Demystifying FDA's Approach to AI/ML in Healthcare: Your Ultimate Guide
Demystifying FDA's Approach to AI/ML in Healthcare: Your Ultimate Guide
Demystifying FDA's Approach to AI/ML in Healthcare: Your Ultimate Guide
Gaurav Agarwal
Apr 4, 2024




As we delve into the realm of cutting-edge artificial intelligence (AI) and machine learning (ML) technologies within the healthcare sector, it becomes imperative to navigate the regulatory landscape, particularly under the purview of the U.S. Food and Drug Administration (FDA). The FDA has recently released a series of discussion papers shedding light on their current perspectives and considerations surrounding the integration of AI/ML in healthcare.
Navigating the Regulatory Terrain
Software as a Medical Device (SaMD) constitutes software intended for medical purposes, independent of hardware medical devices. The International Medical Device Regulators Forum (IMDRF) delineates SaMD as software executing medical functions without being embedded in a physical device. Examples encompass mobile medical applications, clinical decision support software, and AI/ML-based algorithms for medical image analysis, diagnosis, or treatment recommendations.
Presently, AI/ML-based SaMD must adhere to the same regulatory prerequisites as traditional medical devices. This entails establishing a quality system (as per 21 CFR 820) and undergoing premarket review and clearance/approval by the FDA based on risk categorisation. The IMDRF has devised a risk categorisation framework stratifying SaMD into four categories (I-IV) contingent on the significance of the information provided and the healthcare situation's state.
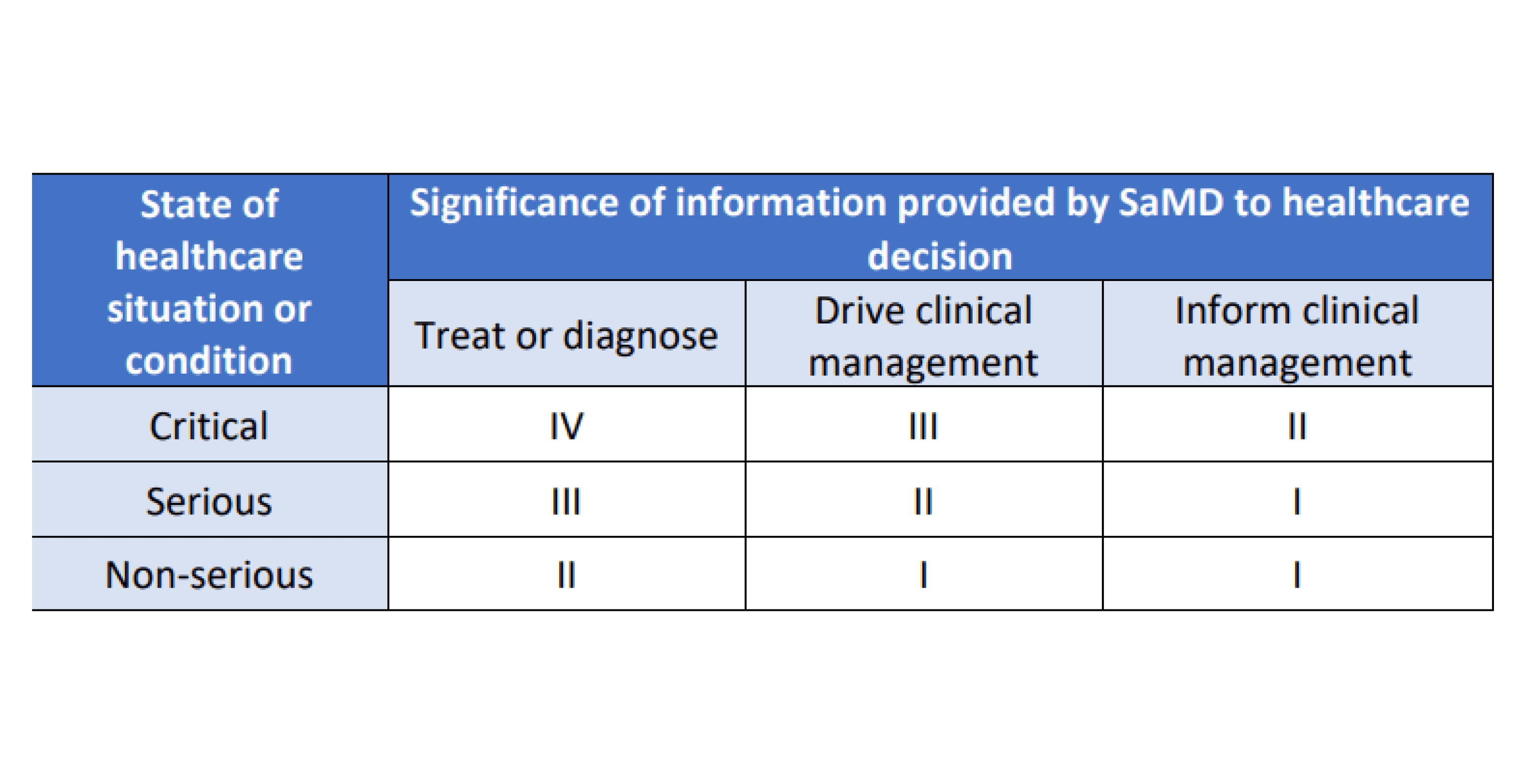
Image Source: FDA Proposed Regulatory Framework
For SaMD necessitating premarket submission (e.g., 510(k), De Novo, or PMA), the FDA typically reviews and clears/approves a "locked" AI/ML algorithm. A locked algorithm yields consistent output for a given set of inputs and remains static over time. Substantial alterations to the locked algorithm generally mandate a new premarket submission and FDA review.
However, this framework isn't ideally suited for the dynamic nature of AI/ML algorithms, which continuously learn and adapt post-market. The iterative, autonomous, and adaptive attributes necessitate a novel regulatory approach facilitating swift product enhancement cycles while upholding safety and effectiveness standards.
Recognising the Potential and Crafting Future Regulations
The FDA acknowledges the transformative potential of AI/ML-based SaMD in healthcare, envisaging advancements in disease detection, diagnosis accuracy, and personalised therapeutics. However, the current regulatory paradigm presents a challenge in balancing the benefits of continual learning with the imperative of regulatory oversight to mitigate patient risks.
In response, the FDA has proposed a novel Total Product Lifecycle (TPLC) regulatory approach. This approach evaluates a company's culture of quality and organisational excellence, ensuring high-quality software development, testing, and performance monitoring. Key tenets of this approach include:
Establishing clear expectations regarding quality systems and good machine learning practices (GMLP)
Image Source: FDA Proposed Regulatory Framework
Conducting premarket review with optional submission of predetermined modification plans by manufacturers.
Mandating manufacturers to monitor and manage modification risks per predetermined plans.
Enabling transparency and real-world performance reporting.
Central to this approach is the concept of a "predetermined change control plan" (PCCP), which manufacturers can submit during premarket review. The PCCP delineates anticipated modifications and protocols for implementation and validation, facilitating controlled iteration while ensuring safety and effectiveness.
Crafting a Predetermined Change Control Plan
The report proposes that a PCCP consists of three main components:
1) a detailed description of planned modifications
2) a modification protocol outlining verification/validation activities for the modifications, and
3) an impact assessment evaluating the benefits/risks of the modifications.
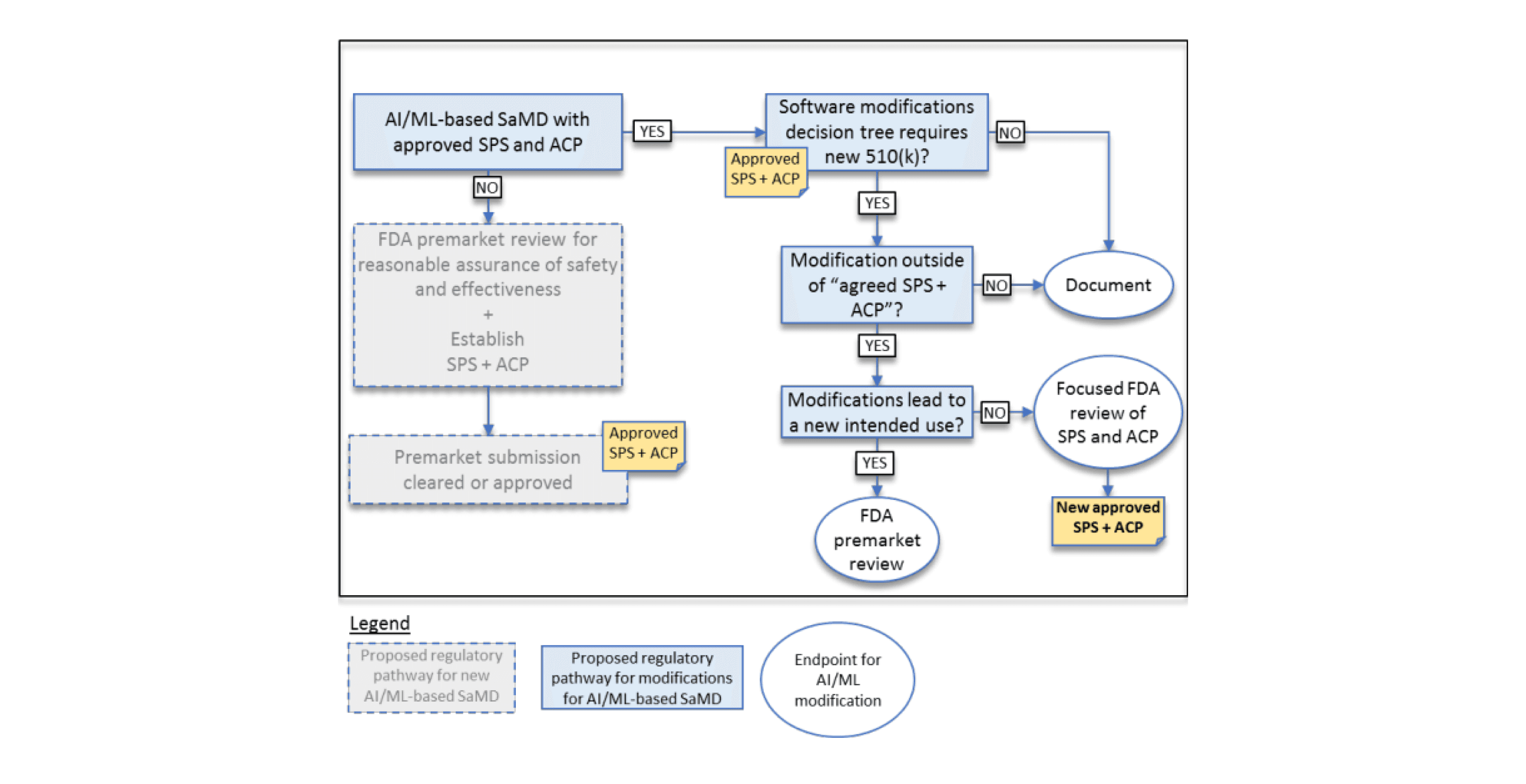
Image Source: FDA Proposed Regulatory Framework
Establishing a PCCP involves clearly defining the scope of anticipated modifications and documenting robust procedures in the Modification Protocol to ensure modifications remain safe and effective. The level of detail required may depend on the SaMD's risk category.
When submitting for marketing authorization, manufacturers can identify if they plan to utilise a PCCP by including the Description of Modifications and Modification Protocol sections in their submission. FDA's review and authorization of an acceptable PCCP would allow the manufacturer to implement certain types of modifications described in the plan without further FDA review, as long as they follow the specified protocols and document their modification activities.
The Description of Modifications section should define the goals and boundaries for what types of modifications may occur, such as performance enhancements, new data inputs, or refined intended uses. Providing clear examples is recommended.
The Modification Protocol should specify how the manufacturer will implement modifications reliably. It should cover:
1) Data management practices for curating, maintaining, and controlling training/test datasets
2) Re-training practices on when/how the algorithm will be re-trained
3) Performance evaluation protocols including metrics, analysis methods, predetermined performance targets
4) Update procedures for testing, deployment, versioning, and communication of algorithm updates
There must be traceability mapping the specific modification types described to the corresponding methods detailed in the Modification Protocol.
The PCCP framework aims to enable controlled iteration of AI/ML SaMD to realise performance improvements while enforcing pre-specified processes and guardrails to manage risks as the device evolves after initial marketing. Manufacturer adherence to approved PCCPs provides ongoing reasonable assurance of safety and effectiveness.
How can RagaAI help here?
Analysis of published resources shows RagaAI platform can help unlock tremendous values with regards to AI/ML compliance dimensions not only now but in future too.
Under the current overarching regulations RagaAI can help determine a locked SAMD and Good ML Practices, helping you achieve compliance with FDA.
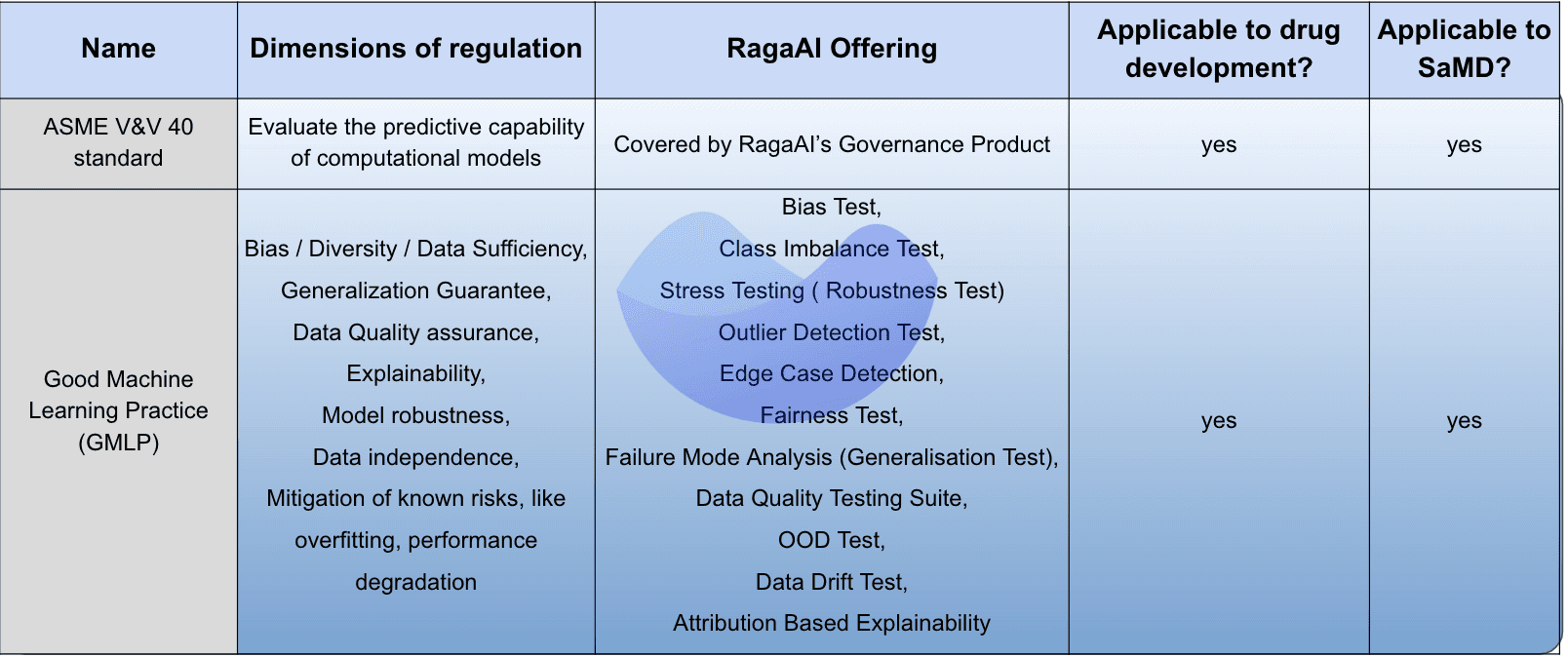
The above image shows mapping of RagaAI offerings against GMLP practices. Source: RagaAI
RagaAI also provides a concrete framework for complying with the practices for AI/ML in Drug Development, which have been formulated with a risk-based approach.
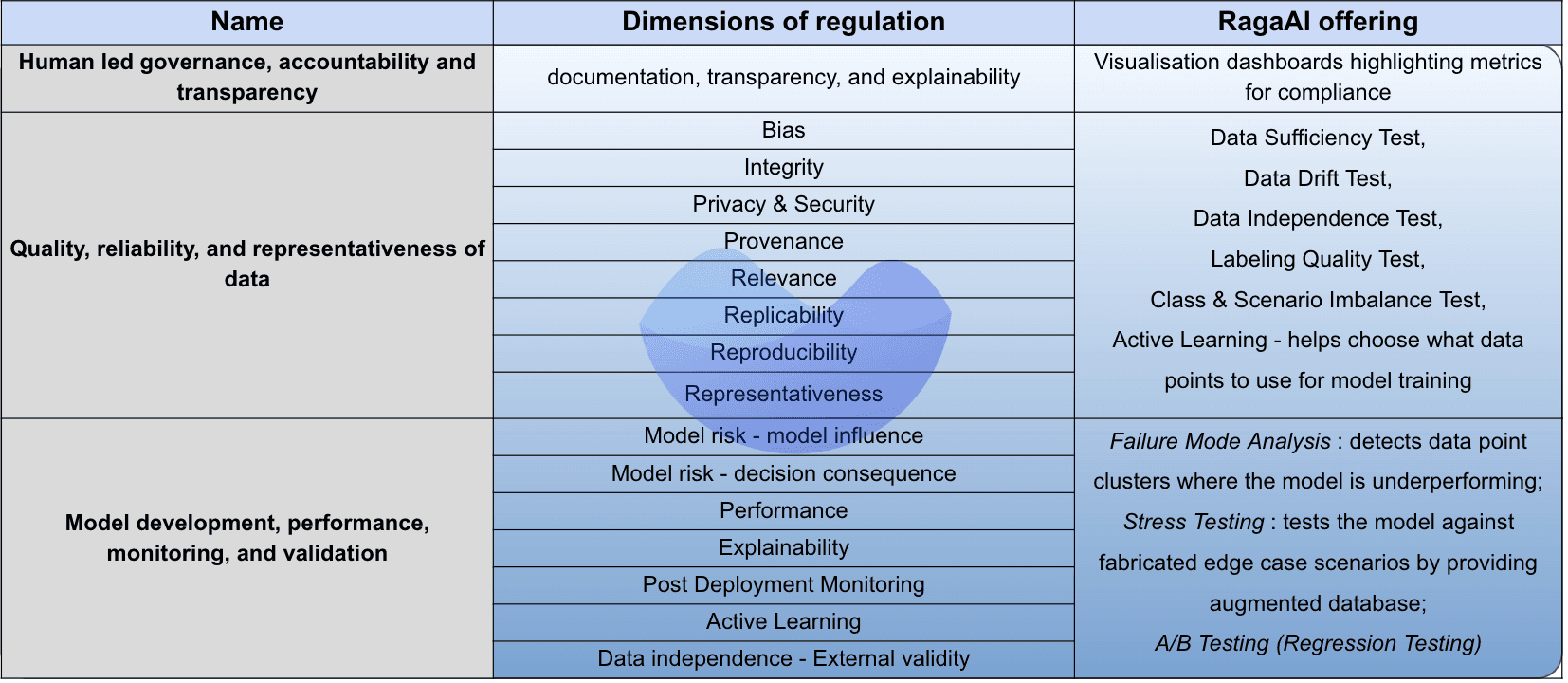
Image Source: RagaAI
The website docs enlist and meticulously present the various tests which can be used to comply with different aspects of FDA regulations for both drug development and SaMD.
Conclusion
While this article only covers the AI Compliance landscape in healthcare from the lens of FDA, there are various regulatory regimes being crafted across the globe. FDA, being on the top of healthcare regulations, provides the best view into how the healthcare industry can approach enabling safe and ethical use of AI in applications. As the healthcare industry is a very high-risk sector, the technology deployed at every step needs to be safe and repairable. Being highly comprehensive, this set of regulations can also be extrapolated, on a high level, to other acute-risk industries with ease.
It is natural to have a lot of questions in your mind after reading this, and unfortunately we cannot cover all the ground in this article.
But we wholeheartedly extend an invitation to share our expertise with whoever wants to take this wobbly ride of AI governance in Healthcare.
Get in touch with our Experts.
Want to know more ? Get in touch with our experts!
As we delve into the realm of cutting-edge artificial intelligence (AI) and machine learning (ML) technologies within the healthcare sector, it becomes imperative to navigate the regulatory landscape, particularly under the purview of the U.S. Food and Drug Administration (FDA). The FDA has recently released a series of discussion papers shedding light on their current perspectives and considerations surrounding the integration of AI/ML in healthcare.
Navigating the Regulatory Terrain
Software as a Medical Device (SaMD) constitutes software intended for medical purposes, independent of hardware medical devices. The International Medical Device Regulators Forum (IMDRF) delineates SaMD as software executing medical functions without being embedded in a physical device. Examples encompass mobile medical applications, clinical decision support software, and AI/ML-based algorithms for medical image analysis, diagnosis, or treatment recommendations.
Presently, AI/ML-based SaMD must adhere to the same regulatory prerequisites as traditional medical devices. This entails establishing a quality system (as per 21 CFR 820) and undergoing premarket review and clearance/approval by the FDA based on risk categorisation. The IMDRF has devised a risk categorisation framework stratifying SaMD into four categories (I-IV) contingent on the significance of the information provided and the healthcare situation's state.
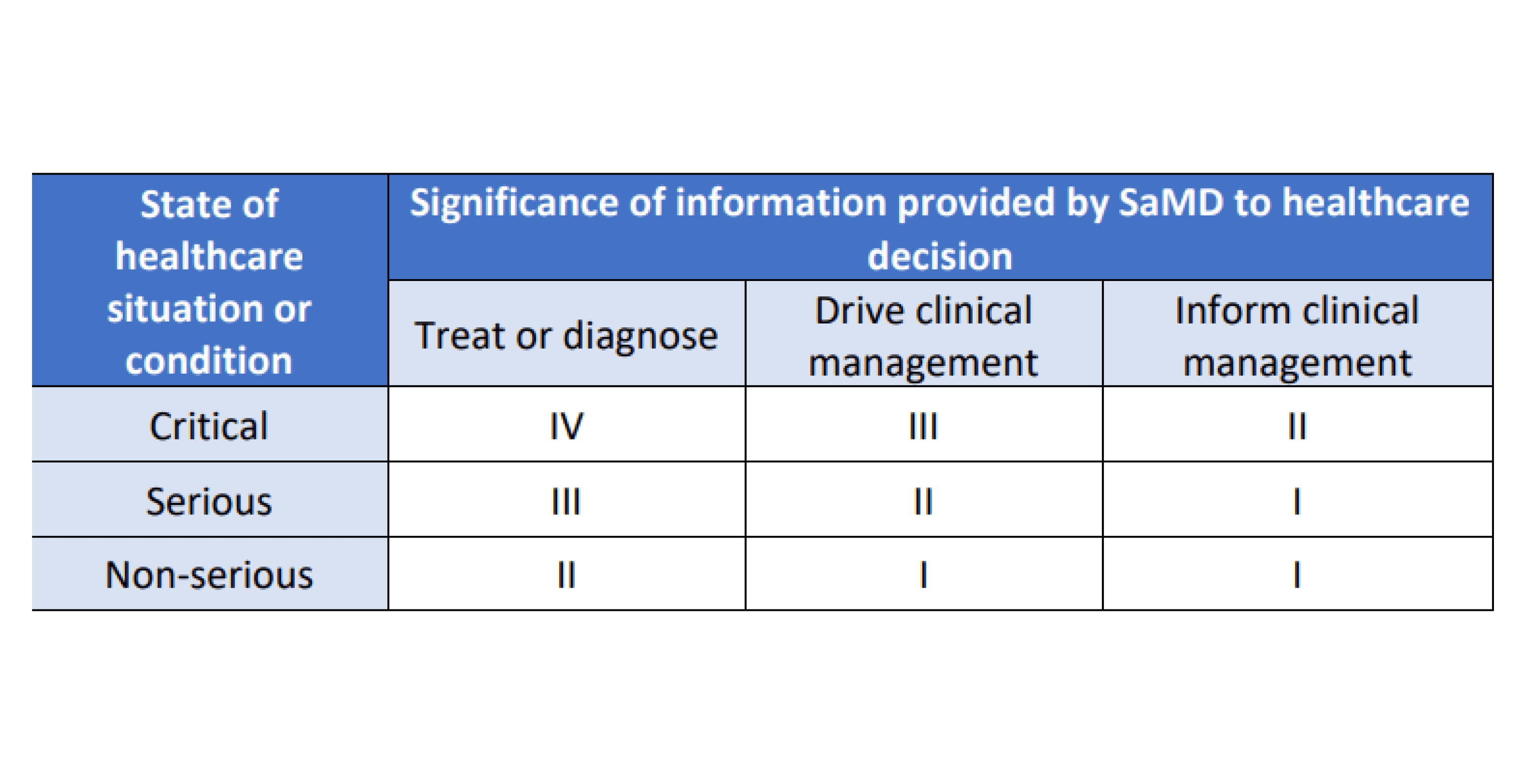
Image Source: FDA Proposed Regulatory Framework
For SaMD necessitating premarket submission (e.g., 510(k), De Novo, or PMA), the FDA typically reviews and clears/approves a "locked" AI/ML algorithm. A locked algorithm yields consistent output for a given set of inputs and remains static over time. Substantial alterations to the locked algorithm generally mandate a new premarket submission and FDA review.
However, this framework isn't ideally suited for the dynamic nature of AI/ML algorithms, which continuously learn and adapt post-market. The iterative, autonomous, and adaptive attributes necessitate a novel regulatory approach facilitating swift product enhancement cycles while upholding safety and effectiveness standards.
Recognising the Potential and Crafting Future Regulations
The FDA acknowledges the transformative potential of AI/ML-based SaMD in healthcare, envisaging advancements in disease detection, diagnosis accuracy, and personalised therapeutics. However, the current regulatory paradigm presents a challenge in balancing the benefits of continual learning with the imperative of regulatory oversight to mitigate patient risks.
In response, the FDA has proposed a novel Total Product Lifecycle (TPLC) regulatory approach. This approach evaluates a company's culture of quality and organisational excellence, ensuring high-quality software development, testing, and performance monitoring. Key tenets of this approach include:
Establishing clear expectations regarding quality systems and good machine learning practices (GMLP)
Image Source: FDA Proposed Regulatory Framework
Conducting premarket review with optional submission of predetermined modification plans by manufacturers.
Mandating manufacturers to monitor and manage modification risks per predetermined plans.
Enabling transparency and real-world performance reporting.
Central to this approach is the concept of a "predetermined change control plan" (PCCP), which manufacturers can submit during premarket review. The PCCP delineates anticipated modifications and protocols for implementation and validation, facilitating controlled iteration while ensuring safety and effectiveness.
Crafting a Predetermined Change Control Plan
The report proposes that a PCCP consists of three main components:
1) a detailed description of planned modifications
2) a modification protocol outlining verification/validation activities for the modifications, and
3) an impact assessment evaluating the benefits/risks of the modifications.
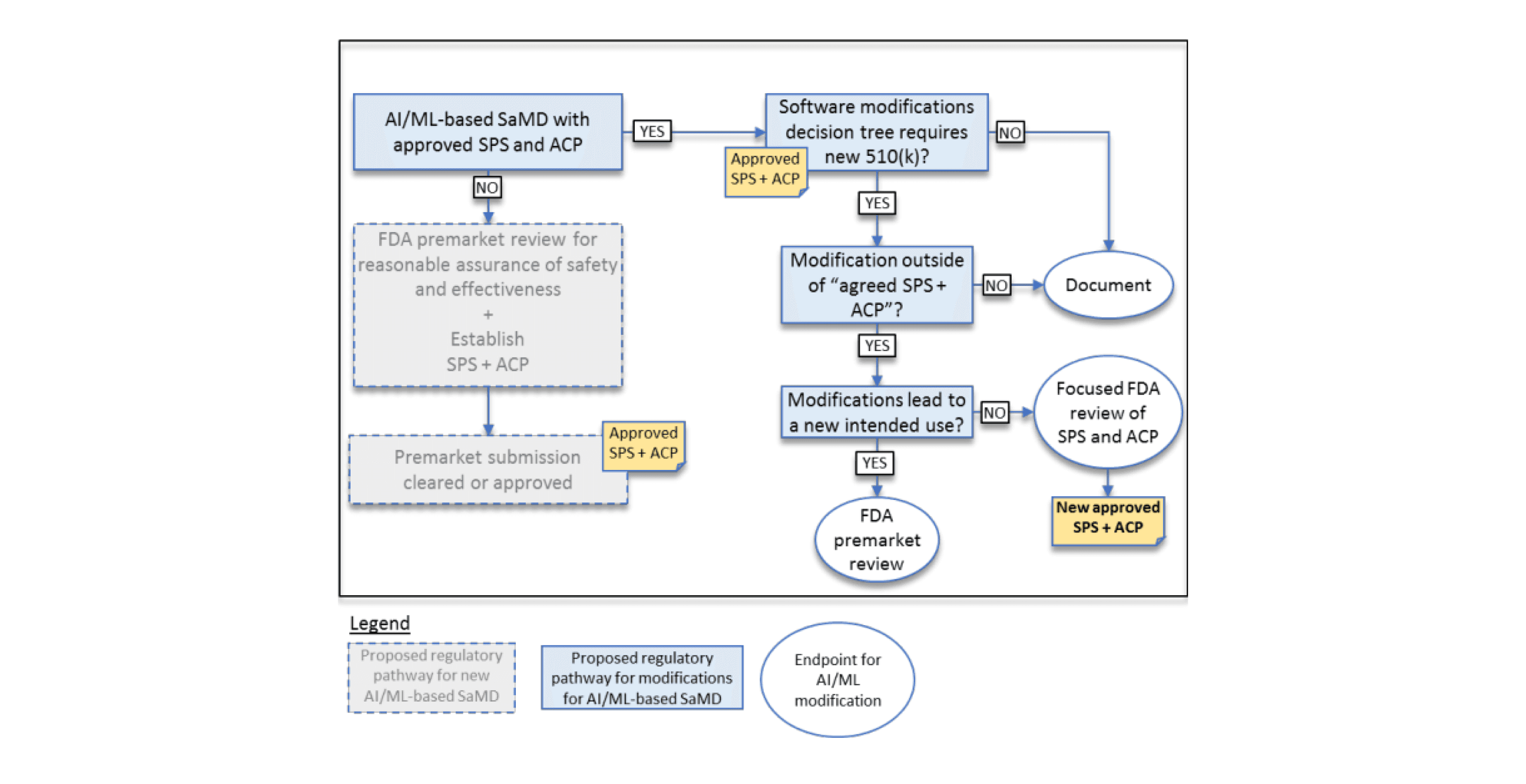
Image Source: FDA Proposed Regulatory Framework
Establishing a PCCP involves clearly defining the scope of anticipated modifications and documenting robust procedures in the Modification Protocol to ensure modifications remain safe and effective. The level of detail required may depend on the SaMD's risk category.
When submitting for marketing authorization, manufacturers can identify if they plan to utilise a PCCP by including the Description of Modifications and Modification Protocol sections in their submission. FDA's review and authorization of an acceptable PCCP would allow the manufacturer to implement certain types of modifications described in the plan without further FDA review, as long as they follow the specified protocols and document their modification activities.
The Description of Modifications section should define the goals and boundaries for what types of modifications may occur, such as performance enhancements, new data inputs, or refined intended uses. Providing clear examples is recommended.
The Modification Protocol should specify how the manufacturer will implement modifications reliably. It should cover:
1) Data management practices for curating, maintaining, and controlling training/test datasets
2) Re-training practices on when/how the algorithm will be re-trained
3) Performance evaluation protocols including metrics, analysis methods, predetermined performance targets
4) Update procedures for testing, deployment, versioning, and communication of algorithm updates
There must be traceability mapping the specific modification types described to the corresponding methods detailed in the Modification Protocol.
The PCCP framework aims to enable controlled iteration of AI/ML SaMD to realise performance improvements while enforcing pre-specified processes and guardrails to manage risks as the device evolves after initial marketing. Manufacturer adherence to approved PCCPs provides ongoing reasonable assurance of safety and effectiveness.
How can RagaAI help here?
Analysis of published resources shows RagaAI platform can help unlock tremendous values with regards to AI/ML compliance dimensions not only now but in future too.
Under the current overarching regulations RagaAI can help determine a locked SAMD and Good ML Practices, helping you achieve compliance with FDA.
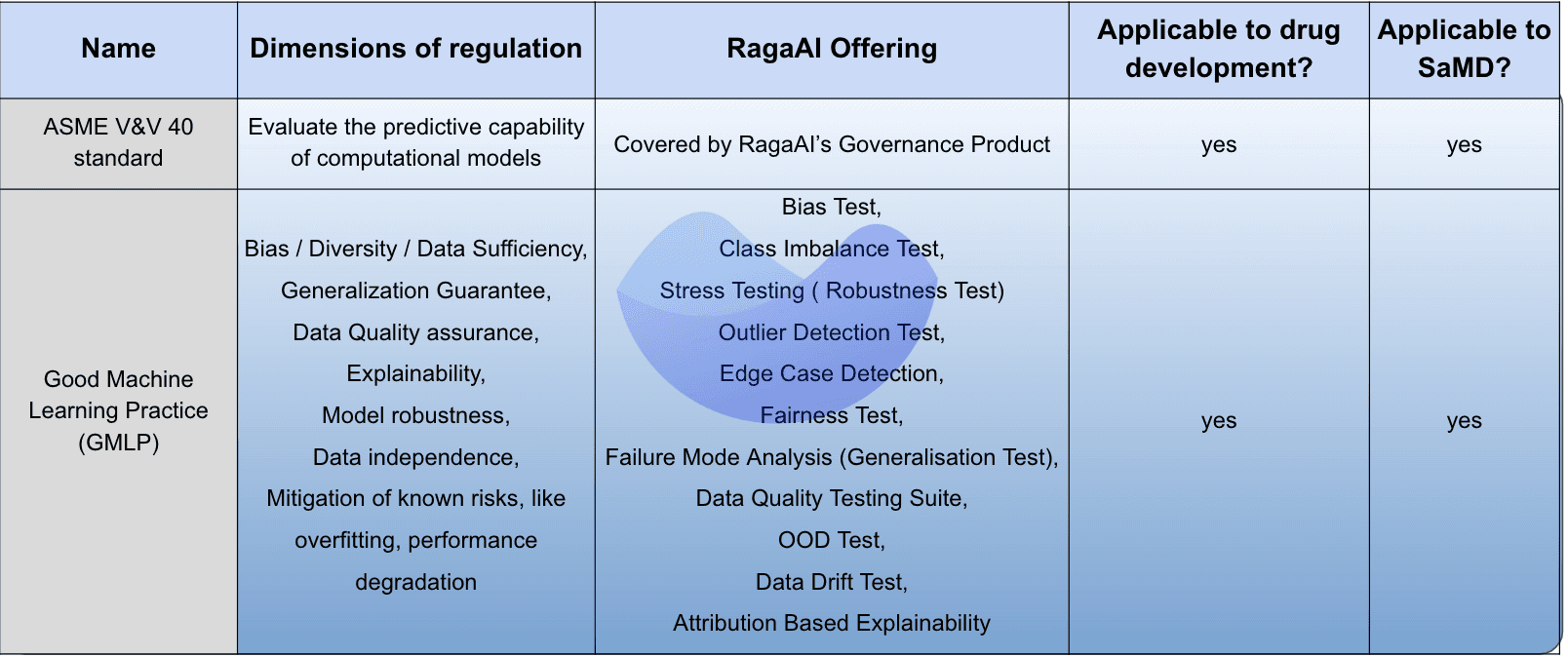
The above image shows mapping of RagaAI offerings against GMLP practices. Source: RagaAI
RagaAI also provides a concrete framework for complying with the practices for AI/ML in Drug Development, which have been formulated with a risk-based approach.
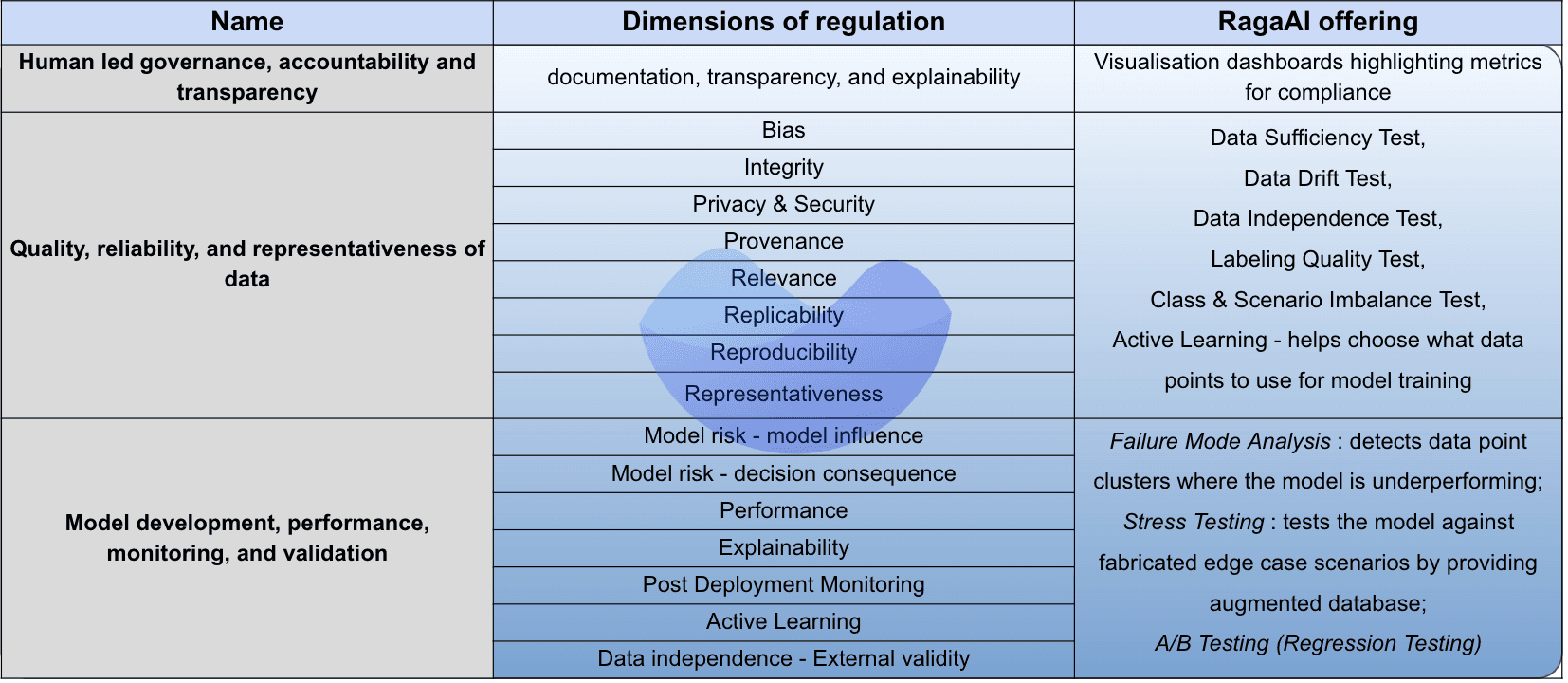
Image Source: RagaAI
The website docs enlist and meticulously present the various tests which can be used to comply with different aspects of FDA regulations for both drug development and SaMD.
Conclusion
While this article only covers the AI Compliance landscape in healthcare from the lens of FDA, there are various regulatory regimes being crafted across the globe. FDA, being on the top of healthcare regulations, provides the best view into how the healthcare industry can approach enabling safe and ethical use of AI in applications. As the healthcare industry is a very high-risk sector, the technology deployed at every step needs to be safe and repairable. Being highly comprehensive, this set of regulations can also be extrapolated, on a high level, to other acute-risk industries with ease.
It is natural to have a lot of questions in your mind after reading this, and unfortunately we cannot cover all the ground in this article.
But we wholeheartedly extend an invitation to share our expertise with whoever wants to take this wobbly ride of AI governance in Healthcare.
Get in touch with our Experts.
Want to know more ? Get in touch with our experts!
As we delve into the realm of cutting-edge artificial intelligence (AI) and machine learning (ML) technologies within the healthcare sector, it becomes imperative to navigate the regulatory landscape, particularly under the purview of the U.S. Food and Drug Administration (FDA). The FDA has recently released a series of discussion papers shedding light on their current perspectives and considerations surrounding the integration of AI/ML in healthcare.
Navigating the Regulatory Terrain
Software as a Medical Device (SaMD) constitutes software intended for medical purposes, independent of hardware medical devices. The International Medical Device Regulators Forum (IMDRF) delineates SaMD as software executing medical functions without being embedded in a physical device. Examples encompass mobile medical applications, clinical decision support software, and AI/ML-based algorithms for medical image analysis, diagnosis, or treatment recommendations.
Presently, AI/ML-based SaMD must adhere to the same regulatory prerequisites as traditional medical devices. This entails establishing a quality system (as per 21 CFR 820) and undergoing premarket review and clearance/approval by the FDA based on risk categorisation. The IMDRF has devised a risk categorisation framework stratifying SaMD into four categories (I-IV) contingent on the significance of the information provided and the healthcare situation's state.
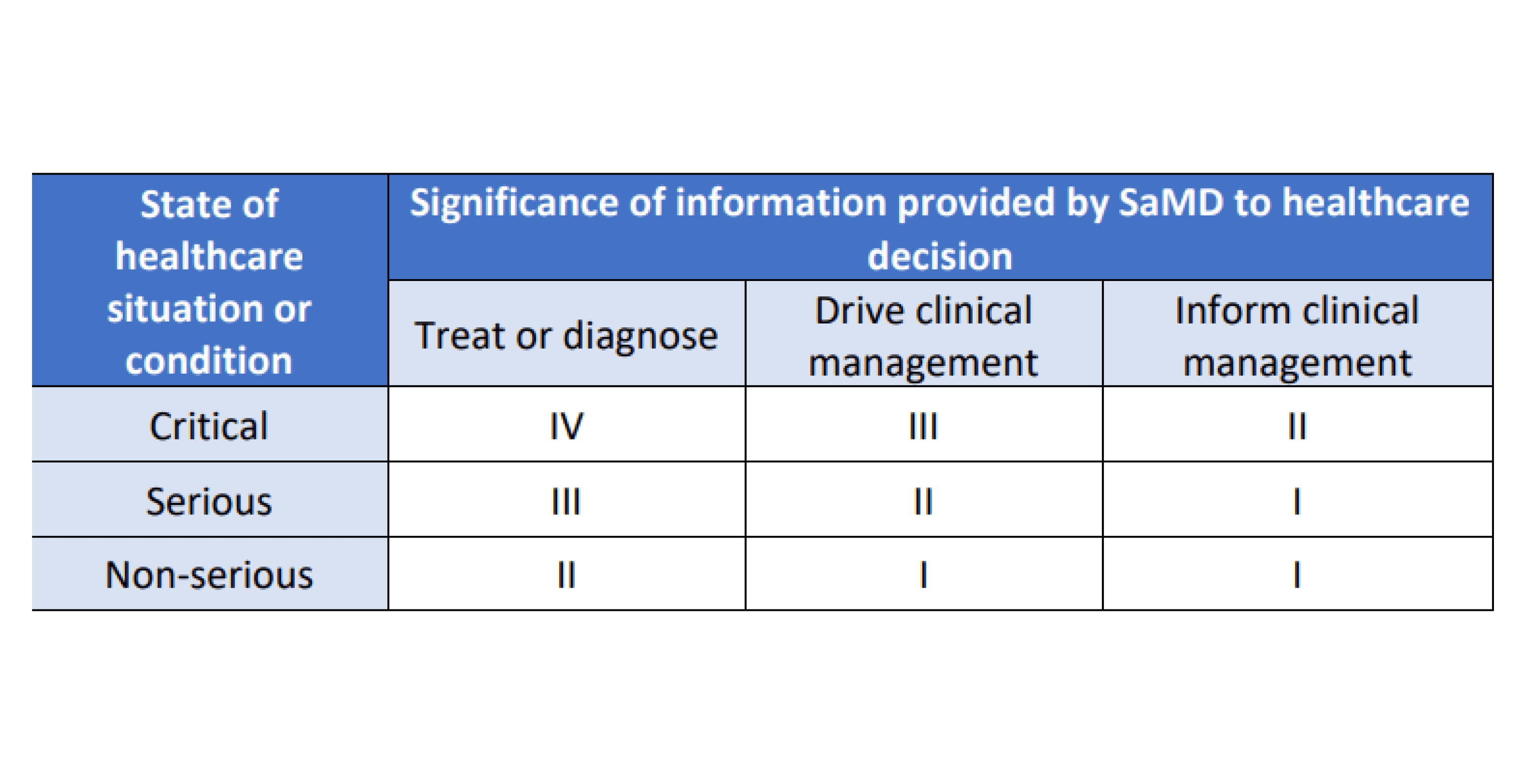
Image Source: FDA Proposed Regulatory Framework
For SaMD necessitating premarket submission (e.g., 510(k), De Novo, or PMA), the FDA typically reviews and clears/approves a "locked" AI/ML algorithm. A locked algorithm yields consistent output for a given set of inputs and remains static over time. Substantial alterations to the locked algorithm generally mandate a new premarket submission and FDA review.
However, this framework isn't ideally suited for the dynamic nature of AI/ML algorithms, which continuously learn and adapt post-market. The iterative, autonomous, and adaptive attributes necessitate a novel regulatory approach facilitating swift product enhancement cycles while upholding safety and effectiveness standards.
Recognising the Potential and Crafting Future Regulations
The FDA acknowledges the transformative potential of AI/ML-based SaMD in healthcare, envisaging advancements in disease detection, diagnosis accuracy, and personalised therapeutics. However, the current regulatory paradigm presents a challenge in balancing the benefits of continual learning with the imperative of regulatory oversight to mitigate patient risks.
In response, the FDA has proposed a novel Total Product Lifecycle (TPLC) regulatory approach. This approach evaluates a company's culture of quality and organisational excellence, ensuring high-quality software development, testing, and performance monitoring. Key tenets of this approach include:
Establishing clear expectations regarding quality systems and good machine learning practices (GMLP)
Image Source: FDA Proposed Regulatory Framework
Conducting premarket review with optional submission of predetermined modification plans by manufacturers.
Mandating manufacturers to monitor and manage modification risks per predetermined plans.
Enabling transparency and real-world performance reporting.
Central to this approach is the concept of a "predetermined change control plan" (PCCP), which manufacturers can submit during premarket review. The PCCP delineates anticipated modifications and protocols for implementation and validation, facilitating controlled iteration while ensuring safety and effectiveness.
Crafting a Predetermined Change Control Plan
The report proposes that a PCCP consists of three main components:
1) a detailed description of planned modifications
2) a modification protocol outlining verification/validation activities for the modifications, and
3) an impact assessment evaluating the benefits/risks of the modifications.
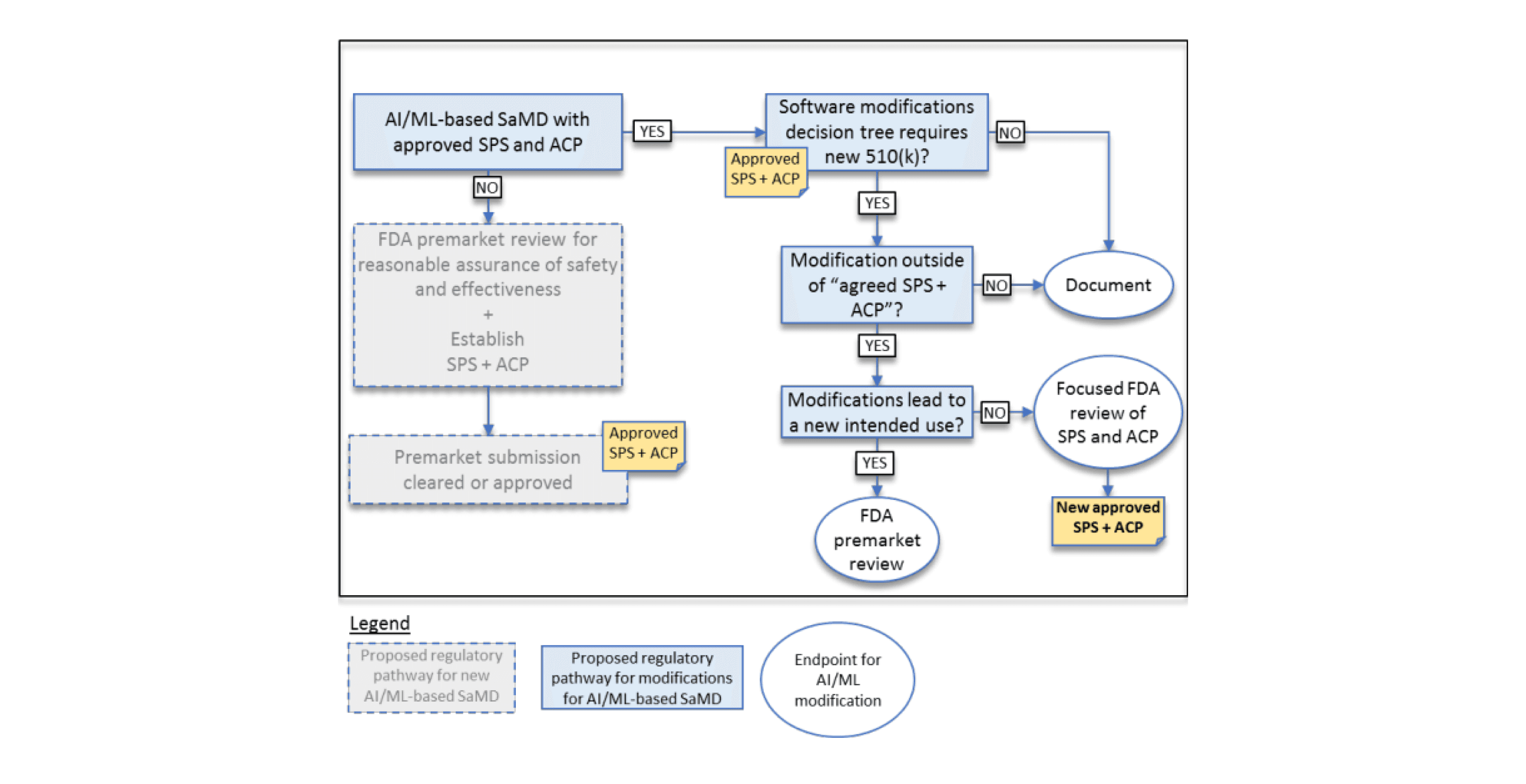
Image Source: FDA Proposed Regulatory Framework
Establishing a PCCP involves clearly defining the scope of anticipated modifications and documenting robust procedures in the Modification Protocol to ensure modifications remain safe and effective. The level of detail required may depend on the SaMD's risk category.
When submitting for marketing authorization, manufacturers can identify if they plan to utilise a PCCP by including the Description of Modifications and Modification Protocol sections in their submission. FDA's review and authorization of an acceptable PCCP would allow the manufacturer to implement certain types of modifications described in the plan without further FDA review, as long as they follow the specified protocols and document their modification activities.
The Description of Modifications section should define the goals and boundaries for what types of modifications may occur, such as performance enhancements, new data inputs, or refined intended uses. Providing clear examples is recommended.
The Modification Protocol should specify how the manufacturer will implement modifications reliably. It should cover:
1) Data management practices for curating, maintaining, and controlling training/test datasets
2) Re-training practices on when/how the algorithm will be re-trained
3) Performance evaluation protocols including metrics, analysis methods, predetermined performance targets
4) Update procedures for testing, deployment, versioning, and communication of algorithm updates
There must be traceability mapping the specific modification types described to the corresponding methods detailed in the Modification Protocol.
The PCCP framework aims to enable controlled iteration of AI/ML SaMD to realise performance improvements while enforcing pre-specified processes and guardrails to manage risks as the device evolves after initial marketing. Manufacturer adherence to approved PCCPs provides ongoing reasonable assurance of safety and effectiveness.
How can RagaAI help here?
Analysis of published resources shows RagaAI platform can help unlock tremendous values with regards to AI/ML compliance dimensions not only now but in future too.
Under the current overarching regulations RagaAI can help determine a locked SAMD and Good ML Practices, helping you achieve compliance with FDA.
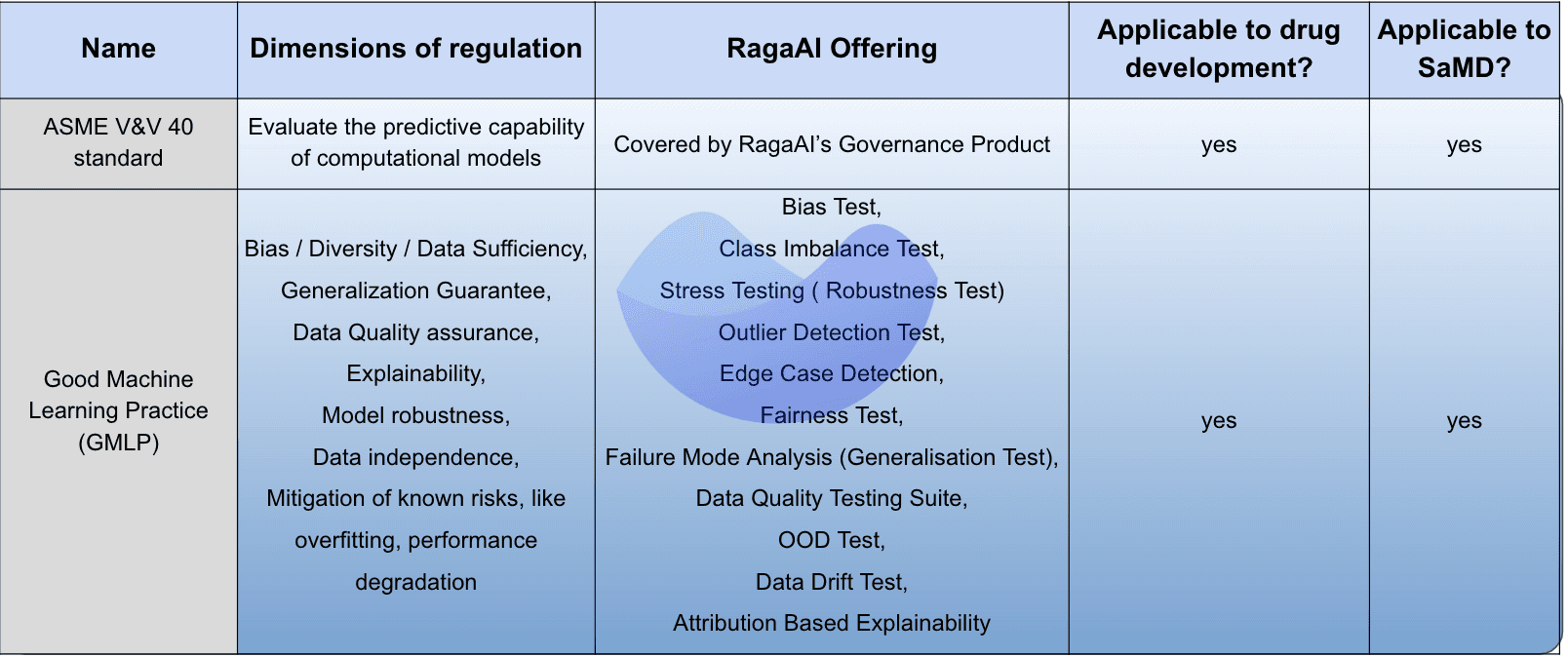
The above image shows mapping of RagaAI offerings against GMLP practices. Source: RagaAI
RagaAI also provides a concrete framework for complying with the practices for AI/ML in Drug Development, which have been formulated with a risk-based approach.
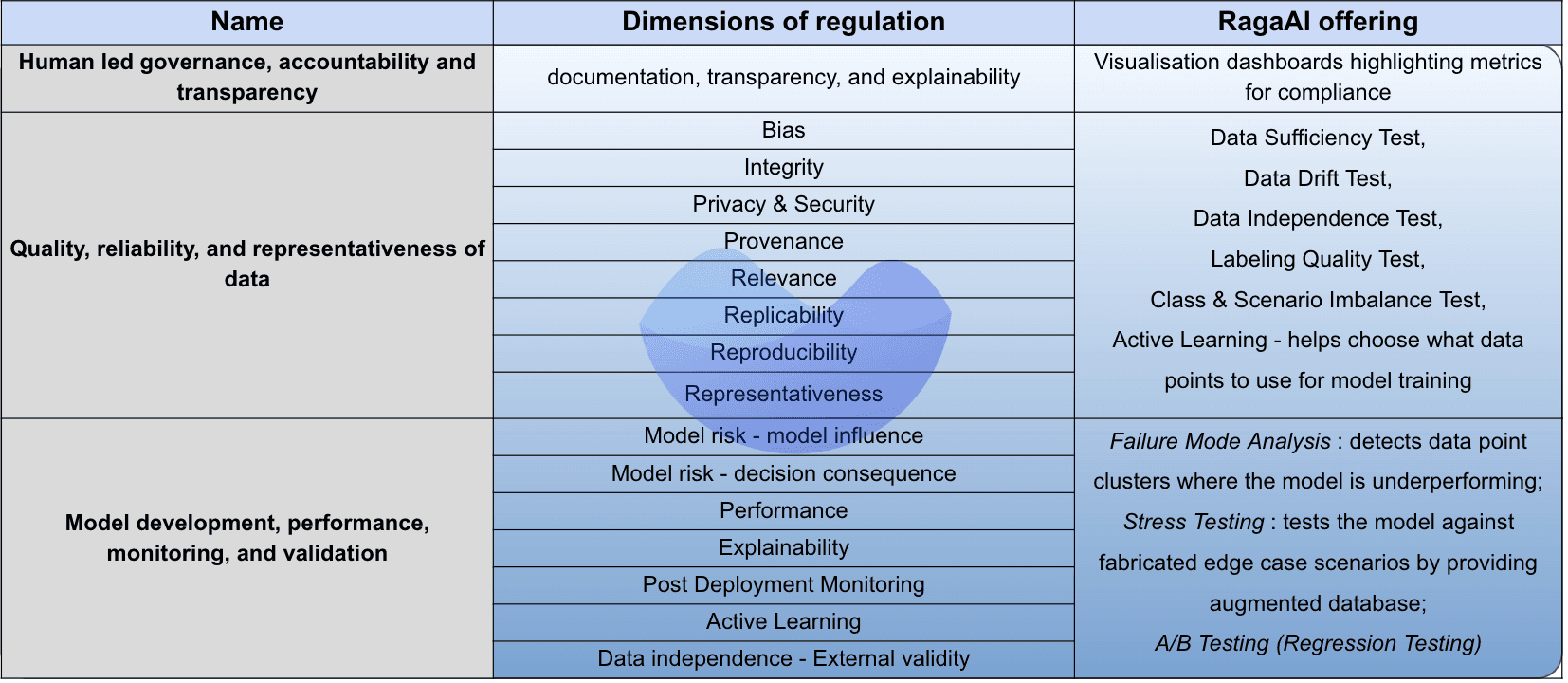
Image Source: RagaAI
The website docs enlist and meticulously present the various tests which can be used to comply with different aspects of FDA regulations for both drug development and SaMD.
Conclusion
While this article only covers the AI Compliance landscape in healthcare from the lens of FDA, there are various regulatory regimes being crafted across the globe. FDA, being on the top of healthcare regulations, provides the best view into how the healthcare industry can approach enabling safe and ethical use of AI in applications. As the healthcare industry is a very high-risk sector, the technology deployed at every step needs to be safe and repairable. Being highly comprehensive, this set of regulations can also be extrapolated, on a high level, to other acute-risk industries with ease.
It is natural to have a lot of questions in your mind after reading this, and unfortunately we cannot cover all the ground in this article.
But we wholeheartedly extend an invitation to share our expertise with whoever wants to take this wobbly ride of AI governance in Healthcare.
Get in touch with our Experts.
Want to know more ? Get in touch with our experts!
As we delve into the realm of cutting-edge artificial intelligence (AI) and machine learning (ML) technologies within the healthcare sector, it becomes imperative to navigate the regulatory landscape, particularly under the purview of the U.S. Food and Drug Administration (FDA). The FDA has recently released a series of discussion papers shedding light on their current perspectives and considerations surrounding the integration of AI/ML in healthcare.
Navigating the Regulatory Terrain
Software as a Medical Device (SaMD) constitutes software intended for medical purposes, independent of hardware medical devices. The International Medical Device Regulators Forum (IMDRF) delineates SaMD as software executing medical functions without being embedded in a physical device. Examples encompass mobile medical applications, clinical decision support software, and AI/ML-based algorithms for medical image analysis, diagnosis, or treatment recommendations.
Presently, AI/ML-based SaMD must adhere to the same regulatory prerequisites as traditional medical devices. This entails establishing a quality system (as per 21 CFR 820) and undergoing premarket review and clearance/approval by the FDA based on risk categorisation. The IMDRF has devised a risk categorisation framework stratifying SaMD into four categories (I-IV) contingent on the significance of the information provided and the healthcare situation's state.
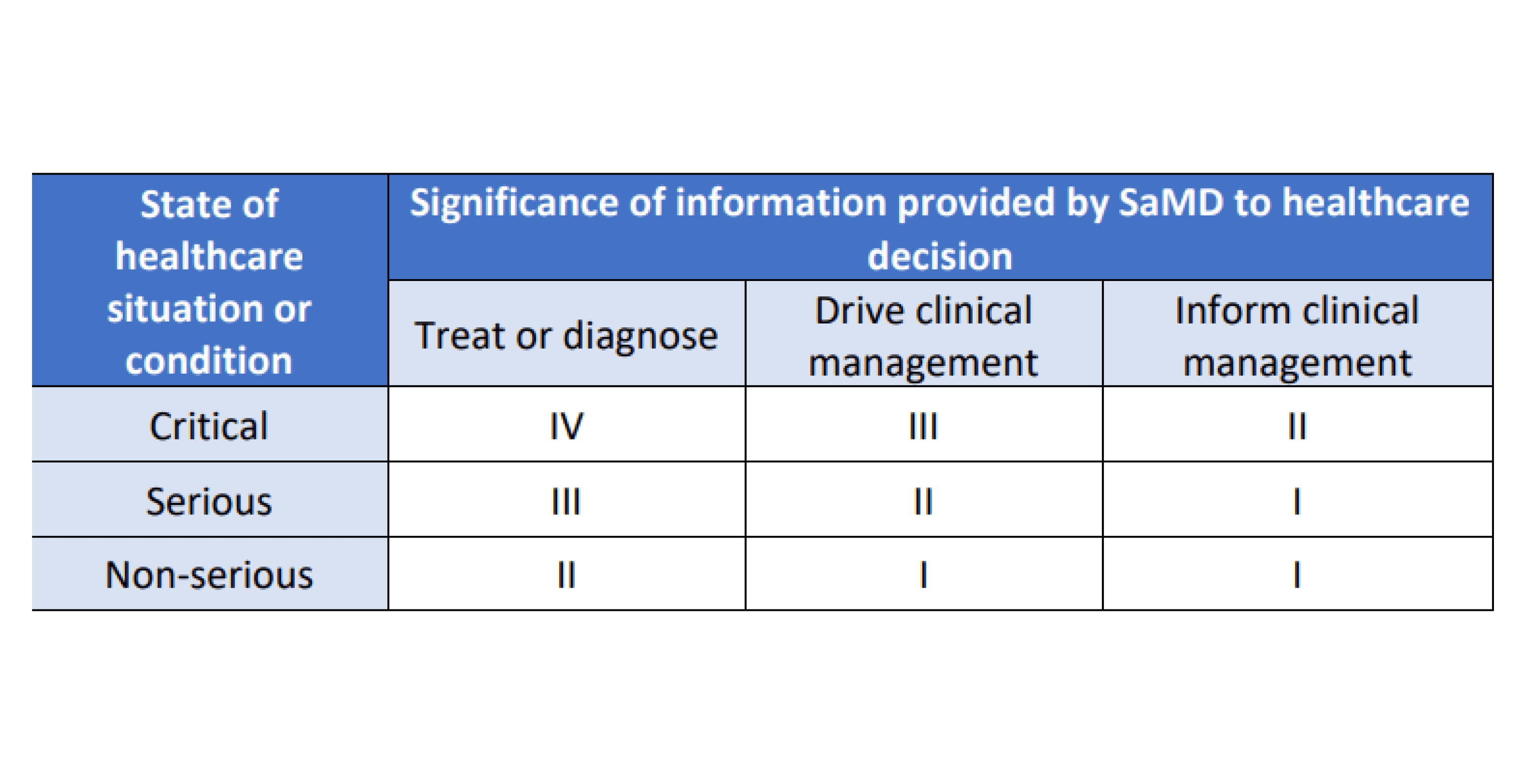
Image Source: FDA Proposed Regulatory Framework
For SaMD necessitating premarket submission (e.g., 510(k), De Novo, or PMA), the FDA typically reviews and clears/approves a "locked" AI/ML algorithm. A locked algorithm yields consistent output for a given set of inputs and remains static over time. Substantial alterations to the locked algorithm generally mandate a new premarket submission and FDA review.
However, this framework isn't ideally suited for the dynamic nature of AI/ML algorithms, which continuously learn and adapt post-market. The iterative, autonomous, and adaptive attributes necessitate a novel regulatory approach facilitating swift product enhancement cycles while upholding safety and effectiveness standards.
Recognising the Potential and Crafting Future Regulations
The FDA acknowledges the transformative potential of AI/ML-based SaMD in healthcare, envisaging advancements in disease detection, diagnosis accuracy, and personalised therapeutics. However, the current regulatory paradigm presents a challenge in balancing the benefits of continual learning with the imperative of regulatory oversight to mitigate patient risks.
In response, the FDA has proposed a novel Total Product Lifecycle (TPLC) regulatory approach. This approach evaluates a company's culture of quality and organisational excellence, ensuring high-quality software development, testing, and performance monitoring. Key tenets of this approach include:
Establishing clear expectations regarding quality systems and good machine learning practices (GMLP)
Image Source: FDA Proposed Regulatory Framework
Conducting premarket review with optional submission of predetermined modification plans by manufacturers.
Mandating manufacturers to monitor and manage modification risks per predetermined plans.
Enabling transparency and real-world performance reporting.
Central to this approach is the concept of a "predetermined change control plan" (PCCP), which manufacturers can submit during premarket review. The PCCP delineates anticipated modifications and protocols for implementation and validation, facilitating controlled iteration while ensuring safety and effectiveness.
Crafting a Predetermined Change Control Plan
The report proposes that a PCCP consists of three main components:
1) a detailed description of planned modifications
2) a modification protocol outlining verification/validation activities for the modifications, and
3) an impact assessment evaluating the benefits/risks of the modifications.
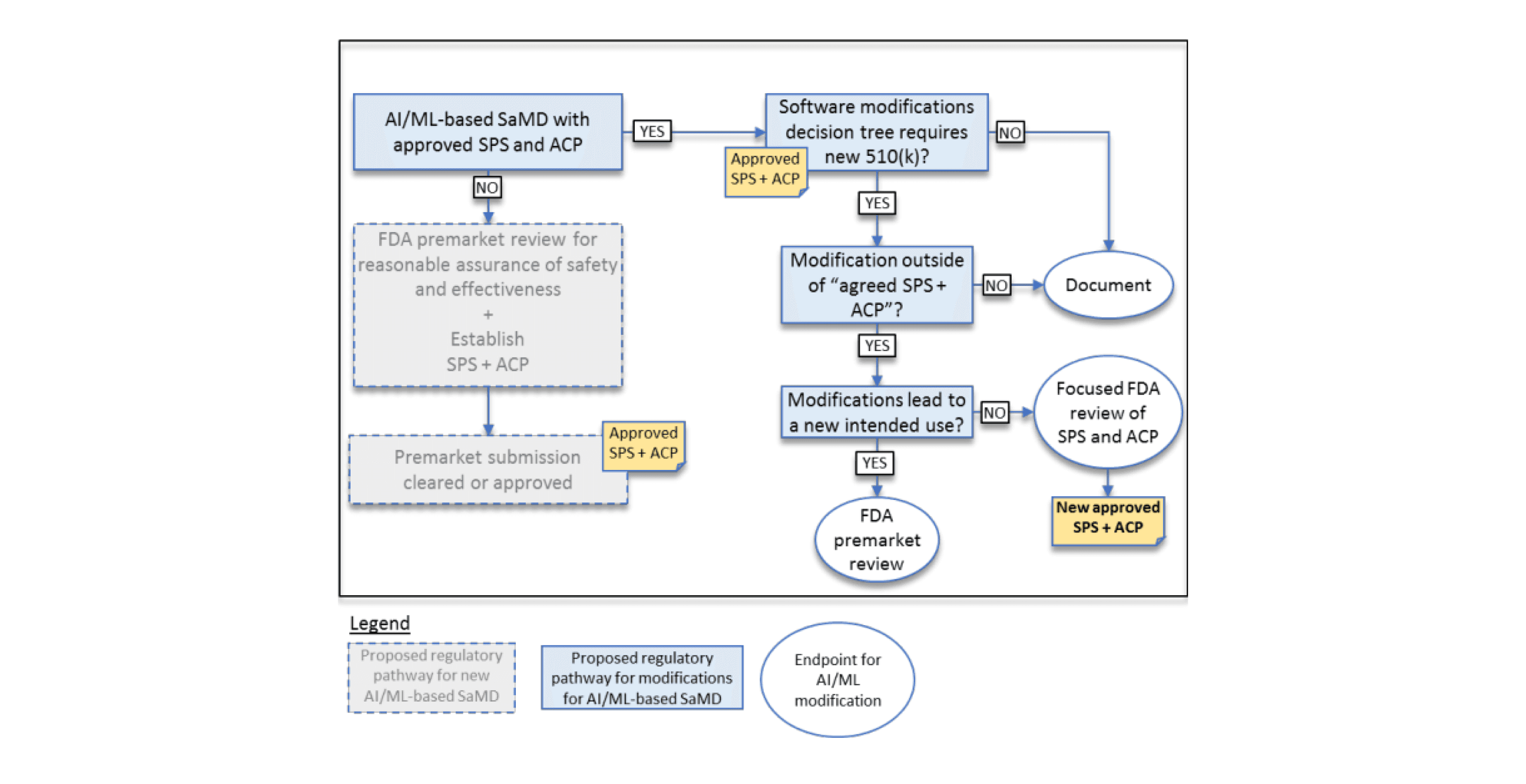
Image Source: FDA Proposed Regulatory Framework
Establishing a PCCP involves clearly defining the scope of anticipated modifications and documenting robust procedures in the Modification Protocol to ensure modifications remain safe and effective. The level of detail required may depend on the SaMD's risk category.
When submitting for marketing authorization, manufacturers can identify if they plan to utilise a PCCP by including the Description of Modifications and Modification Protocol sections in their submission. FDA's review and authorization of an acceptable PCCP would allow the manufacturer to implement certain types of modifications described in the plan without further FDA review, as long as they follow the specified protocols and document their modification activities.
The Description of Modifications section should define the goals and boundaries for what types of modifications may occur, such as performance enhancements, new data inputs, or refined intended uses. Providing clear examples is recommended.
The Modification Protocol should specify how the manufacturer will implement modifications reliably. It should cover:
1) Data management practices for curating, maintaining, and controlling training/test datasets
2) Re-training practices on when/how the algorithm will be re-trained
3) Performance evaluation protocols including metrics, analysis methods, predetermined performance targets
4) Update procedures for testing, deployment, versioning, and communication of algorithm updates
There must be traceability mapping the specific modification types described to the corresponding methods detailed in the Modification Protocol.
The PCCP framework aims to enable controlled iteration of AI/ML SaMD to realise performance improvements while enforcing pre-specified processes and guardrails to manage risks as the device evolves after initial marketing. Manufacturer adherence to approved PCCPs provides ongoing reasonable assurance of safety and effectiveness.
How can RagaAI help here?
Analysis of published resources shows RagaAI platform can help unlock tremendous values with regards to AI/ML compliance dimensions not only now but in future too.
Under the current overarching regulations RagaAI can help determine a locked SAMD and Good ML Practices, helping you achieve compliance with FDA.
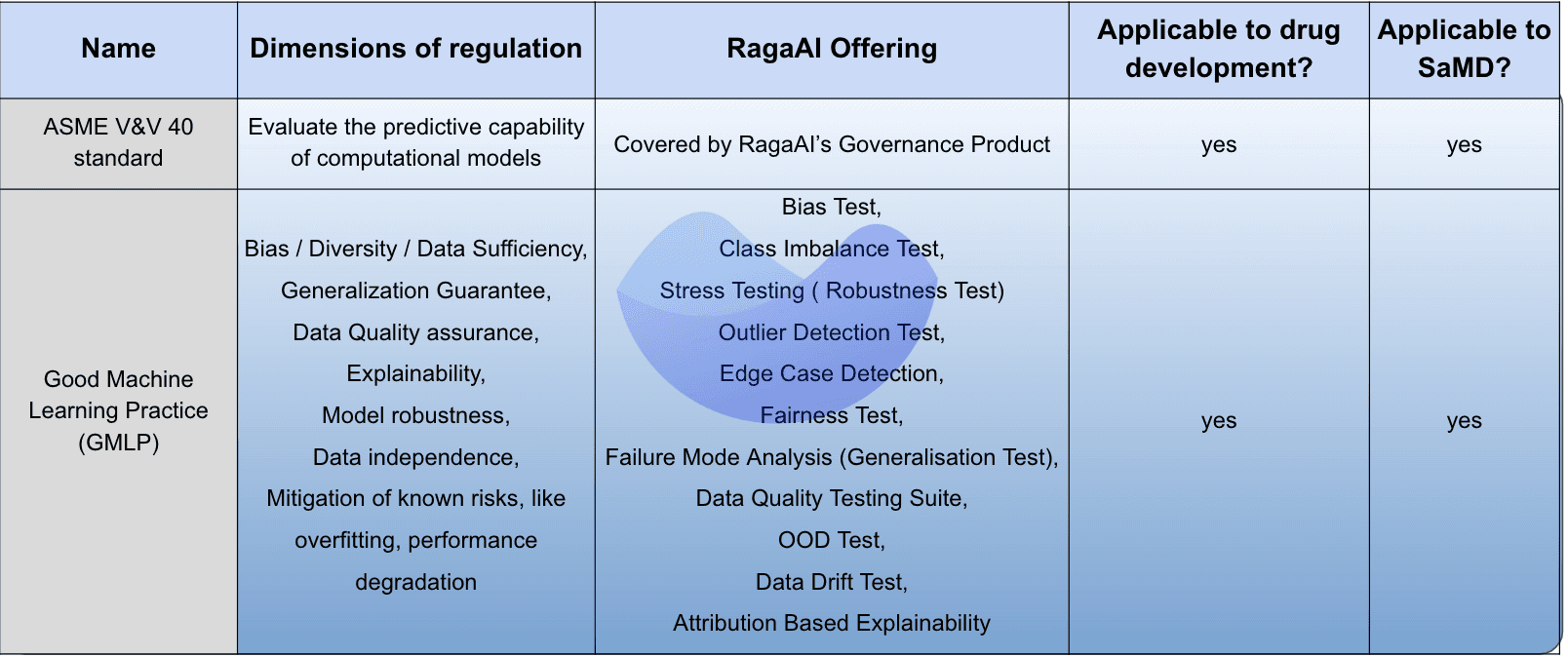
The above image shows mapping of RagaAI offerings against GMLP practices. Source: RagaAI
RagaAI also provides a concrete framework for complying with the practices for AI/ML in Drug Development, which have been formulated with a risk-based approach.
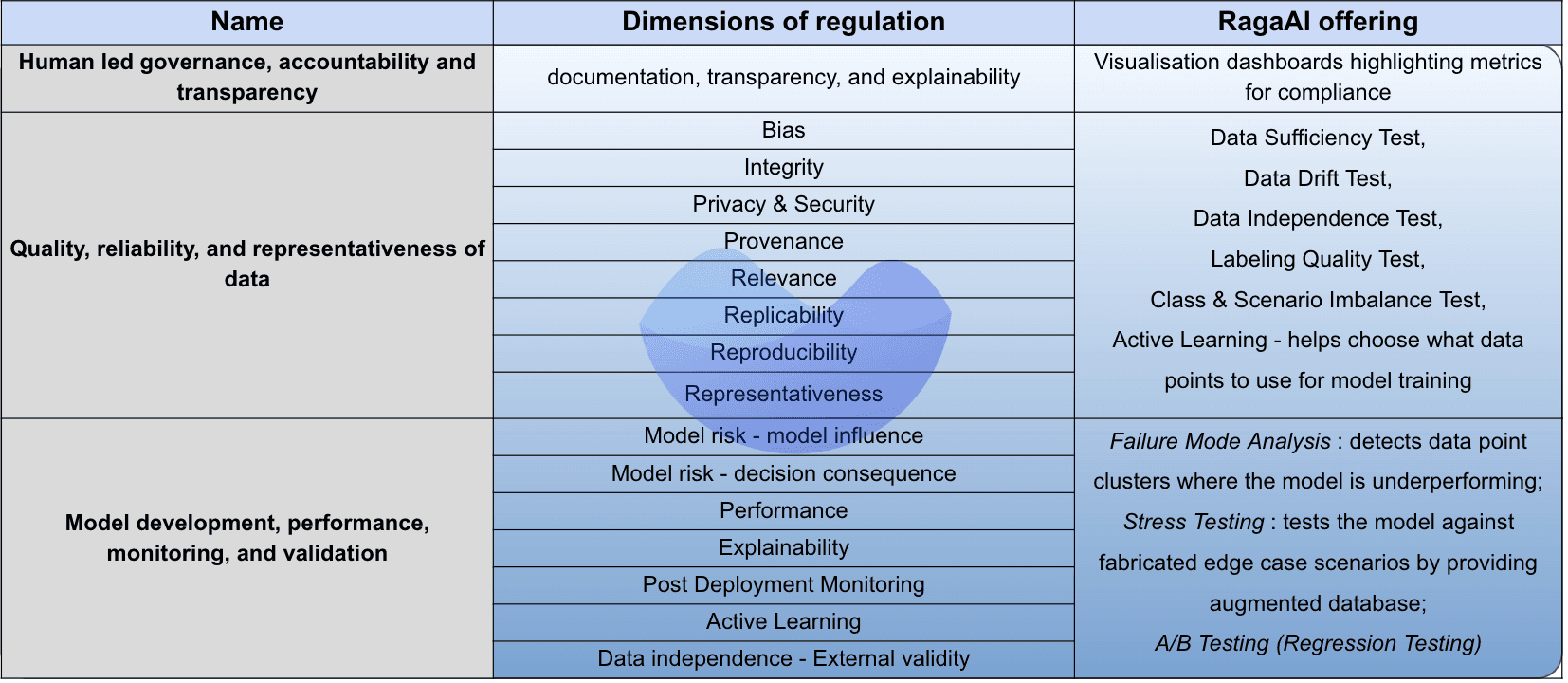
Image Source: RagaAI
The website docs enlist and meticulously present the various tests which can be used to comply with different aspects of FDA regulations for both drug development and SaMD.
Conclusion
While this article only covers the AI Compliance landscape in healthcare from the lens of FDA, there are various regulatory regimes being crafted across the globe. FDA, being on the top of healthcare regulations, provides the best view into how the healthcare industry can approach enabling safe and ethical use of AI in applications. As the healthcare industry is a very high-risk sector, the technology deployed at every step needs to be safe and repairable. Being highly comprehensive, this set of regulations can also be extrapolated, on a high level, to other acute-risk industries with ease.
It is natural to have a lot of questions in your mind after reading this, and unfortunately we cannot cover all the ground in this article.
But we wholeheartedly extend an invitation to share our expertise with whoever wants to take this wobbly ride of AI governance in Healthcare.
Get in touch with our Experts.
Want to know more ? Get in touch with our experts!
As we delve into the realm of cutting-edge artificial intelligence (AI) and machine learning (ML) technologies within the healthcare sector, it becomes imperative to navigate the regulatory landscape, particularly under the purview of the U.S. Food and Drug Administration (FDA). The FDA has recently released a series of discussion papers shedding light on their current perspectives and considerations surrounding the integration of AI/ML in healthcare.
Navigating the Regulatory Terrain
Software as a Medical Device (SaMD) constitutes software intended for medical purposes, independent of hardware medical devices. The International Medical Device Regulators Forum (IMDRF) delineates SaMD as software executing medical functions without being embedded in a physical device. Examples encompass mobile medical applications, clinical decision support software, and AI/ML-based algorithms for medical image analysis, diagnosis, or treatment recommendations.
Presently, AI/ML-based SaMD must adhere to the same regulatory prerequisites as traditional medical devices. This entails establishing a quality system (as per 21 CFR 820) and undergoing premarket review and clearance/approval by the FDA based on risk categorisation. The IMDRF has devised a risk categorisation framework stratifying SaMD into four categories (I-IV) contingent on the significance of the information provided and the healthcare situation's state.
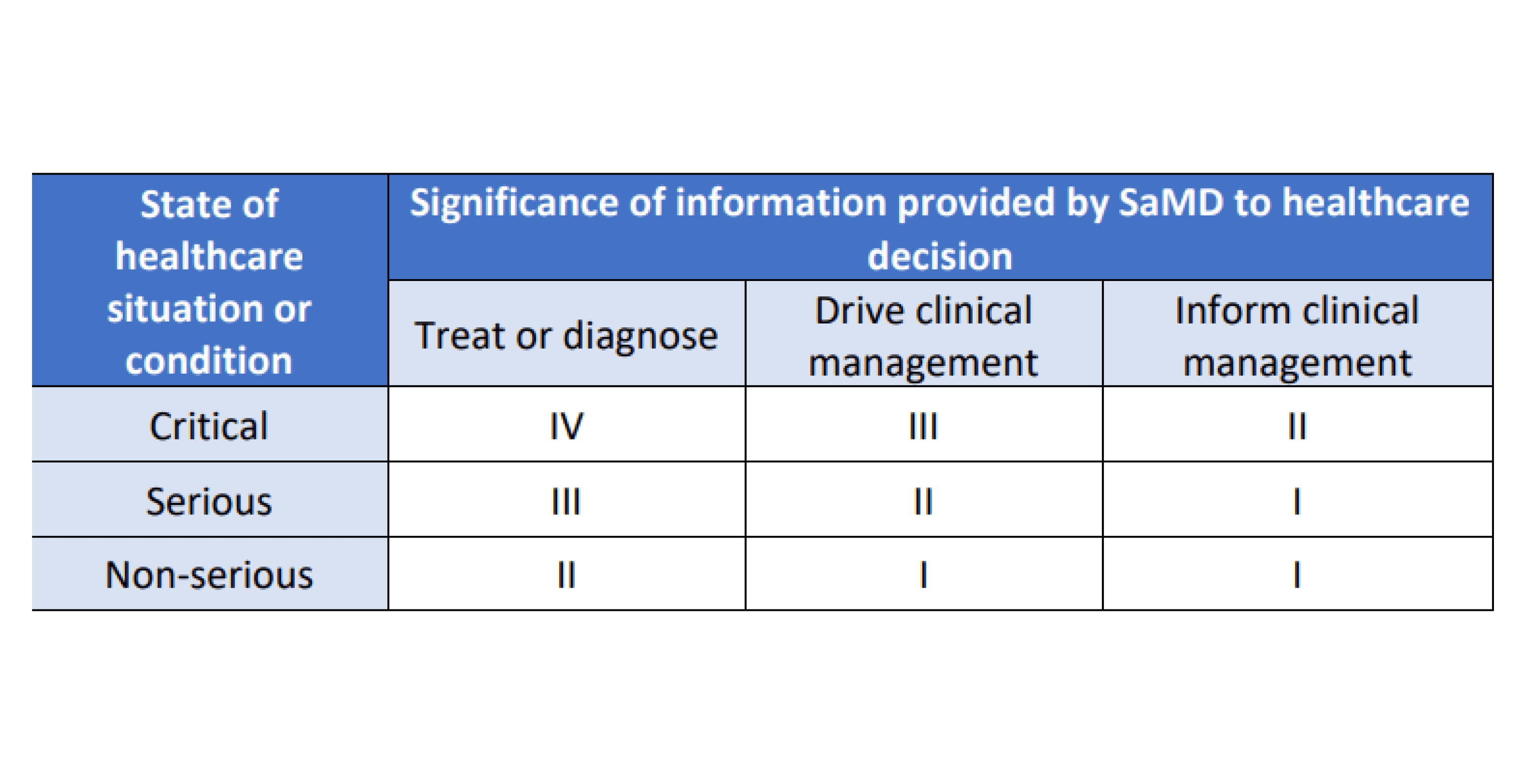
Image Source: FDA Proposed Regulatory Framework
For SaMD necessitating premarket submission (e.g., 510(k), De Novo, or PMA), the FDA typically reviews and clears/approves a "locked" AI/ML algorithm. A locked algorithm yields consistent output for a given set of inputs and remains static over time. Substantial alterations to the locked algorithm generally mandate a new premarket submission and FDA review.
However, this framework isn't ideally suited for the dynamic nature of AI/ML algorithms, which continuously learn and adapt post-market. The iterative, autonomous, and adaptive attributes necessitate a novel regulatory approach facilitating swift product enhancement cycles while upholding safety and effectiveness standards.
Recognising the Potential and Crafting Future Regulations
The FDA acknowledges the transformative potential of AI/ML-based SaMD in healthcare, envisaging advancements in disease detection, diagnosis accuracy, and personalised therapeutics. However, the current regulatory paradigm presents a challenge in balancing the benefits of continual learning with the imperative of regulatory oversight to mitigate patient risks.
In response, the FDA has proposed a novel Total Product Lifecycle (TPLC) regulatory approach. This approach evaluates a company's culture of quality and organisational excellence, ensuring high-quality software development, testing, and performance monitoring. Key tenets of this approach include:
Establishing clear expectations regarding quality systems and good machine learning practices (GMLP)
Image Source: FDA Proposed Regulatory Framework
Conducting premarket review with optional submission of predetermined modification plans by manufacturers.
Mandating manufacturers to monitor and manage modification risks per predetermined plans.
Enabling transparency and real-world performance reporting.
Central to this approach is the concept of a "predetermined change control plan" (PCCP), which manufacturers can submit during premarket review. The PCCP delineates anticipated modifications and protocols for implementation and validation, facilitating controlled iteration while ensuring safety and effectiveness.
Crafting a Predetermined Change Control Plan
The report proposes that a PCCP consists of three main components:
1) a detailed description of planned modifications
2) a modification protocol outlining verification/validation activities for the modifications, and
3) an impact assessment evaluating the benefits/risks of the modifications.
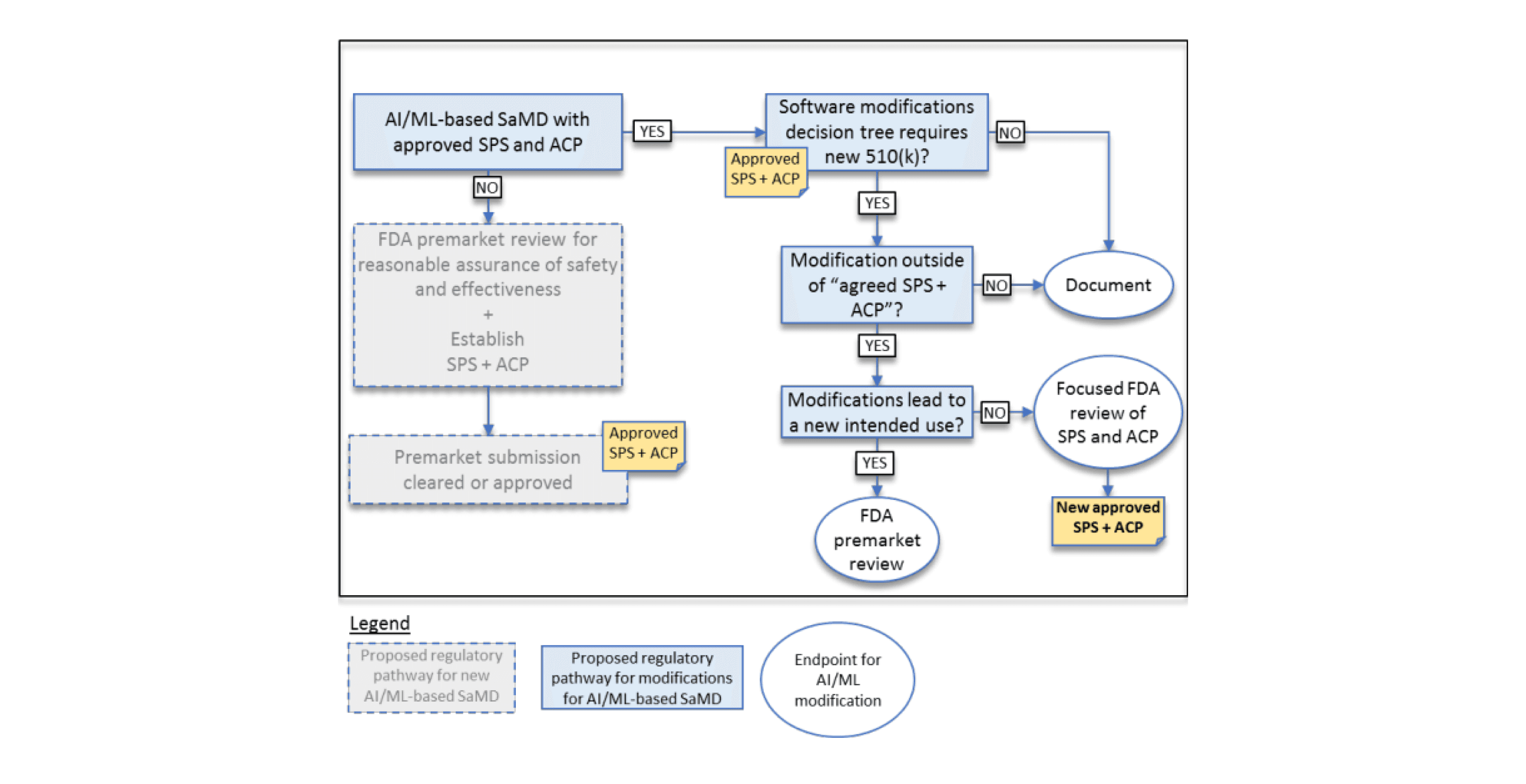
Image Source: FDA Proposed Regulatory Framework
Establishing a PCCP involves clearly defining the scope of anticipated modifications and documenting robust procedures in the Modification Protocol to ensure modifications remain safe and effective. The level of detail required may depend on the SaMD's risk category.
When submitting for marketing authorization, manufacturers can identify if they plan to utilise a PCCP by including the Description of Modifications and Modification Protocol sections in their submission. FDA's review and authorization of an acceptable PCCP would allow the manufacturer to implement certain types of modifications described in the plan without further FDA review, as long as they follow the specified protocols and document their modification activities.
The Description of Modifications section should define the goals and boundaries for what types of modifications may occur, such as performance enhancements, new data inputs, or refined intended uses. Providing clear examples is recommended.
The Modification Protocol should specify how the manufacturer will implement modifications reliably. It should cover:
1) Data management practices for curating, maintaining, and controlling training/test datasets
2) Re-training practices on when/how the algorithm will be re-trained
3) Performance evaluation protocols including metrics, analysis methods, predetermined performance targets
4) Update procedures for testing, deployment, versioning, and communication of algorithm updates
There must be traceability mapping the specific modification types described to the corresponding methods detailed in the Modification Protocol.
The PCCP framework aims to enable controlled iteration of AI/ML SaMD to realise performance improvements while enforcing pre-specified processes and guardrails to manage risks as the device evolves after initial marketing. Manufacturer adherence to approved PCCPs provides ongoing reasonable assurance of safety and effectiveness.
How can RagaAI help here?
Analysis of published resources shows RagaAI platform can help unlock tremendous values with regards to AI/ML compliance dimensions not only now but in future too.
Under the current overarching regulations RagaAI can help determine a locked SAMD and Good ML Practices, helping you achieve compliance with FDA.
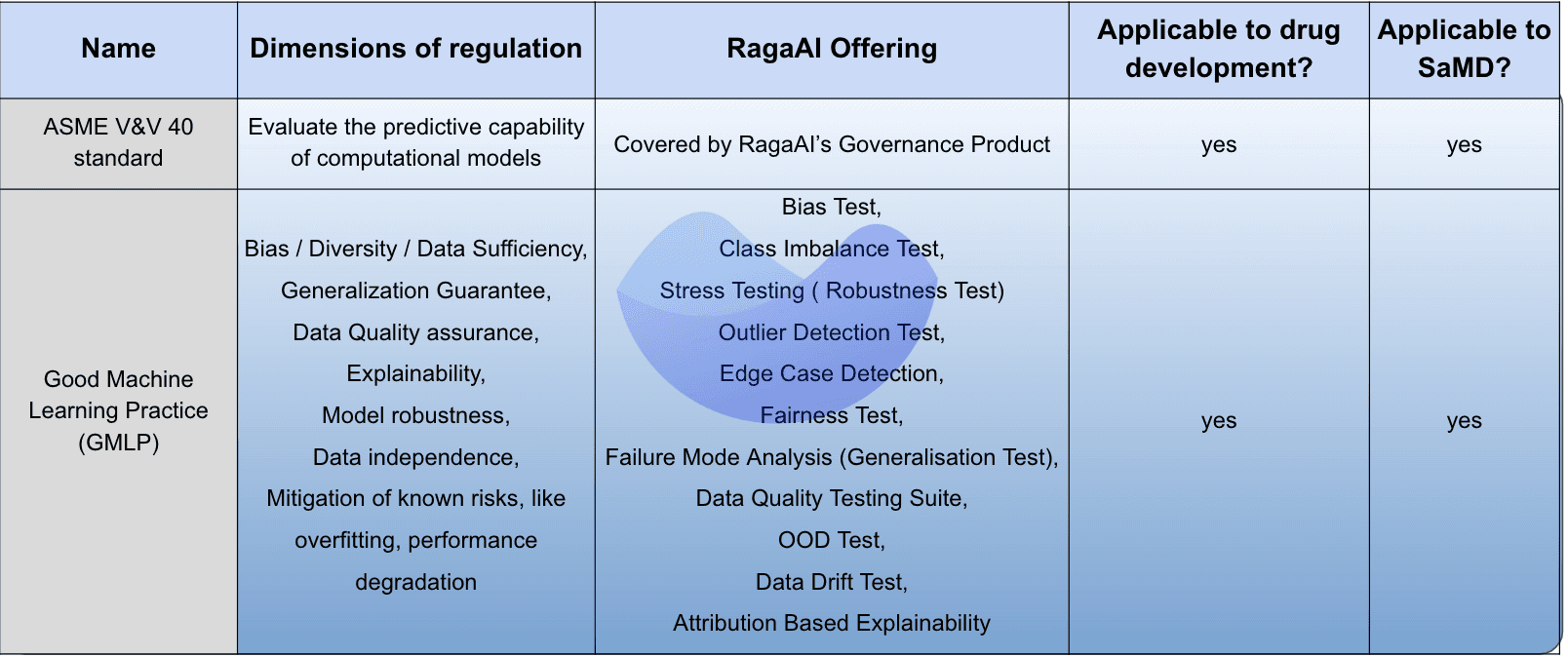
The above image shows mapping of RagaAI offerings against GMLP practices. Source: RagaAI
RagaAI also provides a concrete framework for complying with the practices for AI/ML in Drug Development, which have been formulated with a risk-based approach.
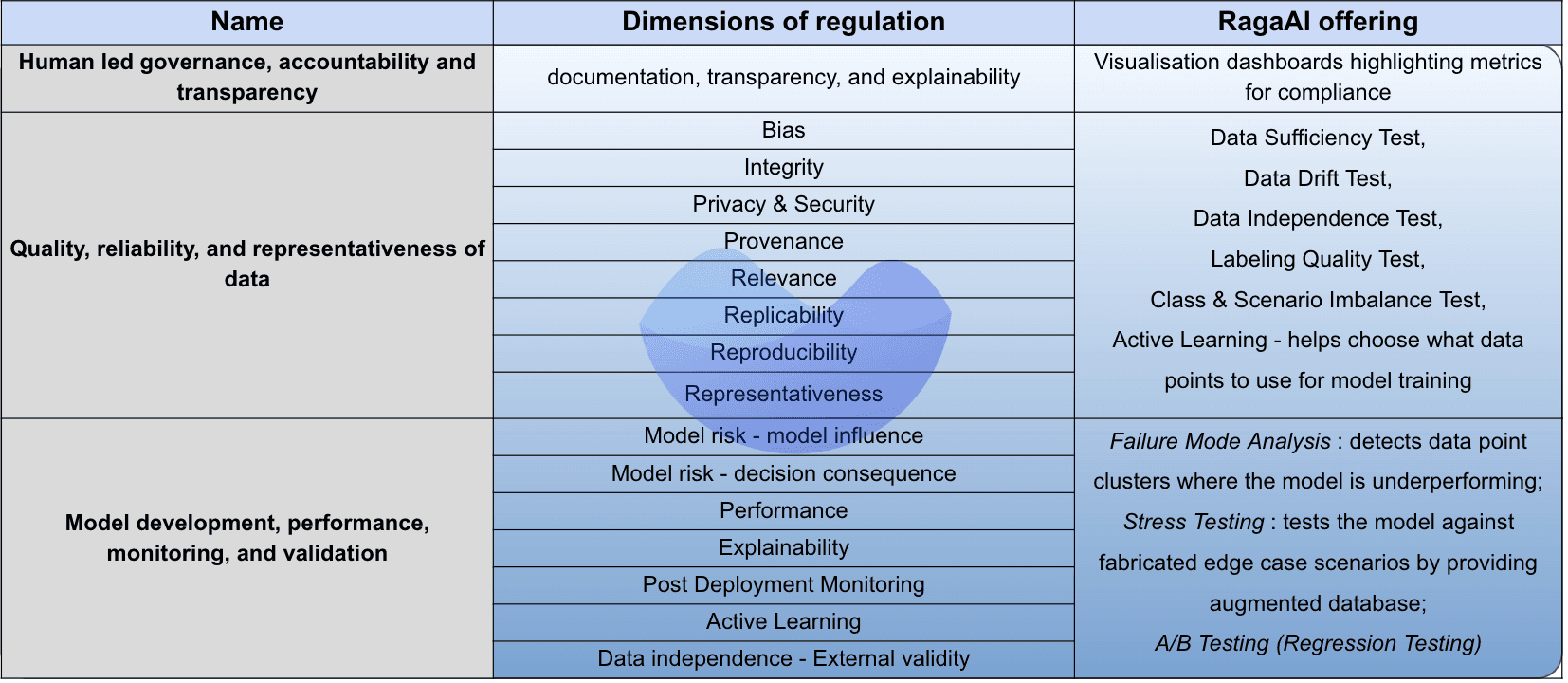
Image Source: RagaAI
The website docs enlist and meticulously present the various tests which can be used to comply with different aspects of FDA regulations for both drug development and SaMD.
Conclusion
While this article only covers the AI Compliance landscape in healthcare from the lens of FDA, there are various regulatory regimes being crafted across the globe. FDA, being on the top of healthcare regulations, provides the best view into how the healthcare industry can approach enabling safe and ethical use of AI in applications. As the healthcare industry is a very high-risk sector, the technology deployed at every step needs to be safe and repairable. Being highly comprehensive, this set of regulations can also be extrapolated, on a high level, to other acute-risk industries with ease.
It is natural to have a lot of questions in your mind after reading this, and unfortunately we cannot cover all the ground in this article.
But we wholeheartedly extend an invitation to share our expertise with whoever wants to take this wobbly ride of AI governance in Healthcare.
Get in touch with our Experts.
Want to know more ? Get in touch with our experts!
Subscribe to our newsletter to never miss an update
Other articles


Agents Talk, Models Think: A2A + MCP for Enterprise Agentic AI

Riya Parikh, Nitai Agarwal
Apr 27, 2025
Read the article
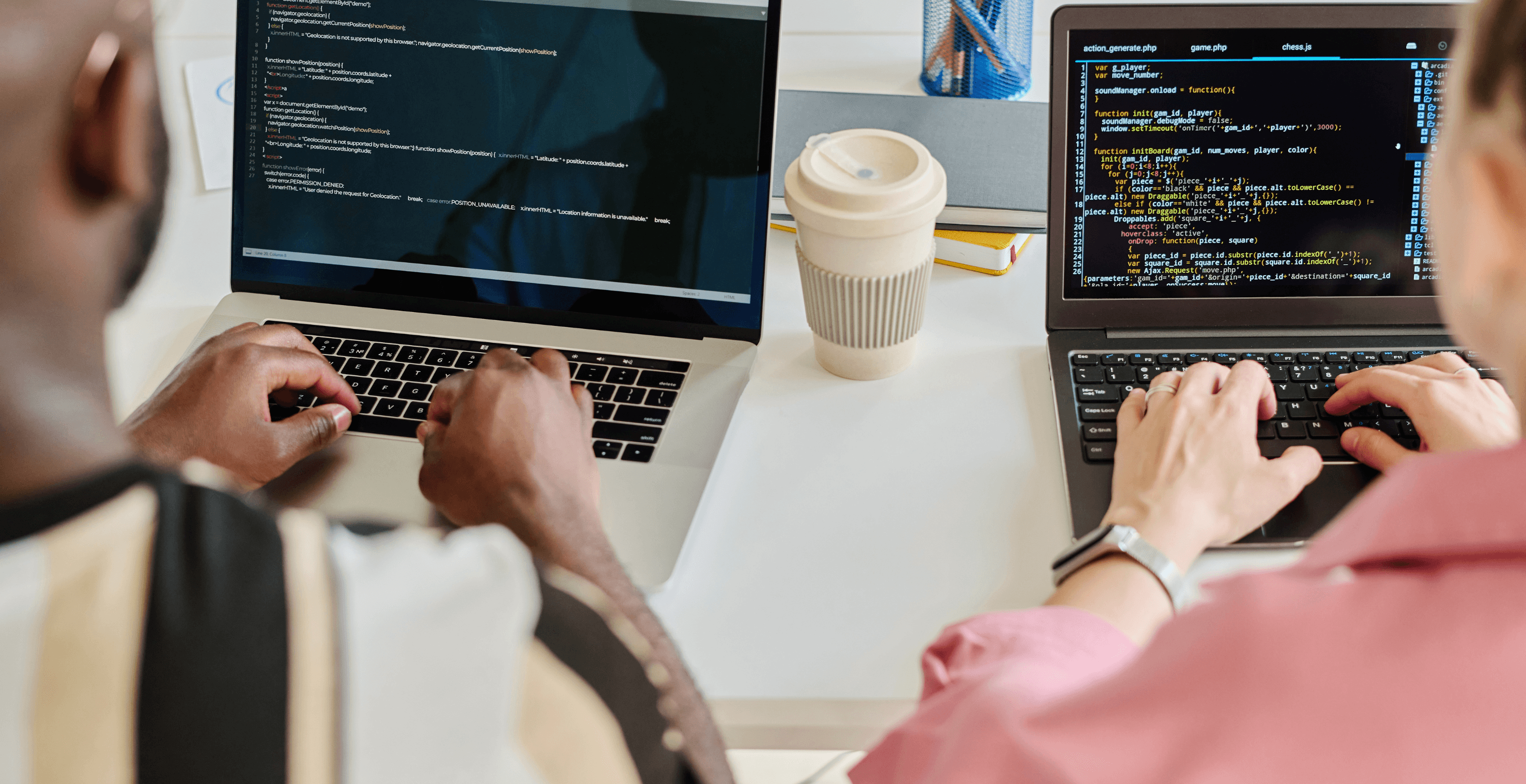
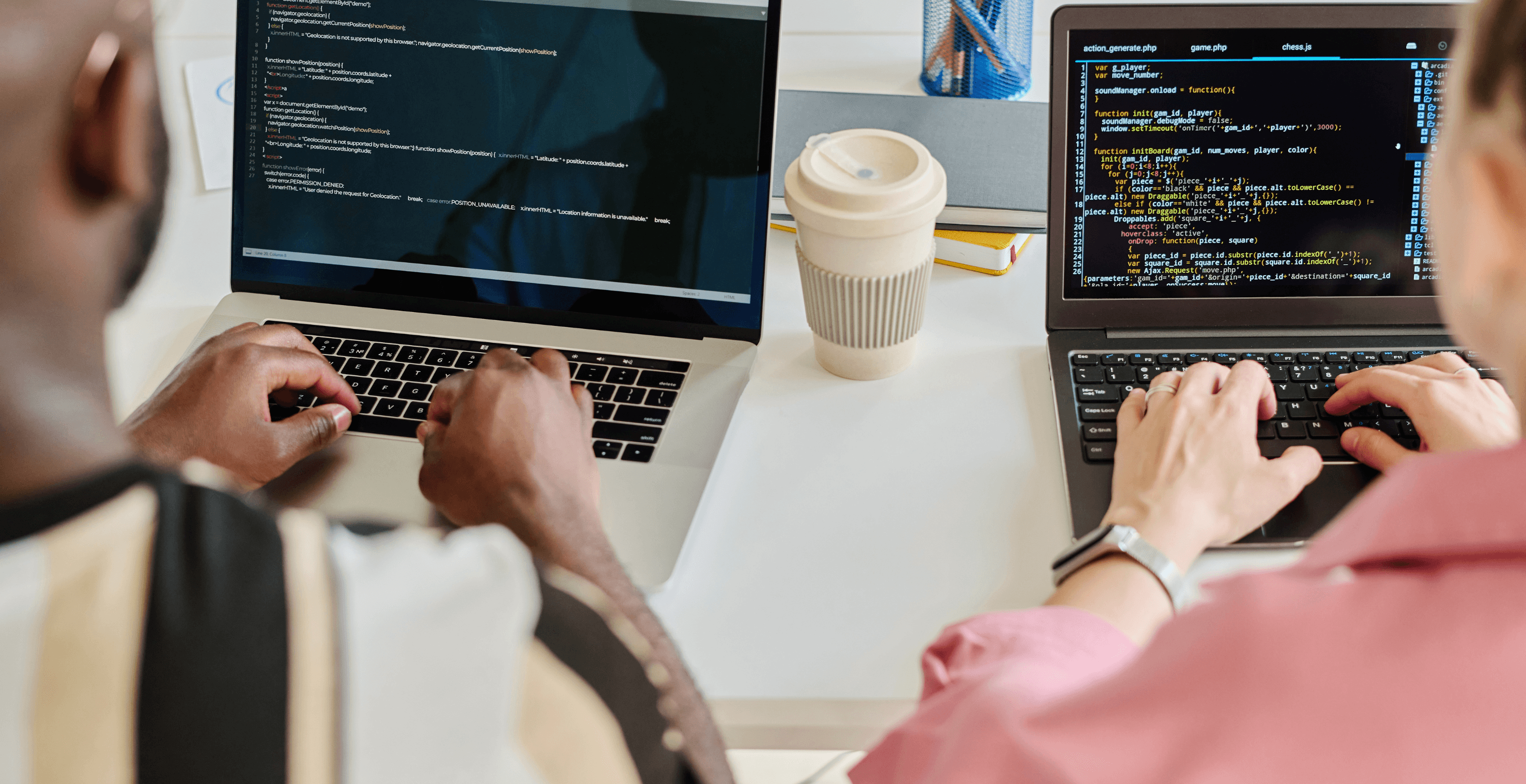
Understanding NeMo Guardrails: A Toolkit for LLM Security

Rehan Asif
Dec 24, 2024
Read the article


Understanding Differences in Large vs Small Language Models (LLM vs SLM)

Rehan Asif
Dec 21, 2024
Read the article