The Impact of AI Governance on Innovation and Development Speed
The Impact of AI Governance on Innovation and Development Speed
The Impact of AI Governance on Innovation and Development Speed
Rehan Asif
Apr 26, 2024
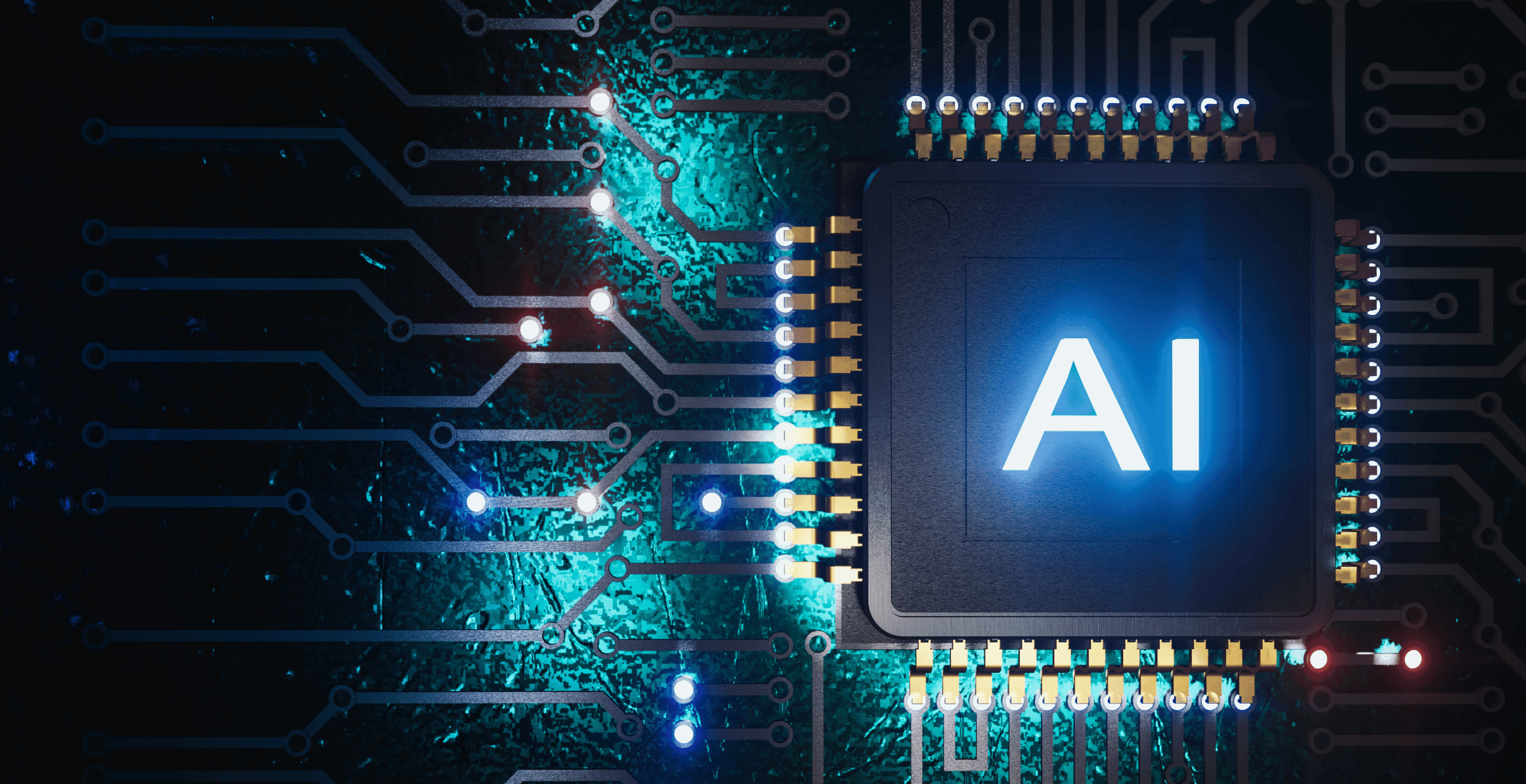
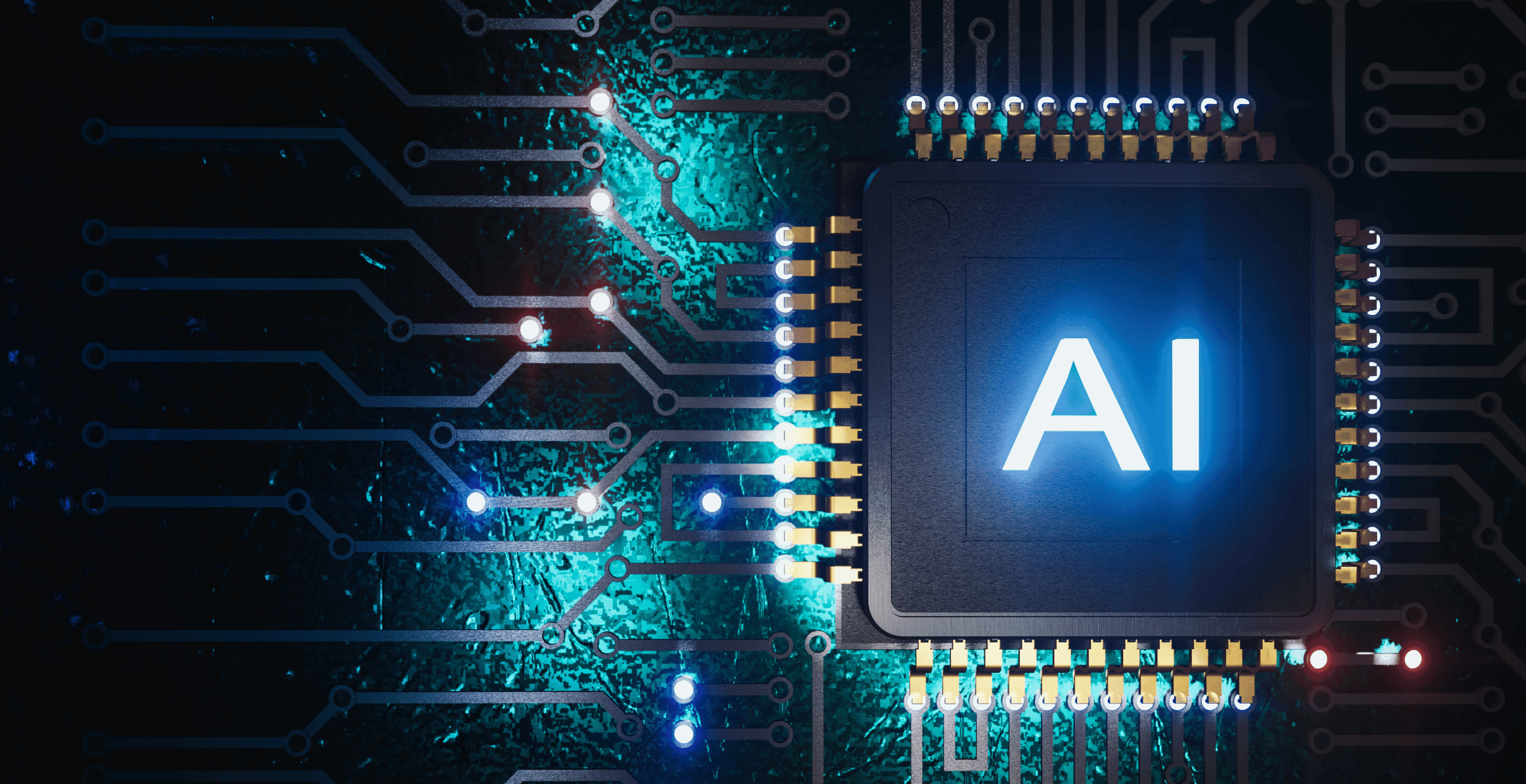
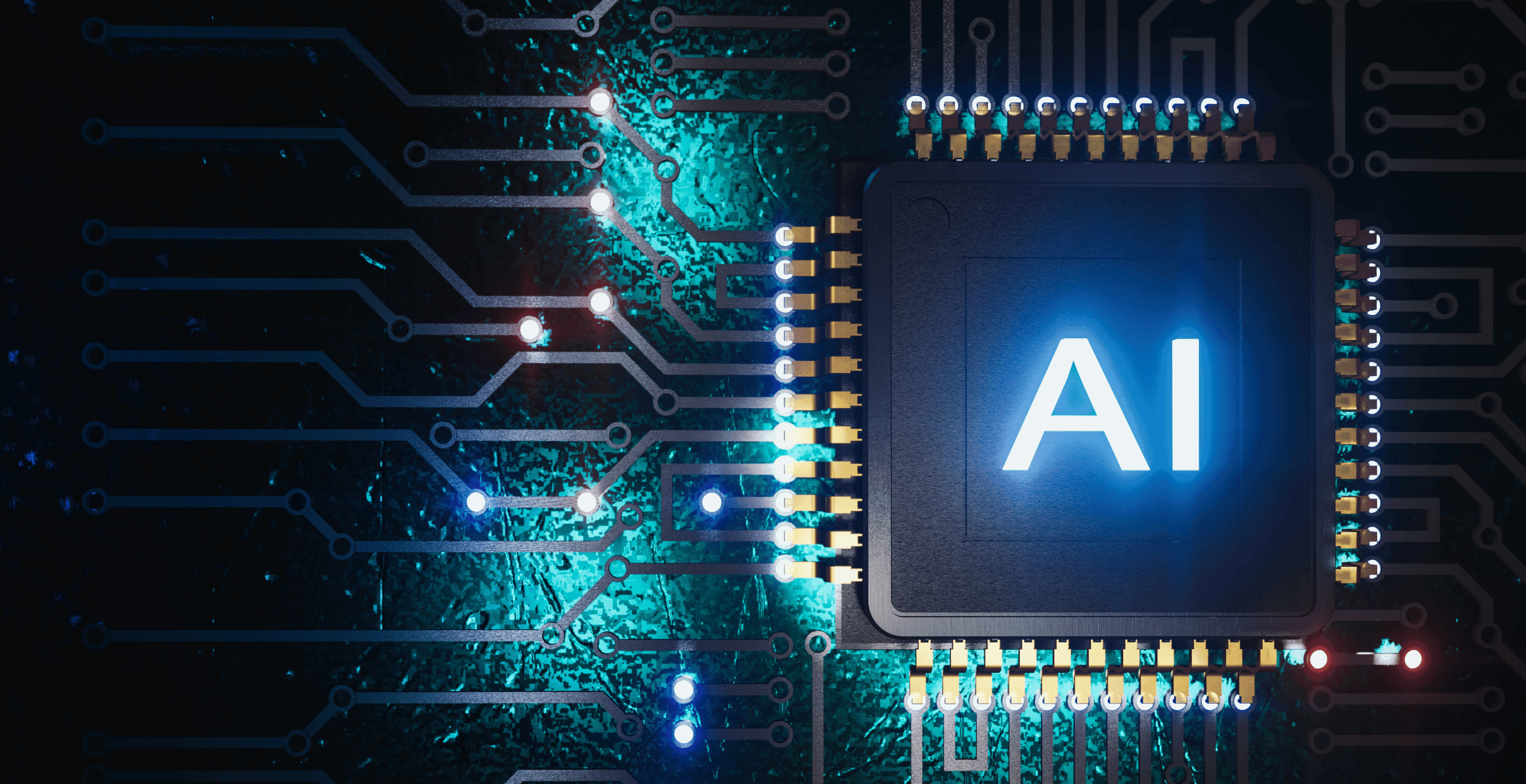
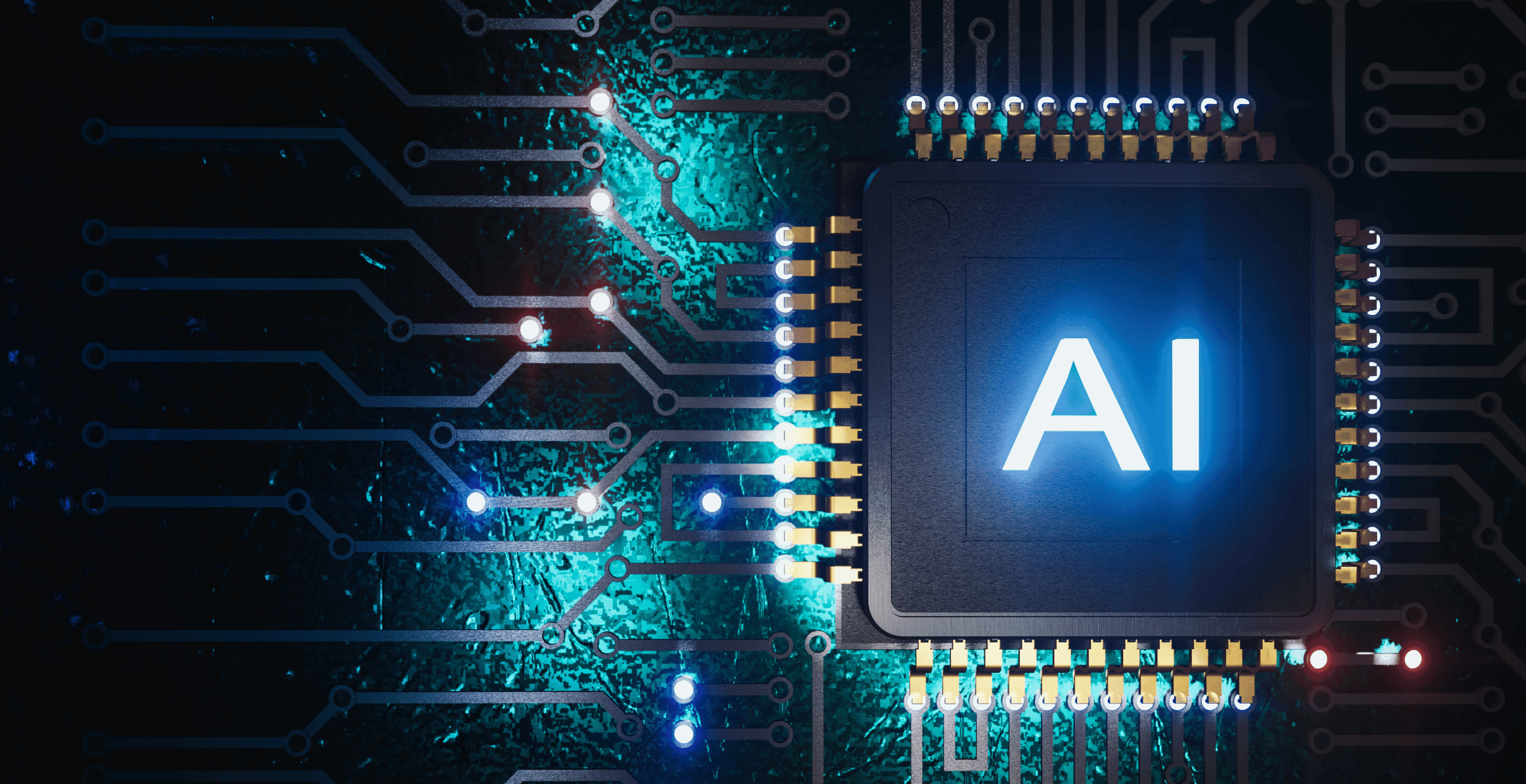
Ever tried to juggle fire while coding? That's child's play compared to balancing AI innovation and governance!
As we navigate through the span of digital evolution, the role of AI governance on innovation and development has become gradually important.
Setting regulations and structures that guide the ethical formation, positioning, and handling of Artificial Intelligence technologies indulges AI governance. This governance is significant not just for ensuring the secured utilization of AI but also for nurturing an environment where inventiveness can succeed without ethical concessions.
Comprehending the balance between communal inventiveness and significant regulation is important. Too little regulation could result in ethical problems and risks, while too much could strangle innovation and drop down technological development.
The Significance of AI Governance in Advancing Innovation
AI technology is transforming how we live and operate, but its quick progress brings quirky challenges. Efficient AI governance is critical in utilizing AI’s prospective responsibly while alleviating threats. Here’s why it matters:-
Guiding AI Development and Deployment Responsibly
As technologies advance, liable AI governance ensures they do so with deliberation for safety and impartiality. Strict instructions and standards are established to help avert misutilization and ensure AI systems work transparently and predictably.
This is crucial not just for ethical reasons but also to preserve public trust in how these technologies are enforced.
Emphasizing Ethical, Legal, and Societal Considerations in AI Use
AI systems can have deep impacts on society, influencing everything from the professional markets to privacy rights. Governance structures must acknowledge these impacts, integrating ethical standards and legitimate needs into the fabric of AI evolution.
Not only does this technique safeguard individuals, but it also helps firms navigate the intricate regulatory landscapes occurring around AI technology.
Promoting Innovation through Clear Governance Frameworks
Transparent, well-defined governance structures are generally believed to foster innovation by providing clear guidelines, although the extent of their positive impact can vary by sector. This lucidity decreases assurance and risk, promising investment and research in new AI applications. Furthermore, governance can promote partnership between investors, indulging policymakers, technologists, and the public, ensuring that AI evolution corresponds with wider social purposes and values.
AI governance isn’t just about supervision; its about ensuring that AI technology develops in a way that aids everyone. By categorizing responsible development, ethical contemplations, and transparent regulatory structures, we can foster a future where AI contributes firmly and legitimately to society.
Challenges Posed by AI Governance to Innovation and Development Speed
Acknowledging the challenges posed by AI governance to inventiveness and development speed is crucial as we continue to incorporate AI technologies into numerous sectors. Here is a closer look at they three major key challenges:
Complex Nature of AI Systems Complicating Governance
AI systems encompass intricate layers of computations and data processing that require expert knowledge to design, interpret, and manage effectively. The intricacy of AI systems challenges the creation of both efficient and understandable governance. Governance structures, therefore, need to concentrate not only on the results but also on the AI progression procedures, integrating clarity and accountability measures.
Balancing Rapid Innovation with the Need for Regulation
The rapid speed of AI development depicts a crucial challenge for regulation. There's a need to carefully craft regulations to promote safe innovation without unnecessarily hindering creative exploration. On the other hand, the potent threats related to AI, like ethical concerns and safety problems, need cautious oversight. Creating adjustable regulatory structures that provide instructions and oversight without restricting creative investigation and technological progression can accomplish this balance.
Difficulties in Updating Regulatory Frameworks to Keep Pace with AI Advancements
AI technology progresses at a much faster rate than the standard legislative procedure can contain. This lag can result in gaps in regulations and where new technologies work without apt instructions, possibly resulting in unethical practices or misutilization.
To acknowledge this, regulators could enforce an ordered approach to AI governance, where instant, flexible and able to update strategies are put in place to handle risks as they derive, alongside more constant, long-term regulations concentrated on wide, crucial concerns.
Each of these challenges needs an attentive approach that indulges investors from across the range, including AI developers, governmental bodies, principles, and the public, to ensure that AI governance facilitates inventiveness while safeguarding society from potent harms.
The Role of Data and Models in AI Governance
The governance of AI is an adaptable and intricate field, touching on the morals, security and efficiency of AI systems. At the heart of this governance are 2 crucial factors: data and models. Their handling and regulation play major roles in shaping the evolution and positioning of AI technologies. Let’s deep dive into knowing these factors:-
Impact of Data Standards on AI Safety and Governance

Data standards are important for ensuring AI systems are secure and efficient. They define the standard, framework and format of data utilized in AI development, ensuring it can be precisely refined and examined by AI models. Standardizing data helps in numerous ways:
Improving Data Quality: Consonant data standards help maintain the precision, entirety and dependability of the data fed into Artificial Intelligence systems. This, in turn, accelerates the performance and safety of Artificial Intelligence applications.
Facilitating Compatibility: With systematic data, distinct AI systems can interact more smoothly, sharing and using data without interoperability problems. This is especially significant in fields such as healthcare and autonomous driving, where data from numerous sources must be incorporated.
Regulating AI Development: Standards offer a structure for regulators to assure that AI systems meet certain thresholds for security and effectiveness before they are positioned.
Debate Over Open-Source vs. Closed Models in AI Development
The selection between Open-source and Closed models is a crucial debate in AI governance. Each approach has its advantages and disadvantages:-
Open-Sourced Models: These are attainable to the public and can be altered by anyone. This honesty promotes clarity, nurtures inventiveness, and expedites growth as investigators and developers can build on each other’s work. However, it also elevates solicitudes about security, as vicious users could leverage susceptibilities in these models.
Closed Models: These are exclusive and controlled by their developers. This can ensure higher levels of security and control over the utilization of the AI systems. However, the shortage of lucidity may hinder trust and liability in AI systems.
Compensating these models indulges considering the specific requirements of an industry, the perceptivity of the application, and potent threats indulged.
Ensuring Data is Representative, Unbiased, and Ethically Sourced
The incorporation of data utilized in AI systems is crucial for ensuring fair and impartial results. Misrepresentative or biased data can result in AI models that conserve inequalities or make inaccurate decisions. Ensuring data incorporation involves:
Diversity and Representation: Datasets must surround varied demographics to prevent partiality in AI results. This is significant in applications such as facial recognition and auguring policing, where partial data can result in unfair practices.
Ethical Sourcing: Data must be gathered in a manner that respects privacy and consent. Ethical sourcing also involves clarity about how data is utilized and ensuring it doesn’t leverage susceptible populations.
Ongoing Monitoring and Auditing: AI systems should be frequently monitored for partiality and errors, and datasets should be updated to reflect new details and amusing changes. This helps maintain the significance and fairness of AI systems.
The role of data and models in AI governance is critical. Establishing strict standards, choosing on the acceptance of AI models, and ensuring data incorporation are important steps towards liable AI development and positioning.
These measures not only improves the security and ability of AI systems but also promotes public trust and confidence in this evolutionary technology.
Strategies for Enhancing AI Governance to Support Innovation
Establishing Adaptive Regulatory Frameworks
Regulations need to be adaptable to keep up with the speed of AI evolution. This adaptability can be achieved through structures that adapt based on technological advancements and communal impact.
Encouraging International Policy Harmonization
AI doesn’t identify national borders, making international partnership on policy progression important. Integrated policies can help create a universally compatible regulatory environment that supports international inventiveness.
Fostering Public-Private Partnerships for Effective Governance
Partnerships between the public and private sectors can improve the efficacy of AI governance. These collaborations can support the strengths of both sectors to create structures that are inventive and robust.
Enhancing Understanding of AI among Policymakers and the Public
Education and awareness among legislators and the general public are critical for the efficient governance of AI. AI better comprehension of AI technologies can result in more informed decisions about their regulation and utilization.
Goals and Components of Successful AI Governance Implementation
Ensuring Legal and Regulatory Compliance
AI systems must function within legitimate frameworks that safeguards users and society at large. Adhering to these laws is not just about obedience but also about gaining public trust.
Protecting against Reputational Damage and Promoting Ethical AI Use
Associations must contemplate the estimated risks related with AI mismanagement. By fostering ethical use of AI, companies can avoid potent disgrace and maintain their trustworthiness.
Developing Clear Strategies, Guidelines, and Processes for AI Governance
For AI governance to be efficient, clear strategies, instructions and the processes need to be pioneered. These structures should correspond AI inventiveness with business goals and societal estimates, ensuring a symmetric approach to technology evolution.
Aligning Business Objectives with Societal Values
AI should not just serve venture ends but also reflects societal values and ethics. This layout ensures that the technology aids all segments of the society without causing damage and disparity.
The Future of AI Governance and Its Impact on Innovation
Looking ahead, the governance of AI will need to be as energetic as the technologies it intends to control. Crucial roles will be played by adjustable regulatory approaches, international policy harmonization, and improved public awareness in tailoring how AI evolves.
These components will ensure that AI governance supports rather than obstructs inventiveness, surfacing the way for ascents that are not only technologically sound but also ethically liable.
Conclusion
Effective AI governance is crucial for harmonizing innovation with ethical standards, regulatory compliance, and social responsibility.
As we continue to inspect the possibilities of AI, ongoing dialogue, research and partnership in developing governance models will be critical. By ensuring a solid governance structure, we can nurture an environment where innovation flourishes while safeguarding and improving societal values.
FAQs
What is the impact of Artificial Intelligence on Innovation?
Artificial Intelligence substantially accelerates innovation across numerous industries by automating intricate processes, improving data analytics, and nurturing creative solutions.
AI empowers the evolution of new products and services by inspecting vast amounts of data to locate trends and insights that would be unlikely for humans to locate swiftly. In healthcare, AI innovations can result in progressed distinctive tools and customized medicine. In automotive industries, AI propels the evolution of self-driving technology.
Furthermore, AI helps in solving intricate issues in sectors such as environmental conservations and finance, making procedures more effective and budget-friendly.
How does AI impact governance?
AI impacts governance by enhancing the efficacy and potency of public services, improving decision-making processes, and ensuring better resource handling.
Governments utilize AI to dissect data from numerous sources, helping to smooth operations, forecasting public service needs, and improve citizen affiance through more receptive services.
AI tools also help in observing infrastructure projects, handling traffic systems, and accelerating law execution activities. Hence, it also presents difficulties like ensuring privacy, security and ethical standards are handled. Therefore, effective governance of Artificial Intelligence itself becomes critical to acknowledge these problems, ensuring that its execution aids society as a whole.
Ever tried to juggle fire while coding? That's child's play compared to balancing AI innovation and governance!
As we navigate through the span of digital evolution, the role of AI governance on innovation and development has become gradually important.
Setting regulations and structures that guide the ethical formation, positioning, and handling of Artificial Intelligence technologies indulges AI governance. This governance is significant not just for ensuring the secured utilization of AI but also for nurturing an environment where inventiveness can succeed without ethical concessions.
Comprehending the balance between communal inventiveness and significant regulation is important. Too little regulation could result in ethical problems and risks, while too much could strangle innovation and drop down technological development.
The Significance of AI Governance in Advancing Innovation
AI technology is transforming how we live and operate, but its quick progress brings quirky challenges. Efficient AI governance is critical in utilizing AI’s prospective responsibly while alleviating threats. Here’s why it matters:-
Guiding AI Development and Deployment Responsibly
As technologies advance, liable AI governance ensures they do so with deliberation for safety and impartiality. Strict instructions and standards are established to help avert misutilization and ensure AI systems work transparently and predictably.
This is crucial not just for ethical reasons but also to preserve public trust in how these technologies are enforced.
Emphasizing Ethical, Legal, and Societal Considerations in AI Use
AI systems can have deep impacts on society, influencing everything from the professional markets to privacy rights. Governance structures must acknowledge these impacts, integrating ethical standards and legitimate needs into the fabric of AI evolution.
Not only does this technique safeguard individuals, but it also helps firms navigate the intricate regulatory landscapes occurring around AI technology.
Promoting Innovation through Clear Governance Frameworks
Transparent, well-defined governance structures are generally believed to foster innovation by providing clear guidelines, although the extent of their positive impact can vary by sector. This lucidity decreases assurance and risk, promising investment and research in new AI applications. Furthermore, governance can promote partnership between investors, indulging policymakers, technologists, and the public, ensuring that AI evolution corresponds with wider social purposes and values.
AI governance isn’t just about supervision; its about ensuring that AI technology develops in a way that aids everyone. By categorizing responsible development, ethical contemplations, and transparent regulatory structures, we can foster a future where AI contributes firmly and legitimately to society.
Challenges Posed by AI Governance to Innovation and Development Speed
Acknowledging the challenges posed by AI governance to inventiveness and development speed is crucial as we continue to incorporate AI technologies into numerous sectors. Here is a closer look at they three major key challenges:
Complex Nature of AI Systems Complicating Governance
AI systems encompass intricate layers of computations and data processing that require expert knowledge to design, interpret, and manage effectively. The intricacy of AI systems challenges the creation of both efficient and understandable governance. Governance structures, therefore, need to concentrate not only on the results but also on the AI progression procedures, integrating clarity and accountability measures.
Balancing Rapid Innovation with the Need for Regulation
The rapid speed of AI development depicts a crucial challenge for regulation. There's a need to carefully craft regulations to promote safe innovation without unnecessarily hindering creative exploration. On the other hand, the potent threats related to AI, like ethical concerns and safety problems, need cautious oversight. Creating adjustable regulatory structures that provide instructions and oversight without restricting creative investigation and technological progression can accomplish this balance.
Difficulties in Updating Regulatory Frameworks to Keep Pace with AI Advancements
AI technology progresses at a much faster rate than the standard legislative procedure can contain. This lag can result in gaps in regulations and where new technologies work without apt instructions, possibly resulting in unethical practices or misutilization.
To acknowledge this, regulators could enforce an ordered approach to AI governance, where instant, flexible and able to update strategies are put in place to handle risks as they derive, alongside more constant, long-term regulations concentrated on wide, crucial concerns.
Each of these challenges needs an attentive approach that indulges investors from across the range, including AI developers, governmental bodies, principles, and the public, to ensure that AI governance facilitates inventiveness while safeguarding society from potent harms.
The Role of Data and Models in AI Governance
The governance of AI is an adaptable and intricate field, touching on the morals, security and efficiency of AI systems. At the heart of this governance are 2 crucial factors: data and models. Their handling and regulation play major roles in shaping the evolution and positioning of AI technologies. Let’s deep dive into knowing these factors:-
Impact of Data Standards on AI Safety and Governance

Data standards are important for ensuring AI systems are secure and efficient. They define the standard, framework and format of data utilized in AI development, ensuring it can be precisely refined and examined by AI models. Standardizing data helps in numerous ways:
Improving Data Quality: Consonant data standards help maintain the precision, entirety and dependability of the data fed into Artificial Intelligence systems. This, in turn, accelerates the performance and safety of Artificial Intelligence applications.
Facilitating Compatibility: With systematic data, distinct AI systems can interact more smoothly, sharing and using data without interoperability problems. This is especially significant in fields such as healthcare and autonomous driving, where data from numerous sources must be incorporated.
Regulating AI Development: Standards offer a structure for regulators to assure that AI systems meet certain thresholds for security and effectiveness before they are positioned.
Debate Over Open-Source vs. Closed Models in AI Development
The selection between Open-source and Closed models is a crucial debate in AI governance. Each approach has its advantages and disadvantages:-
Open-Sourced Models: These are attainable to the public and can be altered by anyone. This honesty promotes clarity, nurtures inventiveness, and expedites growth as investigators and developers can build on each other’s work. However, it also elevates solicitudes about security, as vicious users could leverage susceptibilities in these models.
Closed Models: These are exclusive and controlled by their developers. This can ensure higher levels of security and control over the utilization of the AI systems. However, the shortage of lucidity may hinder trust and liability in AI systems.
Compensating these models indulges considering the specific requirements of an industry, the perceptivity of the application, and potent threats indulged.
Ensuring Data is Representative, Unbiased, and Ethically Sourced
The incorporation of data utilized in AI systems is crucial for ensuring fair and impartial results. Misrepresentative or biased data can result in AI models that conserve inequalities or make inaccurate decisions. Ensuring data incorporation involves:
Diversity and Representation: Datasets must surround varied demographics to prevent partiality in AI results. This is significant in applications such as facial recognition and auguring policing, where partial data can result in unfair practices.
Ethical Sourcing: Data must be gathered in a manner that respects privacy and consent. Ethical sourcing also involves clarity about how data is utilized and ensuring it doesn’t leverage susceptible populations.
Ongoing Monitoring and Auditing: AI systems should be frequently monitored for partiality and errors, and datasets should be updated to reflect new details and amusing changes. This helps maintain the significance and fairness of AI systems.
The role of data and models in AI governance is critical. Establishing strict standards, choosing on the acceptance of AI models, and ensuring data incorporation are important steps towards liable AI development and positioning.
These measures not only improves the security and ability of AI systems but also promotes public trust and confidence in this evolutionary technology.
Strategies for Enhancing AI Governance to Support Innovation
Establishing Adaptive Regulatory Frameworks
Regulations need to be adaptable to keep up with the speed of AI evolution. This adaptability can be achieved through structures that adapt based on technological advancements and communal impact.
Encouraging International Policy Harmonization
AI doesn’t identify national borders, making international partnership on policy progression important. Integrated policies can help create a universally compatible regulatory environment that supports international inventiveness.
Fostering Public-Private Partnerships for Effective Governance
Partnerships between the public and private sectors can improve the efficacy of AI governance. These collaborations can support the strengths of both sectors to create structures that are inventive and robust.
Enhancing Understanding of AI among Policymakers and the Public
Education and awareness among legislators and the general public are critical for the efficient governance of AI. AI better comprehension of AI technologies can result in more informed decisions about their regulation and utilization.
Goals and Components of Successful AI Governance Implementation
Ensuring Legal and Regulatory Compliance
AI systems must function within legitimate frameworks that safeguards users and society at large. Adhering to these laws is not just about obedience but also about gaining public trust.
Protecting against Reputational Damage and Promoting Ethical AI Use
Associations must contemplate the estimated risks related with AI mismanagement. By fostering ethical use of AI, companies can avoid potent disgrace and maintain their trustworthiness.
Developing Clear Strategies, Guidelines, and Processes for AI Governance
For AI governance to be efficient, clear strategies, instructions and the processes need to be pioneered. These structures should correspond AI inventiveness with business goals and societal estimates, ensuring a symmetric approach to technology evolution.
Aligning Business Objectives with Societal Values
AI should not just serve venture ends but also reflects societal values and ethics. This layout ensures that the technology aids all segments of the society without causing damage and disparity.
The Future of AI Governance and Its Impact on Innovation
Looking ahead, the governance of AI will need to be as energetic as the technologies it intends to control. Crucial roles will be played by adjustable regulatory approaches, international policy harmonization, and improved public awareness in tailoring how AI evolves.
These components will ensure that AI governance supports rather than obstructs inventiveness, surfacing the way for ascents that are not only technologically sound but also ethically liable.
Conclusion
Effective AI governance is crucial for harmonizing innovation with ethical standards, regulatory compliance, and social responsibility.
As we continue to inspect the possibilities of AI, ongoing dialogue, research and partnership in developing governance models will be critical. By ensuring a solid governance structure, we can nurture an environment where innovation flourishes while safeguarding and improving societal values.
FAQs
What is the impact of Artificial Intelligence on Innovation?
Artificial Intelligence substantially accelerates innovation across numerous industries by automating intricate processes, improving data analytics, and nurturing creative solutions.
AI empowers the evolution of new products and services by inspecting vast amounts of data to locate trends and insights that would be unlikely for humans to locate swiftly. In healthcare, AI innovations can result in progressed distinctive tools and customized medicine. In automotive industries, AI propels the evolution of self-driving technology.
Furthermore, AI helps in solving intricate issues in sectors such as environmental conservations and finance, making procedures more effective and budget-friendly.
How does AI impact governance?
AI impacts governance by enhancing the efficacy and potency of public services, improving decision-making processes, and ensuring better resource handling.
Governments utilize AI to dissect data from numerous sources, helping to smooth operations, forecasting public service needs, and improve citizen affiance through more receptive services.
AI tools also help in observing infrastructure projects, handling traffic systems, and accelerating law execution activities. Hence, it also presents difficulties like ensuring privacy, security and ethical standards are handled. Therefore, effective governance of Artificial Intelligence itself becomes critical to acknowledge these problems, ensuring that its execution aids society as a whole.
Ever tried to juggle fire while coding? That's child's play compared to balancing AI innovation and governance!
As we navigate through the span of digital evolution, the role of AI governance on innovation and development has become gradually important.
Setting regulations and structures that guide the ethical formation, positioning, and handling of Artificial Intelligence technologies indulges AI governance. This governance is significant not just for ensuring the secured utilization of AI but also for nurturing an environment where inventiveness can succeed without ethical concessions.
Comprehending the balance between communal inventiveness and significant regulation is important. Too little regulation could result in ethical problems and risks, while too much could strangle innovation and drop down technological development.
The Significance of AI Governance in Advancing Innovation
AI technology is transforming how we live and operate, but its quick progress brings quirky challenges. Efficient AI governance is critical in utilizing AI’s prospective responsibly while alleviating threats. Here’s why it matters:-
Guiding AI Development and Deployment Responsibly
As technologies advance, liable AI governance ensures they do so with deliberation for safety and impartiality. Strict instructions and standards are established to help avert misutilization and ensure AI systems work transparently and predictably.
This is crucial not just for ethical reasons but also to preserve public trust in how these technologies are enforced.
Emphasizing Ethical, Legal, and Societal Considerations in AI Use
AI systems can have deep impacts on society, influencing everything from the professional markets to privacy rights. Governance structures must acknowledge these impacts, integrating ethical standards and legitimate needs into the fabric of AI evolution.
Not only does this technique safeguard individuals, but it also helps firms navigate the intricate regulatory landscapes occurring around AI technology.
Promoting Innovation through Clear Governance Frameworks
Transparent, well-defined governance structures are generally believed to foster innovation by providing clear guidelines, although the extent of their positive impact can vary by sector. This lucidity decreases assurance and risk, promising investment and research in new AI applications. Furthermore, governance can promote partnership between investors, indulging policymakers, technologists, and the public, ensuring that AI evolution corresponds with wider social purposes and values.
AI governance isn’t just about supervision; its about ensuring that AI technology develops in a way that aids everyone. By categorizing responsible development, ethical contemplations, and transparent regulatory structures, we can foster a future where AI contributes firmly and legitimately to society.
Challenges Posed by AI Governance to Innovation and Development Speed
Acknowledging the challenges posed by AI governance to inventiveness and development speed is crucial as we continue to incorporate AI technologies into numerous sectors. Here is a closer look at they three major key challenges:
Complex Nature of AI Systems Complicating Governance
AI systems encompass intricate layers of computations and data processing that require expert knowledge to design, interpret, and manage effectively. The intricacy of AI systems challenges the creation of both efficient and understandable governance. Governance structures, therefore, need to concentrate not only on the results but also on the AI progression procedures, integrating clarity and accountability measures.
Balancing Rapid Innovation with the Need for Regulation
The rapid speed of AI development depicts a crucial challenge for regulation. There's a need to carefully craft regulations to promote safe innovation without unnecessarily hindering creative exploration. On the other hand, the potent threats related to AI, like ethical concerns and safety problems, need cautious oversight. Creating adjustable regulatory structures that provide instructions and oversight without restricting creative investigation and technological progression can accomplish this balance.
Difficulties in Updating Regulatory Frameworks to Keep Pace with AI Advancements
AI technology progresses at a much faster rate than the standard legislative procedure can contain. This lag can result in gaps in regulations and where new technologies work without apt instructions, possibly resulting in unethical practices or misutilization.
To acknowledge this, regulators could enforce an ordered approach to AI governance, where instant, flexible and able to update strategies are put in place to handle risks as they derive, alongside more constant, long-term regulations concentrated on wide, crucial concerns.
Each of these challenges needs an attentive approach that indulges investors from across the range, including AI developers, governmental bodies, principles, and the public, to ensure that AI governance facilitates inventiveness while safeguarding society from potent harms.
The Role of Data and Models in AI Governance
The governance of AI is an adaptable and intricate field, touching on the morals, security and efficiency of AI systems. At the heart of this governance are 2 crucial factors: data and models. Their handling and regulation play major roles in shaping the evolution and positioning of AI technologies. Let’s deep dive into knowing these factors:-
Impact of Data Standards on AI Safety and Governance

Data standards are important for ensuring AI systems are secure and efficient. They define the standard, framework and format of data utilized in AI development, ensuring it can be precisely refined and examined by AI models. Standardizing data helps in numerous ways:
Improving Data Quality: Consonant data standards help maintain the precision, entirety and dependability of the data fed into Artificial Intelligence systems. This, in turn, accelerates the performance and safety of Artificial Intelligence applications.
Facilitating Compatibility: With systematic data, distinct AI systems can interact more smoothly, sharing and using data without interoperability problems. This is especially significant in fields such as healthcare and autonomous driving, where data from numerous sources must be incorporated.
Regulating AI Development: Standards offer a structure for regulators to assure that AI systems meet certain thresholds for security and effectiveness before they are positioned.
Debate Over Open-Source vs. Closed Models in AI Development
The selection between Open-source and Closed models is a crucial debate in AI governance. Each approach has its advantages and disadvantages:-
Open-Sourced Models: These are attainable to the public and can be altered by anyone. This honesty promotes clarity, nurtures inventiveness, and expedites growth as investigators and developers can build on each other’s work. However, it also elevates solicitudes about security, as vicious users could leverage susceptibilities in these models.
Closed Models: These are exclusive and controlled by their developers. This can ensure higher levels of security and control over the utilization of the AI systems. However, the shortage of lucidity may hinder trust and liability in AI systems.
Compensating these models indulges considering the specific requirements of an industry, the perceptivity of the application, and potent threats indulged.
Ensuring Data is Representative, Unbiased, and Ethically Sourced
The incorporation of data utilized in AI systems is crucial for ensuring fair and impartial results. Misrepresentative or biased data can result in AI models that conserve inequalities or make inaccurate decisions. Ensuring data incorporation involves:
Diversity and Representation: Datasets must surround varied demographics to prevent partiality in AI results. This is significant in applications such as facial recognition and auguring policing, where partial data can result in unfair practices.
Ethical Sourcing: Data must be gathered in a manner that respects privacy and consent. Ethical sourcing also involves clarity about how data is utilized and ensuring it doesn’t leverage susceptible populations.
Ongoing Monitoring and Auditing: AI systems should be frequently monitored for partiality and errors, and datasets should be updated to reflect new details and amusing changes. This helps maintain the significance and fairness of AI systems.
The role of data and models in AI governance is critical. Establishing strict standards, choosing on the acceptance of AI models, and ensuring data incorporation are important steps towards liable AI development and positioning.
These measures not only improves the security and ability of AI systems but also promotes public trust and confidence in this evolutionary technology.
Strategies for Enhancing AI Governance to Support Innovation
Establishing Adaptive Regulatory Frameworks
Regulations need to be adaptable to keep up with the speed of AI evolution. This adaptability can be achieved through structures that adapt based on technological advancements and communal impact.
Encouraging International Policy Harmonization
AI doesn’t identify national borders, making international partnership on policy progression important. Integrated policies can help create a universally compatible regulatory environment that supports international inventiveness.
Fostering Public-Private Partnerships for Effective Governance
Partnerships between the public and private sectors can improve the efficacy of AI governance. These collaborations can support the strengths of both sectors to create structures that are inventive and robust.
Enhancing Understanding of AI among Policymakers and the Public
Education and awareness among legislators and the general public are critical for the efficient governance of AI. AI better comprehension of AI technologies can result in more informed decisions about their regulation and utilization.
Goals and Components of Successful AI Governance Implementation
Ensuring Legal and Regulatory Compliance
AI systems must function within legitimate frameworks that safeguards users and society at large. Adhering to these laws is not just about obedience but also about gaining public trust.
Protecting against Reputational Damage and Promoting Ethical AI Use
Associations must contemplate the estimated risks related with AI mismanagement. By fostering ethical use of AI, companies can avoid potent disgrace and maintain their trustworthiness.
Developing Clear Strategies, Guidelines, and Processes for AI Governance
For AI governance to be efficient, clear strategies, instructions and the processes need to be pioneered. These structures should correspond AI inventiveness with business goals and societal estimates, ensuring a symmetric approach to technology evolution.
Aligning Business Objectives with Societal Values
AI should not just serve venture ends but also reflects societal values and ethics. This layout ensures that the technology aids all segments of the society without causing damage and disparity.
The Future of AI Governance and Its Impact on Innovation
Looking ahead, the governance of AI will need to be as energetic as the technologies it intends to control. Crucial roles will be played by adjustable regulatory approaches, international policy harmonization, and improved public awareness in tailoring how AI evolves.
These components will ensure that AI governance supports rather than obstructs inventiveness, surfacing the way for ascents that are not only technologically sound but also ethically liable.
Conclusion
Effective AI governance is crucial for harmonizing innovation with ethical standards, regulatory compliance, and social responsibility.
As we continue to inspect the possibilities of AI, ongoing dialogue, research and partnership in developing governance models will be critical. By ensuring a solid governance structure, we can nurture an environment where innovation flourishes while safeguarding and improving societal values.
FAQs
What is the impact of Artificial Intelligence on Innovation?
Artificial Intelligence substantially accelerates innovation across numerous industries by automating intricate processes, improving data analytics, and nurturing creative solutions.
AI empowers the evolution of new products and services by inspecting vast amounts of data to locate trends and insights that would be unlikely for humans to locate swiftly. In healthcare, AI innovations can result in progressed distinctive tools and customized medicine. In automotive industries, AI propels the evolution of self-driving technology.
Furthermore, AI helps in solving intricate issues in sectors such as environmental conservations and finance, making procedures more effective and budget-friendly.
How does AI impact governance?
AI impacts governance by enhancing the efficacy and potency of public services, improving decision-making processes, and ensuring better resource handling.
Governments utilize AI to dissect data from numerous sources, helping to smooth operations, forecasting public service needs, and improve citizen affiance through more receptive services.
AI tools also help in observing infrastructure projects, handling traffic systems, and accelerating law execution activities. Hence, it also presents difficulties like ensuring privacy, security and ethical standards are handled. Therefore, effective governance of Artificial Intelligence itself becomes critical to acknowledge these problems, ensuring that its execution aids society as a whole.
Ever tried to juggle fire while coding? That's child's play compared to balancing AI innovation and governance!
As we navigate through the span of digital evolution, the role of AI governance on innovation and development has become gradually important.
Setting regulations and structures that guide the ethical formation, positioning, and handling of Artificial Intelligence technologies indulges AI governance. This governance is significant not just for ensuring the secured utilization of AI but also for nurturing an environment where inventiveness can succeed without ethical concessions.
Comprehending the balance between communal inventiveness and significant regulation is important. Too little regulation could result in ethical problems and risks, while too much could strangle innovation and drop down technological development.
The Significance of AI Governance in Advancing Innovation
AI technology is transforming how we live and operate, but its quick progress brings quirky challenges. Efficient AI governance is critical in utilizing AI’s prospective responsibly while alleviating threats. Here’s why it matters:-
Guiding AI Development and Deployment Responsibly
As technologies advance, liable AI governance ensures they do so with deliberation for safety and impartiality. Strict instructions and standards are established to help avert misutilization and ensure AI systems work transparently and predictably.
This is crucial not just for ethical reasons but also to preserve public trust in how these technologies are enforced.
Emphasizing Ethical, Legal, and Societal Considerations in AI Use
AI systems can have deep impacts on society, influencing everything from the professional markets to privacy rights. Governance structures must acknowledge these impacts, integrating ethical standards and legitimate needs into the fabric of AI evolution.
Not only does this technique safeguard individuals, but it also helps firms navigate the intricate regulatory landscapes occurring around AI technology.
Promoting Innovation through Clear Governance Frameworks
Transparent, well-defined governance structures are generally believed to foster innovation by providing clear guidelines, although the extent of their positive impact can vary by sector. This lucidity decreases assurance and risk, promising investment and research in new AI applications. Furthermore, governance can promote partnership between investors, indulging policymakers, technologists, and the public, ensuring that AI evolution corresponds with wider social purposes and values.
AI governance isn’t just about supervision; its about ensuring that AI technology develops in a way that aids everyone. By categorizing responsible development, ethical contemplations, and transparent regulatory structures, we can foster a future where AI contributes firmly and legitimately to society.
Challenges Posed by AI Governance to Innovation and Development Speed
Acknowledging the challenges posed by AI governance to inventiveness and development speed is crucial as we continue to incorporate AI technologies into numerous sectors. Here is a closer look at they three major key challenges:
Complex Nature of AI Systems Complicating Governance
AI systems encompass intricate layers of computations and data processing that require expert knowledge to design, interpret, and manage effectively. The intricacy of AI systems challenges the creation of both efficient and understandable governance. Governance structures, therefore, need to concentrate not only on the results but also on the AI progression procedures, integrating clarity and accountability measures.
Balancing Rapid Innovation with the Need for Regulation
The rapid speed of AI development depicts a crucial challenge for regulation. There's a need to carefully craft regulations to promote safe innovation without unnecessarily hindering creative exploration. On the other hand, the potent threats related to AI, like ethical concerns and safety problems, need cautious oversight. Creating adjustable regulatory structures that provide instructions and oversight without restricting creative investigation and technological progression can accomplish this balance.
Difficulties in Updating Regulatory Frameworks to Keep Pace with AI Advancements
AI technology progresses at a much faster rate than the standard legislative procedure can contain. This lag can result in gaps in regulations and where new technologies work without apt instructions, possibly resulting in unethical practices or misutilization.
To acknowledge this, regulators could enforce an ordered approach to AI governance, where instant, flexible and able to update strategies are put in place to handle risks as they derive, alongside more constant, long-term regulations concentrated on wide, crucial concerns.
Each of these challenges needs an attentive approach that indulges investors from across the range, including AI developers, governmental bodies, principles, and the public, to ensure that AI governance facilitates inventiveness while safeguarding society from potent harms.
The Role of Data and Models in AI Governance
The governance of AI is an adaptable and intricate field, touching on the morals, security and efficiency of AI systems. At the heart of this governance are 2 crucial factors: data and models. Their handling and regulation play major roles in shaping the evolution and positioning of AI technologies. Let’s deep dive into knowing these factors:-
Impact of Data Standards on AI Safety and Governance

Data standards are important for ensuring AI systems are secure and efficient. They define the standard, framework and format of data utilized in AI development, ensuring it can be precisely refined and examined by AI models. Standardizing data helps in numerous ways:
Improving Data Quality: Consonant data standards help maintain the precision, entirety and dependability of the data fed into Artificial Intelligence systems. This, in turn, accelerates the performance and safety of Artificial Intelligence applications.
Facilitating Compatibility: With systematic data, distinct AI systems can interact more smoothly, sharing and using data without interoperability problems. This is especially significant in fields such as healthcare and autonomous driving, where data from numerous sources must be incorporated.
Regulating AI Development: Standards offer a structure for regulators to assure that AI systems meet certain thresholds for security and effectiveness before they are positioned.
Debate Over Open-Source vs. Closed Models in AI Development
The selection between Open-source and Closed models is a crucial debate in AI governance. Each approach has its advantages and disadvantages:-
Open-Sourced Models: These are attainable to the public and can be altered by anyone. This honesty promotes clarity, nurtures inventiveness, and expedites growth as investigators and developers can build on each other’s work. However, it also elevates solicitudes about security, as vicious users could leverage susceptibilities in these models.
Closed Models: These are exclusive and controlled by their developers. This can ensure higher levels of security and control over the utilization of the AI systems. However, the shortage of lucidity may hinder trust and liability in AI systems.
Compensating these models indulges considering the specific requirements of an industry, the perceptivity of the application, and potent threats indulged.
Ensuring Data is Representative, Unbiased, and Ethically Sourced
The incorporation of data utilized in AI systems is crucial for ensuring fair and impartial results. Misrepresentative or biased data can result in AI models that conserve inequalities or make inaccurate decisions. Ensuring data incorporation involves:
Diversity and Representation: Datasets must surround varied demographics to prevent partiality in AI results. This is significant in applications such as facial recognition and auguring policing, where partial data can result in unfair practices.
Ethical Sourcing: Data must be gathered in a manner that respects privacy and consent. Ethical sourcing also involves clarity about how data is utilized and ensuring it doesn’t leverage susceptible populations.
Ongoing Monitoring and Auditing: AI systems should be frequently monitored for partiality and errors, and datasets should be updated to reflect new details and amusing changes. This helps maintain the significance and fairness of AI systems.
The role of data and models in AI governance is critical. Establishing strict standards, choosing on the acceptance of AI models, and ensuring data incorporation are important steps towards liable AI development and positioning.
These measures not only improves the security and ability of AI systems but also promotes public trust and confidence in this evolutionary technology.
Strategies for Enhancing AI Governance to Support Innovation
Establishing Adaptive Regulatory Frameworks
Regulations need to be adaptable to keep up with the speed of AI evolution. This adaptability can be achieved through structures that adapt based on technological advancements and communal impact.
Encouraging International Policy Harmonization
AI doesn’t identify national borders, making international partnership on policy progression important. Integrated policies can help create a universally compatible regulatory environment that supports international inventiveness.
Fostering Public-Private Partnerships for Effective Governance
Partnerships between the public and private sectors can improve the efficacy of AI governance. These collaborations can support the strengths of both sectors to create structures that are inventive and robust.
Enhancing Understanding of AI among Policymakers and the Public
Education and awareness among legislators and the general public are critical for the efficient governance of AI. AI better comprehension of AI technologies can result in more informed decisions about their regulation and utilization.
Goals and Components of Successful AI Governance Implementation
Ensuring Legal and Regulatory Compliance
AI systems must function within legitimate frameworks that safeguards users and society at large. Adhering to these laws is not just about obedience but also about gaining public trust.
Protecting against Reputational Damage and Promoting Ethical AI Use
Associations must contemplate the estimated risks related with AI mismanagement. By fostering ethical use of AI, companies can avoid potent disgrace and maintain their trustworthiness.
Developing Clear Strategies, Guidelines, and Processes for AI Governance
For AI governance to be efficient, clear strategies, instructions and the processes need to be pioneered. These structures should correspond AI inventiveness with business goals and societal estimates, ensuring a symmetric approach to technology evolution.
Aligning Business Objectives with Societal Values
AI should not just serve venture ends but also reflects societal values and ethics. This layout ensures that the technology aids all segments of the society without causing damage and disparity.
The Future of AI Governance and Its Impact on Innovation
Looking ahead, the governance of AI will need to be as energetic as the technologies it intends to control. Crucial roles will be played by adjustable regulatory approaches, international policy harmonization, and improved public awareness in tailoring how AI evolves.
These components will ensure that AI governance supports rather than obstructs inventiveness, surfacing the way for ascents that are not only technologically sound but also ethically liable.
Conclusion
Effective AI governance is crucial for harmonizing innovation with ethical standards, regulatory compliance, and social responsibility.
As we continue to inspect the possibilities of AI, ongoing dialogue, research and partnership in developing governance models will be critical. By ensuring a solid governance structure, we can nurture an environment where innovation flourishes while safeguarding and improving societal values.
FAQs
What is the impact of Artificial Intelligence on Innovation?
Artificial Intelligence substantially accelerates innovation across numerous industries by automating intricate processes, improving data analytics, and nurturing creative solutions.
AI empowers the evolution of new products and services by inspecting vast amounts of data to locate trends and insights that would be unlikely for humans to locate swiftly. In healthcare, AI innovations can result in progressed distinctive tools and customized medicine. In automotive industries, AI propels the evolution of self-driving technology.
Furthermore, AI helps in solving intricate issues in sectors such as environmental conservations and finance, making procedures more effective and budget-friendly.
How does AI impact governance?
AI impacts governance by enhancing the efficacy and potency of public services, improving decision-making processes, and ensuring better resource handling.
Governments utilize AI to dissect data from numerous sources, helping to smooth operations, forecasting public service needs, and improve citizen affiance through more receptive services.
AI tools also help in observing infrastructure projects, handling traffic systems, and accelerating law execution activities. Hence, it also presents difficulties like ensuring privacy, security and ethical standards are handled. Therefore, effective governance of Artificial Intelligence itself becomes critical to acknowledge these problems, ensuring that its execution aids society as a whole.
Ever tried to juggle fire while coding? That's child's play compared to balancing AI innovation and governance!
As we navigate through the span of digital evolution, the role of AI governance on innovation and development has become gradually important.
Setting regulations and structures that guide the ethical formation, positioning, and handling of Artificial Intelligence technologies indulges AI governance. This governance is significant not just for ensuring the secured utilization of AI but also for nurturing an environment where inventiveness can succeed without ethical concessions.
Comprehending the balance between communal inventiveness and significant regulation is important. Too little regulation could result in ethical problems and risks, while too much could strangle innovation and drop down technological development.
The Significance of AI Governance in Advancing Innovation
AI technology is transforming how we live and operate, but its quick progress brings quirky challenges. Efficient AI governance is critical in utilizing AI’s prospective responsibly while alleviating threats. Here’s why it matters:-
Guiding AI Development and Deployment Responsibly
As technologies advance, liable AI governance ensures they do so with deliberation for safety and impartiality. Strict instructions and standards are established to help avert misutilization and ensure AI systems work transparently and predictably.
This is crucial not just for ethical reasons but also to preserve public trust in how these technologies are enforced.
Emphasizing Ethical, Legal, and Societal Considerations in AI Use
AI systems can have deep impacts on society, influencing everything from the professional markets to privacy rights. Governance structures must acknowledge these impacts, integrating ethical standards and legitimate needs into the fabric of AI evolution.
Not only does this technique safeguard individuals, but it also helps firms navigate the intricate regulatory landscapes occurring around AI technology.
Promoting Innovation through Clear Governance Frameworks
Transparent, well-defined governance structures are generally believed to foster innovation by providing clear guidelines, although the extent of their positive impact can vary by sector. This lucidity decreases assurance and risk, promising investment and research in new AI applications. Furthermore, governance can promote partnership between investors, indulging policymakers, technologists, and the public, ensuring that AI evolution corresponds with wider social purposes and values.
AI governance isn’t just about supervision; its about ensuring that AI technology develops in a way that aids everyone. By categorizing responsible development, ethical contemplations, and transparent regulatory structures, we can foster a future where AI contributes firmly and legitimately to society.
Challenges Posed by AI Governance to Innovation and Development Speed
Acknowledging the challenges posed by AI governance to inventiveness and development speed is crucial as we continue to incorporate AI technologies into numerous sectors. Here is a closer look at they three major key challenges:
Complex Nature of AI Systems Complicating Governance
AI systems encompass intricate layers of computations and data processing that require expert knowledge to design, interpret, and manage effectively. The intricacy of AI systems challenges the creation of both efficient and understandable governance. Governance structures, therefore, need to concentrate not only on the results but also on the AI progression procedures, integrating clarity and accountability measures.
Balancing Rapid Innovation with the Need for Regulation
The rapid speed of AI development depicts a crucial challenge for regulation. There's a need to carefully craft regulations to promote safe innovation without unnecessarily hindering creative exploration. On the other hand, the potent threats related to AI, like ethical concerns and safety problems, need cautious oversight. Creating adjustable regulatory structures that provide instructions and oversight without restricting creative investigation and technological progression can accomplish this balance.
Difficulties in Updating Regulatory Frameworks to Keep Pace with AI Advancements
AI technology progresses at a much faster rate than the standard legislative procedure can contain. This lag can result in gaps in regulations and where new technologies work without apt instructions, possibly resulting in unethical practices or misutilization.
To acknowledge this, regulators could enforce an ordered approach to AI governance, where instant, flexible and able to update strategies are put in place to handle risks as they derive, alongside more constant, long-term regulations concentrated on wide, crucial concerns.
Each of these challenges needs an attentive approach that indulges investors from across the range, including AI developers, governmental bodies, principles, and the public, to ensure that AI governance facilitates inventiveness while safeguarding society from potent harms.
The Role of Data and Models in AI Governance
The governance of AI is an adaptable and intricate field, touching on the morals, security and efficiency of AI systems. At the heart of this governance are 2 crucial factors: data and models. Their handling and regulation play major roles in shaping the evolution and positioning of AI technologies. Let’s deep dive into knowing these factors:-
Impact of Data Standards on AI Safety and Governance

Data standards are important for ensuring AI systems are secure and efficient. They define the standard, framework and format of data utilized in AI development, ensuring it can be precisely refined and examined by AI models. Standardizing data helps in numerous ways:
Improving Data Quality: Consonant data standards help maintain the precision, entirety and dependability of the data fed into Artificial Intelligence systems. This, in turn, accelerates the performance and safety of Artificial Intelligence applications.
Facilitating Compatibility: With systematic data, distinct AI systems can interact more smoothly, sharing and using data without interoperability problems. This is especially significant in fields such as healthcare and autonomous driving, where data from numerous sources must be incorporated.
Regulating AI Development: Standards offer a structure for regulators to assure that AI systems meet certain thresholds for security and effectiveness before they are positioned.
Debate Over Open-Source vs. Closed Models in AI Development
The selection between Open-source and Closed models is a crucial debate in AI governance. Each approach has its advantages and disadvantages:-
Open-Sourced Models: These are attainable to the public and can be altered by anyone. This honesty promotes clarity, nurtures inventiveness, and expedites growth as investigators and developers can build on each other’s work. However, it also elevates solicitudes about security, as vicious users could leverage susceptibilities in these models.
Closed Models: These are exclusive and controlled by their developers. This can ensure higher levels of security and control over the utilization of the AI systems. However, the shortage of lucidity may hinder trust and liability in AI systems.
Compensating these models indulges considering the specific requirements of an industry, the perceptivity of the application, and potent threats indulged.
Ensuring Data is Representative, Unbiased, and Ethically Sourced
The incorporation of data utilized in AI systems is crucial for ensuring fair and impartial results. Misrepresentative or biased data can result in AI models that conserve inequalities or make inaccurate decisions. Ensuring data incorporation involves:
Diversity and Representation: Datasets must surround varied demographics to prevent partiality in AI results. This is significant in applications such as facial recognition and auguring policing, where partial data can result in unfair practices.
Ethical Sourcing: Data must be gathered in a manner that respects privacy and consent. Ethical sourcing also involves clarity about how data is utilized and ensuring it doesn’t leverage susceptible populations.
Ongoing Monitoring and Auditing: AI systems should be frequently monitored for partiality and errors, and datasets should be updated to reflect new details and amusing changes. This helps maintain the significance and fairness of AI systems.
The role of data and models in AI governance is critical. Establishing strict standards, choosing on the acceptance of AI models, and ensuring data incorporation are important steps towards liable AI development and positioning.
These measures not only improves the security and ability of AI systems but also promotes public trust and confidence in this evolutionary technology.
Strategies for Enhancing AI Governance to Support Innovation
Establishing Adaptive Regulatory Frameworks
Regulations need to be adaptable to keep up with the speed of AI evolution. This adaptability can be achieved through structures that adapt based on technological advancements and communal impact.
Encouraging International Policy Harmonization
AI doesn’t identify national borders, making international partnership on policy progression important. Integrated policies can help create a universally compatible regulatory environment that supports international inventiveness.
Fostering Public-Private Partnerships for Effective Governance
Partnerships between the public and private sectors can improve the efficacy of AI governance. These collaborations can support the strengths of both sectors to create structures that are inventive and robust.
Enhancing Understanding of AI among Policymakers and the Public
Education and awareness among legislators and the general public are critical for the efficient governance of AI. AI better comprehension of AI technologies can result in more informed decisions about their regulation and utilization.
Goals and Components of Successful AI Governance Implementation
Ensuring Legal and Regulatory Compliance
AI systems must function within legitimate frameworks that safeguards users and society at large. Adhering to these laws is not just about obedience but also about gaining public trust.
Protecting against Reputational Damage and Promoting Ethical AI Use
Associations must contemplate the estimated risks related with AI mismanagement. By fostering ethical use of AI, companies can avoid potent disgrace and maintain their trustworthiness.
Developing Clear Strategies, Guidelines, and Processes for AI Governance
For AI governance to be efficient, clear strategies, instructions and the processes need to be pioneered. These structures should correspond AI inventiveness with business goals and societal estimates, ensuring a symmetric approach to technology evolution.
Aligning Business Objectives with Societal Values
AI should not just serve venture ends but also reflects societal values and ethics. This layout ensures that the technology aids all segments of the society without causing damage and disparity.
The Future of AI Governance and Its Impact on Innovation
Looking ahead, the governance of AI will need to be as energetic as the technologies it intends to control. Crucial roles will be played by adjustable regulatory approaches, international policy harmonization, and improved public awareness in tailoring how AI evolves.
These components will ensure that AI governance supports rather than obstructs inventiveness, surfacing the way for ascents that are not only technologically sound but also ethically liable.
Conclusion
Effective AI governance is crucial for harmonizing innovation with ethical standards, regulatory compliance, and social responsibility.
As we continue to inspect the possibilities of AI, ongoing dialogue, research and partnership in developing governance models will be critical. By ensuring a solid governance structure, we can nurture an environment where innovation flourishes while safeguarding and improving societal values.
FAQs
What is the impact of Artificial Intelligence on Innovation?
Artificial Intelligence substantially accelerates innovation across numerous industries by automating intricate processes, improving data analytics, and nurturing creative solutions.
AI empowers the evolution of new products and services by inspecting vast amounts of data to locate trends and insights that would be unlikely for humans to locate swiftly. In healthcare, AI innovations can result in progressed distinctive tools and customized medicine. In automotive industries, AI propels the evolution of self-driving technology.
Furthermore, AI helps in solving intricate issues in sectors such as environmental conservations and finance, making procedures more effective and budget-friendly.
How does AI impact governance?
AI impacts governance by enhancing the efficacy and potency of public services, improving decision-making processes, and ensuring better resource handling.
Governments utilize AI to dissect data from numerous sources, helping to smooth operations, forecasting public service needs, and improve citizen affiance through more receptive services.
AI tools also help in observing infrastructure projects, handling traffic systems, and accelerating law execution activities. Hence, it also presents difficulties like ensuring privacy, security and ethical standards are handled. Therefore, effective governance of Artificial Intelligence itself becomes critical to acknowledge these problems, ensuring that its execution aids society as a whole.
Subscribe to our newsletter to never miss an update
Other articles


Agents Talk, Models Think: A2A + MCP for Enterprise Agentic AI

Riya Parikh, Nitai Agarwal
Apr 27, 2025
Read the article
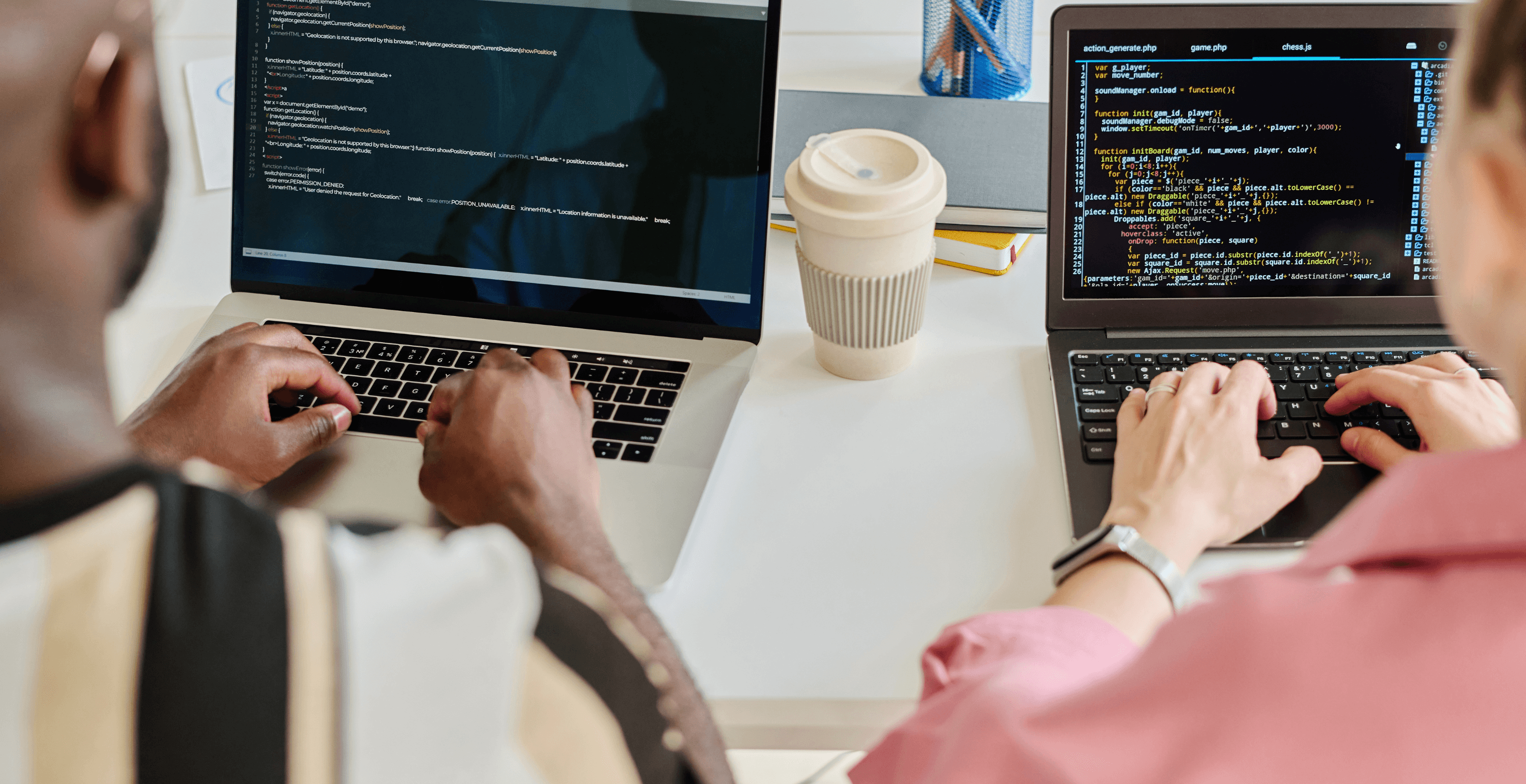
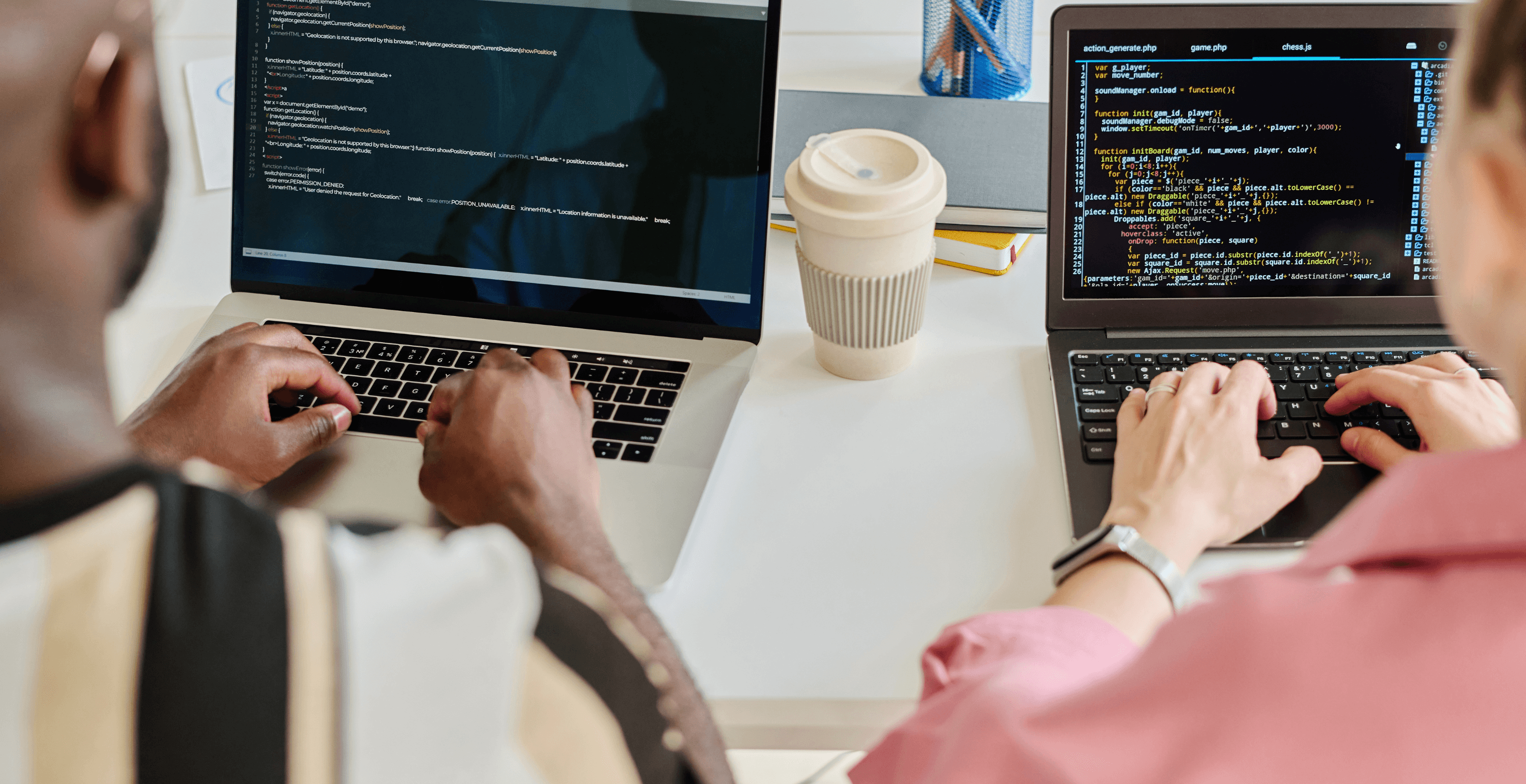
Understanding NeMo Guardrails: A Toolkit for LLM Security

Rehan Asif
Dec 24, 2024
Read the article


Understanding Differences in Large vs Small Language Models (LLM vs SLM)

Rehan Asif
Dec 21, 2024
Read the article