Understanding AI regulations In Finance
Understanding AI regulations In Finance
Understanding AI regulations In Finance
Akshat Gupta
Apr 26, 2024




Artificial Intelligence (AI) is dramatically transforming the financial sector, revolutionizing how companies approach everything from risk management to customer service.
The integration of AI technologies has enabled financial institutions to analyze large volumes of data with unprecedented speed and accuracy, leading to more informed decision-making processes and increased efficiency.
The proliferation of AI in finance is primarily fueled by the exponential growth in data availability and significant advancements in computing power.
Financial institutions can now process complex algorithms that were previously impractical, leveraging AI to gain insights from data patterns that human analysts could easily overlook. This capability enhances predictive analytics in financial markets, customer behavior analysis, and personalized banking services.
Balance Innovation with Financial Stability
Comprehensive regulatory frameworks are critical as AI technologies become more embedded in the financial sector. These regulations are essential to ensure that AI-driven innovations do not undermine the stability of financial systems or lead to unintended consequences such as systemic risks or discriminatory practices.
Proper regulatory measures must strike a balance between fostering innovation and protecting the integrity of the financial system, ensuring that advancements in AI contribute positively to the industry without compromising consumer trust or economic security.
Let's explore the specific AI developments and use cases in the financial sector, examining how these technologies are applied to enhance operations, manage risks, and improve customer engagement.
Read more on AI Developments in Finance
AI Development and Use Cases in Finance
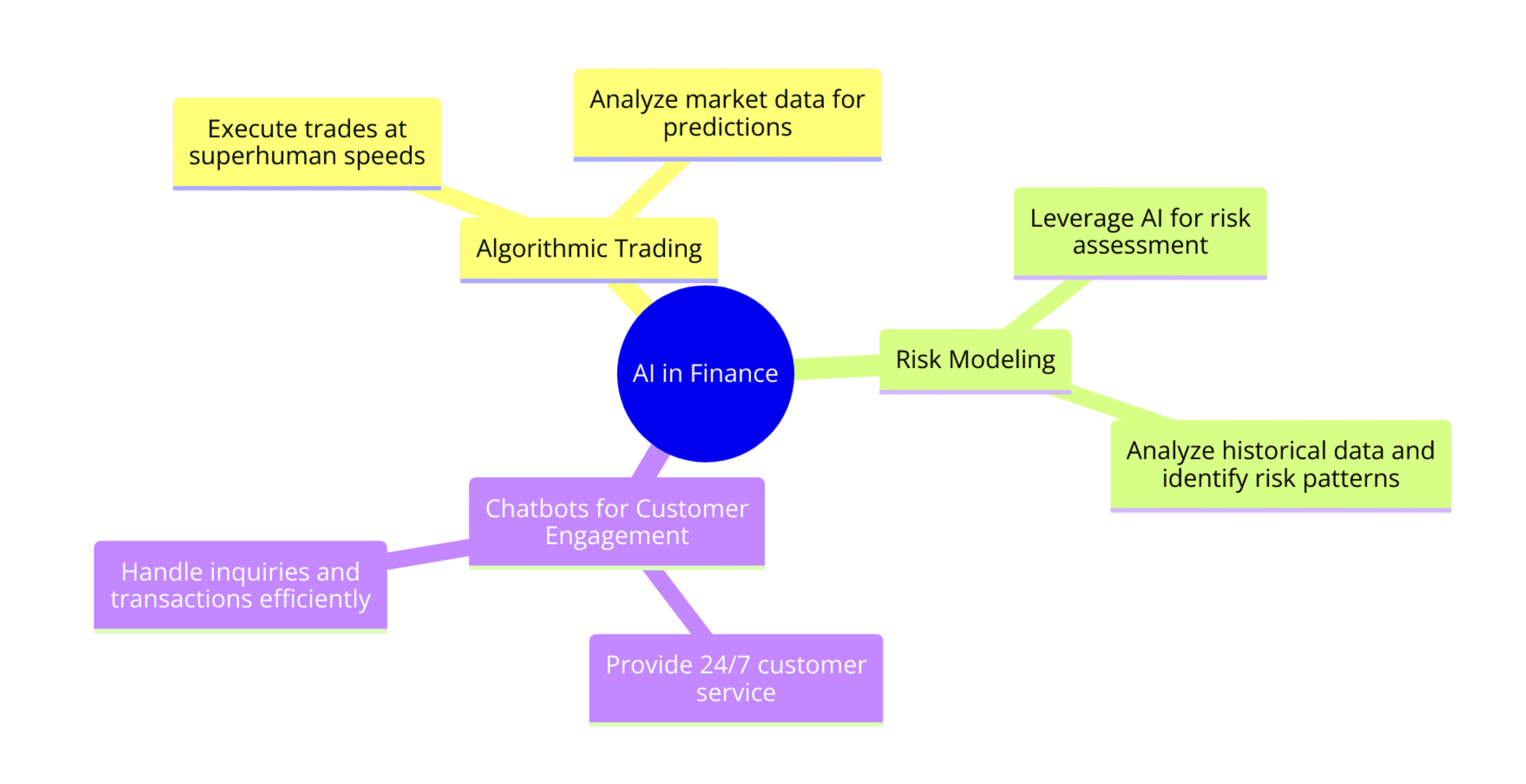
AI technologies have significantly advanced the capabilities of financial institutions in several key areas:
Algorithmic Trading: AI systems can execute trades at superhuman speeds and analyze market data to make predictions, optimizing trading strategies for better returns.
Risk Modeling: Financial institutions can more effectively predict and mitigate potential losses by leveraging AI for risk assessment. AI models can analyze historical data and identify risk patterns that might elude traditional methods.
Chatbots for Customer Engagement: AI-driven chatbots provide 24/7 customer service, handling inquiries and transactions with greater accuracy and efficiency. This not only enhances customer experience but also reduces operational costs.
AI's Role in Enhancing Operational Capacity, Productivity, and Unifying Data Silos
AI is pivotal in streamlining operations within financial institutions. By automating routine tasks, AI frees up human resources for more complex decision-making processes, enhancing overall productivity. Additionally, AI helps break down data silos within organizations by integrating disparate data sources, offering a unified view that facilitates better decision-making.
AI's ability to sift through vast amounts of data and identify patterns makes it an invaluable tool in combating financial crimes:
Anti-Money Laundering (AML): AI systems can track and analyze financial transactions to spot unusual patterns that may indicate money laundering activities, significantly improving the speed and accuracy of AML investigations.
Know-Your-Customer (KYC): AI enhances KYC processes by quickly validating customer data against multiple verification databases, ensuring compliance with regulatory requirements, and reducing the potential for fraud.
Let's explore the global regulatory landscape for AI in finance, detailing international commitments, jurisdiction-specific developments, and key examples of how various regions are addressing the challenges and opportunities presented by AI technologies.
Read more on AI Use Cases
Global Regulatory Landscape for AI in Finance
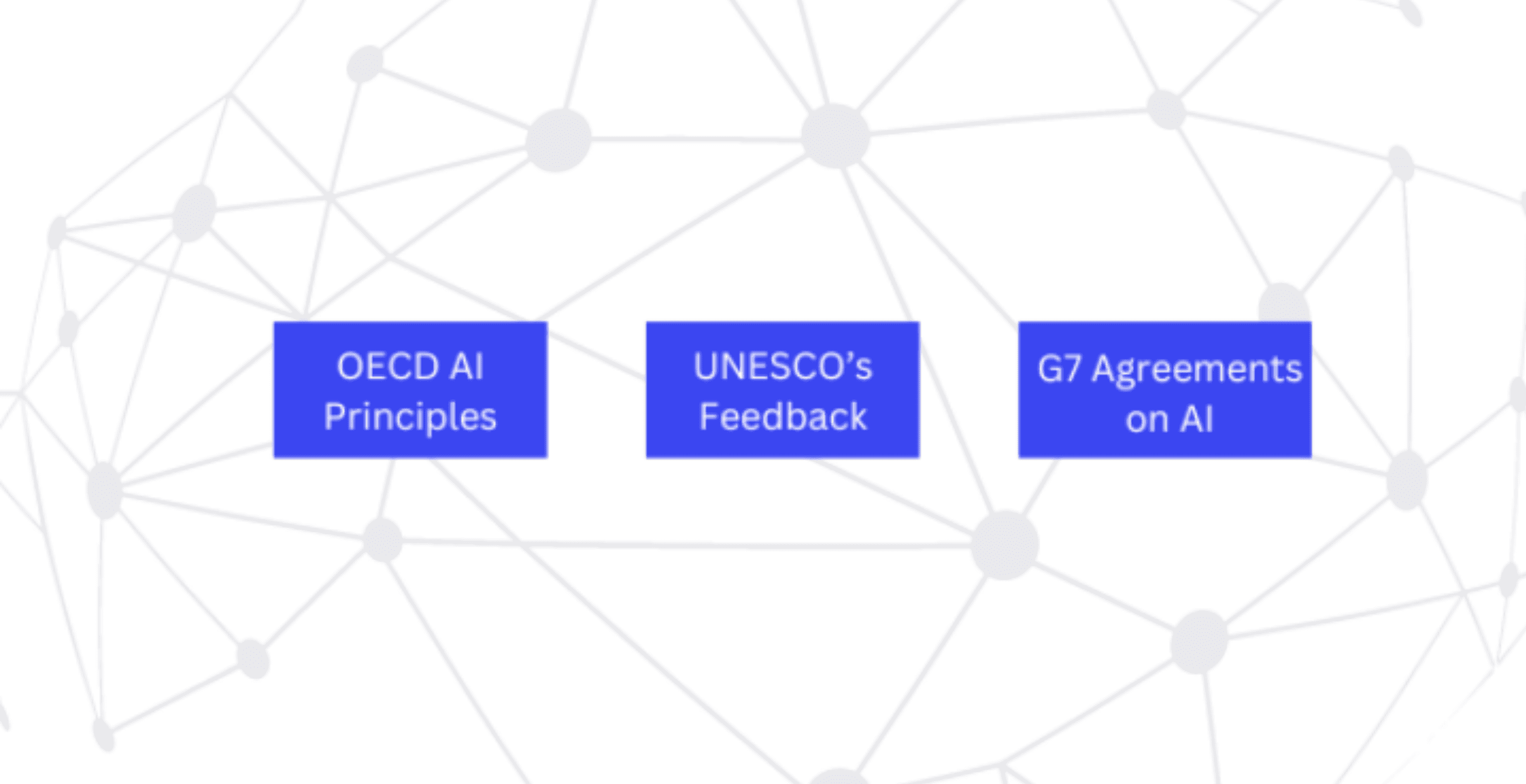
The international community has recognized the need for cohesive regulatory frameworks to guide the development and deployment of AI in finance. Key initiatives include:
OECD AI Principles: These principles focus on fostering innovation and trust in AI technologies while ensuring respect for human rights and democratic values. They emphasize AI systems' transparency, robustness, and safety, particularly in high-stakes sectors like finance.
UNESCO’s Recommendation on the Ethics of AI: This recommendation outlines standards to ensure that AI development respects ethical principles and human values, promoting data privacy and transparency.
G7 Agreements on AI: These agreements among G7 countries aim to harmonize AI regulations across borders, ensuring that AI supports economic growth and societal benefits without compromising financial stability.
Key Jurisdictional Developments in the EU, UK, and Japan
Different regions have taken unique approaches to AI regulation:
European Union (EU): The EU has been at the forefront with the proposed AI Act, which sets clear guidelines for high-risk AI applications in finance, such as ensuring algorithmic transparency and accountability.
United Kingdom (UK): The UK focuses on sector-specific guidelines and has established a sandbox environment where fintech firms can test AI technologies in a controlled regulatory space.
Japan: Japan emphasizes collaboration between the private sector and government to drive AI innovation while ensuring stringent data protection and security measures in financial services.
US State-Level AI Legislation and Federal Interests
In the United States, AI regulation is evolving at both state and federal levels:
State-Level Legislation: States like California and New York have implemented laws specifically addressing AI's use in decision-making processes, including transparency in AI-driven credit scoring systems.
Federal Interests: At the federal level, initiatives such as those from the National Institute of Standards and Technology (NIST) provide guidelines for deploying trustworthy AI systems, focusing on ethical, legal, and societal implications for AI in finance.
AI Regulation in the United States
The global landscape for AI regulation in finance is complex and dynamic, with international and regional frameworks evolving to address the rapid advancements in AI technologies. As we delve deeper into specific regions like the United States and Europe, we'll explore how these regulations.
State-level Legislation and Proposed Federal Laws
In the U.S., AI regulation varies significantly across states, reflecting diverse approaches to balancing innovation with consumer protection and ethical considerations:
State-Level Legislation: States like California have enacted laws that specifically address the transparency of AI systems used in hiring processes. These regulations require companies to disclose when AI is used in making employment decisions. They are designed to prevent biases and ensure fairness in AI applications.
Proposed Federal Laws: At the federal level, there are ongoing discussions about comprehensive AI legislation that would provide a unified framework for the use of AI across various sectors, including finance. Proposed laws focus on enhancing AI systems' transparency, accountability, and security to protect consumers and maintain trust in AI technologies.
Read more on USA AI Policies
Guidance from the NIST
The National Institute of Standards and Technology (NIST) plays a crucial role in setting standards for AI that bolster its safety, reliability, and interoperability. NIST's guidance often includes:
Frameworks for AI Testing and Validation: Ensuring that AI systems are rigorously tested and validated for their intended financial services applications.
Security Protocols for AI Systems: Developing robust security measures to protect sensitive financial data processed by AI, mitigating risks associated with data breaches and cyber-attacks.
AI Bill of Rights
The White House has proposed a Blueprint for an AI Bill of Rights, which outlines key protections that should be afforded to all Americans in the age of AI. This blueprint emphasizes:
Privacy Rights: Safeguarding personal data against misuse and ensuring individuals have control over their information.
Non-discrimination: Ensuring AI systems do not perpetuate biases or discriminatory practices, particularly in critical areas like credit scoring and lending.
Algorithmic Transparency: Requiring that the operations of AI systems be understandable to users, with clear explanations of how decisions are made, especially those impacting financial opportunities and livelihoods.
The regulatory framework in the United States aims to foster an environment where AI can thrive while ensuring it operates in a secure, transparent, and equitable manner.
As AI continues integrating into the financial sector, these regulations will play a pivotal role in shaping its development and ensuring its benefits are realized responsibly. Next, we'll explore how Europe contributes to shaping global AI regulations, particularly in financial services.
Read more on AI Regulations Worldwide
Europe's Role in Shaping AI Regulations
Let's explore Europe's significant role in shaping AI regulations, particularly focusing on the comprehensive frameworks it has established that influence global standards and practices, especially in the financial sector.
The EU AI Act and Its Implications for Financial Services
Europe has been a frontrunner in regulating AI, with the European Union's AI Act representing one of the most ambitious legislative efforts globally. This act categorizes AI applications according to risk levels, imposing stricter requirements on high-risk sectors like finance. Critical provisions for financial services include:
Risk Assessment and Mitigation: Financial institutions must conduct thorough risk assessments of their AI systems to ensure they operate safely and without bias.
Transparency Requirements: AI systems used in finance must be transparent, with clear explanations provided to customers about how their data is used, and decisions are made, especially for AI-driven credit scoring or investment advice.
Europe's influence extends beyond its borders, as it has played a critical role in shaping international AI policies:
OECD AI Principles Endorsement: The EU has been instrumental in developing and endorsing the OECD AI Principles, advocating for responsible stewardship of trustworthy AI globally.
Hiroshima AI Process: Under this initiative, Europe has pushed for a global Code of Conduct for AI, promoting ethical standards and practices that ensure AI's benefits are distributed equitably across societies.
The EU AI Act's Global Impact
The EU AI Act is pivotal for European countries and serves as a benchmark for other regions developing their AI regulations.
Its comprehensive approach is influencing how global markets, including the US and Asia, regulate AI technologies, especially in critical areas such as financial services, where the potential for harm is significant.
The act's focus on ethical considerations and consumer protections sets a high standard for what responsible AI deployment should look like.
Europe's proactive approach to AI regulation, mainly through the EU AI Act, highlights its commitment to ensuring that AI development is balanced with the need to protect consumers and maintain ethical standards. This legislative framework influences European financial markets and sets a global precedent for how AI should be regulated in sensitive sectors.
Next, we'll discuss how institutions can ensure compliance with these regulations and the recommended practices for deploying AI in finance responsibly. Ready to move on to compliance and regulatory recommendations?
Compliance and Regulatory Recommendations
Let's dive into the essential compliance and regulatory recommendations for deploying AI in the financial sector, emphasizing the core principles that ensure these systems are used responsibly and effectively.
Compliance in AI, especially within the financial sector, revolves around several key principles:
Training: AI systems should use diverse and representative data sets to avoid biases and ensure fair outcomes.
Testing: Rigorous testing must be conducted to verify the accuracy and reliability of AI models under various conditions.
Monitoring: Continuous monitoring is essential to quickly identify and address any issues that may arise during the operation of AI systems, ensuring they continue to function as intended.
Auditing: Regular audits are necessary to ensure compliance with all relevant laws and regulations and to maintain transparency and accountability in AI operations.
Inclusion of Legal Counsel in AI Policy Development
Involving legal counsel in developing AI policies is crucial to ensure that all AI implementations comply with existing legal frameworks and ethical standards. Legal experts can provide guidance on regulatory requirements, help assess potential legal risks, and ensure that AI deployments are both effective and compliant with the law.
Prioritizing Data Quality and Security in AI Model Training
Data quality and security are paramount in AI model training:
Data Quality: High-quality data is essential for training effective AI models. This means ensuring the data is accurate, comprehensive, and free of biases.
Data Security: Protecting sensitive information used in AI training is crucial to prevent data breaches and maintain consumer trust. Implementing robust data security measures, such as encryption and access controls, is essential.
Ensuring Thorough Documentation for Auditability of AI Processes
Thorough documentation of AI processes is vital for auditability and compliance. Documentation should include details on the design and development of AI systems, data handling practices, decision-making processes, and any incidents or modifications. This transparency helps regulators and stakeholders understand how AI systems work and assess their compliance with regulatory standards.
These compliance and regulatory recommendations are designed to help financial institutions implement AI responsibly, ensuring that they not only reap the benefits of AI but also maintain the trust and confidence of their customers and regulators. Next, we'll discuss the specific risks associated with AI in financial institutions and how appropriate regulations can help mitigate these risks.
AI Risks and Financial Institution Accountability
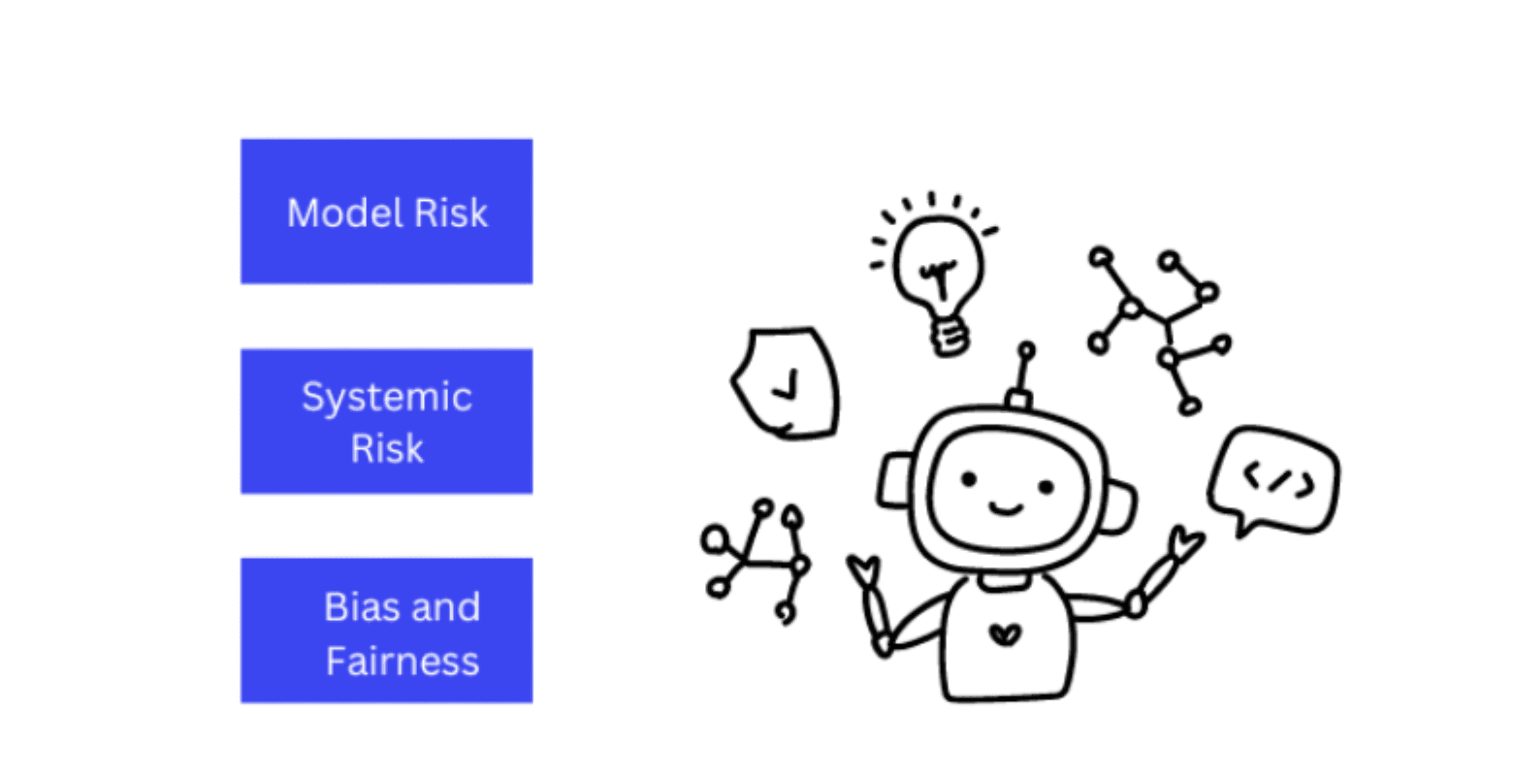
Understanding and mitigating the risks associated with AI in financial institutions is critical for ensuring that these technologies are used responsibly and effectively. As we move forward, we will discuss how financial institutions can prepare for the future of AI, including expected developments in regulations and technologies.
AI technologies, while offering significant benefits in terms of efficiency and decision-making, also pose unique risks that can amplify existing challenges within financial institutions. These risks include:
Model Risk: The risk that AI models may not perform as expected under different market conditions or may fail to adequately handle edge cases.
Systemic Risk: The potential for AI-driven decisions to lead to widespread issues within the financial system, especially if similar AI strategies are adopted across multiple institutions.
Bias and Fairness: AI systems can inadvertently perpetuate or even exacerbate biases present in the training data, leading to unfair outcomes for certain groups of customers.
To effectively manage these risks, robust regulatory frameworks are essential. These frameworks should focus on:
Regulation: Establishing clear guidelines for the development, deployment, and operation of AI systems within financial institutions to ensure they are safe and fair.
Supervision: Regular monitoring by regulatory bodies to ensure that financial institutions comply with AI governance standards and that their AI systems do not pose undue risks to the financial system.
Accountability: Implementing mechanisms that hold institutions accountable for the decisions made by their AI systems, ensuring that there is always a clear line of responsibility.
Read more on EU AI Act Insights
Addressing AI Risks in Practice
Implementing practical measures to manage AI risks involves:
Risk Assessment Protocols: Financial institutions must regularly assess the risks associated with their AI applications, considering factors like data integrity, model robustness, and potential impacts on customers.
Ethics and Compliance Committees: Establishing dedicated committees to oversee AI ethics and compliance can help ensure that AI applications adhere to both internal and external ethical standards.
Transparent Reporting: Institutions should be transparent about their use of AI, including the risks involved and the measures taken to mitigate these risks. This transparency is crucial for maintaining stakeholder trust.
Read more on AI Governance Strategies
Facing the Future of AI in Finance
The landscape of AI regulation in finance is rapidly evolving to keep pace with technological advancements. As AI systems become more complex and integral to financial operations, regulators worldwide are working to update and refine legal frameworks to ensure these technologies are used safely and ethically.
This includes establishing clearer guidelines for accountability, transparency, and data protection in AI applications.
Looking ahead, several key regulatory developments are expected to significantly impact the financial sector:
EU AI Act: This comprehensive legislative framework is set to establish risk-based classifications for AI systems, mandating stringent compliance requirements for high-risk applications in finance.
SEC Proposals: In the United States, the Securities and Exchange Commission (SEC) is proposing new regulations aimed at enhancing transparency in AI-driven investment strategies and financial products, ensuring that investors are adequately informed about the AI methodologies that influence their investments.
Global Harmonization Efforts: There is an increasing call for international cooperation to harmonize AI regulations, which would facilitate smoother cross-border operations of multinational financial institutions and prevent regulatory arbitrage.
The Call for a Harmonized Approach to AI Regulation Internationally
The need for a harmonized international approach to AI regulation in finance cannot be overstated. As financial markets are inherently global, disparate regulatory environments can lead to inconsistencies that complicate compliance for international entities and potentially hinder innovation.
A coordinated effort to establish common standards and practices can help ensure that AI technologies are developed and used in ways that benefit the global community while safeguarding against systemic risks.
Ready to transform your approach to AI with cutting-edge insights and tools? Explore RagaAI's comprehensive suite of AI solutions — from enhancing AI reliability with our guardrails to navigating the complexities of AI governance.
Whether diving into prompt engineering, seeking to mitigate AI biases, or exploring the frontier of AI testing, RagaAI is your partner in pioneering a safer, more efficient AI-driven future. Discover more and join us in shaping the next wave of AI innovation. Let's embark on this journey together with RagaAI.
Artificial Intelligence (AI) is dramatically transforming the financial sector, revolutionizing how companies approach everything from risk management to customer service.
The integration of AI technologies has enabled financial institutions to analyze large volumes of data with unprecedented speed and accuracy, leading to more informed decision-making processes and increased efficiency.
The proliferation of AI in finance is primarily fueled by the exponential growth in data availability and significant advancements in computing power.
Financial institutions can now process complex algorithms that were previously impractical, leveraging AI to gain insights from data patterns that human analysts could easily overlook. This capability enhances predictive analytics in financial markets, customer behavior analysis, and personalized banking services.
Balance Innovation with Financial Stability
Comprehensive regulatory frameworks are critical as AI technologies become more embedded in the financial sector. These regulations are essential to ensure that AI-driven innovations do not undermine the stability of financial systems or lead to unintended consequences such as systemic risks or discriminatory practices.
Proper regulatory measures must strike a balance between fostering innovation and protecting the integrity of the financial system, ensuring that advancements in AI contribute positively to the industry without compromising consumer trust or economic security.
Let's explore the specific AI developments and use cases in the financial sector, examining how these technologies are applied to enhance operations, manage risks, and improve customer engagement.
Read more on AI Developments in Finance
AI Development and Use Cases in Finance
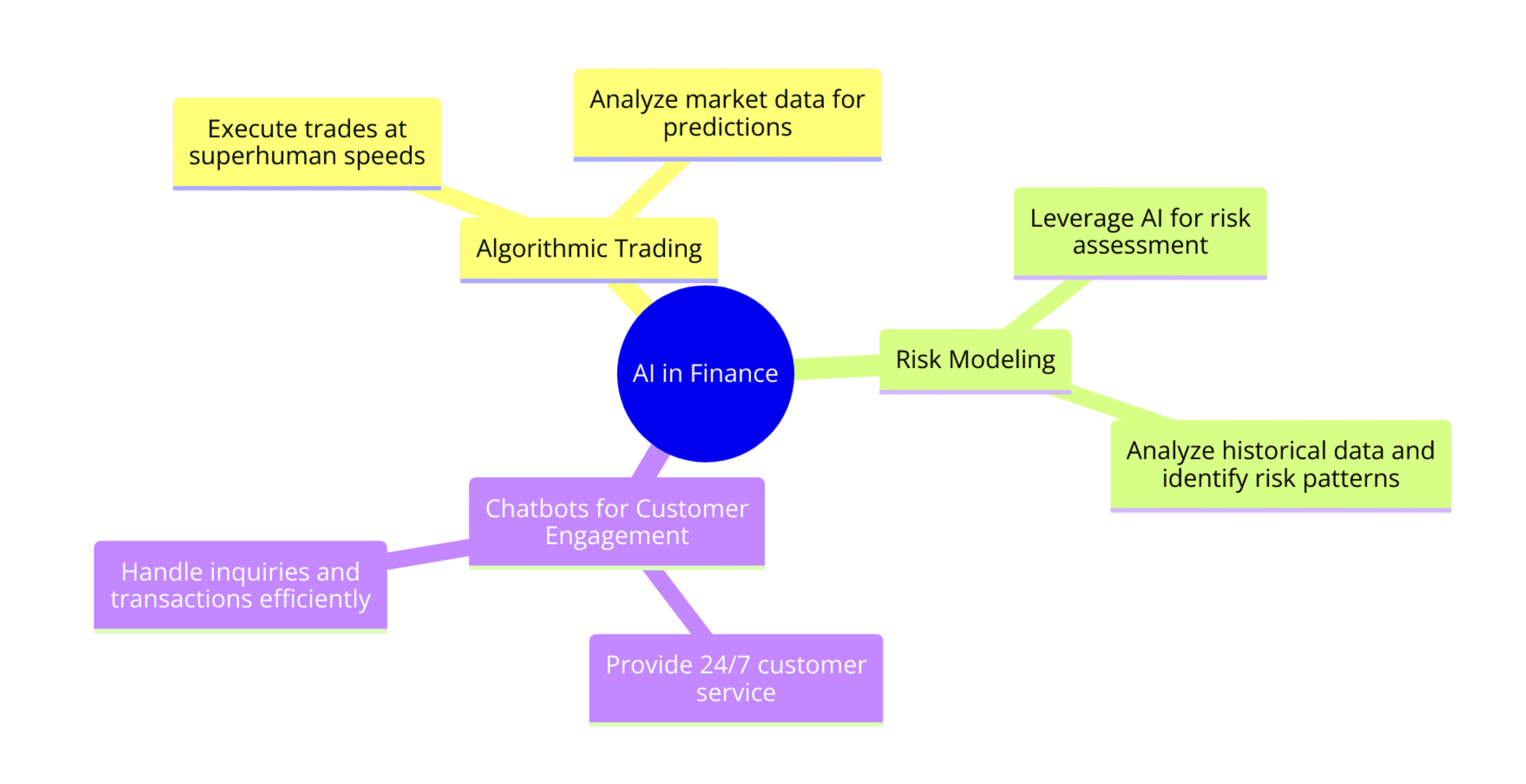
AI technologies have significantly advanced the capabilities of financial institutions in several key areas:
Algorithmic Trading: AI systems can execute trades at superhuman speeds and analyze market data to make predictions, optimizing trading strategies for better returns.
Risk Modeling: Financial institutions can more effectively predict and mitigate potential losses by leveraging AI for risk assessment. AI models can analyze historical data and identify risk patterns that might elude traditional methods.
Chatbots for Customer Engagement: AI-driven chatbots provide 24/7 customer service, handling inquiries and transactions with greater accuracy and efficiency. This not only enhances customer experience but also reduces operational costs.
AI's Role in Enhancing Operational Capacity, Productivity, and Unifying Data Silos
AI is pivotal in streamlining operations within financial institutions. By automating routine tasks, AI frees up human resources for more complex decision-making processes, enhancing overall productivity. Additionally, AI helps break down data silos within organizations by integrating disparate data sources, offering a unified view that facilitates better decision-making.
AI's ability to sift through vast amounts of data and identify patterns makes it an invaluable tool in combating financial crimes:
Anti-Money Laundering (AML): AI systems can track and analyze financial transactions to spot unusual patterns that may indicate money laundering activities, significantly improving the speed and accuracy of AML investigations.
Know-Your-Customer (KYC): AI enhances KYC processes by quickly validating customer data against multiple verification databases, ensuring compliance with regulatory requirements, and reducing the potential for fraud.
Let's explore the global regulatory landscape for AI in finance, detailing international commitments, jurisdiction-specific developments, and key examples of how various regions are addressing the challenges and opportunities presented by AI technologies.
Read more on AI Use Cases
Global Regulatory Landscape for AI in Finance
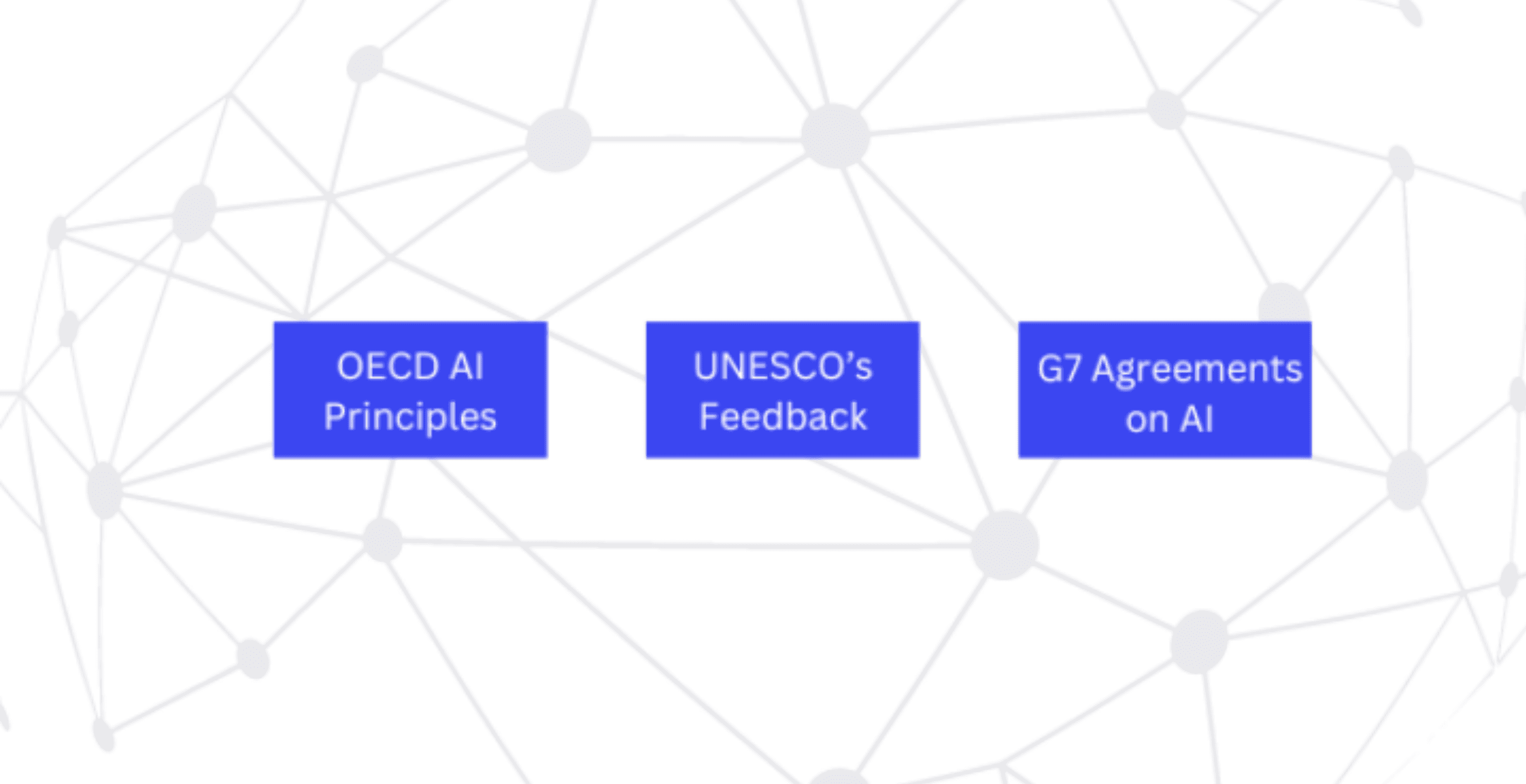
The international community has recognized the need for cohesive regulatory frameworks to guide the development and deployment of AI in finance. Key initiatives include:
OECD AI Principles: These principles focus on fostering innovation and trust in AI technologies while ensuring respect for human rights and democratic values. They emphasize AI systems' transparency, robustness, and safety, particularly in high-stakes sectors like finance.
UNESCO’s Recommendation on the Ethics of AI: This recommendation outlines standards to ensure that AI development respects ethical principles and human values, promoting data privacy and transparency.
G7 Agreements on AI: These agreements among G7 countries aim to harmonize AI regulations across borders, ensuring that AI supports economic growth and societal benefits without compromising financial stability.
Key Jurisdictional Developments in the EU, UK, and Japan
Different regions have taken unique approaches to AI regulation:
European Union (EU): The EU has been at the forefront with the proposed AI Act, which sets clear guidelines for high-risk AI applications in finance, such as ensuring algorithmic transparency and accountability.
United Kingdom (UK): The UK focuses on sector-specific guidelines and has established a sandbox environment where fintech firms can test AI technologies in a controlled regulatory space.
Japan: Japan emphasizes collaboration between the private sector and government to drive AI innovation while ensuring stringent data protection and security measures in financial services.
US State-Level AI Legislation and Federal Interests
In the United States, AI regulation is evolving at both state and federal levels:
State-Level Legislation: States like California and New York have implemented laws specifically addressing AI's use in decision-making processes, including transparency in AI-driven credit scoring systems.
Federal Interests: At the federal level, initiatives such as those from the National Institute of Standards and Technology (NIST) provide guidelines for deploying trustworthy AI systems, focusing on ethical, legal, and societal implications for AI in finance.
AI Regulation in the United States
The global landscape for AI regulation in finance is complex and dynamic, with international and regional frameworks evolving to address the rapid advancements in AI technologies. As we delve deeper into specific regions like the United States and Europe, we'll explore how these regulations.
State-level Legislation and Proposed Federal Laws
In the U.S., AI regulation varies significantly across states, reflecting diverse approaches to balancing innovation with consumer protection and ethical considerations:
State-Level Legislation: States like California have enacted laws that specifically address the transparency of AI systems used in hiring processes. These regulations require companies to disclose when AI is used in making employment decisions. They are designed to prevent biases and ensure fairness in AI applications.
Proposed Federal Laws: At the federal level, there are ongoing discussions about comprehensive AI legislation that would provide a unified framework for the use of AI across various sectors, including finance. Proposed laws focus on enhancing AI systems' transparency, accountability, and security to protect consumers and maintain trust in AI technologies.
Read more on USA AI Policies
Guidance from the NIST
The National Institute of Standards and Technology (NIST) plays a crucial role in setting standards for AI that bolster its safety, reliability, and interoperability. NIST's guidance often includes:
Frameworks for AI Testing and Validation: Ensuring that AI systems are rigorously tested and validated for their intended financial services applications.
Security Protocols for AI Systems: Developing robust security measures to protect sensitive financial data processed by AI, mitigating risks associated with data breaches and cyber-attacks.
AI Bill of Rights
The White House has proposed a Blueprint for an AI Bill of Rights, which outlines key protections that should be afforded to all Americans in the age of AI. This blueprint emphasizes:
Privacy Rights: Safeguarding personal data against misuse and ensuring individuals have control over their information.
Non-discrimination: Ensuring AI systems do not perpetuate biases or discriminatory practices, particularly in critical areas like credit scoring and lending.
Algorithmic Transparency: Requiring that the operations of AI systems be understandable to users, with clear explanations of how decisions are made, especially those impacting financial opportunities and livelihoods.
The regulatory framework in the United States aims to foster an environment where AI can thrive while ensuring it operates in a secure, transparent, and equitable manner.
As AI continues integrating into the financial sector, these regulations will play a pivotal role in shaping its development and ensuring its benefits are realized responsibly. Next, we'll explore how Europe contributes to shaping global AI regulations, particularly in financial services.
Read more on AI Regulations Worldwide
Europe's Role in Shaping AI Regulations
Let's explore Europe's significant role in shaping AI regulations, particularly focusing on the comprehensive frameworks it has established that influence global standards and practices, especially in the financial sector.
The EU AI Act and Its Implications for Financial Services
Europe has been a frontrunner in regulating AI, with the European Union's AI Act representing one of the most ambitious legislative efforts globally. This act categorizes AI applications according to risk levels, imposing stricter requirements on high-risk sectors like finance. Critical provisions for financial services include:
Risk Assessment and Mitigation: Financial institutions must conduct thorough risk assessments of their AI systems to ensure they operate safely and without bias.
Transparency Requirements: AI systems used in finance must be transparent, with clear explanations provided to customers about how their data is used, and decisions are made, especially for AI-driven credit scoring or investment advice.
Europe's influence extends beyond its borders, as it has played a critical role in shaping international AI policies:
OECD AI Principles Endorsement: The EU has been instrumental in developing and endorsing the OECD AI Principles, advocating for responsible stewardship of trustworthy AI globally.
Hiroshima AI Process: Under this initiative, Europe has pushed for a global Code of Conduct for AI, promoting ethical standards and practices that ensure AI's benefits are distributed equitably across societies.
The EU AI Act's Global Impact
The EU AI Act is pivotal for European countries and serves as a benchmark for other regions developing their AI regulations.
Its comprehensive approach is influencing how global markets, including the US and Asia, regulate AI technologies, especially in critical areas such as financial services, where the potential for harm is significant.
The act's focus on ethical considerations and consumer protections sets a high standard for what responsible AI deployment should look like.
Europe's proactive approach to AI regulation, mainly through the EU AI Act, highlights its commitment to ensuring that AI development is balanced with the need to protect consumers and maintain ethical standards. This legislative framework influences European financial markets and sets a global precedent for how AI should be regulated in sensitive sectors.
Next, we'll discuss how institutions can ensure compliance with these regulations and the recommended practices for deploying AI in finance responsibly. Ready to move on to compliance and regulatory recommendations?
Compliance and Regulatory Recommendations
Let's dive into the essential compliance and regulatory recommendations for deploying AI in the financial sector, emphasizing the core principles that ensure these systems are used responsibly and effectively.
Compliance in AI, especially within the financial sector, revolves around several key principles:
Training: AI systems should use diverse and representative data sets to avoid biases and ensure fair outcomes.
Testing: Rigorous testing must be conducted to verify the accuracy and reliability of AI models under various conditions.
Monitoring: Continuous monitoring is essential to quickly identify and address any issues that may arise during the operation of AI systems, ensuring they continue to function as intended.
Auditing: Regular audits are necessary to ensure compliance with all relevant laws and regulations and to maintain transparency and accountability in AI operations.
Inclusion of Legal Counsel in AI Policy Development
Involving legal counsel in developing AI policies is crucial to ensure that all AI implementations comply with existing legal frameworks and ethical standards. Legal experts can provide guidance on regulatory requirements, help assess potential legal risks, and ensure that AI deployments are both effective and compliant with the law.
Prioritizing Data Quality and Security in AI Model Training
Data quality and security are paramount in AI model training:
Data Quality: High-quality data is essential for training effective AI models. This means ensuring the data is accurate, comprehensive, and free of biases.
Data Security: Protecting sensitive information used in AI training is crucial to prevent data breaches and maintain consumer trust. Implementing robust data security measures, such as encryption and access controls, is essential.
Ensuring Thorough Documentation for Auditability of AI Processes
Thorough documentation of AI processes is vital for auditability and compliance. Documentation should include details on the design and development of AI systems, data handling practices, decision-making processes, and any incidents or modifications. This transparency helps regulators and stakeholders understand how AI systems work and assess their compliance with regulatory standards.
These compliance and regulatory recommendations are designed to help financial institutions implement AI responsibly, ensuring that they not only reap the benefits of AI but also maintain the trust and confidence of their customers and regulators. Next, we'll discuss the specific risks associated with AI in financial institutions and how appropriate regulations can help mitigate these risks.
AI Risks and Financial Institution Accountability
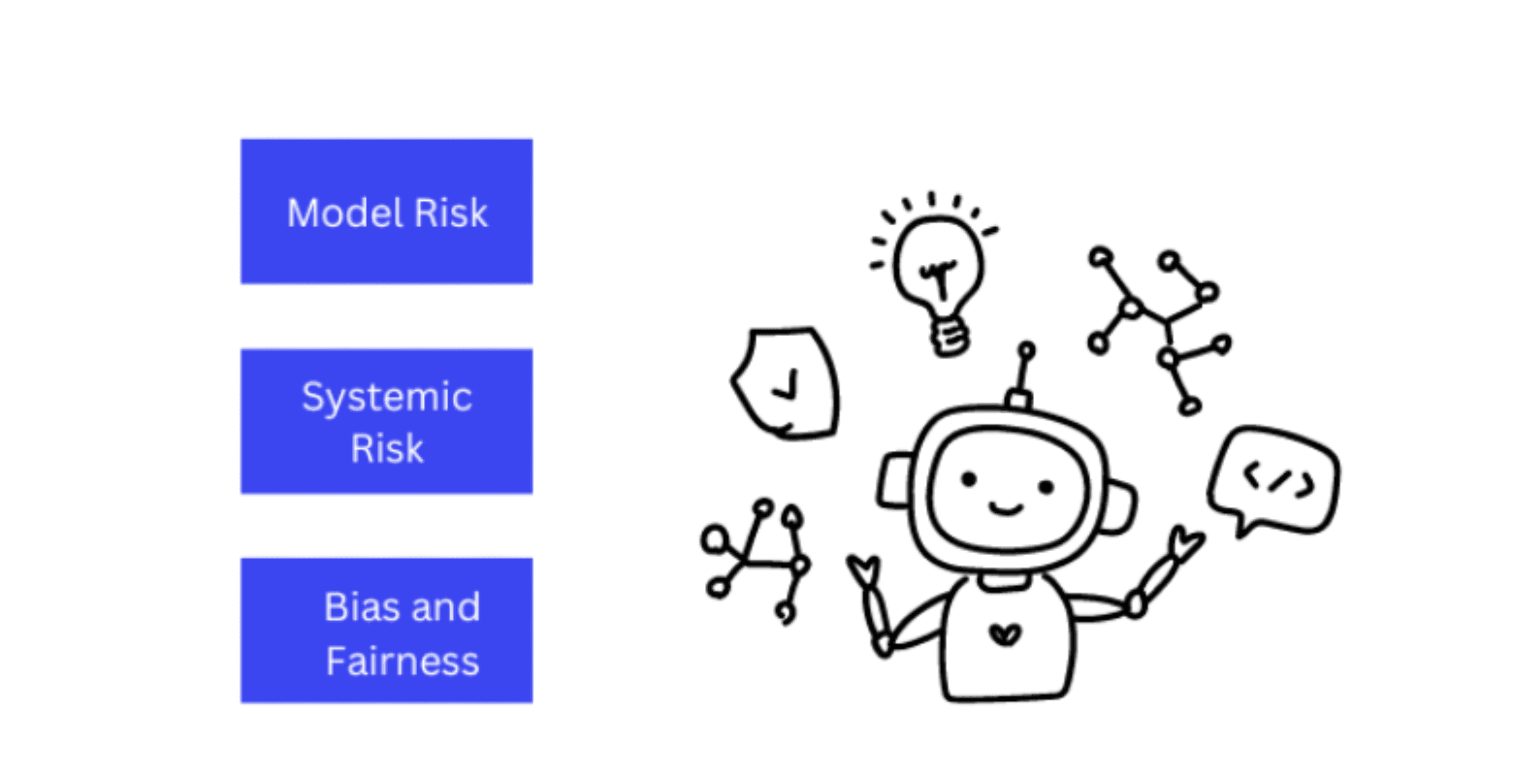
Understanding and mitigating the risks associated with AI in financial institutions is critical for ensuring that these technologies are used responsibly and effectively. As we move forward, we will discuss how financial institutions can prepare for the future of AI, including expected developments in regulations and technologies.
AI technologies, while offering significant benefits in terms of efficiency and decision-making, also pose unique risks that can amplify existing challenges within financial institutions. These risks include:
Model Risk: The risk that AI models may not perform as expected under different market conditions or may fail to adequately handle edge cases.
Systemic Risk: The potential for AI-driven decisions to lead to widespread issues within the financial system, especially if similar AI strategies are adopted across multiple institutions.
Bias and Fairness: AI systems can inadvertently perpetuate or even exacerbate biases present in the training data, leading to unfair outcomes for certain groups of customers.
To effectively manage these risks, robust regulatory frameworks are essential. These frameworks should focus on:
Regulation: Establishing clear guidelines for the development, deployment, and operation of AI systems within financial institutions to ensure they are safe and fair.
Supervision: Regular monitoring by regulatory bodies to ensure that financial institutions comply with AI governance standards and that their AI systems do not pose undue risks to the financial system.
Accountability: Implementing mechanisms that hold institutions accountable for the decisions made by their AI systems, ensuring that there is always a clear line of responsibility.
Read more on EU AI Act Insights
Addressing AI Risks in Practice
Implementing practical measures to manage AI risks involves:
Risk Assessment Protocols: Financial institutions must regularly assess the risks associated with their AI applications, considering factors like data integrity, model robustness, and potential impacts on customers.
Ethics and Compliance Committees: Establishing dedicated committees to oversee AI ethics and compliance can help ensure that AI applications adhere to both internal and external ethical standards.
Transparent Reporting: Institutions should be transparent about their use of AI, including the risks involved and the measures taken to mitigate these risks. This transparency is crucial for maintaining stakeholder trust.
Read more on AI Governance Strategies
Facing the Future of AI in Finance
The landscape of AI regulation in finance is rapidly evolving to keep pace with technological advancements. As AI systems become more complex and integral to financial operations, regulators worldwide are working to update and refine legal frameworks to ensure these technologies are used safely and ethically.
This includes establishing clearer guidelines for accountability, transparency, and data protection in AI applications.
Looking ahead, several key regulatory developments are expected to significantly impact the financial sector:
EU AI Act: This comprehensive legislative framework is set to establish risk-based classifications for AI systems, mandating stringent compliance requirements for high-risk applications in finance.
SEC Proposals: In the United States, the Securities and Exchange Commission (SEC) is proposing new regulations aimed at enhancing transparency in AI-driven investment strategies and financial products, ensuring that investors are adequately informed about the AI methodologies that influence their investments.
Global Harmonization Efforts: There is an increasing call for international cooperation to harmonize AI regulations, which would facilitate smoother cross-border operations of multinational financial institutions and prevent regulatory arbitrage.
The Call for a Harmonized Approach to AI Regulation Internationally
The need for a harmonized international approach to AI regulation in finance cannot be overstated. As financial markets are inherently global, disparate regulatory environments can lead to inconsistencies that complicate compliance for international entities and potentially hinder innovation.
A coordinated effort to establish common standards and practices can help ensure that AI technologies are developed and used in ways that benefit the global community while safeguarding against systemic risks.
Ready to transform your approach to AI with cutting-edge insights and tools? Explore RagaAI's comprehensive suite of AI solutions — from enhancing AI reliability with our guardrails to navigating the complexities of AI governance.
Whether diving into prompt engineering, seeking to mitigate AI biases, or exploring the frontier of AI testing, RagaAI is your partner in pioneering a safer, more efficient AI-driven future. Discover more and join us in shaping the next wave of AI innovation. Let's embark on this journey together with RagaAI.
Artificial Intelligence (AI) is dramatically transforming the financial sector, revolutionizing how companies approach everything from risk management to customer service.
The integration of AI technologies has enabled financial institutions to analyze large volumes of data with unprecedented speed and accuracy, leading to more informed decision-making processes and increased efficiency.
The proliferation of AI in finance is primarily fueled by the exponential growth in data availability and significant advancements in computing power.
Financial institutions can now process complex algorithms that were previously impractical, leveraging AI to gain insights from data patterns that human analysts could easily overlook. This capability enhances predictive analytics in financial markets, customer behavior analysis, and personalized banking services.
Balance Innovation with Financial Stability
Comprehensive regulatory frameworks are critical as AI technologies become more embedded in the financial sector. These regulations are essential to ensure that AI-driven innovations do not undermine the stability of financial systems or lead to unintended consequences such as systemic risks or discriminatory practices.
Proper regulatory measures must strike a balance between fostering innovation and protecting the integrity of the financial system, ensuring that advancements in AI contribute positively to the industry without compromising consumer trust or economic security.
Let's explore the specific AI developments and use cases in the financial sector, examining how these technologies are applied to enhance operations, manage risks, and improve customer engagement.
Read more on AI Developments in Finance
AI Development and Use Cases in Finance
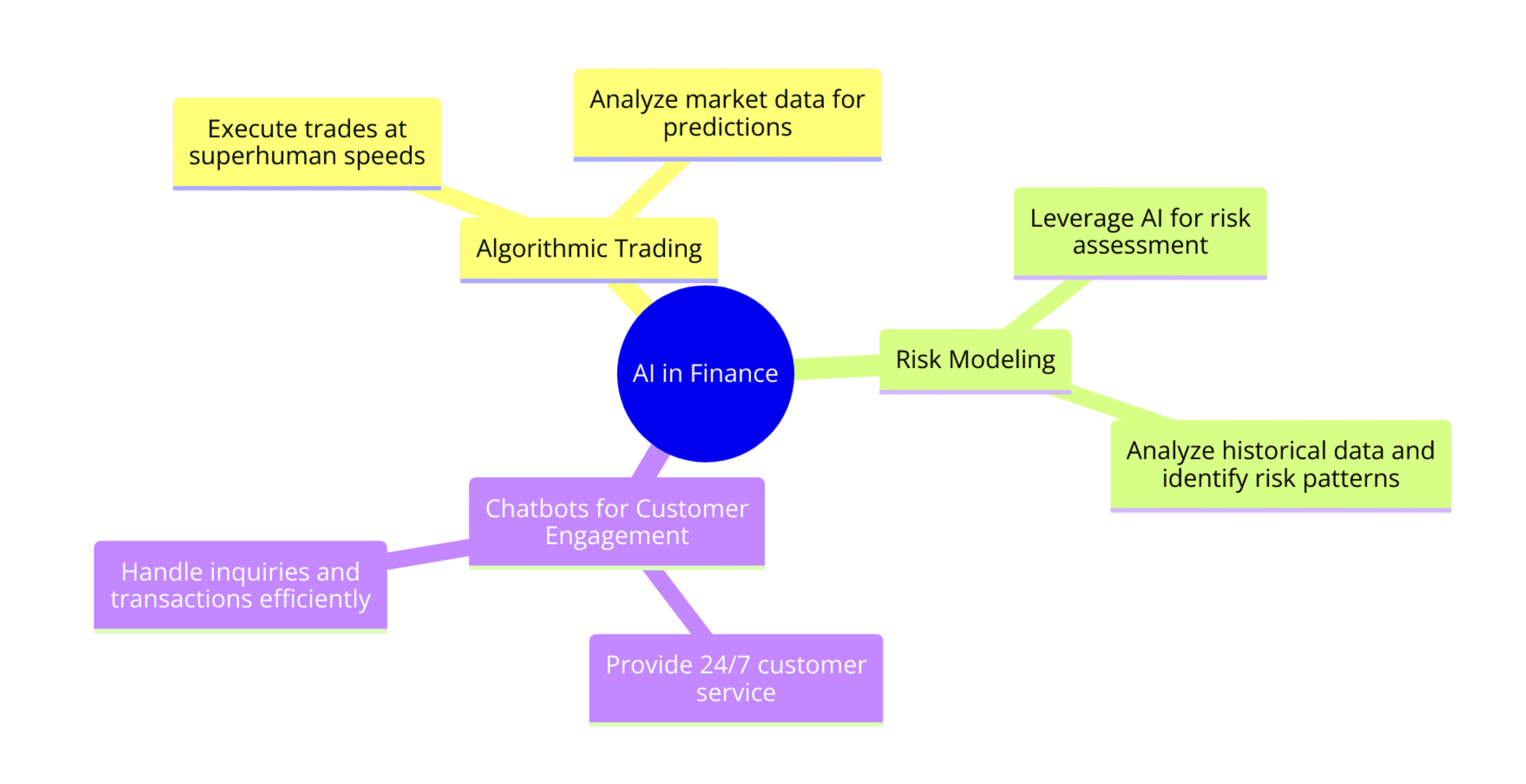
AI technologies have significantly advanced the capabilities of financial institutions in several key areas:
Algorithmic Trading: AI systems can execute trades at superhuman speeds and analyze market data to make predictions, optimizing trading strategies for better returns.
Risk Modeling: Financial institutions can more effectively predict and mitigate potential losses by leveraging AI for risk assessment. AI models can analyze historical data and identify risk patterns that might elude traditional methods.
Chatbots for Customer Engagement: AI-driven chatbots provide 24/7 customer service, handling inquiries and transactions with greater accuracy and efficiency. This not only enhances customer experience but also reduces operational costs.
AI's Role in Enhancing Operational Capacity, Productivity, and Unifying Data Silos
AI is pivotal in streamlining operations within financial institutions. By automating routine tasks, AI frees up human resources for more complex decision-making processes, enhancing overall productivity. Additionally, AI helps break down data silos within organizations by integrating disparate data sources, offering a unified view that facilitates better decision-making.
AI's ability to sift through vast amounts of data and identify patterns makes it an invaluable tool in combating financial crimes:
Anti-Money Laundering (AML): AI systems can track and analyze financial transactions to spot unusual patterns that may indicate money laundering activities, significantly improving the speed and accuracy of AML investigations.
Know-Your-Customer (KYC): AI enhances KYC processes by quickly validating customer data against multiple verification databases, ensuring compliance with regulatory requirements, and reducing the potential for fraud.
Let's explore the global regulatory landscape for AI in finance, detailing international commitments, jurisdiction-specific developments, and key examples of how various regions are addressing the challenges and opportunities presented by AI technologies.
Read more on AI Use Cases
Global Regulatory Landscape for AI in Finance
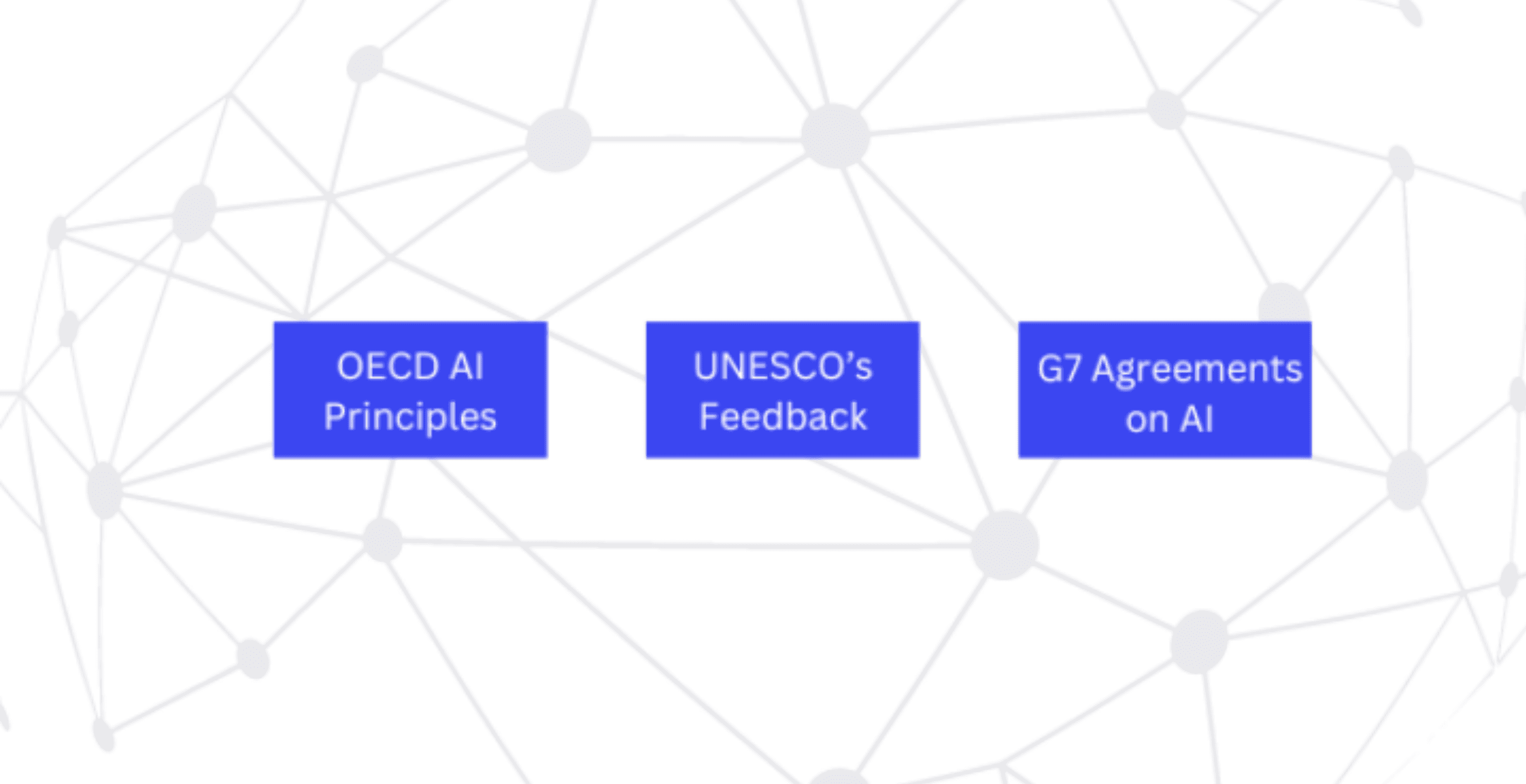
The international community has recognized the need for cohesive regulatory frameworks to guide the development and deployment of AI in finance. Key initiatives include:
OECD AI Principles: These principles focus on fostering innovation and trust in AI technologies while ensuring respect for human rights and democratic values. They emphasize AI systems' transparency, robustness, and safety, particularly in high-stakes sectors like finance.
UNESCO’s Recommendation on the Ethics of AI: This recommendation outlines standards to ensure that AI development respects ethical principles and human values, promoting data privacy and transparency.
G7 Agreements on AI: These agreements among G7 countries aim to harmonize AI regulations across borders, ensuring that AI supports economic growth and societal benefits without compromising financial stability.
Key Jurisdictional Developments in the EU, UK, and Japan
Different regions have taken unique approaches to AI regulation:
European Union (EU): The EU has been at the forefront with the proposed AI Act, which sets clear guidelines for high-risk AI applications in finance, such as ensuring algorithmic transparency and accountability.
United Kingdom (UK): The UK focuses on sector-specific guidelines and has established a sandbox environment where fintech firms can test AI technologies in a controlled regulatory space.
Japan: Japan emphasizes collaboration between the private sector and government to drive AI innovation while ensuring stringent data protection and security measures in financial services.
US State-Level AI Legislation and Federal Interests
In the United States, AI regulation is evolving at both state and federal levels:
State-Level Legislation: States like California and New York have implemented laws specifically addressing AI's use in decision-making processes, including transparency in AI-driven credit scoring systems.
Federal Interests: At the federal level, initiatives such as those from the National Institute of Standards and Technology (NIST) provide guidelines for deploying trustworthy AI systems, focusing on ethical, legal, and societal implications for AI in finance.
AI Regulation in the United States
The global landscape for AI regulation in finance is complex and dynamic, with international and regional frameworks evolving to address the rapid advancements in AI technologies. As we delve deeper into specific regions like the United States and Europe, we'll explore how these regulations.
State-level Legislation and Proposed Federal Laws
In the U.S., AI regulation varies significantly across states, reflecting diverse approaches to balancing innovation with consumer protection and ethical considerations:
State-Level Legislation: States like California have enacted laws that specifically address the transparency of AI systems used in hiring processes. These regulations require companies to disclose when AI is used in making employment decisions. They are designed to prevent biases and ensure fairness in AI applications.
Proposed Federal Laws: At the federal level, there are ongoing discussions about comprehensive AI legislation that would provide a unified framework for the use of AI across various sectors, including finance. Proposed laws focus on enhancing AI systems' transparency, accountability, and security to protect consumers and maintain trust in AI technologies.
Read more on USA AI Policies
Guidance from the NIST
The National Institute of Standards and Technology (NIST) plays a crucial role in setting standards for AI that bolster its safety, reliability, and interoperability. NIST's guidance often includes:
Frameworks for AI Testing and Validation: Ensuring that AI systems are rigorously tested and validated for their intended financial services applications.
Security Protocols for AI Systems: Developing robust security measures to protect sensitive financial data processed by AI, mitigating risks associated with data breaches and cyber-attacks.
AI Bill of Rights
The White House has proposed a Blueprint for an AI Bill of Rights, which outlines key protections that should be afforded to all Americans in the age of AI. This blueprint emphasizes:
Privacy Rights: Safeguarding personal data against misuse and ensuring individuals have control over their information.
Non-discrimination: Ensuring AI systems do not perpetuate biases or discriminatory practices, particularly in critical areas like credit scoring and lending.
Algorithmic Transparency: Requiring that the operations of AI systems be understandable to users, with clear explanations of how decisions are made, especially those impacting financial opportunities and livelihoods.
The regulatory framework in the United States aims to foster an environment where AI can thrive while ensuring it operates in a secure, transparent, and equitable manner.
As AI continues integrating into the financial sector, these regulations will play a pivotal role in shaping its development and ensuring its benefits are realized responsibly. Next, we'll explore how Europe contributes to shaping global AI regulations, particularly in financial services.
Read more on AI Regulations Worldwide
Europe's Role in Shaping AI Regulations
Let's explore Europe's significant role in shaping AI regulations, particularly focusing on the comprehensive frameworks it has established that influence global standards and practices, especially in the financial sector.
The EU AI Act and Its Implications for Financial Services
Europe has been a frontrunner in regulating AI, with the European Union's AI Act representing one of the most ambitious legislative efforts globally. This act categorizes AI applications according to risk levels, imposing stricter requirements on high-risk sectors like finance. Critical provisions for financial services include:
Risk Assessment and Mitigation: Financial institutions must conduct thorough risk assessments of their AI systems to ensure they operate safely and without bias.
Transparency Requirements: AI systems used in finance must be transparent, with clear explanations provided to customers about how their data is used, and decisions are made, especially for AI-driven credit scoring or investment advice.
Europe's influence extends beyond its borders, as it has played a critical role in shaping international AI policies:
OECD AI Principles Endorsement: The EU has been instrumental in developing and endorsing the OECD AI Principles, advocating for responsible stewardship of trustworthy AI globally.
Hiroshima AI Process: Under this initiative, Europe has pushed for a global Code of Conduct for AI, promoting ethical standards and practices that ensure AI's benefits are distributed equitably across societies.
The EU AI Act's Global Impact
The EU AI Act is pivotal for European countries and serves as a benchmark for other regions developing their AI regulations.
Its comprehensive approach is influencing how global markets, including the US and Asia, regulate AI technologies, especially in critical areas such as financial services, where the potential for harm is significant.
The act's focus on ethical considerations and consumer protections sets a high standard for what responsible AI deployment should look like.
Europe's proactive approach to AI regulation, mainly through the EU AI Act, highlights its commitment to ensuring that AI development is balanced with the need to protect consumers and maintain ethical standards. This legislative framework influences European financial markets and sets a global precedent for how AI should be regulated in sensitive sectors.
Next, we'll discuss how institutions can ensure compliance with these regulations and the recommended practices for deploying AI in finance responsibly. Ready to move on to compliance and regulatory recommendations?
Compliance and Regulatory Recommendations
Let's dive into the essential compliance and regulatory recommendations for deploying AI in the financial sector, emphasizing the core principles that ensure these systems are used responsibly and effectively.
Compliance in AI, especially within the financial sector, revolves around several key principles:
Training: AI systems should use diverse and representative data sets to avoid biases and ensure fair outcomes.
Testing: Rigorous testing must be conducted to verify the accuracy and reliability of AI models under various conditions.
Monitoring: Continuous monitoring is essential to quickly identify and address any issues that may arise during the operation of AI systems, ensuring they continue to function as intended.
Auditing: Regular audits are necessary to ensure compliance with all relevant laws and regulations and to maintain transparency and accountability in AI operations.
Inclusion of Legal Counsel in AI Policy Development
Involving legal counsel in developing AI policies is crucial to ensure that all AI implementations comply with existing legal frameworks and ethical standards. Legal experts can provide guidance on regulatory requirements, help assess potential legal risks, and ensure that AI deployments are both effective and compliant with the law.
Prioritizing Data Quality and Security in AI Model Training
Data quality and security are paramount in AI model training:
Data Quality: High-quality data is essential for training effective AI models. This means ensuring the data is accurate, comprehensive, and free of biases.
Data Security: Protecting sensitive information used in AI training is crucial to prevent data breaches and maintain consumer trust. Implementing robust data security measures, such as encryption and access controls, is essential.
Ensuring Thorough Documentation for Auditability of AI Processes
Thorough documentation of AI processes is vital for auditability and compliance. Documentation should include details on the design and development of AI systems, data handling practices, decision-making processes, and any incidents or modifications. This transparency helps regulators and stakeholders understand how AI systems work and assess their compliance with regulatory standards.
These compliance and regulatory recommendations are designed to help financial institutions implement AI responsibly, ensuring that they not only reap the benefits of AI but also maintain the trust and confidence of their customers and regulators. Next, we'll discuss the specific risks associated with AI in financial institutions and how appropriate regulations can help mitigate these risks.
AI Risks and Financial Institution Accountability
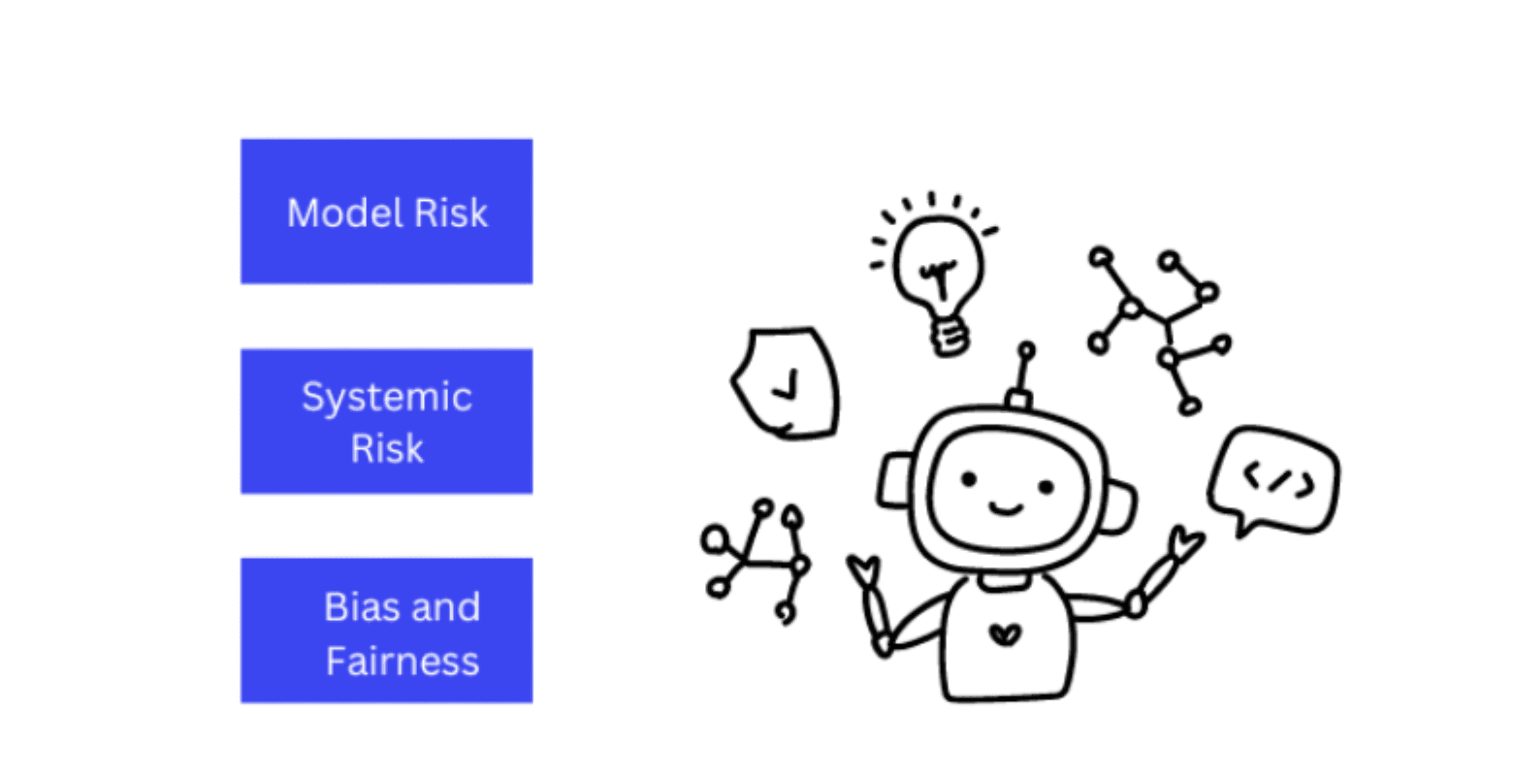
Understanding and mitigating the risks associated with AI in financial institutions is critical for ensuring that these technologies are used responsibly and effectively. As we move forward, we will discuss how financial institutions can prepare for the future of AI, including expected developments in regulations and technologies.
AI technologies, while offering significant benefits in terms of efficiency and decision-making, also pose unique risks that can amplify existing challenges within financial institutions. These risks include:
Model Risk: The risk that AI models may not perform as expected under different market conditions or may fail to adequately handle edge cases.
Systemic Risk: The potential for AI-driven decisions to lead to widespread issues within the financial system, especially if similar AI strategies are adopted across multiple institutions.
Bias and Fairness: AI systems can inadvertently perpetuate or even exacerbate biases present in the training data, leading to unfair outcomes for certain groups of customers.
To effectively manage these risks, robust regulatory frameworks are essential. These frameworks should focus on:
Regulation: Establishing clear guidelines for the development, deployment, and operation of AI systems within financial institutions to ensure they are safe and fair.
Supervision: Regular monitoring by regulatory bodies to ensure that financial institutions comply with AI governance standards and that their AI systems do not pose undue risks to the financial system.
Accountability: Implementing mechanisms that hold institutions accountable for the decisions made by their AI systems, ensuring that there is always a clear line of responsibility.
Read more on EU AI Act Insights
Addressing AI Risks in Practice
Implementing practical measures to manage AI risks involves:
Risk Assessment Protocols: Financial institutions must regularly assess the risks associated with their AI applications, considering factors like data integrity, model robustness, and potential impacts on customers.
Ethics and Compliance Committees: Establishing dedicated committees to oversee AI ethics and compliance can help ensure that AI applications adhere to both internal and external ethical standards.
Transparent Reporting: Institutions should be transparent about their use of AI, including the risks involved and the measures taken to mitigate these risks. This transparency is crucial for maintaining stakeholder trust.
Read more on AI Governance Strategies
Facing the Future of AI in Finance
The landscape of AI regulation in finance is rapidly evolving to keep pace with technological advancements. As AI systems become more complex and integral to financial operations, regulators worldwide are working to update and refine legal frameworks to ensure these technologies are used safely and ethically.
This includes establishing clearer guidelines for accountability, transparency, and data protection in AI applications.
Looking ahead, several key regulatory developments are expected to significantly impact the financial sector:
EU AI Act: This comprehensive legislative framework is set to establish risk-based classifications for AI systems, mandating stringent compliance requirements for high-risk applications in finance.
SEC Proposals: In the United States, the Securities and Exchange Commission (SEC) is proposing new regulations aimed at enhancing transparency in AI-driven investment strategies and financial products, ensuring that investors are adequately informed about the AI methodologies that influence their investments.
Global Harmonization Efforts: There is an increasing call for international cooperation to harmonize AI regulations, which would facilitate smoother cross-border operations of multinational financial institutions and prevent regulatory arbitrage.
The Call for a Harmonized Approach to AI Regulation Internationally
The need for a harmonized international approach to AI regulation in finance cannot be overstated. As financial markets are inherently global, disparate regulatory environments can lead to inconsistencies that complicate compliance for international entities and potentially hinder innovation.
A coordinated effort to establish common standards and practices can help ensure that AI technologies are developed and used in ways that benefit the global community while safeguarding against systemic risks.
Ready to transform your approach to AI with cutting-edge insights and tools? Explore RagaAI's comprehensive suite of AI solutions — from enhancing AI reliability with our guardrails to navigating the complexities of AI governance.
Whether diving into prompt engineering, seeking to mitigate AI biases, or exploring the frontier of AI testing, RagaAI is your partner in pioneering a safer, more efficient AI-driven future. Discover more and join us in shaping the next wave of AI innovation. Let's embark on this journey together with RagaAI.
Artificial Intelligence (AI) is dramatically transforming the financial sector, revolutionizing how companies approach everything from risk management to customer service.
The integration of AI technologies has enabled financial institutions to analyze large volumes of data with unprecedented speed and accuracy, leading to more informed decision-making processes and increased efficiency.
The proliferation of AI in finance is primarily fueled by the exponential growth in data availability and significant advancements in computing power.
Financial institutions can now process complex algorithms that were previously impractical, leveraging AI to gain insights from data patterns that human analysts could easily overlook. This capability enhances predictive analytics in financial markets, customer behavior analysis, and personalized banking services.
Balance Innovation with Financial Stability
Comprehensive regulatory frameworks are critical as AI technologies become more embedded in the financial sector. These regulations are essential to ensure that AI-driven innovations do not undermine the stability of financial systems or lead to unintended consequences such as systemic risks or discriminatory practices.
Proper regulatory measures must strike a balance between fostering innovation and protecting the integrity of the financial system, ensuring that advancements in AI contribute positively to the industry without compromising consumer trust or economic security.
Let's explore the specific AI developments and use cases in the financial sector, examining how these technologies are applied to enhance operations, manage risks, and improve customer engagement.
Read more on AI Developments in Finance
AI Development and Use Cases in Finance
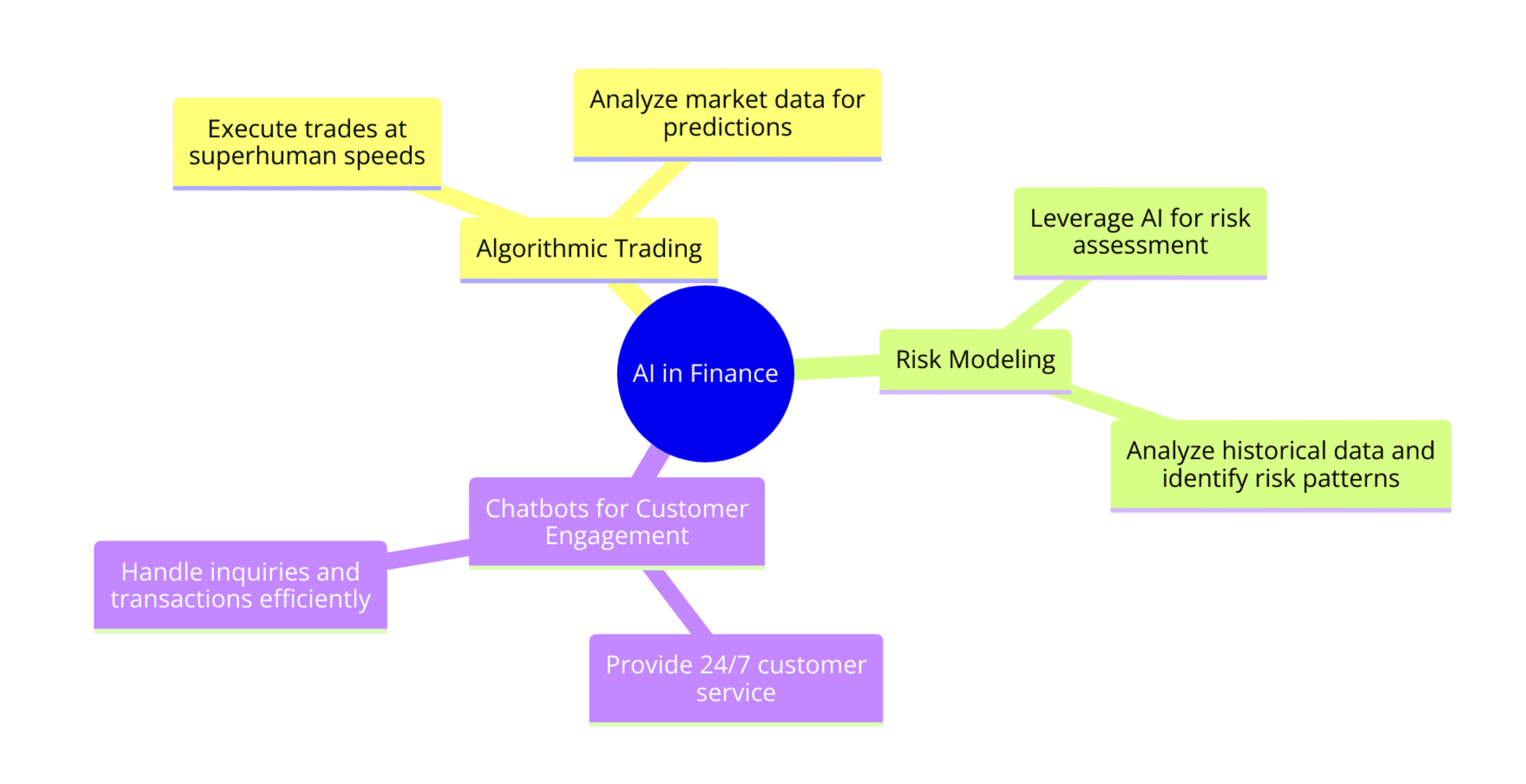
AI technologies have significantly advanced the capabilities of financial institutions in several key areas:
Algorithmic Trading: AI systems can execute trades at superhuman speeds and analyze market data to make predictions, optimizing trading strategies for better returns.
Risk Modeling: Financial institutions can more effectively predict and mitigate potential losses by leveraging AI for risk assessment. AI models can analyze historical data and identify risk patterns that might elude traditional methods.
Chatbots for Customer Engagement: AI-driven chatbots provide 24/7 customer service, handling inquiries and transactions with greater accuracy and efficiency. This not only enhances customer experience but also reduces operational costs.
AI's Role in Enhancing Operational Capacity, Productivity, and Unifying Data Silos
AI is pivotal in streamlining operations within financial institutions. By automating routine tasks, AI frees up human resources for more complex decision-making processes, enhancing overall productivity. Additionally, AI helps break down data silos within organizations by integrating disparate data sources, offering a unified view that facilitates better decision-making.
AI's ability to sift through vast amounts of data and identify patterns makes it an invaluable tool in combating financial crimes:
Anti-Money Laundering (AML): AI systems can track and analyze financial transactions to spot unusual patterns that may indicate money laundering activities, significantly improving the speed and accuracy of AML investigations.
Know-Your-Customer (KYC): AI enhances KYC processes by quickly validating customer data against multiple verification databases, ensuring compliance with regulatory requirements, and reducing the potential for fraud.
Let's explore the global regulatory landscape for AI in finance, detailing international commitments, jurisdiction-specific developments, and key examples of how various regions are addressing the challenges and opportunities presented by AI technologies.
Read more on AI Use Cases
Global Regulatory Landscape for AI in Finance
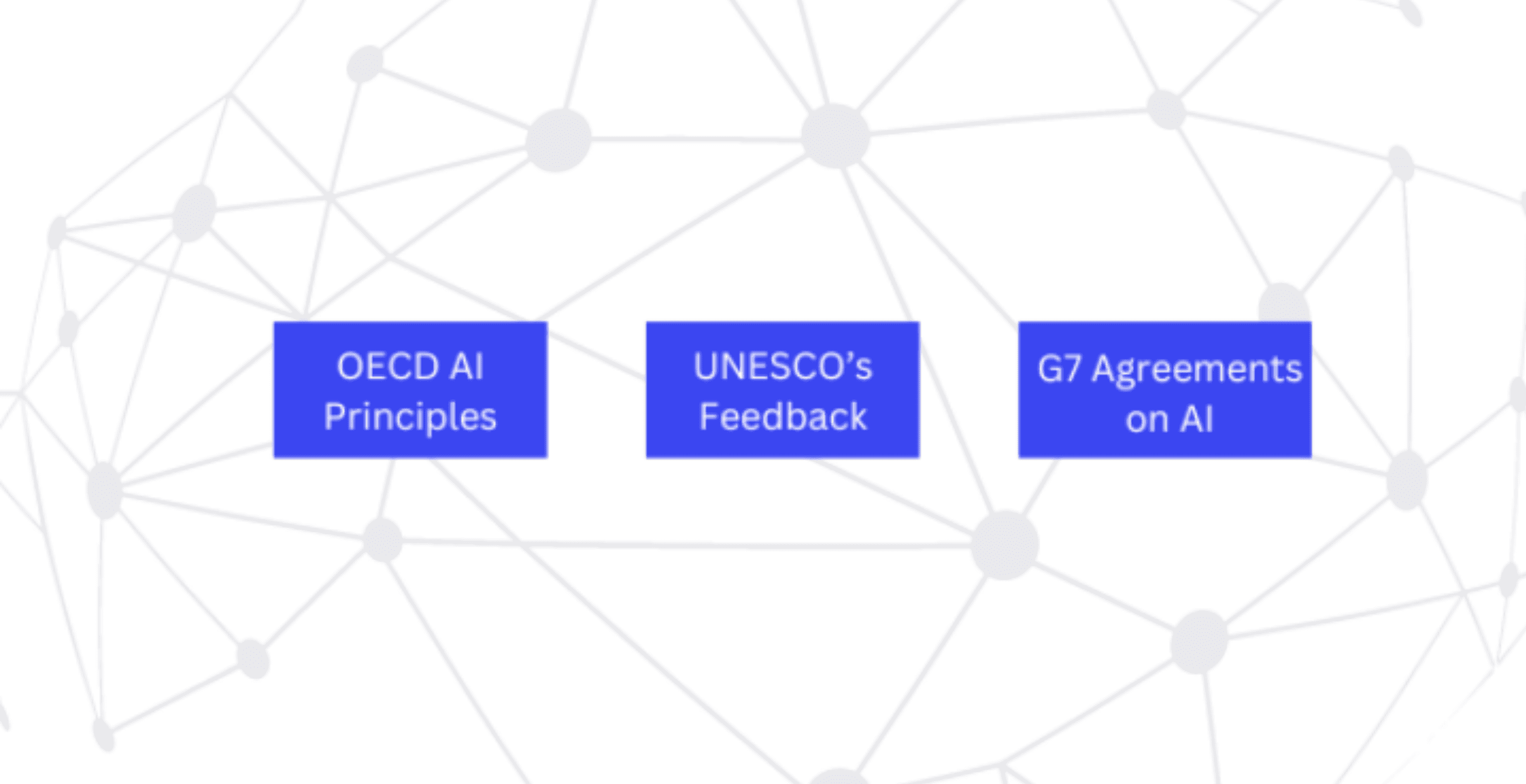
The international community has recognized the need for cohesive regulatory frameworks to guide the development and deployment of AI in finance. Key initiatives include:
OECD AI Principles: These principles focus on fostering innovation and trust in AI technologies while ensuring respect for human rights and democratic values. They emphasize AI systems' transparency, robustness, and safety, particularly in high-stakes sectors like finance.
UNESCO’s Recommendation on the Ethics of AI: This recommendation outlines standards to ensure that AI development respects ethical principles and human values, promoting data privacy and transparency.
G7 Agreements on AI: These agreements among G7 countries aim to harmonize AI regulations across borders, ensuring that AI supports economic growth and societal benefits without compromising financial stability.
Key Jurisdictional Developments in the EU, UK, and Japan
Different regions have taken unique approaches to AI regulation:
European Union (EU): The EU has been at the forefront with the proposed AI Act, which sets clear guidelines for high-risk AI applications in finance, such as ensuring algorithmic transparency and accountability.
United Kingdom (UK): The UK focuses on sector-specific guidelines and has established a sandbox environment where fintech firms can test AI technologies in a controlled regulatory space.
Japan: Japan emphasizes collaboration between the private sector and government to drive AI innovation while ensuring stringent data protection and security measures in financial services.
US State-Level AI Legislation and Federal Interests
In the United States, AI regulation is evolving at both state and federal levels:
State-Level Legislation: States like California and New York have implemented laws specifically addressing AI's use in decision-making processes, including transparency in AI-driven credit scoring systems.
Federal Interests: At the federal level, initiatives such as those from the National Institute of Standards and Technology (NIST) provide guidelines for deploying trustworthy AI systems, focusing on ethical, legal, and societal implications for AI in finance.
AI Regulation in the United States
The global landscape for AI regulation in finance is complex and dynamic, with international and regional frameworks evolving to address the rapid advancements in AI technologies. As we delve deeper into specific regions like the United States and Europe, we'll explore how these regulations.
State-level Legislation and Proposed Federal Laws
In the U.S., AI regulation varies significantly across states, reflecting diverse approaches to balancing innovation with consumer protection and ethical considerations:
State-Level Legislation: States like California have enacted laws that specifically address the transparency of AI systems used in hiring processes. These regulations require companies to disclose when AI is used in making employment decisions. They are designed to prevent biases and ensure fairness in AI applications.
Proposed Federal Laws: At the federal level, there are ongoing discussions about comprehensive AI legislation that would provide a unified framework for the use of AI across various sectors, including finance. Proposed laws focus on enhancing AI systems' transparency, accountability, and security to protect consumers and maintain trust in AI technologies.
Read more on USA AI Policies
Guidance from the NIST
The National Institute of Standards and Technology (NIST) plays a crucial role in setting standards for AI that bolster its safety, reliability, and interoperability. NIST's guidance often includes:
Frameworks for AI Testing and Validation: Ensuring that AI systems are rigorously tested and validated for their intended financial services applications.
Security Protocols for AI Systems: Developing robust security measures to protect sensitive financial data processed by AI, mitigating risks associated with data breaches and cyber-attacks.
AI Bill of Rights
The White House has proposed a Blueprint for an AI Bill of Rights, which outlines key protections that should be afforded to all Americans in the age of AI. This blueprint emphasizes:
Privacy Rights: Safeguarding personal data against misuse and ensuring individuals have control over their information.
Non-discrimination: Ensuring AI systems do not perpetuate biases or discriminatory practices, particularly in critical areas like credit scoring and lending.
Algorithmic Transparency: Requiring that the operations of AI systems be understandable to users, with clear explanations of how decisions are made, especially those impacting financial opportunities and livelihoods.
The regulatory framework in the United States aims to foster an environment where AI can thrive while ensuring it operates in a secure, transparent, and equitable manner.
As AI continues integrating into the financial sector, these regulations will play a pivotal role in shaping its development and ensuring its benefits are realized responsibly. Next, we'll explore how Europe contributes to shaping global AI regulations, particularly in financial services.
Read more on AI Regulations Worldwide
Europe's Role in Shaping AI Regulations
Let's explore Europe's significant role in shaping AI regulations, particularly focusing on the comprehensive frameworks it has established that influence global standards and practices, especially in the financial sector.
The EU AI Act and Its Implications for Financial Services
Europe has been a frontrunner in regulating AI, with the European Union's AI Act representing one of the most ambitious legislative efforts globally. This act categorizes AI applications according to risk levels, imposing stricter requirements on high-risk sectors like finance. Critical provisions for financial services include:
Risk Assessment and Mitigation: Financial institutions must conduct thorough risk assessments of their AI systems to ensure they operate safely and without bias.
Transparency Requirements: AI systems used in finance must be transparent, with clear explanations provided to customers about how their data is used, and decisions are made, especially for AI-driven credit scoring or investment advice.
Europe's influence extends beyond its borders, as it has played a critical role in shaping international AI policies:
OECD AI Principles Endorsement: The EU has been instrumental in developing and endorsing the OECD AI Principles, advocating for responsible stewardship of trustworthy AI globally.
Hiroshima AI Process: Under this initiative, Europe has pushed for a global Code of Conduct for AI, promoting ethical standards and practices that ensure AI's benefits are distributed equitably across societies.
The EU AI Act's Global Impact
The EU AI Act is pivotal for European countries and serves as a benchmark for other regions developing their AI regulations.
Its comprehensive approach is influencing how global markets, including the US and Asia, regulate AI technologies, especially in critical areas such as financial services, where the potential for harm is significant.
The act's focus on ethical considerations and consumer protections sets a high standard for what responsible AI deployment should look like.
Europe's proactive approach to AI regulation, mainly through the EU AI Act, highlights its commitment to ensuring that AI development is balanced with the need to protect consumers and maintain ethical standards. This legislative framework influences European financial markets and sets a global precedent for how AI should be regulated in sensitive sectors.
Next, we'll discuss how institutions can ensure compliance with these regulations and the recommended practices for deploying AI in finance responsibly. Ready to move on to compliance and regulatory recommendations?
Compliance and Regulatory Recommendations
Let's dive into the essential compliance and regulatory recommendations for deploying AI in the financial sector, emphasizing the core principles that ensure these systems are used responsibly and effectively.
Compliance in AI, especially within the financial sector, revolves around several key principles:
Training: AI systems should use diverse and representative data sets to avoid biases and ensure fair outcomes.
Testing: Rigorous testing must be conducted to verify the accuracy and reliability of AI models under various conditions.
Monitoring: Continuous monitoring is essential to quickly identify and address any issues that may arise during the operation of AI systems, ensuring they continue to function as intended.
Auditing: Regular audits are necessary to ensure compliance with all relevant laws and regulations and to maintain transparency and accountability in AI operations.
Inclusion of Legal Counsel in AI Policy Development
Involving legal counsel in developing AI policies is crucial to ensure that all AI implementations comply with existing legal frameworks and ethical standards. Legal experts can provide guidance on regulatory requirements, help assess potential legal risks, and ensure that AI deployments are both effective and compliant with the law.
Prioritizing Data Quality and Security in AI Model Training
Data quality and security are paramount in AI model training:
Data Quality: High-quality data is essential for training effective AI models. This means ensuring the data is accurate, comprehensive, and free of biases.
Data Security: Protecting sensitive information used in AI training is crucial to prevent data breaches and maintain consumer trust. Implementing robust data security measures, such as encryption and access controls, is essential.
Ensuring Thorough Documentation for Auditability of AI Processes
Thorough documentation of AI processes is vital for auditability and compliance. Documentation should include details on the design and development of AI systems, data handling practices, decision-making processes, and any incidents or modifications. This transparency helps regulators and stakeholders understand how AI systems work and assess their compliance with regulatory standards.
These compliance and regulatory recommendations are designed to help financial institutions implement AI responsibly, ensuring that they not only reap the benefits of AI but also maintain the trust and confidence of their customers and regulators. Next, we'll discuss the specific risks associated with AI in financial institutions and how appropriate regulations can help mitigate these risks.
AI Risks and Financial Institution Accountability
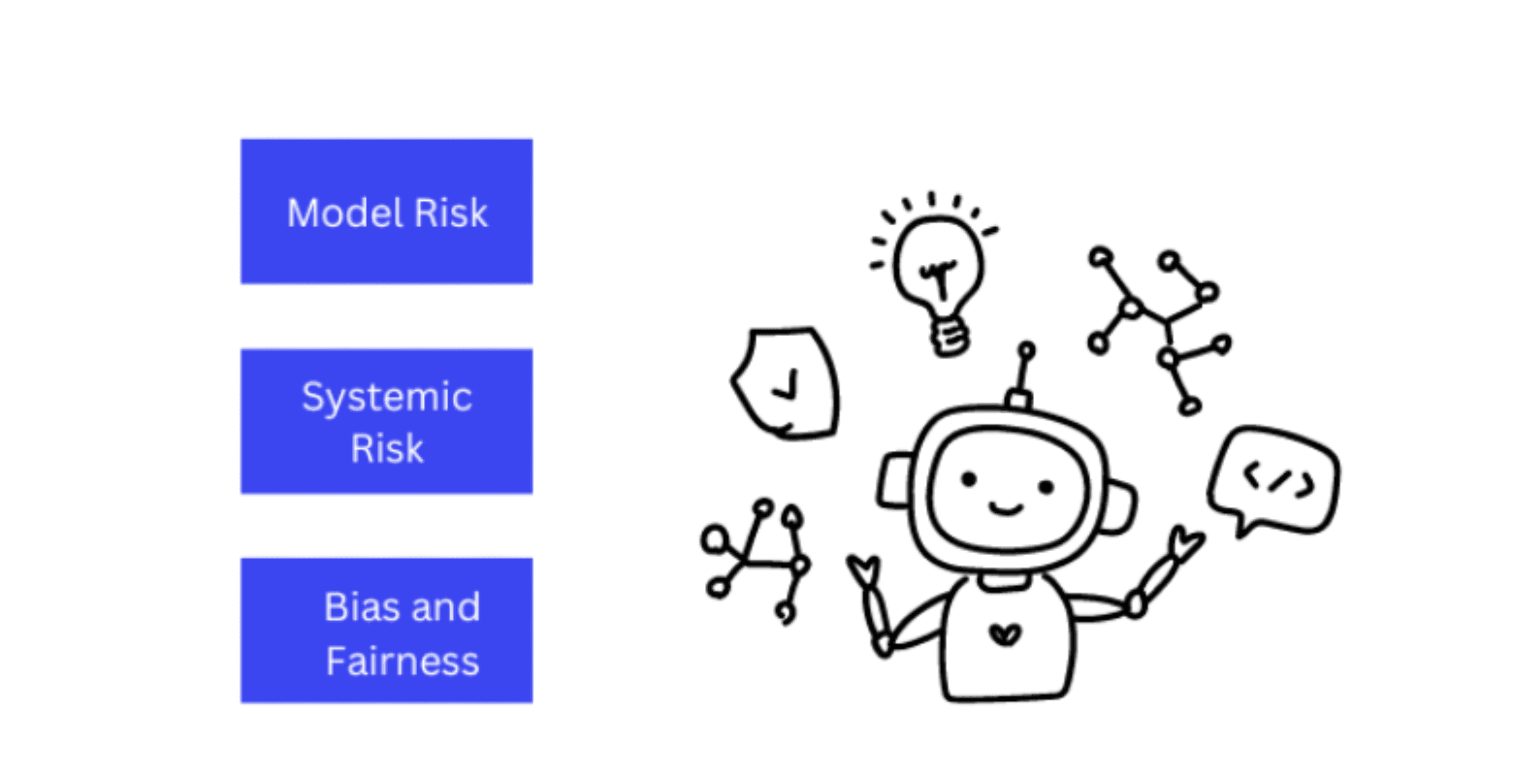
Understanding and mitigating the risks associated with AI in financial institutions is critical for ensuring that these technologies are used responsibly and effectively. As we move forward, we will discuss how financial institutions can prepare for the future of AI, including expected developments in regulations and technologies.
AI technologies, while offering significant benefits in terms of efficiency and decision-making, also pose unique risks that can amplify existing challenges within financial institutions. These risks include:
Model Risk: The risk that AI models may not perform as expected under different market conditions or may fail to adequately handle edge cases.
Systemic Risk: The potential for AI-driven decisions to lead to widespread issues within the financial system, especially if similar AI strategies are adopted across multiple institutions.
Bias and Fairness: AI systems can inadvertently perpetuate or even exacerbate biases present in the training data, leading to unfair outcomes for certain groups of customers.
To effectively manage these risks, robust regulatory frameworks are essential. These frameworks should focus on:
Regulation: Establishing clear guidelines for the development, deployment, and operation of AI systems within financial institutions to ensure they are safe and fair.
Supervision: Regular monitoring by regulatory bodies to ensure that financial institutions comply with AI governance standards and that their AI systems do not pose undue risks to the financial system.
Accountability: Implementing mechanisms that hold institutions accountable for the decisions made by their AI systems, ensuring that there is always a clear line of responsibility.
Read more on EU AI Act Insights
Addressing AI Risks in Practice
Implementing practical measures to manage AI risks involves:
Risk Assessment Protocols: Financial institutions must regularly assess the risks associated with their AI applications, considering factors like data integrity, model robustness, and potential impacts on customers.
Ethics and Compliance Committees: Establishing dedicated committees to oversee AI ethics and compliance can help ensure that AI applications adhere to both internal and external ethical standards.
Transparent Reporting: Institutions should be transparent about their use of AI, including the risks involved and the measures taken to mitigate these risks. This transparency is crucial for maintaining stakeholder trust.
Read more on AI Governance Strategies
Facing the Future of AI in Finance
The landscape of AI regulation in finance is rapidly evolving to keep pace with technological advancements. As AI systems become more complex and integral to financial operations, regulators worldwide are working to update and refine legal frameworks to ensure these technologies are used safely and ethically.
This includes establishing clearer guidelines for accountability, transparency, and data protection in AI applications.
Looking ahead, several key regulatory developments are expected to significantly impact the financial sector:
EU AI Act: This comprehensive legislative framework is set to establish risk-based classifications for AI systems, mandating stringent compliance requirements for high-risk applications in finance.
SEC Proposals: In the United States, the Securities and Exchange Commission (SEC) is proposing new regulations aimed at enhancing transparency in AI-driven investment strategies and financial products, ensuring that investors are adequately informed about the AI methodologies that influence their investments.
Global Harmonization Efforts: There is an increasing call for international cooperation to harmonize AI regulations, which would facilitate smoother cross-border operations of multinational financial institutions and prevent regulatory arbitrage.
The Call for a Harmonized Approach to AI Regulation Internationally
The need for a harmonized international approach to AI regulation in finance cannot be overstated. As financial markets are inherently global, disparate regulatory environments can lead to inconsistencies that complicate compliance for international entities and potentially hinder innovation.
A coordinated effort to establish common standards and practices can help ensure that AI technologies are developed and used in ways that benefit the global community while safeguarding against systemic risks.
Ready to transform your approach to AI with cutting-edge insights and tools? Explore RagaAI's comprehensive suite of AI solutions — from enhancing AI reliability with our guardrails to navigating the complexities of AI governance.
Whether diving into prompt engineering, seeking to mitigate AI biases, or exploring the frontier of AI testing, RagaAI is your partner in pioneering a safer, more efficient AI-driven future. Discover more and join us in shaping the next wave of AI innovation. Let's embark on this journey together with RagaAI.
Artificial Intelligence (AI) is dramatically transforming the financial sector, revolutionizing how companies approach everything from risk management to customer service.
The integration of AI technologies has enabled financial institutions to analyze large volumes of data with unprecedented speed and accuracy, leading to more informed decision-making processes and increased efficiency.
The proliferation of AI in finance is primarily fueled by the exponential growth in data availability and significant advancements in computing power.
Financial institutions can now process complex algorithms that were previously impractical, leveraging AI to gain insights from data patterns that human analysts could easily overlook. This capability enhances predictive analytics in financial markets, customer behavior analysis, and personalized banking services.
Balance Innovation with Financial Stability
Comprehensive regulatory frameworks are critical as AI technologies become more embedded in the financial sector. These regulations are essential to ensure that AI-driven innovations do not undermine the stability of financial systems or lead to unintended consequences such as systemic risks or discriminatory practices.
Proper regulatory measures must strike a balance between fostering innovation and protecting the integrity of the financial system, ensuring that advancements in AI contribute positively to the industry without compromising consumer trust or economic security.
Let's explore the specific AI developments and use cases in the financial sector, examining how these technologies are applied to enhance operations, manage risks, and improve customer engagement.
Read more on AI Developments in Finance
AI Development and Use Cases in Finance
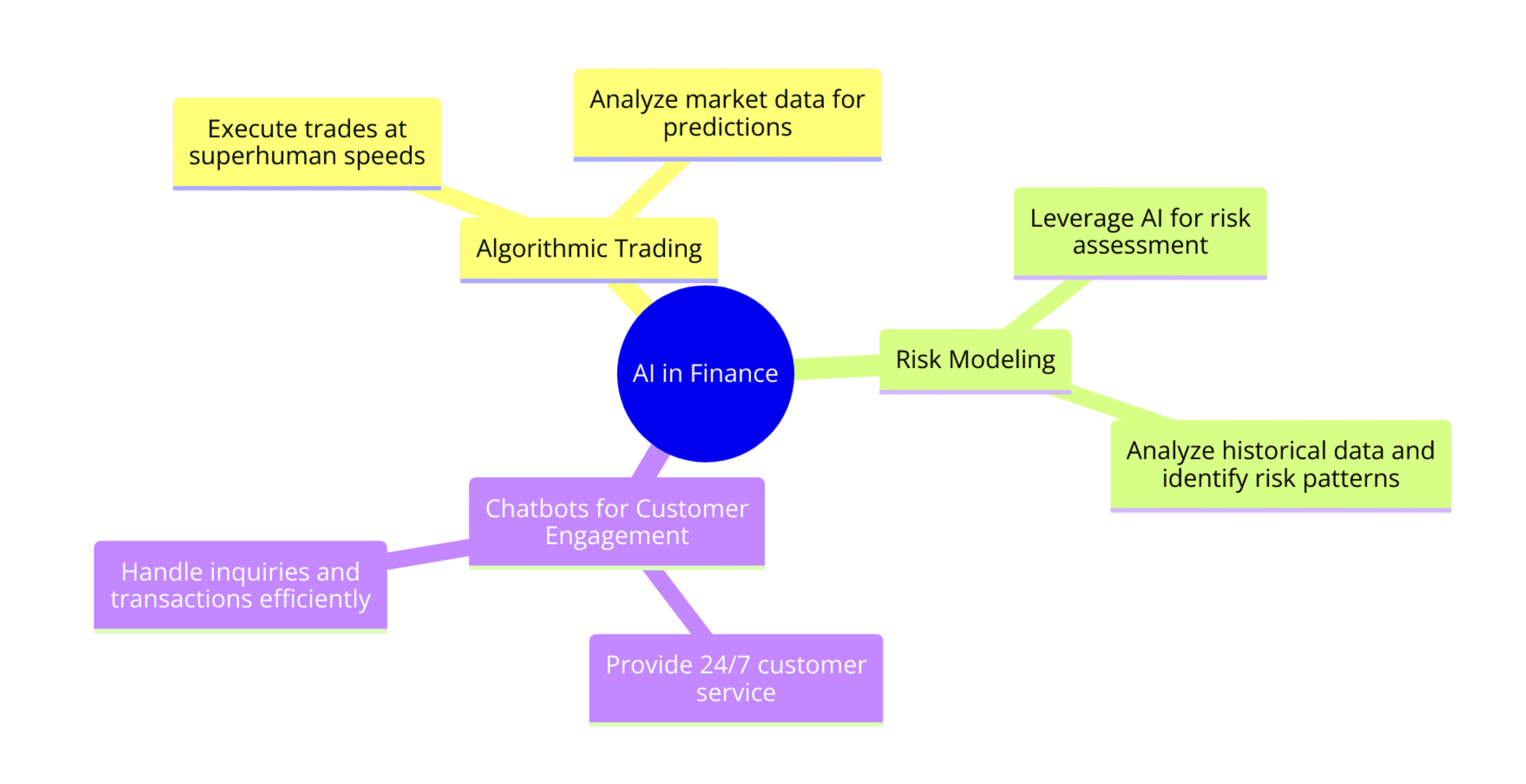
AI technologies have significantly advanced the capabilities of financial institutions in several key areas:
Algorithmic Trading: AI systems can execute trades at superhuman speeds and analyze market data to make predictions, optimizing trading strategies for better returns.
Risk Modeling: Financial institutions can more effectively predict and mitigate potential losses by leveraging AI for risk assessment. AI models can analyze historical data and identify risk patterns that might elude traditional methods.
Chatbots for Customer Engagement: AI-driven chatbots provide 24/7 customer service, handling inquiries and transactions with greater accuracy and efficiency. This not only enhances customer experience but also reduces operational costs.
AI's Role in Enhancing Operational Capacity, Productivity, and Unifying Data Silos
AI is pivotal in streamlining operations within financial institutions. By automating routine tasks, AI frees up human resources for more complex decision-making processes, enhancing overall productivity. Additionally, AI helps break down data silos within organizations by integrating disparate data sources, offering a unified view that facilitates better decision-making.
AI's ability to sift through vast amounts of data and identify patterns makes it an invaluable tool in combating financial crimes:
Anti-Money Laundering (AML): AI systems can track and analyze financial transactions to spot unusual patterns that may indicate money laundering activities, significantly improving the speed and accuracy of AML investigations.
Know-Your-Customer (KYC): AI enhances KYC processes by quickly validating customer data against multiple verification databases, ensuring compliance with regulatory requirements, and reducing the potential for fraud.
Let's explore the global regulatory landscape for AI in finance, detailing international commitments, jurisdiction-specific developments, and key examples of how various regions are addressing the challenges and opportunities presented by AI technologies.
Read more on AI Use Cases
Global Regulatory Landscape for AI in Finance
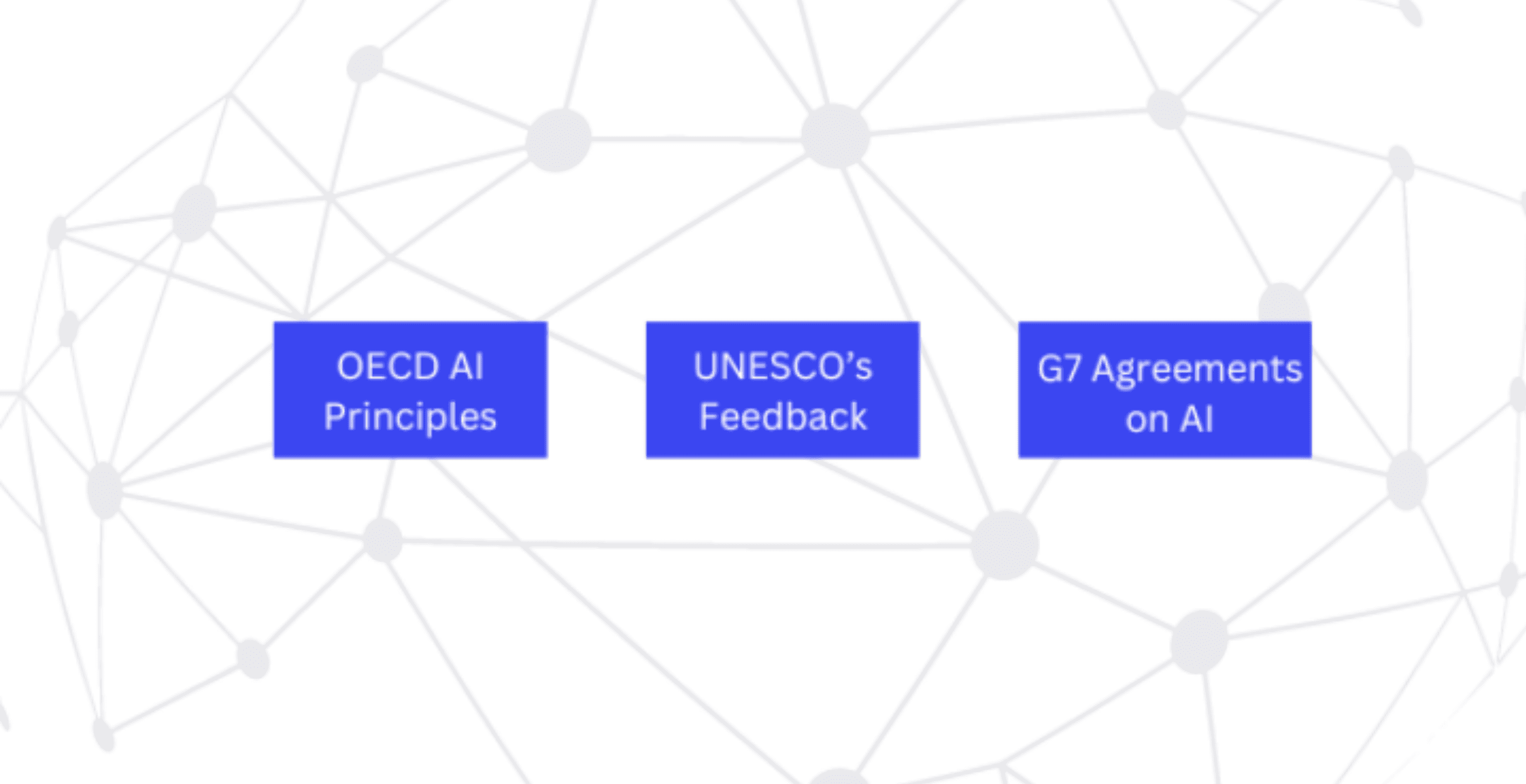
The international community has recognized the need for cohesive regulatory frameworks to guide the development and deployment of AI in finance. Key initiatives include:
OECD AI Principles: These principles focus on fostering innovation and trust in AI technologies while ensuring respect for human rights and democratic values. They emphasize AI systems' transparency, robustness, and safety, particularly in high-stakes sectors like finance.
UNESCO’s Recommendation on the Ethics of AI: This recommendation outlines standards to ensure that AI development respects ethical principles and human values, promoting data privacy and transparency.
G7 Agreements on AI: These agreements among G7 countries aim to harmonize AI regulations across borders, ensuring that AI supports economic growth and societal benefits without compromising financial stability.
Key Jurisdictional Developments in the EU, UK, and Japan
Different regions have taken unique approaches to AI regulation:
European Union (EU): The EU has been at the forefront with the proposed AI Act, which sets clear guidelines for high-risk AI applications in finance, such as ensuring algorithmic transparency and accountability.
United Kingdom (UK): The UK focuses on sector-specific guidelines and has established a sandbox environment where fintech firms can test AI technologies in a controlled regulatory space.
Japan: Japan emphasizes collaboration between the private sector and government to drive AI innovation while ensuring stringent data protection and security measures in financial services.
US State-Level AI Legislation and Federal Interests
In the United States, AI regulation is evolving at both state and federal levels:
State-Level Legislation: States like California and New York have implemented laws specifically addressing AI's use in decision-making processes, including transparency in AI-driven credit scoring systems.
Federal Interests: At the federal level, initiatives such as those from the National Institute of Standards and Technology (NIST) provide guidelines for deploying trustworthy AI systems, focusing on ethical, legal, and societal implications for AI in finance.
AI Regulation in the United States
The global landscape for AI regulation in finance is complex and dynamic, with international and regional frameworks evolving to address the rapid advancements in AI technologies. As we delve deeper into specific regions like the United States and Europe, we'll explore how these regulations.
State-level Legislation and Proposed Federal Laws
In the U.S., AI regulation varies significantly across states, reflecting diverse approaches to balancing innovation with consumer protection and ethical considerations:
State-Level Legislation: States like California have enacted laws that specifically address the transparency of AI systems used in hiring processes. These regulations require companies to disclose when AI is used in making employment decisions. They are designed to prevent biases and ensure fairness in AI applications.
Proposed Federal Laws: At the federal level, there are ongoing discussions about comprehensive AI legislation that would provide a unified framework for the use of AI across various sectors, including finance. Proposed laws focus on enhancing AI systems' transparency, accountability, and security to protect consumers and maintain trust in AI technologies.
Read more on USA AI Policies
Guidance from the NIST
The National Institute of Standards and Technology (NIST) plays a crucial role in setting standards for AI that bolster its safety, reliability, and interoperability. NIST's guidance often includes:
Frameworks for AI Testing and Validation: Ensuring that AI systems are rigorously tested and validated for their intended financial services applications.
Security Protocols for AI Systems: Developing robust security measures to protect sensitive financial data processed by AI, mitigating risks associated with data breaches and cyber-attacks.
AI Bill of Rights
The White House has proposed a Blueprint for an AI Bill of Rights, which outlines key protections that should be afforded to all Americans in the age of AI. This blueprint emphasizes:
Privacy Rights: Safeguarding personal data against misuse and ensuring individuals have control over their information.
Non-discrimination: Ensuring AI systems do not perpetuate biases or discriminatory practices, particularly in critical areas like credit scoring and lending.
Algorithmic Transparency: Requiring that the operations of AI systems be understandable to users, with clear explanations of how decisions are made, especially those impacting financial opportunities and livelihoods.
The regulatory framework in the United States aims to foster an environment where AI can thrive while ensuring it operates in a secure, transparent, and equitable manner.
As AI continues integrating into the financial sector, these regulations will play a pivotal role in shaping its development and ensuring its benefits are realized responsibly. Next, we'll explore how Europe contributes to shaping global AI regulations, particularly in financial services.
Read more on AI Regulations Worldwide
Europe's Role in Shaping AI Regulations
Let's explore Europe's significant role in shaping AI regulations, particularly focusing on the comprehensive frameworks it has established that influence global standards and practices, especially in the financial sector.
The EU AI Act and Its Implications for Financial Services
Europe has been a frontrunner in regulating AI, with the European Union's AI Act representing one of the most ambitious legislative efforts globally. This act categorizes AI applications according to risk levels, imposing stricter requirements on high-risk sectors like finance. Critical provisions for financial services include:
Risk Assessment and Mitigation: Financial institutions must conduct thorough risk assessments of their AI systems to ensure they operate safely and without bias.
Transparency Requirements: AI systems used in finance must be transparent, with clear explanations provided to customers about how their data is used, and decisions are made, especially for AI-driven credit scoring or investment advice.
Europe's influence extends beyond its borders, as it has played a critical role in shaping international AI policies:
OECD AI Principles Endorsement: The EU has been instrumental in developing and endorsing the OECD AI Principles, advocating for responsible stewardship of trustworthy AI globally.
Hiroshima AI Process: Under this initiative, Europe has pushed for a global Code of Conduct for AI, promoting ethical standards and practices that ensure AI's benefits are distributed equitably across societies.
The EU AI Act's Global Impact
The EU AI Act is pivotal for European countries and serves as a benchmark for other regions developing their AI regulations.
Its comprehensive approach is influencing how global markets, including the US and Asia, regulate AI technologies, especially in critical areas such as financial services, where the potential for harm is significant.
The act's focus on ethical considerations and consumer protections sets a high standard for what responsible AI deployment should look like.
Europe's proactive approach to AI regulation, mainly through the EU AI Act, highlights its commitment to ensuring that AI development is balanced with the need to protect consumers and maintain ethical standards. This legislative framework influences European financial markets and sets a global precedent for how AI should be regulated in sensitive sectors.
Next, we'll discuss how institutions can ensure compliance with these regulations and the recommended practices for deploying AI in finance responsibly. Ready to move on to compliance and regulatory recommendations?
Compliance and Regulatory Recommendations
Let's dive into the essential compliance and regulatory recommendations for deploying AI in the financial sector, emphasizing the core principles that ensure these systems are used responsibly and effectively.
Compliance in AI, especially within the financial sector, revolves around several key principles:
Training: AI systems should use diverse and representative data sets to avoid biases and ensure fair outcomes.
Testing: Rigorous testing must be conducted to verify the accuracy and reliability of AI models under various conditions.
Monitoring: Continuous monitoring is essential to quickly identify and address any issues that may arise during the operation of AI systems, ensuring they continue to function as intended.
Auditing: Regular audits are necessary to ensure compliance with all relevant laws and regulations and to maintain transparency and accountability in AI operations.
Inclusion of Legal Counsel in AI Policy Development
Involving legal counsel in developing AI policies is crucial to ensure that all AI implementations comply with existing legal frameworks and ethical standards. Legal experts can provide guidance on regulatory requirements, help assess potential legal risks, and ensure that AI deployments are both effective and compliant with the law.
Prioritizing Data Quality and Security in AI Model Training
Data quality and security are paramount in AI model training:
Data Quality: High-quality data is essential for training effective AI models. This means ensuring the data is accurate, comprehensive, and free of biases.
Data Security: Protecting sensitive information used in AI training is crucial to prevent data breaches and maintain consumer trust. Implementing robust data security measures, such as encryption and access controls, is essential.
Ensuring Thorough Documentation for Auditability of AI Processes
Thorough documentation of AI processes is vital for auditability and compliance. Documentation should include details on the design and development of AI systems, data handling practices, decision-making processes, and any incidents or modifications. This transparency helps regulators and stakeholders understand how AI systems work and assess their compliance with regulatory standards.
These compliance and regulatory recommendations are designed to help financial institutions implement AI responsibly, ensuring that they not only reap the benefits of AI but also maintain the trust and confidence of their customers and regulators. Next, we'll discuss the specific risks associated with AI in financial institutions and how appropriate regulations can help mitigate these risks.
AI Risks and Financial Institution Accountability
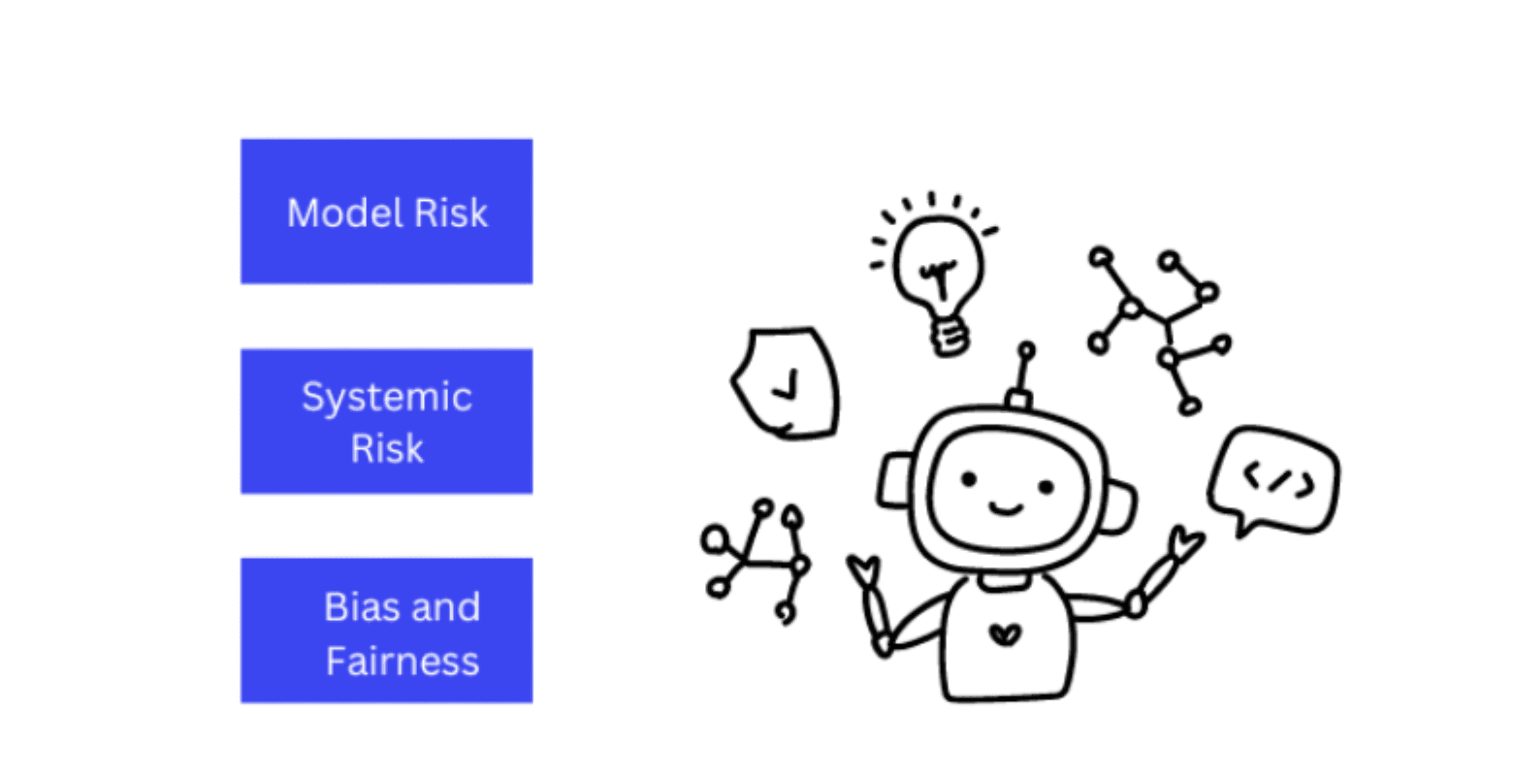
Understanding and mitigating the risks associated with AI in financial institutions is critical for ensuring that these technologies are used responsibly and effectively. As we move forward, we will discuss how financial institutions can prepare for the future of AI, including expected developments in regulations and technologies.
AI technologies, while offering significant benefits in terms of efficiency and decision-making, also pose unique risks that can amplify existing challenges within financial institutions. These risks include:
Model Risk: The risk that AI models may not perform as expected under different market conditions or may fail to adequately handle edge cases.
Systemic Risk: The potential for AI-driven decisions to lead to widespread issues within the financial system, especially if similar AI strategies are adopted across multiple institutions.
Bias and Fairness: AI systems can inadvertently perpetuate or even exacerbate biases present in the training data, leading to unfair outcomes for certain groups of customers.
To effectively manage these risks, robust regulatory frameworks are essential. These frameworks should focus on:
Regulation: Establishing clear guidelines for the development, deployment, and operation of AI systems within financial institutions to ensure they are safe and fair.
Supervision: Regular monitoring by regulatory bodies to ensure that financial institutions comply with AI governance standards and that their AI systems do not pose undue risks to the financial system.
Accountability: Implementing mechanisms that hold institutions accountable for the decisions made by their AI systems, ensuring that there is always a clear line of responsibility.
Read more on EU AI Act Insights
Addressing AI Risks in Practice
Implementing practical measures to manage AI risks involves:
Risk Assessment Protocols: Financial institutions must regularly assess the risks associated with their AI applications, considering factors like data integrity, model robustness, and potential impacts on customers.
Ethics and Compliance Committees: Establishing dedicated committees to oversee AI ethics and compliance can help ensure that AI applications adhere to both internal and external ethical standards.
Transparent Reporting: Institutions should be transparent about their use of AI, including the risks involved and the measures taken to mitigate these risks. This transparency is crucial for maintaining stakeholder trust.
Read more on AI Governance Strategies
Facing the Future of AI in Finance
The landscape of AI regulation in finance is rapidly evolving to keep pace with technological advancements. As AI systems become more complex and integral to financial operations, regulators worldwide are working to update and refine legal frameworks to ensure these technologies are used safely and ethically.
This includes establishing clearer guidelines for accountability, transparency, and data protection in AI applications.
Looking ahead, several key regulatory developments are expected to significantly impact the financial sector:
EU AI Act: This comprehensive legislative framework is set to establish risk-based classifications for AI systems, mandating stringent compliance requirements for high-risk applications in finance.
SEC Proposals: In the United States, the Securities and Exchange Commission (SEC) is proposing new regulations aimed at enhancing transparency in AI-driven investment strategies and financial products, ensuring that investors are adequately informed about the AI methodologies that influence their investments.
Global Harmonization Efforts: There is an increasing call for international cooperation to harmonize AI regulations, which would facilitate smoother cross-border operations of multinational financial institutions and prevent regulatory arbitrage.
The Call for a Harmonized Approach to AI Regulation Internationally
The need for a harmonized international approach to AI regulation in finance cannot be overstated. As financial markets are inherently global, disparate regulatory environments can lead to inconsistencies that complicate compliance for international entities and potentially hinder innovation.
A coordinated effort to establish common standards and practices can help ensure that AI technologies are developed and used in ways that benefit the global community while safeguarding against systemic risks.
Ready to transform your approach to AI with cutting-edge insights and tools? Explore RagaAI's comprehensive suite of AI solutions — from enhancing AI reliability with our guardrails to navigating the complexities of AI governance.
Whether diving into prompt engineering, seeking to mitigate AI biases, or exploring the frontier of AI testing, RagaAI is your partner in pioneering a safer, more efficient AI-driven future. Discover more and join us in shaping the next wave of AI innovation. Let's embark on this journey together with RagaAI.
Subscribe to our newsletter to never miss an update
Other articles


Agents Talk, Models Think: A2A + MCP for Enterprise Agentic AI

Riya Parikh, Nitai Agarwal
Apr 27, 2025
Read the article
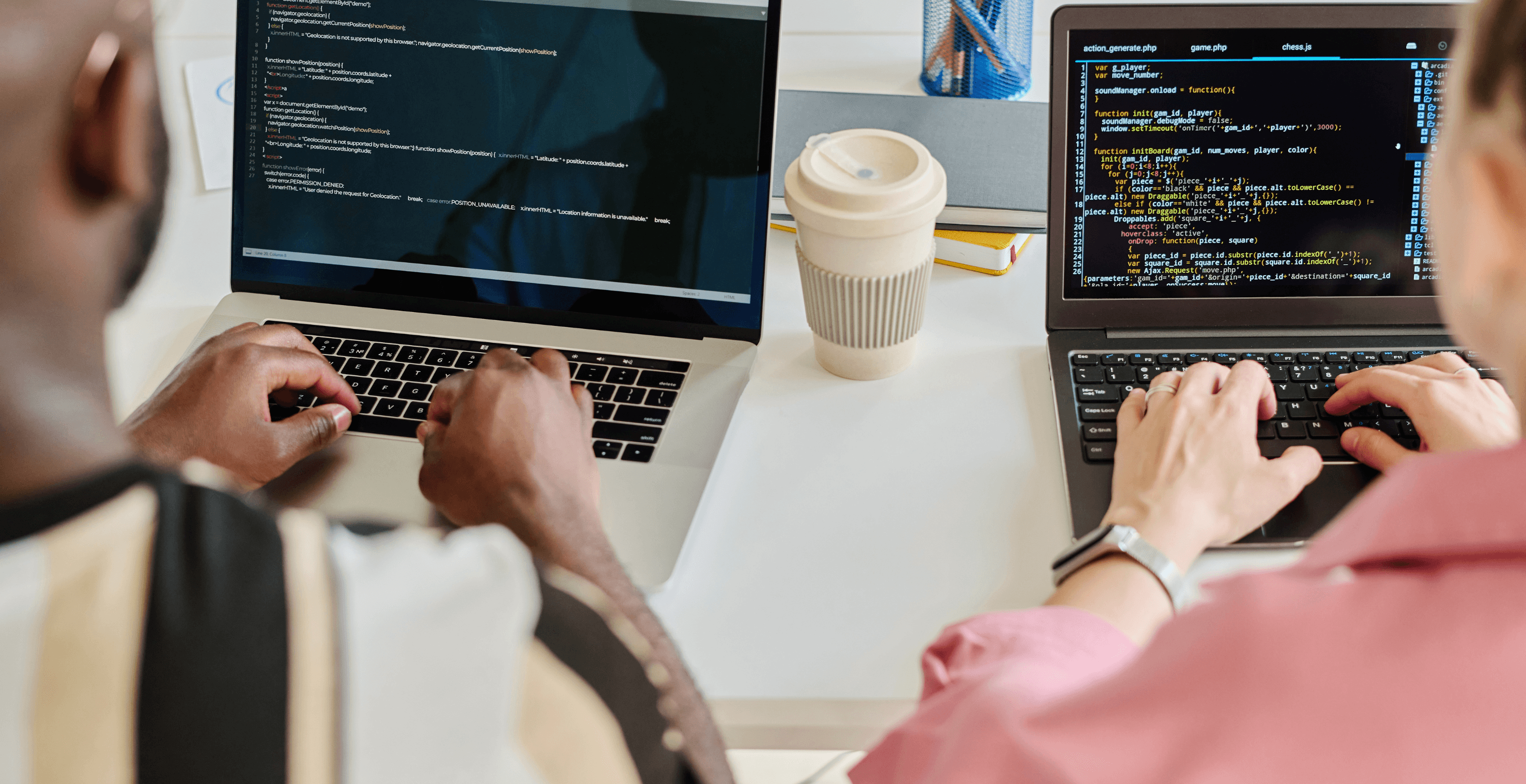
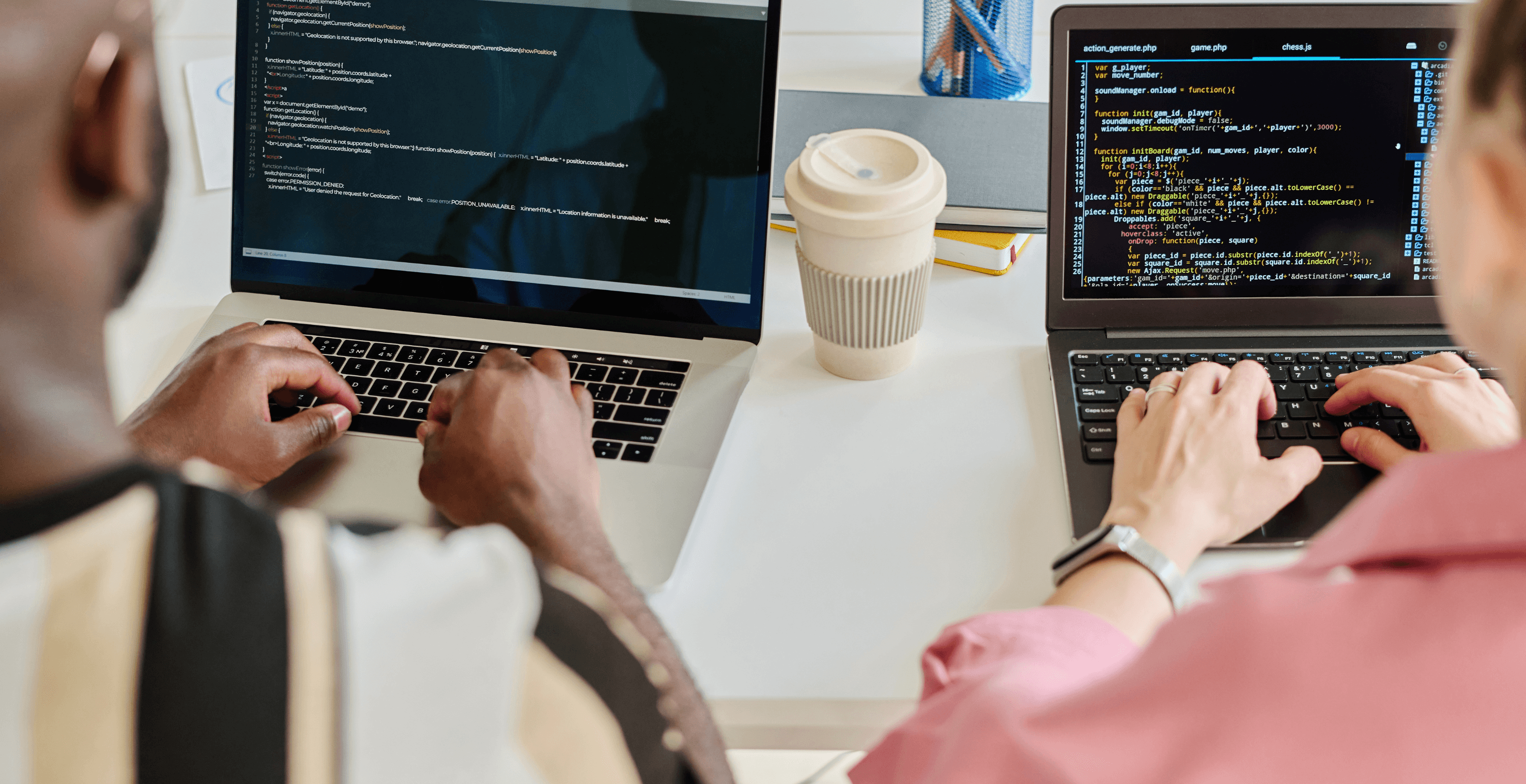
Understanding NeMo Guardrails: A Toolkit for LLM Security

Rehan Asif
Dec 24, 2024
Read the article


Understanding Differences in Large vs Small Language Models (LLM vs SLM)

Rehan Asif
Dec 21, 2024
Read the article