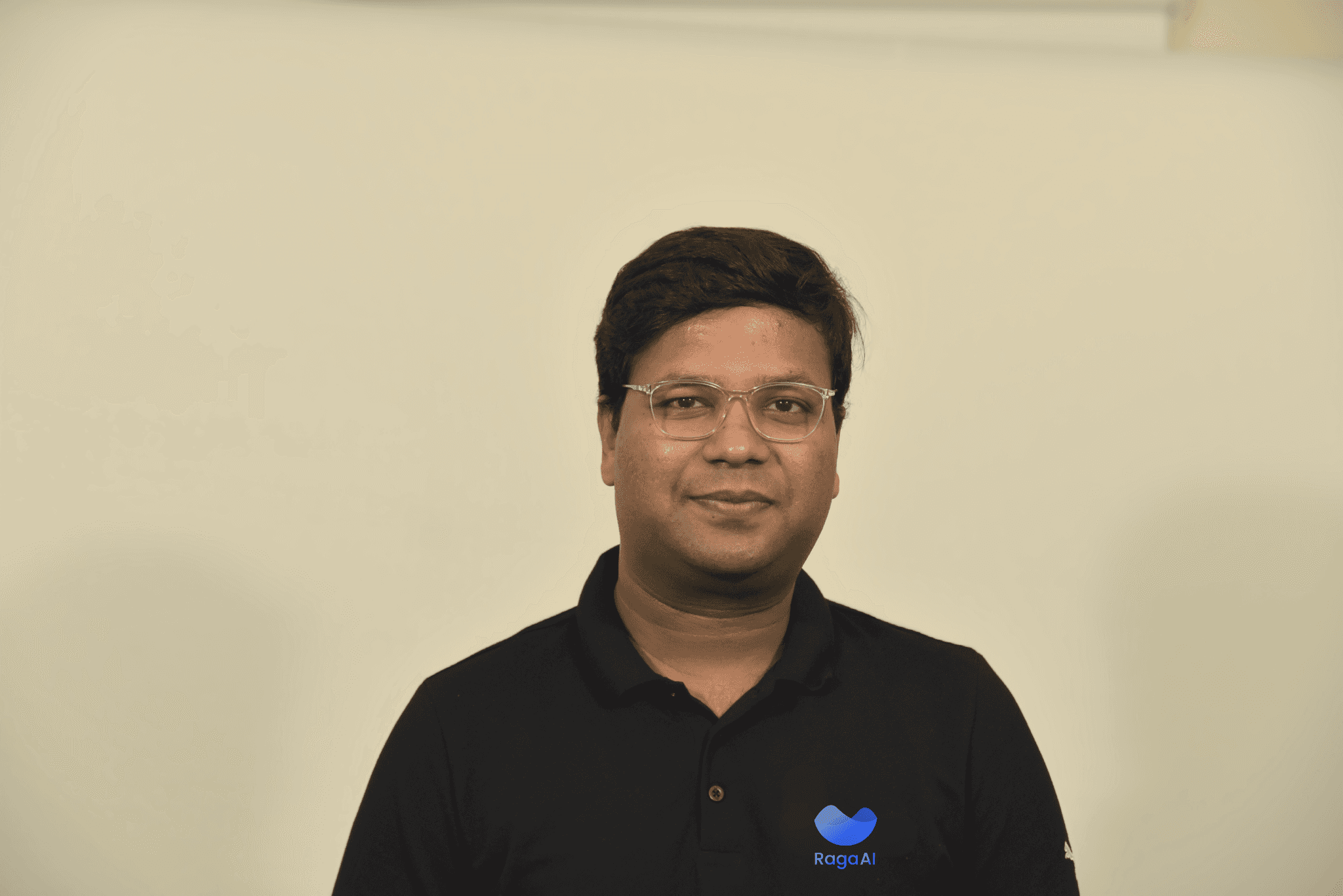
Jigar Gupta
Sep 22, 2024
Read the article
Transforming Conversational AI with Large Language Models

Rehan Asif
Aug 30, 2024
Read the article
Deploying Generative AI Agents with Local LLMs

Rehan Asif
Aug 30, 2024
Read the article
Exploring Different Types of AI Agents with Key Examples
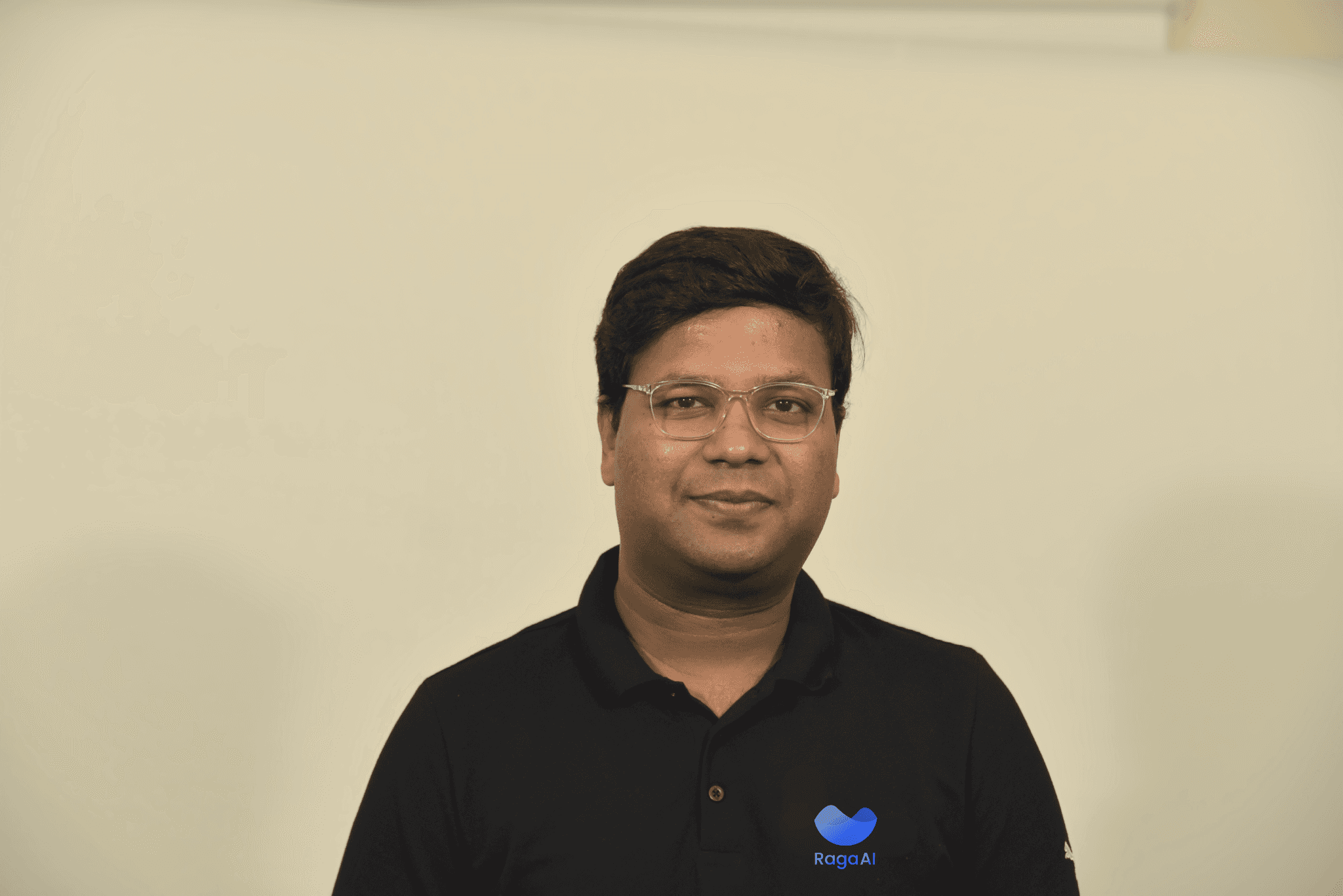
Jigar Gupta
Aug 30, 2024
Read the article
Creating Your Own Personal LLM Agents: Introduction to Implementation

Rehan Asif
Aug 30, 2024
Read the article
Exploring Agentic AI Architecture and Design Patterns
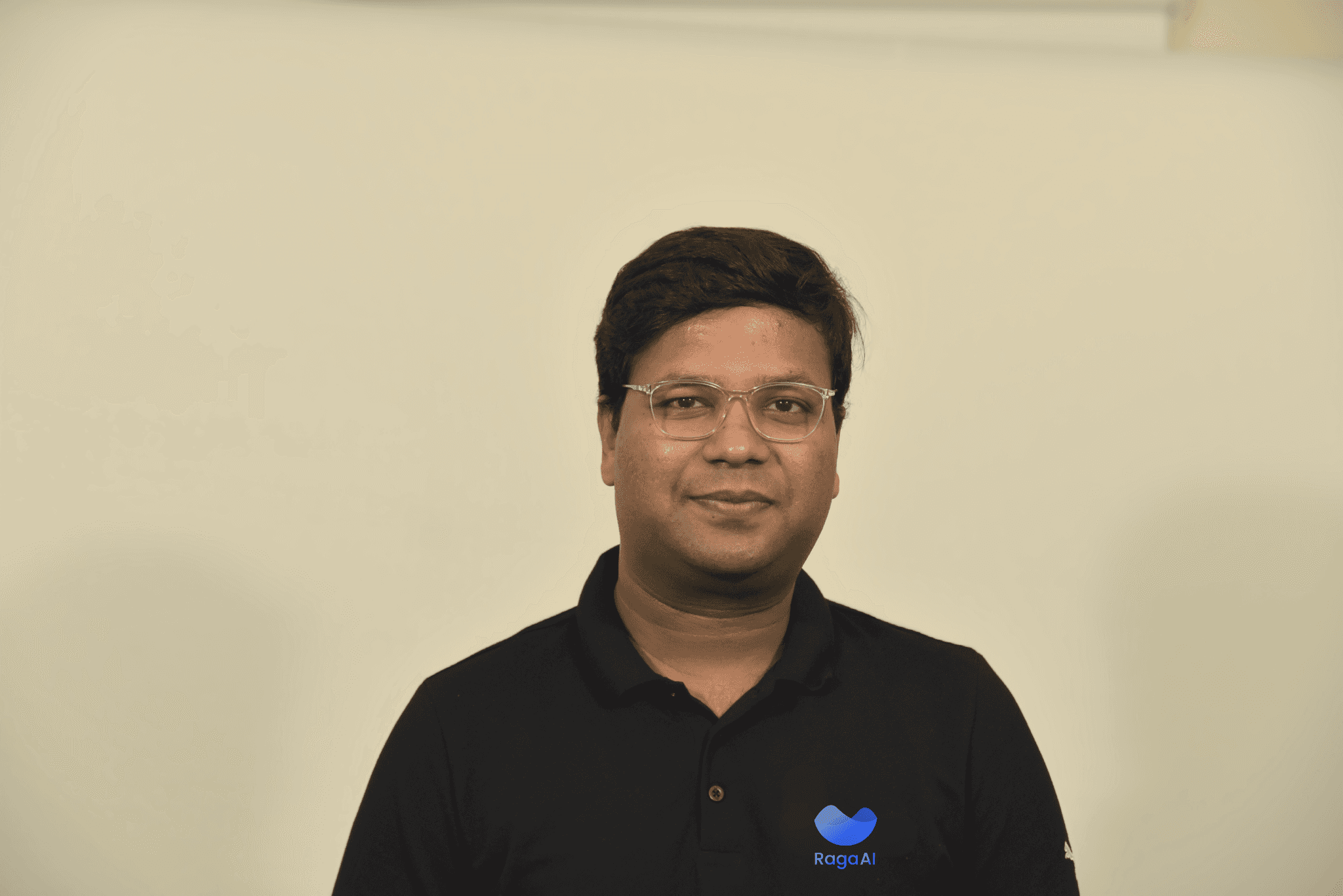
Jigar Gupta
Aug 30, 2024
Read the article
Building Your First LLM Agent Framework Application

Rehan Asif
Aug 29, 2024
Read the article
Multi-Agent Design and Collaboration Patterns

Rehan Asif
Aug 29, 2024
Read the article
Creating Your Own LLM Agent Application from Scratch

Rehan Asif
Aug 29, 2024
Read the article
Solving LLM Token Limit Issues: Understanding and Approaches

Rehan Asif
Aug 29, 2024
Read the article
Understanding the Impact of Inference Cost on Generative AI Adoption
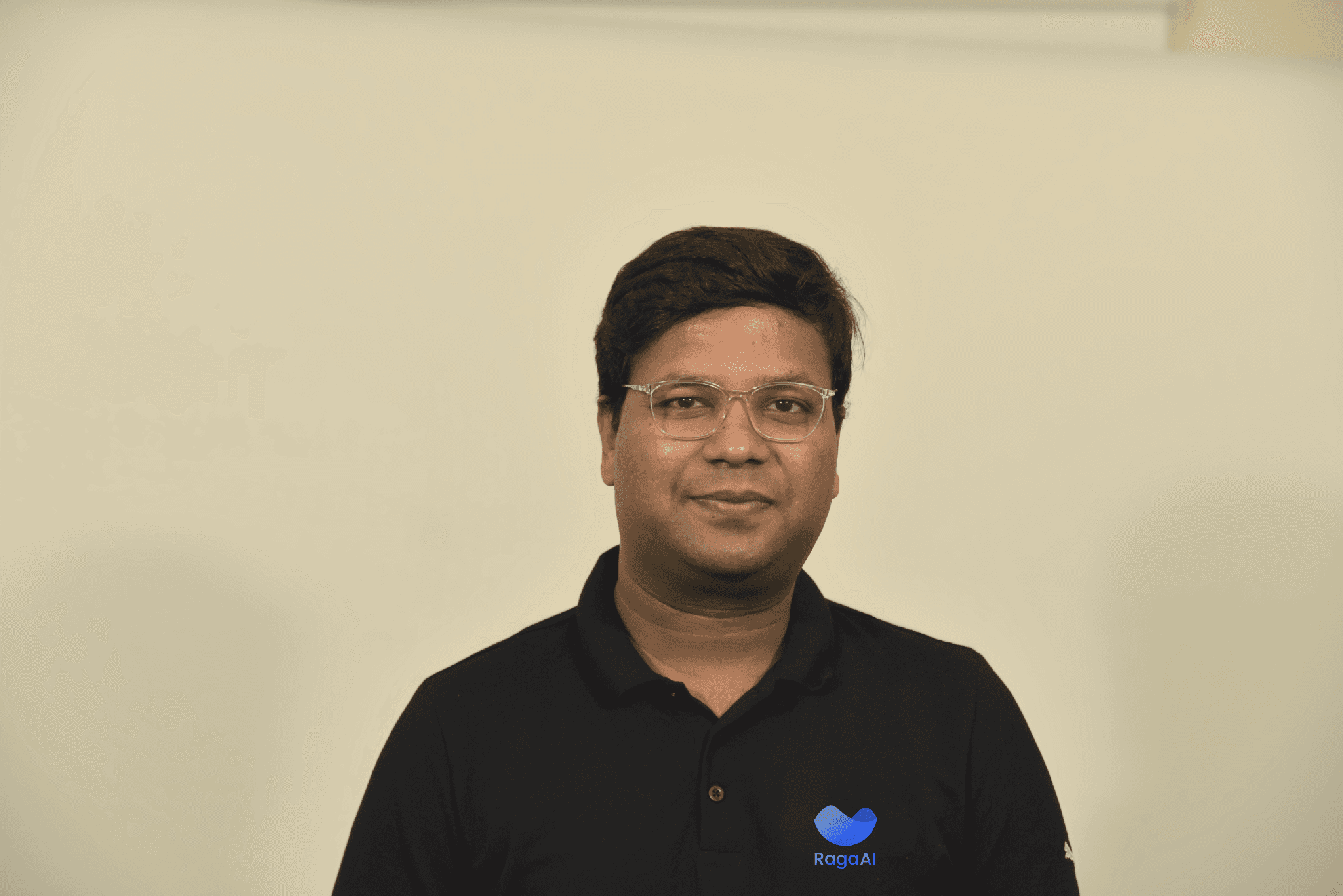
Jigar Gupta
Aug 28, 2024
Read the article
Data Security: Risks, Solutions, Types and Best Practices
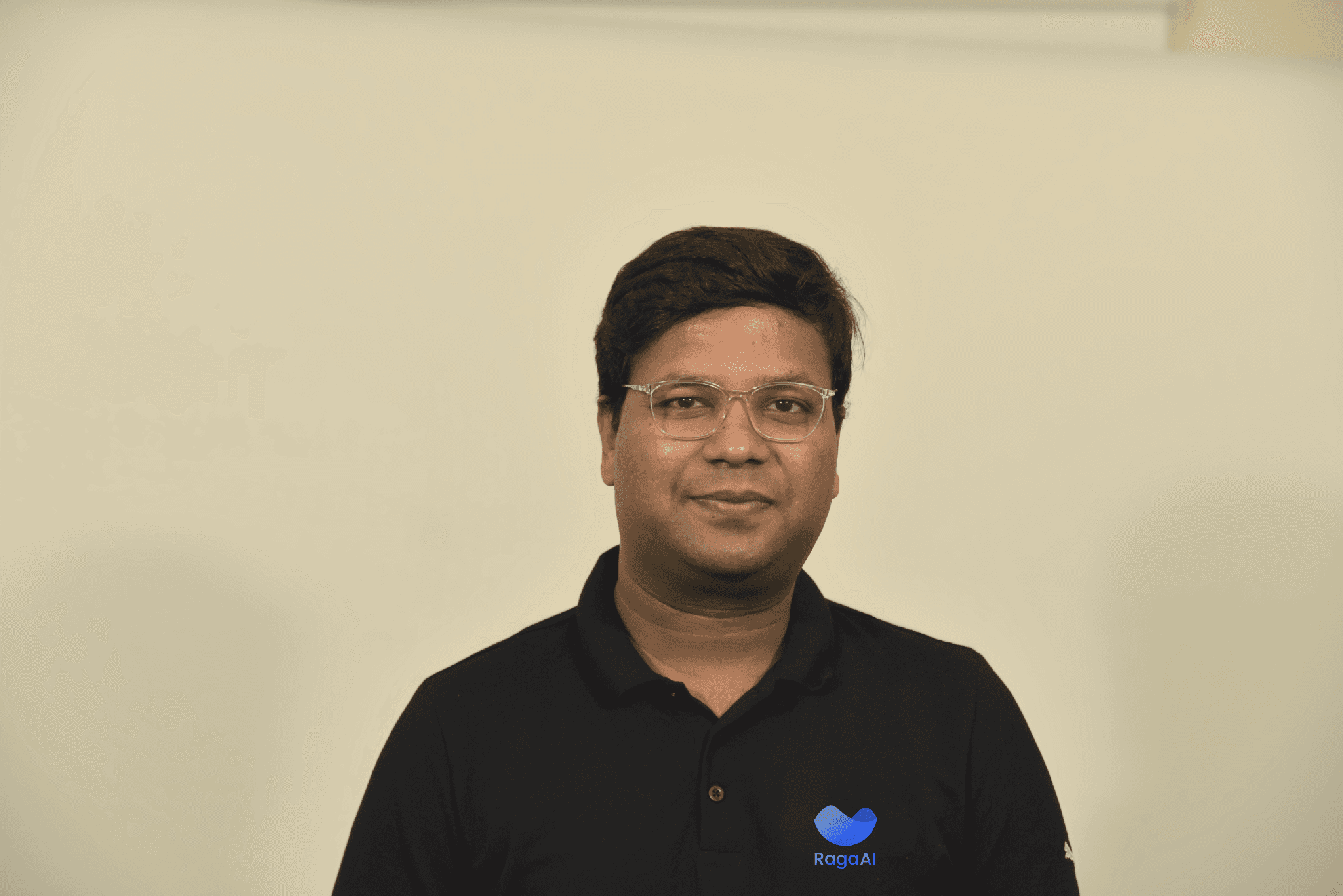
Jigar Gupta
Aug 28, 2024
Read the article
Getting Contextual Understanding Right for RAG Applications
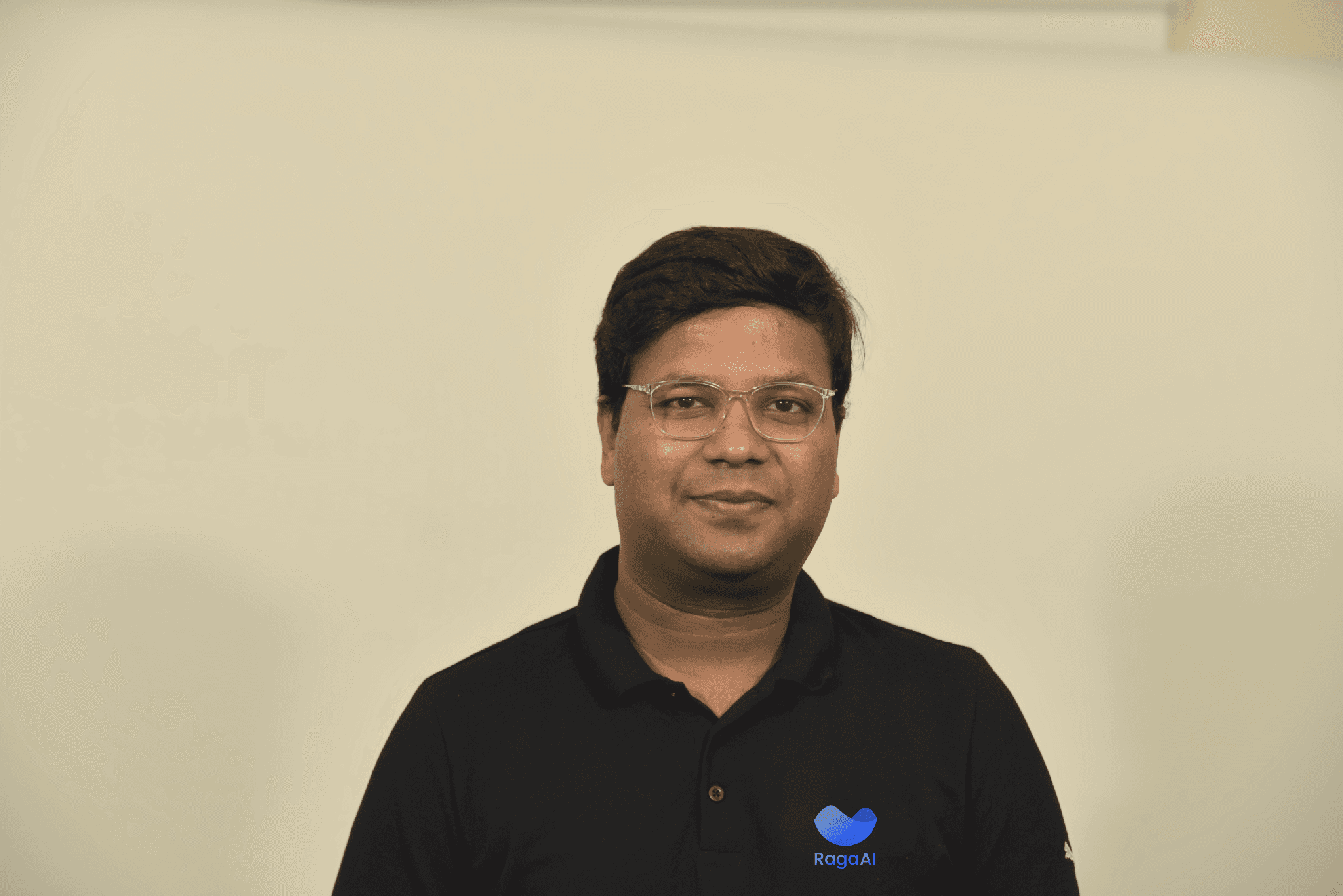
Jigar Gupta
Aug 28, 2024
Read the article
Understanding Data Fragmentation and Strategies to Overcome It
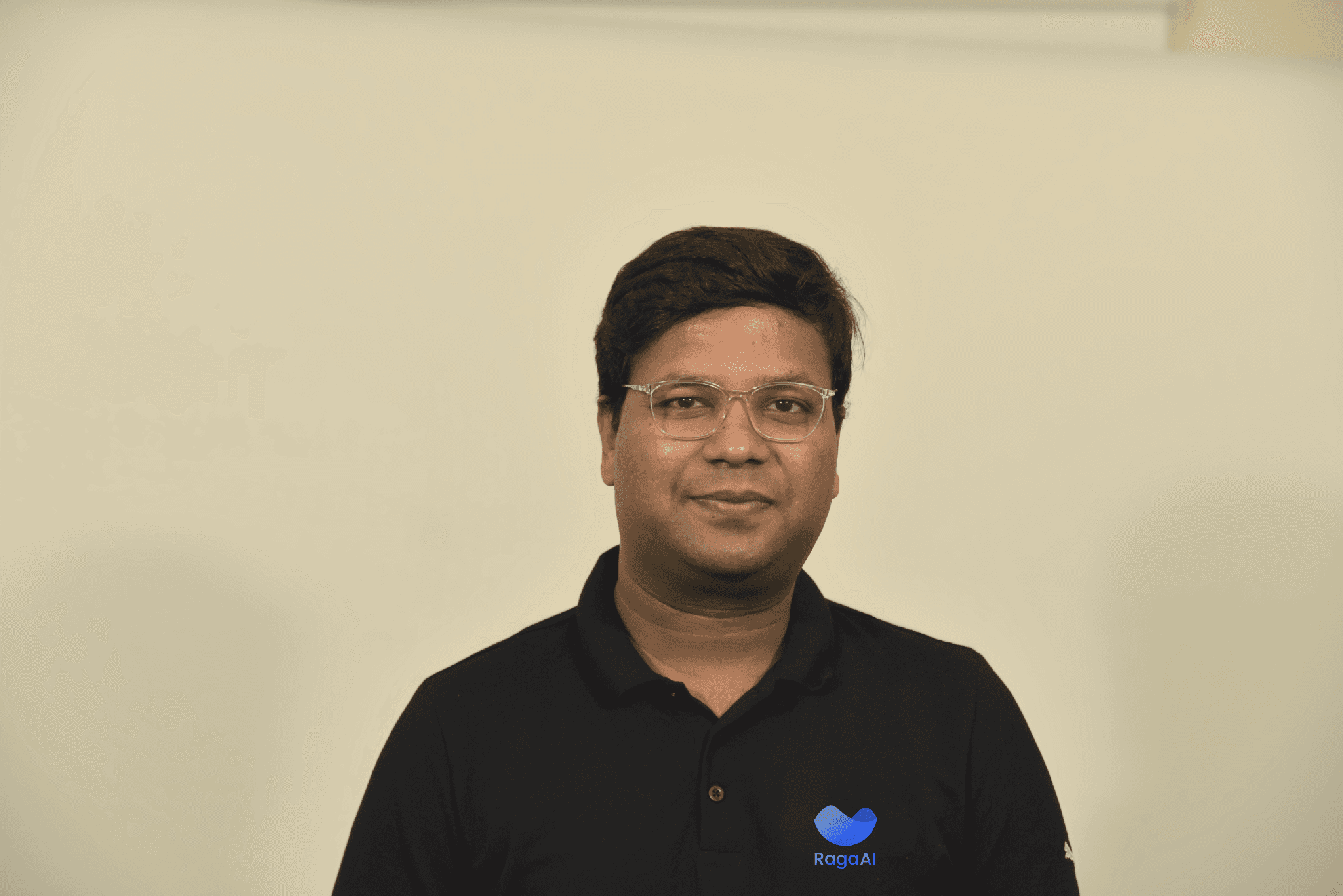
Jigar Gupta
Aug 28, 2024
Read the article
Understanding Techniques and Applications for Grounding LLMs in Data

Rehan Asif
Aug 28, 2024
Read the article
Advantages Of Using LLMs For Rapid Application Development

Rehan Asif
Aug 28, 2024
Read the article
Understanding React Agent in LangChain Engineering

Rehan Asif
Aug 28, 2024
Read the article
Using RagaAI Catalyst to Evaluate LLM Applications

Gaurav Agarwal
Aug 20, 2024
Read the article
Step-by-Step Guide on Training Large Language Models

Rehan Asif
Aug 19, 2024
Read the article
Understanding LLM Agent Architecture

Rehan Asif
Aug 19, 2024
Read the article
Understanding the Need and Possibilities of AI Guardrails Today
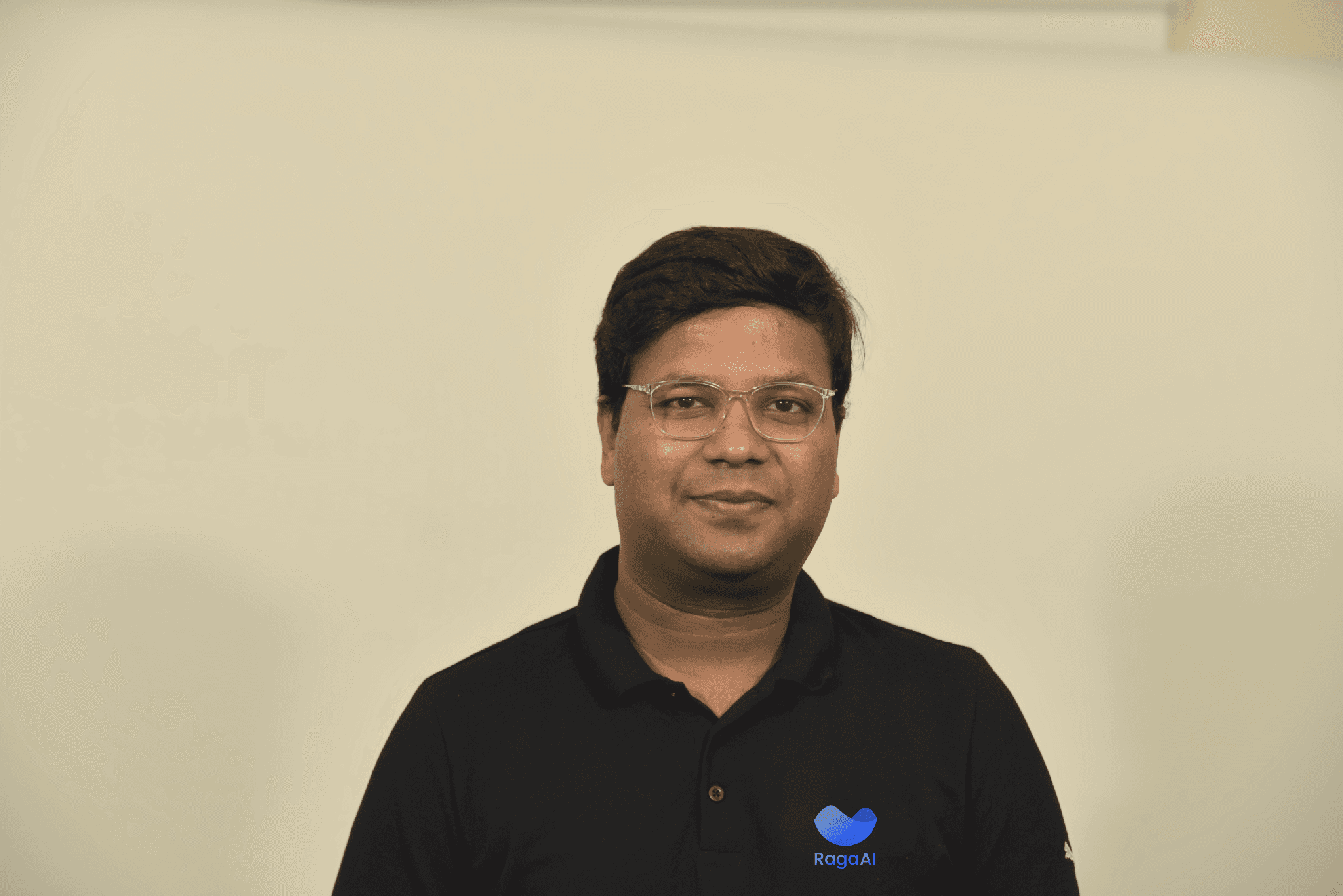
Jigar Gupta
Aug 19, 2024
Read the article
How to Prepare Quality Dataset for LLM Training

Rehan Asif
Aug 14, 2024
Read the article
Understanding Multi-Agent LLM Framework and Its Performance Scaling

Rehan Asif
Aug 15, 2024
Read the article
Understanding and Tackling Data Drift: Causes, Impact, and Automation Strategies
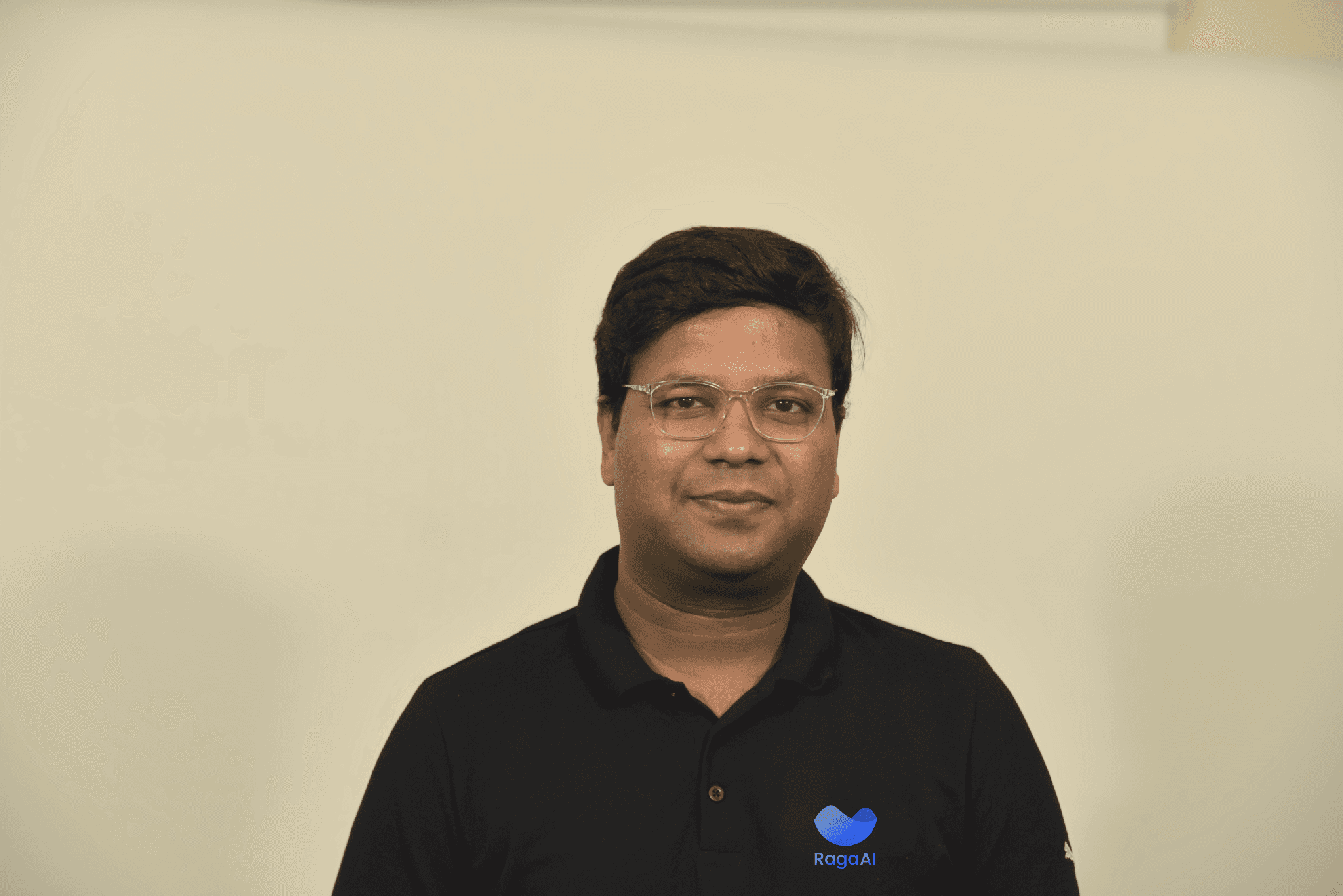
Jigar Gupta
Aug 14, 2024
Read the article
Introducing RagaAI Catalyst: Best in class automated LLM evaluation with 93% Human Alignment
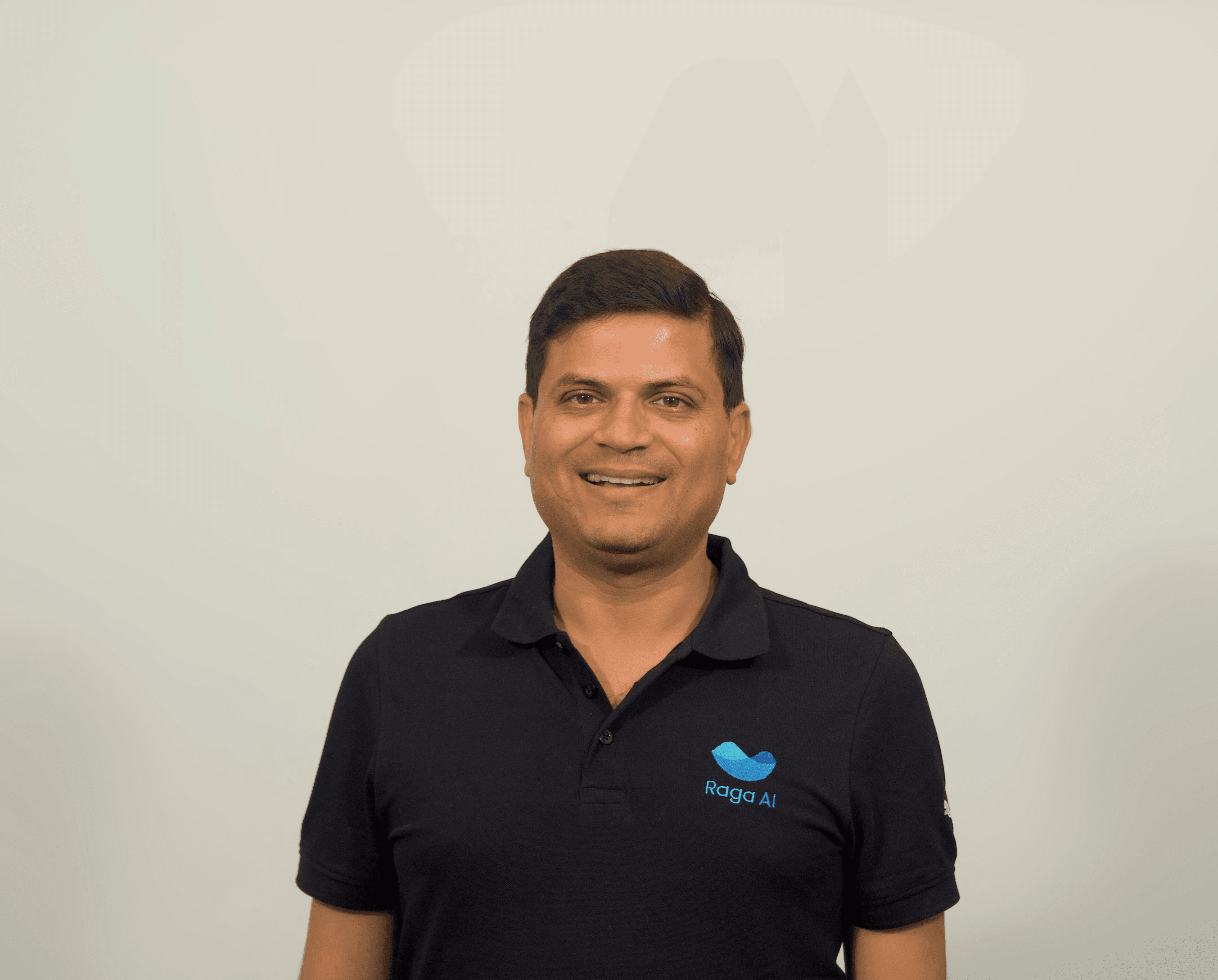
Gaurav Agarwal
Jul 15, 2024
Read the article
Key Pillars and Techniques for LLM Observability and Monitoring

Rehan Asif
Jul 24, 2024
Read the article
Introduction to What is LLM Agents and How They Work?

Rehan Asif
Jul 24, 2024
Read the article
Analysis of the Large Language Model Landscape Evolution

Rehan Asif
Jul 24, 2024
Read the article
Marketing Success With Retrieval Augmented Generation (RAG) Platforms
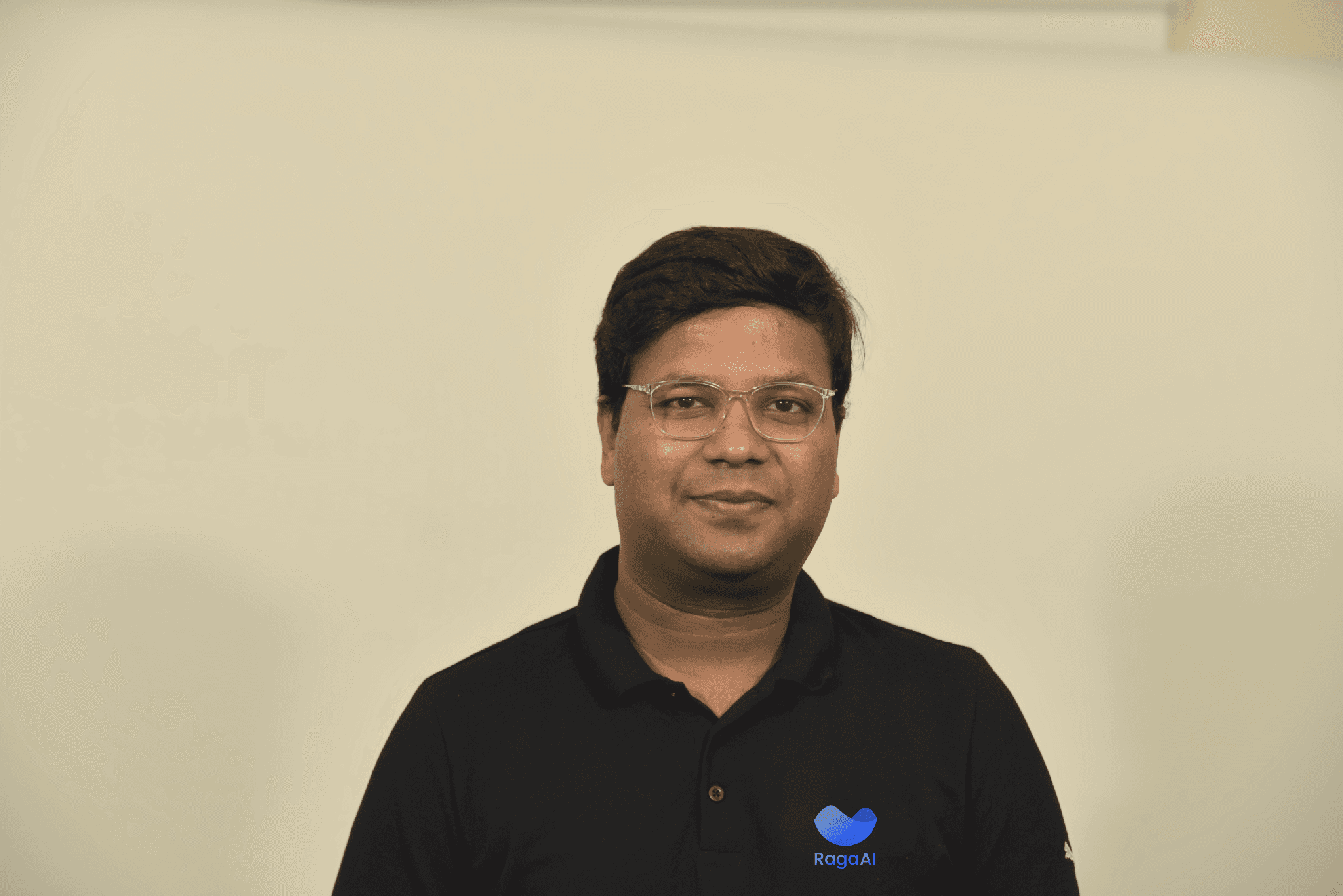
Jigar Gupta
Jul 24, 2024
Read the article
Developing AI Agent Strategies Using GPT
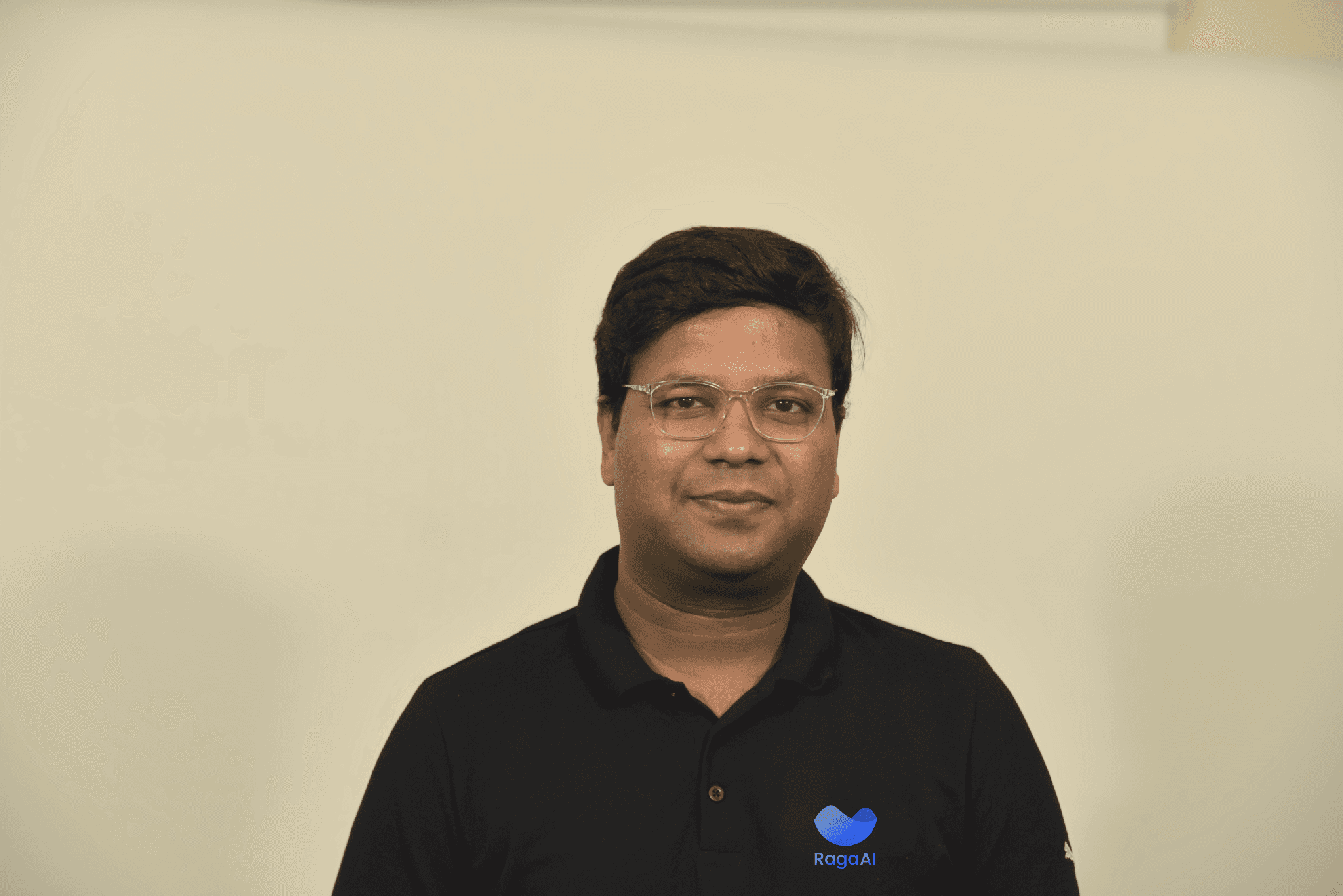
Jigar Gupta
Jul 24, 2024
Read the article
Identifying Triggers for Retraining AI Models to Maintain Performance
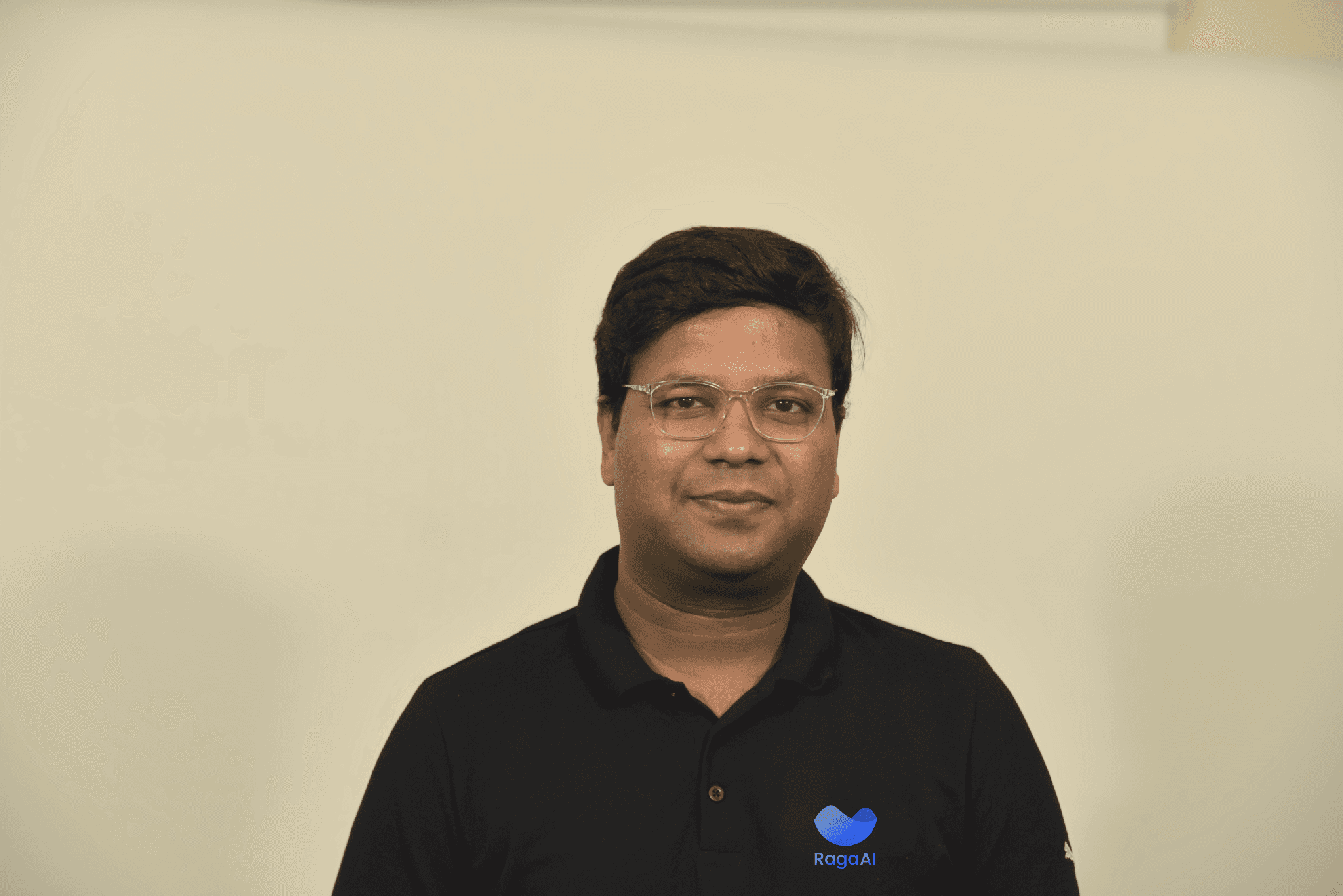
Jigar Gupta
Jul 16, 2024
Read the article
Agentic Design Patterns In LLM-Based Applications

Rehan Asif
Jul 16, 2024
Read the article
Generative AI And Document Question Answering With LLMs
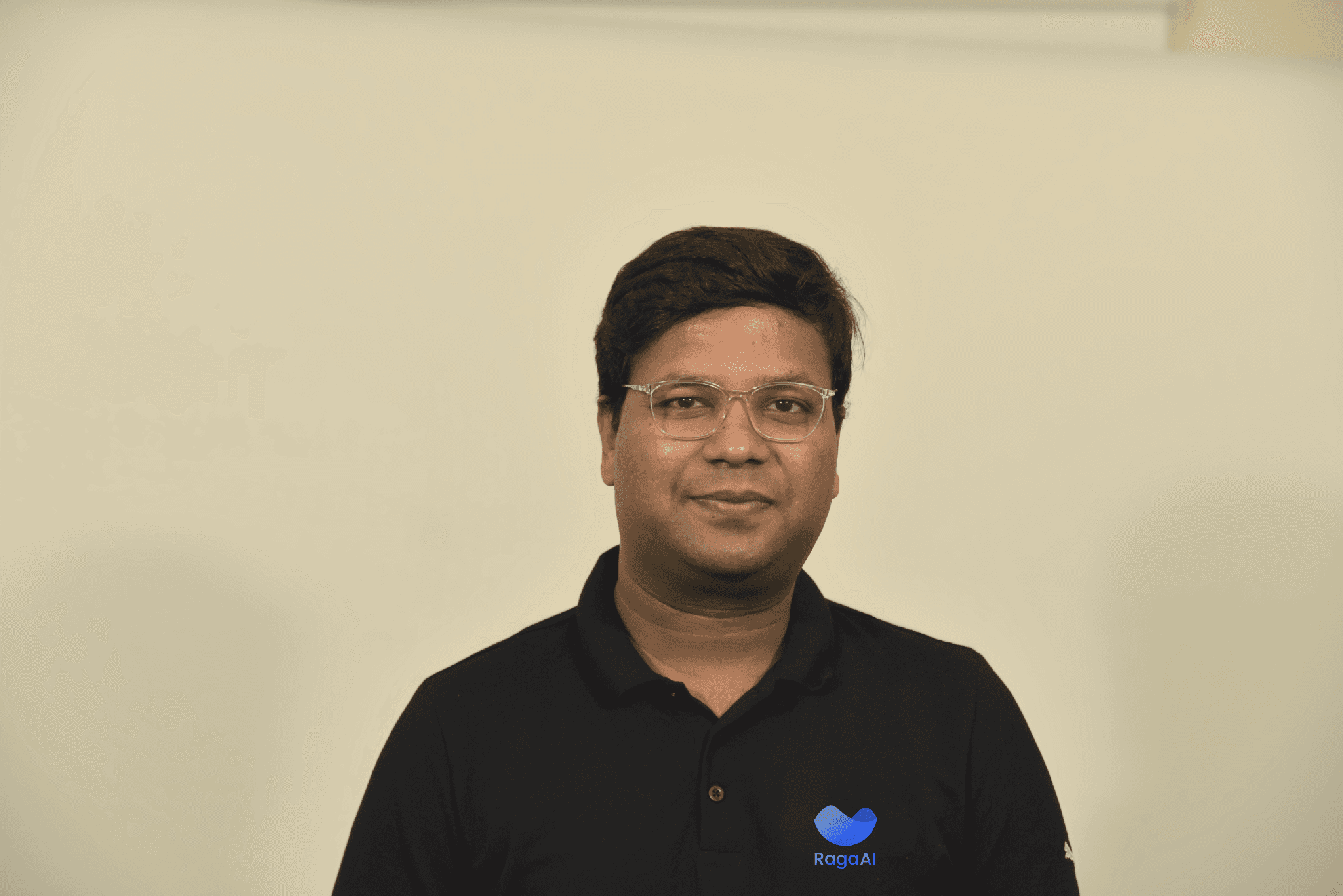
Jigar Gupta
Jul 15, 2024
Read the article
How to Fine-Tune ChatGPT for Your Use Case - Step by Step Guide
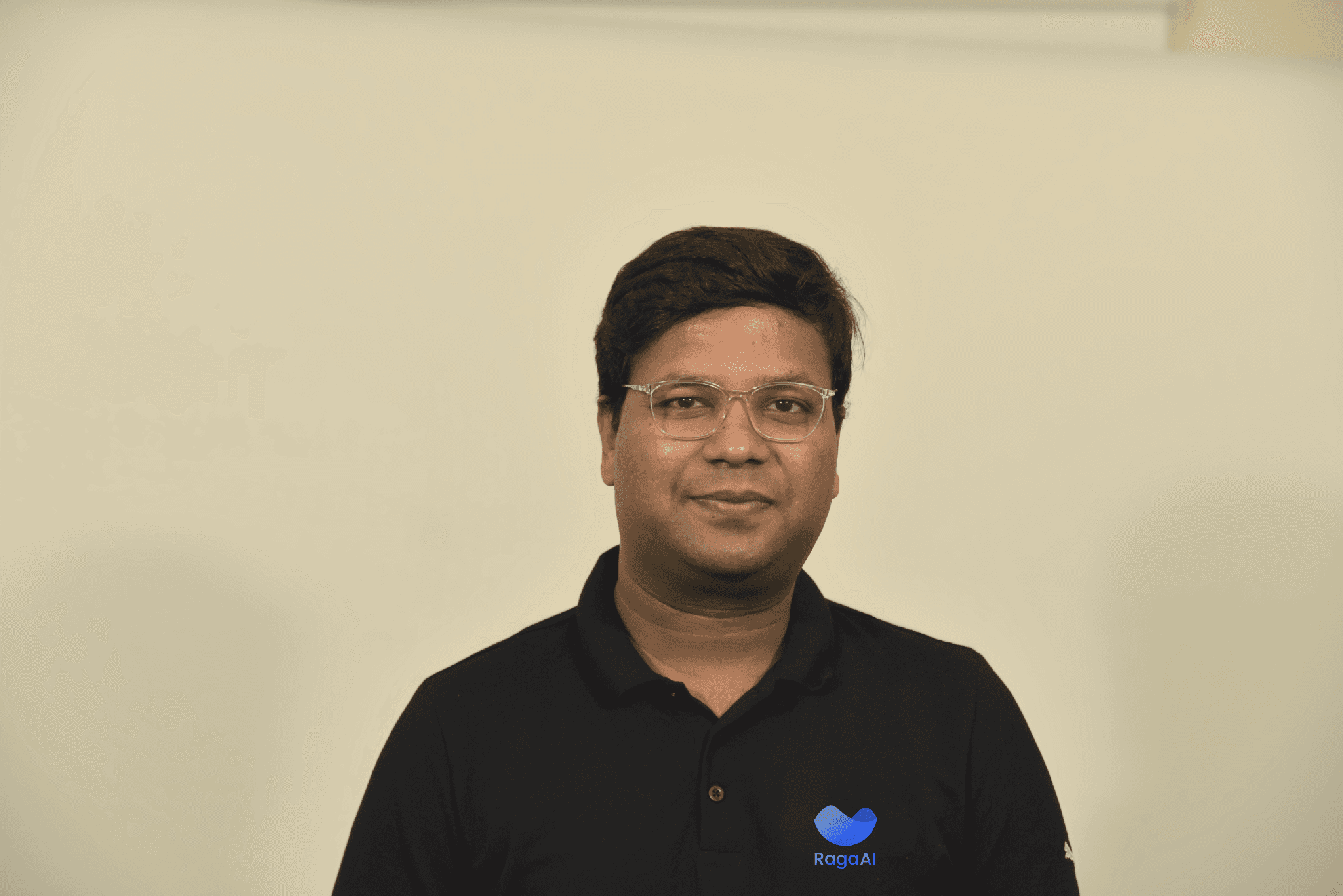
Jigar Gupta
Jul 15, 2024
Read the article
Security and LLM Firewall Controls

Rehan Asif
Jul 15, 2024
Read the article
Understanding the Use of Guardrail Metrics in Ensuring LLM Safety

Rehan Asif
Jul 13, 2024
Read the article
Exploring the Future of LLM and Generative AI Infrastructure

Rehan Asif
Jul 13, 2024
Read the article
Comprehensive Guide to RLHF and Fine Tuning LLMs from Scratch

Rehan Asif
Jul 13, 2024
Read the article
Using Synthetic Data To Enrich RAG Applications
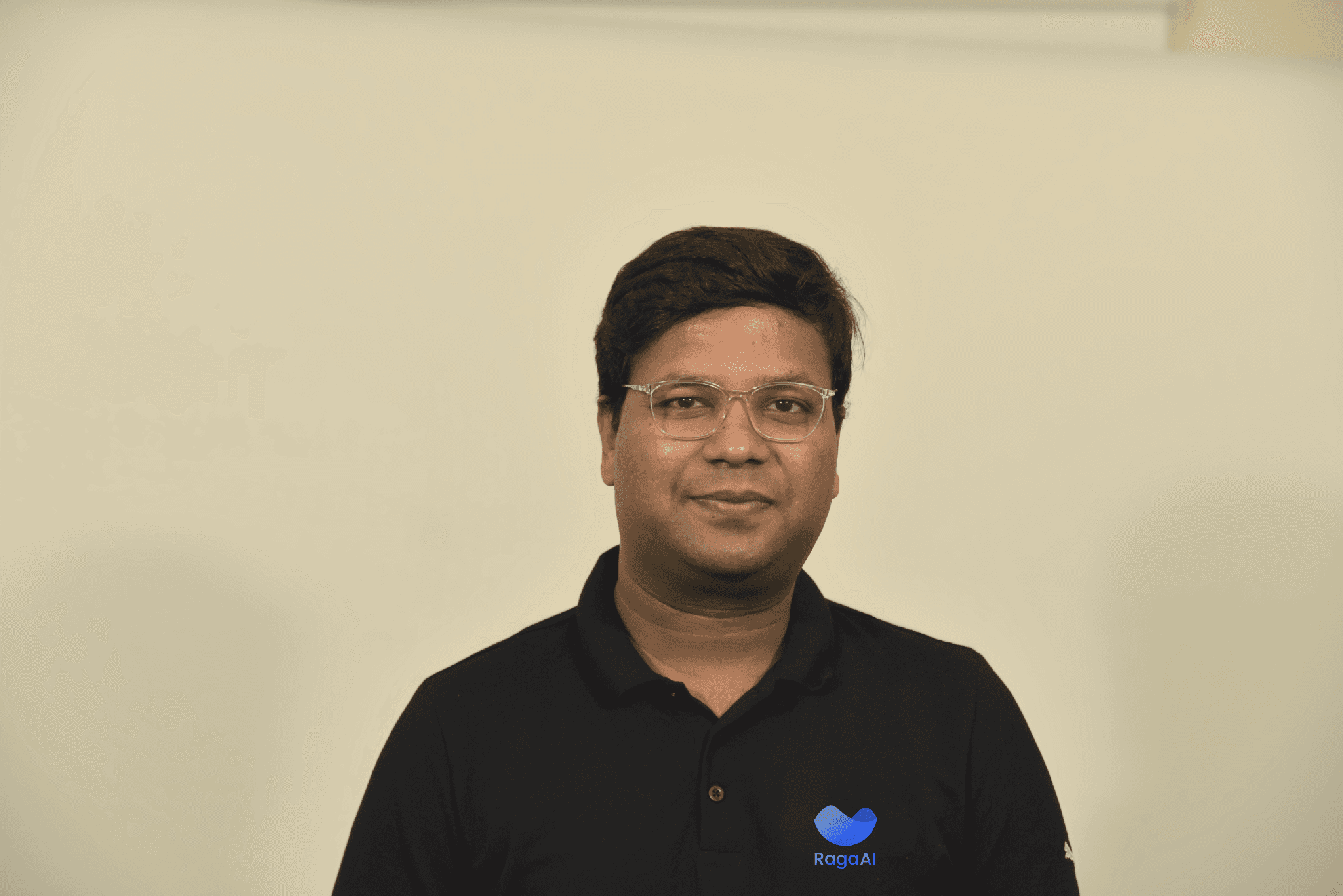
Jigar Gupta
Jul 13, 2024
Read the article
Comparing Different Large Language Model (LLM) Frameworks

Rehan Asif
Jul 12, 2024
Read the article
Integrating AI Models with Continuous Integration Systems
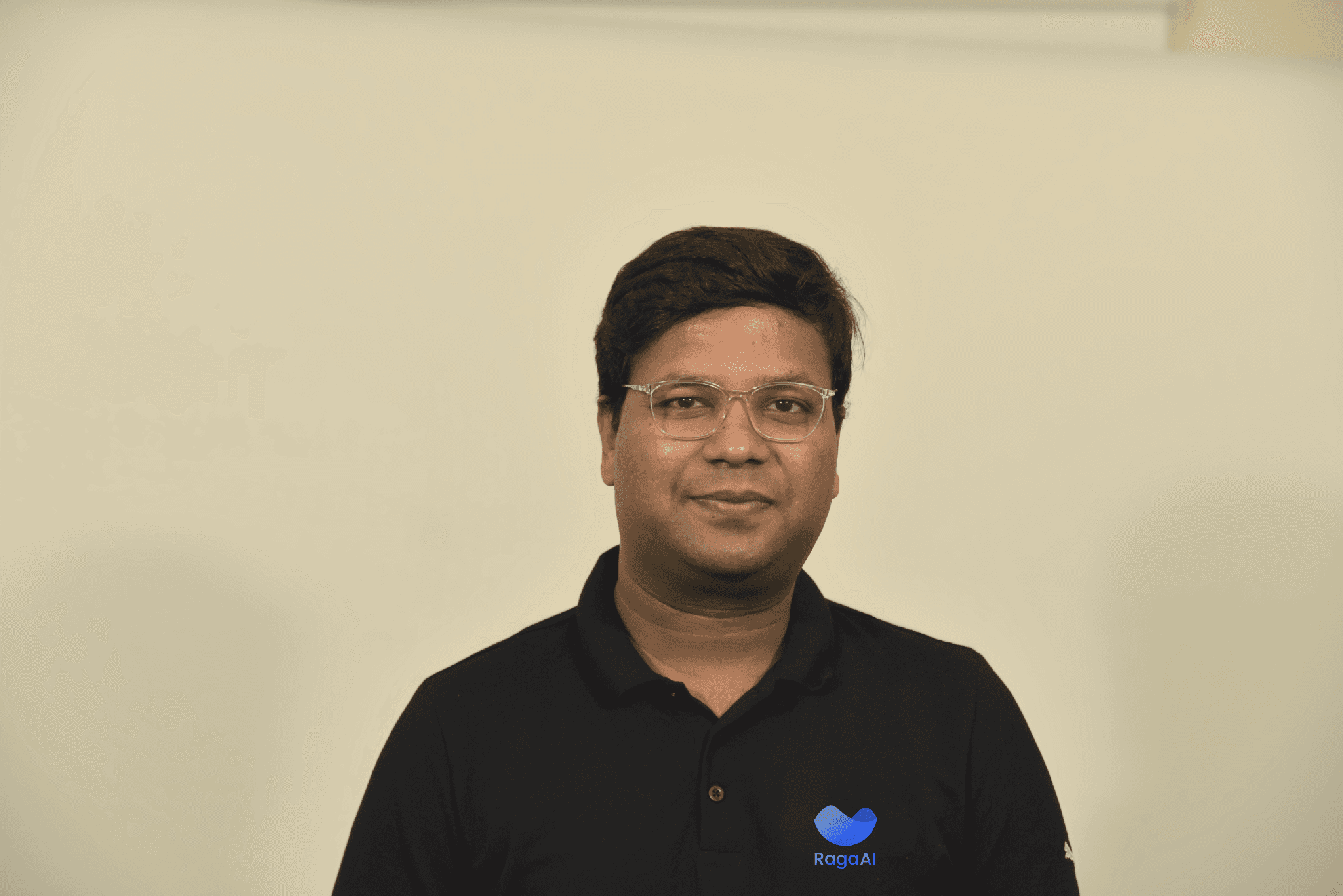
Jigar Gupta
Jul 12, 2024
Read the article
Understanding Retrieval Augmented Generation for Large Language Models: A Survey
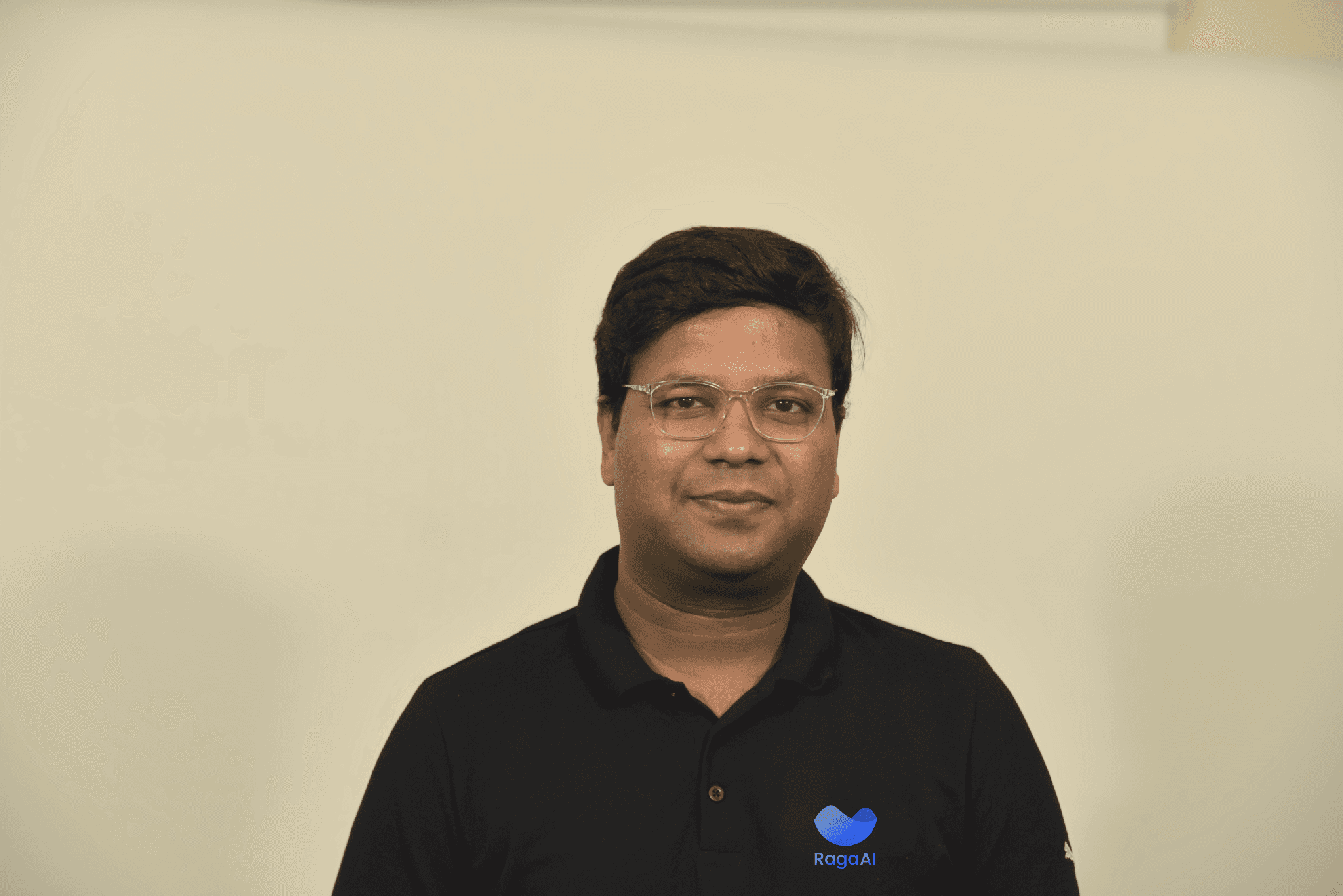
Jigar Gupta
Jul 12, 2024
Read the article
Leveraging AI For Enhanced Retail Customer Experiences
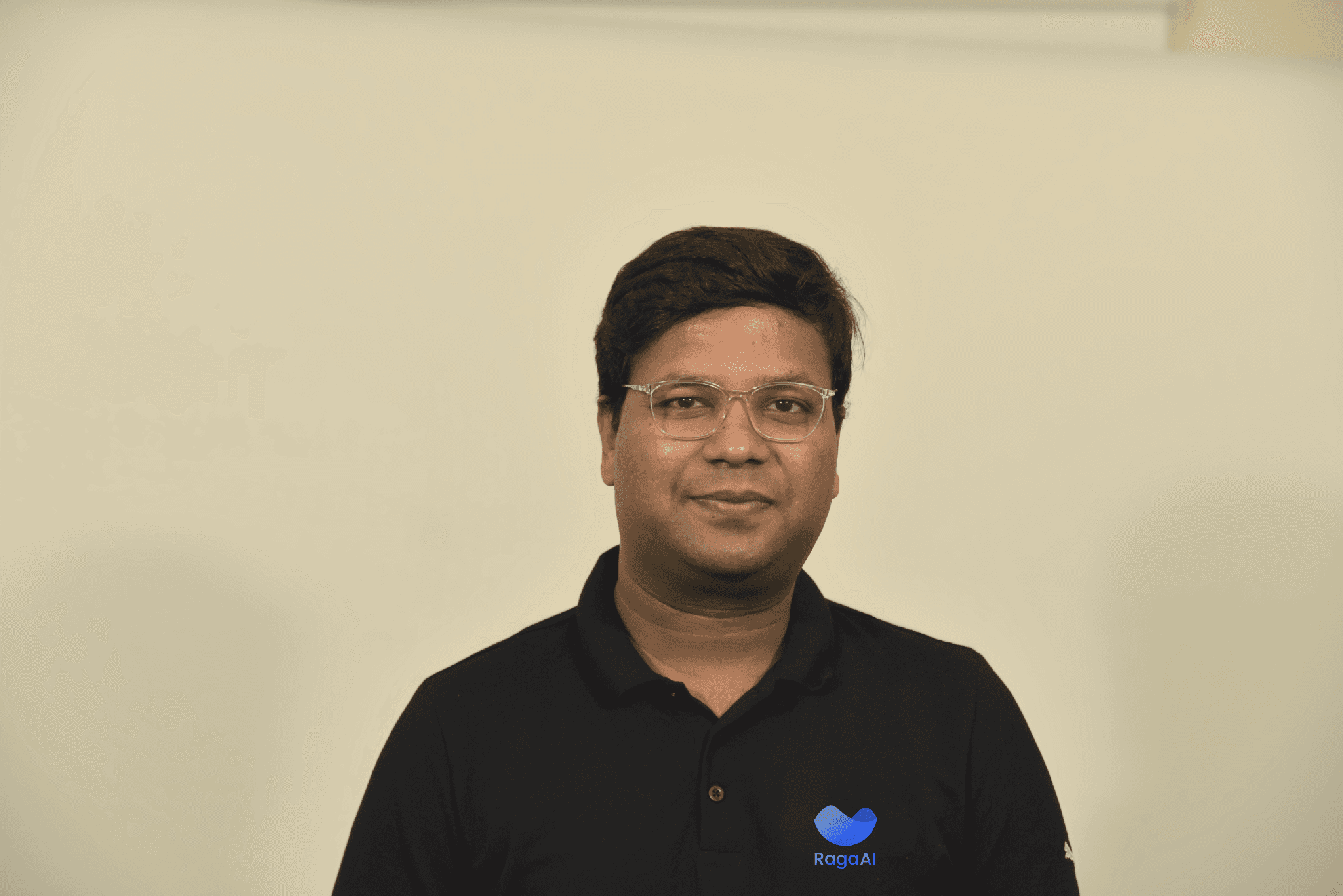
Jigar Gupta
Jul 1, 2024
Read the article
Enhancing Enterprise Search Using RAG and LLMs

Rehan Asif
Jul 1, 2024
Read the article
Importance of Accuracy and Reliability in Tabular Data Models
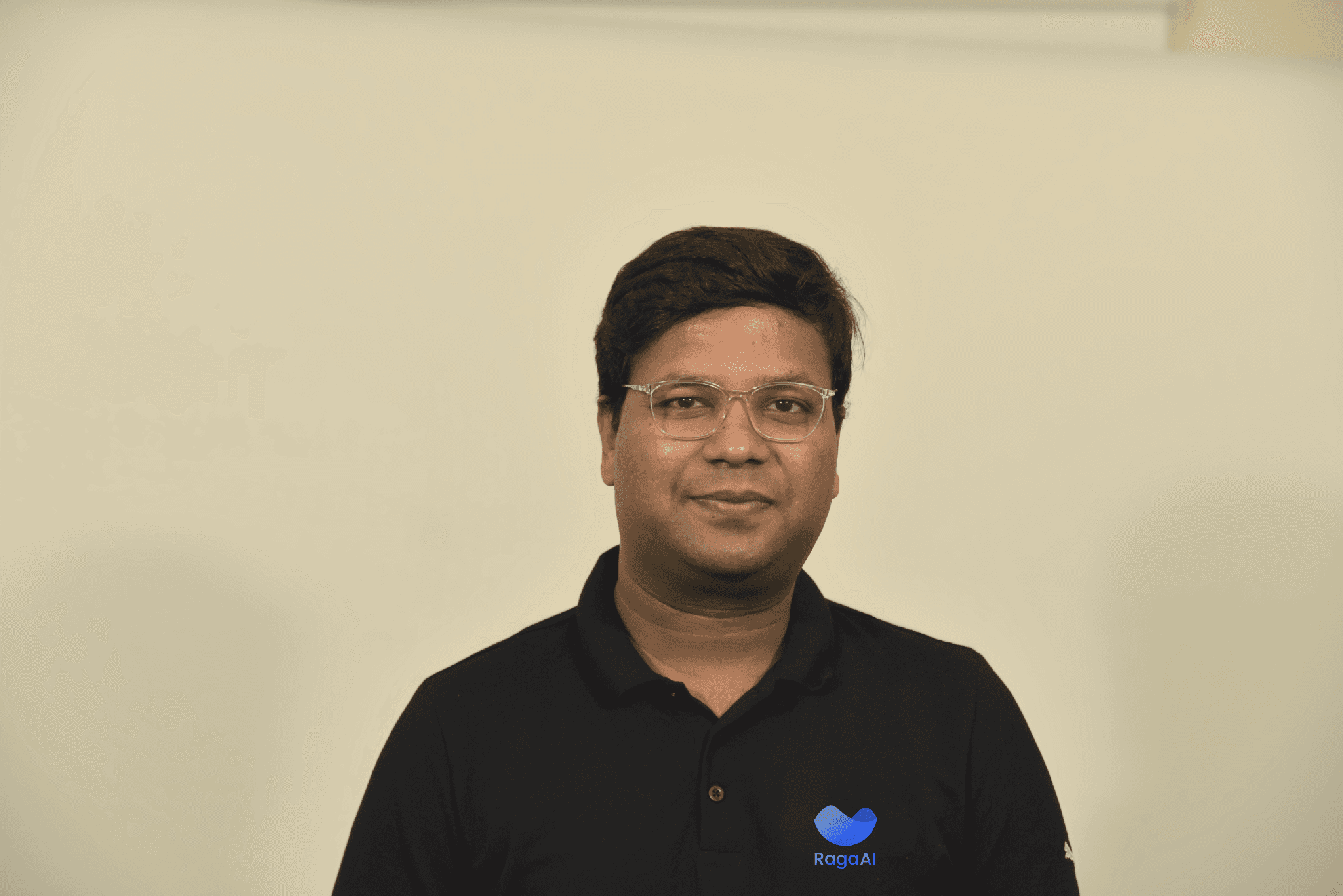
Jigar Gupta
Jul 1, 2024
Read the article
Information Retrieval And LLMs: RAG Explained

Rehan Asif
Jul 1, 2024
Read the article
Introduction to LLM Powered Autonomous Agents

Rehan Asif
Jul 1, 2024
Read the article
Guide on Unified Multi-Dimensional LLM Evaluation and Benchmark Metrics

Rehan Asif
Jul 1, 2024
Read the article
Innovations In AI For Healthcare
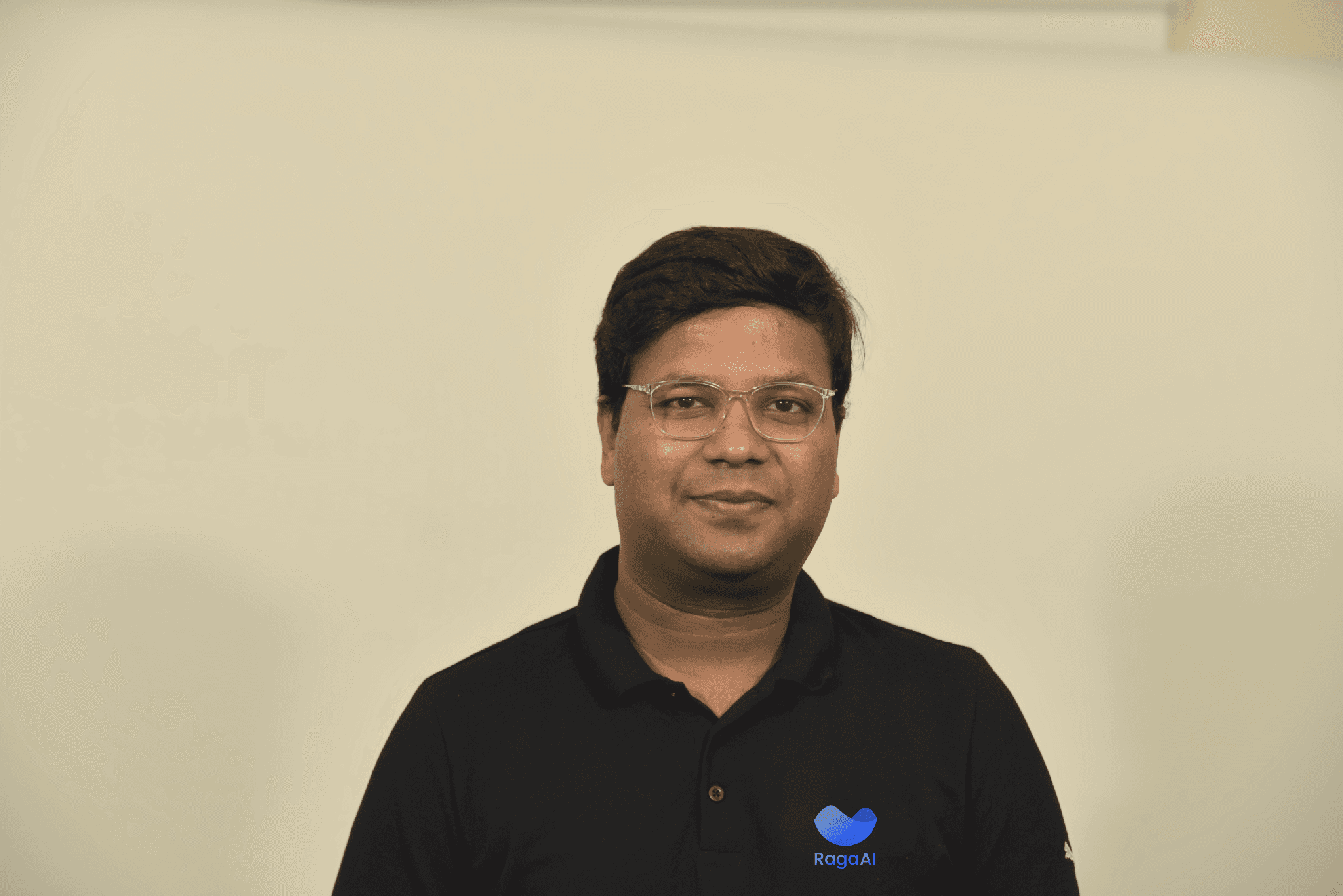
Jigar Gupta
Jun 24, 2024
Read the article
Implementing AI-Driven Inventory Management For The Retail Industry
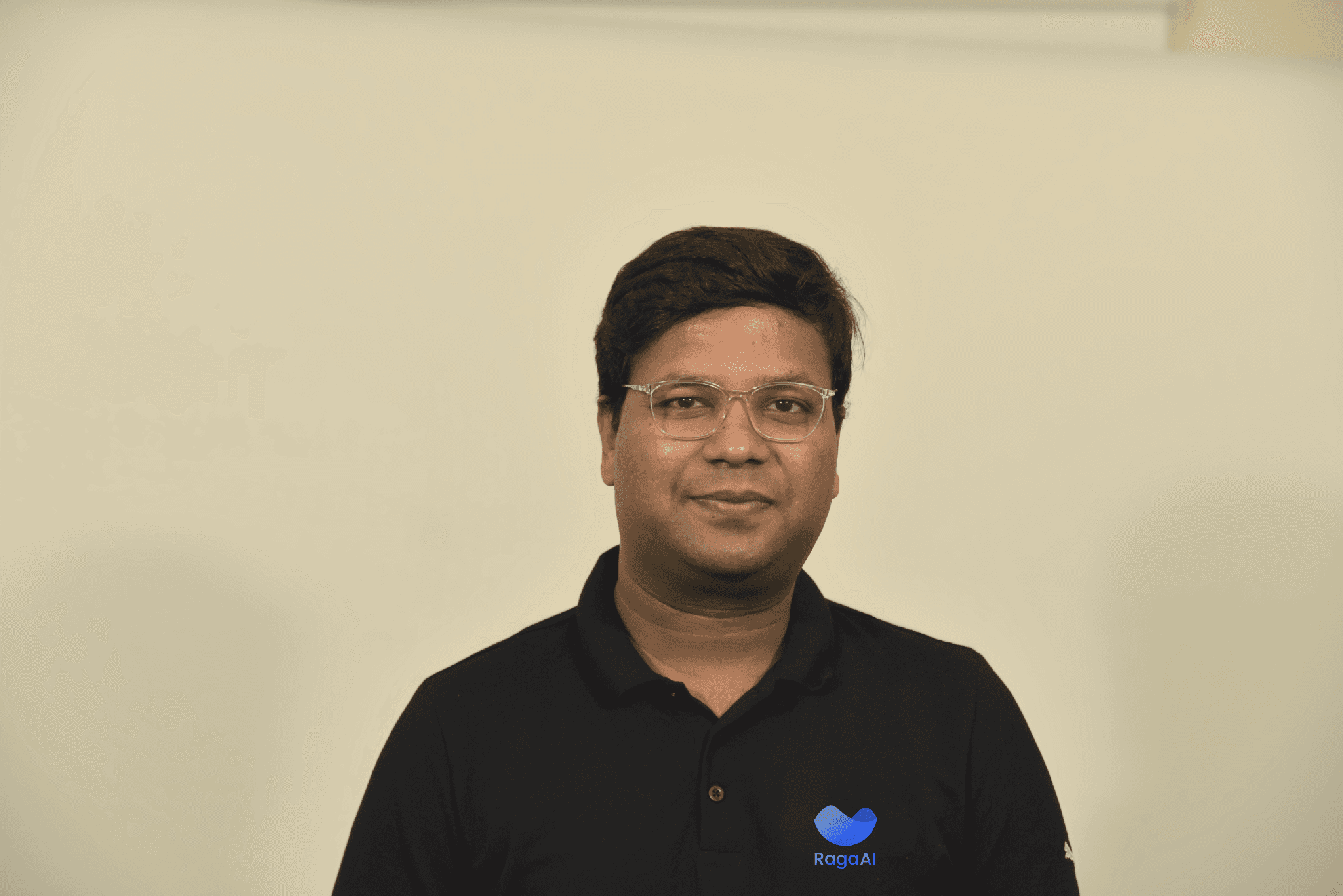
Jigar Gupta
Jun 24, 2024
Read the article
Practical Retrieval Augmented Generation: Use Cases And Impact
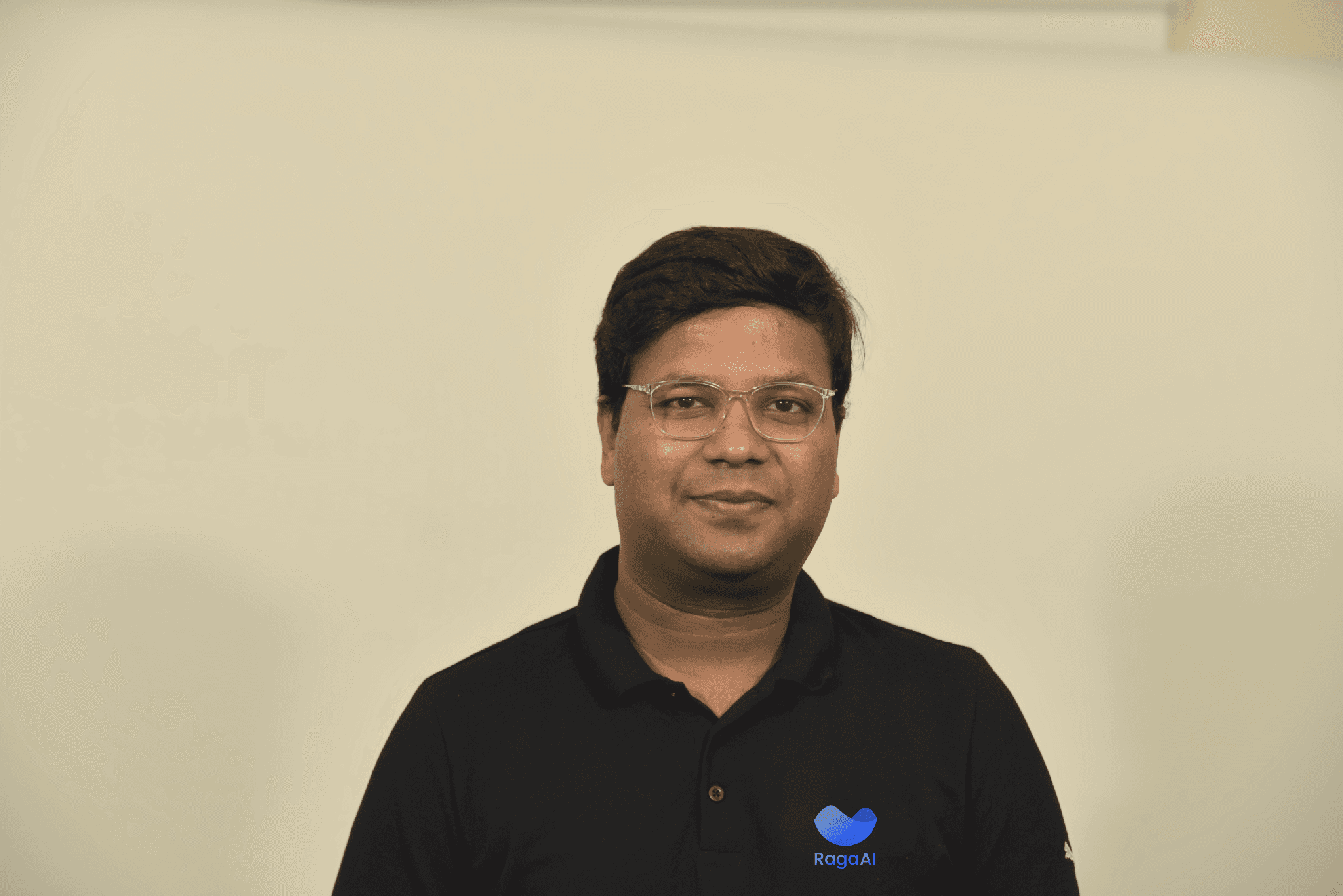
Jigar Gupta
Jun 24, 2024
Read the article
LLM Pre-Training and Fine-Tuning Differences

Rehan Asif
Jun 23, 2024
Read the article
20 LLM Project Ideas For Beginners Using Large Language Models

Rehan Asif
Jun 23, 2024
Read the article
Understanding LLM Parameters: Tuning Top-P, Temperature And Tokens

Rehan Asif
Jun 23, 2024
Read the article
Understanding Large Action Models In AI

Rehan Asif
Jun 23, 2024
Read the article
Building And Implementing Custom LLM Guardrails

Rehan Asif
Jun 12, 2024
Read the article
Understanding LLM Alignment: A Simple Guide

Rehan Asif
Jun 12, 2024
Read the article
Practical Strategies For Self-Hosting Large Language Models

Rehan Asif
Jun 12, 2024
Read the article
Practical Guide For Deploying LLMs In Production

Rehan Asif
Jun 12, 2024
Read the article
The Impact Of Generative Models On Content Creation
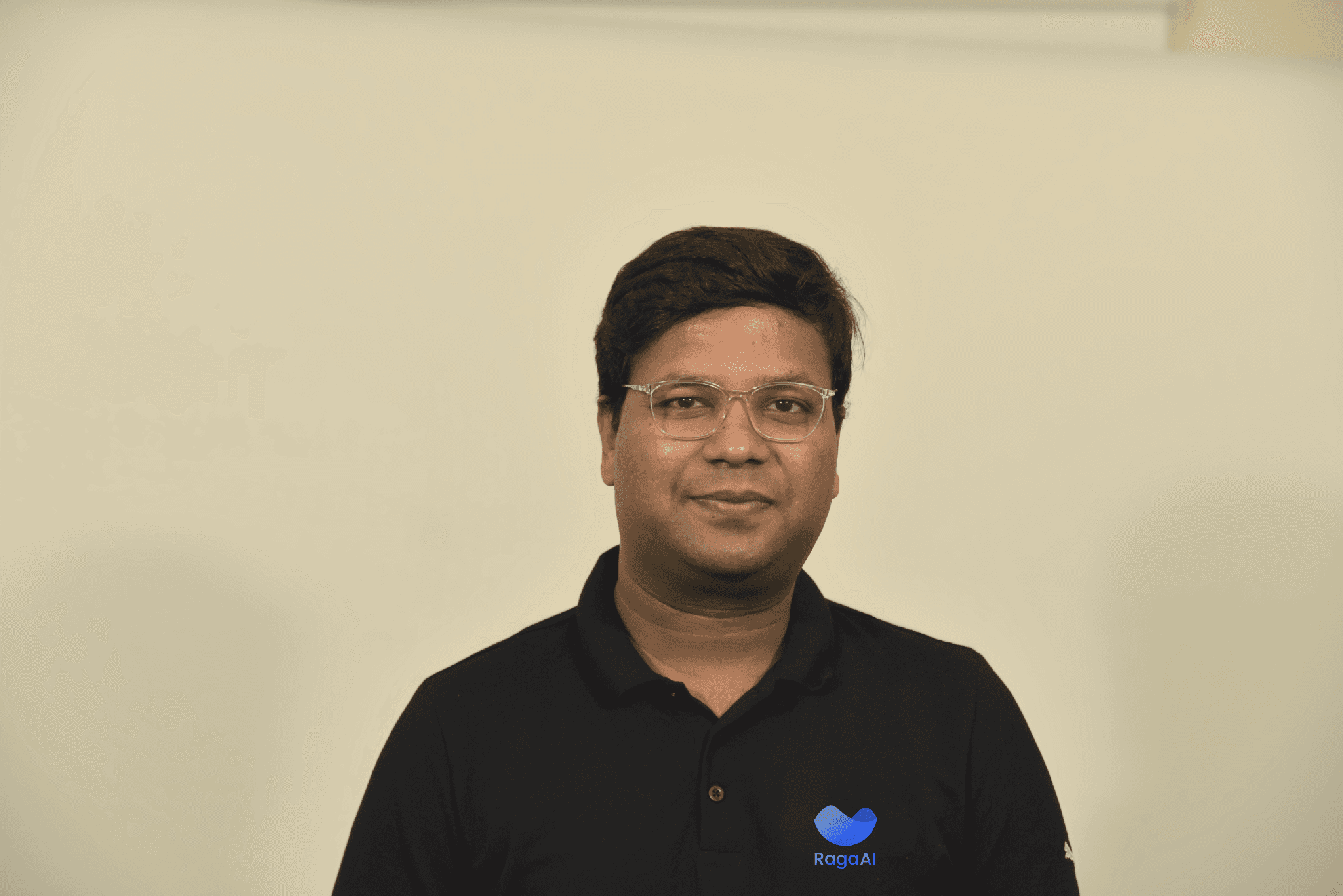
Jigar Gupta
Jun 12, 2024
Read the article
Implementing Regression Tests In AI Development
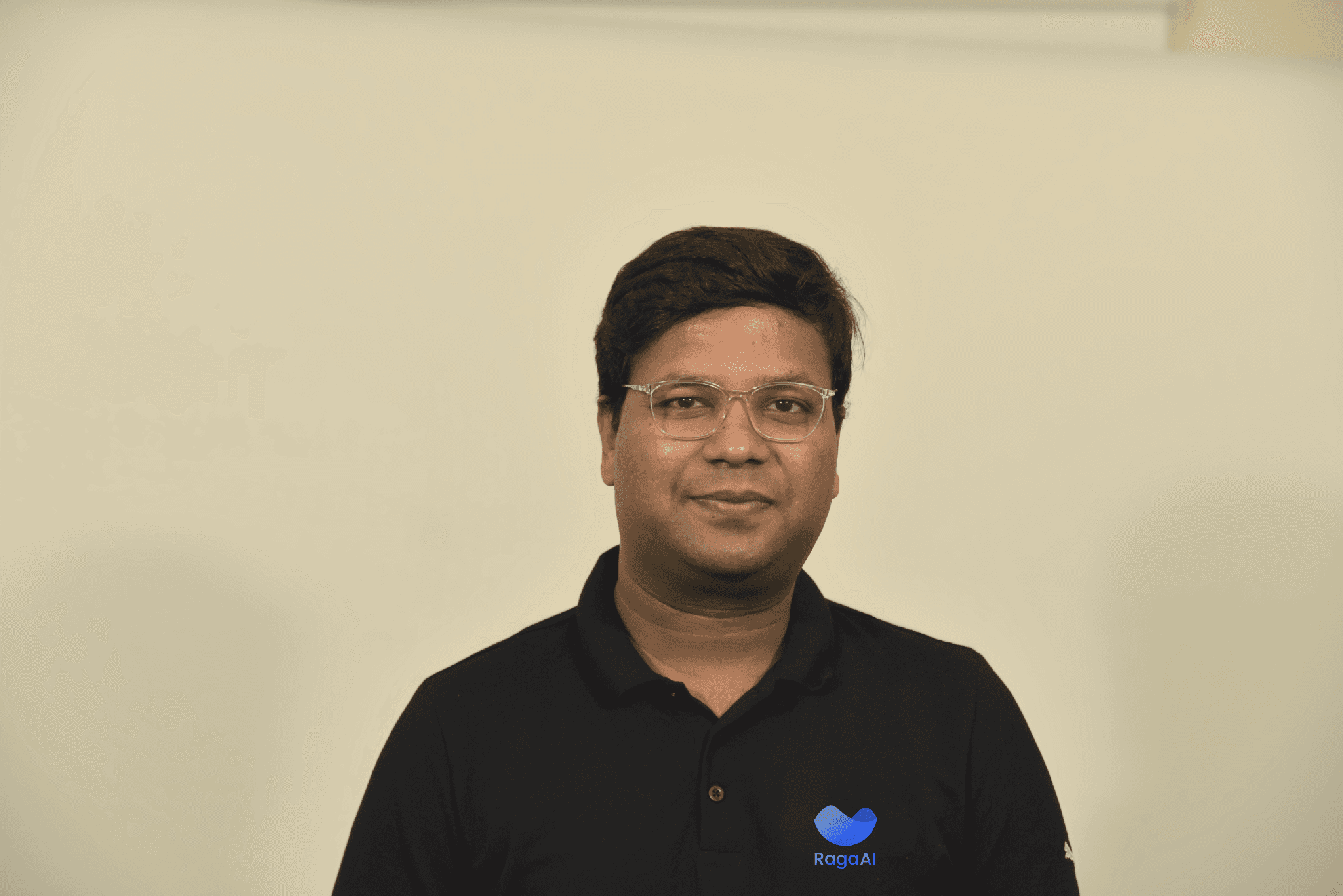
Jigar Gupta
Jun 12, 2024
Read the article
In-Depth Case Studies in AI Model Testing: Exploring Real-World Applications and Insights
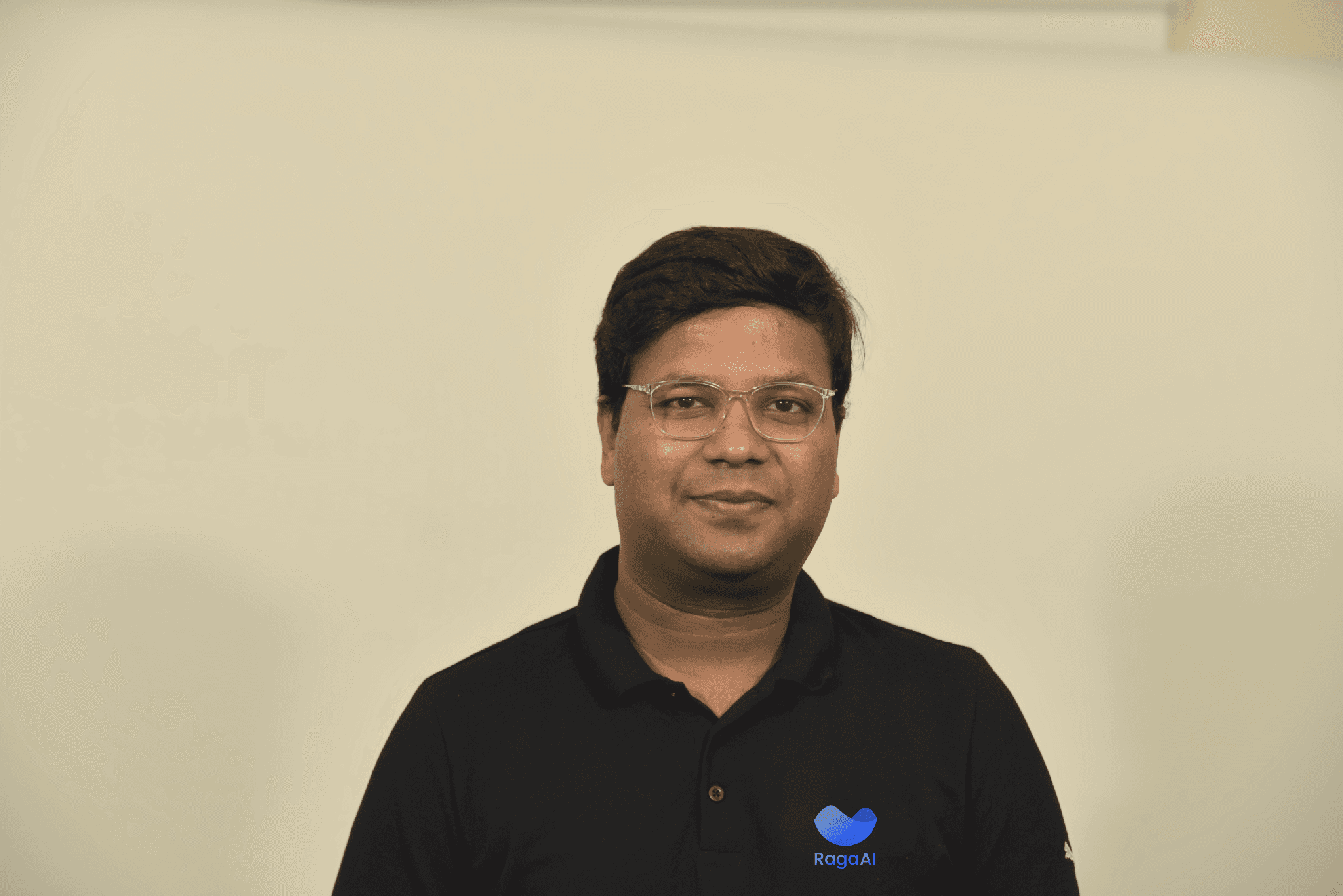
Jigar Gupta
Jun 11, 2024
Read the article
Techniques and Importance of Stress Testing AI Systems
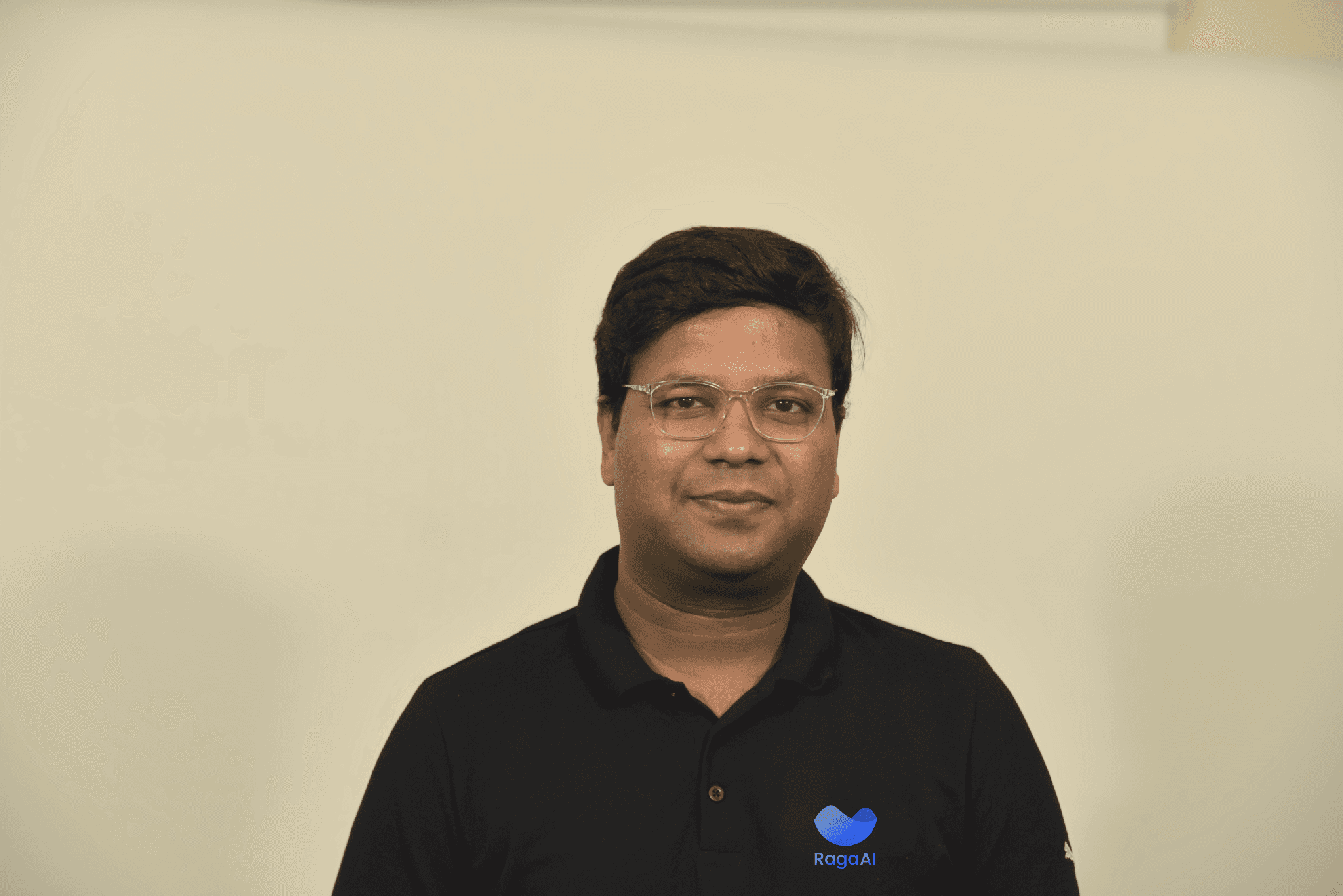
Jigar Gupta
Jun 11, 2024
Read the article
Navigating Global AI Regulations and Standards

Rehan Asif
Jun 10, 2024
Read the article
The Cost of Errors In AI Application Development

Rehan Asif
Jun 10, 2024
Read the article
Best Practices In Data Governance For AI

Rehan Asif
Jun 10, 2024
Read the article
Success Stories And Case Studies Of AI Adoption Across Industries
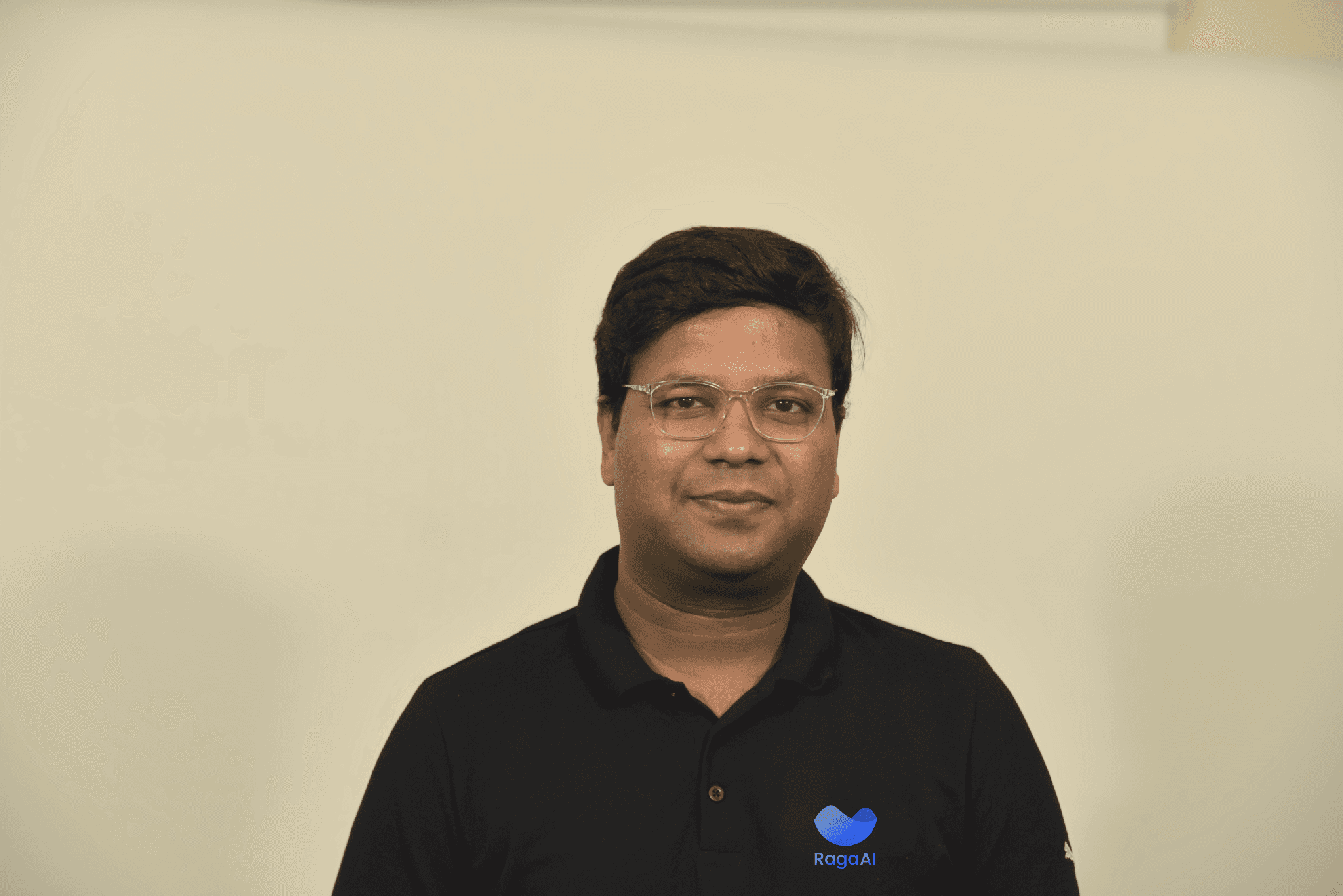
Jigar Gupta
May 1, 2024
Read the article
Exploring The Frontiers Of Deep Learning Applications
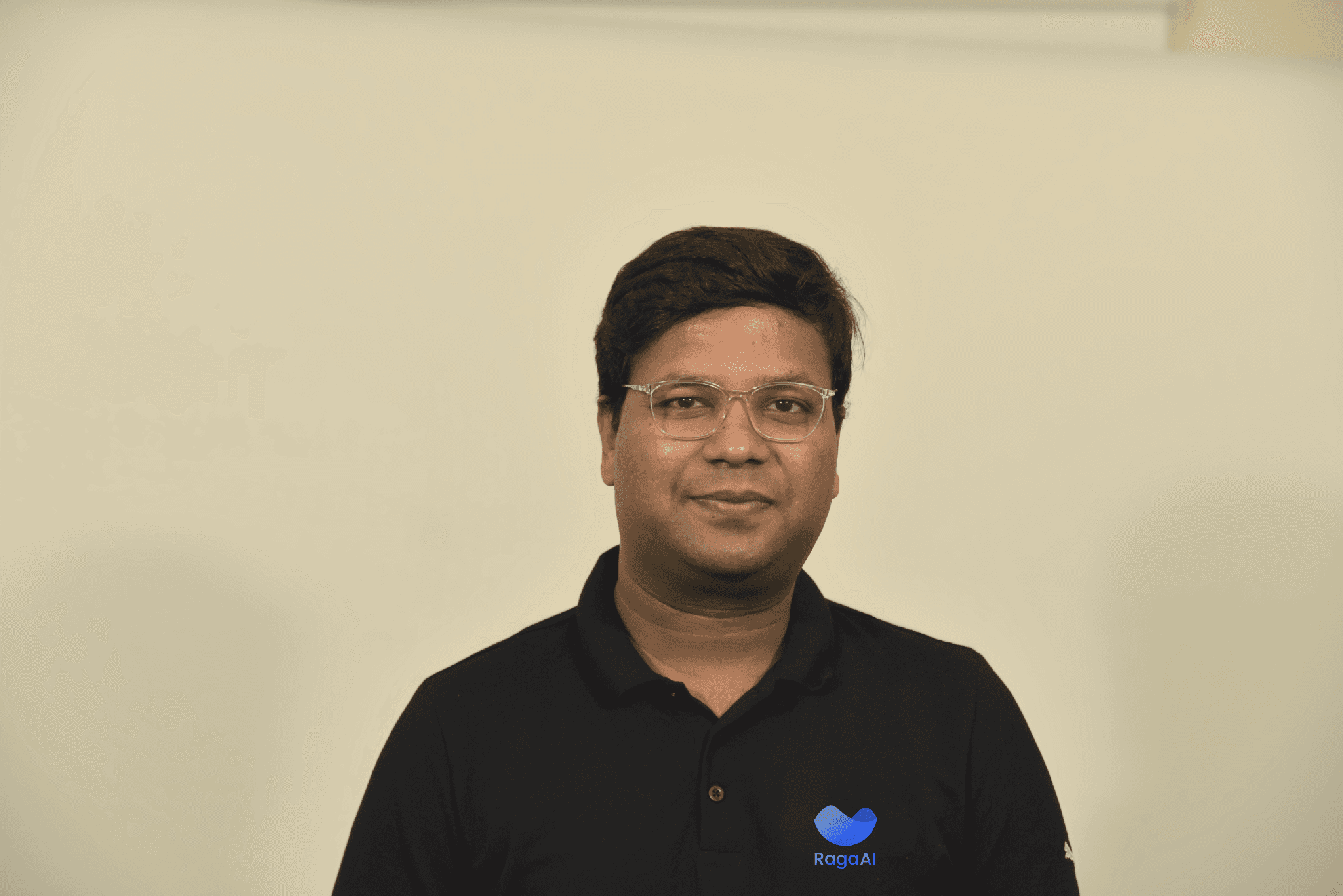
Jigar Gupta
May 1, 2024
Read the article
Integration Of RAG Platforms With Existing Enterprise Systems
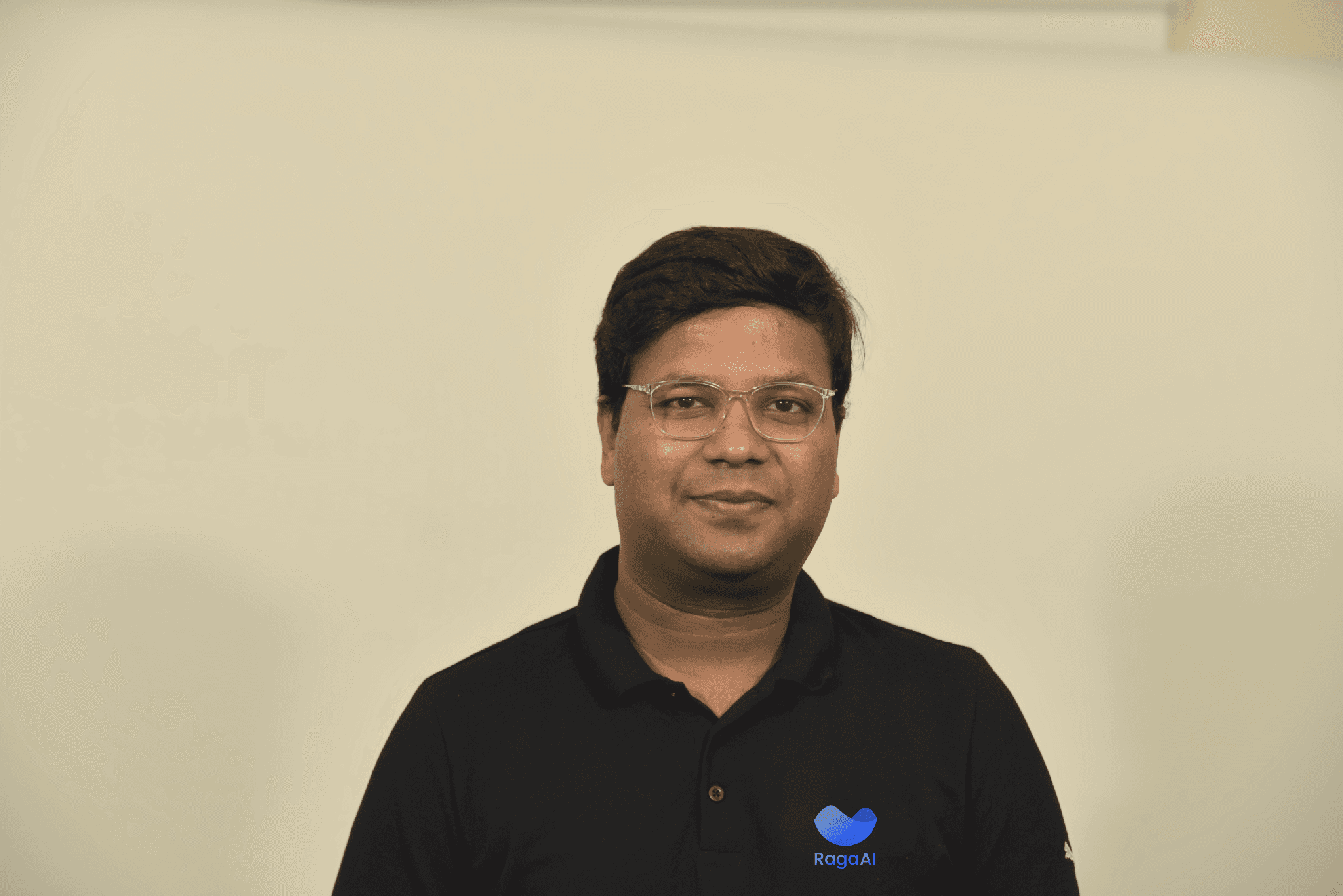
Jigar Gupta
Apr 30, 2024
Read the article
Multimodal LLMS Using Image And Text

Rehan Asif
Apr 30, 2024
Read the article
Understanding ML Model Monitoring In Production

Rehan Asif
Apr 30, 2024
Read the article
Strategic Approach To Testing AI-Powered Applications And Systems

Rehan Asif
Apr 30, 2024
Read the article
Navigating GDPR Compliance for AI Applications

Rehan Asif
Apr 26, 2024
Read the article
The Impact of AI Governance on Innovation and Development Speed

Rehan Asif
Apr 26, 2024
Read the article
Best Practices For Testing Computer Vision Models
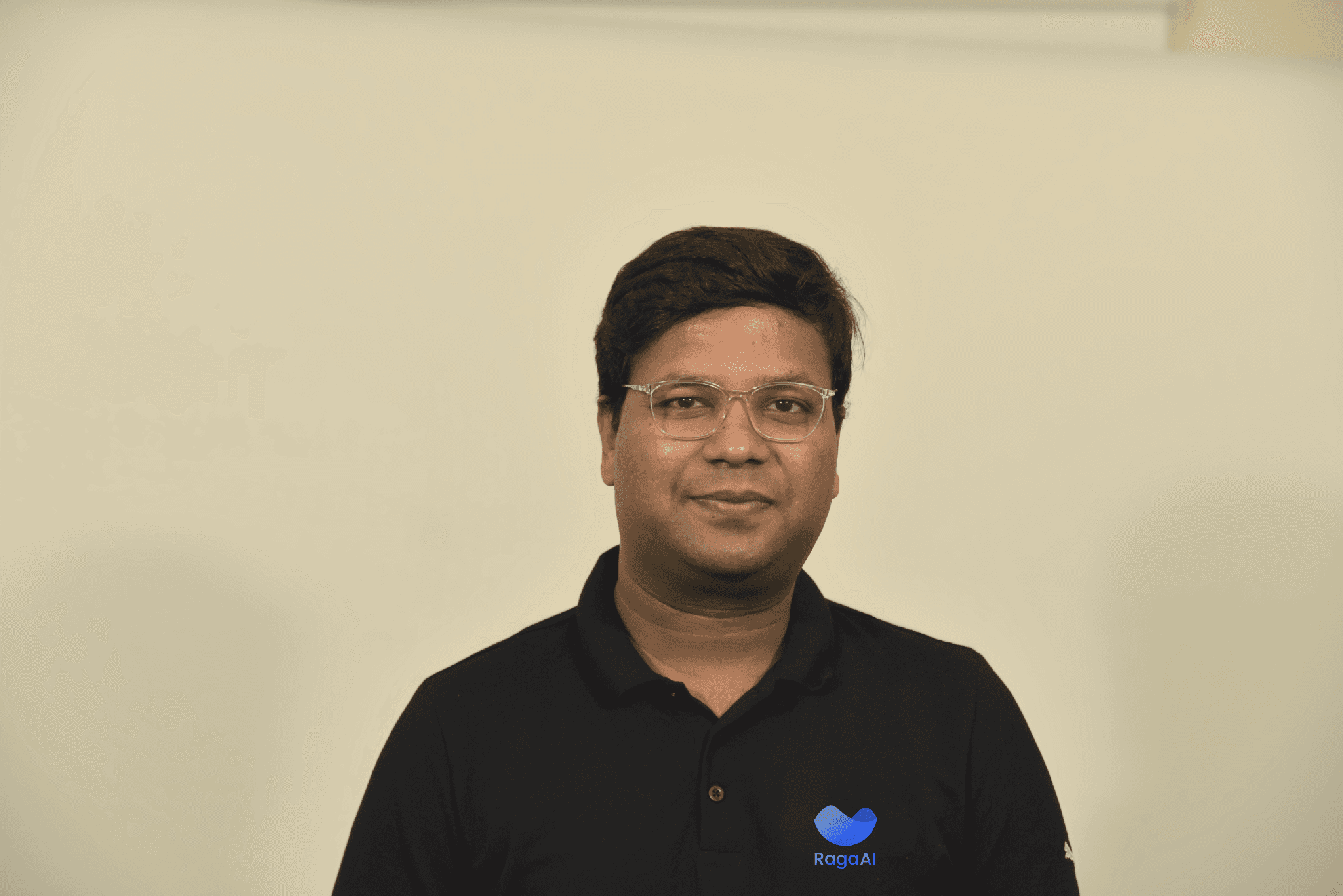
Jigar Gupta
Apr 25, 2024
Read the article
Building Low-Code LLM Apps with Visual Programming

Rehan Asif
Apr 26, 2024
Read the article
Understanding AI regulations In Finance

Akshat Gupta
Apr 26, 2024
Read the article
Compliance Automation: Getting Started with Regulatory Management

Akshat Gupta
Apr 25, 2024
Read the article
Practical Guide to Fine-Tuning OpenAI GPT Models Using Python

Rehan Asif
Apr 24, 2024
Read the article
Comparing Different Large Language Models (LLM)

Rehan Asif
Apr 23, 2024
Read the article
Evaluating Large Language Models: Methods And Metrics

Rehan Asif
Apr 22, 2024
Read the article
Significant AI Errors, Mistakes, Failures, and Flaws Companies Encounter

Akshat Gupta
Apr 21, 2024
Read the article
Challenges and Strategies for Implementing Enterprise LLM

Rehan Asif
Apr 20, 2024
Read the article
Enhancing Computer Vision with Synthetic Data: Advantages and Generation Techniques
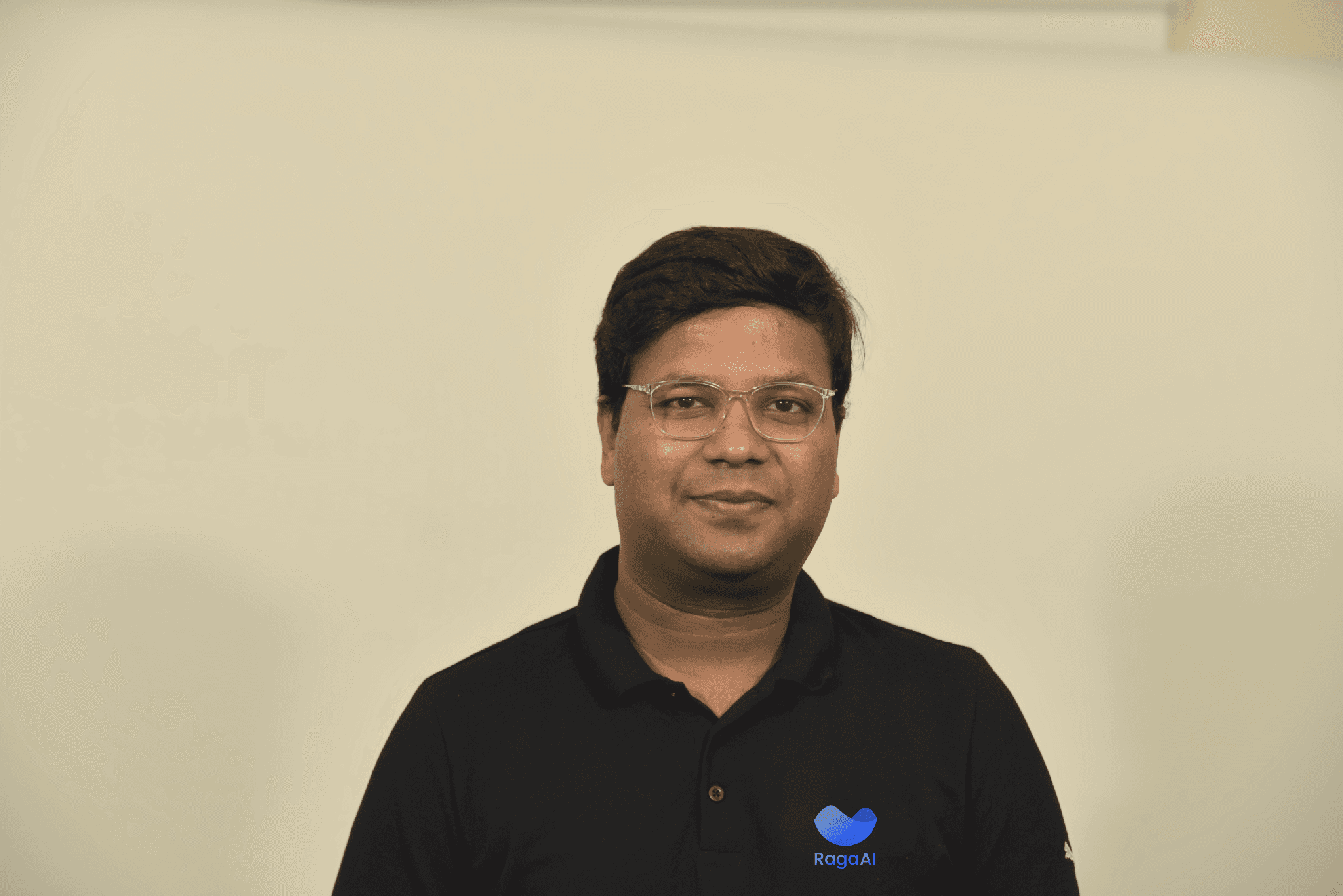
Jigar Gupta
Apr 20, 2024
Read the article
Building Trust In Artificial Intelligence Systems

Akshat Gupta
Apr 19, 2024
Read the article
A Brief Guide To LLM Parameters: Tuning and Optimization

Rehan Asif
Apr 18, 2024
Read the article
Unlocking The Potential Of Computer Vision Testing: Key Techniques And Tools
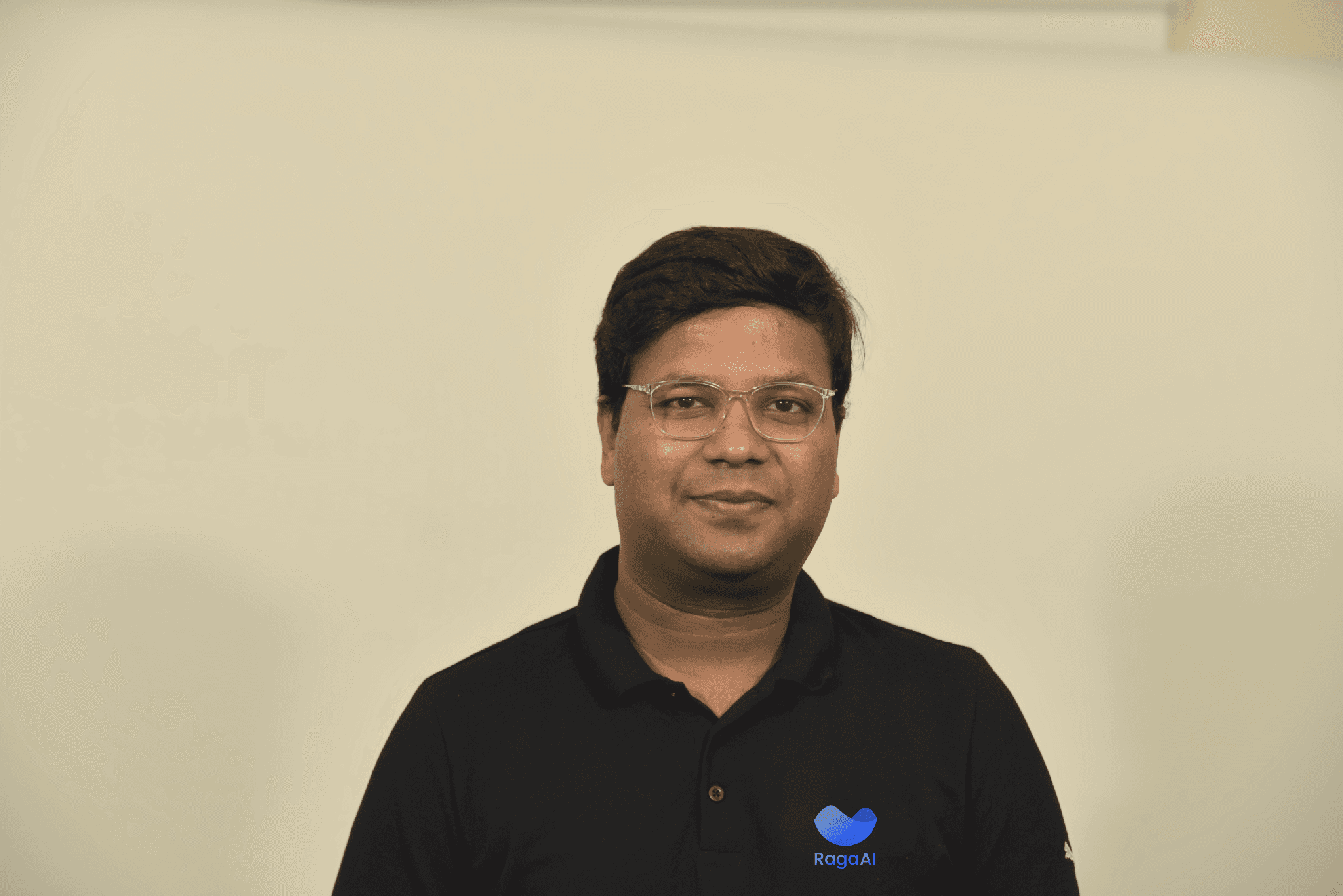
Jigar Gupta
Apr 17, 2024
Read the article
Understanding AI Regulatory Compliance And Its Importance

Akshat Gupta
Apr 16, 2024
Read the article
Understanding The Basics Of AI Governance

Akshat Gupta
Apr 15, 2024
Read the article
Understanding Prompt Engineering: A Guide

Rehan Asif
Apr 15, 2024
Read the article
Examples And Strategies To Mitigate AI Bias In Real-Life

Akshat Gupta
Apr 14, 2024
Read the article
Understanding The Basics Of LLM Fine-tuning With Custom Data

Rehan Asif
Apr 13, 2024
Read the article
Overview Of Key Concepts In AI Safety And Security
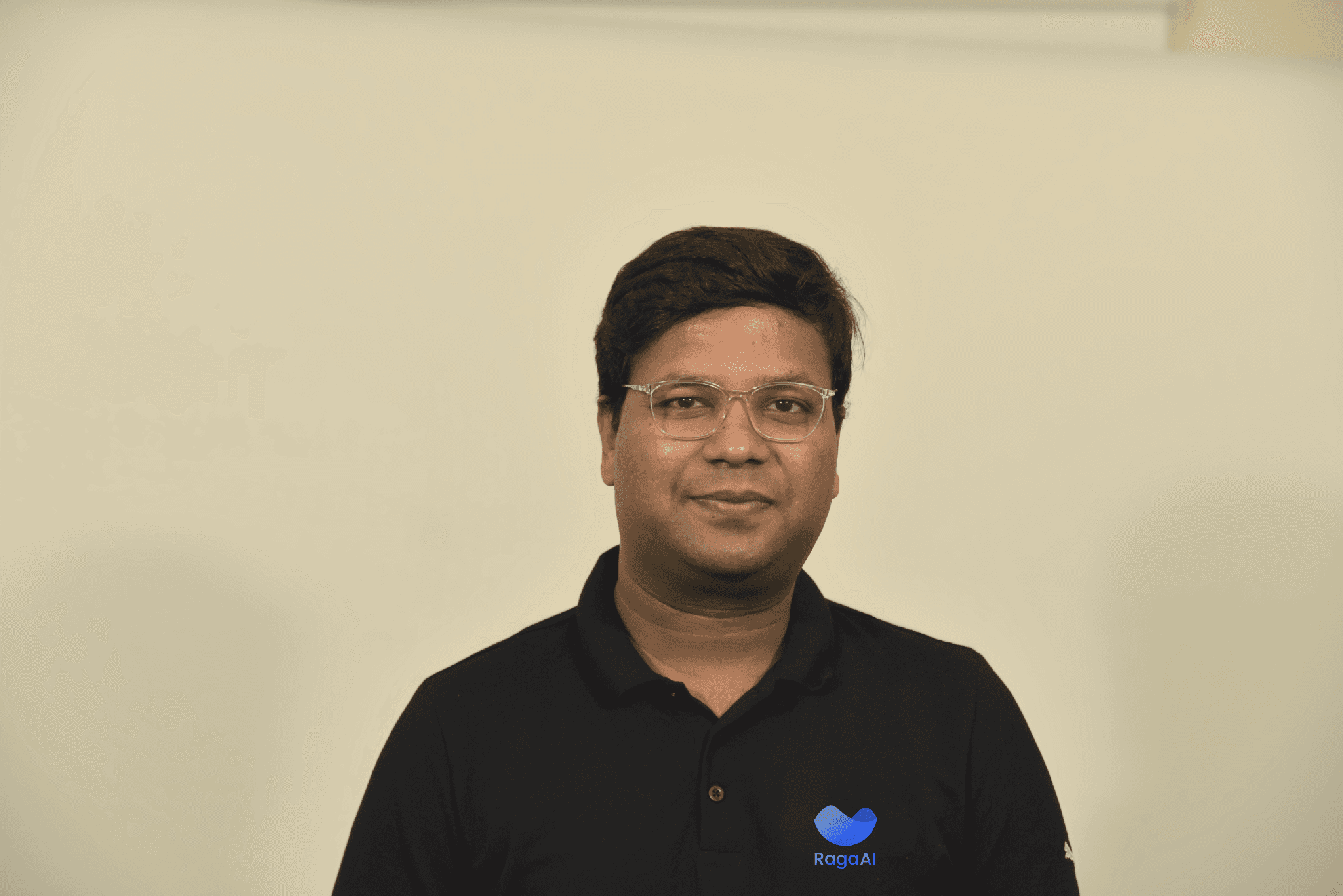
Jigar Gupta
Apr 12, 2024
Read the article
Understanding Hallucinations In LLMs

Rehan Asif
Apr 7, 2024
Read the article
Demystifying FDA's Approach to AI/ML in Healthcare: Your Ultimate Guide
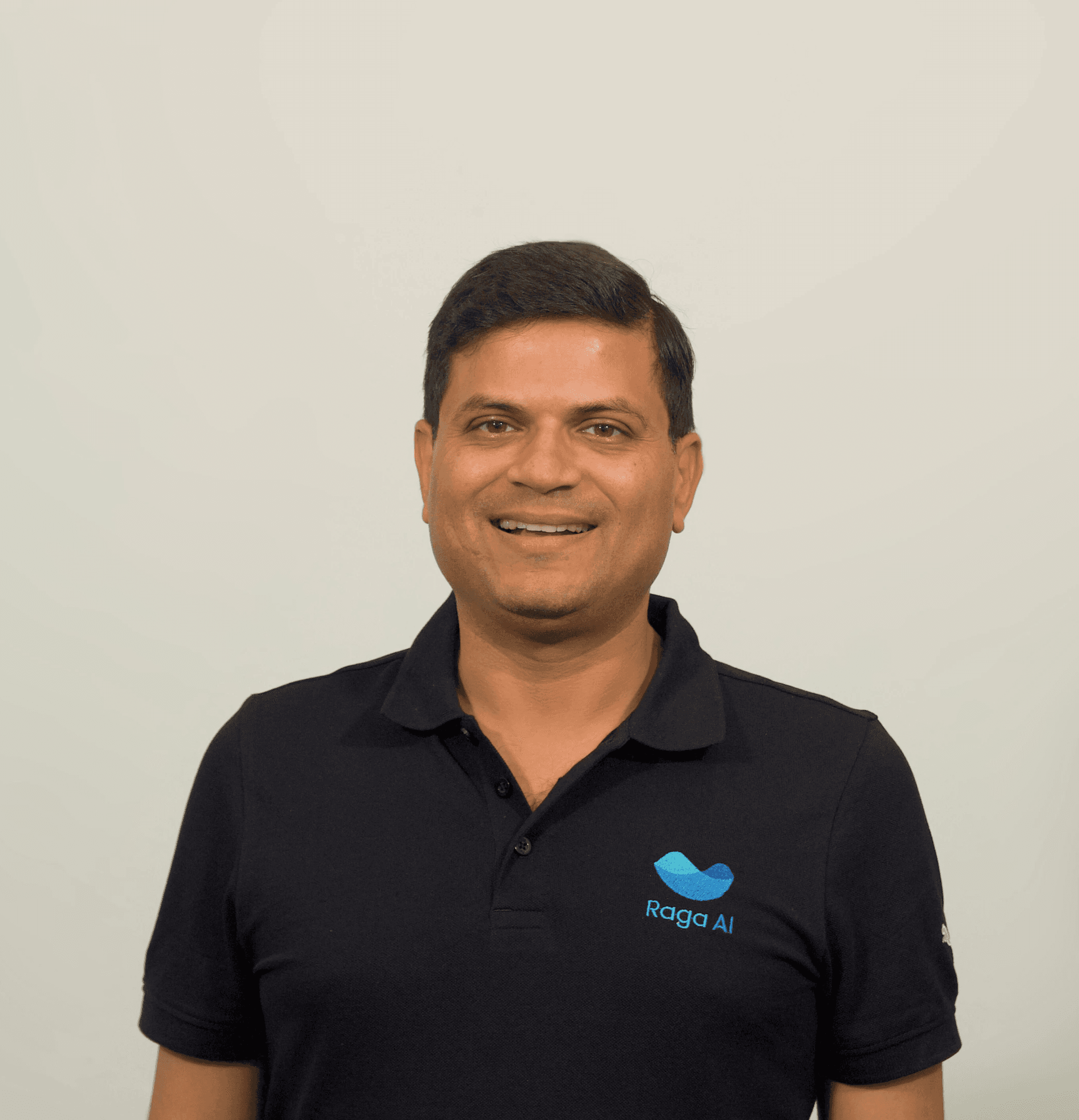
Gaurav Agarwal
Apr 4, 2024
Read the article
Navigating AI Governance in Aerospace Industry

Akshat Gupta
Apr 3, 2024
Read the article
The White House Executive Order on Safe and Trustworthy AI

Jigar Gupta
Mar 29, 2024
Read the article
The EU AI Act - All you need to know
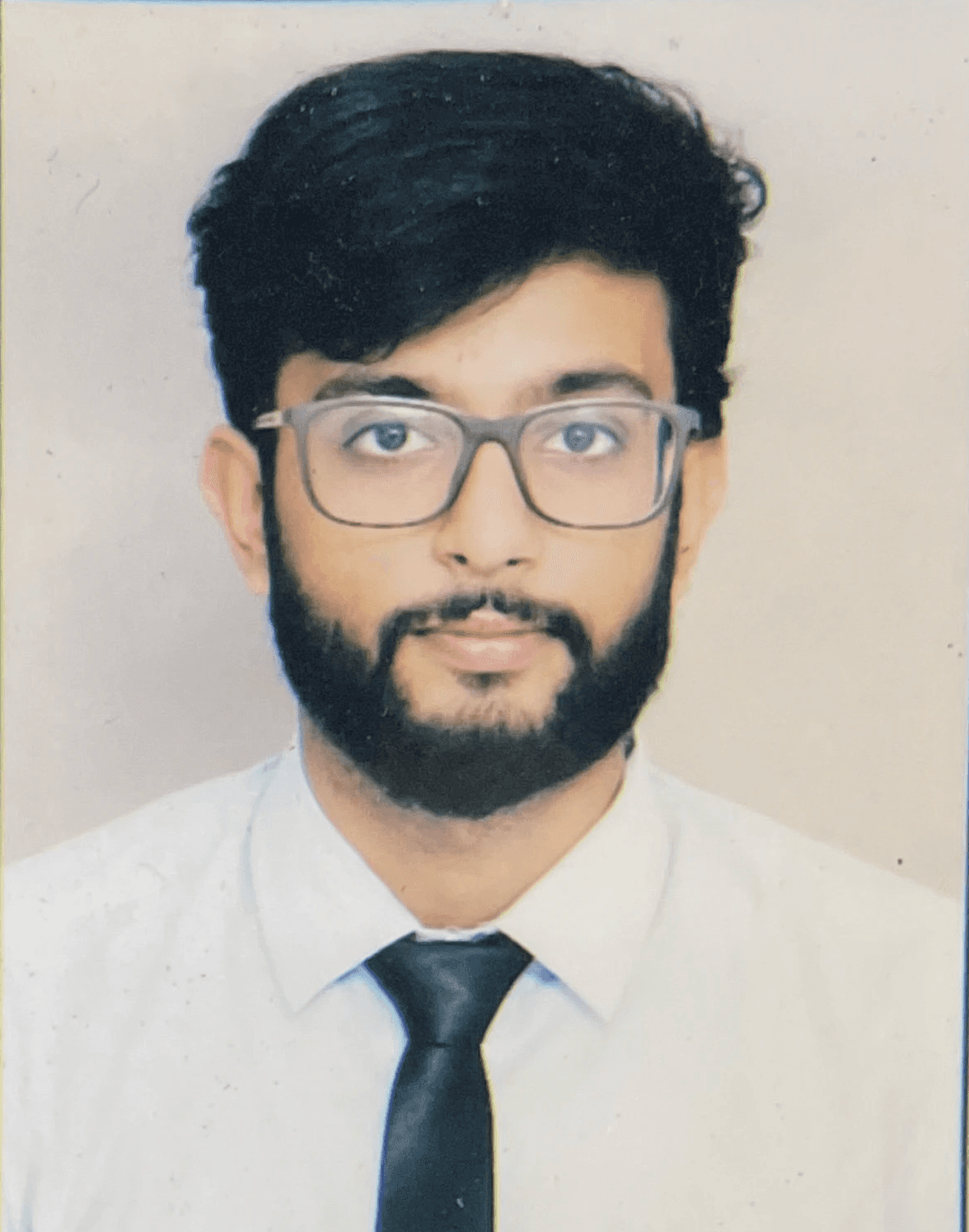
Akshat Gupta
Mar 27, 2024
Read the article
Enhancing Edge AI with RagaAI Integration on NVIDIA Metropolis

Siddharth Jain
Mar 15, 2024
Read the article
RagaAI releases the most comprehensive open-source LLM Evaluation and Guardrails package

Gaurav Agarwal
Mar 7, 2024
Read the article
A Guide to Evaluating LLM Applications and enabling Guardrails using Raga-LLM-Hub

Rehan Asif
Mar 7, 2024
Read the article
Identifying edge cases within CelebA Dataset using RagaAI testing Platform

Rehan Asif
Feb 15, 2024
Read the article
How to Detect and Fix AI Issues with RagaAI
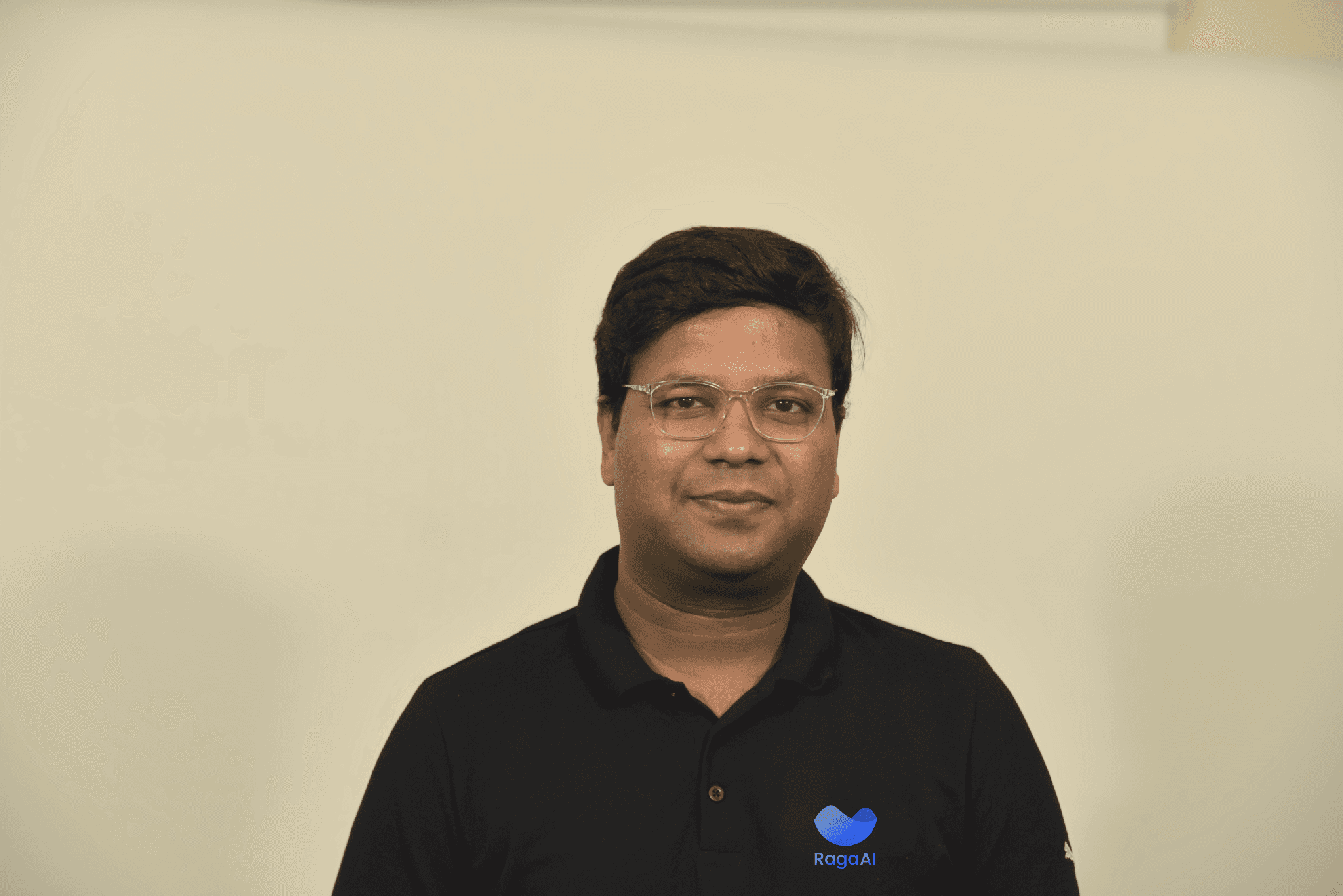
Jigar Gupta
Feb 16, 2024
Read the article
Detection of Labelling Issue in CIFAR-10 Dataset using RagaAI Platform
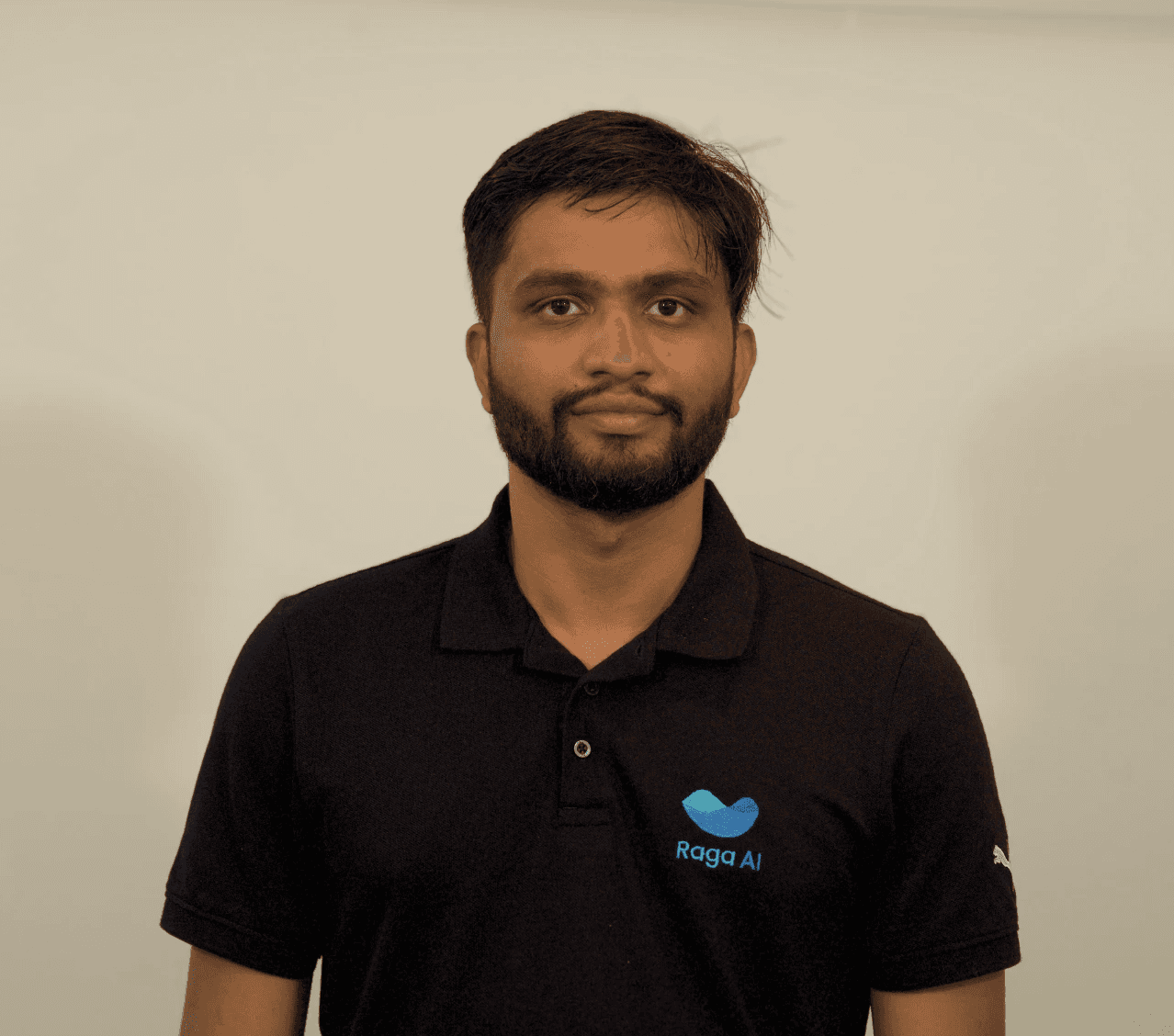
Rehan Asif
Feb 5, 2024
Read the article
RagaAI emerges from Stealth with the most Comprehensive Testing Platform for AI
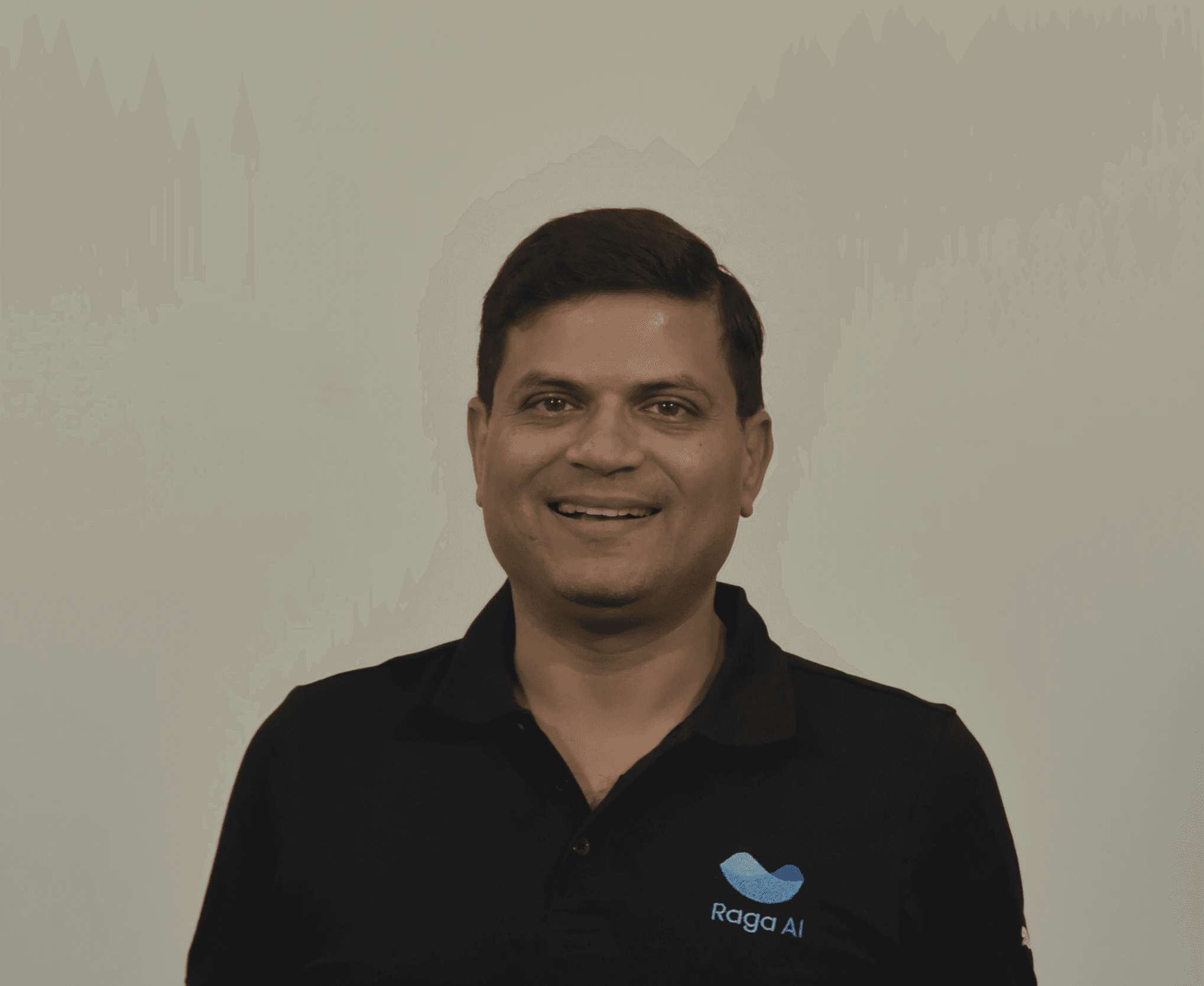
Gaurav Agarwal
Jan 23, 2024
Read the article
AI’s Missing Piece: Comprehensive AI Testing

Gaurav Agarwal
Jan 11, 2024
Read the article
Introducing RagaAI - The Future of AI Testing
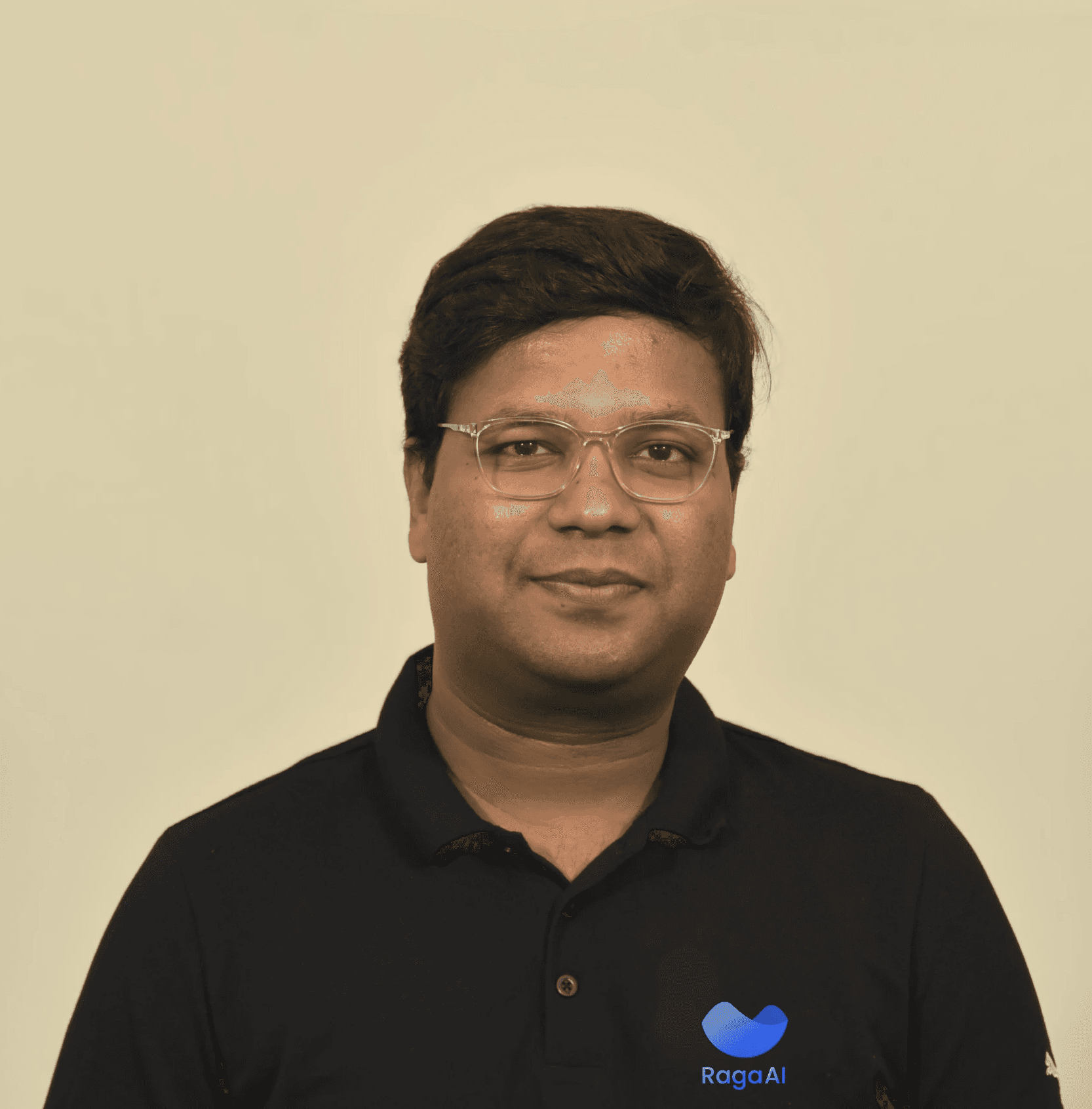
Jigar Gupta
Jan 14, 2024
Read the article
Introducing RagaAI DNA: The Multi-modal Foundation Model for AI Testing
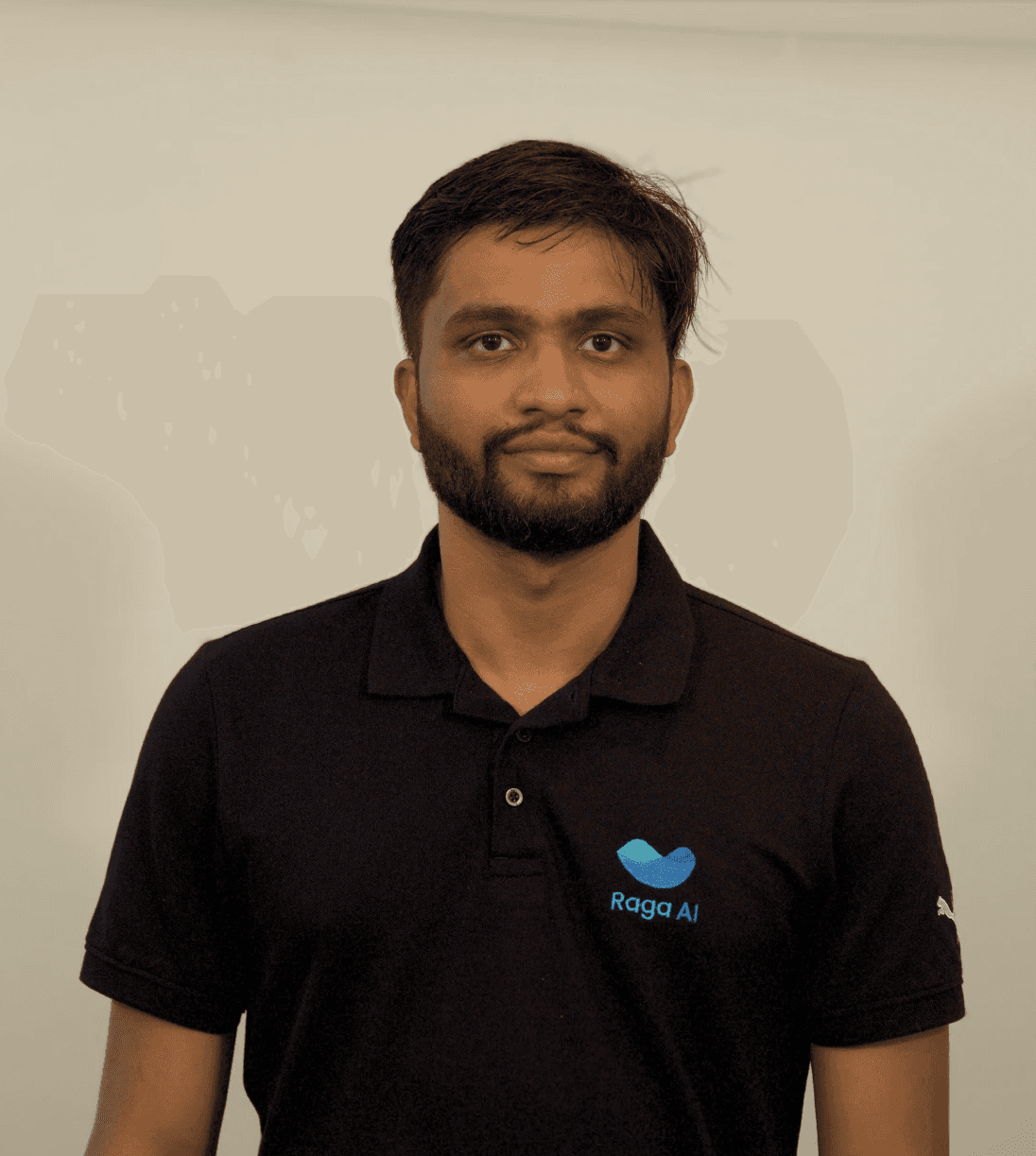
Rehan Asif
Jan 13, 2024
Read the article