Using Synthetic Data To Enrich RAG Applications
Jigar Gupta
Jul 13, 2024
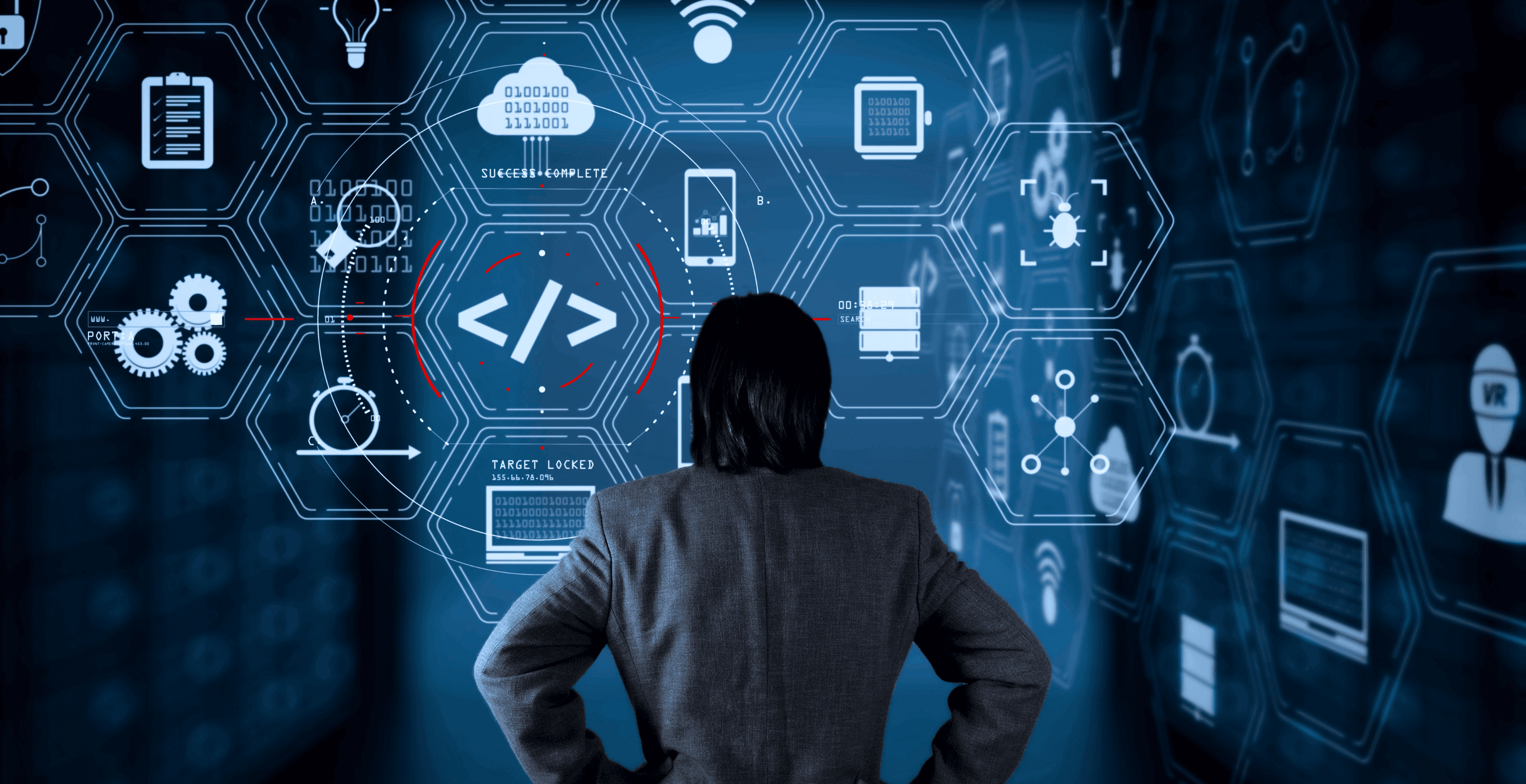
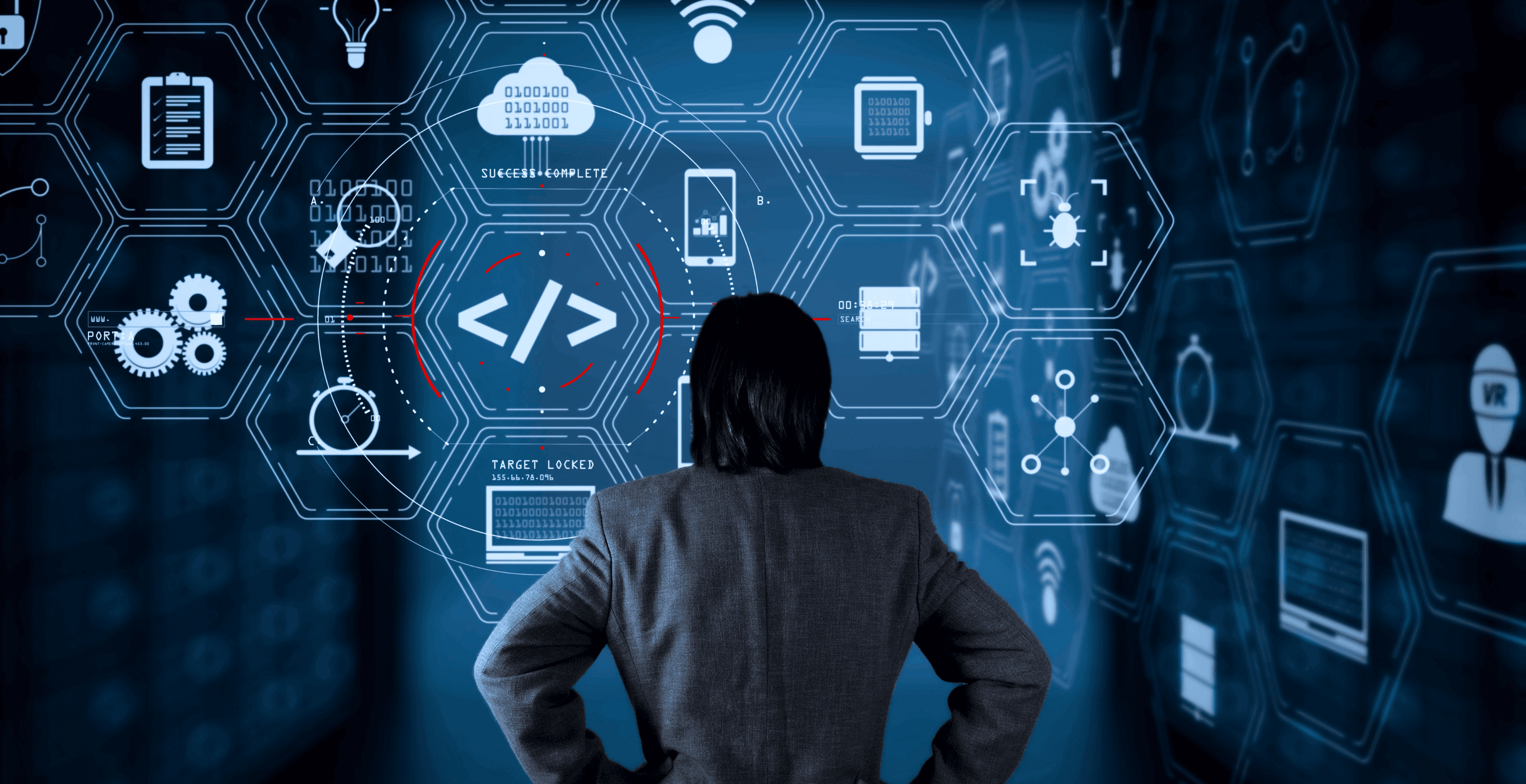
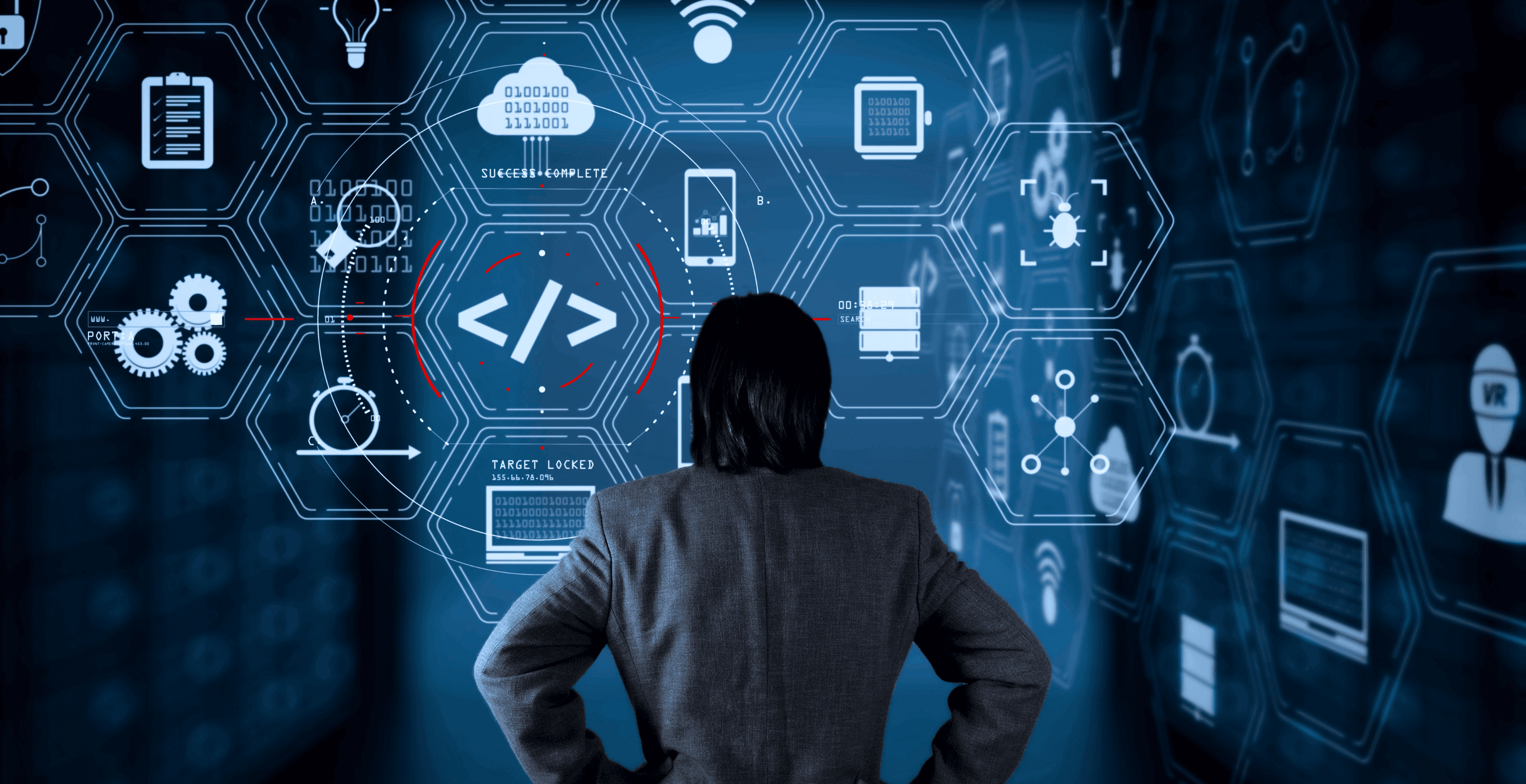
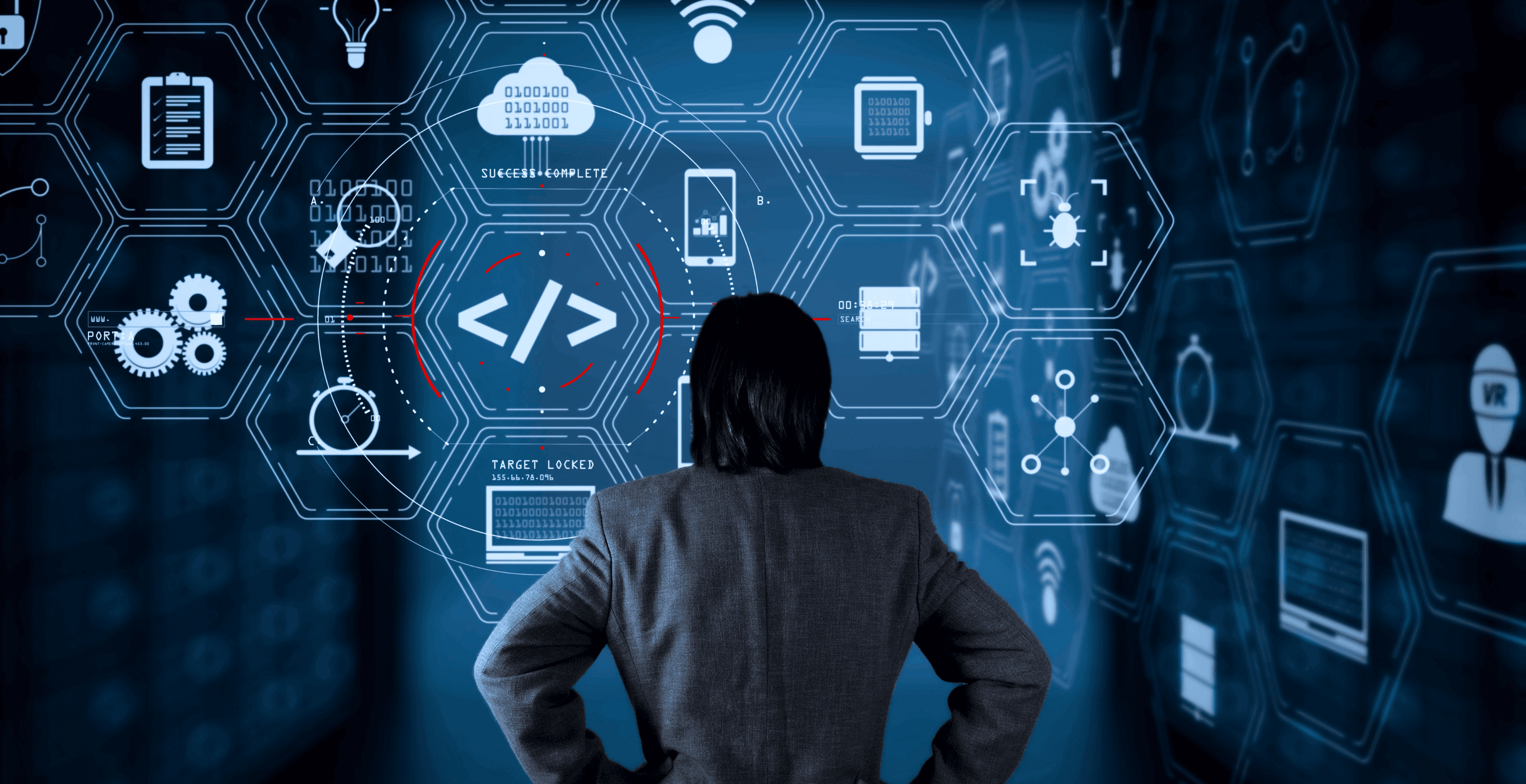
Retrieval-Augmented Generation (RAG) applications have transformed natural language processing (NLP) by unifying retrieval and generation methods to produce more precise and contextually pertinent responses. However, these applications often brawl with the challenges of limited or partial training data. This can hamper their performance and dependability. Here’s where artificial data comes into play. By generating synthetic data, you can acknowledge these issues, making your RAG applications more rigid and efficient. Synthetic data isn’t just a substitute; it is a strategic tool to improve data enrichment.
Overview of Synthetic Data Generation Techniques
Rule-Based Techniques
Template-Based Generation:
Template-based generation allows for the creation of artificial data by defining a set of predefined templates. These templates act as outlines, permitting you to create data specimens by populating the templates with pertinent values. This technique is direct and ensures correspondence and framework in the generated data. For example, in generating artificial customer profiles, you might utilize a template such as “Name: {First Name} {Last Name}, Age: {Age}, Email: {Email},” where the substitutes are replaced with values from a predefined list or a random generator.
Grammar-Based Generation:
Grammar-based generation indulges using formal grammars to produce artificial data generation. Defining a set of grammatical regulations describes the framework of your data, and generating data iteratively applies these regulations. This approach is specifically useful when you are required to generate syntactically intricate data, like programming code or natural language sentences. For instance, you might define a grammar for producing arithmetic expressions, citing rules for operators and operands, which can then generate an assortment of logical expressions.
Domain-Specific Synthetic Data Generation
Domain-Specific synthetic data generation involves creating artificial data customized to a concrete field or industry. This information mirrors real-world synopsis, helping ventures and investigators perform inspection, testing and model training without the restrictions and privacy concerns linked with actual data. It uses advanced algorithms and methods to counterfeit data that firmly resembles the attributes and motifs found within a precise domain, like e-commerce, healthcare and finance.
Machine-Learning Based Techniques
Generative Adversarial Networks (GANs)
Generative Adversarial Networks (GANs) provide a precise technique for artificial data creation. Two sensory networks, a generator and a discriminator, contend against each other. The generator generates artificial data, while the discriminator assesses its genuineness. Through this conflicting process, the generator enhances its ability to generate pragmatic data. GANs have shown phenomenal success in creating high-quality images, but they can also be applied to other kinds of data, like text and audio.
Variational Autoencoders (VAEs)
Variational Autoencoders (VAEs) are another striking method for producing artificial data. Training a VAE involves encrypting input data into a dormant space and then decrypting it back into the original formation. During this procedure, the VAE learns to create new data points by selecting from the inactive space. VAEs are specifically useful for creating data with constant variations and can be applied to tasks like image combination and anomaly detection.
Language Models and Text Generation
One highly uses language models, like GPT-3, for text generation. Training these models on a huge corpora of text data permits them to create coherent and conditionally pertinent text. By refining the model on precise datasets, you can generate artificial text that imitates the style and content of the training data. This method is precious for tasks such as automated content creation, chatbot replies, and NLP applications.
Hybrid Approaches Combining Rules and Machine Learning
Hybrid approaches use the strengths of both restrained-based and machine learning methods. Unifying predefined regulations with machine learning models produces artificial data that follows precise restrictions while handling changeability and realism. For example, you might incorporate a grammar-based technique to depict the framework of the data and then engage a GAN or VAE to fill in the details, ensuring both compatibility and variety in the produced data.
Evaluation Metrics for Synthetic Data Quality
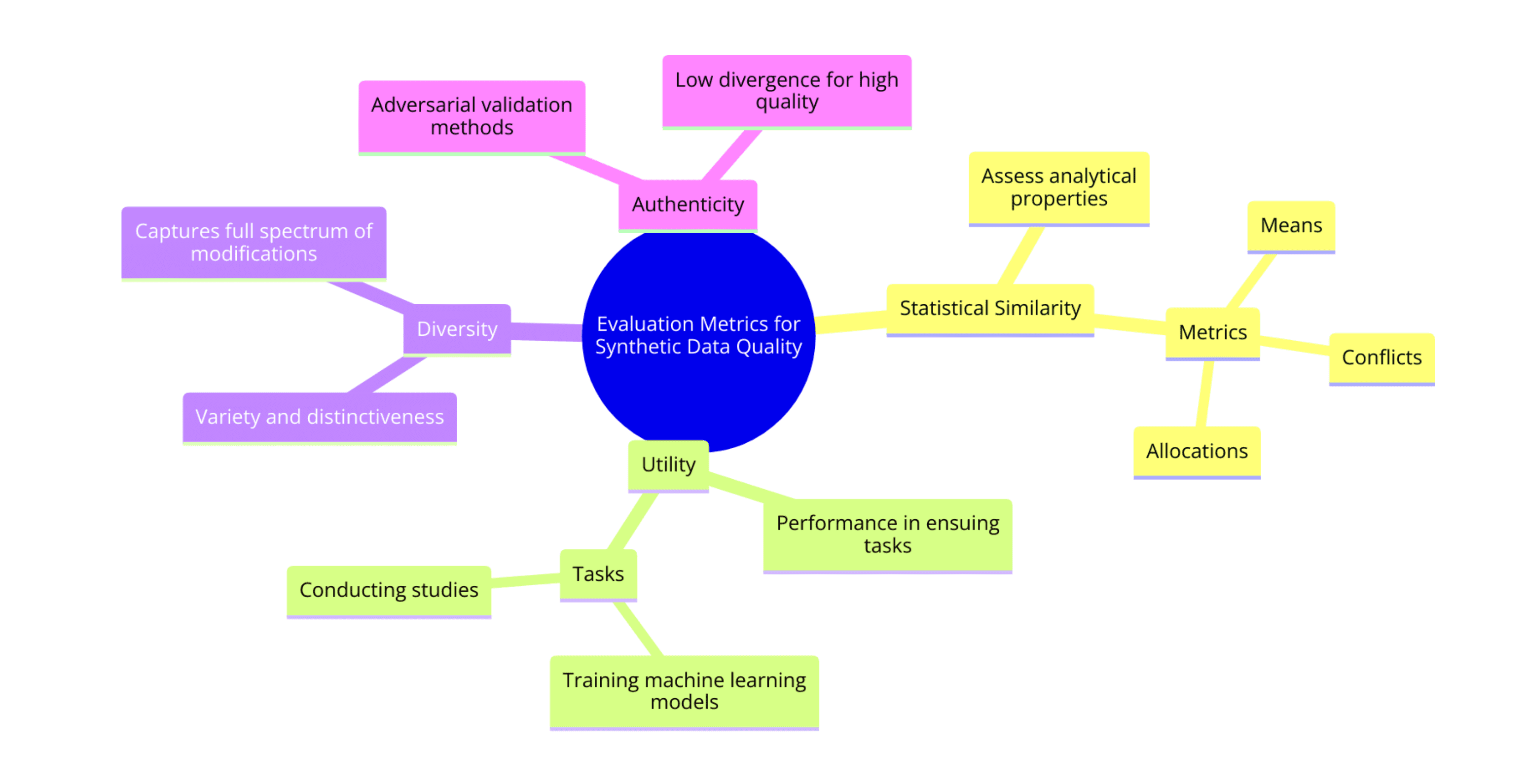
To examine the quality of synthetic data, several calculation metrics can be engaged. Common metrics include:
Statistical Similarity: Assess how closely the artificial data matches the analytical properties of the real data, like means, conflicts and allocations.
Utility: Assess how well the artificial data performs in ensuing tasks, like training machine learning models or conducting studies.
Diversity: Examine the variety and distinctiveness of the artificial data to ensure it catches the full spectrum of modifications present in the real data.
Authenticity: Utilize adversarial validation methods to analyze how noticeable the artificial data is from real data, planning for low divergence to specify high quality.
By applying these metrics, you can ensure that the artificial data you produce is both pragmatic and useful for your deliberated applications.
Applications of Synthetic Data in RAG Systems
Augmenting and Diversifying Training Data
Domain Adaptation and Transfer Learning
When you operate with RAG systems, synthetic data can play a critical role in domain adaptation and transfer learning. By producing synthetic data that closely imitates the target domain, efficiently aqueducting the gap between distinct data domains becomes possible. This technique permits you to refine pre-trained models on artificial datasets, permitting them to adjust to new domains without the requirement for comprehensive real-world data. Transfer learning becomes more effective, as the artificial data offers a rigid foundation for the models to construct upon.
Addressing Data Scarcity and Bias
The shortage of high-quality training data presents a predominant challenge in constructing RAG systems. Synthetic data can help you conquer this obstacle by producing large volumes of data that resemble real-world datasets. Not only does this procedure satisfy data scarcity, but it also allows for the introduction of variety into the training data, acknowledging possible bias. By carefully composing artificial data to include underrepresented outlines and edge cases, ensuring that models become more rigid and less liable to impartiality becomes your responsibility.
Enhancing Retrieval Components
Expanding Knowledge Bases and Corpora
For RAG systems, the standard and thoroughness of the knowledge base or corpus are uppermost. Synthetic data permits you to develop these resources substantially. By producing synthetic documents, accelerating existing corpora with pertinent details that might be missing from the original dataset becomes achievable. This enlargement improves the length and depth of the knowledge base, offering an affluent context for the retrieval procedure.
Improving Retrieval Performance and Relevance
Synthetic data can also enhance the performance and pertinence of the retrieval elements in RAG systems. By integrating artificial data that reflects the queries and details required of users, you can refine retrieval algorithms to better comprehend and respond to varied queries. This tailored approach ensures that the retrieval system returns more precise and pertinent outcomes improving the overall user experience.
Enriching Generation Components
Fine-Tuning Language Models
In the generation stage of RAG systems, synthetic data proves indispensable for fine-tuning language models. By training models on synthetic data that indulges numerous verbal styles and contexts, you can improve their ability to produce coherent and conditionally pertinent responses. This fine-tuning process ensures that the language models are better prepared to manage the intricacies of natural language, leading to more engaging and natural results.
Improving Fluency, Coherence, and Factual Accuracy
Finally, synthetic data can help you enhance the proficiency, coherence and accuracy of produced content. By producing artificial datasets that accentuate these standards, you can train models to prioritize them in their results. This focus results in the creation of text that is not only grammatically accurate and logically constant but also factually precise. The outcome is a more dependable and trustworthy RAG system that users can rely on for high-quality details.
Alright, now that we've navigated the ins and outs of synthetic data and its impact on RAG applications, let's dive deeper into the ethical jungle. How do we ensure that our pursuit of advancement respects privacy and dodges the pitfalls of bias? Hang tight, because this next section is crucial.
Challenges and Considerations
Privacy and Ethical Concerns
Privacy and ethical concerns are predominant when dealing with artificial data. Synthetic data must be produced in a way that doesn’t accidentally disclose sensitive details from the original datasets. Ensuring obscurity while handling the usefulness of the data is challenging. Ethical considerations also lengthened to how this data is utilized. For example, synthetic data shouldn’t be used to betray or mislead investors. Establishing clear instructions and following ethical standards are needed by organizations to navigate these concerns efficiently.
Ensuring Synthetic Data Quality and Realism
Ensuring quality and authenticity of synthetic data is crucial. Poor-quality synthetic can result in imprecise models and undependable results. One often uses methods like Generative Adversarial Networks (GANs) and Variational Autoencoders (VAEs) to generate high-quality synthetic data. These methods must be carefully measured to produce data that closely reflects real-world circumstances. Verification processes, including cross-verification with real-data, are important to validate the loyalty and usefulness of synthetic datasets.
Potential for Amplifying Biases and Artifacts
Synthetic data generation can accidentally distend impartiality present in the original dataset. If the source data contains impartiality, these can be regenerated and even enlarged in the artificial data. Acknowledging these problems needs rigid pre-processing and bias mitigation methods. It’s important to locate and eliminate biases in the training data before producing synthetic datasets. Constant observation and adaptation are important to decrease the risk of bias mitigation.
Balancing Synthetic and Real-World Data
Balancing the use of synthetic and real-world data is a fragile task. While synthetic data offers benefits such as scalability and privacy, it should complement rather than replace real-world data. The blend approach, using both synthetic and real data, often yields the best outcomes. Real-world data is essential for verification and evaluation reasons, ensuring that models trained on synthetic data stays pertinent and precise.
Computational Resources and Scalability
Important arithmetical resources are required to produce high-quality synthetic data. The processes involved, especially with advanced methods such as GANs, need strong hardware and significant processing time. Scalability becomes a solicitude as the volume of data increases. Investing in rigid infrastructure and upgrading their arithmetical workflows is a must for organizations to manage these demands effectively. Cloud calculating and allocating processing are possible solutions to acknowledge scalability difficulties.
Case Studies and Real-World Examples
Organizations and Research Groups Using Synthetic Data for RAG Applications
At the vanguard of using synthetic data for Retrieval-Augmented Generation (RAG) applications are several organizations and research groups. For instance, OpenAI uses synthetic data to improve the training of its language models. Similarly, IBM Research has developed synthetic data solutions to enhance their AI-Driven analytics platforms. These organizations use synthetic data to conquer restrictions in data attainability and improve model performance.
Successful Use Cases and Outcomes
The utilization of synthetic data in autonomous vehicle development represents one prestigious success story. Huge amounts of synthetic data are produced by companies such as Waymo and Tesla to affect driving scenarios. The approach has expedited the development and testing of their autonomous systems. Another instance is the healthcare sector, where synthetic data is utilized to expand the datasets of patients, assisting in the progression of diagnostic models without compromising the privacy of patients. These use cases substantiate the practical advantages and effectiveness of synthetic data.
Lessons Learned and Best Practices
From these applications, numerous lessons become apparent. Initially, it is important to handle a rigid verification process to ensure synthetic data quality. Secondly, acknowledging impartiality from the beginning can avert problems down the line. Third, a hybrid approach mixing synthetic with real data, often yields superior outcomes. Finally, investing in scalable infrastructure and embracing cloud-based solutions can lessen arithmetical challenges. These best practices provide a strategy for organizations looking to use synthetic data efficiently.
Conclusion
Synthetic data plays a crucial role in enriching RAG applications, acknowledging shortage of data and bias, and improving overall model performance. Going forward, the liable and ethical utilization of synthetic data will be critical for boosting its advantages. By using synthetic data considerably , you can uncover new possibilities in your RAG applications, making them more rigid, precise and fair.
Retrieval-Augmented Generation (RAG) applications have transformed natural language processing (NLP) by unifying retrieval and generation methods to produce more precise and contextually pertinent responses. However, these applications often brawl with the challenges of limited or partial training data. This can hamper their performance and dependability. Here’s where artificial data comes into play. By generating synthetic data, you can acknowledge these issues, making your RAG applications more rigid and efficient. Synthetic data isn’t just a substitute; it is a strategic tool to improve data enrichment.
Overview of Synthetic Data Generation Techniques
Rule-Based Techniques
Template-Based Generation:
Template-based generation allows for the creation of artificial data by defining a set of predefined templates. These templates act as outlines, permitting you to create data specimens by populating the templates with pertinent values. This technique is direct and ensures correspondence and framework in the generated data. For example, in generating artificial customer profiles, you might utilize a template such as “Name: {First Name} {Last Name}, Age: {Age}, Email: {Email},” where the substitutes are replaced with values from a predefined list or a random generator.
Grammar-Based Generation:
Grammar-based generation indulges using formal grammars to produce artificial data generation. Defining a set of grammatical regulations describes the framework of your data, and generating data iteratively applies these regulations. This approach is specifically useful when you are required to generate syntactically intricate data, like programming code or natural language sentences. For instance, you might define a grammar for producing arithmetic expressions, citing rules for operators and operands, which can then generate an assortment of logical expressions.
Domain-Specific Synthetic Data Generation
Domain-Specific synthetic data generation involves creating artificial data customized to a concrete field or industry. This information mirrors real-world synopsis, helping ventures and investigators perform inspection, testing and model training without the restrictions and privacy concerns linked with actual data. It uses advanced algorithms and methods to counterfeit data that firmly resembles the attributes and motifs found within a precise domain, like e-commerce, healthcare and finance.
Machine-Learning Based Techniques
Generative Adversarial Networks (GANs)
Generative Adversarial Networks (GANs) provide a precise technique for artificial data creation. Two sensory networks, a generator and a discriminator, contend against each other. The generator generates artificial data, while the discriminator assesses its genuineness. Through this conflicting process, the generator enhances its ability to generate pragmatic data. GANs have shown phenomenal success in creating high-quality images, but they can also be applied to other kinds of data, like text and audio.
Variational Autoencoders (VAEs)
Variational Autoencoders (VAEs) are another striking method for producing artificial data. Training a VAE involves encrypting input data into a dormant space and then decrypting it back into the original formation. During this procedure, the VAE learns to create new data points by selecting from the inactive space. VAEs are specifically useful for creating data with constant variations and can be applied to tasks like image combination and anomaly detection.
Language Models and Text Generation
One highly uses language models, like GPT-3, for text generation. Training these models on a huge corpora of text data permits them to create coherent and conditionally pertinent text. By refining the model on precise datasets, you can generate artificial text that imitates the style and content of the training data. This method is precious for tasks such as automated content creation, chatbot replies, and NLP applications.
Hybrid Approaches Combining Rules and Machine Learning
Hybrid approaches use the strengths of both restrained-based and machine learning methods. Unifying predefined regulations with machine learning models produces artificial data that follows precise restrictions while handling changeability and realism. For example, you might incorporate a grammar-based technique to depict the framework of the data and then engage a GAN or VAE to fill in the details, ensuring both compatibility and variety in the produced data.
Evaluation Metrics for Synthetic Data Quality
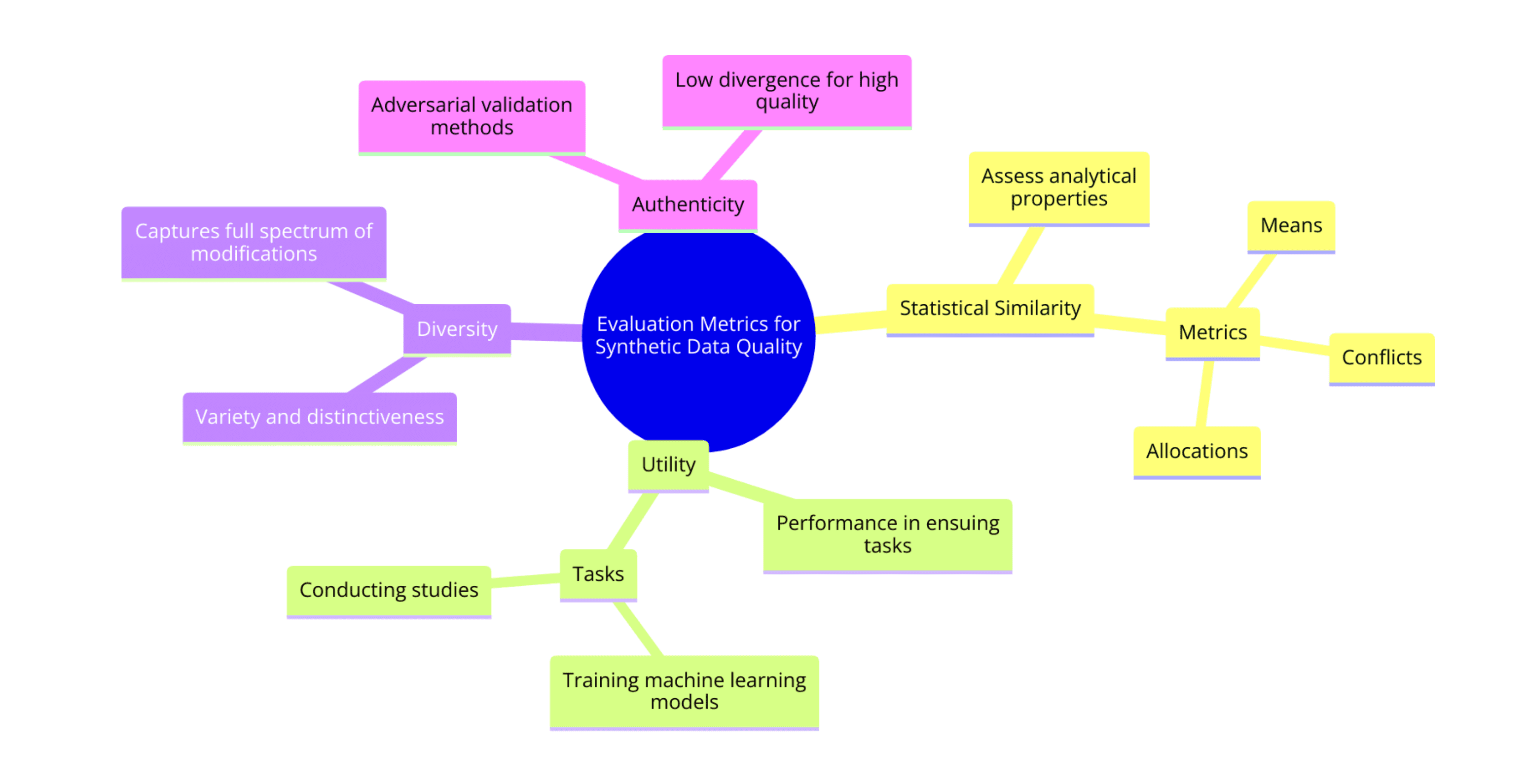
To examine the quality of synthetic data, several calculation metrics can be engaged. Common metrics include:
Statistical Similarity: Assess how closely the artificial data matches the analytical properties of the real data, like means, conflicts and allocations.
Utility: Assess how well the artificial data performs in ensuing tasks, like training machine learning models or conducting studies.
Diversity: Examine the variety and distinctiveness of the artificial data to ensure it catches the full spectrum of modifications present in the real data.
Authenticity: Utilize adversarial validation methods to analyze how noticeable the artificial data is from real data, planning for low divergence to specify high quality.
By applying these metrics, you can ensure that the artificial data you produce is both pragmatic and useful for your deliberated applications.
Applications of Synthetic Data in RAG Systems
Augmenting and Diversifying Training Data
Domain Adaptation and Transfer Learning
When you operate with RAG systems, synthetic data can play a critical role in domain adaptation and transfer learning. By producing synthetic data that closely imitates the target domain, efficiently aqueducting the gap between distinct data domains becomes possible. This technique permits you to refine pre-trained models on artificial datasets, permitting them to adjust to new domains without the requirement for comprehensive real-world data. Transfer learning becomes more effective, as the artificial data offers a rigid foundation for the models to construct upon.
Addressing Data Scarcity and Bias
The shortage of high-quality training data presents a predominant challenge in constructing RAG systems. Synthetic data can help you conquer this obstacle by producing large volumes of data that resemble real-world datasets. Not only does this procedure satisfy data scarcity, but it also allows for the introduction of variety into the training data, acknowledging possible bias. By carefully composing artificial data to include underrepresented outlines and edge cases, ensuring that models become more rigid and less liable to impartiality becomes your responsibility.
Enhancing Retrieval Components
Expanding Knowledge Bases and Corpora
For RAG systems, the standard and thoroughness of the knowledge base or corpus are uppermost. Synthetic data permits you to develop these resources substantially. By producing synthetic documents, accelerating existing corpora with pertinent details that might be missing from the original dataset becomes achievable. This enlargement improves the length and depth of the knowledge base, offering an affluent context for the retrieval procedure.
Improving Retrieval Performance and Relevance
Synthetic data can also enhance the performance and pertinence of the retrieval elements in RAG systems. By integrating artificial data that reflects the queries and details required of users, you can refine retrieval algorithms to better comprehend and respond to varied queries. This tailored approach ensures that the retrieval system returns more precise and pertinent outcomes improving the overall user experience.
Enriching Generation Components
Fine-Tuning Language Models
In the generation stage of RAG systems, synthetic data proves indispensable for fine-tuning language models. By training models on synthetic data that indulges numerous verbal styles and contexts, you can improve their ability to produce coherent and conditionally pertinent responses. This fine-tuning process ensures that the language models are better prepared to manage the intricacies of natural language, leading to more engaging and natural results.
Improving Fluency, Coherence, and Factual Accuracy
Finally, synthetic data can help you enhance the proficiency, coherence and accuracy of produced content. By producing artificial datasets that accentuate these standards, you can train models to prioritize them in their results. This focus results in the creation of text that is not only grammatically accurate and logically constant but also factually precise. The outcome is a more dependable and trustworthy RAG system that users can rely on for high-quality details.
Alright, now that we've navigated the ins and outs of synthetic data and its impact on RAG applications, let's dive deeper into the ethical jungle. How do we ensure that our pursuit of advancement respects privacy and dodges the pitfalls of bias? Hang tight, because this next section is crucial.
Challenges and Considerations
Privacy and Ethical Concerns
Privacy and ethical concerns are predominant when dealing with artificial data. Synthetic data must be produced in a way that doesn’t accidentally disclose sensitive details from the original datasets. Ensuring obscurity while handling the usefulness of the data is challenging. Ethical considerations also lengthened to how this data is utilized. For example, synthetic data shouldn’t be used to betray or mislead investors. Establishing clear instructions and following ethical standards are needed by organizations to navigate these concerns efficiently.
Ensuring Synthetic Data Quality and Realism
Ensuring quality and authenticity of synthetic data is crucial. Poor-quality synthetic can result in imprecise models and undependable results. One often uses methods like Generative Adversarial Networks (GANs) and Variational Autoencoders (VAEs) to generate high-quality synthetic data. These methods must be carefully measured to produce data that closely reflects real-world circumstances. Verification processes, including cross-verification with real-data, are important to validate the loyalty and usefulness of synthetic datasets.
Potential for Amplifying Biases and Artifacts
Synthetic data generation can accidentally distend impartiality present in the original dataset. If the source data contains impartiality, these can be regenerated and even enlarged in the artificial data. Acknowledging these problems needs rigid pre-processing and bias mitigation methods. It’s important to locate and eliminate biases in the training data before producing synthetic datasets. Constant observation and adaptation are important to decrease the risk of bias mitigation.
Balancing Synthetic and Real-World Data
Balancing the use of synthetic and real-world data is a fragile task. While synthetic data offers benefits such as scalability and privacy, it should complement rather than replace real-world data. The blend approach, using both synthetic and real data, often yields the best outcomes. Real-world data is essential for verification and evaluation reasons, ensuring that models trained on synthetic data stays pertinent and precise.
Computational Resources and Scalability
Important arithmetical resources are required to produce high-quality synthetic data. The processes involved, especially with advanced methods such as GANs, need strong hardware and significant processing time. Scalability becomes a solicitude as the volume of data increases. Investing in rigid infrastructure and upgrading their arithmetical workflows is a must for organizations to manage these demands effectively. Cloud calculating and allocating processing are possible solutions to acknowledge scalability difficulties.
Case Studies and Real-World Examples
Organizations and Research Groups Using Synthetic Data for RAG Applications
At the vanguard of using synthetic data for Retrieval-Augmented Generation (RAG) applications are several organizations and research groups. For instance, OpenAI uses synthetic data to improve the training of its language models. Similarly, IBM Research has developed synthetic data solutions to enhance their AI-Driven analytics platforms. These organizations use synthetic data to conquer restrictions in data attainability and improve model performance.
Successful Use Cases and Outcomes
The utilization of synthetic data in autonomous vehicle development represents one prestigious success story. Huge amounts of synthetic data are produced by companies such as Waymo and Tesla to affect driving scenarios. The approach has expedited the development and testing of their autonomous systems. Another instance is the healthcare sector, where synthetic data is utilized to expand the datasets of patients, assisting in the progression of diagnostic models without compromising the privacy of patients. These use cases substantiate the practical advantages and effectiveness of synthetic data.
Lessons Learned and Best Practices
From these applications, numerous lessons become apparent. Initially, it is important to handle a rigid verification process to ensure synthetic data quality. Secondly, acknowledging impartiality from the beginning can avert problems down the line. Third, a hybrid approach mixing synthetic with real data, often yields superior outcomes. Finally, investing in scalable infrastructure and embracing cloud-based solutions can lessen arithmetical challenges. These best practices provide a strategy for organizations looking to use synthetic data efficiently.
Conclusion
Synthetic data plays a crucial role in enriching RAG applications, acknowledging shortage of data and bias, and improving overall model performance. Going forward, the liable and ethical utilization of synthetic data will be critical for boosting its advantages. By using synthetic data considerably , you can uncover new possibilities in your RAG applications, making them more rigid, precise and fair.
Retrieval-Augmented Generation (RAG) applications have transformed natural language processing (NLP) by unifying retrieval and generation methods to produce more precise and contextually pertinent responses. However, these applications often brawl with the challenges of limited or partial training data. This can hamper their performance and dependability. Here’s where artificial data comes into play. By generating synthetic data, you can acknowledge these issues, making your RAG applications more rigid and efficient. Synthetic data isn’t just a substitute; it is a strategic tool to improve data enrichment.
Overview of Synthetic Data Generation Techniques
Rule-Based Techniques
Template-Based Generation:
Template-based generation allows for the creation of artificial data by defining a set of predefined templates. These templates act as outlines, permitting you to create data specimens by populating the templates with pertinent values. This technique is direct and ensures correspondence and framework in the generated data. For example, in generating artificial customer profiles, you might utilize a template such as “Name: {First Name} {Last Name}, Age: {Age}, Email: {Email},” where the substitutes are replaced with values from a predefined list or a random generator.
Grammar-Based Generation:
Grammar-based generation indulges using formal grammars to produce artificial data generation. Defining a set of grammatical regulations describes the framework of your data, and generating data iteratively applies these regulations. This approach is specifically useful when you are required to generate syntactically intricate data, like programming code or natural language sentences. For instance, you might define a grammar for producing arithmetic expressions, citing rules for operators and operands, which can then generate an assortment of logical expressions.
Domain-Specific Synthetic Data Generation
Domain-Specific synthetic data generation involves creating artificial data customized to a concrete field or industry. This information mirrors real-world synopsis, helping ventures and investigators perform inspection, testing and model training without the restrictions and privacy concerns linked with actual data. It uses advanced algorithms and methods to counterfeit data that firmly resembles the attributes and motifs found within a precise domain, like e-commerce, healthcare and finance.
Machine-Learning Based Techniques
Generative Adversarial Networks (GANs)
Generative Adversarial Networks (GANs) provide a precise technique for artificial data creation. Two sensory networks, a generator and a discriminator, contend against each other. The generator generates artificial data, while the discriminator assesses its genuineness. Through this conflicting process, the generator enhances its ability to generate pragmatic data. GANs have shown phenomenal success in creating high-quality images, but they can also be applied to other kinds of data, like text and audio.
Variational Autoencoders (VAEs)
Variational Autoencoders (VAEs) are another striking method for producing artificial data. Training a VAE involves encrypting input data into a dormant space and then decrypting it back into the original formation. During this procedure, the VAE learns to create new data points by selecting from the inactive space. VAEs are specifically useful for creating data with constant variations and can be applied to tasks like image combination and anomaly detection.
Language Models and Text Generation
One highly uses language models, like GPT-3, for text generation. Training these models on a huge corpora of text data permits them to create coherent and conditionally pertinent text. By refining the model on precise datasets, you can generate artificial text that imitates the style and content of the training data. This method is precious for tasks such as automated content creation, chatbot replies, and NLP applications.
Hybrid Approaches Combining Rules and Machine Learning
Hybrid approaches use the strengths of both restrained-based and machine learning methods. Unifying predefined regulations with machine learning models produces artificial data that follows precise restrictions while handling changeability and realism. For example, you might incorporate a grammar-based technique to depict the framework of the data and then engage a GAN or VAE to fill in the details, ensuring both compatibility and variety in the produced data.
Evaluation Metrics for Synthetic Data Quality
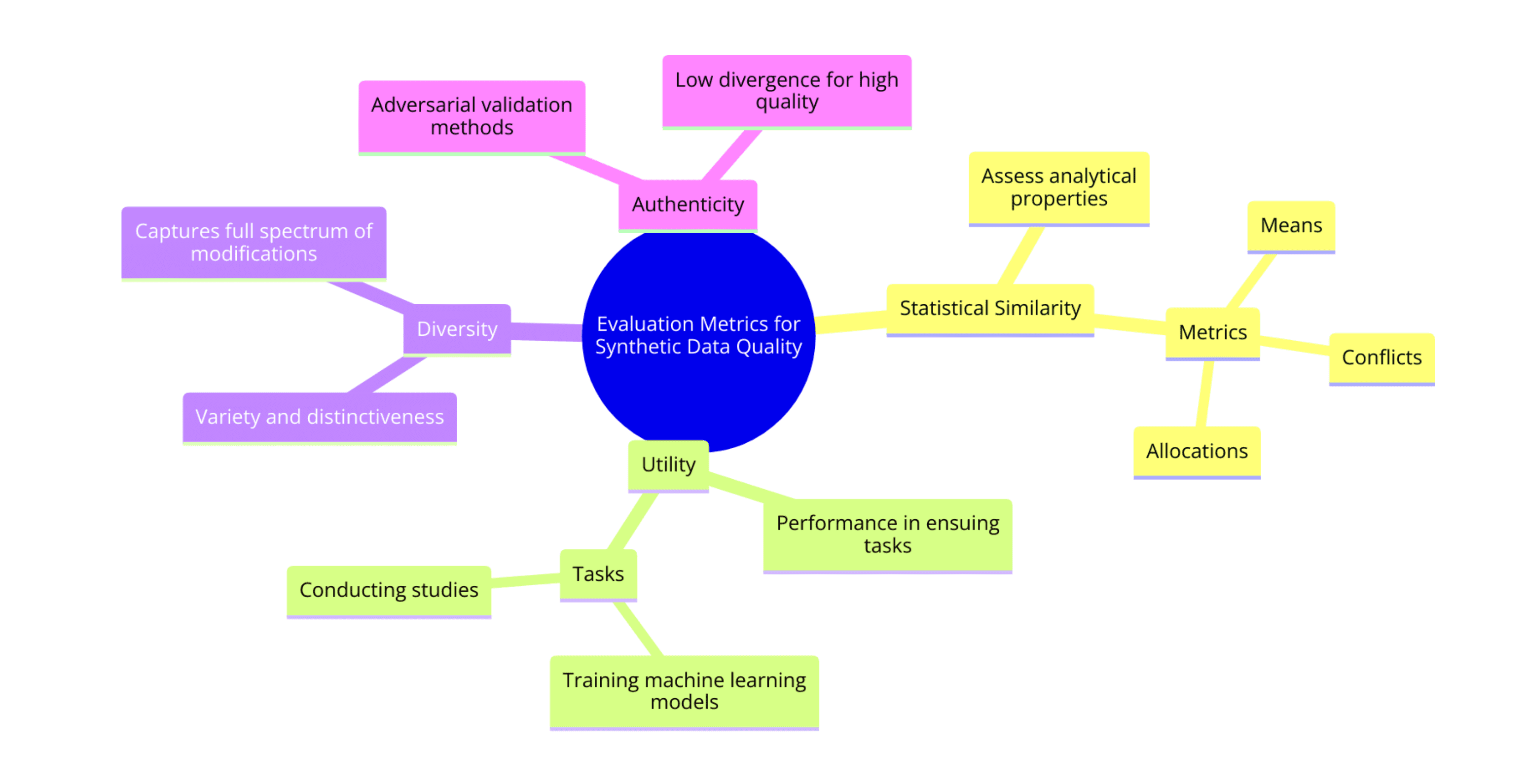
To examine the quality of synthetic data, several calculation metrics can be engaged. Common metrics include:
Statistical Similarity: Assess how closely the artificial data matches the analytical properties of the real data, like means, conflicts and allocations.
Utility: Assess how well the artificial data performs in ensuing tasks, like training machine learning models or conducting studies.
Diversity: Examine the variety and distinctiveness of the artificial data to ensure it catches the full spectrum of modifications present in the real data.
Authenticity: Utilize adversarial validation methods to analyze how noticeable the artificial data is from real data, planning for low divergence to specify high quality.
By applying these metrics, you can ensure that the artificial data you produce is both pragmatic and useful for your deliberated applications.
Applications of Synthetic Data in RAG Systems
Augmenting and Diversifying Training Data
Domain Adaptation and Transfer Learning
When you operate with RAG systems, synthetic data can play a critical role in domain adaptation and transfer learning. By producing synthetic data that closely imitates the target domain, efficiently aqueducting the gap between distinct data domains becomes possible. This technique permits you to refine pre-trained models on artificial datasets, permitting them to adjust to new domains without the requirement for comprehensive real-world data. Transfer learning becomes more effective, as the artificial data offers a rigid foundation for the models to construct upon.
Addressing Data Scarcity and Bias
The shortage of high-quality training data presents a predominant challenge in constructing RAG systems. Synthetic data can help you conquer this obstacle by producing large volumes of data that resemble real-world datasets. Not only does this procedure satisfy data scarcity, but it also allows for the introduction of variety into the training data, acknowledging possible bias. By carefully composing artificial data to include underrepresented outlines and edge cases, ensuring that models become more rigid and less liable to impartiality becomes your responsibility.
Enhancing Retrieval Components
Expanding Knowledge Bases and Corpora
For RAG systems, the standard and thoroughness of the knowledge base or corpus are uppermost. Synthetic data permits you to develop these resources substantially. By producing synthetic documents, accelerating existing corpora with pertinent details that might be missing from the original dataset becomes achievable. This enlargement improves the length and depth of the knowledge base, offering an affluent context for the retrieval procedure.
Improving Retrieval Performance and Relevance
Synthetic data can also enhance the performance and pertinence of the retrieval elements in RAG systems. By integrating artificial data that reflects the queries and details required of users, you can refine retrieval algorithms to better comprehend and respond to varied queries. This tailored approach ensures that the retrieval system returns more precise and pertinent outcomes improving the overall user experience.
Enriching Generation Components
Fine-Tuning Language Models
In the generation stage of RAG systems, synthetic data proves indispensable for fine-tuning language models. By training models on synthetic data that indulges numerous verbal styles and contexts, you can improve their ability to produce coherent and conditionally pertinent responses. This fine-tuning process ensures that the language models are better prepared to manage the intricacies of natural language, leading to more engaging and natural results.
Improving Fluency, Coherence, and Factual Accuracy
Finally, synthetic data can help you enhance the proficiency, coherence and accuracy of produced content. By producing artificial datasets that accentuate these standards, you can train models to prioritize them in their results. This focus results in the creation of text that is not only grammatically accurate and logically constant but also factually precise. The outcome is a more dependable and trustworthy RAG system that users can rely on for high-quality details.
Alright, now that we've navigated the ins and outs of synthetic data and its impact on RAG applications, let's dive deeper into the ethical jungle. How do we ensure that our pursuit of advancement respects privacy and dodges the pitfalls of bias? Hang tight, because this next section is crucial.
Challenges and Considerations
Privacy and Ethical Concerns
Privacy and ethical concerns are predominant when dealing with artificial data. Synthetic data must be produced in a way that doesn’t accidentally disclose sensitive details from the original datasets. Ensuring obscurity while handling the usefulness of the data is challenging. Ethical considerations also lengthened to how this data is utilized. For example, synthetic data shouldn’t be used to betray or mislead investors. Establishing clear instructions and following ethical standards are needed by organizations to navigate these concerns efficiently.
Ensuring Synthetic Data Quality and Realism
Ensuring quality and authenticity of synthetic data is crucial. Poor-quality synthetic can result in imprecise models and undependable results. One often uses methods like Generative Adversarial Networks (GANs) and Variational Autoencoders (VAEs) to generate high-quality synthetic data. These methods must be carefully measured to produce data that closely reflects real-world circumstances. Verification processes, including cross-verification with real-data, are important to validate the loyalty and usefulness of synthetic datasets.
Potential for Amplifying Biases and Artifacts
Synthetic data generation can accidentally distend impartiality present in the original dataset. If the source data contains impartiality, these can be regenerated and even enlarged in the artificial data. Acknowledging these problems needs rigid pre-processing and bias mitigation methods. It’s important to locate and eliminate biases in the training data before producing synthetic datasets. Constant observation and adaptation are important to decrease the risk of bias mitigation.
Balancing Synthetic and Real-World Data
Balancing the use of synthetic and real-world data is a fragile task. While synthetic data offers benefits such as scalability and privacy, it should complement rather than replace real-world data. The blend approach, using both synthetic and real data, often yields the best outcomes. Real-world data is essential for verification and evaluation reasons, ensuring that models trained on synthetic data stays pertinent and precise.
Computational Resources and Scalability
Important arithmetical resources are required to produce high-quality synthetic data. The processes involved, especially with advanced methods such as GANs, need strong hardware and significant processing time. Scalability becomes a solicitude as the volume of data increases. Investing in rigid infrastructure and upgrading their arithmetical workflows is a must for organizations to manage these demands effectively. Cloud calculating and allocating processing are possible solutions to acknowledge scalability difficulties.
Case Studies and Real-World Examples
Organizations and Research Groups Using Synthetic Data for RAG Applications
At the vanguard of using synthetic data for Retrieval-Augmented Generation (RAG) applications are several organizations and research groups. For instance, OpenAI uses synthetic data to improve the training of its language models. Similarly, IBM Research has developed synthetic data solutions to enhance their AI-Driven analytics platforms. These organizations use synthetic data to conquer restrictions in data attainability and improve model performance.
Successful Use Cases and Outcomes
The utilization of synthetic data in autonomous vehicle development represents one prestigious success story. Huge amounts of synthetic data are produced by companies such as Waymo and Tesla to affect driving scenarios. The approach has expedited the development and testing of their autonomous systems. Another instance is the healthcare sector, where synthetic data is utilized to expand the datasets of patients, assisting in the progression of diagnostic models without compromising the privacy of patients. These use cases substantiate the practical advantages and effectiveness of synthetic data.
Lessons Learned and Best Practices
From these applications, numerous lessons become apparent. Initially, it is important to handle a rigid verification process to ensure synthetic data quality. Secondly, acknowledging impartiality from the beginning can avert problems down the line. Third, a hybrid approach mixing synthetic with real data, often yields superior outcomes. Finally, investing in scalable infrastructure and embracing cloud-based solutions can lessen arithmetical challenges. These best practices provide a strategy for organizations looking to use synthetic data efficiently.
Conclusion
Synthetic data plays a crucial role in enriching RAG applications, acknowledging shortage of data and bias, and improving overall model performance. Going forward, the liable and ethical utilization of synthetic data will be critical for boosting its advantages. By using synthetic data considerably , you can uncover new possibilities in your RAG applications, making them more rigid, precise and fair.
Retrieval-Augmented Generation (RAG) applications have transformed natural language processing (NLP) by unifying retrieval and generation methods to produce more precise and contextually pertinent responses. However, these applications often brawl with the challenges of limited or partial training data. This can hamper their performance and dependability. Here’s where artificial data comes into play. By generating synthetic data, you can acknowledge these issues, making your RAG applications more rigid and efficient. Synthetic data isn’t just a substitute; it is a strategic tool to improve data enrichment.
Overview of Synthetic Data Generation Techniques
Rule-Based Techniques
Template-Based Generation:
Template-based generation allows for the creation of artificial data by defining a set of predefined templates. These templates act as outlines, permitting you to create data specimens by populating the templates with pertinent values. This technique is direct and ensures correspondence and framework in the generated data. For example, in generating artificial customer profiles, you might utilize a template such as “Name: {First Name} {Last Name}, Age: {Age}, Email: {Email},” where the substitutes are replaced with values from a predefined list or a random generator.
Grammar-Based Generation:
Grammar-based generation indulges using formal grammars to produce artificial data generation. Defining a set of grammatical regulations describes the framework of your data, and generating data iteratively applies these regulations. This approach is specifically useful when you are required to generate syntactically intricate data, like programming code or natural language sentences. For instance, you might define a grammar for producing arithmetic expressions, citing rules for operators and operands, which can then generate an assortment of logical expressions.
Domain-Specific Synthetic Data Generation
Domain-Specific synthetic data generation involves creating artificial data customized to a concrete field or industry. This information mirrors real-world synopsis, helping ventures and investigators perform inspection, testing and model training without the restrictions and privacy concerns linked with actual data. It uses advanced algorithms and methods to counterfeit data that firmly resembles the attributes and motifs found within a precise domain, like e-commerce, healthcare and finance.
Machine-Learning Based Techniques
Generative Adversarial Networks (GANs)
Generative Adversarial Networks (GANs) provide a precise technique for artificial data creation. Two sensory networks, a generator and a discriminator, contend against each other. The generator generates artificial data, while the discriminator assesses its genuineness. Through this conflicting process, the generator enhances its ability to generate pragmatic data. GANs have shown phenomenal success in creating high-quality images, but they can also be applied to other kinds of data, like text and audio.
Variational Autoencoders (VAEs)
Variational Autoencoders (VAEs) are another striking method for producing artificial data. Training a VAE involves encrypting input data into a dormant space and then decrypting it back into the original formation. During this procedure, the VAE learns to create new data points by selecting from the inactive space. VAEs are specifically useful for creating data with constant variations and can be applied to tasks like image combination and anomaly detection.
Language Models and Text Generation
One highly uses language models, like GPT-3, for text generation. Training these models on a huge corpora of text data permits them to create coherent and conditionally pertinent text. By refining the model on precise datasets, you can generate artificial text that imitates the style and content of the training data. This method is precious for tasks such as automated content creation, chatbot replies, and NLP applications.
Hybrid Approaches Combining Rules and Machine Learning
Hybrid approaches use the strengths of both restrained-based and machine learning methods. Unifying predefined regulations with machine learning models produces artificial data that follows precise restrictions while handling changeability and realism. For example, you might incorporate a grammar-based technique to depict the framework of the data and then engage a GAN or VAE to fill in the details, ensuring both compatibility and variety in the produced data.
Evaluation Metrics for Synthetic Data Quality
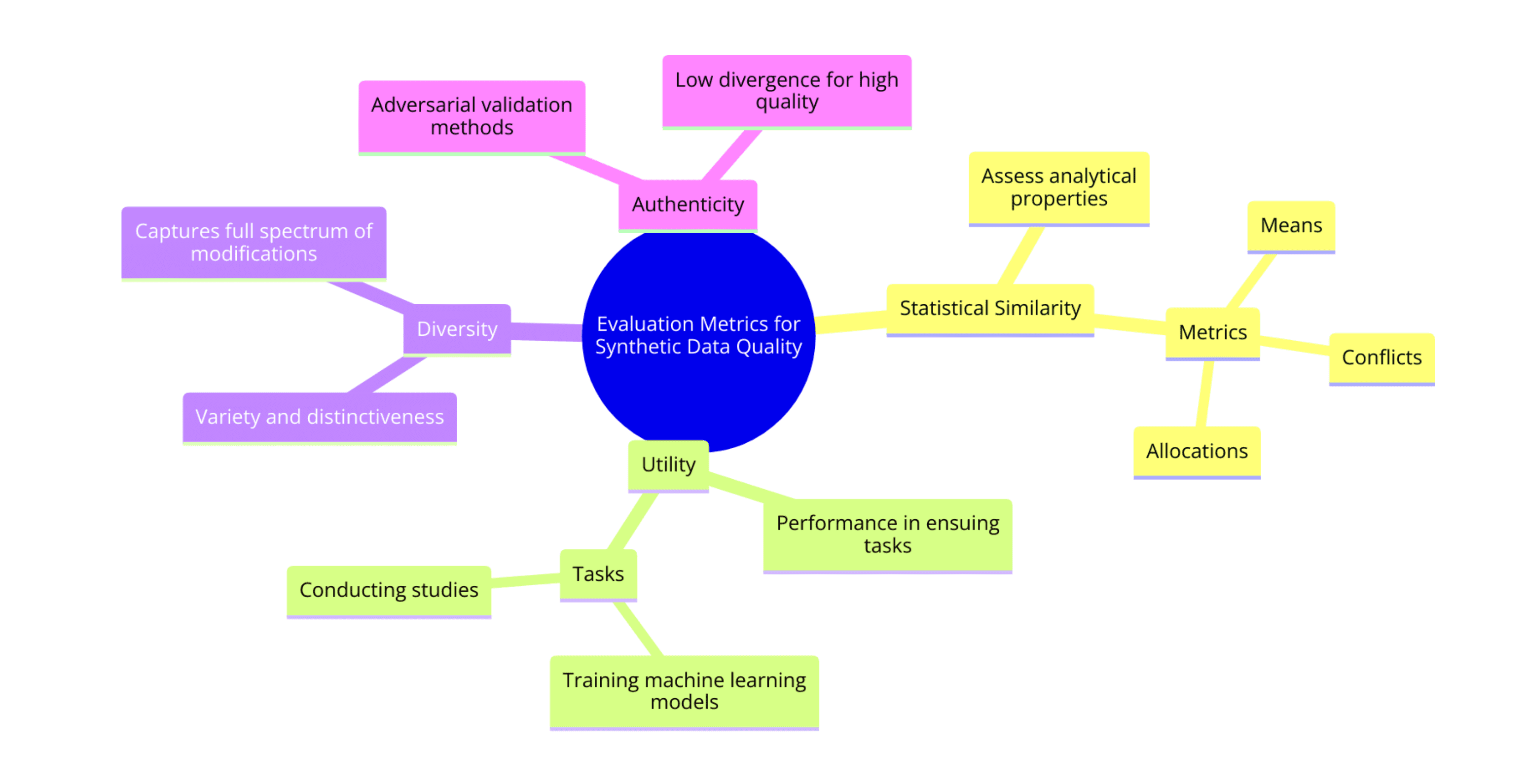
To examine the quality of synthetic data, several calculation metrics can be engaged. Common metrics include:
Statistical Similarity: Assess how closely the artificial data matches the analytical properties of the real data, like means, conflicts and allocations.
Utility: Assess how well the artificial data performs in ensuing tasks, like training machine learning models or conducting studies.
Diversity: Examine the variety and distinctiveness of the artificial data to ensure it catches the full spectrum of modifications present in the real data.
Authenticity: Utilize adversarial validation methods to analyze how noticeable the artificial data is from real data, planning for low divergence to specify high quality.
By applying these metrics, you can ensure that the artificial data you produce is both pragmatic and useful for your deliberated applications.
Applications of Synthetic Data in RAG Systems
Augmenting and Diversifying Training Data
Domain Adaptation and Transfer Learning
When you operate with RAG systems, synthetic data can play a critical role in domain adaptation and transfer learning. By producing synthetic data that closely imitates the target domain, efficiently aqueducting the gap between distinct data domains becomes possible. This technique permits you to refine pre-trained models on artificial datasets, permitting them to adjust to new domains without the requirement for comprehensive real-world data. Transfer learning becomes more effective, as the artificial data offers a rigid foundation for the models to construct upon.
Addressing Data Scarcity and Bias
The shortage of high-quality training data presents a predominant challenge in constructing RAG systems. Synthetic data can help you conquer this obstacle by producing large volumes of data that resemble real-world datasets. Not only does this procedure satisfy data scarcity, but it also allows for the introduction of variety into the training data, acknowledging possible bias. By carefully composing artificial data to include underrepresented outlines and edge cases, ensuring that models become more rigid and less liable to impartiality becomes your responsibility.
Enhancing Retrieval Components
Expanding Knowledge Bases and Corpora
For RAG systems, the standard and thoroughness of the knowledge base or corpus are uppermost. Synthetic data permits you to develop these resources substantially. By producing synthetic documents, accelerating existing corpora with pertinent details that might be missing from the original dataset becomes achievable. This enlargement improves the length and depth of the knowledge base, offering an affluent context for the retrieval procedure.
Improving Retrieval Performance and Relevance
Synthetic data can also enhance the performance and pertinence of the retrieval elements in RAG systems. By integrating artificial data that reflects the queries and details required of users, you can refine retrieval algorithms to better comprehend and respond to varied queries. This tailored approach ensures that the retrieval system returns more precise and pertinent outcomes improving the overall user experience.
Enriching Generation Components
Fine-Tuning Language Models
In the generation stage of RAG systems, synthetic data proves indispensable for fine-tuning language models. By training models on synthetic data that indulges numerous verbal styles and contexts, you can improve their ability to produce coherent and conditionally pertinent responses. This fine-tuning process ensures that the language models are better prepared to manage the intricacies of natural language, leading to more engaging and natural results.
Improving Fluency, Coherence, and Factual Accuracy
Finally, synthetic data can help you enhance the proficiency, coherence and accuracy of produced content. By producing artificial datasets that accentuate these standards, you can train models to prioritize them in their results. This focus results in the creation of text that is not only grammatically accurate and logically constant but also factually precise. The outcome is a more dependable and trustworthy RAG system that users can rely on for high-quality details.
Alright, now that we've navigated the ins and outs of synthetic data and its impact on RAG applications, let's dive deeper into the ethical jungle. How do we ensure that our pursuit of advancement respects privacy and dodges the pitfalls of bias? Hang tight, because this next section is crucial.
Challenges and Considerations
Privacy and Ethical Concerns
Privacy and ethical concerns are predominant when dealing with artificial data. Synthetic data must be produced in a way that doesn’t accidentally disclose sensitive details from the original datasets. Ensuring obscurity while handling the usefulness of the data is challenging. Ethical considerations also lengthened to how this data is utilized. For example, synthetic data shouldn’t be used to betray or mislead investors. Establishing clear instructions and following ethical standards are needed by organizations to navigate these concerns efficiently.
Ensuring Synthetic Data Quality and Realism
Ensuring quality and authenticity of synthetic data is crucial. Poor-quality synthetic can result in imprecise models and undependable results. One often uses methods like Generative Adversarial Networks (GANs) and Variational Autoencoders (VAEs) to generate high-quality synthetic data. These methods must be carefully measured to produce data that closely reflects real-world circumstances. Verification processes, including cross-verification with real-data, are important to validate the loyalty and usefulness of synthetic datasets.
Potential for Amplifying Biases and Artifacts
Synthetic data generation can accidentally distend impartiality present in the original dataset. If the source data contains impartiality, these can be regenerated and even enlarged in the artificial data. Acknowledging these problems needs rigid pre-processing and bias mitigation methods. It’s important to locate and eliminate biases in the training data before producing synthetic datasets. Constant observation and adaptation are important to decrease the risk of bias mitigation.
Balancing Synthetic and Real-World Data
Balancing the use of synthetic and real-world data is a fragile task. While synthetic data offers benefits such as scalability and privacy, it should complement rather than replace real-world data. The blend approach, using both synthetic and real data, often yields the best outcomes. Real-world data is essential for verification and evaluation reasons, ensuring that models trained on synthetic data stays pertinent and precise.
Computational Resources and Scalability
Important arithmetical resources are required to produce high-quality synthetic data. The processes involved, especially with advanced methods such as GANs, need strong hardware and significant processing time. Scalability becomes a solicitude as the volume of data increases. Investing in rigid infrastructure and upgrading their arithmetical workflows is a must for organizations to manage these demands effectively. Cloud calculating and allocating processing are possible solutions to acknowledge scalability difficulties.
Case Studies and Real-World Examples
Organizations and Research Groups Using Synthetic Data for RAG Applications
At the vanguard of using synthetic data for Retrieval-Augmented Generation (RAG) applications are several organizations and research groups. For instance, OpenAI uses synthetic data to improve the training of its language models. Similarly, IBM Research has developed synthetic data solutions to enhance their AI-Driven analytics platforms. These organizations use synthetic data to conquer restrictions in data attainability and improve model performance.
Successful Use Cases and Outcomes
The utilization of synthetic data in autonomous vehicle development represents one prestigious success story. Huge amounts of synthetic data are produced by companies such as Waymo and Tesla to affect driving scenarios. The approach has expedited the development and testing of their autonomous systems. Another instance is the healthcare sector, where synthetic data is utilized to expand the datasets of patients, assisting in the progression of diagnostic models without compromising the privacy of patients. These use cases substantiate the practical advantages and effectiveness of synthetic data.
Lessons Learned and Best Practices
From these applications, numerous lessons become apparent. Initially, it is important to handle a rigid verification process to ensure synthetic data quality. Secondly, acknowledging impartiality from the beginning can avert problems down the line. Third, a hybrid approach mixing synthetic with real data, often yields superior outcomes. Finally, investing in scalable infrastructure and embracing cloud-based solutions can lessen arithmetical challenges. These best practices provide a strategy for organizations looking to use synthetic data efficiently.
Conclusion
Synthetic data plays a crucial role in enriching RAG applications, acknowledging shortage of data and bias, and improving overall model performance. Going forward, the liable and ethical utilization of synthetic data will be critical for boosting its advantages. By using synthetic data considerably , you can uncover new possibilities in your RAG applications, making them more rigid, precise and fair.
Retrieval-Augmented Generation (RAG) applications have transformed natural language processing (NLP) by unifying retrieval and generation methods to produce more precise and contextually pertinent responses. However, these applications often brawl with the challenges of limited or partial training data. This can hamper their performance and dependability. Here’s where artificial data comes into play. By generating synthetic data, you can acknowledge these issues, making your RAG applications more rigid and efficient. Synthetic data isn’t just a substitute; it is a strategic tool to improve data enrichment.
Overview of Synthetic Data Generation Techniques
Rule-Based Techniques
Template-Based Generation:
Template-based generation allows for the creation of artificial data by defining a set of predefined templates. These templates act as outlines, permitting you to create data specimens by populating the templates with pertinent values. This technique is direct and ensures correspondence and framework in the generated data. For example, in generating artificial customer profiles, you might utilize a template such as “Name: {First Name} {Last Name}, Age: {Age}, Email: {Email},” where the substitutes are replaced with values from a predefined list or a random generator.
Grammar-Based Generation:
Grammar-based generation indulges using formal grammars to produce artificial data generation. Defining a set of grammatical regulations describes the framework of your data, and generating data iteratively applies these regulations. This approach is specifically useful when you are required to generate syntactically intricate data, like programming code or natural language sentences. For instance, you might define a grammar for producing arithmetic expressions, citing rules for operators and operands, which can then generate an assortment of logical expressions.
Domain-Specific Synthetic Data Generation
Domain-Specific synthetic data generation involves creating artificial data customized to a concrete field or industry. This information mirrors real-world synopsis, helping ventures and investigators perform inspection, testing and model training without the restrictions and privacy concerns linked with actual data. It uses advanced algorithms and methods to counterfeit data that firmly resembles the attributes and motifs found within a precise domain, like e-commerce, healthcare and finance.
Machine-Learning Based Techniques
Generative Adversarial Networks (GANs)
Generative Adversarial Networks (GANs) provide a precise technique for artificial data creation. Two sensory networks, a generator and a discriminator, contend against each other. The generator generates artificial data, while the discriminator assesses its genuineness. Through this conflicting process, the generator enhances its ability to generate pragmatic data. GANs have shown phenomenal success in creating high-quality images, but they can also be applied to other kinds of data, like text and audio.
Variational Autoencoders (VAEs)
Variational Autoencoders (VAEs) are another striking method for producing artificial data. Training a VAE involves encrypting input data into a dormant space and then decrypting it back into the original formation. During this procedure, the VAE learns to create new data points by selecting from the inactive space. VAEs are specifically useful for creating data with constant variations and can be applied to tasks like image combination and anomaly detection.
Language Models and Text Generation
One highly uses language models, like GPT-3, for text generation. Training these models on a huge corpora of text data permits them to create coherent and conditionally pertinent text. By refining the model on precise datasets, you can generate artificial text that imitates the style and content of the training data. This method is precious for tasks such as automated content creation, chatbot replies, and NLP applications.
Hybrid Approaches Combining Rules and Machine Learning
Hybrid approaches use the strengths of both restrained-based and machine learning methods. Unifying predefined regulations with machine learning models produces artificial data that follows precise restrictions while handling changeability and realism. For example, you might incorporate a grammar-based technique to depict the framework of the data and then engage a GAN or VAE to fill in the details, ensuring both compatibility and variety in the produced data.
Evaluation Metrics for Synthetic Data Quality
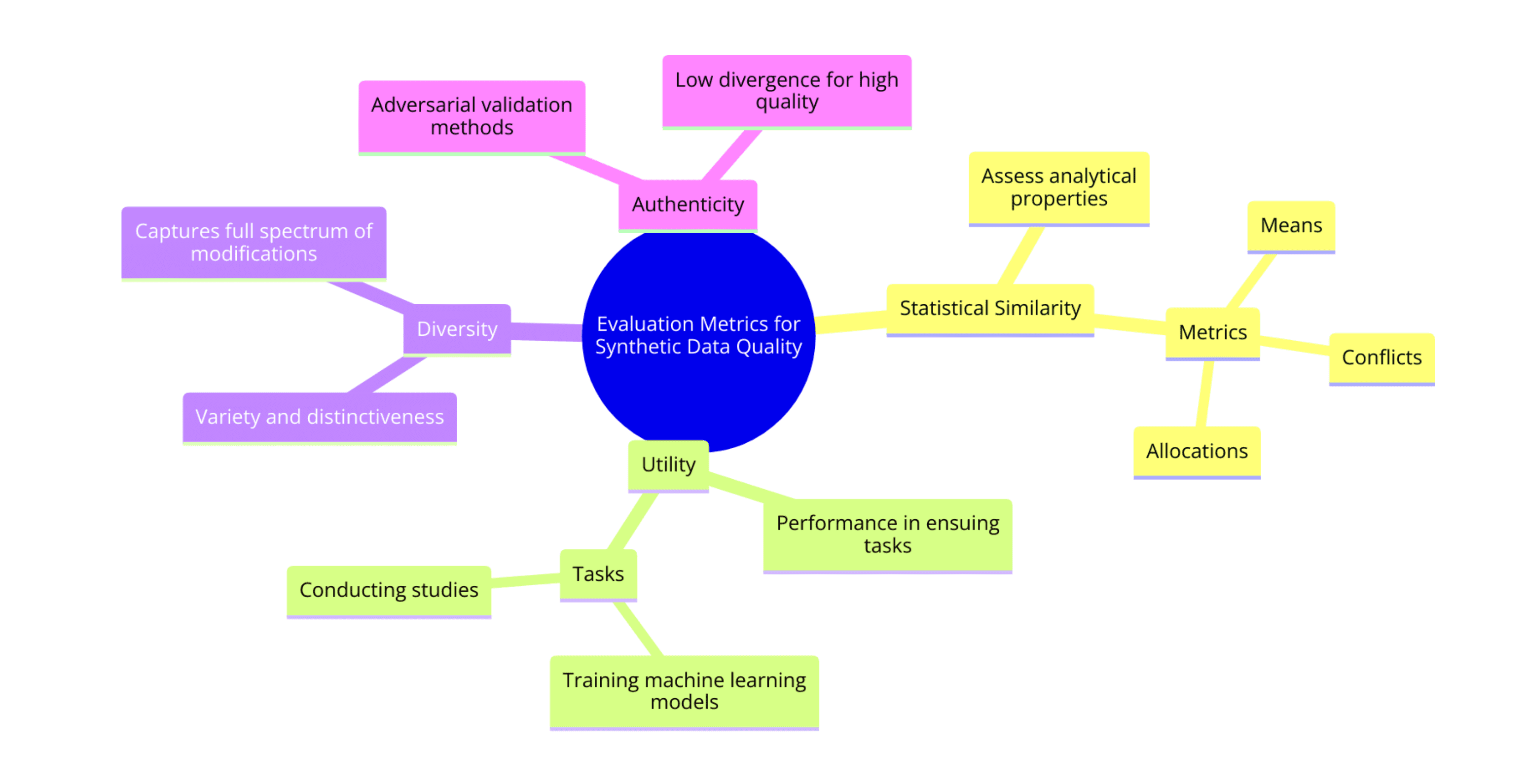
To examine the quality of synthetic data, several calculation metrics can be engaged. Common metrics include:
Statistical Similarity: Assess how closely the artificial data matches the analytical properties of the real data, like means, conflicts and allocations.
Utility: Assess how well the artificial data performs in ensuing tasks, like training machine learning models or conducting studies.
Diversity: Examine the variety and distinctiveness of the artificial data to ensure it catches the full spectrum of modifications present in the real data.
Authenticity: Utilize adversarial validation methods to analyze how noticeable the artificial data is from real data, planning for low divergence to specify high quality.
By applying these metrics, you can ensure that the artificial data you produce is both pragmatic and useful for your deliberated applications.
Applications of Synthetic Data in RAG Systems
Augmenting and Diversifying Training Data
Domain Adaptation and Transfer Learning
When you operate with RAG systems, synthetic data can play a critical role in domain adaptation and transfer learning. By producing synthetic data that closely imitates the target domain, efficiently aqueducting the gap between distinct data domains becomes possible. This technique permits you to refine pre-trained models on artificial datasets, permitting them to adjust to new domains without the requirement for comprehensive real-world data. Transfer learning becomes more effective, as the artificial data offers a rigid foundation for the models to construct upon.
Addressing Data Scarcity and Bias
The shortage of high-quality training data presents a predominant challenge in constructing RAG systems. Synthetic data can help you conquer this obstacle by producing large volumes of data that resemble real-world datasets. Not only does this procedure satisfy data scarcity, but it also allows for the introduction of variety into the training data, acknowledging possible bias. By carefully composing artificial data to include underrepresented outlines and edge cases, ensuring that models become more rigid and less liable to impartiality becomes your responsibility.
Enhancing Retrieval Components
Expanding Knowledge Bases and Corpora
For RAG systems, the standard and thoroughness of the knowledge base or corpus are uppermost. Synthetic data permits you to develop these resources substantially. By producing synthetic documents, accelerating existing corpora with pertinent details that might be missing from the original dataset becomes achievable. This enlargement improves the length and depth of the knowledge base, offering an affluent context for the retrieval procedure.
Improving Retrieval Performance and Relevance
Synthetic data can also enhance the performance and pertinence of the retrieval elements in RAG systems. By integrating artificial data that reflects the queries and details required of users, you can refine retrieval algorithms to better comprehend and respond to varied queries. This tailored approach ensures that the retrieval system returns more precise and pertinent outcomes improving the overall user experience.
Enriching Generation Components
Fine-Tuning Language Models
In the generation stage of RAG systems, synthetic data proves indispensable for fine-tuning language models. By training models on synthetic data that indulges numerous verbal styles and contexts, you can improve their ability to produce coherent and conditionally pertinent responses. This fine-tuning process ensures that the language models are better prepared to manage the intricacies of natural language, leading to more engaging and natural results.
Improving Fluency, Coherence, and Factual Accuracy
Finally, synthetic data can help you enhance the proficiency, coherence and accuracy of produced content. By producing artificial datasets that accentuate these standards, you can train models to prioritize them in their results. This focus results in the creation of text that is not only grammatically accurate and logically constant but also factually precise. The outcome is a more dependable and trustworthy RAG system that users can rely on for high-quality details.
Alright, now that we've navigated the ins and outs of synthetic data and its impact on RAG applications, let's dive deeper into the ethical jungle. How do we ensure that our pursuit of advancement respects privacy and dodges the pitfalls of bias? Hang tight, because this next section is crucial.
Challenges and Considerations
Privacy and Ethical Concerns
Privacy and ethical concerns are predominant when dealing with artificial data. Synthetic data must be produced in a way that doesn’t accidentally disclose sensitive details from the original datasets. Ensuring obscurity while handling the usefulness of the data is challenging. Ethical considerations also lengthened to how this data is utilized. For example, synthetic data shouldn’t be used to betray or mislead investors. Establishing clear instructions and following ethical standards are needed by organizations to navigate these concerns efficiently.
Ensuring Synthetic Data Quality and Realism
Ensuring quality and authenticity of synthetic data is crucial. Poor-quality synthetic can result in imprecise models and undependable results. One often uses methods like Generative Adversarial Networks (GANs) and Variational Autoencoders (VAEs) to generate high-quality synthetic data. These methods must be carefully measured to produce data that closely reflects real-world circumstances. Verification processes, including cross-verification with real-data, are important to validate the loyalty and usefulness of synthetic datasets.
Potential for Amplifying Biases and Artifacts
Synthetic data generation can accidentally distend impartiality present in the original dataset. If the source data contains impartiality, these can be regenerated and even enlarged in the artificial data. Acknowledging these problems needs rigid pre-processing and bias mitigation methods. It’s important to locate and eliminate biases in the training data before producing synthetic datasets. Constant observation and adaptation are important to decrease the risk of bias mitigation.
Balancing Synthetic and Real-World Data
Balancing the use of synthetic and real-world data is a fragile task. While synthetic data offers benefits such as scalability and privacy, it should complement rather than replace real-world data. The blend approach, using both synthetic and real data, often yields the best outcomes. Real-world data is essential for verification and evaluation reasons, ensuring that models trained on synthetic data stays pertinent and precise.
Computational Resources and Scalability
Important arithmetical resources are required to produce high-quality synthetic data. The processes involved, especially with advanced methods such as GANs, need strong hardware and significant processing time. Scalability becomes a solicitude as the volume of data increases. Investing in rigid infrastructure and upgrading their arithmetical workflows is a must for organizations to manage these demands effectively. Cloud calculating and allocating processing are possible solutions to acknowledge scalability difficulties.
Case Studies and Real-World Examples
Organizations and Research Groups Using Synthetic Data for RAG Applications
At the vanguard of using synthetic data for Retrieval-Augmented Generation (RAG) applications are several organizations and research groups. For instance, OpenAI uses synthetic data to improve the training of its language models. Similarly, IBM Research has developed synthetic data solutions to enhance their AI-Driven analytics platforms. These organizations use synthetic data to conquer restrictions in data attainability and improve model performance.
Successful Use Cases and Outcomes
The utilization of synthetic data in autonomous vehicle development represents one prestigious success story. Huge amounts of synthetic data are produced by companies such as Waymo and Tesla to affect driving scenarios. The approach has expedited the development and testing of their autonomous systems. Another instance is the healthcare sector, where synthetic data is utilized to expand the datasets of patients, assisting in the progression of diagnostic models without compromising the privacy of patients. These use cases substantiate the practical advantages and effectiveness of synthetic data.
Lessons Learned and Best Practices
From these applications, numerous lessons become apparent. Initially, it is important to handle a rigid verification process to ensure synthetic data quality. Secondly, acknowledging impartiality from the beginning can avert problems down the line. Third, a hybrid approach mixing synthetic with real data, often yields superior outcomes. Finally, investing in scalable infrastructure and embracing cloud-based solutions can lessen arithmetical challenges. These best practices provide a strategy for organizations looking to use synthetic data efficiently.
Conclusion
Synthetic data plays a crucial role in enriching RAG applications, acknowledging shortage of data and bias, and improving overall model performance. Going forward, the liable and ethical utilization of synthetic data will be critical for boosting its advantages. By using synthetic data considerably , you can uncover new possibilities in your RAG applications, making them more rigid, precise and fair.
Subscribe to our newsletter to never miss an update
Subscribe to our newsletter to never miss an update
Other articles
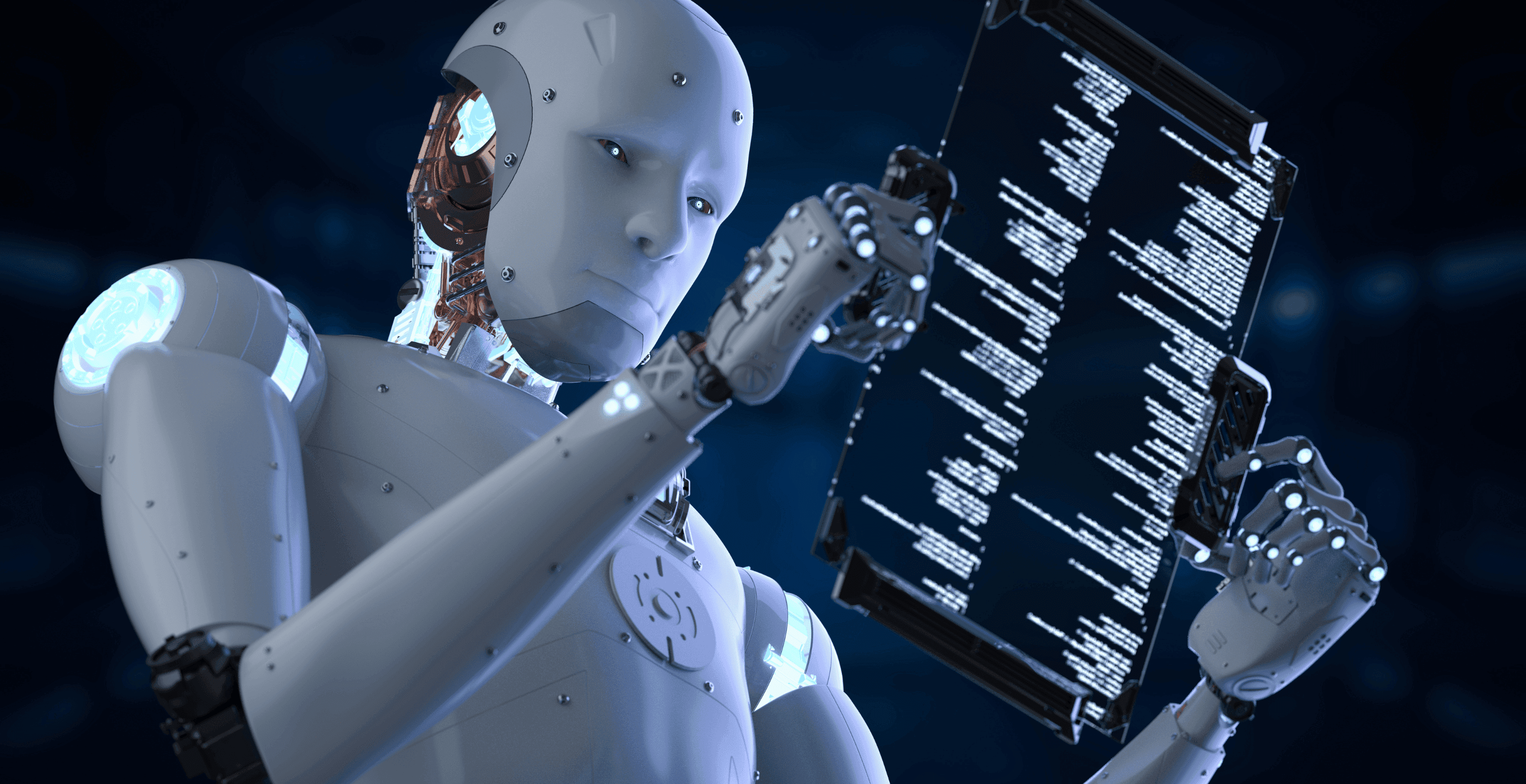
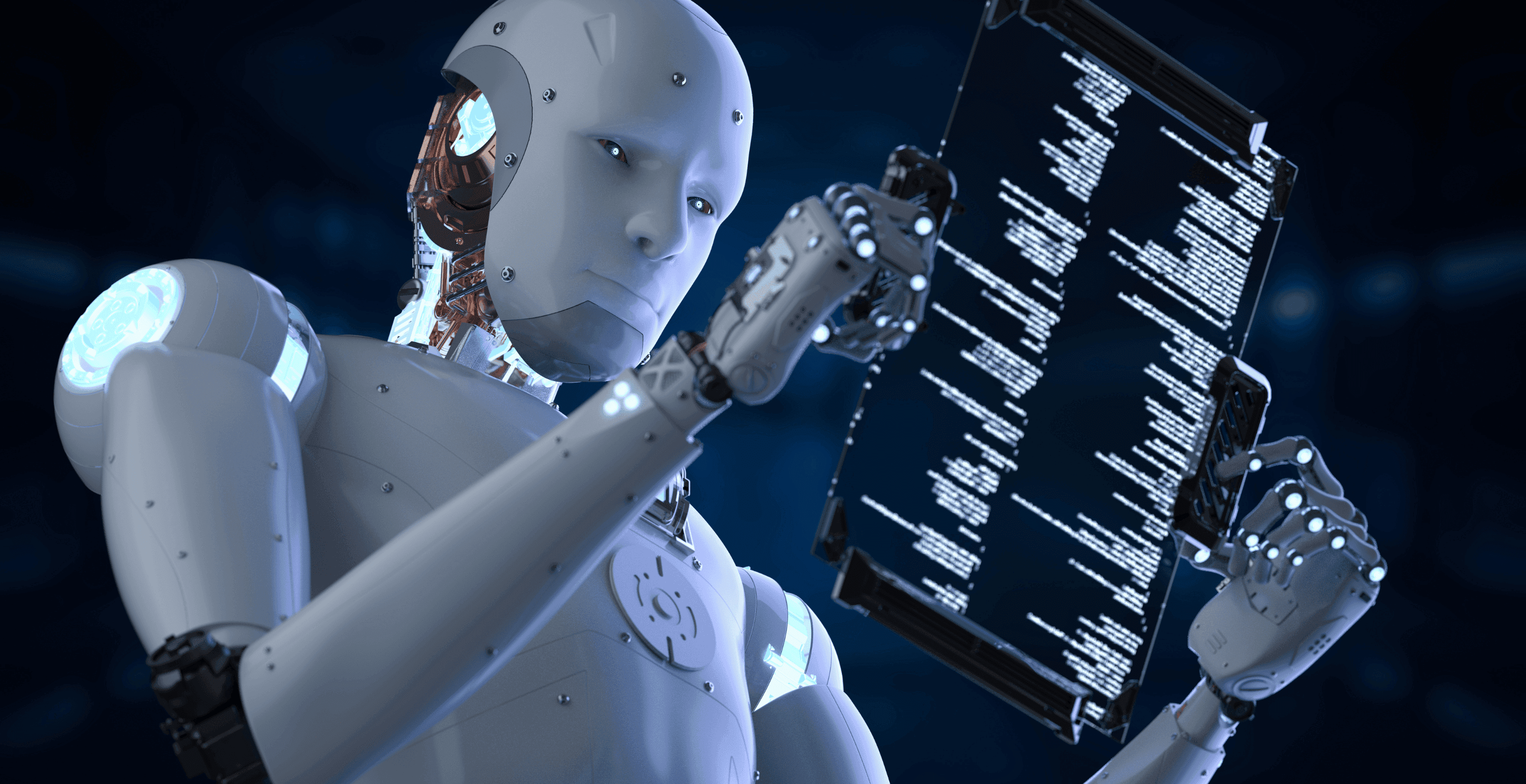
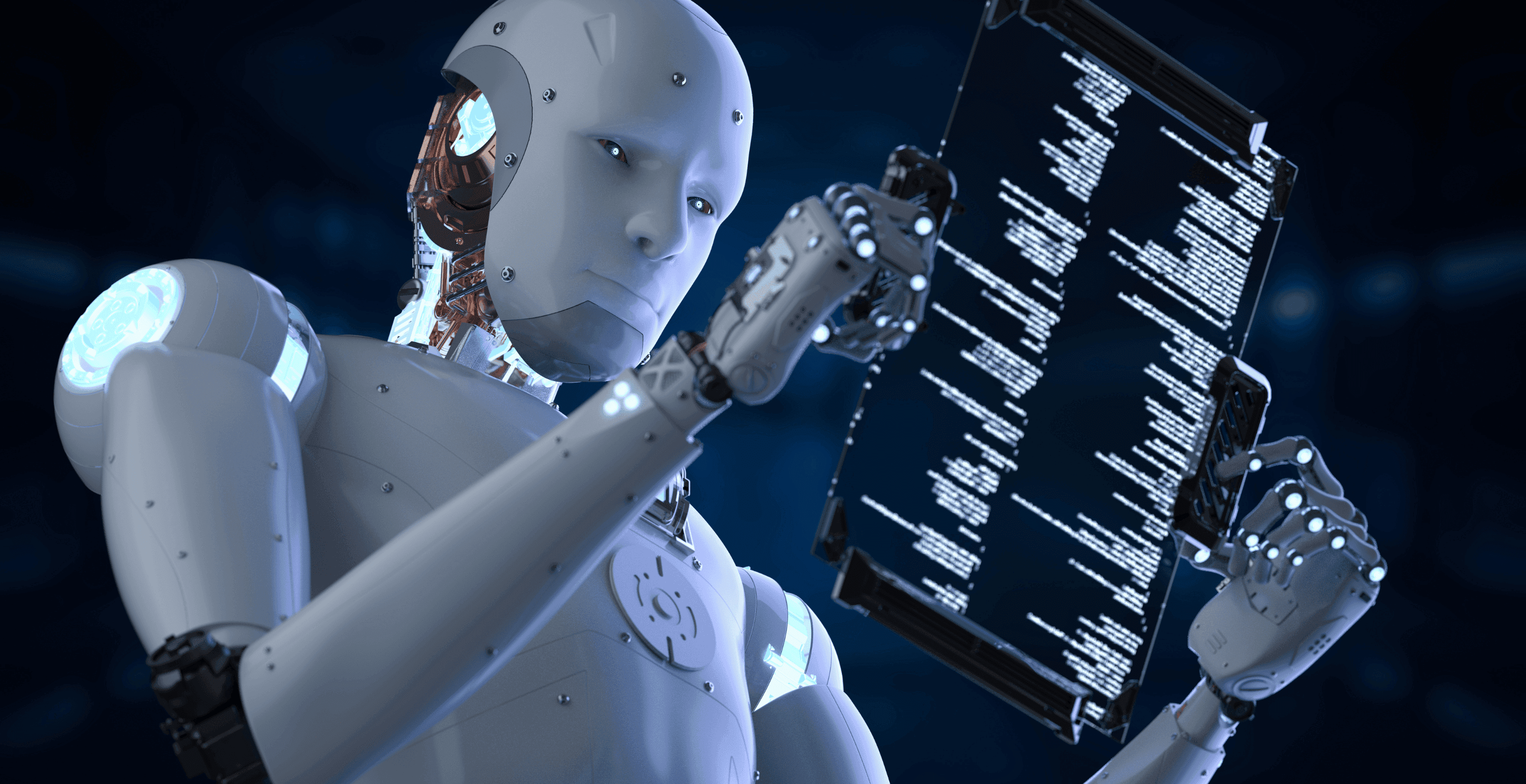
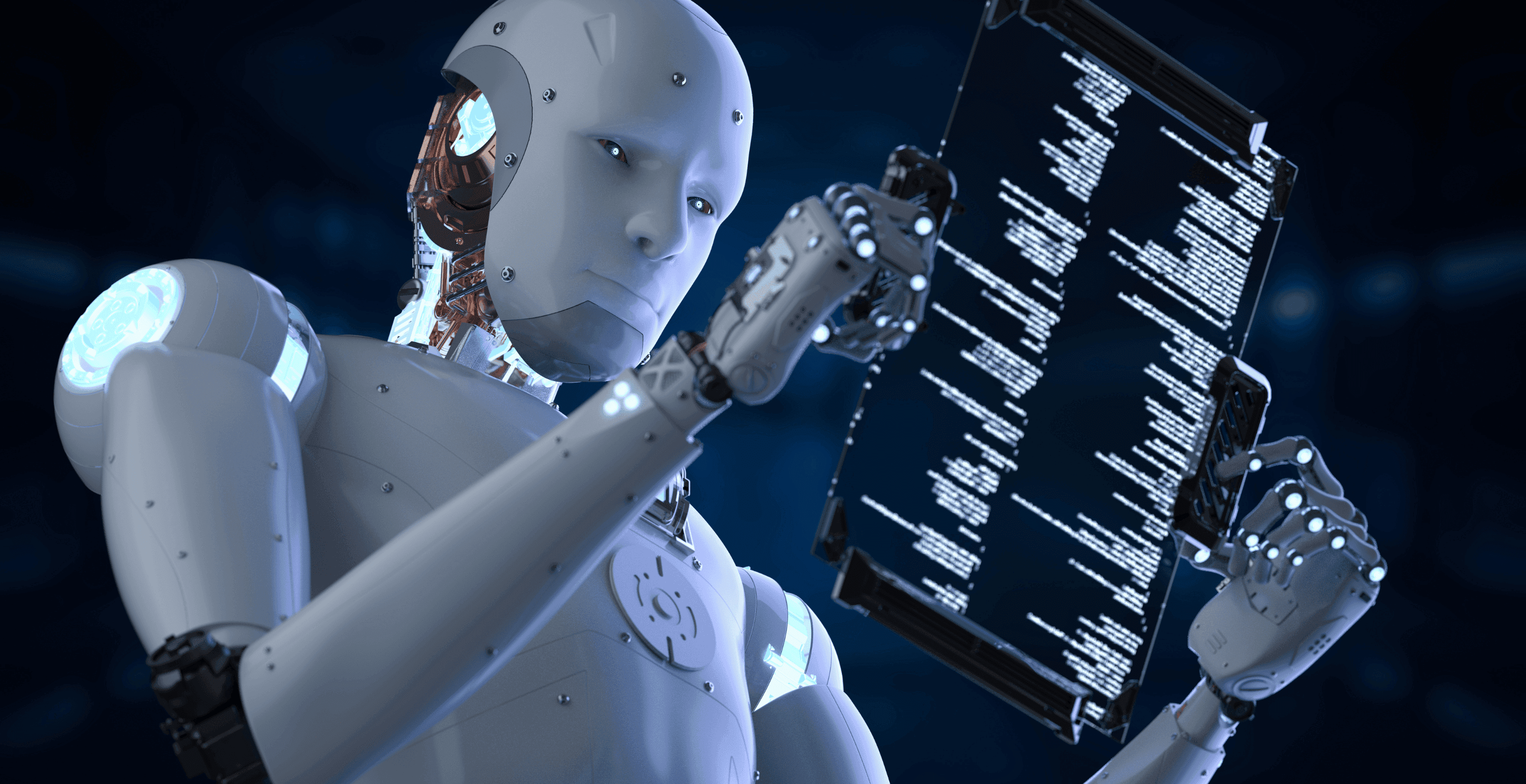
Exploring Intelligent Agents in AI

Rehan Asif
Jan 3, 2025
Read the article
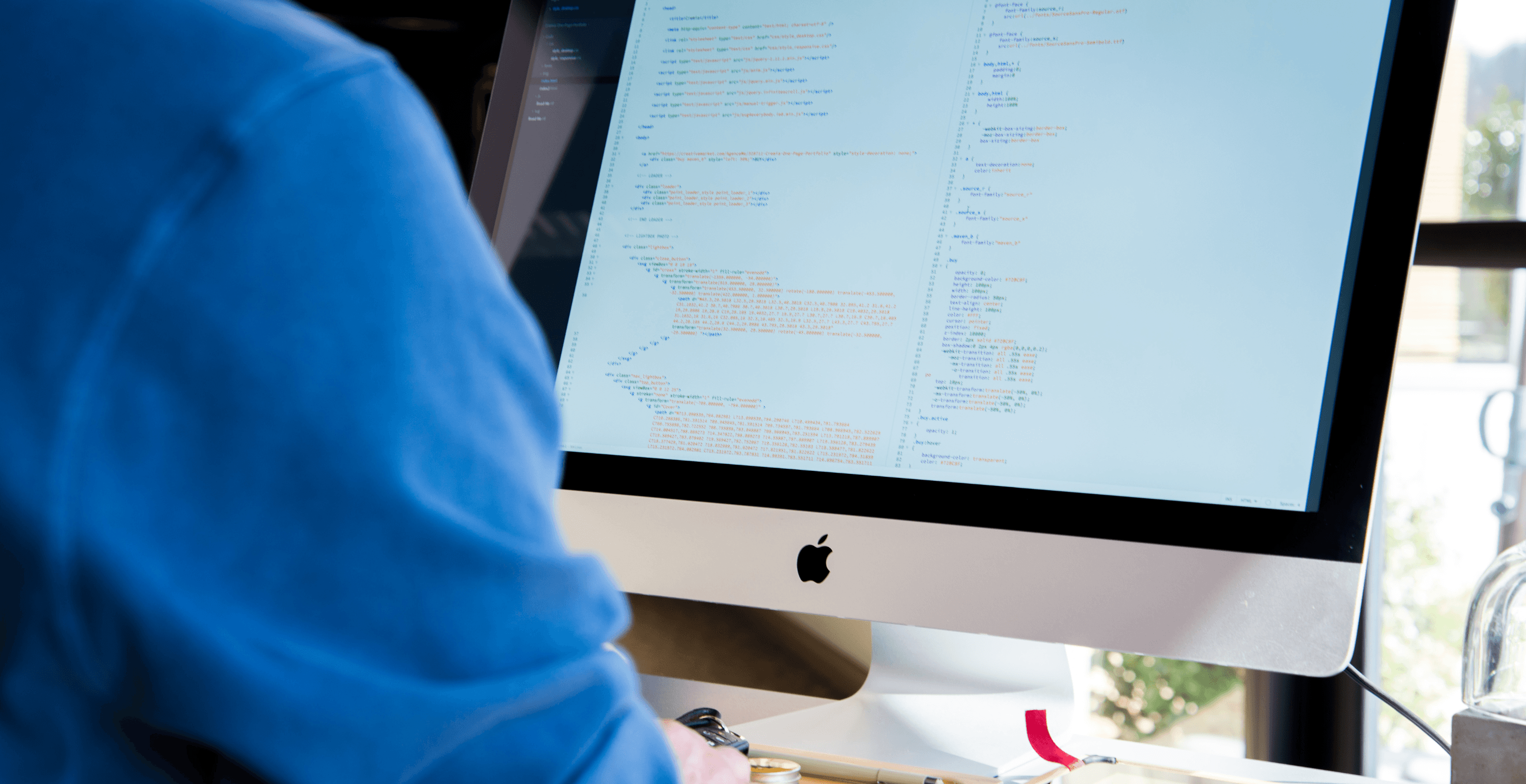
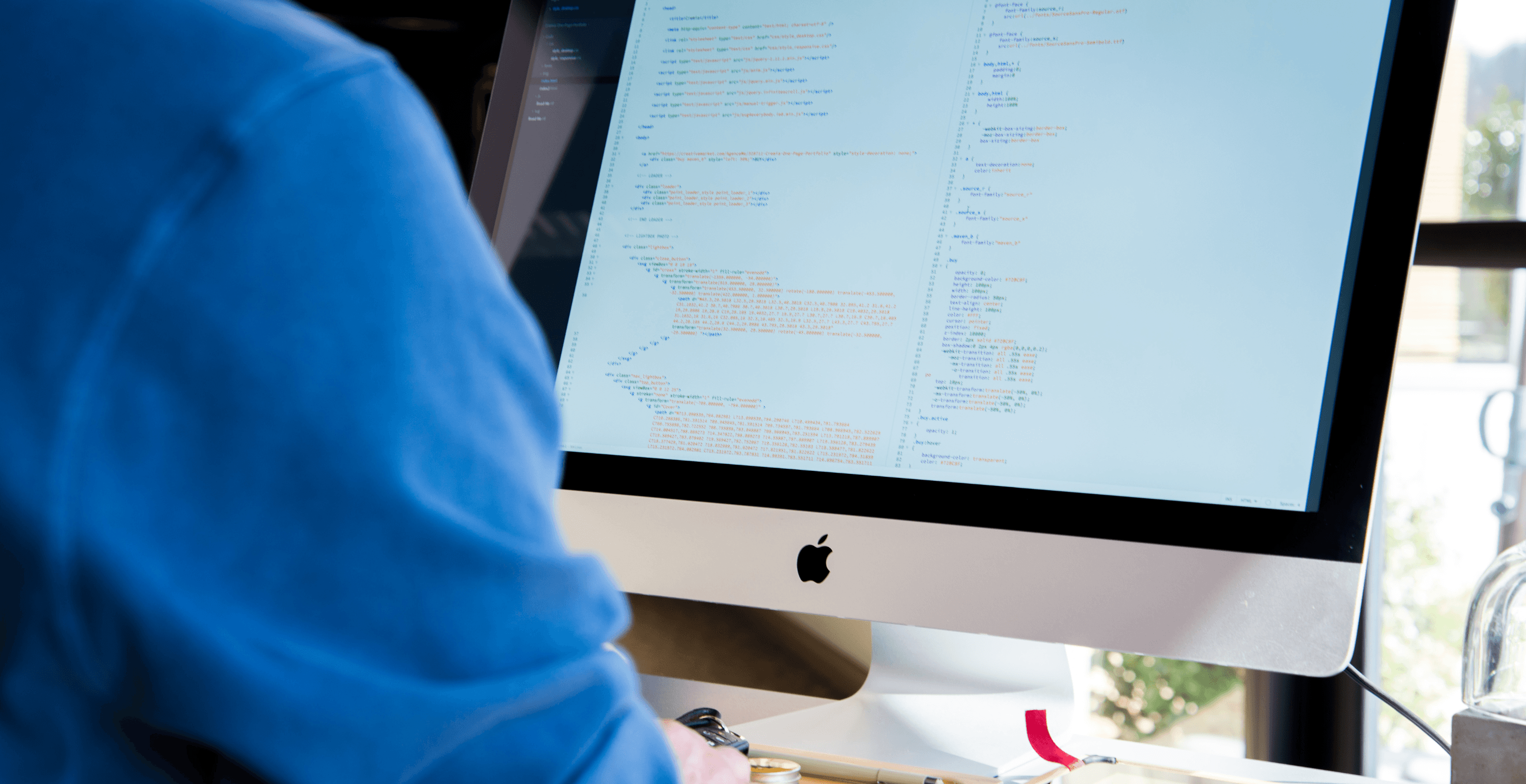
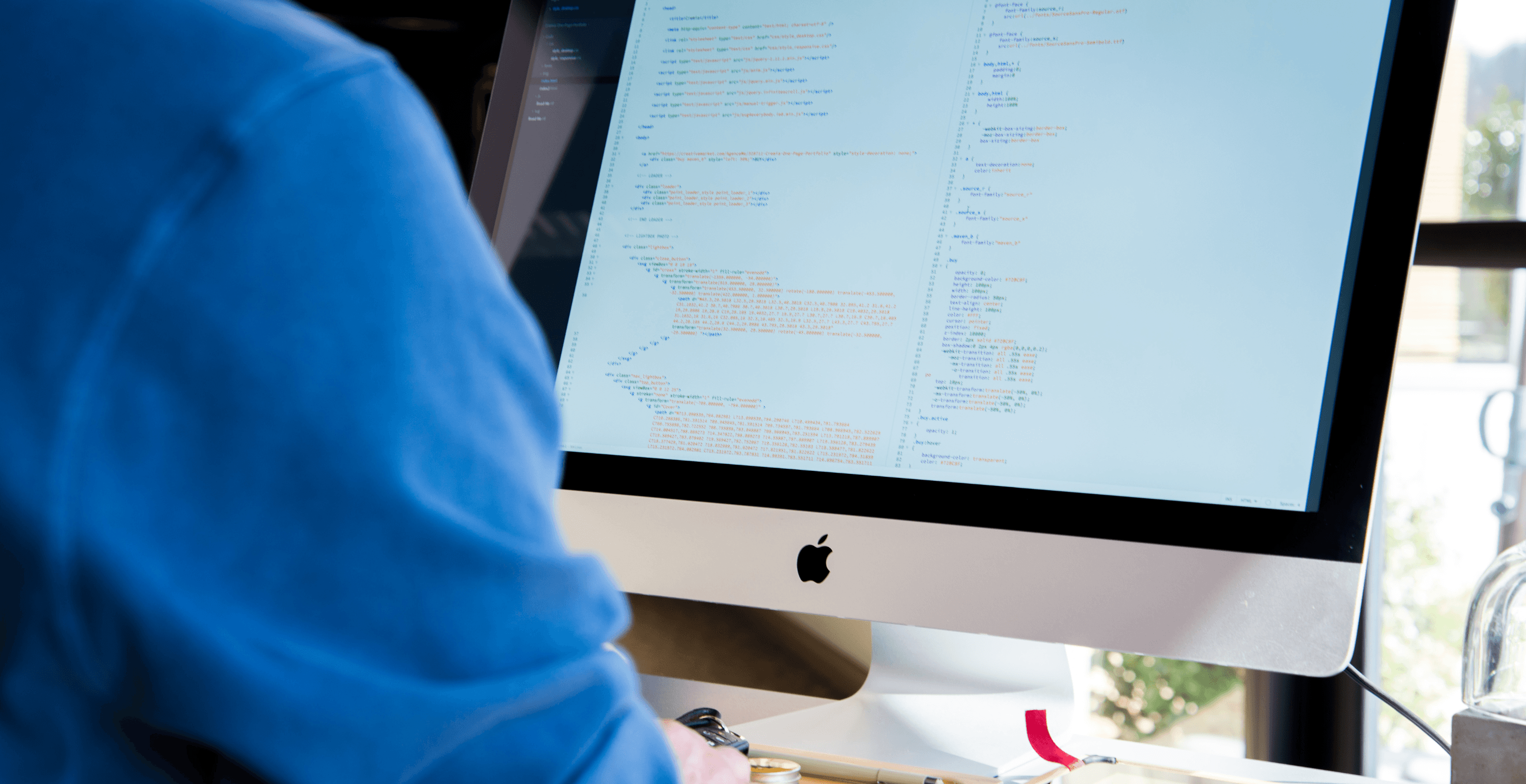
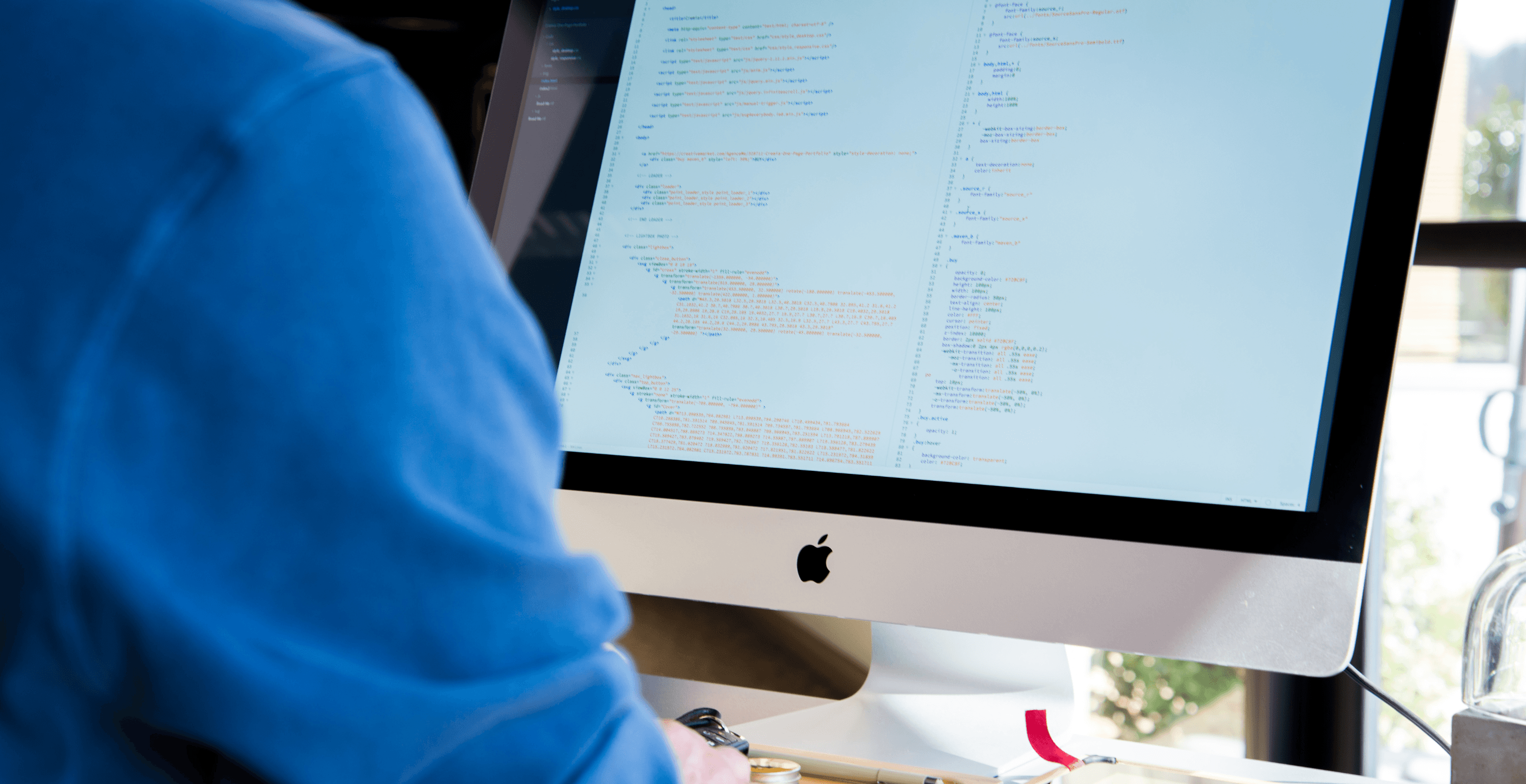
Understanding What AI Red Teaming Means for Generative Models
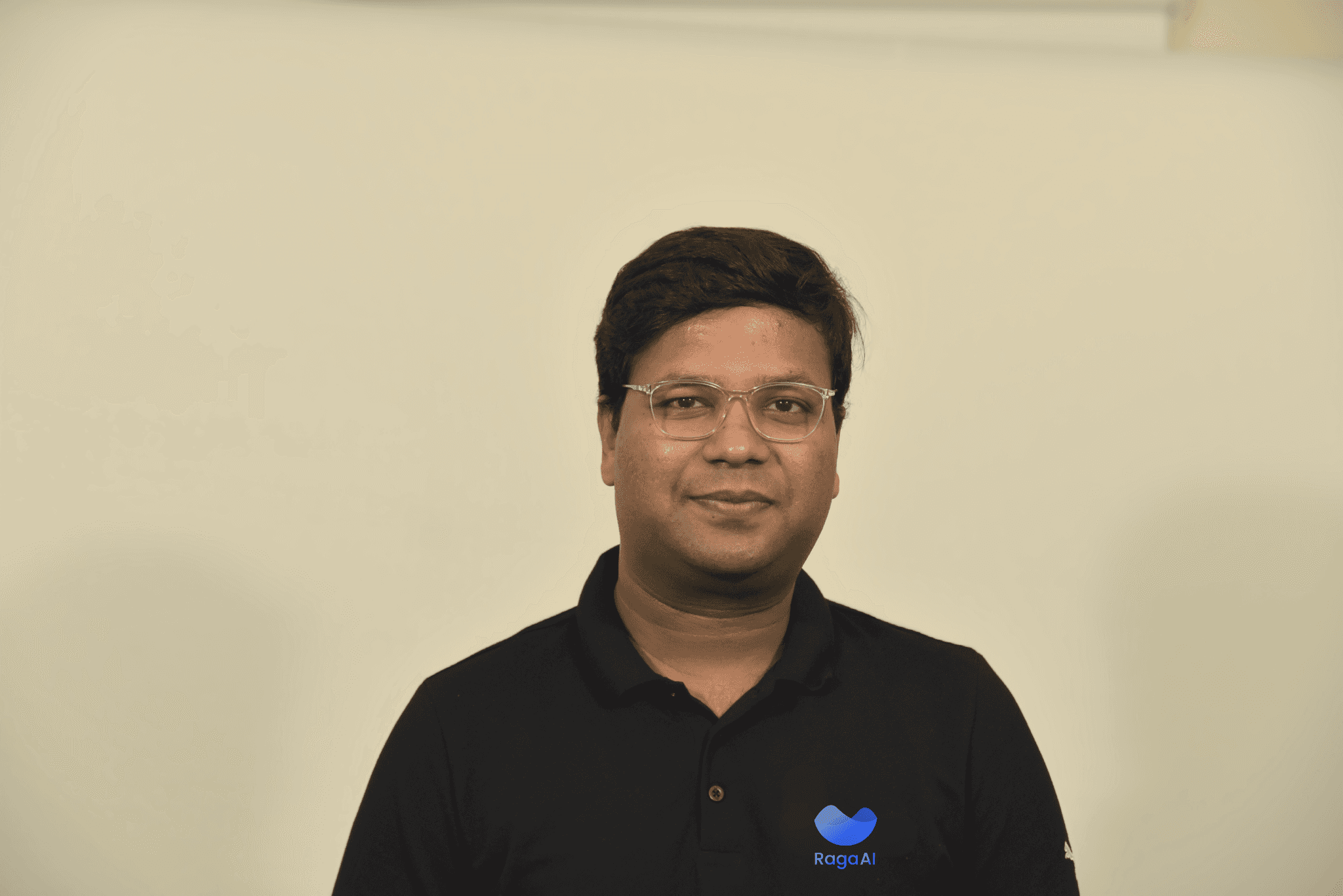
Jigar Gupta
Dec 30, 2024
Read the article
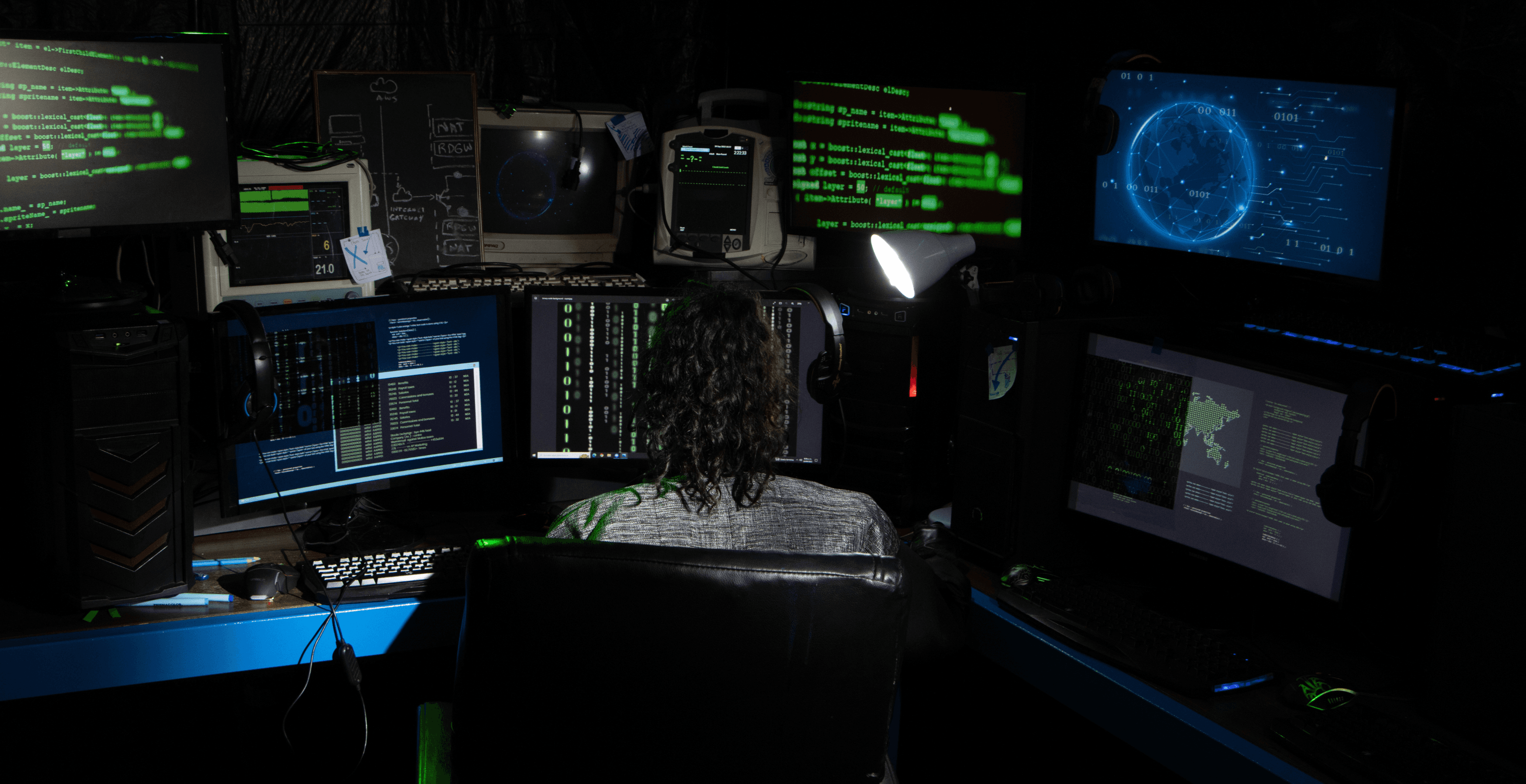
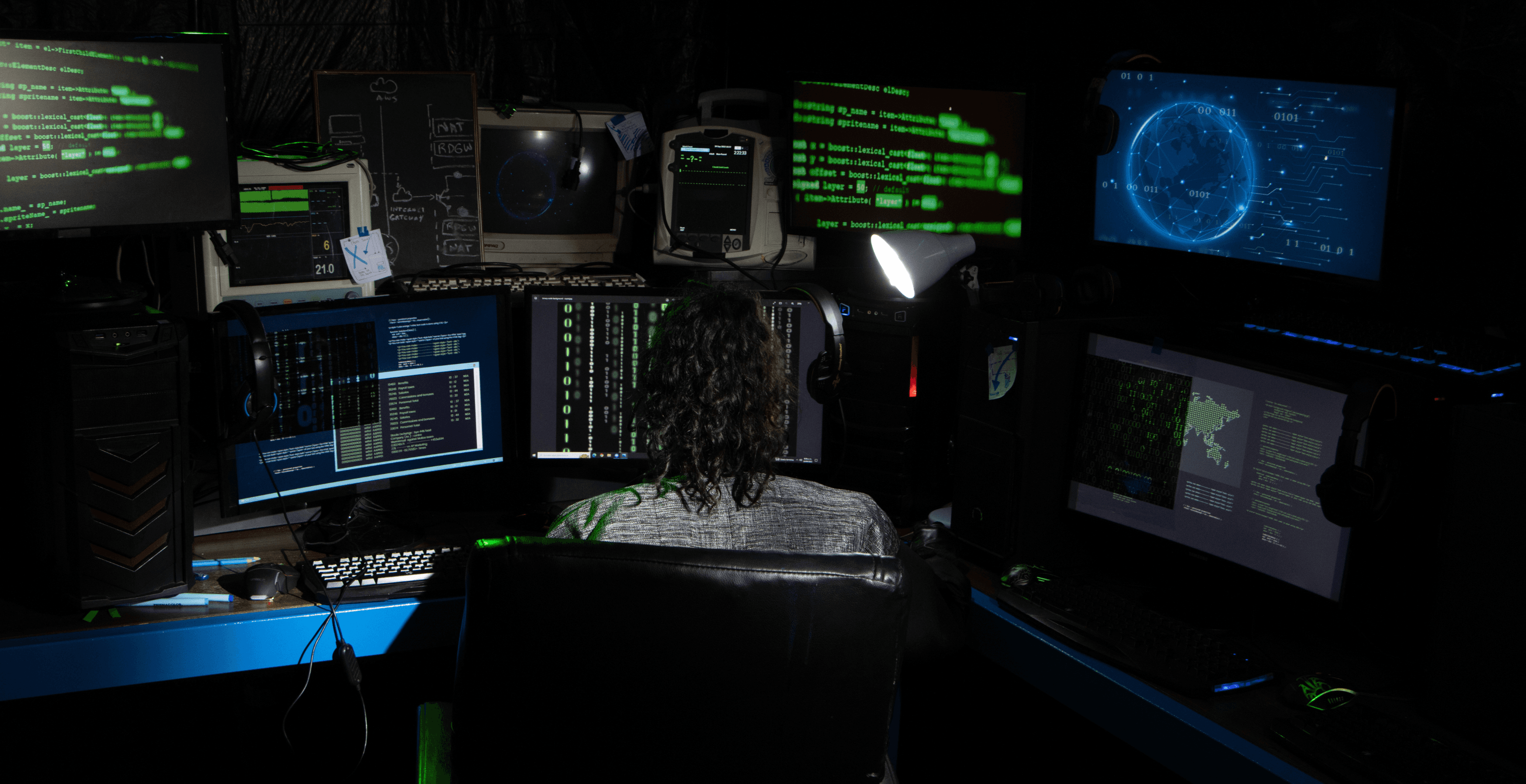
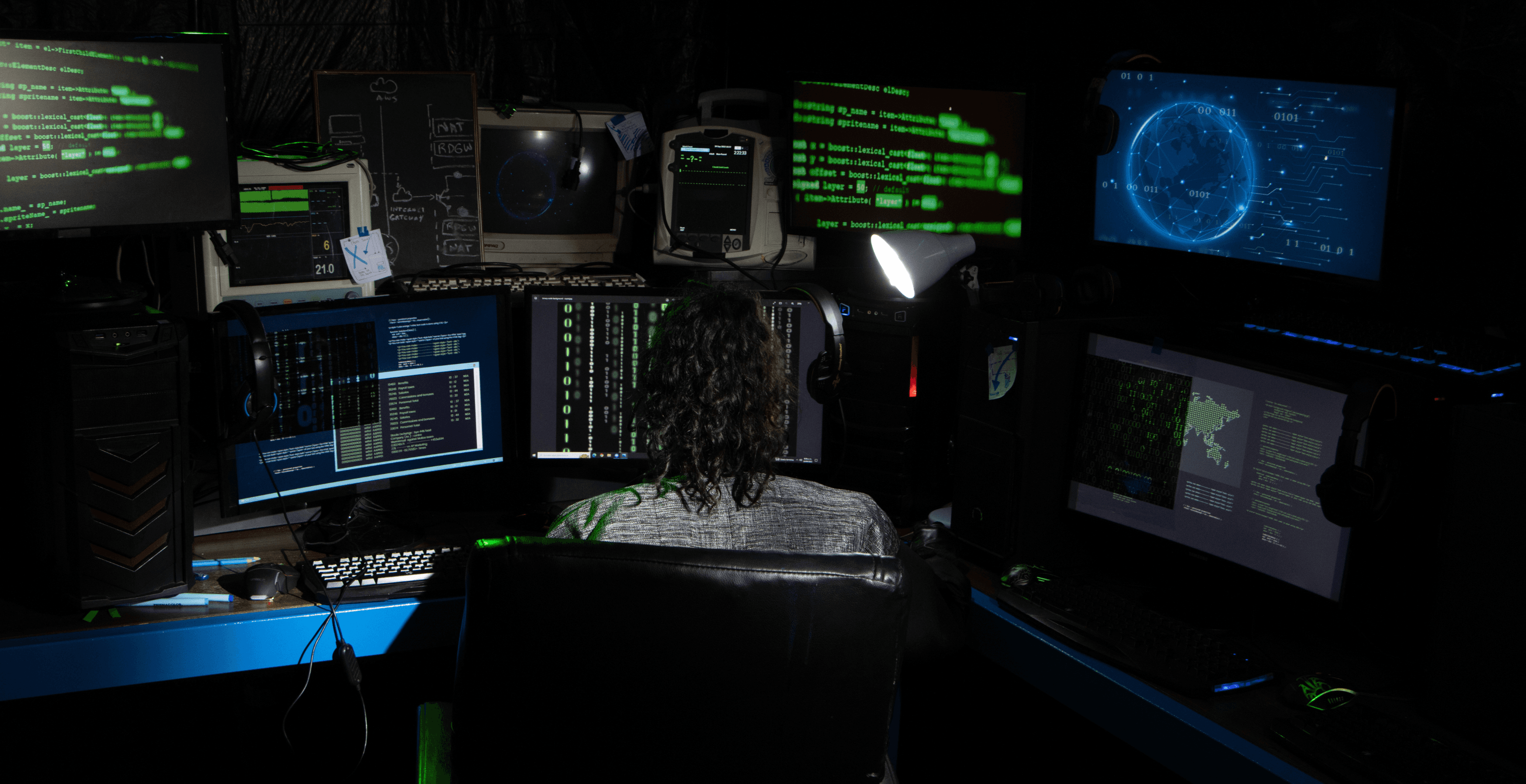
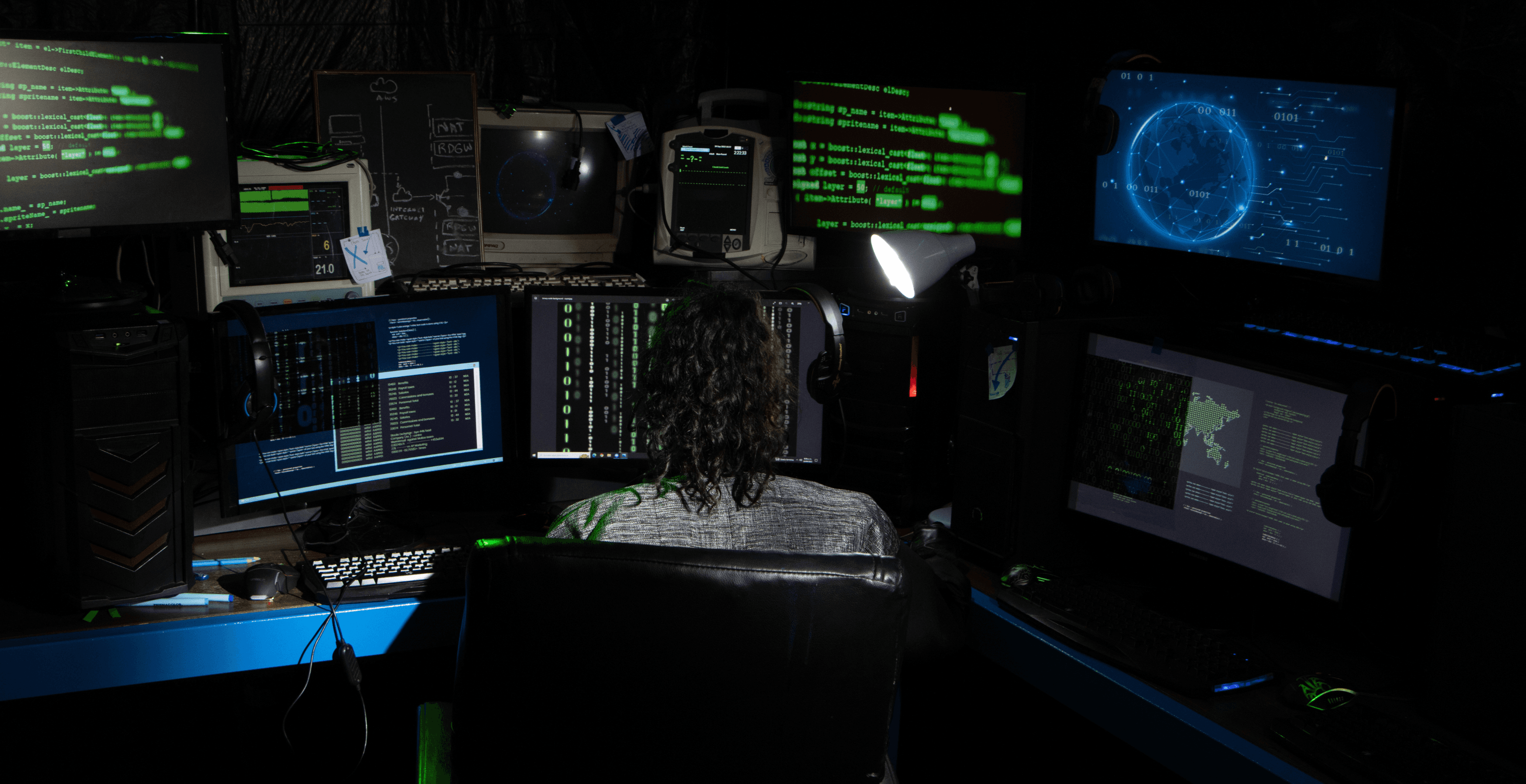
RAG vs Fine-Tuning: Choosing the Best AI Learning Technique
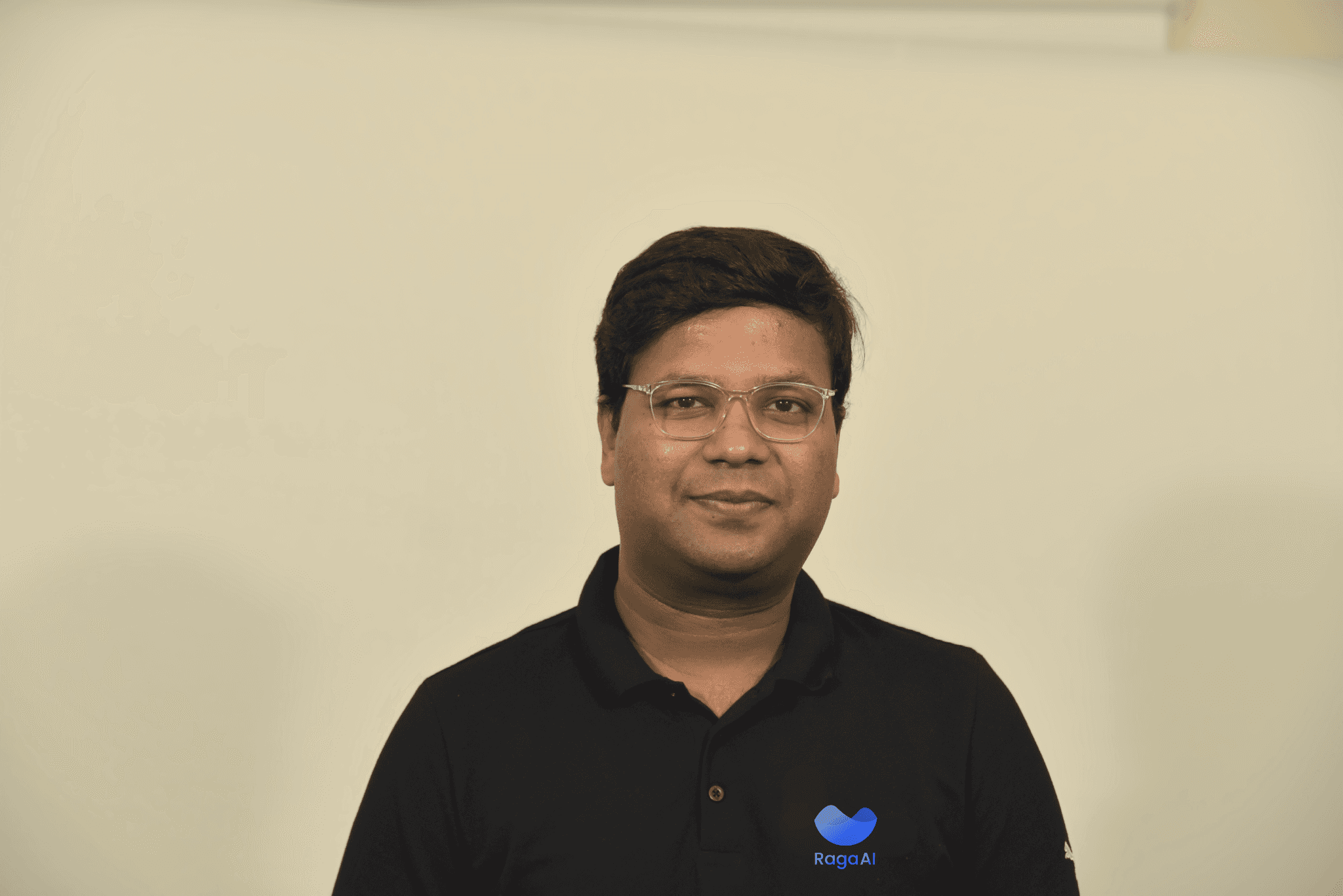
Jigar Gupta
Dec 27, 2024
Read the article
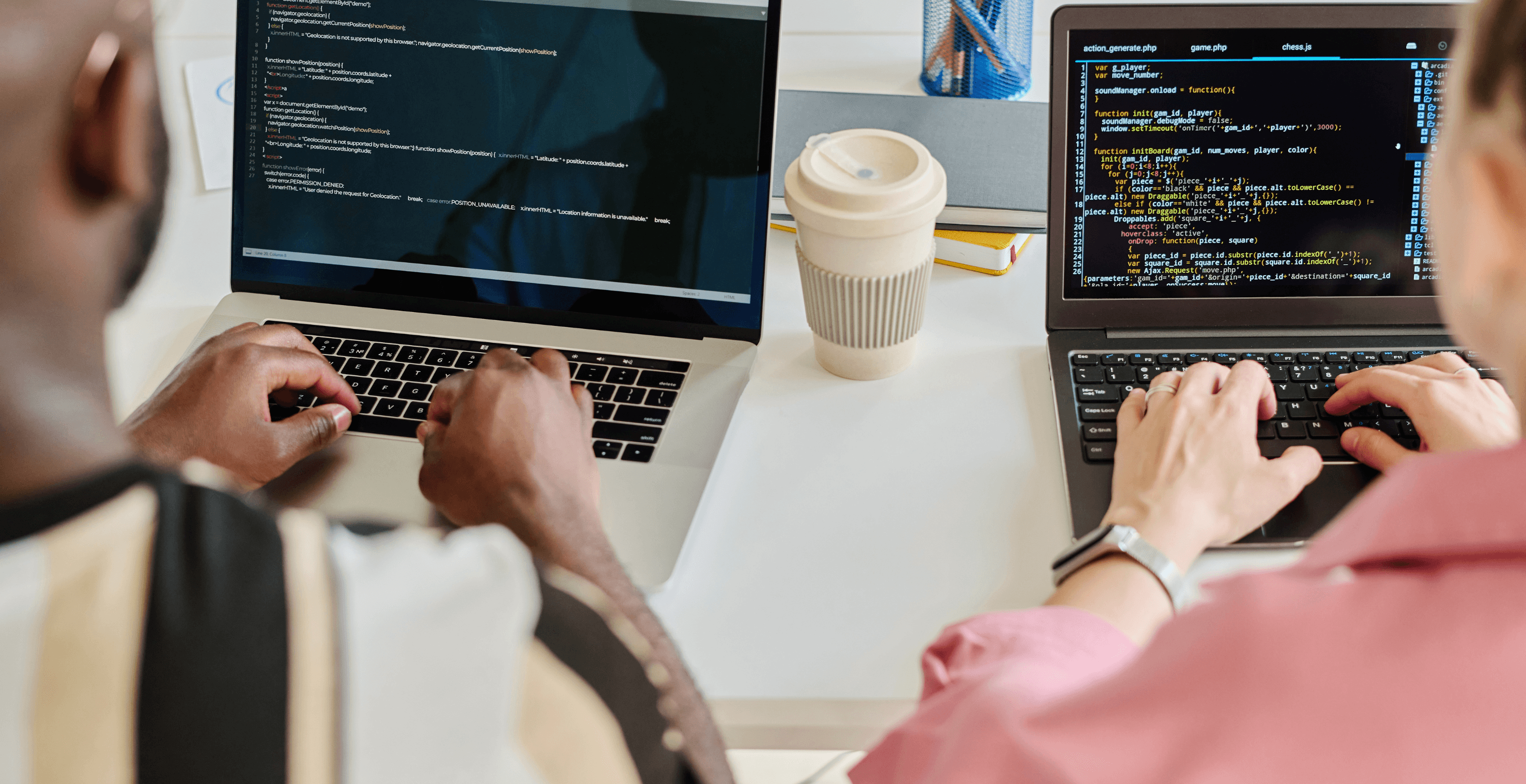
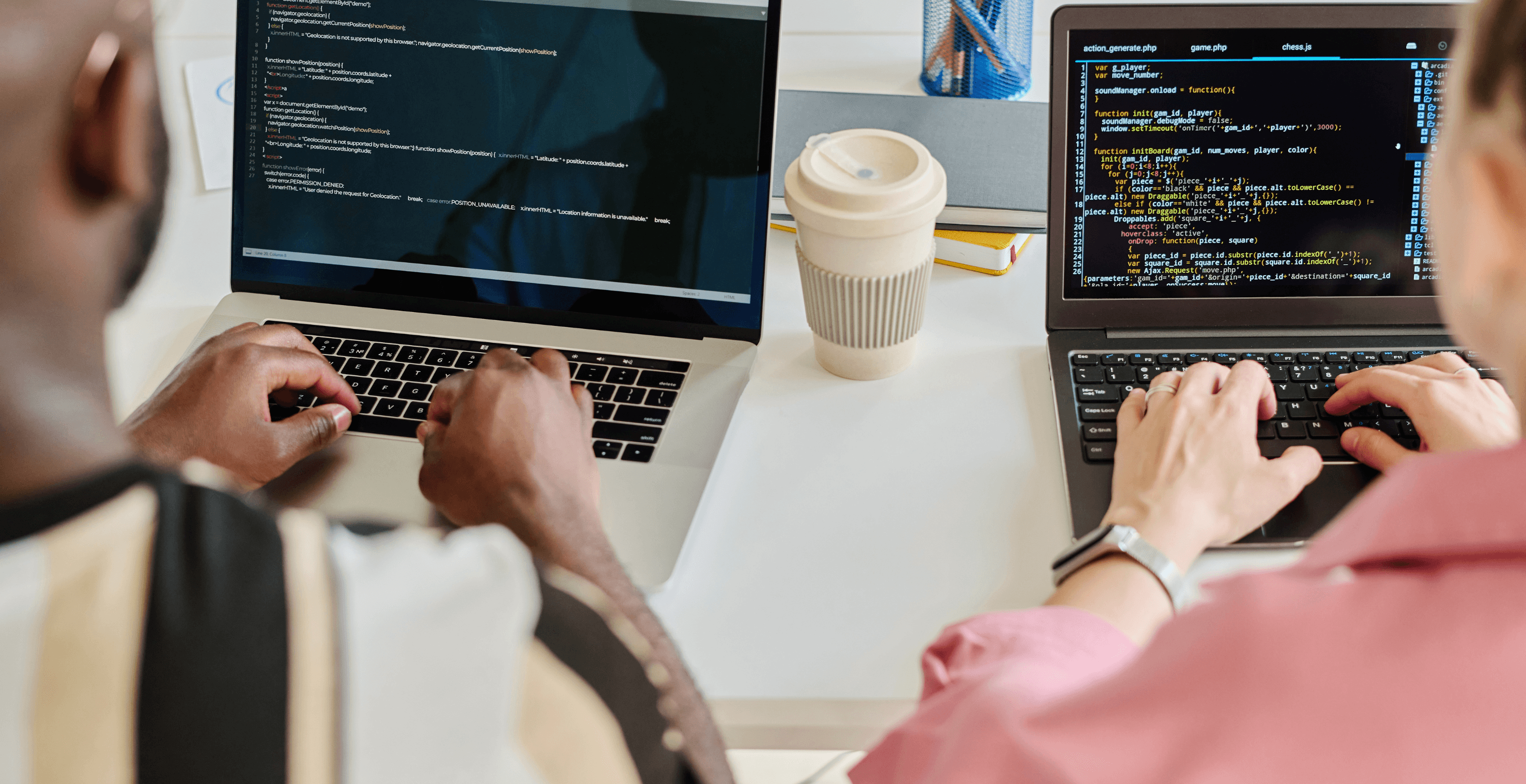
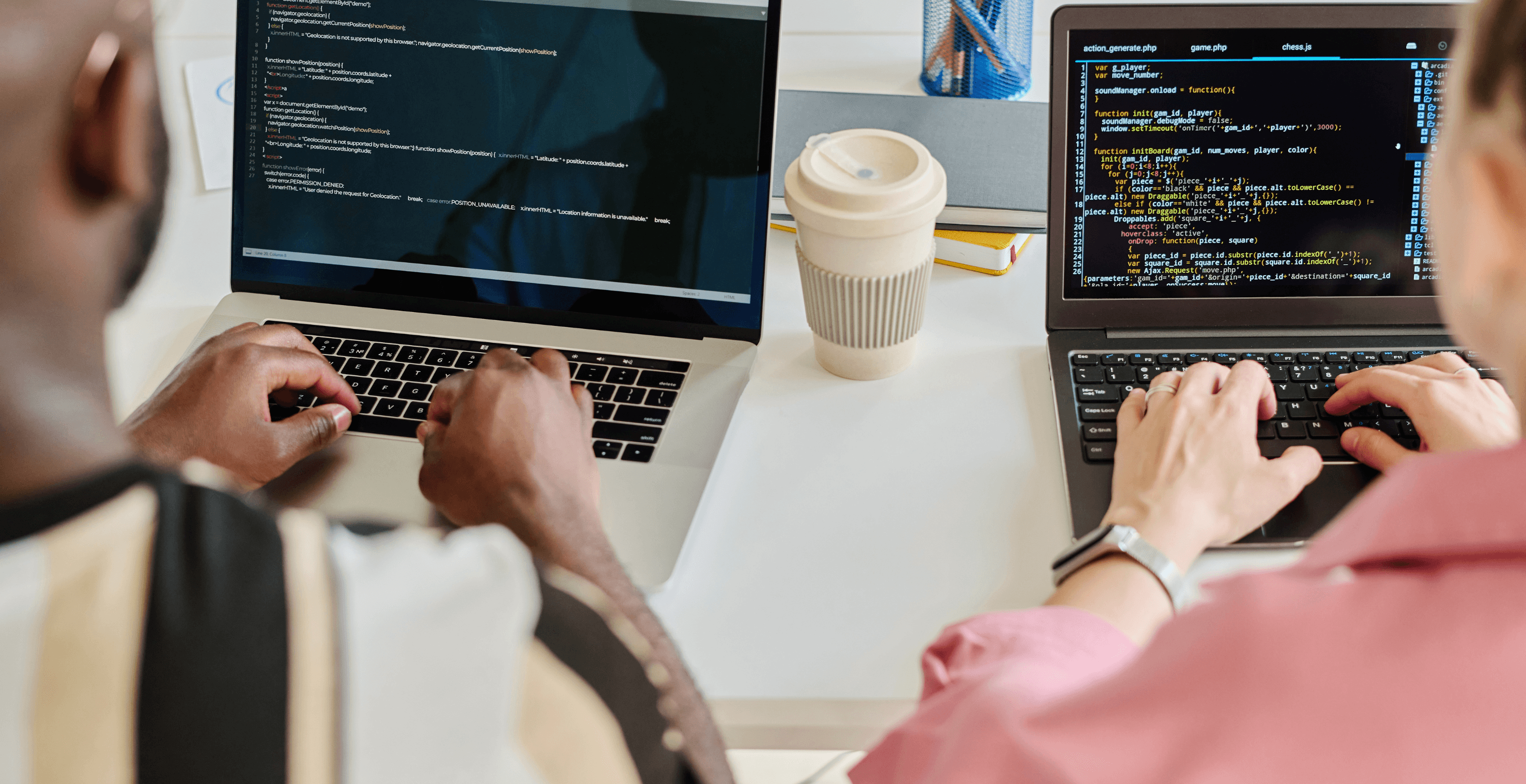
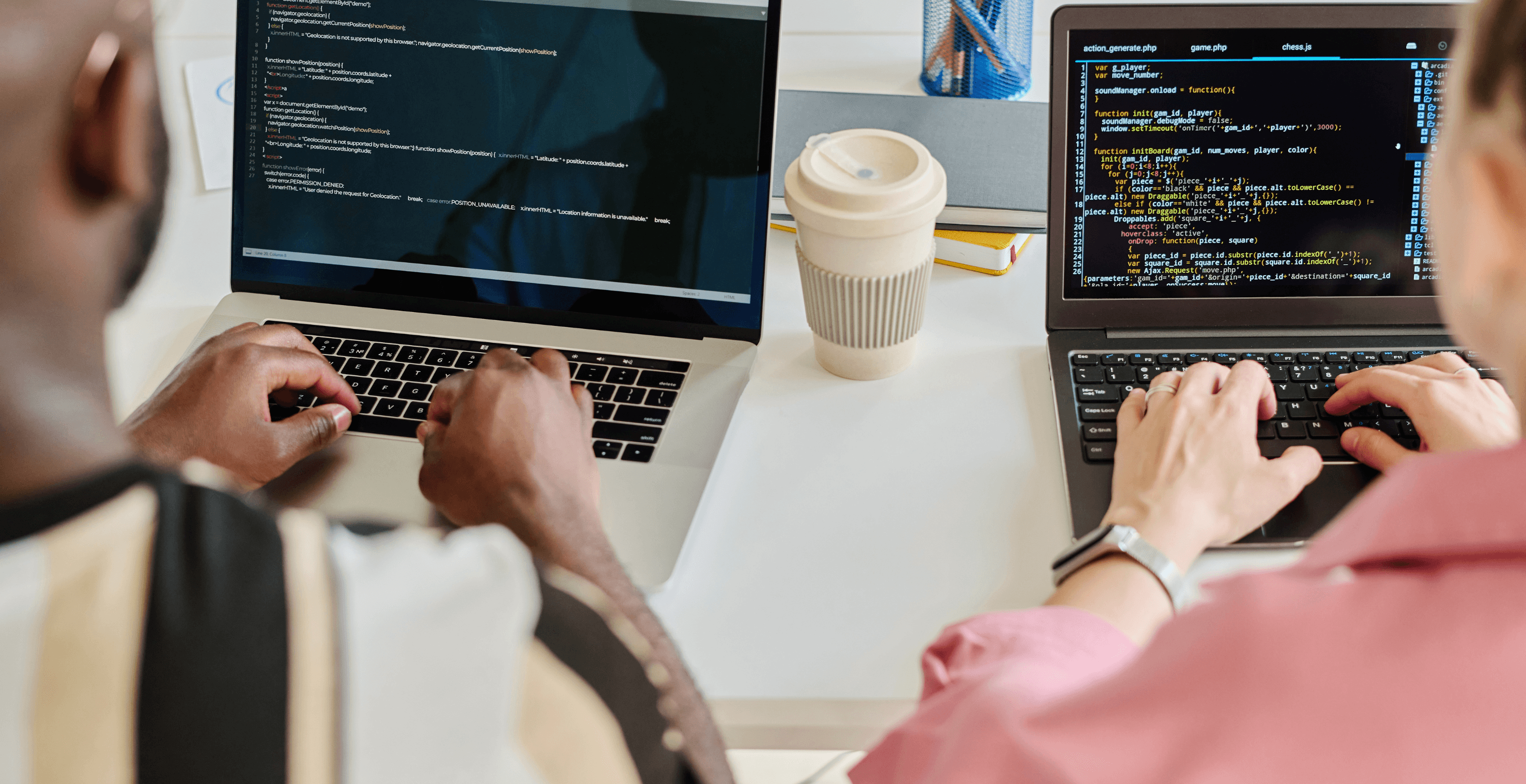
Understanding NeMo Guardrails: A Toolkit for LLM Security

Rehan Asif
Dec 24, 2024
Read the article




Understanding Differences in Large vs Small Language Models (LLM vs SLM)

Rehan Asif
Dec 21, 2024
Read the article




Understanding What an AI Agent is: Key Applications and Examples
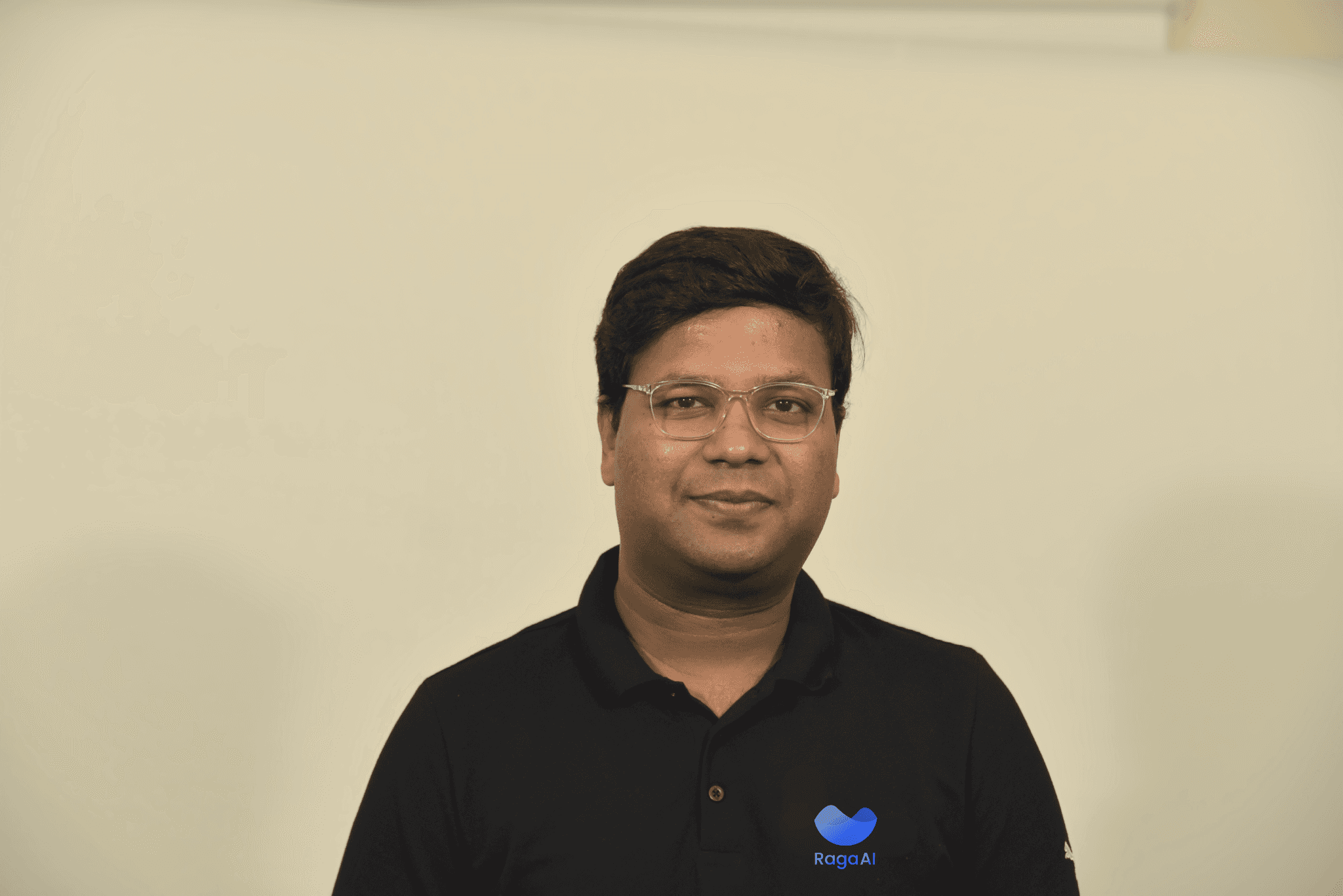
Jigar Gupta
Dec 17, 2024
Read the article




Prompt Engineering and Retrieval Augmented Generation (RAG)
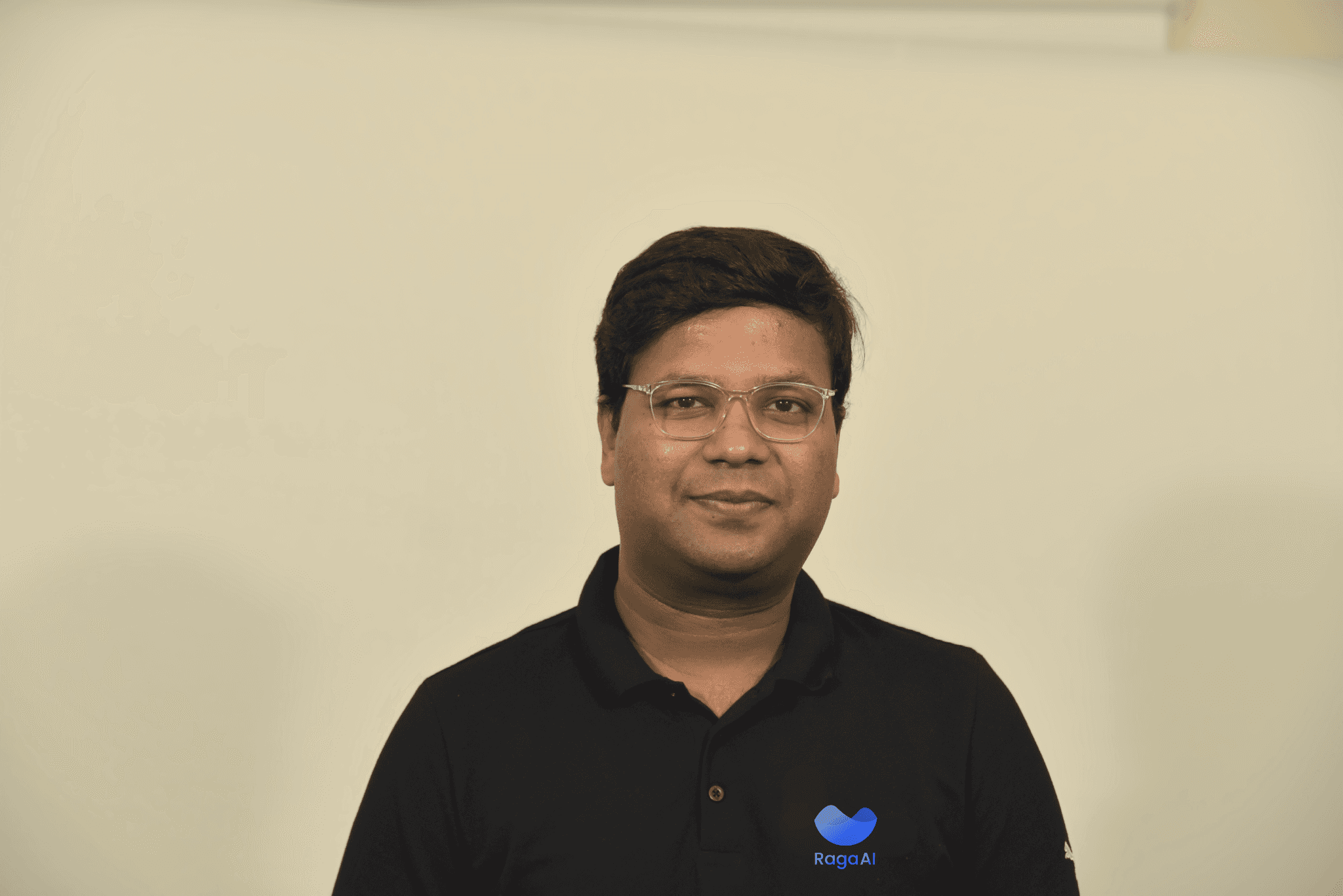
Jigar Gupta
Dec 12, 2024
Read the article




Exploring How Multimodal Large Language Models Work

Rehan Asif
Dec 9, 2024
Read the article
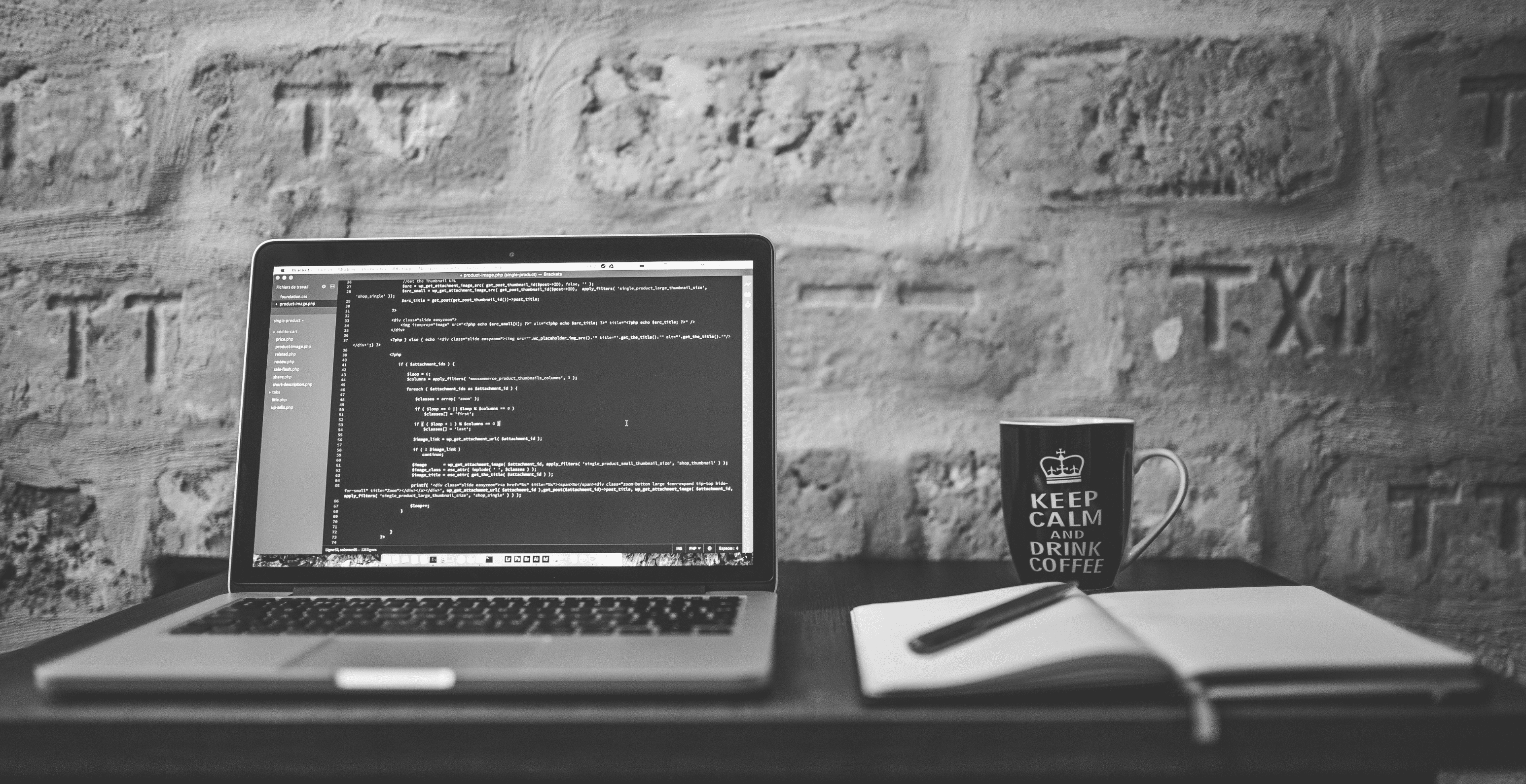
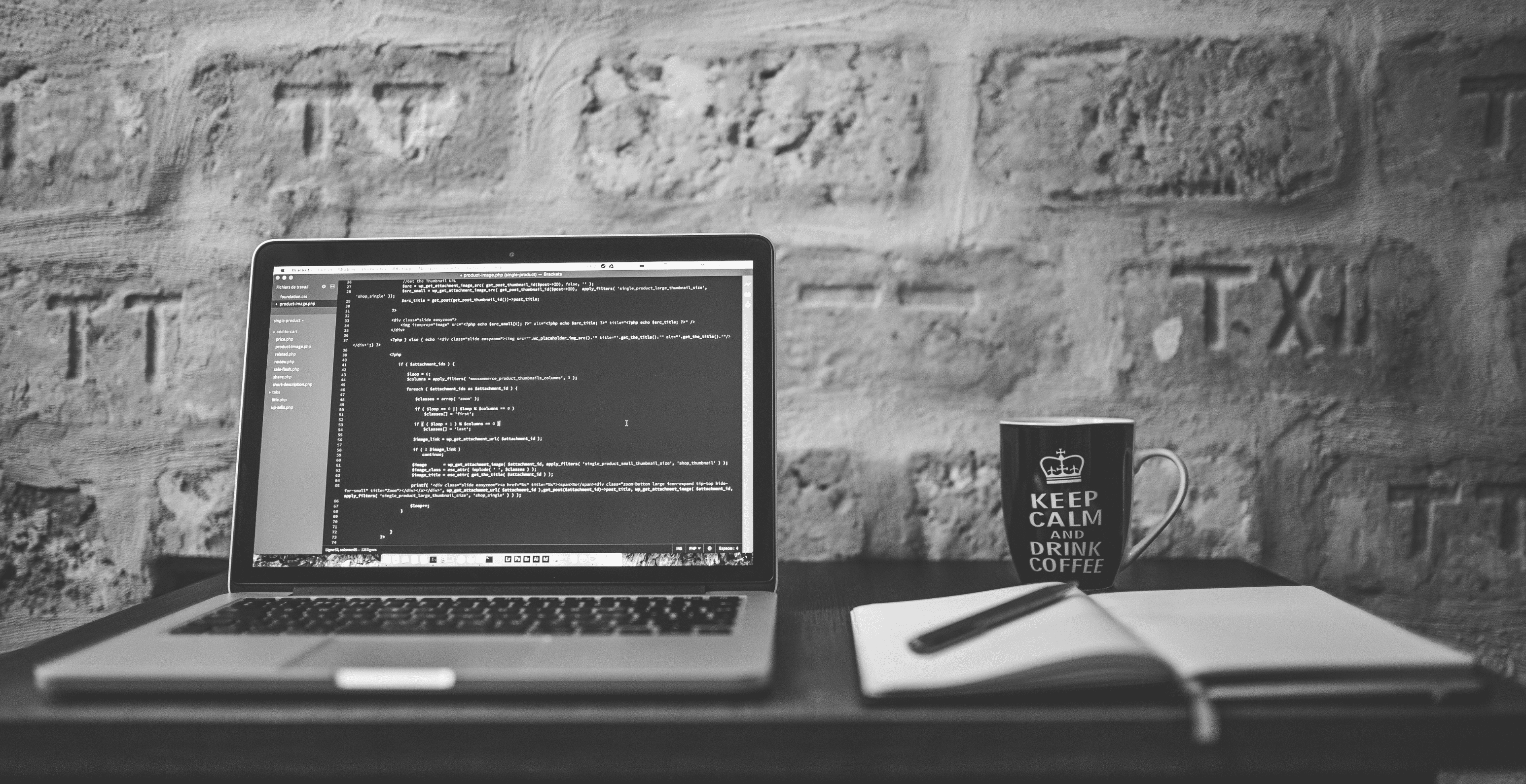
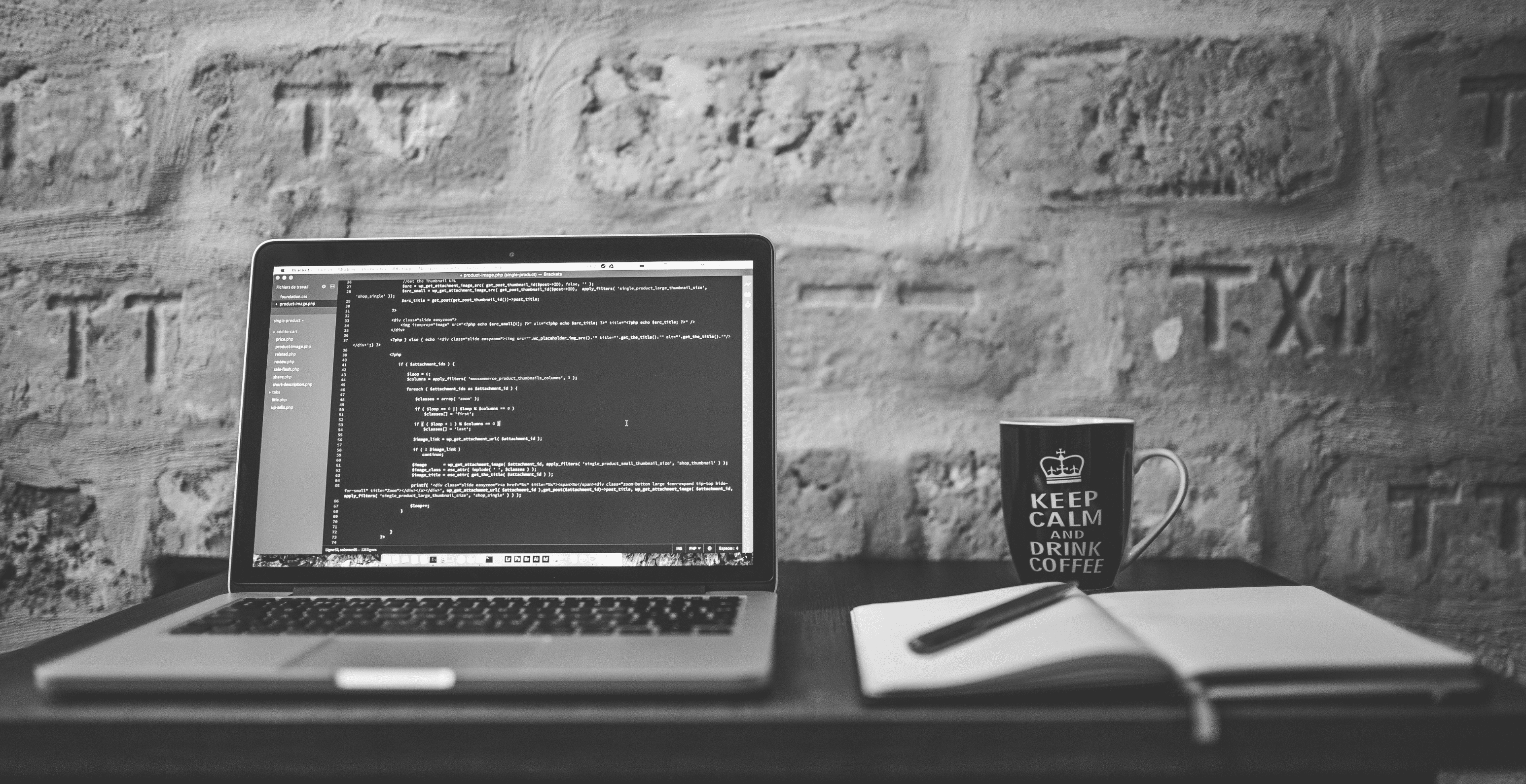
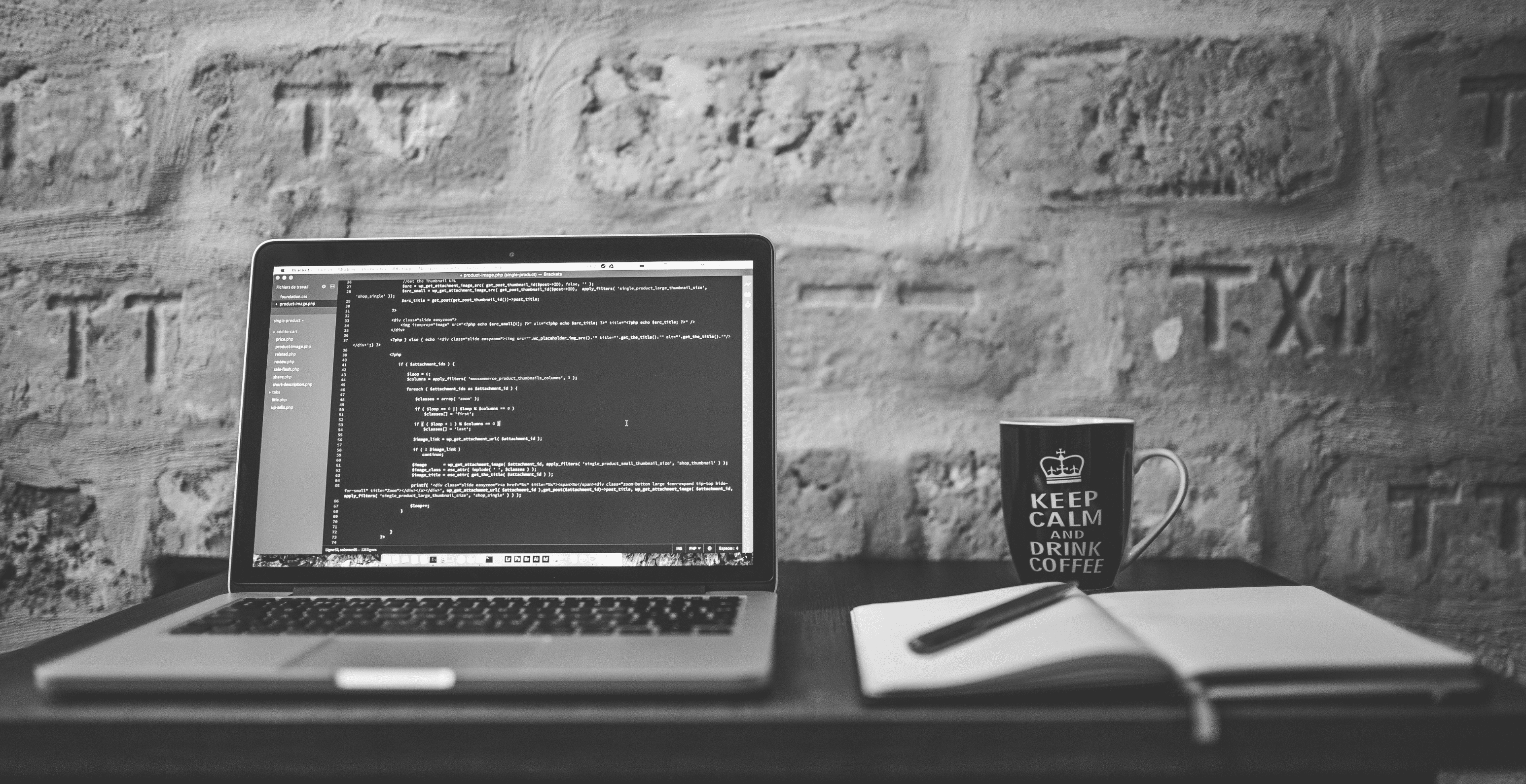
Evaluating and Enhancing LLM-as-a-Judge with Automated Tools

Rehan Asif
Dec 6, 2024
Read the article




Optimizing Performance and Cost by Caching LLM Queries

Rehan Asif
Dec 3, 2024
Read the article




LoRA vs RAG: Full Model Fine-Tuning in Large Language Models
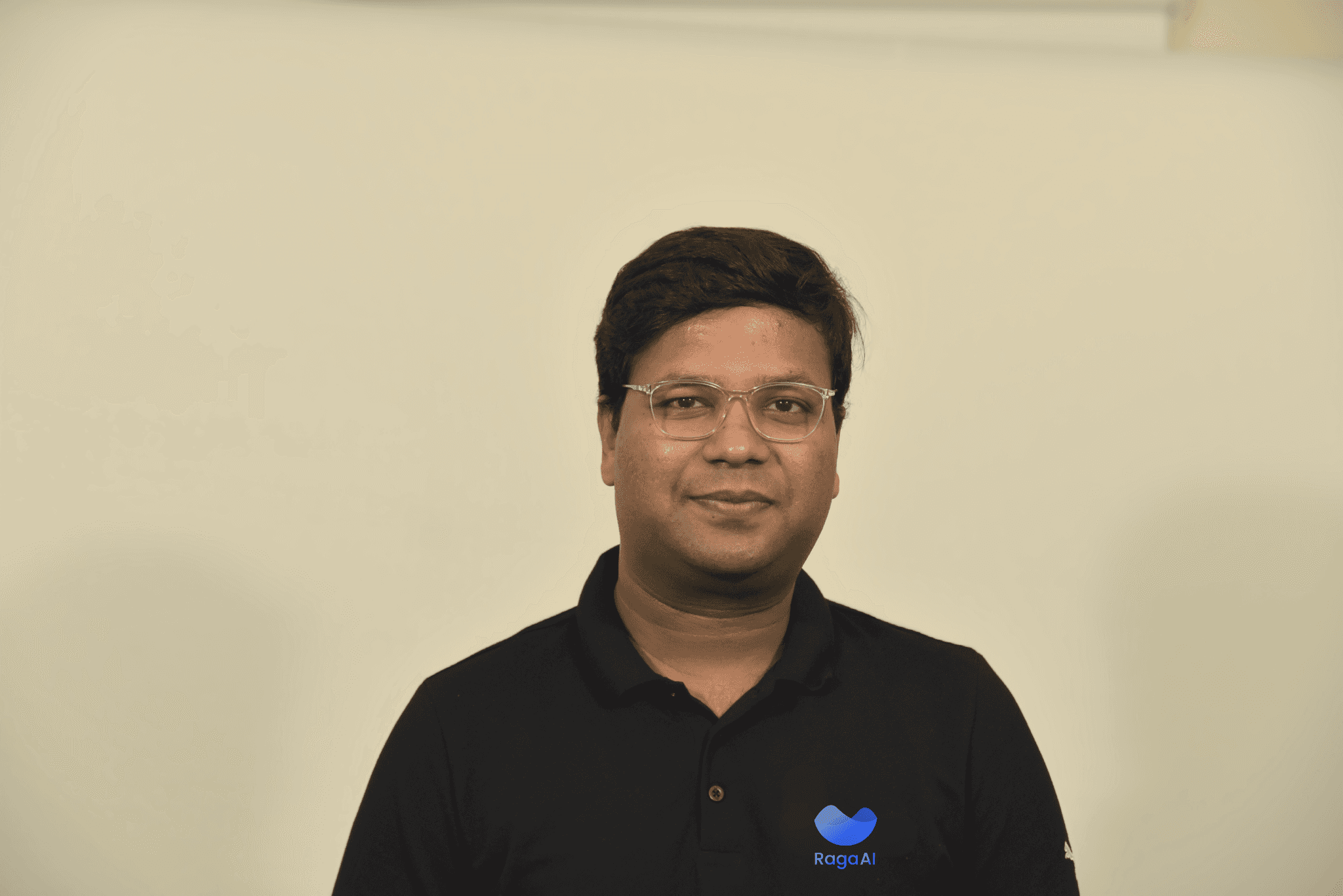
Jigar Gupta
Nov 30, 2024
Read the article
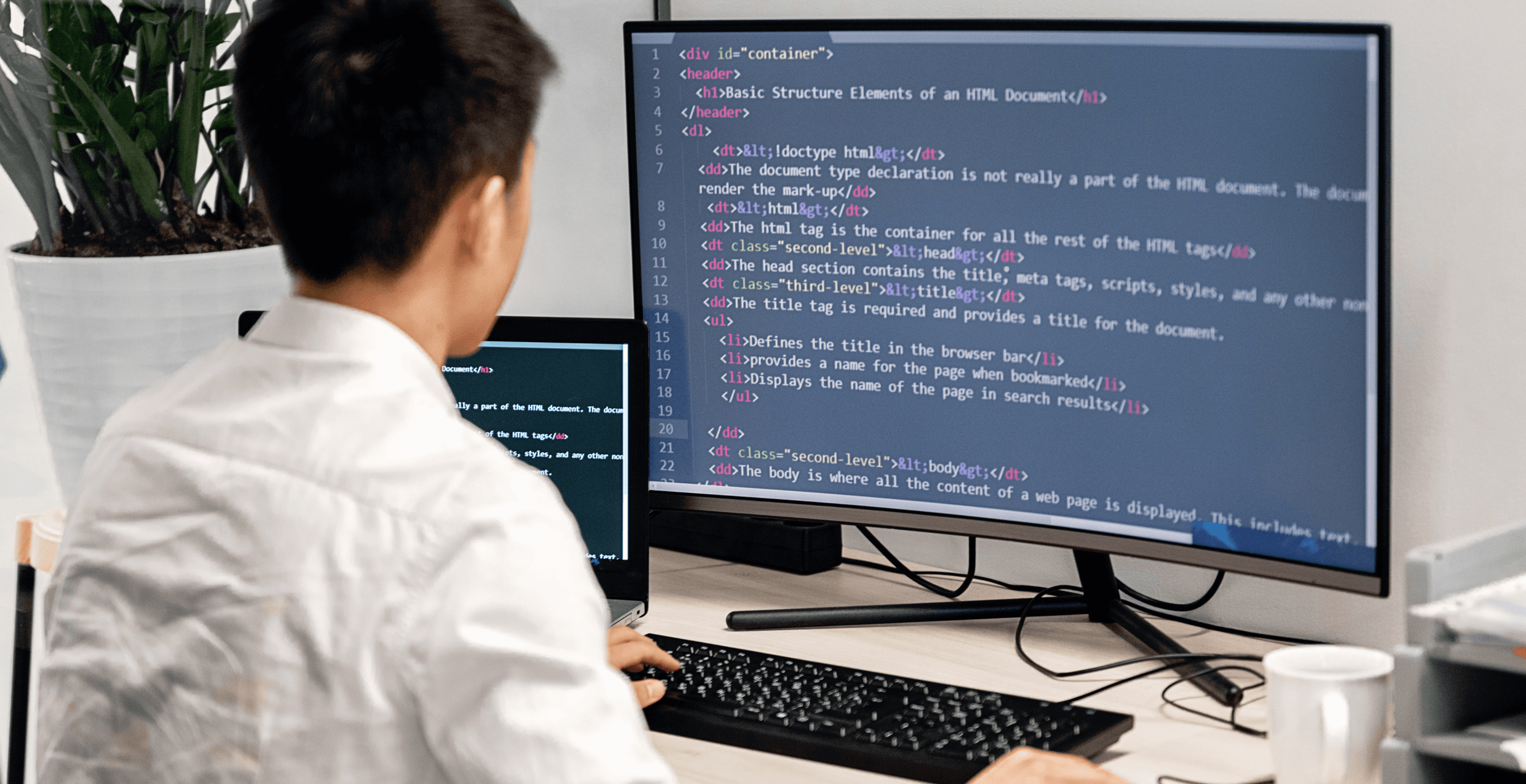
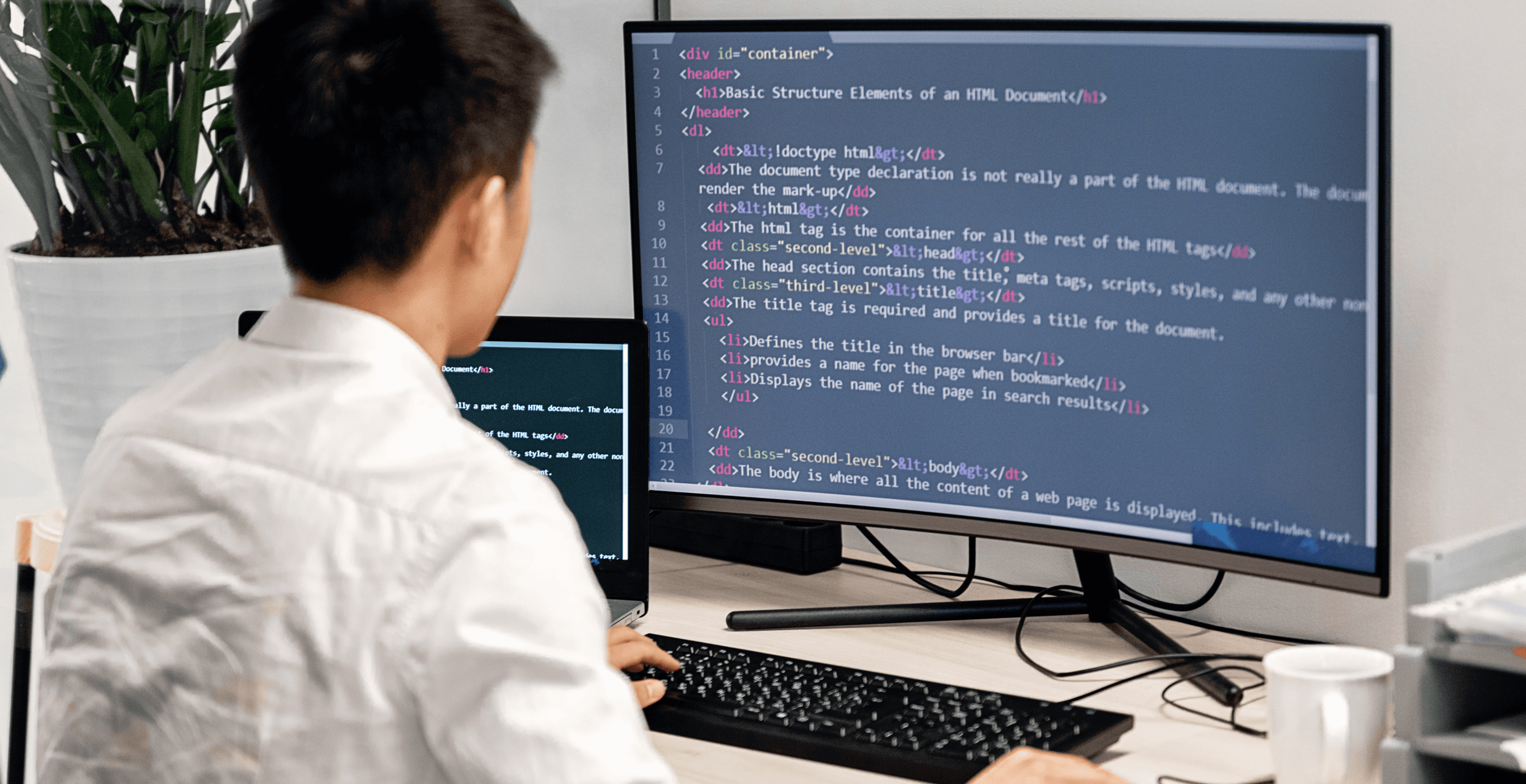
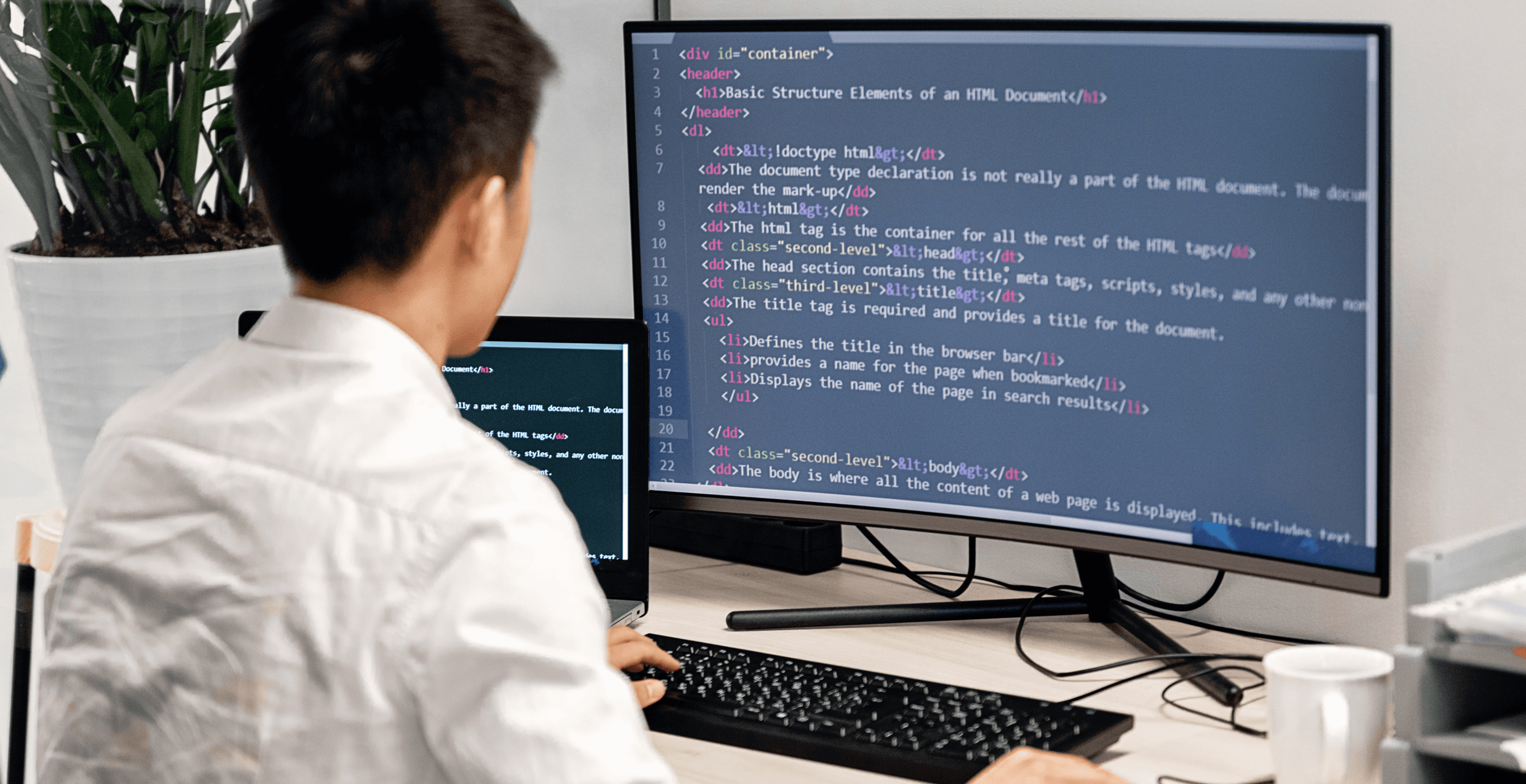
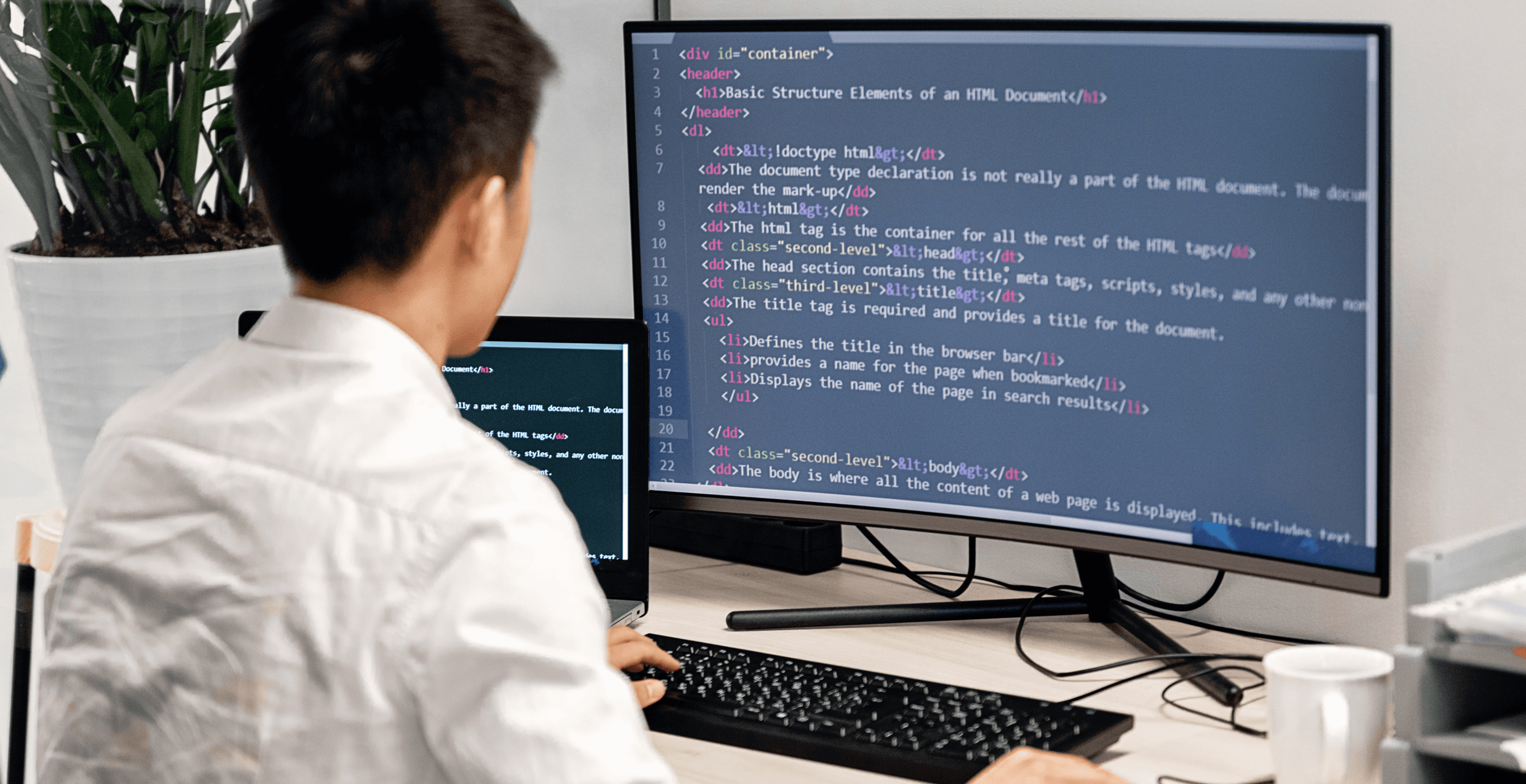
Steps to Train LLM on Personal Data

Rehan Asif
Nov 28, 2024
Read the article




Step by Step Guide to Building RAG-based LLM Applications with Examples

Rehan Asif
Nov 27, 2024
Read the article
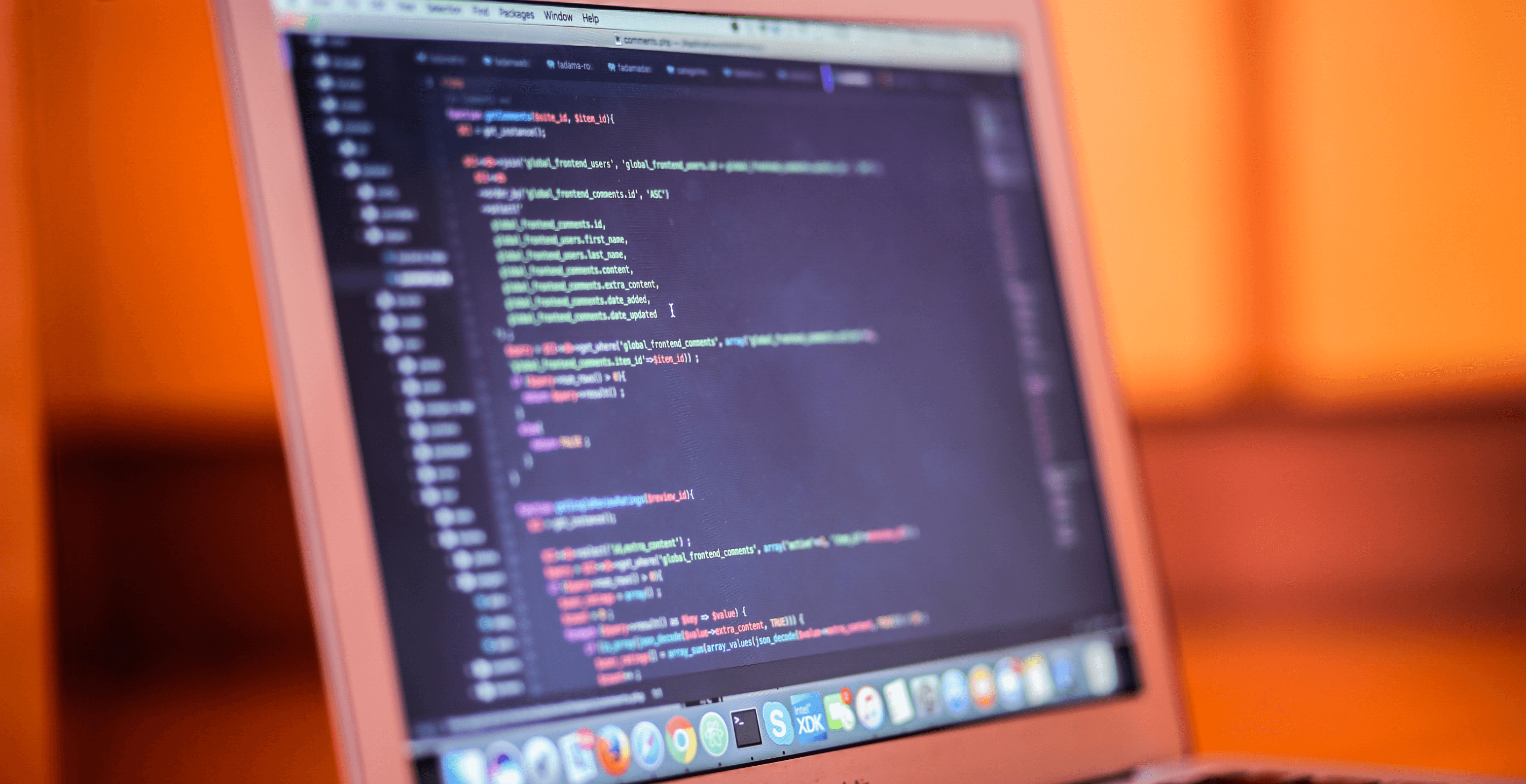
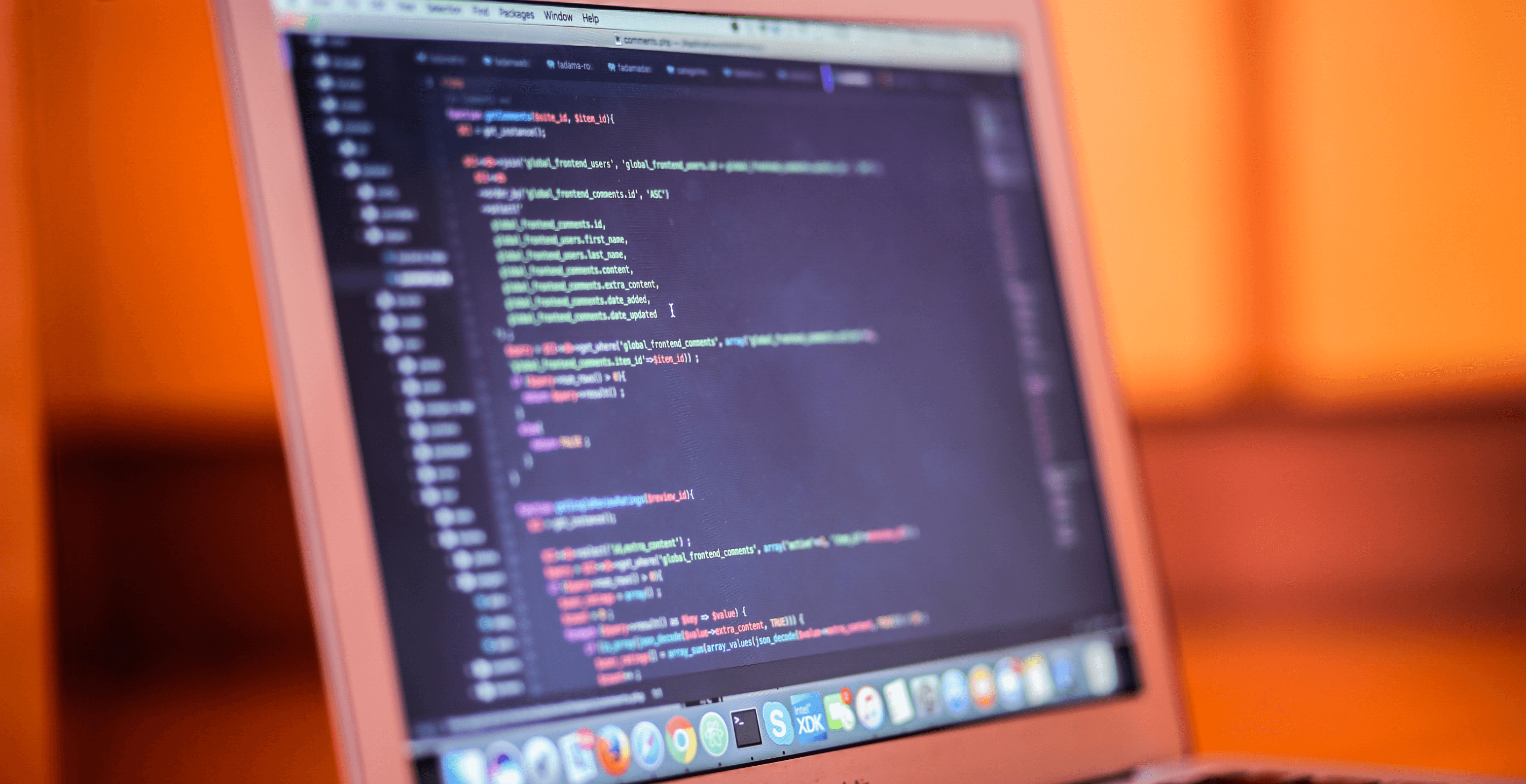
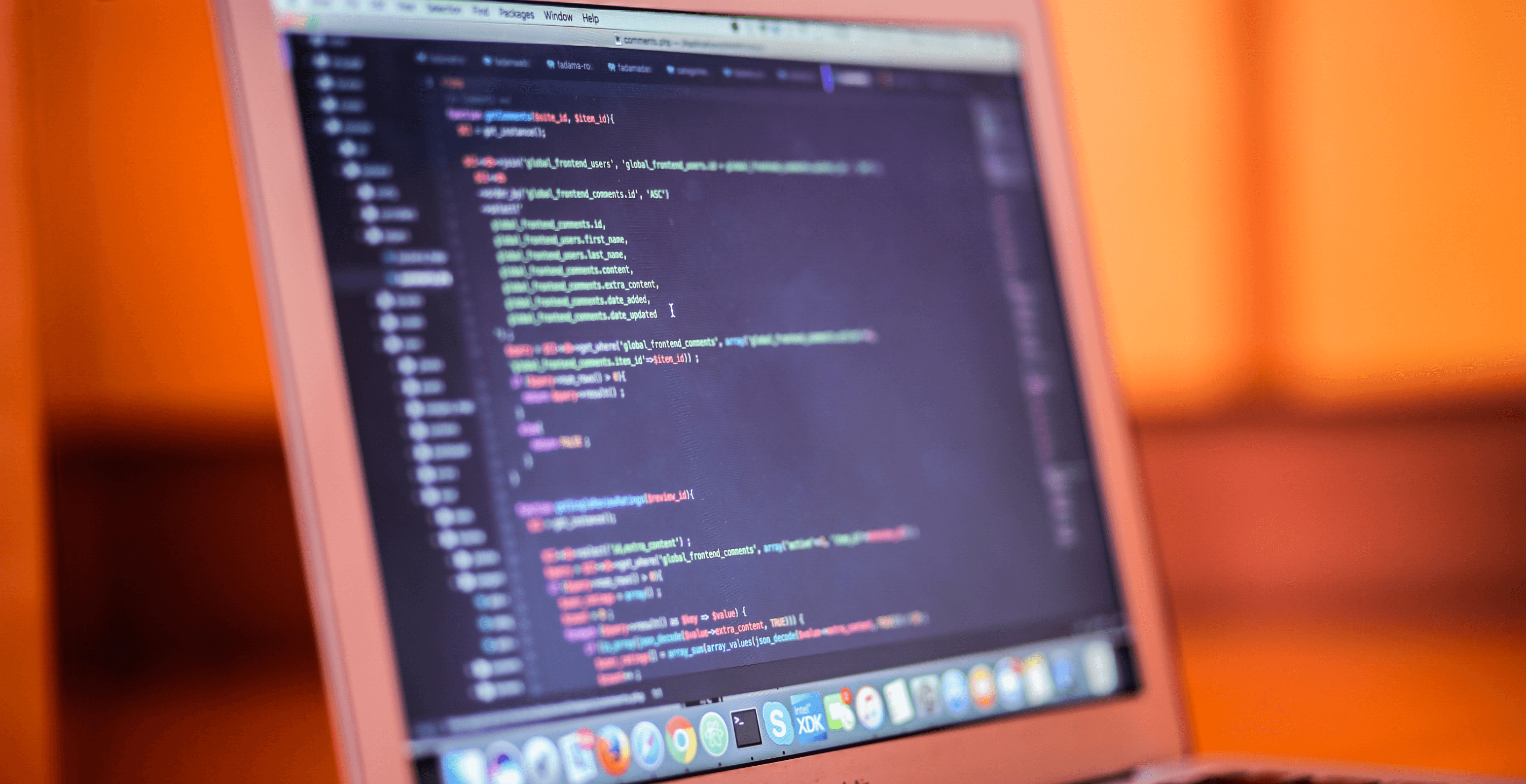
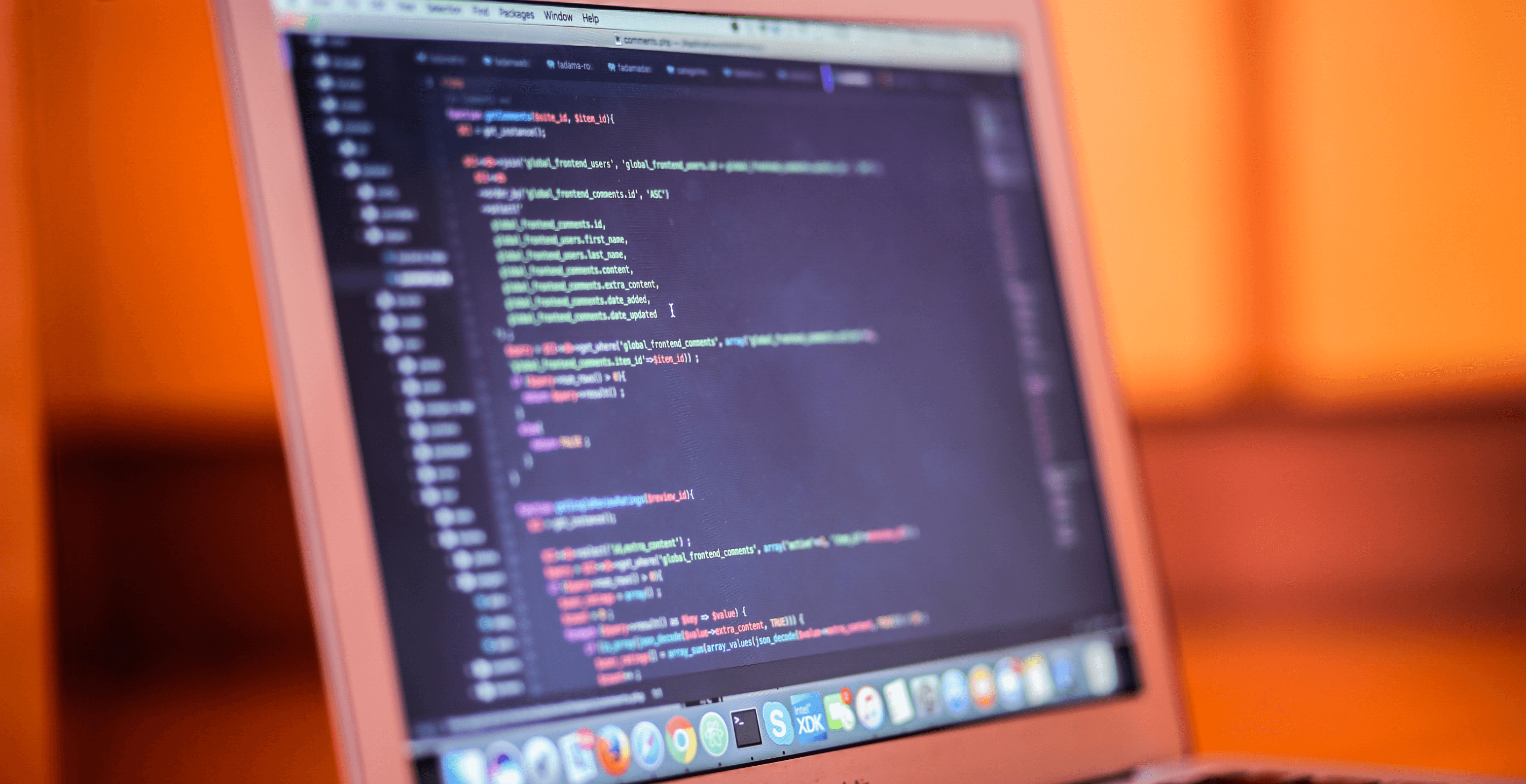
Building AI Agentic Workflows with Multi-Agent Collaboration
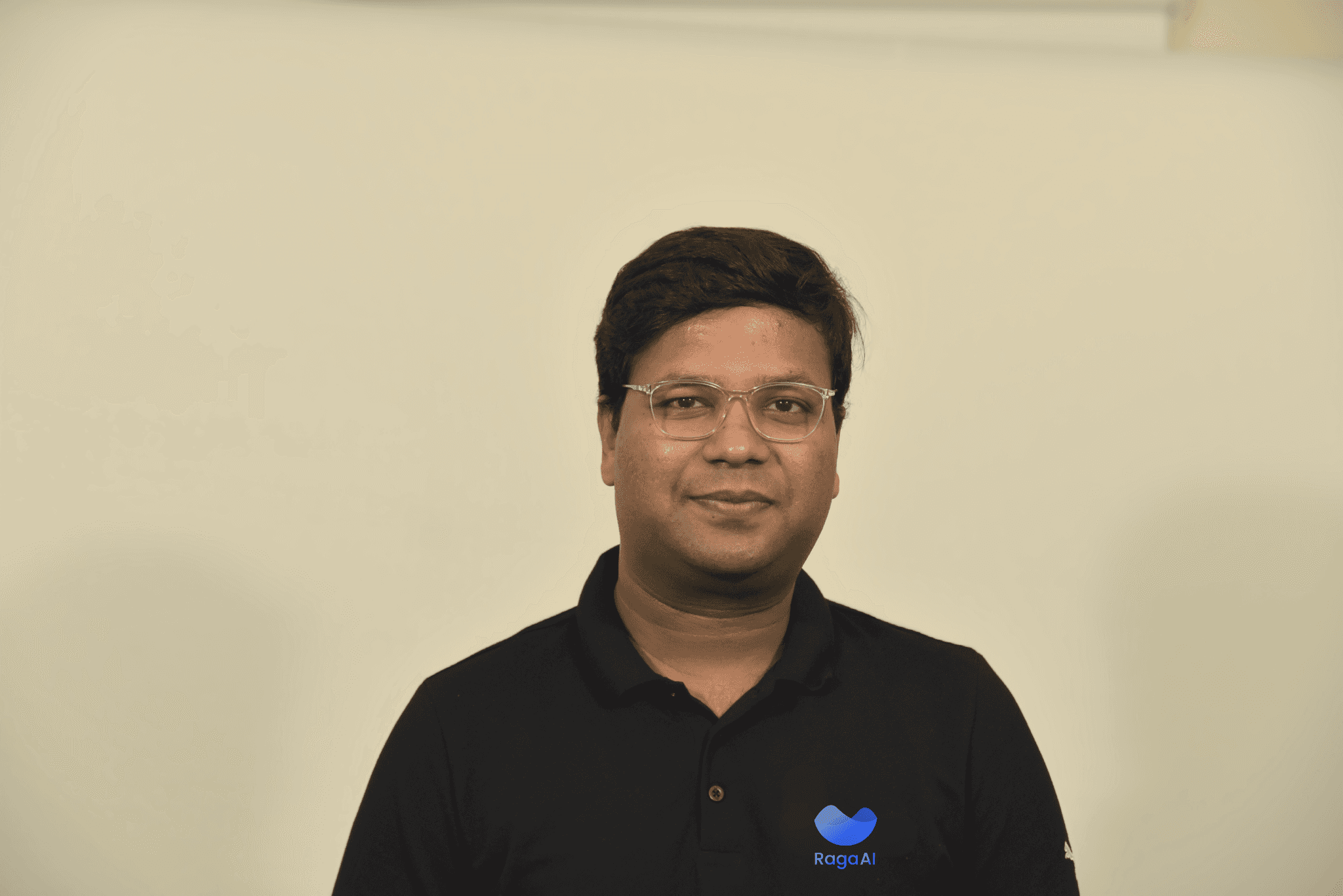
Jigar Gupta
Nov 25, 2024
Read the article




Top Large Language Models (LLMs) in 2024

Rehan Asif
Nov 22, 2024
Read the article
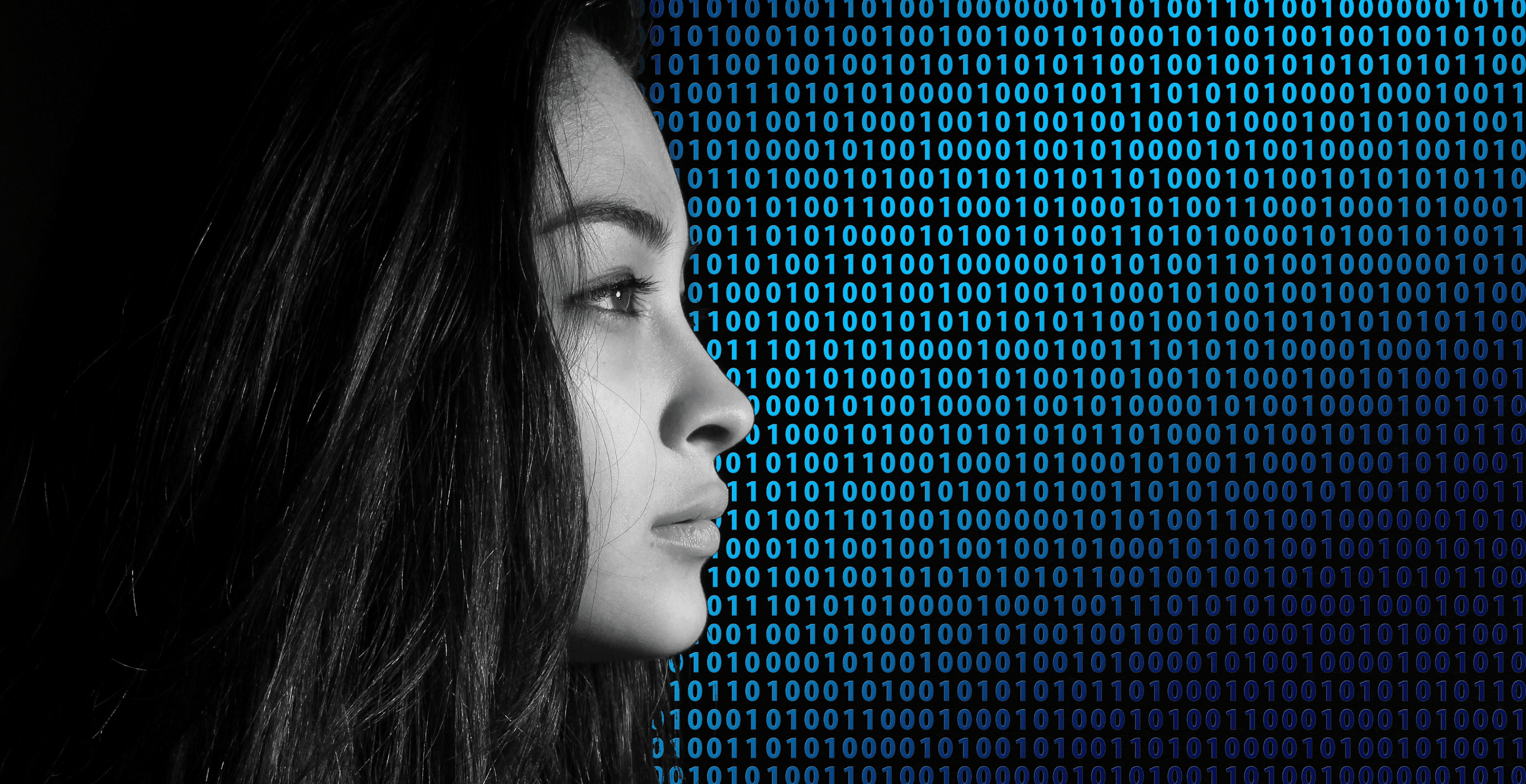
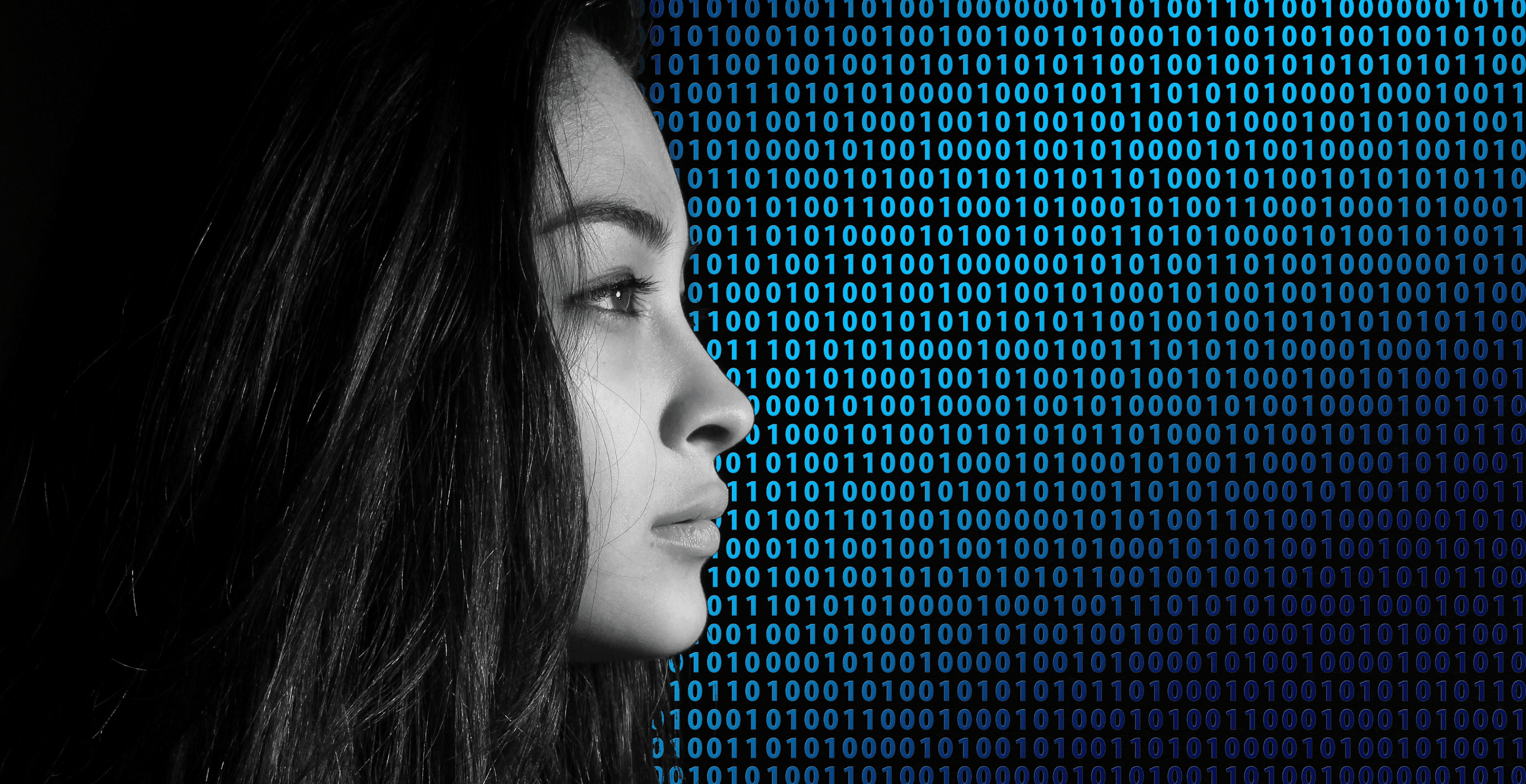
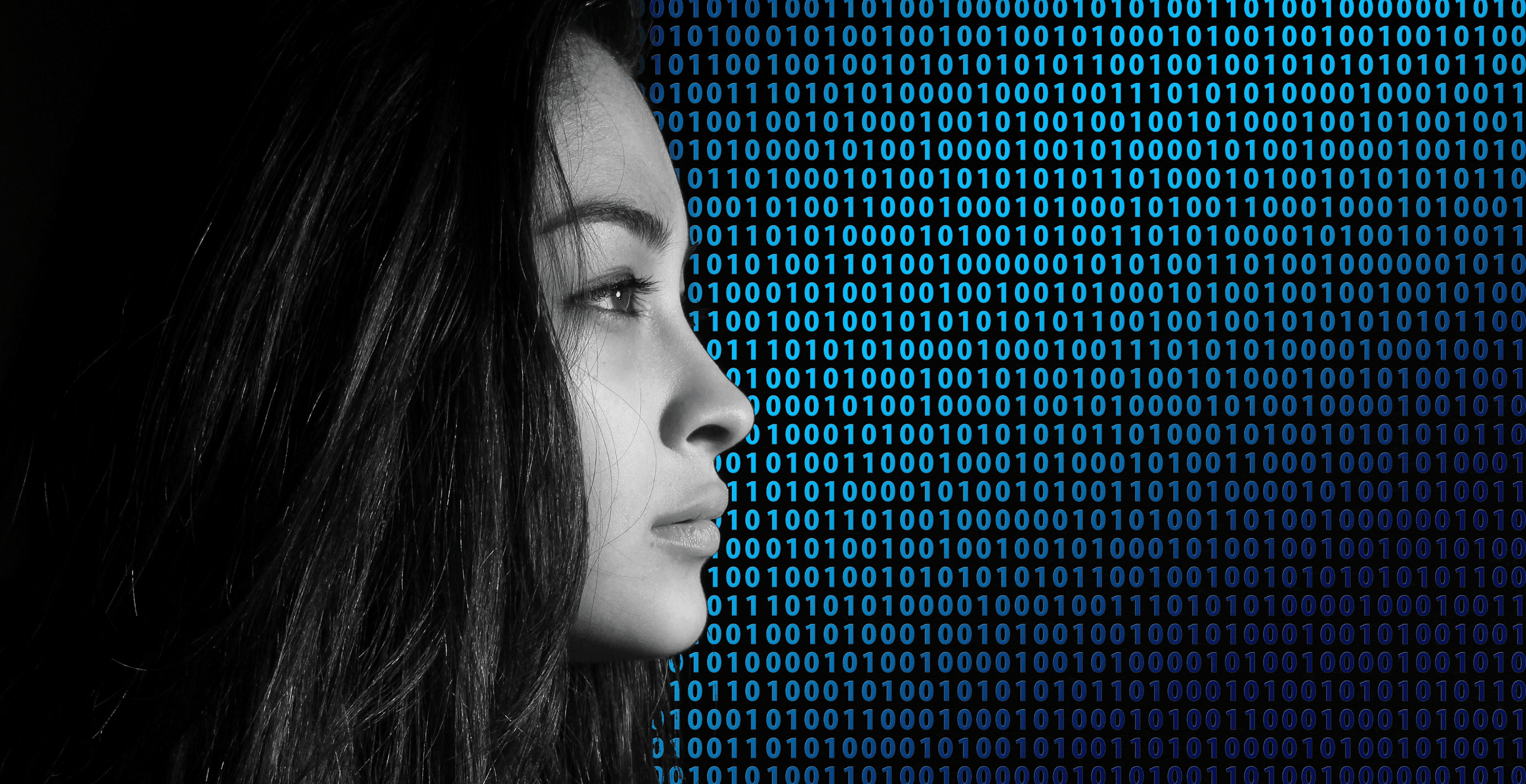
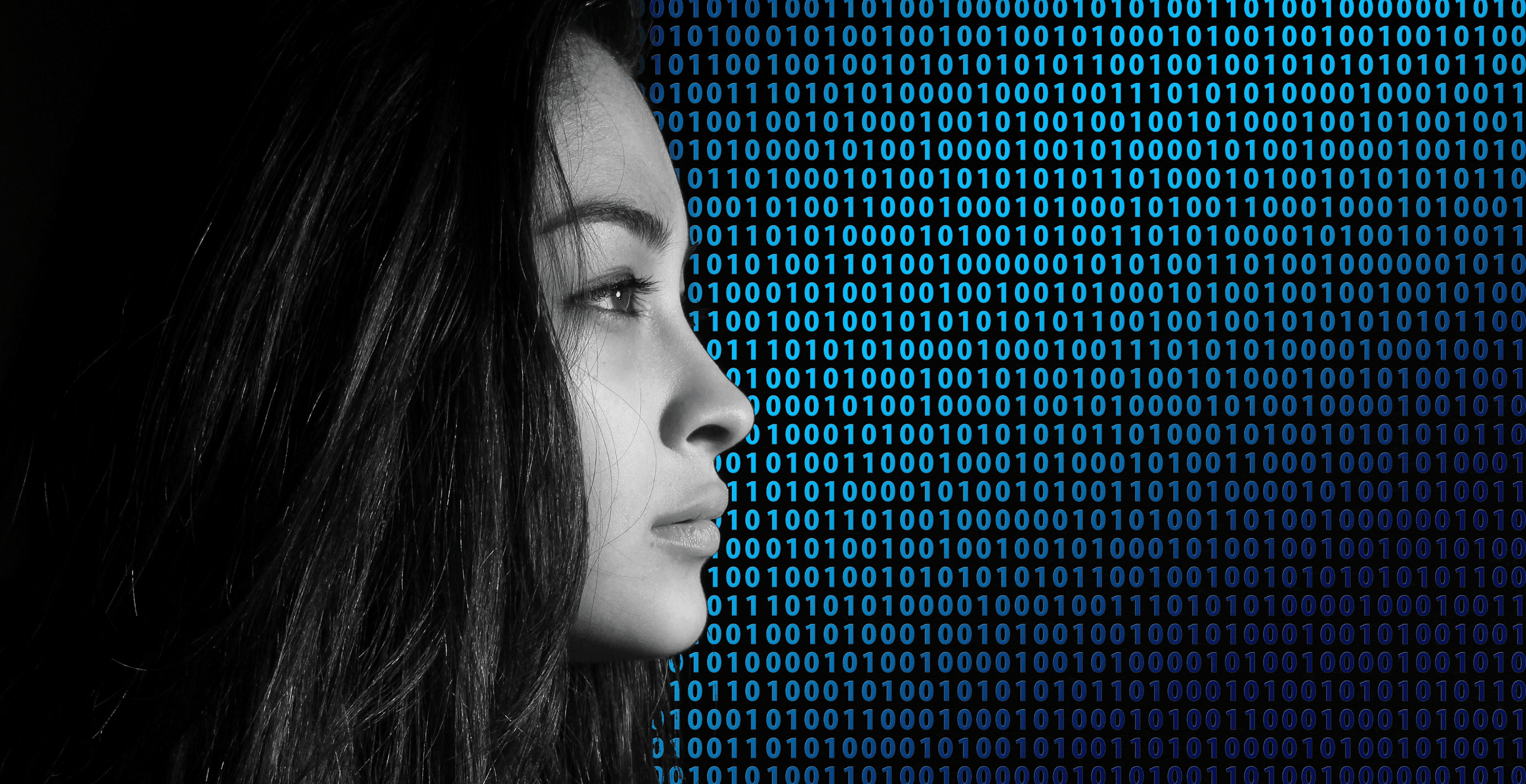
Creating Apps with Large Language Models

Rehan Asif
Nov 21, 2024
Read the article
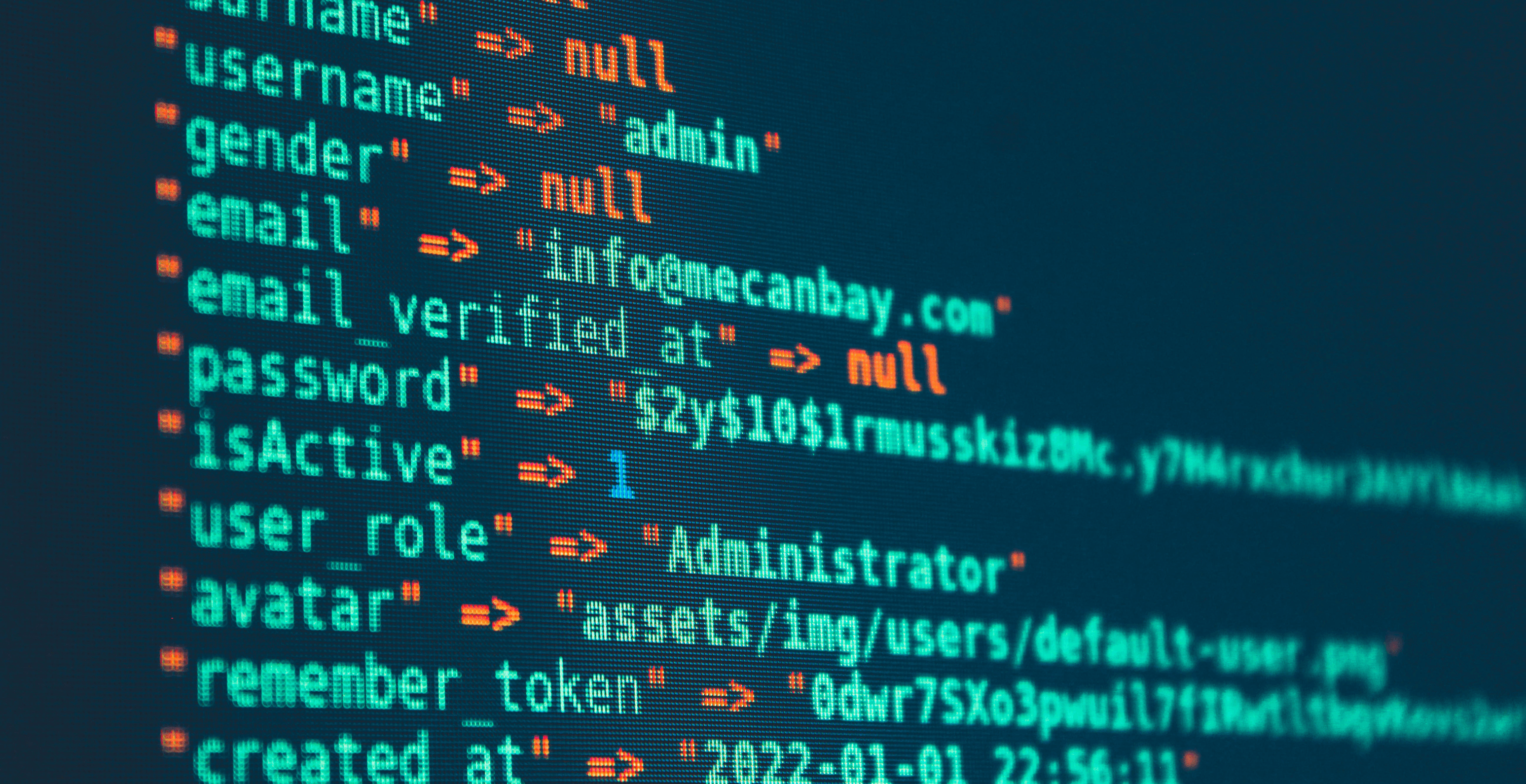
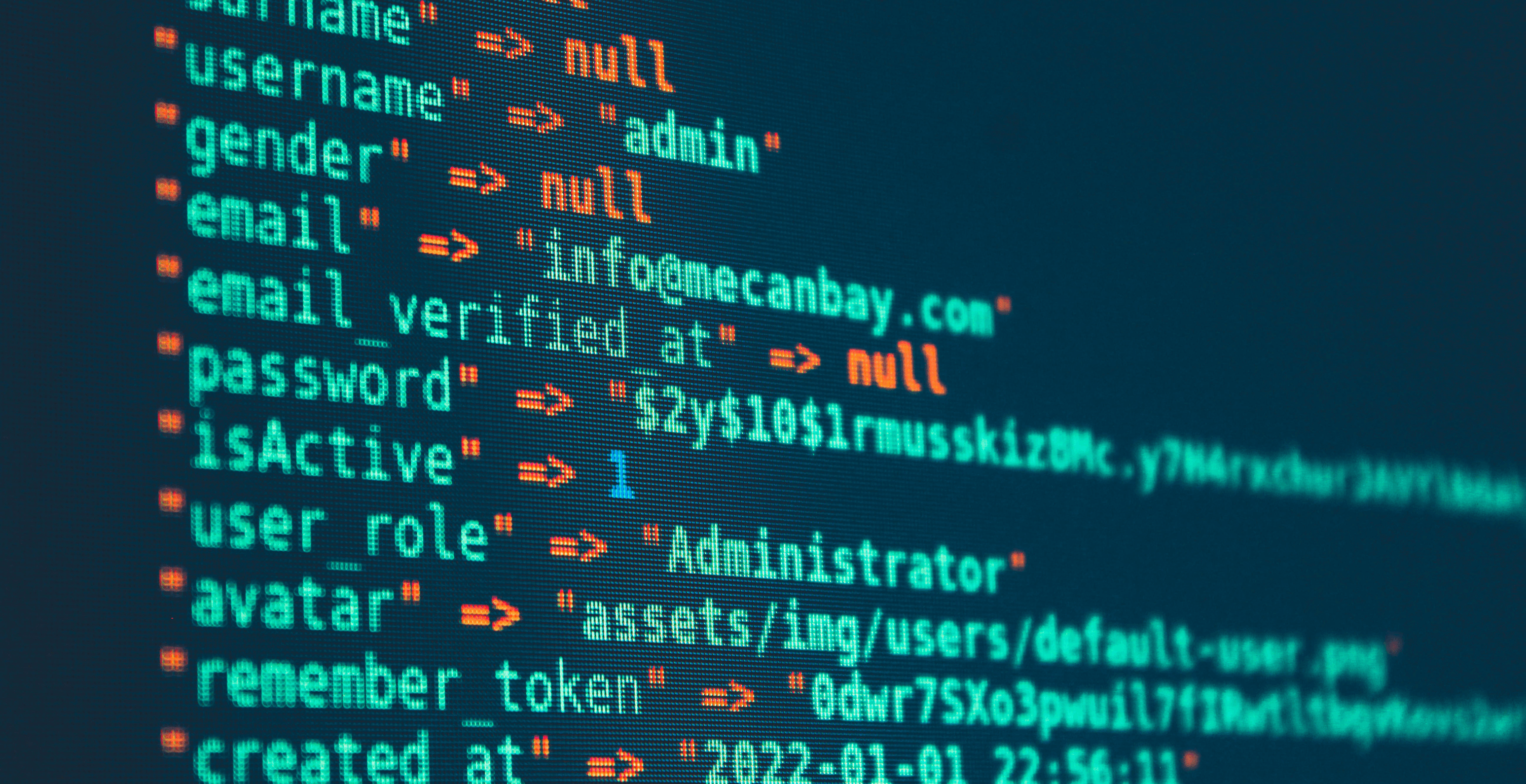
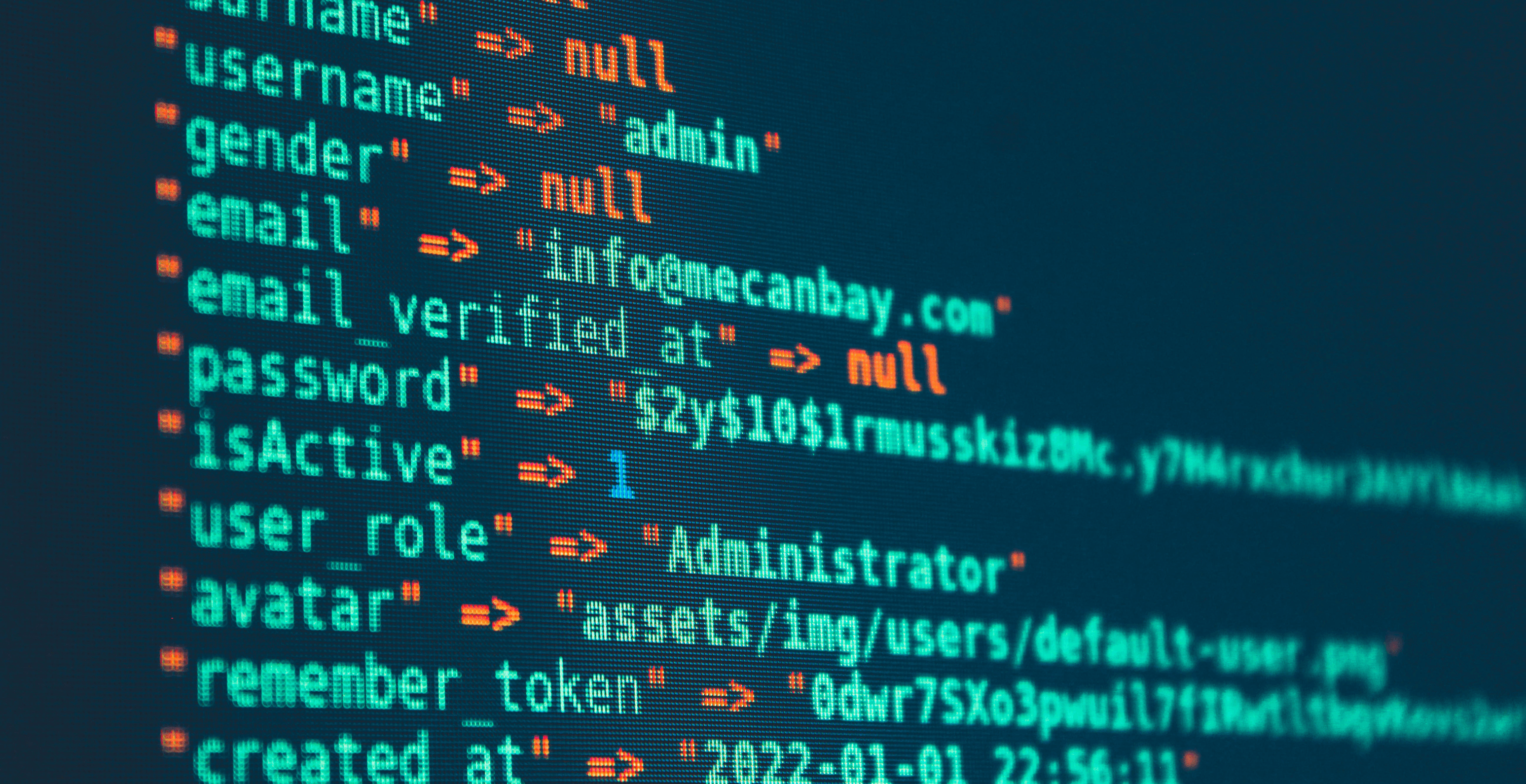
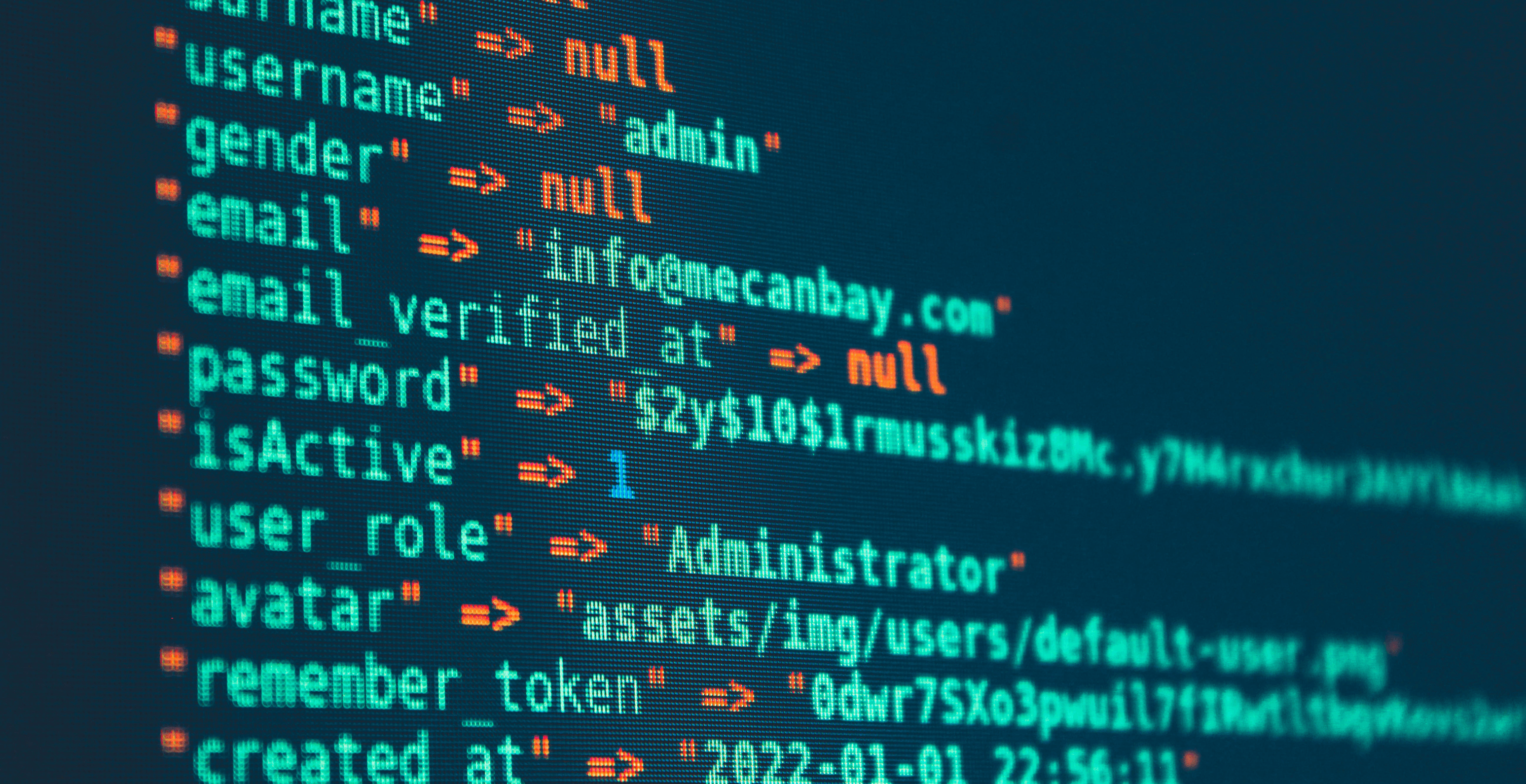
Best Practices In Data Governance For AI
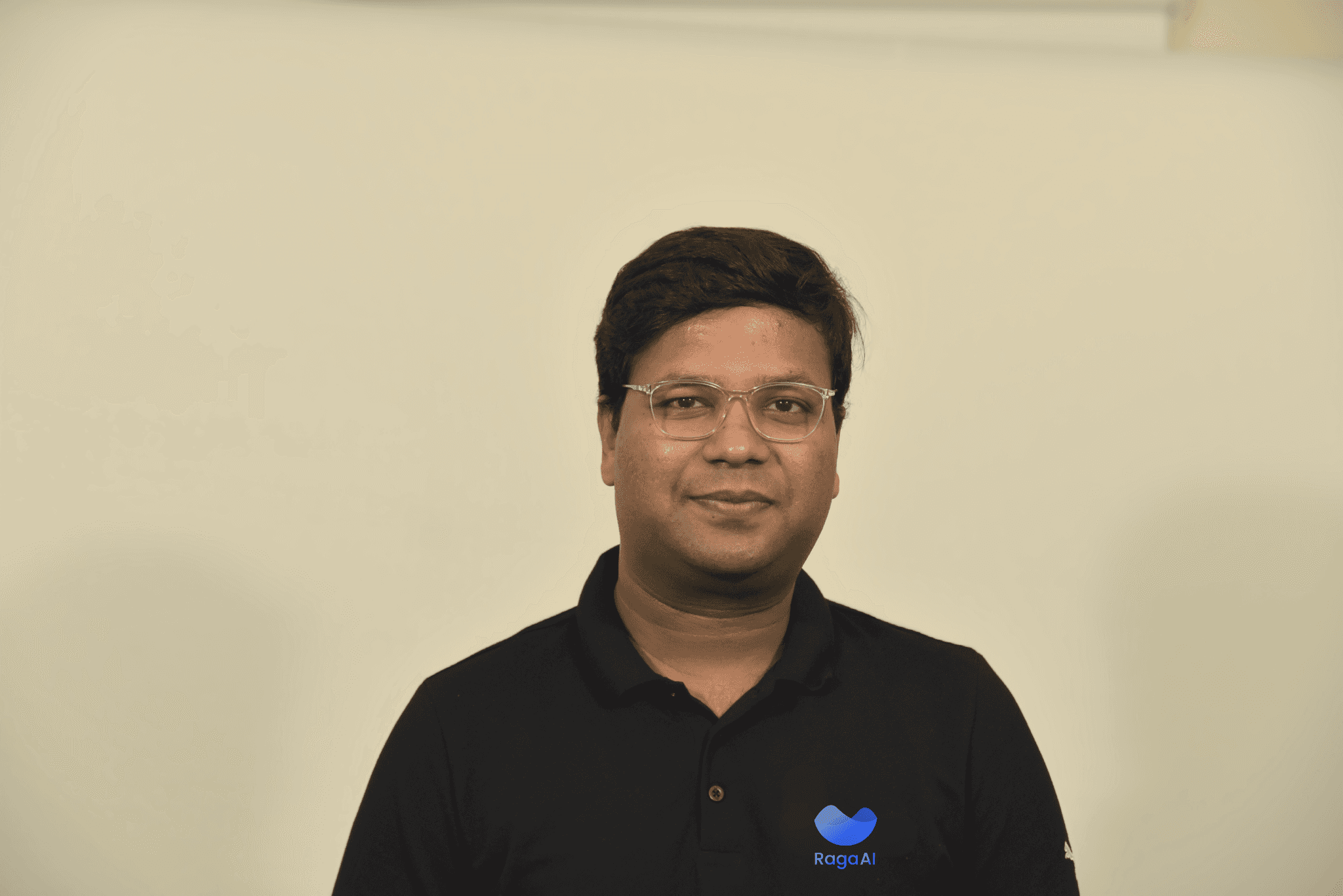
Jigar Gupta
Nov 17, 2024
Read the article
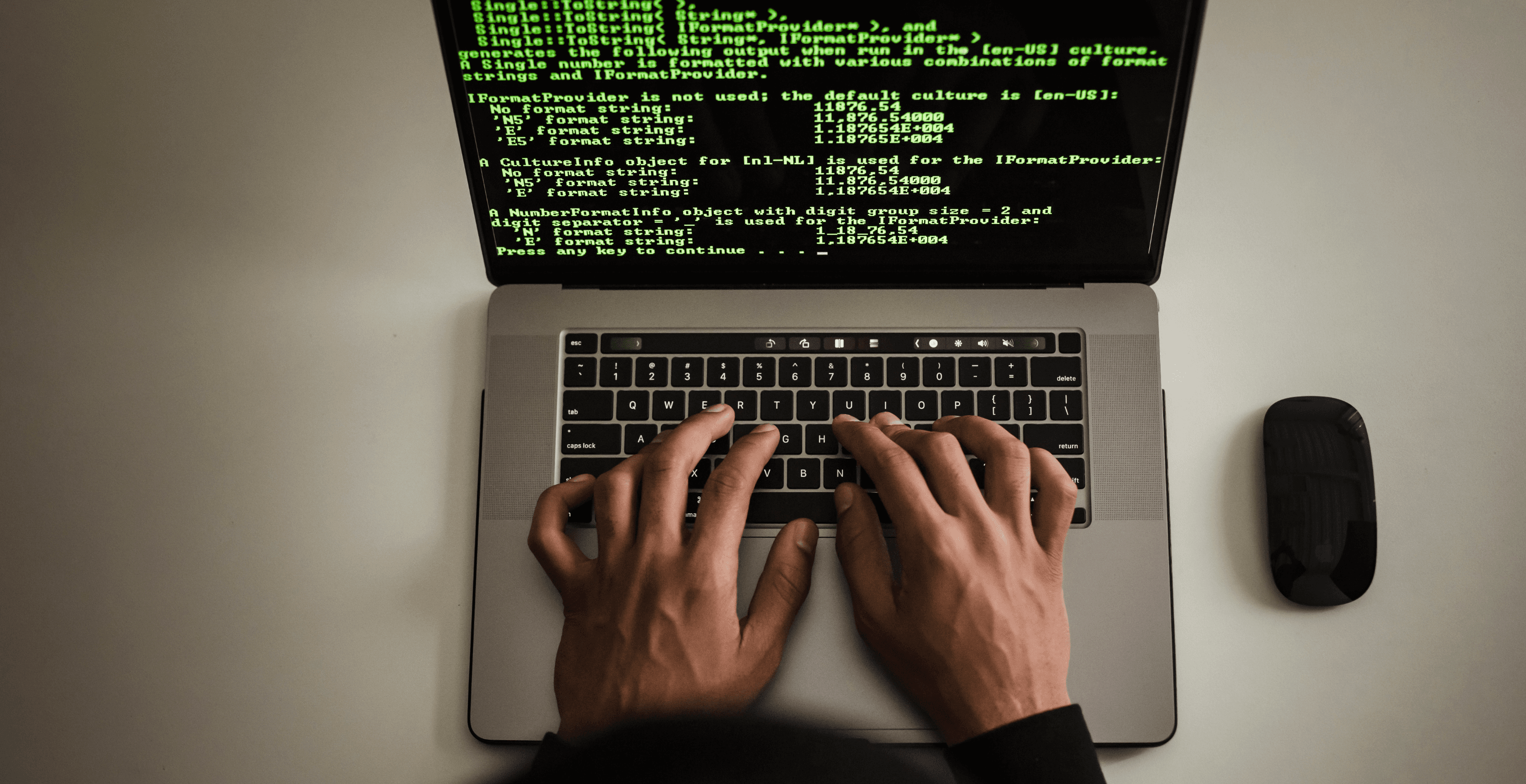
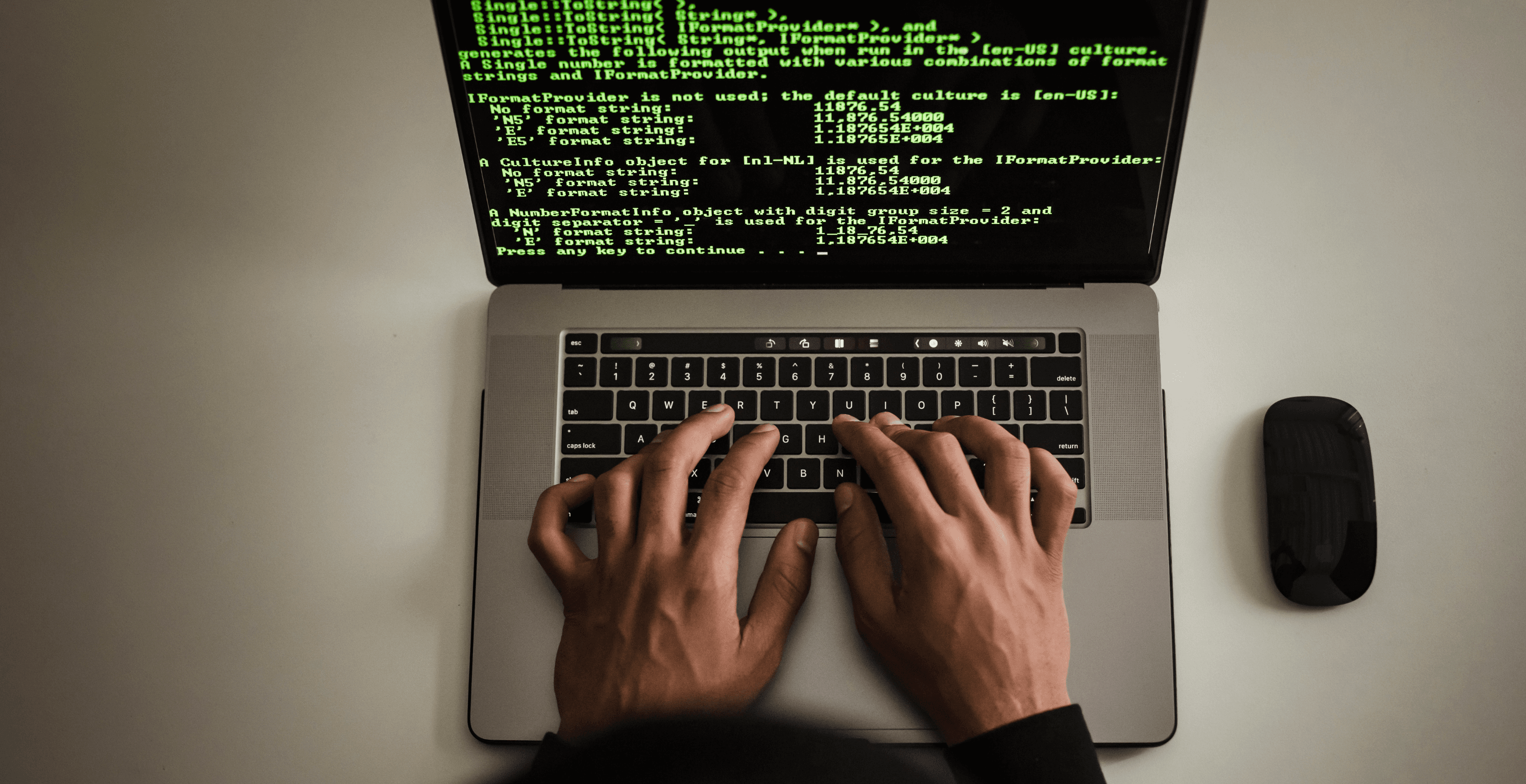
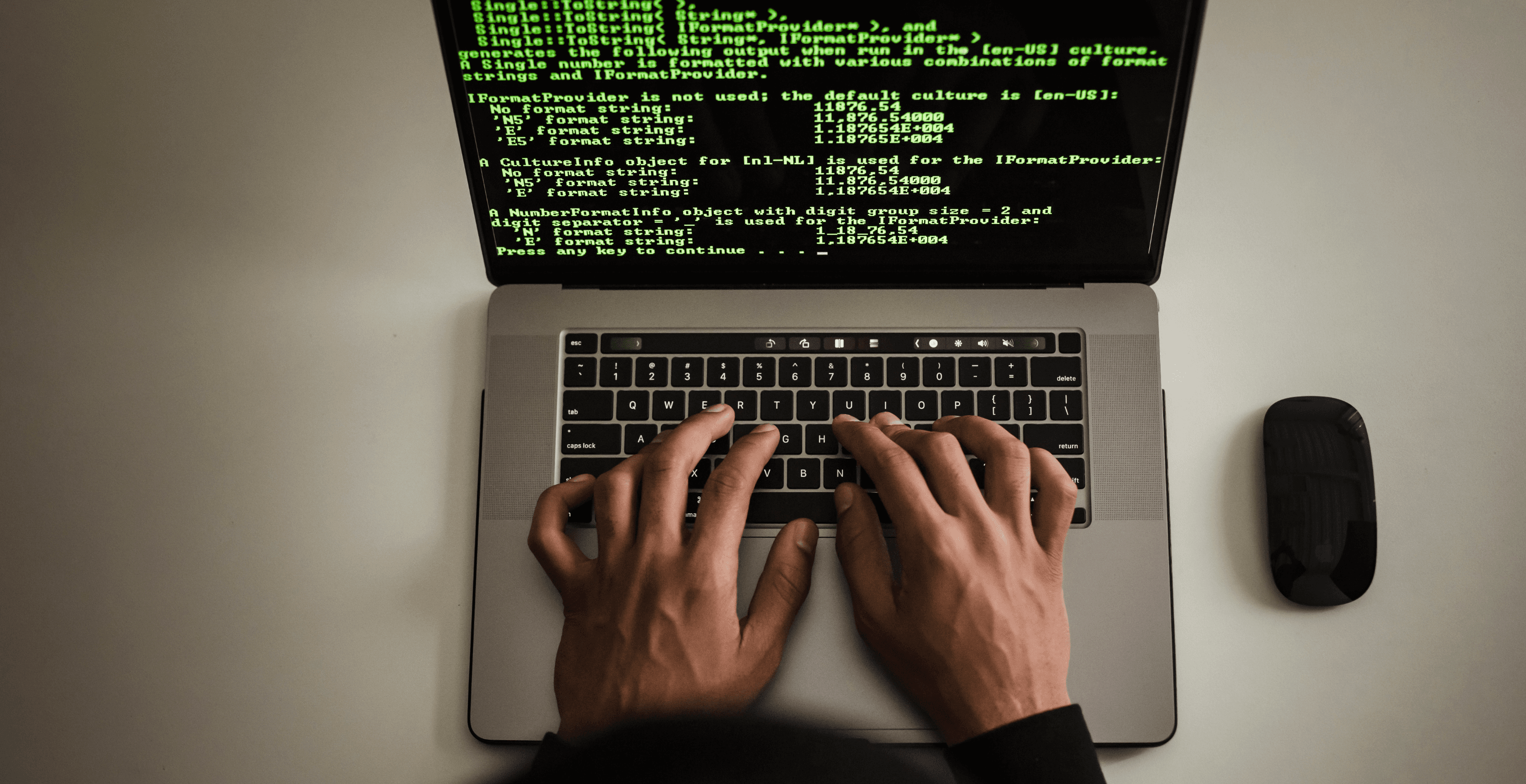
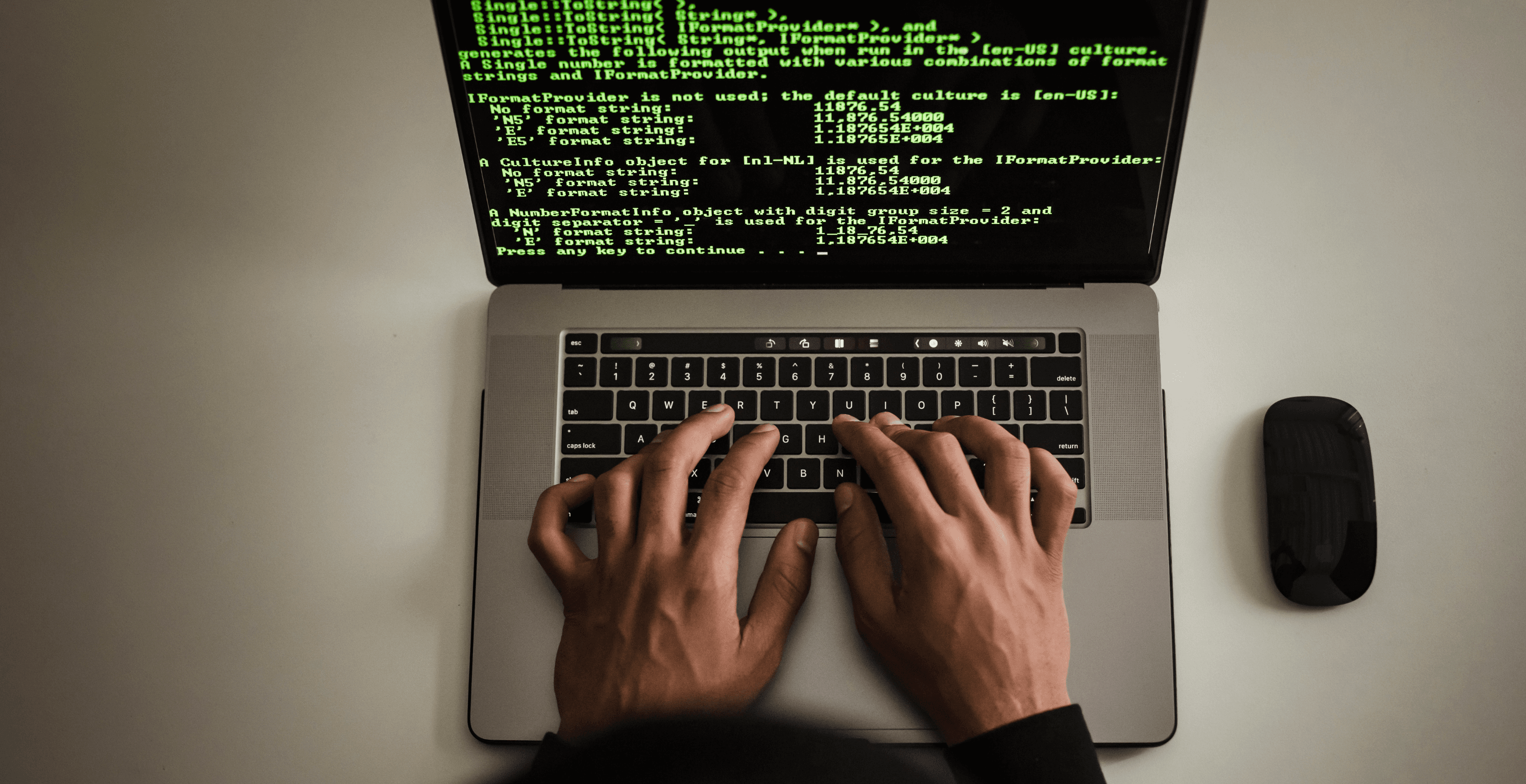
Transforming Conversational AI with Large Language Models

Rehan Asif
Nov 15, 2024
Read the article
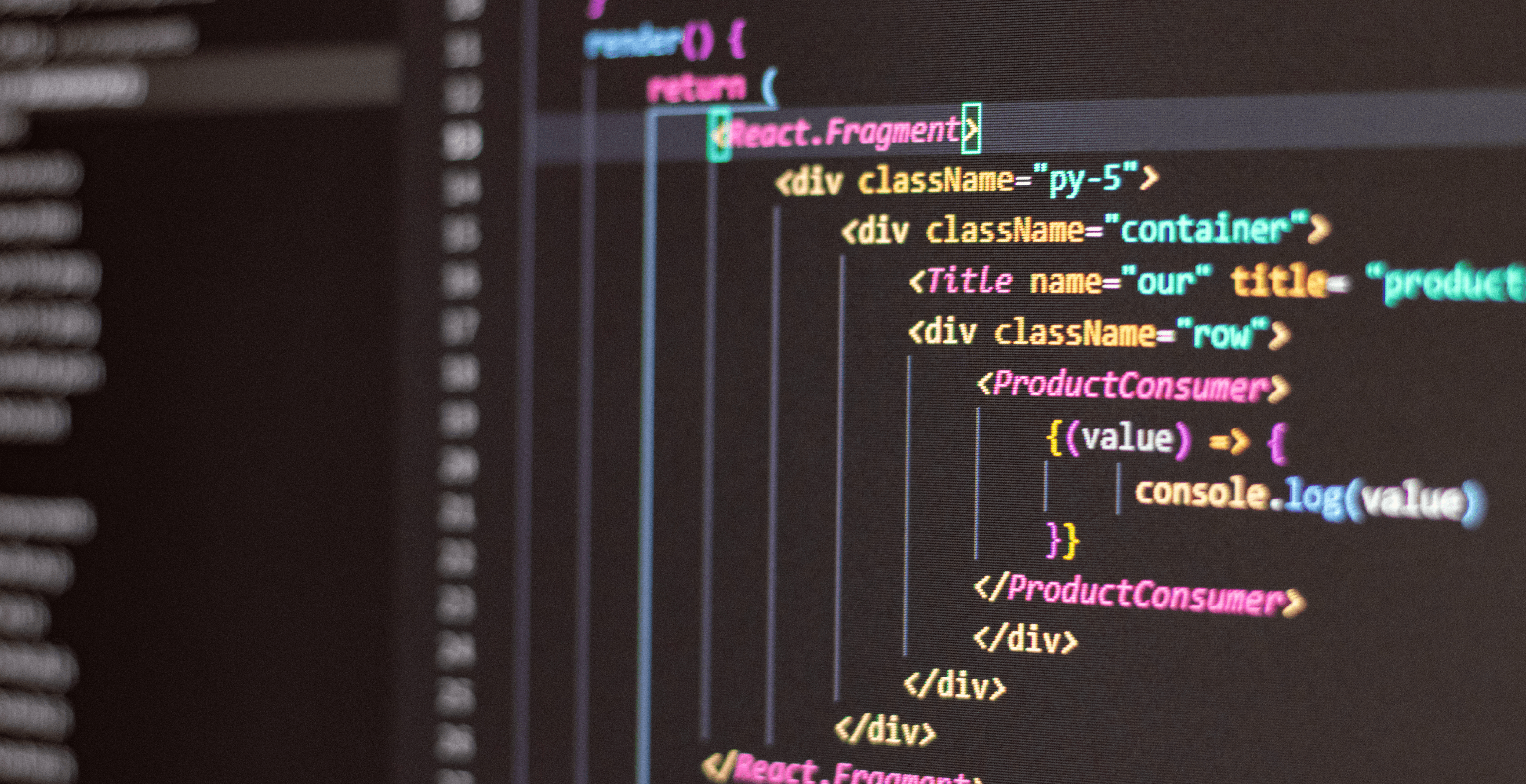
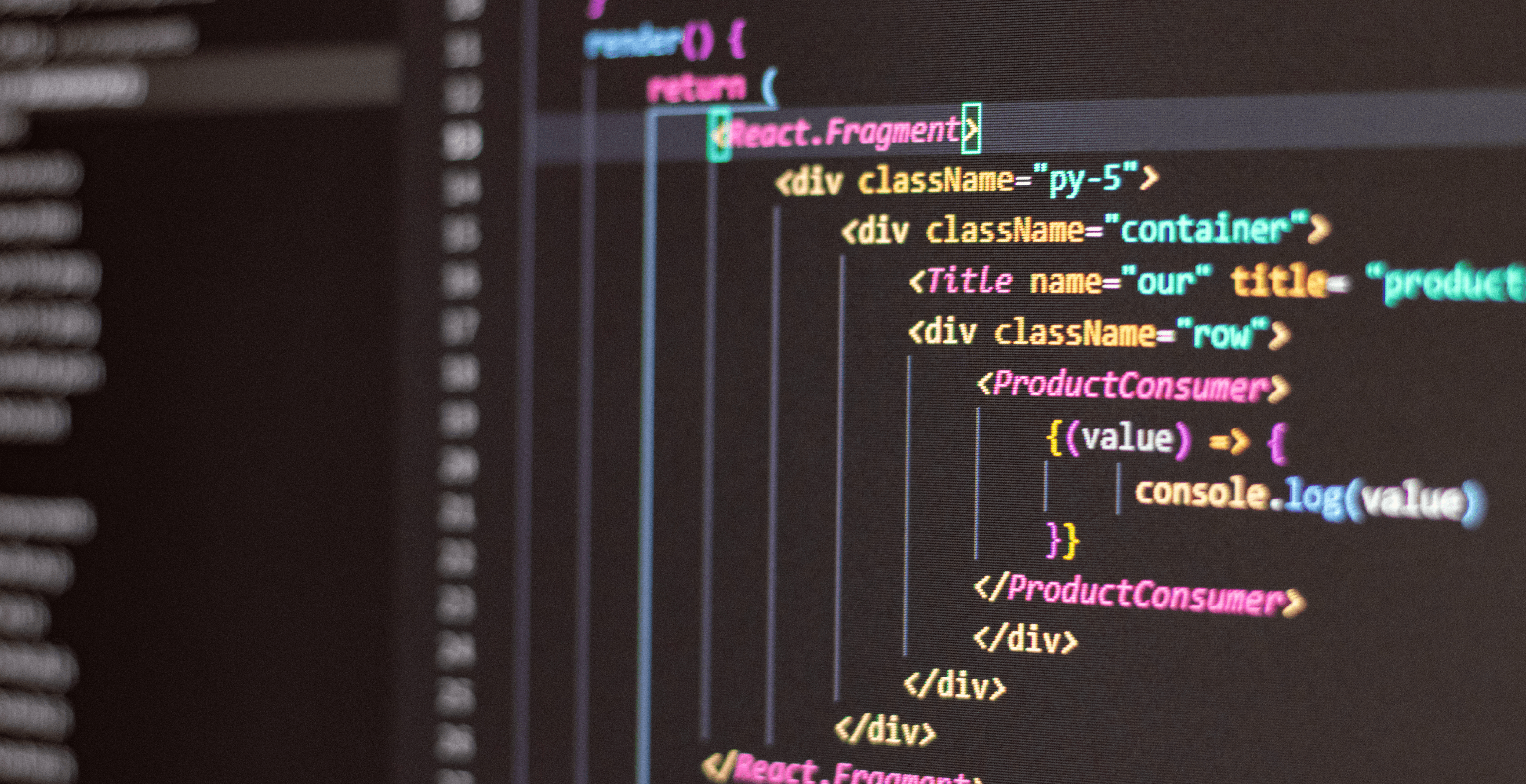
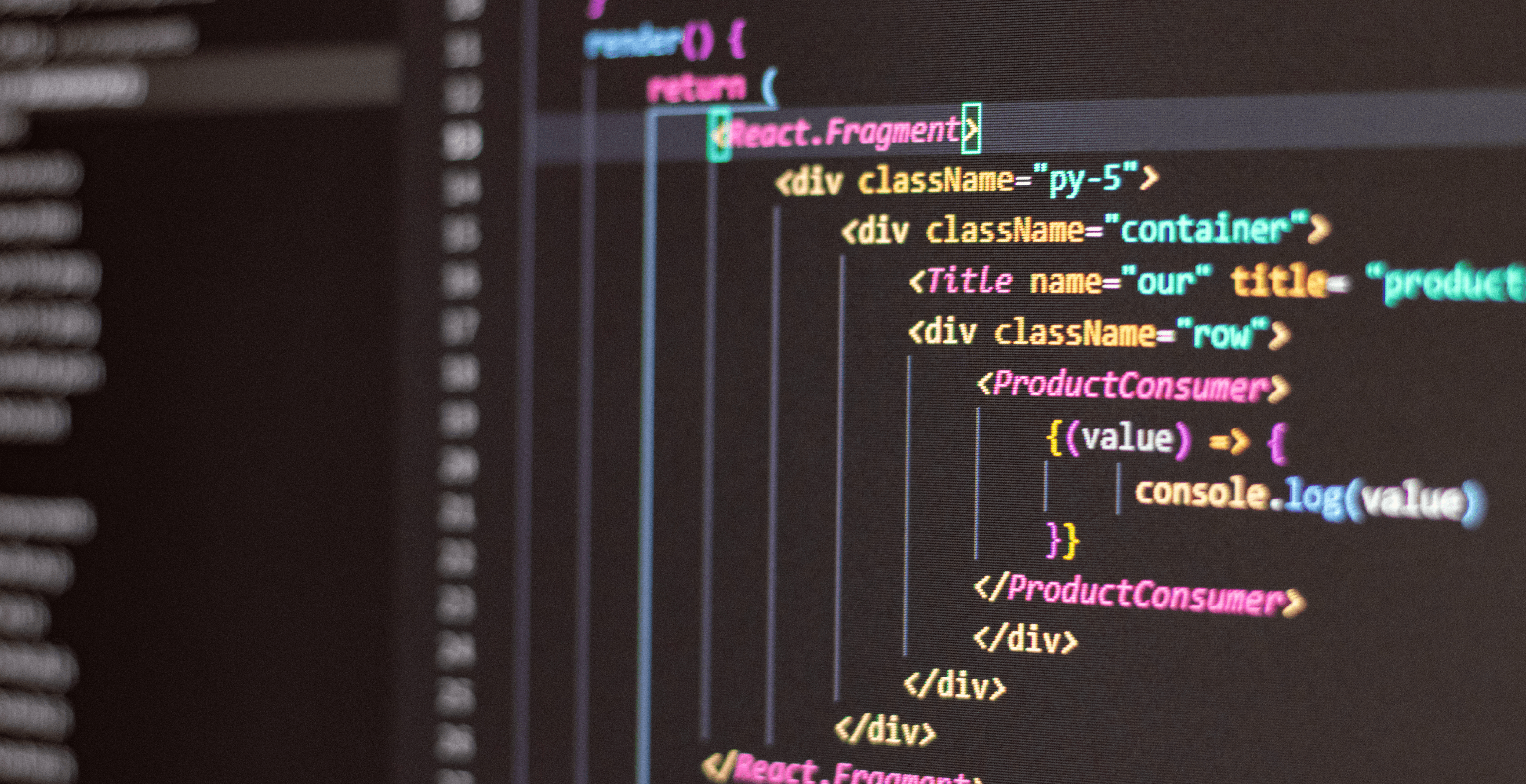
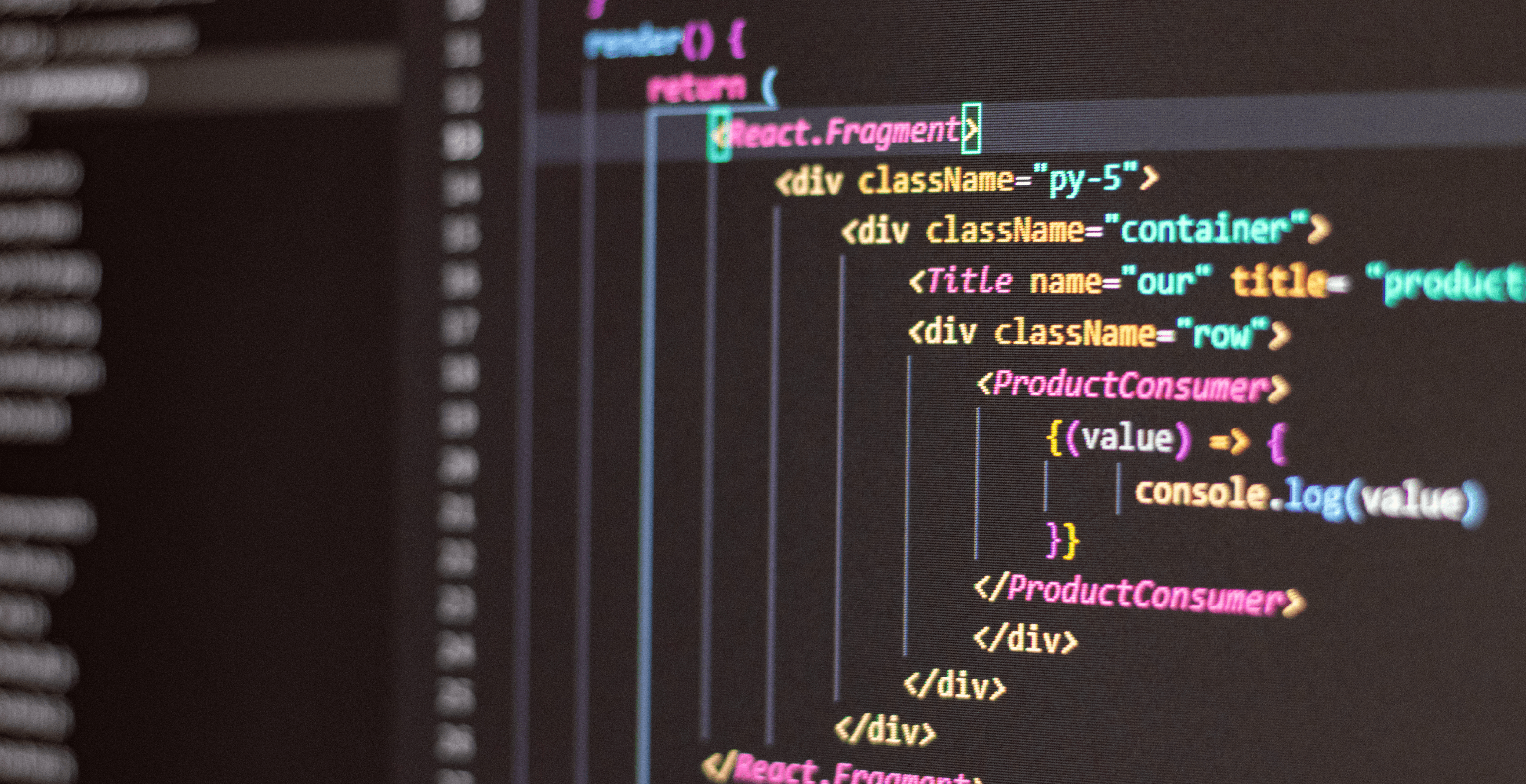
Deploying Generative AI Agents with Local LLMs

Rehan Asif
Nov 13, 2024
Read the article




Exploring Different Types of AI Agents with Key Examples
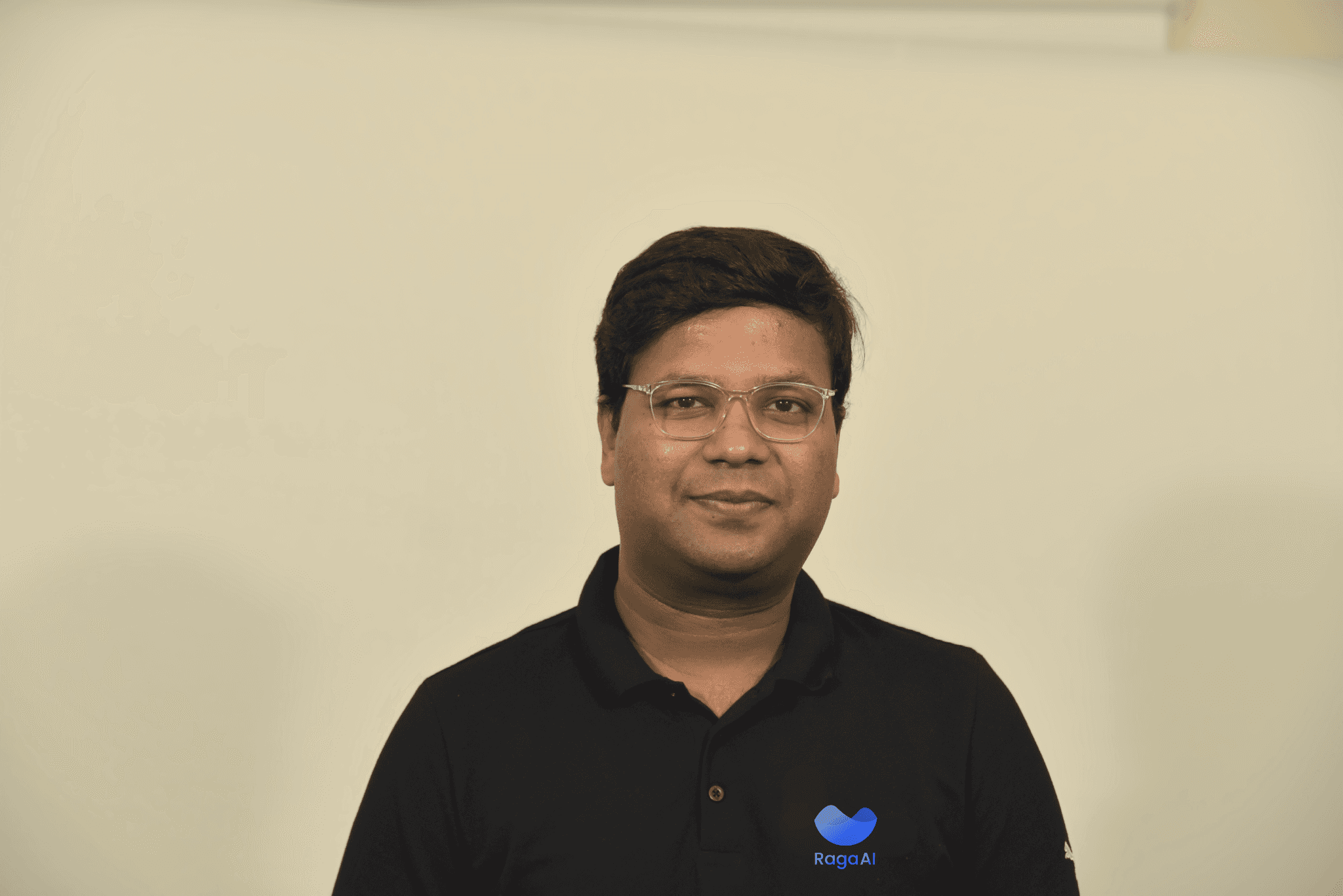
Jigar Gupta
Nov 11, 2024
Read the article




Creating Your Own Personal LLM Agents: Introduction to Implementation

Rehan Asif
Nov 8, 2024
Read the article




Exploring Agentic AI Architecture and Design Patterns
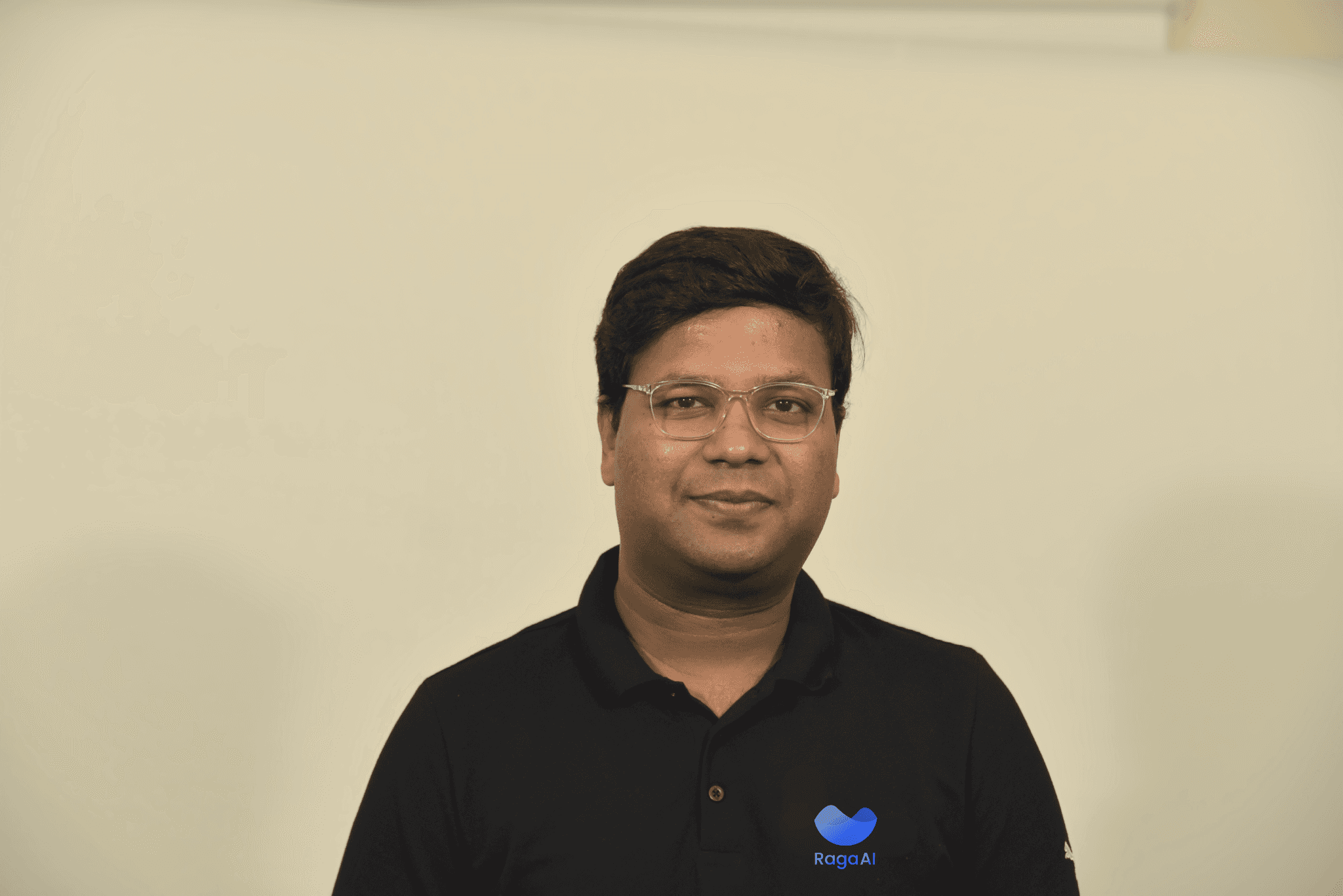
Jigar Gupta
Nov 6, 2024
Read the article
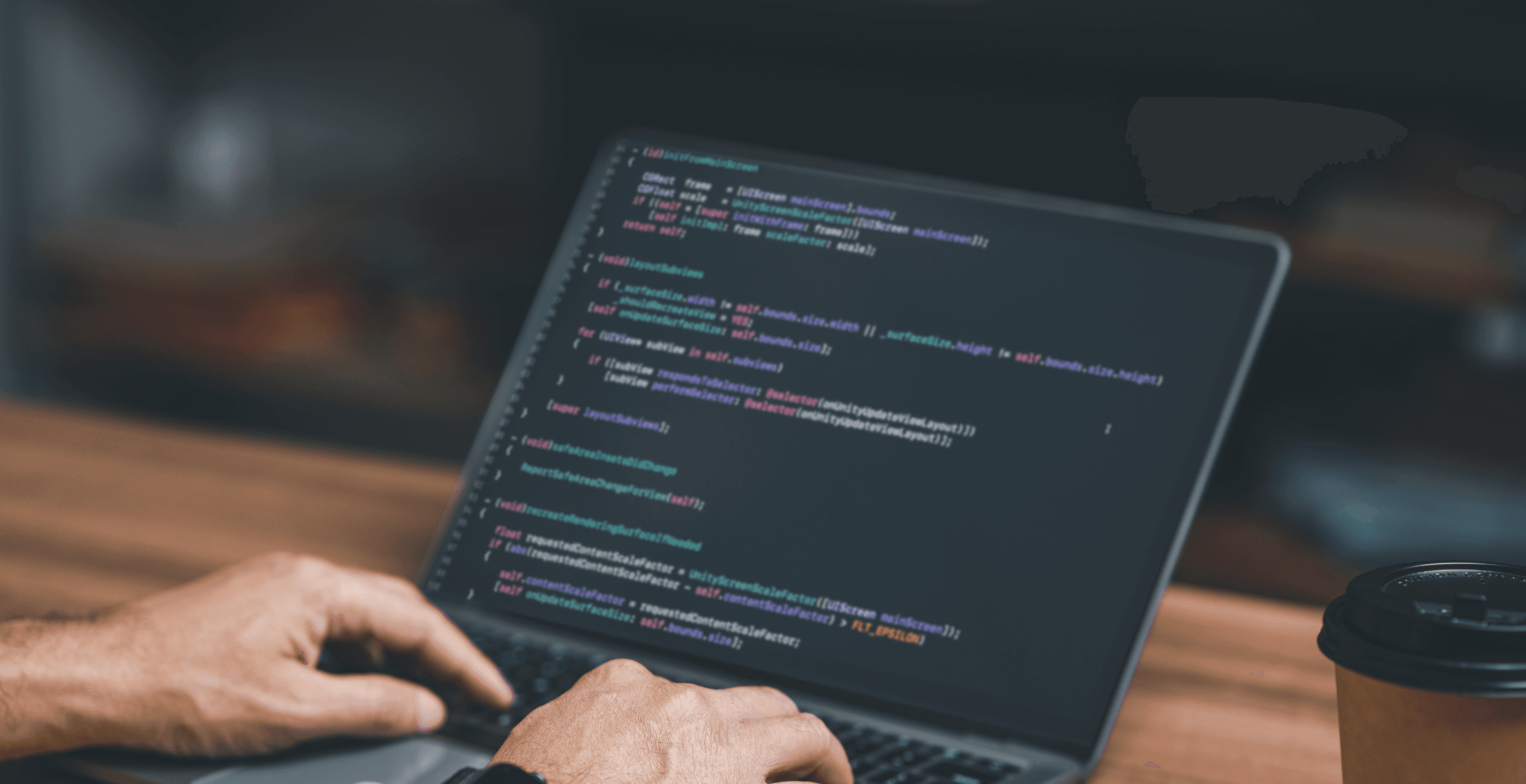
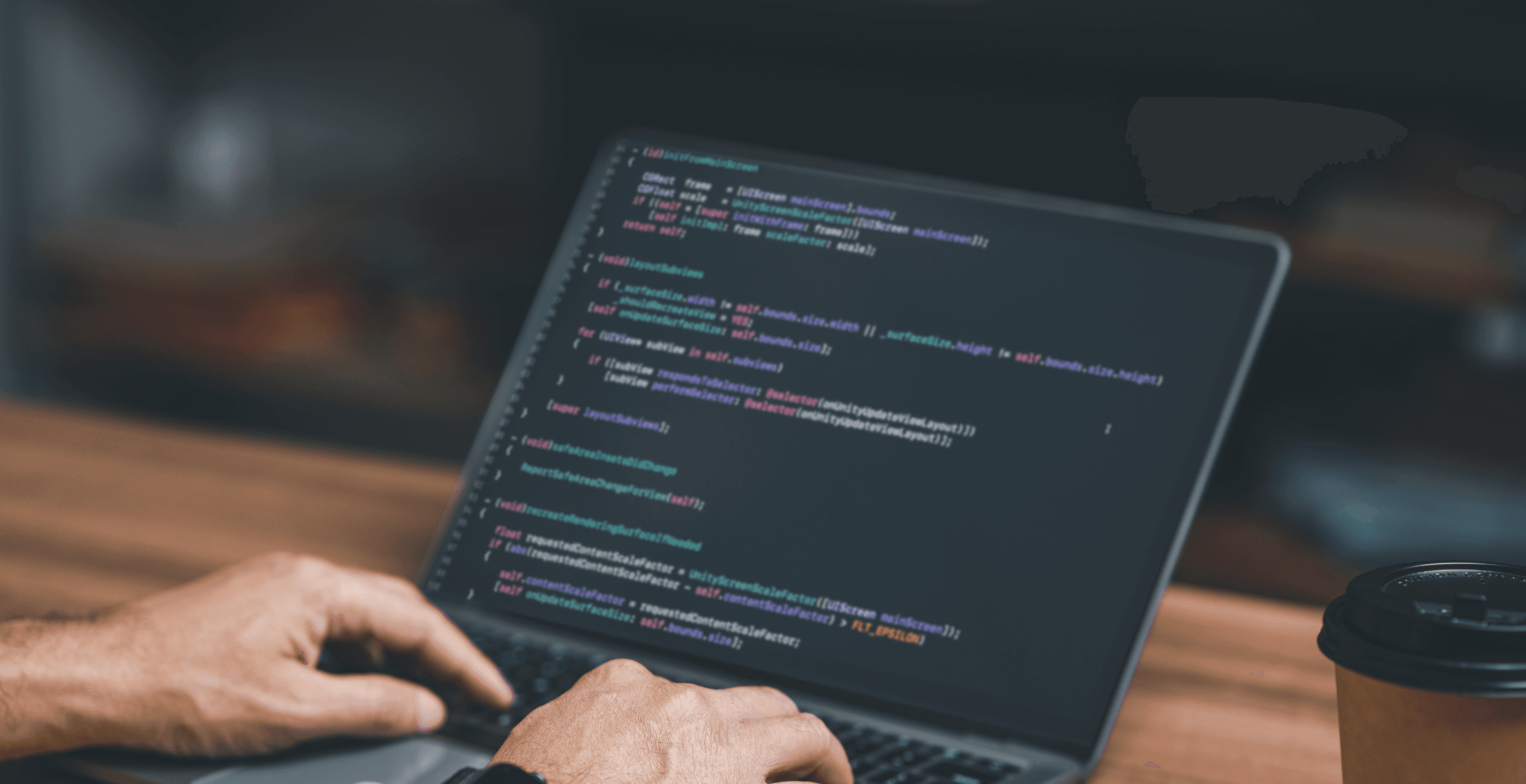
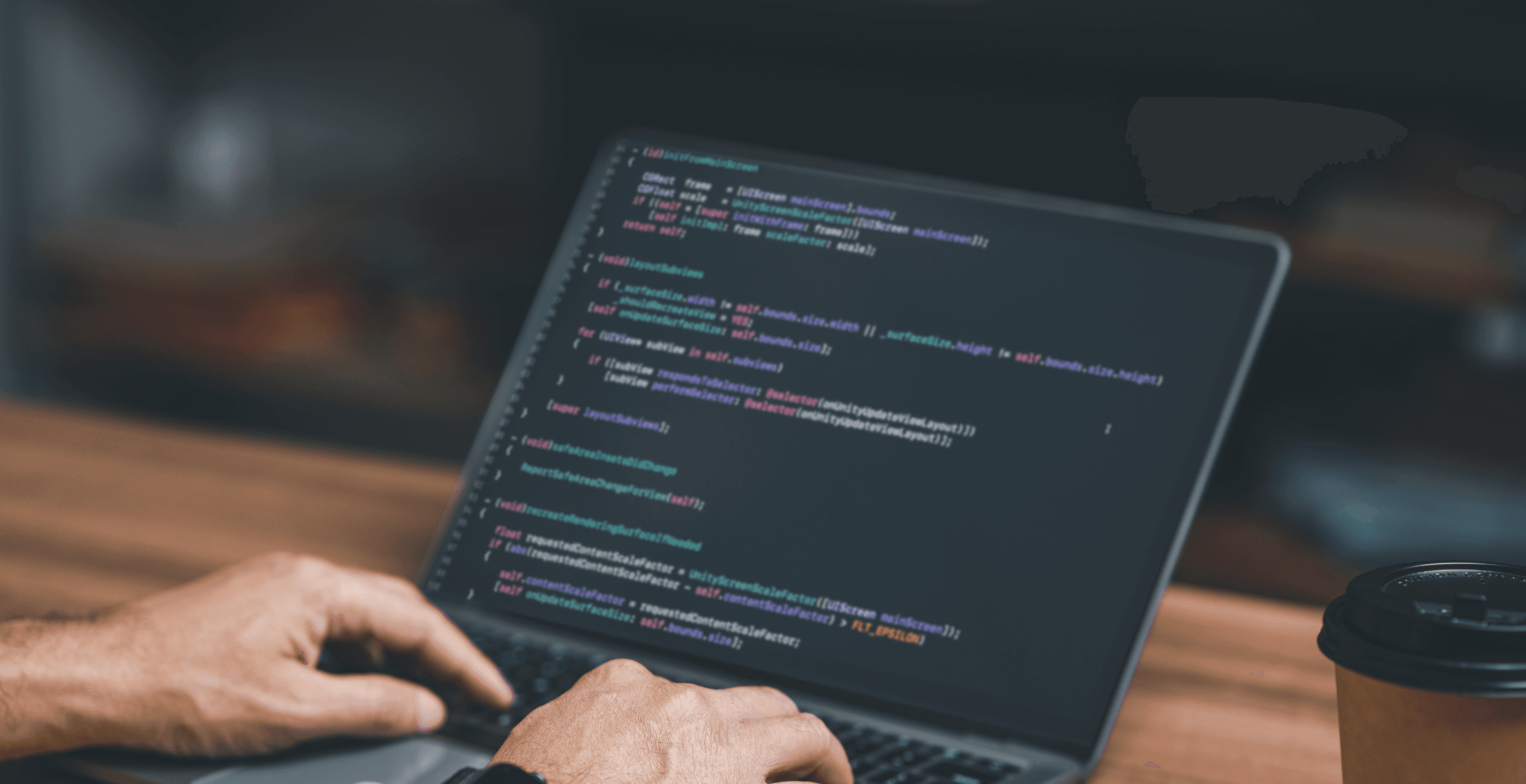
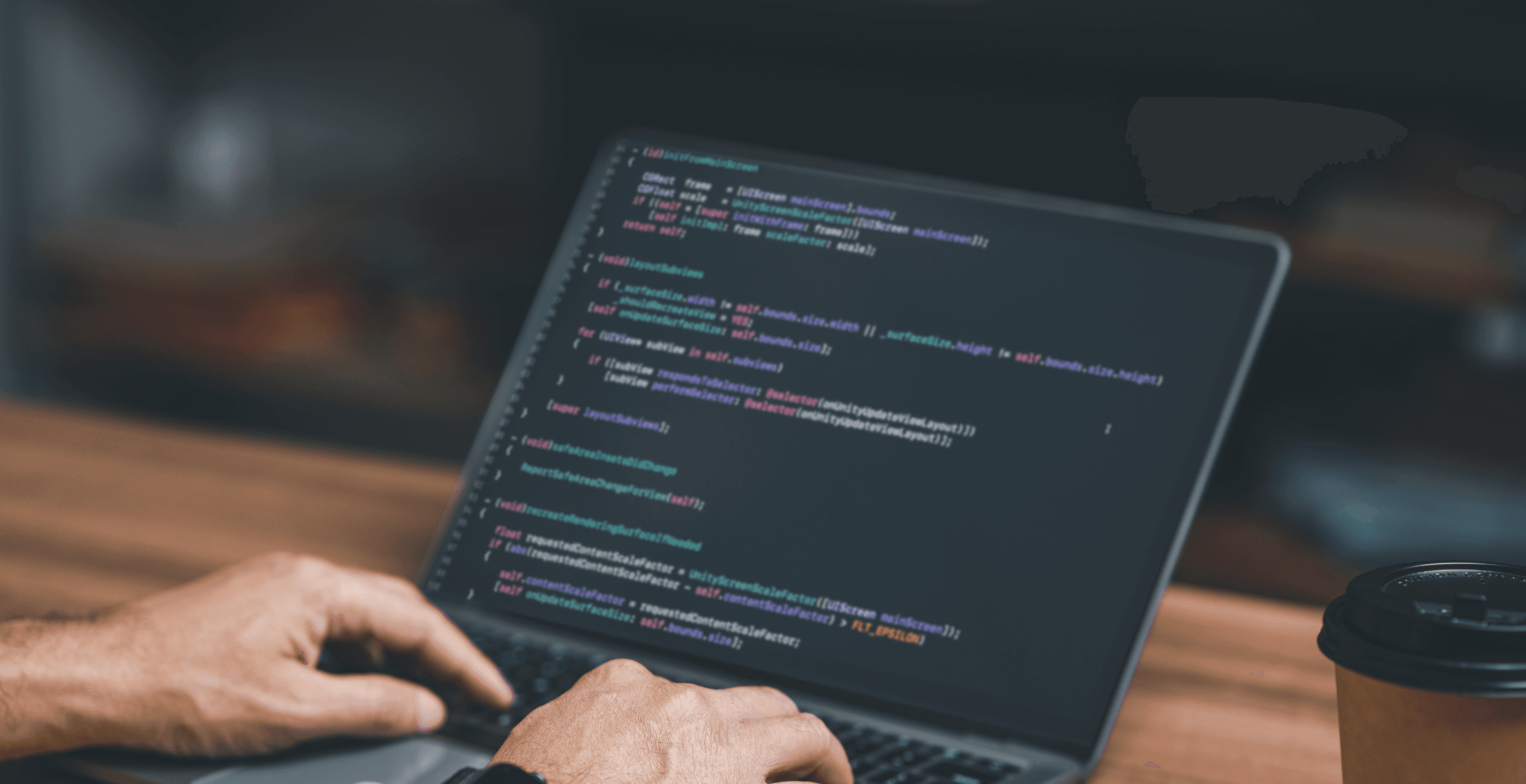
Building Your First LLM Agent Framework Application

Rehan Asif
Nov 4, 2024
Read the article




Multi-Agent Design and Collaboration Patterns

Rehan Asif
Nov 1, 2024
Read the article




Creating Your Own LLM Agent Application from Scratch

Rehan Asif
Oct 30, 2024
Read the article




Solving LLM Token Limit Issues: Understanding and Approaches

Rehan Asif
Oct 27, 2024
Read the article
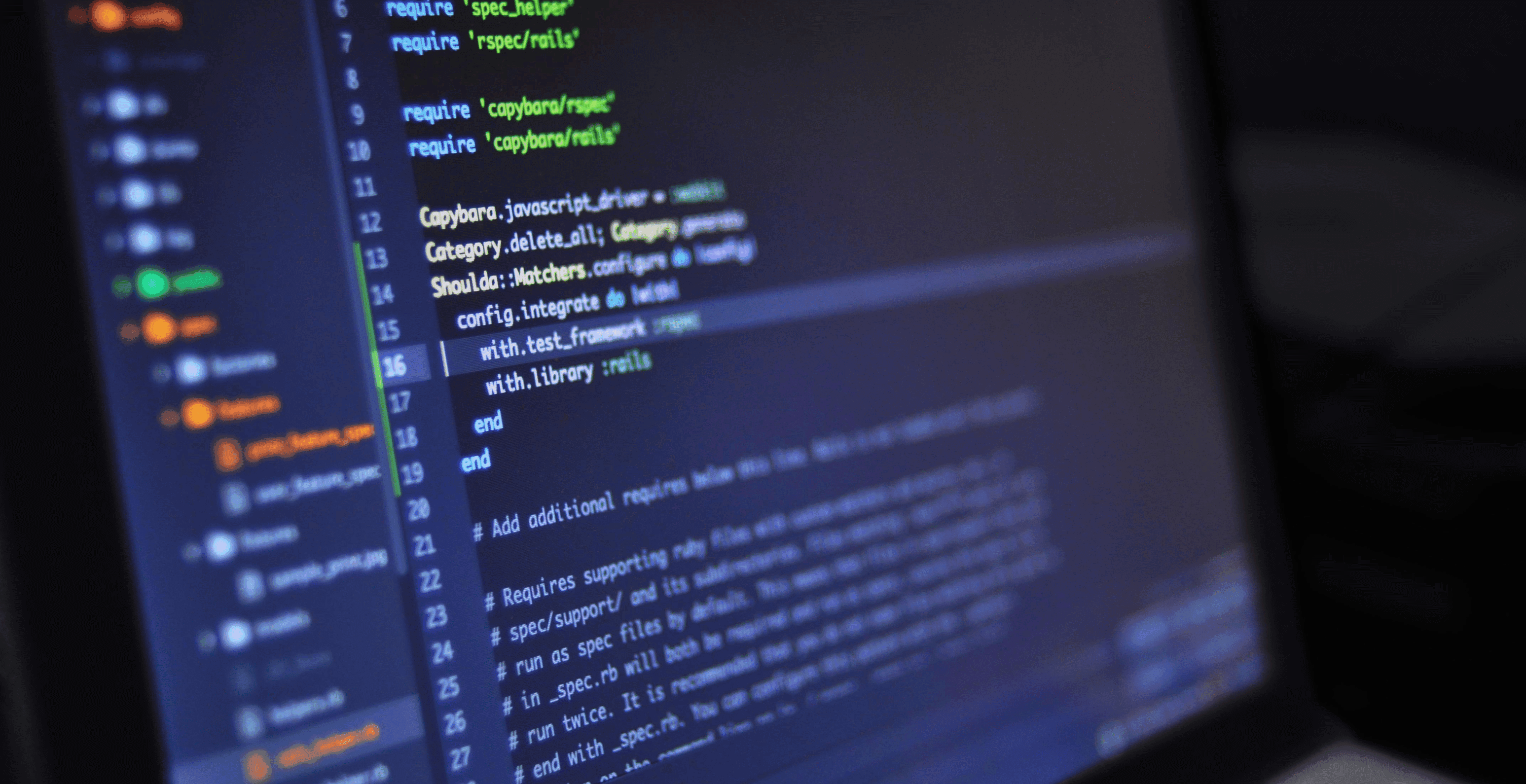
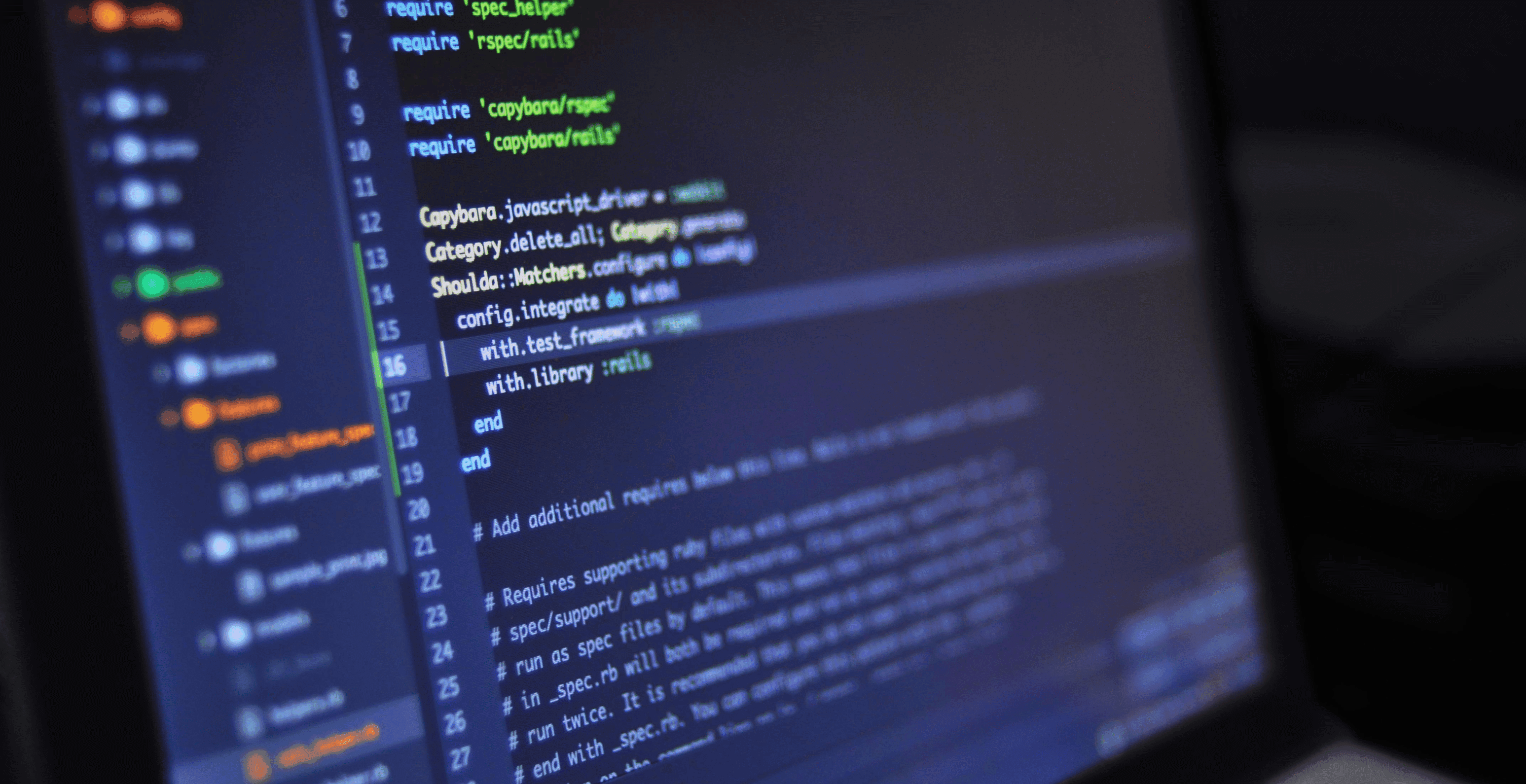
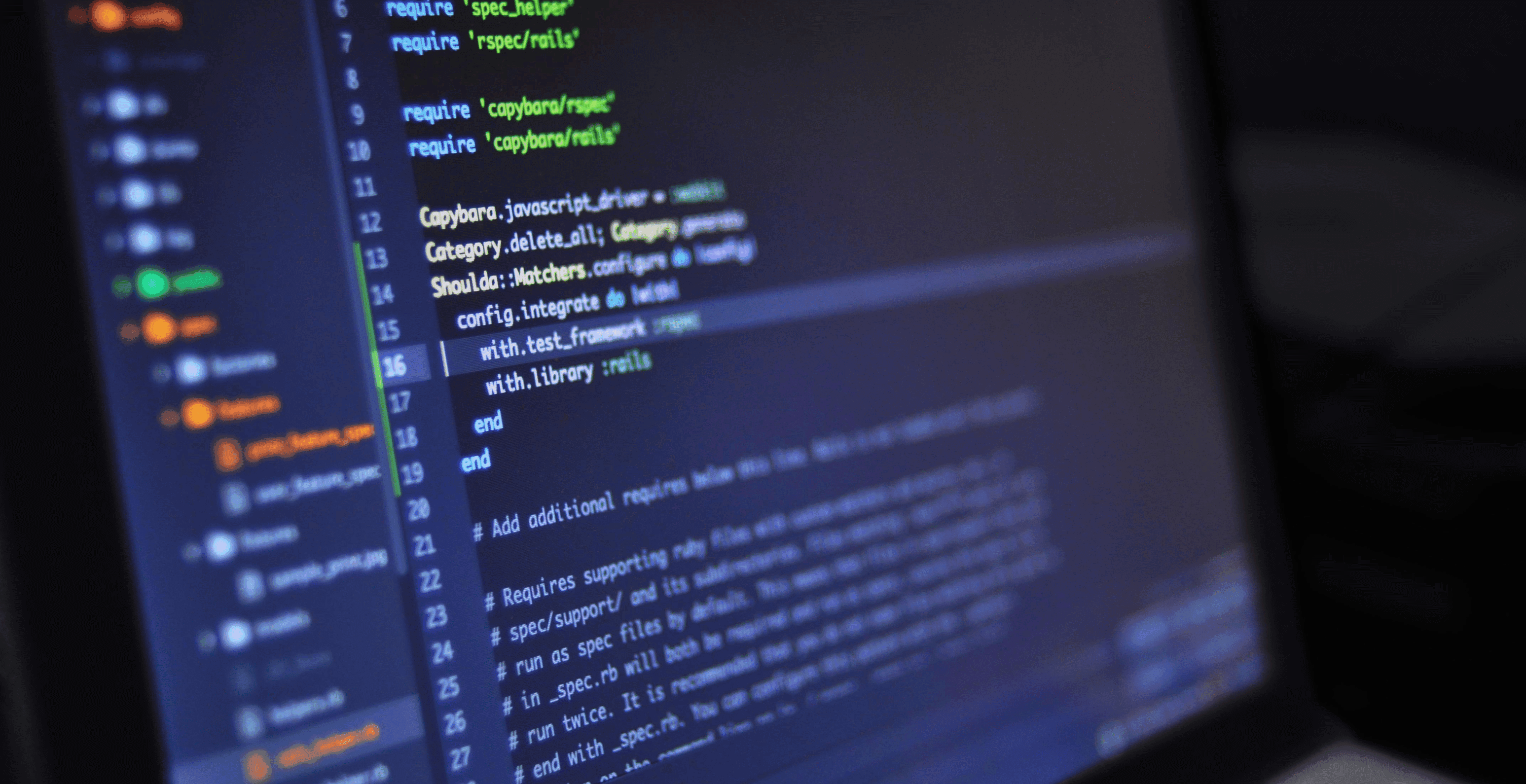
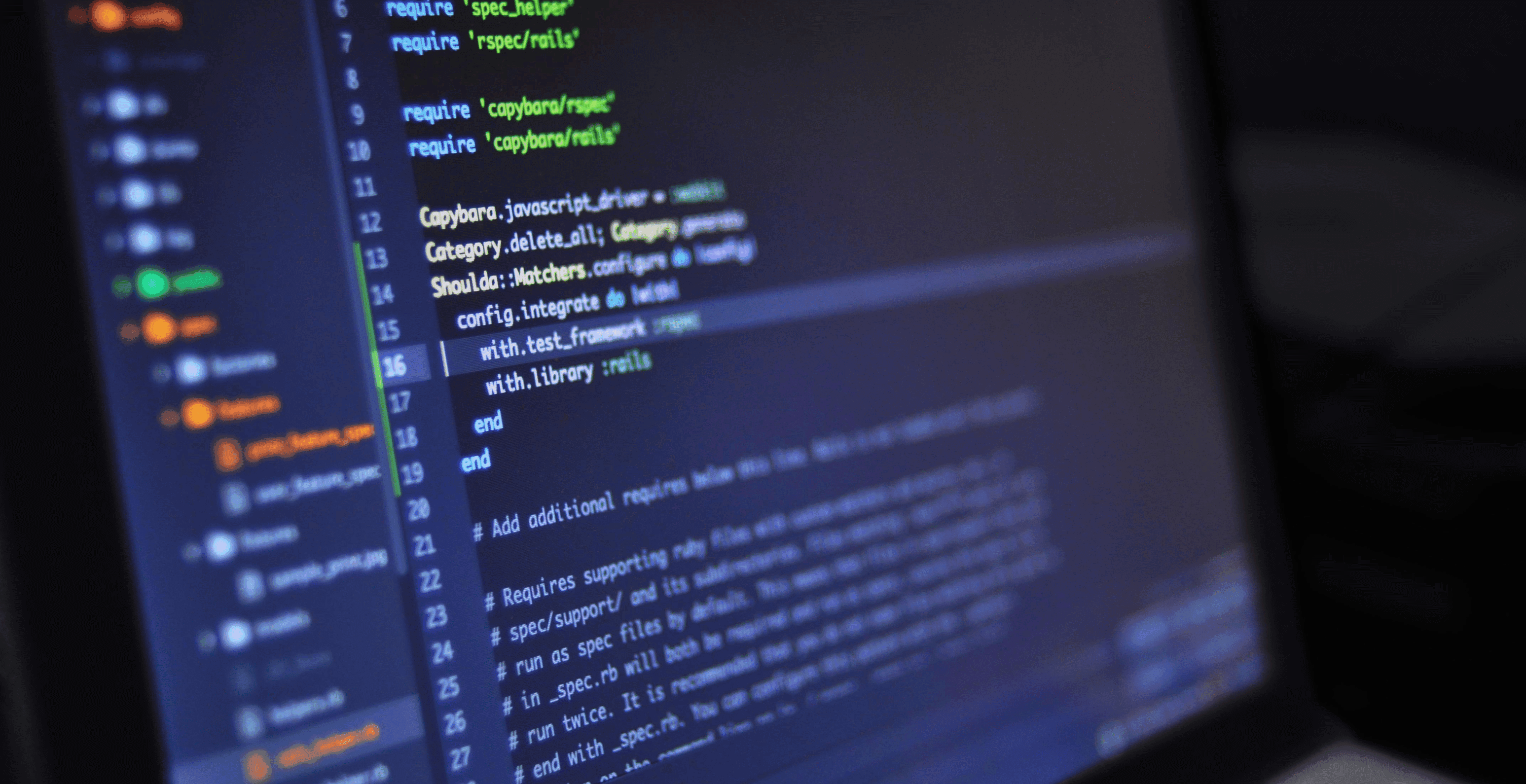
Understanding the Impact of Inference Cost on Generative AI Adoption
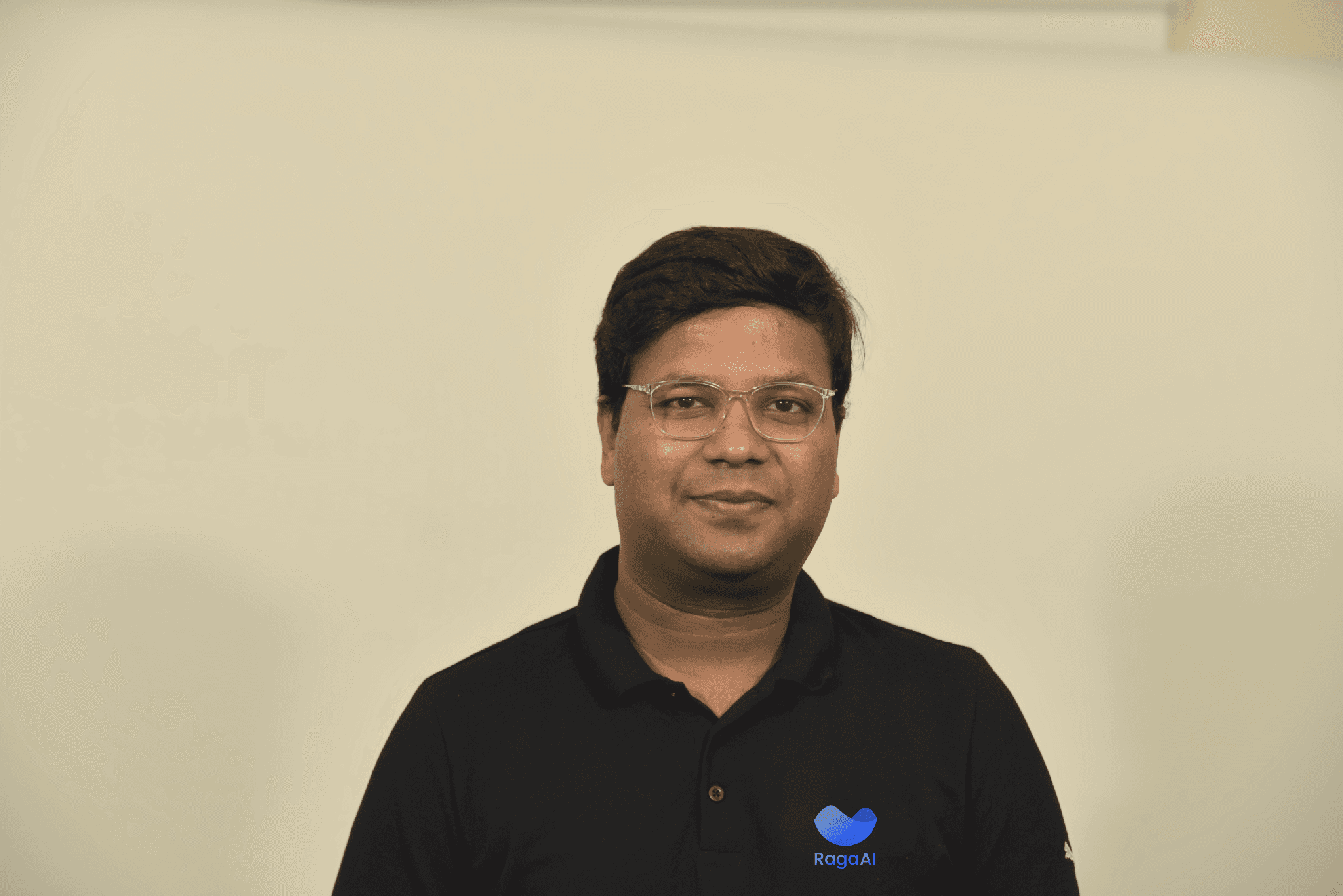
Jigar Gupta
Oct 24, 2024
Read the article




Data Security: Risks, Solutions, Types and Best Practices
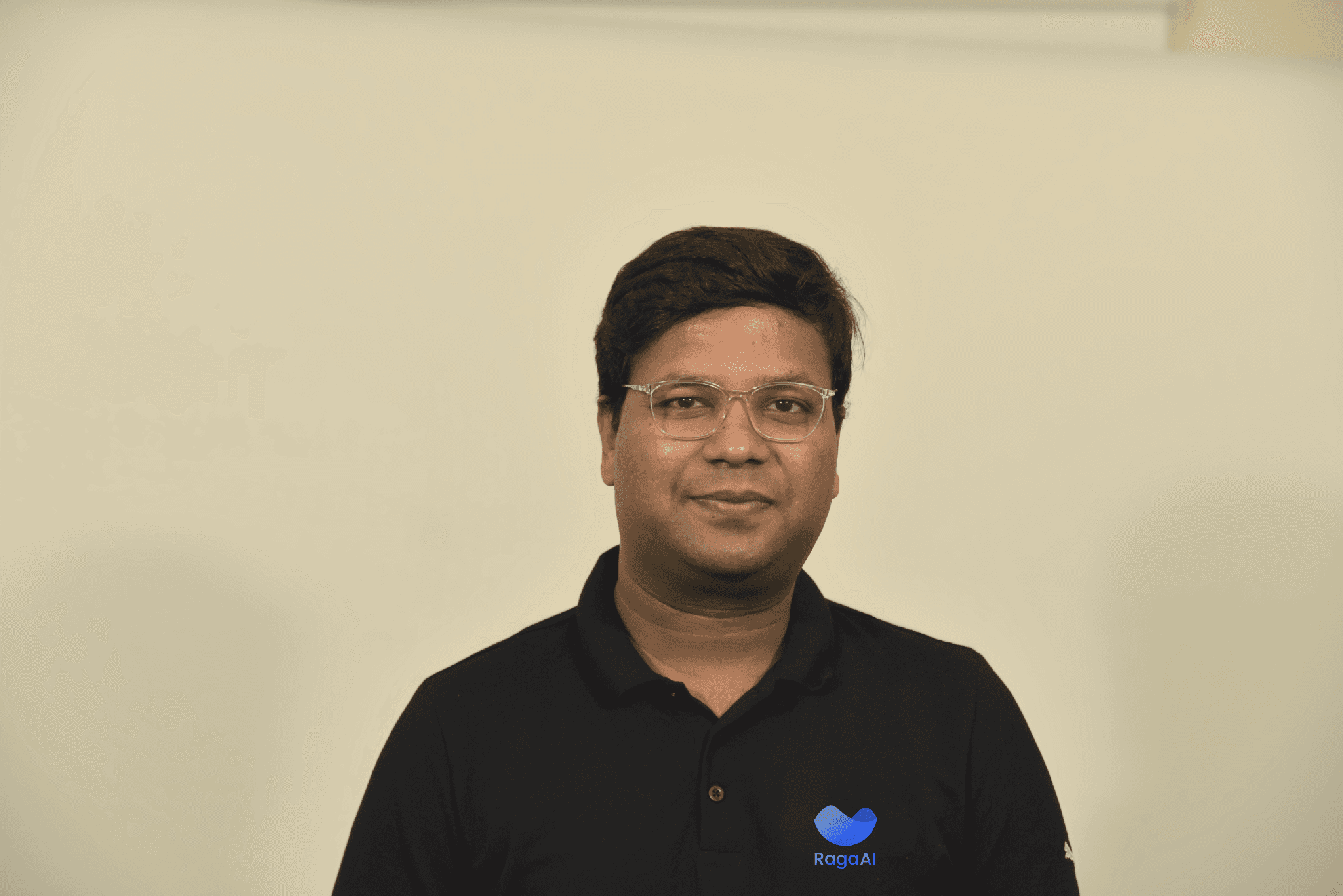
Jigar Gupta
Oct 21, 2024
Read the article
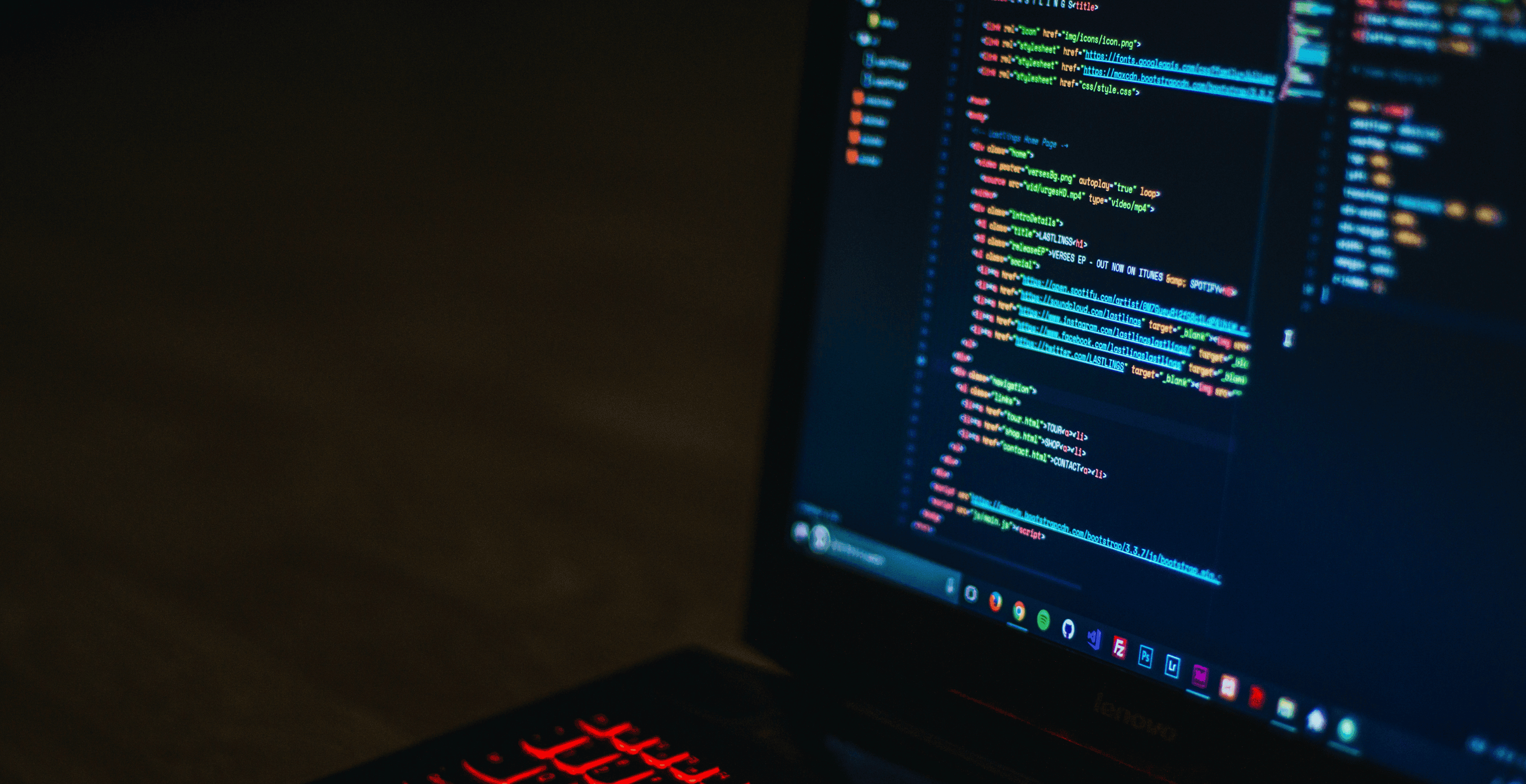
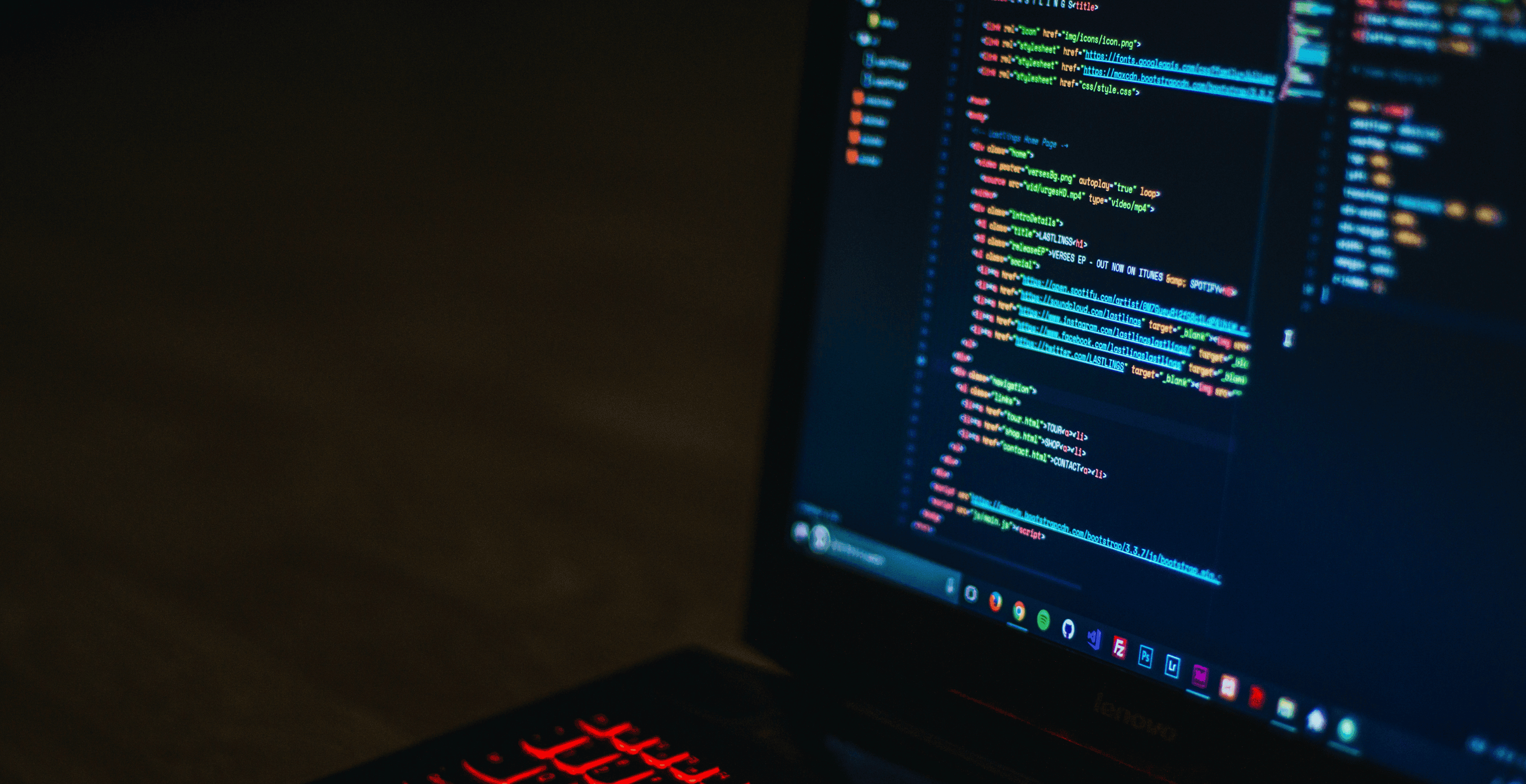
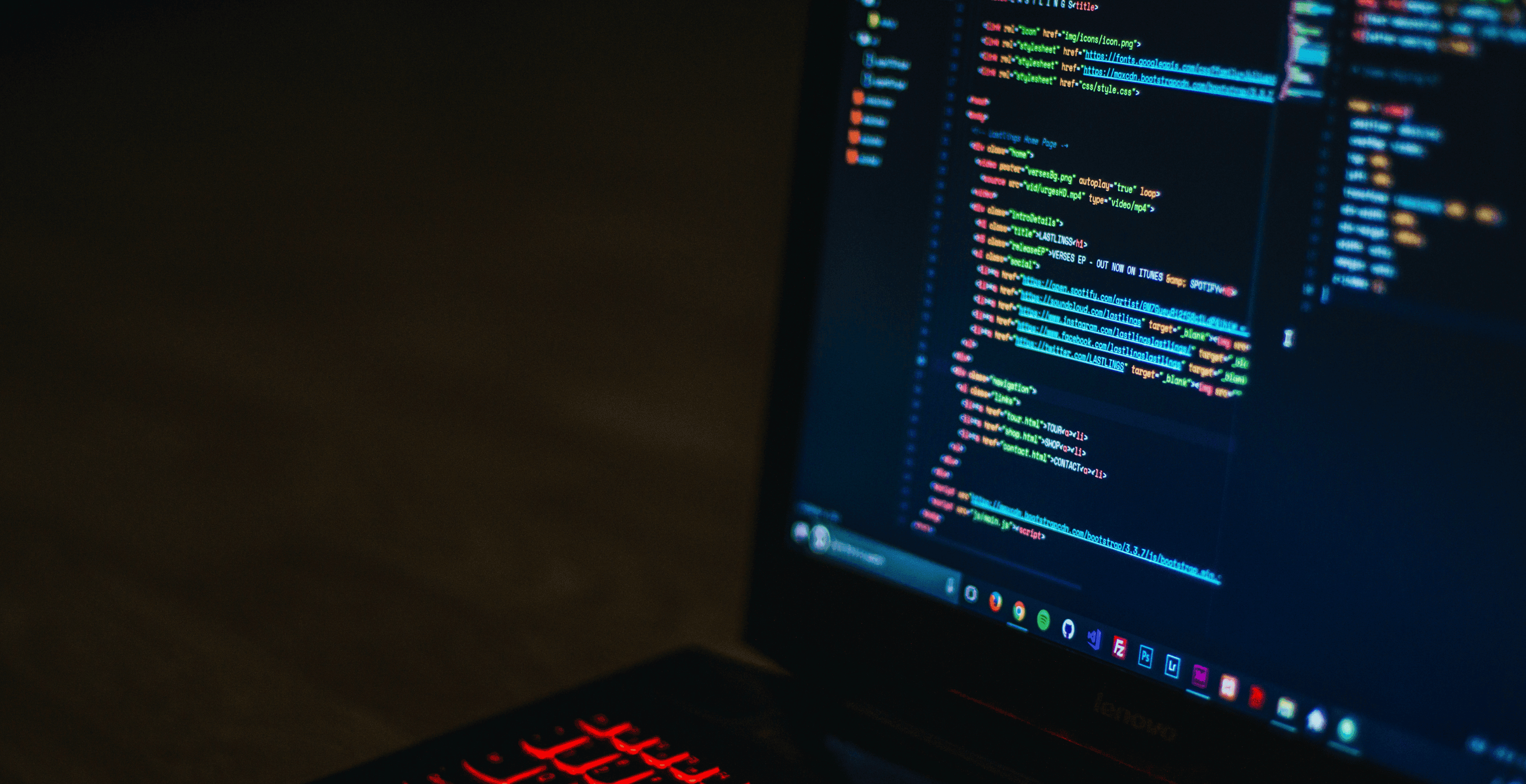
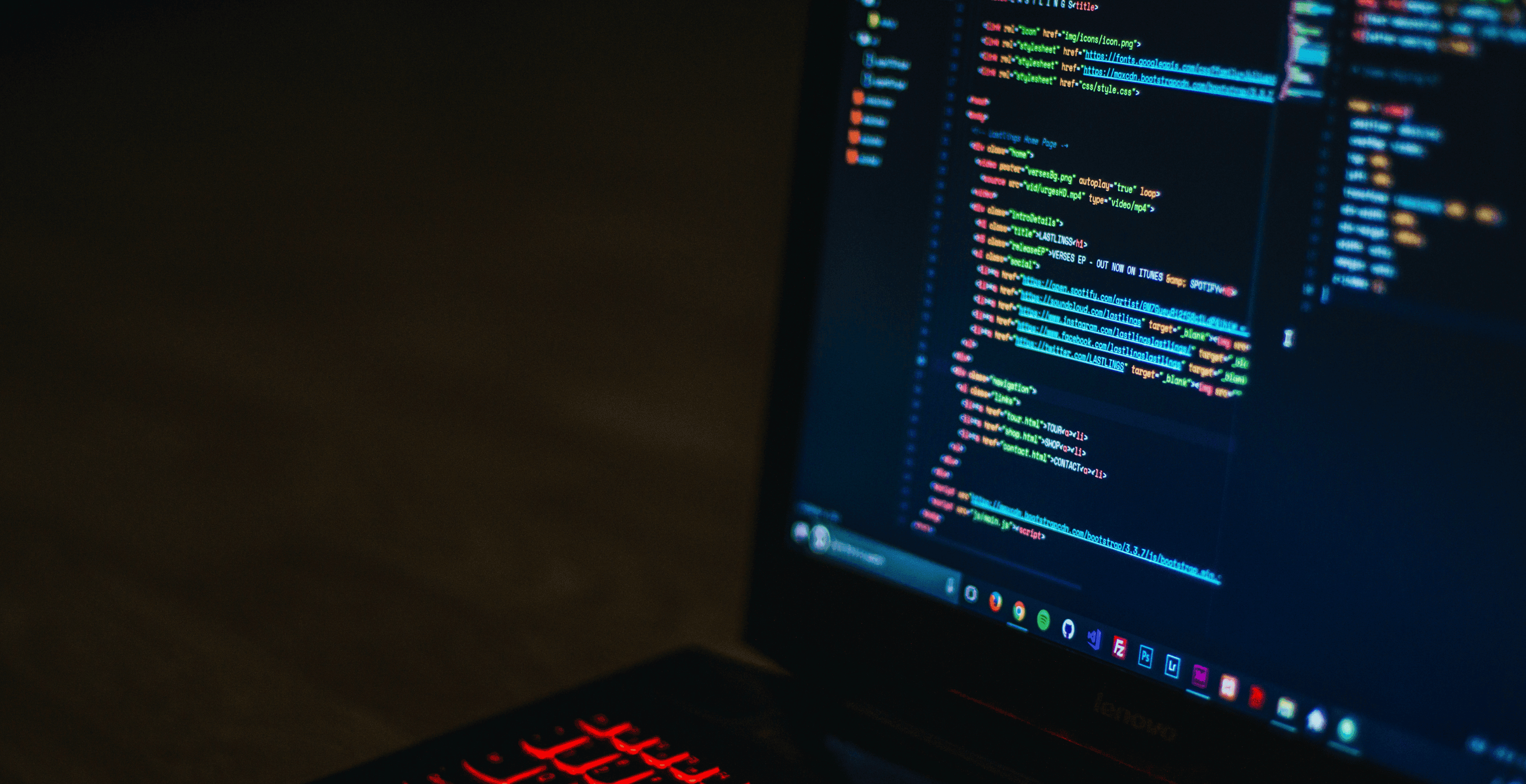
Getting Contextual Understanding Right for RAG Applications
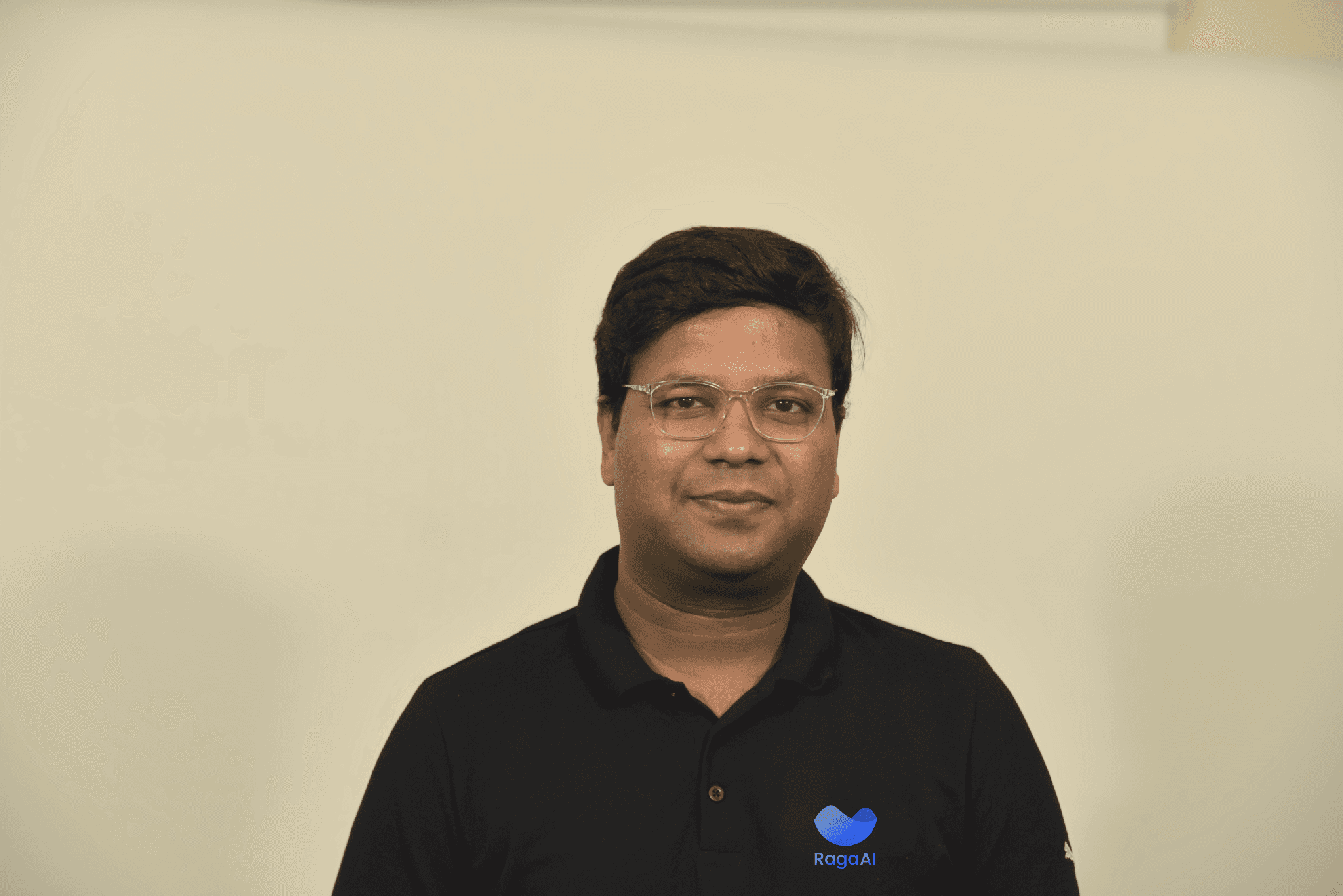
Jigar Gupta
Oct 19, 2024
Read the article
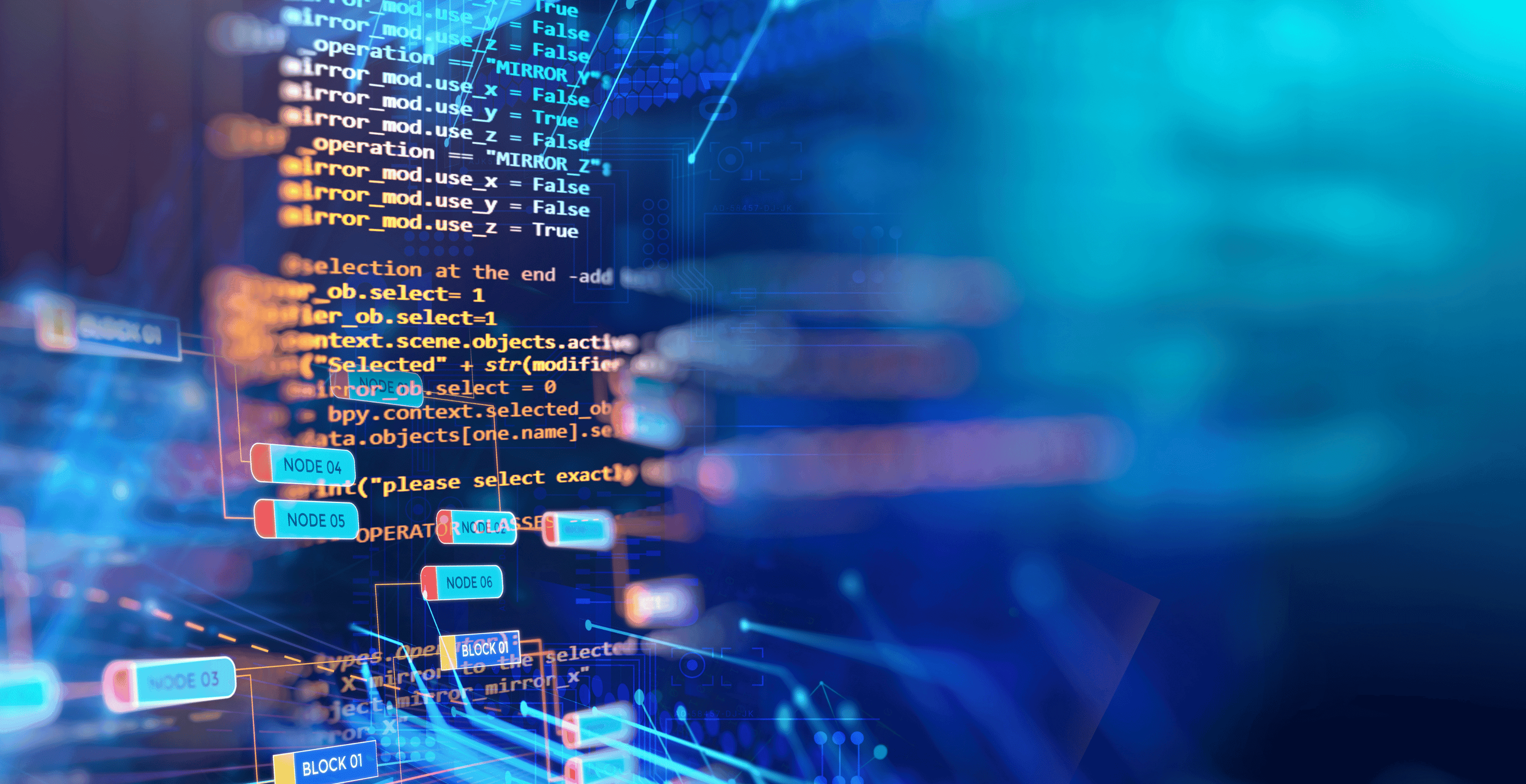
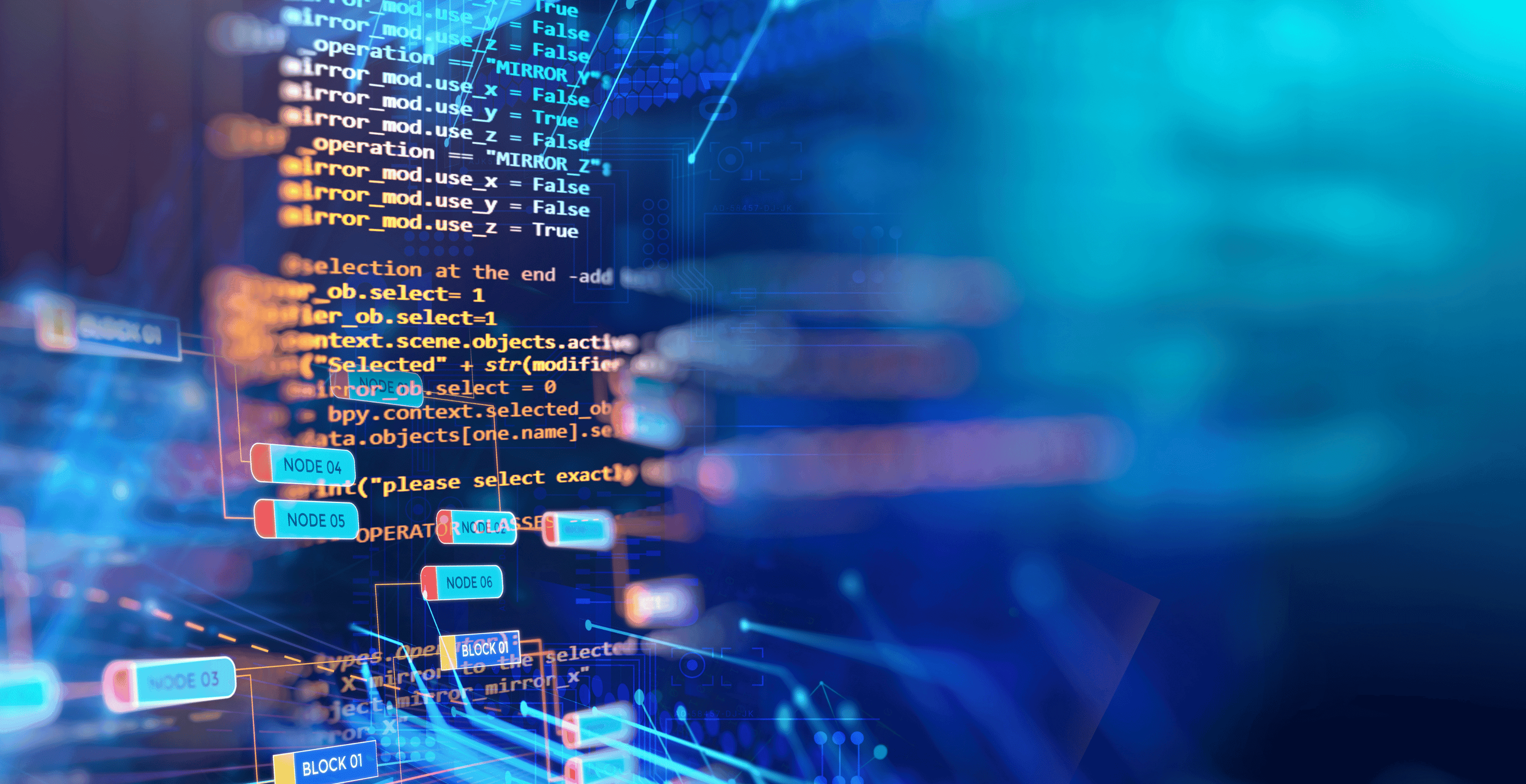
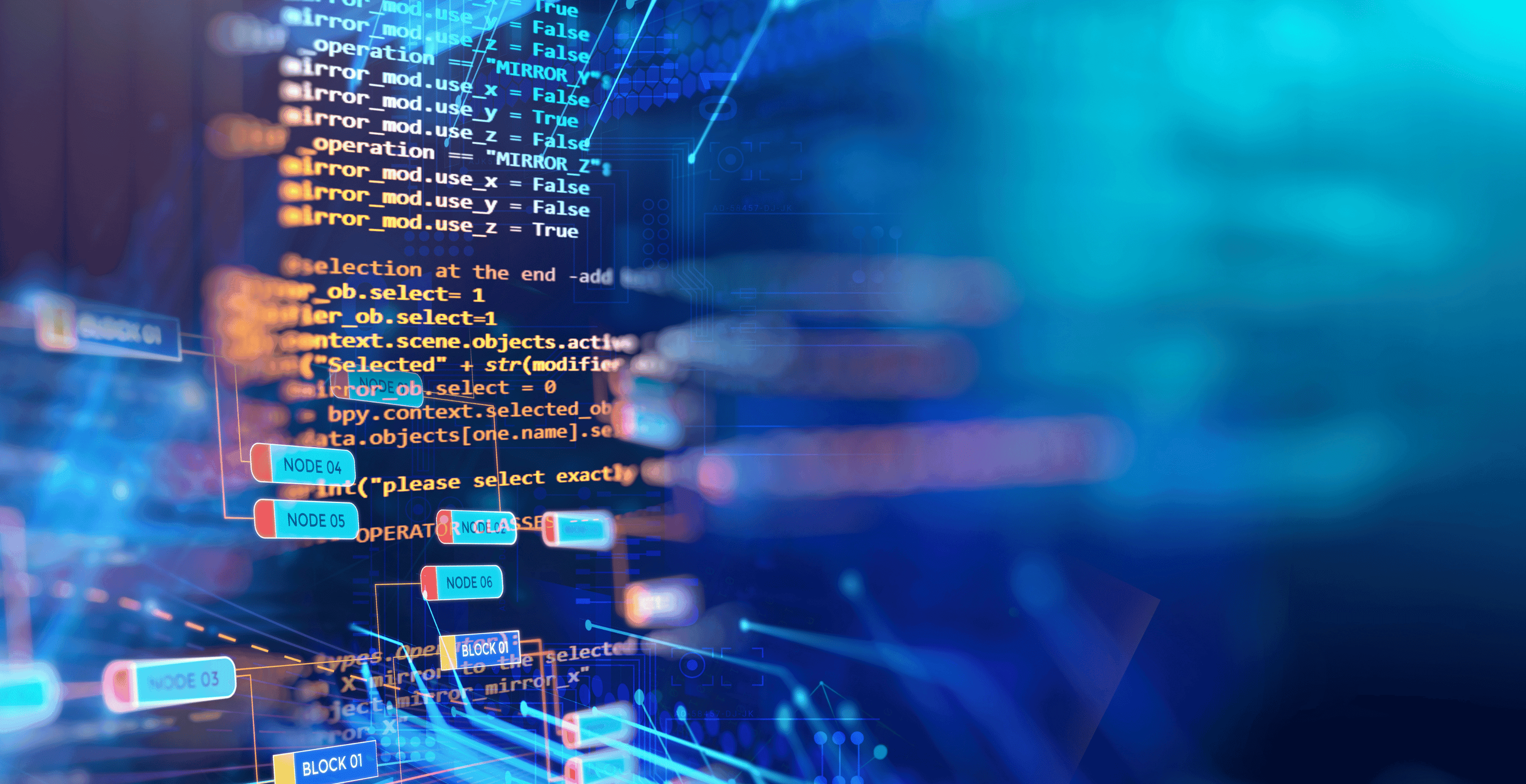
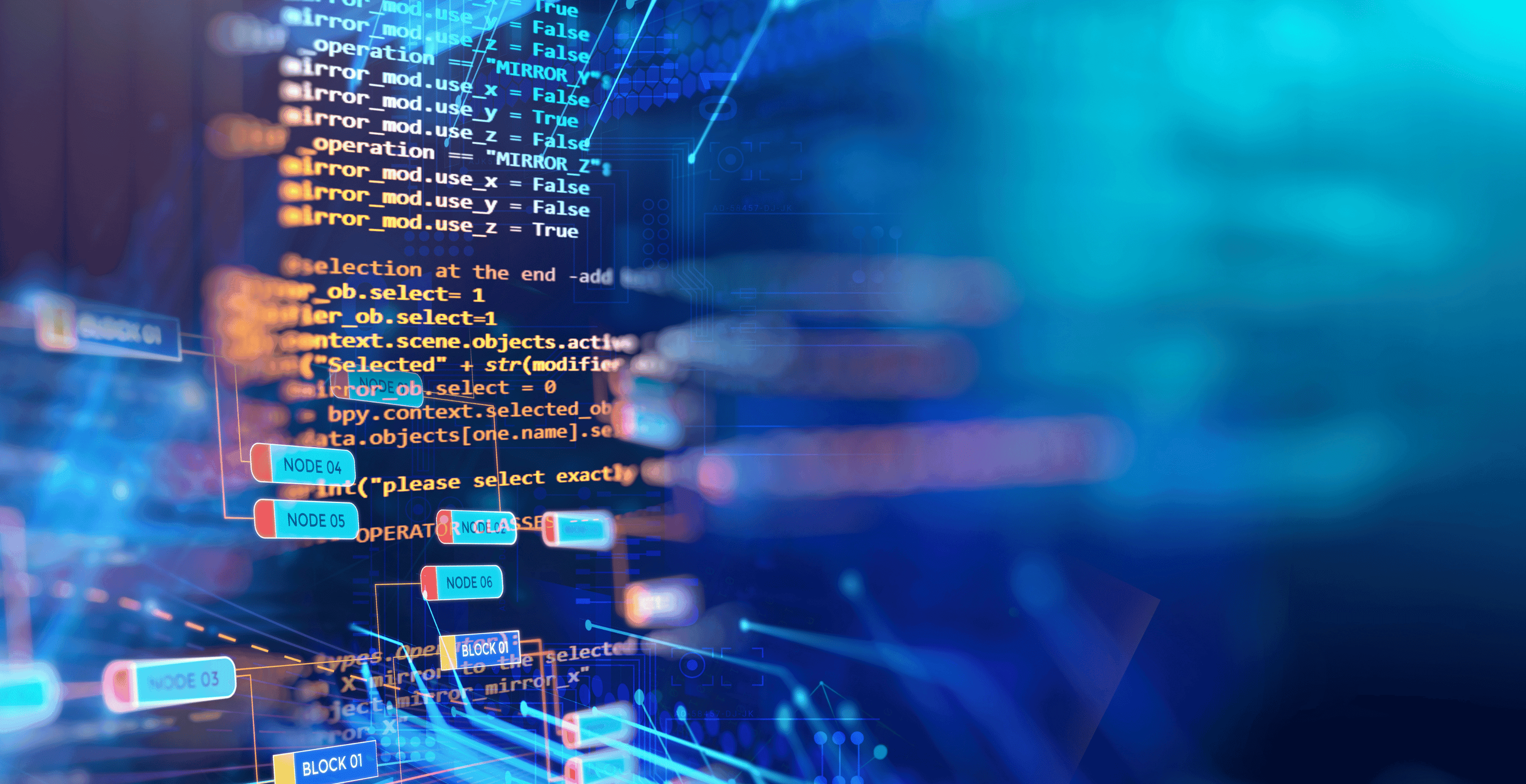
Understanding Data Fragmentation and Strategies to Overcome It
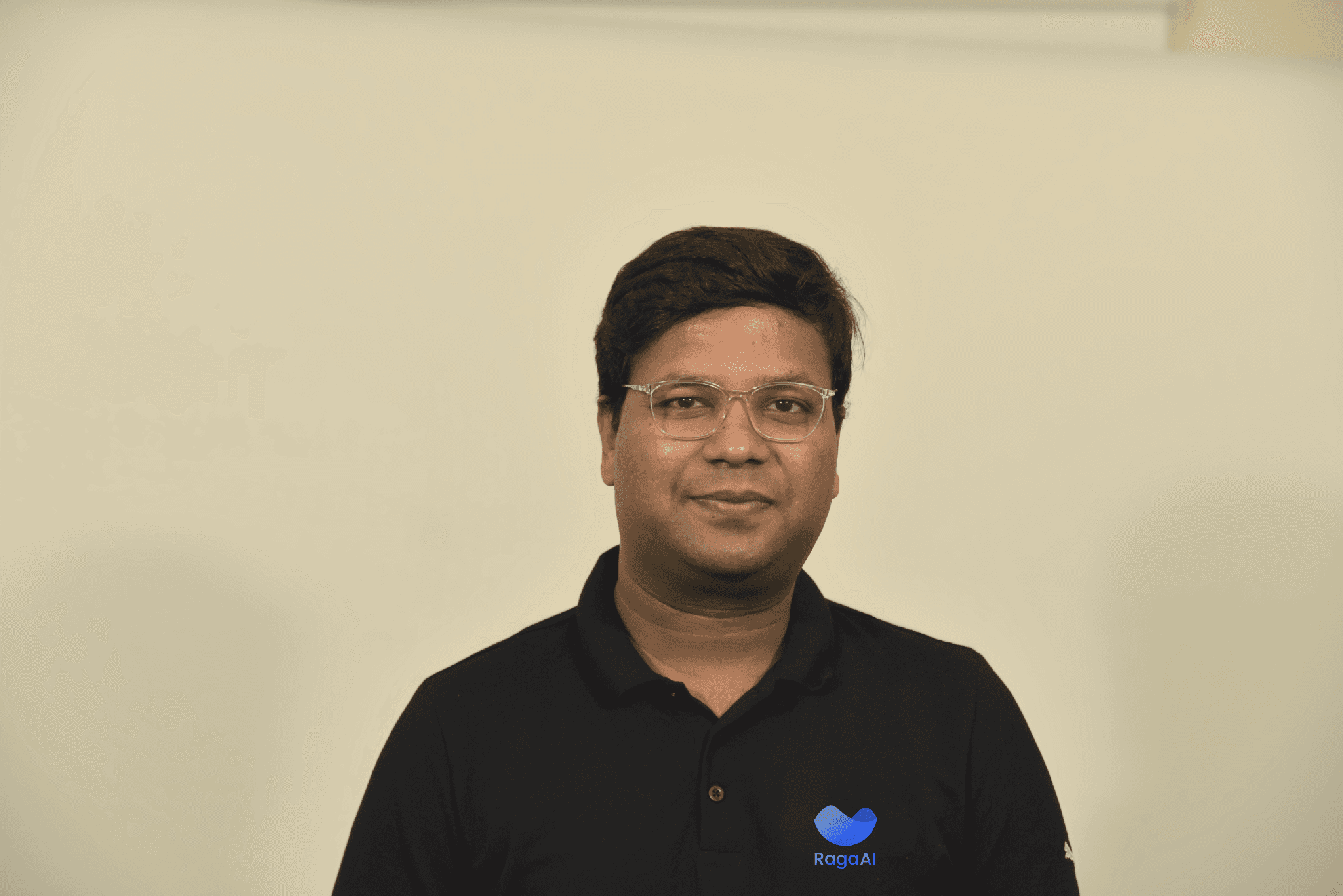
Jigar Gupta
Oct 16, 2024
Read the article
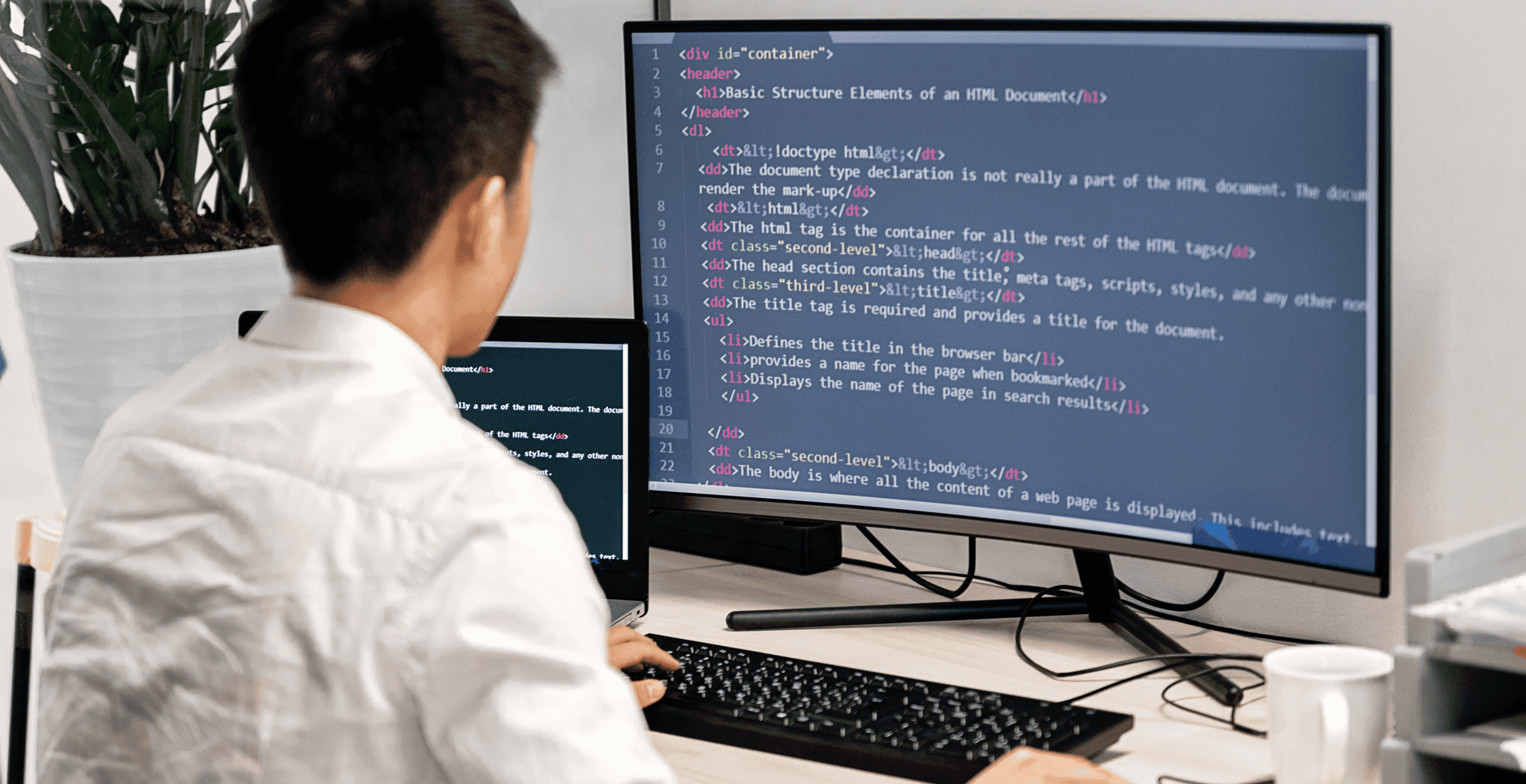
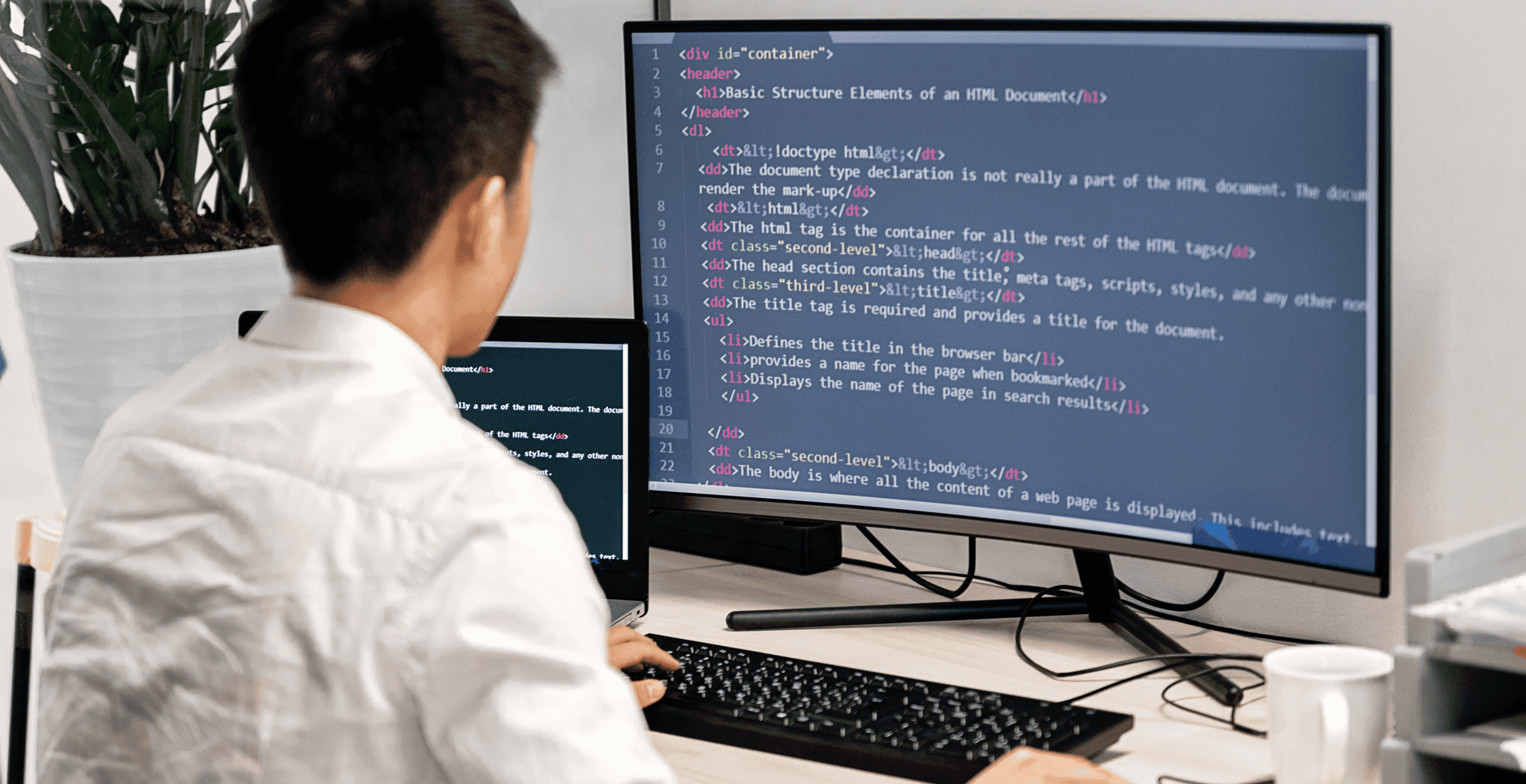
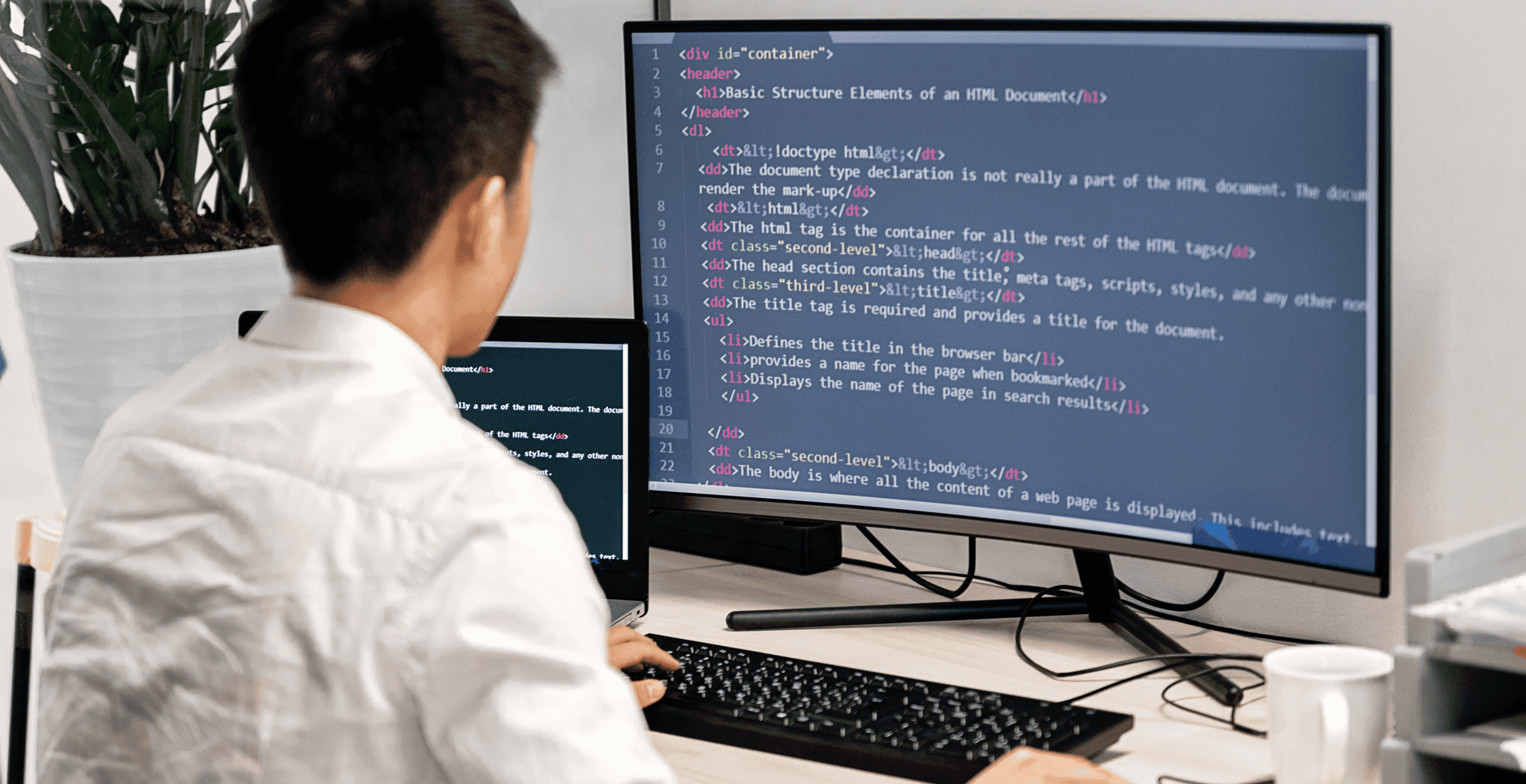
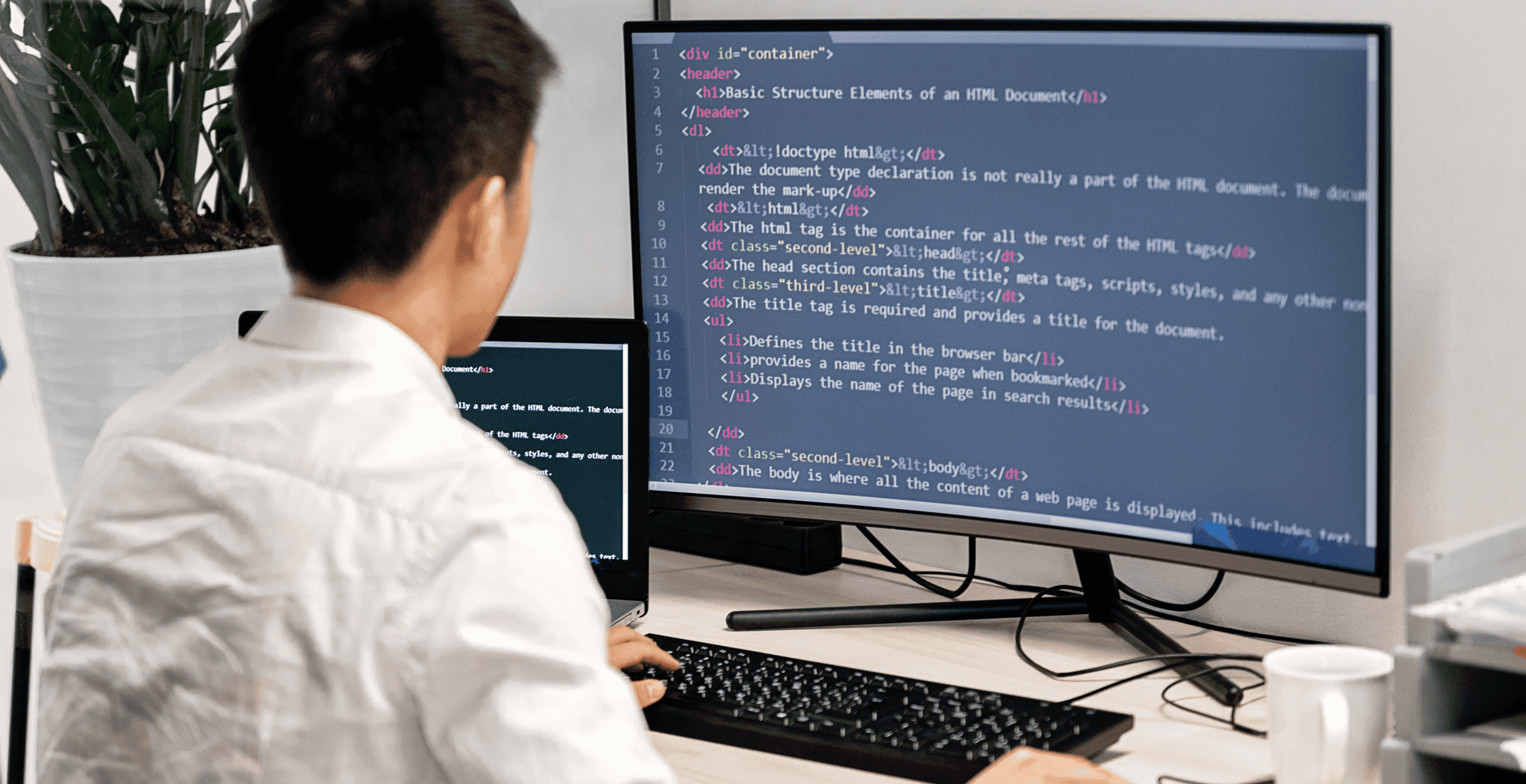
Understanding Techniques and Applications for Grounding LLMs in Data

Rehan Asif
Oct 13, 2024
Read the article




Advantages Of Using LLMs For Rapid Application Development

Rehan Asif
Oct 10, 2024
Read the article
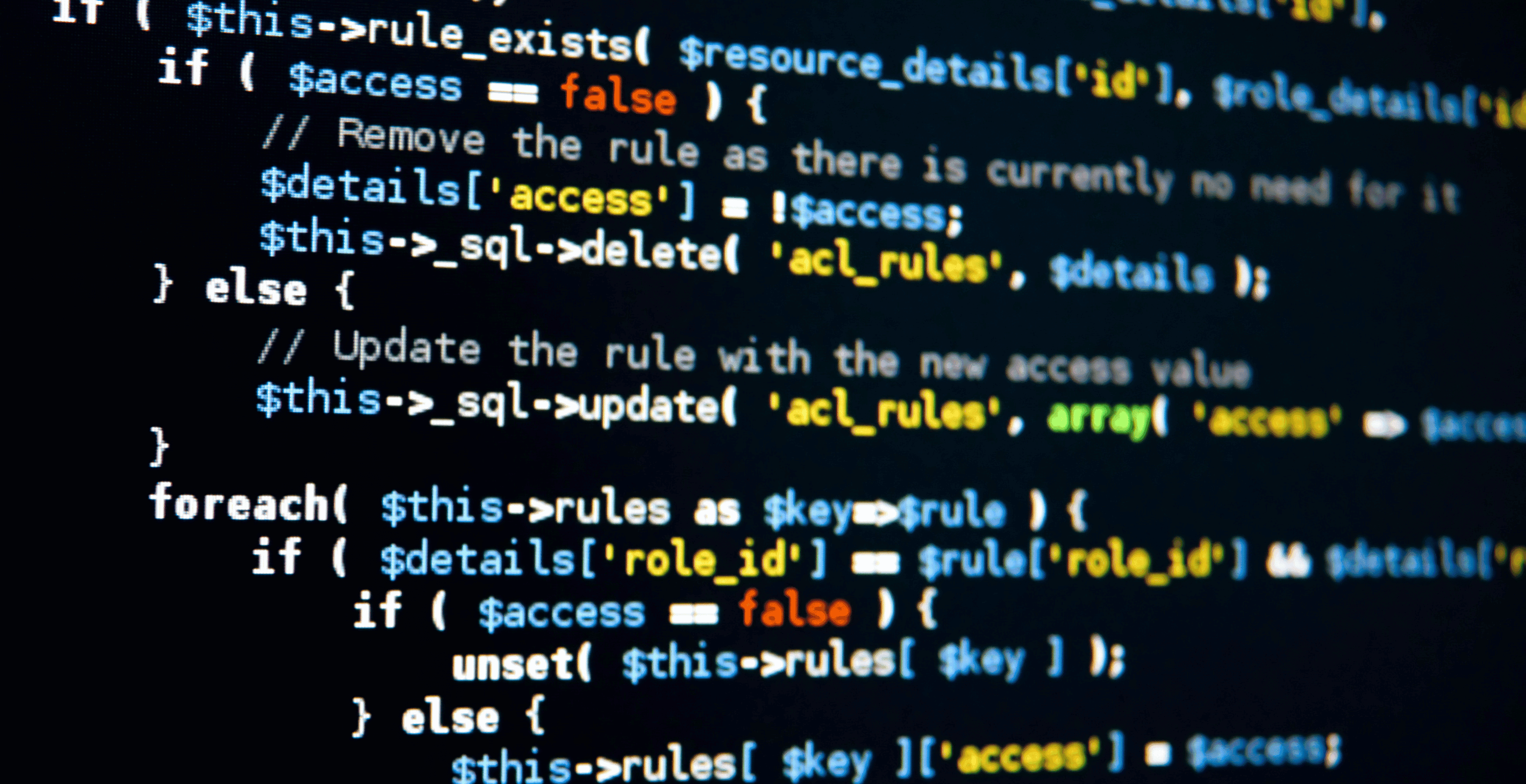
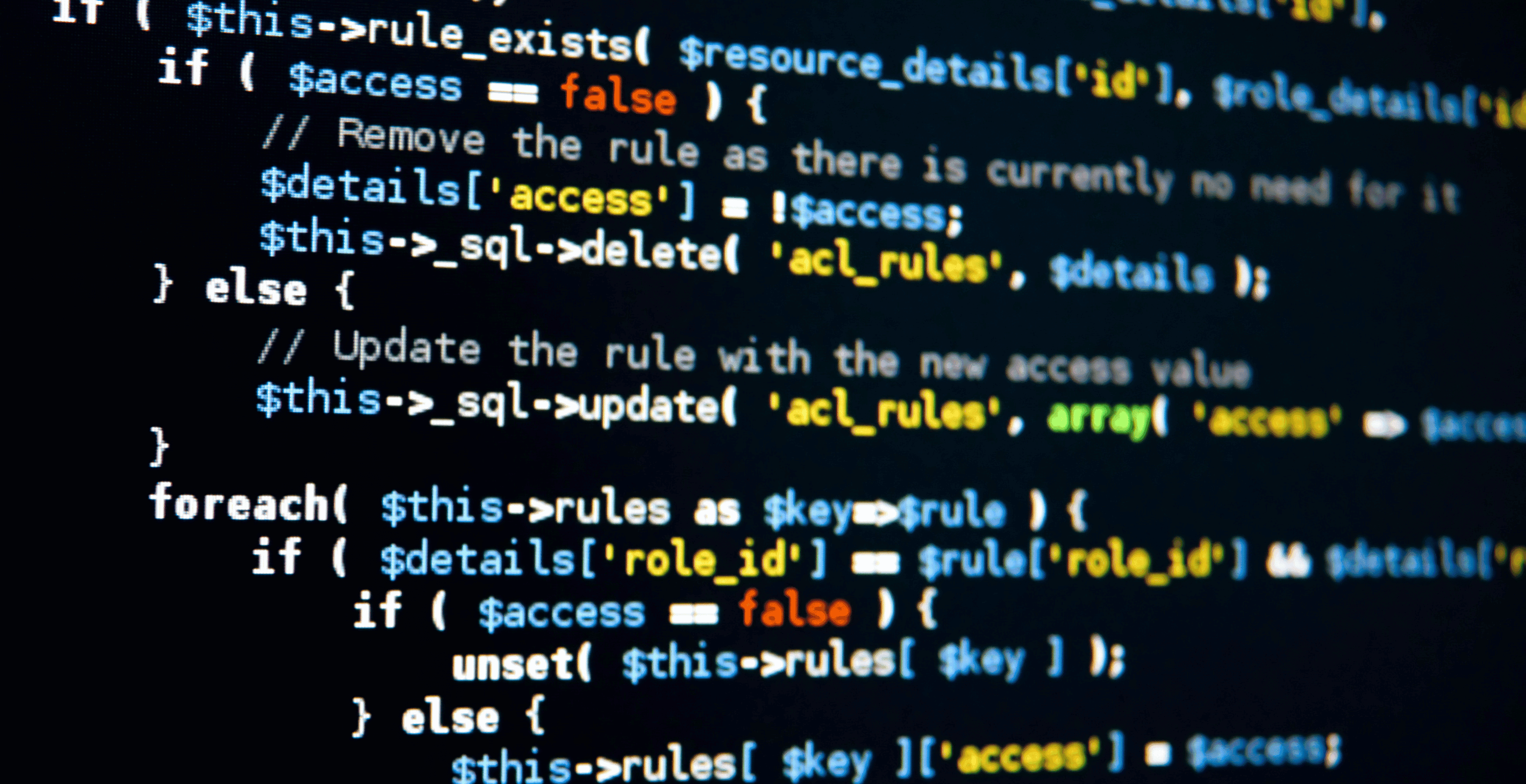
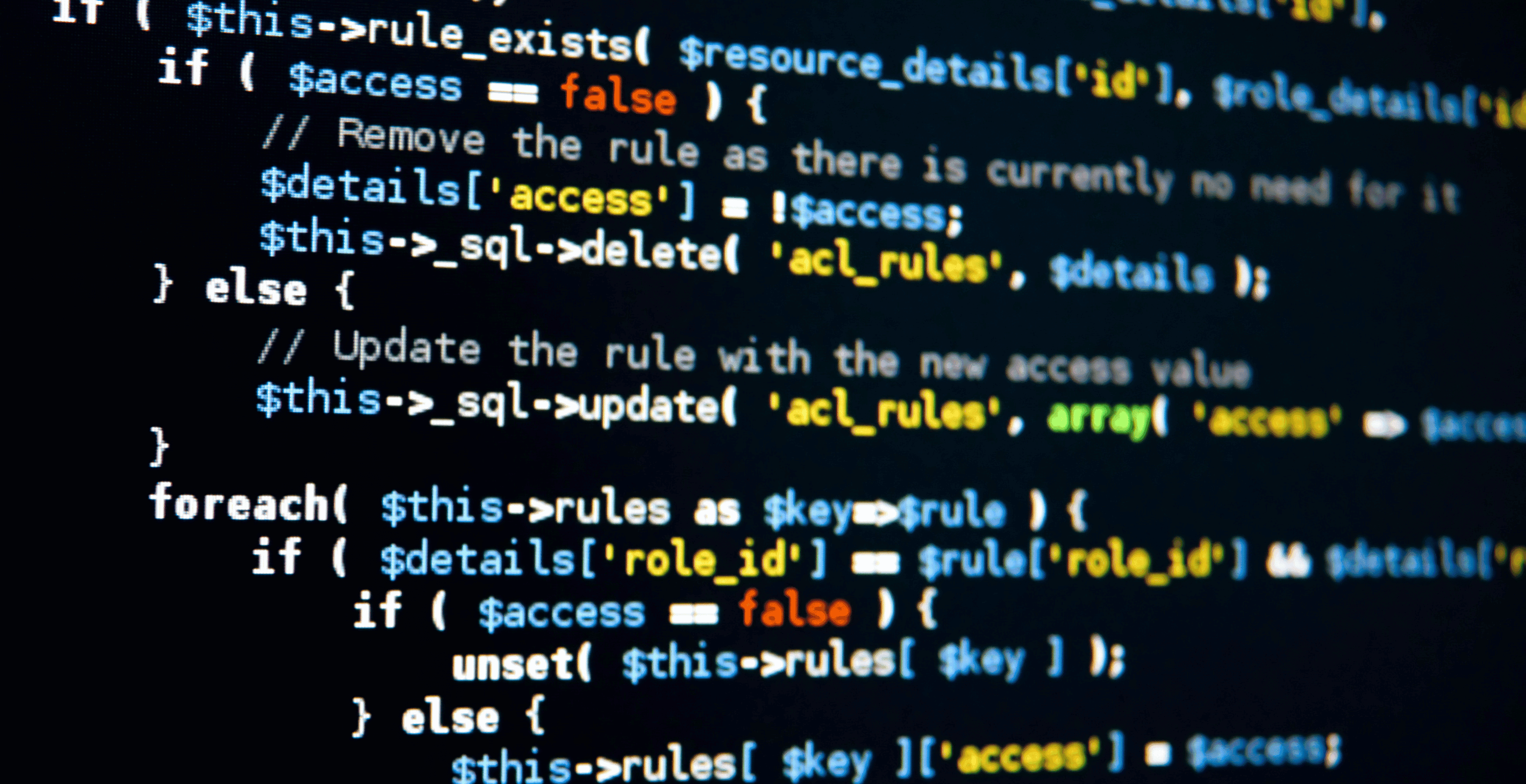
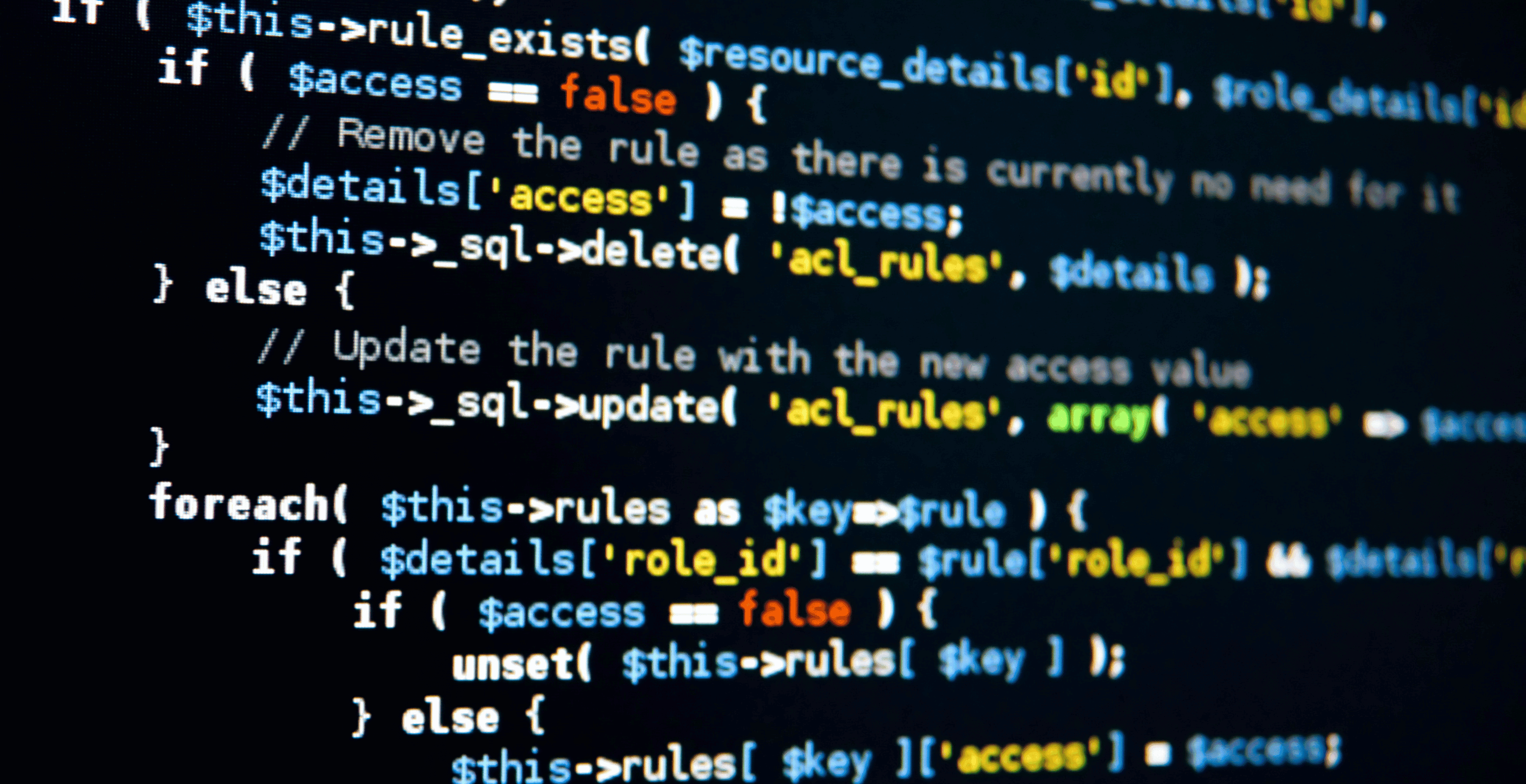
Understanding React Agent in LangChain Engineering

Rehan Asif
Oct 7, 2024
Read the article
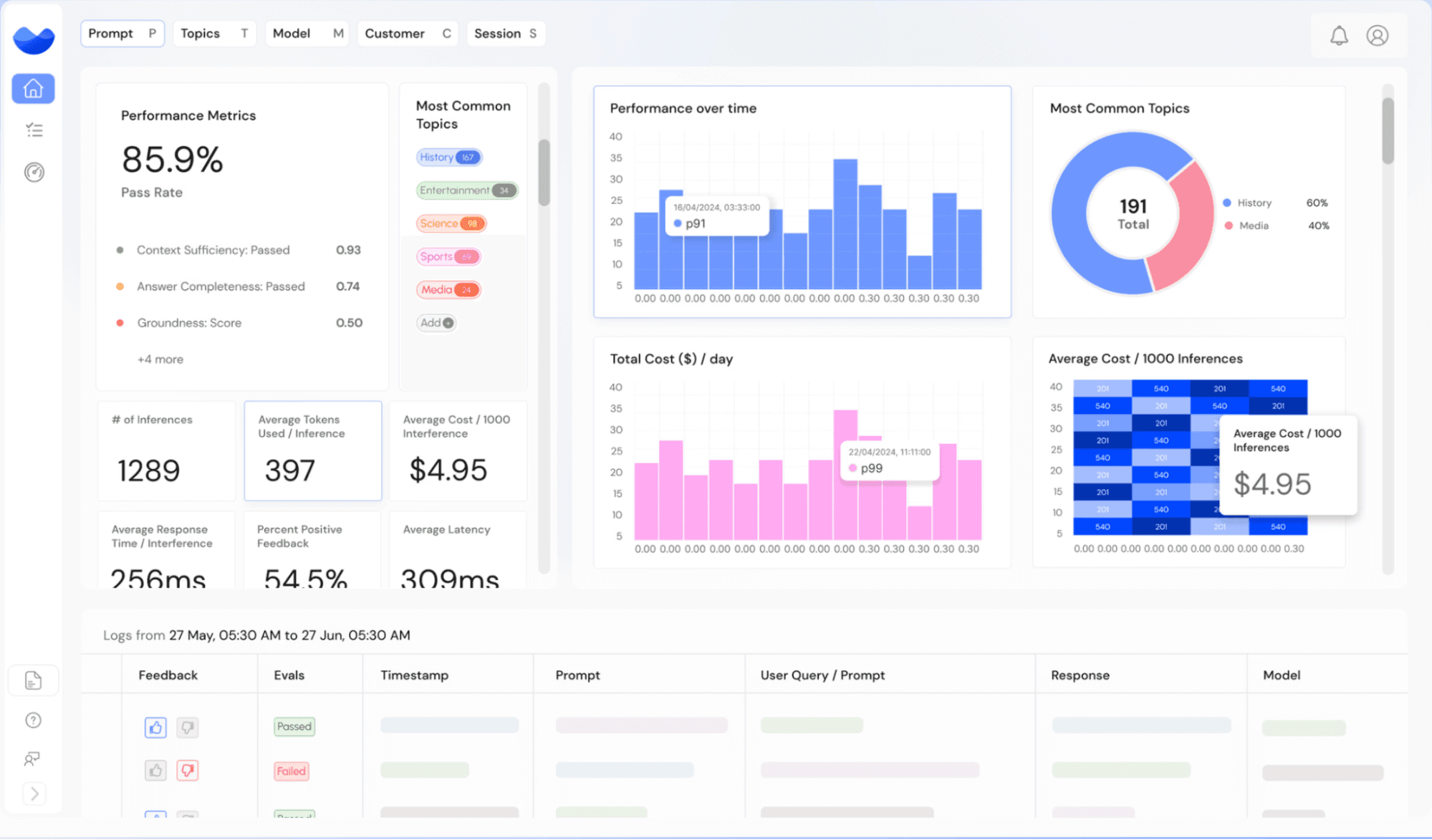
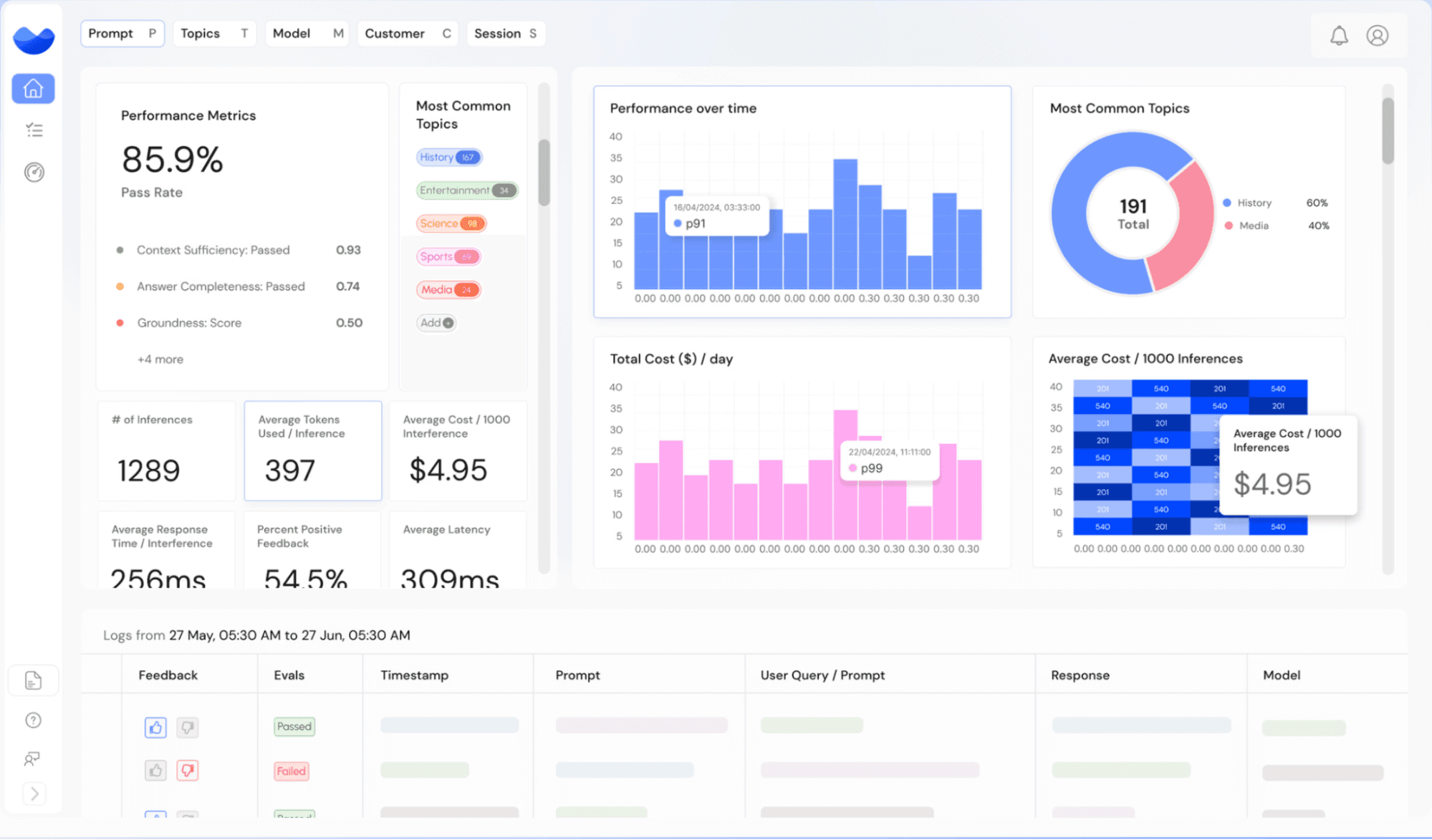
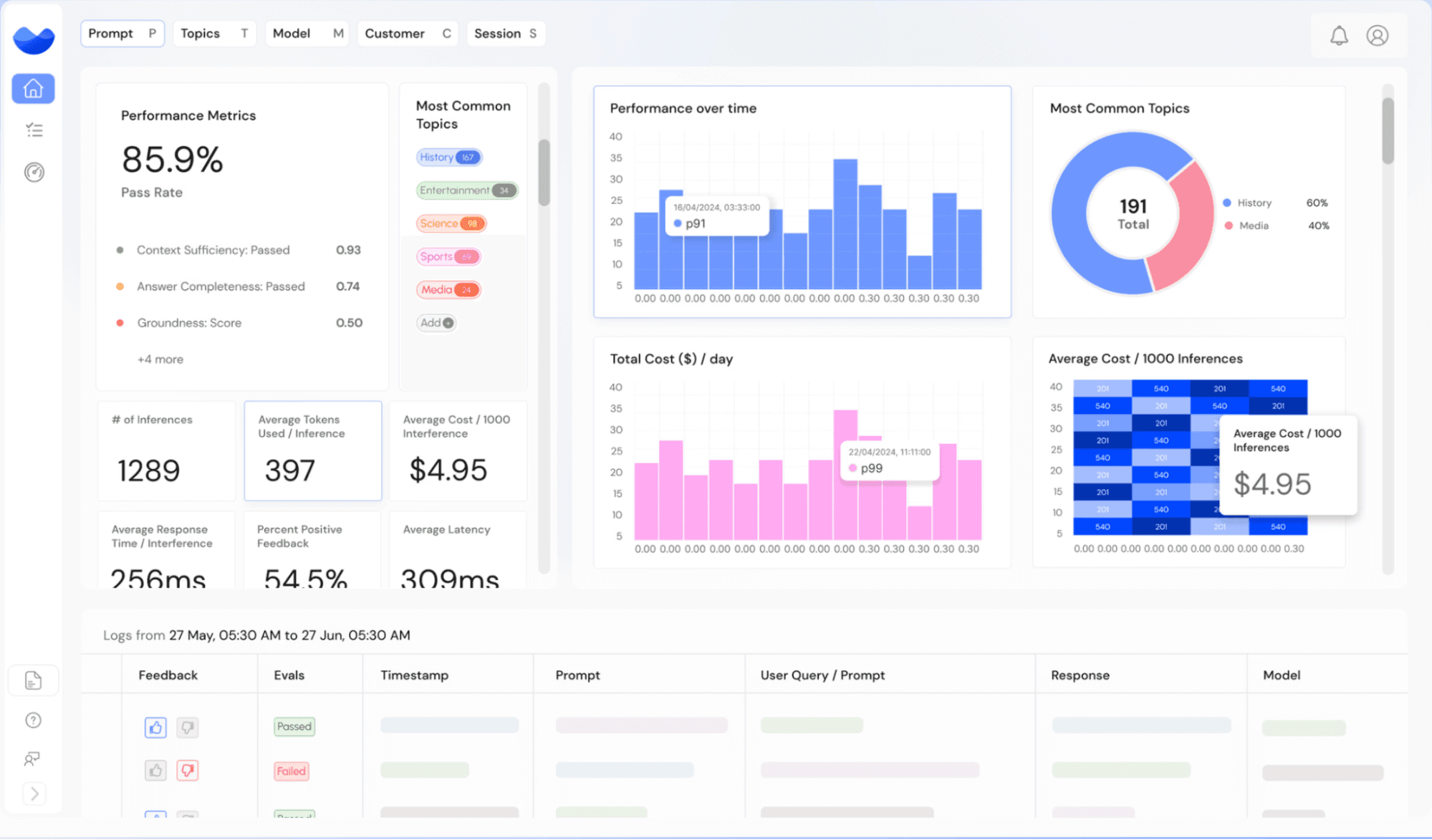
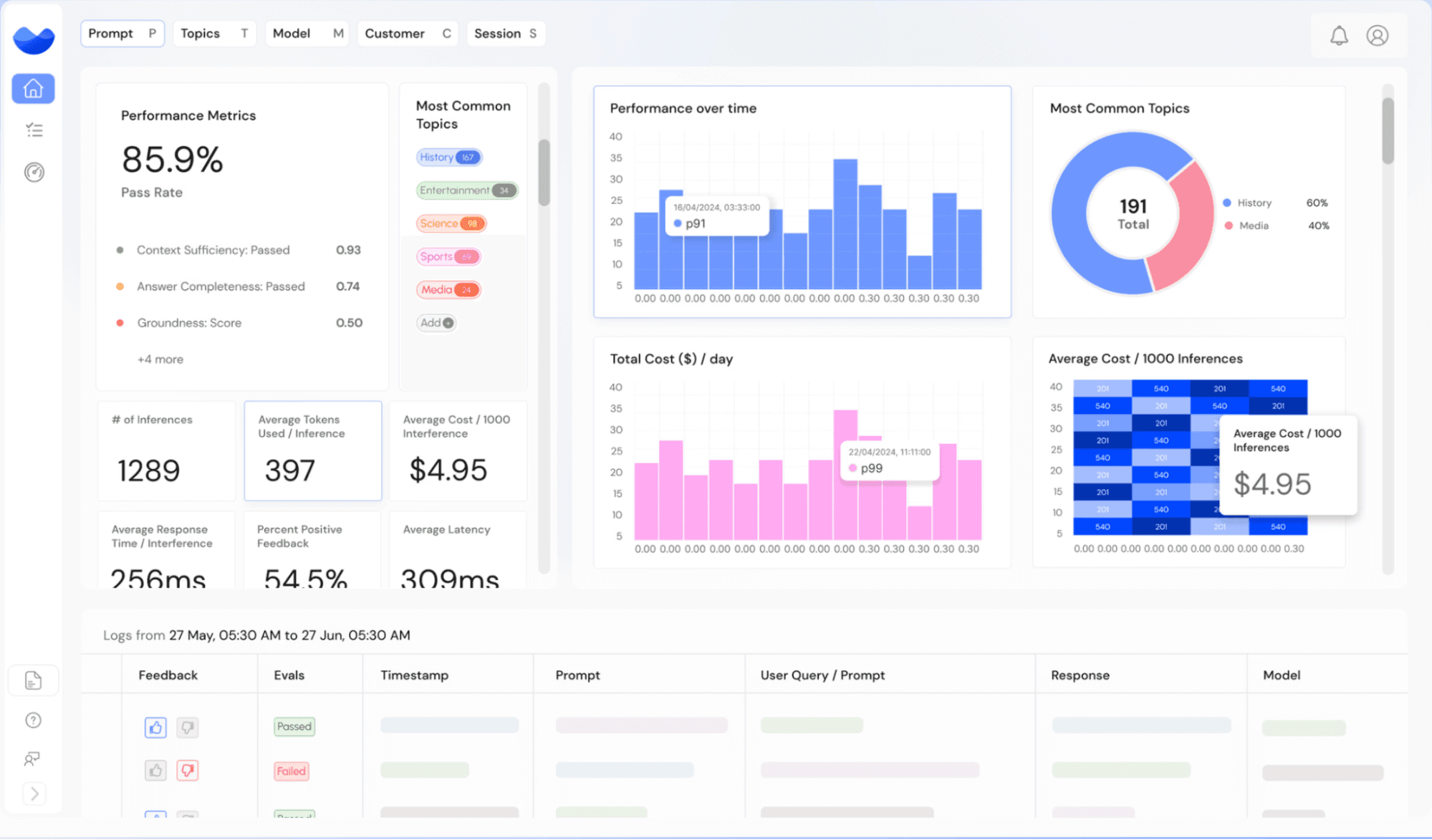
Using RagaAI Catalyst to Evaluate LLM Applications

Gaurav Agarwal
Oct 4, 2024
Read the article




Step-by-Step Guide on Training Large Language Models

Rehan Asif
Oct 1, 2024
Read the article
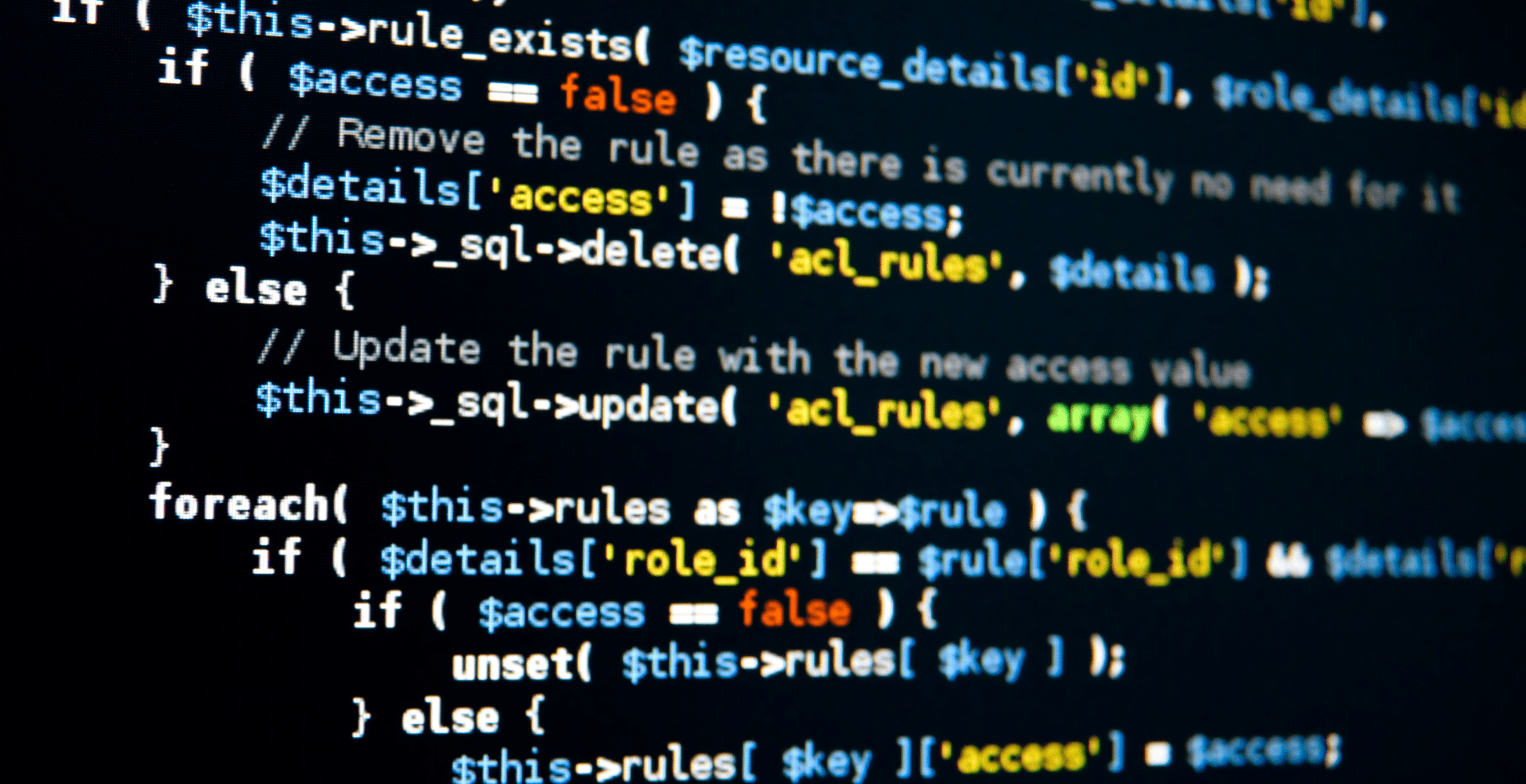
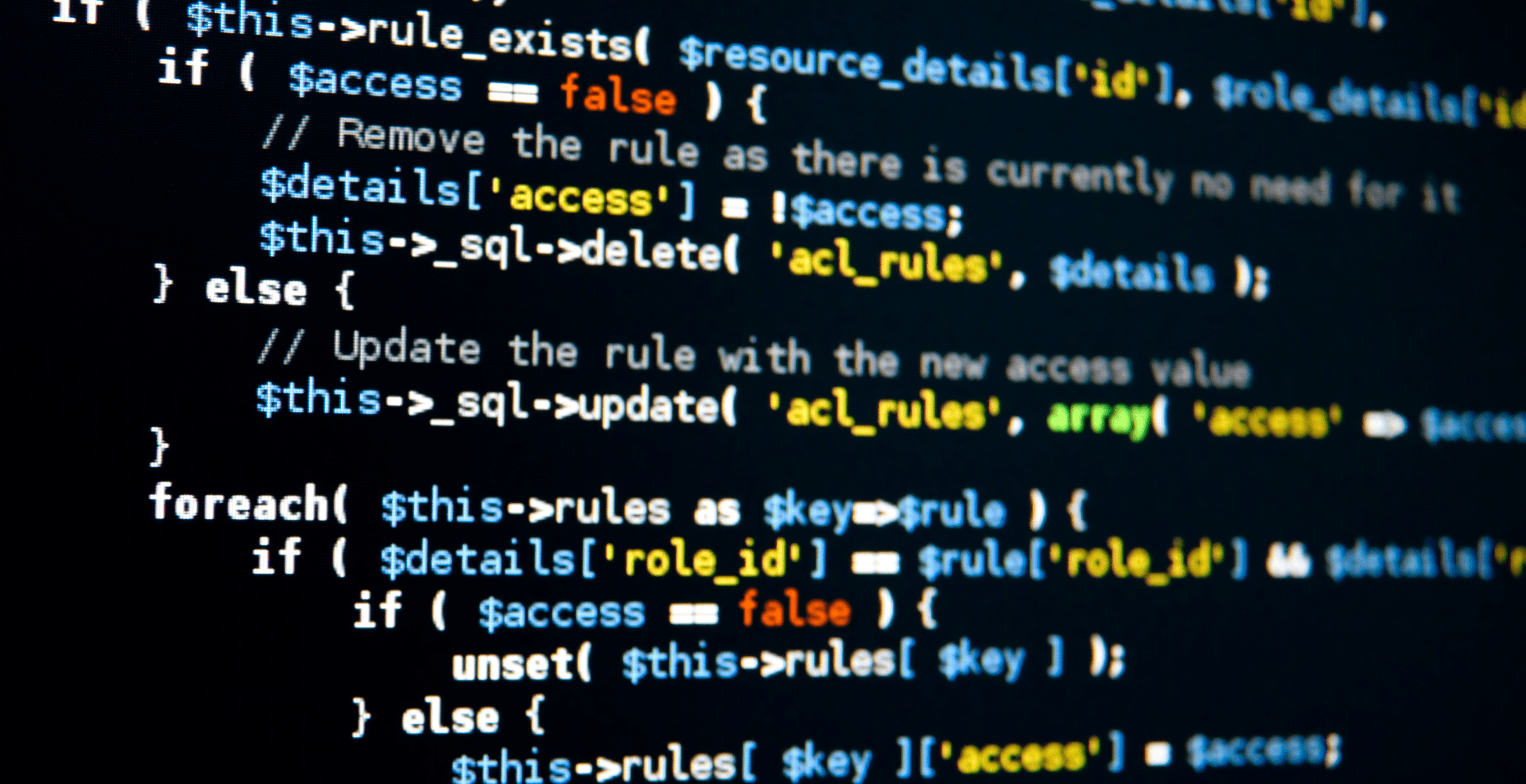
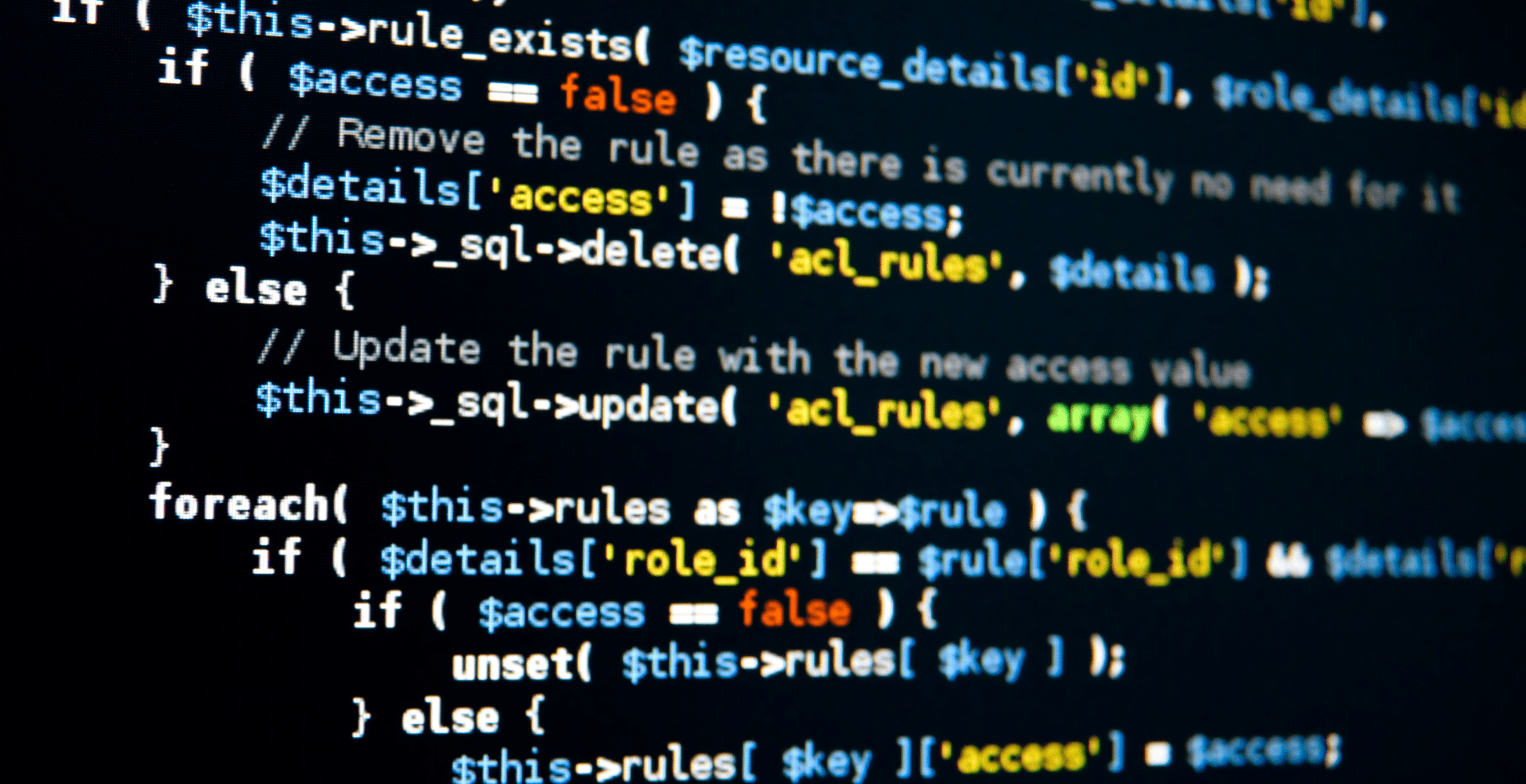
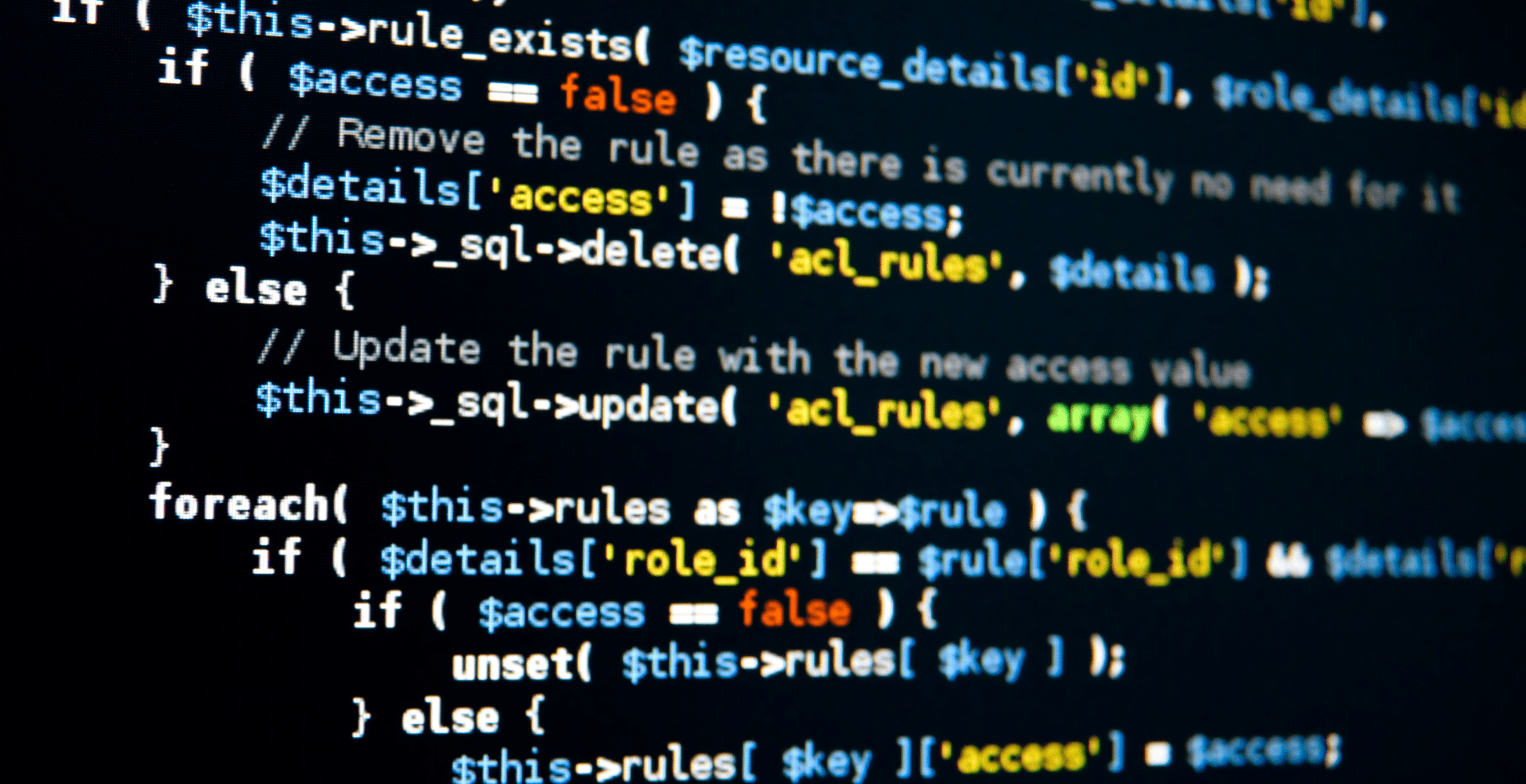
Understanding LLM Agent Architecture

Rehan Asif
Aug 19, 2024
Read the article




Understanding the Need and Possibilities of AI Guardrails Today
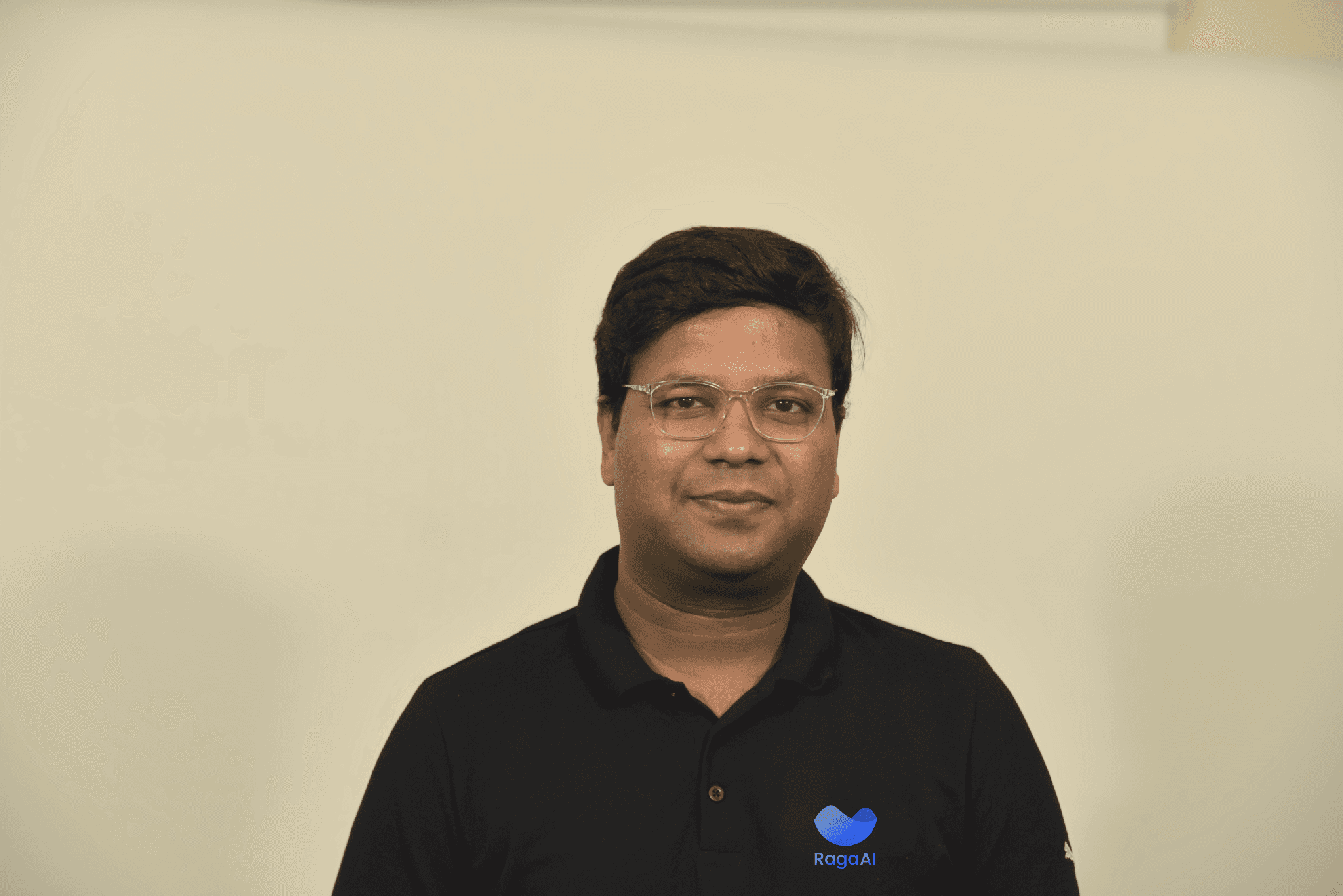
Jigar Gupta
Aug 19, 2024
Read the article




How to Prepare Quality Dataset for LLM Training

Rehan Asif
Aug 14, 2024
Read the article




Understanding Multi-Agent LLM Framework and Its Performance Scaling

Rehan Asif
Aug 15, 2024
Read the article
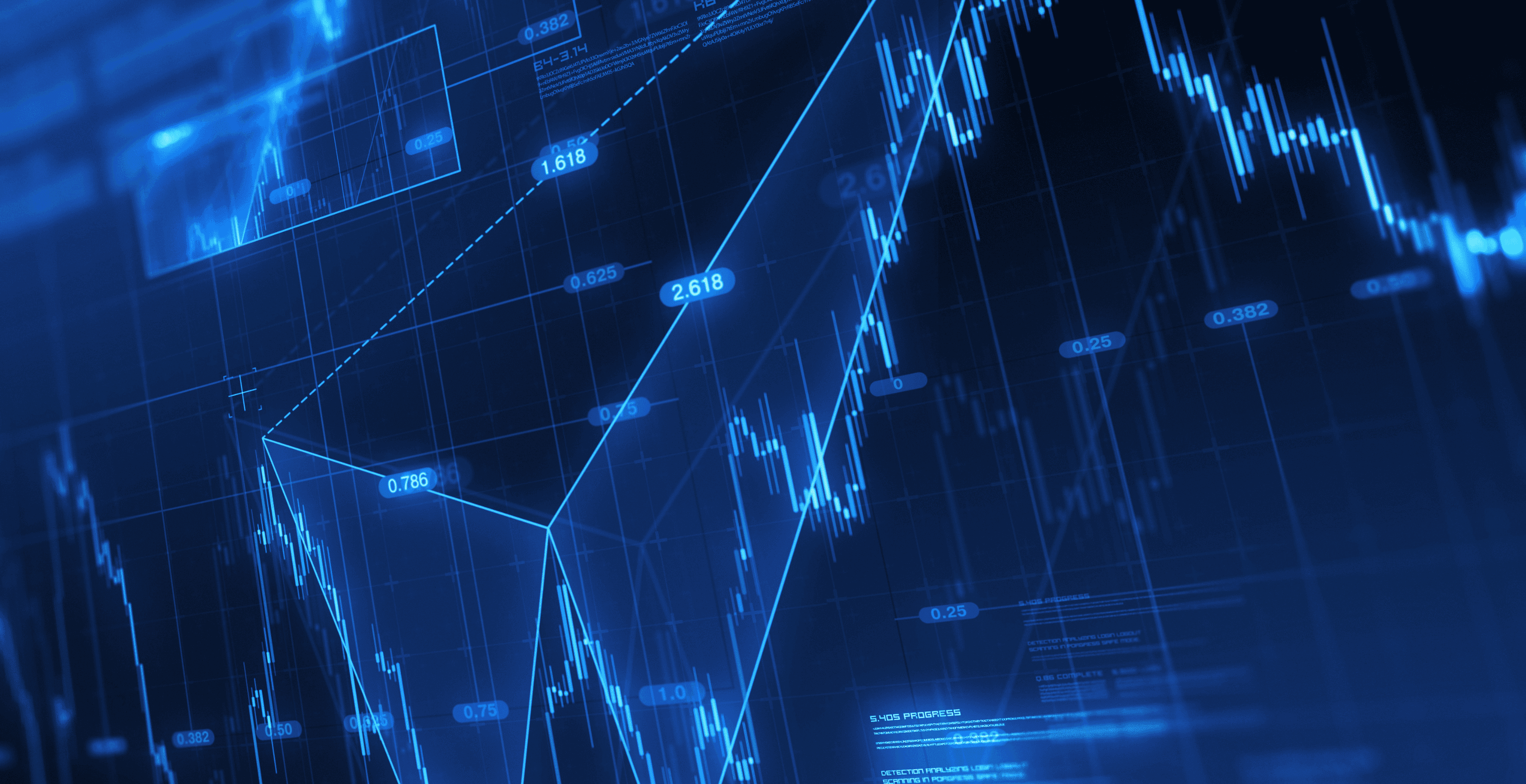
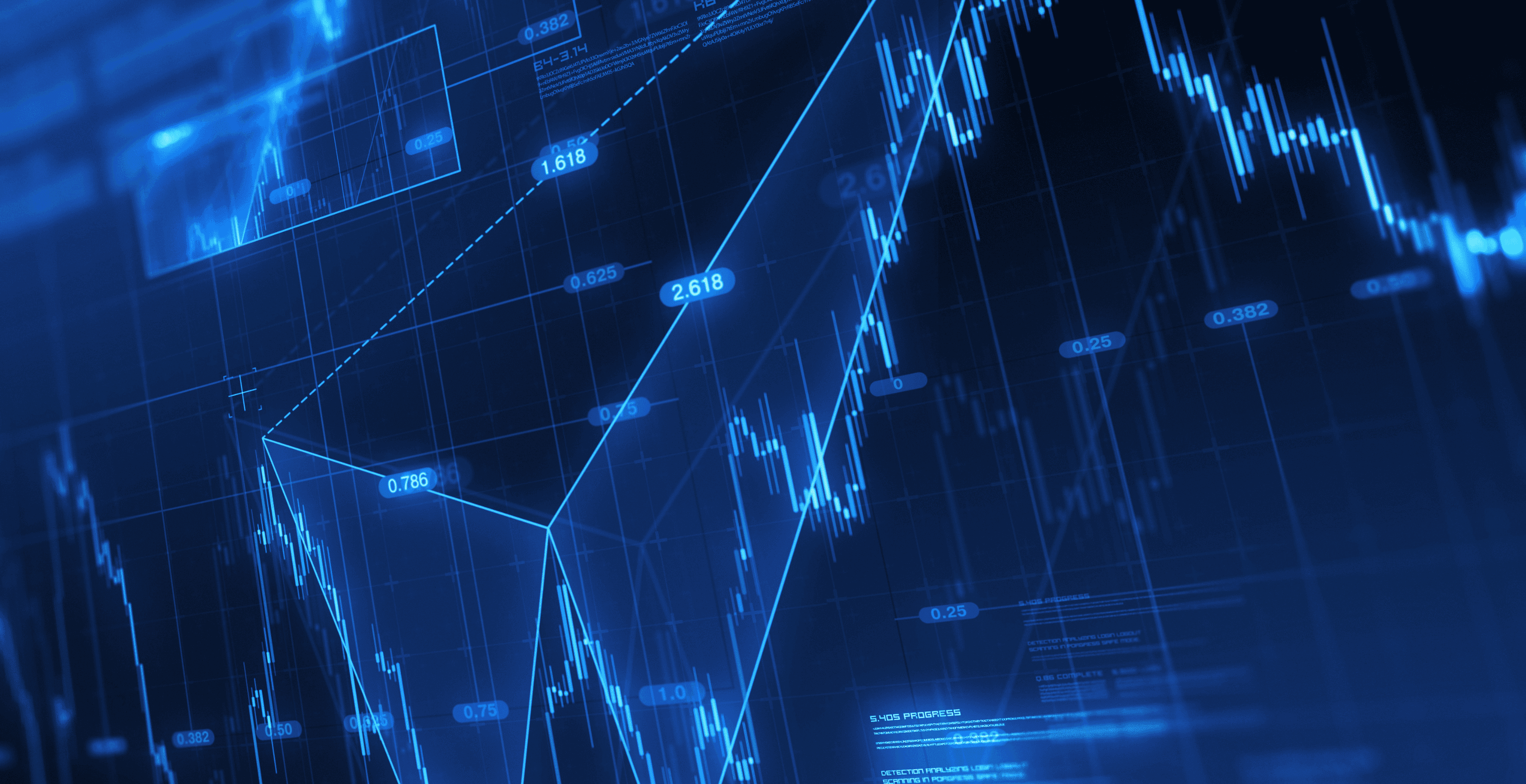
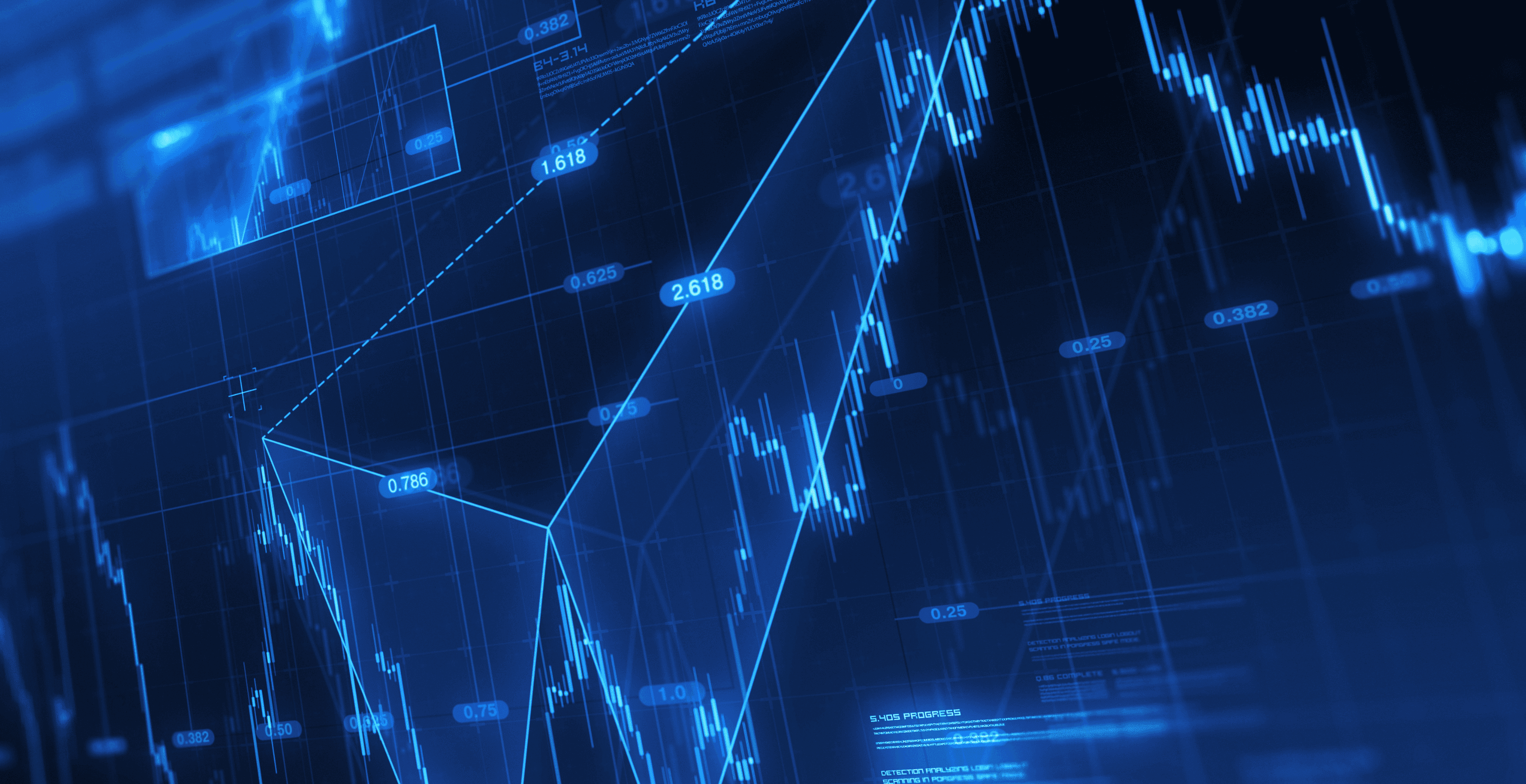
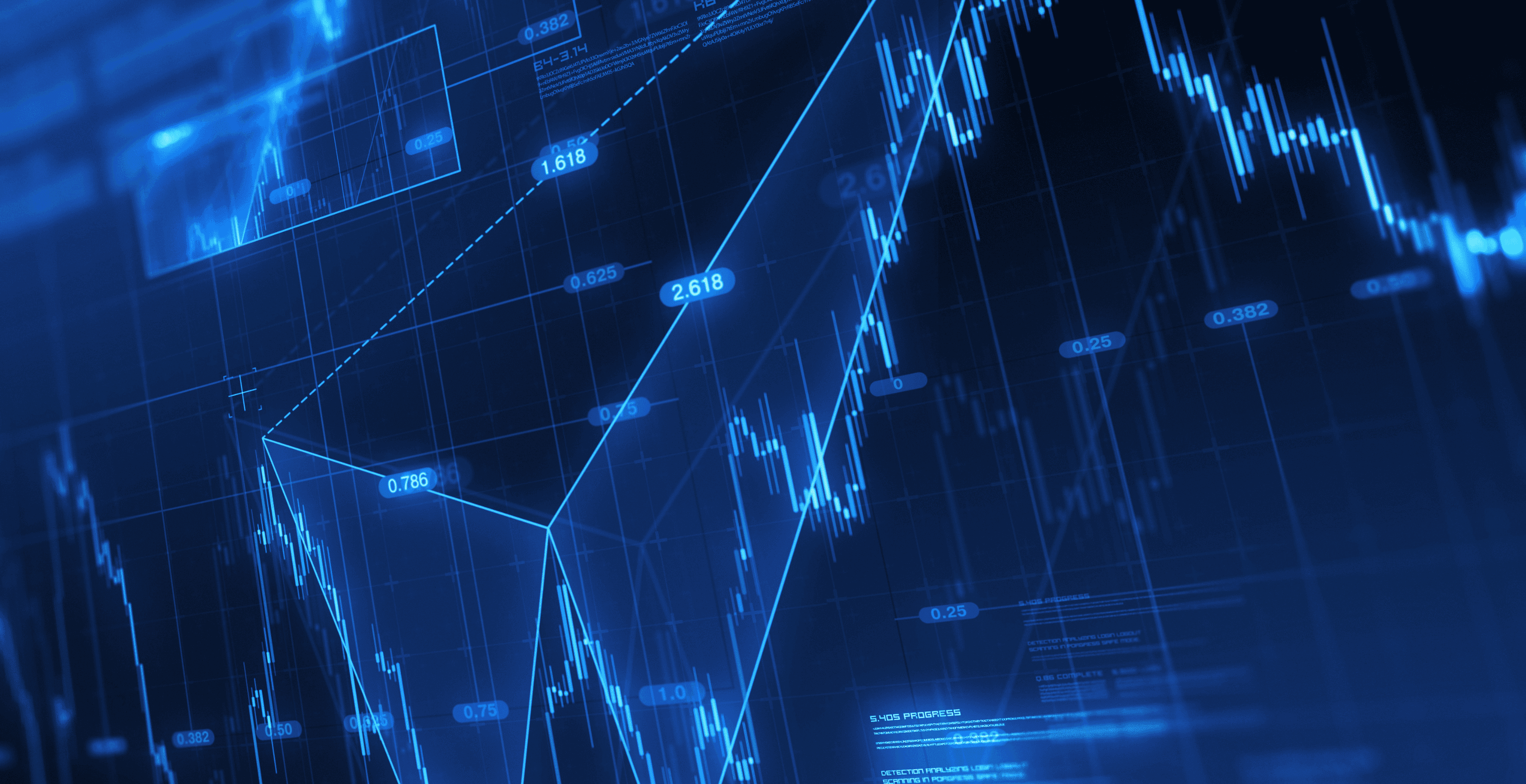
Understanding and Tackling Data Drift: Causes, Impact, and Automation Strategies
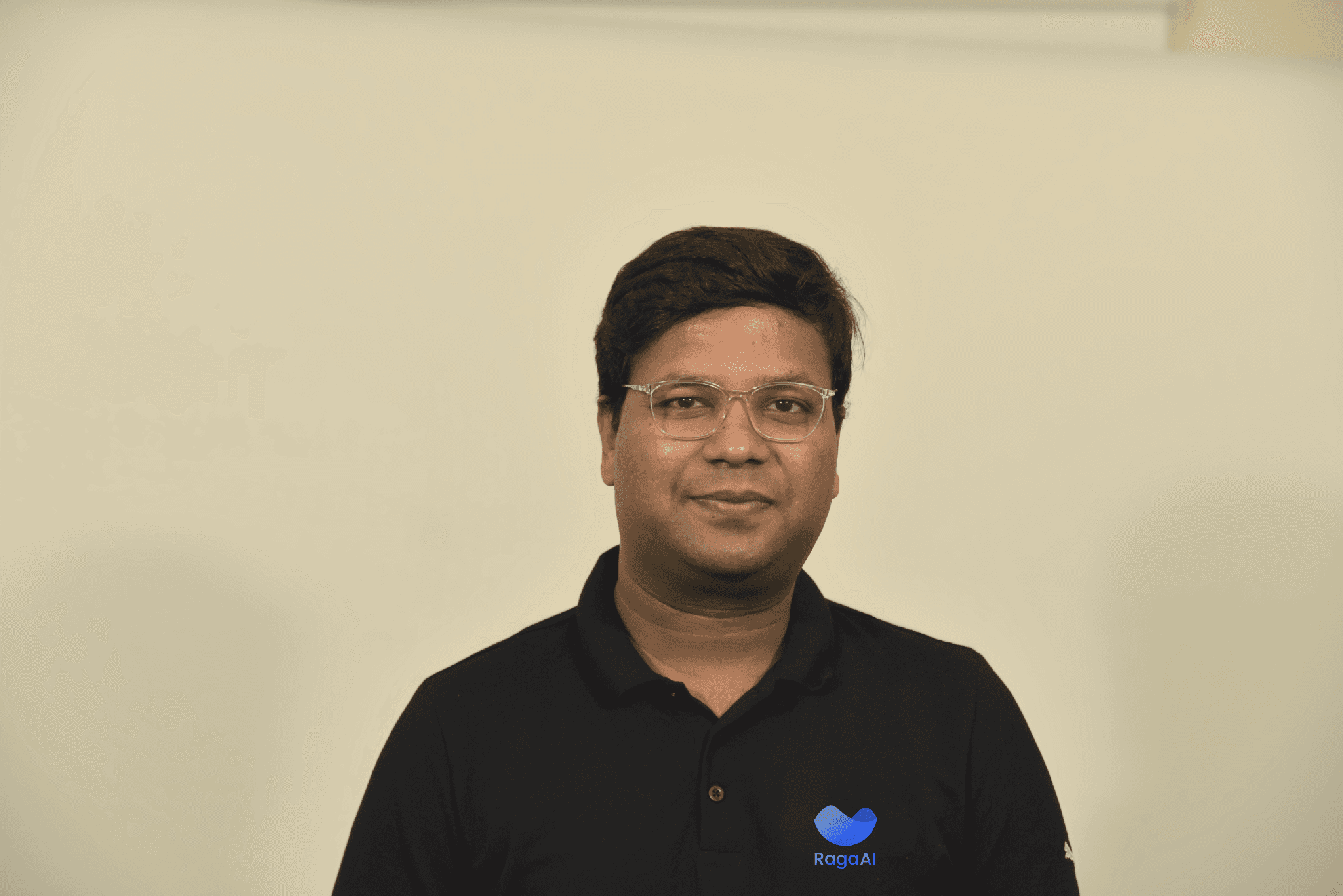
Jigar Gupta
Aug 14, 2024
Read the article




Introducing RagaAI Catalyst: Best in class automated LLM evaluation with 93% Human Alignment
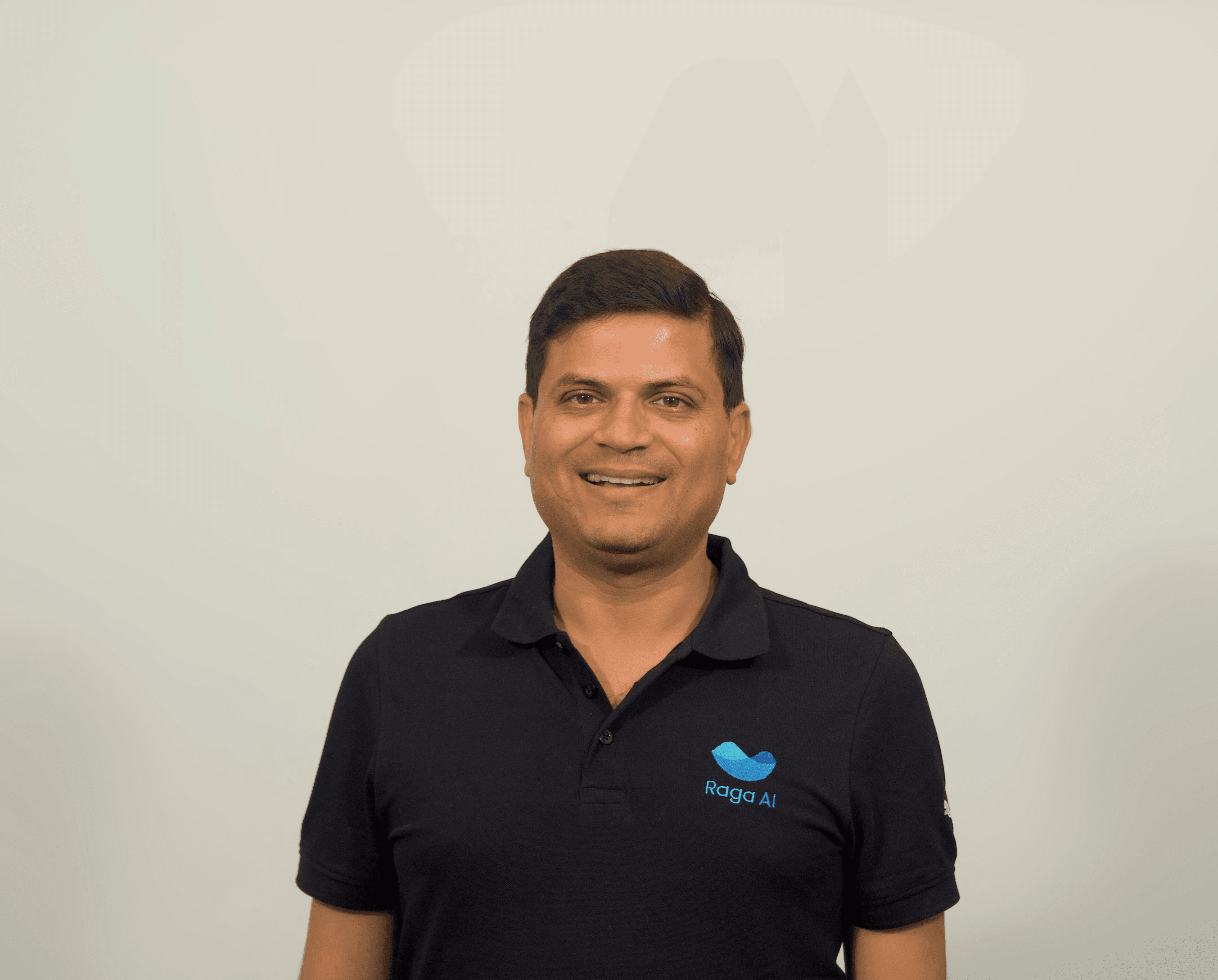
Gaurav Agarwal
Jul 15, 2024
Read the article




Key Pillars and Techniques for LLM Observability and Monitoring

Rehan Asif
Jul 24, 2024
Read the article
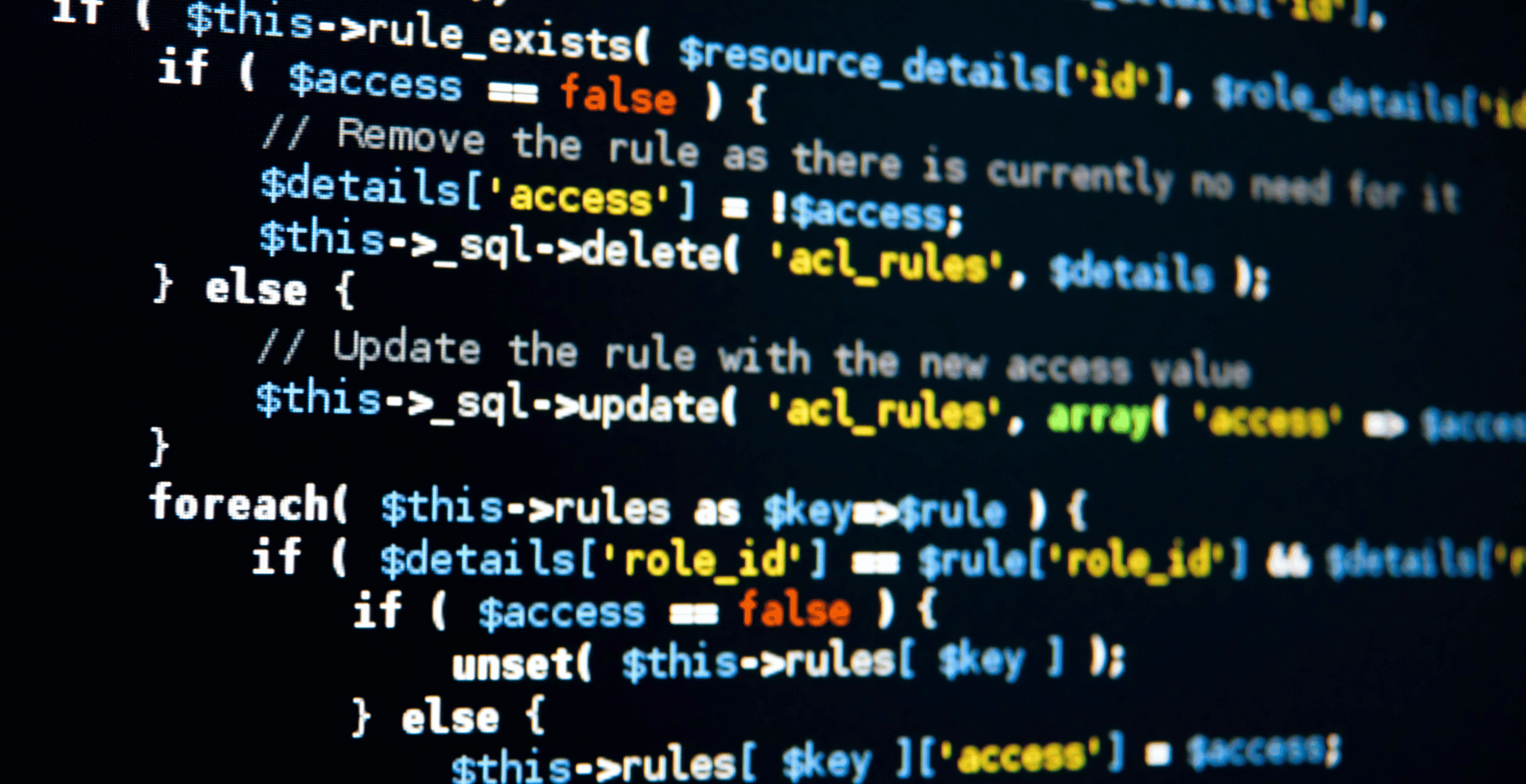
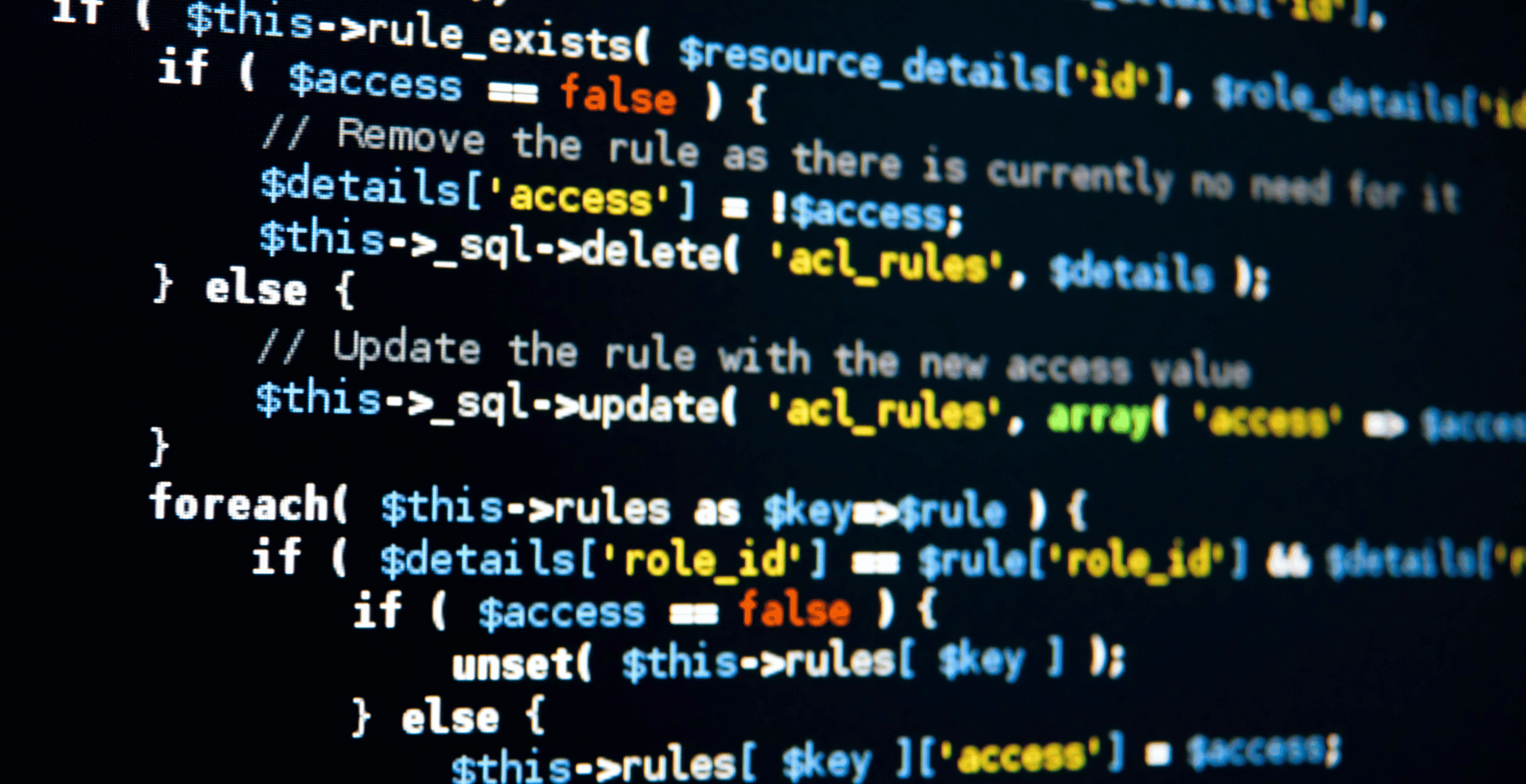
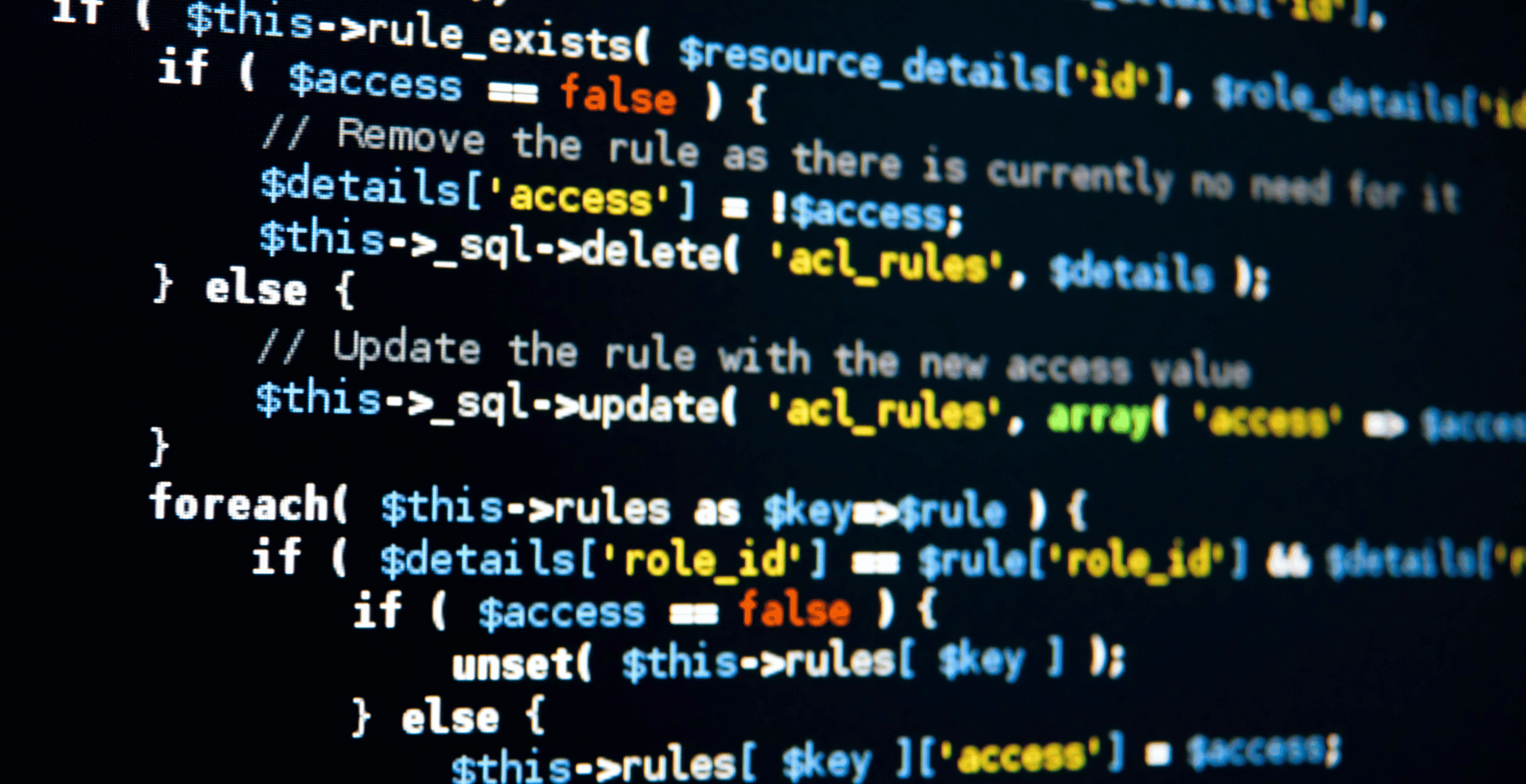
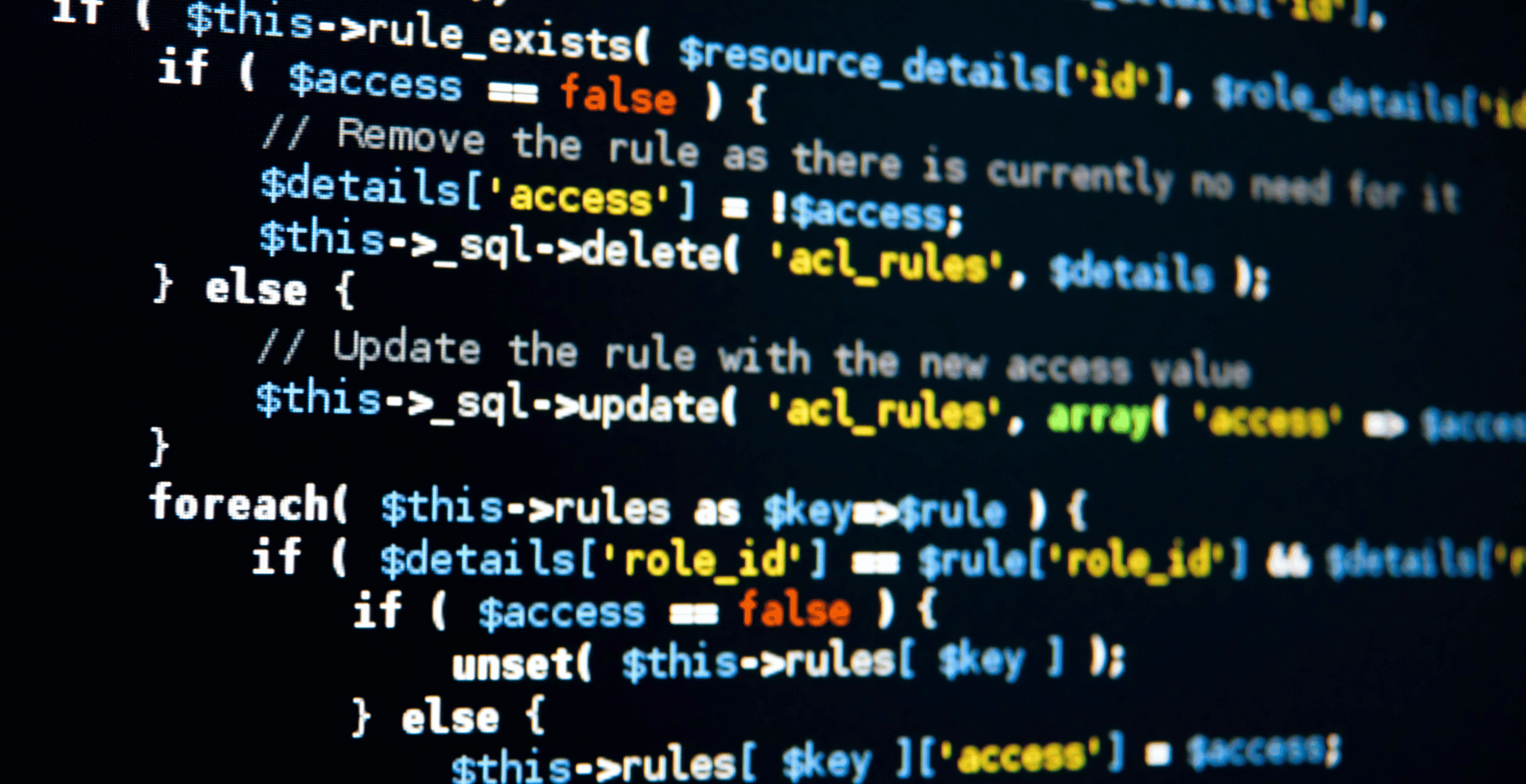
Introduction to What is LLM Agents and How They Work?

Rehan Asif
Jul 24, 2024
Read the article




Analysis of the Large Language Model Landscape Evolution

Rehan Asif
Jul 24, 2024
Read the article




Marketing Success With Retrieval Augmented Generation (RAG) Platforms
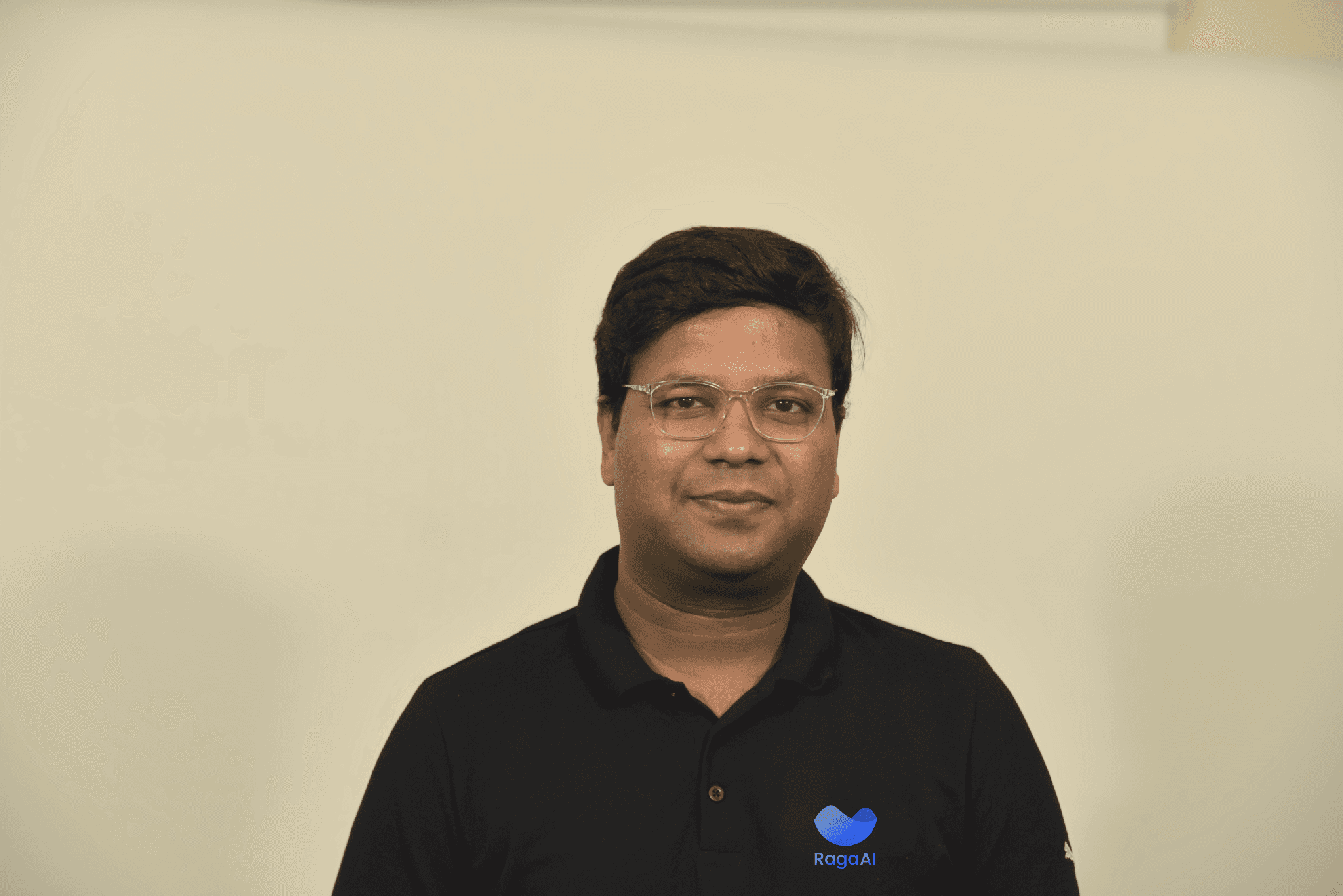
Jigar Gupta
Jul 24, 2024
Read the article




Developing AI Agent Strategies Using GPT
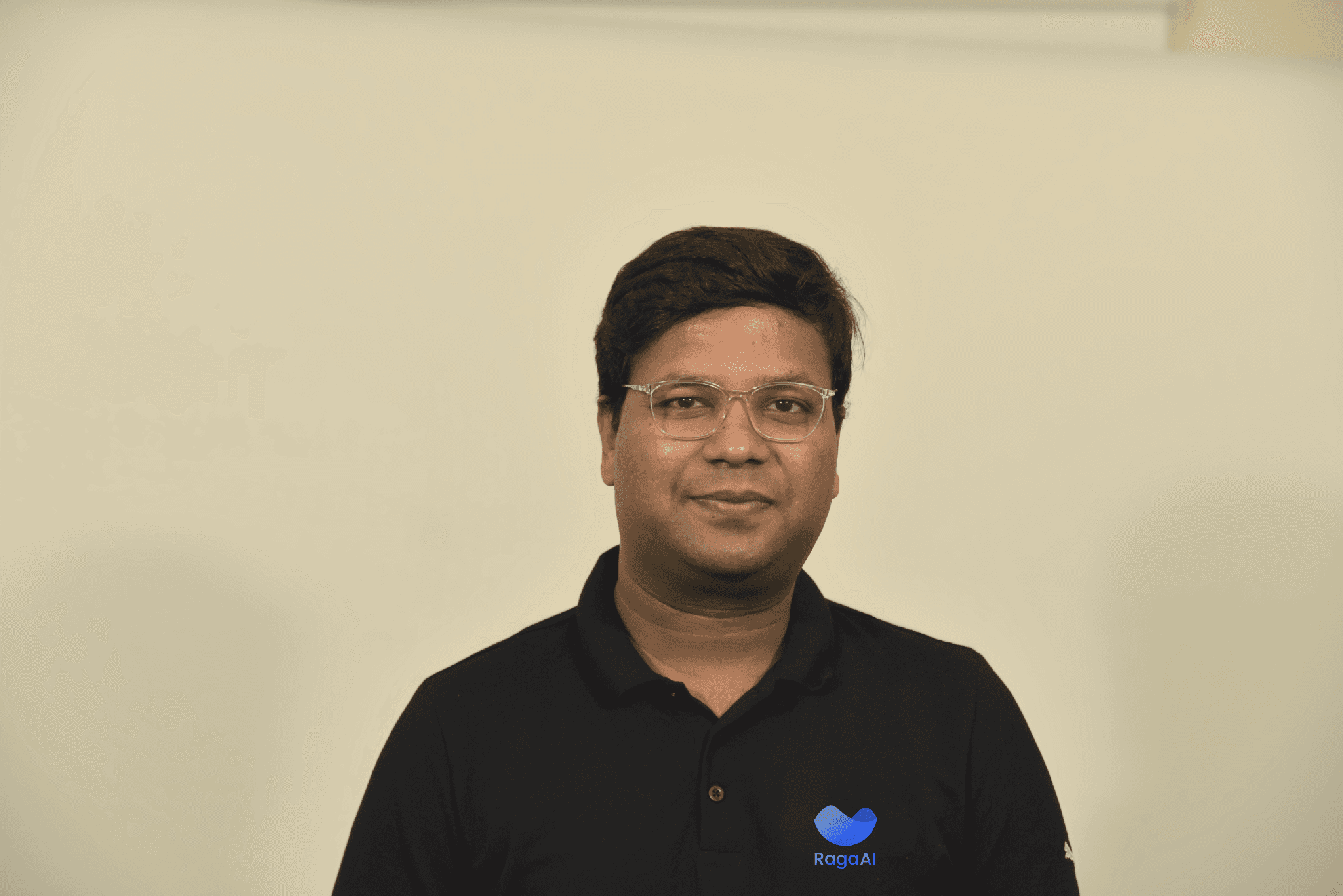
Jigar Gupta
Jul 24, 2024
Read the article




Identifying Triggers for Retraining AI Models to Maintain Performance
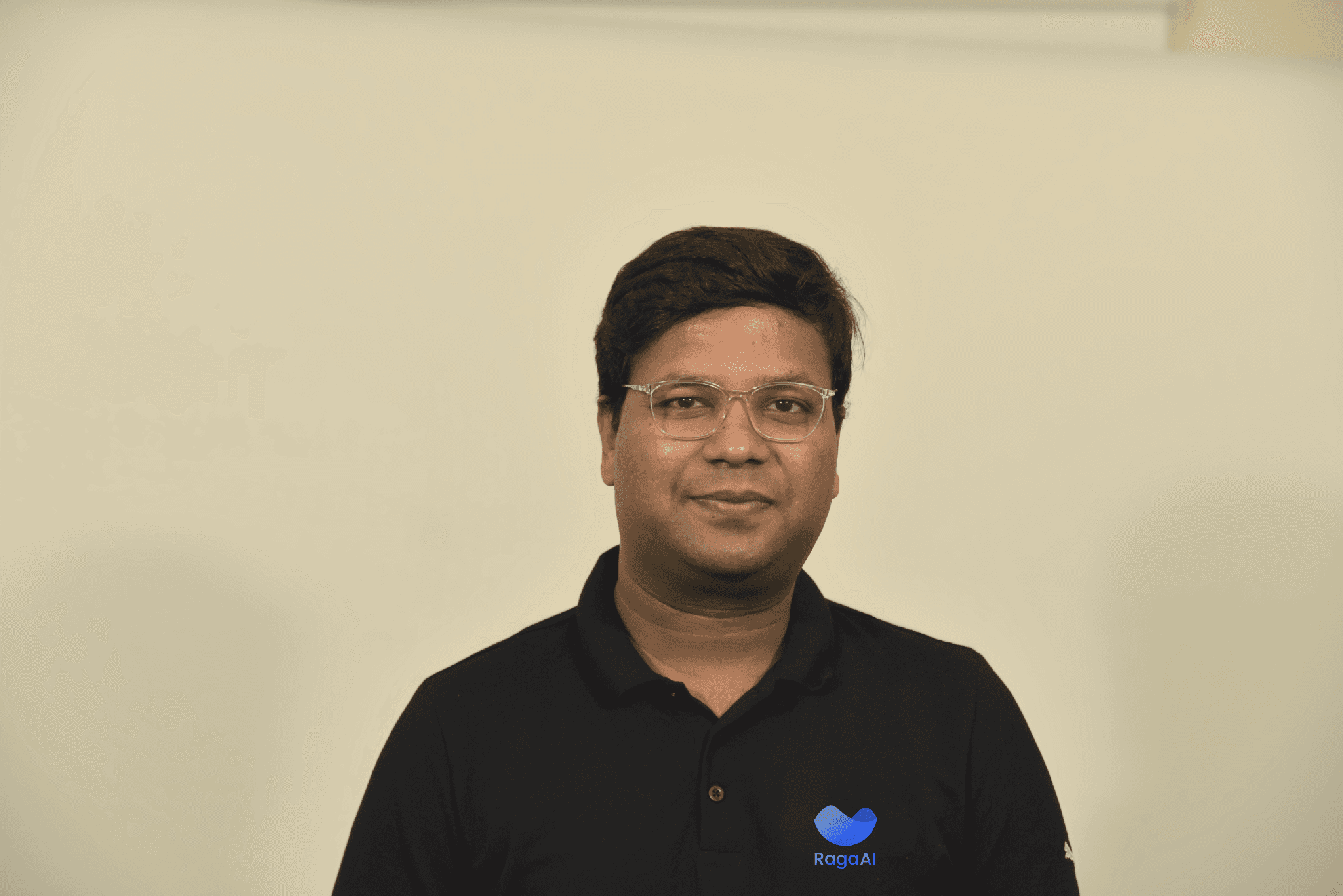
Jigar Gupta
Jul 16, 2024
Read the article
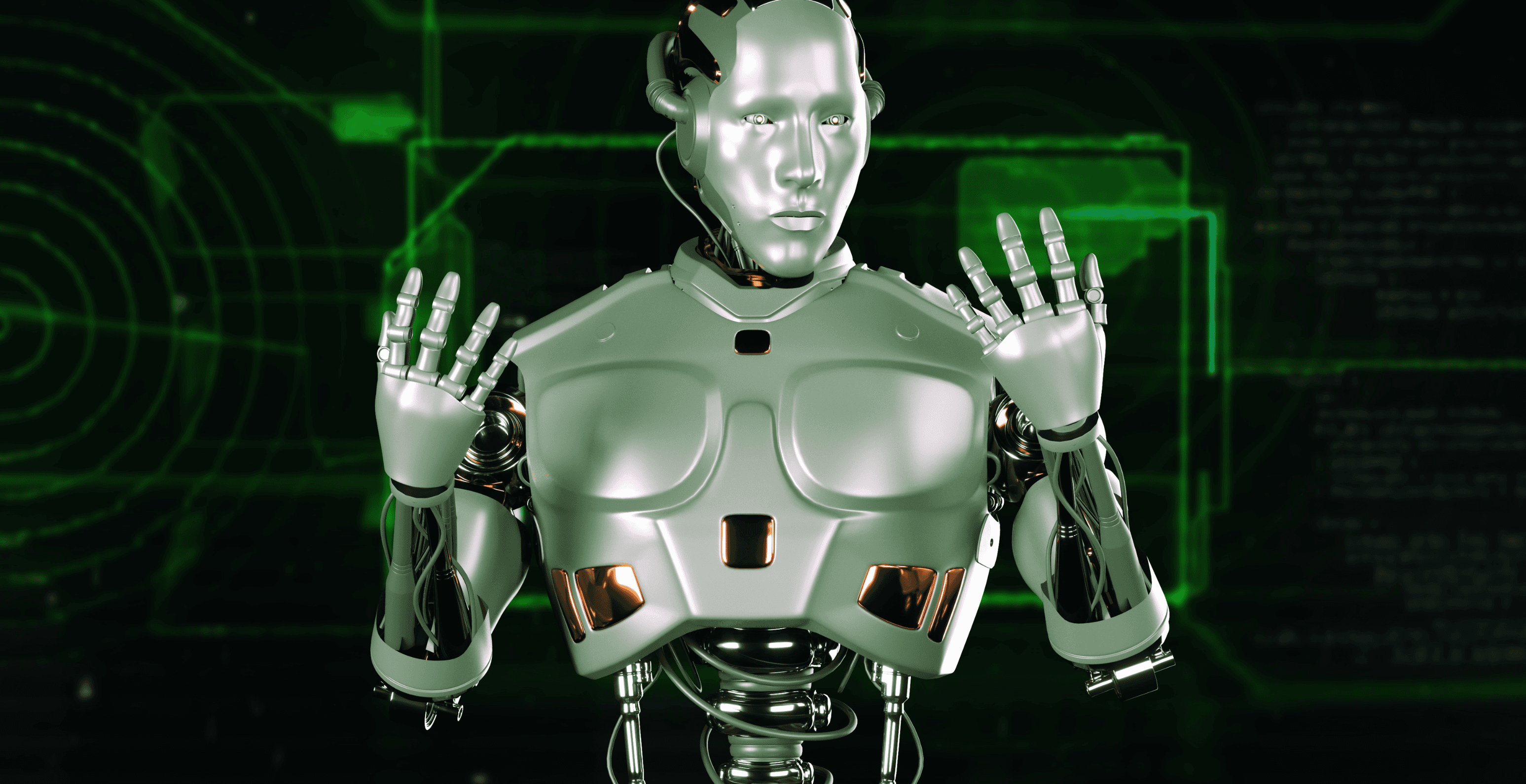
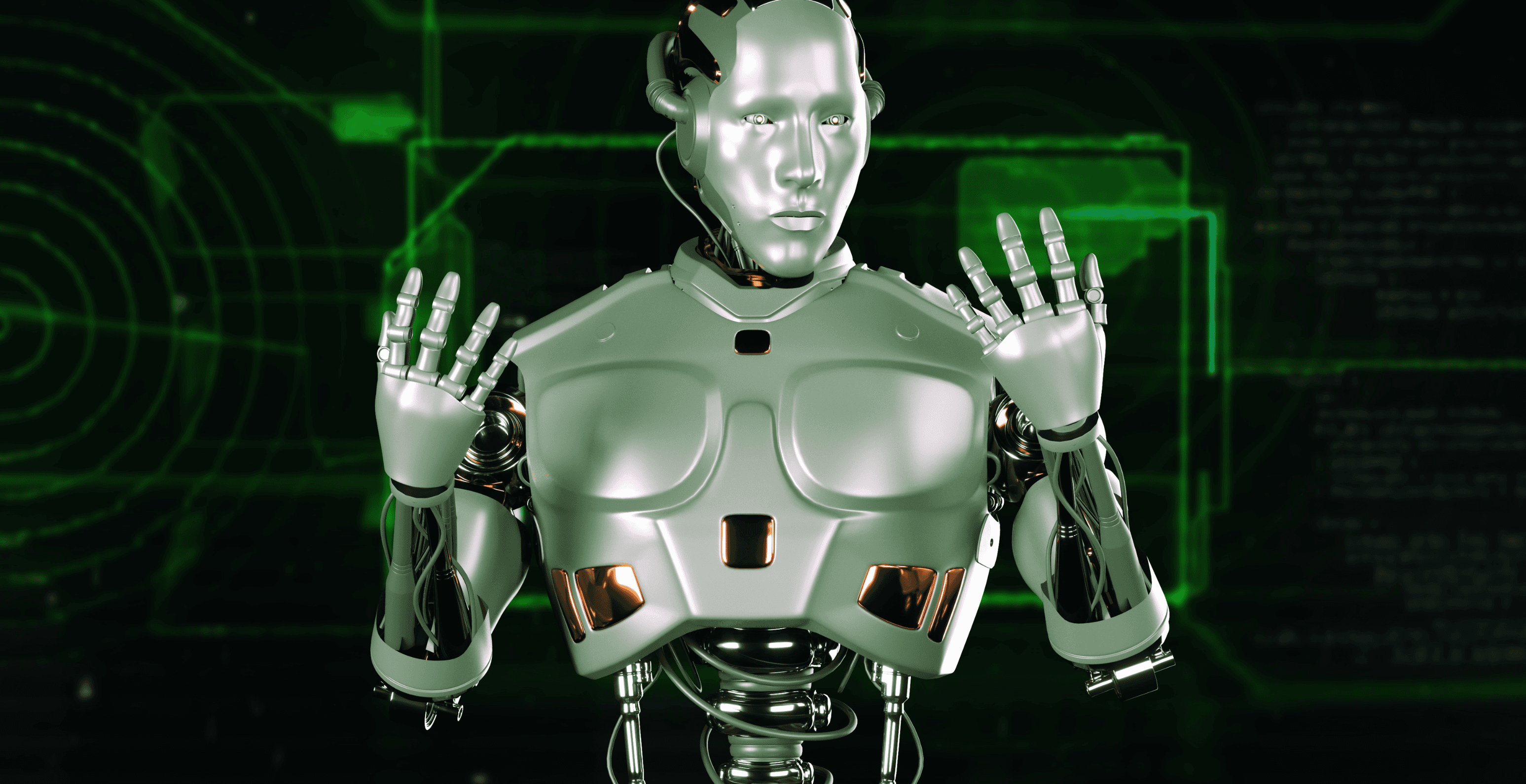
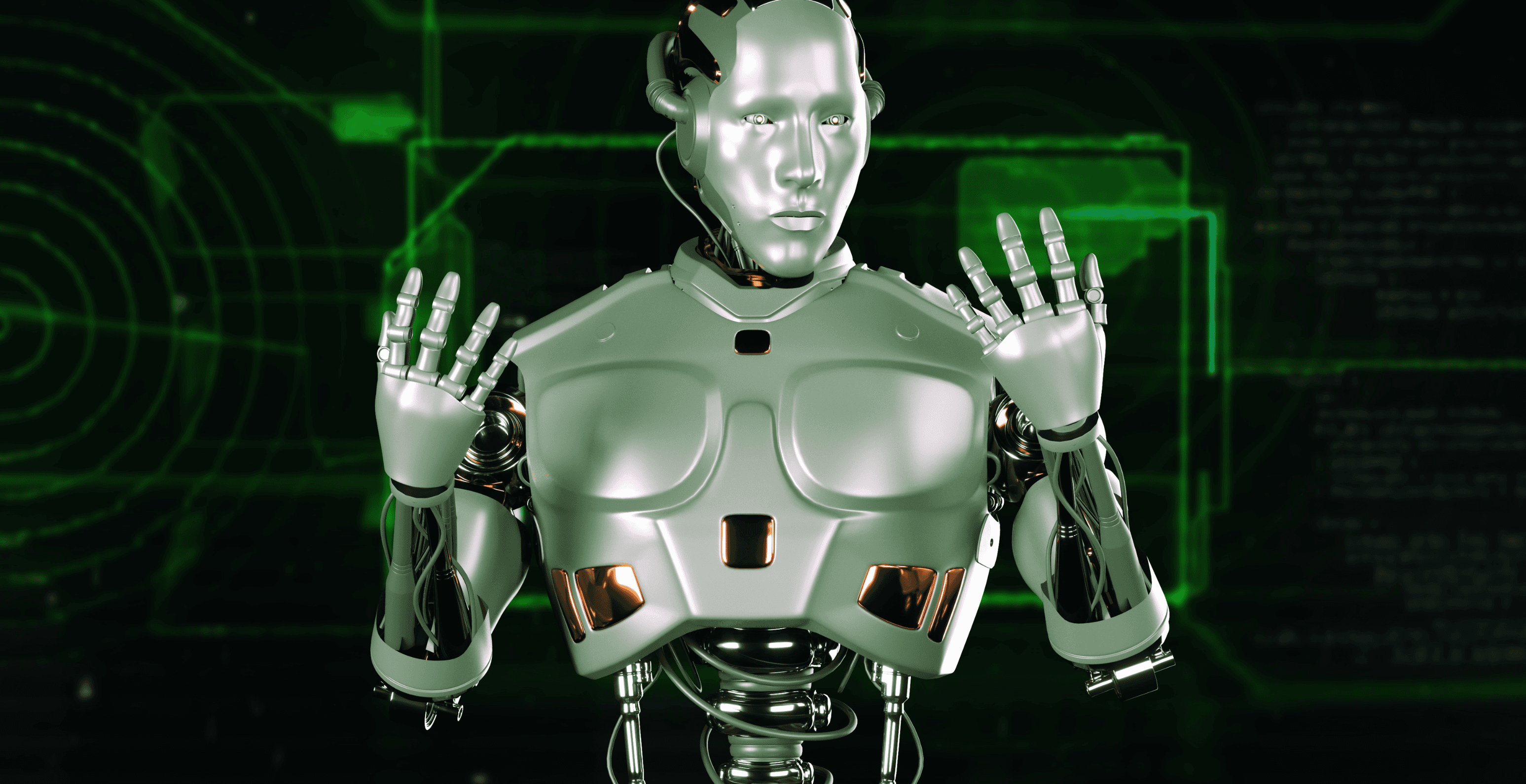
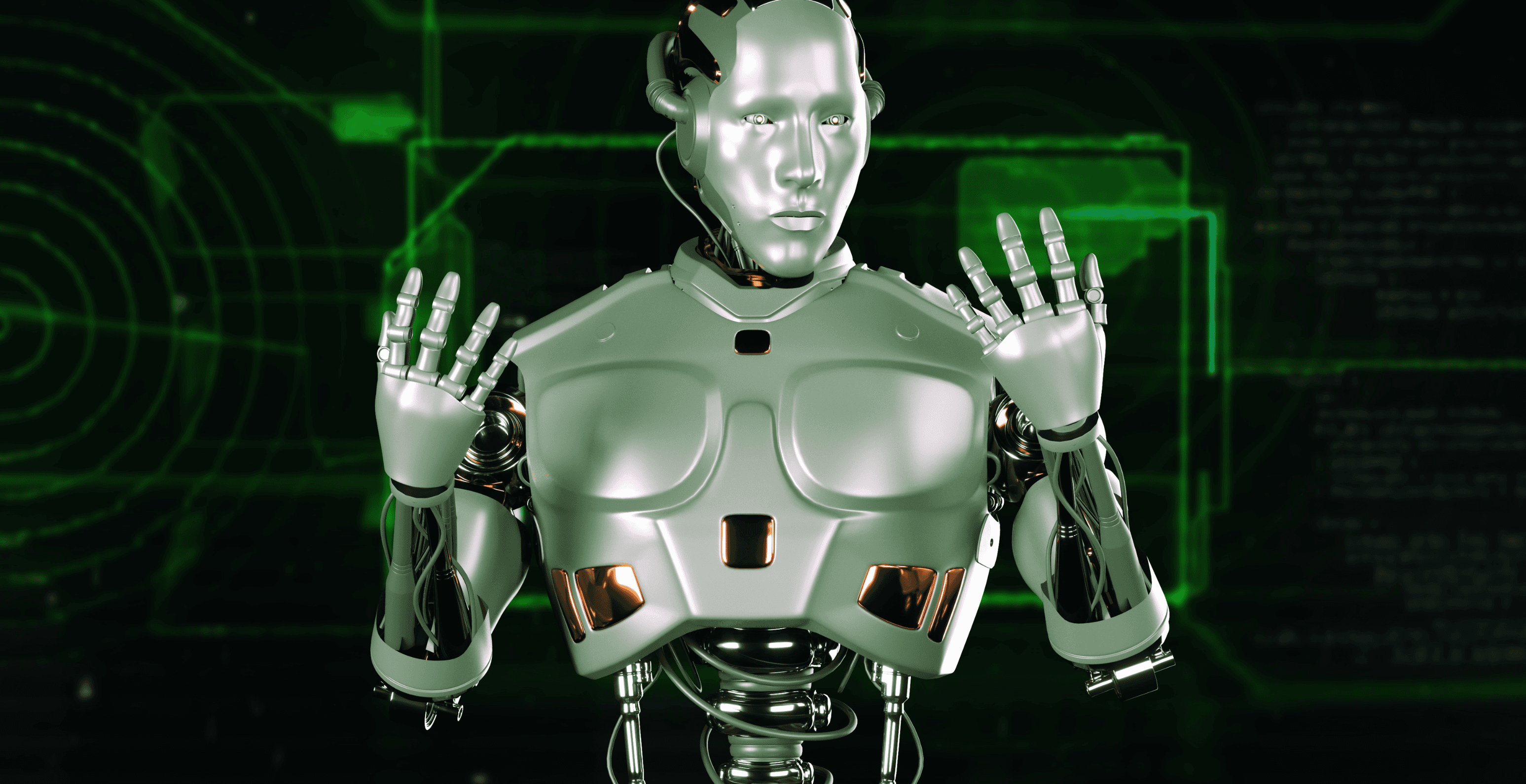
Agentic Design Patterns In LLM-Based Applications

Rehan Asif
Jul 16, 2024
Read the article
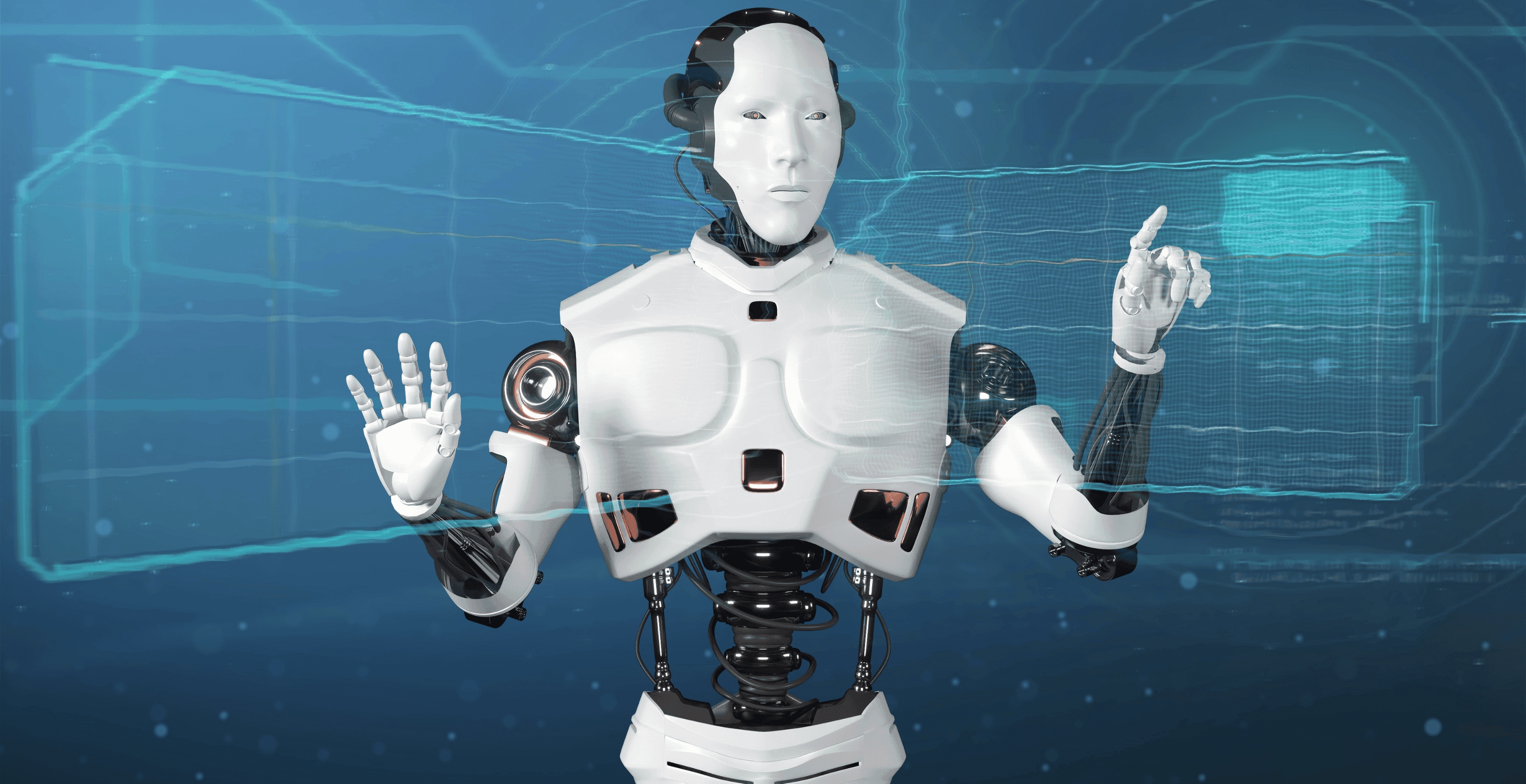
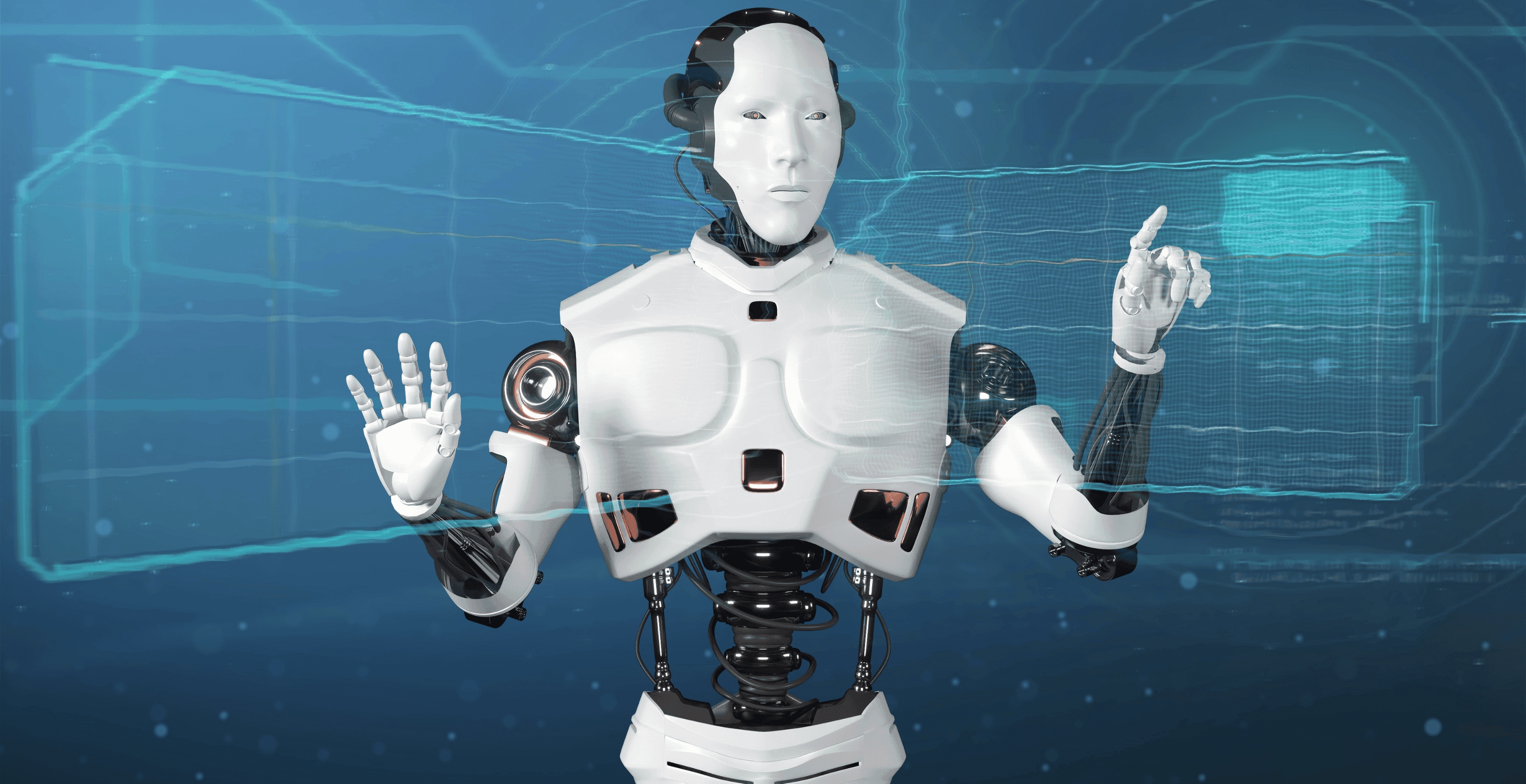
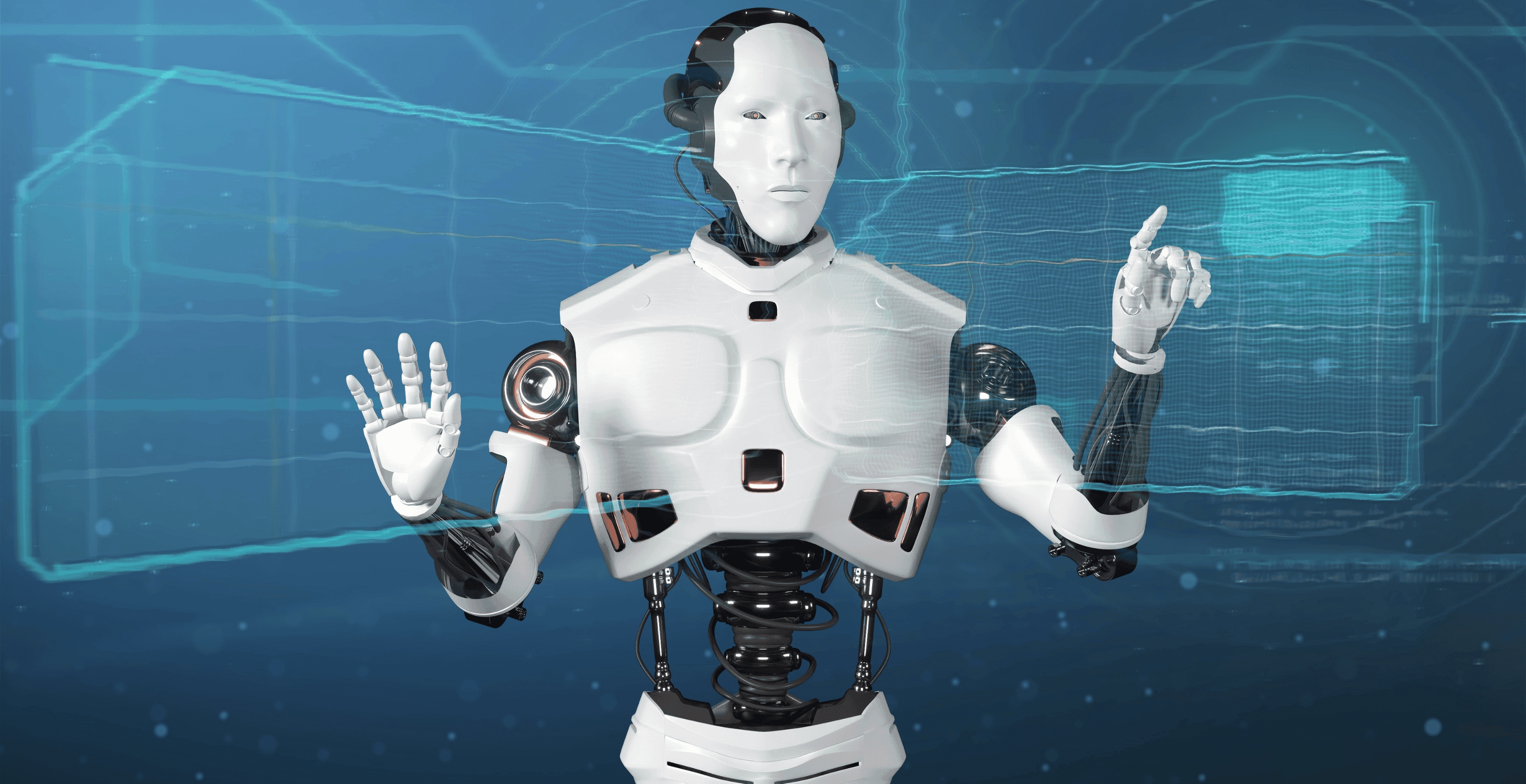
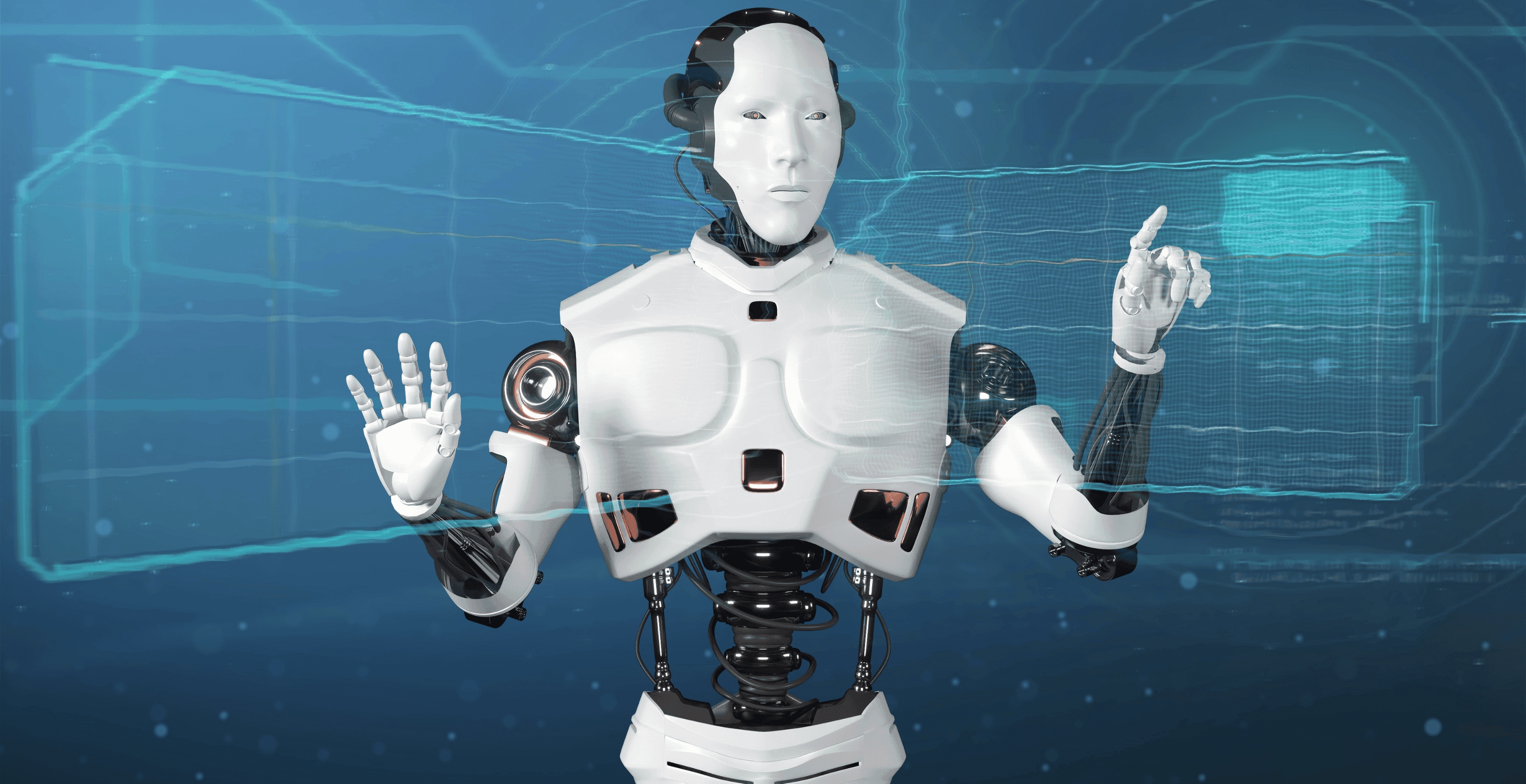
Generative AI And Document Question Answering With LLMs
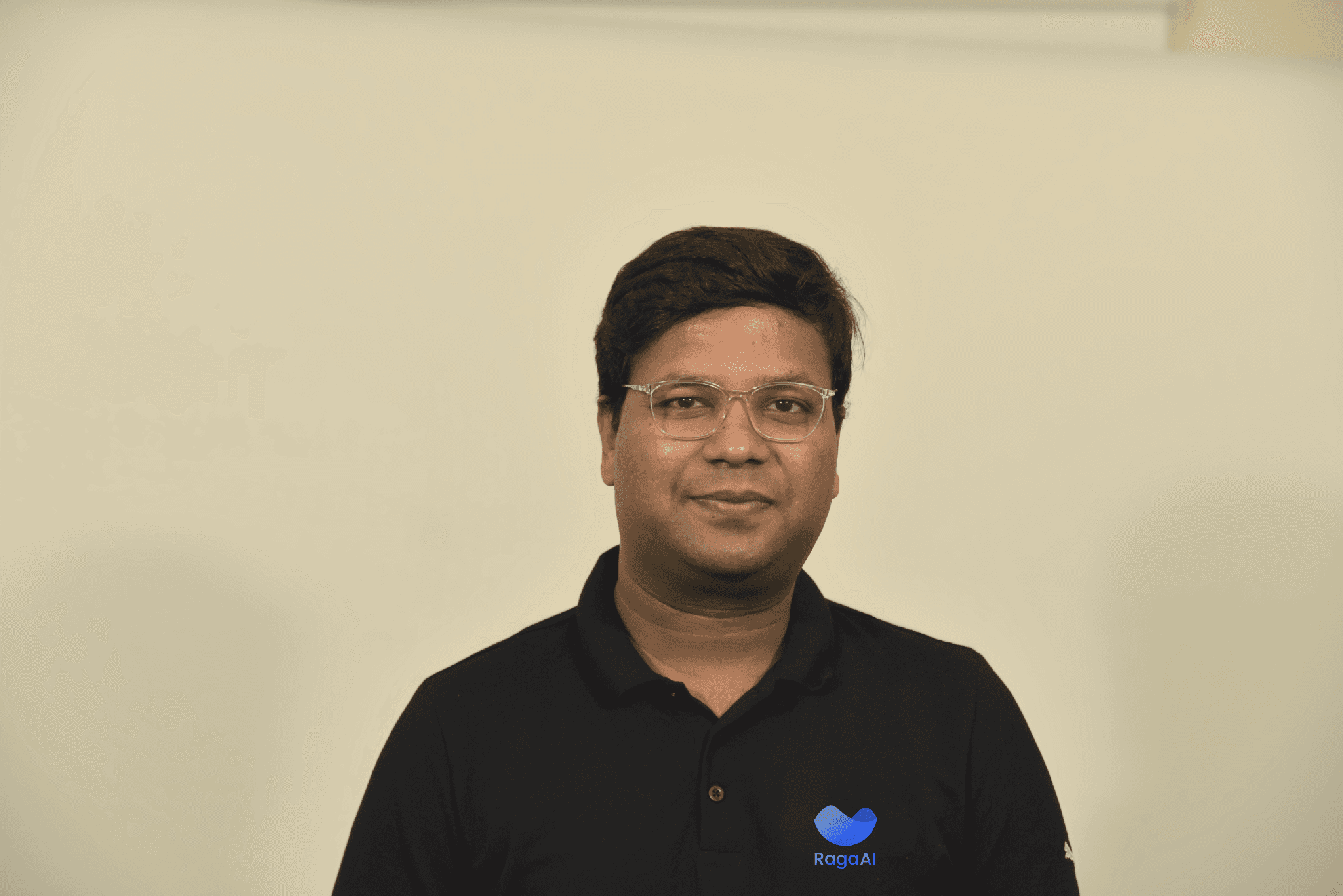
Jigar Gupta
Jul 15, 2024
Read the article




How to Fine-Tune ChatGPT for Your Use Case - Step by Step Guide
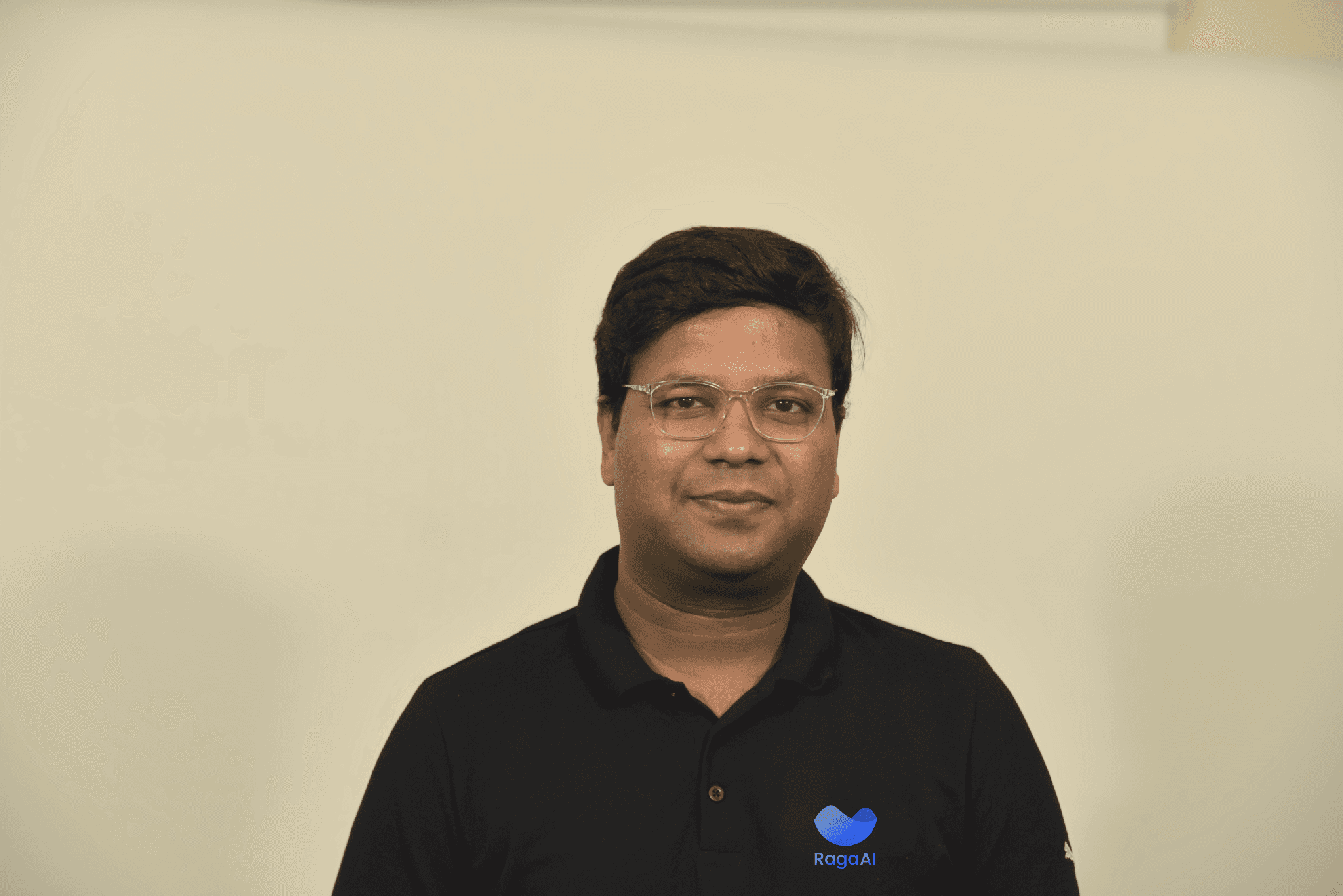
Jigar Gupta
Jul 15, 2024
Read the article




Security and LLM Firewall Controls

Rehan Asif
Jul 15, 2024
Read the article
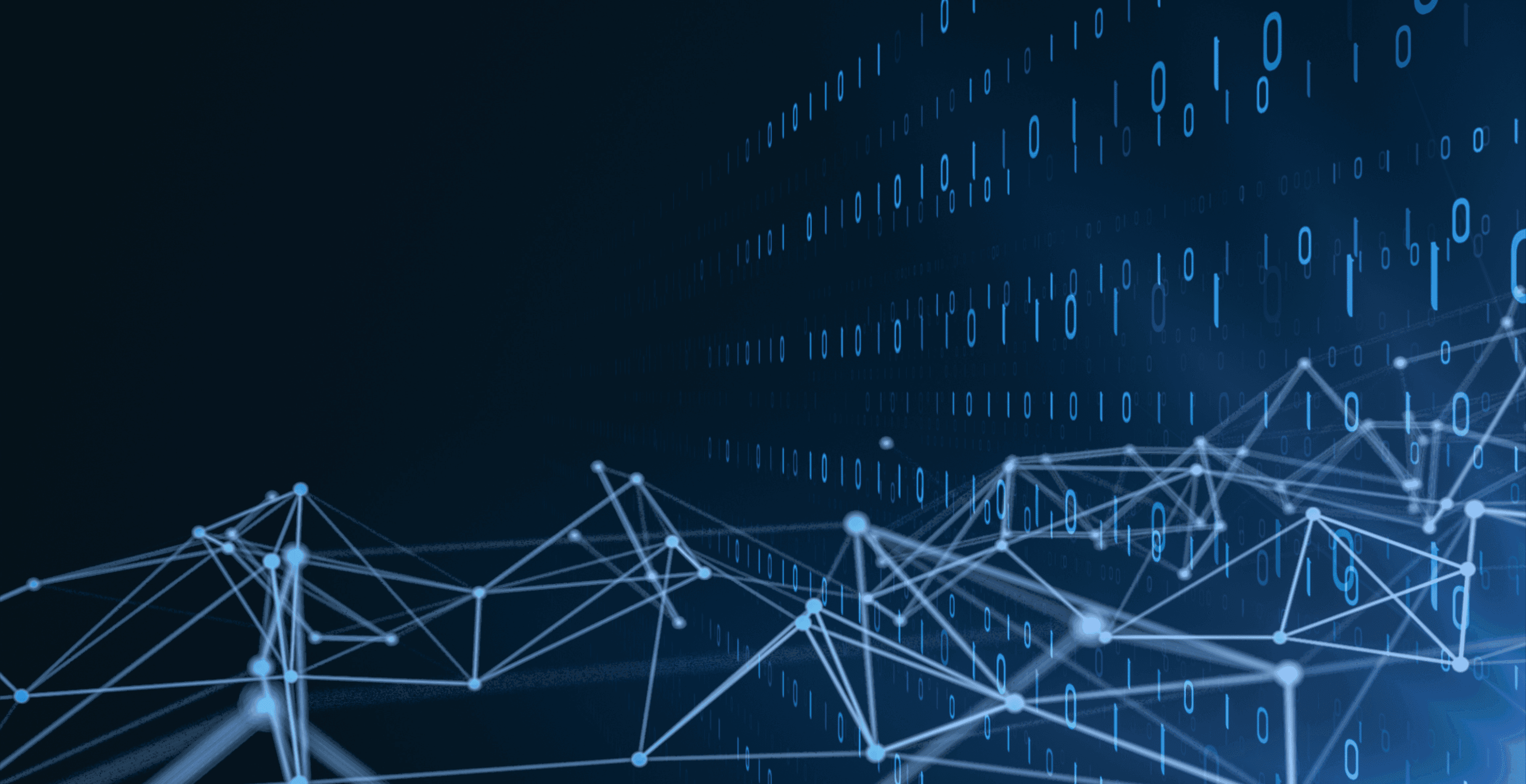
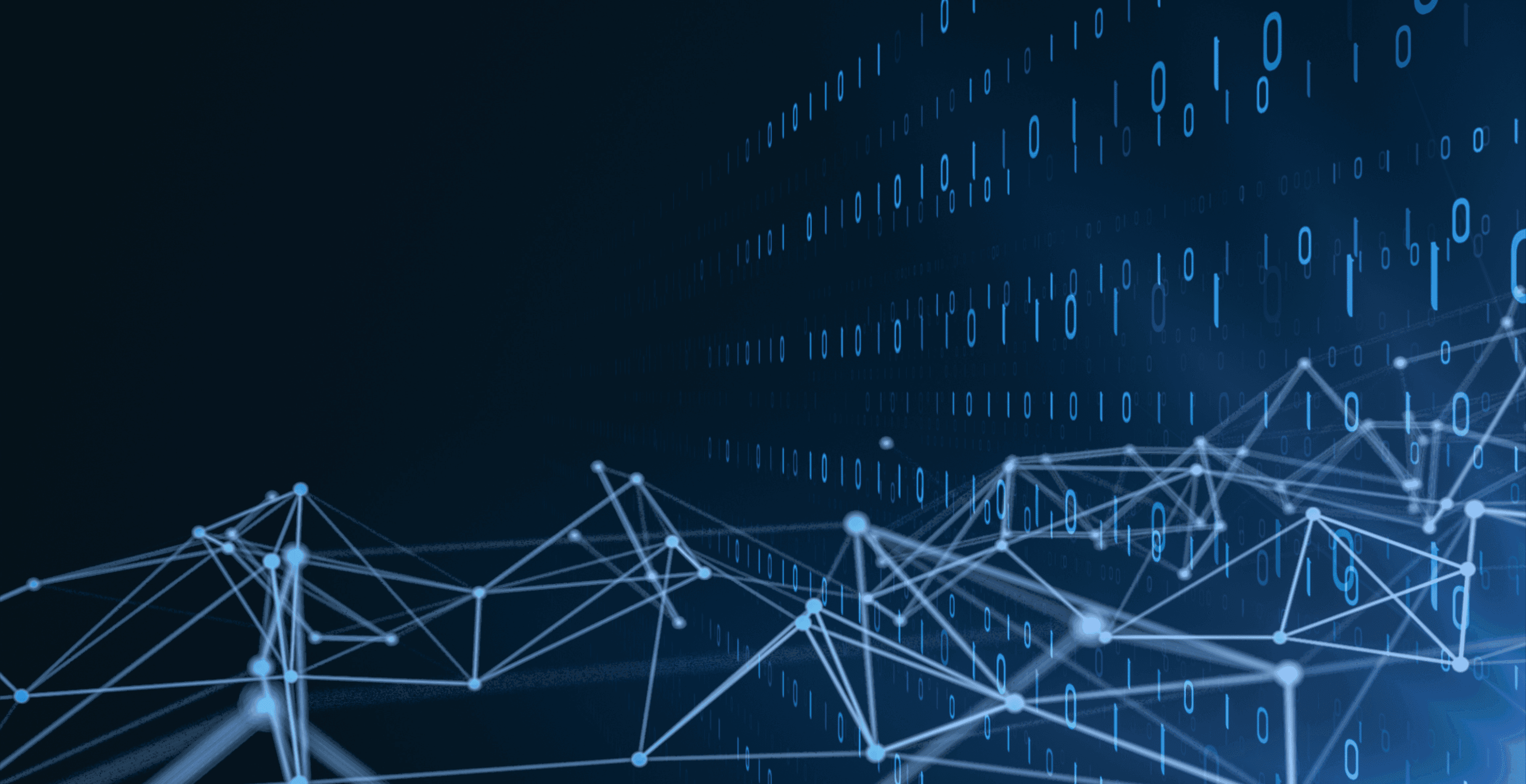
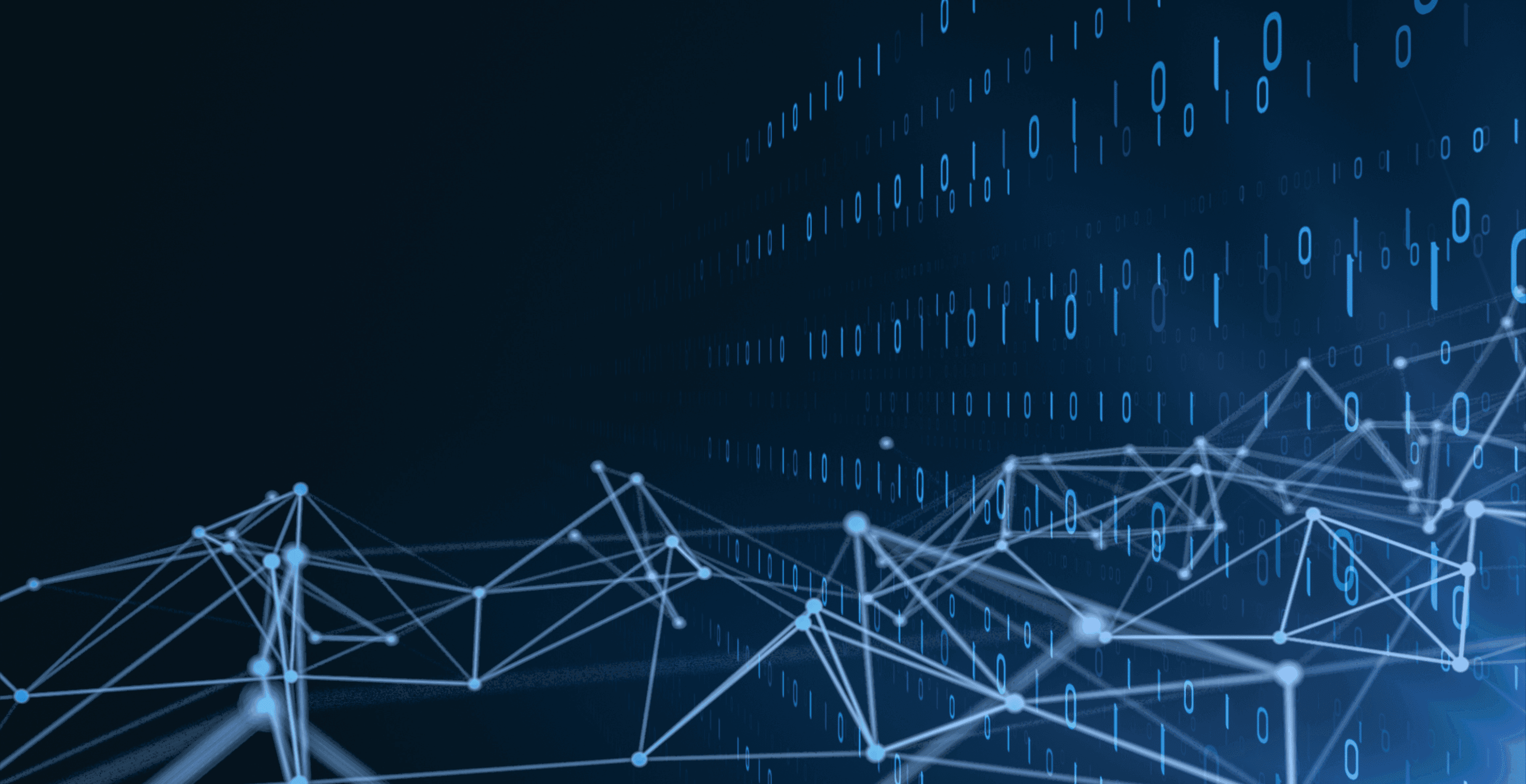
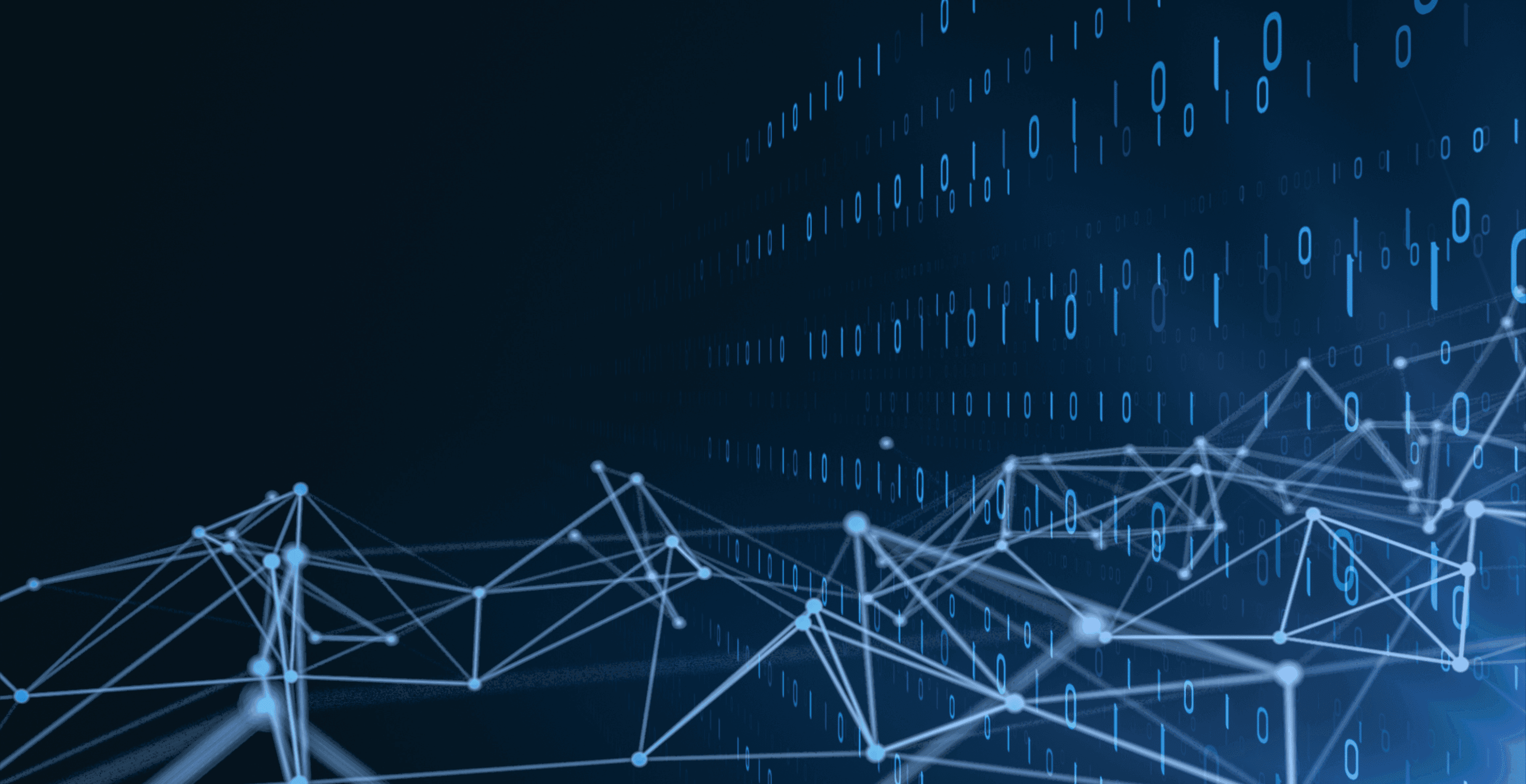
Understanding the Use of Guardrail Metrics in Ensuring LLM Safety

Rehan Asif
Jul 13, 2024
Read the article
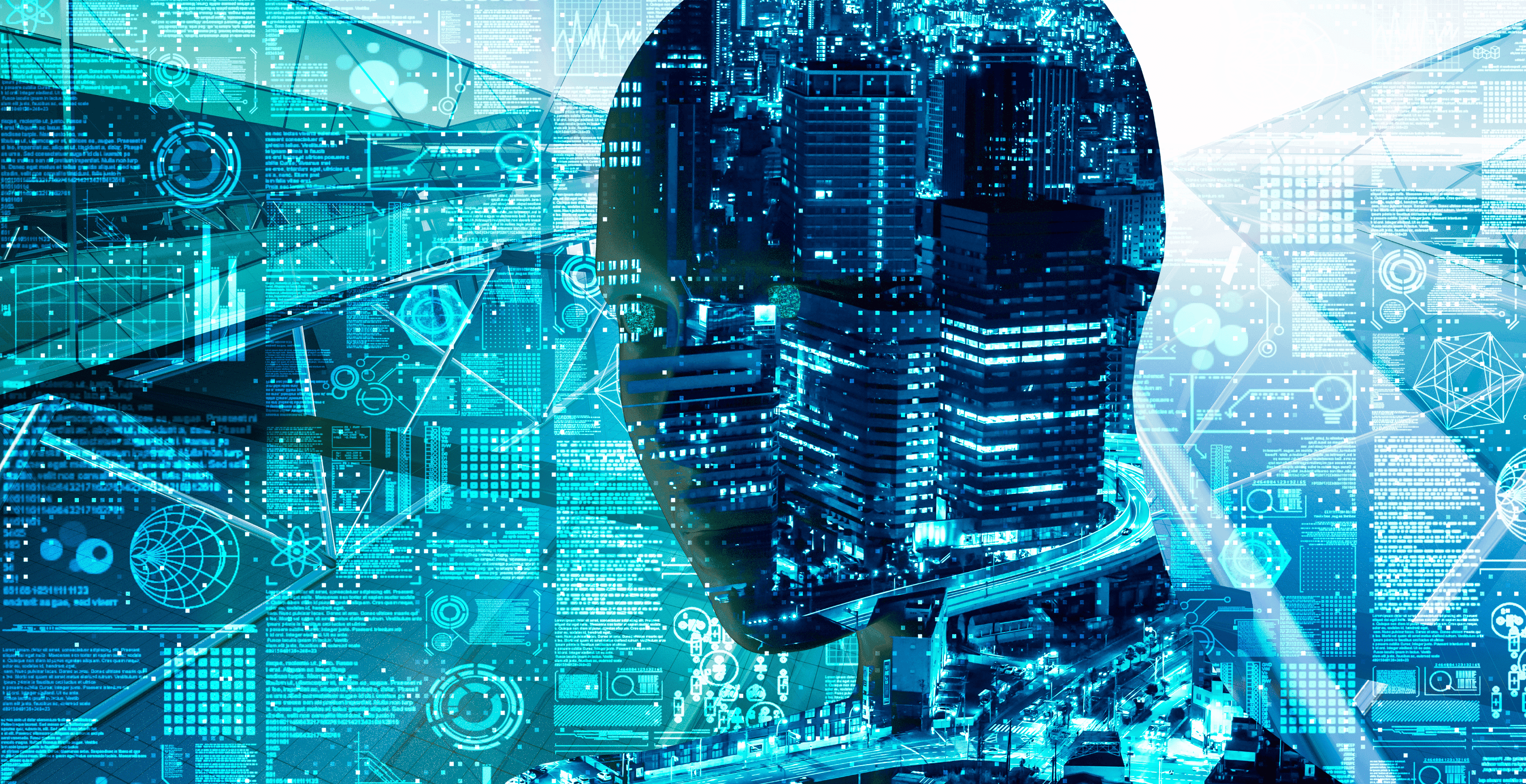
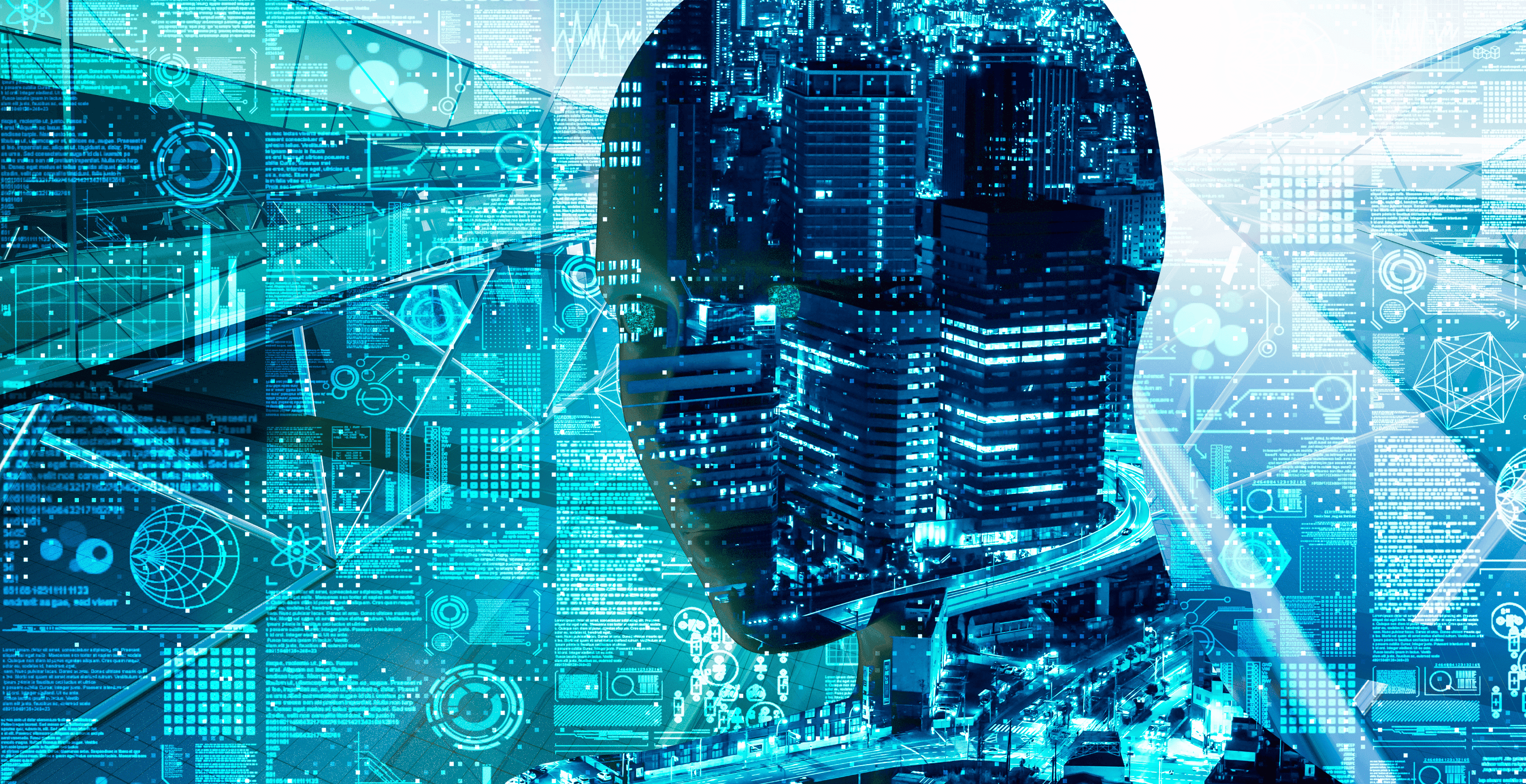
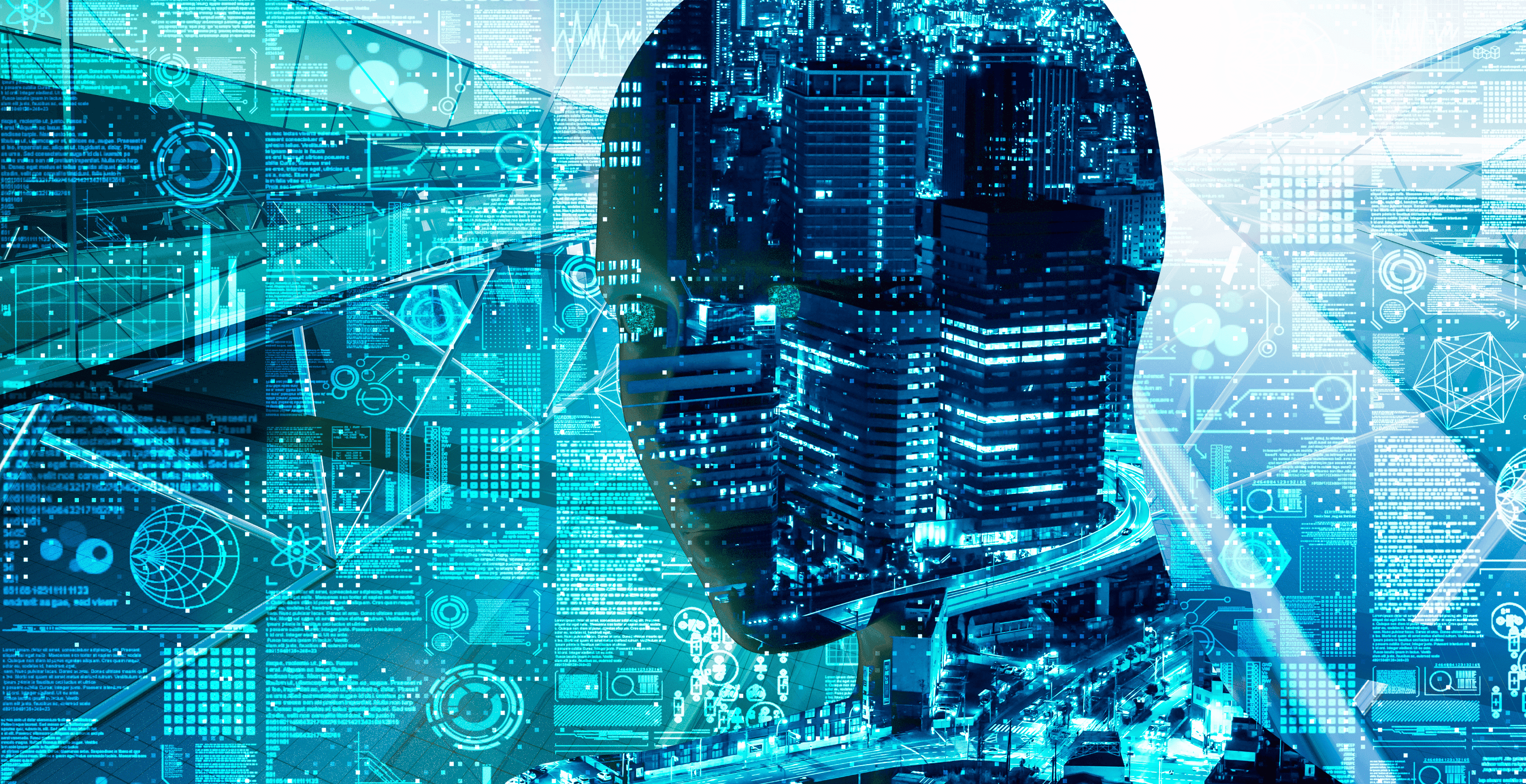
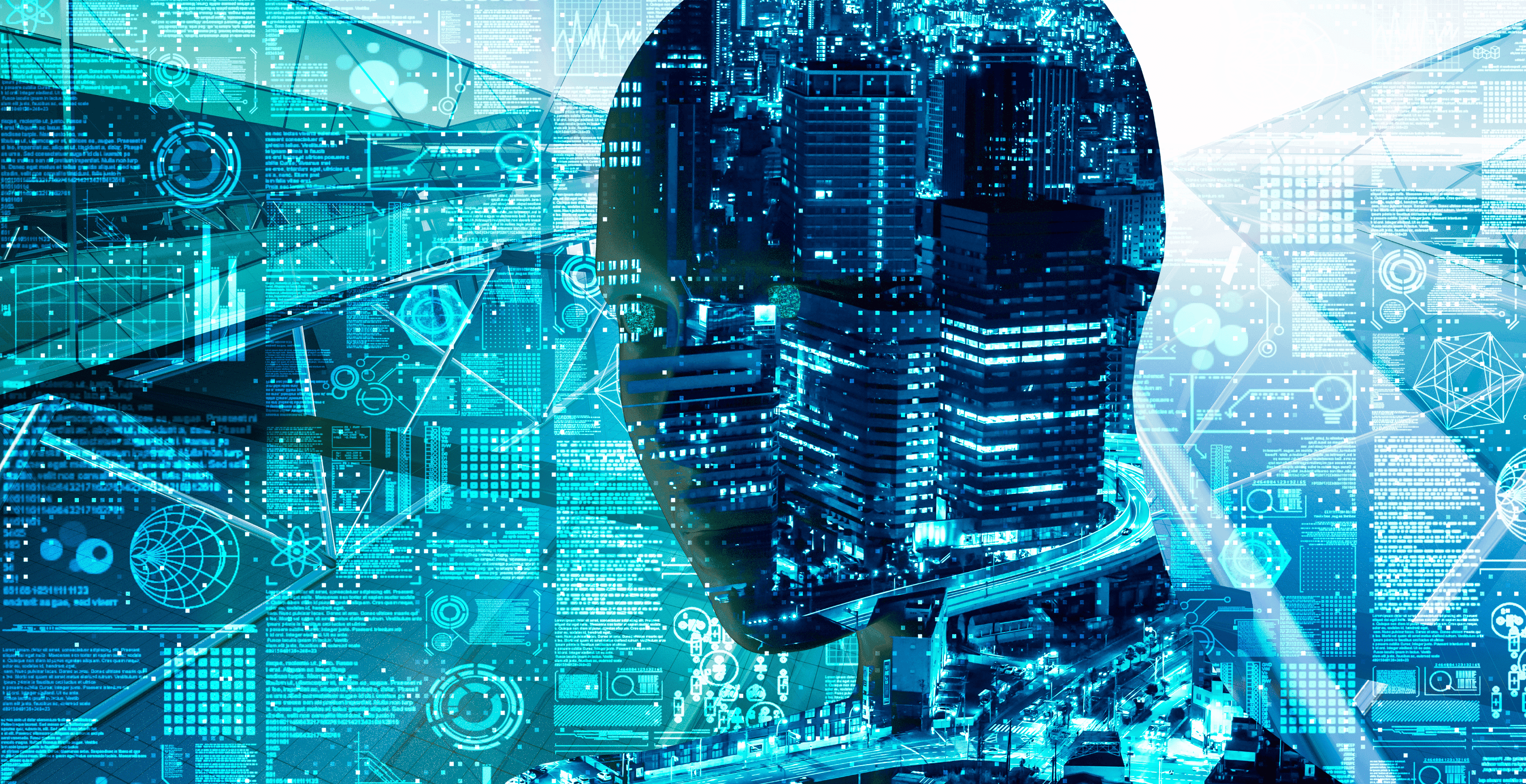
Exploring the Future of LLM and Generative AI Infrastructure

Rehan Asif
Jul 13, 2024
Read the article




Comprehensive Guide to RLHF and Fine Tuning LLMs from Scratch

Rehan Asif
Jul 13, 2024
Read the article
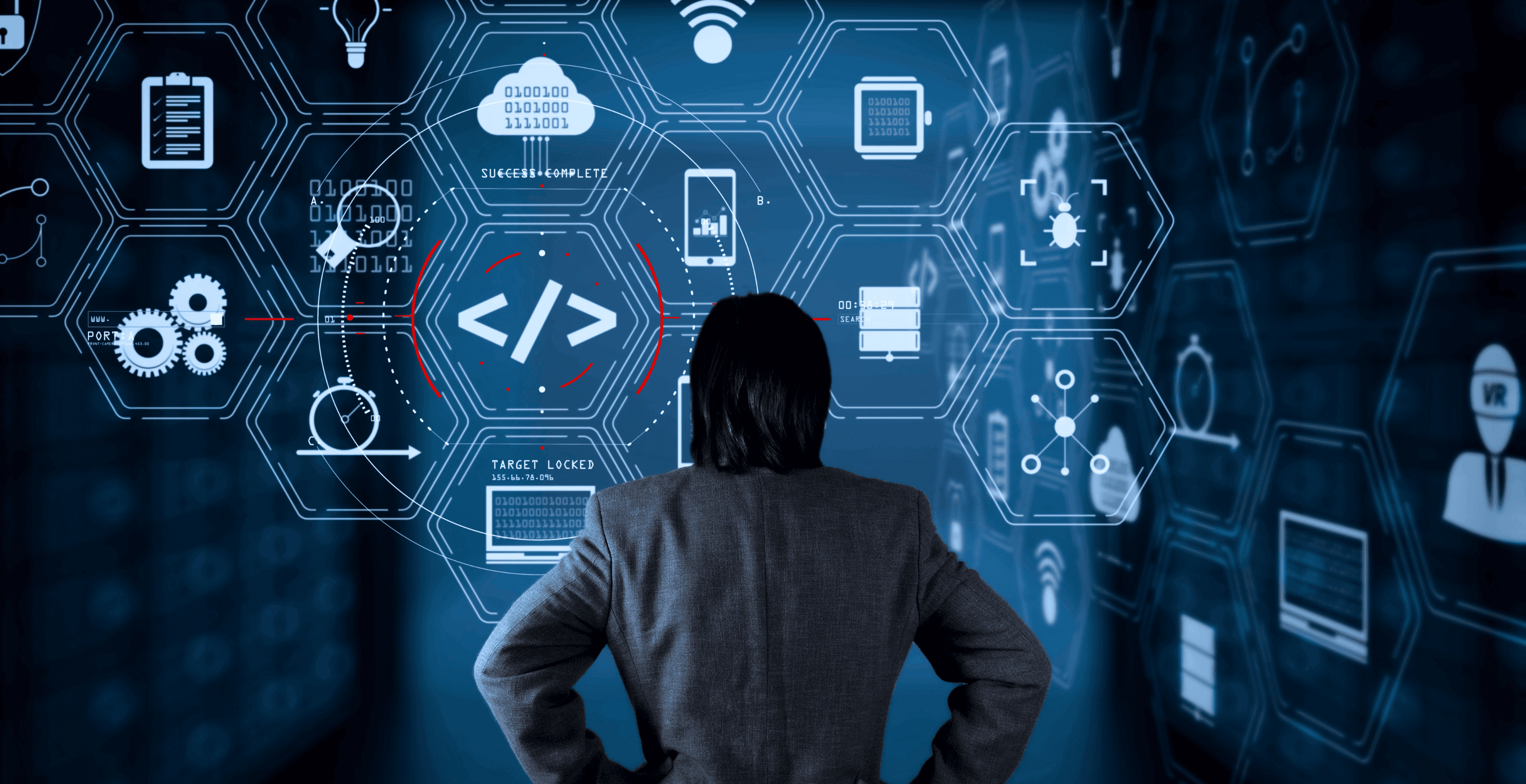
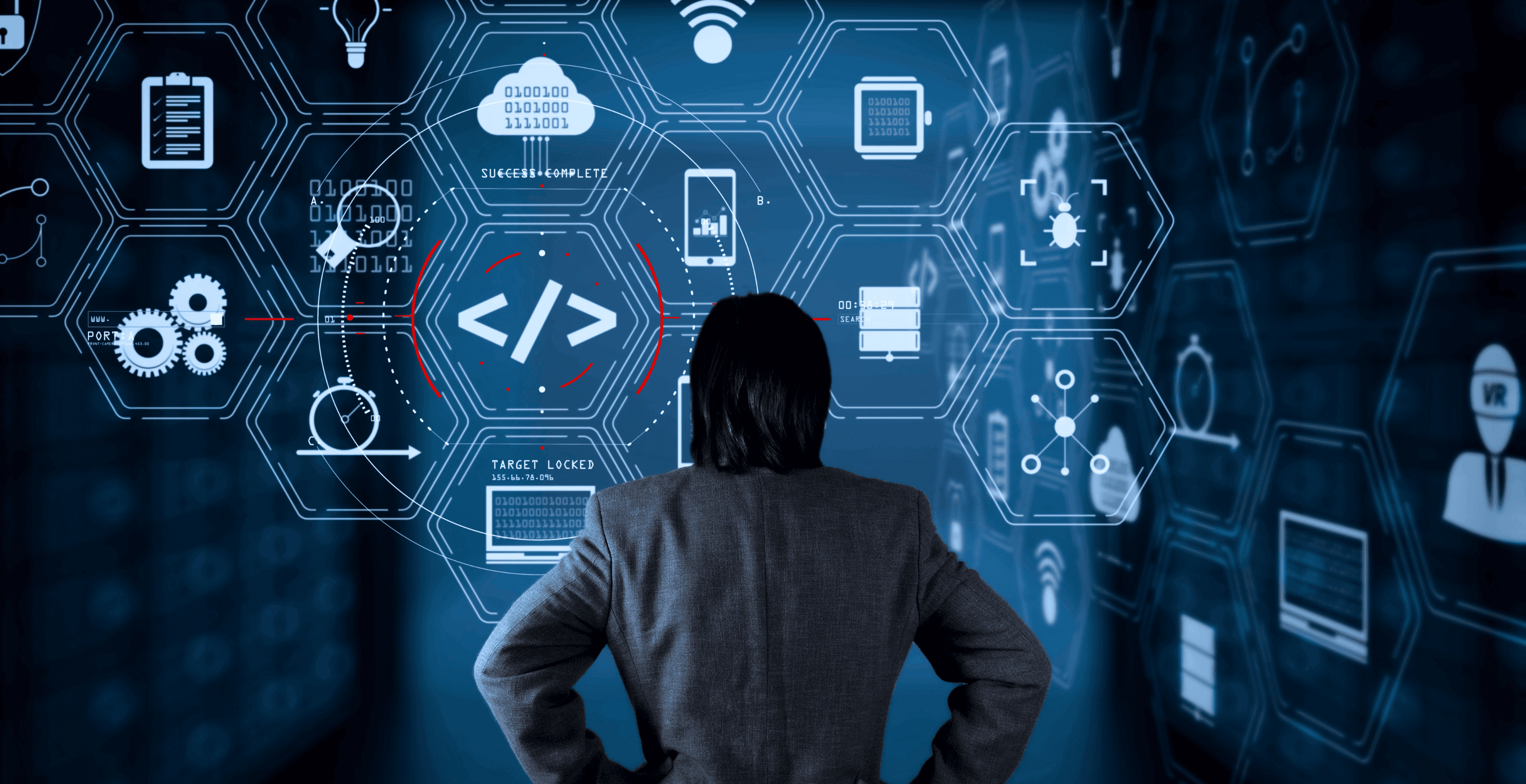
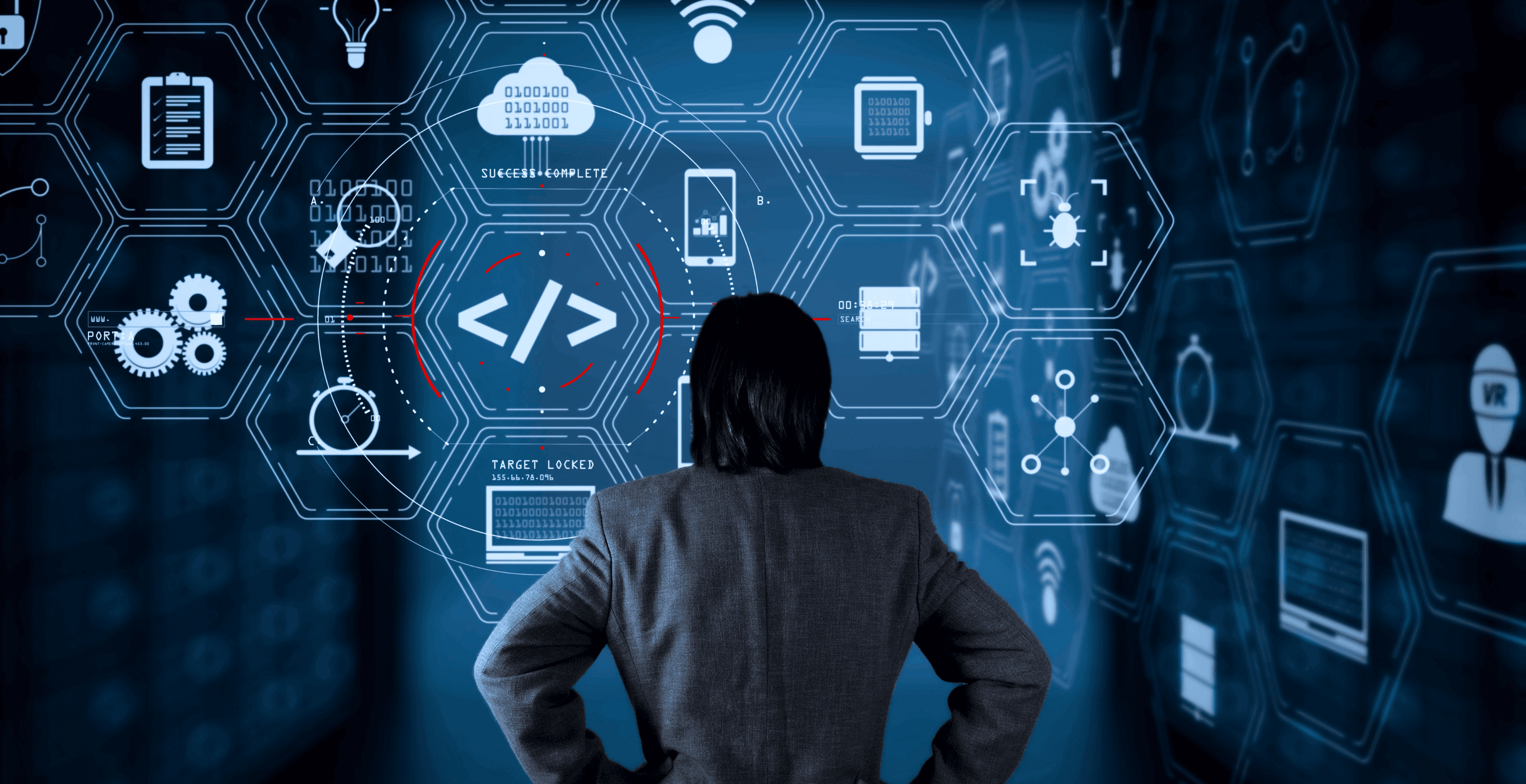
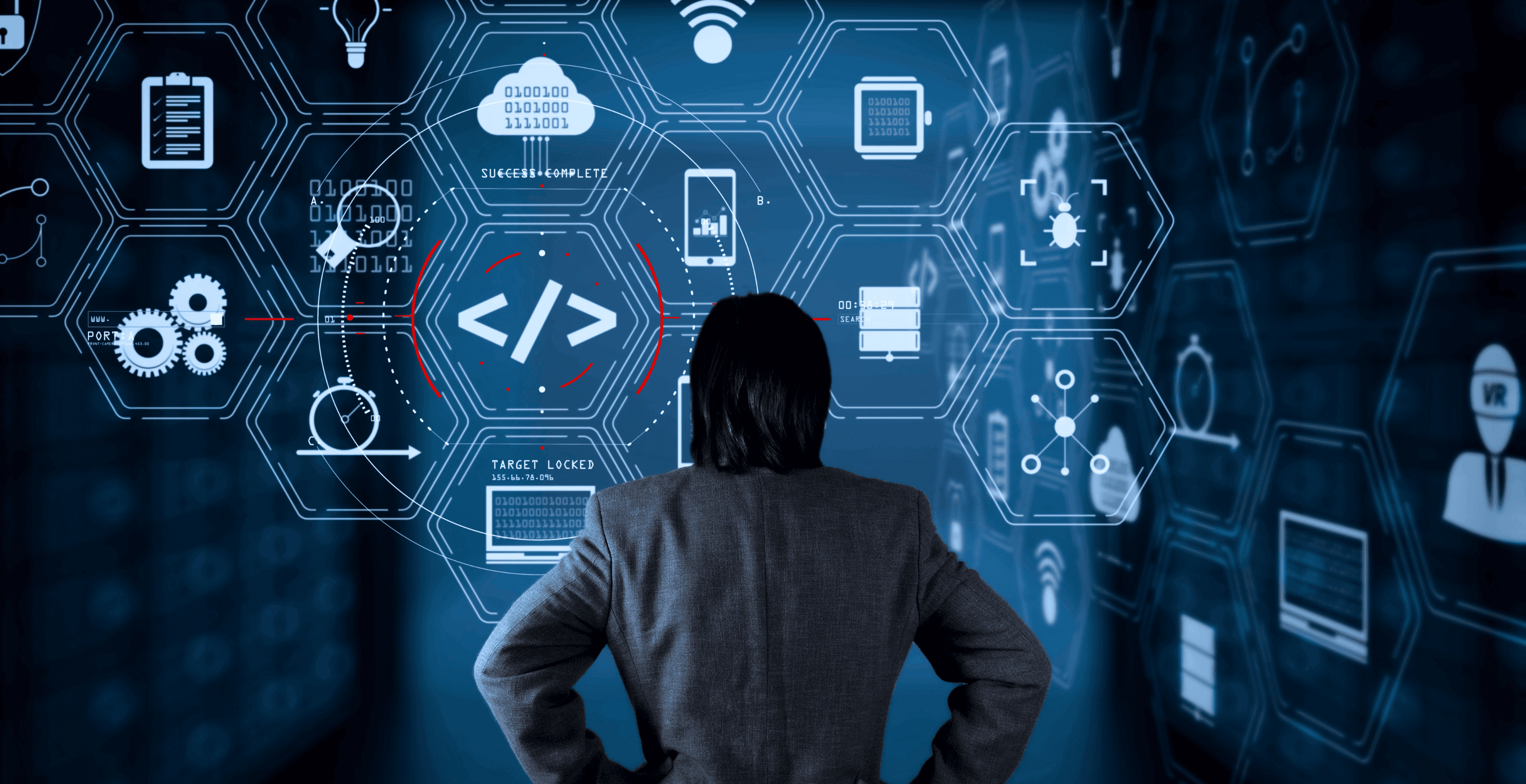
Using Synthetic Data To Enrich RAG Applications
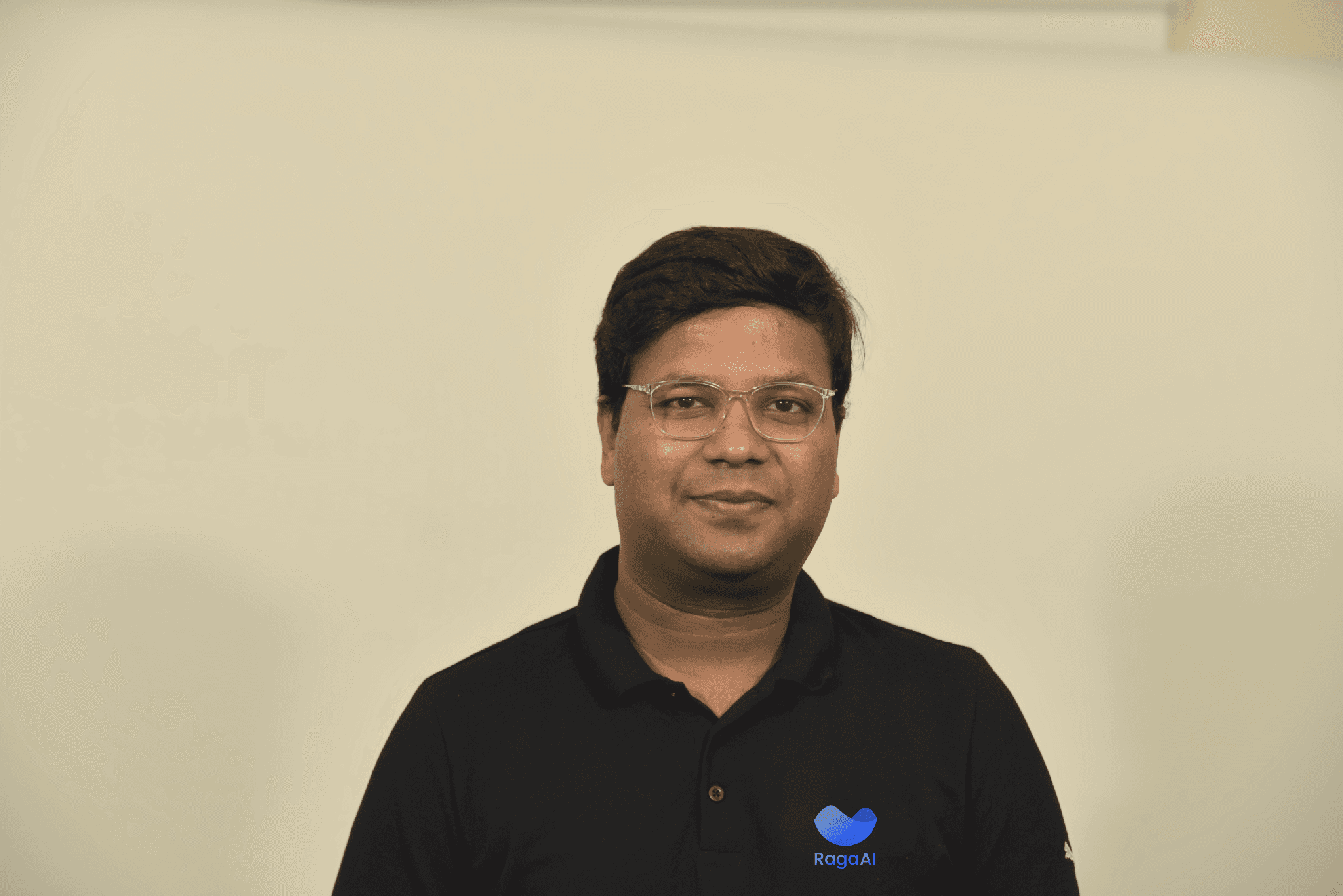
Jigar Gupta
Jul 13, 2024
Read the article




Comparing Different Large Language Model (LLM) Frameworks

Rehan Asif
Jul 12, 2024
Read the article
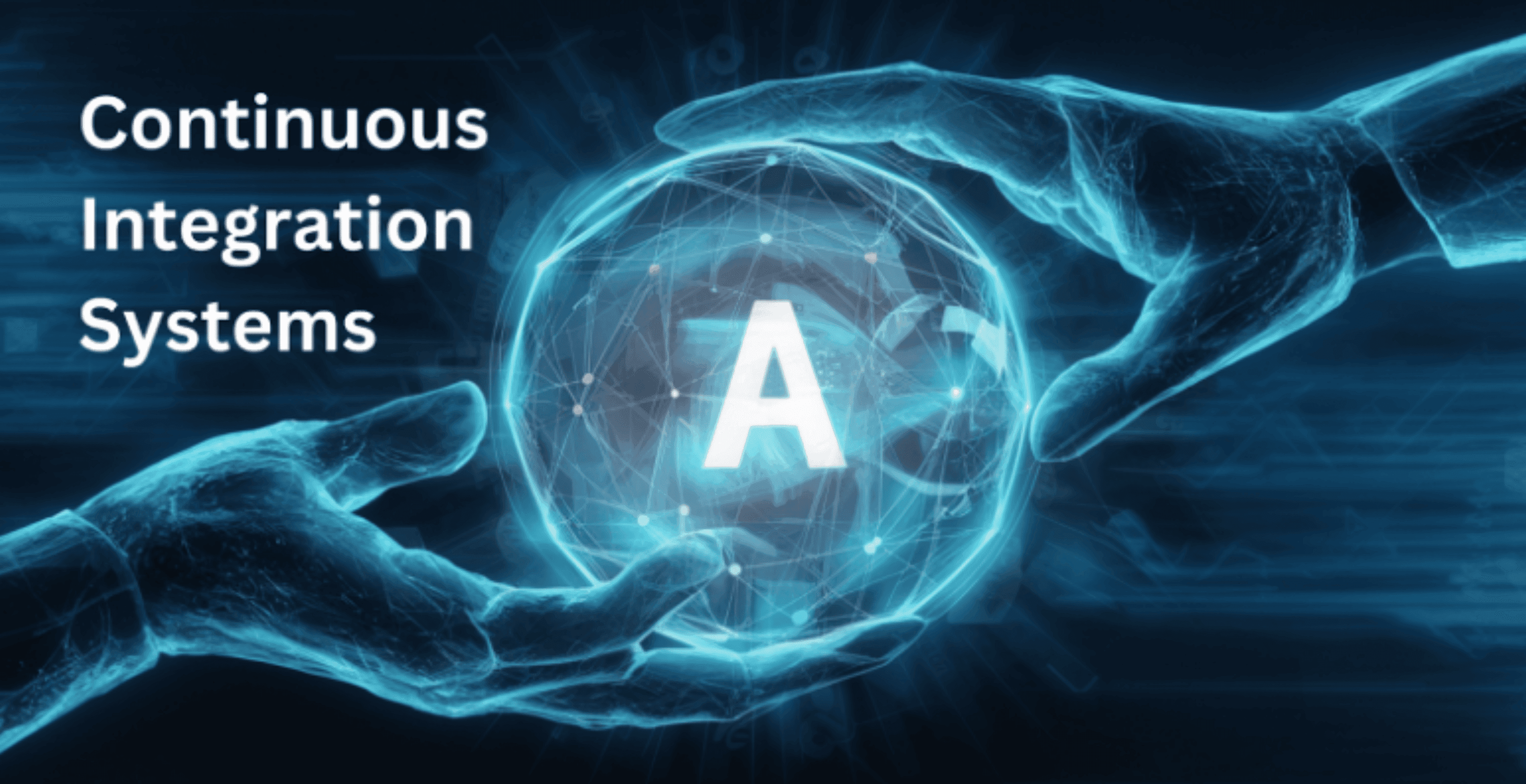
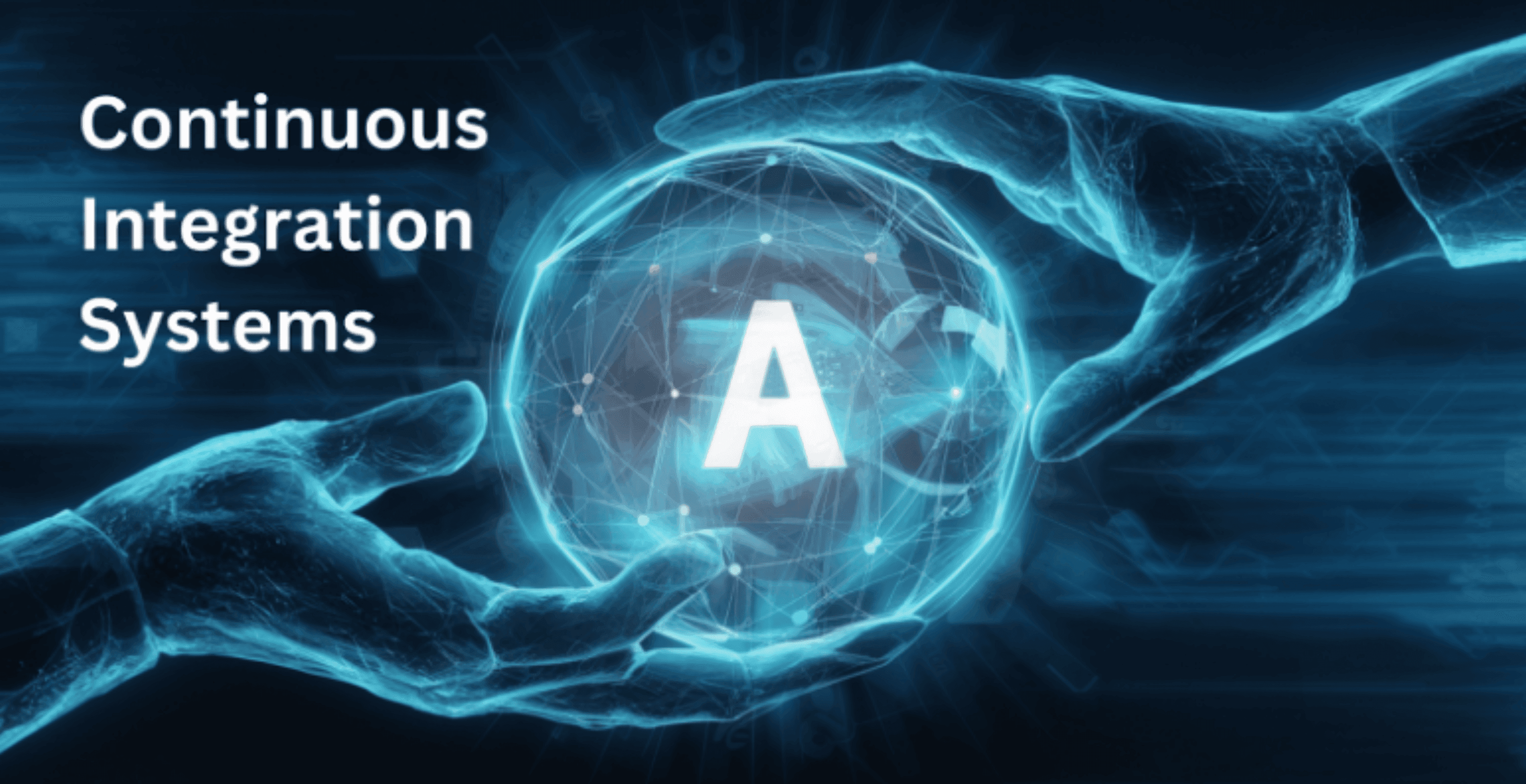
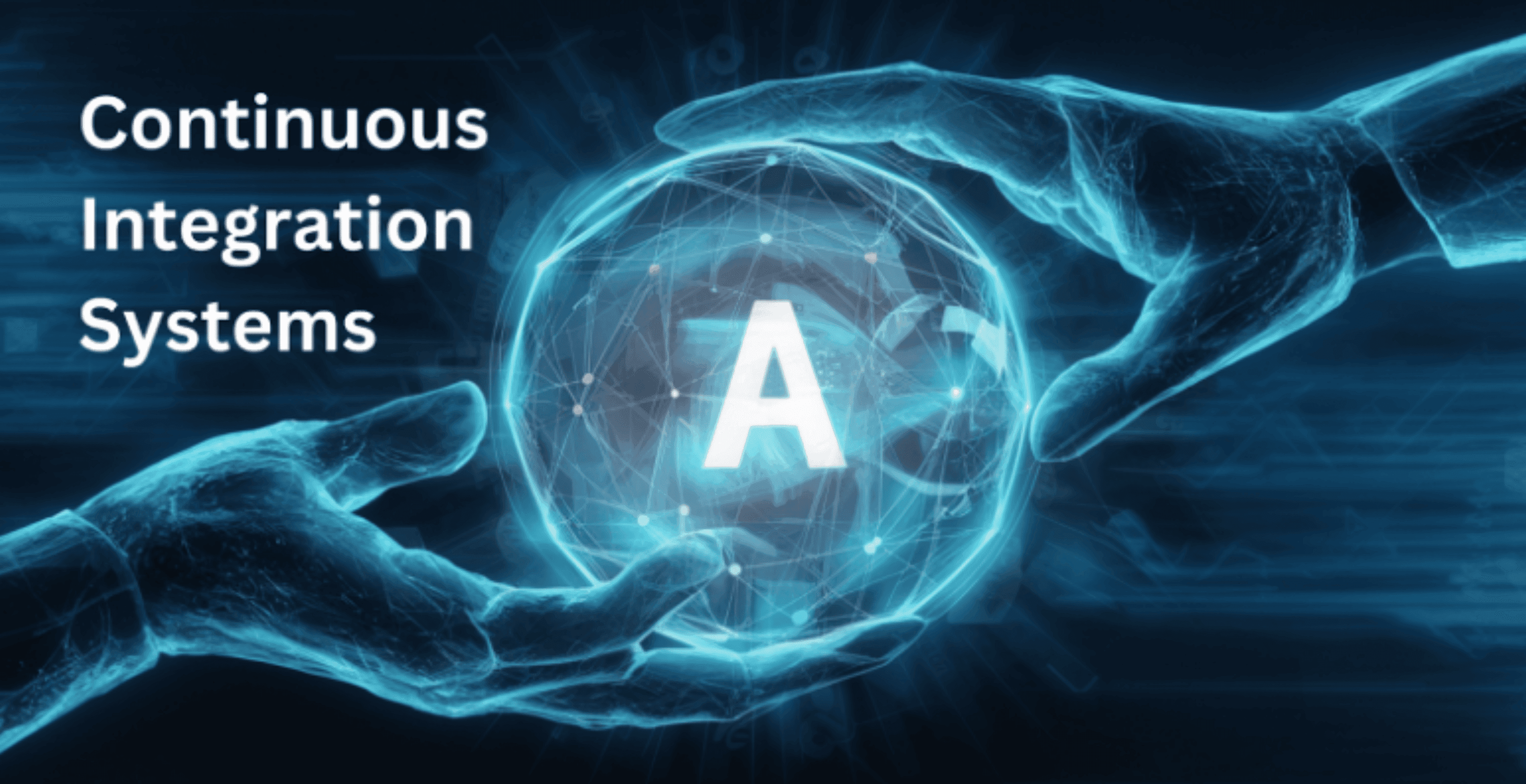
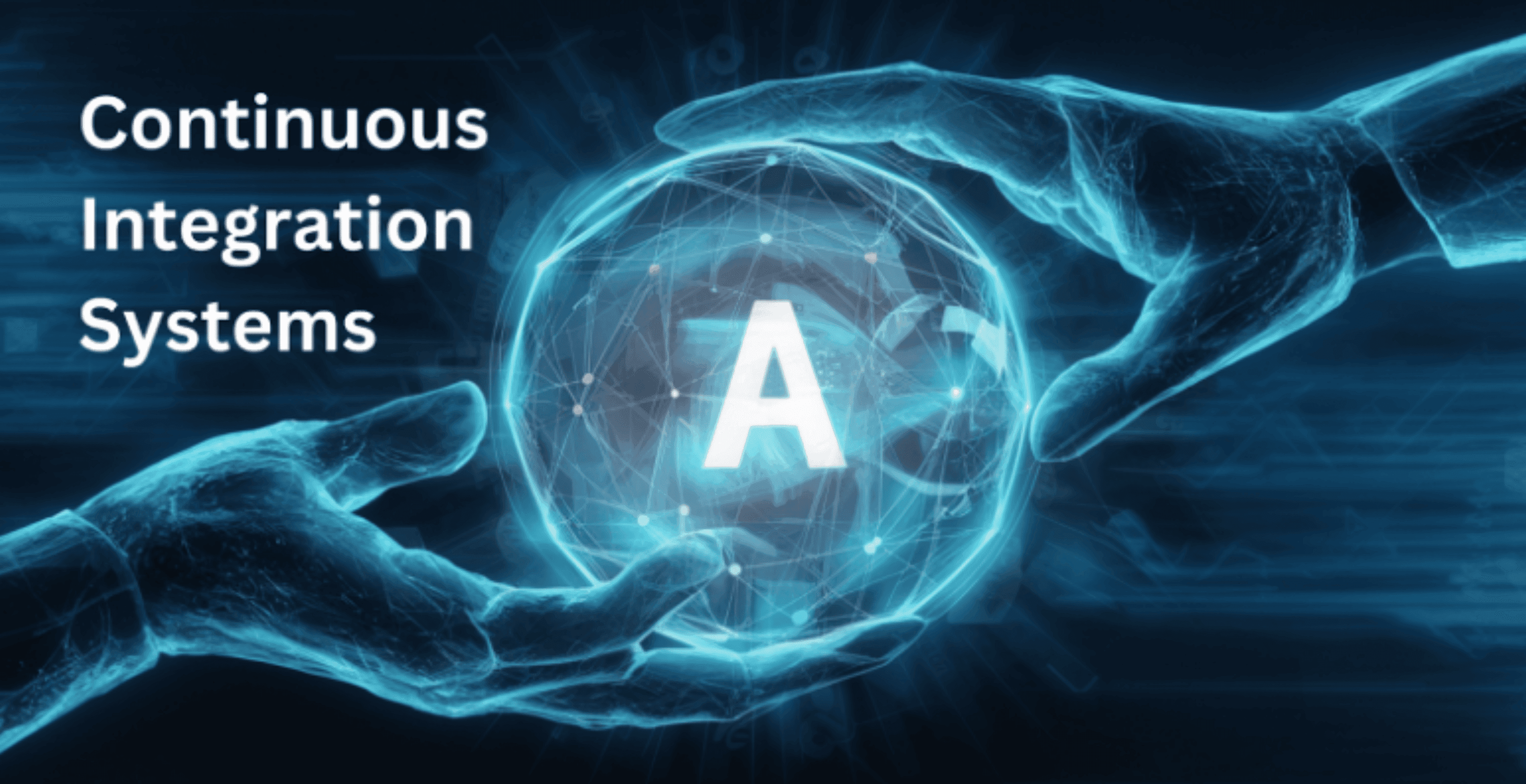
Integrating AI Models with Continuous Integration Systems
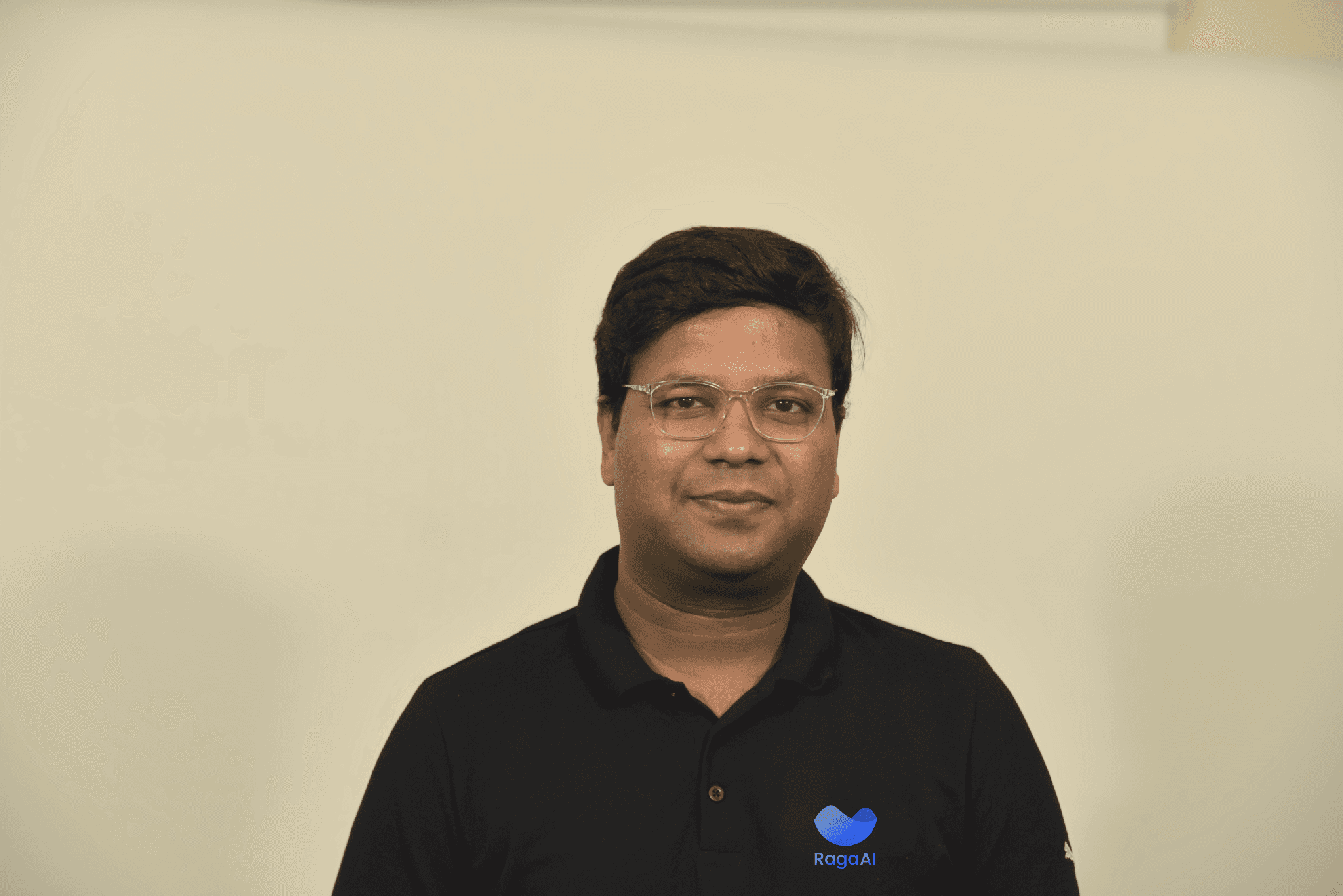
Jigar Gupta
Jul 12, 2024
Read the article




Understanding Retrieval Augmented Generation for Large Language Models: A Survey
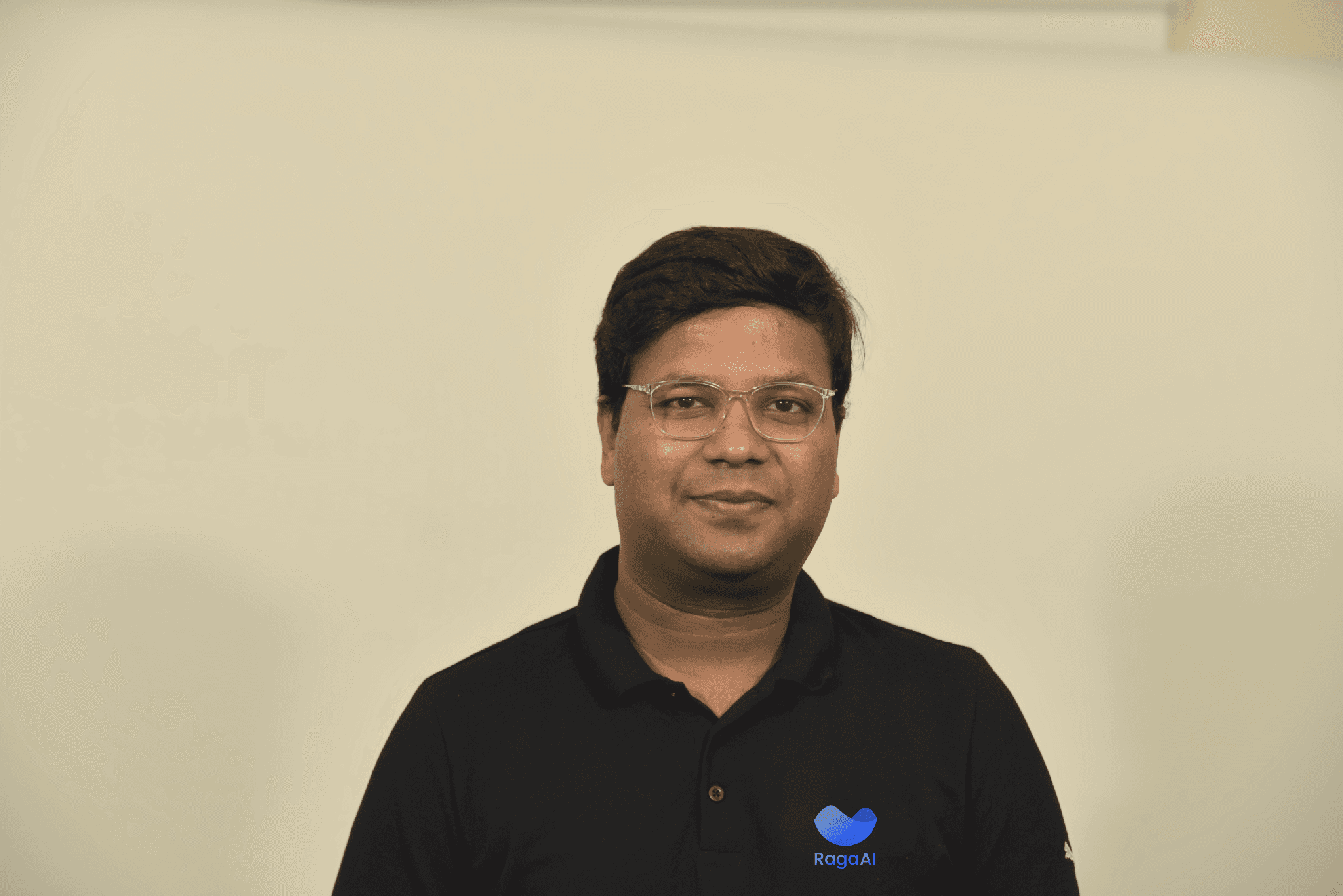
Jigar Gupta
Jul 12, 2024
Read the article




Leveraging AI For Enhanced Retail Customer Experiences
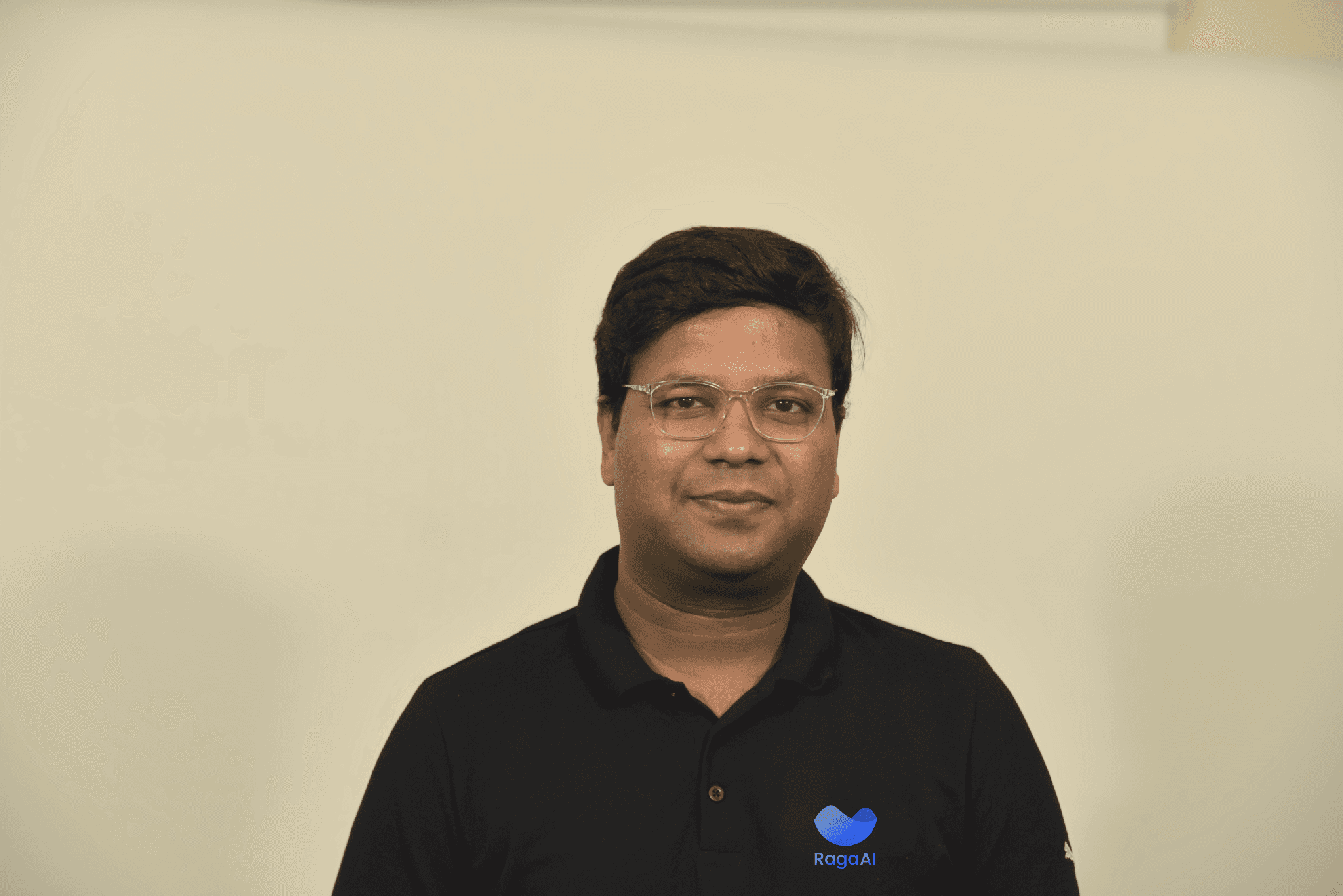
Jigar Gupta
Jul 1, 2024
Read the article
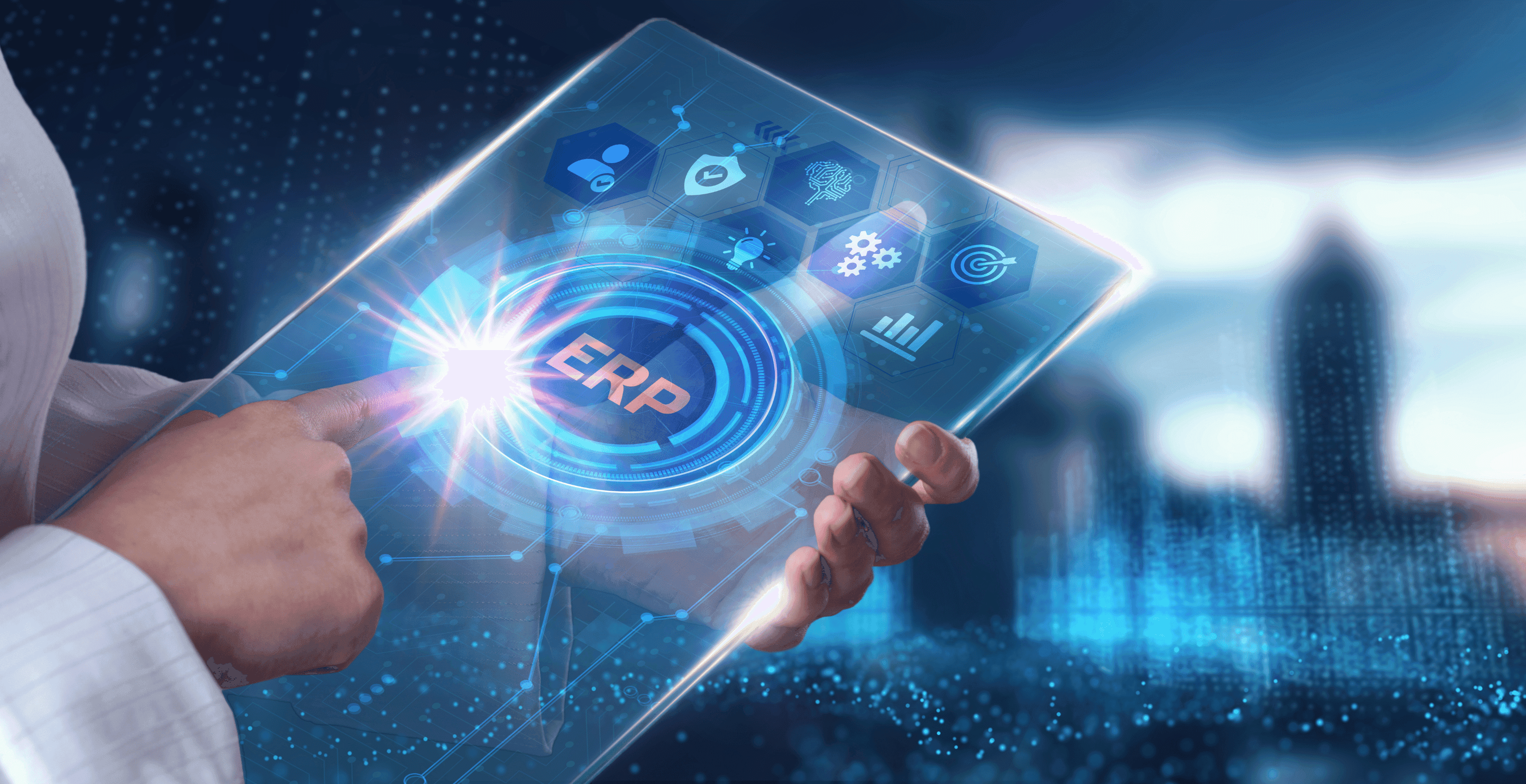
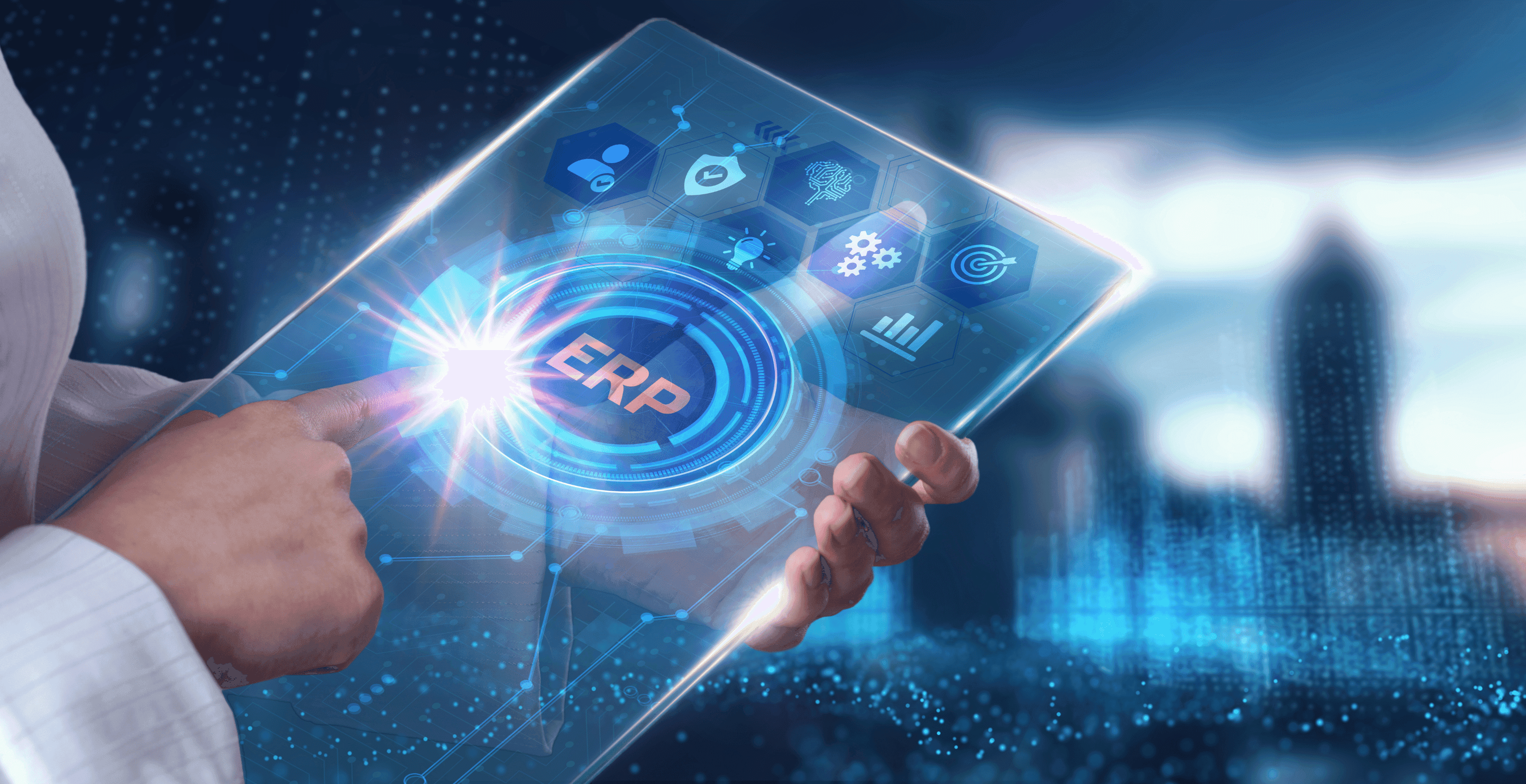
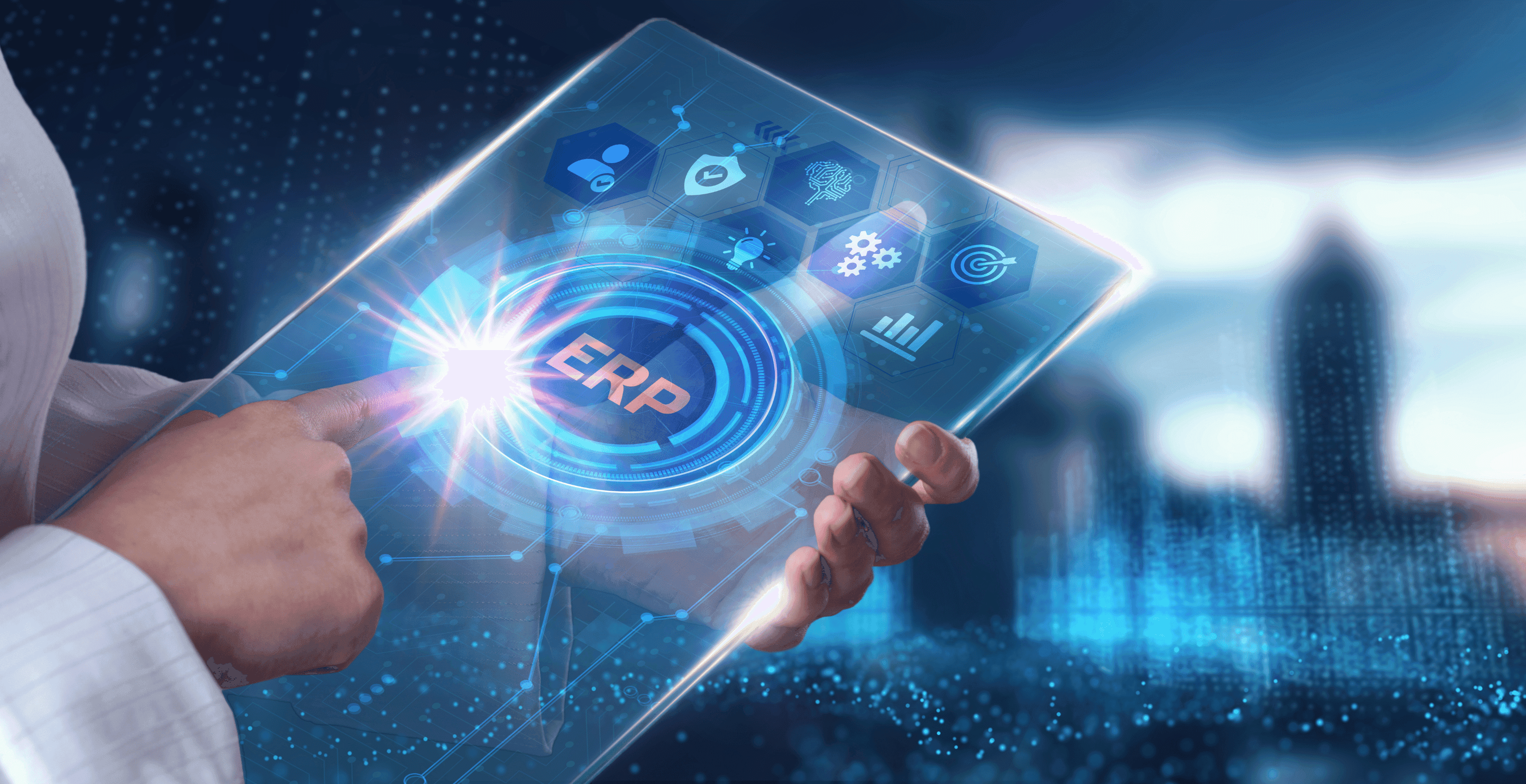
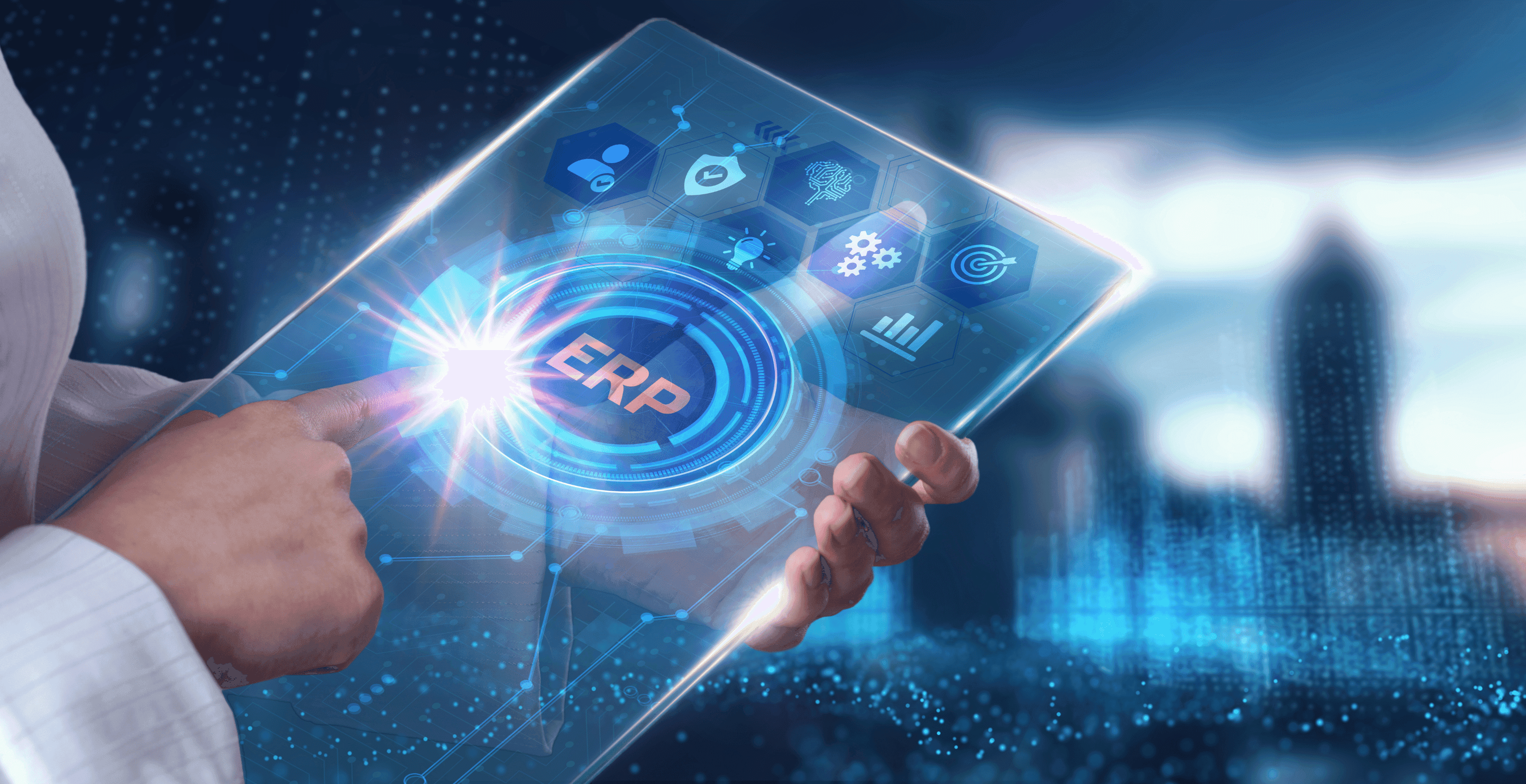
Enhancing Enterprise Search Using RAG and LLMs

Rehan Asif
Jul 1, 2024
Read the article
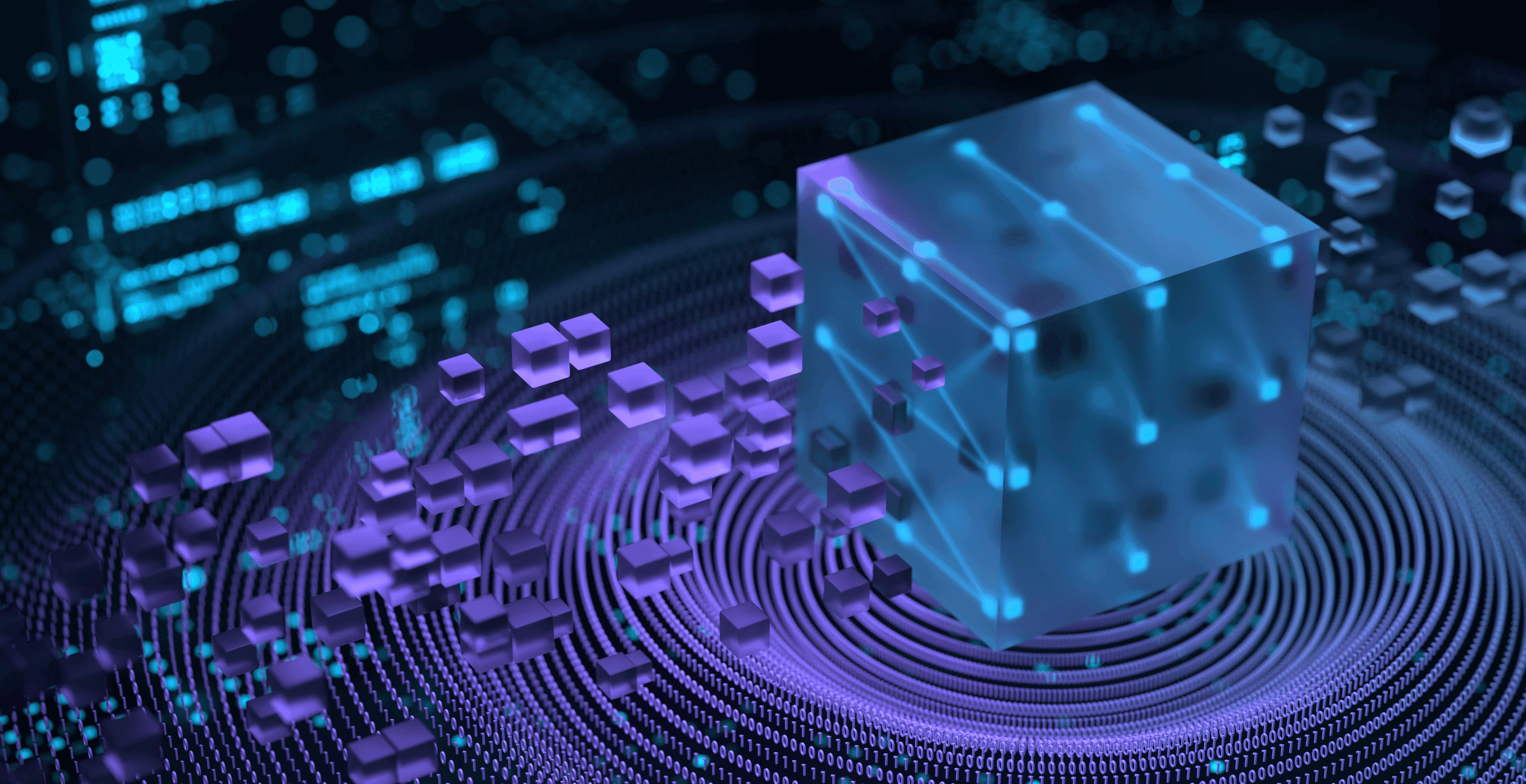
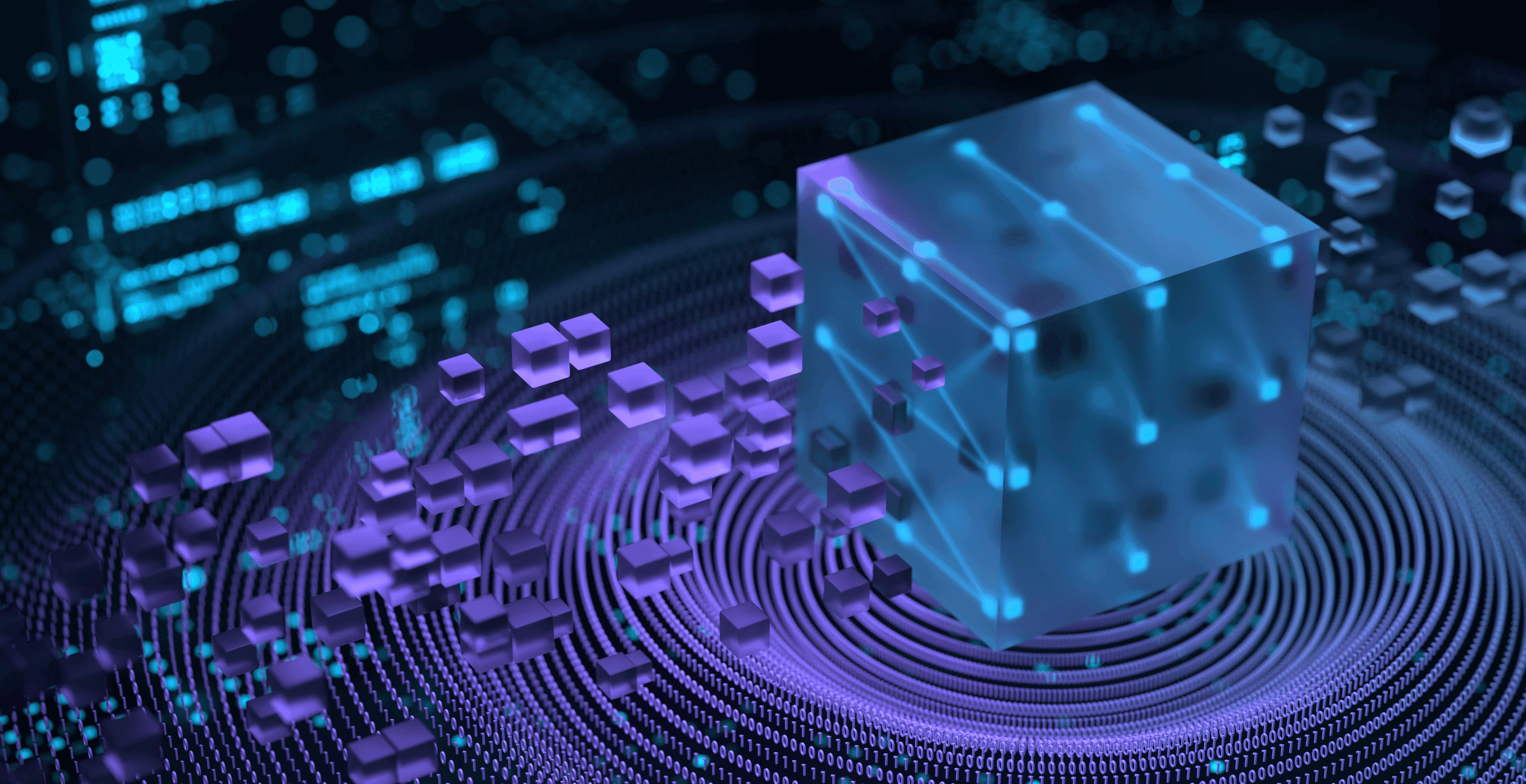
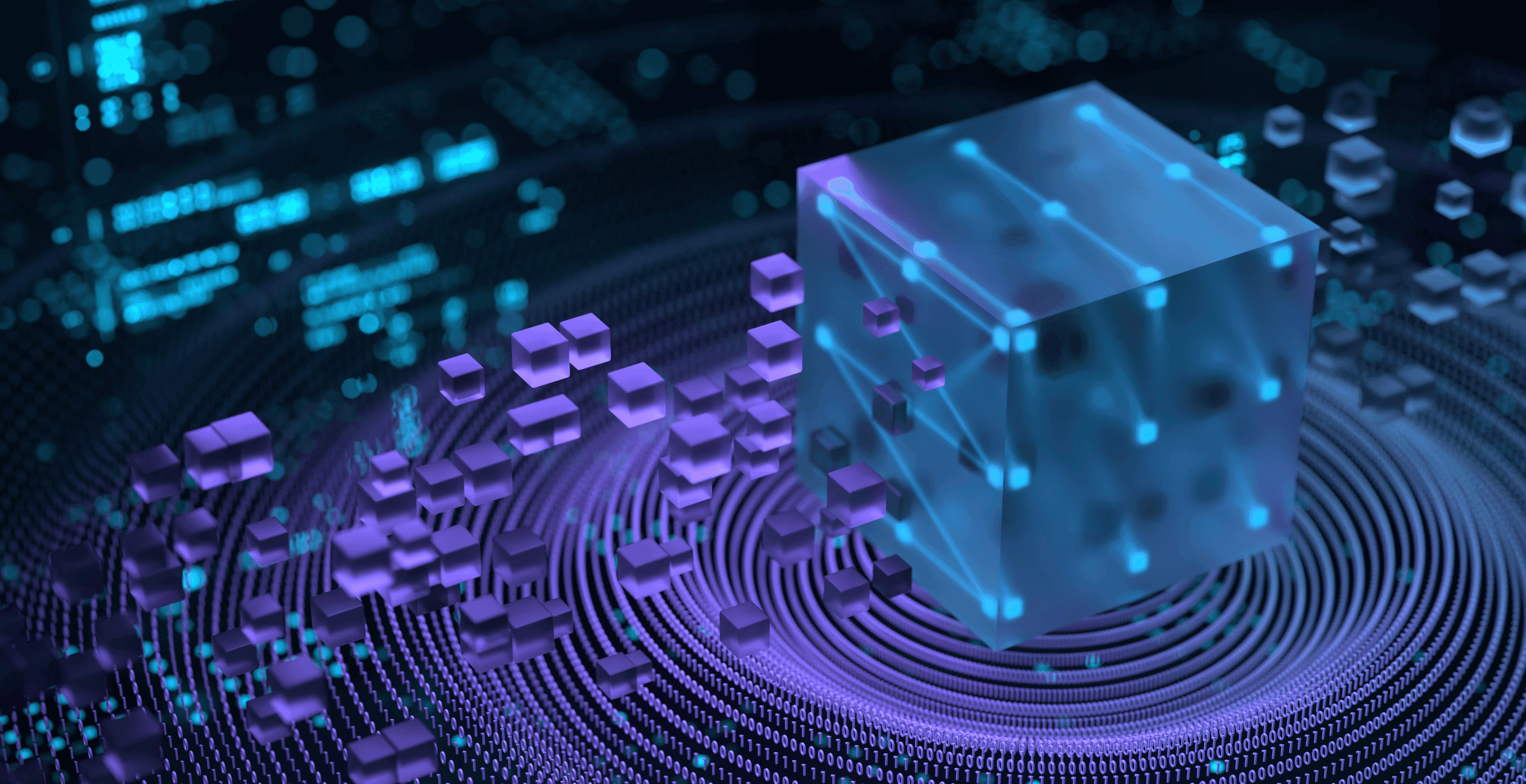
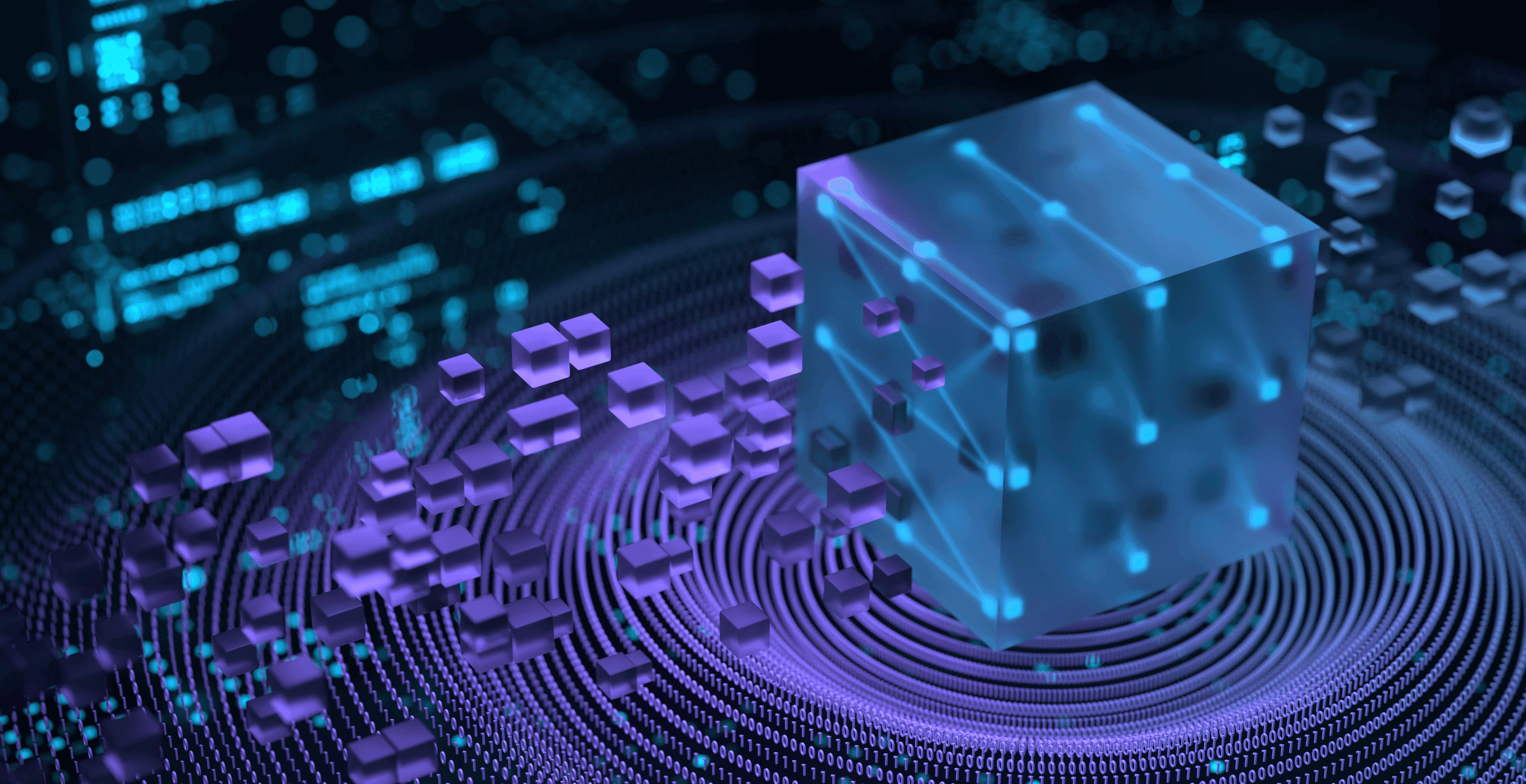
Importance of Accuracy and Reliability in Tabular Data Models
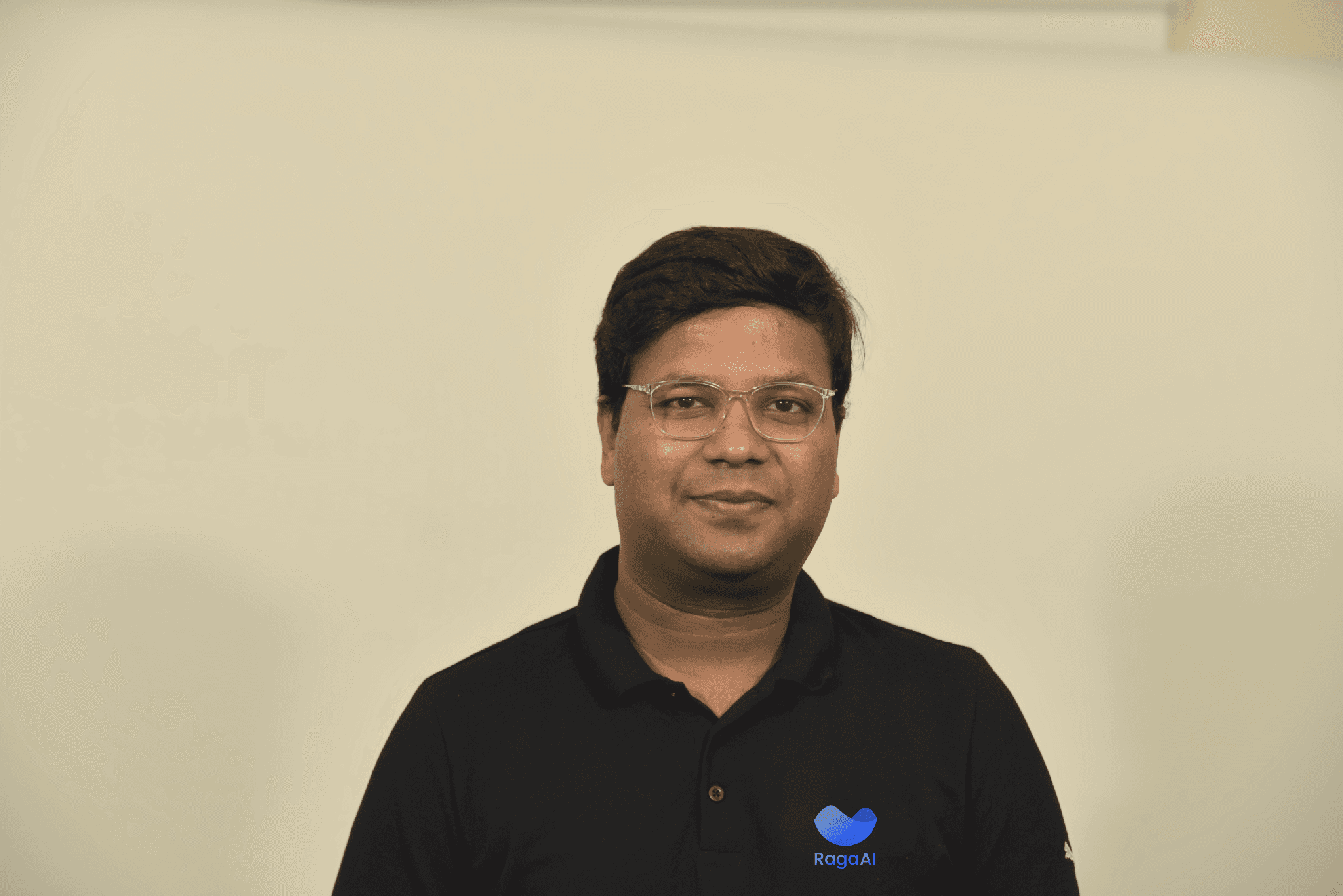
Jigar Gupta
Jul 1, 2024
Read the article
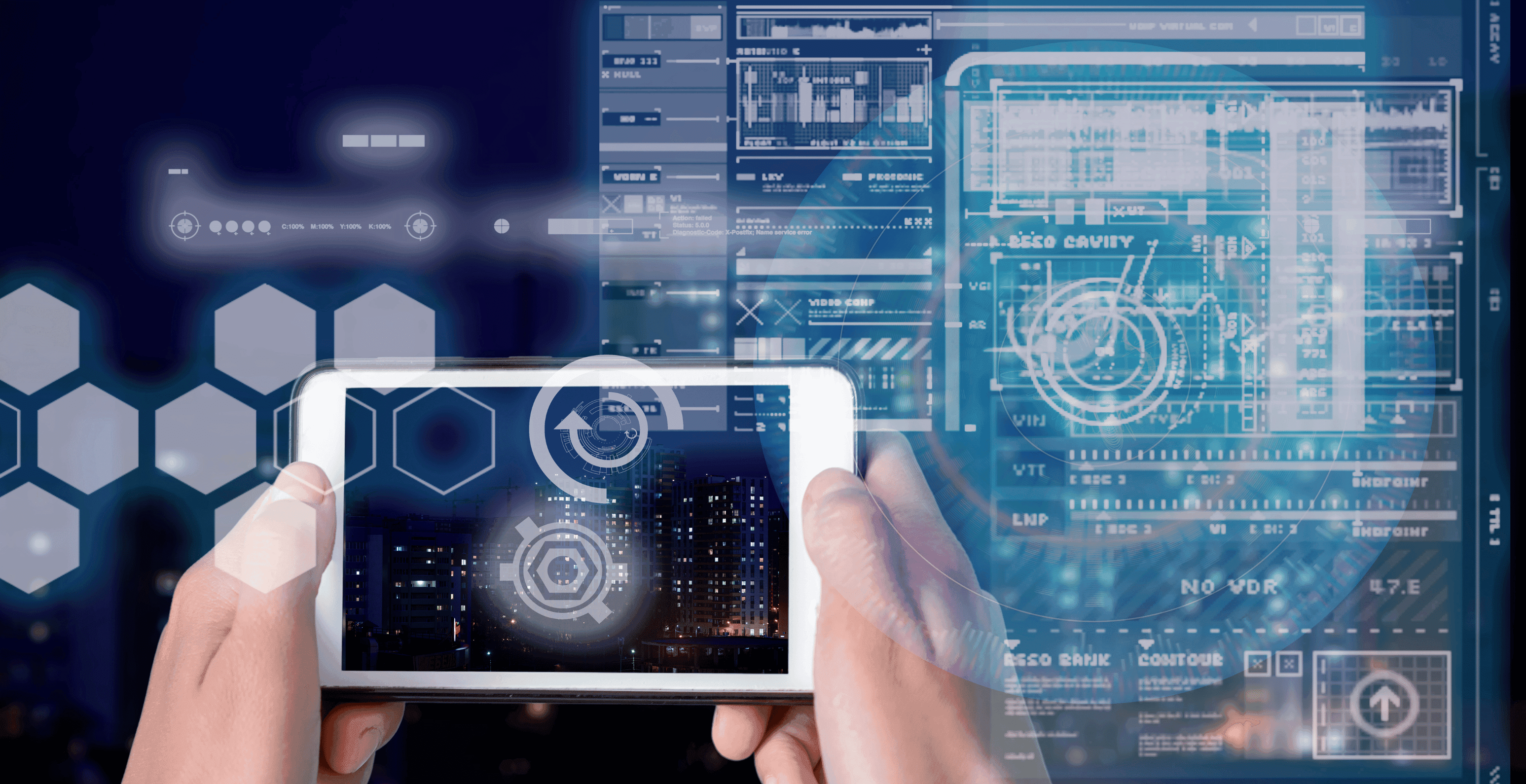
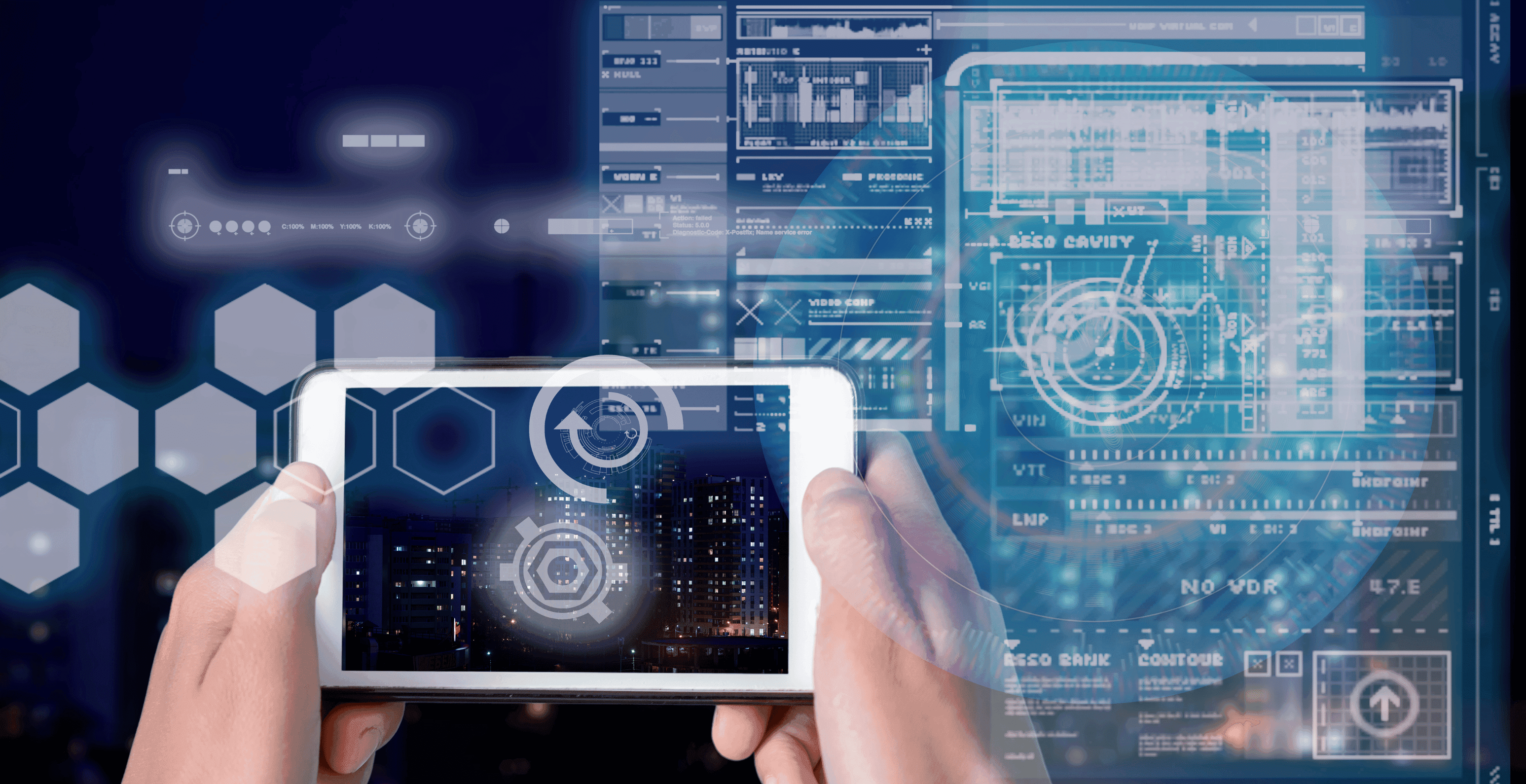
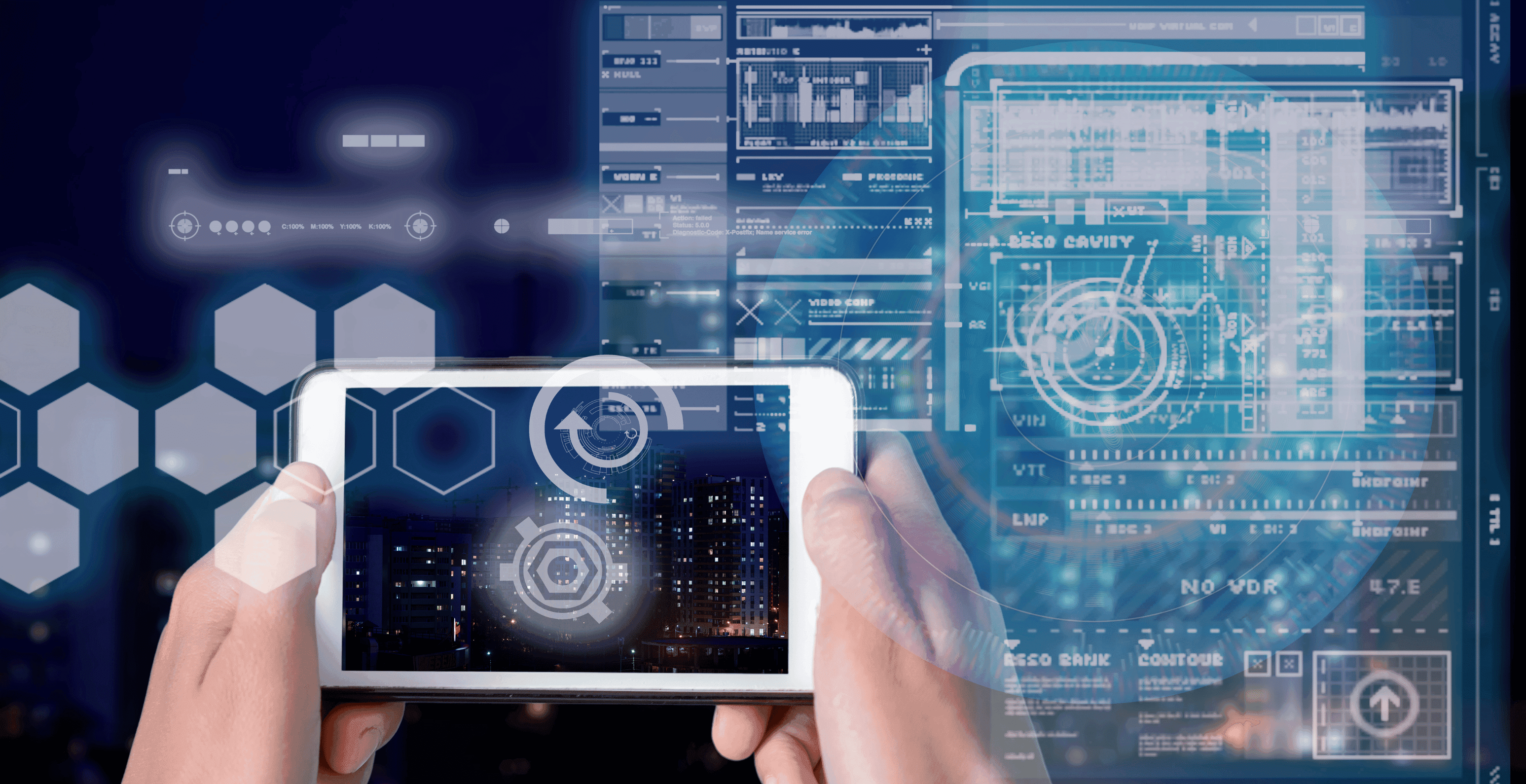
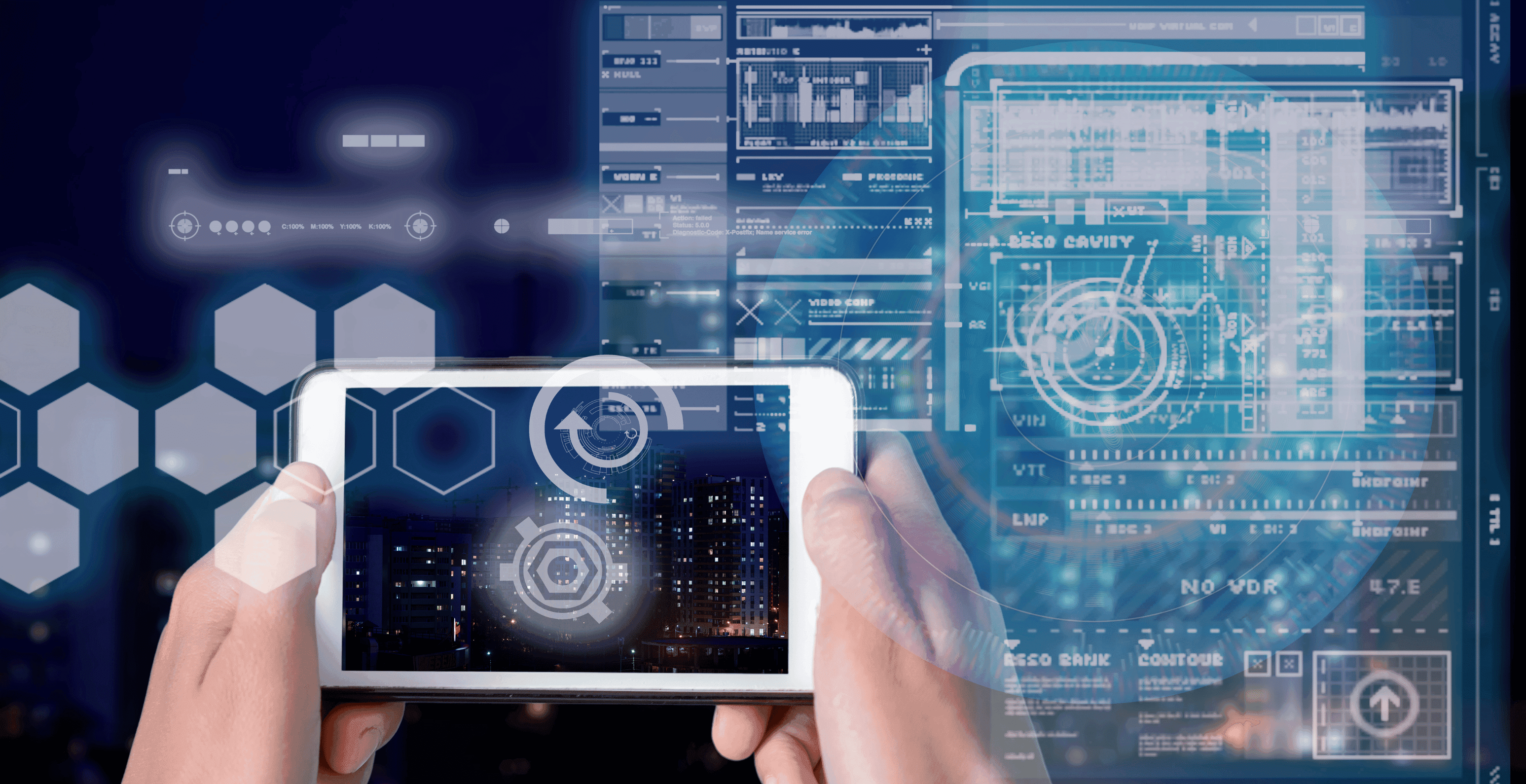
Information Retrieval And LLMs: RAG Explained

Rehan Asif
Jul 1, 2024
Read the article




Introduction to LLM Powered Autonomous Agents

Rehan Asif
Jul 1, 2024
Read the article
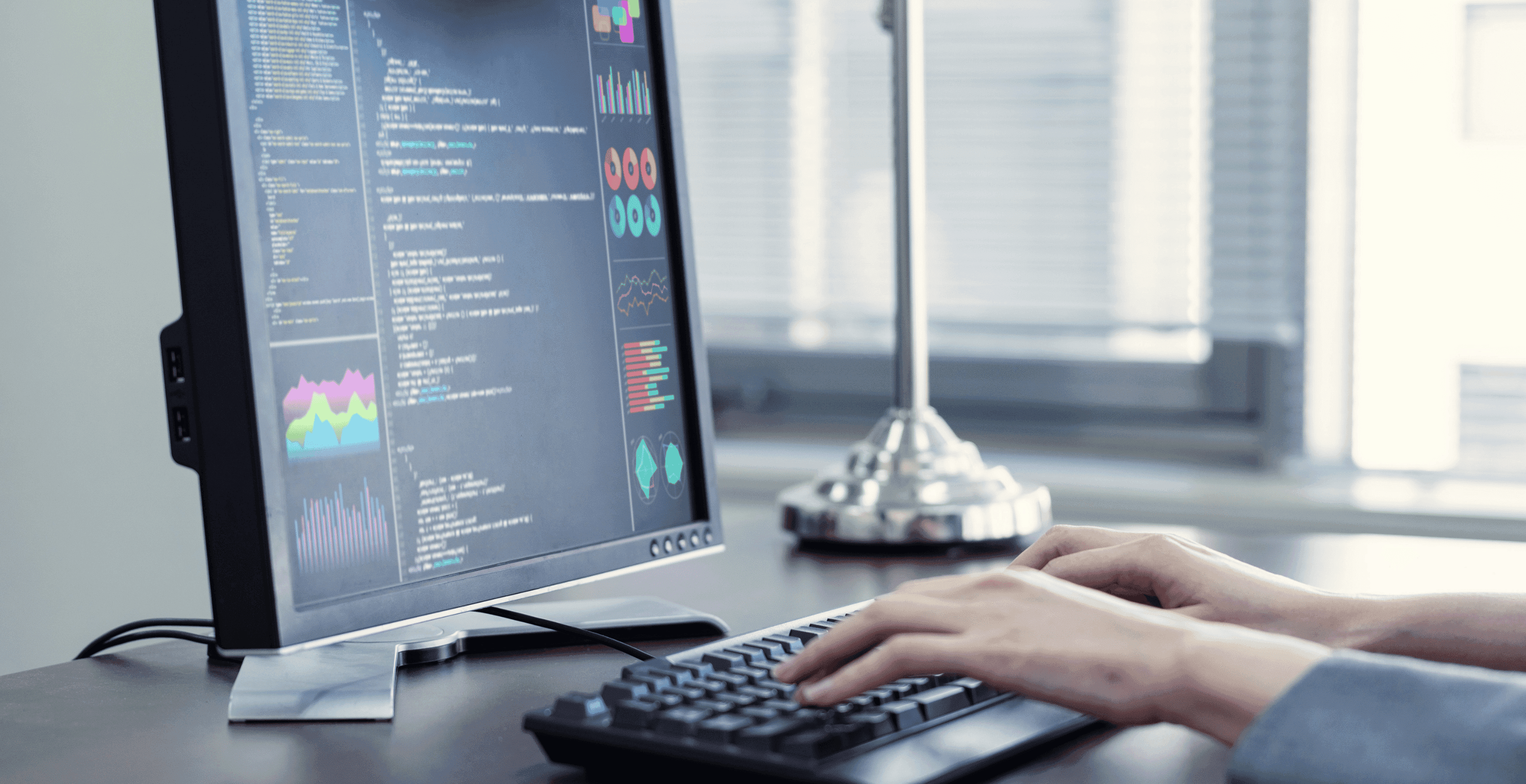
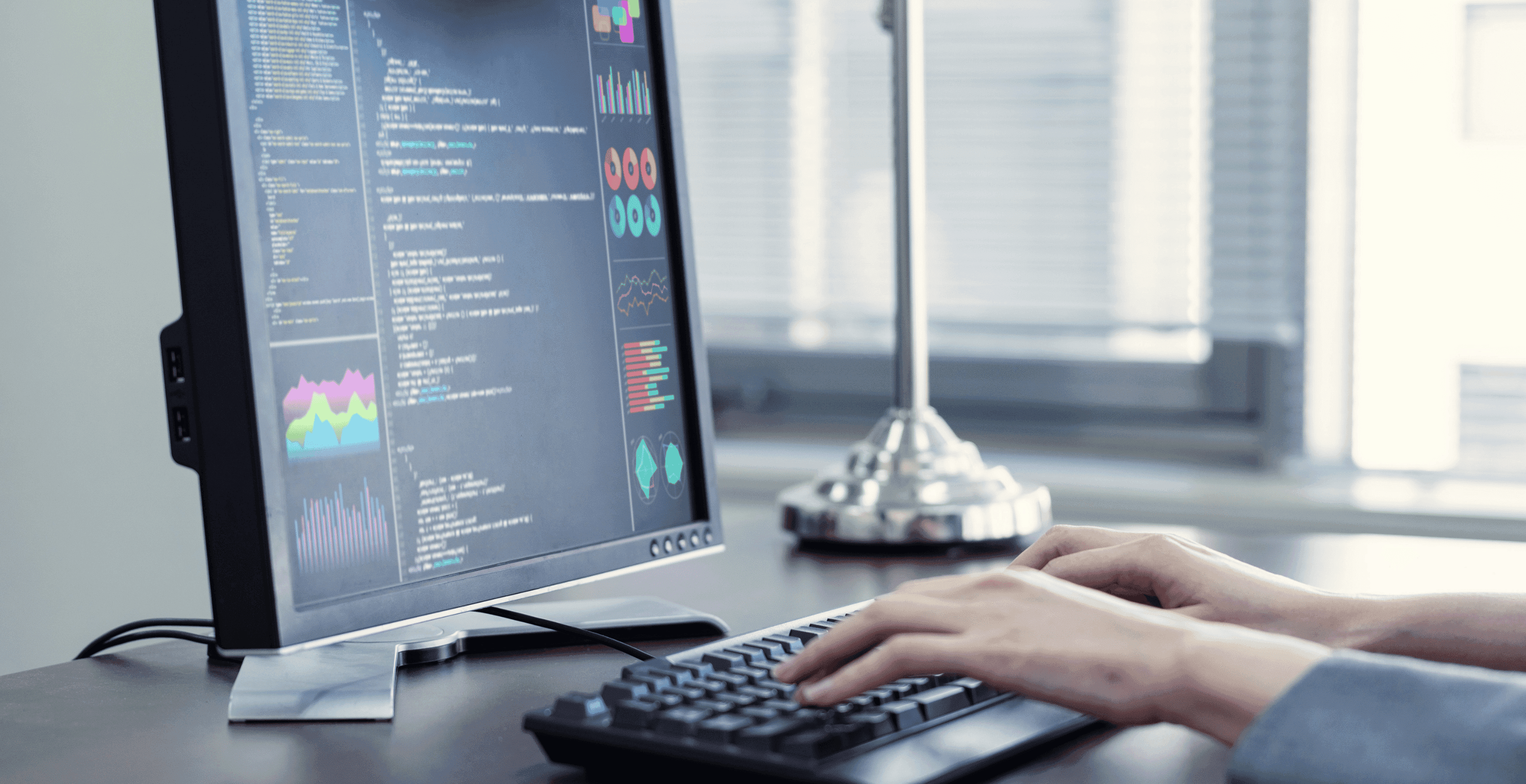
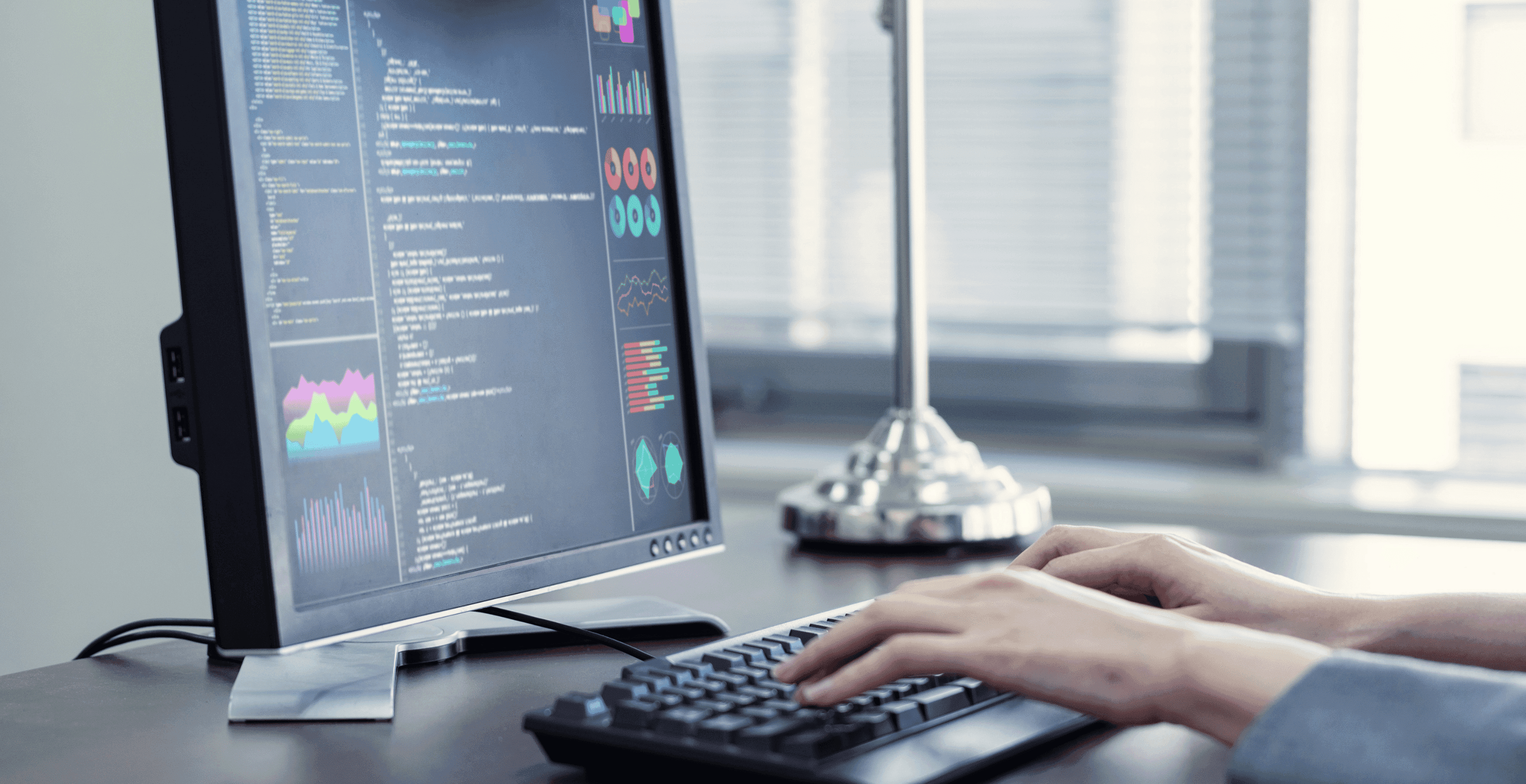
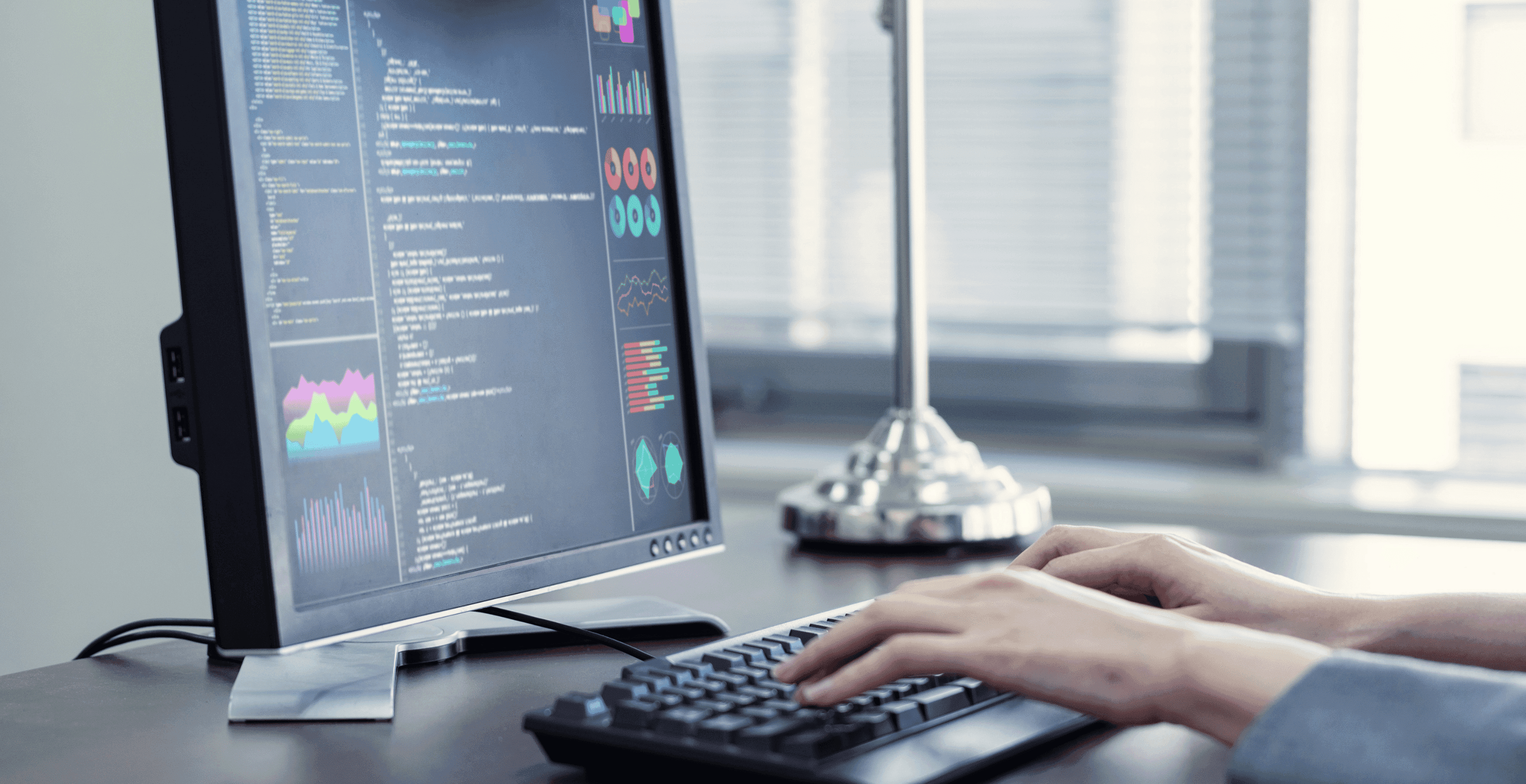
Guide on Unified Multi-Dimensional LLM Evaluation and Benchmark Metrics

Rehan Asif
Jul 1, 2024
Read the article




Innovations In AI For Healthcare
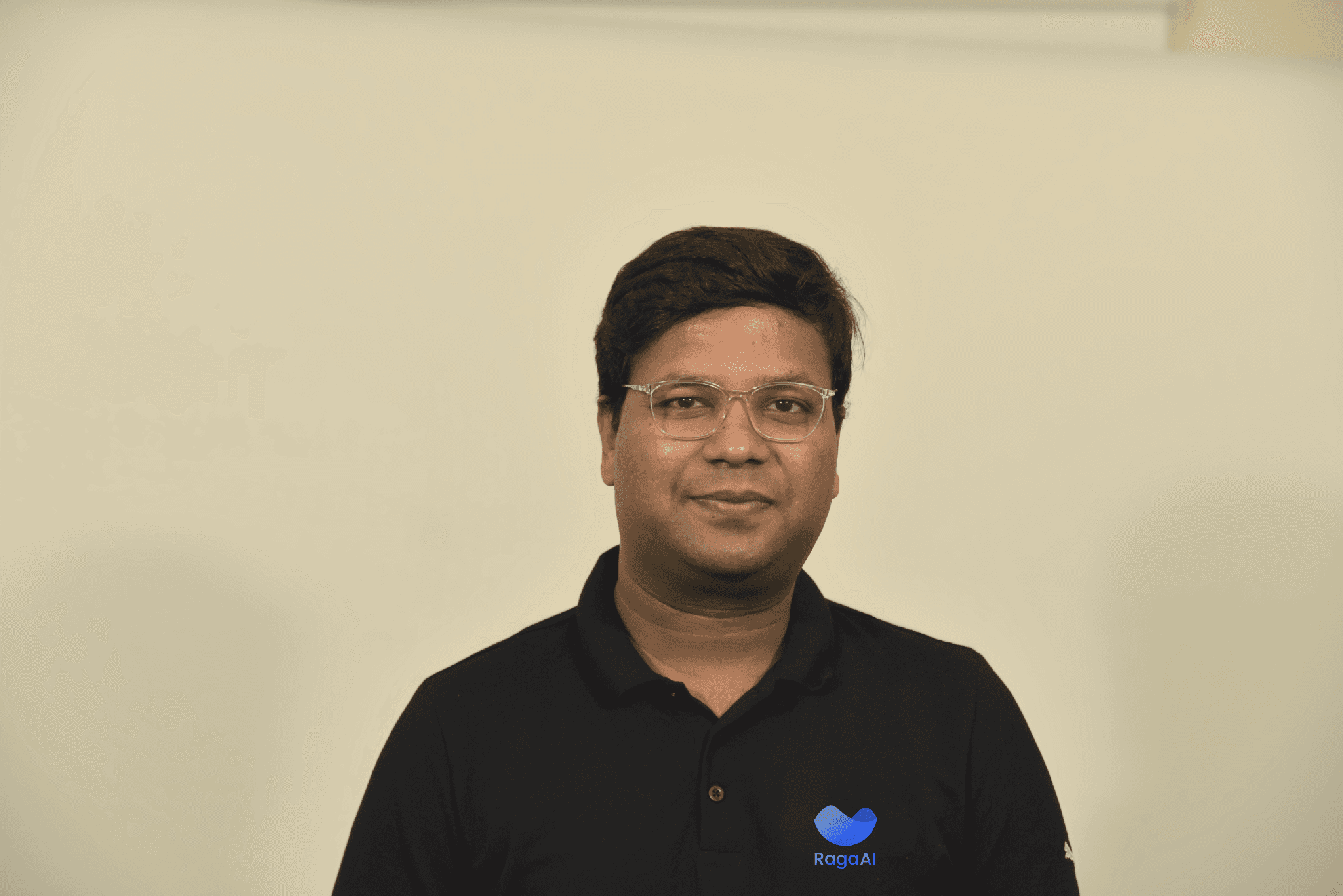
Jigar Gupta
Jun 24, 2024
Read the article




Implementing AI-Driven Inventory Management For The Retail Industry
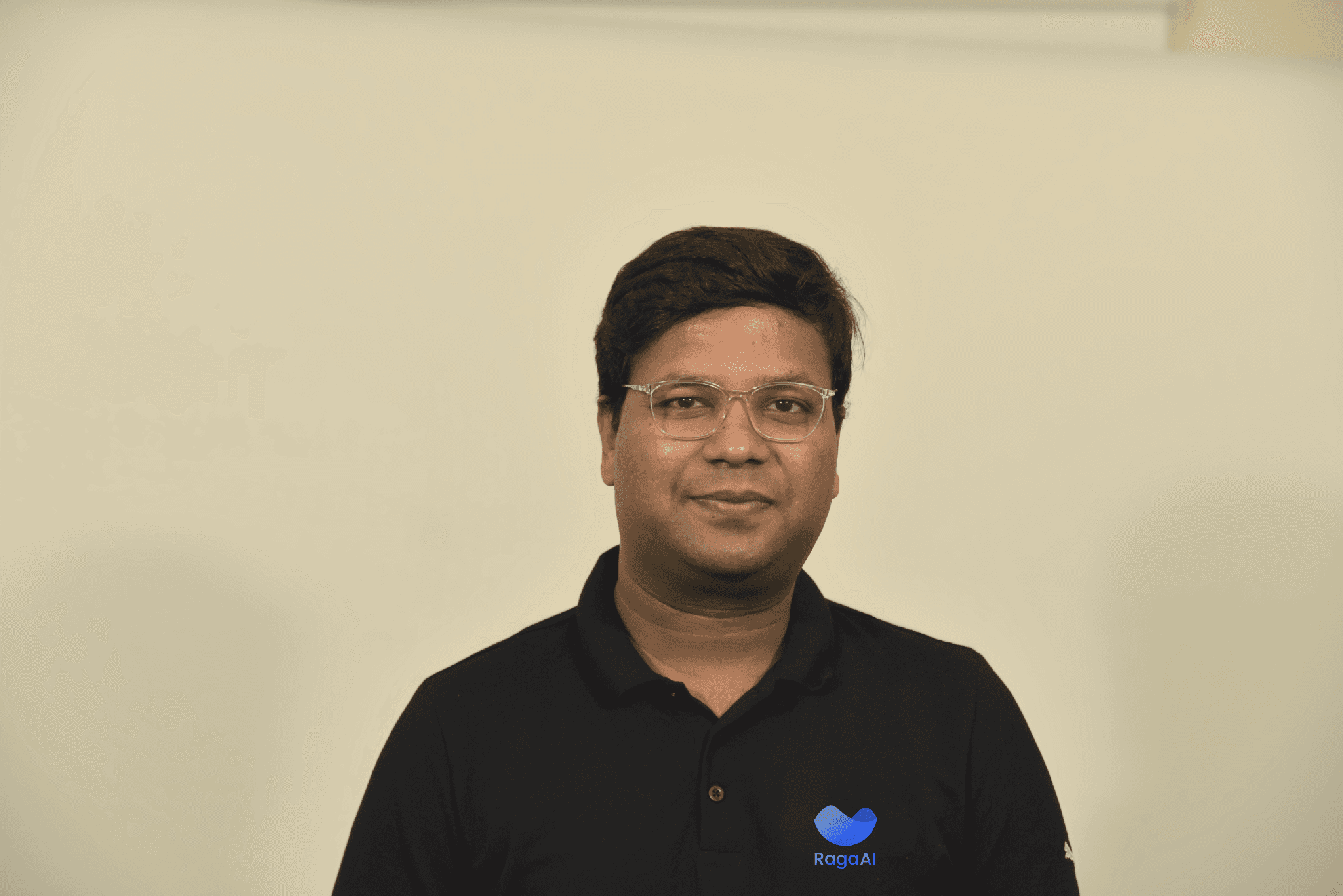
Jigar Gupta
Jun 24, 2024
Read the article




Practical Retrieval Augmented Generation: Use Cases And Impact
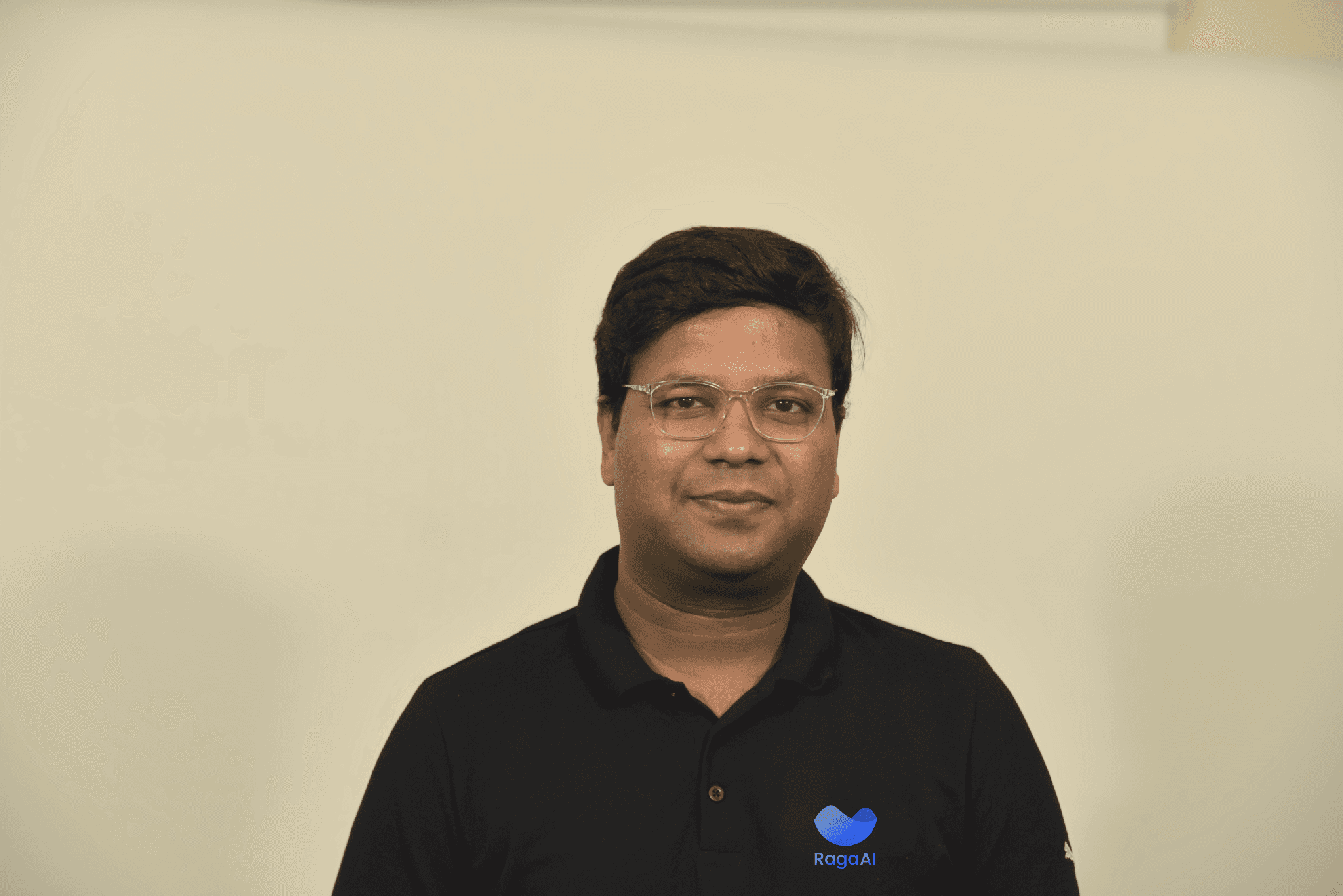
Jigar Gupta
Jun 24, 2024
Read the article




LLM Pre-Training and Fine-Tuning Differences

Rehan Asif
Jun 23, 2024
Read the article




20 LLM Project Ideas For Beginners Using Large Language Models

Rehan Asif
Jun 23, 2024
Read the article




Understanding LLM Parameters: Tuning Top-P, Temperature And Tokens

Rehan Asif
Jun 23, 2024
Read the article




Understanding Large Action Models In AI

Rehan Asif
Jun 23, 2024
Read the article




Building And Implementing Custom LLM Guardrails

Rehan Asif
Jun 12, 2024
Read the article




Understanding LLM Alignment: A Simple Guide

Rehan Asif
Jun 12, 2024
Read the article
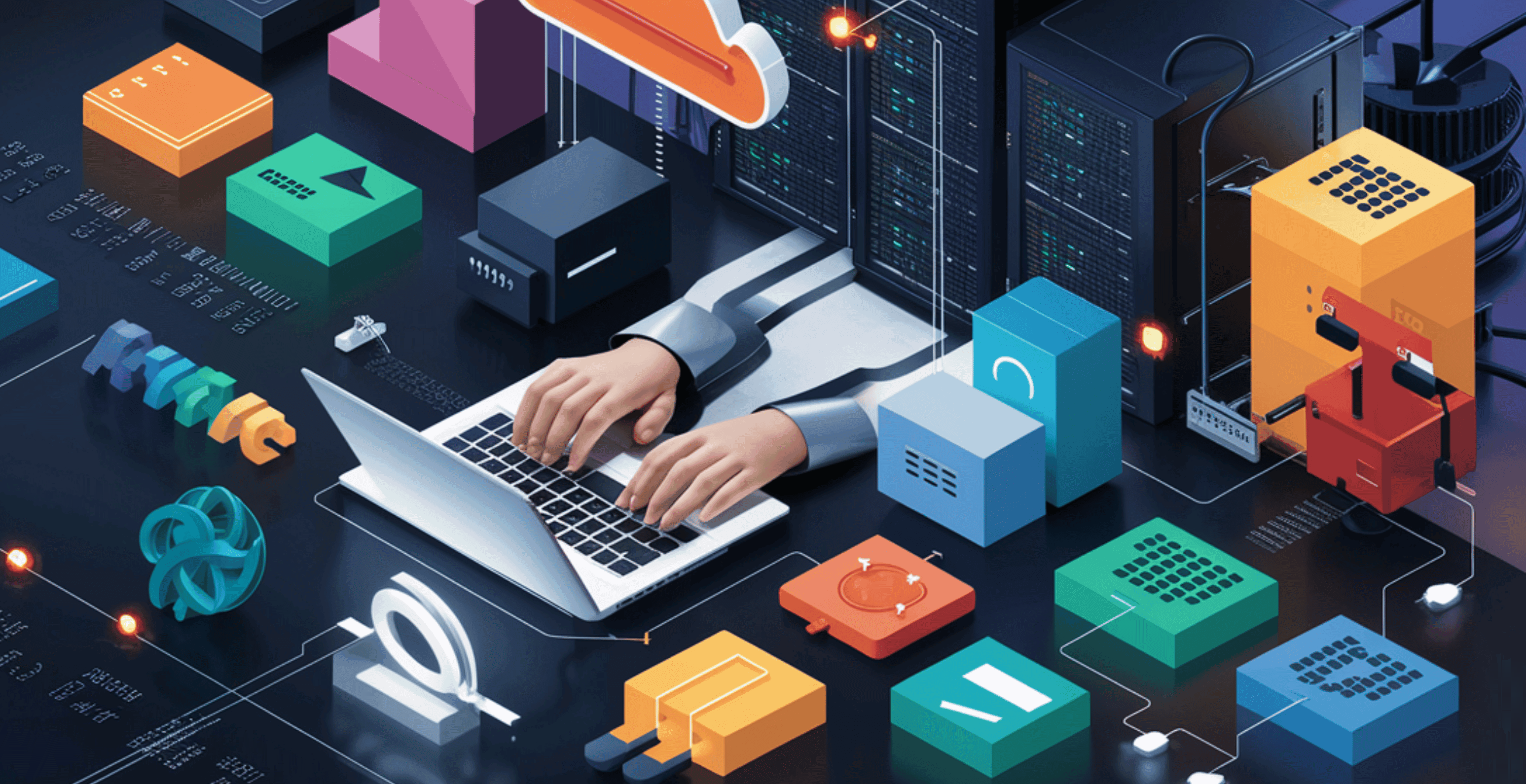
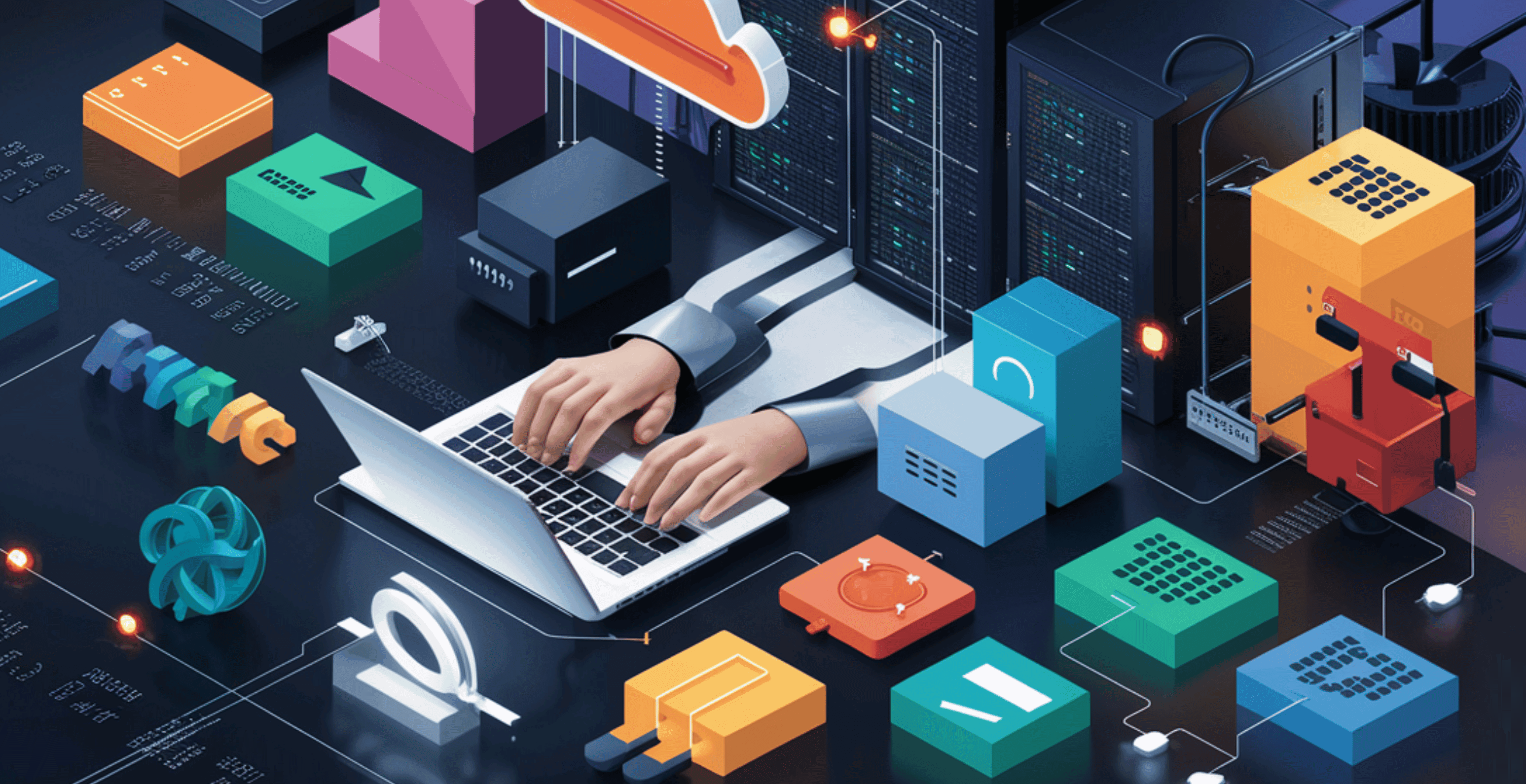
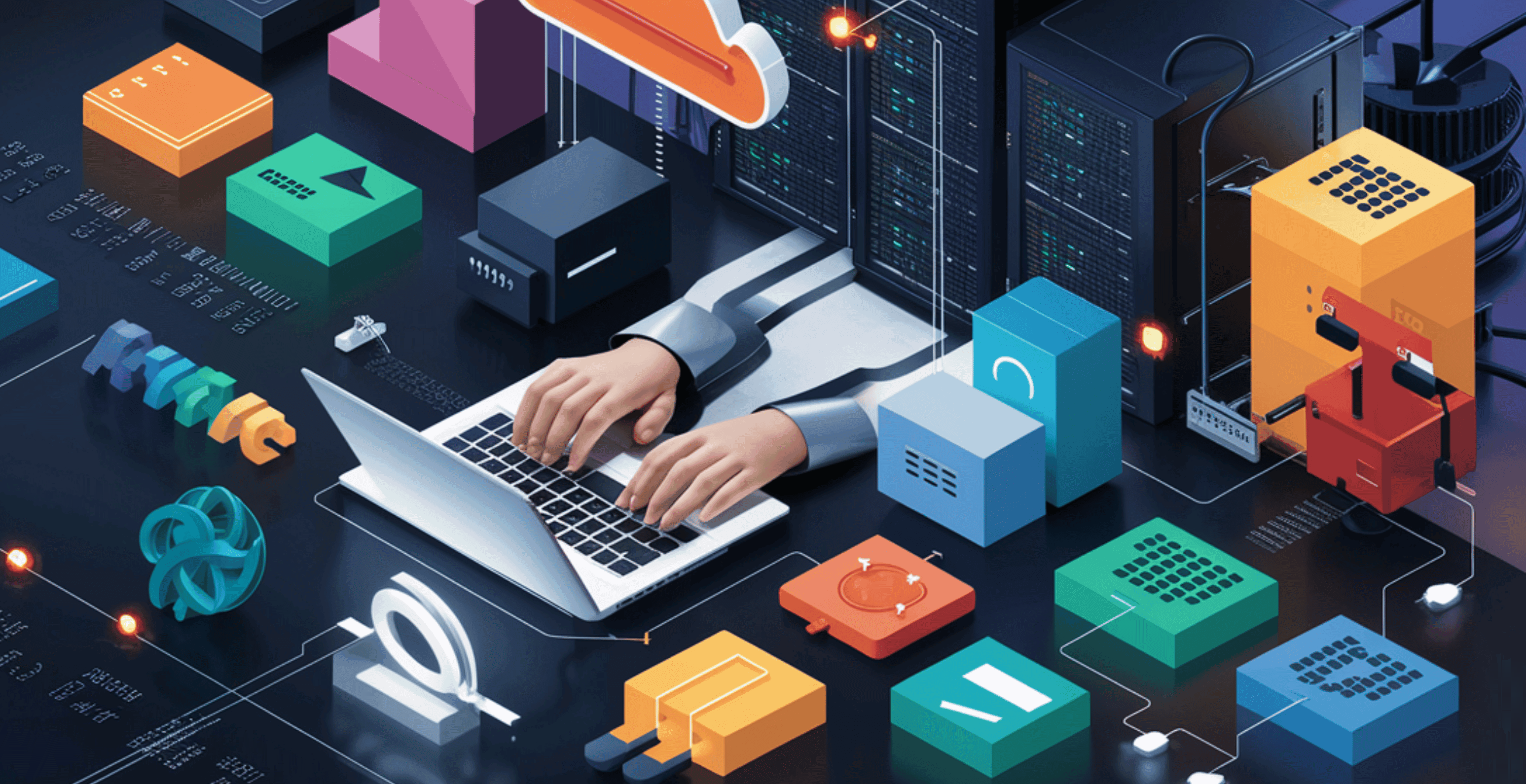
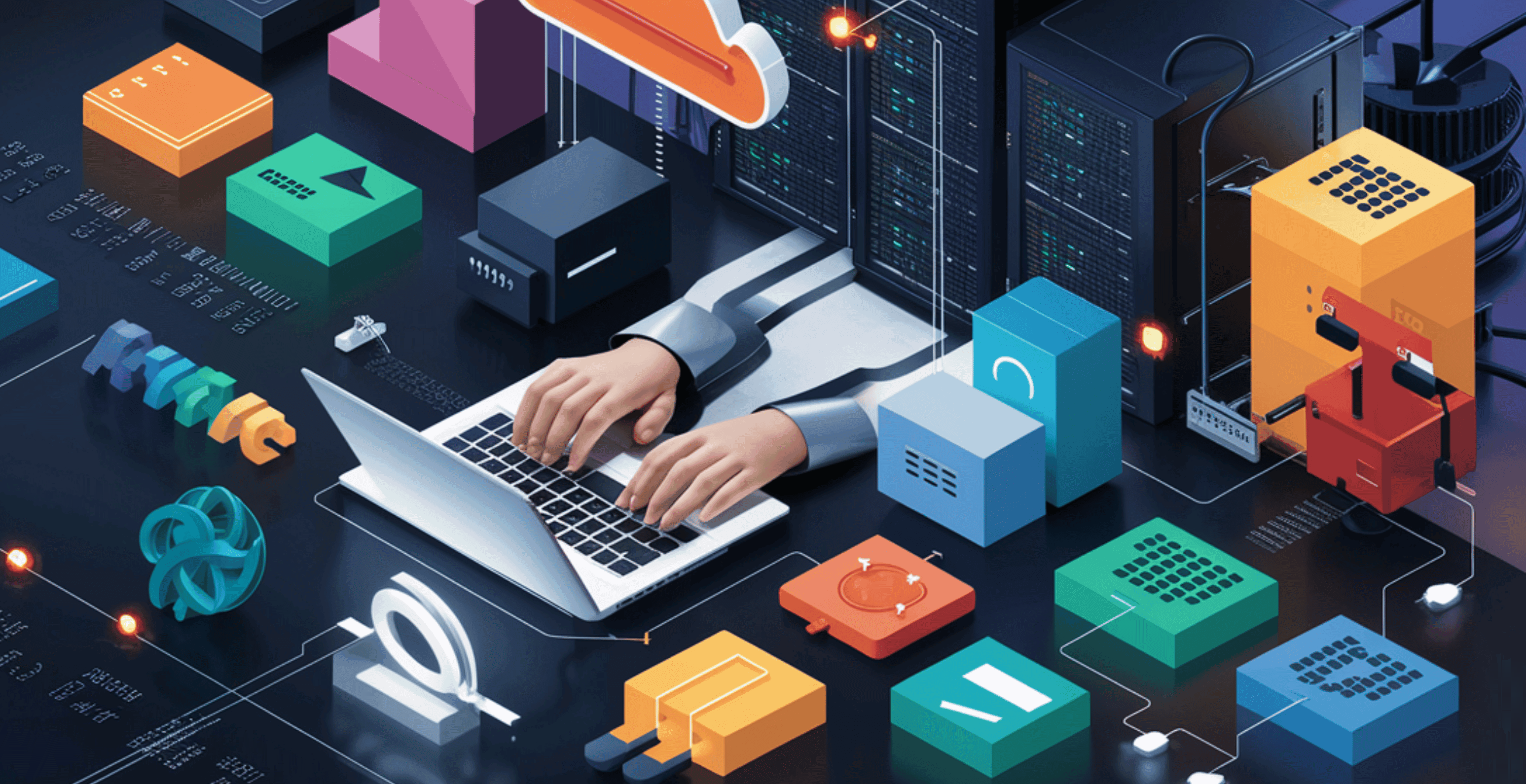
Practical Strategies For Self-Hosting Large Language Models

Rehan Asif
Jun 12, 2024
Read the article
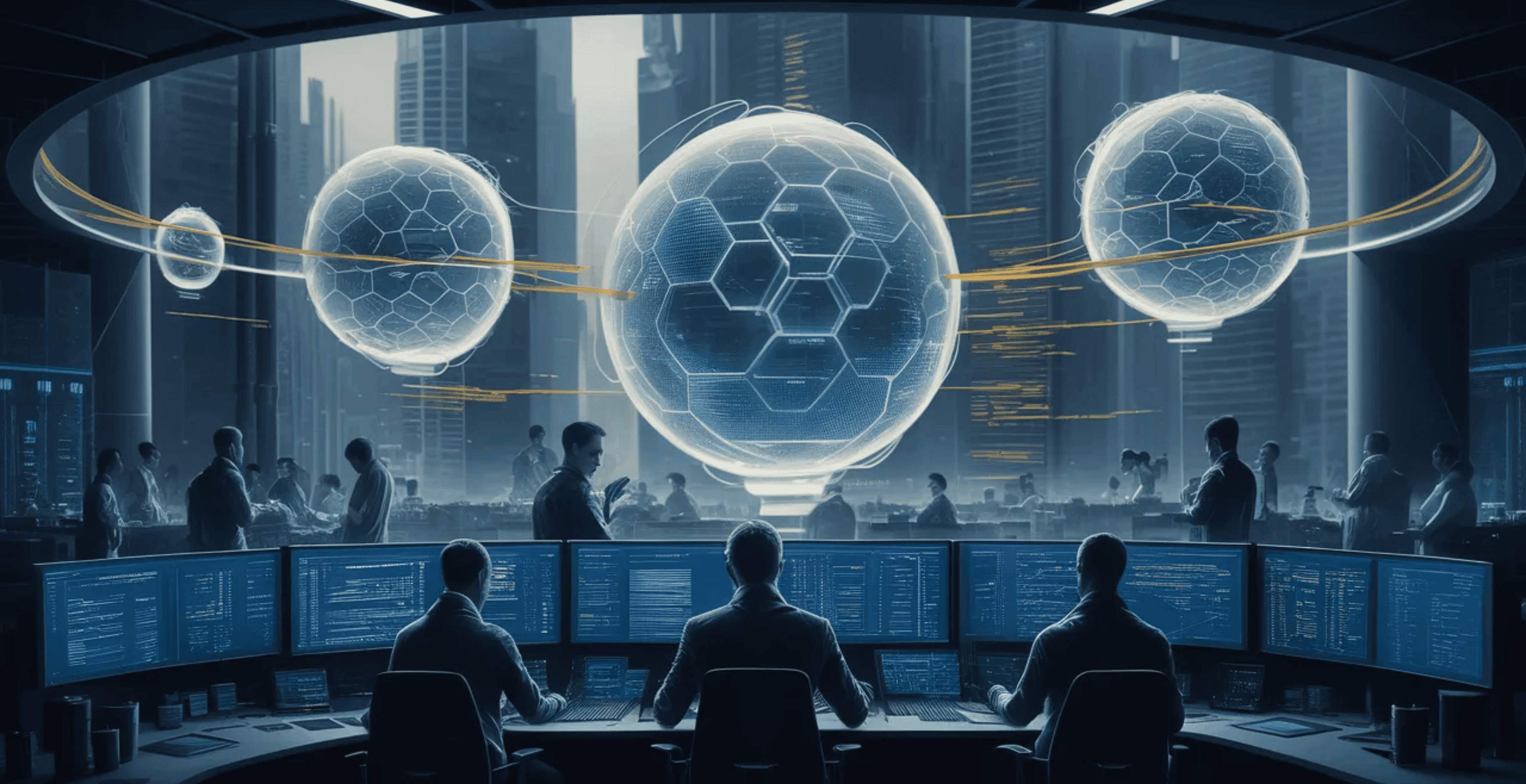
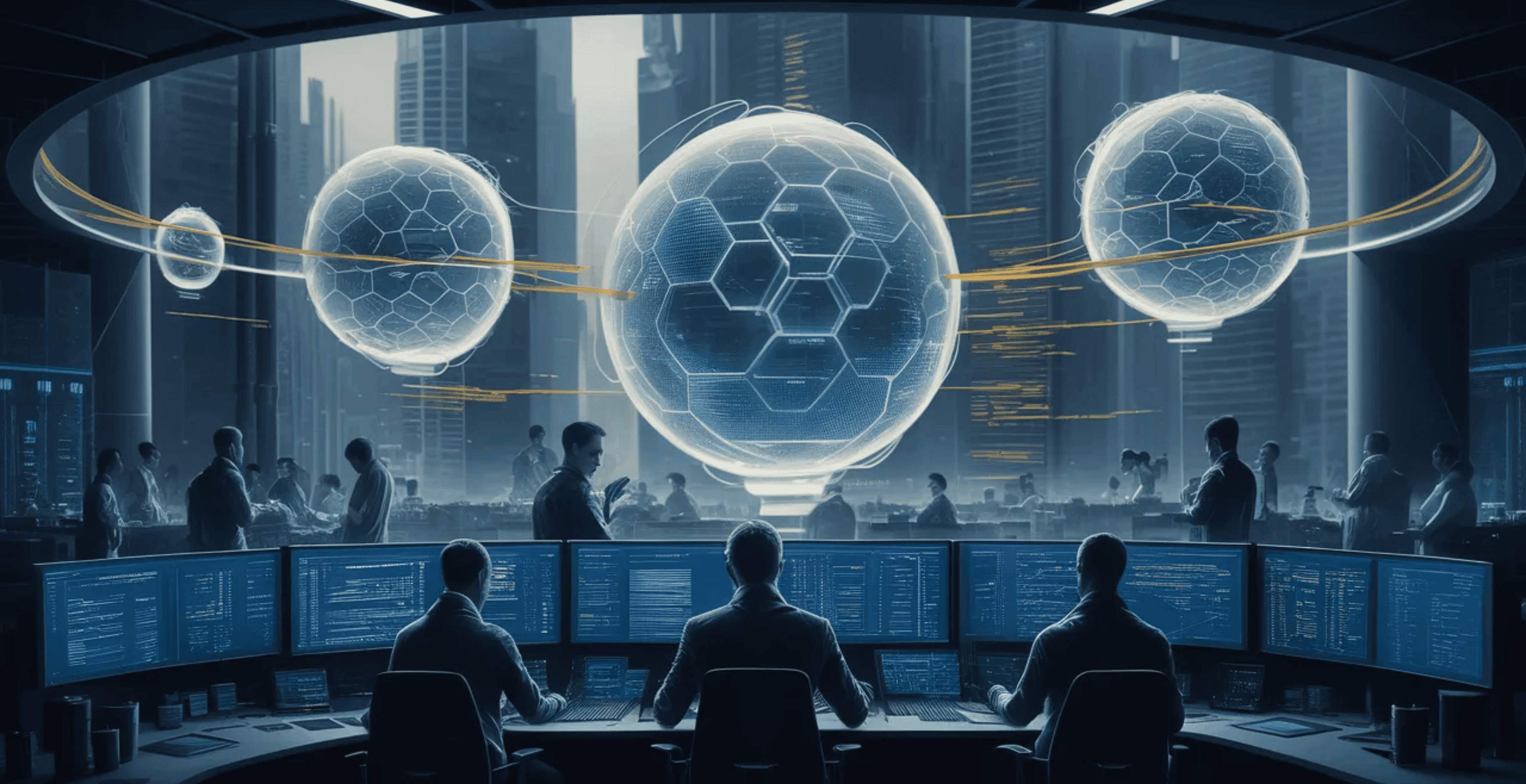
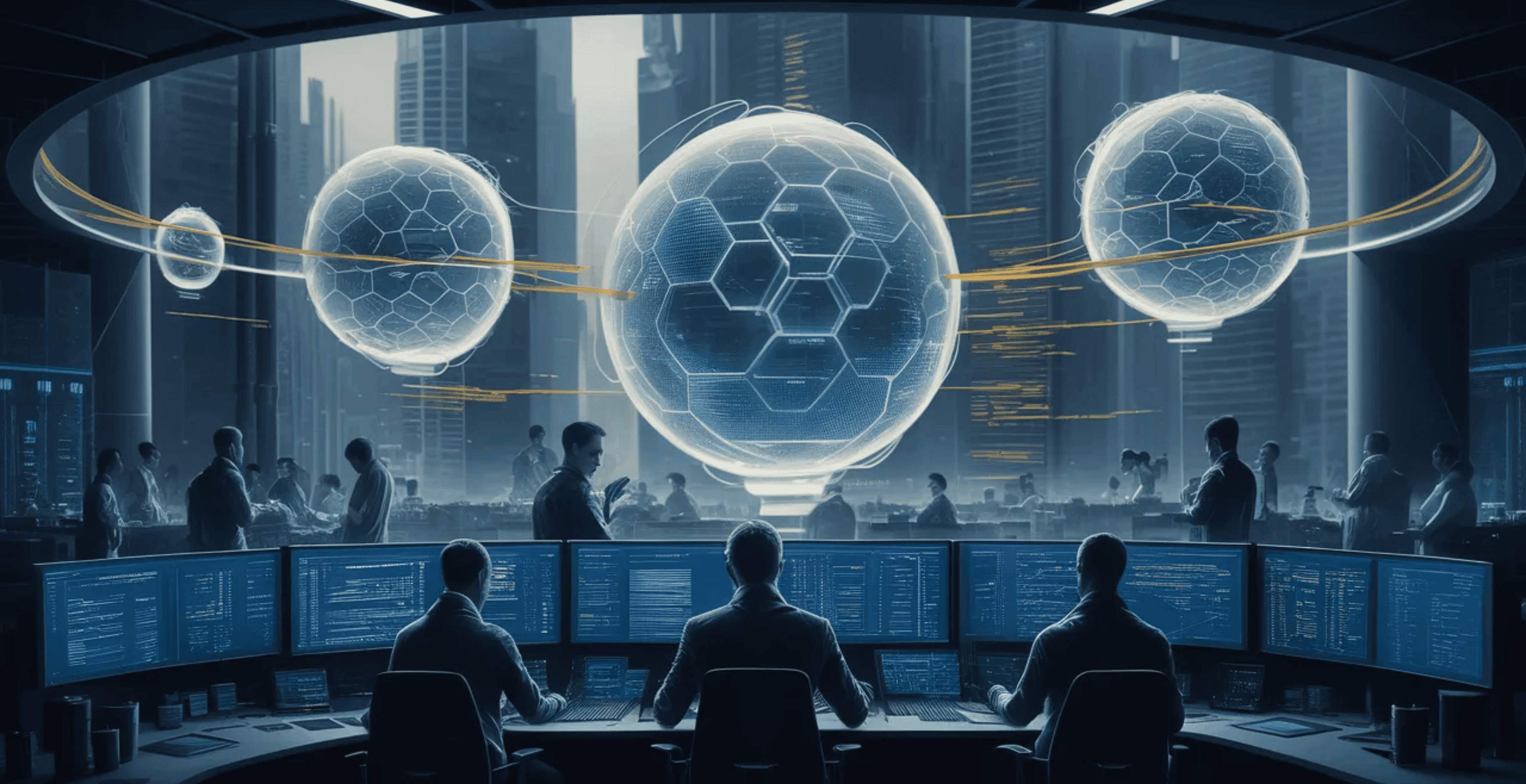
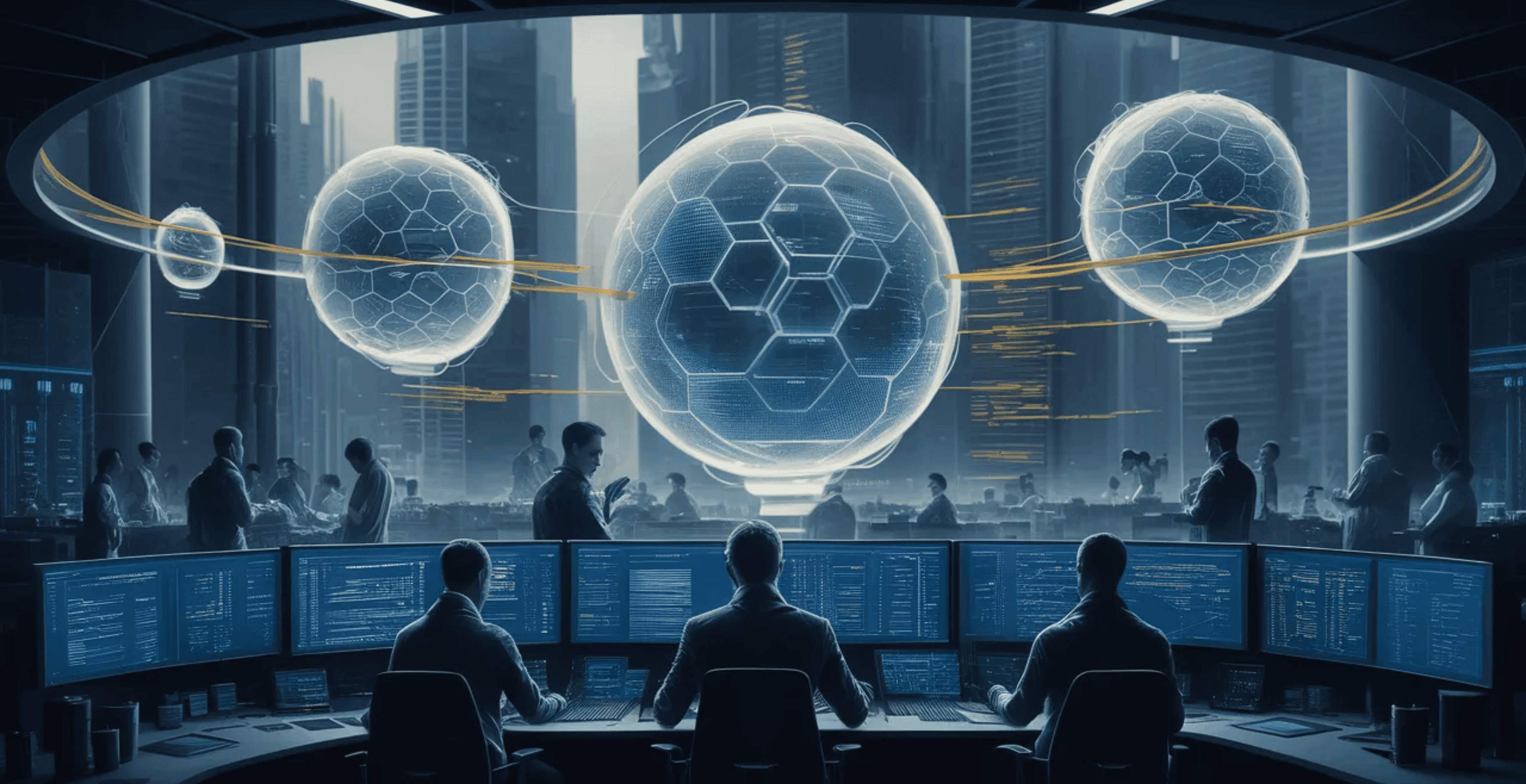
Practical Guide For Deploying LLMs In Production

Rehan Asif
Jun 12, 2024
Read the article
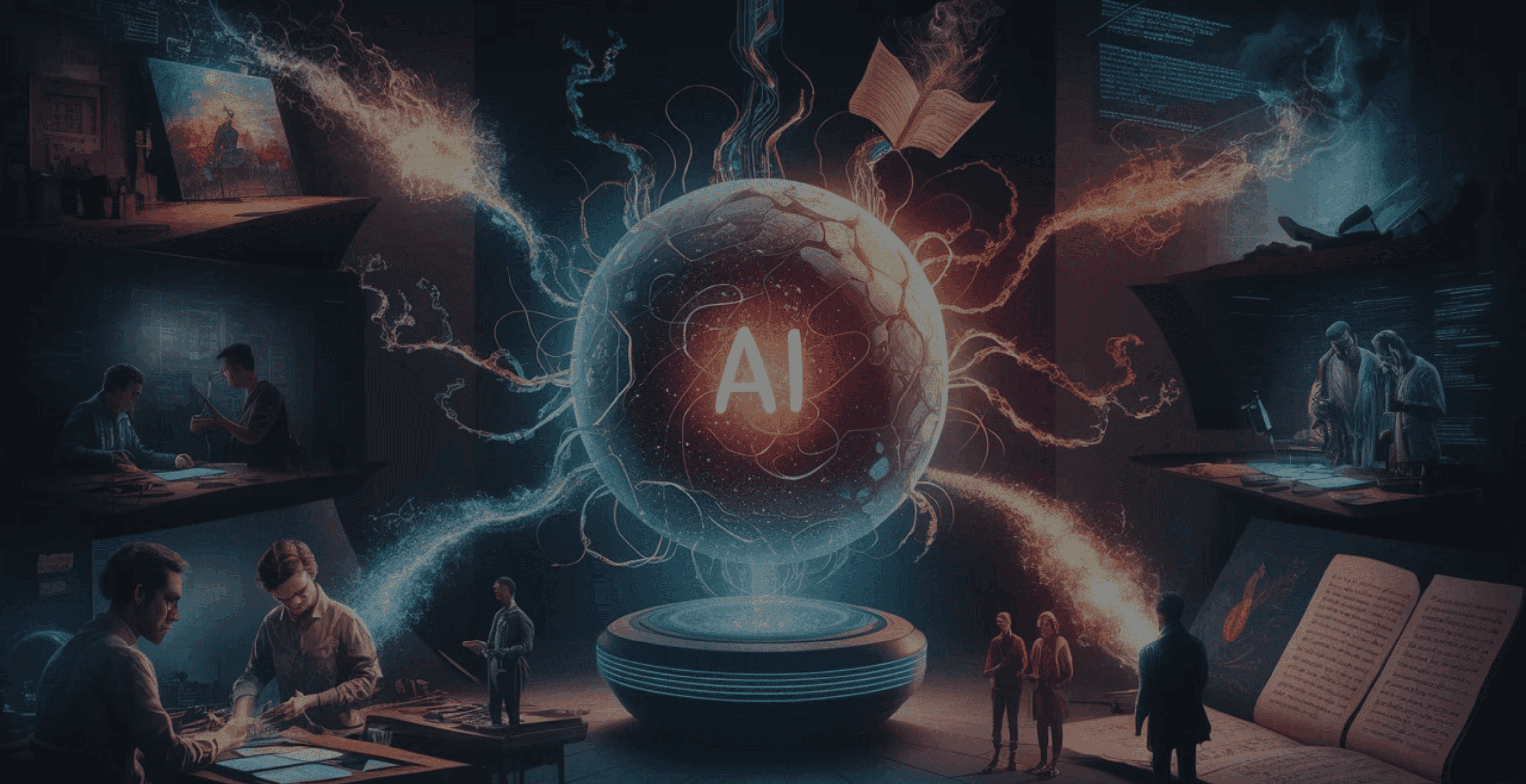
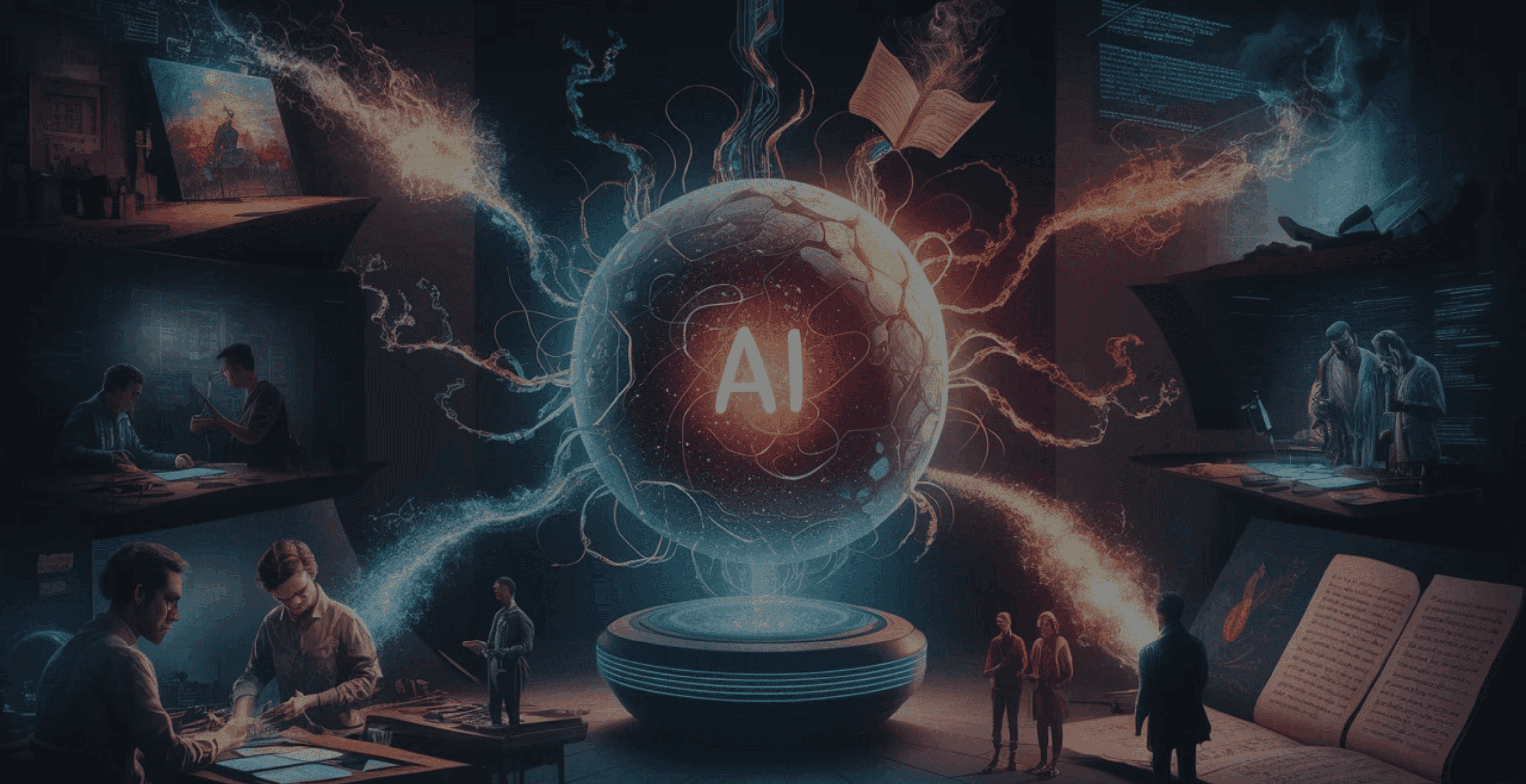
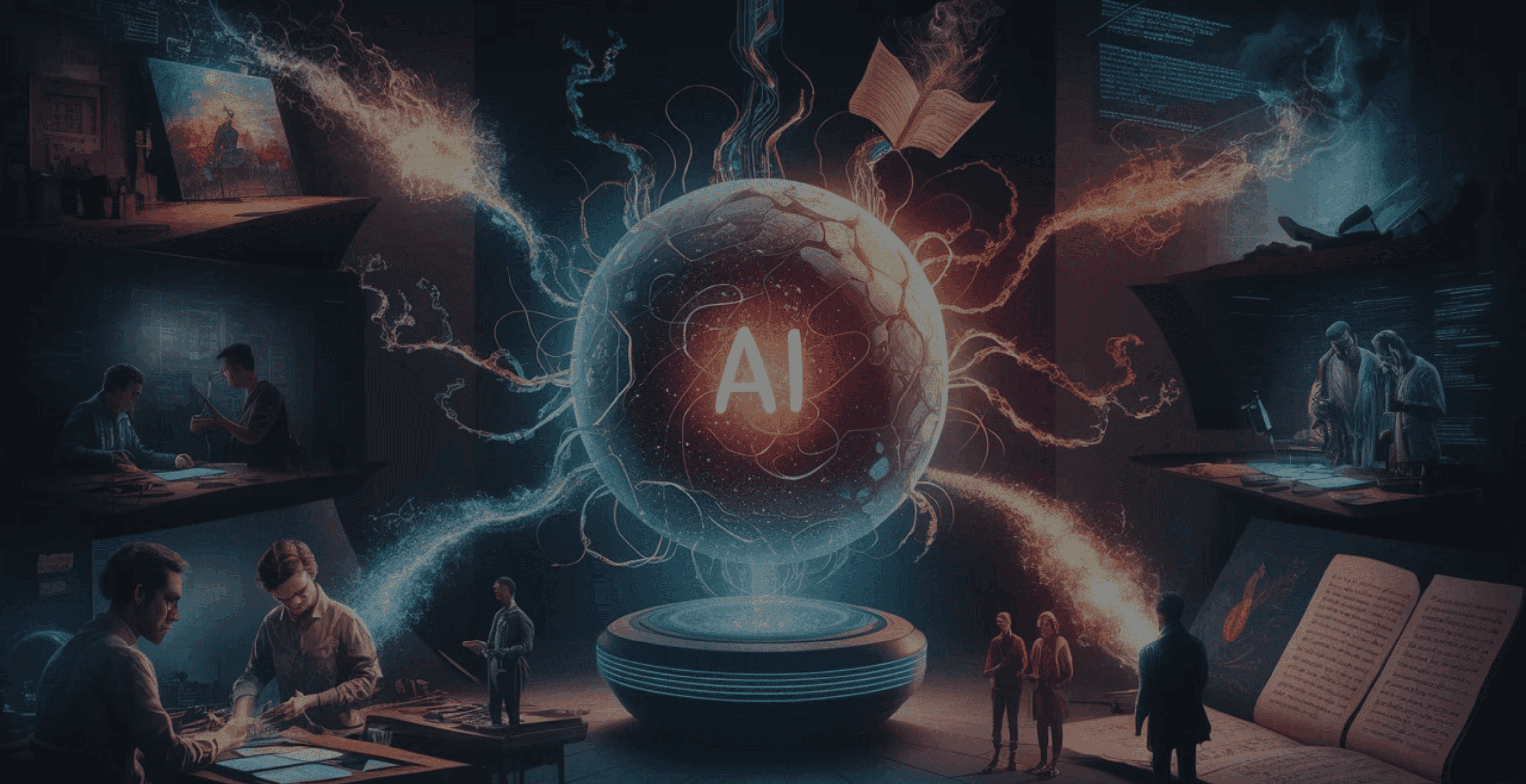
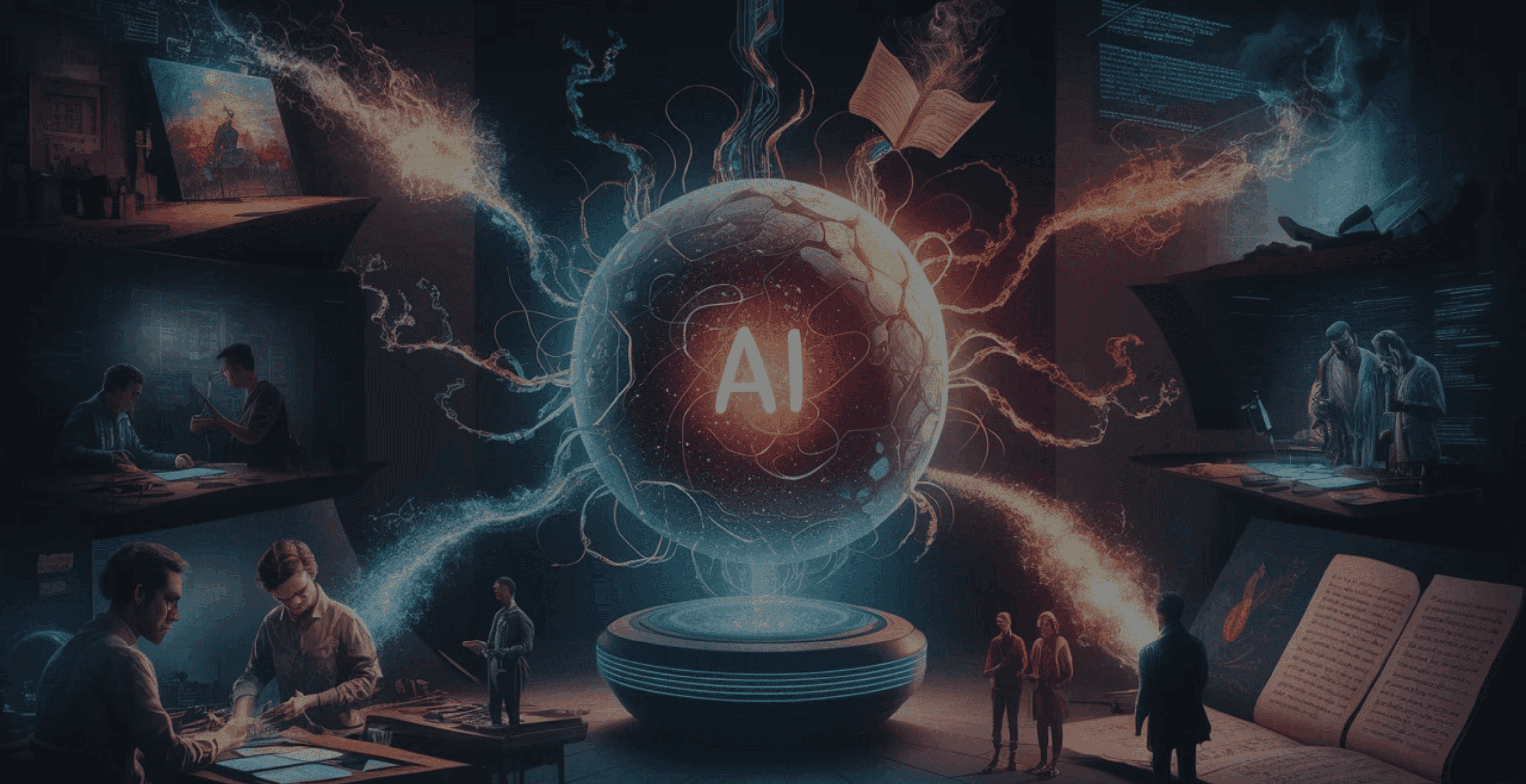
The Impact Of Generative Models On Content Creation
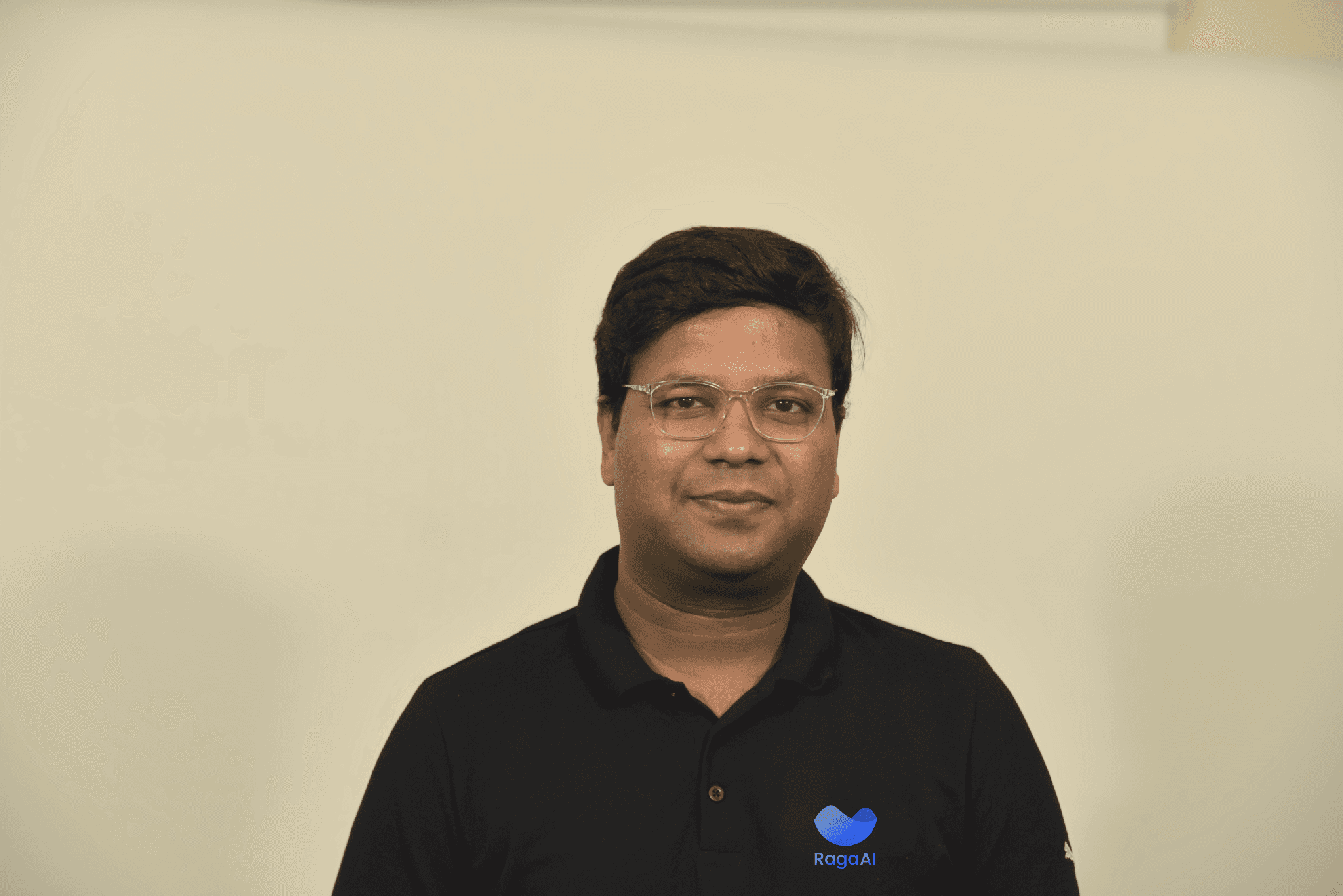
Jigar Gupta
Jun 12, 2024
Read the article




Implementing Regression Tests In AI Development
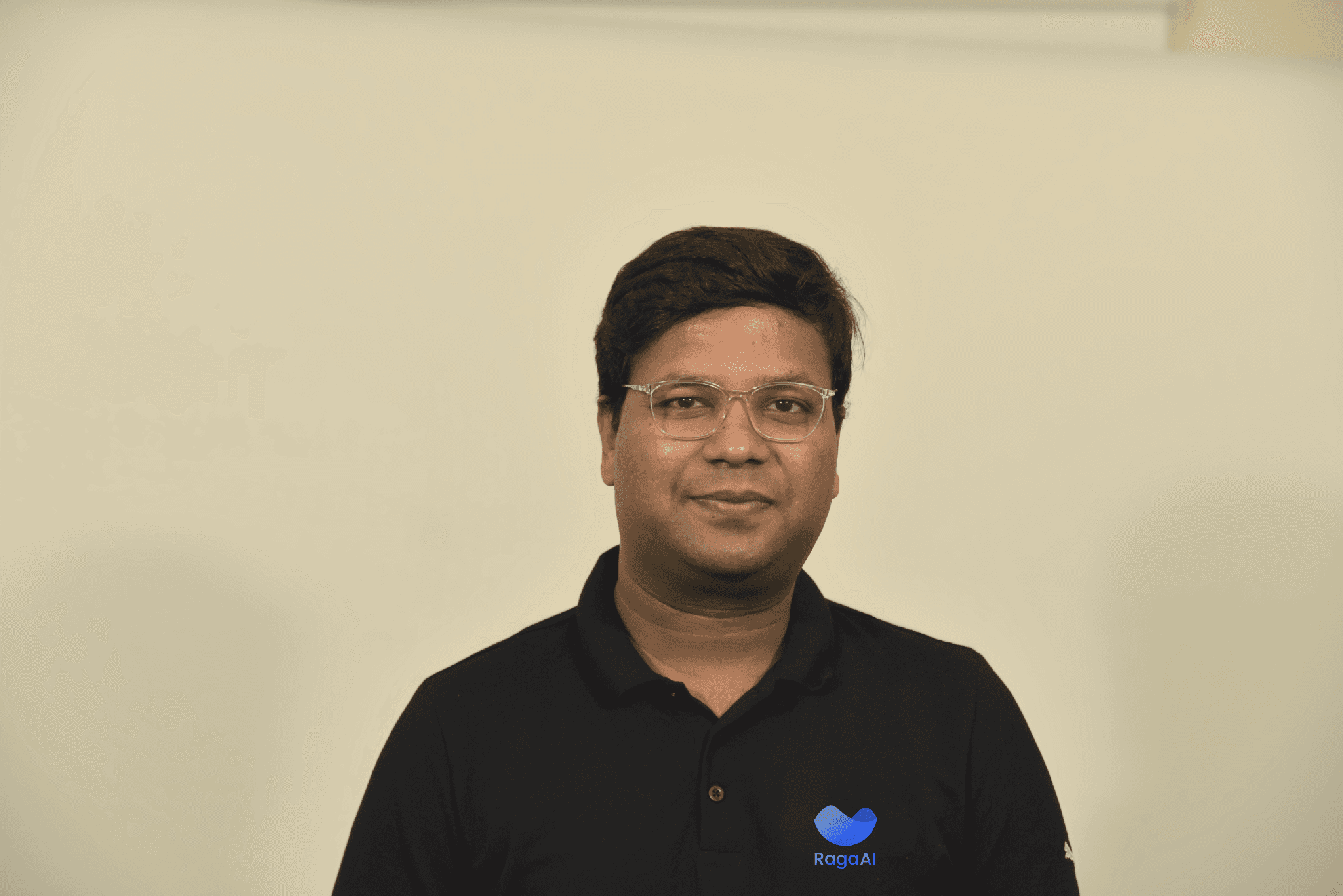
Jigar Gupta
Jun 12, 2024
Read the article
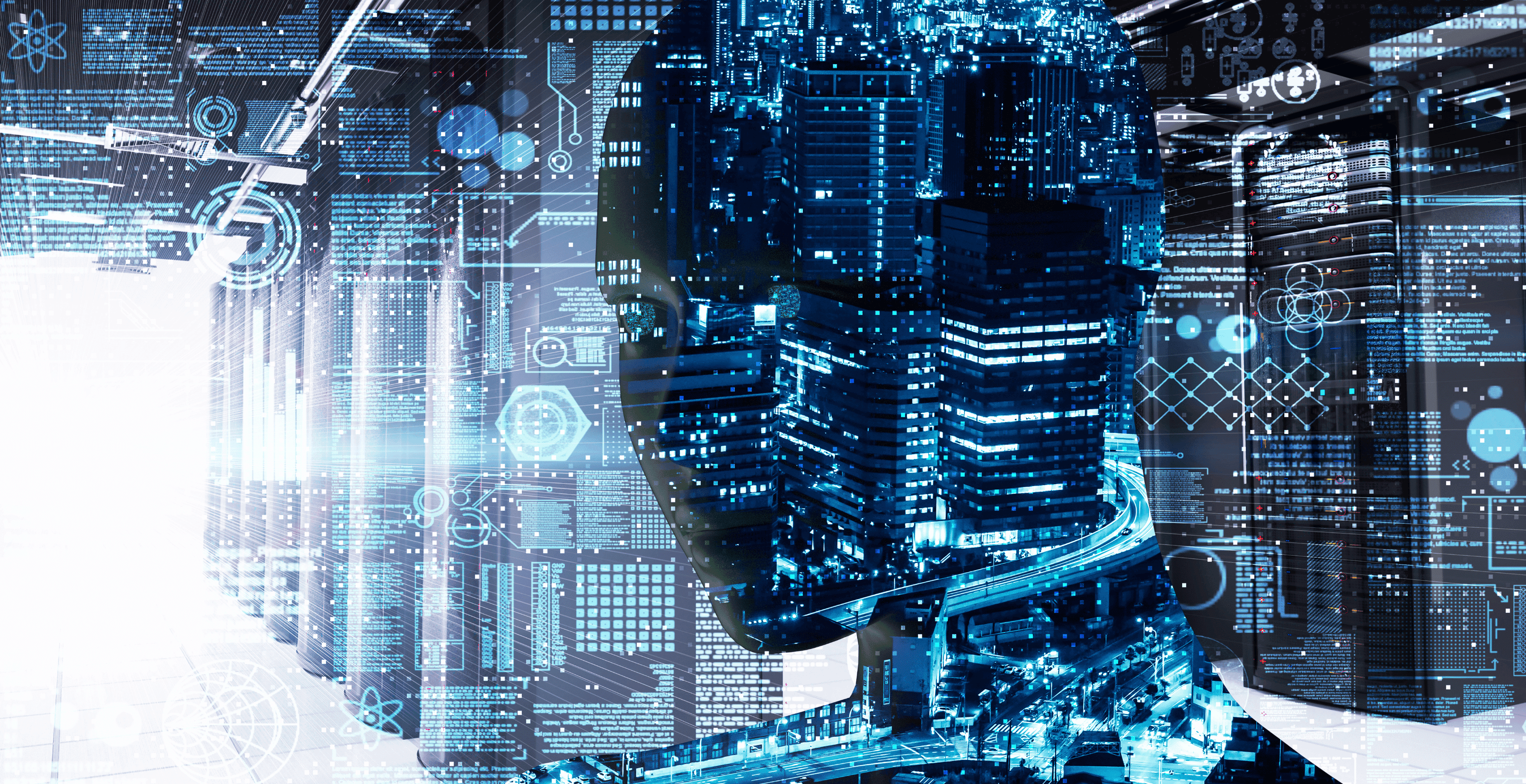
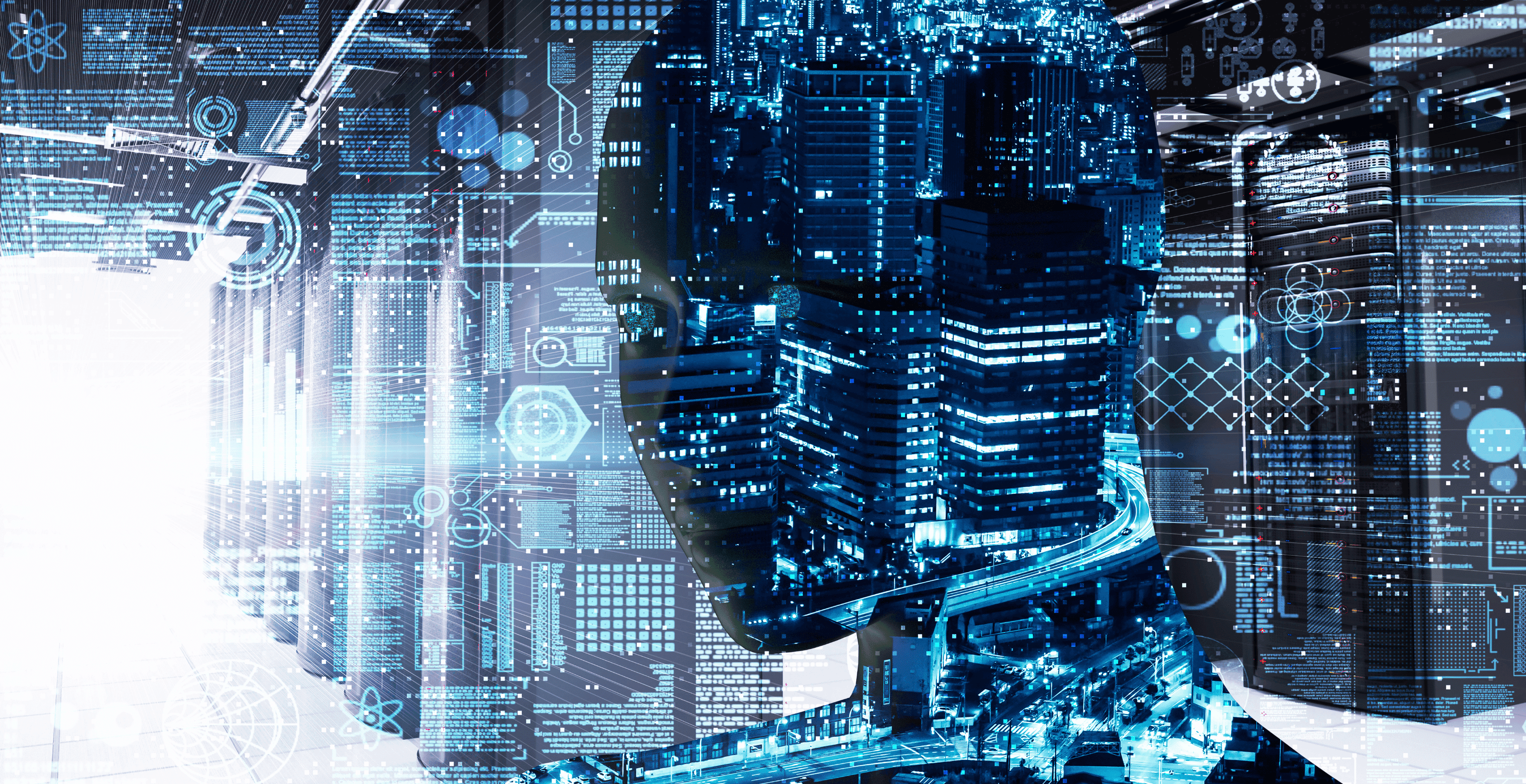
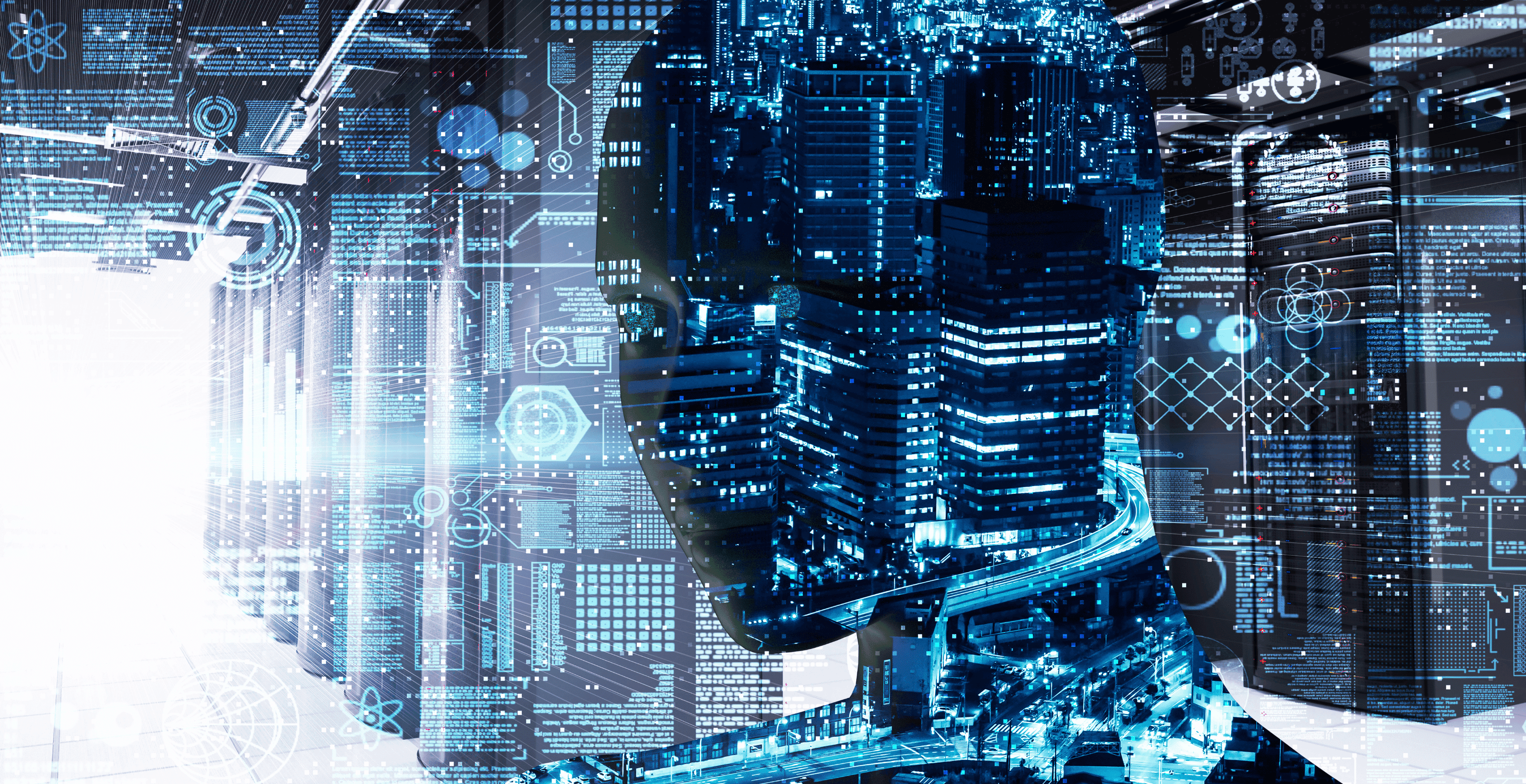
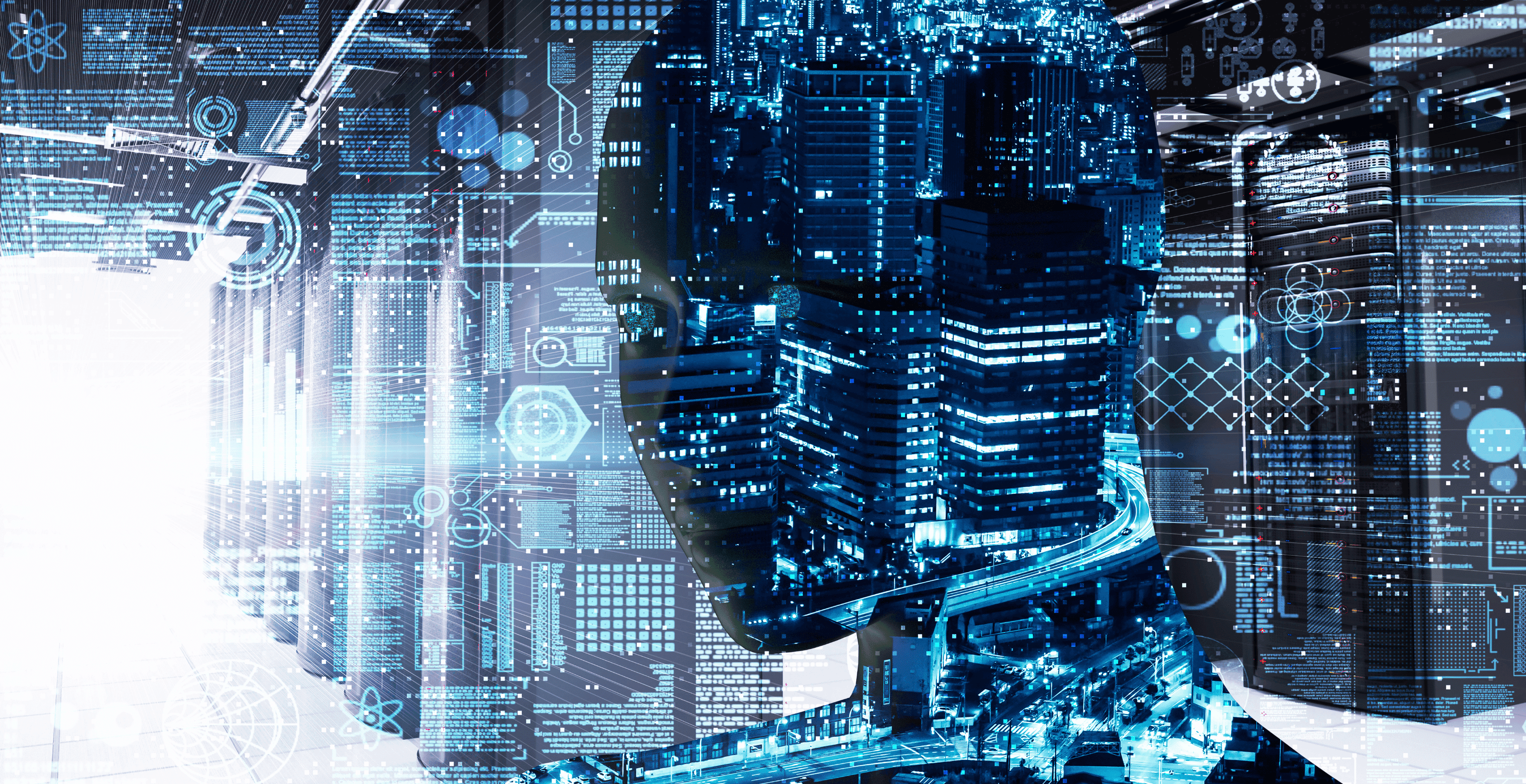
In-Depth Case Studies in AI Model Testing: Exploring Real-World Applications and Insights
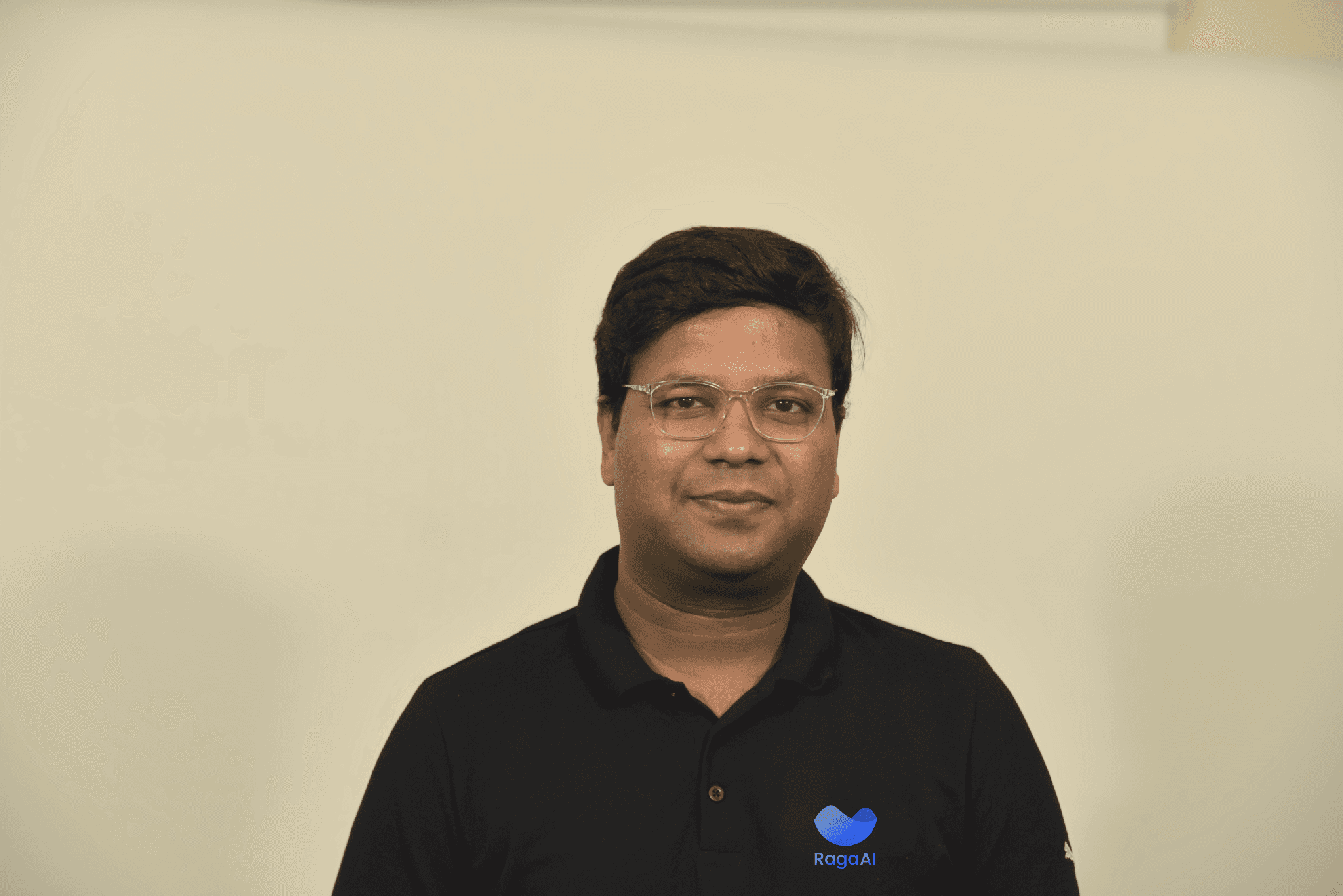
Jigar Gupta
Jun 11, 2024
Read the article




Techniques and Importance of Stress Testing AI Systems
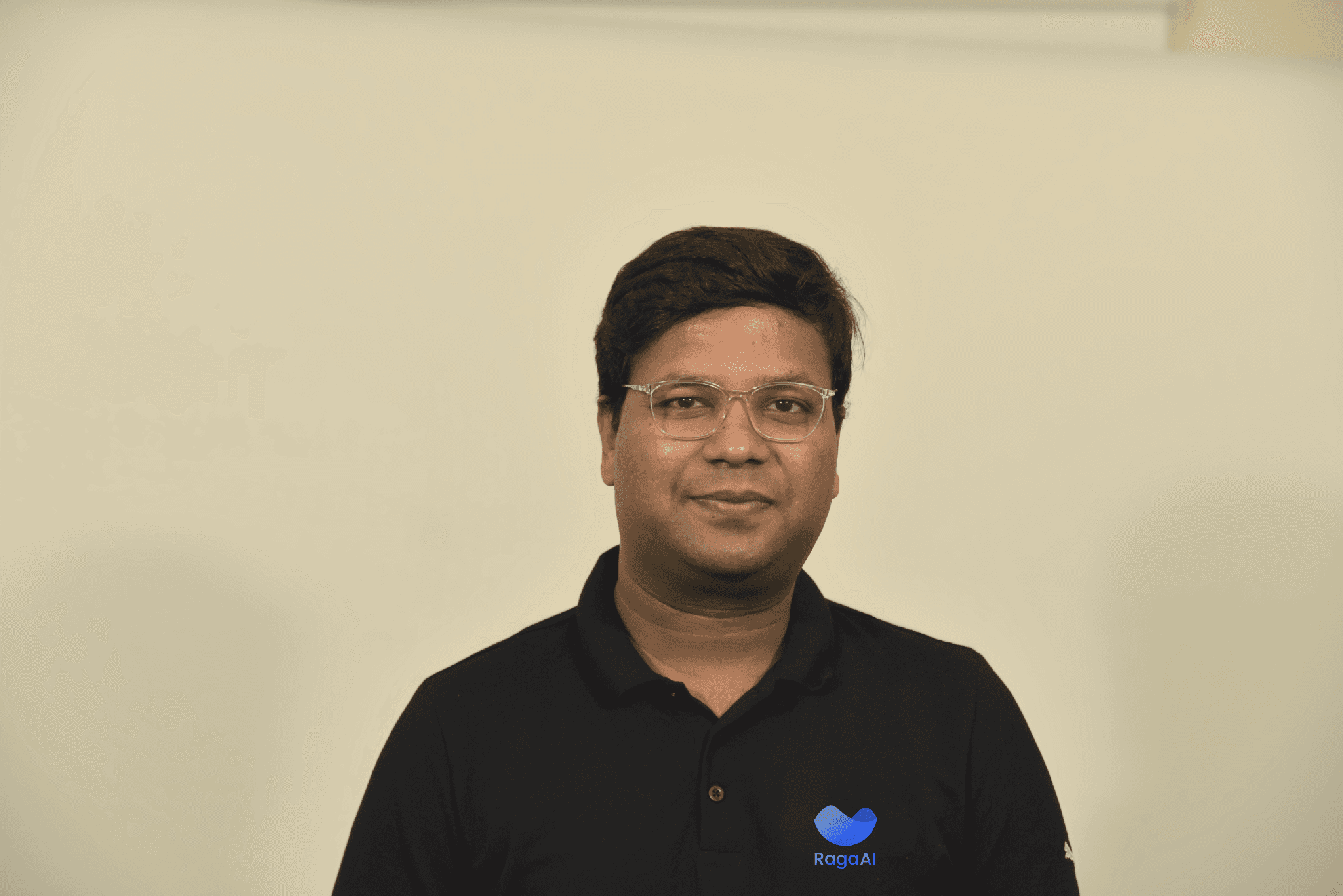
Jigar Gupta
Jun 11, 2024
Read the article
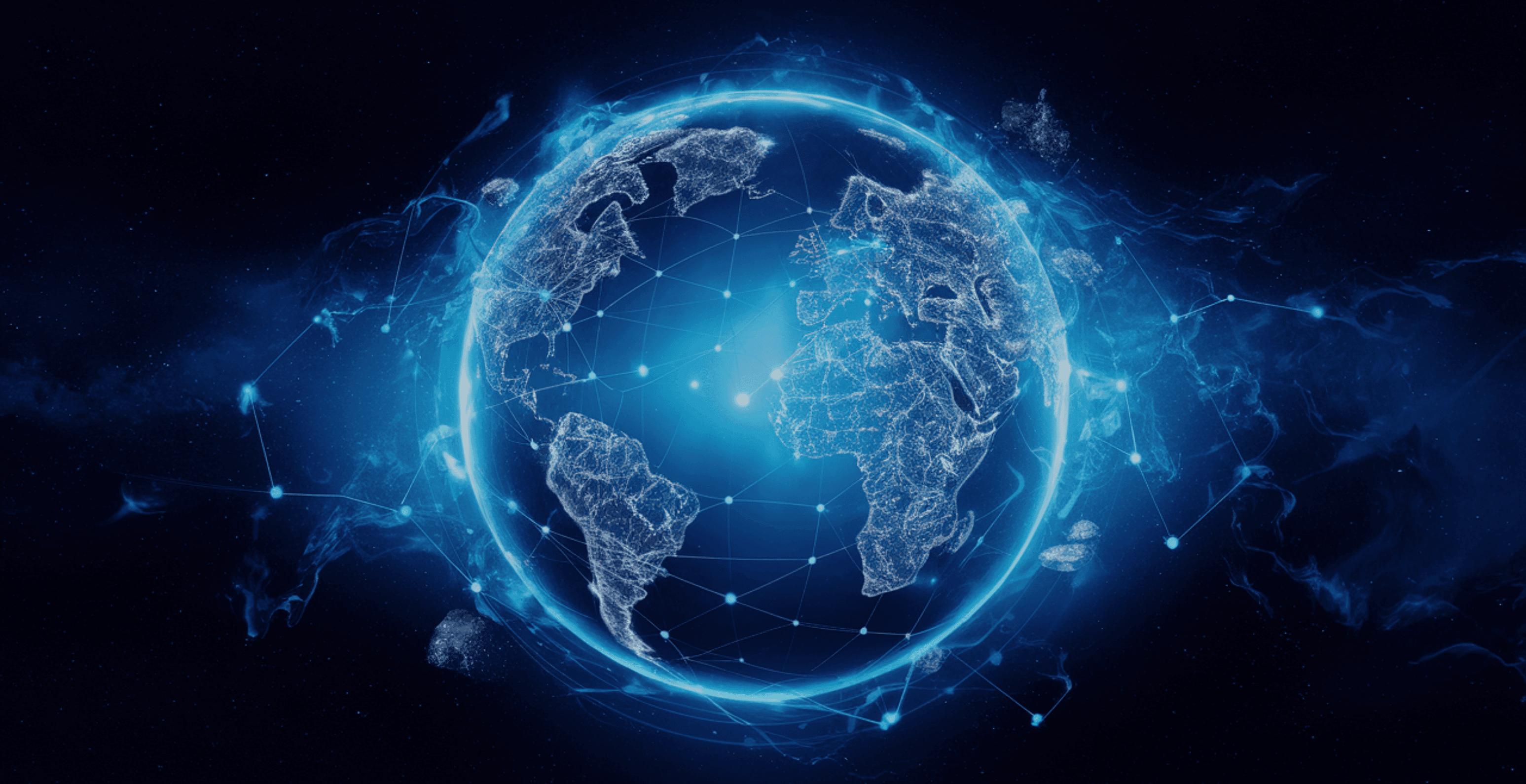
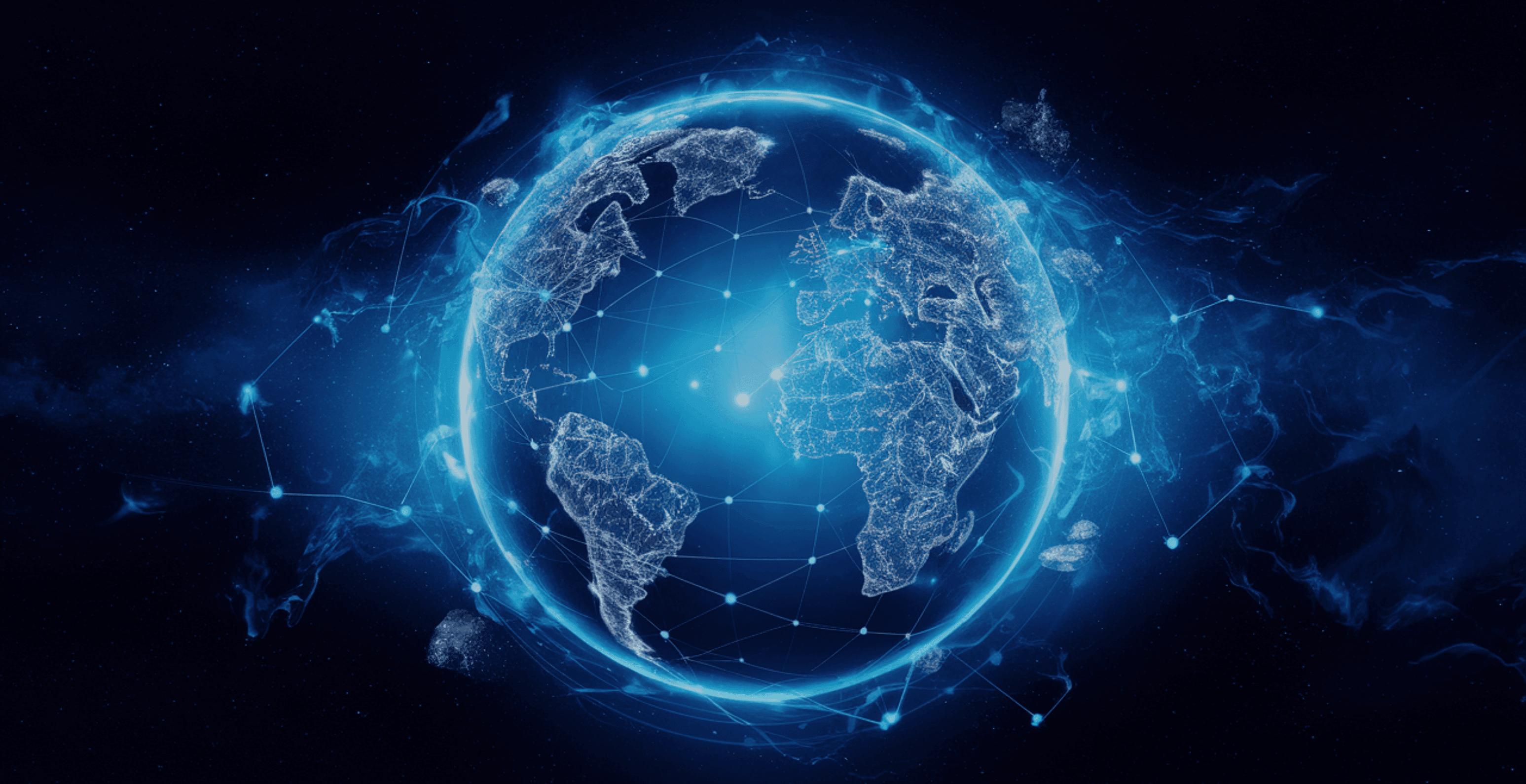
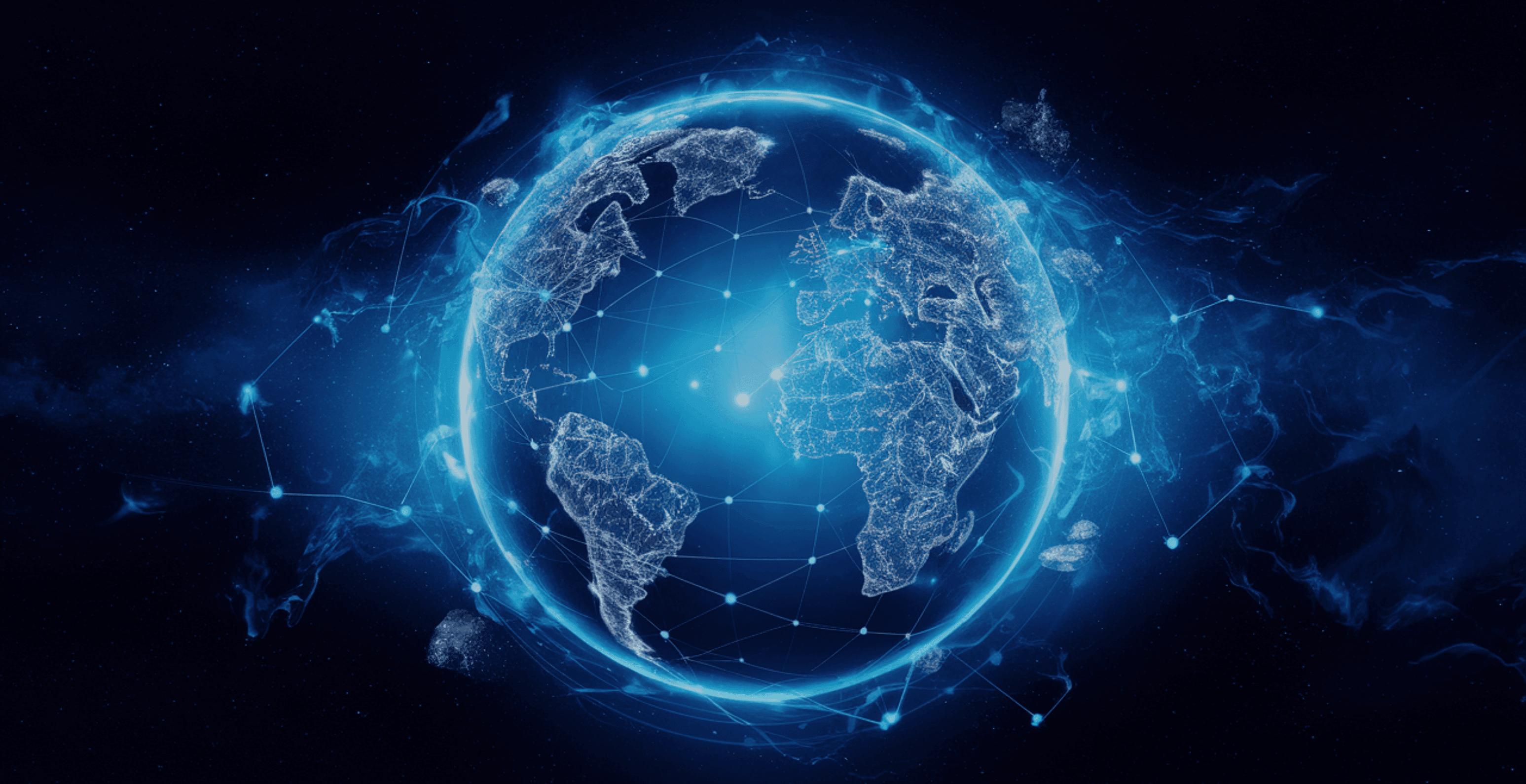
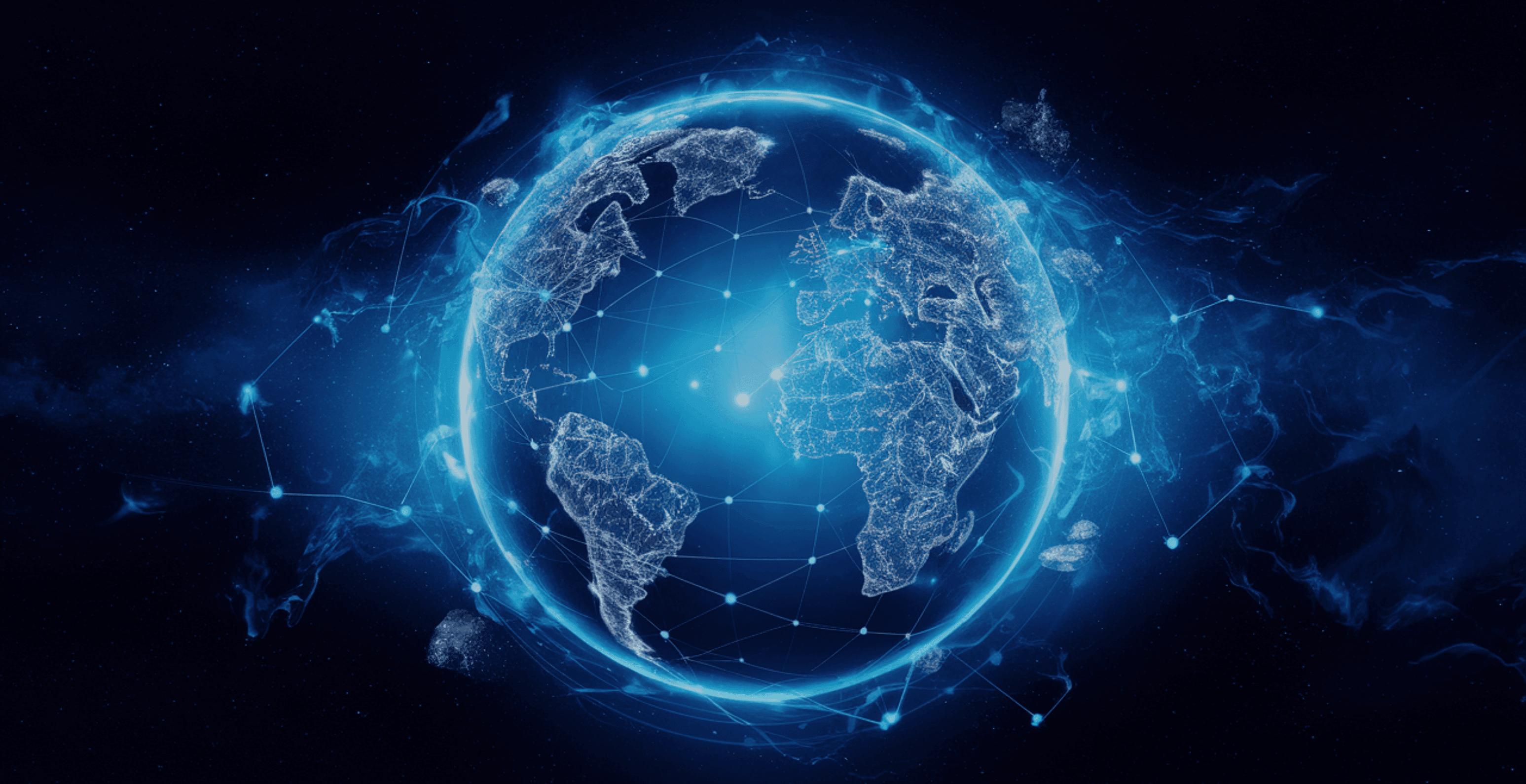
Navigating Global AI Regulations and Standards

Rehan Asif
Jun 10, 2024
Read the article




The Cost of Errors In AI Application Development

Rehan Asif
Jun 10, 2024
Read the article
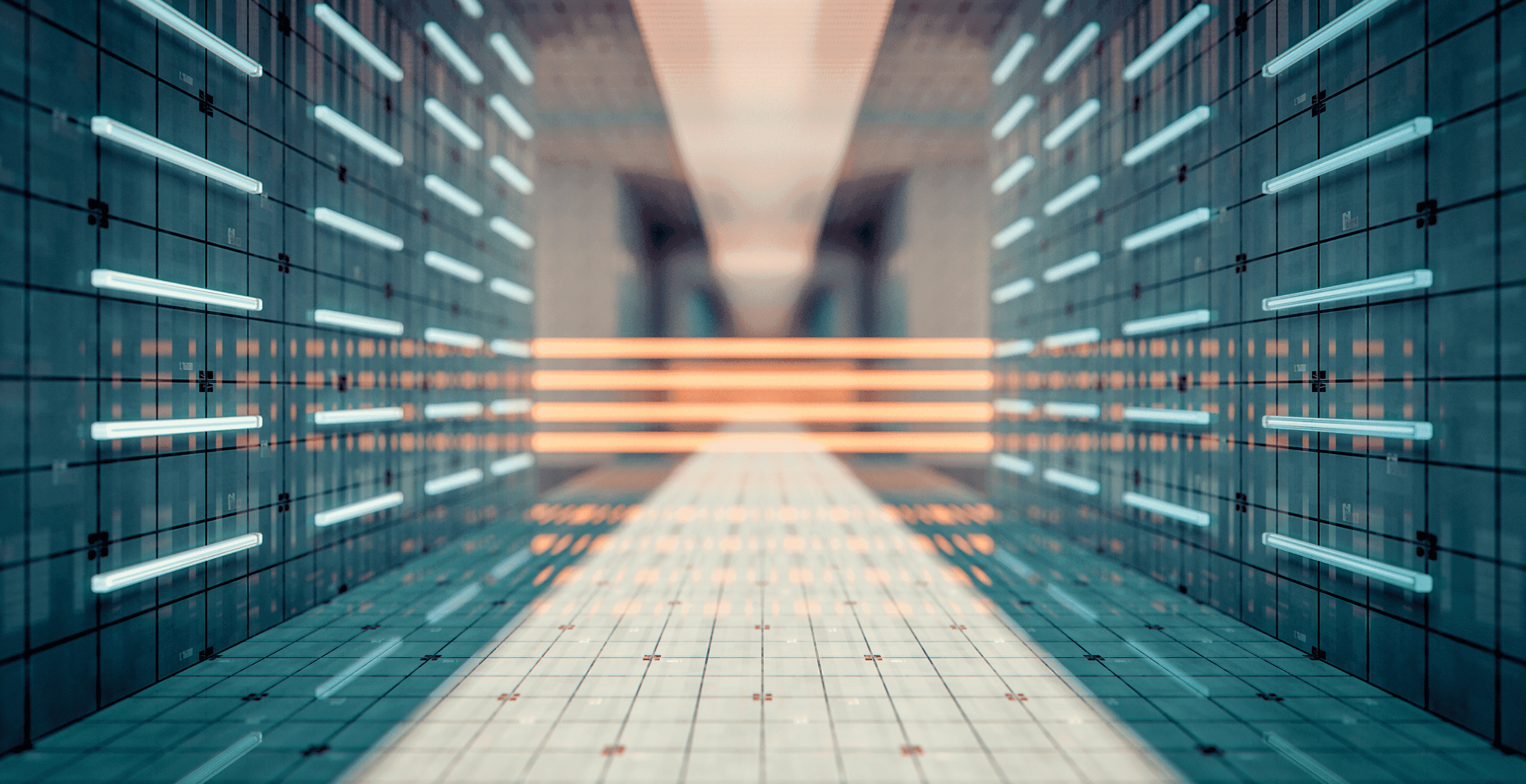
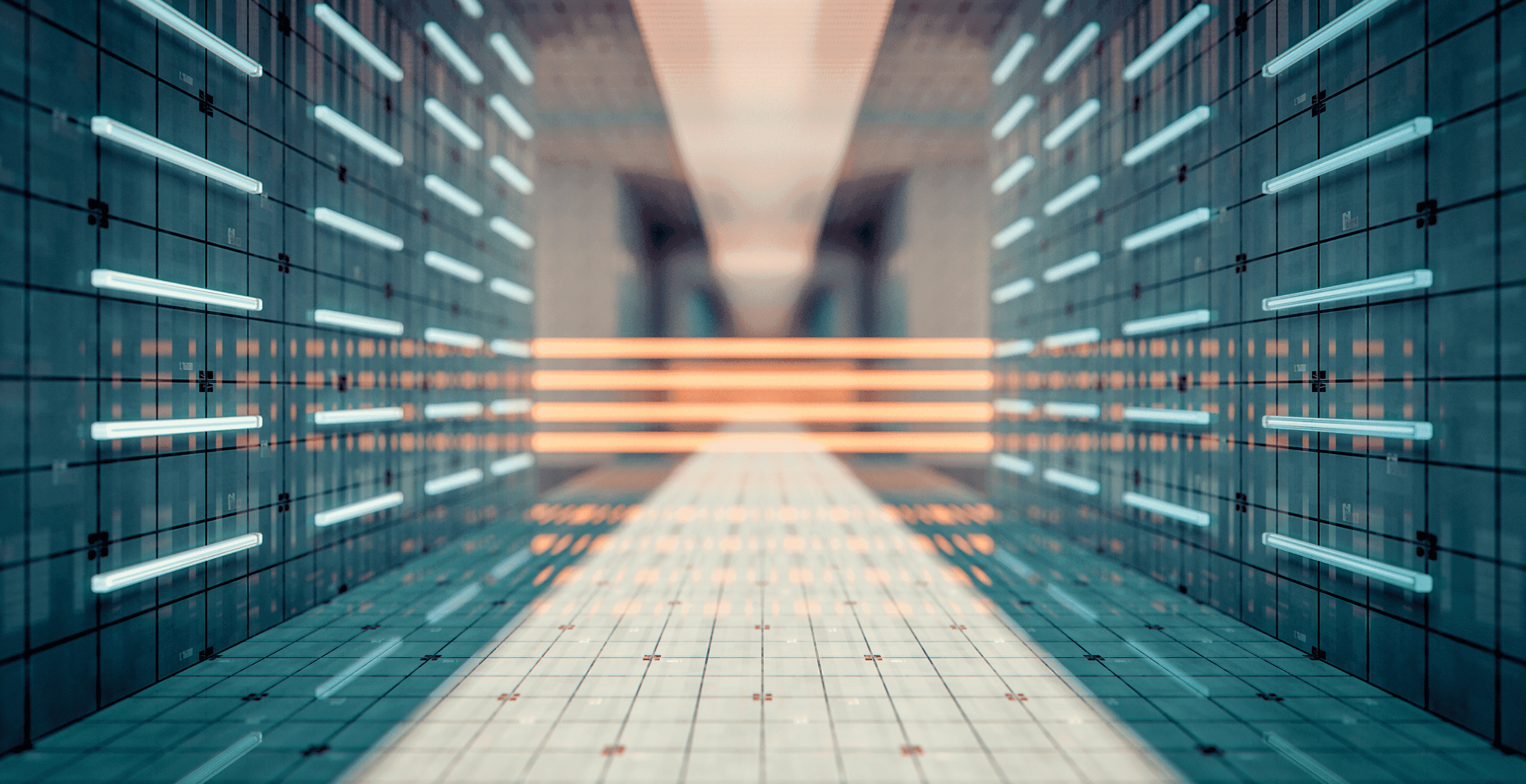
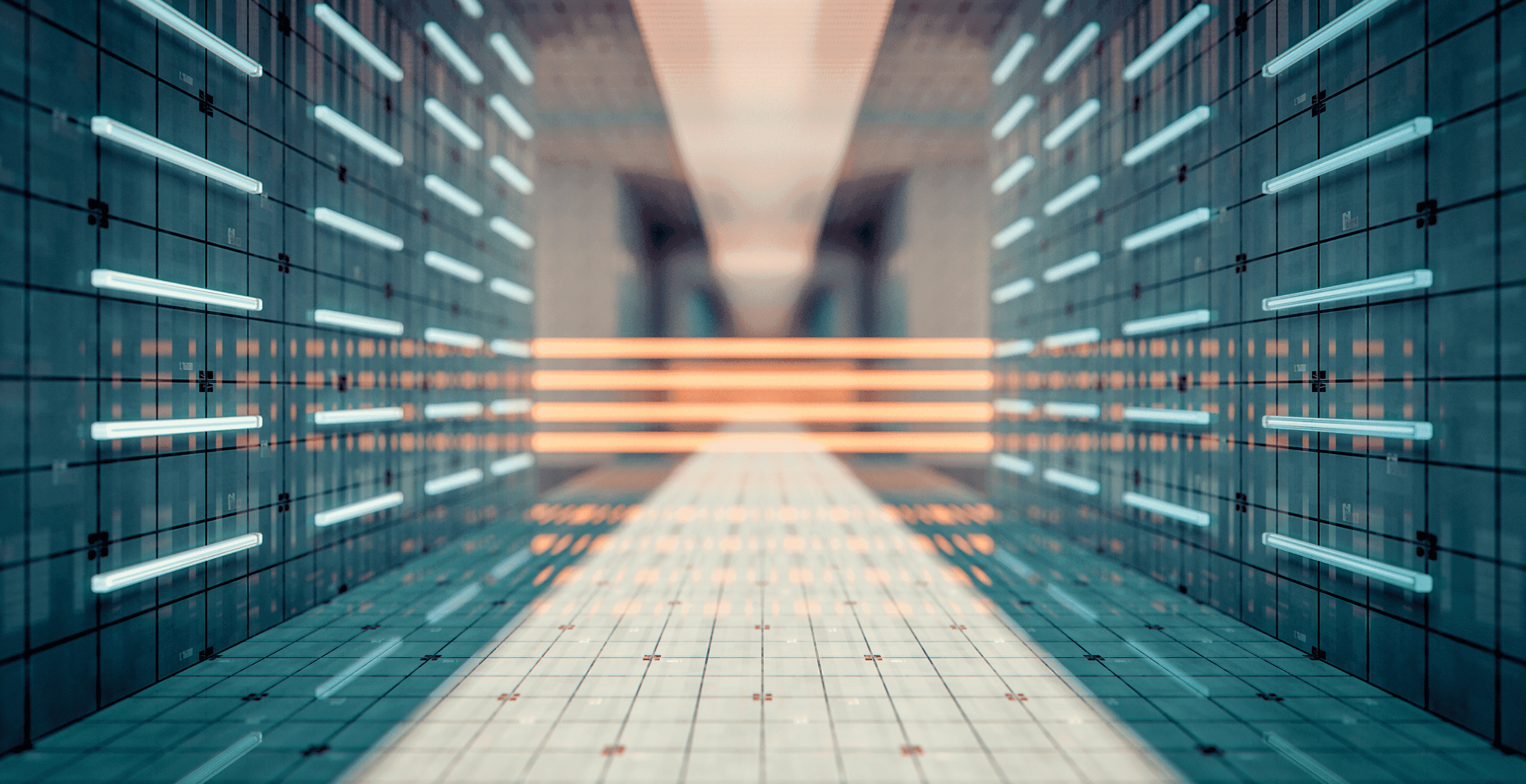
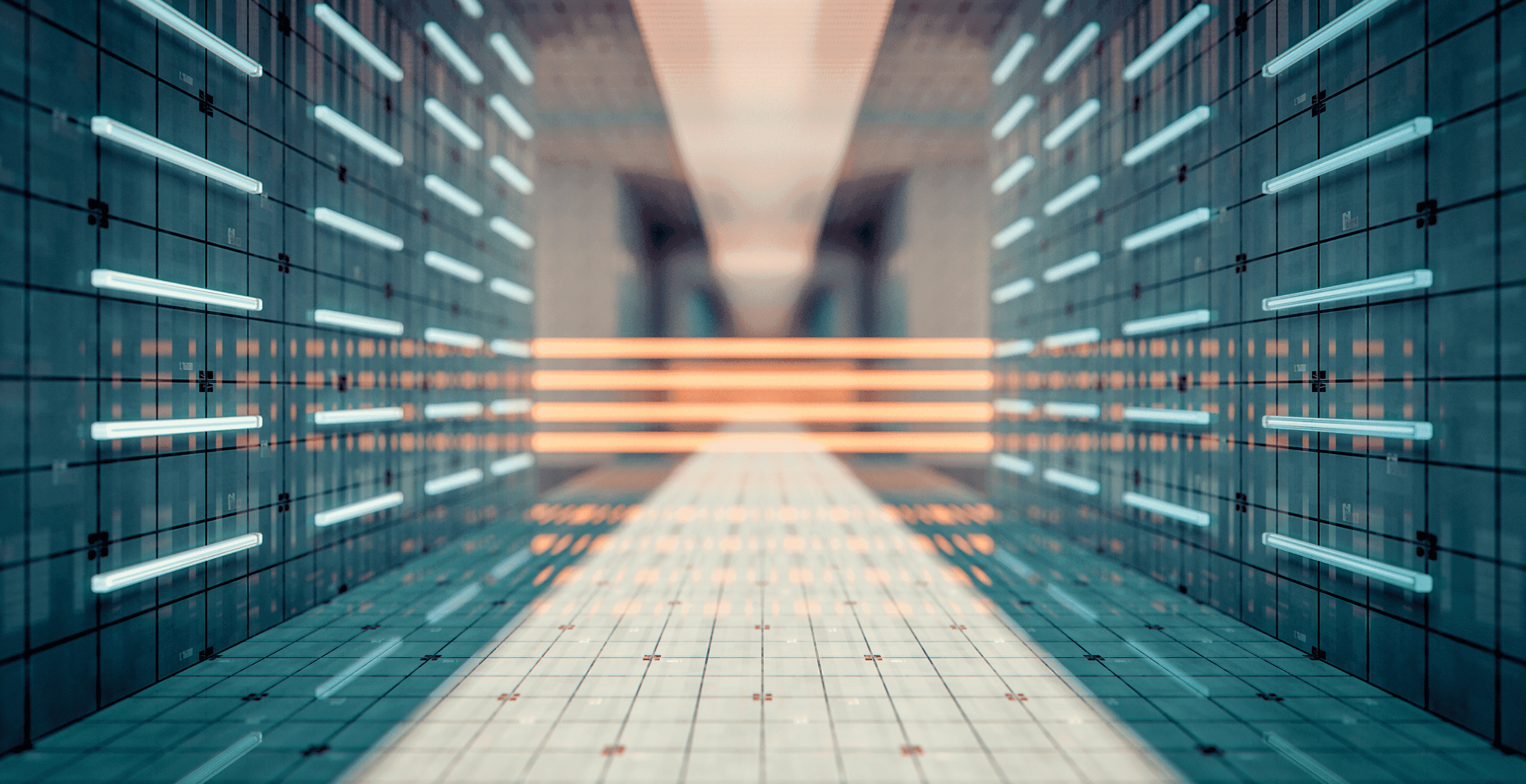
Best Practices In Data Governance For AI

Rehan Asif
Jun 10, 2024
Read the article




Success Stories And Case Studies Of AI Adoption Across Industries
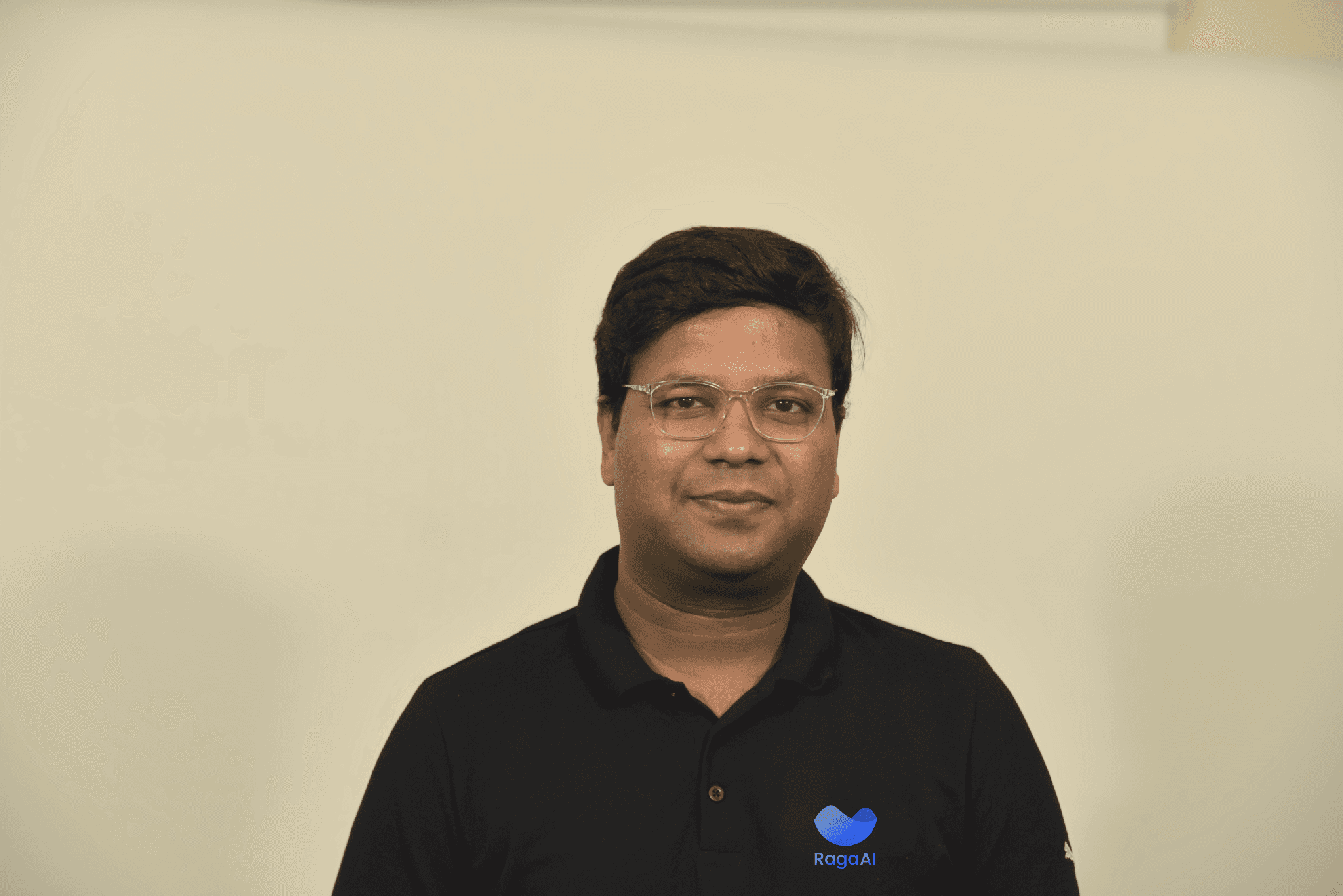
Jigar Gupta
May 1, 2024
Read the article
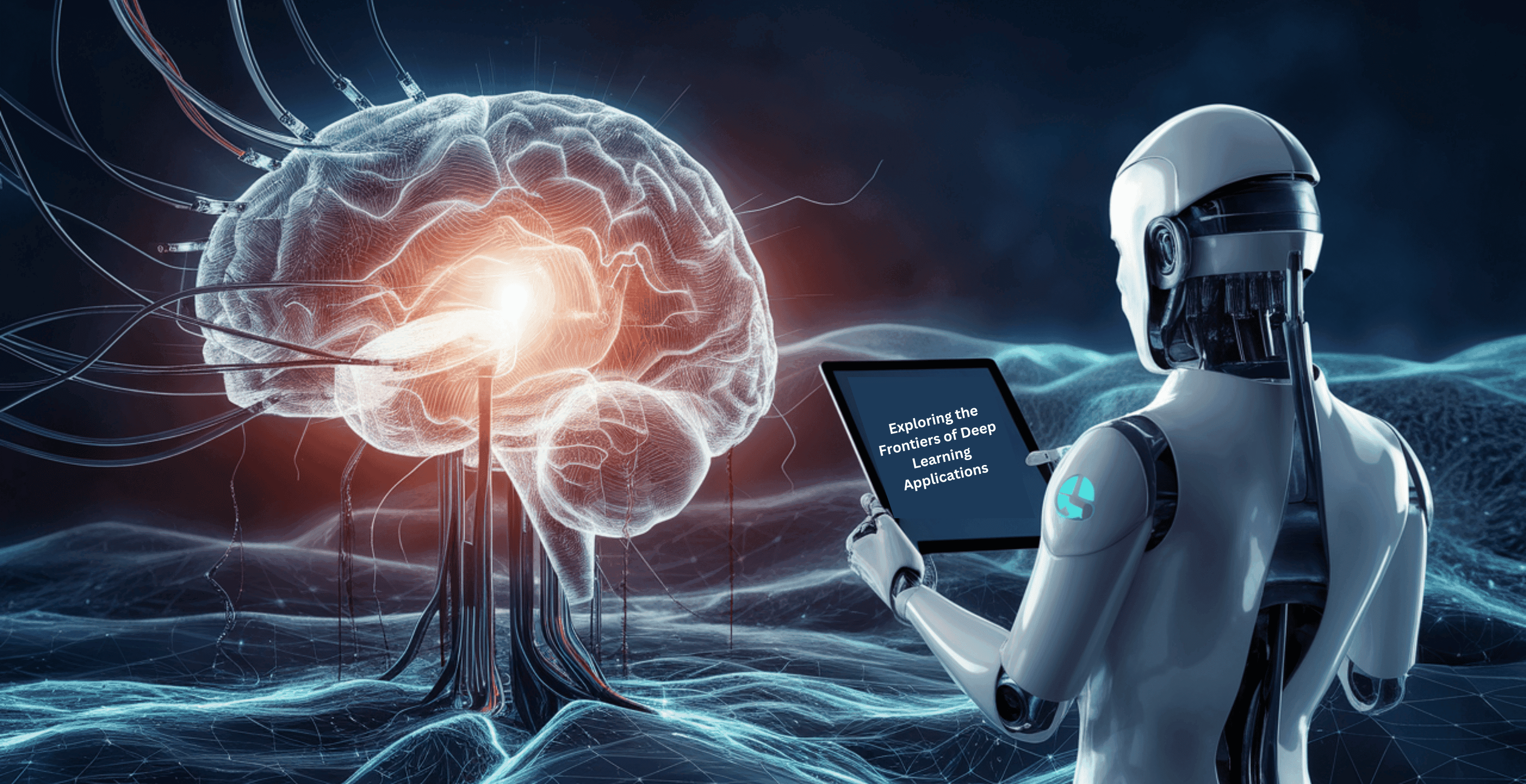
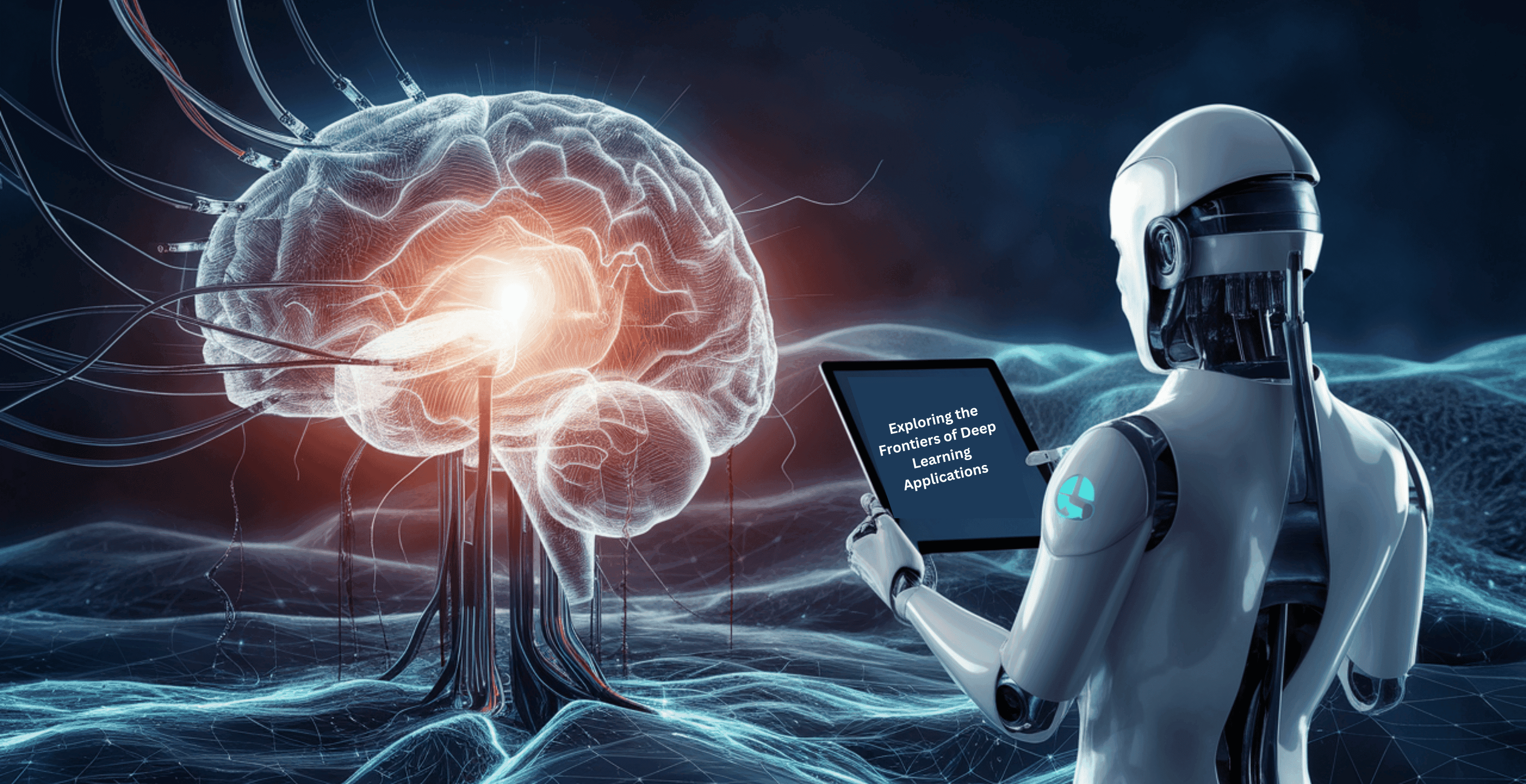
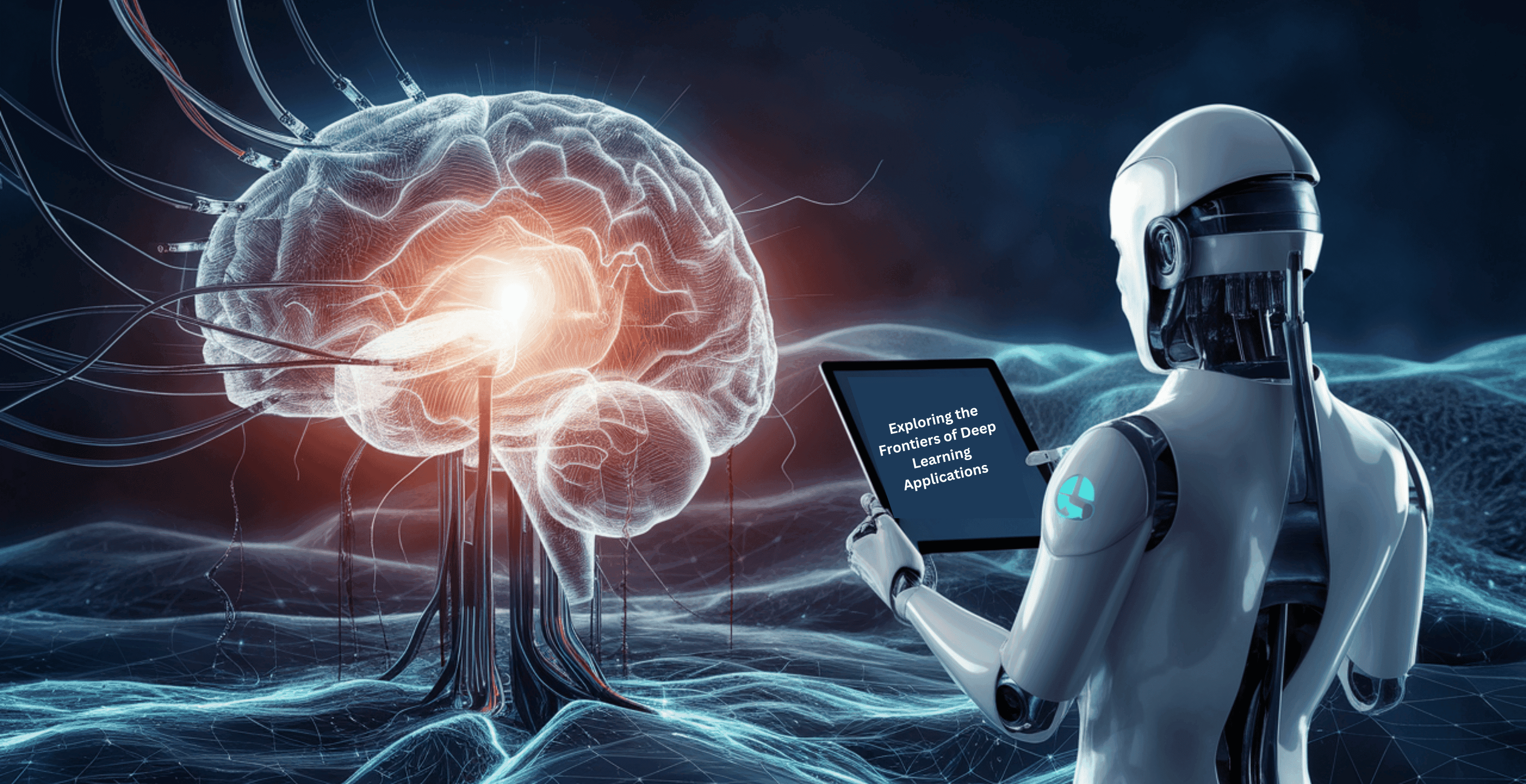
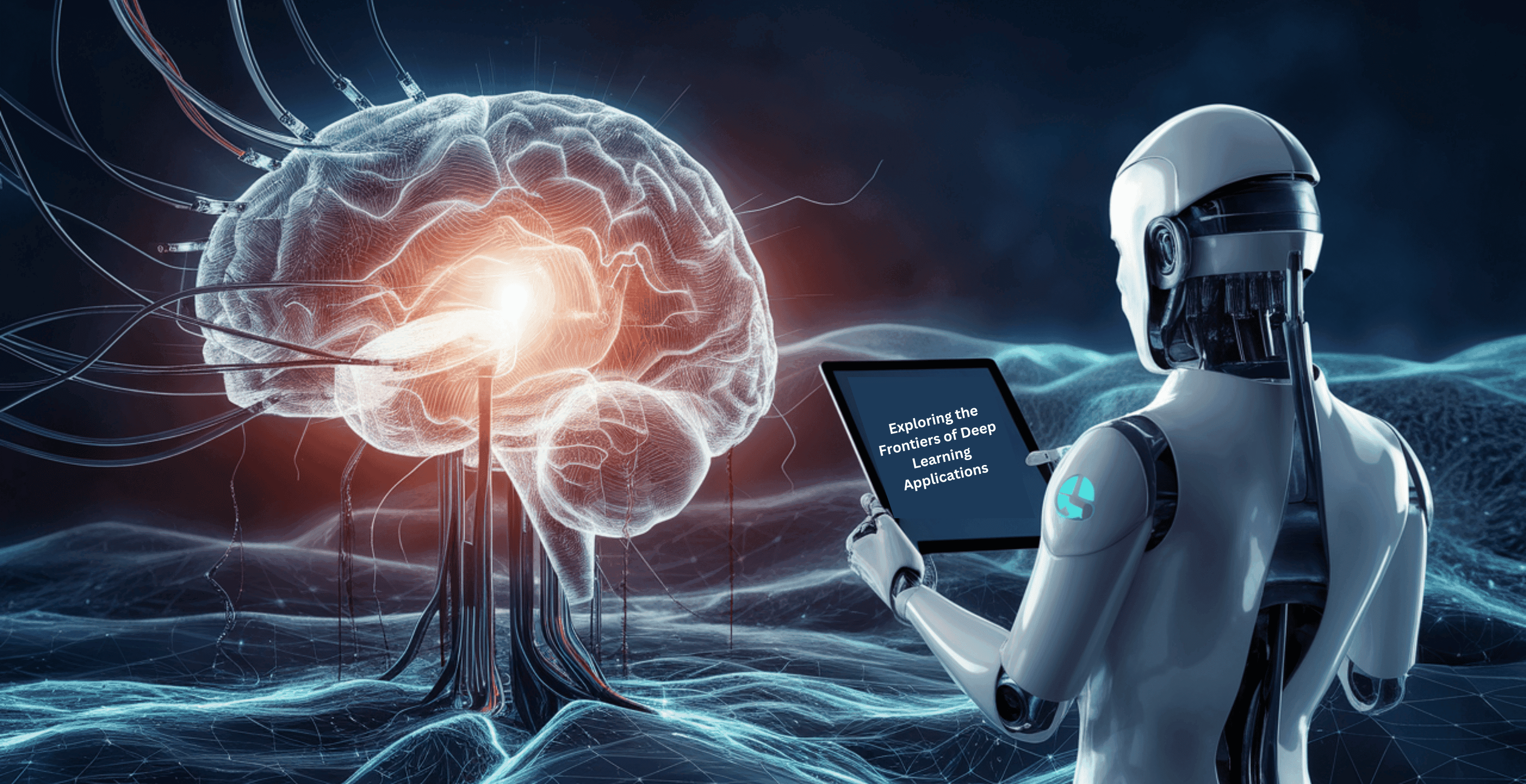
Exploring The Frontiers Of Deep Learning Applications
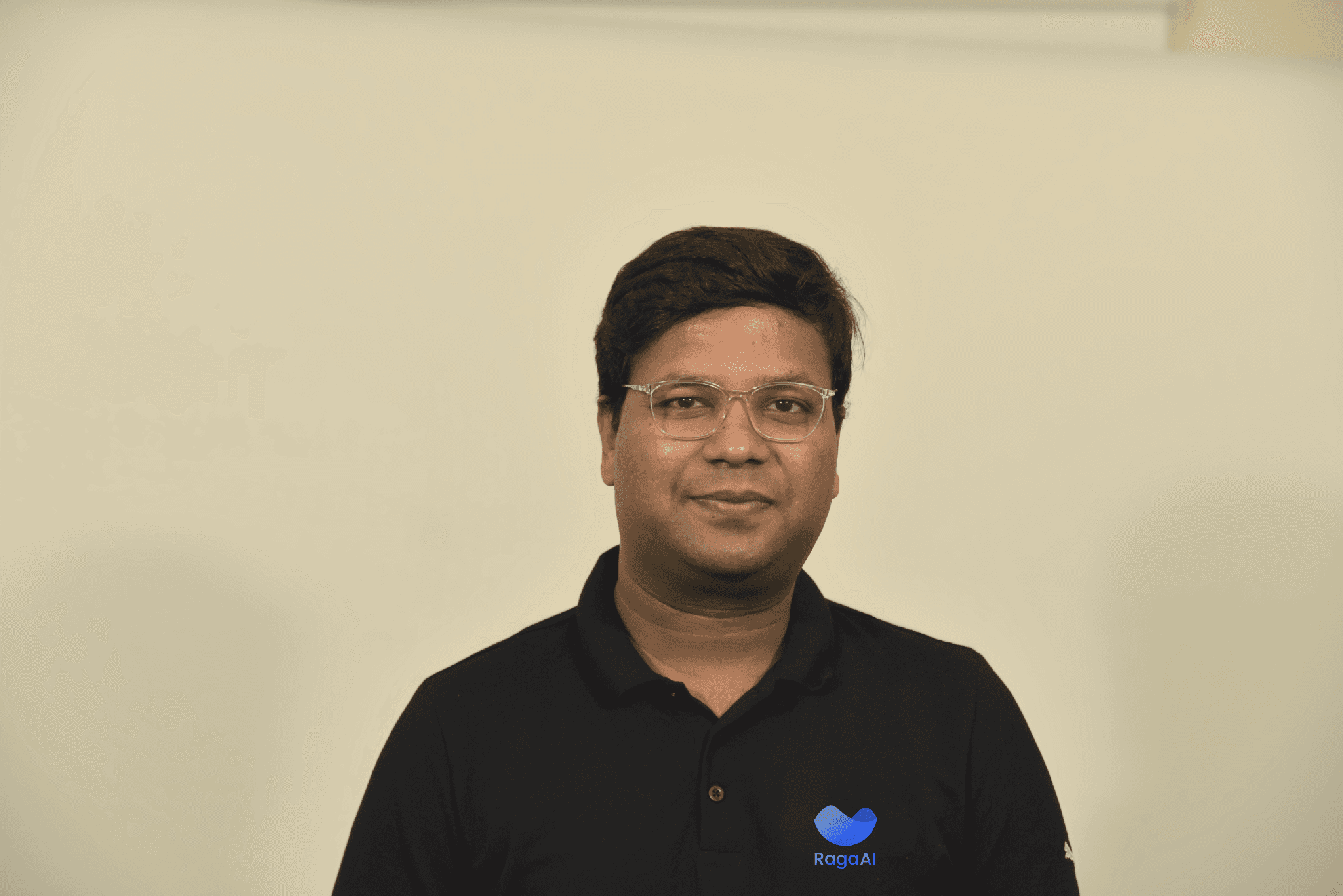
Jigar Gupta
May 1, 2024
Read the article




Integration Of RAG Platforms With Existing Enterprise Systems
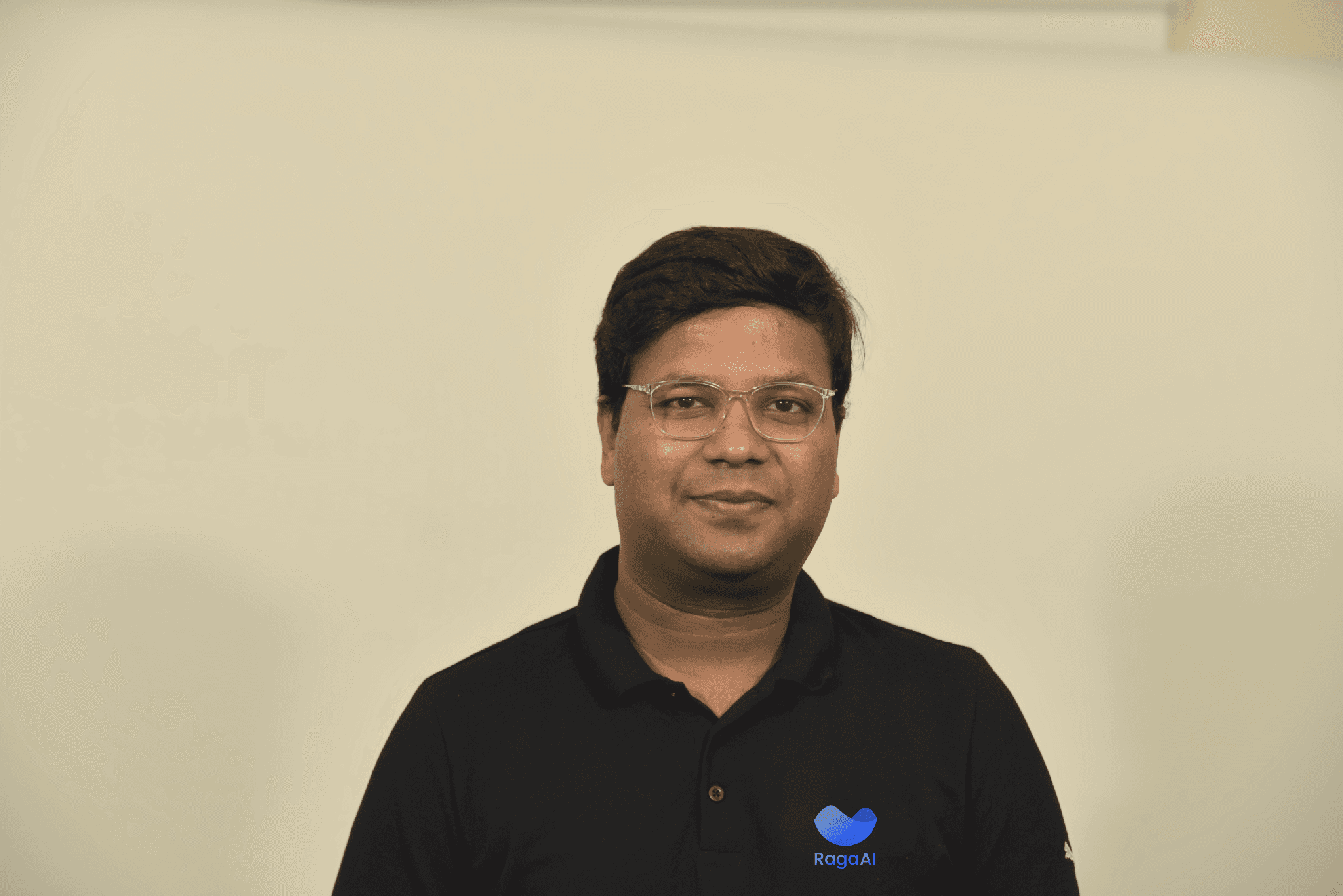
Jigar Gupta
Apr 30, 2024
Read the article




Multimodal LLMS Using Image And Text

Rehan Asif
Apr 30, 2024
Read the article




Understanding ML Model Monitoring In Production

Rehan Asif
Apr 30, 2024
Read the article
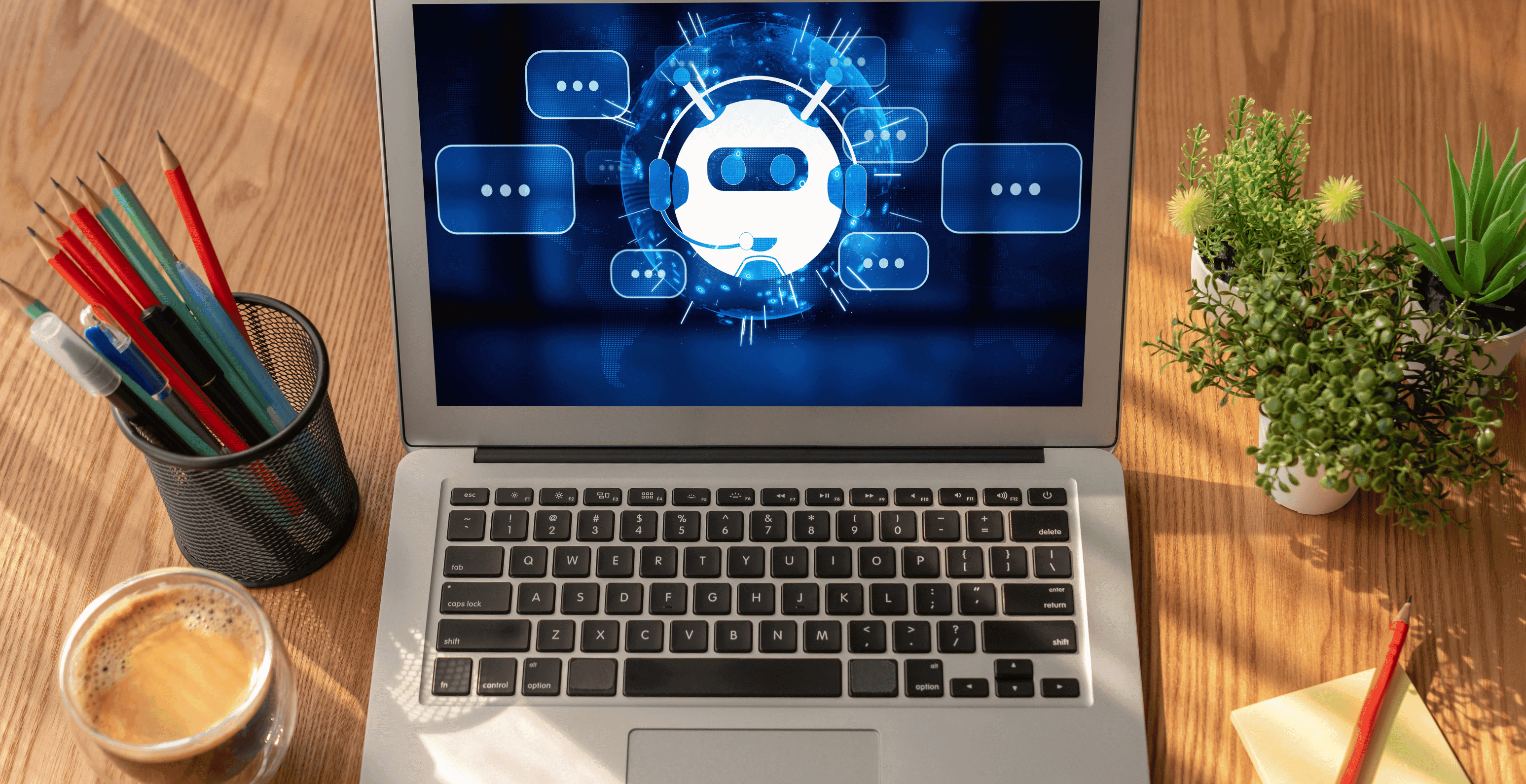
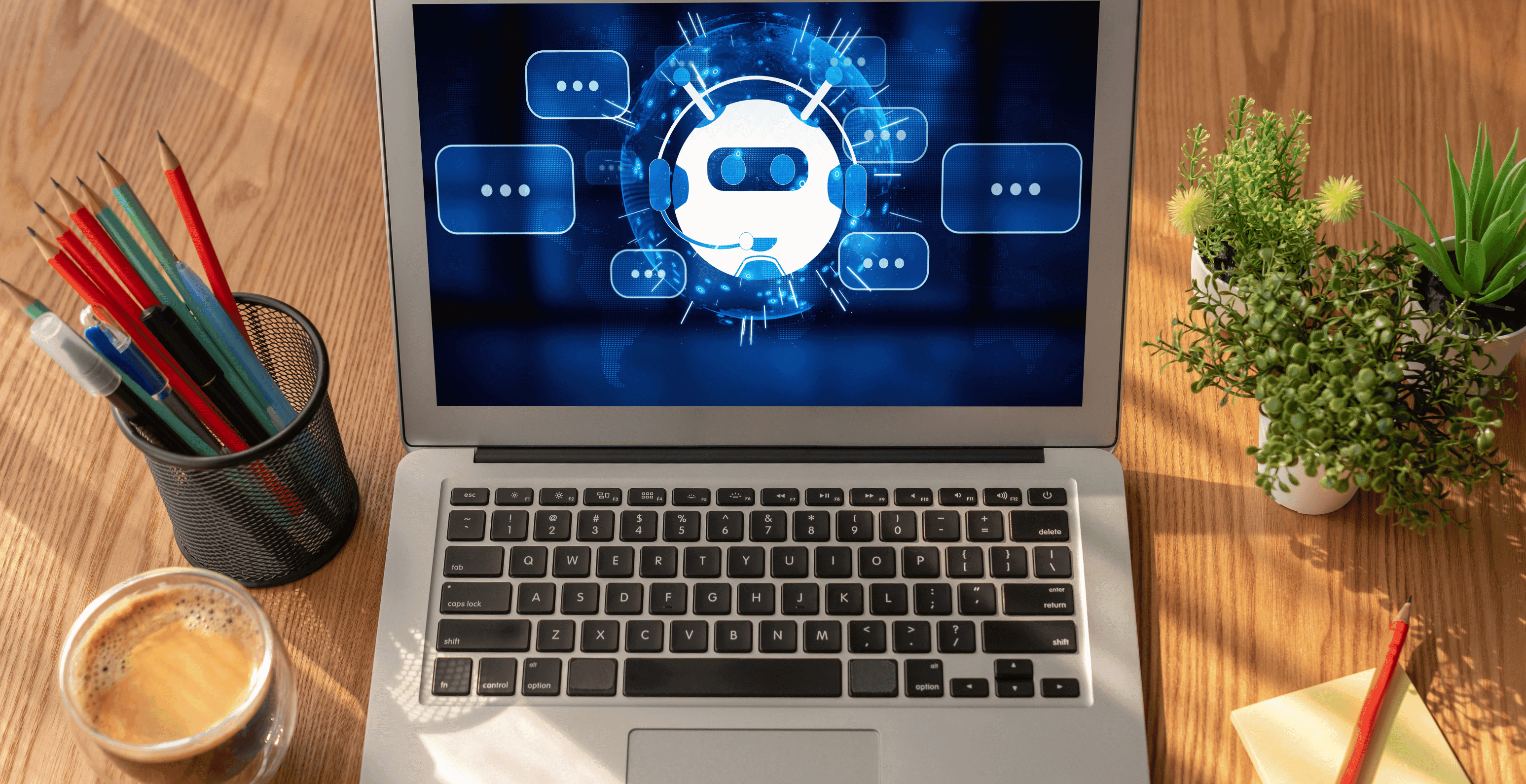
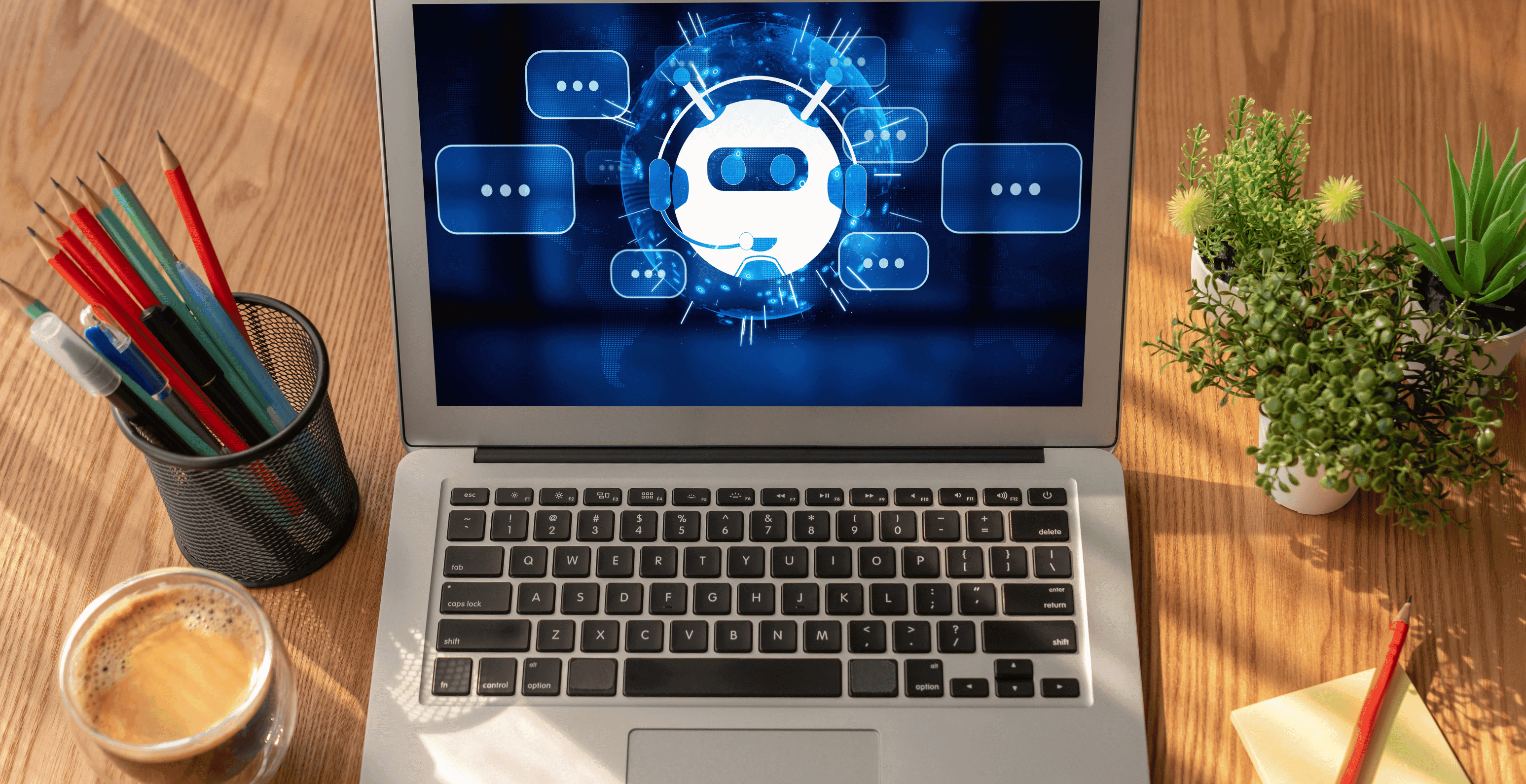
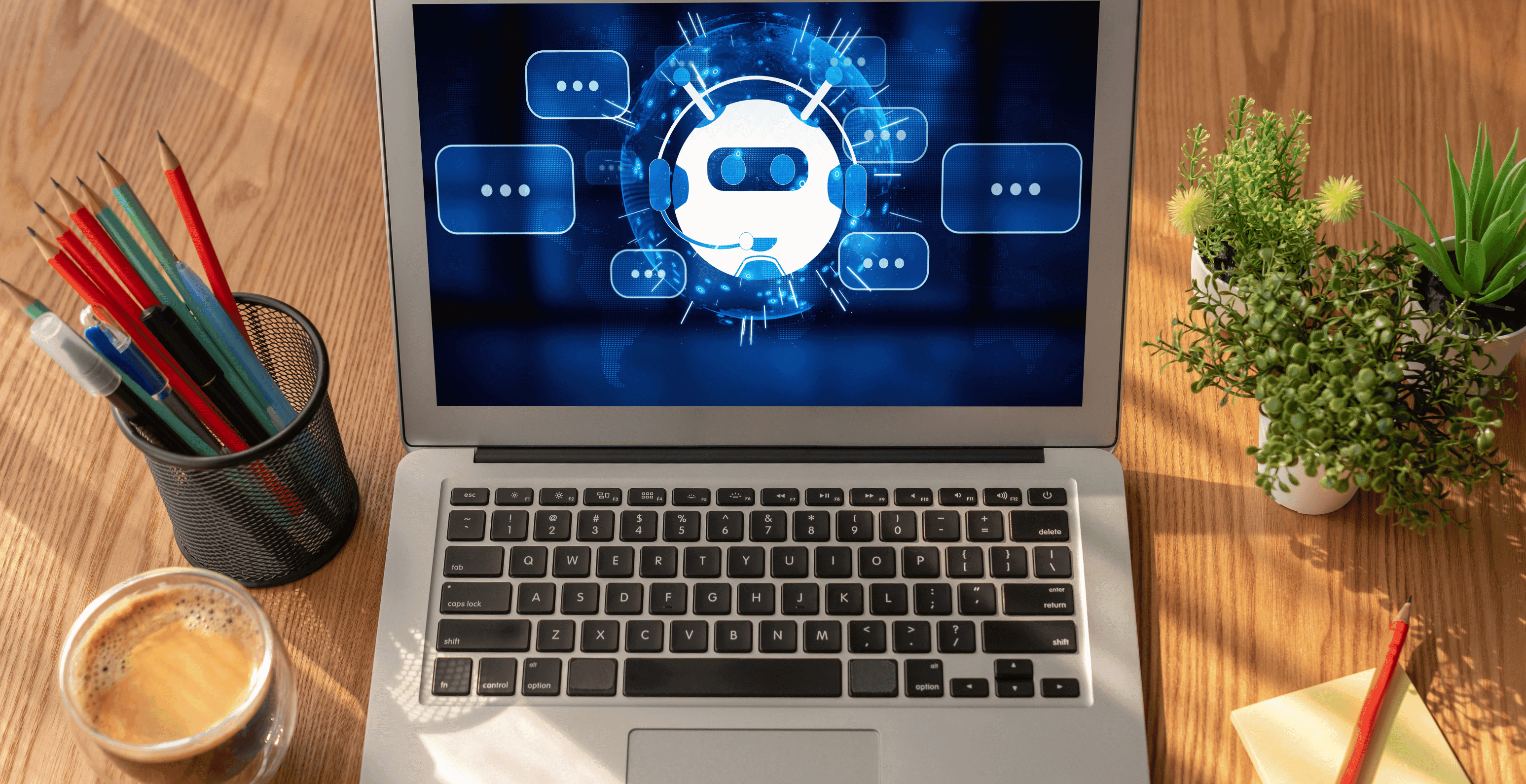
Strategic Approach To Testing AI-Powered Applications And Systems

Rehan Asif
Apr 30, 2024
Read the article




Navigating GDPR Compliance for AI Applications

Rehan Asif
Apr 26, 2024
Read the article
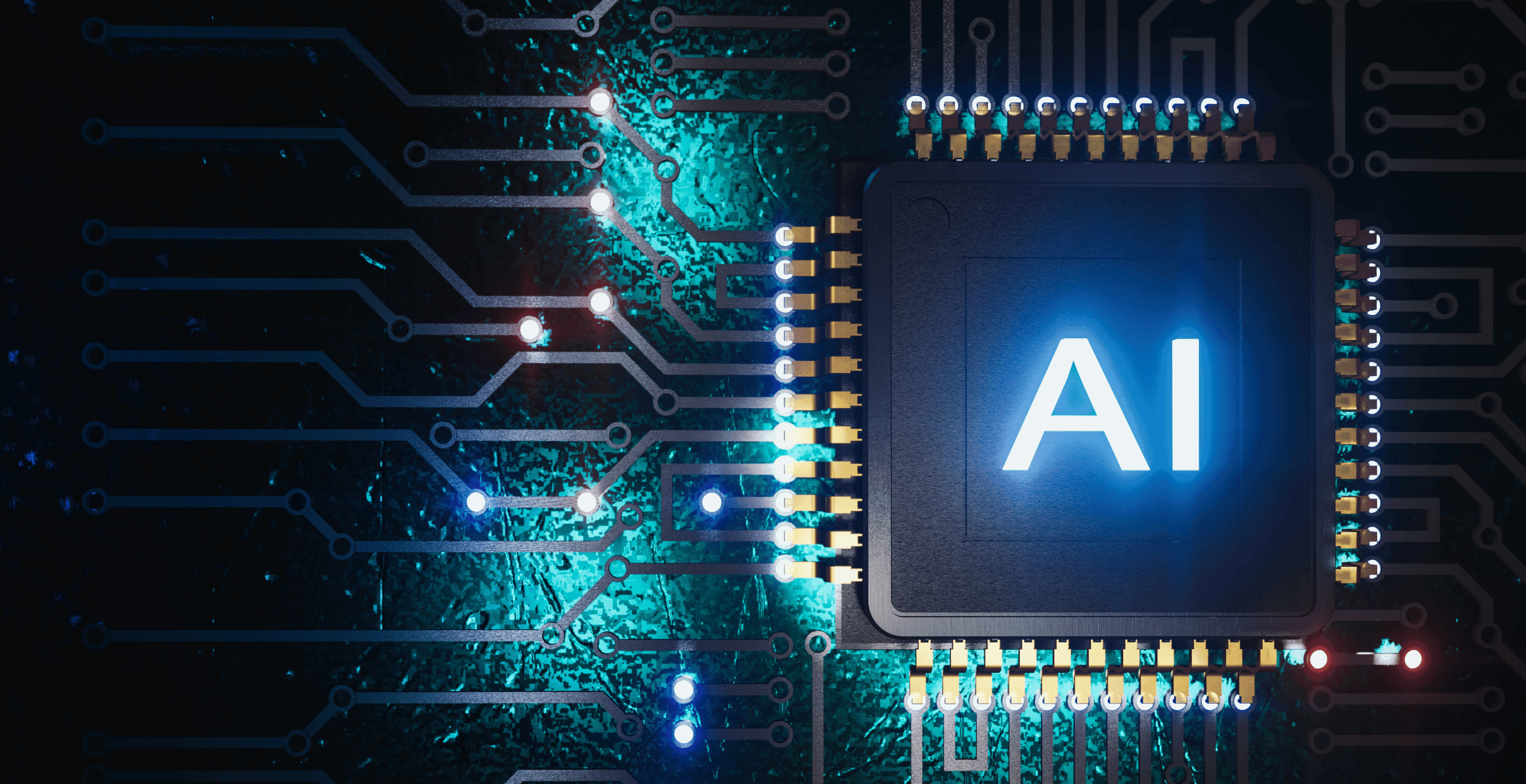
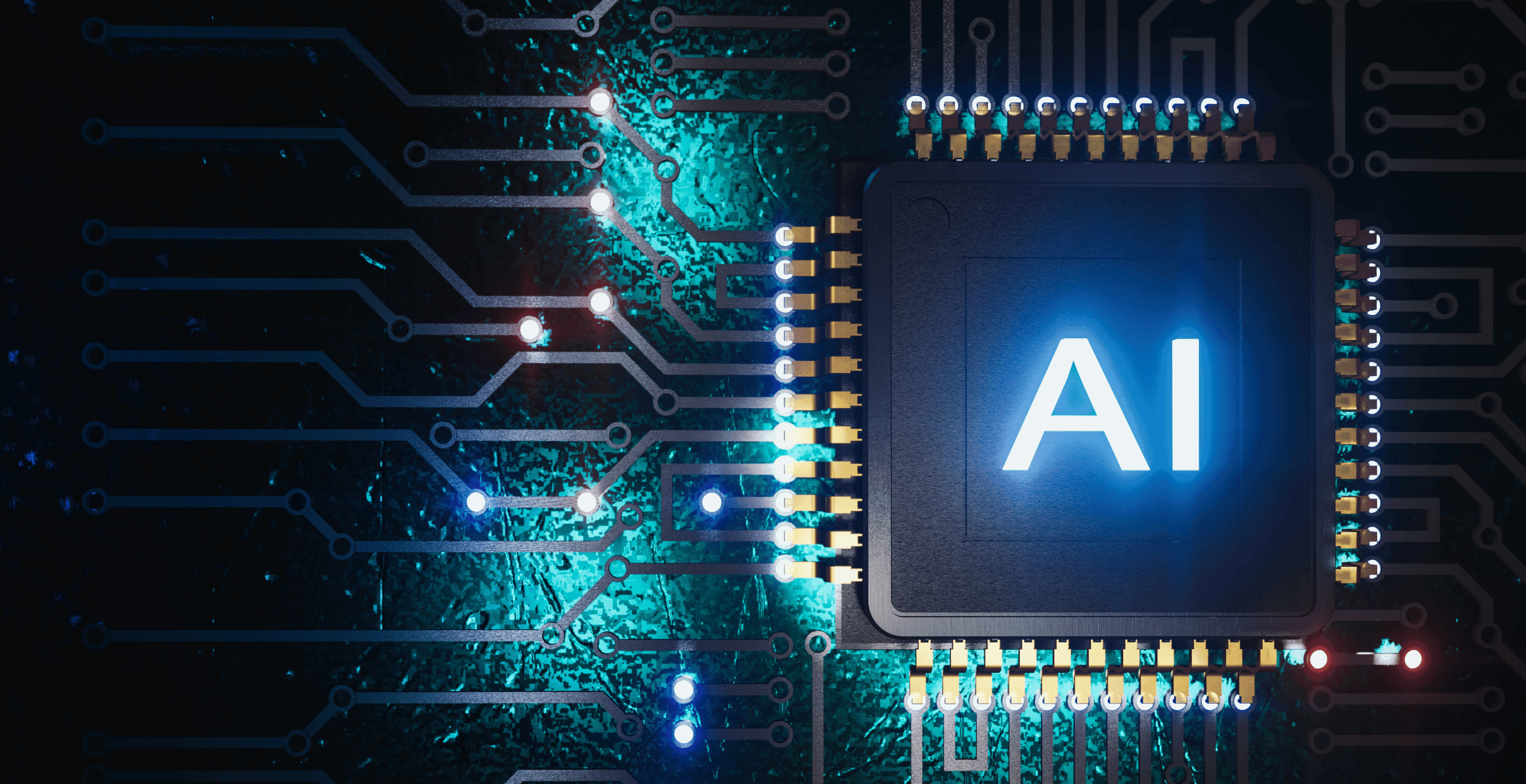
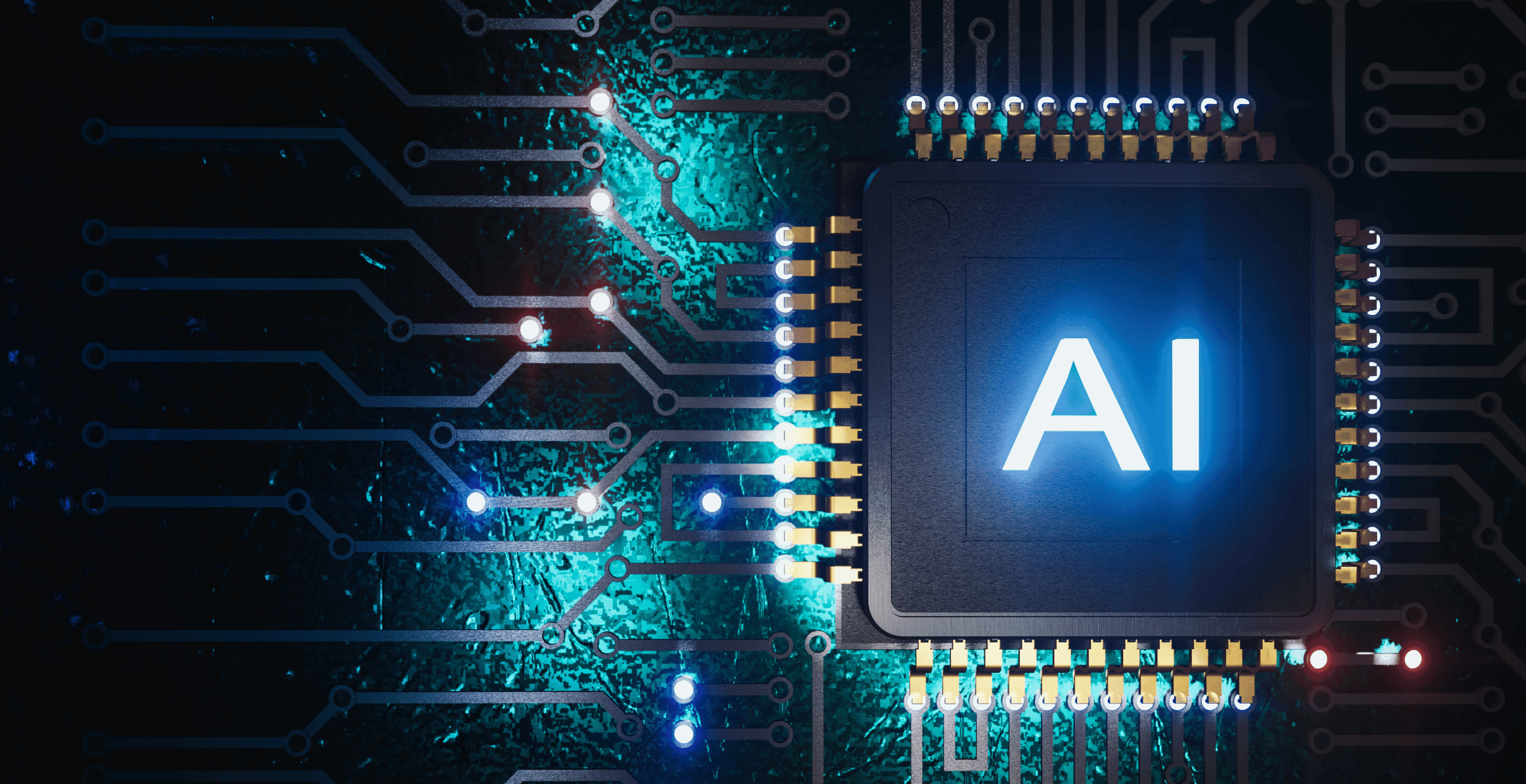
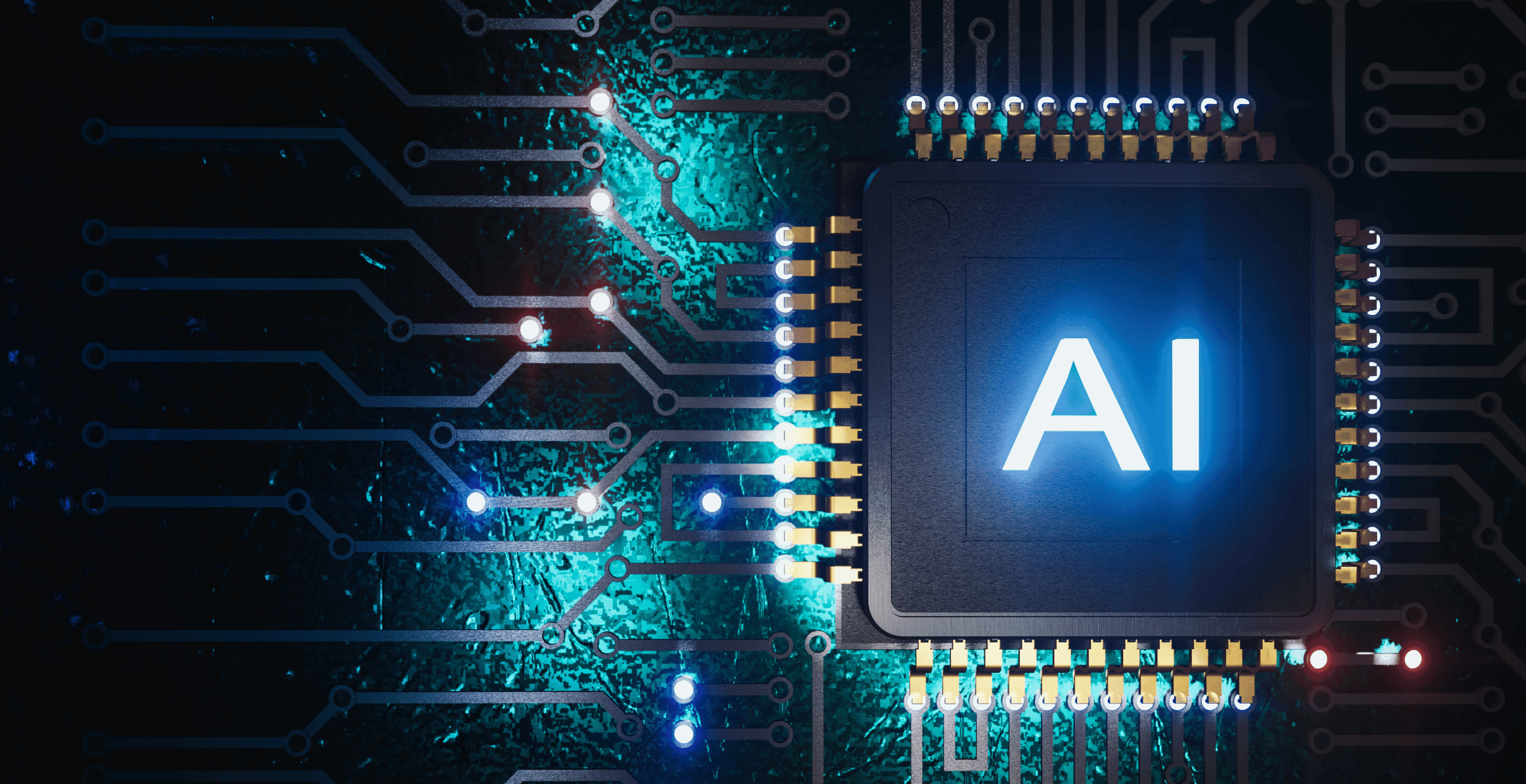
The Impact of AI Governance on Innovation and Development Speed

Rehan Asif
Apr 26, 2024
Read the article
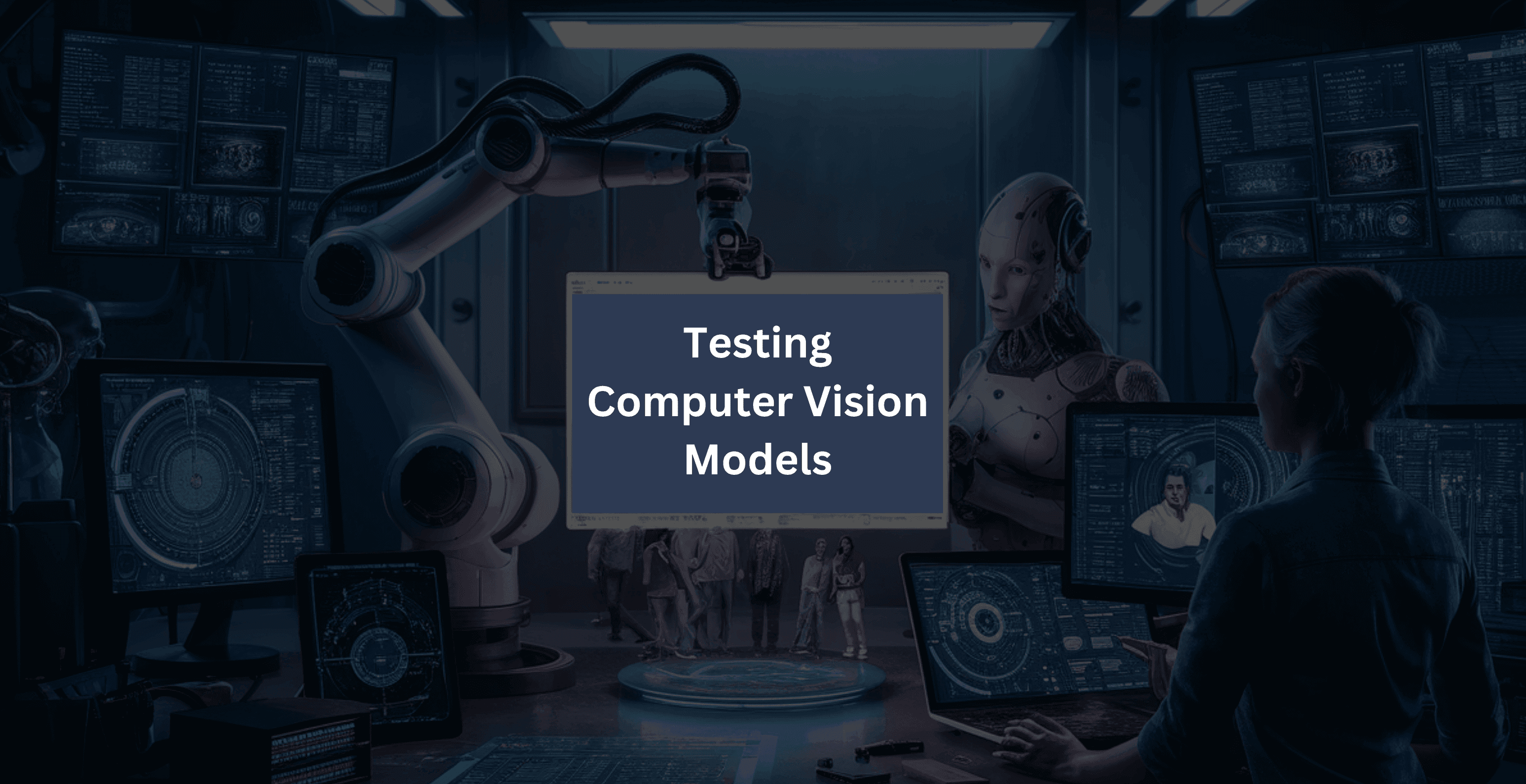
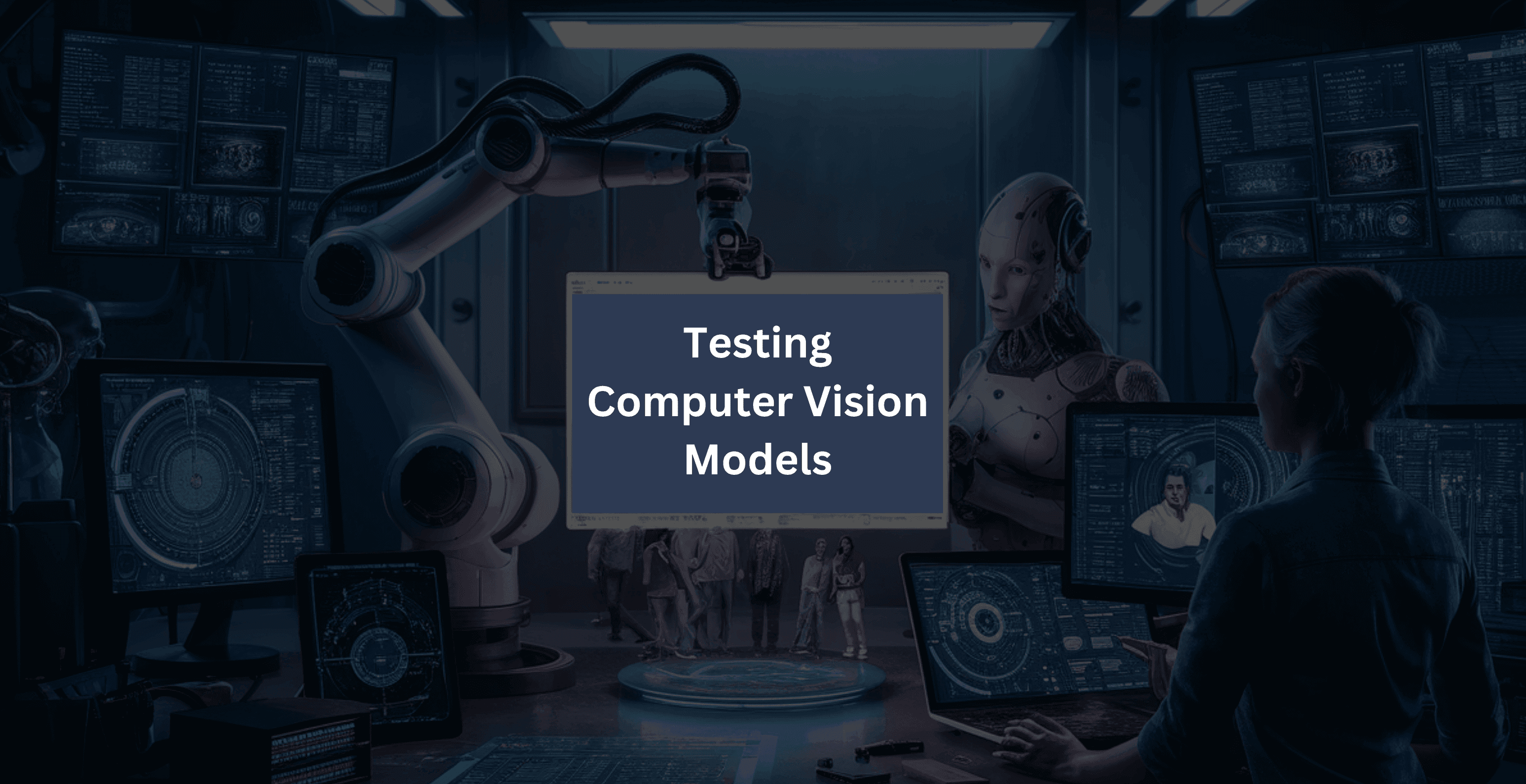
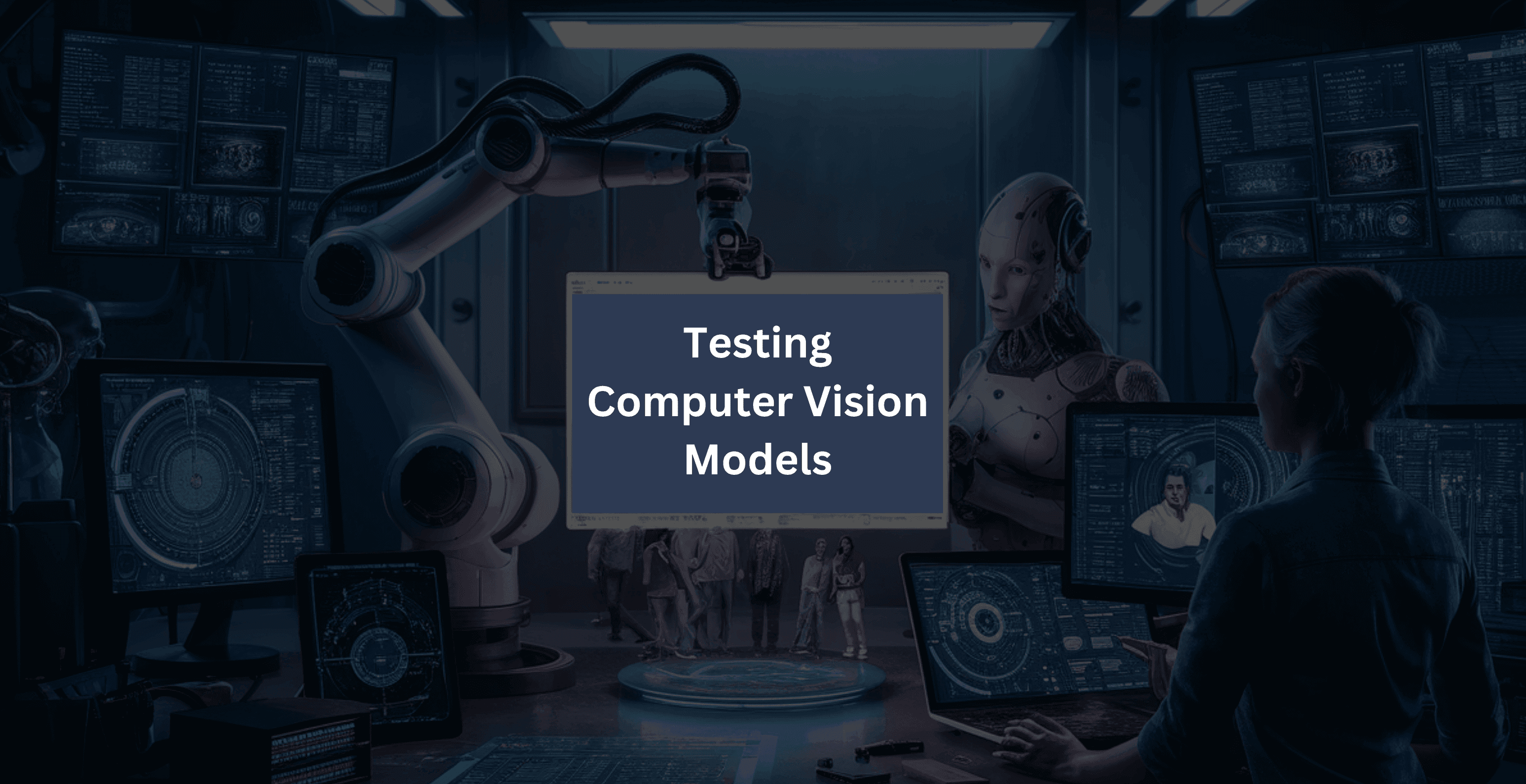
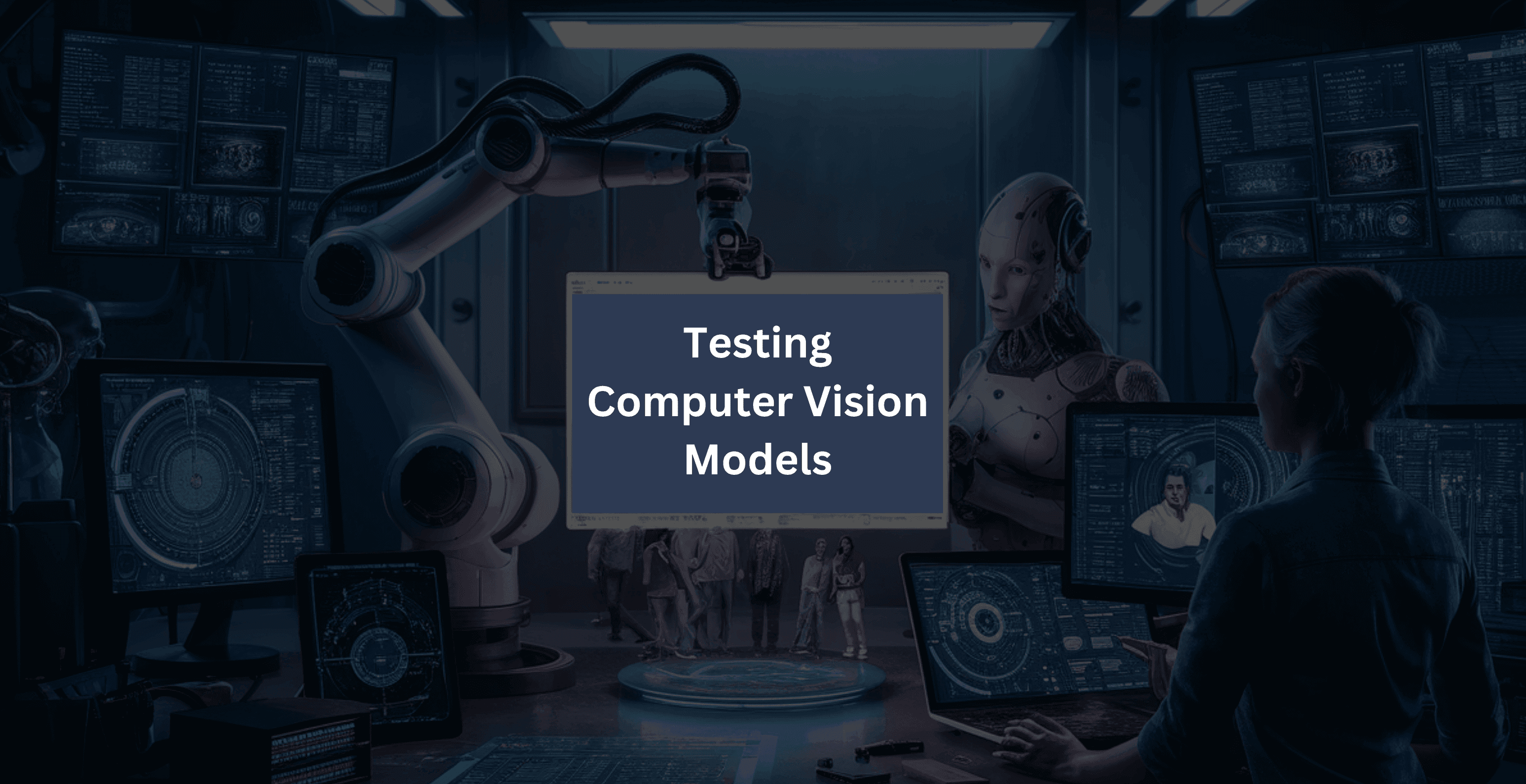
Best Practices For Testing Computer Vision Models
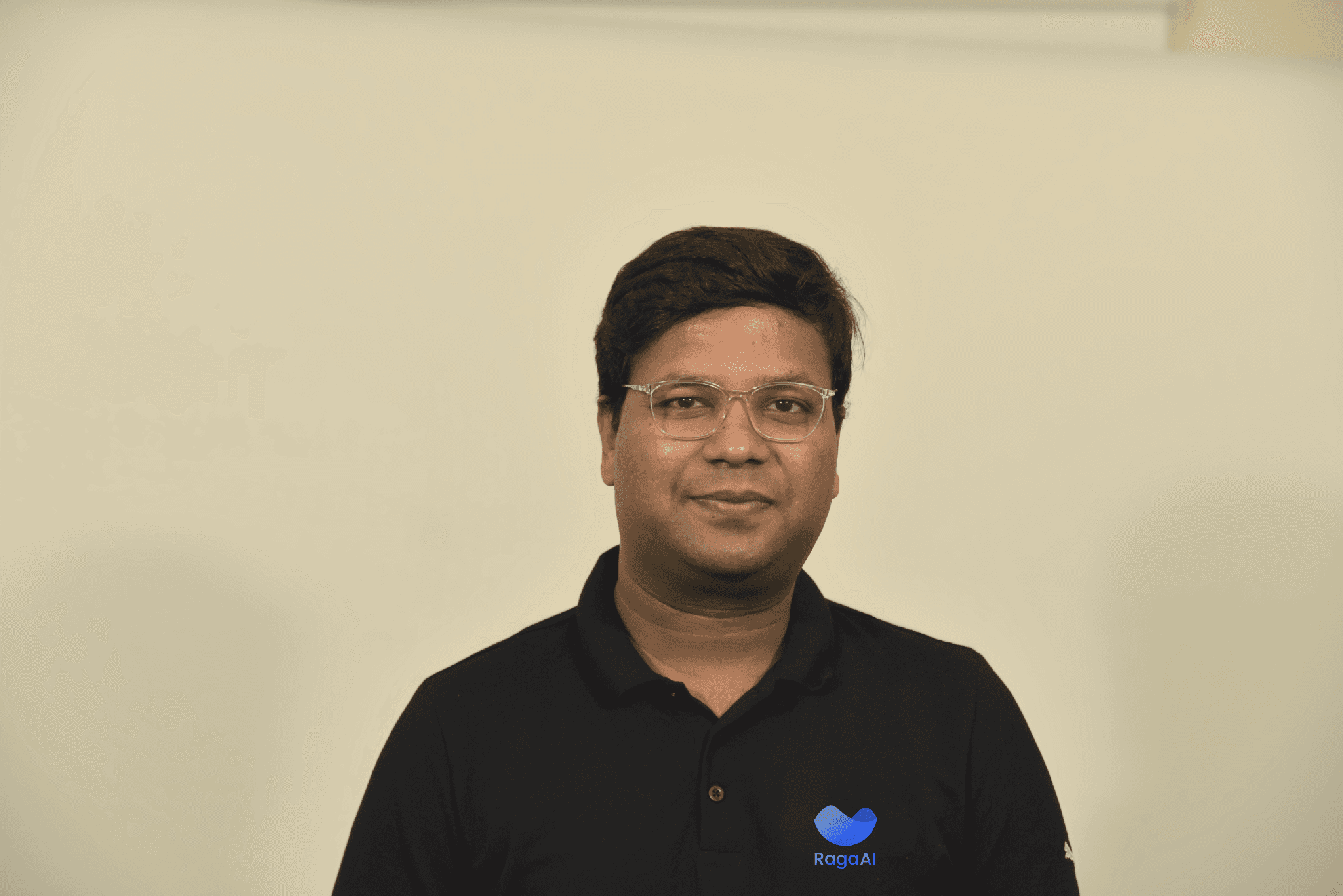
Jigar Gupta
Apr 25, 2024
Read the article
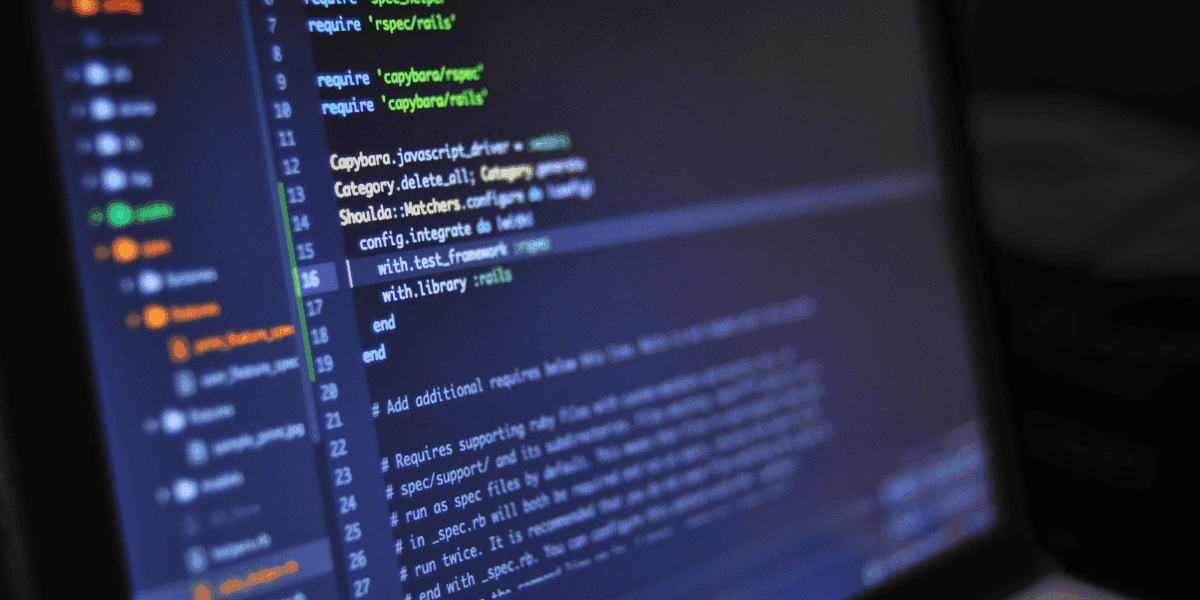
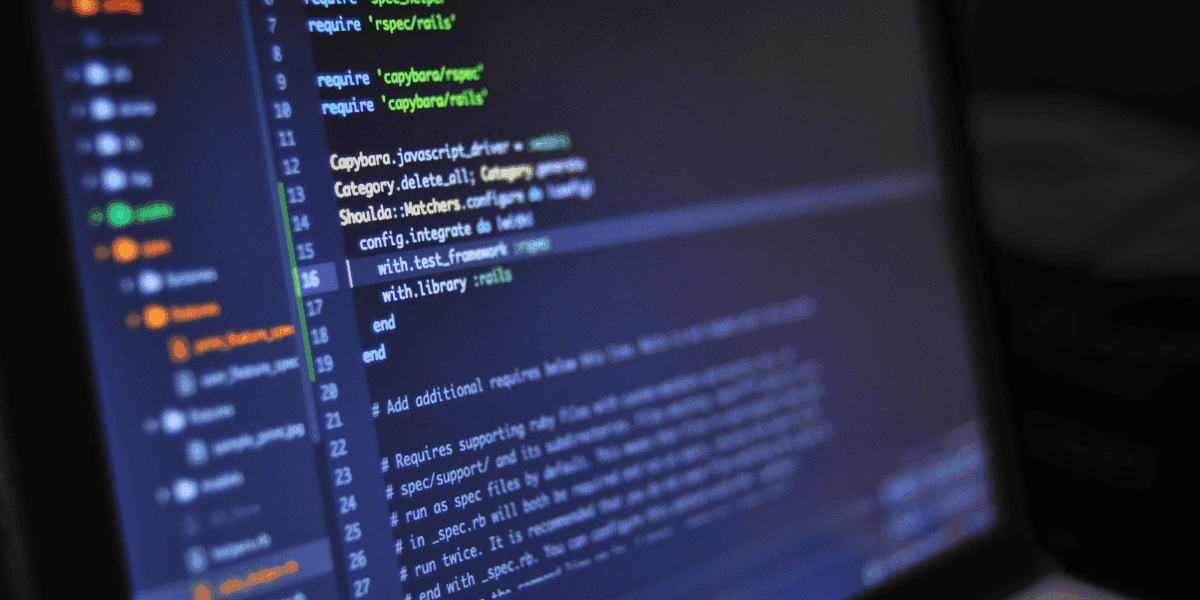
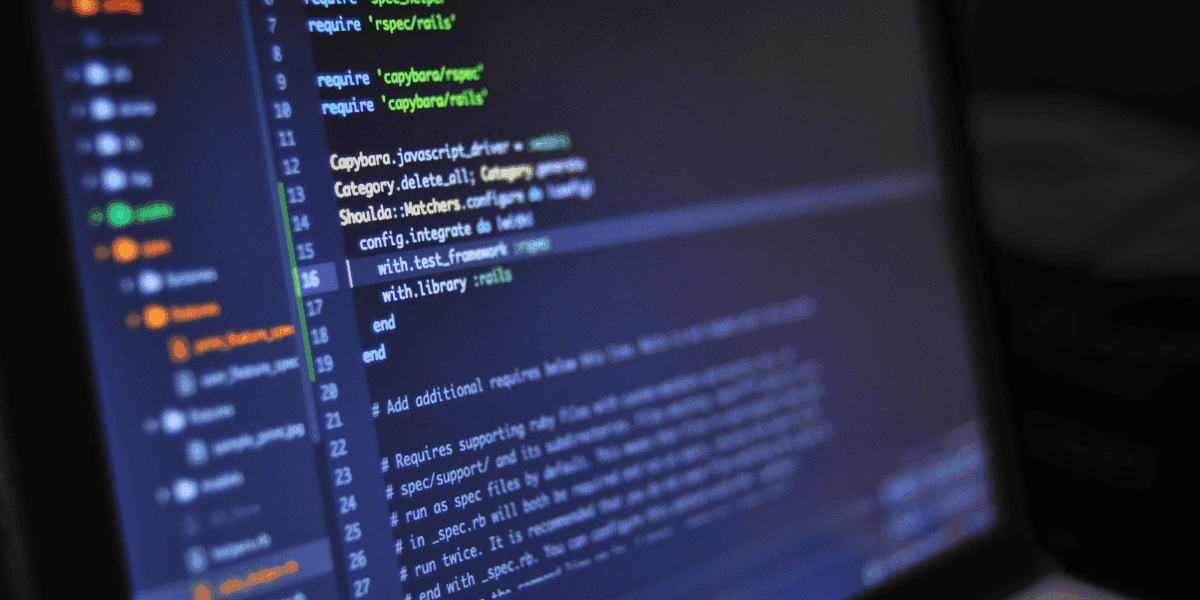
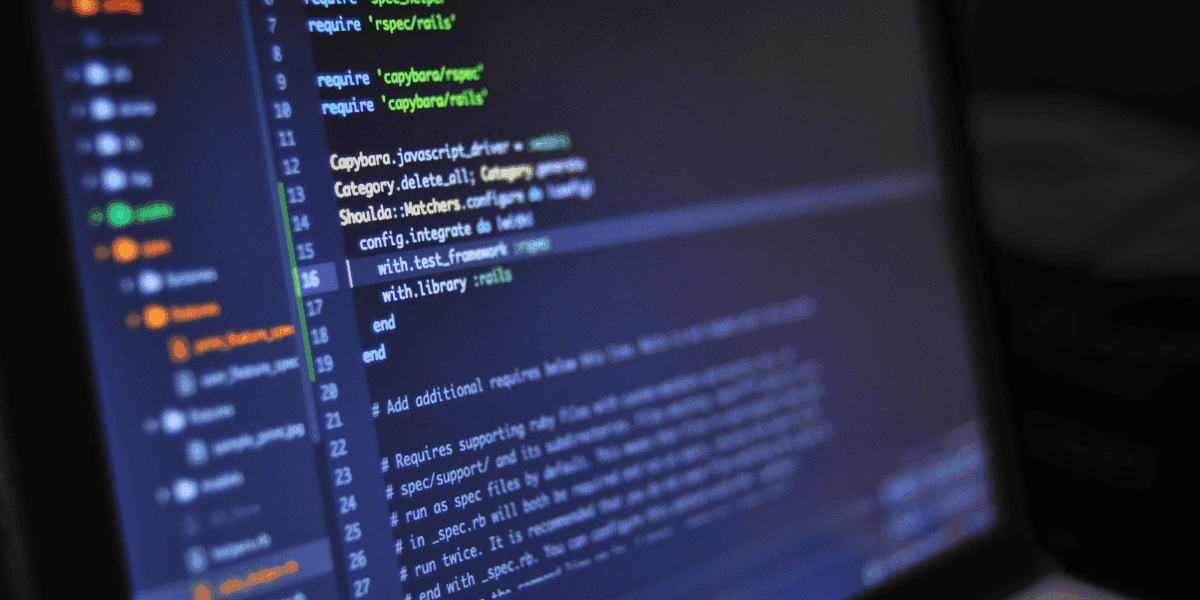
Building Low-Code LLM Apps with Visual Programming

Rehan Asif
Apr 26, 2024
Read the article




Understanding AI regulations In Finance

Akshat Gupta
Apr 26, 2024
Read the article




Compliance Automation: Getting Started with Regulatory Management

Akshat Gupta
Apr 25, 2024
Read the article
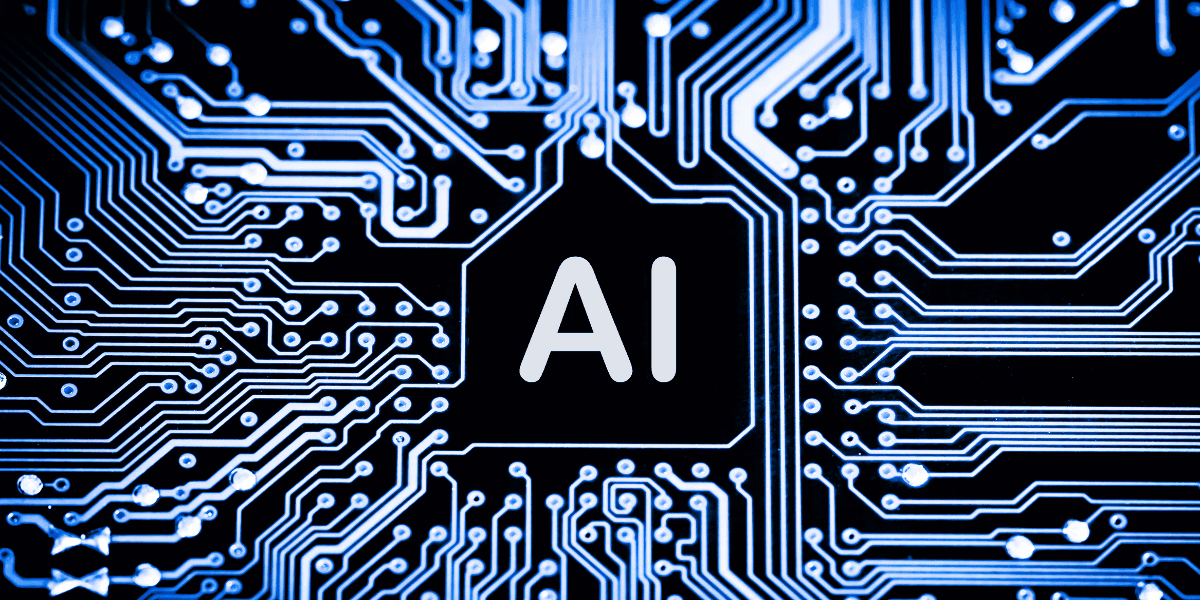
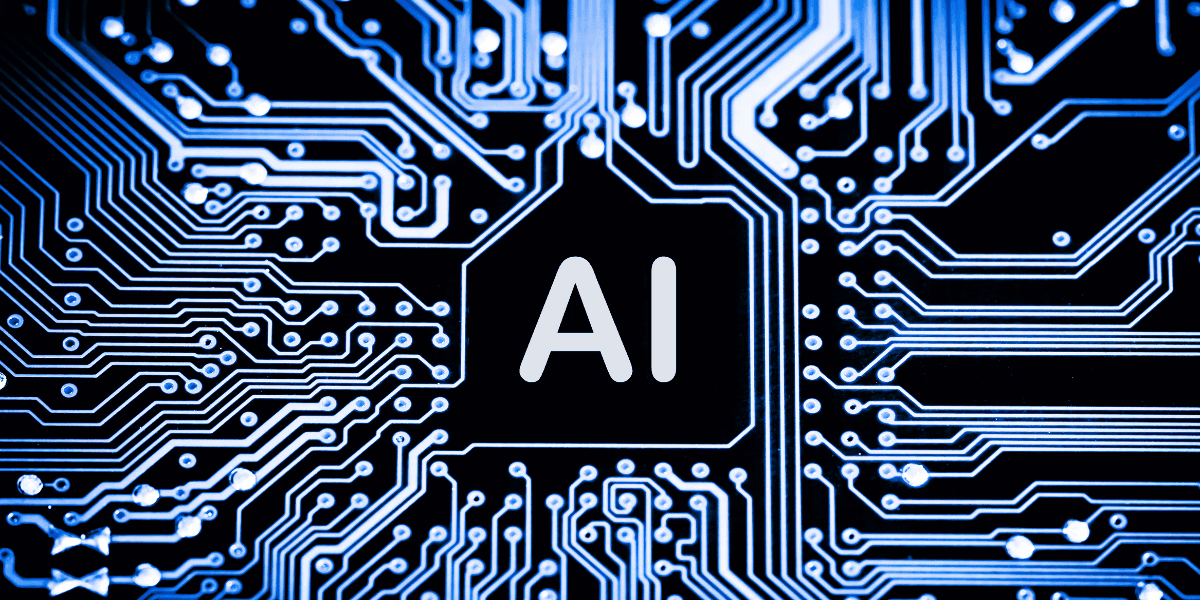
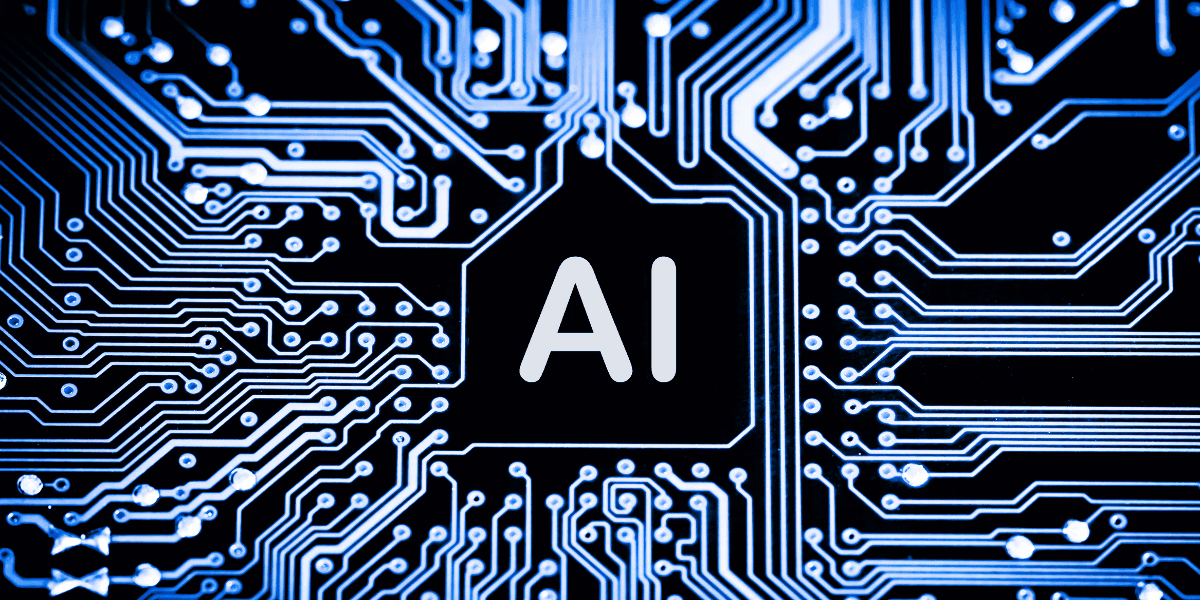
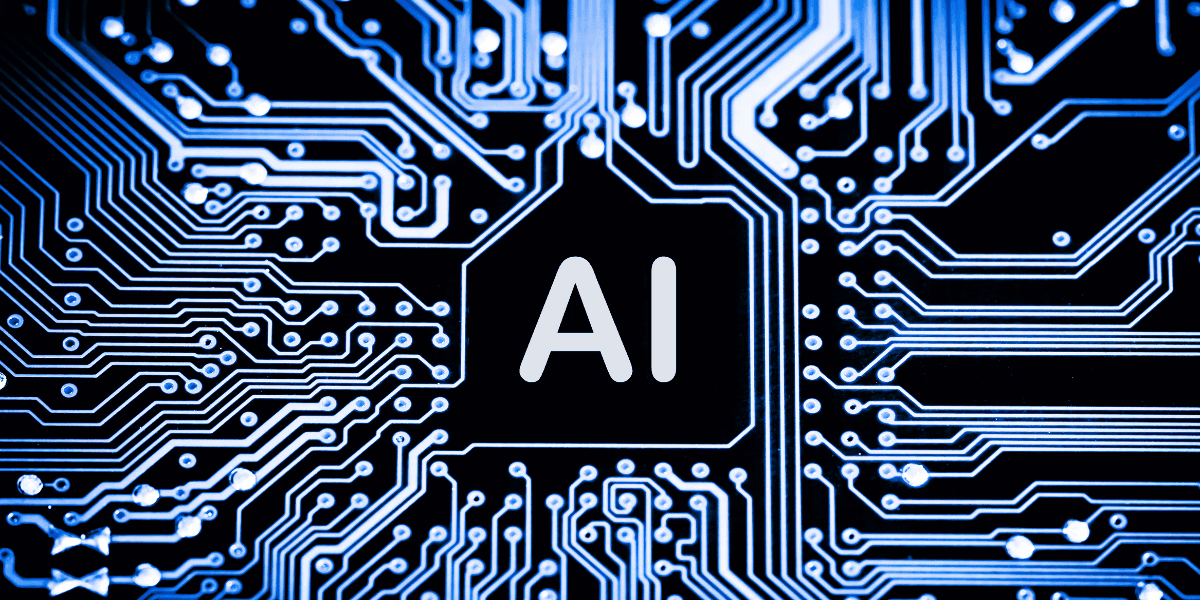
Practical Guide to Fine-Tuning OpenAI GPT Models Using Python

Rehan Asif
Apr 24, 2024
Read the article




Comparing Different Large Language Models (LLM)

Rehan Asif
Apr 23, 2024
Read the article




Evaluating Large Language Models: Methods And Metrics

Rehan Asif
Apr 22, 2024
Read the article




Significant AI Errors, Mistakes, Failures, and Flaws Companies Encounter

Akshat Gupta
Apr 21, 2024
Read the article




Challenges and Strategies for Implementing Enterprise LLM

Rehan Asif
Apr 20, 2024
Read the article




Enhancing Computer Vision with Synthetic Data: Advantages and Generation Techniques
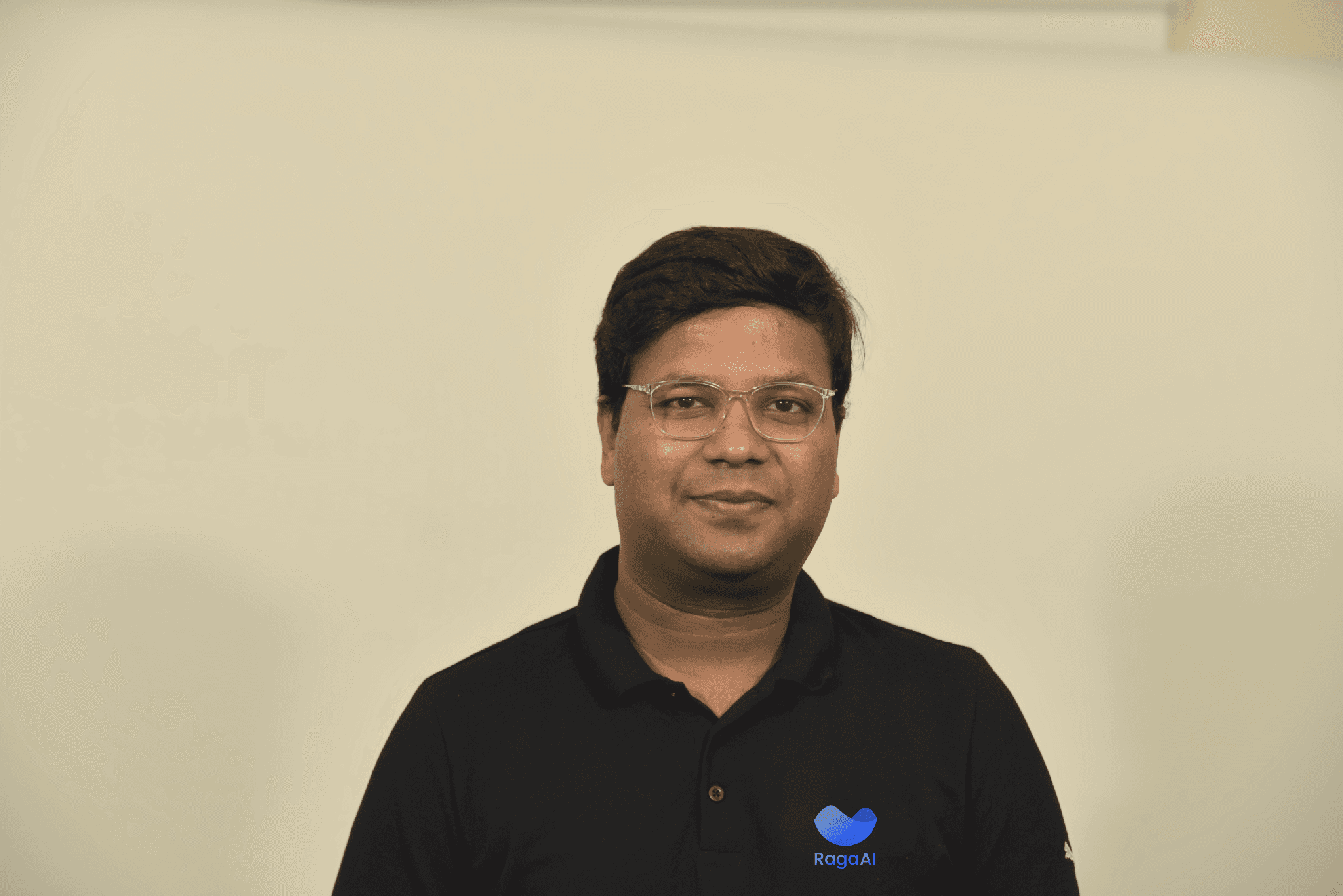
Jigar Gupta
Apr 20, 2024
Read the article
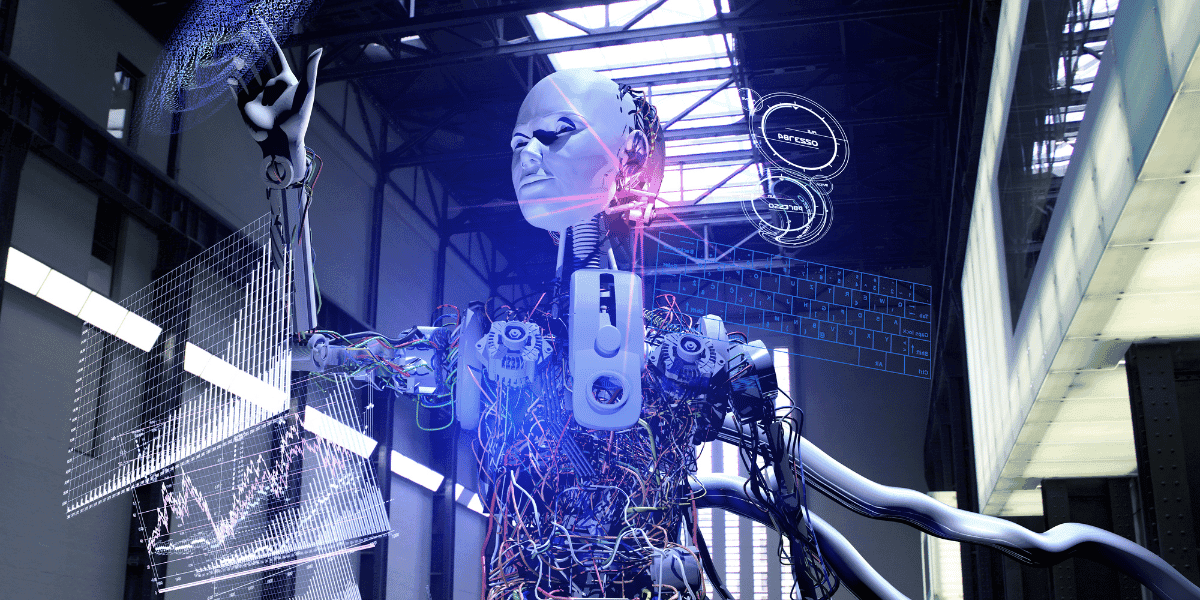
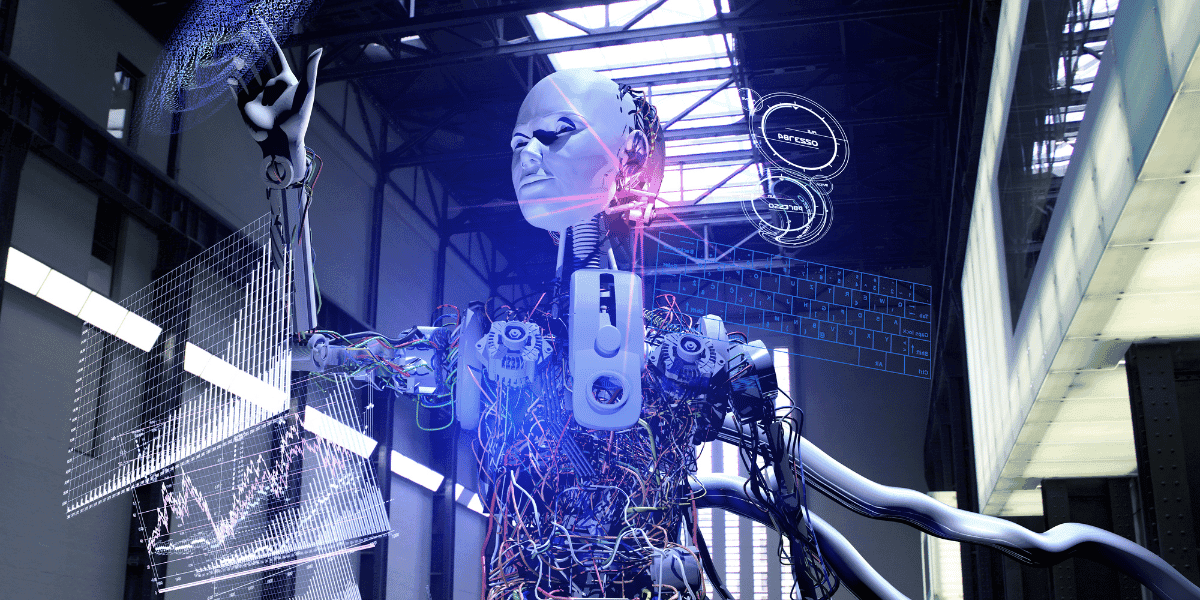
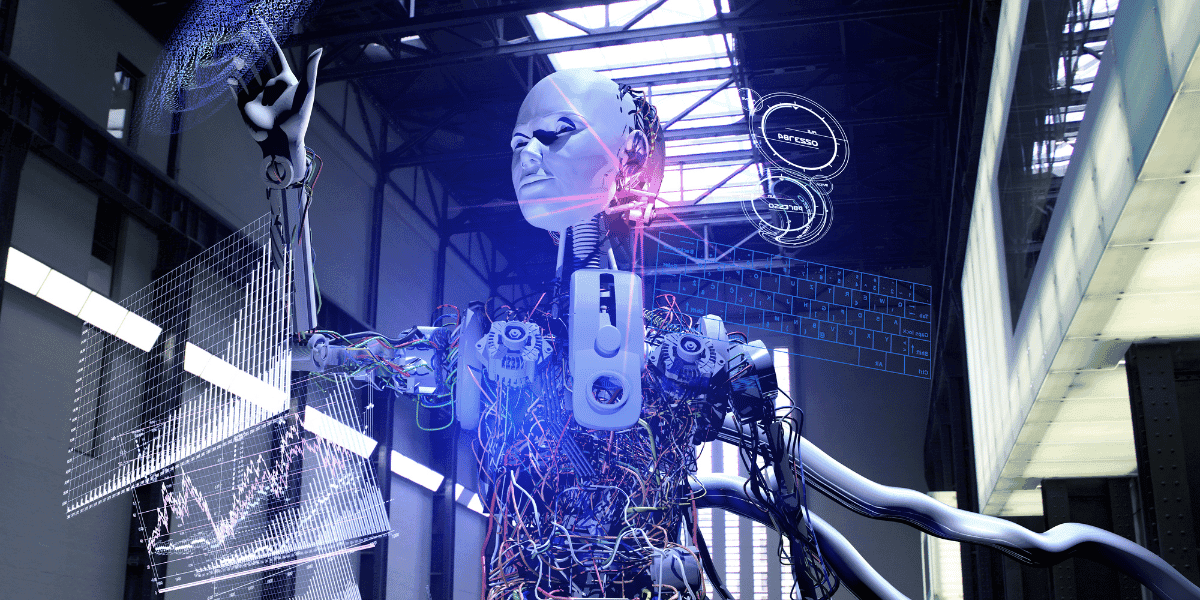
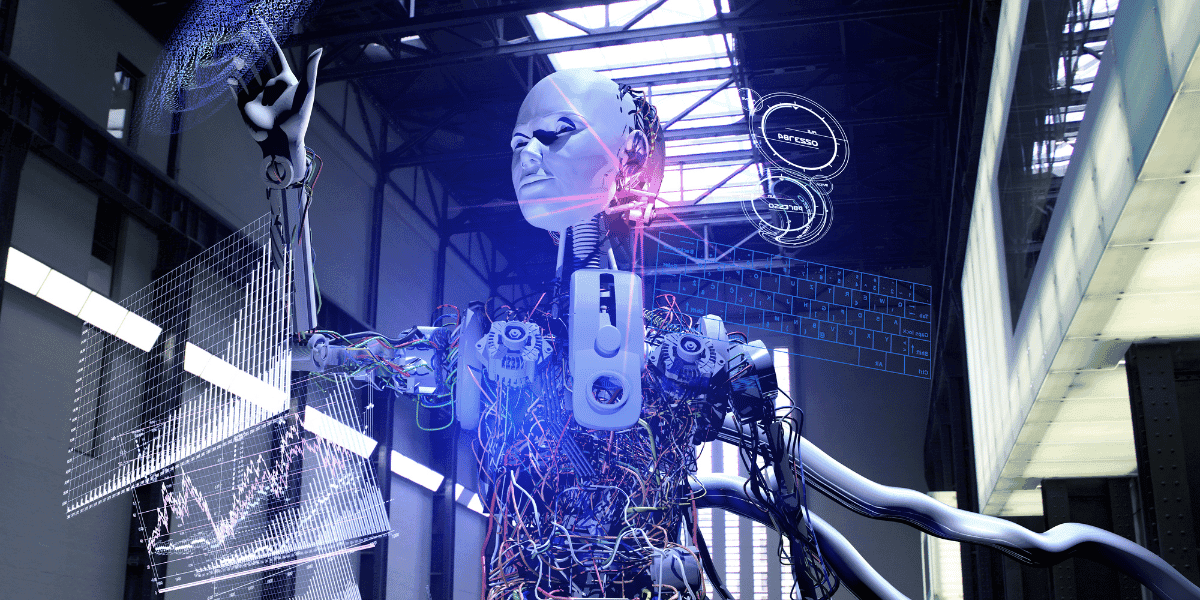
Building Trust In Artificial Intelligence Systems

Akshat Gupta
Apr 19, 2024
Read the article




A Brief Guide To LLM Parameters: Tuning and Optimization

Rehan Asif
Apr 18, 2024
Read the article




Unlocking The Potential Of Computer Vision Testing: Key Techniques And Tools
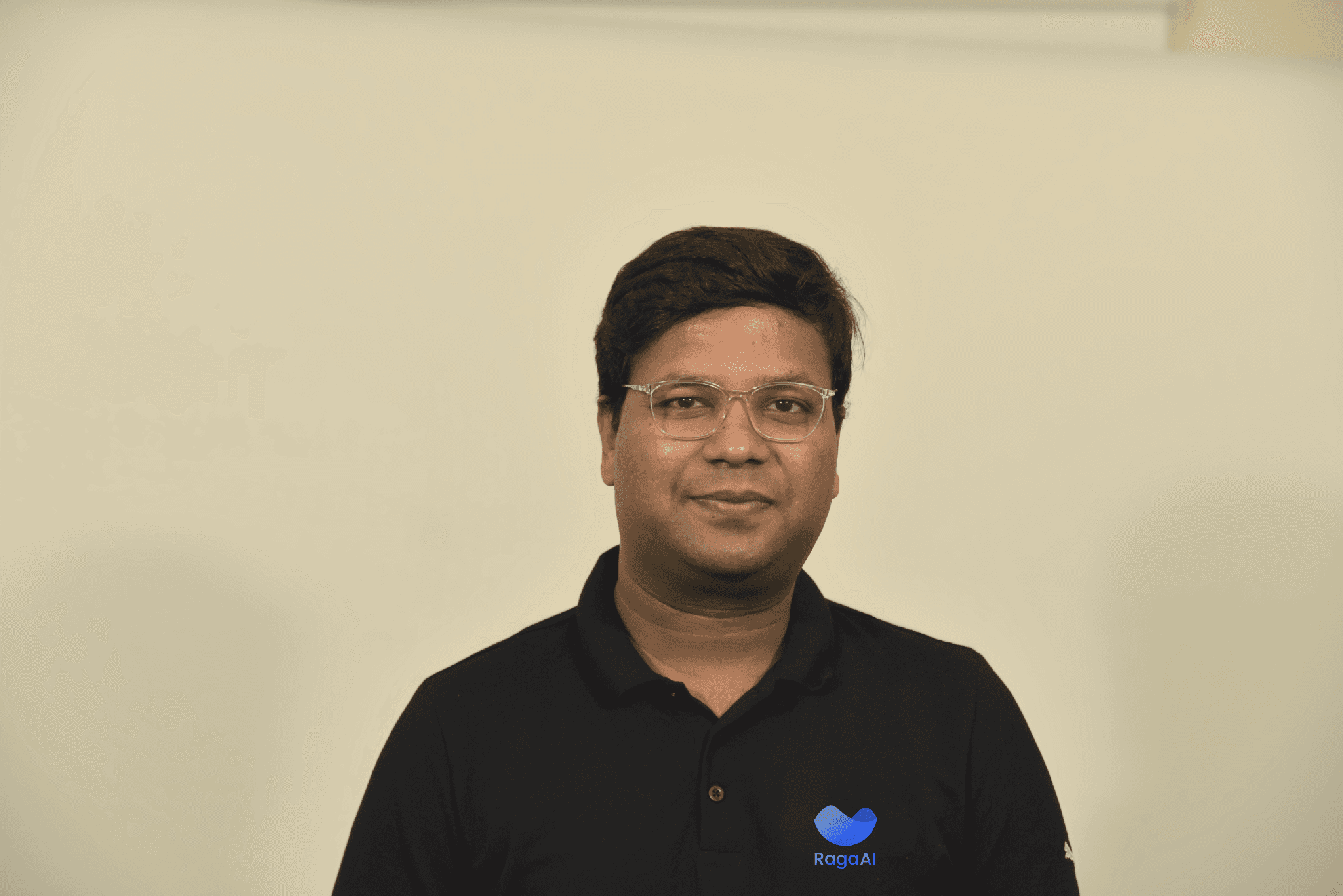
Jigar Gupta
Apr 17, 2024
Read the article




Understanding AI Regulatory Compliance And Its Importance

Akshat Gupta
Apr 16, 2024
Read the article
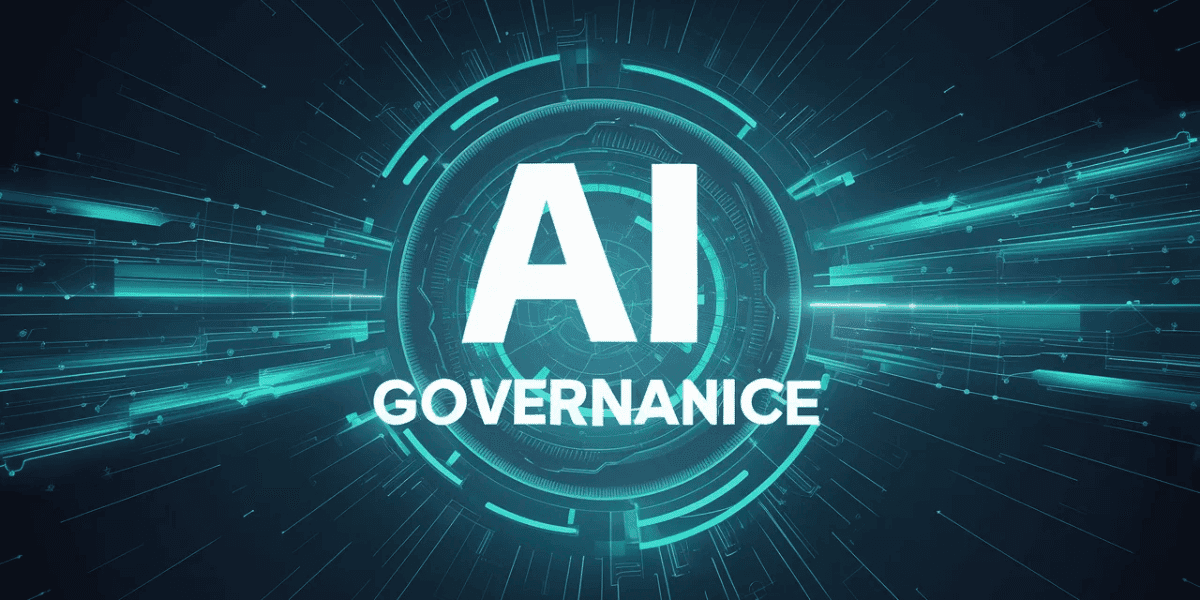
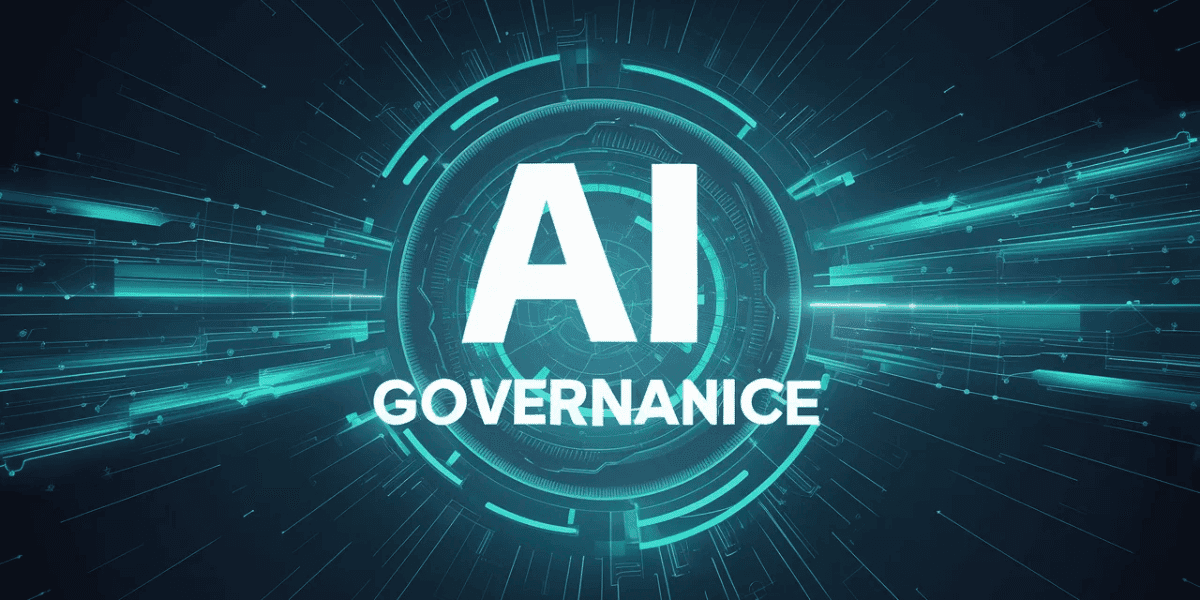
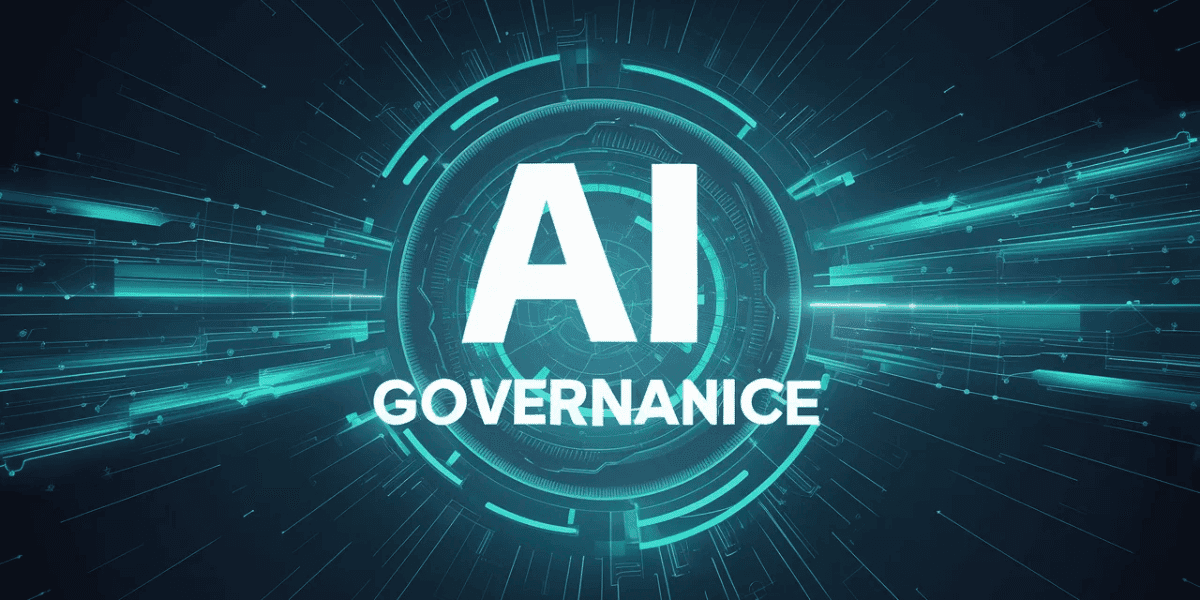
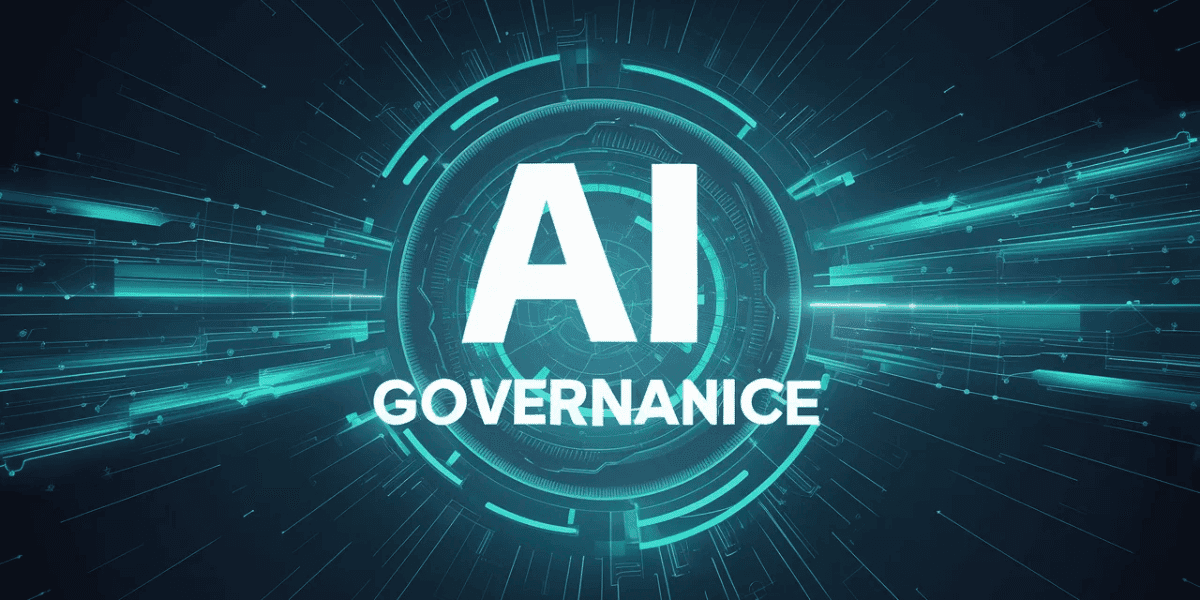
Understanding The Basics Of AI Governance

Akshat Gupta
Apr 15, 2024
Read the article




Understanding Prompt Engineering: A Guide

Rehan Asif
Apr 15, 2024
Read the article
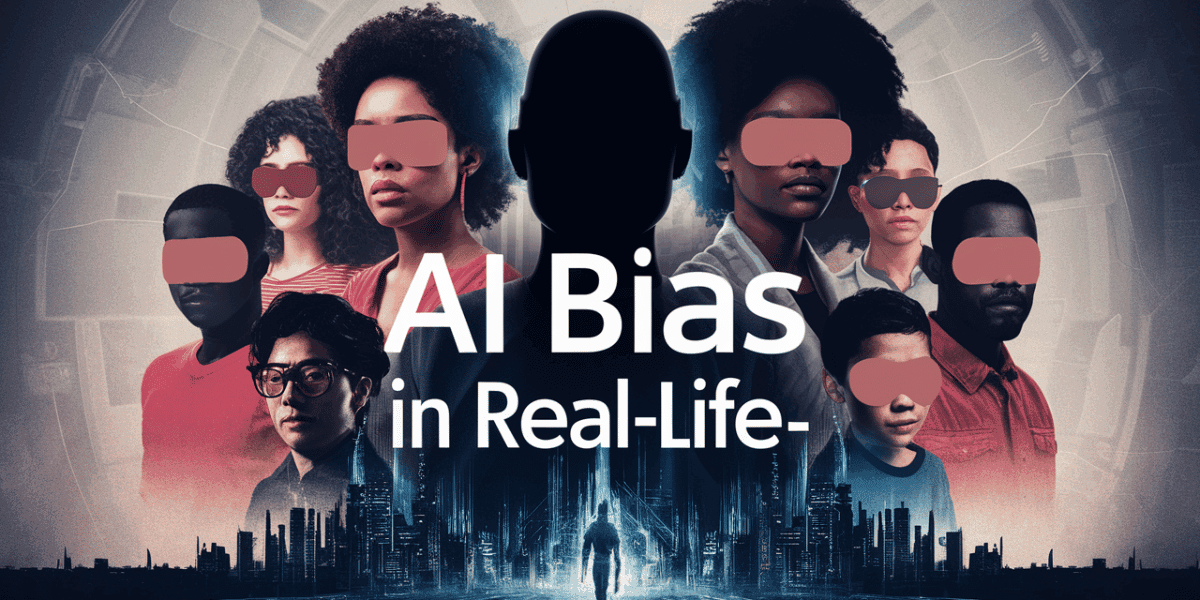
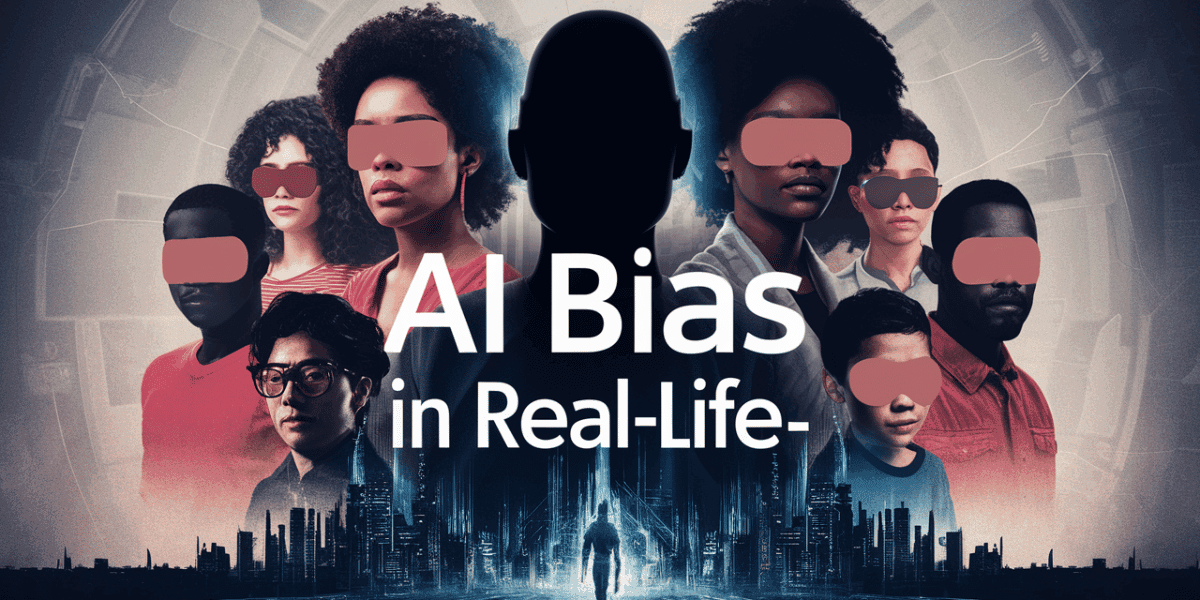
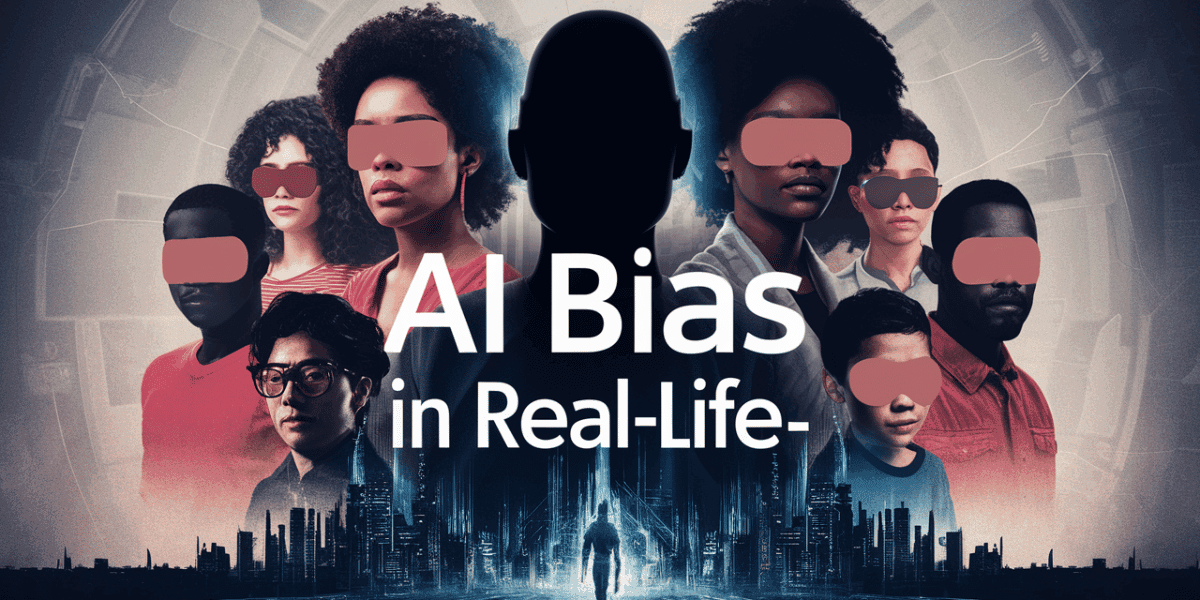
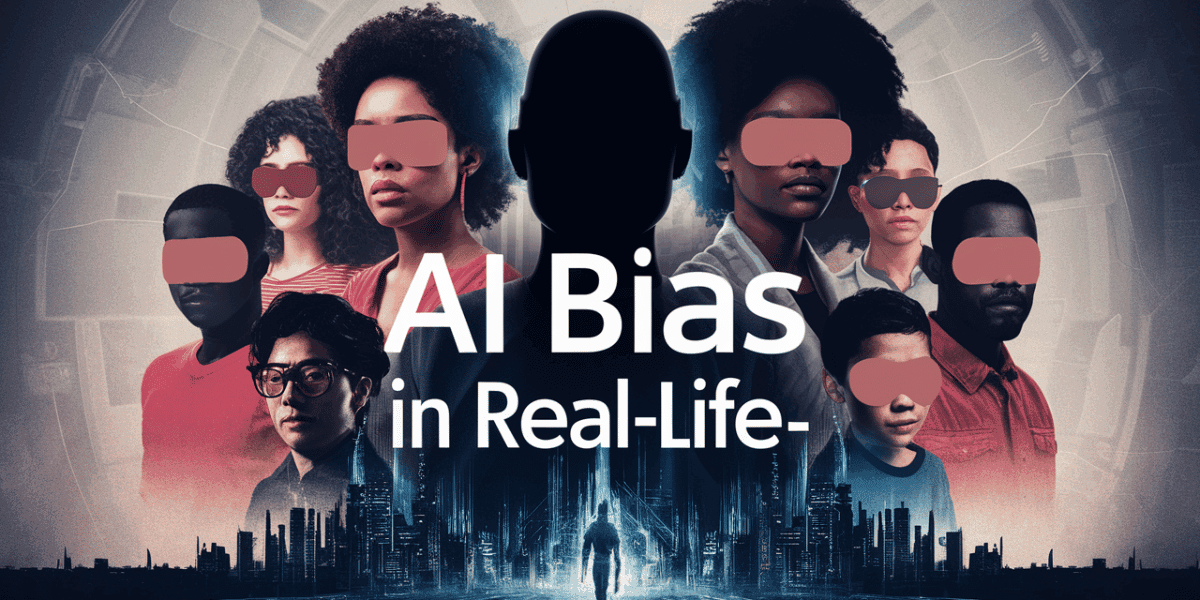
Examples And Strategies To Mitigate AI Bias In Real-Life

Akshat Gupta
Apr 14, 2024
Read the article




Understanding The Basics Of LLM Fine-tuning With Custom Data

Rehan Asif
Apr 13, 2024
Read the article
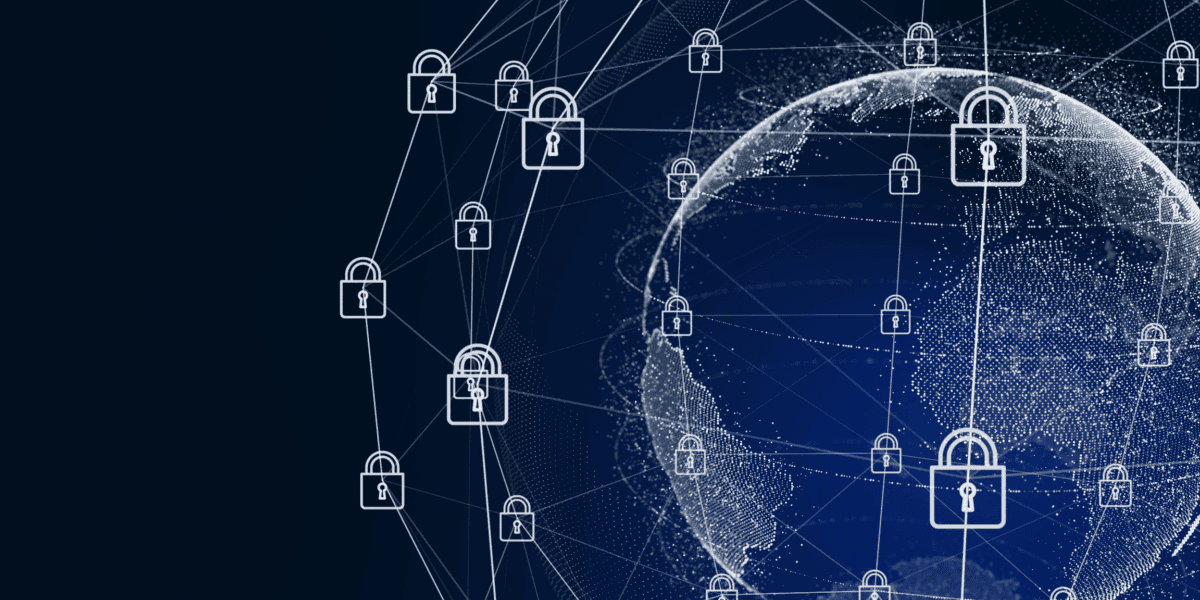
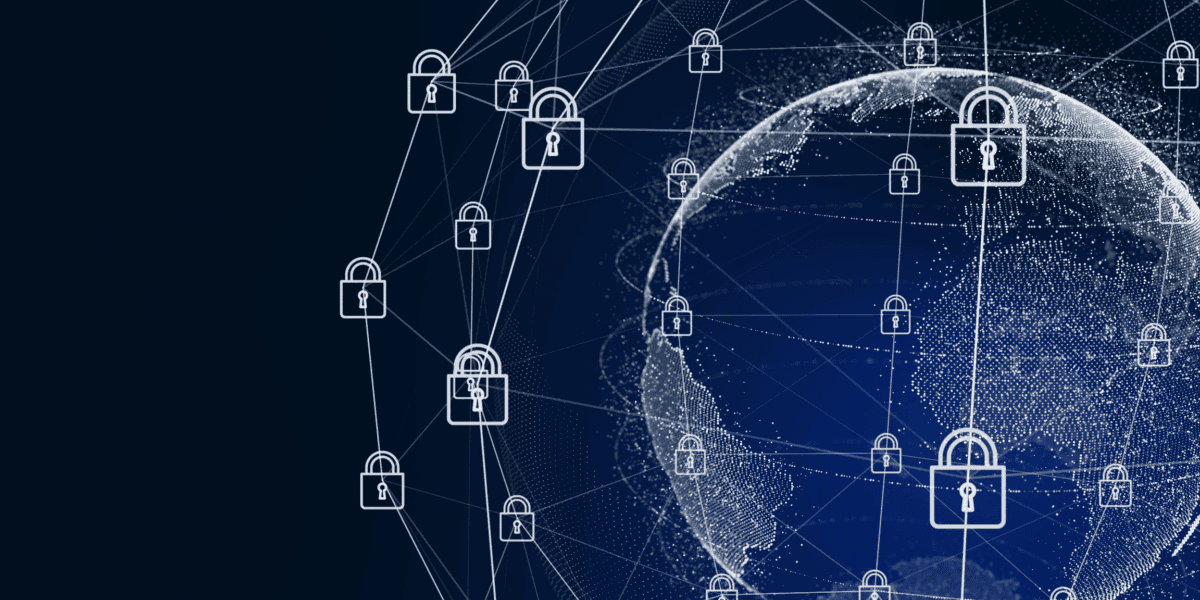
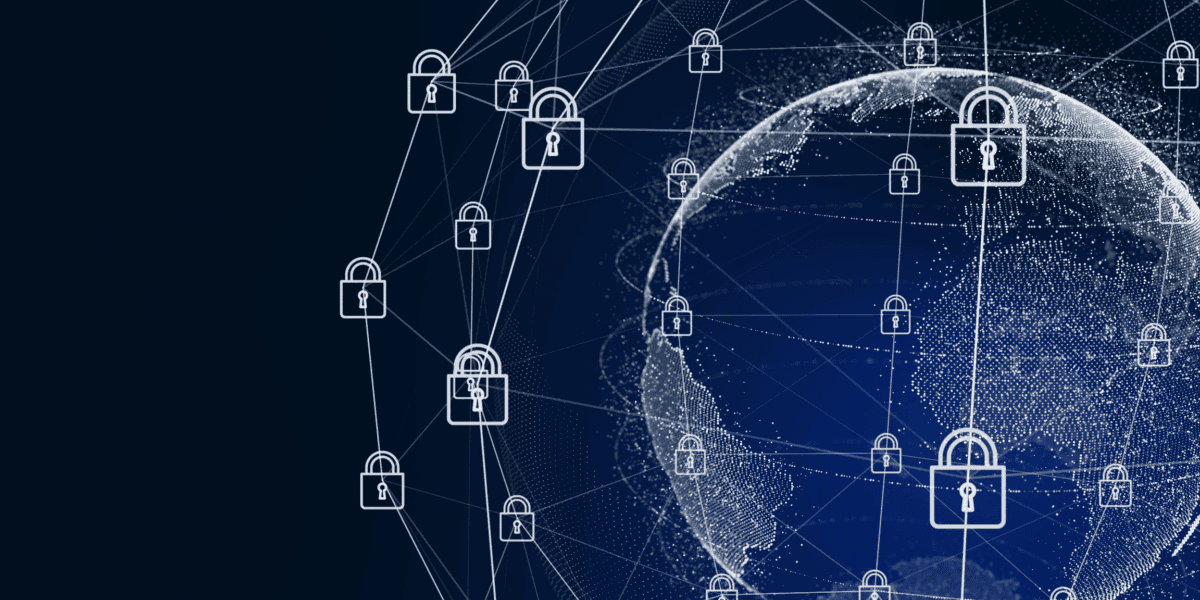
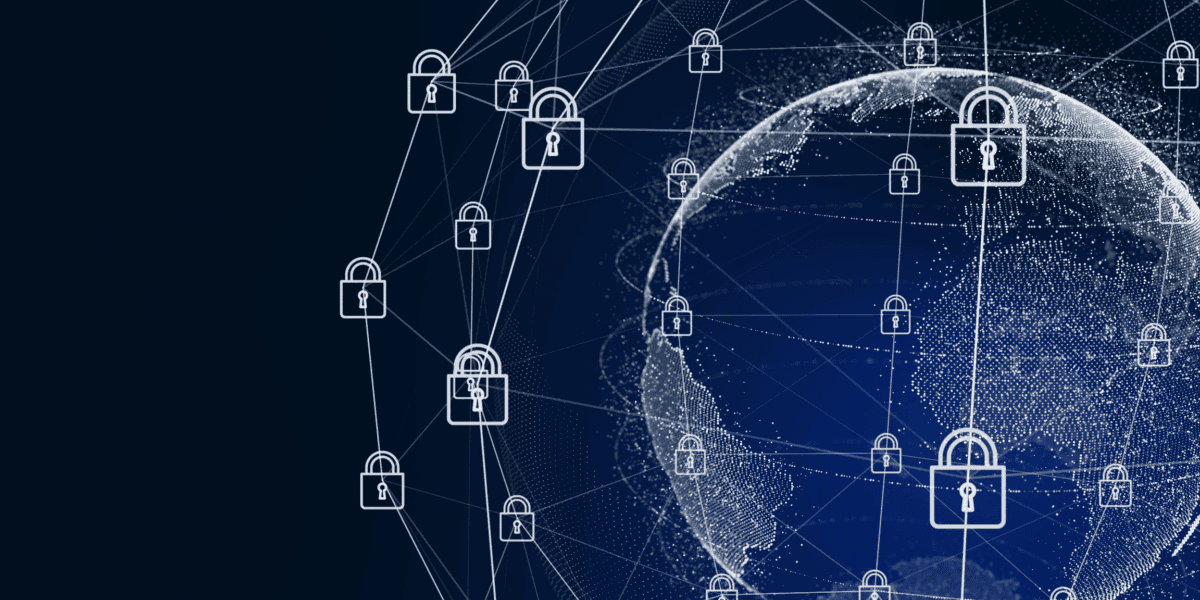
Overview Of Key Concepts In AI Safety And Security
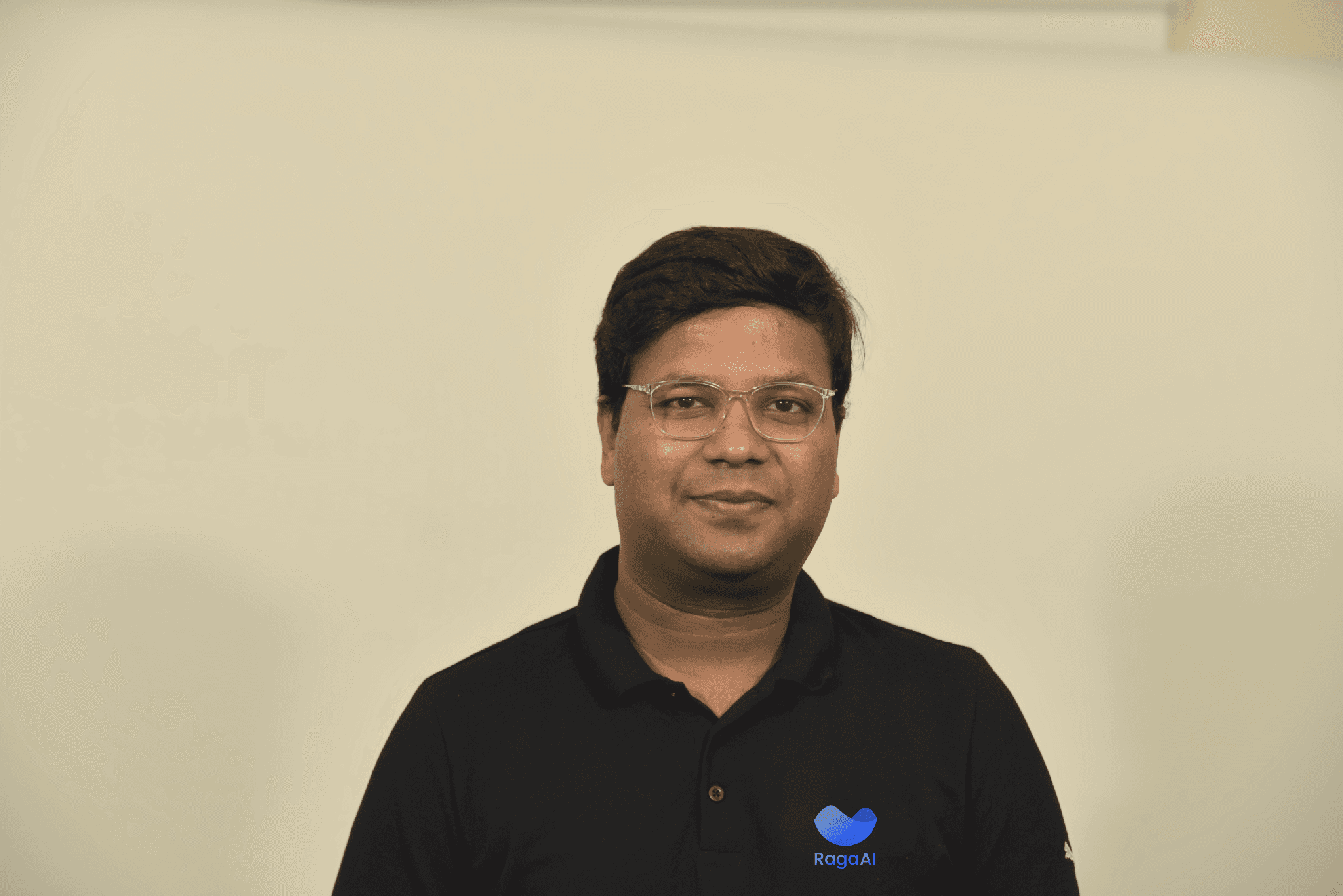
Jigar Gupta
Apr 12, 2024
Read the article




Understanding Hallucinations In LLMs

Rehan Asif
Apr 7, 2024
Read the article




Demystifying FDA's Approach to AI/ML in Healthcare: Your Ultimate Guide
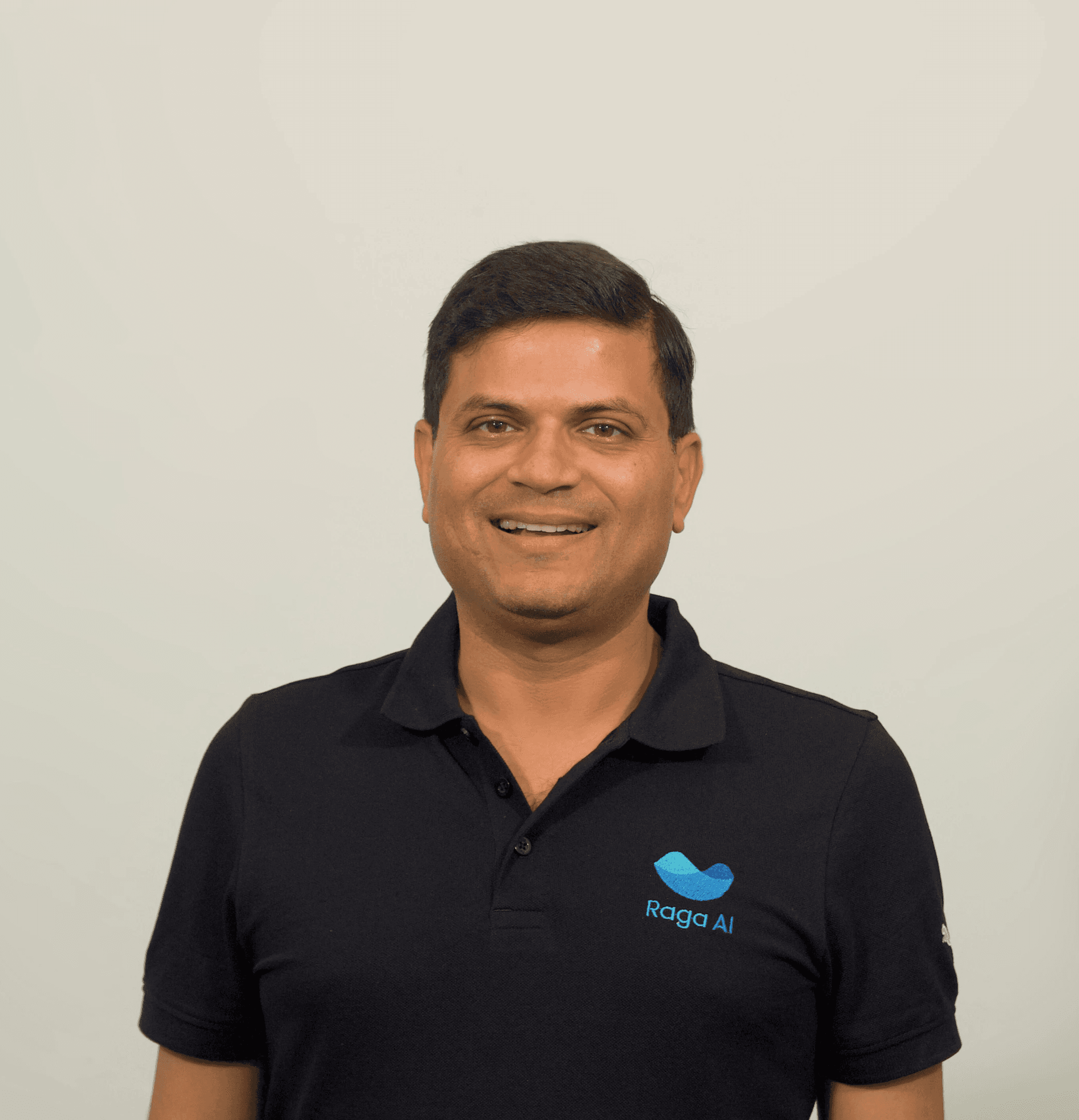
Gaurav Agarwal
Apr 4, 2024
Read the article




Navigating AI Governance in Aerospace Industry

Akshat Gupta
Apr 3, 2024
Read the article




The White House Executive Order on Safe and Trustworthy AI

Jigar Gupta
Mar 29, 2024
Read the article




The EU AI Act - All you need to know
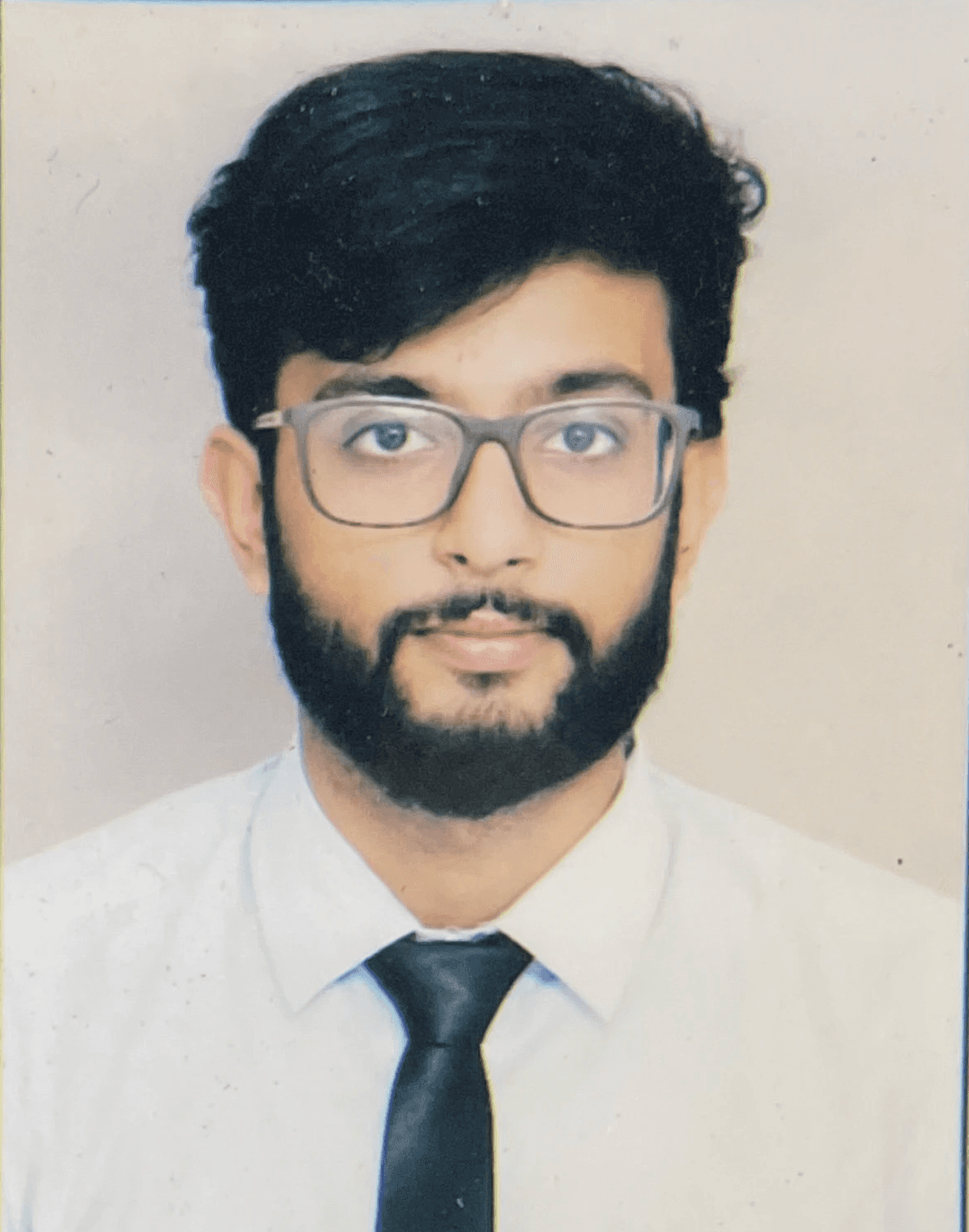
Akshat Gupta
Mar 27, 2024
Read the article
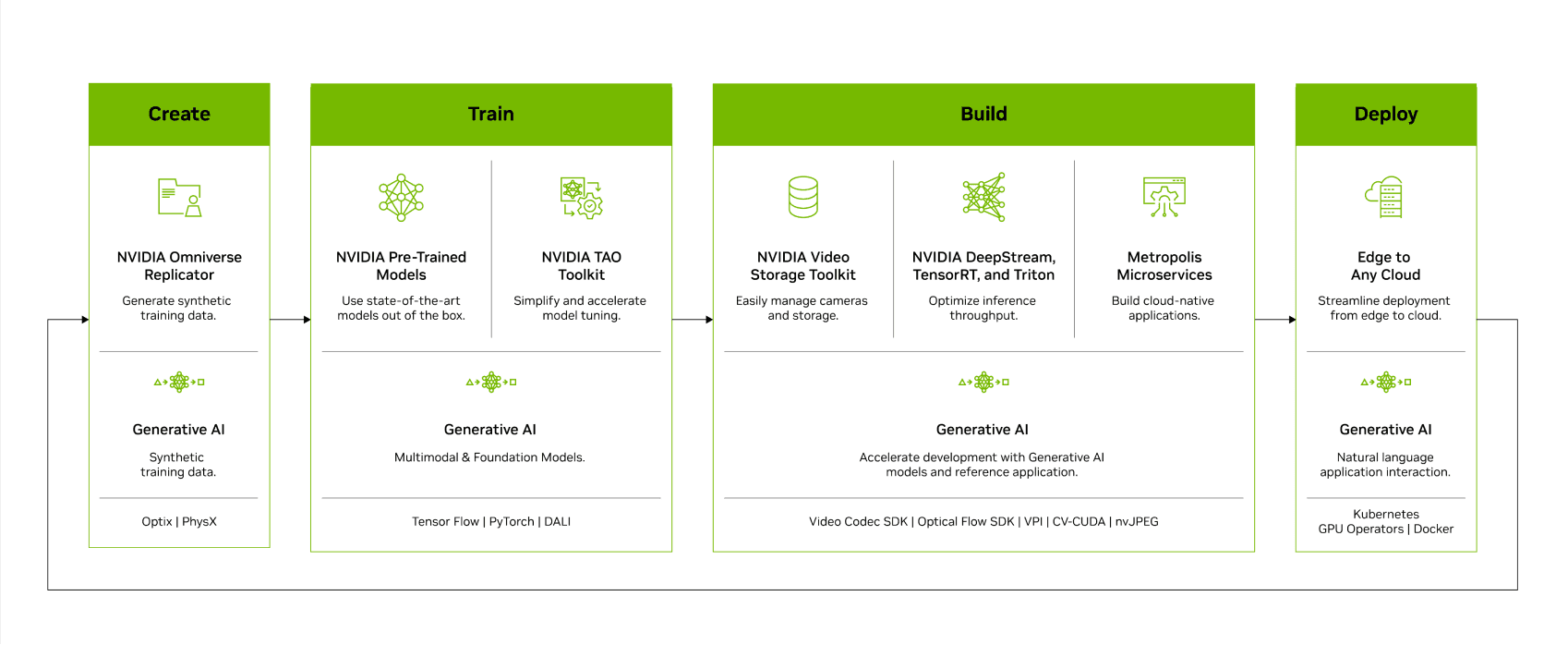
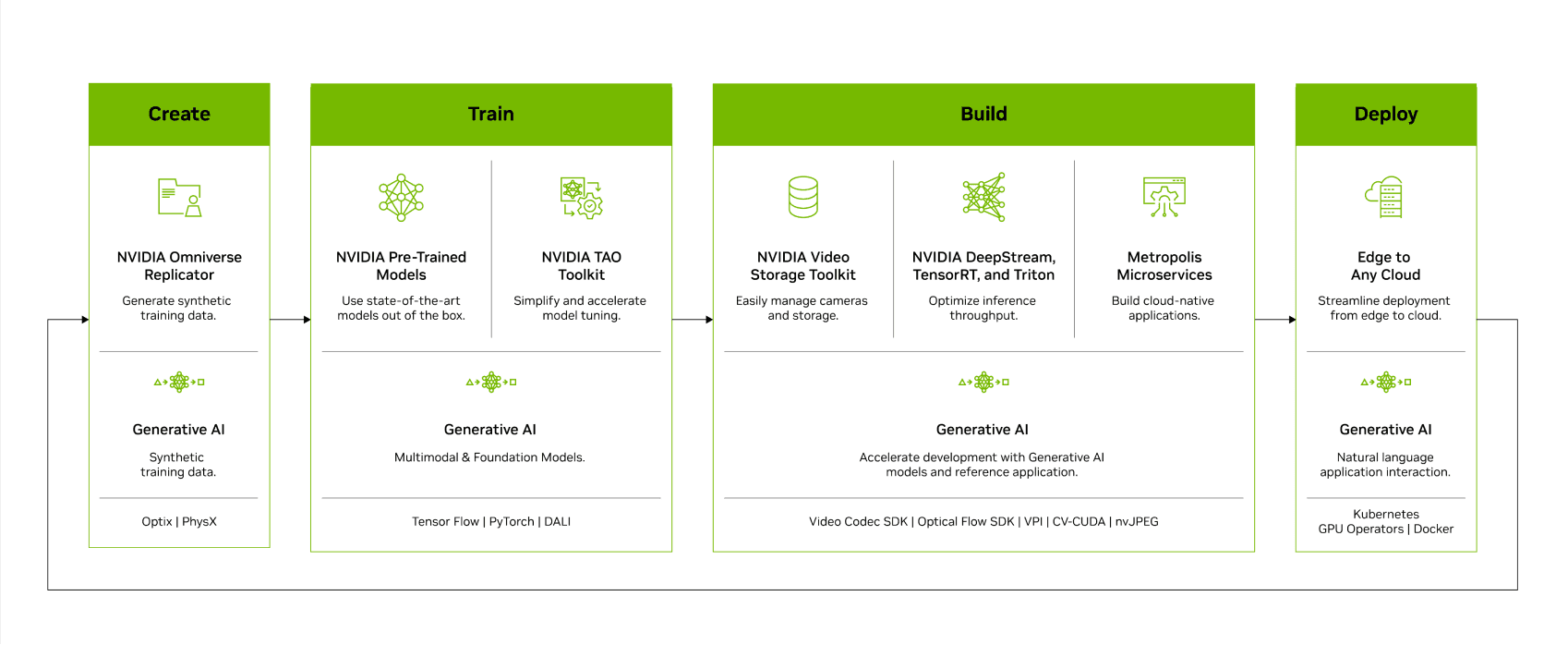
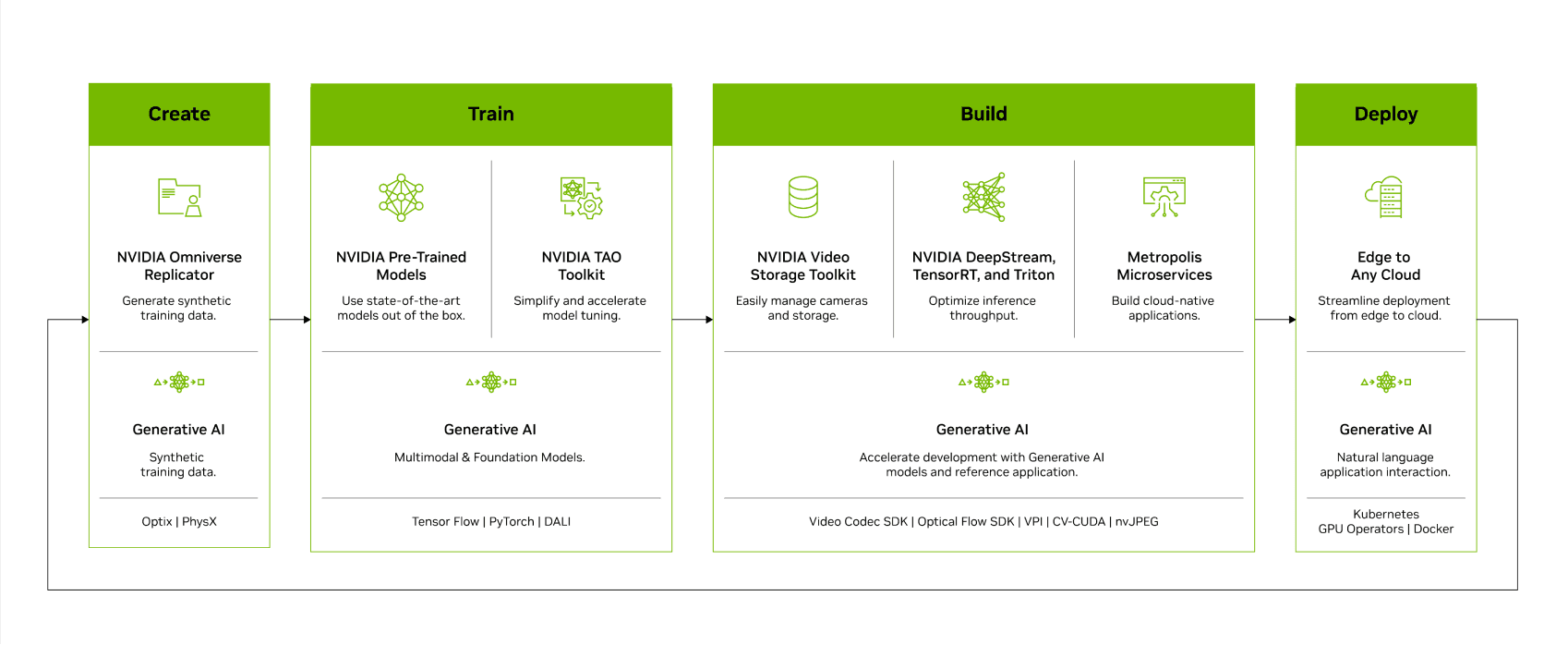
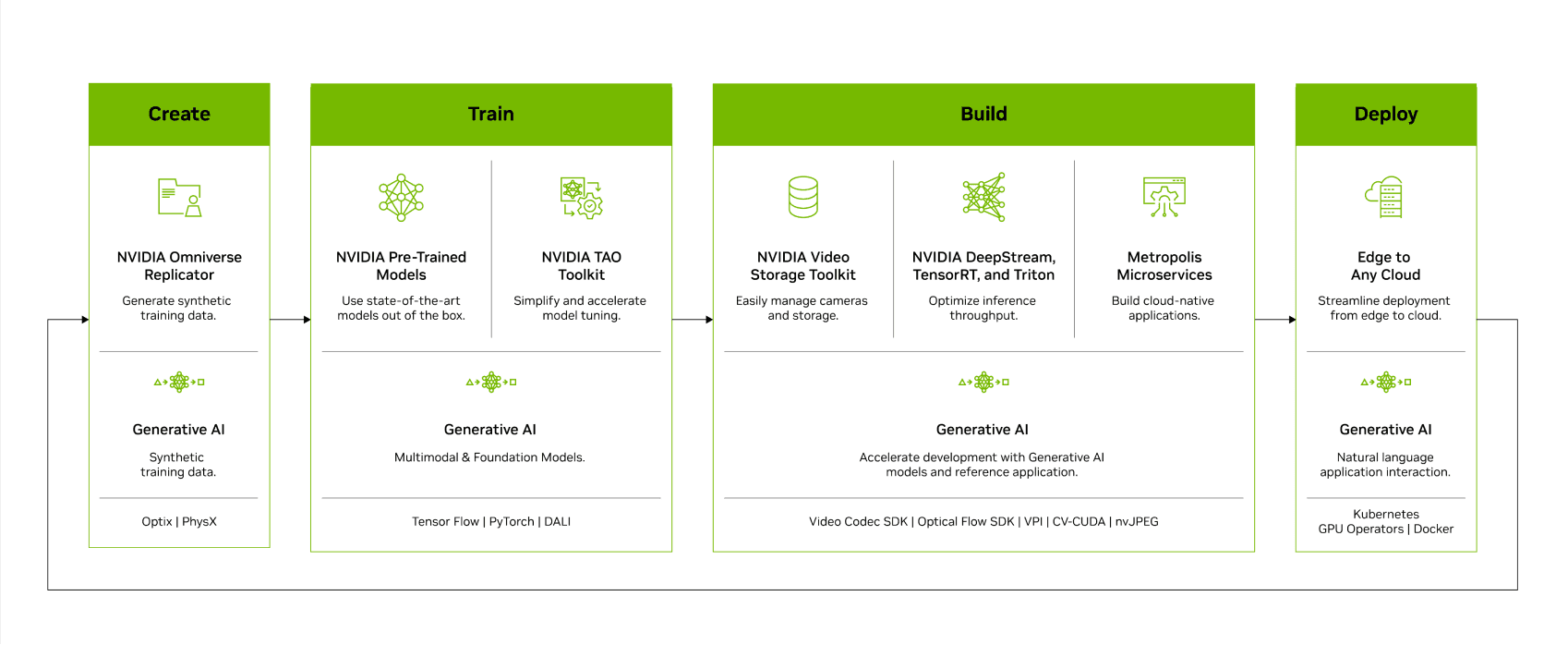
Enhancing Edge AI with RagaAI Integration on NVIDIA Metropolis

Siddharth Jain
Mar 15, 2024
Read the article




RagaAI releases the most comprehensive open-source LLM Evaluation and Guardrails package

Gaurav Agarwal
Mar 7, 2024
Read the article
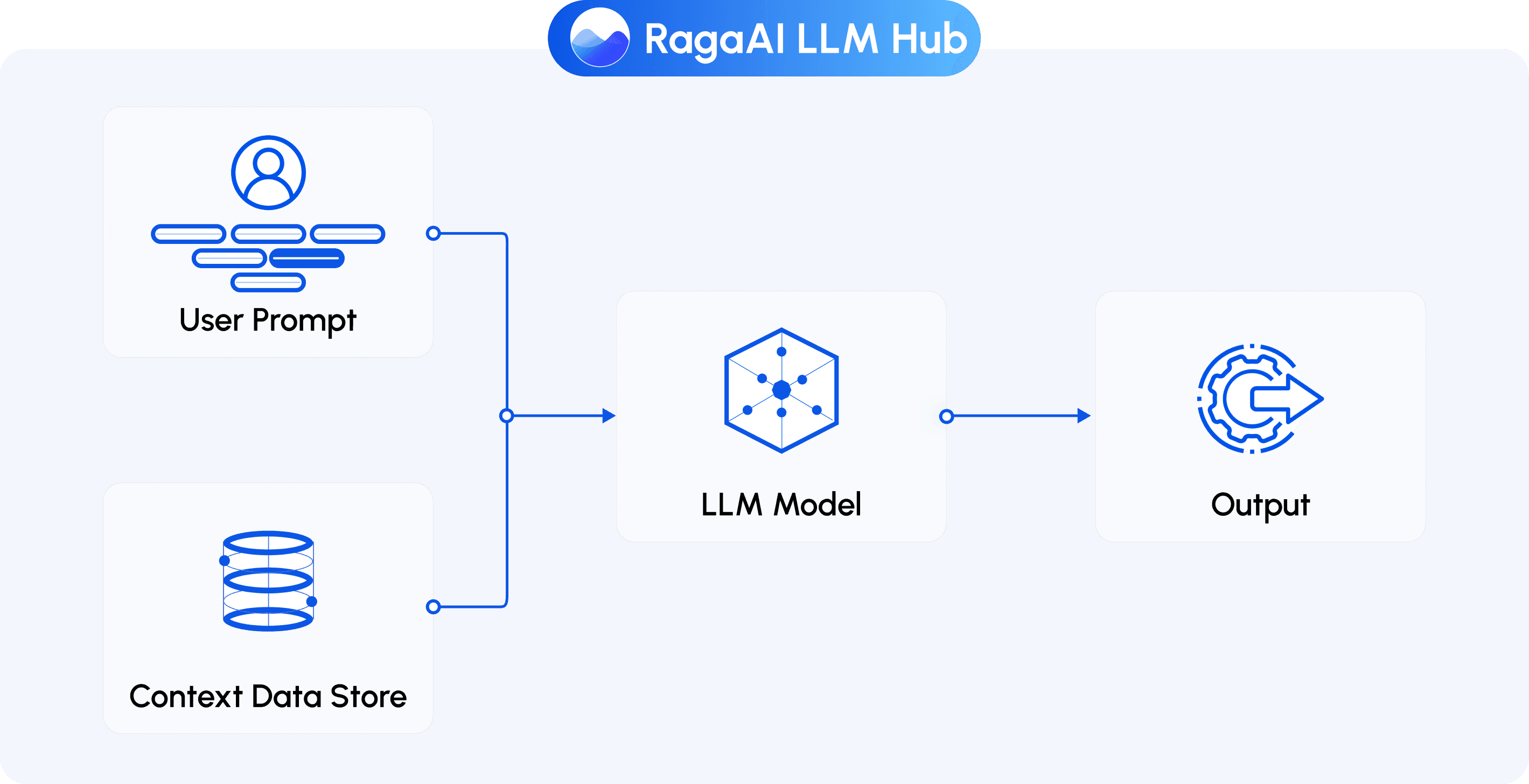
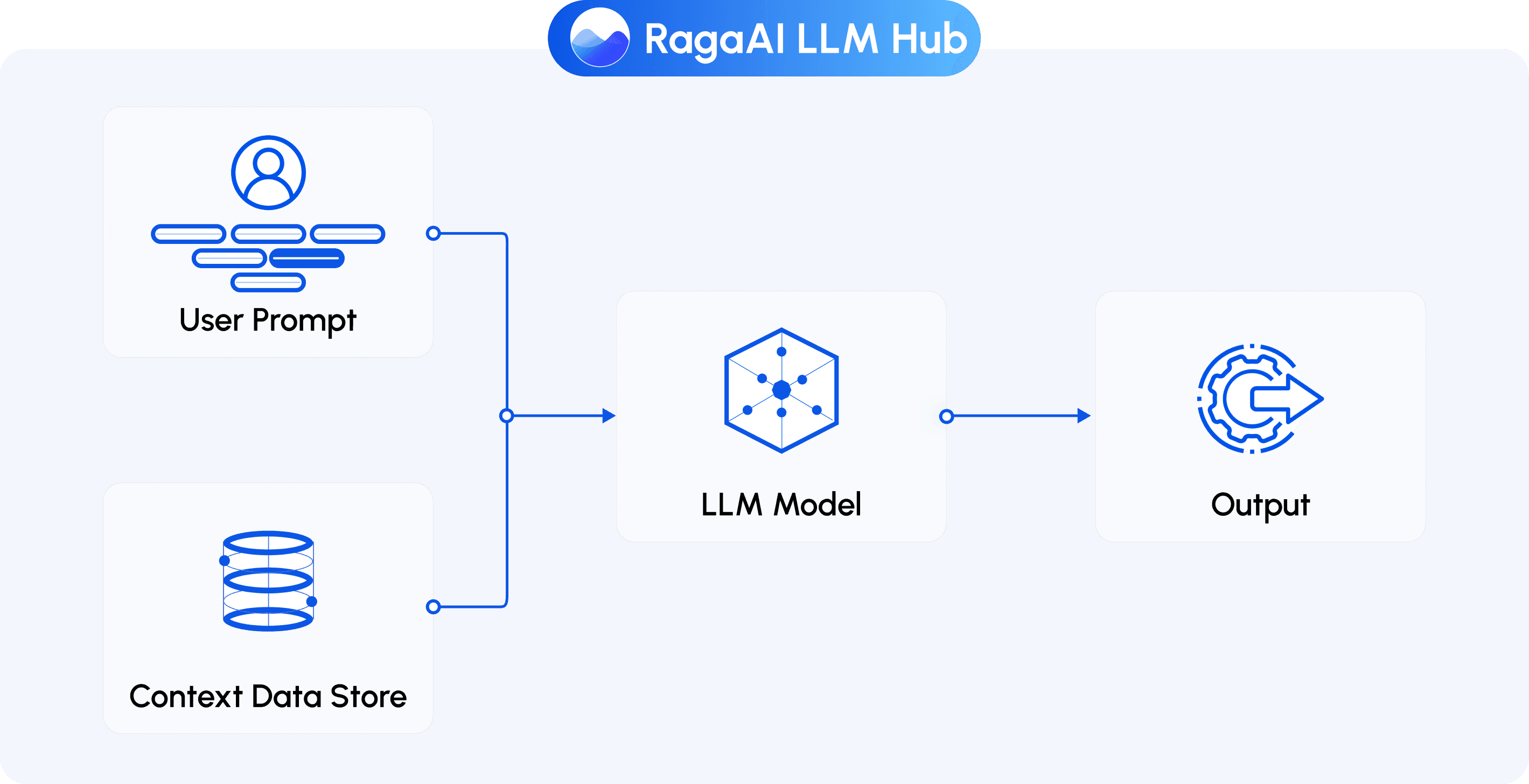
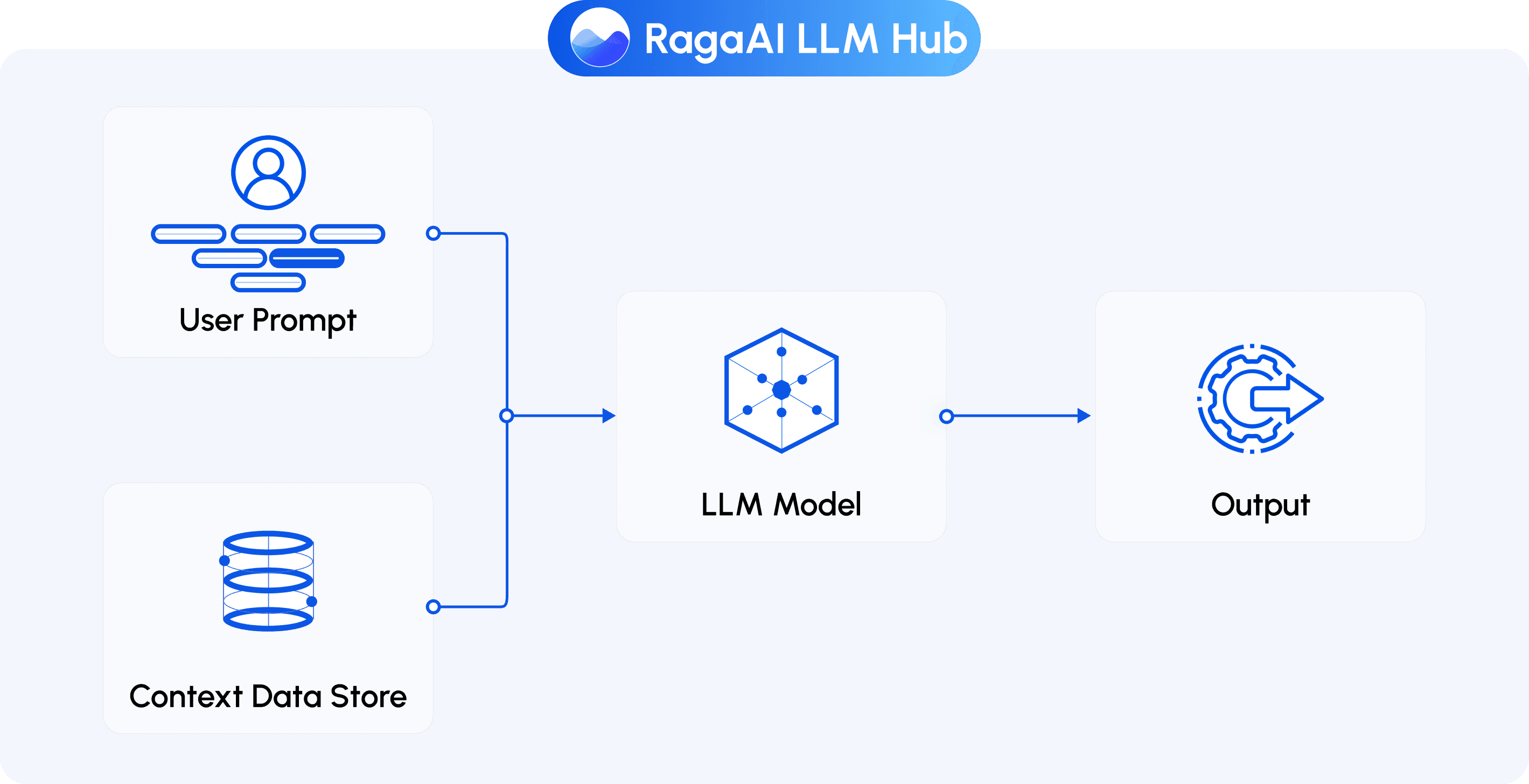
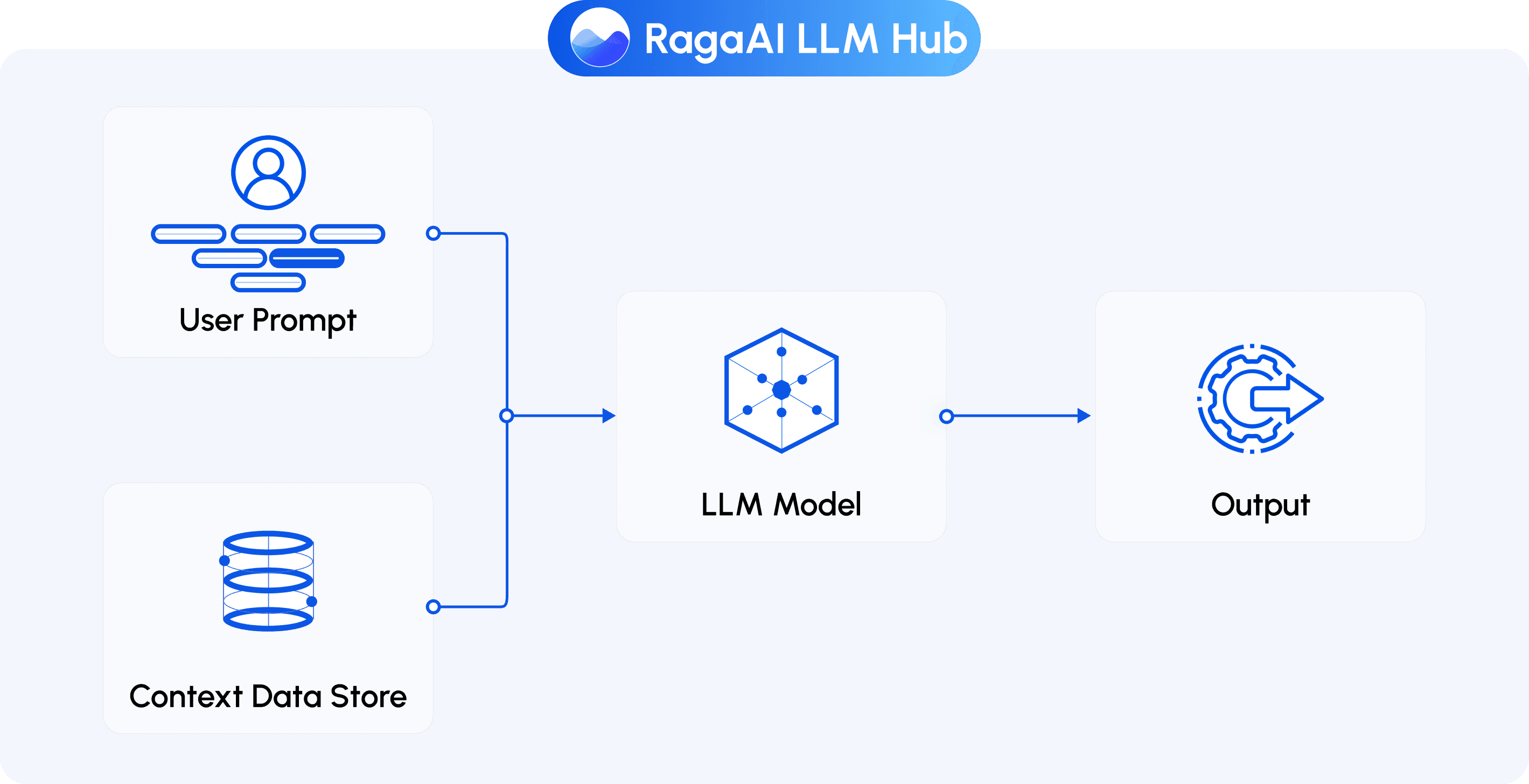
A Guide to Evaluating LLM Applications and enabling Guardrails using Raga-LLM-Hub

Rehan Asif
Mar 7, 2024
Read the article




Identifying edge cases within CelebA Dataset using RagaAI testing Platform

Rehan Asif
Feb 15, 2024
Read the article
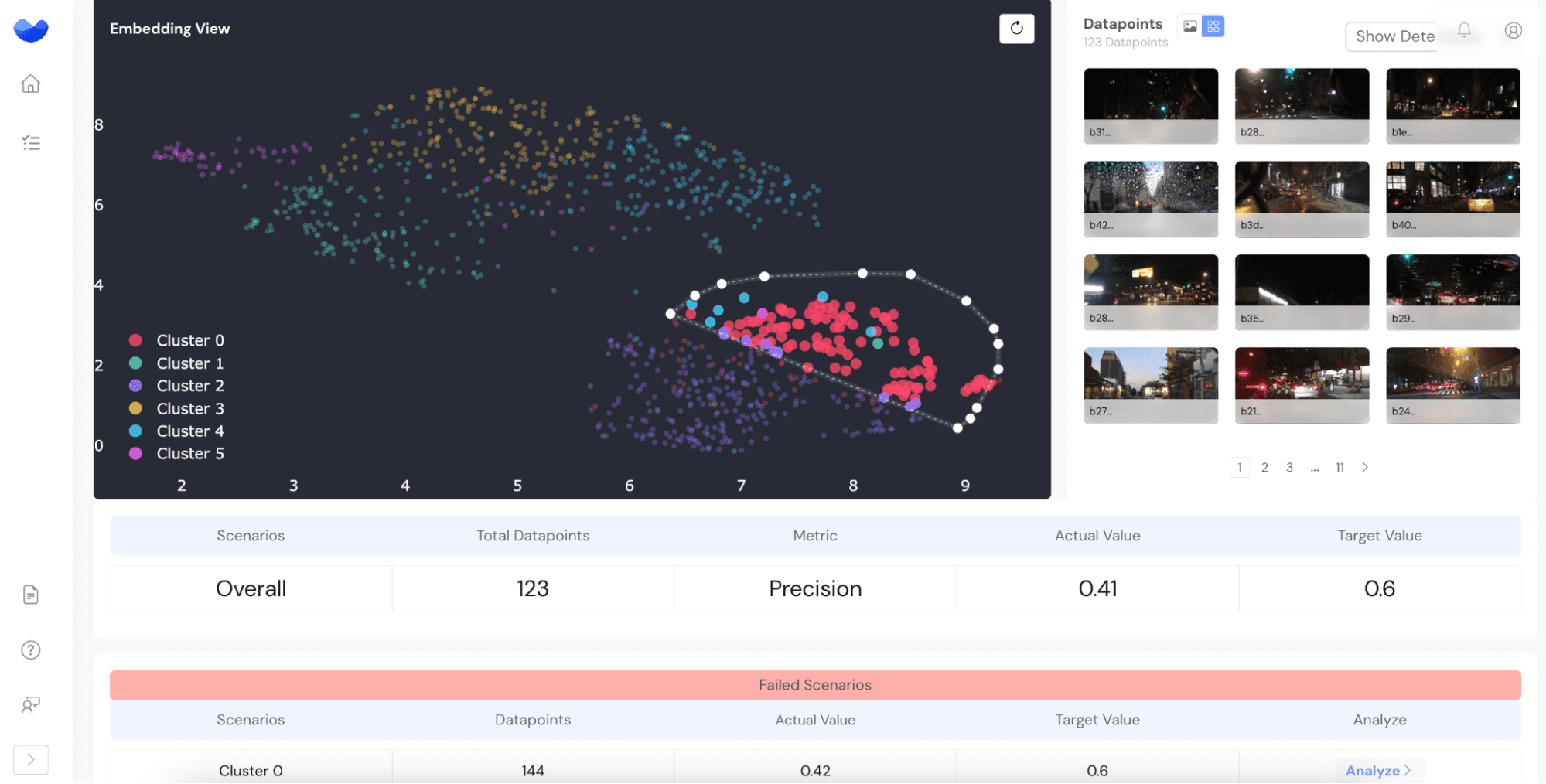
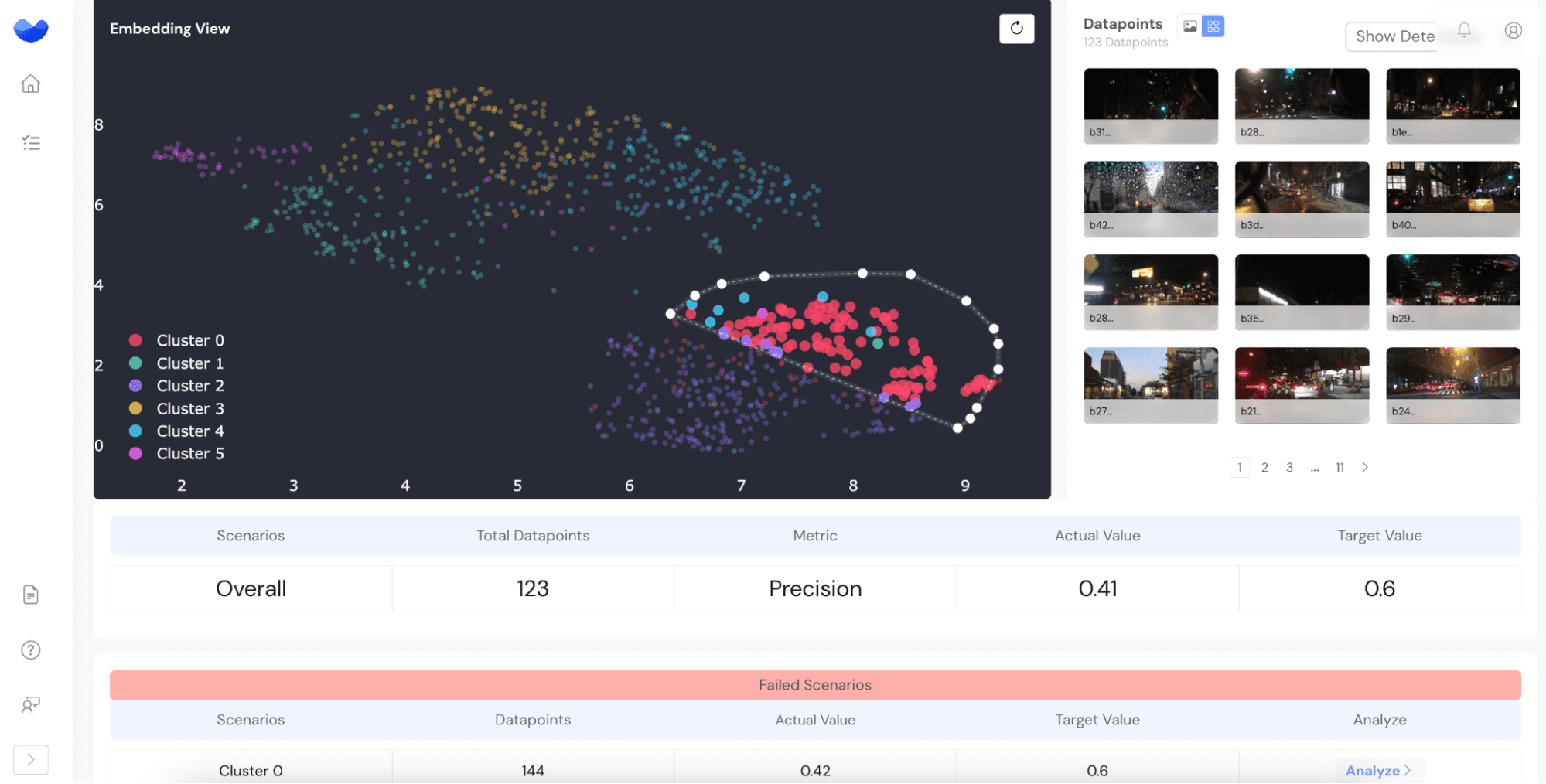
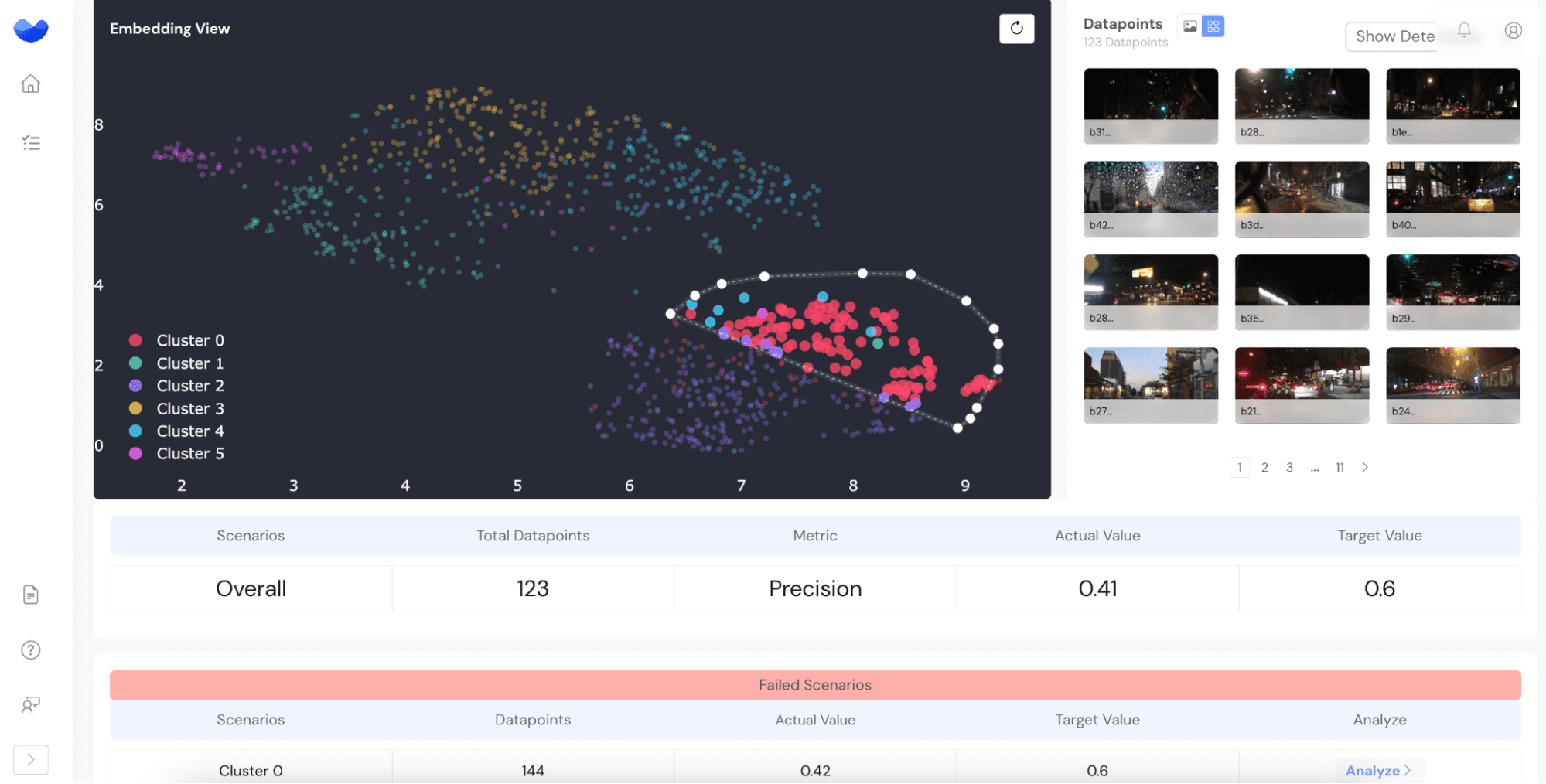
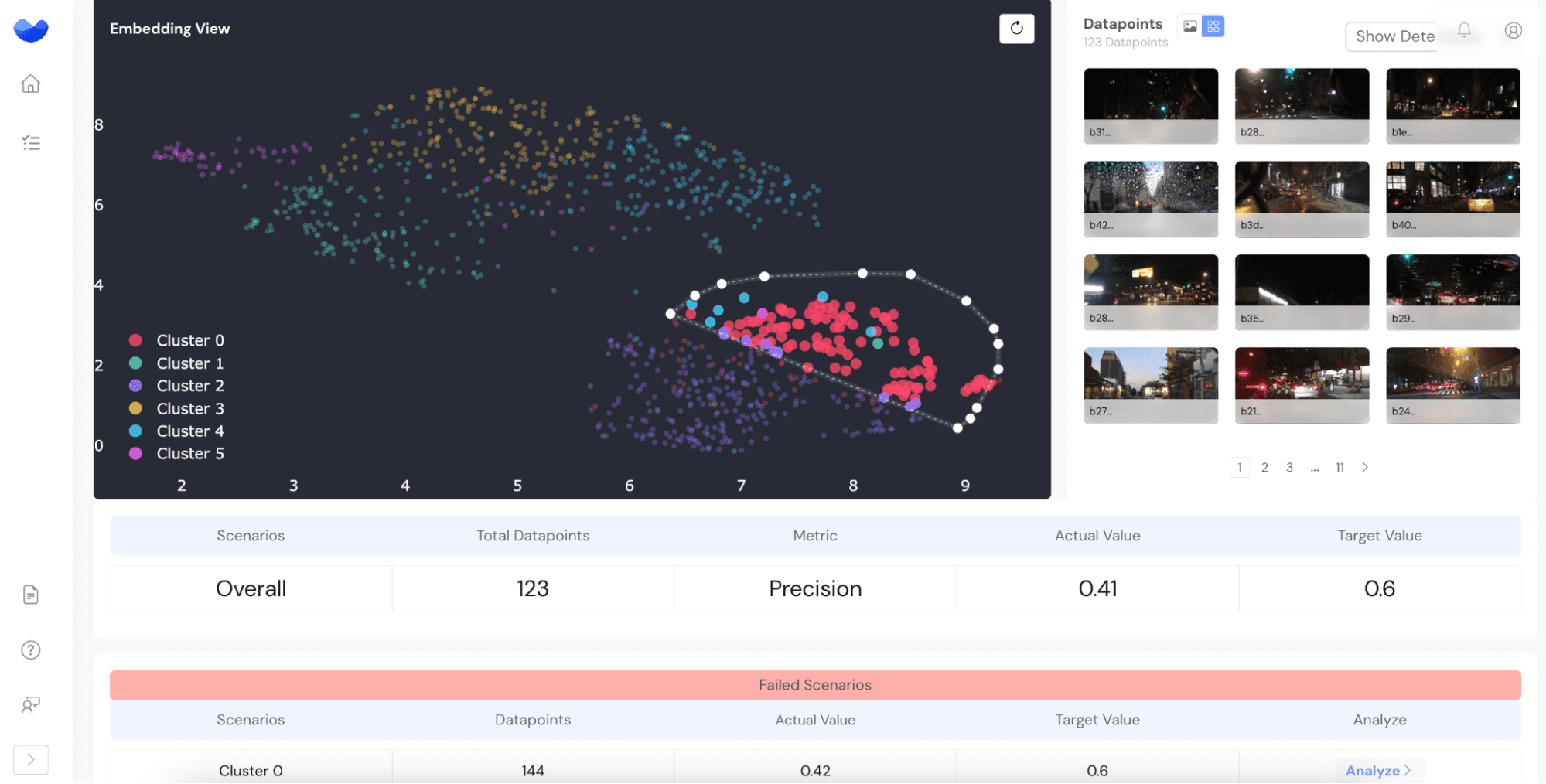
How to Detect and Fix AI Issues with RagaAI
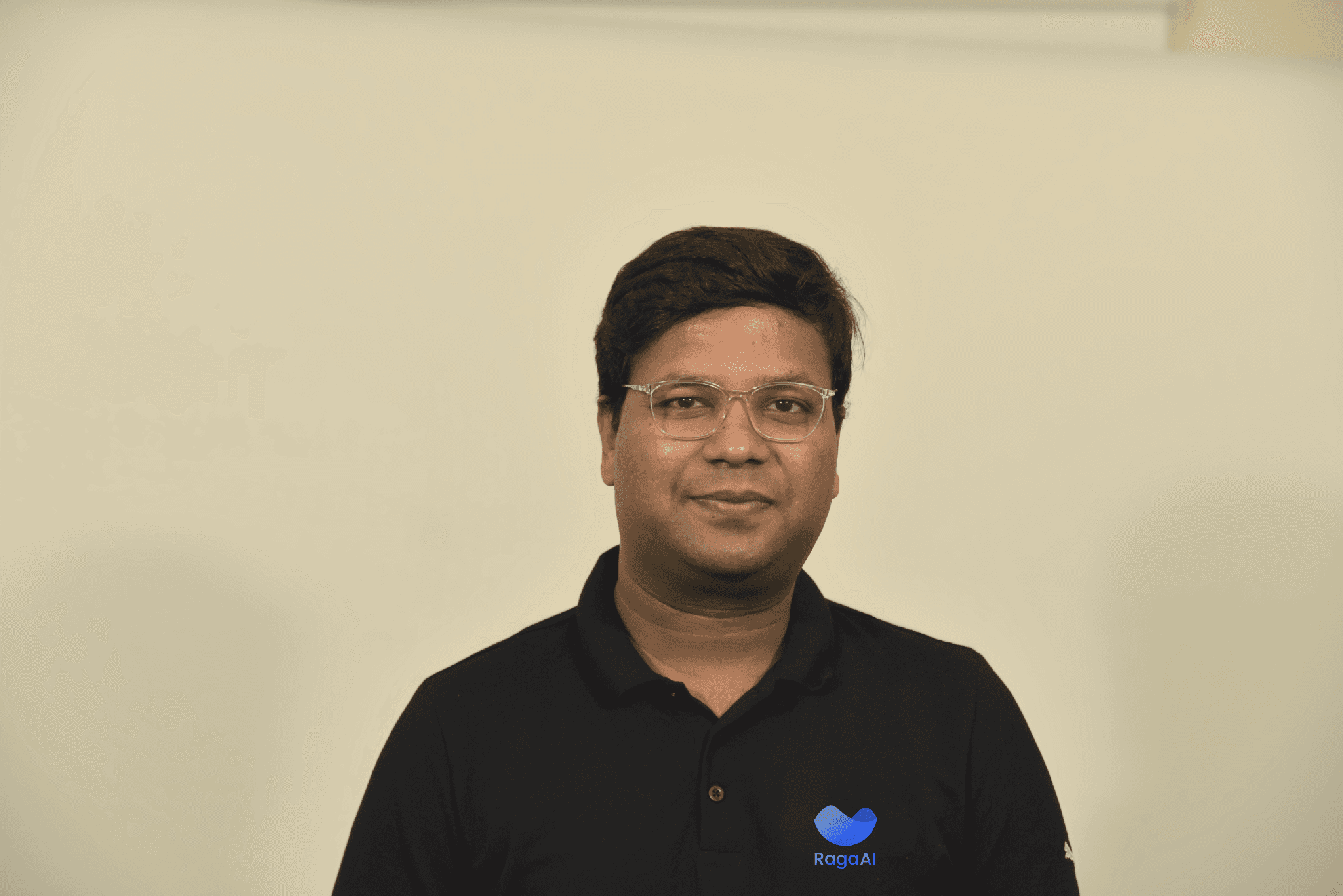
Jigar Gupta
Feb 16, 2024
Read the article
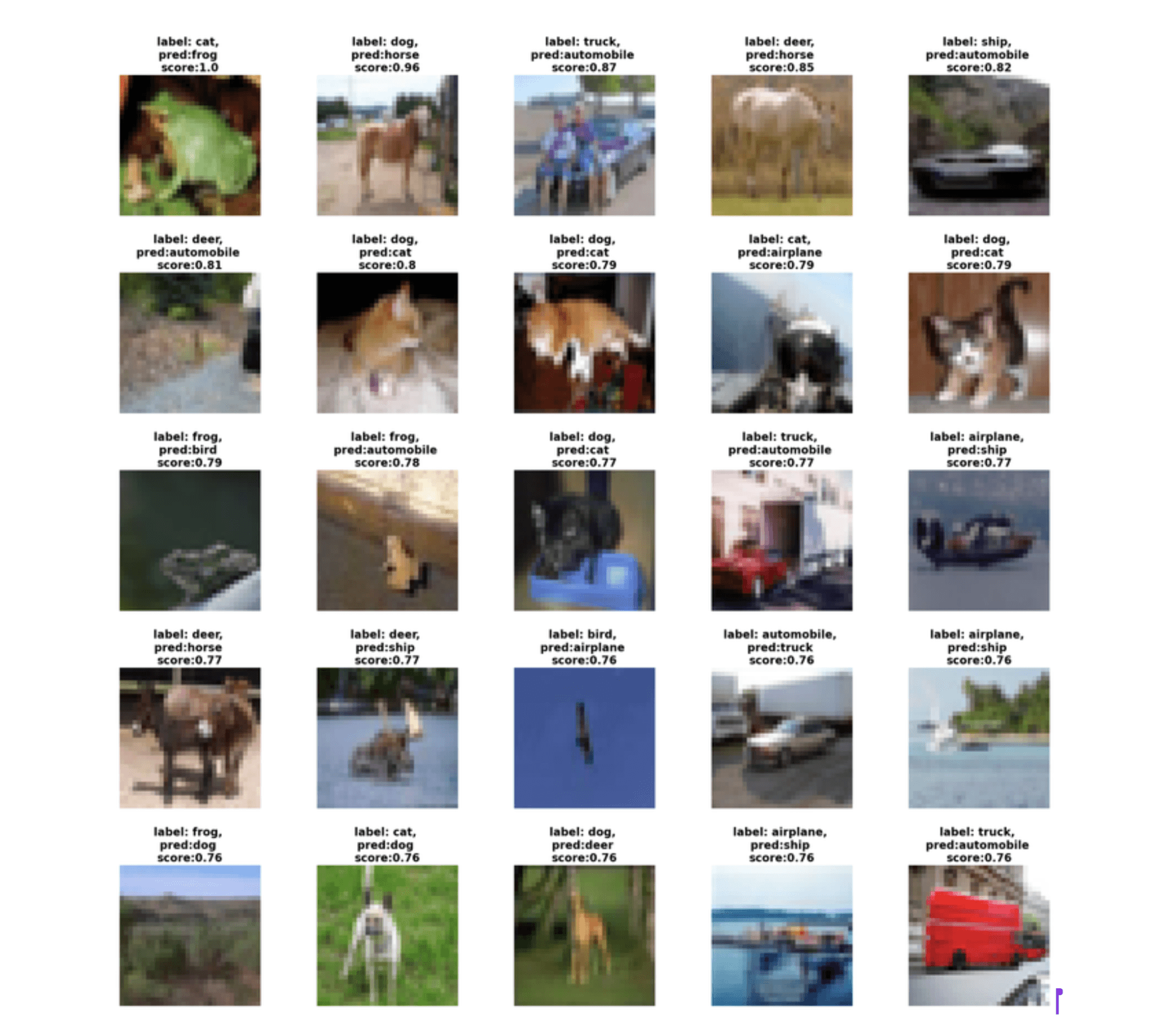
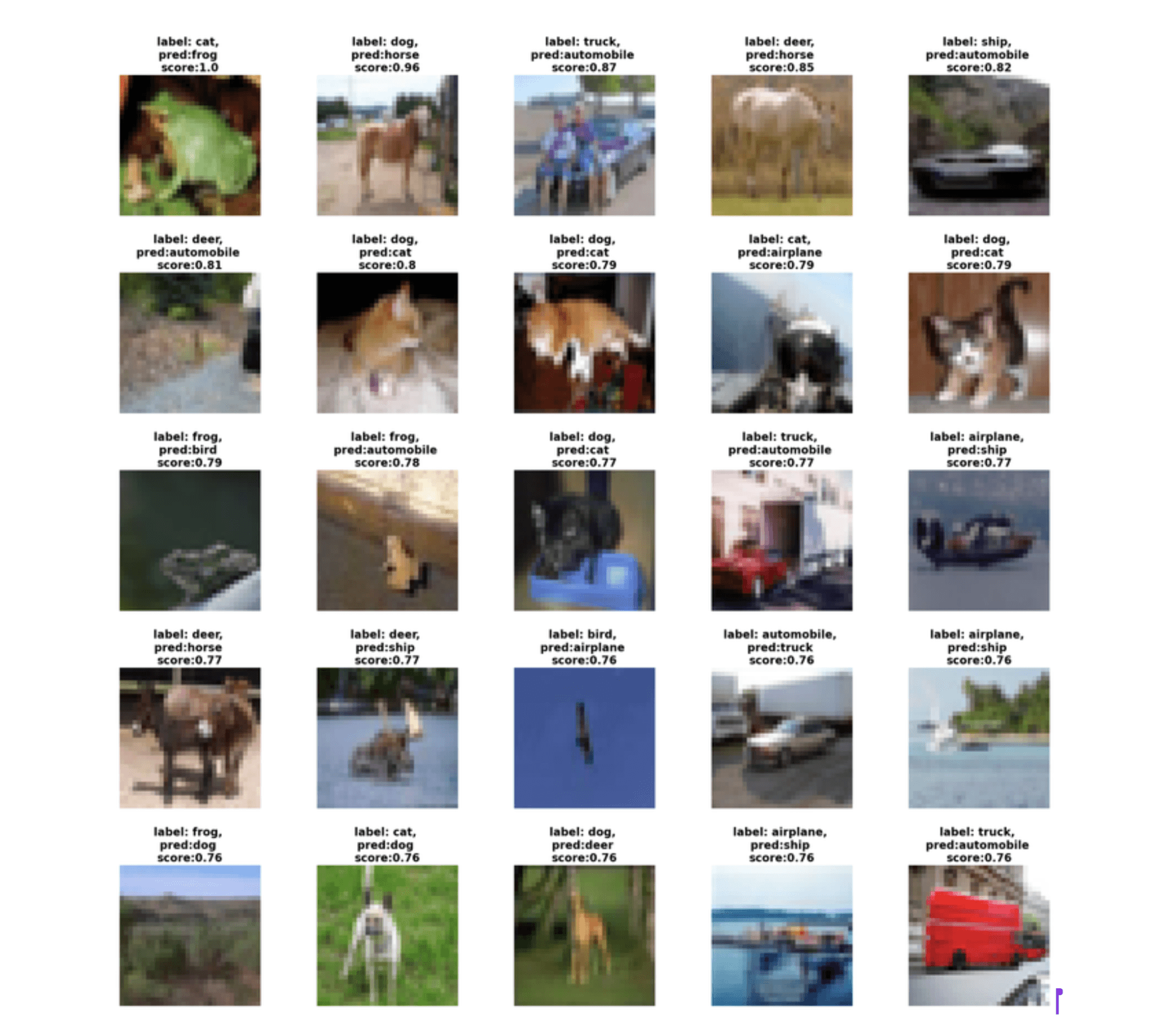
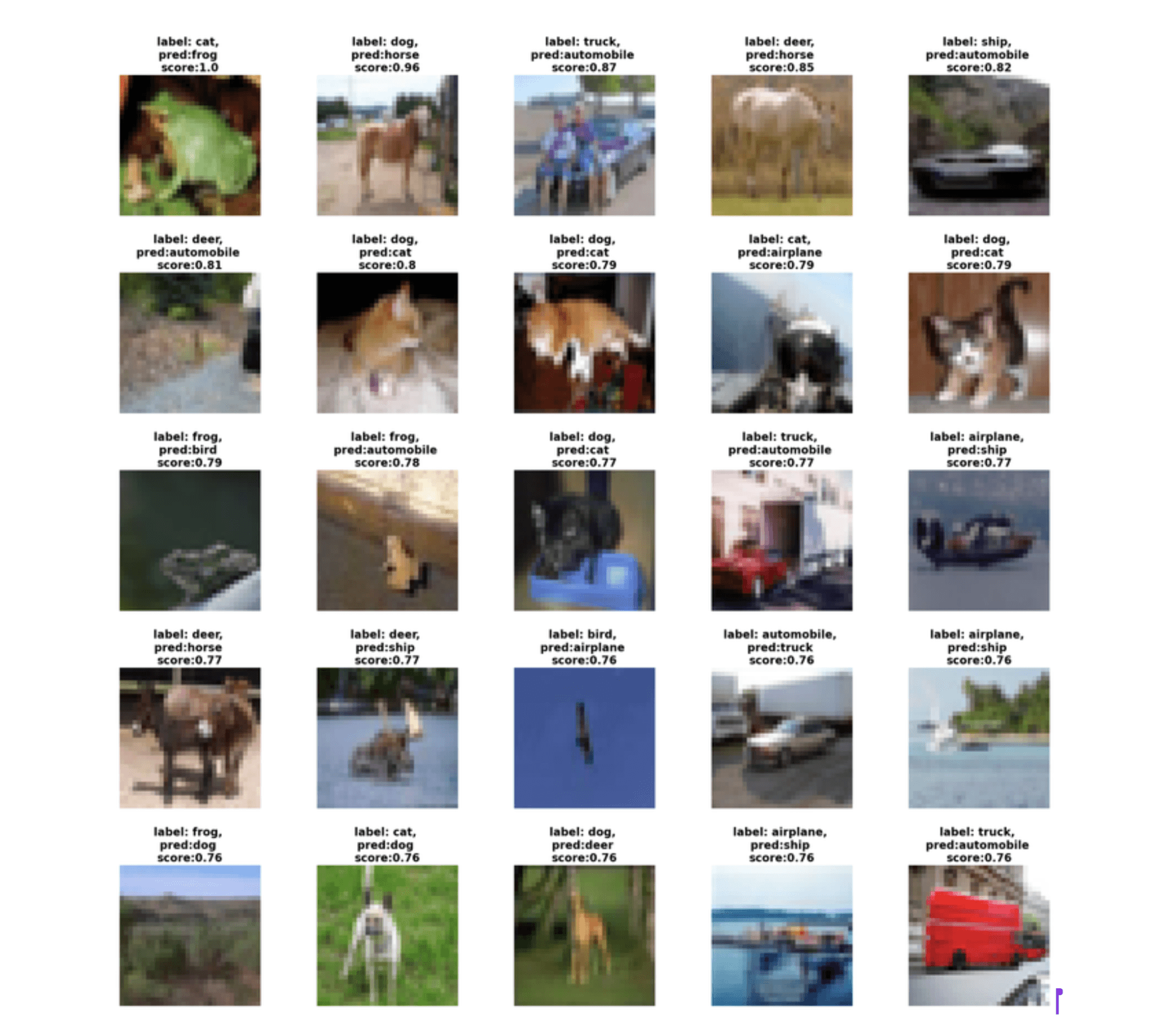
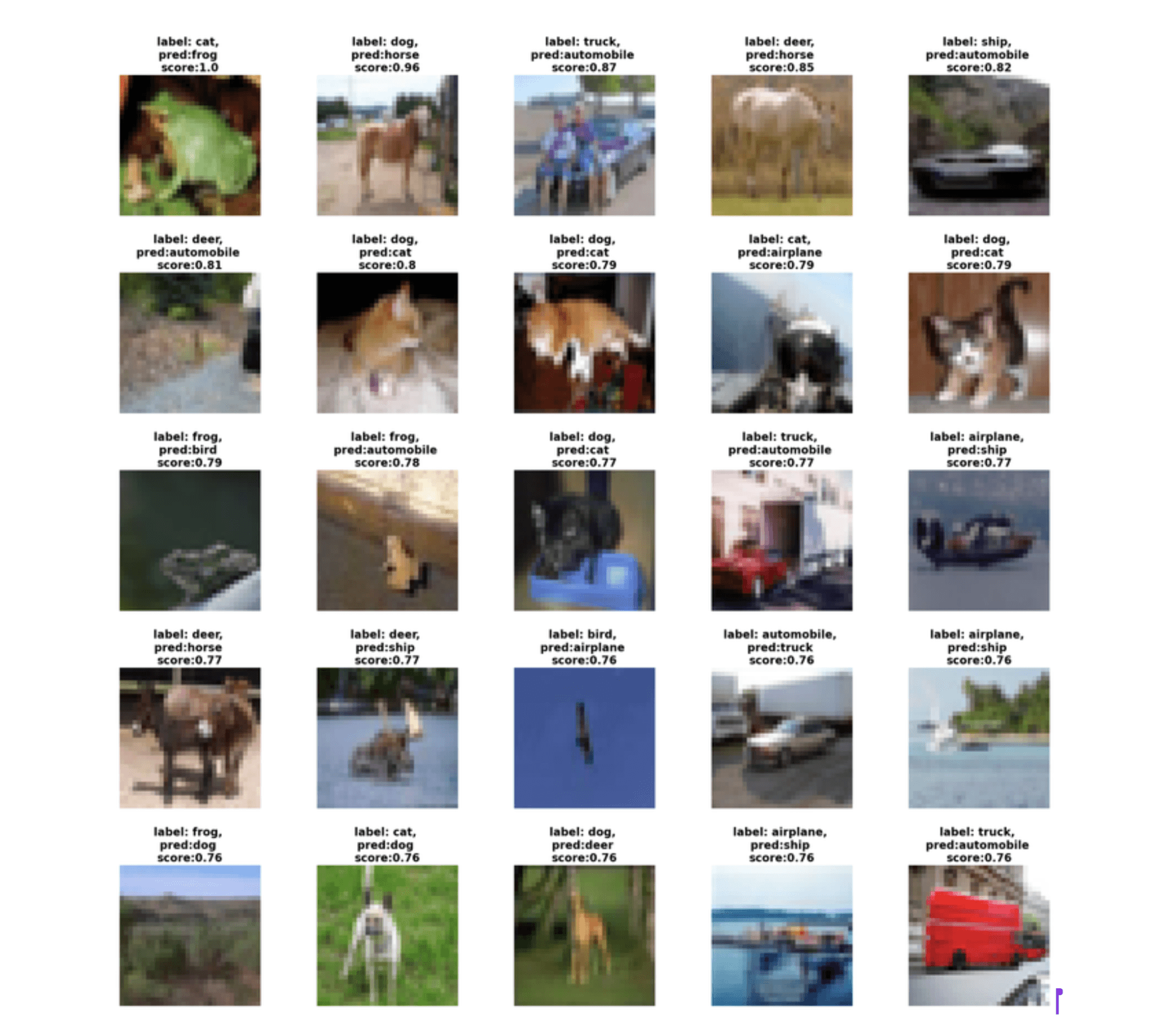
Detection of Labelling Issue in CIFAR-10 Dataset using RagaAI Platform
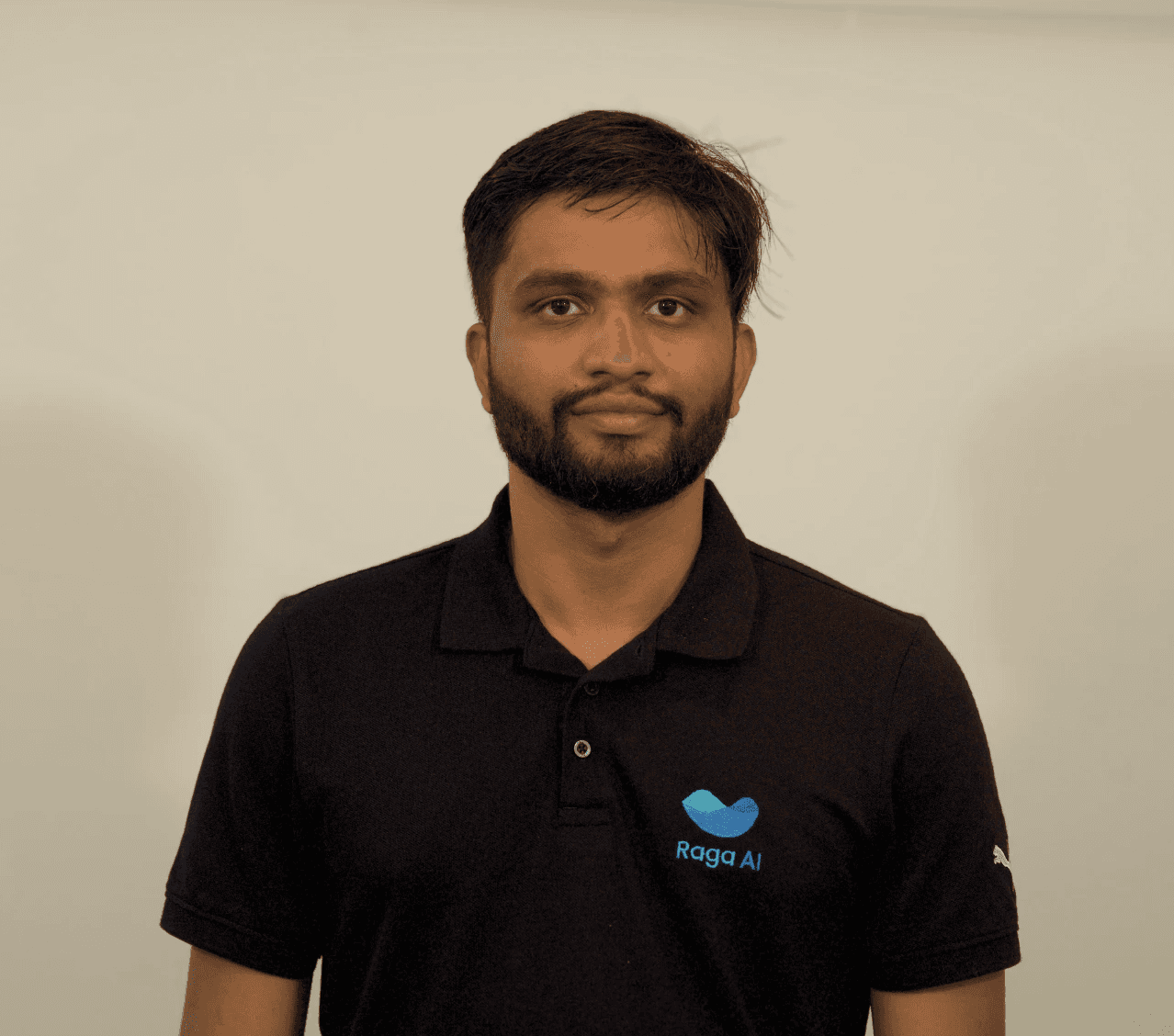
Rehan Asif
Feb 5, 2024
Read the article
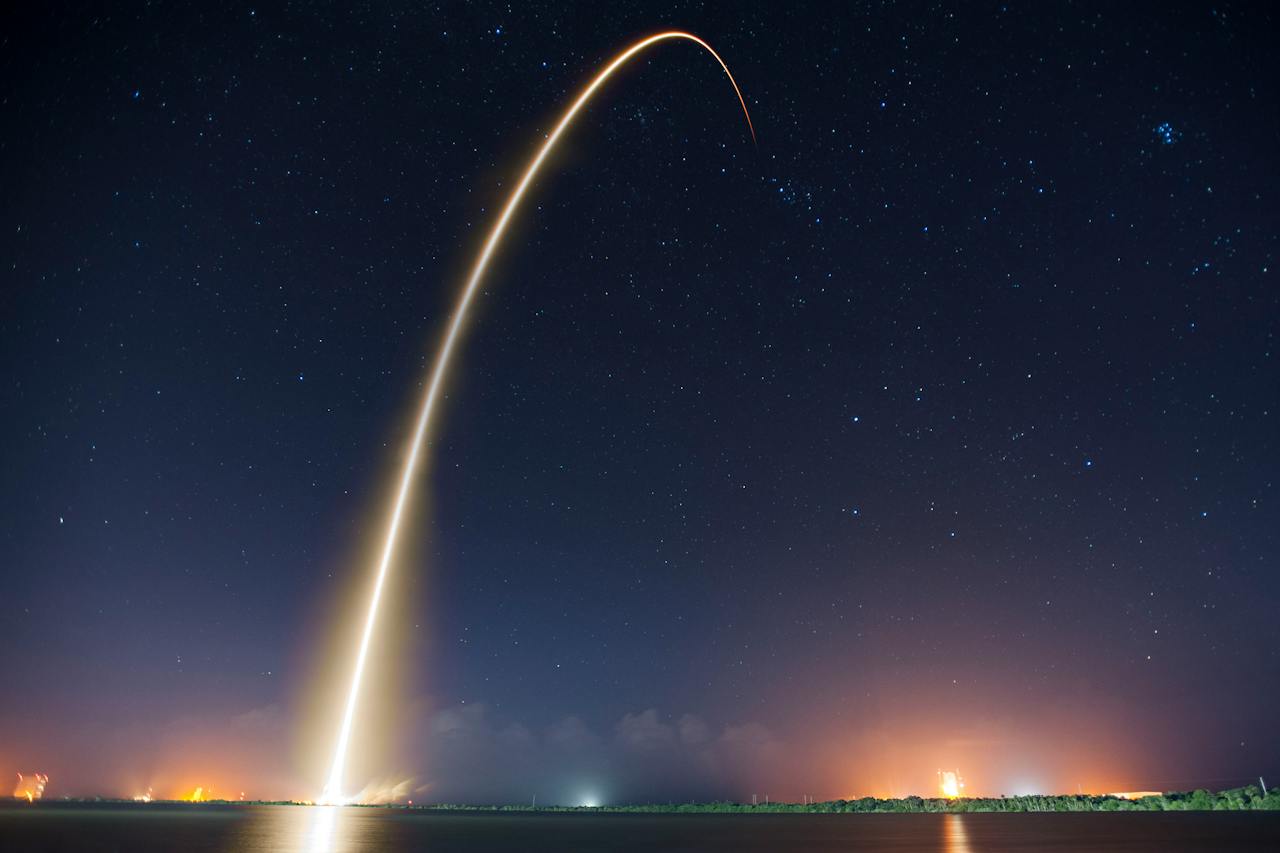
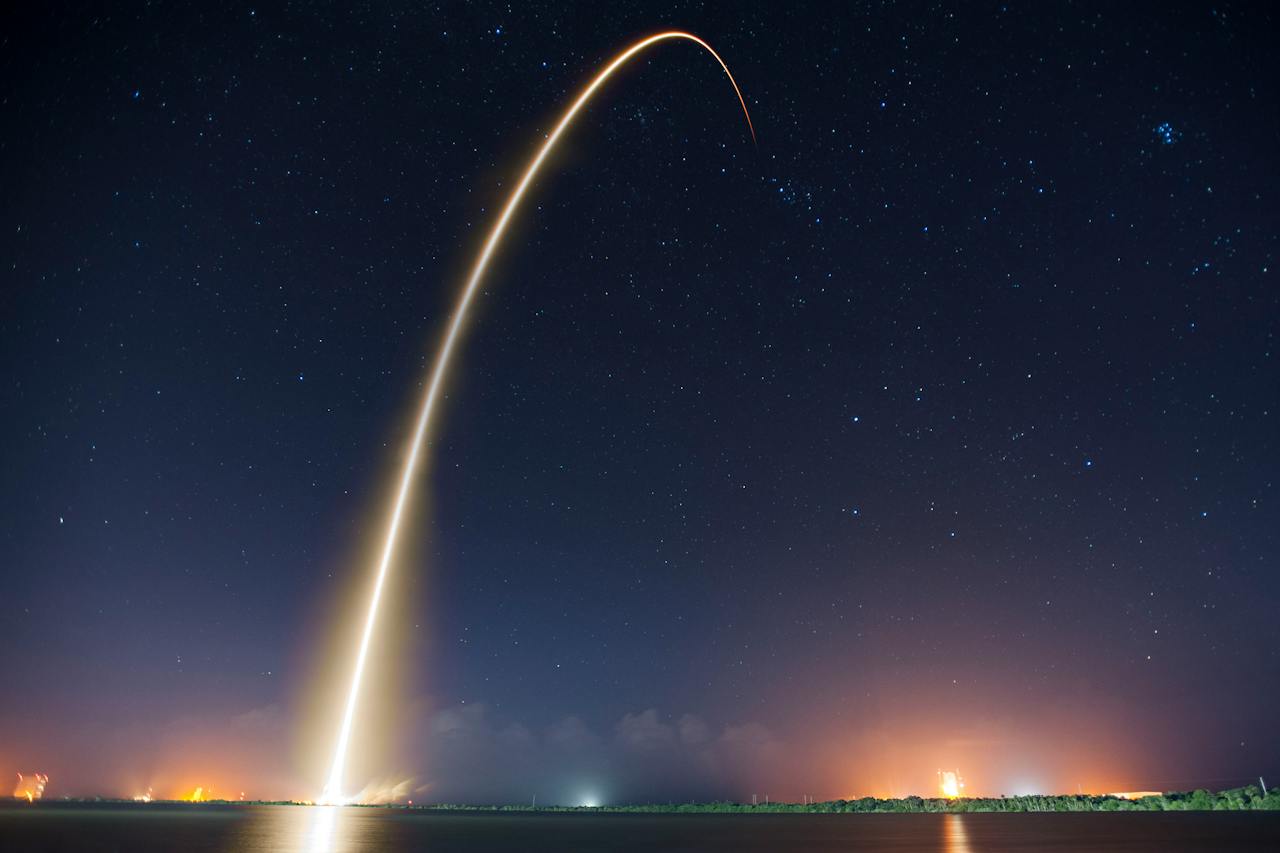
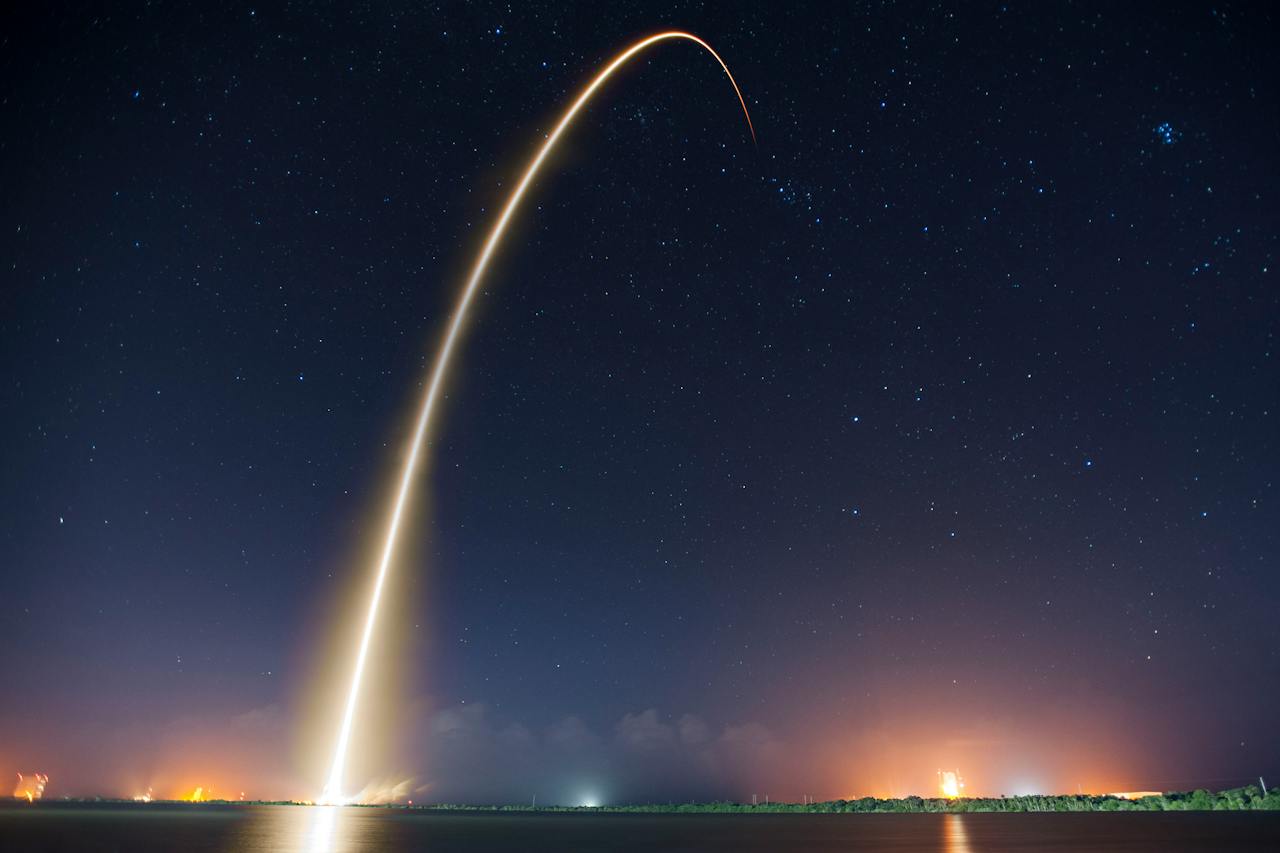
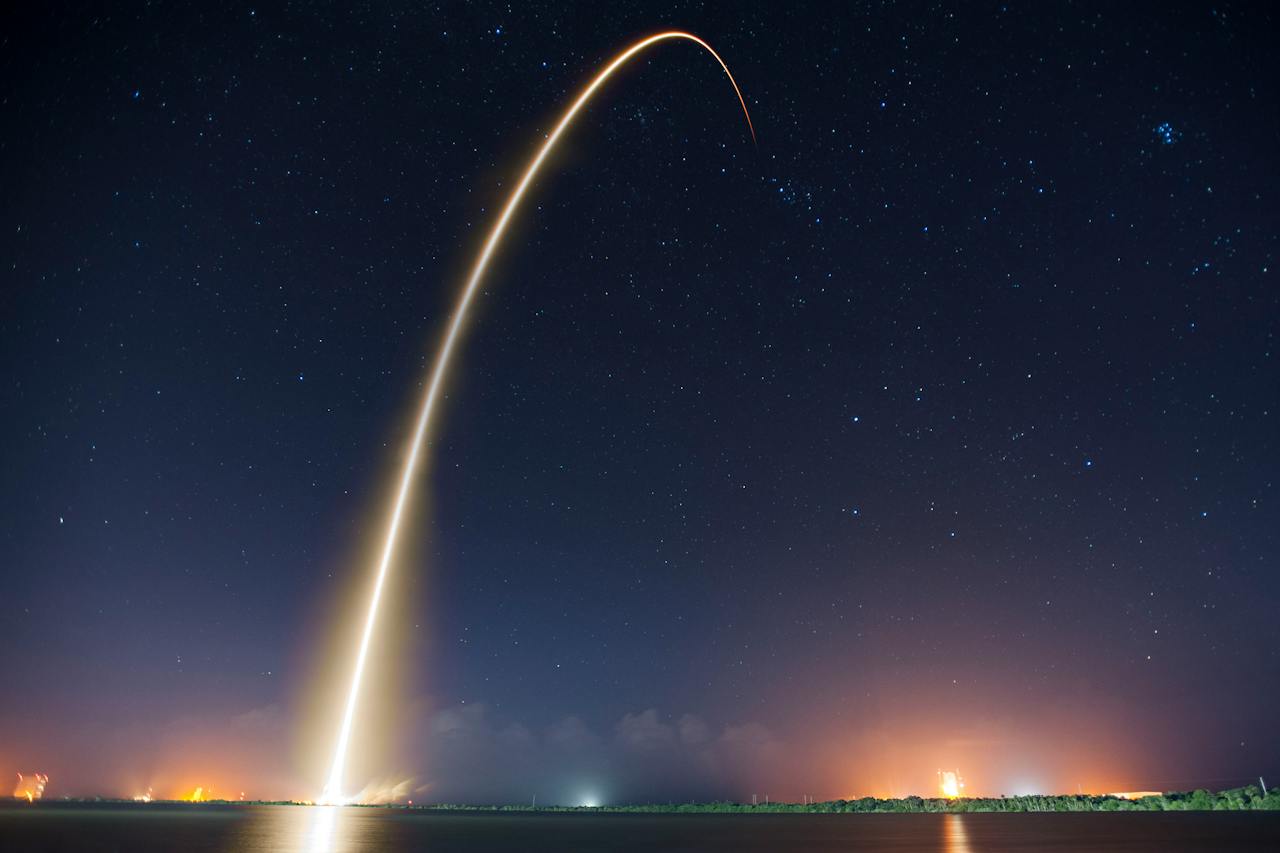
RagaAI emerges from Stealth with the most Comprehensive Testing Platform for AI
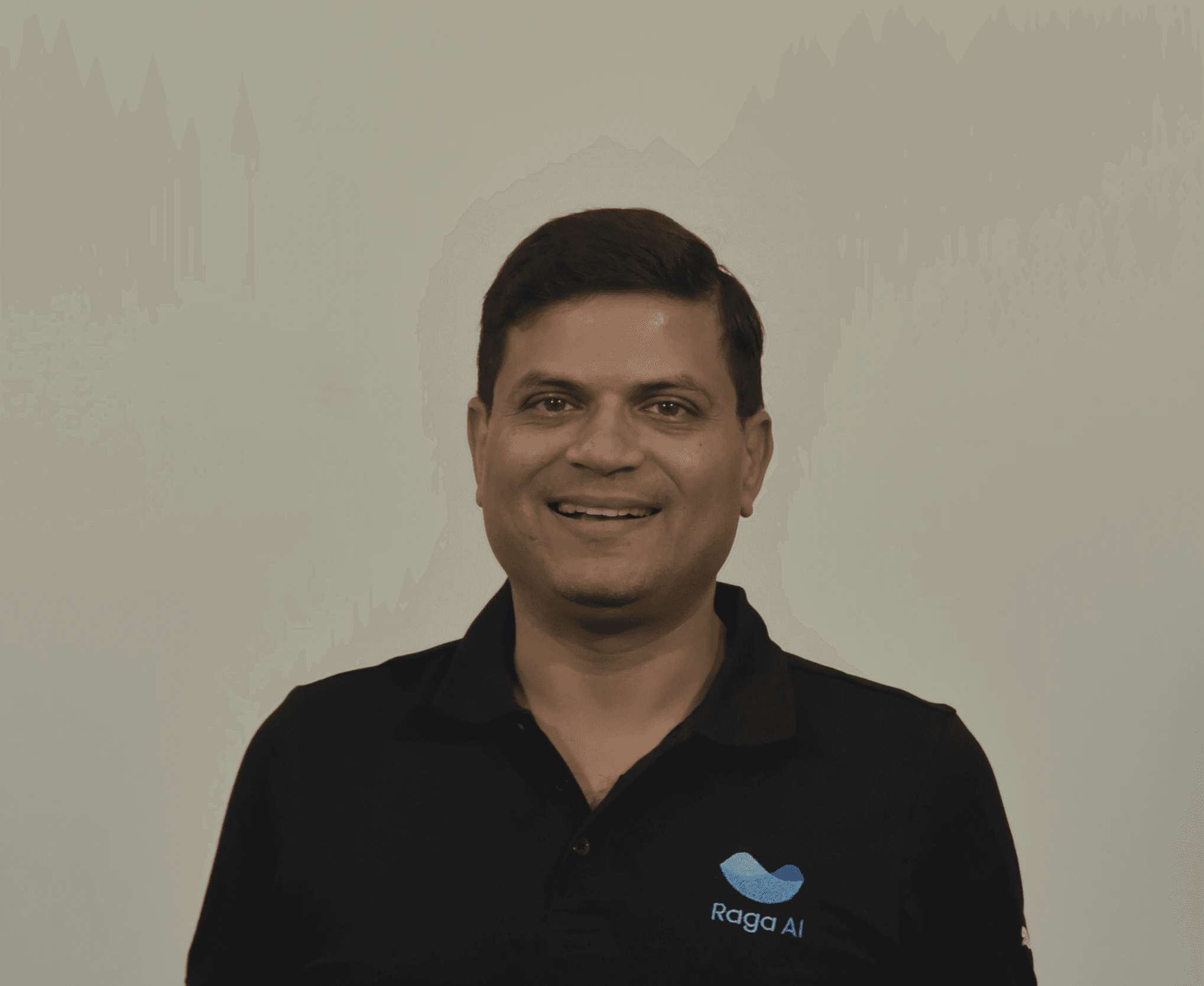
Gaurav Agarwal
Jan 23, 2024
Read the article




AI’s Missing Piece: Comprehensive AI Testing

Gaurav Agarwal
Jan 11, 2024
Read the article




Introducing RagaAI - The Future of AI Testing
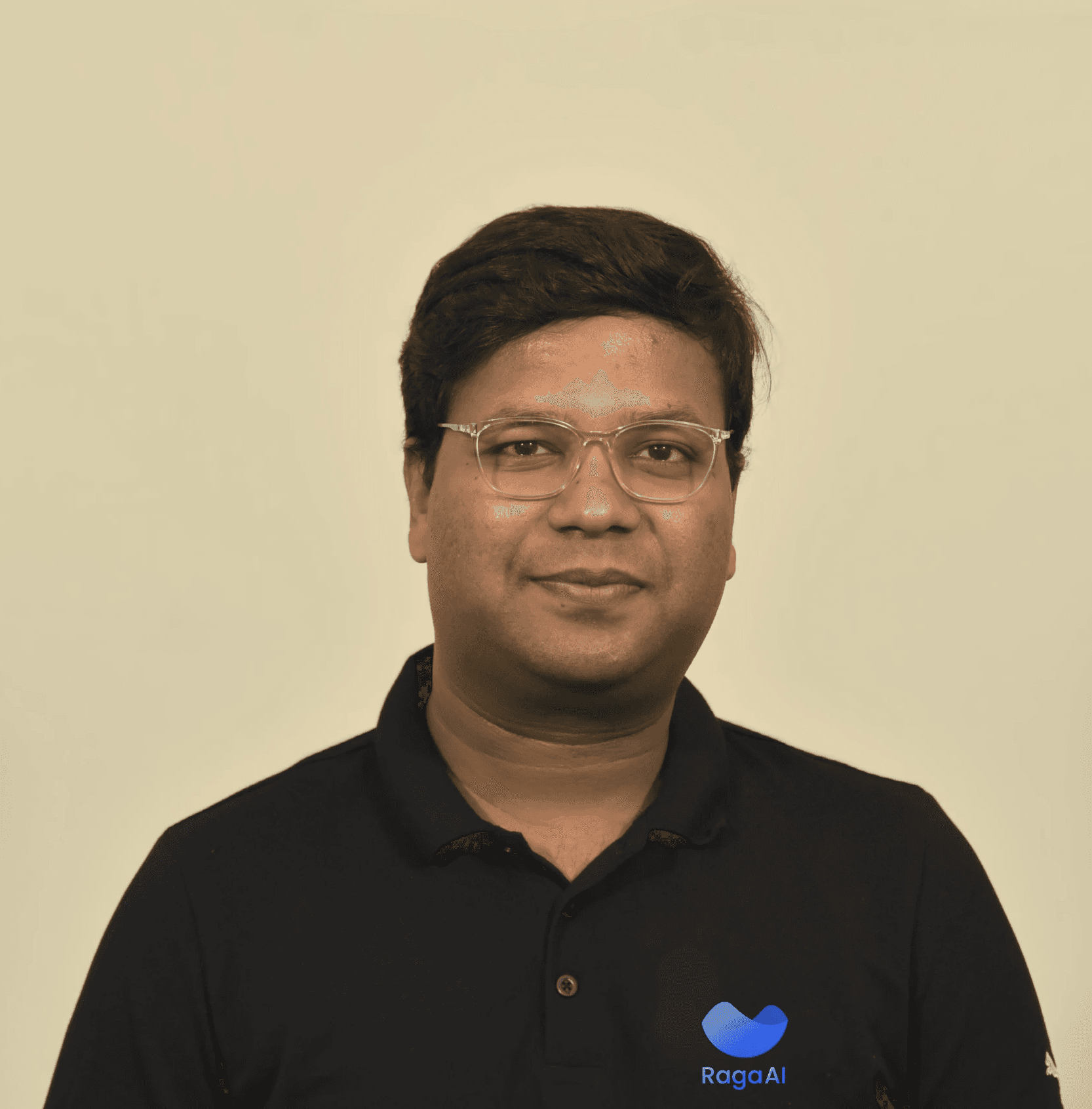
Jigar Gupta
Jan 14, 2024
Read the article




Introducing RagaAI DNA: The Multi-modal Foundation Model for AI Testing
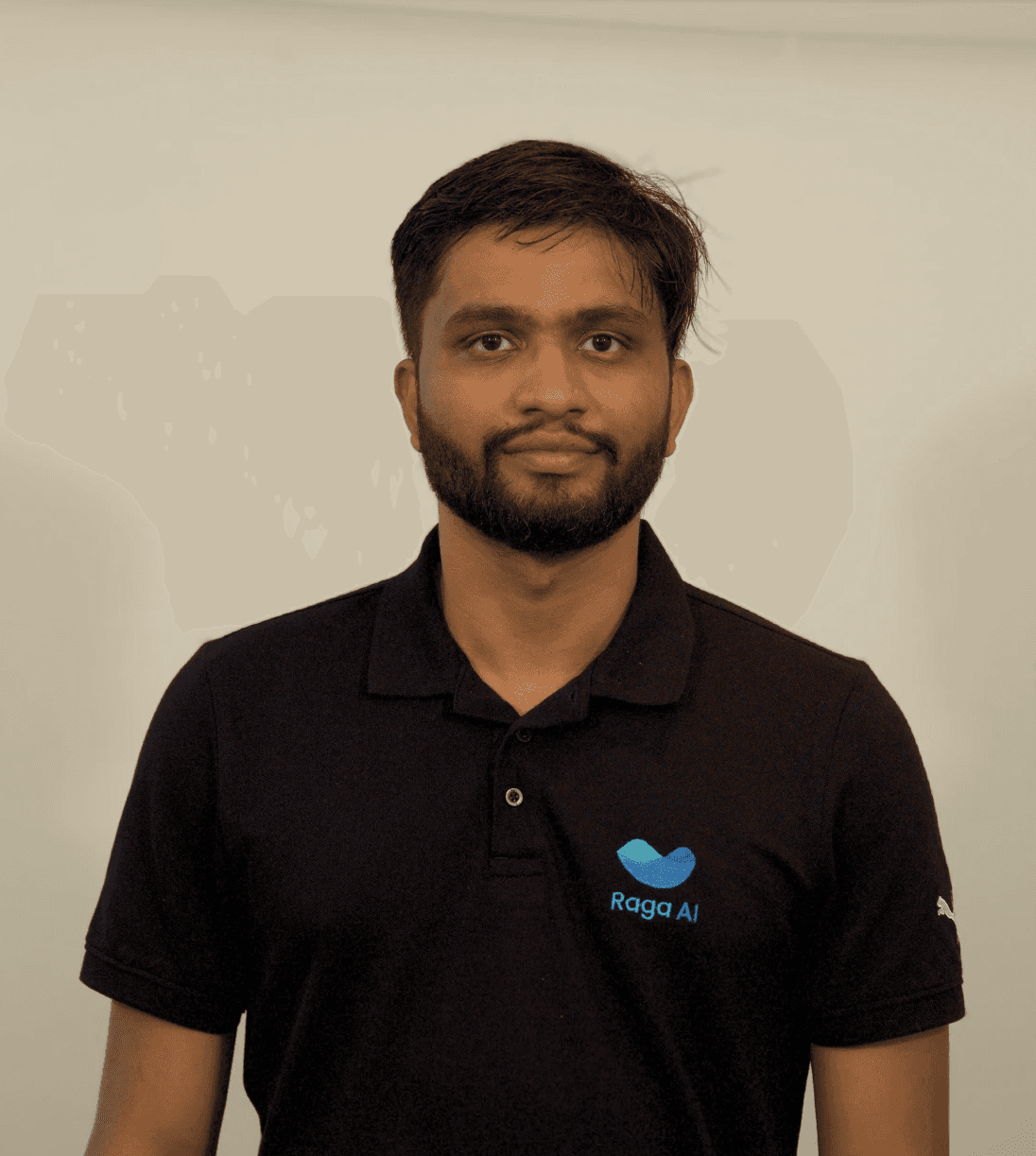
Rehan Asif
Jan 13, 2024
Read the article
Get Started With RagaAI®
Book a Demo
Schedule a call with AI Testing Experts

Get Started With RagaAI®
Book a Demo
Schedule a call with AI Testing Experts
